Risk Prediction Models for Lung Cancer: A Systematic Review
Affiliations.
- 1 Department of School of Health and Related Research, University of Sheffield, Sheffield, United Kingdom. Electronic address: [email protected].
- 2 Department of School of Health and Related Research, University of Sheffield, Sheffield, United Kingdom.
- PMID: 26712102
- DOI: 10.1016/j.cllc.2015.11.007
Many lung cancer risk prediction models have been published but there has been no systematic review or comprehensive assessment of these models to assess how they could be used in screening. We performed a systematic review of lung cancer prediction models and identified 31 articles that related to 25 distinct models, of which 11 considered epidemiological factors only and did not require a clinical input. Another 11 articles focused on models that required a clinical assessment such as a blood test or scan, and 8 articles considered the 2-stage clonal expansion model. More of the epidemiological models had been externally validated than the more recent clinical assessment models. There was varying discrimination, the ability of a model to distinguish between cases and controls, with an area under the curve between 0.57 and 0.879 and calibration, the model's ability to assign an accurate probability to an individual. In our review we found that further validation studies need to be considered; especially for the Prostate, Lung, Colorectal, and Ovarian (PLCO) Cancer Screening Trial 2012 Model Version (PLCOM2012) and Hoggart models, which recorded the best overall performance. Future studies will need to focus on prediction rules, such as optimal risk thresholds, for models for selective screening trials. Only 3 validation studies considered prediction rules when validating the models and overall the models were validated using varied tests in distinct populations, which made direct comparisons difficult. To improve this, multiple models need to be tested on the same data set with considerations for sensitivity, specificity, model accuracy, and positive predictive values at the optimal risk thresholds.
Keywords: Clinical utility; Prediction models; Prediction models' design; Prediction models' discrimination; Validation results.
Copyright © 2016 Elsevier Inc. All rights reserved.
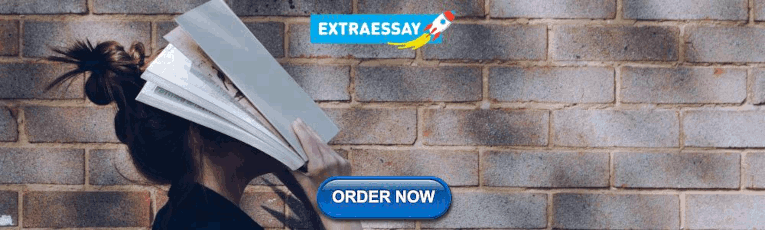
Publication types
- Research Support, Non-U.S. Gov't
- Systematic Review
- Decision Support Techniques*
- Early Detection of Cancer / methods
- Lung Neoplasms / diagnosis*
- Lung Neoplasms / epidemiology
- Models, Statistical
- Predictive Value of Tests
- Risk Assessment
- Risk Factors
- Sensitivity and Specificity
Log in using your username and password
- Search More Search for this keyword Advanced search
- Latest content
- Current issue
- Instructions for Authors
- BMJ Journals More You are viewing from: Google Indexer
You are here
- Online First
- Chemotherapy decision-making in advanced lung cancer: a prospective qualitative study
- Article Text
- Article info
- Citation Tools
- Rapid Responses
- Article metrics

- Annmarie Nelson 1 ,
- http://orcid.org/0000-0002-9867-3806 Mirella Longo 1 ,
- Anthony Byrne 1 ,
- Stephanie Sivell 1 ,
- http://orcid.org/0000-0001-5425-2383 Simon Noble 1 ,
- Jason Lester 2 ,
- Lesley Radley 3 ,
- David Jones 3 ,
- Catherine Sampson 1 and
- Despina Anagnostou 1
- 1 Marie Curie Palliative Care Research Centre, School of Medicine , Cardiff University , Cardiff , South Glamorgan , UK
- 2 Velindre Cancer Centre , Cardiff , Cardiff , UK
- 3 Cardiff University , Cardiff , South Glamorgan , UK
- Correspondence to Professor Annmarie Nelson, Marie Curie Palliative Care Research Centre, School of Medicine, Cardiff University, Cardiff cf14 4ys, South Glamorgan, UK; nelsona9{at}Cardiff.ac.uk
Objective To study how treatment decisions are made alongside the lung cancer clinical pathway.
Methods A prospective, multicentre, multimethods, five-stage, qualitative study. Mediated discourse, thematic, framework and narrative analysis were used to analyse the transcripts.
Results 51 health professionals, 15 patients with advanced lung cancer, 15 family members and 18 expert stakeholders were recruited from three UK NHS trusts. Multidisciplinary team (MDT) members constructed treatment recommendations around patient performance status, pathology, clinical information and imaging. Information around patients’ social context, needs and preferences were limited. The provisional nature of MDTs treatment recommendations was not always linked to future discussions with the patient along the pathway, that is, patients’ interpretation of their prognosis, treatment discussions occurring prior to seeing the oncologist. This together with the rapid disease trajectory placed additional stress on the oncologist, who had to introduce a different treatment option from that recommended by the MDT or patient’s expectations. Palliative treatment was not referred to explicitly as such, due to its potential for confusion. Patients were unaware of the purpose of each consultation and did not fully understand the non-curative intent of treatment pathways. Patients’ priorities were framed around social and family needs, such as being able to attend a family event.
Conclusion Missed opportunities for information giving, affect both clinicians and patients; the pathway for patients with non-small cell lung cancer focuses on clinical management at the expense of patient-centred care. Treatment decisions are a complex process and patients draw conclusions from healthcare interactions prior to the oncology clinic, which prioritises aggressive treatment and influences decisions.
- communication
- supportive care
- clinical assessment
- quality of life
This is an open access article distributed in accordance with the Creative Commons Attribution Non Commercial (CC BY-NC 4.0) license, which permits others to distribute, remix, adapt, build upon this work non-commercially, and license their derivative works on different terms, provided the original work is properly cited, appropriate credit is given, any changes made indicated, and the use is non-commercial. See: http://creativecommons.org/licenses/by-nc/4.0/ .
https://doi.org/10.1136/bmjspcare-2020-002395
Statistics from Altmetric.com
Request permissions.
If you wish to reuse any or all of this article please use the link below which will take you to the Copyright Clearance Center’s RightsLink service. You will be able to get a quick price and instant permission to reuse the content in many different ways.
Introduction
New developments in immunotherapy offer promising treatment for advanced lung cancer. 1 However, a considerable number of patients with non-small cell lung cancer (NSCLC) present with locally advanced or metastatic disease and are currently unsuitable for curative treatment with a median survival of 6–8 months. 2 The fear of destroying hope places a huge burden on the clinicians who may resort to the treatment imperative; 3 patients’ limited understanding of treatment with palliative intent 4 5 further hinders the patients’ opportunity to consider all available options and to make an informed decision. Treatment decisions become time bound, complex and require information and awareness for both patients and clinicians.
Chemotherapy with palliative intent is used for symptom control and to improve quality of life; however, systemic anticancer treatment (SACT) may have unintentionally detrimental effects. For patients in advanced stage, including NSCLC, SACT can increase early treatment-related mortality, 6–10 toxicities, emergency and inpatient admissions 11 and affect quality of life, even in patients with good performance status. 12 Around 10% of patients with lung cancer are dying within 30 days of SACT and this is arguably overly aggressive care. The urgency of administering treatments, in an attempt to mitigate deterioration, can also result in underpreparation of patients and families at the end of life 13 14 and patients become less likely to die in their preferred place of death. 15 Documentation of end of life discussions and discontinuation of treatment is rare; 16 17 however, early links with palliative care teams improve the timing of final chemotherapy administration and transition to hospice services. 18–20 Patients opting out of SACT 21 show greater acceptance of terminal status, focus on the present and on time with family.
The WHO places responsiveness and participation as key to achieving integrated people-centred health services and advocates care in respect of people’s preferences. 22 Clinical pathways aim to support decisions along the treatment pathway; however, in oncology, these seem to focus on when to start anticancer treatment—not when to stop. Studying the treatment decision-making process, alongside the patient clinical pathway, helps to understand the interaction of factors at different levels and how these influence the success or the failure of patient-centred care in the context of these very sensitive consultations. This study aimed to understand how palliative chemotherapy treatment decisions are determined and what intervention could support Patients with NSCLC and clinicians when considering AntiCancer Therapy (the PACT study). The paper adheres to Consolidated criteria for Reporting Qualitative research guidelines ( online supplementary 1 ; online supplementary table e1 ).
Supplemental material
The PACT study was a five-stage, prospective, qualitative multicentre, multimethods study. The original protocol 23 was adhered to with the exception of using narrative analysis in place of interpretative phenomenological analysis as clear narratives emerged in the data. A conceptual framework was built around study aim and objectives ( figure 1 ).
- Download figure
- Open in new tab
- Download powerpoint
Patients with non-small cell lung cancer and clinicians when considering AntiCancer Therapy (PACT) conceptual framework. MDT, multidisciplinary team.
Participants
A stakeholder approach was used to purposively sample study participants. For phases 1–4, clinicians and patients were recruited from three hospitals set within three University Health Boards in Wales. UK experts with an interest in lung cancer were invited to the expert consensus event. The data collected throughout phases 2–4 included the same patient/doctor dyad. Patient and public involvement (PPI) was incorporated throughout the study and in accordance with the national standards.
Approach and consent
Patients with a diagnosis of NSCLC and due to attend their first appointment with the oncologist were eligible to be part of the study. Multidisciplinary team (MDT) members were recruited and consented first. Clinicians identified eligible participants and provided them with an information sheet and a cover letter which included a reply slip where they would specify their contact details if they wished to be contacted about the study. Consent from clinicians, patients, companions and experts attending the consensus event was taken by the researchers (CS, DS, ML). Audio recording and fields notes were used throughout.
Data analysis
Inductive thematic analysis was used initially to aid understanding of the data before specific methodologies were overlaid. Principles of mediated discourse analysis were applied to MDT meetings to understand team dynamics and working practices, the role played by key MDT members and factors influencing allocation of patients to treatment pathways. Narrative analysis was used to study the consultations between the patient/companion and clinician and follow-up face-to-face interviews. Narrative analysis highlighted the priorities of the participants in single consultations; it also enabled the decision-making trajectory to be modelled through a generic consultation with priorities for each participant indicated at each phase. The OPTION (Observing PaTient InvOlvemeNt) tool 24 was used as a consultation process framework to assess how clinicians involved patients in consultations under key clinical domains ( online supplementary 2 ; online supplementary table e2 ); this was reflected in the interview guide ( online supplementary 4 ). Phase 5 drew together expert stakeholders and provided the platform where information from phases 1 to 4 could be shared, questioned and prioritised by all parties. Group discussions were held and covered seven areas of enquiry (eg, patients’ preparedness; online supplementary 4 , online supplementary table e3 ). The composition of groups ensured that while the focus was on the patient, the intervention would receive input from carers and health professionals at each stage of the clinical pathway. A deductive thematic analysis (coding tree focus group 3— online supplementary 5 , online supplementary tables e4-e10 ) of the group discussions was initially organised around responses to the areas of enquiry and then transformed into a series of statements of suggested practice attached to the clinical pathway. Data saturation was determined by the researchers. NVIVO (V.12) was used to organise the data.
Research team reflexivity
The research team comprised clinical oncology consultants, palliative care consultants, patient representatives and experienced postdoctoral researchers with expertise in healthcare research, decision-making, ethnography, discourse analysis and narrative analysis. This variety helped to balance knowledge and assumptions, personal experiences and biases towards aims of treatment, or the dynamic relationships of patients and healthcare professionals. Data collection was undertaken by experienced and trained researchers (DS, CS, ML) not associated with patients or clinical sites.
Between March 2015 and January 2017, a total of 99 participants were recruited into the study ( online supplementary 6 ; online supplementary table e11 ). Participants who declined to take part were not recorded.
PPI involvement
One public contributor (PC) contributed to the development of the research proposal. During the study, two PCs commented on the: information available to patients and their families; jargon used at MDT meetings; and patient recruitment strategies. They took part in the consensus day. They read a sample of anonymised transcripts of patient–clinician consultations and contributed to validate, interpret and contextualise the findings. The PCs continue to be involved in the dissemination of the study results and have agreed to join the follow-up study.
Lung cancer MDT meetings
Each MDT meeting followed local site routines; however, the primary purpose was to provide accurate diagnosis and recommend treatment pathways. Meetings usually included a chest physician, an oncologist, a radiologist, a pathologist, a lung clinical nurse specialist (CNS) and an MDT coordinator. Palliative care consultants were not always present. Patients were primarily represented through technology ( figure 2 ). Patient-focused clinical and social information was limited.
Presenting patient information at the multidisciplinary team meeting.
Radiology and pathology reports were the key references for making treatment recommendations, with the chest physician and the oncologist making key contributions to the decision-making discussions. Performance status was significant in moderating discussion of treatment options. However, treatment decisions were sometimes based on a historical performance status (quotation 1— online supplementary 7 , online supplementary table e12 ).
The provisional nature of a treatment recommendation was not always clearly linked to future discussions with the patient at identified key points on the pathway, that is, considering what the chest physician would discuss and how the patient’s interpretation of their prognosis and any treatment discussions would be conveyed to the oncologist.
Oncology clinic
Four themes emerged from the analysis of the oncology consultation and follow-up interviews: process and priorities were key themes influencing the choice/availability of treatment options and decision-making in the oncology consultation; and the concept of palliative treatment intent and prognosis were key challenges to communication of options. The intersection between these four themes influenced the explicit discussion of options and terminal prognoses and gave insights into the uncertainty for patients around treatment options (quotation 2— online supplementary 7 , online supplementary table e12 ).
Patients were generally unaware that the oncology consultation was a treatment decision-making event and carried expectations about their treatment plan originating earlier by prior events on the clinical pathway (for instance, discussion with the chest physician) (quotations 3 and 4— online supplementary 7 , online supplementary table e12 ). This suggests a series of missed opportunities for ‘preparedness’ from both parties.
The oncology consultation evolved over several stages; at each stage, each stakeholder held specific priorities ( figure 3 ).
Example of narrative analysis applied to the oncology consultation.
Patients focused on relationships, placing their disease in the context of everyday life and were concerned with what would happen next. Treatment context was focused on external priorities, such as being able to attend a special family event, and existing relationships with health professionals, all of which influenced their perception and knowledge of a particular treatment option (quotation 5— online supplementary 7 , online supplementary table e12 ). Key issues were challenges for successful communication of their priorities, expertise and constancy within health professional relationships, and the need for adequate support to live with the uncertainty of their disease trajectory.
Oncologists were concerned with establishing rapport, were aware of time pressures and followed their own established structure. Performance status description alone did not provide the necessary contextual information for the oncologist who often had to re-evaluate the patient at the time of consultation. Key issues were communicating other treatment options (quotation 6— online supplementary 7 , online supplementary table e12 ), managing uncertainty; particularly where the proposed MDT treatment decision trajectory had changed or where expectations from the chest physician consultation were no longer possible (quotation 7— online supplementary 7 , online supplementary table e12 ).
Companions acted as the patient advocate and their role was to confirm patients’ knowledge, needs and preference (quotation 8— online supplementary 7 , online supplementary table e12 ) and provide information on the patient’s quality of life. Key issues were preparing the patient to be as well as possible for treatment, having a consistent point of contact with the health professional team, and being aware of potentially different coping styles between patients and carers.
CNS s advocated for the patient perspective and highlighted relationships, patient’s home context and priorities, where they were known to them (quotation 9— online supplementary 7 , online supplementary table e12 ). Key issues were the need for ongoing contact, explain terminology and outline treatment practicalities again, and giving the patient opportunities to reflect on their decisions.
Situations where a patient did not wish to discuss prognosis explicitly presented challenges for the oncologist (quotation 10— online supplementary 7 , online supplementary table e12 ). Similar challenges arose when there was no formal documentation of how prognosis had been discussed with, and understood by, the patient and carer. Communication skills were key to the confidence of the oncologist in navigating the consultation without directly discussing prognosis.
Palliative intent of treatment
The most common treatment option offered was palliative chemotherapy, where the word ‘palliative’ was unspoken and remained implicit in the description (quotation 11— online supplementary 7 , online supplementary table e12 ). There was potential for broad interpretation around the possibility of chemotherapy extending life (quotation 12— online supplementary 7 , online supplementary table e12 ), and patient misunderstanding of the purpose as being palliative. However, when palliative was used, this often led to confusion as patients were uncertain about what exactly the oncologist was referring to.
The distinction between palliative treatments perceived as ‘active’ was significant, which indicated the lack of a positive alternative supportive care option. This was reinforced by oncologists’ discussion of their reluctance to use palliative terminology to avoid distress, suggesting ambivalence or lack of understanding around how palliative chemotherapy is presented (quotation 13— online supplementary 7 , online supplementary table e12 ).
Treatment decision around SACT
Patients opting for SACT without questioning the oncologist had often discussed the proposed treatment option with the chest physician or CNS and had placed their trust in those health professionals. A further deciding factor was where patients felt that their only option was SACT, compounded by the lack of clear definition of what constitutes an active palliative pathway as a viable option.
Opting out of a proposed treatment was challenging for patients; patients did not want ‘active treatment’, as described by oncologists, for quality of life reasons (quotation 14— online supplementary 7 , online supplementary table e12 ) but were compromised further when oncologists positioned palliative chemotherapy as an option that had to be overtly refused. Table 1 displays the treatment pathways and survival of our study participants.
- View inline
Patients’ planned and actual treatment pathway
Consensus day: defining the key components of the intervention
The findings from phases 1 to 4 set the framework underpinning the design of the intervention. These consistently highlight a lack of ‘preparedness’ along the clinical pathway ( figure 4 ).
Statements of suggested practice mapped to the clinical pathway. MDT, multidisciplinary team; NHS, National Health Service.
This mapping signifies a departure from a traditional clinical pathway, providing information on all treatment options early, and identifying patients’ priorities and preferences to inform MDT recommendations in advance of the oncology consultation. The data confirm that a future intervention should include a systematic collection and bidirectional flow of relevant information along the pathway, starting from primary care.
Tailored qualitative methods were used to understand how palliative chemotherapy treatment decisions are determined in advanced lung cancer. Data collection began with the MDT meetings where treatment discussions begin. MDT members constructed treatment recommendations mainly around patient performance status, pathology, clinical information and imaging. Patients’ needs were not fully addressed because information about their social needs and preferences was limited. 25
Measures of performance status did not fully capture patients’ overall function and in this context where risks and benefits are finely balanced, alternative indicators regarding patients’ ability to tolerate treatment, for example, frailty, cachexia should be considered. 26–28 Here, the palliative care ethos of keeping people as well as possible for as long as possible highlights the risks of tipping the balance—between preserving patients’ function, and ability to take part in everyday activities, to hastening decline from avoidable toxicities. With increasing evidence to support the benefits of specialist palliative care for patients with advanced cancer, 29 there is an argument against avoidable harm by SACT, and disenfranchisement of patients’ access to better care.
Prognosis was the link for the oncologist to discuss available treatment options, and was compromised either by situations where a patient expressed a clear desire not to refer explicitly to prognosis, or where there was lack of information sharing among clinicians about how prognosis had been interpreted by the patient. 30 Further, for patients with NSCLC, decision-making is a continuous process 17 rather than a single event. With a rapid disease trajectory, the health of the patient might change significantly before the oncology appointment. The oncologist may have to re-evaluate the patient and consider a different treatment option to that recommended at the MDT. 31 This proved particularly demanding for less experienced clinicians; training protocols have been developed but they did not always result in increased competency. 32 Clinicians’ attitude towards hope is also contributory to limited disclosure as Atul Gawande 33 describes it “Suppose I was wrong, I wondered, and she proved to be that miracle patient who survived metastatic lung cancer?” (p168). In addition to this, clinicians might be hesitant to crush patients’ expectations when discussing palliative cancer treatments. Communication styles 34 35 can affect patients’ experiences and treatment choices, new approaches to treatment decisions, such as the global Choosing Wisely initiative 36 encourage the use of prompt questions by clinicians and patients to weigh up the risks and benefits of treatment including the potential trigger question, ‘What if I do nothing’.
Issues relating to palliative terminology often arose; 4 however, patients’ understanding of ‘palliative’ can be improved 37 and, differentiating between palliative care and end-of-life care, to what is effectively supportive oncology, could also improve referral. 38 From a population perspective, societal challenges have been identified, including a public acceptance of death as part of being human, and the education of patients and families about treatment goals for cancer. 39 40
The study adopted a coproductive approach, which ensured that all relevant stakeholders contributed to inform the intervention. The robust multiqualitative design allowed the study of the patient journey from different perspectives and levels. Patients represented geographical, and socioeconomic diversity, and different communities of professional practice. This study did not aim to contest or verify the appropriateness of the treatment decisions made. However, from the analysis of the treatment decision-making process, it emerged that there is a series of missed opportunities along the clinical pathway and these impacted on both clinicians and patients. Embedding patients’ values and preferences into the treatment decision-making process may facilitate difficult consultations, reduce aggressive end-of-life treatments, and reflect more closely patients’ goals and motives in their everyday lives.
Conclusions
Current cancer clinical pathways are not designed to include the circumstances, priorities and preferences of patients taking part in these highly sensitive consultations. The optimal management of advanced patients with NSCLC necessitates going beyond the treatment of symptoms by reflecting what matters to the patients within their personal context.
This patient population is characterised by a complex intersection of terminal disease with a short prognosis and declining functioning. Decisions around treatment towards end of life have a particular acuity, the limited patient understanding of the aims of treatment and the challenges faced by the clinicians who might resort to the treatment imperative may result in a clinical pathway heavily weighted towards SACT.
In the palliative context, the concept of hope realigns more realistically from hope of a cure to hope of comfort and quality of life. This study promotes opportunities for engagement with the patient (and family) and clinicians at different levels of the pathway to identify goals of care echoing the patient’s view of a life worth living.
Although these findings reflect the narratives of the study participants and the researchers’ interpretation of these, they also reflect elements easily applicable to many patients diagnosed with advanced cancer.
- Hellmann MD ,
- Rizvi NA , et al
- Connell CM ,
- De Vries RG , et al
- McIlfatrick S ,
- McCorry NK , et al
- Catalano PJ ,
- Cronin A , et al
- O’Hara C , et al
- O'Brien MR ,
- Whitehead B ,
- Murphy PN , et al
- Hamilton K , et al
- Wallington M ,
- Bomb M , et al
- Neville BA ,
- Landrum MB , et al
- Prigerson HG ,
- Shah MA , et al
- Burgers JA ,
- Murray SA ,
- Kendall M ,
- Mitchell G , et al
- Higginson IJ ,
- Sen-Gupta GJ
- Helde-Frankling M ,
- Runesdotter S , et al
- Traeger L ,
- Rapoport C ,
- Wright E , et al
- Jackson VA , et al
- Wright AA ,
- Ray A , et al
- Walling A ,
- Dy S , et al
- Anagnostou D ,
- Noble S , et al
- Hutchings H ,
- Edwards A , et al
- Arora S , et al
- Rockwood K ,
- MacKnight C , et al
- Gambassi G ,
- van Kan GA ,
- Abellan van Kan G , et al
- Moffatt H ,
- Moorhouse P ,
- Mallery L , et al
- Ziegler LE ,
- Craigs CL ,
- West RM , et al
- Laryionava K ,
- Reiter-Theil S , et al
- Trinidad SB ,
- Hopley EK , et al
- Johnson LA ,
- Morse R , et al
- Henselmans I ,
- Van Laarhoven HW ,
- Van der Vloodt J , et al
- Levinson W ,
- Kallewaard M ,
- Bhatia RS , et al
- Carpenter BD
- El-Jawahri A , et al
- Harrington SE ,
Twitter @annmarienelson0, @MirellaLongo18
Contributors AN conceived and designed the study; drafted the manuscript. AN, SN, AB, SS and JL secured funding for the study. DA, CS and ML led the collection and analysis of the data. All authors made substantial contribution to the analysis and interpretation of the data. The public contributors (LR and DJ) supported the study throughout and carried out the validation of the findings. ML and CS contributed to the drafting of the manuscript. All authors reviewed and commented on the drafts of this manuscript. All authors read and approved the final manuscript. CS and DA are joint last authors.
Funding This study was funded by The Velindre Stepping Stones Appeal within Velindre NHS Trust Charitable Funds; Grant No 2013/009. Sponsored by Cardiff University; and coordinated by the Marie Curie core-funded Marie Curie Palliative Care Research Centre at Cardiff University. AN, AB, ML, SS and SN’s posts are funded by Marie Curie Cancer Care core grant funding (grant reference MCCC-FCO-17-C).
Competing interests None declared.
Patient consent for publication Not required.
Ethics approval Ethical approval was granted (REC 14/WA/1103).
Provenance and peer review Not commissioned; internally peer reviewed.
Data availability statement Data are available upon reasonable request. Anonymised narratives from the transcripts will be available upon request.
Read the full text or download the PDF:
Thank you for visiting nature.com. You are using a browser version with limited support for CSS. To obtain the best experience, we recommend you use a more up to date browser (or turn off compatibility mode in Internet Explorer). In the meantime, to ensure continued support, we are displaying the site without styles and JavaScript.
- View all journals
- My Account Login
- Explore content
- About the journal
- Publish with us
- Sign up for alerts
- Open access
- Published: 14 January 2022
Deep learning-based algorithm for lung cancer detection on chest radiographs using the segmentation method
- Akitoshi Shimazaki 1 ,
- Daiju Ueda 1 , 2 ,
- Antoine Choppin 3 ,
- Akira Yamamoto 1 ,
- Takashi Honjo 1 ,
- Yuki Shimahara 3 &
- Yukio Miki 1
Scientific Reports volume 12 , Article number: 727 ( 2022 ) Cite this article
20k Accesses
45 Citations
7 Altmetric
Metrics details
- Lung cancer
- Radiography
We developed and validated a deep learning (DL)-based model using the segmentation method and assessed its ability to detect lung cancer on chest radiographs. Chest radiographs for use as a training dataset and a test dataset were collected separately from January 2006 to June 2018 at our hospital. The training dataset was used to train and validate the DL-based model with five-fold cross-validation. The model sensitivity and mean false positive indications per image (mFPI) were assessed with the independent test dataset. The training dataset included 629 radiographs with 652 nodules/masses and the test dataset included 151 radiographs with 159 nodules/masses. The DL-based model had a sensitivity of 0.73 with 0.13 mFPI in the test dataset. Sensitivity was lower in lung cancers that overlapped with blind spots such as pulmonary apices, pulmonary hila, chest wall, heart, and sub-diaphragmatic space (0.50–0.64) compared with those in non-overlapped locations (0.87). The dice coefficient for the 159 malignant lesions was on average 0.52. The DL-based model was able to detect lung cancers on chest radiographs, with low mFPI.
Similar content being viewed by others
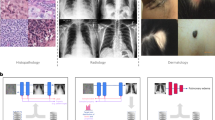
Generative models improve fairness of medical classifiers under distribution shifts
Ira Ktena, Olivia Wiles, … Sven Gowal
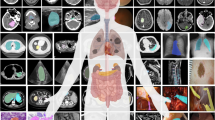
Segment anything in medical images
Jun Ma, Yuting He, … Bo Wang
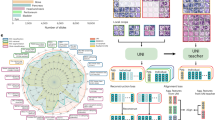
Towards a general-purpose foundation model for computational pathology
Richard J. Chen, Tong Ding, … Faisal Mahmood
Introduction
Lung cancer is the primary cause of cancer death worldwide, with 2.09 million new cases and 1.76 million people dying from lung cancer in 2018 1 . Four case-controlled studies from Japan reported in the early 2000s that the combined use of chest radiographs and sputum cytology in screening was effective for reducing lung cancer mortality 2 . In contrast, two randomized controlled trials conducted from 1980 to 1990 concluded that screening with chest radiographs was not effective in reducing mortality in lung cancer 3 , 4 . Although the efficacy of chest radiographs in lung cancer screening remains controversial, chest radiographs are more cost-effective, easier to access, and deliver lower radiation dose compared with low-dose computed tomography (CT). A further disadvantage of chest CT is excessive false positive (FP) results. It has been reported that 96% of nodules detected by low-dose CT screening are FPs, which commonly leads to unnecessary follow-up and invasive examinations 5 . Chest radiography is inferior to chest CT in terms of sensitivity but superior in terms of specificity. Taking these characteristics into consideration, the development of a computer-aided diagnosis (CAD) model for chest radiograph would have value by improving sensitivity while maintaining low FP results.
The recent application of convolutional neural networks (CNN), a field of deep learning (DL) 6 , 7 , has led to dramatic, state-of-the-art improvements in radiology 8 . DL-based models have also shown promise for nodule/mass detection on chest radiographs 9 , 10 , 11 , 12 , 13 , which have reported sensitivities in the range of 0.51–0.84 and mean number of FP indications per image (mFPI) of 0.02–0.34. In addition, radiologist performance for detecting nodules was better with these CAD models than without them 9 . In clinical practice, it is often challenging for radiologists to detect nodules and to differentiate between benign and malignant nodules. Normal anatomical structures often appear as if they are nodules, which is why radiologists must pay careful attention to the shape and marginal properties of nodules. As these problems are caused by the conditions rather than the ability of the radiologist, even skillful radiologists can misdiagnose 14 , 15 .
There are two main methods for detecting lesions using DL: detection and segmentation. The detection method is a region-level classification, whereas the segmentation method is a pixel-level classification. The segmentation method can provide more detailed information than the detection method. In clinical practice, classifying the size of a lesion at the pixel-level increases the likelihood of making a correct diagnosis. Pixel-level classification also makes it easier to follow up on changes in lesion size and shape, since the shape can be used as a reference during detection. It also makes it possible to consider not only the long and short diameters but also the area of the lesion when determining the effect of treatment 16 . However, to our knowledge, there are no studies using the segmentation method to detect pathologically proven lung cancer on chest radiographs.
The purpose of this study was to train and validate a DL-based model capable of detecting lung cancer on chest radiographs using the segmentation method, and to evaluate the characteristics of this DL-based model to improve sensitivity while maintaining low FP results.
The following points summarize the contributions of this article:
This study developed a deep learning-based model for detection and segmentation of lung cancer on chest radiographs.
Our dataset is high quality because all the nodules/masses were pathologically proven lung cancers, and these lesions were pixel-level annotated by two radiologists.
The segmentation method was more informative than the classification or detection methods, which is useful not only for the detection of lung cancer but also for follow-up and treatment efficacy.
Materials and methods
Study design.
We retrospectively collected consecutive chest radiographs from patients who had been pathologically diagnosed with lung cancer at our hospital. Radiologists annotated the lung cancer lesions on these chest radiographs. A DL-based model for detecting lung cancer on radiographs was trained and validated with the annotated radiographs. The model was then tested with an independent dataset for detecting lung cancers. The protocol for this study was comprehensively reviewed and approved by the Ethical Committee of Osaka City University Graduate School of Medicine (No. 4349). Because the radiographs had been acquired during daily clinical practice and informed consent for their use in research had been obtained from patients, the Ethical Committee of Osaka City University Graduate School of Medicine waived the need for further informed consent. All methods were performed in accordance with the relevant guidelines and regulations.
Eligibility and ground truth labelling
Two datasets were used to train and test the DL-based model, a training dataset and a test dataset. We retrospectively collected consecutive chest radiographs from patients pathologically diagnosed with lung cancer at our hospital. The training dataset was comprised of chest radiographs obtained between January 2006 and June 2017, and the test dataset contained those obtained between July 2017 and June 2018. The inclusion criteria were as follows: (a) pathologically proven lung cancer in a surgical specimen; (b) age > 40 years at the time of the preoperative chest radiograph; (c) chest CT performed within 1 month of the preoperative chest radiograph. If the patient had multiple chest radiographs that matched the above criteria, the latest radiograph was selected. Most of these chest radiographs were taken as per routine before hospitalization and were not intended to detect lung cancer. Chest radiographs on which radiologists could not identify the lesion, even with reference to CT, were excluded from analysis. For eligible radiographs, the lesions were annotated by two general radiologists (A.S. and D.U.), with 6 and 7 years of experience in chest radiography, using ITK-SNAP version 3.6.0 ( http://www.itksnap.org/ ) . These annotations were defined as ground truths. The radiologists had access to the chest CT and surgical reports and evaluated the lesion characteristics including size, location, and edge. If > 50% of the edge of the nodule was traceable, the nodule was considered to have a “traceable edge”; if not, it was termed an “untraceable edge”.
Model development
We adopted the CNN architecture using segmentation method. The segmentation method outputs more information than the detection method (which present a bounding box) or the classification method (which determine the malignancy from a single image). Maximal diameter of the tumor is particularly important in clinical practice. Since the largest diameter of the tumor often coincides with an oblique direction, not the horizontal nor the vertical direction, it is difficult to measure with detection methods which present a bounding box. Our CNN architecture was based on the encoder-decoder architecture to output segmentation 17 . The encoder-decoder architecture has a bottleneck structure, which reduces the resolution of the feature map and improves the model robustness to noise and overfitting 18 .
In addition, one characteristic of this DL-based model is that it used both a normal chest radiograph and a black-and-white inversion of a chest radiograph. This is an augmentation that makes use of the experience of radiologists 19 . It is known that black-and-white inversion makes it easier to confirm the presence of lung lesions overlapping blind spots. We considered that this augmentation could be effective for this model as well, so we applied a CNN architecture to each of the normal and inverted images and then an ensemble model using these two architectures 20 . Supplementary Fig. S1 online shows detailed information of the model.
Using chest radiographs from the training dataset, the model was trained and validated from scratch, utilizing five-fold cross-validation. The model when the value of the loss function was the smallest within 100 epochs using Adam (learning rate = 0.001, beta_1 = 0.9, beta_2 = 0.999, epsilon = 0.00000001, decay = 0.0) was adopted as the best-performing.
Model assessment
A detection performance test was performed on a per-lesion basis using the test dataset to evaluate whether the model could identify malignant lesions on radiographs. The model calculated the probability of malignancy in a lesion detected on chest radiographs as an integer between 0 and 255. If the center of output generated by the model was within the ground truth, it was considered true positive (TP). All other outputs were FPs. When two or more TPs were proposed by the model for one ground truth, they were considered as one TP. If there was no output from the model for one ground truth, it was one FN. Two radiologists (A.S. and D.U.) retrospectively referred to the radiograph and CT to evaluate what structures were detected by the FP output. The dice coefficient was also used to evaluate segmentation performance.
Statistical analysis
In the detection performance test, metrics were evaluated on a per-lesion basis. We used the free-response receiver-operating characteristic (FROC) curve to evaluate whether the bounding boxes proposed by the model accurately identified malignant cancers in radiographs 21 . The vertical axis of the FROC curve is sensitivity and the horizontal axis is mFPI. Sensitivity is the number of TPs that the model was able to identify divided by the number of ground truths. The mFPI is the number of FPs that the model mistakenly presented divided by the number of radiographs in the dataset. Thus, the FROC curve shows sensitivity as a function of the number of FPs shown on the image.
One of the authors (D.U.) performed all analyses, using R version 3.6.0 ( https://www.r-project.org/ ). The FROC curves were plotted by R software. All statistical inferences were performed with two-sided 5% significance level.
Figure 1 shows a flowchart of the eligibility criteria for the chest radiographs. For the training dataset, 629 radiographs with 652 nodules/masses were collected from 629 patients (age range 40–91 years, mean age 70 ± 9.0 years, 221 women). For the test dataset, 151 radiographs with 159 nodules/masses were collected from 151 patients (age range 43–84 years, mean age 70 ± 9.0 years, 57 women) (Table 1 ).
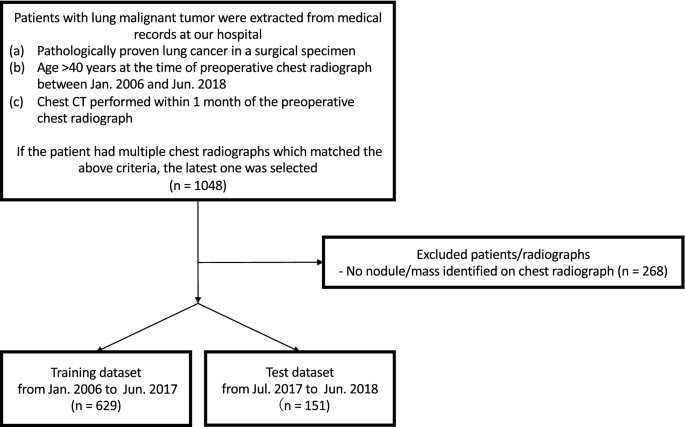
Flowchart of dataset selection.
The DL-based model had sensitivity of 0.73 with 0.13 mFPI in the test dataset (Table 2 ). The FROC curve is shown in Fig. 2 . The highest sensitivity the model attained was 1.00 for cancers with a diameter of 31–50 mm, and the second highest sensitivity was 0.85 for those with a diameter > 50 mm. For lung cancers that overlapped with blind spots such as the pulmonary apices, pulmonary hila, chest wall, heart, or sub-diaphragmatic space, sensitivity was 0.52, 0.64, 0.52, 0.56, and 0.50, respectively. The sensitivity of lesions with traceable edges on radiographs was 0.87, and that for untraceable edges was 0.21. Detailed results are shown in Table 2 .
The dice coefficient for all 159 lesions was on average 0.52 ± 0.37 (standard deviation, SD). For 116 lesions detected by the model, the dice coefficient was on average 0.71 ± 0.24 (SD). The dice coefficient for all 71 lesions overlapping blind spots was 0.34 ± 0.38 (SD). For 39 lesions detected by the model that overlapped with blind spots, the dice coefficient was 0.62 ± 0.29 (SD).
Of the 20 FPs, 19 could be identified as some kind of structure on the chest radiograph by radiologists (Table 3 ). In these 20 FPs, 13 overlapped with blind spots. There were 43 FNs, ranging in size from 9 to 72 mm (mean 21 ± 15 mm), 32 of which overlapped with blind spots (Table 4 ). There were four FNs > 50 mm, all of which overlapped with blind spots. Figure 3 shows representative cases of our model. Figure 4 shows overlapping of a FP output with normal anatomical structures and Fig. 5 shows a FN lung cancer that overlapped with a blind spot. Supplementary Fig. S2 online shows visualized images of the first and last layers. An ablation study to use black-and-white inversion images is shown in Supplementary Data online.
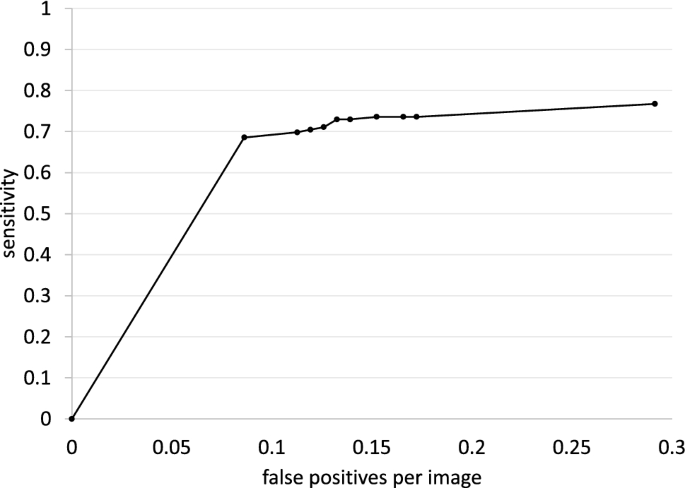
Free-response receiver-operating characteristic curve for the test dataset.
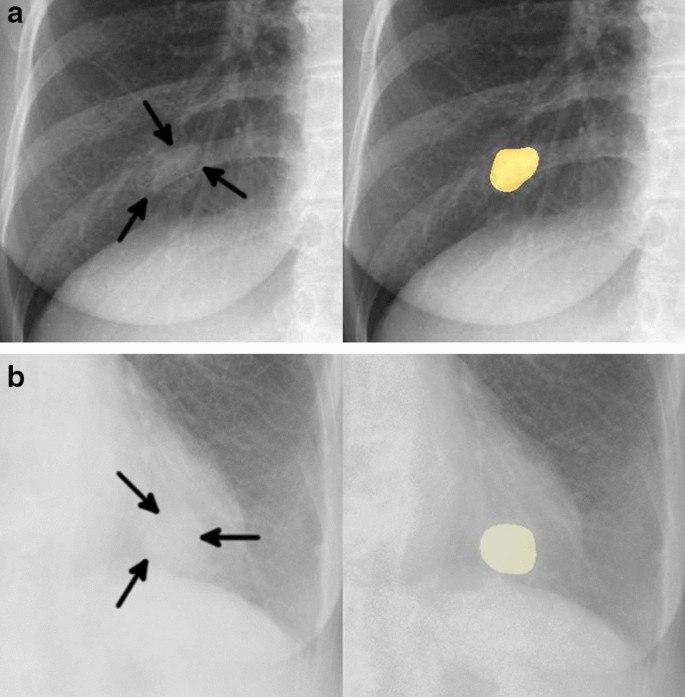
Two representative true positive cases. The images on the left are original images, and those on the right are images output by our model. ( a ) A 48-year-old woman with a nodule in the right lower lobe that was diagnosed as adenocarcinoma. The nodule was confused with rib and vessels (arrows). The model detected the nodule in the right middle lung field. ( b ) A 74-year-old woman with a nodule in the left lower lobe that was diagnosed as squamous cell carcinoma. The nodule overlapped with the heart (arrows). The lesion was identifiable by the model because its edges were traceable.
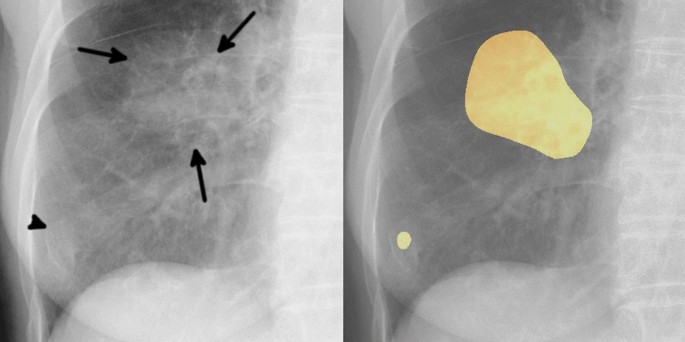
Example of one false positive case. The image on the left is an original image, and the image on the right is an image output by our model. An 81-year-old woman with a mass in the right lower lobe that was diagnosed as squamous cell carcinoma. The mass in the right middle lung field (arrows) was carcinoma. Our model detected this lesion, and also detected a slightly calcified nodule in the right lower lung field (arrowhead). This nodule was an old fracture of the right tenth rib, but was misidentified as a malignant lesion because its shape was obscured by overlap with the right eighth rib and breast.
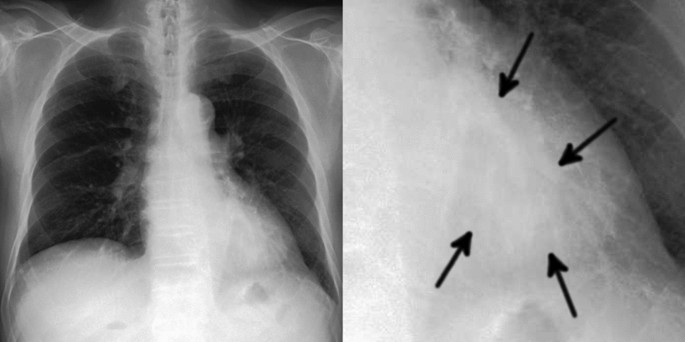
Example of one false negative case. The image on the left is a gross image, and the image on the right is an enlarged image of the lesion. A 68-year-old man with a mass in the left lower lobe that was diagnosed as adenocarcinoma. This lesion overlapped with the heart and is only faintly visible (arrows). Our model failed to detect the mass.
In this study, we developed a model for detecting lung cancer on chest radiographs and evaluated its performance. Adding pixel-level classification of lesions in the proposed DL-based model resulted in sensitivity of 0.73 with 0.13 mFPI in the test dataset.
To our knowledge, ours is the first study to use the segmentation method to detect pathologically proven lung cancer on chest radiographs. We found several studies that used classification or detection methods to detect lung cancer on chest radiographs, but not the segmentation method. Since the segmentation method has more information about the detected lesions than the classification or detection methods, it has advantages not only in the detection of lung cancer but also in follow-up and treatment efficacy. We achieved performance as high as that in similar previous studies 9 , 10 , 11 , 12 , 13 using DL-based lung nodule detection models, with fewer training data. It is particularly noteworthy that the present method achieved low mFPI. In previous studies, sensitivity and mFPI were 0.51–0.84 and 0.02–0.34, respectively, and used 3,500–13,326 radiographs with nodules or masses as the training data, compared with the 629 radiographs used in the present study. Although comparisons to these studies are difficult because the test datasets were different, our accuracy was similar to that of the detection models employed in most of the previous studies. We performed pixel-level classification of the lesions based on the segmentation method and included for analysis only lesions that were pathologically proven to be malignant, based on examination of surgically resected specimens. All previous studies 9 , 10 , 11 , 12 , 13 have included potentially benign lesions, clinically malignant lesions, or pathologically malignant lesions by biopsy in their training data. Therefore, our model may be able to analyze the features of the malignant lesions in more detail. In regard with the CNN, we created this model based on Inception-ResNet-v2 17 , which combines the Inception structure and the Residual connection. In the Inception-ResNet block, convolutional filters of multiple sizes are combined with residual connections. The use of residual connections not only avoids the degradation problem caused by deep structures but also reduces the training time. In theory, the combination of these features further improves the recognition accuracy and learning efficiency 17 . By using this model with combining normal and black-white-inversion images, our results achieved comparable or better performance with fewer training data than previous studies. In regard with the robustness of the model, we consider this model to be relatively robust against imaging conditions or body shape because we consecutively collected the dataset and did not set any exclusion criteria based on imaging conditions or body shape.
The dice coefficient for 159 malignant lesions was on average 0.52. On the other hand, for the 116 lesions detected by the model, the dice coefficient was on average 0.71. These values provide a benchmark for the segmentation performance of lung cancer on chest radiograph. The 71 lesions which overlapped with blind spots tended to have a low dice coefficient with an average of 0.34, but for 39 lesions detected by the model that overlapped with blind spots, the average dice coefficient was 0.62. This means that lesions overlapping blind spots were not only difficult to detect, but also had low accuracy in segmentation. On the other hand, the segmentation accuracy was relatively high for lesions that were detected by the model even if they overlapped with the blind spots.
Two interesting tendencies were found after retrospectively examining the characteristics of FP outputs. First, 95% (19/20) FPs could be visually recognized on chest radiographs as nodule/mass-like structures. The model identified some nodule-like structures (FPs), which overlapped with vascular shadows and ribs. This is also the case for radiologists in daily practice. Second, nodules with calcification overlapped with normal anatomical structures tended to be misdiagnosed by the model (FPs). Five FPs were non-malignant calcified lung nodules on CT and also overlapped with the heart, clavicle or ribs. As the model was trained only on malignant nodules without calcification in the training dataset, calcified nodules should not be identified in theory. Most calcified nodules are actually not identified by the model, however, this was not the case for calcified nodules that overlapped with normal anatomical structures. In other word, there is a possibility that the model could misidentify the lesion as a malignant if the features of calcification that should signal a benign lesion are masked by normal anatomical structures.
When we investigated FNs, we found that nodules in blind spots and metastatic nodules tended to be FNs. With regard to blind spots, our model showed a decrease in sensitivity for lesions that overlapped with normal anatomical structures. It was difficult for the model to identify lung cancers that overlapped with blind spots even when the tumor size was large (Fig. 5 ). In all FNs larger than 50 mm, there was wide overlap with normal anatomical structures, for the possible reason that it becomes difficult for the model to detect subtle density differences in lesions that overlapped with large structures such as the heart. With regard to metastatic nodules, 33% (14/43) metastatic lung cancers were FNs. These metastatic nodules ranged in size from 10 to 20 mm (mean 14 ± 3.8 mm) and were difficult to visually identify on radiographs, even with reference to CT. In fact, the radiologists had overlooked most of the small metastatic nodules at first and could only identify them retrospectively, with knowledge of the type of lung cancer and their locations.
There are some limitations of this study. The model was developed using a dataset collected from a single hospital. Although our model achieved high sensitivity with low FPs, the number of FPs may be higher in a screening cohort and the impact of this should be considered. Furthermore, an observer’s performance study is needed to evaluate the clinical utility of the model. In this study, we included only chest radiographs containing malignant nodules/masses. The fact that we used only pathologically proven lung cancers and pixel-level annotations by two radiologists in our dataset is a strength of our study, on the other hand, it may reduce the detection rate of benign nodules/masses. This is often not a problem in clinical practice. Technically, all areas other than the malignant nodules/masses could be trained as normal areas. However, normal images should be mixed in and tested to evaluate the model for detailed examination in clinical practice.
In conclusion, a DL-based model developed using the segmentation method showed high performance in the detection of lung cancer on chest radiographs. Compared with CT, chest radiographs have advantages in terms of accessibility, cost effectiveness, and low radiation dose. However, the known effectiveness of the model for lung cancer detection is limited. We believe that a CAD model with higher performance can support clinical detection and interpretation of malignant lesions on chest radiographs and offers additive value in lung cancer detection.
Bray, F. et al. Global cancer statistics 2018: GLOBOCAN estimates of incidence and mortality worldwide for 36 cancers in 185 countries. CA Cancer J. Clin. 68 , 394–424. https://doi.org/10.3322/caac.21492 (2018).
Article PubMed Google Scholar
Sagawa, M. et al. The efficacy of lung cancer screening conducted in 1990s: Four case–control studies in Japan. Lung Cancer 41 , 29–36. https://doi.org/10.1016/s0169-5002(03)00197-1 (2003).
Fontana, R. S. et al. Lung cancer screening: The Mayo program. J. Occup. Med. 28 , 746–750. https://doi.org/10.1097/00043764-198608000-00038 (1986).
Article CAS PubMed Google Scholar
Kubik, A. et al. Lack of benefit from semi-annual screening for cancer of the lung: Follow-up report of a randomized controlled trial on a population of high-risk males in Czechoslovakia. Int. J. Cancer 45 , 26–33. https://doi.org/10.1002/ijc.2910450107 (1990).
Raghu, V. K. et al. Feasibility of lung cancer prediction from low-dose CT scan and smoking factors using causal models. Thorax 74 , 643–649. https://doi.org/10.1136/thoraxjnl-2018-212638 (2019).
Hinton, G. Deep learning—a technology with the potential to transform health care. JAMA 320 , 1101–1102. https://doi.org/10.1001/jama.2018.11100 (2018).
LeCun, Y., Bengio, Y. & Hinton, G. Deep learning. Nature 521 , 436–444. https://doi.org/10.1038/nature14539 (2015).
Article ADS CAS PubMed Google Scholar
Ueda, D., Shimazaki, A. & Miki, Y. Technical and clinical overview of deep learning in radiology. Jpn. J. Radiol. 37 , 15–33. https://doi.org/10.1007/s11604-018-0795-3 (2019).
Nam, J. G. et al. Development and validation of deep learning–based automatic detection algorithm for malignant pulmonary nodules on chest radiographs. Radiology 290 , 218–228. https://doi.org/10.1148/radiol.2018180237 (2019).
Park, S. et al. Deep learning-based detection system for multiclass lesions on chest radiographs: Comparison with observer readings. Eur. Radiol. 30 , 1359–1368. https://doi.org/10.1007/s00330-019-06532-x (2020).
Yoo, H., Kim, K. H., Singh, R., Digumarthy, S. R. & Kalra, M. K. Validation of a deep learning algorithm for the detection of malignant pulmonary nodules in chest radiographs. JAMA Netw. Open 3 , e2017135. https://doi.org/10.1001/jamanetworkopen.2020.17135 (2020).
Article PubMed PubMed Central Google Scholar
Sim, Y. et al. Deep convolutional neural network–based software improves radiologist detection of malignant lung nodules on chest radiographs. Radiology 294 , 199–209. https://doi.org/10.1148/radiol.2019182465 (2020).
Hwang, E. J. et al. Development and validation of a deep learning-based automated detection algorithm for major thoracic diseases on chest radiographs. JAMA Netw. Open 2 , e191095. https://doi.org/10.1001/jamanetworkopen.2019.1095 (2019).
Manser, R. et al. Screening for lung cancer. Cochrane Database Syst Rev CD001991. https://doi.org/10.1002/14651858.CD001991.pub3 (2013).
Berlin, L. Radiologic errors, past, present and future. Diagnosis (Berl) 1 , 79–84. https://doi.org/10.1515/dx-2013-0012 (2014).
Article Google Scholar
From the RECIST committee. Schwartz, L.H. et al. RECIST 1.1-Update and clarification. Eur. J. Cancer. 62 , 132–137. https://doi.org/10.1016/j.ejca.2016.03.081 (2016).
Szegedy, C., Ioffe, S., Vanhoucke, V. & Alemi, A. A. Inception-v4, Inception-ResNet and the Impact of Residual Connections on Learning . In Proceedings of the Thirty-First AAAI Conference on Artificial Intelligence , 4278–4284 (AAAI Press, San Francisco, California, USA, 2017).
Matějka P. et al. Neural Network Bottleneck Features for Language Identification. In Proceedings of Odyssey 2014. vol. 2014. International Speech Communication Association , 299–304 (2014).
Sheline, M. E. et al. The diagnosis of pulmonary nodules: Comparison between standard and inverse digitized images and conventional chest radiographs. Am. J. Roentgenol. 152 (2), 261–263. https://doi.org/10.2214/ajr.152.2.261 (1989).
Article CAS Google Scholar
Wang, G., Hao, J., Ma, J. & Jiang, H. A comparative assessment of ensemble learning for credit scoring. Expert. Syst. Appl. 38 (1), 223–230 (2011).
Bunch, P., Hamilton, J., Sanderson, G. & Simmons, A. A free response approach to the measurement and characterization of radiographic observer performance. Proc. SPIE 127 , 124–135. https://doi.org/10.1117/12.955926 (1977).
Article ADS Google Scholar
Download references
Acknowledgements
We are grateful to LPIXEL Inc. for joining this study.
Author information
Authors and affiliations.
Department of Diagnostic and Interventional Radiology, Graduate School of Medicine, Osaka City University, Osaka, Japan
Akitoshi Shimazaki, Daiju Ueda, Akira Yamamoto, Takashi Honjo & Yukio Miki
Smart Life Science Lab, Center for Health Science Innovation, Osaka City University, Osaka, Japan
LPIXEL Inc, Tokyo, Japan
Antoine Choppin & Yuki Shimahara
You can also search for this author in PubMed Google Scholar
Contributions
All authors contributed to the study conception and design. Material preparation, data collection and analysis were performed by A.S., D.U., A.Y. and T.H. Model development was performed by A.C. and Y.S. The first draft of the manuscript was written by A.S. and all authors commented on previous versions of the manuscript. All authors read and approved the final manuscript.
Corresponding author
Correspondence to Daiju Ueda .
Ethics declarations
Competing interests.
Akitoshi Shimazaki has no relevant relationships to disclose. Daiju Ueda has no relevant relationships to disclose. Antoine Choppin is an employee of LPIXEL Inc. Akira Yamamoto has no relevant relationships to disclose. Takashi Honjo has no relevant relationships to disclose. Yuki Shimahara is the CEO of LPIXEL Inc. Yukio Miki has no relevant relationships to disclose.
Additional information
Publisher's note.
Springer Nature remains neutral with regard to jurisdictional claims in published maps and institutional affiliations.
Supplementary Information
Supplementary information., rights and permissions.
Open Access This article is licensed under a Creative Commons Attribution 4.0 International License, which permits use, sharing, adaptation, distribution and reproduction in any medium or format, as long as you give appropriate credit to the original author(s) and the source, provide a link to the Creative Commons licence, and indicate if changes were made. The images or other third party material in this article are included in the article's Creative Commons licence, unless indicated otherwise in a credit line to the material. If material is not included in the article's Creative Commons licence and your intended use is not permitted by statutory regulation or exceeds the permitted use, you will need to obtain permission directly from the copyright holder. To view a copy of this licence, visit http://creativecommons.org/licenses/by/4.0/ .
Reprints and permissions
About this article
Cite this article.
Shimazaki, A., Ueda, D., Choppin, A. et al. Deep learning-based algorithm for lung cancer detection on chest radiographs using the segmentation method. Sci Rep 12 , 727 (2022). https://doi.org/10.1038/s41598-021-04667-w
Download citation
Received : 04 August 2021
Accepted : 29 December 2021
Published : 14 January 2022
DOI : https://doi.org/10.1038/s41598-021-04667-w
Share this article
Anyone you share the following link with will be able to read this content:
Sorry, a shareable link is not currently available for this article.
Provided by the Springer Nature SharedIt content-sharing initiative
This article is cited by
Deep volcanic residual u-net for nodal metastasis (nmet) identification from lung cancer.
- M. Ramkumar
- K. Kalirajan
Biomedical Engineering Letters (2024)
Fairness of artificial intelligence in healthcare: review and recommendations
- Taichi Kakinuma
- Shinji Naganawa
Japanese Journal of Radiology (2024)
Lung tumor analysis using a thrice novelty block classification approach
- S. L. Soniya
- T. Ajith Bosco Raj
Signal, Image and Video Processing (2023)
Radon transform-based improved single seeded region growing segmentation for lung cancer detection using AMPWSVM classification approach
- K. Vijila Rani
- M. Eugine Prince
An efficient transfer learning approach for prediction and classification of SARS – COVID -19
- Krishna Kumar Joshi
- Kamlesh Gupta
- Jitendra Agrawal
Multimedia Tools and Applications (2023)
By submitting a comment you agree to abide by our Terms and Community Guidelines . If you find something abusive or that does not comply with our terms or guidelines please flag it as inappropriate.
Quick links
- Explore articles by subject
- Guide to authors
- Editorial policies
Sign up for the Nature Briefing: Cancer newsletter — what matters in cancer research, free to your inbox weekly.

EDITORIAL article
Editorial: lung adenocarcinoma: from genomics to immunotherapy.
- 1 Department of Central Laboratory, Cancer Hospital of Dalian University of Technology, Liaoning Cancer Hospital and Institute, Shenyang, China
- 2 Cancer Genomics and Systems Biology Lab, Department of Medical Biotechnologies, University of Siena, Siena, Italy
- 3 Department of Medical Biotechnologies, Med Biotech Hub and Competence Centre, University of Siena, Siena, Italy
Editorial on the Research Topic Lung adenocarcinoma: from genomics to immunotherapy
Introduction
Lung cancer is the second most common type of cancer and is the leading cause of cancer death globally. In 2018, almost 2.1 million new cases were diagnosed, accounting for ∼12% of the cancer burden worldwide ( Sung et al., 2021 ). The malignant stage of lung cancer is known as lung adenocarcinoma, which is the most common and is diagnosed in both smokers and non-smokers.
There are two main types of lung cancer, the non-small cell lung cancer (NSCLC) and the small cell lung cancer (SCLC). Genomic studies have indicated that more than 80% of lung malignancies are classified as NSCLC, of which adenocarcinoma is the predominant subtype. In metastatic patients, although significant progress has been made for tumors harboring druggable mutations such as EGFR, the majority of those is lacking of such mutations and the prognosis remains poor. Platinum doublet chemotherapy has been the mainstay first-line treatment for patients who are diagnosed with metastatic lung adenocarcinoma without a targetable mutation ( Bodor et al., 2018 ).
In recent years, immunotherapy has emerged as a treatment option that has shown a strong response in a subset of patients. The immune agents block crucial checkpoints and regulate the immune response, but the tumor cells evade the patient’s immune system. By blocking these receptor–ligand interactions, a particular subset of T cells is activated to recognize and respond to tumor cells. While such responses to immunotherapy are promising, they have only been effective in ∼20% of patients ( Murciano-Goroff et al., 2020 ).
Therefore, there is an urgent need to understand the underlying mechanism of lung adenocarcinoma from genome to immunotherapy. To address this unmet need, this Research Topic will focus on advancements related to lung adenocarcinoma (LUAD) and the identification of novel biomarkers as new therapy-determining or companion prognostic tools for the development of precise mechanism-based treatments.
Novel prognostic biomarkers for lung adenocarcinoma
The original articles published in the present Research Topic updated about novel prognostic biomarkers in lung adenocarcinoma patients through in silico approaches. In particular, Wang et al. F’s group assessed the roles of unlocking phenotypic plasticity (UPP) in immune status, prognosis, and treatment in patients with LUAD based on the cancer genome atlas (TCGA) database ( https://www.frontiersin.org/journals/genetics/articles/10.3389/fgene.2022.941567/full ). They proposed UPP as a new and reliable prognosis indicator to predict the patient’s overall survival and help the clinician to predict therapeutic responses and make individualized treatment plans.
Similarly, Zhou X et al. investigated the expression of indolethylamine N-methyltransferase (INMT) and its clinical value as a prognostic biomarker in LUAD based on TCGA and Gene Expression Omnibus (GEO) databases ( https://www.frontiersin.org/journals/genetics/articles/10.3389/fgene.2022.946848/full ). They found that INMT expression was significantly downregulated in LUAD, and the low expression of INMT was associated with poor prognosis but favorable immunotherapy response in LUAD.
Song Y et al. highlighted the association of necroptosis with LUAD and its potential use in guiding immunotherapy based on transcriptomic and clinical data of patients from TCGA and GEO databases ( https://www.frontiersin.org/journals/genetics/articles/10.3389/fgene.2022.1027741/full ). They analyzed 902 samples and identified a prognostic signature of five necroptosis-related genes that could be used to predict the prognosis of LUAD patients.
Additionally, Zhu X’s group focused their attention on the role of basement membranes (BMs) and their related genes for prognosis prediction in LUAD patients from TCGA and GEO databases ( https://www.frontiersin.org/journals/genetics/articles/10.3389/fgene.2023.1100560/full ). They used a training set of data and a verification cohort and identified a prognostic signature of ten BM-associated genes that could be used to predict the prognosis of LUAD patients and guide personalized treatment.
Zhang et al. investigated the relationship between cuproptosis and long non-coding RNAs (lncRNAs) in carcinogenesis and prognosis/treatment of LUAD patients based on transcriptomic data of 507 samples from TCGA database ( https://www.frontiersin.org/journals/pharmacology/articles/10.3389/fphar.2023.1236655/full ). They constructed a prognostic model associated with the prognosis of patients with LUAD undergoing therapy and confirmed their results through in-vitro experiments.
Finally, Liu R’s group has dedicated its work to studying the correlation between neutrophils and tumor development in LUAD based on data from the TCGA database and in-vitro experiments, identifying 30 hub genes that were significantly associated with neutrophil infiltration and developing a neutrophil scoring system associated with prognosis, and tumor immune microenvironment.
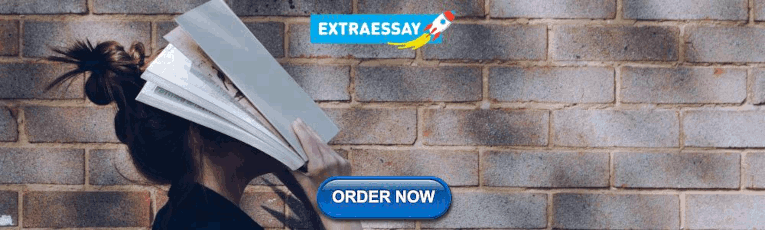
Relevant case reports
The present Research Topic also contains interesting, unusual, and noteworthy case reports that can help clinicians and scientists identify new trends, evaluate new therapeutic effects, as well as create new research questions. In particular, Hodges A et al. presented a 62-year female with Lynch syndrome, who developed an EGFR-positive lung adenocarcinoma highlighting the complex interplay of genetic cancer predisposition syndromes and the development of spontaneous driver mutations in the disease course and the subsequent management of tumors arising ( https://www.frontiersin.org/journals/oncology/articles/10.3389/fonc.2023.1193503/full ).
Li H et al. presented a 35-year female with a rare lung cancer exhibiting choriocarcinoma features demonstrating the potential of chemo-immunotherapy in treating this aggressive subtype of lung cancer ( https://www.frontiersin.org/journals/oncology/articles/10.3389/fonc.2024.1324057/abstract ).
Last but not least, Quanqing L et al. presented a 67-year female with a squamous cell carcinoma (NSCLC) that transforms into small cell carcinoma (SCLC) after five cycles of immunotherapy targeting PD-1 treatment (Sintilimab) of NSCLC ( https://www.frontiersin.org/journals/oncology/articles/10.3389/fonc.2024.1329152/full ). This histological transformation could represent a potential mechanism of cancer therapeutic resistance.
In conclusion, this Research Topic highlights the importance of good prognostic biomarkers in determining the most effective treatment and revolutionizing cancer precision medicine. The Research Topic of articles provides a comprehensive overview of current advancements in prognostic and therapeutic lung cancer biomarkers offering a substantive framework that informs ongoing scientific inquiry and clinical practice, aiming to improve the understanding and management of LUAD patients.
Author contributions
YM: Conceptualization, Validation, Writing–original draft, Writing–review and editing. MP: Conceptualization, Data curation, Validation, Writing–original draft, Writing–review and editing. EF: Conceptualization, Data curation, Supervision, Writing–original draft, Writing–review and editing.
The author(s) declare that no financial support was received for the research, authorship, and/or publication of this article.
Acknowledgments
We deeply thank all the authors and reviewers who have participated in this Research Topic.
Conflict of interest
The authors declare that the research was conducted in the absence of any commercial or financial relationships that could be construed as a potential conflict of interest.
The author(s) declared that they were an editorial board member of Frontiers, at the time of submission. This had no impact on the peer review process and the final decision.
Publisher’s note
All claims expressed in this article are solely those of the authors and do not necessarily represent those of their affiliated organizations, or those of the publisher, the editors and the reviewers. Any product that may be evaluated in this article, or claim that may be made by its manufacturer, is not guaranteed or endorsed by the publisher.
Bodor, J. N., Kasireddy, V., and Borghaei, H. (2018). First-line therapies for metastatic lung adenocarcinoma without a driver mutation. J. Oncol. Pract. 14 (9), 529–535. doi:10.1200/JOP.18.00250
PubMed Abstract | CrossRef Full Text | Google Scholar
Murciano-Goroff, Y. R., Warner, A. B., and Wolchok, J. D. (2020). The future of cancer immunotherapy: microenvironment-targeting combinations. Cell Res. 30 (6), 507–519. doi:10.1038/s41422-020-0337-2
Sung, H., Ferlay, J., Siegel, R. L., Laversanne, M., Soerjomataram, I., Jemal, A., et al. (2021). Global cancer statistics 2020: GLOBOCAN estimates of incidence and mortality worldwide for 36 cancers in 185 countries. Ca. Cancer J. Clin. 71 (3), 209–249. doi:10.3322/caac.21660
Keywords: solid cancer, cancer biomarkers, cancer prognosis, disease monitoring, lung cancer, precision medicine
Citation: Meng Y, Palmieri M and Frullanti E (2024) Editorial: Lung adenocarcinoma: from genomics to immunotherapy. Front. Genet. 15:1399127. doi: 10.3389/fgene.2024.1399127
Received: 11 March 2024; Accepted: 27 March 2024; Published: 09 April 2024.
Edited and reviewed by:
Copyright © 2024 Meng, Palmieri and Frullanti. This is an open-access article distributed under the terms of the Creative Commons Attribution License (CC BY). The use, distribution or reproduction in other forums is permitted, provided the original author(s) and the copyright owner(s) are credited and that the original publication in this journal is cited, in accordance with accepted academic practice. No use, distribution or reproduction is permitted which does not comply with these terms.
*Correspondence: Elisa Frullanti, [email protected]
† These authors share last authorship
Disclaimer: All claims expressed in this article are solely those of the authors and do not necessarily represent those of their affiliated organizations, or those of the publisher, the editors and the reviewers. Any product that may be evaluated in this article or claim that may be made by its manufacturer is not guaranteed or endorsed by the publisher.
- Help & Support
Stopping Lung Cancer Before It Progresses
by Vince Tedjasaputra, Ph.D. | April 17, 2024
- Lung Cancer
Lung cancer is still the leading cause of death by cancer in the U.S., with someone diagnosed about every two minutes. To address this, the American Lung Association has renewed a partnership with LUNGevity to fund innovative research to intercept lung cancer—that is, detecting and treating pre-cancerous cells before they have a chance to become malignant.
Accelerating Innovation
This partnership is not a typical research endeavor; it is part of the American Lung Association Research Institute’s new Accelerator Program , which seeks to form collaborations with government, industry and other non-profit organizations like LUNGevity who have a shared mission to improve lung health.
"Early detection of lung cancer is crucial to saving lives,” says Harold Wimmer, President and CEO of the American Lung Association, stressing how critical this partnership is. “By investing in research to intercept lung cancer at its earliest stages, we have the potential to revolutionize how we approach this disease and improve outcomes for patients."
Leading the Team
Heading this ambitious initiative are Dr. Avrum Spira , Boston Medical Center, and Dr. Steven Dubinett University of California, Los Angeles, who are esteemed leaders in the field of lung cancer research. Their collaborative project, titled “Intercept Lung Cancer Through Immune, Imaging & Molecular Evaluation In TIME,” seeks to define how lung cancer progresses.
By utilizing cutting-edge technologies like robot-assisted bronchoscopy, they aim to establish a timeline of pre-cancerous cell evolution into malignant cancer—a pivotal step toward early detection and intervention.
"This project represents an evolution of our ongoing efforts to understand and intercept lung cancer before it progresses,” reflects Dr. Spira. “By unraveling the molecular and immune mechanisms underlying lung cancer development, we can develop targeted strategies for early detection and intervention."
Continuing the Legacy of Impactful Lung Cancer Research
The partnership builds on a successful previous collaborative effort, which included research funded by the American Lung Association, LUNGevity Foundation and Stand Up To Cancer. This collaboration has already yielded significant findings, from mapping the pre-cancer genome to identifying enzymes and proteins associated with pre-malignant cells.
Here are a few of the recent breakthrough findings published by the Lung Cancer Interception Team:
- Unlocking Mechanisms of Cancer Treatment Resistance : The discovery of an enzyme called APOBEC3B, that influences how lung cancer develops resistance to EGFR-targeted therapy, which holds the promise of enhancing treatment effectiveness ( Nature Genetics, December 2023 ).
- Modeling how cancer cells change their DNA : A novel computational method allows scientists to study how cancer cells change their DNA, leading to innovations that can improve lung cancer treatments ( PLOS Computational Biology, October 2023 ).
- Insights into Immune Evasion : A comprehensive review which sheds light on how cancer cells evade detection by immune systems, providing a roadmap for the development of novel immunotherapeutic strategies ( Immunity, October 2023 ).
- Targeting Pre-Cancerous Cells : Identification of important gene regulation and function in early stages of lung cancer development paves the way for targeted treatments ( Journal of Experimental and Clinical Cancer Research, May 2023 ).
- Precision in Metastasis Detection : Establishment of the timing and patterns of metastasis in non-small cell lung cancer guides tissue sampling strategies, leading to improved therapies and patient outcomes ( Nature, April 2023 ).
To read more about each of these groundbreaking studies and others published by the Lung Cancer Interception Team, visit our Research News page, which provides easy-to-understand summaries of most all Lung Association-funded research studies.
Sustaining Momentum in Lung Cancer Research
The insights gained from the Lung Cancer Interception Team’s previous research lay the groundwork for the innovative approaches and discoveries that will define the next phase of lung cancer interception. That is why this renewed partnership is notable, as Drs. Spira and Dubinett’s team have gained serious momentum from their prior work.
"Our past achievements serve as a springboard for future success. By building upon the foundation laid by previous research, we can push the boundaries of what's possible in lung cancer interception,” says Dr. Spira. “Together, we are charting a course toward a future where lung cancer is intercepted, not just treated."
To learn more about the incredible research being conducted by the Lung Cancer Interception Team, visit lung.org/intercept-cancer .
If you or a loved one are looking for support or more information about lung cancer, visit lung.org/lung-cancer .
Related Blogs
April 17, 2024
“It’s So Important to Never Miss a Scan”
April 2, 2024
Spreading the Word about Lung Cancer, One Story at a Time
February 26, 2024
Blog last updated: April 17, 2024
Triple Your Impact
Donate this Giving Week to honor 120 years of advancing lung health and saving lives. Your donation will make more lifesaving milestones possible.
Make a Donation
Your tax-deductible donation funds lung disease and lung cancer research, new treatments, lung health education, and more.
Become a Lung Health Insider
Join over 700,000 people who receive the latest news about lung health, including research, lung disease, air quality, quitting tobacco, inspiring stories and more!
Thank you! You will now receive email updates from the American Lung Association.
Select Your Location
Select your location to view local American Lung Association events and news near you.
Change Language
Lung helpline.
Talk to our lung health experts at the American Lung Association. Our service is free and we are here to help you.
1-800-LUNG-USA
(1-800-586-4872)

An official website of the United States government
The .gov means it’s official. Federal government websites often end in .gov or .mil. Before sharing sensitive information, make sure you’re on a federal government site.
The site is secure. The https:// ensures that you are connecting to the official website and that any information you provide is encrypted and transmitted securely.
- Publications
- Account settings
Preview improvements coming to the PMC website in October 2024. Learn More or Try it out now .
- Advanced Search
- Journal List
- HHS Author Manuscripts

COMPLICATED GRIEF SYMPTOMS IN CAREGIVERS OF PERSONS WITH LUNG CANCER: THE ROLE OF FAMILY CONFLICT, INTRAPSYCHIC STRAINS, AND HOSPICE UTILIZATION *
Guided by a stress process conceptual model, this study examines social and psychological determinants of complicated grief symptoms focusing on family conflict, intrapsychic strains, and the potential moderating effect of care quality and hospice utilization. Relying on data from 152 spouse and adult child lung cancer caregiver survey respondents, drawn from an ancillary study of the Assessment of Cancer CarE and SatiSfaction (ACCESS) in Wisconsin, hierarchical multiple regression analysis was used to examine determinants of complicated grief. After controlling for contextual factors and time since death, complicated grief symptoms were higher among care-givers with less education, among families with lower prior conflict but higher conflict at the end-of-life, who had family members who had difficulty accepting the illness, and who were caring for patients with greater fear of death. Additionally, hospice utilization moderated the effect of fear of death on complicated grief. Findings suggest that family conflict, intrapsychic strains, and hospice utilization may help to explain the variability found in complicated grief symptoms among bereaved caregivers. Implications for enhancing complicated grief assessment tools and preventative interventions across the continuum of cancer care are highlighted.
INTRODUCTION
Every year approximately 160,000 persons, primarily elders, die from lung cancer; the leading cause of cancer mortality in the United States ( American Cancer Society, 2009 ). Given the rapid deteriorating course of illness, prevalence of uncontrolled symptoms ( Griffin, Koch, Nelson, & Cooley, 2007 ), and stigma associated with lung cancer that is reported by smokers and non-smokers ( Chapple, Ziebland, & McPherson, 2004 ), it is not surprising that these families report high levels of distress during the dying process ( Cameron, Franche, Cheung, & Stewart, 2002 ). Compared to the general population, significant others of lung cancer patients score lower on psychological well-being and experience a decline in family functioning over the course of illness that continues after the death ( Persson, Östlund, Wennman-Larsen, Wengström, & Gustavsson, 2008 ). Little research has been done on how family conflict and experiences during treatment and at the end-of-life may influence the bereavement outcomes of family caregivers.
There is tremendous variability in how individuals adapt following the death of a family member; the vast majority of whom are adequately supported, and do not benefit from intervention ( Jordan & Neimeyer, 2003 ). Given the high risk of suicide and other adverse mental and physical health outcomes ( Latham & Prigerson, 2004 ), understanding what contributes to complicated grief symptomatology is necessary to identify caregivers at risk and to develop relevant interventions. Preliminary evidence suggests that family conflict and intrapsychic strains at the end-of-life that are commonly experienced by family members of persons with lung cancer ( Cameron et al., 2002 ), may contribute to bereavement adjustment difficulties ( Kramer, Boelk, & Auer, 2006 ). The purpose of this article was to examine the predictors of complicated grief symptoms focusing on the role of family conflict, intrapsychic strains, and the potential moderating effect of care quality and hospice utilization.
Theoretical Framework
Stress process theories have been influential in informing an extensive body of research on the impact of family caregiving ( Kramer & Vitaliano, 1994 ), and more recently caregiving and bereavement outcomes ( Burton, Haley, Small, Finley, Dillinger-Vasille, & Schonwetter, 2008 ). Aside from background and contextual considerations, the stress process model is made up of four primary domains: primary stressors, secondary stressors, moderators, and outcomes ( Aneshensel, Pearlin, Mullan, Zarit, & Whitlatch, 1995 ; Pearlin, Mullan, Semple, & Skaff, 1990 ).
Primary Stressors
Patient clinical care needs.
Primary stressors are the difficult circumstances experienced by family care-givers that stem from the patient’s care needs ( Pearlin et al., 1990 ). Lung cancer patients have a high prevalence of uncontrolled distressing physical symptoms; the most common are pain, shortness of breath, nausea or vomiting, and fatigue ( Griffin et al., 2007 ). These symptoms exacerbate co-occurring psychological symptoms such as patient depression and anxiety ( Portenoy, Payne, & Jacobson, 1999 ), and family distress ( McMillan & Small, 2007 ). Caregivers experience better bereavement adjustment when these symptoms are minimized and quality of life is enhanced ( Grande, Farquhar, & Barclay, 2004 ).
Secondary Stressors
Role strains.
“Secondary stressors are the ways that primary stressors influence and disrupt other areas of the caregiver’s life” ( Gustavsson & Dal Santo, 2008 , p. 292), and are associated with worse bereavement outcomes ( Bernard & Guarnaccia, 2003 ). Pearlin and colleagues (1990) identified family conflict as a central role strain that may arise in caregiving families. Based on prior research, suggesting that greater bereavement distress among family caregivers is more likely among families in conflict ( Kramer et al., 2006 ) and who have poorer family functioning ( Kissane, Bloch, Miach, Smith, Seddon, & Keks, 1997 ), it is hypothesized that family conflict will be associated with more complicated grief symptoms.
Intrapsychic Strains
Intrapsychic strains are psychological difficulties occurring within the mind that may be influenced by primary stressors. Two intrapsychic strains that may be particularly relevant to lung cancer caregivers are difficulty accepting a highly stigmatized illness and the patient’s fear of death, that is distressing to witness ( Chapple et al., 2004 ). “Acceptance” has been put forth as an essential component of illness adjustment, and a growing body of literature has focused on the correlates of acceptance among patients with acute and chronic heath conditions ( Stuifbergen, Becker, Blozis, & Beal, 2008 ). Grieving may be hampered when one has difficulty accepting illness and related feelings of loss ( Prigerson & Maciejewski, 2008 ). Although an extensive body of literature has developed investigating the predictors and outcomes of death anxiety among elders, the seriously ill and professional caregivers ( Neimeyer, Wittkowski, & Moser, 2004 ), and personal fear of death as associated with bereavement outcomes ( Barr & Cacciatore, 2008 ), little attention has been given to how the patient’s fear of death might influence caregiver bereavement. Compared to other cancer patient populations, lung cancer patients exhibit the highest rates of psychological disorders, and existential distress is elevated closer to death ( Lichtenthal, Nilsson, Zhang, Trice, Kissane, & Prigerson, 2009 ). Emotional distress is higher among caregivers of patients perceived to have death anxiety ( Morita, Akechi, Ikenaga, Inoue, Kohara, Matsubara, et al., 2007 ). It is hypothesized that difficulty accepting the illness and patient fear of death will contribute to complicated grief symptoms.
Resources are the various supports that one has to manage stressful experiences and these are thought to moderate the relationship between stressors and outcomes ( Pearlin et al., 1990 ). Two resources that are particularly salient to the needs of lung cancer patients and families are quality of care and hospice utilization. Better care quality is associated with higher quality of life for care-givers of patients with lung cancer ( Ryan, Howell, Jones, & Hardy, 2008 ), and bereaved family members who are adequately supported by health care providers experience lower levels of psychiatric illness following hospital deaths ( Siegel, Hayes, Vanderwerker, Loseth, & Prigerson, 2008 ). Support received prior to death, is a stronger predictor of bereavement adjustment than support received after death ( Bass, Bowman, & Noelker, 1991 ), and perceptions of care quality are associated with better bereavement adjustment ( Grande et al., 2004 ).
Hospice programs are specifically designed to provide end-of-life care in order to manage distressing symptoms, maximize patient quality of life, address needs of family, and is touted as the optimal care model for patients with advanced lung cancer ( Griffin, Koch, Ackerman, & Cole, 2003 ). Family members report greater satisfaction with hospice care than care in other settings ( Yancey, Greger, & Coburn, 1990 ), and improved family well-being and functioning ( Godkin, Krant, & Doster, 1983–84 ). Findings regarding hospice utilization and the influence on bereavement outcomes are mixed. Some find utilization to have a positive influence ( Christakis & Iwashyna, 2003 ; Steele, 1990 ), but others report no relationship ( Grande et al., 2004 ; Yancey, Greger, & Coburn, 1990 ). According to the stress process model, we hypothesize that the impact of family conflict and intrapsychic strains on complicated grief symptoms will be intensified when resources are low and diminished when they are plentiful.
Complicated Grief Symptoms
Salient outcomes of the stress process include psychological well-being indicators related to the individual’s “ability to sustain themselves in their social roles” ( Pearlin et al., 1990 , p. 590). Complicated grief symptoms interfere with daily life and the fulfillment of social roles ( Prigerson, Maciejewski, Reynolds, Bierhals, Newsom, Fasiczka, et al., 1995 ) and are experienced by 10%–20% of the population ( Prigerson & Jacobs, 2001 ). Although there are important features that distinguish it from bereavement related depression, anxiety and Posttraumatic Stress Disorder (PTSD) ( Zhang, El-Jawahri, & Prigerson, 2006 ), one study reports as many as 75% of complicated grievers also meet criteria for PTSD ( Tolstikova, Fleming, & Chartier, 2005 ). Given that complicated grievers experience intense, persistent emotional and functional impairment ( Schulz, Boerner, Shear, Zhang, & Gitlin, 2006 ), identifying the contributing factors of this particular outcome is an important goal of bereavement research.
Background and Context—Control Variables
Pearlin et al. (1990 , p. 585) propose that the effects of “ascribed statuses, such as age, gender, ethnicity, along with education … and economic attainments are expected to be threaded throughout the entire stress process,” influence the primary and secondary stressors individuals are exposed to, the resources they have access to, and the outcomes they experience. As such it is important to control for these and other contextual variables. Other contextual variables include advanced care planning and the family context. Among several forms of advanced care planning, discussions with significant others is considered an essential component of the planning process ( Hammes & Rooney, 1998 ). Stress and post-death depression and anxiety among relatives may be reduced when caregivers know the patient’s preferences for care ( Detering, Hancock, Reade, & Silvester, 2010 ; Tilden, Tolle, Garland, & Nelson, 1995 ), and evidence suggests that “end-of-life discussions may have cascading benefits for patients and their caregivers” including better caregiver bereavement adjustment ( Wright, Zhang, Ray, Mack, Trice, Balboni, et al., 2008 , p. 1670). Although little attention has been given to the family context, higher bereavement risk was found among those with young children at home ( Beckwith, Beckwith, Gray, & Micsko, 1990 ) and a history of conflict was associated with guilt following death which negatively effects bereavement adaptation ( Mullan, 1992 ). In addition, a history of conflict is a strong predictor of family conflict at the end-of-life ( Kramer, Kavanaugh, Trentham-Dietz, Walsh, & Yonker, 2009 ), and needs to be taken into consideration in order to understand how conflict at the end-of-life might influence bereavement outcomes.
This study will identify and control for the background and contextual variables that are significantly associated with complicated grief among care-givers of deceased lung cancer patients and will test the following two hypotheses, which were generated from this review of the literature and the stress process model.
Hypothesis 1: After controlling for time since death, history of family conflict, and other contextual and primary stressor variables, secondary stressors (i.e., family conflict at the end-of-life, family member difficulty accepting the illness, and patient fear of death) will significantly predict complicated grief symptoms.
Hypothesis 2: The quality of care provided to the patient and family and hospice utilization will moderate the effect of secondary stressors on complicated grief symptoms.
Data Sources
The results reported here are drawn from an ancillary mail survey of family members of persons who died with lung cancer, drawn from the larger Assessment of Cancer CarE and SatiSfaction (ACCESS) study conducted in the state of Wisconsin. Sampling and survey methods from the ACCESS and ancillary lung cancer family study are described in detail by Trentham-Dietz and Walsh (2008) and Kramer et al. (2009) respectively. In brief, of 358 primary family caregivers of persons who died with lung cancer, who were identified in a statewide registry and who had contact information available, 205 (57%) agreed to receive the study questionnaire, and 169 returned surveys (82% of those who received the survey; 47% of total contacted). Consistent with the national profile of informal end-of-life caregivers ( Wolff, Dy, Frick, & Kasper, 2007 ), the majority, 158 (93%) of respondents were spouses ( n = 141) or adult children ( n = 17). The analytic sample is confined to 152 of these respondents. These included 148 who answered all of the complicated grief items, and four others who had completed 50% or more of the items. In these cases we used the person mean substitution method ( Downey & King, 1998 ) found to be the optimal technique for imputing missing data when half of the items are present ( Hawthorne & Elliott, 2005 ). The time between death and completion of the surveys averaged 19 months ( SD = 7.37), and ranged from .9 to 34 months.
Dependent Variable—Complicated Grief
In 1995 the Inventory of Complicated Grief (ICG) was introduced as the first measure to assess maladaptive grief symptoms ( Prigerson et al., 1995 ), and has since been well validated and widely used. The ICG-revised (ICG-R) is a more contemporary version of the ICG further refined by an expert consensus panel ( Prigerson & Jacobs, 2001 ). Using 5-point scales to represent increasing levels of symptom severity respondents rated nine items addressing symptoms of yearning and longing for the deceased, trouble accepting the death, difficulty trusting people, bitterness over the death, inability to move forward, sense of emotional numbness, sense of edginess, feeling the future holds no prospect for fulfillment without the deceased, and feeling life is empty or meaningless without the deceased. This measure is highly correlated with clinical assessments of complicated grief and distinguishes complicated grief from typical grief symptoms in terms of the prediction of morbidity, persistence and intensity ( Prigerson & Maciejewski, 2005 ). A mean score was calculated. Cronbach’s α was .90.
Two measures of patient clinical care needs were assessed. An index of four common physical symptoms associated with lung cancer was assessed by asking: “Did your family member have: a) pain; b) shortness of breath; c) nausea or vomiting; or d) severe tiredness or fatigue during or after any of his/her cancer treatments?” Cronbach’s α was .66. A psychological symptoms index was assessed by asking “Did your family member experience: a) loss of hope or depression; and/or b) anxiety during or after any of his/her cancer treatments?” Cronbach’s α was .57.
Role strains
A four-item Family Conflict at the End-of Life (FC-EOL) scale was developed for this study, based on findings from a prior qualitative study ( Kramer et al., 2006 ), a literature review, and prior clinical experience. Respondents were asked to use a 5-point scale (1 = not at all to 5 = very much ) to answer the following four questions “As you think about the decisions that your family member and the rest of the family faced near the end-of-life, how much did any family members: Disagree or argue with one another? Feel resentment toward one another? Feel anger toward one another? Insult or yell at one another?” A mean score for family conflict was calculated. Cronbach’s α was .93; item to total correlations ranged from .72 to .88.
Intrapsychic strains
Family difficulty accepting the illness was assessed with response to the question “How much did any family members find it hard to accept the illness?” using a 5-point continuum to indicate the degree of difficulty (0 = not at all to 4 = very much ). Patient fear of death was measured by a 5-point rating of agreement (1 = strongly disagree to 5 = strongly agree ) with the statement “After his/her lung cancer diagnosis, my family member was afraid to die.”
Moderators—Resources
Patient quality of care was assessed using nine items adapted from the Toolkit of Instruments to Measure End-of-Life Care ( Teno, 2004 ). Using a 5-point response set (0 = not at all to 5 = very much ), respondents rated the extent to which the patient was treated with kindness and respect, and their physical, psychological, spiritual, and informational needs were adequately addressed. Cronbach’s α was .86. Family quality of care was assessed using five items adapted from the Toolkit of Instruments ( Teno, 2004 ) to rate family care quality. Respondents rated the extent to which the treatment staff included them in decisions, were sensitive to their feelings, paid attention to their wishes for care, and were helpful in explaining the patient’s condition, using a 5-point response set (0 = not at all to 5 = very much ). Cronbach’s α was .84. Hospice utilization was determined with the question “Was hospice involved in the medical care of your family member?” (0 = no ; 1 = yes ).
Background and Context
Demographic characteristics included sociodemographic variables such as age (continuous variable that was dichotomized; 0 = under 65 ; 1 = 65 or over ), gender (0 = male ; 1 = female ), race (0 = Non-Hispanic White ; 1 = Black ), income (four income categories included less than $12,000, $15,000–$29,999, $30,000–$49,999, and $50,000+), education (two categories that include high school or less, and some college or advanced degree), and relationship to the deceased (0 = adult child ; 1 = spouse ). Care preferences specified was ascertained by asking: “Did your family member have specific wishes or plans about the types of medical treatment he/she wanted while dying?” (0 = no ; 1 = yes ). Regarding family context we assessed presence of children in the home (0 = no ; 1 = yes ), and history of family conflict prior to illness with a two-item scale developed by (first author). Respondents were asked “Prior to [the decedent’s] illness, how much did any of your family members: ‘have serious arguments with one another;’ and ‘insult or yell at one another’?” Response categories range from 0 = not at all to 4 = very much . Cronbach’s α was .94.
Analytic Plan
Details regarding strategies for handling missing data are reported elsewhere ( Kramer et al., 2009 ). The analysis has four parts. First, we present descriptive statistics and the results of the two-tailed t tests and F tests comparing the means for complicated grief on all values of the categorical background and contextual variables. Second, we present the descriptives and bivariate correlations for the analytic variables. Third, to test the first hypothesis, we used hierarchical multiple regression analysis to control for the time since death, contextual variables, and primary stressors. Given the limited sample size and the number of independent variables, aside from time since death and history of family conflict, only the variables that were significantly correlated with complicated grief were chosen for the analysis to allow for a more parsimonious model. Omitted contextual variables were analyzed as covariates in an exploratory regression analysis, and their presence did not alter appreciably the relationships between conflict, intra-psychic strains, and complicated grief. Finally, to test hypothesis 2 and examine the extent to which resources would have a stress-buffering effect, interaction terms were computed by multiplying each of the selected secondary stressor variables by each of the three resources variables, which were centered before forming the multiplicative terms ( Jaccard, Turrisi, & Wan, 1990 ). Separate regression analyses were run to identify any significant interactions. One interaction was a significant predictor of complicated grief and as such, the regression analysis included two additional blocks to control for resources and this interaction effect respectively. The more conservative two-tailed tests of significance were used to interpret the results.
Sample Characteristics and Contextual Correlates of Complicated Grief
Sample characteristics and complicated grief means for categorical background and contextual variables are described in Table 1 . The majority of respondents were female, Non-Hispanic White, spouses, with less than a college education. The sample fell fairly evenly into the under and over age 65 groupings. Consistent with the national profile of end-of-life caregivers, the mean age was 63 for family members ( Wolff et al., 2007 ), and 66 for patients ( SD = 8.82; range = 43–80), indicating that this was primarily an older sample. Less than half of the patients had expressed specific wishes for end-of-life care (39%) and the majority (68%) received hospice care. Complicated grief symptom were greater among those who were younger ( t = 2.96, p ≤ .01), less educated ( t = 2.65, p ≤ .01), and who had more children in the home ( t = 2.49, p ≤ .01). No differences were found by gender, race, income, or relationship, and grief was higher among those caring for patients who had expressed their end-of-life care wishes ( t = 2.52, p ≤ .01).
Sample Characteristics and Complicated Grief Means for Categorical Background and Contextual Variables ( N = 152)
Table 2 presents the means and standard deviations of the analytic variables as well as the zero-order correlations among them. Complicated grief symptoms were not correlated with a history of family conflict, but were significantly correlated with physical ( r = .28, p ≤ .001) and psychological symptoms of the patient ( r = .34, p ≤ .001).
Correlations and Descriptives for Analytic Variables
Secondary Stressors and Complicated Grief: Hypothesis 1
We hypothesized that after controlling for time since death and a history of family conflict, and other contextual and primary stressor variables, that higher levels of family conflict at the end-of-life, family member difficulty accepting the illness and patient fear of death will contribute to complicated grief symptoms. Table 3 presents the results of the regression analysis for complicated grief. As shown in Step 3, after controlling for contextual and primary stressor variables, secondary stressors uniquely explained 15% of the variance in complicated grief symptoms, the most important two being family conflict at the end-of-life ( b = .23, p ≤ .01) and family member difficulty accepting the illness ( b = .34, p ≤ .001).
Hierarchical Regression Analysis for Complicated Grief Symptoms
Moderators of Complicated Grief Symptoms: Hypothesis 2
We hypothesized that the quality of care provided to the patient and family and hospice utilization will buffer the effect of secondary stressors on complicated grief symptoms. As shown in the final model of Table 3 , net of the other predictors, an interaction between patient fear of death and hospice care utilization significantly predicted complicated grief symptoms ( b = −.16, p ≤ .05). We graphed this interaction using the estimated regression coefficients for combinations of low and high patient fear of death by hospice or no hospice status; all other variables evaluated at their mean (see Figure 1 for a portrayal of this interaction effect). Patient fear of death contributed to complicated grief when hospice care was not utilized, and supports the stress-buffering hypothesis.

Interaction between patient fear of death and hospice utilization on complicated grief.
The regression equation explained 45% of the total variance in complicated grief symptoms. In the final model, in addition to the moderator variable (i.e., patient fear of death by hospice utilization), significantly more complicated grief symptoms were found among caregivers without college education ( b = −.25, p ≤ .001), who were caring for patients who had specified their end-of-life care preferences ( b = .13, p ≤ .10), and had more psychological symptoms ( b = .15, p ≤ .10), and fear of dying ( b = .15, p ≤ .05), who had family members who expressed difficulty accepting the illness ( b = .33, p ≤ .001), and who had low levels of a history of family conflict ( b = −.21, p ≤ .05), but higher levels of family conflict near the end-of-life ( b = .21, p ≤ .05).
The most compelling findings from this study concern the importance of secondary stressors in predicting complicated grief symptoms and hospice utilization in moderating the stress process. Secondary stressors uniquely explained 15% of the variance in complicated grief symptoms and both family conflict and the two intrapsychic strains were significant predictors in the final model. Kissane and colleagues ( Kissane & Bloch, 2002 ; Kissane, McKenzie, Bloch, Moskowitz, McKenzie, & O’Neill, 2006 ) have well documented a relationship between “maladaptive family functioning” and bereavement outcomes. Our results suggest that although not very common, as evidenced by the strong correlation between prior conflict and conflict at the end-of-life (i.e., r = .62), that families with lower levels of prior conflict and higher levels of conflict associated with difficult decisions at the end-of-life may be at most risk. Developing and testing interventions to meet the needs of families at risk of experiencing conflict at the end-of-life (see Kramer et al., 2009 ), to better support families during critical junctures in decision making, and in the aftermath of unresolved conflict across the continuum of cancer care are important goals for future research and practice.
National guidelines and preferred practices for palliative care have been established that emphasize the importance of assessing and treating social and psychological responses to illness that often contribute to patient and family suffering ( Hultman, Keene-Reder, & Dahlin, 2008 ). Our findings suggest that assessment and treatment of these needs should not be relegated to palliative care only. Family difficulty accepting illness and patient fear of death both represent psychological needs that may be present very soon after the diagnosis. Oncologists, family physicians, and other interdisciplinary care providers need to be mindful of the intrapsychic strains that are stimulated by a lung cancer diagnosis and consider psychotherapeutic interventions or referrals to address them. Cognitive-existential group therapy has been used successfully to address death anxiety among cancer patients ( Kissane et al., 1997 ). Similar models might be employed to support lung cancer patients and family members to address fear of death and difficulty accepting the illness.
Although no direct bivariate relationship was found between hospice utilization and complicated grief, hospice enrollment did serve to buffer the effect of patient fear of death and the outcome. The decedent’s fear of death, as reported by the survivor, did not lead to complicated grief when hospice was used, but did have a negative effect on bereavement outcomes for those not enrolled. Hospice professionals are found to have less death anxiety and more death related experience than other health care professionals ( Carr & Merriman, 1995–1996 ), and may have greater competency to address fear of death among patients. Of course this is an empirical question, but may help to explain our findings. Hospice care is recommended as the optimal care model of patients with advanced lung cancer ( Griffin et al., 2003 ), but is typically underutilized by lung cancer patients ( McCarthy, Burns, Davis, & Phillips, 2003 ). In addition to encouraging efforts to promote timely hospice referral, other initiatives to increase the knowledge, skills, and competencies of oncology and other health care providers for routinely assessing and treating patient fear of death may help prevent complicated bereavement.
Not surprisingly, bivariate analysis revealed strong relationships between lower quality of care of the patient and family and higher levels of complicated grief. Given the documented concerns regarding care quality for lung cancer patients and their families ( Krishnasamy, Wilkie, & Haviland, 2001 ), this is an important finding. The relationship between care quality and complicated grief was no longer statistically significant in the multivariate model when contextual and stressor variables were included and care quality did not moderate the effect of stressors on grief. Routine care may not sufficiently address the distinctive problems associated with secondary stressors. More intensive and targeted interventions to meet these distinctive needs may be required.
Several limitations should be acknowledged when interpreting these results. The cross-sectional design and reliance on retrospective reports of care and conflict at the end-of-life are important limitations. Current affective states may affect retrospective appraisals of subjective measures. Prospective studies are necessary to evaluate the direction of relationships and capture experiences as they occur, but do present ethical and methodological challenges of their own ( Casarett, Knebel, & Helmers, 2003 ). Although the interaction between hospice utilization and patient fear of death was interpretable, it may be a chance finding given the multiple exploratory tests used to evaluate possible interaction effects. Other studies are needed to test for replication. Some of our measures are limited such as the clinical care needs of the patient that measured the number of symptoms rather than symptom severity that may underestimate the relationship between symptoms and complicated grief. In addition, we did not collect any data on the end-of-life care treatment preferences that were expressed by patients or the extent to which they were carried out, which seriously limits our ability to interpret the unanticipated positive correlation between care preferences specified and grief symptoms. It is possible that the patient’s expressed end-of-life care wishes were not fulfilled or were a source of disagreement among family members. Finally, the nonrepresentative nature of the sample, seriously limits the generalizability of study findings. Although the ACCESS study sought a representative statewide sample and over sampled by race, less than half of the eligible families completed the survey, and the sample was primarily older White female spouses. In addition, the hospice utilization rate of our sample is higher than that of lung cancer Medicare beneficiaries (i.e., 68% compared to 27%; McCarthy et al., 2003 ), and the overall rates of family conflict were fairly modest ( Kramer et al., 2009 ).
Despite its limitations, this study was guided by a theoretical framework to provide a more comprehensive understanding of the complex determinants of the bereavement process with attention to domains not previously considered in research. Results provide substantial support documenting a relationship between family conflict, intrapsychic strains and complicated grief, and a moderating influence of hospice utilization. Risk assessment tools used to identify persons at risk for complicated grief ( Melliar-Smith, 2002 ) could be expanded to include assessment of patient symptoms, intrapsychic strains, prior and end-of-life conflict, and caregiver education. Future research to replicate these findings and to illuminate understanding of the primary and secondary stressors and resources that might influence the bereavement process, as well as development and testing of interventions to address these are warranted.
Acknowledgments
The authors gratefully acknowledge the bereaved family members for their participation in this study, and the contributions of our colleagues who participated in the design of the ACCESS survey.
* This study used data from the Assessment of Cancer CarE and SatiSfaction (ACCESS), survey, a project of the Wisconsin Comprehensive Cancer Control Program. Funding for the WI CCC Program is provided by The Centers for Disease Control & Prevention, The Wisconsin Department of Health Services, The Wisconsin Partnership Fund for a Healthy Future, and the UW-Paul P. Carbone Comprehensive Cancer Center. This article was prepared with support from the National Institute of Mental Health (T32 MH65185 to Jan. Greenberg).
- American Cancer Society. Cancer facts and figures 2009. Atlanta, GA: American Cancer Society; 2009. [ Google Scholar ]
- Aneshensel CS, Pearlin LI, Mullan JT, Zarit SH, Whitlatch CJ. Profiles in caregiving: The unexpected career. New York: Academic Press; 1995. [ Google Scholar ]
- Barr P, Cacciatore JE. Personal fear of death and grief in bereaved mothers. Death Studies. 2008; 32 :445–460. [ PubMed ] [ Google Scholar ]
- Bass DM, Bowman K, Noelker LS. The influence of caregiving and bereavement support on adjusting to an older relative’s death. The Gerontologist. 1991; 31 :32–42. [ PubMed ] [ Google Scholar ]
- Beckwith BE, Beckwith SK, Gray TL, Micsko MM. Identification of spouses at high risk during bereavement: A preliminary assessment of Parkes and Weiss’ Risk Index. Hospice Journal. 1990; 6 (3):35–46. [ PubMed ] [ Google Scholar ]
- Bernard LL, Guarnaccia CA. Two models of caregiver strain and bereavement adjustment: A comparison of husband and daughter caregivers of breast cancer hospice patients. The Gerontologist. 2003; 43 :808–816. [ PubMed ] [ Google Scholar ]
- Burton AM, Haley WE, Small BJ, Finley MR, Dillinger-Vasille M, Schonwetter R. Predictors of well-being in bereaved former hospice care-givers: The role of caregiving stressors, appraisals, and social resources. Palliative and Supportive Care. 2008; 6 :149–158. [ PubMed ] [ Google Scholar ]
- Cameron J, Franche R, Cheung AM, Stewart DE. Lifestyle interference and emotional distress in family caregivers of advanced cancer patients. Cancer. 2002; 94 :521–527. [ PubMed ] [ Google Scholar ]
- Carr M, Merriman MP. Comparison of death attitudes among hospice workers and health care professionals in other settings. Omega. 1995–1996; 32 :287–301. [ Google Scholar ]
- Casarett D, Knebel A, Helmers K. Ethical challenges of palliative care research. Journal of Pain and Symptom Management. 2003; 25 (4):S3–S5. [ PubMed ] [ Google Scholar ]
- Chapple A, Ziebland S, McPherson A. Stigma, shame, and blame experienced by patients with lung cancer: Qualitative study. British Medical Journal. 2004; 328 (7454):1470–1474. [ PMC free article ] [ PubMed ] [ Google Scholar ]
- Christakis NA, Iwashyna TJ. The health impact of health care on families: A matched cohort study of hospice use by decedents and mortality outcomes in surviving, widowed spouses. Social Science & Medicine. 2003; 57 :465–475. [ PubMed ] [ Google Scholar ]
- Detering KM, Hancock AD, Reade MC, Silvester W. The impact of advance care planning on end of life care in elderly patients: A randomised controlled trial. British Medical Journal. 2010; 340 doi: 10.1136/bmj.c1345. [ PMC free article ] [ PubMed ] [ CrossRef ] [ Google Scholar ]
- Downey RG, King C. Missing data in Likert ratings: A comparison of replacement methods. Journal of General Psychology. 1998; 125 :175–191. [ PubMed ] [ Google Scholar ]
- Godkin MA, Krant MJ, Doster NJ. The impact of hospice care on families. International Journal of Psychiatry in Medicine. 1983–84; 13 (2):153–165. [ PubMed ] [ Google Scholar ]
- Grande GE, Farquhar MC, Barclay SIG. Caregiver bereavement outcome: Relationship with hospice at home, satisfaction with care, and home death. Journal of Palliative Care. 2004; 20 (2):69–77. [ PubMed ] [ Google Scholar ]
- Griffin JP, Koch KA, Ackerman TF, Cole FH., Jr End-of-life care in patients with lung cancer. CHEST: Supplement. 2003; 123 :312S–331S. [ PubMed ] [ Google Scholar ]
- Griffin JP, Koch KA, Nelson JE, Cooley ME. Palliative care consultation, quality-of-life measurements, and bereavement for end-of-life care in patients with lung cancer. CHEST: Supplement. 2007; 132 :404S–422S. [ PubMed ] [ Google Scholar ]
- Gustavson K, Dal Santo TS. Caregiver service use: A complex story of care at the end of life. Journal of Social Work in End-of-Life & Palliative Care. 2008; 4 :286–311. [ Google Scholar ]
- Hammes BJ, Rooney BL. Death and end-of-life planning in one midwestern community. Archives of Internal Medicine. 1998; 158 :383–390. [ PubMed ] [ Google Scholar ]
- Hawthorne G, Elliott P. Imputing cross-sectional missing data: Comparison of common techniques. Australian and New Zealand Journal of Psychiatry. 2005; 39 :583–590. [ PubMed ] [ Google Scholar ]
- Hultman T, Keene Reder EA, Dahlin CM. Improving psychological and psychiatric aspects of palliative care: The national consensus project and the national quality forum preferred practices for palliative and hospice care. Omega. 2008; 57 :323–339. [ PubMed ] [ Google Scholar ]
- Jaccard J, Turrisi R, Wan CK. Interaction effects in multiple regression. Newbury Park, CA: Sage; 1990. [ PubMed ] [ Google Scholar ]
- Jordan JR, Neimeyer RA. Does grief counseling work? Death Studies. 2003; 27 :765–786. [ PubMed ] [ Google Scholar ]
- Kissane DW, Bloch S. Family focused grief therapy: A model of family-centered care during palliative care and bereavement. Philadelphia, PA: Open University Press; 2002. [ Google Scholar ]
- Kissane DW, Bloch S, Miach P, Smith GC, Seddon A, Keks N. Cognitive-existential group therapy for patients with primary breast cancer—Techniques and themes. Psycho-Oncology. 1997; 6 :25–33. [ PubMed ] [ Google Scholar ]
- Kissane DW, McKenzie M, Bloch S, Moskowitz C, McKenzie DP, O’Neill J. Family focused grief therapy: A randomized, controlled trial in palliative care and bereavement. American Journal of Psychiatry. 2006; 163 (7):1208–1218. [ PubMed ] [ Google Scholar ]
- Kramer BJ, Boelk A, Auer C. Family conflict at the end-of-life: Lessons learned in a model program for vulnerable older adults. Journal of Palliative Medicine. 2006; 9 :791–801. [ PubMed ] [ Google Scholar ]
- Kramer BJ, Kavanaugh M, Trentham-Dietz A, Walsh M, Yonker JA. Predictors of family conflict at the end of life: The experience of spouses and adult children of persons with lung cancer. The Gerontologist. 2009 doi: 10.1093/geront/gnp 121. [ PMC free article ] [ PubMed ] [ CrossRef ] [ Google Scholar ]
- Kramer BJ, Vitaliano PP. Coping: A review of the theoretical frameworks and the measures used among caregivers of individuals with dementia. Journal of Gerontological Social Work. 1994; 23 (1/2):151–174. [ Google Scholar ]
- Krishnasamy M, Wilkie E, Haviland J. Lung cancer health care needs assessment: Patients’ and informal carers’ responses to a national mail questionnaire survey. Palliative Medicine. 2001; 15 :213–227. [ PubMed ] [ Google Scholar ]
- Latham AE, Prigerson HG. Suicidality and bereavement: Complicated grief as psychiatric disorder presenting greatest risk for suicidality. Suicide and Life-Threatening Behavior. 2004; 34 :350–362. [ PMC free article ] [ PubMed ] [ Google Scholar ]
- Lichtenthal WG, Nilsson M, Zhang B, Trice ED, Kissane DW, Prigerson HG. Do rates of mental disorders and existential distress among advanced stage cancer patients increase as death approaches. Psycho-Oncology. 2009; 18 :50–61. [ PMC free article ] [ PubMed ] [ Google Scholar ]
- McCarthy EP, Burns RB, Davis RB, Phillips RS. Barriers to hospice care among older patients dying with lung and colorectal cancer. Journal of Clinical Oncology. 2003; 21 :728–735. [ PubMed ] [ Google Scholar ]
- McMillan SC, Small BJ. Using the COPE intervention for family caregivers to improve symptoms of hospice homecare patients: A clinical trial. Oncology Nursing Forum. 2007; 34 :313–321. [ PubMed ] [ Google Scholar ]
- Melliar-Smith C. The risk assessment of bereavement in a palliative care setting. International Journal of Palliative Nursing. 2002; 8 :281–287. [ PubMed ] [ Google Scholar ]
- Morita T, Akechi T, Ikenaga M, Inoue S, Kohara H, Matsubara T, et al. Terminal decline: Recommendations from bereaved families’ experiences. Journal of Pain and Symptom Management. 2007; 34 :579–589. [ PubMed ] [ Google Scholar ]
- Mullan JT. The bereaved caregiver: A prospective study of changes in well-being. The Gerontologist. 1992; 32 :673–683. [ PubMed ] [ Google Scholar ]
- Neimeyer RA, Wittkowski J, Moser RP. Psychological research on death attitudes: An overview and evaluation. Death Studies. 2004; 28 :309–340. [ PubMed ] [ Google Scholar ]
- Pearlin L, Mullan J, Semple M, Skaff M. Caregiving and the stress process: An overview of concepts and their measures. The Gerontologist. 1990; 30 (5):583–594. [ PubMed ] [ Google Scholar ]
- Persson C, Östlund U, Wennman-Larsen A, Wengström Y, Gustavsson P. Health-related quality of life in significant others of patients dying from lung cancer. Palliative Medicine. 2008; 22 :239–247. [ PubMed ] [ Google Scholar ]
- Portenoy R, Payne D, Jacobson P. Breakthrough pain: Characteristics and impacts in patients with cancer pain. Pain. 1999; 81 :129–134. [ PubMed ] [ Google Scholar ]
- Prigerson HG, Jacobs SC. Traumatic grief as a distinct disorder: A rationale, consensus criteria, and a preliminary empirical test. In: Stroebe MS, Hansson RO, Stroebe W, Schut H, editors. Handbook of bereavement research: Consequences, coping and care. Washington, DC: American Psychological Association; 2001. pp. 613–637. [ Google Scholar ]
- Prigerson HG, Maciejewski PK. A call for sound empirical testing and evaluation of criteria for complicated grief proposed for DSM-V. Omega. 2005; 52 :9. [ Google Scholar ]
- Prigerson HG, Maciejewski PK. Grief and acceptance as opposite sides of the same coin: Setting a research agenda to study peaceful acceptance of loss. British Journal of Psychiatry. 2008; 193 :435–437. [ PubMed ] [ Google Scholar ]
- Prigerson HG, Maciejewski PK, Reynolds CF, Bierhals AJ, Newsom JT, Fasiczka A, et al. Inventory of complicated grief: A scale to measure maladaptive symptoms of loss. Psychiatry Research. 1995; 59 :65–79. [ PubMed ] [ Google Scholar ]
- Ryan RP, Howell V, Jones J, Hardy EJ. Lung cancer, caring for the caregivers. A qualitative study of providing pro-active social support targeted to the carers of patients with lung cancer. Palliative Medicine. 2008; 22 :233–238. [ PubMed ] [ Google Scholar ]
- Schulz R, Boerner K, Shear K, Zhang S, Gitlin LN. Predictors of complicated grief among dementia caregivers: A prospective study of bereavement. American Journal of Geriatric Psychiatry. 2006; 14 :650–656. [ PubMed ] [ Google Scholar ]
- Siegel MD, Hayes E, Vanderwerker LC, Loseth DB, Prigerson HG. Psychiatric illness in the next of kin in patients who die in the intensive care unit. Critical Care Medicine. 2008; 36 :1722–1728. [ PubMed ] [ Google Scholar ]
- Steele LL. The death surround: Factors influencing the grief experience of survivors. Oncology Nursing Forum. 1990; 17 :235–241. [ PubMed ] [ Google Scholar ]
- Stuifbergen A, Becker H, Blozis S, Beal C. Conceptualization and development of the acceptance of chronic health conditions scale. Issues in Mental Health Nursing. 2008; 29 :101–114. [ PubMed ] [ Google Scholar ]
- Teno JM. TIME: Toolkit of instruments to measure end of life care. 2004 Retrieved August 1, 2000 from http://www.chcr.brown.edu/pcoc/linkstoinstrumhtm.htm .
- Tilden VP, Tolle SW, Garland MJ, Nelson CA. Decisions about life-sustaining treatments. Archives of Internal Medicine. 1995; 155 :633–638. [ PubMed ] [ Google Scholar ]
- Tolstikova K, Fleming S, Chartier B. Grief, complicated grief, and trauma: The role of the search for meaning, impaired self-reference, and death anxiety. Illness, Crisis & Loss. 2005; 18 :293–313. [ Google Scholar ]
- Trentham-Dietz A, Walsh MC. Summary of results. Madison, WI: University of Wisconsin Paul P. Carbone Comprehensive Cancer Center; 2008. ACCESS: Assessment of Cancer CarE and SatiSfaction. [ Google Scholar ]
- Wolff JL, Dy SM, Frick KD, Kasper JD. End-of-life care: Findings from a national survey of informal caregivers. Annals of Internal Medicine. 2007; 167 :40–46. [ PubMed ] [ Google Scholar ]
- Wright AA, Zhang B, Ray A, Mack JW, Trice E, Balboni T, et al. Associations between end-of-life discussions, patient mental health, medical care near death, and caregiver bereavement adjustment. Journal of the American Medical Association. 2008; 300 :1665–1673. [ PMC free article ] [ PubMed ] [ Google Scholar ]
- Yancey D, Greger HA, Coburn P. Determinants of grief resolution in cancer death. Journal of Palliative Care. 1990; 6 (4):24–31. [ PubMed ] [ Google Scholar ]
- Zhang A, El-Jawahri A, Prigerson HG. Update on bereavement research: Evidence-based guidelines for the diagnosis and treatment of complicated bereavement. Journal of Palliative Medicine. 2006; 9 :1188–1203. [ PubMed ] [ Google Scholar ]
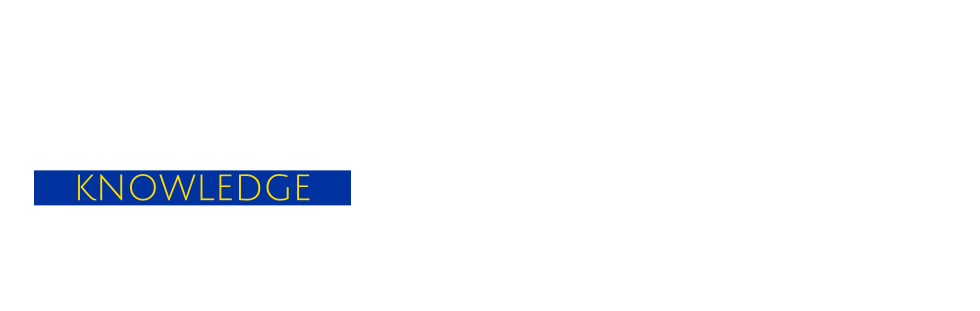
UKnowledge > College of Medicine > Toxicology and Cancer Biology > Theses & Dissertations
Theses and Dissertations--Toxicology and Cancer Biology
Theses/dissertations from 2024 2024.
Elucidation of Mismatch Repair Regulation by ABL1: Advantages/Disadvantages of Tyrosine Kinase Inhibitor Treatment , Hannah Daniels
ACQUIRED TREATMENT RESISTANCE IN PROSTATE CANCER VIA THE PRODUCTION OF RADIATION DERIVED EXTRACELLULAR VESICLES CONTAINING MITOCHONDRIAL PROTEINS , Caitlin Miller
Theses/Dissertations from 2023 2023
ELUCIDATING THE FUNCTIONAL IMPORTANCE OF PEROXIREDOXIN IV IN PROSTATE CANCER AND ITS SECRETION MECHANISM , Na Ding
Targeting EZH2 to Improve Outcomes of Lung Squamous Cell Carcinoma , Tanner DuCote
UNDERSTANDING AND TARGETING THE TPH1-SEROTONIN-HTR3A AXIS IN SMALL CELL LUNG CANCER , Yanning Hao
CONSERVED NOVEL INTERACTIONS BETWEEN POST-REPLICATIVE REPAIR AND MISMATCH REPAIR PROTEINS HAVE DIFFERENTIAL EFFECTS ON DNA REPAIR PATHWAYS , Anna K. Miller
UNDERSTANDING THE ROLE OF PEROXIREDOXIN IV IN COLORECTAL CANCER DEVELOPMENT , Pratik Thapa
BEYOND MITOSIS, PLK1-MEDIATED PHOSPHORYLATION RE-WIRES CANCER METABOLISM AND PROMOTES CANCER PROGRESSION , Qiongsi Zhang
Theses/Dissertations from 2022 2022
ELUCIDATING THE ROLE OF POLYCOMB REPRESSIVE COMPLEX 2 IN LUNG STEM CELL FATE AND LUNG DISEASE , Aria Byrd
SEX DIMORPHISM IN HEMATOPOIESIS AND BONE MARROW NICHE , xiaojing cui
EXTRACELLULAR VESICLES AND CANCER THERAPY: AN INSIGHT INTO THE ROLE OF OXIDATIVE STRESS , Jenni Ho
OVERCOMING RESISTANCE TO SG-ARIS IN CASTRATION-RESISTANT PROSTATE CANCER , Chaohao Li
Theses/Dissertations from 2021 2021
THE TUMOR SUPPRESSOR PAR-4 REGULATES HYPERTROPHIC OBESITY , Nathalia Araujo
Epigenetic States Regulate Tumor Aggressiveness and Response to Targeted Therapies in Lung Adenocarcinoma , Fan Chen
DELINEATING THE ROLE OF FATTY ACID METABOLISM TO IMPROVE THERAPEUTIC STRATEGIES FOR COLORECTAL CANCER , James Drury
DEVELOPMENT OF TOOLS FOR ATOM-LEVEL INTERPRETATION OF STABLE ISOTOPE-RESOLVED METABOLOMICS DATASETS , Huan Jin
MECHANISMS OF CADMIUM-INDUCED AND EPIDERMAL GROWTH FACTOR RECEPTOR MUTATION-DRIVEN LUNG TUMORIGENESIS , Hsuan-Pei Lin
SCIENCE-BASED REGULATION OF PHARMACOLOGICAL SUBSTANCES IN COMPETITION HORSES , Jacob Machin
A NOVEL ROLE FOR NEUROTENSIN IN REGULATION OF STEM CELL FUNCTION IN THE SMALL INTESTINE , Stephanie Rock
Theses/Dissertations from 2020 2020
NOVEL POST-TRANSLATIONAL MODIFICATION AND FUNCTION OF FUS: THE RELEVANCE TO AMYOTROPHIC LATERAL SCLEROSIS , Alexandra Arenas
Prostate Cancer Resistance to Cabazitaxel Chemotherapy , Diane Begemann
Examining the Role of Metabolic Pathways as Therapeutic Modalities for Triple Negative Breast Cancer , Jeremy Andrew Johnson
THE ROLE OF NEURAL PRECURSOR CELL EXPRESSED DEVELOPMENTALLY DOWN-REGULATED PROTEIN 9 IN ENHANCED AGGRESSIVENESS OF HEXAVALENT CHROMIUM TRANSFORMED BRONCHIAL EPITHELIAL CELLS , Peter Van Wie
Theses/Dissertations from 2019 2019
A COMPROMISED LIVER ALTERS PCB TOXICITY AND NUTRIENT METABOLISM , Jazmyne D. L. Barney
Advanced Search
- Notify me via email or RSS
Browse by Author
- Collections
- Disciplines
Author Corner
- Submit Research
New Title Here
Below. --> connect.
- Law Library
- Special Collections
- Copyright Resource Center
- Graduate School
- Scholars@UK
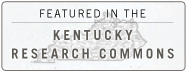
- We’d like your feedback
Home | About | FAQ | My Account | Accessibility Statement
Privacy Copyright
University of Kentucky ®
An Equal Opportunity University Accreditation Directory Email Privacy Policy Accessibility Disclosures
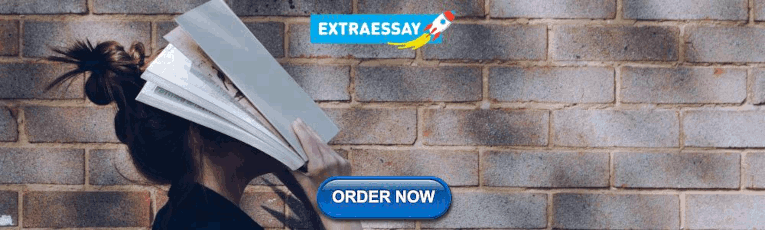
IMAGES
VIDEO
COMMENTS
Lung cancer remains a devastating diagnosis to receive. The goal of this thesis was to better understand the impact of lung cancer and study how to integrate palliative and supportive care.
Lung cancer is one of the most frequently diagnosed cancers and the leading cause of cancer-related deaths worldwide with an estimated 2 million new cases and 1·76 million deaths per year. Substantial improvements in our understanding of disease biology, application of predictive biomarkers, and refinements in treatment have led to remarkable ...
The 100 most cited articles in lung cancer are listed in Table 1, arranged in descending order according to the number of times cited. The number of citations ranged from 7751 to 889, and the mean number of citations per article was 1879.82 ±1264.78 (range: 7751-889). We found that the most cited article (times cited: 7751) on lung cancer ...
Lung Cancer Screening Program Revenue April 2016 verses 2017. A 2012 study found lung cancer screening to be one of the most cost-effective. screening tests available at about $11,000 per life-year saved, compared with $18,000 for. colon cancer screening, $31,000 for breast cancer screening, and $50,000 for cervical.
both limited-stage small cell lung cancer patients and extensive-stage small cell lung cancer patients throughout the study period. Lu et al. (2019) demonstrated that the average incidence of lung cancer was 59.0/100,000 people for the years from 1973 to 2015. Specifically, the incidence rate was at an apex in 1992 and then constantly declined.
the Cancer Dyspnea Scale (CDS) developed by Tanaka and colleagues (2000). The scale. measures three factors: 1) sense of effort; 2) sense of anxiety; and 3) sense of discomfort. Quality of life will be measured by the Assessment of Quality of Life at the End of Life. (AQEL) scale, developed by Henoch et al (2010).
This thesis will be defended in public on Wednesday 24 February 2016 at 11:00 hrs by Ali Saber Hosseinabadi born on 11 September 1981 in Rasht, Iran . Supervisors ... Lung cancer is a result of multiple genetic alterations that accumulate during life21. In 1982, a human gene with transforming activity was identified in a lung ...
In addition, I would like to thank my Thesis Research Advisory Committee Member, Dr. Kimberly Fisher, program directors of the Masters of Science in Clinical Investigation program, Drs. Robert Goldberg and Kate Lapane, Drs. Robert ... Lung cancer is the leading cause of cancer death and the second most common cancer
2. Gender issues in lung cancer risk 8 2.1 Differences between women and men in lung cancer incidence, prevalence and mortality 8 -Differentials by geographical region 11 -Differentials in relation to social diversity 13 2.2 Sex, gender and lung cancer: explaining the differences between men and women 13 -Gender-linked factors 14
Lung cancer has four common types: lung nodules, non-small cell lung cancer (NSCLC), small cell lung cancer (SCLC), and mesothelioma; the most common of which is non-small cell lung cancer [6]. It is important that research and modeling is done on lung cancer in an attempt to better understand and counteract this disease. 1.1 A549 Cells One ...
Fig. 1. The Main Cause of Lung Cancer. Symptoms used to diagnose lung cancer including, yellow fingers, anxiety, chronic illness, fatigueness, allergies, wheezing, roaring, coughing up blood, even small amounts, hoarseness, shortness of breath, bone pain, headache, difficulty in swallowing and chest pain [10].
Lung cancer, the most common cause of cancer-related death in men and women, is responsible for 1.3 million deaths worldwide annually, as of 2004 The most common symptoms are shortness of breath ...
Abstract. Many lung cancer risk prediction models have been published but there has been no systematic review or comprehensive assessment of these models to assess how they could be used in screening. We performed a systematic review of lung cancer prediction models and identified 31 articles that related to 25 distinct models, of which 11 ...
This study demonstrates accurate lung cancer classification and prediction using technologies enabled by machine learning and image processing. To begin, photographs must be collected. Following that, the images are preprocessed using a geometric mean filter. This eventually leads to an increase in image quality.
Objective To study how treatment decisions are made alongside the lung cancer clinical pathway. Methods A prospective, multicentre, multimethods, five-stage, qualitative study. Mediated discourse, thematic, framework and narrative analysis were used to analyse the transcripts. Results 51 health professionals, 15 patients with advanced lung cancer, 15 family members and 18 expert stakeholders ...
Lung cancer is the primary cause of cancer death worldwide, with 2.09 million new cases and 1.76 million people dying from lung cancer in 2018 1.Four case-controlled studies from Japan reported in ...
represented over 25% of all cancer fatalities.1. The National Cancer Database (NCDB) is a hospital-based outcomes data resource that is. estimated to capture 70% of newly diagnosed lung cancers in the United States. The. NCDB is jointly sponsored by the American College of Surgeons and the American.
Design. This is an exploratory study that used a phenomenological-hermeneutic approach (Dreyer & Pedersen, 2009) to deeply understand the connotation of MoL in patients with advanced lung cancer, and to reveal the complexity of MoL and its impact on self.A phenomenological perspective attaches importance to rich contextualized descriptions based on experience, and helps the researcher to look ...
Lung cancer is a very deadly disease. Over half of people with lung cancer die within one year of being diagnosed. Only 16 percent of patients diagnosed with lung cancer are still alive after five years, compared with over 90 percent of breast and prostate cancer patients. 7 The low survival rate contributes to perceptions that
Cancer-related fatigue (CRF) is common in lung cancer survivors and can occur at any point in the care continuum. CRF encompasses physical, emotional, and/or cognitive tiredness; is related to cancer or cancer treatments; and interferes with multiple dimensions of health-related quality of life (1-3).CRF is poorly understood, underrecognized, and undertreated.
Introduction. Lung cancer is the second most common type of cancer and is the leading cause of cancer death globally. In 2018, almost 2.1 million new cases were diagnosed, accounting for ∼12% of the cancer burden worldwide (Sung et al., 2021).The malignant stage of lung cancer is known as lung adenocarcinoma, which is the most common and is diagnosed in both smokers and non-smokers.
Lung cancer is still the leading cause of death by cancer in the U.S., with someone diagnosed about every two minutes. To address this, the American Lung Association has renewed a partnership with LUNGevity to fund innovative research to intercept lung cancer—that is, detecting and treating pre-cancerous cells before they have a chance to become malignant.
Patient Clinical Care Needs . Primary stressors are the difficult circumstances experienced by family care-givers that stem from the patient's care needs (Pearlin et al., 1990).Lung cancer patients have a high prevalence of uncontrolled distressing physical symptoms; the most common are pain, shortness of breath, nausea or vomiting, and fatigue (Griffin et al., 2007).
trials in multiple cancers, including lung cancer. • Determined that defective apoptosis, or programmed cell death, plays a large role in the emergence of resistance to targeted therapy in lung cancer, leading to phase 1 clinical trial (NCT02520778) testing of a combination therapy of an apoptotic stimulator with a targeted therapy in lung ...
elucidating the role of polycomb repressive complex 2 in lung stem cell fate and lung disease, aria byrd. pdf. sex dimorphism in hematopoiesis and bone marrow niche, xiaojing cui. pdf. extracellular vesicles and cancer therapy: an insight into the role of oxidative stress, jenni ho. pdf