remote sensing and gis Recently Published Documents
Total documents.
- Latest Documents
- Most Cited Documents
- Contributed Authors
- Related Sources
- Related Keywords
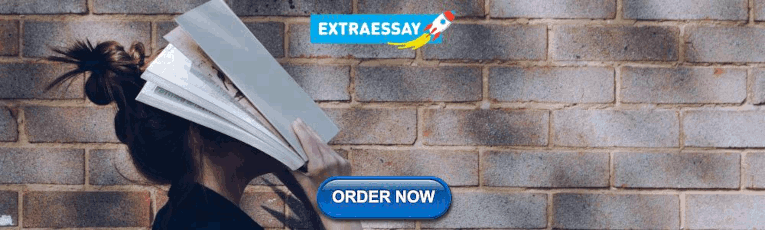
Expected spatio-temporal variation of groundwater deficit by integrating groundwater modeling, remote sensing, and GIS techniques
Relative shannon’s entropy approach for quantifying urban growth using remote sensing and gis: a case study of cuttack city, odisha, india, review of conceptual models of estimating the spatio-temporal variations of water depth using remote sensing and gis for the management of dams and reservoirs, temporal assessment of sedimentation in siruvani reservoir using remote sensing and gis, evaluating the groundwater potential of wadi al-jizi, sultanate of oman, by integrating remote sensing and gis techniques, diachronic study of land cover of the medjerda watershed and estimation of rusle-c factor using ndvi-based equation, remote sensing, and gis, slum categorization for efficient development plan—a case study of udhampur city, jammu and kashmir using remote sensing and gis, morphometric analysis of damodar river sub-watershed, jharkhand, india, using remote sensing and gis techniques, land use/land cover change detection and validation of swat model on vishow sub-basin using remote sensing and gis techniques, zoning groundwater potential recharge using remote sensing and gis technique in the red river delta plain.
Abstract The Red River delta plain is the second largest delta in Vietnam and is located in the North of the country with an area of 14,860 km2 and residing more than 22.5 million inhabitants. Groundwater is mainly exploited in Quaternary sedimentary aquifers with a total discharge of about 3 million m3/day. Some localities have shown signs of over-exploitation such as in Hanoi and in Nam Dinh, which may lead to related problems such as depletion, subsidence, saltwater intrusion, and water pollution. In order to be able to sustainably exploit groundwater, the groundwater potential recharge needs to be estimated. There have been many studies using different methods to estimate the groundwater recharge and to zone potential recharge. In the study area, there are several studies for groundwater recharge, but some are still uncertain because of using indirect methods, some are locally estimated in specific areas. Therefore, the objective of this study is to apply remote sensing and GIS to zone the groundwater potential recharge and its verification by using radioactive isotope 3H analysis in the Red River delta plain. Various types of satellite images have been used and interpreted to detect the different thematic layers which concern the groundwater potential recharge. GIS has been applied as a platform for analysis and integration of thematic layers for zonation, finally. Field trip and water sampling for chemical and radioactive 3H analysis were also conducted. Zones with low, moderate, and high groundwater potential recharge have been delineated with good agreement from the direct estimation of groundwater recharge by radioactive isotopes 3H.
Export Citation Format
Share document.

Thank you for visiting nature.com. You are using a browser version with limited support for CSS. To obtain the best experience, we recommend you use a more up to date browser (or turn off compatibility mode in Internet Explorer). In the meantime, to ensure continued support, we are displaying the site without styles and JavaScript.
- View all journals
- Explore content
- About the journal
- Publish with us
- Sign up for alerts
- Review Article
- Published: 15 September 2013
The role of satellite remote sensing in climate change studies
- Jun Yang 1 ,
- Peng Gong 1 , 2 , 3 ,
- Rong Fu 4 ,
- Minghua Zhang 5 ,
- Jingming Chen 6 , 7 ,
- Shunlin Liang 8 , 9 ,
- Bing Xu 8 , 10 ,
- Jiancheng Shi 2 &
- Robert Dickinson 4
Nature Climate Change volume 3 , pages 875–883 ( 2013 ) Cite this article
17k Accesses
325 Citations
55 Altmetric
Metrics details
- Attribution
- Climate-change impacts
An Erratum to this article was published on 20 December 2013
A Corrigendum to this article was published on 29 October 2013
This article has been updated
Satellite remote sensing has provided major advances in understanding the climate system and its changes, by quantifying processes and spatio-temporal states of the atmosphere, land and oceans. In this Review, we highlight some important discoveries about the climate system that have not been detected by climate models and conventional observations; for example, the spatial pattern of sea-level rise and the cooling effects of increased stratospheric aerosols. New insights are made feasible by the unparalleled global- and fine-scale spatial coverage of satellite observations. Nevertheless, the short duration of observation series and their uncertainties still pose challenges for capturing the robust long-term trends of many climate variables. We point out the need for future work and future systems to make better use of remote sensing in climate change studies.
This is a preview of subscription content, access via your institution
Access options
Subscribe to this journal
Receive 12 print issues and online access
195,33 € per year
only 16,28 € per issue
Buy this article
- Purchase on Springer Link
- Instant access to full article PDF
Prices may be subject to local taxes which are calculated during checkout
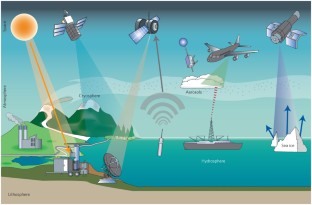
Similar content being viewed by others
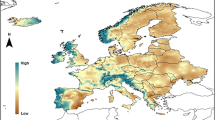
A multidecadal assessment of climate indices over Europe
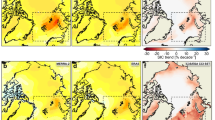
Exceptional warming over the Barents area
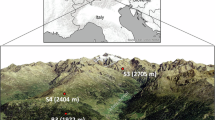
Six years of high-resolution climatic data collected along an elevation gradient in the Italian Alps
Change history, 27 september 2013.
In the version of this Review Article originally published, on page 877, the mass balance of glaciers in the Karakarom region should have been 0.11±0.2 m yr −1 . On page 879, in the second paragraph of the ‘Aerosols’ section, the estimated value for the direct radiative forcing should have been −1.0±0.34 W m −2 . These errors have now been corrected in the HTML and PDF versions of the Review Article.
02 December 2013
In the version of this Review Article originally published, the temperature anomaly trends for RSS and UAH in Fig. 2b,c should have been positive values. These errors have now been corrected in the online versions of the Review Article. In the previous corrigendum, the mass balance of glaciers in the Karakarom region should have read 0.11±0.22 m yr -1 .
Overpeck, J. T., Meehl, G. A., Bony, S. & Easterling, D. R. Climate data challenges in the 21st century. Science 331 , 700 (2011).
CAS Google Scholar
Yates, H. W. Measurement of the Earth radiation balance as an instrument design problem. Appl. Opt. 16 , 297–299 (1977).
Li, J., Wang, M. H. & Ho, Y. S. Trends in research on global climate change: A Science Citation Index Expanded-based analysis. Glob. Planet. Change 77 , 13–20 (2011).
Google Scholar
Bontemps, S. et al. Revisiting land cover observations to address the needs of the climate modelling community. Biogeosci. Discuss. 8 , 7713–7740 (2011).
Gong, P. et al. Finer resolution observation and monitoring of global land cover: first mapping results with Landsat TM and ETM+ data. Int. J. Remote Sens. 34 , 2607–2654 (2013).
Ghent, D., Kaduk, J., Remedios, J. & Balzter, H. Data assimilation into land surface models: The implications for climate feedbacks. Int. J. Remote Sens. 32 , 617–632 (2011).
Saha, S. et al. The NCEP climate forecast system reanalysis. Bull. Am. Meteorol. Soc. 91 , 1015–1057 (2010).
Global Climate Observing System Systematic Observation Requirements for Satellite-based Data Products for Climate: 2011 Update GCOS-154 (World Meteorological Organization, 2011).
Joyce, K. E., Belliss, S. E., Samsonov, S. V., McNeill, S. J. & Glassey, P. J. A review of the status of satellite remote sensing and image processing techniques for mapping natural hazards and disasters. Prog. Phys. Geog. 33 , 183–207 (2009).
Trenberth, K. & Hurrell, J. How accurate are satellite thermometers—Reply. Nature 389 , 342–343 (1997).
Karl, T. R. et al. Observation needs for climate information, prediction and application: Capabilities of existing and future observing systems. Procedia Environ. Sci. 1 , 192–205 (2010).
Thies, B. & Bendix, J. Satellite based remote sensing of weather and climate: Recent achievements and future perspectives. Meteorol. Appl. 18 , 262–295 (2011).
Pachauri, R. K. & Reisinger, A. (eds) IPCC Climate Change 2007: Synthesis Report (IPCC, 2007).
Hansen, J. & Lebedeff, S. Global trends of measured surface air temperature. J. Geophys. Res. 92 , 345–313 (1987).
Pielke, R. A. et al. Unresolved issues with the assessment of multidecadal global land surface temperature trends. J. Geophys. Res. 112 , D24S08 (2007).
Reynolds, R. W., Rayner, N. A., Smith, T. M., Stokes, D. C. & Wang, W. An improved in situ and satellite SST analysis for climate. J. Clim. 15 , 1609–1625 (2002).
Large, W. G. & Yeager, S. G. On the observed trends and changes in global sea surface temperature and air-sea heat fluxes (1984–2006). J. Clim. 25 , 6123–6135 (2012).
Zhang, H. et al. An integrated global observing system for sea surface temperature using satellites and in situ data: Research to operations. Bull. Am. Meteorol. Soc. 90 , 31–38 (2009).
Deser, C., Phillips, A. S. & Alexander, M. A. Twentieth century tropical sea surface temperature trends revisited. Geophys. Res. Lett 37 , L10701 (2010).
Karnauskas, K. B., Seager, R., Kaplan, A., Kushnir, Y. & Cane, M. A. Observed strengthening of the zonal sea surface temperature gradient across the Equatorial Pacific Ocean. J. Clim. 22 , 4316–4321 (2010).
Schwaller, M. R. & Robert Morris, K. A ground validation network for the global precipitation measurement mission. J. Atmos. Ocean. Tech. 28 , 301–319 (2011).
Manabe, S. & Wetherald, R. T. Thermal equilibrium of the atmosphere with a given distribution of relative humidity. J. Atmos. Sci. 24 , 241–259 (1967).
Spencer, R. W. & Christy, J. R. Precise monitoring of global temperature trends from satellites. Science 247 , 1558–1562 (1990).
Thorne, P. W., Lanzante, J. R., Peterson, T. C., Seidel, D. J. & Shine, K. P. Tropospheric temperature trends: History of an ongoing controversy. WIREs Clim. Change 2 , 66–88 (2011).
Mears, C. A. & Wentz, F. J. Construction of the remote sensing systems V3. 2 atmospheric temperature records from the MSU and AMSU microwave sounders. J. Atmos. Ocean. Tech. 26 , 1040–1056 (2009).
Xu, J. & Powell, A. Jr Uncertainty of the stratospheric/tropospheric temperature trends in 1979–2008: Multiple Satellite MSU, radiosonde, and reanalysis datasets. Atmos. Chem. Phys. Discuss. 11 , 10727–10732 (2011).
Christy, J. R., Spencer, R. W. & Norris, W. B. The role of remote sensing in monitoring global bulk tropospheric temperatures. Int. J. Remote Sens. 32 , 671–685 (2011).
Fu, Q., Manabe, S. & Johanson, C. M. On the warming in the tropical upper troposphere: Models versus observations. Geophys. Res. Lett. 38 , L15704 (2011).
Frei, A. A new generation of satellite snow observations for large scale earth system studies. Geog. Compass 3 , 879–902 (2009).
Brown, R. D. & Robinson, D. A. Northern Hemisphere spring snow cover variability and change over 1922–2010 including an assessment of uncertainty. Cryosphere. 5 , 219–229 (2011).
Bulygina, O., Groisman, P. Y., Razuvaev, V. & Korshunova, N. Changes in snow cover characteristics over Northern Eurasia since 1966. Environ. Res. Lett. 6 , 045204 (2011).
Parkinson, C. & Cavalieri, D. Antarctic sea ice variability and trends. Cryosphere Discuss. 6 , 931–956 (2012).
Liu, J. & Curry, J. A. Accelerated warming of the Southern Ocean and its impacts on the hydrological cycle and sea ice. Proc. Natl Acad. Sci. USA 107 , 14987 (2010).
Cavalieri, D. & Parkinson, C. Arctic sea ice variability and trends, 1979–2010 Cryosphere. 6 , 881–889 (2012).
Kattsov, V. M. et al. Arctic sea ice change: A grand challenge of climate science. J. Glaciol. 56 , 1115–1121 (2011).
Maslanik, J., Stroeve, J., Fowler, C. & Emery, W. Distribution and trends in Arctic sea ice age through spring 2011. Geophys. Res. Lett. 38 , L13502 (2011).
Horwath, M., Legresy, B., Remy, F., Blarel, F. & Lemoine, J.-M. Consistent patterns of Antarctic ice sheet interannual variations from ENVISAT radar altimetry and GRACE satellite gravimetry. Geophys. J. Int. 189 , 863–876 (2012).
Shepherd, A. et al. A reconciled estimate of ice-sheet mass balance. Science 338 , 1183–1189 (2012).
Rignot, E. et al. Accelerated ice discharge from the Antarctic Peninsula following the collapse of Larsen B ice shelf. Geophys. Res. Lett. 31 , L18401 (2004).
Joughin, I., Alley, R. B. & Holland, D. M. Ice-sheet response to oceanic forcing. Science 338 , 1172–1176 (2012).
Scherler, D., Bookhagen, B. & Strecker, M. R. Spatially variable response of Himalayan glaciers to climate change affected by debris cover. Nature Geosci. 4 , 156–159 (2011).
Jacob, T., Wahr, J., Pfeffer, W. T. & Swenson, S. Recent contributions of glaciers and ice caps to sea level rise. Nature 482 , 514–518 (2012).
Gardelle, J., Berthier, E. & Arnaud, Y. Slight mass gain of Karakoram glaciers in the early twenty-first century. Nature Geosci. 5 , 322–325 (2012).
Yao, T. et al. Different glacier status with atmospheric circulations in Tibetan Plateau and surroundings. Nature Clim. Change 2 , 663–667 (2012).
Cazenave, A. & Remy, F. Sea level and climate: Measurements and causes of changes. WIREs Clim. Change 2 , 647–662 (2011).
Church, J. A. & White, N. J. Sea-level rise from the late 19th to the early 21st century. Surv. Geophys. 32 , 585–602 (2011).
Leuliette, E. W. & Willis, J. K. Balancing the sea level budget. Oceanography 24 , 122–129 (2011).
Fu, L. L., Chelton, D. B., Le Traon, P. Y. & Morrow, R. Eddy dynamics from satellite altimetry. Oceanography 23 , 14–25 (2010).
Willis, J. K., Chambers, D. P., Kuo, C. Y. & Shum, C. K. Global sea level rise: Recent progress and challenges for the decade to come. Oceanography 23 , 26–35 (2010).
Prandi, P., Ablain, M., Cazenave, A. & Picot, N. A new estimation of mean sea level in the Arctic ocean from satellite altimetry. Mar. Geod. 35 , 61–81 (2012).
Hansen, J. et al. Climate simulations for 1880–2003 with GISS modelE. Clim. Dynam. 29 , 661–696 (2007).
Scafetta, N. & West, B. J. Phenomenological solar contribution to the 1900–2000 global surface warming. Geophys. Res. Lett. 33 , L05708 (2006).
Benestad, R. & Schmidt, G. Solar trends and global warming. J. Geophys. Res. 114 , D14101 (2009).
Harder, J. W., Fontenla, J. M., Pilewskie, P., Richard, E. C. & Woods, T. N. Trends in solar spectral irradiance variability in the visible and infrared. Geophys. Res. Lett. 36 , L07801 (2009).
Haigh, J. D., Winning, A. R., Toumi, R. & Harder, J. W. An influence of solar spectral variations on radiative forcing of climate. Nature 467 , 696–699 (2010).
Ineson, S. et al. Solar forcing of winter climate variability in the Northern Hemisphere. Nature Geosci. 4 , 753–757 (2011).
Lean, J. L. & DeLand, M. T. How does the Sun's spectrum wary? J. Clim. 25 , 2555–2560 (2012).
Matthes, K. Atmospheric science: Solar cycle and climate predictions. Nature Geosci. 4 , 735–736 (2011).
Quaas, J., Boucher, O., Bellouin, N. & Kinne, S. Satellite-based estimate of the direct and indirect aerosol climate forcing. J. Geophys. Res 113 , D05204 (2008).
Zhao, T. X. P., Loeb, N. G., Laszlo, I. & Zhou, M. Global component aerosol direct radiative effect at the top of atmosphere. Int. J. Remote Sens. 32 , 633–655 (2011).
Cermak, J., Wild, M., Knutti, R., Mishchenko, M. I. & Heidinger, A. K. Consistency of global satellite-derived aerosol and cloud data sets with recent brightening observations. Geophys. Res. Lett. 37 , L21704 (2010).
De Meij, A., Pozzer, A. & Lelieveld, J. Trend analysis in aerosol optical depths and pollutant emission estimates between 2000 and 2009. Atmos. Environ. 51 , 75–85 (2012).
Solomon, S. et al. The persistently variable “Background” stratospheric aerosol layer and global climate change. Science 333 , 866–870 (2011).
Vernier, J. P. et al. Major influence of tropical volcanic eruptions on the stratospheric aerosol layer during the last decade. Geophys. Res. Lett. 38 , L12807 (2011).
Penner, J. E., Xu, L. & Wang, M. Satellite methods underestimate indirect climate forcing by aerosols. Proc. Natl Acad. Sci. USA 108 , 13404–13408 (2011).
Quaas, J., Boucher, O., Bellouin, N. & Kinne, S. Which of satellite- or model-based estimates is closer to reality for aerosol indirect forcing? Proc. Natl Acad. Sci. USA 108 , E1099–E1099 (2011).
Choi, Y. S., Lindzen, R. S., Ho, C. H. & Kim, J. Space observations of cold-cloud phase change. Proc. Natl Acad. Sci. USA 107 , 11211–11216 (2010).
Jiang, J. H. et al. Clean and polluted clouds: Relationships among pollution, ice clouds, and precipitation in South America. Geophys. Res. Lett. 35 , L14804 (2008).
Allan, R. P. Combining satellite data and models to estimate cloud radiative effect at the surface and in the atmosphere. Meteorol. Appl. 18 , 324–333 (2011).
Dessler, A. E. A determination of the cloud feedback from climate variations over the past decade. Science 330 , 1523–1527 (2010).
Davies, R. & Molloy, M. Global cloud height fluctuations measured by MISR on Terra from 2000 to 2010. Geophys. Res. Lett. 39 , L03701 (2012).
Taylor, P. C. The role of clouds: An introduction and rapporteur report. Surv. Geophys. 33 , 609–617 (2012).
Schmidt, G. A., Ruedy, R. A., Miller, R. L. & Lacis, A. A. Attribution of the present-day total greenhouse effect. J. Geophys. Res. 115 , D20106 (2010).
Dessler, A. E. & Davis, S. M. Trends in tropospheric humidity from reanalysis systems. J. Geophys. Res. 115 (2010).
Trenberth, K. E., Fasullo, J. & Smith, L. Trends and variability in column-integrated atmospheric water vapor. Clim. Dynam. 24 , 741–758 (2005).
Gu, G. & Adler, R. F. Large-scale, inter-annual relations among surface temperature, water vapour and precipitation with and without ENSO and volcano forcings. Int. J. Climatol. 32 , 1782–1791 (2012).
Shi, L. & Bates, J. J. Three decades of intersatellite-calibrated high-resolution infrared radiation sounder upper tropospheric water vapor. J. Geophys. Res. D Atmos. 116 (2011).
Jin, X., Li, J., Schmit, T. J. & Goldberg, M. D. Evaluation of radiative transfer models in atmospheric profiling with broadband infrared radiance measurements. Int. J. Remote Sens. 32 , 863–874 (2011).
Solomon, S. et al. Contributions of stratospheric water vapor to decadal changes in the rate of global warming. Science 327 , 1219 (2010).
Randel, W. J. Variability and trends in stratospheric temperature and water vapor. Geophys. Monog. Series 190 , 123–135 (2010).
Fueglistaler, S. Stepwise changes in stratospheric water vapor? J. Geophys. Res. D Atmos. 117 , D13302 (2012).
Wentz, F. J., Ricciardulli, L., Hilburn, K. & Mears, C. How much more rain will global warming bring? Science 317 , 233–235 (2007).
Liepert, B. G. & Previdi, M. Do models and observations disagree on the rainfall response to global warming? J. Clim. 22 , 3156–3166 (2009).
Liu, C. & Allan, R. P. Multisatellite observed responses of precipitation and its extremes to interannual climate variability. J. Geophys. Res. 117 , D03101 (2012).
Gruber, A. & Levizzani, V. Assessment of Global Precipitation Products WCRP-128 50 (World Climate Research Programme, 2008).
Gebregiorgis, A. S. & Hossain, F. Understanding the dependence of satellite rainfall uncertainty on topography and climate for hydrologic model simulation. IEEE Trans. Geosci. Remote Sens. 51 , 704–718 (2013).
Wang, B., Liu, J., Kim, H. J., Webster, P. J. & Yim, S. Y. Recent change of the global monsoon precipitation (1979–2008). Clim. Dynam. 39 , 1123–1135 (2012).
Trenberth, K. E., Moore, B., Karl, T. R. & Nobre, C. Monitoring and prediction of the earth's climate: A future perspective. J. Clim. 19 , 5001–5008 (2006).
Henson, S. A. et al. Detection of anthropogenic climate change in satellite records of ocean chlorophyll and productivity. Biogeosciences 7 , 621–640 (2010).
Douglas, B. C. Global sea rise: A redetermination. Surv. Geophys. 18 , 279–292 (1997).
Kahn, B. H. et al. Temperature and water vapor variance scaling in global models: Comparisons to satellite and aircraft data. J. Atmos. Sci. 68 , 2156–2168 (2011).
Datla, R., Kessel, R., Smith, A., Kacker, R. & Pollock, D. Review Article: Uncertainty analysis of remote sensing optical sensor data: Guiding principles to achieve metrological consistency. Int. J. Remote Sens. 31 , 867–880 (2010).
Mears, C. A. & Wentz, F. J. The effect of diurnal correction on satellite-derived lower tropospheric temperature. Science 309 , 1548 (2005).
Mei, L. et al. Validation and analysis of aerosol optical thickness retrieval over land. Int. J. Remote Sens. 33 , 781–803 (2012).
Screen, J. Sudden increase in Antarctic sea ice: Fact or artifact? Geophys. Res. Lett. 38 , L13702 (2011).
Ohring, G., Wielicki, B., Spencer, R., Emery, B. & Datla, R. Satellite instrument calibration for measuring global climate change: Report of a workshop. Bull. Am. Meteorol. Soc. 86 , 1303–1313 (2005).
Li, Z. et al. Uncertainties in satellite remote sensing of aerosols and impact on monitoring its long-term trend: A review and perspective. Ann. Geophys 27 , 2755–2770 (2009).
Crow, W. T. et al. Upscaling sparse ground-based soil moisture observations for the validation of coarse-resolution satellite soil moisture products. Rev. Geophys. 50 , 1–20 (2012).
Liu, Y., Liu, R. & CHen, J. M. Retrospective retrieval of long-term consistent global leaf area index (1981–2010) maps from combined AVHRR and MODIS data J. Geophys. Res. 117 , G04003 (2012).
Knapp, K. R. et al. Globally Gridded Satellite observations for climate studies. Bull. Am. Meteorol. Soc. 92 , 893–907 (2011).
Download references
Acknowledgements
This work was supported by the National High-tech Research and Development Program of China (Grant No. 2009AA12200101) and the National Key Basic Research Program of China (Grant No. 2010CB530300).
Author information
Authors and affiliations.
Ministry of Education Key Laboratory for Earth System Modeling, Center for Earth System Science, Tsinghua University, Beijing, 100084, China
Jun Yang & Peng Gong
State Key Lab of Remote Sensing Science, jointly sponsored by the Institute of Remote Sensing and Digital Earth, Chinese Academy of Science, and Beijing Normal University, Beijing, 100101, China
Peng Gong & Jiancheng Shi
Department of Environmental Science, Policy and Management, University of California, Berkeley, 94720, California, USA
Jackson School of Geosciences, University of Texas, Austin, 78712, Texas, USA
Rong Fu & Robert Dickinson
School of Marine and Atmospheric Sciences, State University of New York, Stony Brook, 11794, New York, USA
Minghua Zhang
International Institute for Earth System Science, Nanjing University, Nanjing, 210093, China
Jingming Chen
Department of Geography and City Planning, University of Toronto, Toronto, M5S 3G3, Ontario, Canada
College of Global Change and Earth System Science, Beijing Normal University, Beijing, 100875, China
Shunlin Liang & Bing Xu
Department of Geography, University of Maryland, College Park, 20742, Maryland, USA
Shunlin Liang
College of Environmental Science and Engineering, Tsinghua University, Beijing, 100084, China
You can also search for this author in PubMed Google Scholar
Contributions
J.Y. and P.G. designed the framework of the Review. All authors contributed to writing.
Corresponding author
Correspondence to Peng Gong .
Ethics declarations
Competing interests.
The authors declare no competing financial interests.
Supplementary information
Supplementary information.
This file contains supplementary tables 1-3 and additional references for table S1. (PDF 1110 kb)
Rights and permissions
Reprints and permissions
About this article
Cite this article.
Yang, J., Gong, P., Fu, R. et al. The role of satellite remote sensing in climate change studies. Nature Clim Change 3 , 875–883 (2013). https://doi.org/10.1038/nclimate1908
Download citation
Received : 23 October 2012
Accepted : 23 April 2013
Published : 15 September 2013
Issue Date : October 2013
DOI : https://doi.org/10.1038/nclimate1908
Share this article
Anyone you share the following link with will be able to read this content:
Sorry, a shareable link is not currently available for this article.
Provided by the Springer Nature SharedIt content-sharing initiative
This article is cited by
Nature-based solutions can help restore degraded grasslands and increase carbon sequestration in the tibetan plateau.
- Yingxin Wang
Communications Earth & Environment (2024)
Remote sensing and GIS techniques in Monitoring and mapping Land System Change in semi-arid environments
- YongAn Zhao
- Mohamed Abdelkareem
- Fathy Abdalla
Environmental Earth Sciences (2024)
Study on Digital Twin Technologies for Watershed Information Modeling (WIM): A Systematic Literature Review and Bibliometric Analysis
- Hongchen Liu
- Haijiang Li
Archives of Computational Methods in Engineering (2024)
Real-time shipborne multi-GNSS atmospheric water vapor retrieval over the South China Sea
GPS Solutions (2023)
The main inherent uncertainty sources in trend estimation based on satellite remote sensing data
- Jianguang Wen
Theoretical and Applied Climatology (2023)
Quick links
- Explore articles by subject
- Guide to authors
- Editorial policies
Sign up for the Nature Briefing newsletter — what matters in science, free to your inbox daily.

IEEE Account
- Change Username/Password
- Update Address
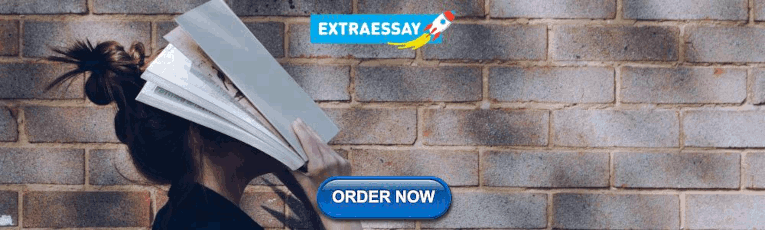
Purchase Details
- Payment Options
- Order History
- View Purchased Documents
Profile Information
- Communications Preferences
- Profession and Education
- Technical Interests
- US & Canada: +1 800 678 4333
- Worldwide: +1 732 981 0060
- Contact & Support
- About IEEE Xplore
- Accessibility
- Terms of Use
- Nondiscrimination Policy
- Privacy & Opting Out of Cookies
A not-for-profit organization, IEEE is the world's largest technical professional organization dedicated to advancing technology for the benefit of humanity. © Copyright 2024 IEEE - All rights reserved. Use of this web site signifies your agreement to the terms and conditions.
Journal of the Indian Society of Remote Sensing
- Shailesh Nayak
Societies and partnerships
Latest issue
Volume 52, Issue 8
Latest articles
Spatial and spectral analysis of resampling algorithms in image fusion of optical and microwave satellite images: a case study over western himalayas.
- Rajinder Kaur
- Sartajvir Singh
- Ganesh Kumar Sethi
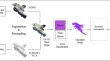
DEALB: A Post-classification Framework for Regionalizing Local Climate Zones in the Urban Environment
- Mrunali Vaidya
- Ravindra Keskar
- Rajashree Kotharkar
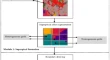
Automatic Building Extraction from Multispectral LiDAR Using Novel 3D Spatial Indices and Deep Learning Point CNN
- Asmaa A. Mandouh
- Mahmoud El Nokrashy O. Ali
- Sayed A. Mohamed
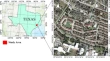
Assessment and Evaluation of Flood Vulnerability of Chhota Udepur District, Gujarat, India Using Analytical Hierarchy Process: A Case Study
- Dhara Kakwani
- Gargi Asodariya
- Bablu Prasad
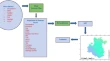
Assessing Regional Precipitation Patterns Using Multiple Global Satellite-Based Datasets in the Upper Citarum Watershed, Indonesia
- Muhammad Iqbal Habibie
- Robby Arifandri
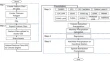
Journal updates
Special issue on advanced polarimetric radar remote sensing techniques and applications.
Lead Guest Editor: Prof. Gulab Singh, Indian Institute of Technology Bombay, Mumbai
Guest Editors:
1. Dr. Raj Kumar, Space Applications Centre (ISRO), India
2. Dr. Thomas Ainsworth, Kent State University, USA
3. Prof. Josaphat Tetuko Sri Sumantyo, Chiba University, Japan
4. Dr. Jorge Arigony, Universidade Federal do Rio Grande, Brazil
Submission Deadline: May 31, 2024
Special Issue on Science and Applications from ISRO’s EOS-06/Oceansat-3 Mission
Dr Mini Raman, Former Scientist, SAC-ISRO, Ahmedabad; Email: [email protected]
Dr Rashmi Sharma, Deputy Director, SAC-ISRO, Ahmedabad; Email: [email protected]
Dr Prakash Chauhan, Director, NRSC-ISRO, Hyderabad; Email: [email protected]
Submission Deadline: August 31, 2024
Journal information
- Astrophysics Data System (ADS)
- CAB Abstracts
- Engineering Village – GEOBASE
- Google Scholar
- Japanese Science and Technology Agency (JST)
- OCLC WorldCat Discovery Service
- Science Citation Index Expanded (SCIE)
- Semantic Scholar
- TD Net Discovery Service
- UGC-CARE List (India)
Rights and permissions
Editorial policies
© Indian Society of Remote Sensing
- Find a journal
- Publish with us
- Track your research
Information
- Author Services
Initiatives
You are accessing a machine-readable page. In order to be human-readable, please install an RSS reader.
All articles published by MDPI are made immediately available worldwide under an open access license. No special permission is required to reuse all or part of the article published by MDPI, including figures and tables. For articles published under an open access Creative Common CC BY license, any part of the article may be reused without permission provided that the original article is clearly cited. For more information, please refer to https://www.mdpi.com/openaccess .
Feature papers represent the most advanced research with significant potential for high impact in the field. A Feature Paper should be a substantial original Article that involves several techniques or approaches, provides an outlook for future research directions and describes possible research applications.
Feature papers are submitted upon individual invitation or recommendation by the scientific editors and must receive positive feedback from the reviewers.
Editor’s Choice articles are based on recommendations by the scientific editors of MDPI journals from around the world. Editors select a small number of articles recently published in the journal that they believe will be particularly interesting to readers, or important in the respective research area. The aim is to provide a snapshot of some of the most exciting work published in the various research areas of the journal.
Original Submission Date Received: .
- Active Journals
- Find a Journal
- Proceedings Series
- For Authors
- For Reviewers
- For Editors
- For Librarians
- For Publishers
- For Societies
- For Conference Organizers
- Open Access Policy
- Institutional Open Access Program
- Special Issues Guidelines
- Editorial Process
- Research and Publication Ethics
- Article Processing Charges
- Testimonials
- Preprints.org
- SciProfiles
- Encyclopedia
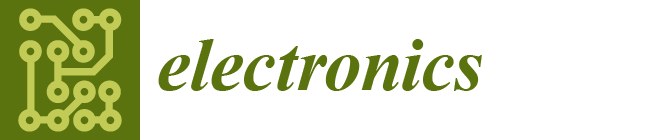
Article Menu
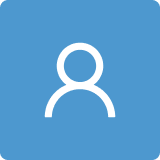
- Subscribe SciFeed
- Recommended Articles
- Author Biographies
- Google Scholar
- on Google Scholar
- Table of Contents
Find support for a specific problem in the support section of our website.
Please let us know what you think of our products and services.
Visit our dedicated information section to learn more about MDPI.
JSmol Viewer
A review of research on spectrum sensing based on deep learning.
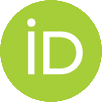
1. Introduction
- Traditional approaches to radio spectrum sensing are studied, and a detailed summary of the strengths and weaknesses of each method is provided.
- The applications of CNNs, long and short-term memory networks (LSTMs), combinatorial neural networks, and other types of neural networks in radio spectrum sensing are described. A comprehensive review of deep-learning-based spectrum-sensing algorithms for the period 2021 to 2023 is presented.
- The paper concludes with a summary of the applications, current state of research, and challenges faced by deep learning in cooperative spectrum sensing.
2. Spectrum-Sensing Methods
2.1. spectrum-sensing modeling and performance metrics.
- Detection probability ( P d ): This is the probability that a secondary user detects the presence of the primary user when the primary user is present, denoted by P d = P r H 1 ∣ H 1 .
- False alarm probability ( P f ): This is the probability that a secondary user incorrectly believes that the primary user exists when the primary user does not exist, denoted by f = P r H 1 ∣ H 0 .
- Missed detection probability ( P m ): This is the probability that a secondary user mistakenly believes that the primary user does not exist when the primary user exists, which can be expressed as P m = P r H 0 ∣ H 1 . The sum of the probability of having a detection and the probability of missing a detection is 1, which can be expressed as P m + P d = 1 .
2.2. Single-Node Spectrum Sensing
- Spectrum monitoring and management: Single-node spectrum sensing monitors and manages spectrum utilization in specific frequency bands. It uses techniques to determine the utilization, interference, and possible availability of free spectrum in that band.
- Spectrum sharing: Single-node spectrum sensing enables dynamic spectrum sharing. Nodes can monitor the available spectrum and share this information with other devices or networks to improve the efficient utilization of spectrum resources.
- Interference detection and elimination: Single-node spectrum awareness detects potential sources of interference by monitoring the surrounding spectrum environment and taking appropriate measures to eliminate interference. This improves the performance and reliability of the communication system.
- Traditional algorithms for single-node spectrum sensing mainly include energy detection algorithms, cyclostationary feature algorithms, and matched filter detection algorithms [ 25 ]. In the next section, these traditional methods are explained in detail, basic simulation experiments are performed, and finally, the advantages and disadvantages of each method are compared and summarized.
2.2.1. Energy Detection
2.2.2. cyclostationary feature detection, 2.2.3. matched filter detection.
- PU signal uncertainty: Under the current spectrum management mechanism, each PU has absolute priority to use its working frequency band, and if a local node or secondary user requires prior knowledge of a PU’s signal, such as modulation coding method or timing, the PU is not obliged to provide it. Therefore, it is very difficult for the SUs to perceive the signal uncertainty in the PUs in the real spectrum.
- Complexity of real environments: The spectrum sensing range of a single node is generally physically limited, as it may be influenced by propagation distance and large obstacles such as plateaus and trees. This limits the range and accuracy of the spectrum resources that nodes can perceive. In wide area communication systems, multiple nodes may need to be deployed to extend the sensing range.
- Spectrum resource competition: A single node performing spectrum sensing may compete with other devices for the same spectrum resources. This can lead to a decrease in the accuracy of the spectrum sensing and may require additional coordination mechanisms to manage the allocation of spectrum resources.
2.3. Cooperative Spectrum Sensing
2.3.1. centralized cooperative spectrum detection, 2.3.2. distributed cooperative spectrum detection, 2.3.3. relay-assisted cooperative spectrum detection, 2.4. summary of conventional spectrum-sensing methods, 3. deep-learning-based spectrum-sensing methods, 3.1. application of convolutional neural networks to spectrum sensing, 3.1.1. convolutional neural networks, 3.1.2. spectrum-sensing method based on convolutional neural network.
- A CNN-based spectrum-sensing method for signal time–frequency domain information
3.1.3. Residual Network
3.1.4. application of residual neural networks to spectrum sensing, 3.2. application of long short-term memory networks to spectrum sensing, 3.2.1. lstm, 3.2.2. lstm-based spectrum-sensing methods, 3.3. other neural networks in spectrum sensing, 4. deep-learning-based cooperative spectrum sensing, 4.1. applications of deep neural networks in cooperative spectrum sensing, 4.2. applications of deep reinforcement learning to cooperative spectrum sensing.
Author | Year | Module | Performance | Application Scenario |
---|---|---|---|---|
W. Lee et al. [ ] | 2019 | CNN-DCS | DCS (HD): Pd = 95% (Pf = 0.5); DCS (SD): Pd = 95.2% (Pf = 0.5) | Under harsh sensing conditions, CSS with correlated individual spectrum sensing. |
Chen Z et al. [ ] | 2020 | CSS-CNN | Pd = 90% (−16 dB, 20 SUs, Pf = 0.01) | Distributed secondary users accept perceptual samples in severe channel fading and shadowing environments. |
P. Shachi et al. [ ] | 2020 | CNN | Test accuracy: 98.34% (scenario 3); 100% (scenario 2) | Spectrum sensing performance analysis in dynamic scenarios with different noise floors and spaces. |
Hang Liu et al. [ ] | 2019 | EL+semi-soft FC | Pd = 96% (−18 dB, 60 SUs) | CSS for cognitive radio systems under OFDM-based signal. |
Myke D. M. Valadão et al. [ ] | 2022 | ResNet | Accuracy = 92% (NSD: −114 dBm −174 dBm, 5 SUs) | Dynamic displacement of the user within a given area over a time period. |
Raghunatha Rao D et al. [ ] | 2022 | Deep ResNet data-cleansing algorithm | Pd = 95.78% (the matrix size of 10 × 5, Rician channel) | Sensing spectrum availability with crowd sensors via DNR. |
Dimpal Janu et al. [ ] | 2023 | GCN-CSS | Pd = 100% (−8 dB, Pf = 0.1) | Dynamics of the wireless environment in CR networks. |
Shuai Liu et al. [ ] | 2021 | DDQN | Average cumulative collision rate = 0.06. Average cumulative reward = 0.91 | Dynamic cooperative spectrum-sensing environments where multiple PUs or multiple SUs can encounter conflicts. |
Yunzeng Li et al. [ ] | 2020 | DQN | Modified decision accuracy = 100% (index of system scenarios = 2) | Dynamic spectrum sensing in wireless networks containing N correlated channels. |
Jalil S Q et al. [ ] | 2021 | CQL | Detection accuracy = 70% (−14 dB) | Improved detection accuracy of SUs against PUs and reduced energy consumption. |
Cai P et al. [ ] | 2020 | DQN+ coordination graph | Average reward of the SUs in the CG = 0.29 (number of time slots = 1500) | Certain obstacles in the physical environment allow CR to occur under the associated decay and shadows. |
Anal Paul et al. [ ] | 2022 | DQL | Pd = 90% (−10 dB) | FC in CSS is subject to data forgery attacks. |
Yu Zhang et al. [ ] | 2019 | DQN+HCB-H | Average reward of all agents = 77% (number of time slots = 1500) | Each SU gathers information from the environment and other SUs to determine its sensing strategy, which can be structured in two different time slots. |
5. Performance Comparison of Conventional Methods and Deep-Learning-Based Spectrum Sensing
6. challenges and perspectives, 6.1. further improvement of detection performance and robustness at a low snr, 6.2. investigating the robustness of sensing systems under various malicious attacks, 6.3. study of spectrum sensing under small-sample conditions, 6.4. study of dynamic threshold settings, 6.5. further research on the application of self-encoders in spectrum sensing, 7. conclusions, author contributions, data availability statement, conflicts of interest.
- Kao, H.W.; Wu, E.H.K. QoE Sustainability on 5G and Beyond 5G Networks. IEEE Wirel. Commun. 2023 , 30 , 118–125. [ Google Scholar ] [ CrossRef ]
- Chen, W.; Demirkol, I.; Mostafa, M.; Perotti, A. Mobile Communications And Networks [Series Editorial]. IEEE Commun. Mag. 2021 , 59 , 52–53. [ Google Scholar ] [ CrossRef ]
- Union, I.T. Estimated Spectrum Bandwidth Requirements for the Future Development of IMT-2000 and IMT-Advanced. 2006. Available online: https://www.itu.int/pub/R-REP-M.2078/zh (accessed on 5 October 2023.).
- Ali, M.; Yasir, M.N.; Bhatti, D.M.S.; Nam, H. Optimization of spectrum utilization efficiency in cognitive radio networks. IEEE Wirel. Commun. Lett. 2022 , 12 , 426–430. [ Google Scholar ] [ CrossRef ]
- Mitola, J. Cognitive Radio. An Integrated Agent Architecture for Software Defined Radio. 2002. Available online: https://www.semanticscholar.org/paper/Cognitive-Radio-An-Integrated-Agent-Architecture-Mitola/82dc0e2ea785f4870816764c25f3d9ae856d9809 (accessed on 5 October 2023).
- Zhao, Y.; Wang, C.; Hu, M.; Wu, H.; Lin, F.; Liu, Z. A cooperative wideband spectrum sensing framework in the frequency domain for resource allocation with 5G-NR. Measurement 2022 , 191 , 110756. [ Google Scholar ] [ CrossRef ]
- Lim, C.H. Spectrum sensing for a radar signal with pulse compression in cognitive radios. IEEE Commun. Lett. 2022 , 26 , 3074–3078. [ Google Scholar ] [ CrossRef ]
- Junior, M.M.; Guo, B. Sensing spectrum sharing based massive MIMO radar for drone tracking and interception. PLoS ONE 2022 , 17 , e0268834. [ Google Scholar ] [ CrossRef ]
- Rafiqi, H.; Mahendru, G.; Gupta, S.H. Effect of Relay-based Communication on Probability of Detection for Spectrum Sensing in LoRaWAN. Wirel. Pers. Commun. 2023 , 130 , 2345–2366. [ Google Scholar ] [ CrossRef ]
- Ali, A.; Hamouda, W. Advances on spectrum sensing for cognitive radio networks: Theory and applications. IEEE Commun. Surv. Tutor. 2016 , 19 , 1277–1304. [ Google Scholar ] [ CrossRef ]
- Dai, L.; Jiao, R.; Adachi, F.; Poor, H.V.; Hanzo, L. Deep learning for wireless communications: An emerging interdisciplinary paradigm. IEEE Wirel. Commun. 2020 , 27 , 133–139. [ Google Scholar ] [ CrossRef ]
- Seo, D.; Nam, H. Deep rp-cnn for burst signal detection in cognitive radios. IEEE Access 2020 , 8 , 167164–167171. [ Google Scholar ] [ CrossRef ]
- Alkhouri, I.; Joneidi, M.; Hejazi, F.; Rahnavard, N. Large-scale spectrum occupancy learning via tensor decomposition and LSTM networks. In Proceedings of the 2020 IEEE International Radar Conference (RADAR), Washington, DC, USA, 28–30 April 2020; IEEE: Piscataway, NJ, USA, 2020; pp. 677–682. [ Google Scholar ]
- Du, K.; Wan, P.; Wang, Y.; Ai, X.; Chen, H. Spectrum sensing method based on information geometry and deep neural network. Entropy 2020 , 22 , 94. [ Google Scholar ] [ CrossRef ] [ PubMed ]
- Gai, J.; Xue, X.; Li, Z.; Zhang, L. Spectrum Sensing Method Based on Residual Cellular Network. IEEE Access 2022 , 10 , 61354–61365. [ Google Scholar ] [ CrossRef ]
- Tan, Y.; Jing, X. Cooperative spectrum sensing based on convolutional neural networks. Appl. Sci. 2021 , 11 , 4440. [ Google Scholar ] [ CrossRef ]
- Chen, Z.; Xu, Y.Q.; Wang, H.; Guo, D. Federated learning-based cooperative spectrum sensing in cognitive radio. IEEE Commun. Lett. 2021 , 26 , 330–334. [ Google Scholar ] [ CrossRef ]
- Yucek, T.; Arslan, H. A survey of spectrum sensing algorithms for cognitive radio applications. IEEE Commun. Surv. Tutorials 2009 , 11 , 116–130. [ Google Scholar ] [ CrossRef ]
- Claudino, L.; Abrao, T. Spectrum sensing methods for cognitive radio networks: A review. Wirel. Pers. Commun. 2017 , 95 , 5003–5037. [ Google Scholar ] [ CrossRef ]
- Gattoua, C.; Chakkor, O.; Aytouna, F. An overview of cooperative spectrum sensing based on machine learning techniques. In Proceedings of the 2020 IEEE 2nd International Conference on Electronics, Control, Optimization and Computer Science (ICECOCS), Kenitra, Morocco, 2–3 December 2020; IEEE: Piscataway, NJ, USA, 2020; pp. 1–8. [ Google Scholar ]
- Janu, D.; Singh, K.; Kumar, S. Machine learning for cooperative spectrum sensing and sharing: A survey. Trans. Emerg. Telecommun. Technol. 2022 , 33 , e4352. [ Google Scholar ] [ CrossRef ]
- Obite, F.; Usman, A.D.; Okafor, E. An overview of deep reinforcement learning for spectrum sensing in cognitive radio networks. Digit. Signal Process. 2021 , 113 , 103014. [ Google Scholar ] [ CrossRef ]
- Syed, S.N.; Lazaridis, P.I.; Khan, F.A.; Ahmed, Q.Z.; Hafeez, M.; Ivanov, A.; Poulkov, V.; Zaharis, Z.D. Deep Neural Networks for Spectrum Sensing: A Review. IEEE Access 2023 , 11 , 89591–89615. [ Google Scholar ] [ CrossRef ]
- Huang, X.L.; Xu, Y.; Wu, J.; Zhang, W. Noncooperative spectrum sensing with historical sensing data mining in cognitive radio. IEEE Trans. Veh. Technol. 2017 , 66 , 8863–8871. [ Google Scholar ] [ CrossRef ]
- Mahajan, K.; Garg, U. An enhancement to the existing cyclostationary feature detection in CRN. Multimed. Tools Appl. 2022 , 81 , 37087–37099. [ Google Scholar ] [ CrossRef ]
- Luo, J.; Zhang, G.; Yan, C. An energy detection-based spectrum-sensing method for cognitive radio. Wirel. Commun. Mob. Comput. 2022 , 2022 , 3933336. [ Google Scholar ] [ CrossRef ]
- Saad, M.A.; Mustafa, S.; Ali, M.H.; Hashim, M.; Ismail, M.B.; Ali, A.H. Spectrum sensing and energy detection in cognitive networks. Indones. J. Electr. Eng. Comput. Sci. 2020 , 17 , 465–472. [ Google Scholar ] [ CrossRef ]
- Sudhamani, C.; Saxena, A.; Aswini, V. Improved detection performance of energy detection based spectrum sensing in cognitive radio networks. Int. J. Sensors Wirel. Commun. Control 2021 , 11 , 957–962. [ Google Scholar ] [ CrossRef ]
- Ilgin, F.Y. Double Threshold Energy Based Spectrum Sensing with Copulas. Wirel. Pers. Commun. 2022 , 126 , 2937–2948. [ Google Scholar ] [ CrossRef ]
- Wu, W.; Wang, Z.; Yuan, L.; Zhou, F.; Lang, F.; Wang, B.; Wu, Q. IRS-enhanced energy detection for spectrum sensing in cognitive radio networks. IEEE Wirel. Commun. Lett. 2021 , 10 , 2254–2258. [ Google Scholar ] [ CrossRef ]
- Yang, M.; Li, Y.; Liu, X.; Tang, W. Cyclostationary feature detection based spectrum sensing algorithm under complicated electromagnetic environment in cognitive radio networks. China Commun. 2015 , 12 , 35–44. [ Google Scholar ] [ CrossRef ]
- Nouri, M.; Behroozi, H.; Mallat, N.K.; Aghdam, S.A. A wideband 5G cyclostationary spectrum sensing method by kernel least mean square algorithm for cognitive radio networks. IEEE Trans. Circ. Syst. II Express Briefs 2021 , 68 , 2700–2704. [ Google Scholar ] [ CrossRef ]
- Mathew, S.G.; Samuel, C.P. A Novel Low-Complexity Cyclostationary Feature Detection Using Sub-Nyquist Samples for Wideband Spectrum Sensing. Circuits Syst. Signal Process. 2021 , 40 , 6371–6386. [ Google Scholar ] [ CrossRef ]
- Brito, A.; Sebastião, P.; Velez, F.J. Hybrid matched filter detection spectrum sensing. IEEE Access 2021 , 9 , 165504–165516. [ Google Scholar ] [ CrossRef ]
- Zhang, C.; Li, J.; Li, B.; Ma, W. Blind Matching Filtering Algorithm for Spectrum Sensing under Multi-Path Channel Environment. Electronics 2023 , 12 , 2499. [ Google Scholar ] [ CrossRef ]
- Kabeel, A.A.; Hussein, A.H.; Khalaf, A.A.; Hamed, H.F. A utilization of multiple antenna elements for matched filter based spectrum sensing performance enhancement in cognitive radio system. AEU-Int. J. Electron. Commun. 2019 , 107 , 98–109. [ Google Scholar ] [ CrossRef ]
- Cichoń, K.; Kliks, A.; Bogucka, H. Energy-efficient cooperative spectrum sensing: A survey. IEEE Commun. Surv. Tutor. 2016 , 18 , 1861–1886. [ Google Scholar ] [ CrossRef ]
- Shinde, S.C.; Jadhav, A.N. Centralized cooperative spectrum sensing with energy detecion in cognitive radio and optimization. In Proceedings of the 2016 IEEE International Conference on Recent Trends in Electronics, Information & Communication Technology (RTEICT), Bangalore, India, 20–21 May 2016; pp. 1002–1006. [ Google Scholar ] [ CrossRef ]
- Na, W.; Yoon, J.; Cho, S.; Griffith, D.; Golmie, N. Centralized cooperative directional spectrum sensing for cognitive radio networks. IEEE Trans. Mob. Comput. 2017 , 17 , 1260–1274. [ Google Scholar ] [ CrossRef ]
- Khaf, S.; Alkhodary, M.T.; Kaddoum, G. Partially cooperative scalable spectrum sensing in cognitive radio networks under SDF attacks. IEEE Internet Things J. 2021 , 9 , 8901–8912. [ Google Scholar ] [ CrossRef ]
- Yun, C. An underwater cooperative spectrum sharing protocol for a centralized underwater cognitive acoustic network. Sensors 2022 , 22 , 5754. [ Google Scholar ] [ CrossRef ]
- Mokhtar, R.A.; Saeed, R.A.; Alhumyani, H.; Khayyat, M.; Abdel-Khalek, S. Cluster mechanism for sensing data report using robust collaborative distributed spectrum sensing. Cluster Comput. 2021 , 25 , 2541–2556. [ Google Scholar ] [ CrossRef ]
- Gharib, A.; Ejaz, W.; Ibnkahla, M. Enhanced multiband multiuser cooperative spectrum sensing for distributed CRNs. IEEE Trans. Cogn. Commun. Netw. 2019 , 6 , 256–270. [ Google Scholar ] [ CrossRef ]
- Akyildiz, I.F.; Lo, B.F.; Balakrishnan, R. Cooperative spectrum sensing in cognitive radio networks: A survey. Phys. Commun. 2011 , 4 , 40–62. [ Google Scholar ] [ CrossRef ]
- Russakovsky, O.; Deng, J.; Su, H.; Krause, J.; Satheesh, S.; Ma, S.; Huang, Z.; Karpathy, A.; Khosla, A.; Bernstein, M.; et al. Imagenet large scale visual recognition challenge. Int. J. Comput. Vis. 2015 , 115 , 211–252. [ Google Scholar ] [ CrossRef ]
- Zastrow, M. South Korea Trumpets $ 860-million AI Fund after AlphaGo’Shock. 2016. Available online: https://www.nature.com/articles/nature.2016.19595#citeas (accessed on 5 October 2023).
- Kocoń, J.; Cichecki, I.; Kaszyca, O.; Kochanek, M.; Szydło, D.; Baran, J.; Bielaniewicz, J.; Gruza, M.; Janz, A.; Kanclerz, K.; et al. ChatGPT: Jack of all trades, master of none. Inf. Fusion 2023 , 99 , 101861. [ Google Scholar ] [ CrossRef ]
- Qin, Z.; Ye, H.; Li, G.Y.; Juang, B.H.F. Deep learning in physical layer communications. IEEE Wirel. Commun. 2019 , 26 , 93–99. [ Google Scholar ] [ CrossRef ]
- Xing, H.; Qin, H.; Luo, S.; Dai, P.; Xu, L.; Cheng, X. Spectrum sensing in cognitive radio: A deep learning based model. Trans. Emerg. Telecommun. Technol. 2022 , 33 , e4388. [ Google Scholar ] [ CrossRef ]
- Li, Z.; Liu, F.; Yang, W.; Peng, S.; Zhou, J. A survey of convolutional neural networks: Analysis, applications, and prospects. IEEE Trans. Neural Netw. Learn. Syst. 2021 , 12 , 6999–7019. [ Google Scholar ] [ CrossRef ] [ PubMed ]
- Liu, D.; Zhang, L.; Lai, X.; Liu, H. Image feature selection embedded distribution differences between classes for convolutional neural network. Appl. Soft Comput. 2022 , 131 , 109715. [ Google Scholar ] [ CrossRef ]
- Antoni, J.; Xin, G.; Hamzaoui, N. Fast computation of the spectral correlation. Mech. Syst. Signal Process. 2017 , 92 , 248–277. [ Google Scholar ] [ CrossRef ]
- Chen, Z.; Xu, Y.Q.; Wang, H.; Guo, D. Deep STFT-CNN for spectrum sensing in cognitive radio. IEEE Commun. Lett. 2020 , 25 , 864–868. [ Google Scholar ] [ CrossRef ]
- El-Shafai, W.; Fawzi, A.; Sedik, A.; Zekry, A.; El-Banby, G.M.; Khalaf, A.A.; Abd El-Samie, F.E.; Abd-Elnaby, M. Convolutional neural network model for spectrum sensing in cognitive radio systems. Int. J. Commun. Syst. 2022 , 35 , e5072. [ Google Scholar ] [ CrossRef ]
- Cai, L.; Cao, K.; Wu, Y.; Zhou, Y. Spectrum sensing based on spectrogram-aware CNN for cognitive radio network. IEEE Wirel. Commun. Lett. 2022 , 11 , 2135–2139. [ Google Scholar ] [ CrossRef ]
- Liu, C.; Wang, J.; Liu, X.; Liang, Y.C. Deep CM-CNN for spectrum sensing in cognitive radio. IEEE J. Sel. Areas Commun. 2019 , 37 , 2306–2321. [ Google Scholar ] [ CrossRef ]
- Wang, Q.; Guo, B. CNN-SVM Spectrum Sensing in Cognitive Radio Based on Signal Covariance Matrix. In Proceedings of the Journal of Physics: Conference Series; IOP Publishing: Bristol, UK, 2022; Volume 2395, p. 012052. [ Google Scholar ]
- Zhang, J.; He, Z.Q.; Rui, H.; Xu, X. Multiband joint spectrum sensing via covariance matrix-aware convolutional neural network. IEEE Commun. Lett. 2022 , 26 , 1578–1582. [ Google Scholar ] [ CrossRef ]
- Duan, Y.; Huang, F.; Xu, L.; Gulliver, T.A. Intelligent spectrum sensing algorithm for cognitive internet of vehicles based on KPCA and improved CNN. Peer-Netw. Appl. 2023 , 16 , 2202–2217. [ Google Scholar ] [ CrossRef ]
- Tekbıyık, K.; Akbunar, Ö.; Ekti, A.R.; Görçin, A.; Kurt, G.K.; Qaraqe, K.A. Spectrum sensing and signal identification with deep learning based on spectral correlation function. IEEE Trans. Veh. Technol. 2021 , 70 , 10514–10527. [ Google Scholar ] [ CrossRef ]
- Chae, K.; Kim, Y. DS2MA: A Deep Learning-Based Spectrum Sensing Scheme for a Multi-Antenna Receiver. IEEE Wirel. Commun. Lett. 2023 , 12 , 952–956. [ Google Scholar ] [ CrossRef ]
- Mao, A.; Mohri, M.; Zhong, Y. Cross-entropy loss functions: Theoretical analysis and applications. arXiv 2023 , arXiv:2304.07288. [ Google Scholar ]
- Suriya, M.; Sumithra, M. Enhancing cooperative spectrum sensing in flying cell towers for disaster management using convolutional neural networks. In Proceedings of the EAI International Conference on Big Data Innovation for Sustainable Cognitive Computing: BDCC 2018, Coimbatore, India, 13–15 December 2018; Springer: Berlin/Heidelberg, Germany, 2020; pp. 181–190. [ Google Scholar ]
- He, K.; Zhang, X.; Ren, S.; Sun, J. Deep residual learning for image recognition. In Proceedings of the IEEE Conference on Computer Vision and Pattern Recognition, Las Vegas, NV, USA, 27–30 June 2016; pp. 770–778. [ Google Scholar ]
- Chandra, S.S.; Upadhye, A.; Saravanan, P.; Gurugopinath, S.; Muralishankar, R. Deep Neural Network Architectures for Spectrum Sensing Using Signal Processing Features. In Proceedings of the 2021 IEEE International Conference on Distributed Computing, VLSI, Electrical Circuits and Robotics (DISCOVER), Nitte, India, 19–20 November 2021; IEEE: Piscataway, NJ, USA, 2021; pp. 129–134. [ Google Scholar ]
- Atalik, A.; Köse, A.; Gastpar, M. Differential Entropy of the Conditional Expectation Under Additive Gaussian Noise. IEEE Trans. Signal Process. 2022 , 70 , 4851–4866. [ Google Scholar ] [ CrossRef ]
- Hu, Q.; Luo, Z.; Xiao, W. Supervised Learning Spectrum Sensing Method via Geometric Power Feature. Electronics 2023 , 12 , 1616. [ Google Scholar ] [ CrossRef ]
- Sahasranand, K. The p-norm of circulant matrices via Fourier analysis. Concr. Oper. 2022 , 9 , 1–5. [ Google Scholar ] [ CrossRef ]
- Ren, X.; Mosavat-Jahromi, H.; Cai, L.; Kidston, D. Spatio-temporal spectrum load prediction using convolutional neural network and ResNet. IEEE Trans. Cogn. Commun. Netw. 2021 , 8 , 502–513. [ Google Scholar ] [ CrossRef ]
- Fan, J.; Cheng, J. Matrix completion by deep matrix factorization. Neural Netw. 2018 , 98 , 34–41. [ Google Scholar ] [ CrossRef ]
- Banerjee, A.; Akhtar, M.N.; Navascués, M. Local α -fractal interpolation function. Eur. Phys. J. Spec. Top. 2023 , 232 , 1043–1050. [ Google Scholar ] [ CrossRef ] [ PubMed ]
- Gai, J.; Zhang, L.; Wei, Z. Spectrum Sensing Based on STFT-ImpResNet for Cognitive Radio. Electronics 2022 , 11 , 2437. [ Google Scholar ] [ CrossRef ]
- Zhen, P.; Zhang, B.; Chen, Z.; Guo, D.; Ma, W. Spectrum Sensing Method Based on Wavelet Transform and Residual Network. IEEE Wirel. Commun. Lett. 2022 , 11 , 2517–2521. [ Google Scholar ] [ CrossRef ]
- Zhang, D.; Zhang, D. Wavelet transform. In Fundamentals of Image Data Mining: Analysis, Features, Classification and Retrieval ; Springer International Publishing: Cham, Switzerland, 2019; pp. 35–44. [ Google Scholar ]
- Xiao, J.; Zhou, Z. Research progress of RNN language model. In Proceedings of the 2020 IEEE International Conference on Artificial Intelligence and Computer Applications (ICAICA), Dalian, China, 27–29 June 2020; IEEE: Piscataway, NJ, USA, 2020; pp. 1285–1288. [ Google Scholar ]
- Sherstinsky, A. Fundamentals of recurrent neural network (RNN) and long short-term memory (LSTM) network. Phys. D Nonlinear Phenom. 2020 , 404 , 132306. [ Google Scholar ] [ CrossRef ]
- Balwani, N.; Patel, D.K.; Soni, B.; López-Benítez, M. Long short-term memory based spectrum sensing scheme for cognitive radio. In Proceedings of the 2019 IEEE 30th Annual International Symposium on Personal, Indoor and Mobile Radio Communications (PIMRC), Istanbul, Turkey, 8–11 September 2019; IEEE: Piscataway, NJ, USA, 2019; pp. 1–6. [ Google Scholar ]
- Soni, B.; Patel, D.K.; López-Benítez, M. Long short-term memory based spectrum sensing scheme for cognitive radio using primary activity statistics. IEEE Access 2020 , 8 , 97437–97451. [ Google Scholar ] [ CrossRef ]
- Bkassiny, M. A Deep Learning-based Signal Classification Approach for Spectrum Sensing using Long Short-Term Memory (LSTM) Networks. In Proceedings of the 2022 6th International Conference on Information Technology, Information Systems and Electrical Engineering (ICITISEE), Yogyakarta, Indonesia, 13–14 December 2022; IEEE: Piscataway, NJ, USA, 2022; pp. 667–672. [ Google Scholar ]
- Chen, W.; Wu, H.; Ren, S. Cm-lstm based spectrum sensing. Sensors 2022 , 22 , 2286. [ Google Scholar ] [ CrossRef ]
- Yu, L.; Chen, J.; Ding, G.; Tu, Y.; Yang, J.; Sun, J. Spectrum prediction based on Taguchi method in deep learning with long short-term memory. IEEE Access 2018 , 6 , 45923–45933. [ Google Scholar ] [ CrossRef ]
- Freddi, A.; Salmon, M.; Freddi, A.; Salmon, M. Introduction to the Taguchi method. In Design Principles and Methodologies: From Conceptualization to First Prototyping with Examples and Case Studies ; Springer: Berlin/Heidelberg, Germany, 2019; pp. 159–180. [ Google Scholar ]
- Arunachalam, G.; SureshKumar, P. Optimized Deep Learning Model for Effective Spectrum Sensing in Dynamic SNR Scenario. Comput. Syst. Sci. Eng. 2023 , 45 , 1279–1294. [ Google Scholar ] [ CrossRef ]
- Al Daweri, M.S.; Abdullah, S.; Ariffin, K.Z. A migration-based cuttlefish algorithm with short-term memory for optimization problems. IEEE Access 2020 , 8 , 70270–70292. [ Google Scholar ] [ CrossRef ]
- Shao, F.; Shen, Z. How can artificial neural networks approximate the brain? Front. Psychol. 2023 , 13 , 970214. [ Google Scholar ] [ CrossRef ]
- Ruiyan, D.; Fan, G.; Xianchao, W.; Dandan, Z.; Fulai, L. Wavelet transform and artificial neural network based spectrum sensing in cognitive radio. In Proceedings of the Journal of Physics: Conference Series; IOP Publishing: Bristol, UK, 2019; Volume 1169, p. 012064. [ Google Scholar ]
- Nasser, A.; Chaitou, M.; Mansour, A.; Yao, K.C.; Charara, H. A deep neural network model for hybrid spectrum sensing in cognitive radio. Wirel. Pers. Commun. 2021 , 118 , 281–299. [ Google Scholar ] [ CrossRef ]
- Wang, Y.; Xu, W.; Qin, Z.; Zhang, Y.; Gao, H.; Pan, M.; Lin, J. Deep neural network-based robust spectrum sensing: Exploiting phase difference distribution. In Proceedings of the ICC 2021-IEEE International Conference on Communications, Montreal, QC, Canada, 14–23 June 2021; IEEE: Piscataway, NJ, USA, 2021; pp. 1–7. [ Google Scholar ]
- Irie, K.; Kumar, S.; Nirschl, M.; Liao, H. RADMM: Recurrent adaptive mixture model with applications to domain robust language modeling. In Proceedings of the 2018 IEEE International Conference on Acoustics, Speech and Signal Processing (ICASSP), Calgary, AB, Canada, 15–20 April 2018; IEEE: Piscataway, NJ, USA, 2018; pp. 6079–6083. [ Google Scholar ]
- Zhang, X.; Ma, Y.; Liu, Y.; Wu, S.; Jiao, J.; Gao, Y.; Zhang, Q. Robust DNN-based Recovery of Wideband Spectrum Signals. IEEE Wireless Commun. Lett. 2023 , 12 , 1712–1715. [ Google Scholar ] [ CrossRef ]
- Farahani, A.; Voghoei, S.; Rasheed, K.; Arabnia, H.R. A brief review of domain adaptation. In Advances in Data Science and Information Engineering: Proceedings from ICDATA 2020 and IKE 2020 ; Springer: Berlin/Heidelberg, Germany, 2021; pp. 877–894. [ Google Scholar ]
- Zhao, R.; Ruan, Y.; Li, Y.; Li, T.; Zhang, R. CCD-GAN for Domain Adaptation in Time-frequency Localization based Wideband Spectrum Sensing. IEEE Commun. Lett. 2023 , 27 , 2521–2525. [ Google Scholar ] [ CrossRef ]
- Zhuang, F.; Qi, Z.; Duan, K.; Xi, D.; Zhu, Y.; Zhu, H.; Xiong, H.; He, Q. A comprehensive survey on transfer learning. Proc. IEEE 2020 , 109 , 43–76. [ Google Scholar ] [ CrossRef ]
- Li, X.; Hu, Z.; Shen, C.; Wu, H.; Zhao, Y. TFF_aDCNN: A Pre-Trained Base Model for Intelligent Wideband Spectrum Sensing. IEEE Trans. Veh. Technol. 2023 , 72 , 12912–12926. [ Google Scholar ] [ CrossRef ]
- Lee, W.; Kim, M.; Cho, D.H. Deep cooperative sensing: Cooperative spectrum sensing based on convolutional neural networks. IEEE Trans. Veh. Technol. 2019 , 68 , 3005–3009. [ Google Scholar ] [ CrossRef ]
- Chen, Z.; Guo, D.; Zhang, J. Deep learning for cooperative spectrum sensing in cognitive radio. In Proceedings of the 2020 IEEE 20th International Conference on Communication Technology (ICCT), Nanning, China, 28–31 October 2020; IEEE: Piscataway, NJ, USA, 2020; pp. 741–745. [ Google Scholar ]
- Shachi, P.; Sudhindra, K.; Suma, M. Convolutional neural network for cooperative spectrum sensing with spatio-temporal dataset. In Proceedings of the 2020 International Conference on Artificial Intelligence and Signal Processing (AISP), Amaravati, India, 10–12 January 2020; IEEE: Piscataway, NJ, USA, 2020; pp. 1–5. [ Google Scholar ]
- Liu, H.; Zhu, X.; Fujii, T. Ensemble deep learning based cooperative spectrum sensing with semi-soft stacking fusion center. In Proceedings of the 2019 IEEE Wireless Communications and Networking Conference (WCNC), Marrakesh, Morocco, 15–18 April 2019; IEEE: Piscataway, NJ, USA, 2019; pp. 1–6. [ Google Scholar ]
- Valadão, M.D.; Amoedo, D.A.; Pereira, A.M.; Tavares, S.A.; Furtado, R.S.; Carvalho, C.B.; Da Costa, A.L.; Júnior, W.S. Cooperative spectrum sensing system using residual convolutional neural network. In Proceedings of the 2022 IEEE International Conference on Consumer Electronics (ICCE), Las Vegas, NV, USA, 7–9 January 2022; IEEE: Piscataway, NJ, USA, 2022; pp. 1–5. [ Google Scholar ]
- Rao, D.R.; Prasad, T.J.; Prasad, M.G. Deep Learning based Cooperative Spectrum Sensing with Crowd Sensors using Data Cleansing Algorithm. In Proceedings of the 2022 International Conference on Edge Computing and Applications (ICECAA), Tamilnadu, India, 13–15 October 2022; IEEE: Piscataway, NJ, USA, 2022; pp. 1276–1281. [ Google Scholar ]
- Zhou, J.; Cui, G.; Hu, S.; Zhang, Z.; Yang, C.; Liu, Z.; Wang, L.; Li, C.; Sun, M. Graph neural networks: A review of methods and applications. AI Open 2020 , 1 , 57–81. [ Google Scholar ] [ CrossRef ]
- Janu, D.; Kumar, S.; Singh, K. A Graph Convolution Network Based Adaptive Cooperative Spectrum Sensing in Cognitive Radio Network. IEEE Trans. Veh. Technol. 2022 , 72 , 2269–2279. [ Google Scholar ] [ CrossRef ]
- Henderson, P.; Islam, R.; Bachman, P.; Pineau, J.; Precup, D.; Meger, D. Deep reinforcement learning that matters. In Proceedings of the AAAI Conference on Artificial Intelligence, New Orleans, LA, USA, 2–7 February 2018; Volume 32. [ Google Scholar ]
- Liu, S.; He, J.; Wu, J. Dynamic cooperative spectrum sensing based on deep multi-user reinforcement learning. Appl. Sci. 2021 , 11 , 1884. [ Google Scholar ] [ CrossRef ]
- Fan, J.; Wang, Z.; Xie, Y.; Yang, Z. A theoretical analysis of deep Q-learning. In Proceedings of the Learning for Dynamics and Control; PMLR: New York, NY, USA, 2020; pp. 486–489. [ Google Scholar ]
- Li, Y.; Zhang, W.; Wang, C.X.; Sun, J.; Liu, Y. Deep reinforcement learning for dynamic spectrum sensing and aggregation in multi-channel wireless networks. IEEE Trans. Cogn. Commun. Netw. 2020 , 6 , 464–475. [ Google Scholar ] [ CrossRef ]
- Jalil, S.Q.; Chalup, S.; Rehmani, M.H. Cognitive radio spectrum sensing and prediction using deep reinforcement learning. In Proceedings of the 2021 International Joint Conference on Neural Networks (IJCNN), Shenzhen, China, 18–22 July 2021; IEEE: Piscataway, NJ, USA, 2021; pp. 1–8. [ Google Scholar ]
- Cai, P.; Zhang, Y.; Pan, C. Coordination graph-based deep reinforcement learning for cooperative spectrum sensing under correlated fading. IEEE Wirel. Commun. Lett. 2020 , 9 , 1778–1781. [ Google Scholar ] [ CrossRef ]
- Paul, A.; Mishra, A.K.; Shreevastava, S.; Tiwari, A.K. Deep Reinforcement Learning based reliable spectrum sensing under SSDF attacks in Cognitive Radio networks. J. Netw. Comput. Appl. 2022 , 205 , 103454. [ Google Scholar ] [ CrossRef ]
- Zhang, Y.; Cai, P.; Pan, C.; Zhang, S. Multi-agent deep reinforcement learning-based cooperative spectrum sensing with upper confidence bound exploration. IEEE Access 2019 , 7 , 118898–118906. [ Google Scholar ] [ CrossRef ]
- Axell, E.; Leus, G.; Larsson, E.G.; Poor, H.V. Spectrum sensing for cognitive radio: State-of-the-art and recent advances. IEEE 1560 Signal Process. Mag. 2012 , 29 , 101–116. [ Google Scholar ] [ CrossRef ]
- Zeng, Y.; Liang, Y.C.; Hoang, A.T.; Zhang, R. A review on spectrum sensing for cognitive radio: Challenges and solutions. EURASIP J. Adv. Signal Process. 2010 , 2010 , 1–15. [ Google Scholar ] [ CrossRef ]
- Solanki, S.; Dehalwar, V.; Choudhary, J. Deep learning for spectrum sensing in cognitive radio. Symmetry 2021 , 13 , 147. [ Google Scholar ] [ CrossRef ]
- Zheng, S.; Ye, L.; Wang, X.; Chen, J.; Zhou, H.; Lou, C.; Zhao, Z.; Yang, X. Primary user adversarial attacks on deep learning-based spectrum sensing and the defense method. China Commun. 2021 , 18 , 94–107. [ Google Scholar ] [ CrossRef ]
- Zhang, Y.; Zhao, Z. Limited data spectrum sensing based on semi-supervised deep neural network. IEEE Access 2021 , 9 , 166423–166435. [ Google Scholar ] [ CrossRef ]
- Abdalzaher, M.S.; Elwekeil, M.; Wang, T.; Zhang, S. A deep autoencoder trust model for mitigating jamming attack in IoT assisted by cognitive radio. IEEE Syst. J. 2021 , 16 , 3635–3645. [ Google Scholar ] [ CrossRef ]
- Pan, G.; Wu, Q.; Ding, G.; Wang, W.; Li, J.; Xu, F.; Zhou, B. Deep Stacked Autoencoder Based Long-Term Spectrum Prediction Using Real-World Data. IEEE Trans. Cogn. Commun. Netw. 2023 , 9 , 534–548. [ Google Scholar ] [ CrossRef ]
Click here to enlarge figure
Article | Year | Main Contribution |
---|---|---|
A Survey of Spectrum Sensing Algorithms for Cognitive Radio Applications [ ] | 2009 | Evaluating spectrum usage in multiple dimensions. It discussed the external sensing algorithms and other alternative sensing methods. |
Spectrum Sensing Methods for Cognitive Radio Networks: A Review [ ] | 2017 | Several primary conventional spectrum-sensing techniques in CR are analyzed, and the effectiveness and shortcomings of some mature SS-CRN techniques are verified through simulation. |
An Overview of Cooperative Spectrum Sensing based on Machine Learning Techniques [ ] | 2020 | Comparison of the performance of three machine learning algorithms with conventional spectrum-sensing algorithms under two channels, AWGN and Rayleigh. |
An Overview of Deep Reinforcement Learning for Spectrum Sensing in Cognitive Radio Networks [ ] | 2021 | It presented a comprehensive overview of state-of-the-art research in the field of DRL for SS in CR. Proposing future challenges for deep reinforcement learning in spectrum sensing. |
Machine Learning for Cooperative Spectrum Sensing and Sharing: A survey [ ] | 2021 | It summarized various ML-based algorithms in the CSS and DSS domains. It justifies the applicability of supervised, unsupervised, and reinforcement ML algorithms in the CSS domain. |
Deep Neural Networks for Spectrum Sensing: A Review [ ] | 2023 | The paper describes the application of several DL algorithms to SS. The paper outlines the application of traditional ML algorithms with simple ANNs in SS The importance of RF datasets with migration learning is further discussed. |
This Review | 2023 | Outlines and compares the conventional SS approach with the CSS approach. A comprehensive overview of the application and development of CNNs, LSTMs, and other deep neural networks in SS. Further overview of the application and development of deep neural networks and deep reinforcement learning in CSS. |
Method of Spectrum Sensing | Advantages | Disadvantages |
---|---|---|
Energy Detection | The lowest complexity. Does not require pre-determined knowledge. | Selection of the threshold for detecting primary users. Inability to differentiate interference from primary users and noise. |
Matched Filter Detection | The probability of false detection is achieved in a short time. | It requires perfect knowledge of the PU signaling features. Large power consumption. |
Cyclostationary Feature Detection | Just need to know the channel status information. | Large amount of calculations and high complexity. |
Spectrum Sensing | Method | Applicable Scenario |
---|---|---|
Single-node spectrum sensing | Energy detection | When the noise level in the system is known or can be estimated, or when the statistical information about the signal is not known. |
Cyclostationary feature detection | Suitable for detecting cyclical signals, such as modulated signals and communication signals, as well as spectrum sensing in complex channel environments. | |
Matched filter detection | Known master user signal information. High-phase synchronization. | |
Cooperative spectrum sensing | Centralized cooperative spectrum sensing | Relatively stable network structure and abundant device computing resources. There is a need for unified decision making based on sensed data. Scenarios with high requirements for security and monitoring of spectrum usage [ ]. |
Distributed cooperative spectrum detection | Local information can be shared between cognitive nodes relatively frequently and fluently. Control channel resources are relatively abundant. Want to improve fault tolerance [ ]. | |
Relay-assisted cooperative spectrum detection | Environments where certain nodes cannot communicate directly with the fusion center or other nodes due to geographic location or other factors [ ]. |
Author | Year | Input | Model | Detection Probability | Advantage |
---|---|---|---|---|---|
Zhibo Chen et al. [ ] | 2021 | Time–frequency matrix | STFT-CNN | 90% (−15 dB, Pf = 0.1) | Considerable SNR robustness. Independent of signal and noise assumptions. |
Walid El-Shafai et al. [ ] | 2022 | Spectrogram images of the received signals | CNN | 98% (−10 dB) | Can efficiently discriminate between signal and noise at different SNRs. |
Lianning Cai et al. [ ] | 2022 | Spectrogram of the signal obtained by STFT | S-CNN | 90% (−8 dB, Pf = 0.1) | The method is insensitive to the modulation order. |
Chang Liu et al. [ ] | 2022 | Sample covariance matrix | CM-CNN | 93% (−14 dB, Pf = 0.1) | The proposed scheme can automatically learn more discriminative features. |
Qi Wang et al. [ ] | 2022 | Covariance matrix of the signal | CNN-SVM | 97% (−14 dB, Pf = 0.4) | Replace the softmax function in CNN with SVM to obtain the classifier to improve detection performance. |
Jintao Zhang et al. [ ] | 2022 | Multi-band sample covariance matrices | MJCM-CNN | 98% (−16 dB, Pf = 0.1) | The proposed method can efficiently learn potential dependent features across frequency bands. Performance improvement over CM-CNN. |
Yanyan Duan et al. [ ] | 2023 | Covariance matrix | KPCA-CNN | 97% (−3 dB) | The algorithm makes full use of the raw information and improves the access of cognitive users to the cognitive vehicular network. |
Kursat Tekbiyik et al. [ ] | 2021 | Spectral correlation function | SCF-CNN | 96% (6 dB) | Better performance than other DL methods under stringent channel conditions. |
Keunhong Chae et al. [ ] | 2023 | A matrix composed of auto-correlation functions for each antenna | DS2MA-CNN | 95% (−22 dB, Pf = 0.1, correlation coefficient = 0.3) | The proposed model has the best performance under impulsive noise channel. |
Author | Year | Input | Model | Detection Probability | Advantage |
---|---|---|---|---|---|
S. S. Chandra et al. [ ] | 2021 | ES feature | ResNet | 90.5% (−10 dB) | Comparing the other models with the same feature inputs, ResNet has the best performance. |
X. Ren et al. [ ] | 2022 | Spatio-temporal feature | NN-ResNet | 90% (−11 dB) | Ability to make better predictions with fewer sensors and lower error rates. |
Gai J et al. [ ] | 2022 | Two-dimensional time–frequency matrix | STFT-ImpResNet | 94% (−19 dB, noise power = 1.5 dBW) | The robustness and detection probability of this model is better than STFT-CNN, SVM, etc. |
Zhen P et al. [ ] | 2022 | Time–frequency matrix obtained after signal processing by wavelet variation | WT-ResNet | 91% (−14 dB) | Improved detection of non-stationary signals in low signal-to-noise ratio environments. |
Author | Year | Input | Model | Performances | Advantage |
---|---|---|---|---|---|
Balwani N et al. [ ] | 2019 | The previous sensing event and present sensing event | LSTM | Pd = 91% (−10 dB) | Ability to improve detection probability and classification accuracy in low signal-to-noise ratio environments. |
Soni B et al. [ ] | 2020 | Temporal correlation | LSTM-SS | Pd = 99% (−10 dB) | Innovative proposal of PAS-SS to improve detection probability by calculating PU activity statistics. |
Bkassiny M [ ] | 2022 | Raw data and cyclostationary feature | LSTM | Pd = 99.4% (20 dB) | The model is suitable for classification by modulation type and pulse shape of communication signals. |
Chen W et al. [ ] | 2022 | Covariance matrix of the signals received by the array | CM-LSTM | Pd = 100% (−10 dB) | The model performs better than other existing methods in a multi-antenna environment. |
Yu L et al. [ ] | 2018 | Data of single spectrum point with temporal correlation | Taguchi method + LSTM | CDF = 90% (RMSE = 15) | Optimizing the network by introducing Taguchi’s method to reduce time consumption and computational resources. |
Arunachalam G et al. [ ] | 2023 | Energy and correlation features | Cuttle Fish + LSTM | Pd = 90% (−20 dB) | The model achieves lower complexity and low computational overhead compared to other existing LSTM models. |
Author | Year | Input | Model | Performances | Advantage |
---|---|---|---|---|---|
Ruiyan D U et al. [ ] | 2019 | Cyclostationary features of training samples | Wavelet Transform + ANN | Pd = 90% (−15 dB) | Enhanced detection performance in low-SNR environments. |
Nasser A et al. [ ] | 2021 | ED, ACD, EVM, EVMM | HHS + ANN | Pd = 89% (−15 dB) | The model can improve the detection probability by learning the information provided by multiple detectors. |
Wang Y et al. [ ] | 2021 | Characterization between the phase-difference distributions of noise-perturbed signals and Gaussian noise | PDD, BPDD | PDD: Pd = 90% (−15 dB, Pf = 0.5, AWGN channels) BPDD: Pd = 83% (−15 dB, Pf = 0.5, AWGN) | When the carrier frequency of the sensed signal is unknown, the proposed BPDD model outperforms other existing schemes. |
Zhang X et al. [ ] | 2023 | Wideband spectrum signals | ADMM + DNN | MSE = 0.75 (−10 dB) | The model accelerates convergence at lower sampling rates and is robust at low signal-to-noise ratios. |
Zhao R et al. [ ] | 2023 | Features such as correlation, texture, shape, and power in spectrograms | CCD-GAN | Precision = 100% (Recall = 0.4, MAP = 95.04) | The model can effectively solve the domain adaptive problem and improve the migration learning to further enhance the generalization ability. |
Li X et al. [ ] | 2023 | Characteristics of data distribution in different time periods | TFF aDCNN | Recovery Probability of Support Set = 0.91 (−8 dB) | The proposed model performs well with very low SNRs and fewer sampled channels. |
Spectrum Sensing | References | Method/Model | Performance | Application Scenario |
---|---|---|---|---|
Based on conventional methods | [ , , ] | Energy detection | Performs well in high-SNR and stable noise environments. | Suitable for scenarios where the main user signal information is not known, the phase requirement is low, and the SNR is not low. |
[ , ] | Cyclostationary feature detection | Performs well in sensing modulated signals or signals with cyclostationary feature, but high algorithmic complexity leads to long sensing time and low network throughput. | Scenarios that need to distinguish between noise energy and signal energy. Knows partial information about the PU signal and can work in lower-SNR environments. | |
[ , , ] | Matched filter detection | Better performance than energy detection in ideal environments. | Knowing a priori information about the signal, higher SNR, and higher phase synchronization. | |
[ , , , , , , ] | Cooperative spectrum sensing | Eliminates noisy signals and interference from malicious users for better spectrum monitoring and sharing than single-node spectrum sensing. | The environment allows for effective linkage of sensory information from multiple nodes or devices. The environment suffers from channel multipath fading, shadowing effects, and receiver instabilities. | |
Based on deep learning | [ , , , , , , , , ] | CNN | By extracting higher-order statistical features of the signal, it is possible to achieve higher detection probabilities at low SNRs, and the model is also more robust. | Do not need a priori information about the signal and want to improve the detection probability and robustness at low SNRs. |
[ , , , ] | ResNet | Improvements on CNN models and, in general, some performance gains over CNNs. | Want to further increase the depth of the CNN, thus achieving better performance and avoiding the problem of gradient drop in DCNNs. | |
[ , , , , , ] | LSTM | Higher accuracy can be achieved at a low SNR through efficient learning of raw signal data or correlation information between signals. | The need to learn complex and noisy spectrum data for tasks that require long sequences with long-term dependencies. | |
[ , , , , ] | DQN | It allows the system to make more accurate decisions in dynamic environments and improves the robustness and accuracy of sensing models. | Looking to address distributed decision making, synchronization issues, security, and robustness in CSS, or to improve sensing accuracy in multi-user and dynamic scenarios. |
The statements, opinions and data contained in all publications are solely those of the individual author(s) and contributor(s) and not of MDPI and/or the editor(s). MDPI and/or the editor(s) disclaim responsibility for any injury to people or property resulting from any ideas, methods, instructions or products referred to in the content. |
Share and Cite
Zhang, Y.; Luo, Z. A Review of Research on Spectrum Sensing Based on Deep Learning. Electronics 2023 , 12 , 4514. https://doi.org/10.3390/electronics12214514
Zhang Y, Luo Z. A Review of Research on Spectrum Sensing Based on Deep Learning. Electronics . 2023; 12(21):4514. https://doi.org/10.3390/electronics12214514
Zhang, Yixuan, and Zhongqiang Luo. 2023. "A Review of Research on Spectrum Sensing Based on Deep Learning" Electronics 12, no. 21: 4514. https://doi.org/10.3390/electronics12214514
Article Metrics
Article access statistics, further information, mdpi initiatives, follow mdpi.
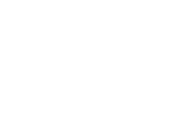
Subscribe to receive issue release notifications and newsletters from MDPI journals
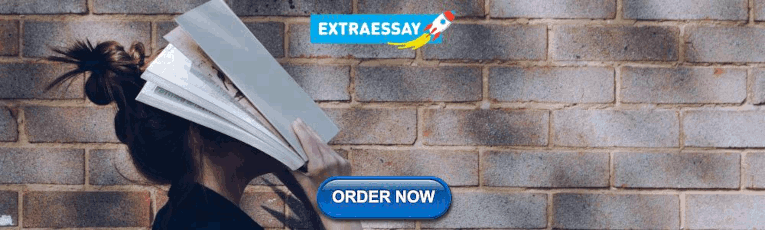
IMAGES
VIDEO
COMMENTS
Explore the latest full-text research PDFs, articles, conference papers, preprints and more on REMOTE SENSING AND GIS. Find methods information, sources, references or conduct a literature review ...
Geovisualisation is a developing field of computing science with the fundamental approach that displaying visual representations of data assists humans in generating ideas and hypotheses about the data set (e.g., [3, 4, 9]).In the applied sciences, coupling remote sensing and GIS-based mapping are helpful for data visualisation, spatial analysis, and a better understanding of the functioning ...
Remote Sensing is an international, peer-reviewed, open access journal about the science and application of remote sensing technology, and is published semimonthly online by MDPI. The Remote Sensing Society of Japan (RSSJ) and the Japan Society of Photogrammetry and Remote Sensing (JSPRS) are affiliated with Remote Sensing, and their members receive a discount on the article processing charge.
Sensing and Bio-Sensing Research is an open access journal devoted to research, design development and application of all bio-sensing and sensing technologies. The editors will accept research papers, reviews, field trials and validation studies of obvious relevance, which describe important new concepts, underpin understanding of the areas of interest or provide important insights into the ...
Advanced Photonics Research is a leading open access photonics journal publishing research across the thriving field ... Search for more papers by this author. Timothy D. Wilkinson, Timothy D. Wilkinson ... sensors based on fiber-optics have been developed rapidly because of their excellent sensing performances and capability to function in ...
Overview. Sensing and Imaging is a peer-reviewed journal focusing on theoretical and experimental advancements in sensing and imaging technologies in engineering and science.The journal has a broad and multidisciplinary scope, including all varieties of sensor technology (physical, chemical, and biological) and relevant image/video processing ...
Groundwater Potential . Remote Sensing And Gis . River Delta . Red River . Red River Delta . Thematic Layers . Delta Plain . Potential Recharge. Abstract The Red River delta plain is the second largest delta in Vietnam and is located in the North of the country with an area of 14,860 km2 and residing more than 22.5 million inhabitants.
The first three review papers from three leading Institutions of Indian Space Research Organisation (ISRO) highlight on the diversity and developments in remote sensing (1) sensor systems, (2) applications aimed at societal development and national building, and (3) data processing and analysis using soft computing techniques.Misra (2017) has reviewed the Indian remote sensing sensor system ...
Research Topics. An exciting journal in its field which focuses on physical and quantitative approaches to remote sensing of the land, oceans, biosphere, atmosphere and space at local and global levels.
The International Journal of Remote Sensing accepts original research articles and review papers. The journal operates a single-anonymized peer review policy. Authors can choose to publish gold open access in this journal. RSPSoc members can publish their work Gold Open Access for a discounted article publishing charge (APC).
In this study, the major DL concepts pertinent to remote-sensing are introduced, and more than 200 publications in this field, most of which were published during the last two years, are reviewed and analyzed. Initially, a meta-analysis was conducted to analyze the status of remote sensing DL studies in terms of the study targets, DL model (s ...
The second part of this paper focuses on the way remote sensing contributes to answer to the specific requirements of different stakeholders for several key agricultural applications. Finally, we provide a synthesis of the emerging opportunities that should strengthen the role of remote sensing in providing operational, efficient and long-term ...
Group focused on Earth Science Remote Sensing | Explore the latest full-text research PDFs, articles, conference papers, preprints and more on REMOTE SENSING. Find methods information, sources ...
Optical Sensors Section publishes original peer-reviewed papers covering all aspects of optical sensing. Both theoretical and experimental papers and comprehensive reviews are considered. This Section addresses all aspects of optical sensing, including source and detection technologies, sensing architectures, physical effects for sensing ...
Research Open Access 02 Jul 2024 Light: Science & Applications. Volume: 13, P: 149. ... Hybrid Rayleigh-Brillouin-Raman distributed sensing system: Coded pulse pairs are employed for ...
Satellite remote sensing (SRS) — which acquires information about the Earth's surface, subsurface and atmosphere remotely from sensors on board satellites (including geodetic satellites) — is ...
Abstract. Remote Sensing (RS) refers to the science of identification of earth surface features and estimation of their geo-biophysical properties using electromagnetic radiation as a medium of ...
2. Optical Fiber Sensors. An optical fiber is a cylindrical dielectric waveguide, where both the core and the cladding are composed of glass or plastic, and the surrounding coatings used to protect the optical fiber are made of acrylate or polyimide materials. Optical fibers can be multi-mode or single-mode.
Compressive Sensing (CS) is a new sensing modality, which compresses the signal being acquired at the time of sensing. Signals can have sparse or compressible representation either in original domain or in some transform domain. Relying on the sparsity of the signals, CS allows us to sample the signal at a rate much below the Nyquist sampling rate. Also, the varied reconstruction algorithms of ...
A Feature Paper should be a substantial original Article that involves several techniques or approaches, provides an outlook for future research directions and describes possible research applications. Feature papers are submitted upon individual invitation or recommendation by the scientific editors and must receive positive feedback from the ...
Journal information. Electronic ISSN. 0974-3006. Print ISSN. 0255-660X. Co-Publisher information. Co-publication with the Indian Society of Remote Sensing. Visit Co-Publisher Site: Indian Society of Remote Sensing. Abstracted and indexed in.
information, data something like temperature, pressure, photograph, etc. Remote sensing is the process of acquiring information, detecting, analyzing, monitoring the physical. characteristics of ...
The research objective of this paper is to summarize the algorithm of cognitive radio spectrum-sensing technology combined with deep learning technology. To review the advantages of deep-learning-based spectrum-sensing algorithms, this paper first introduces the traditional spectrum-sensing methods. It summarizes and compares the advantages and ...