IEEE Account
- Change Username/Password
- Update Address
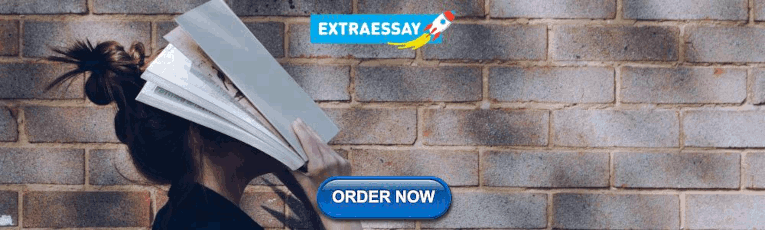
Purchase Details
- Payment Options
- Order History
- View Purchased Documents
Profile Information
- Communications Preferences
- Profession and Education
- Technical Interests
- US & Canada: +1 800 678 4333
- Worldwide: +1 732 981 0060
- Contact & Support
- About IEEE Xplore
- Accessibility
- Terms of Use
- Nondiscrimination Policy
- Privacy & Opting Out of Cookies
A not-for-profit organization, IEEE is the world's largest technical professional organization dedicated to advancing technology for the benefit of humanity. © Copyright 2024 IEEE - All rights reserved. Use of this web site signifies your agreement to the terms and conditions.
Data Science
Featured article, related topics, top conferences on data science, top videos on data science.
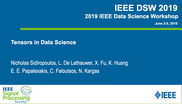
Xplore Articles related to Data Science
Periodicals related to data science, e-books related to data science, courses related to data science, top organizations on data science, most published xplore authors for data science.
Effect of Data Characteristics Inconsistency on Medium and Long-Term Runoff Forecasting by Machine Learning
Share this page:
In the application of medium and long-term runoff forecasting, machine learning has some problems, such as high learning cost, limited computing cost, and difficulty in satisfying statistical data assumptions in some regions, leading to difficulty in popularization in the hydrology industry. In the case of a few data, it is one of the ways to solve the problem to analyze the data characteristics consistency. This paper analyzes the statistical hypothesis of machine learning and runoff data characteristics such as periodicity and mutation. Aiming at the effect of data characteristics inconsistency on three representative machine learning models (multiple linear regression, random forest, back propagation neural network), a simple correction/improvement method suitable for engineering was proposed. The model results were verified in the Danjiangkou area, China. The results show that the errors of the three models have the same distribution as the periodic characteristics of the runoff periods, and the correction/improvement based on periodicity and mutation characteristics can improve the forecasting accuracy of the three models. The back propagation neural network model is most sensitive to the data characteristics consistency.
View this article on IEEE Xplore
Efficiency Optimization Design That Considers Control of Interior Permanent Magnet Synchronous Motors Based on Machine Learning for Automotive Application
Interior permanent magnet synchronous motors have become widely used as traction motors in environmentally friendly vehicles. Interior permanent magnet synchronous motors have a high degree of design freedom and time-consuming finite element analysis is required for their characteristics analysis, which results in a long design period. Here, we propose a method for fast efficiency maximization design that uses a machine-learning-based surrogate model. The surrogate model predicts motor parameters and iron loss with the same accuracy as that of finite element analysis but in a much shorter time. Furthermore, using the current and speed conditions in addition to geometry information as input to the surrogate model enables design optimization that considers motor control. The proposed method completed multi-objective multi-constraint optimization for multi-dimensional geometric parameters, which is prohibitively time-consuming using finite element analysis, in a few hours. The proposed shapes reduced losses under a vehicle test cycle compared with the initial shape. The proposed method was applied to motors with three rotor topologies to verify its generality.
Published in the IEEE Vehicular Technology Society Section
An Intelligent IoT Sensing System for Rail Vehicle Running States Based on TinyML
Real-time identification of the running state is one of the key technologies for a smart rail vehicle. However, it is a challenge to accurately real-time sense the complex running states of the rail vehicle on an Internet-of-Things (IoT) edge device. Traditional systems usually upload a large amount of real-time data from the vehicle to the cloud for identification, which is laborious and inefficient. In this paper, an intelligent identification method for rail vehicle running state is proposed based on Tiny Machine Learning (TinyML) technology, and an IoT system is developed with small size and low energy consumption. The system uses a Micro-Electro-Mechanical System (MEMS) sensor to collect acceleration data for machine learning training. A neural network model for recognizing the running state of rail vehicles is built and trained by defining a machine learning running state classification model. The trained recognition model is deployed to the IoT edge device at the vehicle side, and an offset time window method is utilized for real-time state sensing. In addition, the sensing results are uploaded to the IoT server for visualization. The experiments on the subway vehicle showed that the system could identify six complex running states in real-time with over 99% accuracy using only one IoT microcontroller. The model with three axes converges faster than the model with one. The model recognition accuracy remained above 98% and 95%, under different installation positions on the rail vehicle and the zero-drift phenomenon of the MEMS acceleration sensor, respectively. The presented method and system can also be extended to edge-aware applications of equipment such as automobiles and ships.
Code Generation Using Machine Learning: A Systematic Review
Recently, machine learning (ML) methods have been used to create powerful language models for a broad range of natural language processing tasks. An important subset of this field is that of generating code of programming languages for automatic software development. This review provides a broad and detailed overview of studies for code generation using ML. We selected 37 publications indexed in arXiv and IEEE Xplore databases that train ML models on programming language data to generate code. The three paradigms of code generation we identified in these studies are description-to-code, code-to-description, and code-to-code. The most popular applications that work in these paradigms were found to be code generation from natural language descriptions, documentation generation, and automatic program repair, respectively. The most frequently used ML models in these studies include recurrent neural networks, transformers, and convolutional neural networks. Other neural network architectures, as well as non-neural techniques, were also observed. In this review, we have summarized the applications, models, datasets, results, limitations, and future work of 37 publications. Additionally, we include discussions on topics general to the literature reviewed. This includes comparing different model types, comparing tokenizers, the volume and quality of data used, and methods for evaluating synthesized code. Furthermore, we provide three suggestions for future work for code generation using ML.
Combining Citation Network Information and Text Similarity for Research Article Recommender Systems
Researchers often need to gather a comprehensive set of papers relevant to a focused topic, but this is often difficult and time-consuming using existing search methods. For example, keyword searching suffers from difficulties with synonyms and multiple meanings. While some automated research-paper recommender systems exist, these typically depend on either a researcher’s entire library or just a single paper, resulting in either a quite broad or a quite narrow search. With these issues in mind, we built a new research-paper recommender system that utilizes both citation information and textual similarity of abstracts to provide a highly focused set of relevant results. The input to this system is a set of one or more related papers, and our system searches for papers that are closely related to the entire set. This framework helps researchers gather a set of papers that are closely related to a particular topic of interest, and allows control over which cross-section of the literature is located. We show the effectiveness of this recommender system by using it to recreate the references of review papers. We also show its utility as a general similarity metric between scientific articles by performing unsupervised clustering on sets of scientific articles. We release an implementation, ExCiteSearch (bitbucket.org/mmmontemore/excitesearch), to allow researchers to apply this framework to locate relevant scientific articles.
Novel Multi Center and Threshold Ternary Pattern Based Method for Disease Detection Method Using Voice
Smart health is one of the most popular and important components of smart cities. It is a relatively new context-aware healthcare paradigm influenced by several fields of expertise, such as medical informatics, communications and electronics, bioengineering, ethics, to name a few. Smart health is used to improve healthcare by providing many services such as patient monitoring, early diagnosis of disease and so on. The artificial neural network (ANN), support vector machine (SVM) and deep learning models, especially the convolutional neural network (CNN), are the most commonly used machine learning approaches where they proved to be performance in most cases. Voice disorders are rapidly spreading especially with the development of medical diagnostic systems, although they are often underestimated. Smart health systems can be an easy and fast support to voice pathology detection. The identification of an algorithm that discriminates between pathological and healthy voices with more accuracy is needed to obtain a smart and precise mobile health system. The main contribution of this paper consists of proposing a multiclass-pathologic voice classification using a novel multileveled textural feature extraction with iterative feature selector. Our approach is a simple and efficient voice-based algorithm in which a multi-center and multi threshold based ternary pattern is used (MCMTTP). A more compact multileveled features are then obtained by sample-based discretization techniques and Neighborhood Component Analysis (NCA) is applied to select features iteratively. These features are finally integrated with MCMTTP to achieve an accurate voice-based features detection. Experimental results of six classifiers with three diagnostic diseases (frontal resection, cordectomy and spastic dysphonia) show that the fused features are more suitable for describing voice-based disease detection.
*Published in the IEEE Electronics Packaging Society Section within IEEE Access .
Machine Learning Empowered Spectrum Sharing in Intelligent Unmanned Swarm Communication Systems: Challenges, Requirements and Solutions
The unmanned swarm system (USS) has been seen as a promising technology, and will play an extremely important role in both the military and civilian fields such as military strikes, disaster relief and transportation business. As the “nerve center” of USS, the unmanned swarm communication system (USCS) provides the necessary information transmission medium so as to ensure the system stability and mission implementation. However, challenges caused by multiple tasks, distributed collaboration, high dynamics, ultra-dense and jamming threat make it hard for USCS to manage limited spectrum resources. To tackle with such problems, the machine learning (ML) empowered intelligent spectrum management technique is introduced in this paper. First, based on the challenges of the spectrum resource management in USCS, the requirement of spectrum sharing is analyzed from the perspective of spectrum collaboration and spectrum confrontation. We found that suitable multi-agent collaborative decision making is promising to realize effective spectrum sharing in both two perspectives. Therefore, a multi-agent learning framework is proposed which contains mobile-computing-assisted and distributed structures. Based on the framework, we provide case studies. Finally, future research directions are discussed.
Harnessing Artificial Intelligence Capabilities to Improve Cybersecurity
Cybersecurity is a fast-evolving discipline that is always in the news over the last decade, as the number of threats rises and cybercriminals constantly endeavor to stay a step ahead of law enforcement. Over the years, although the original motives for carrying out cyberattacks largely remain unchanged, cybercriminals have become increasingly sophisticated with their techniques. Traditional cybersecurity solutions are becoming inadequate at detecting and mitigating emerging cyberattacks. Advances in cryptographic and Artificial Intelligence (AI) techniques (in particular, machine learning and deep learning) show promise in enabling cybersecurity experts to counter the ever-evolving threat posed by adversaries. Here, we explore AI’s potential in improving cybersecurity solutions, by identifying both its strengths and weaknesses. We also discuss future research opportunities associated with the development of AI techniques in the cybersecurity field across a range of application domains.
A Study on the Elimination of Thermal Reflections
Recently, thermal cameras have been used in various surveillance and monitoring systems. In particular, in camera-based surveillance systems, algorithms are being developed for detecting and recognizing objects from images acquired in dark environments. However, it is difficult to detect and recognize an object due to the thermal reflections generated in the image obtained from a thermal camera. For example, thermal reflection often occurs on a structure or the floor near an object, similar to shadows or mirror reflections. In this case, the object and the areas of thermal reflection overlap or are connected to each other and are difficult to separate. Thermal reflection also occurs on nearby walls, which can be detected as artifacts when an object is not associated with this phenomenon. In addition, the size and pixel value of the thermal reflection area vary greatly depending on the material of the area and the environmental temperature. In this case, the patterns and pixel values of the thermal reflection and the object are similar to each other and difficult to differentiate. These problems reduce the accuracy of object detection and recognition methods. In addition, no studies have been conducted on the elimination of thermal reflection of objects under different environmental conditions. Therefore, to address these challenges, we propose a method of detecting reflections in thermal images based on deep learning and their elimination via post-processing. Experiments using a self-collected database (Dongguk thermal image database (DTh-DB), Dongguk items and vehicles database (DI&V-DB)) and an open database showed that the performance of the proposed method is superior compared to that of other state-of-the-art approaches.
Machine Learning Designs, Implementations and Techniques
Submission Deadline: 15 February 2020
IEEE Access invites manuscript submissions in the area of Machine Learning Designs, Implementations and Techniques.
Most modern machine learning research is devoted to improving the accuracy of prediction. However, less attention is paid to deployment of machine and deep learning systems, supervised /unsupervised techniques for mining healthcare data, and time series similarity and irregular temporal data analysis. Most deployments are in the cloud, with abundant and scalable resources, and a free choice of computation platform. However, with the advent of intelligent physical devices—such as intelligent robots or self-driven cars—the resources are more limited, and the latency may be strictly bounded.
To address these questions, the focus of this Special Section in IEEE Access is on machine and deep learning designs, implementations and techniques, including both system level topics and other research questions related to the general use and framework of machine learning algorithms.
The topics of interest include, but are not limited to:
- Real time implementation of machine and deep learning,
- System level implementation, considering full pipeline from raw data until the decision layer
- Novel and innovative applications with strong emphasis on design and implementation
- Novel approaches for Temporal / Spatial/Spatio-Temporal Association analysis
- Pattern discovery from Time stamped Temporal and Interval databases
- High performance data mining in cloud
- Novel approaches for handling Uncertain and Imbalanced data
- Supervised/Unsupervised techniques for mining healthcare data
- Deep learning for translational bio-informatics
- Periodic/Sequential pattern mining
- Evolutionary algorithms
- Privacy-Preserving Data mining
- Time series similarity and Irregular temporal data analysis
- Mining Text Web and Social network data
- Imputation techniques for Temporal data
- Causality and Event Processing
- Applications of Data Mining in Anomaly and Intrusion detection
- Applications to medical informatics
We also highly recommend the submission of multimedia with each article as it significantly increases the visibility, downloads, and citations of articles.
Associate Editor: Shadi A. Aljawarneh, Jordan University of Science and Technology, Jordan
Guest Editors:
- Oguz Bayat, Altinbas University, Turkey
- Juan A. Lara, Madrid Open University, Udima, Spain
- Robert P. Schumaker, University of Texas at Tyler, USA
Relevant IEEE Access Special Sections:
- Visual Analysis for CPS Data
- Emerging Approaches to Cyber Security
- Data-Enabled Intelligence for Digital Health
IEEE Access Editor-in-Chief: Prof. Derek Abbott, University of Adelaide
Article submission: Contact Associate Editor and submit manuscript to: http://ieee.atyponrex.com/journal/ieee-access
For inquiries regarding this Special Section, please contact: [email protected] , [email protected] .
At a Glance
- Journal: IEEE Access
- Format: Open Access
- Frequency: Continuous
- Submission to Publication: 4-6 weeks (typical)
- Topics: All topics in IEEE
- Average Acceptance Rate: 27%
- Impact Factor: 3.4
- Model: Binary Peer Review
- Article Processing Charge: US $1,995
Featured Articles
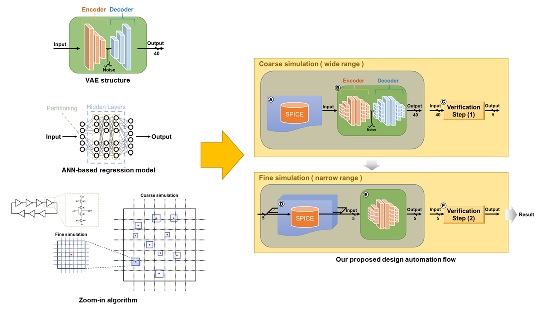
AMS Circuit Design Optimization Technique Based on ANN Regression Model With VAE Structure
View in IEEE Xplore
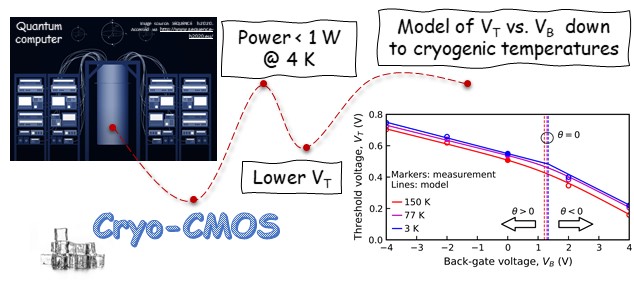
Novel Approach to FDSOI Threshold Voltage Model Validated at Cryogenic Temperatures
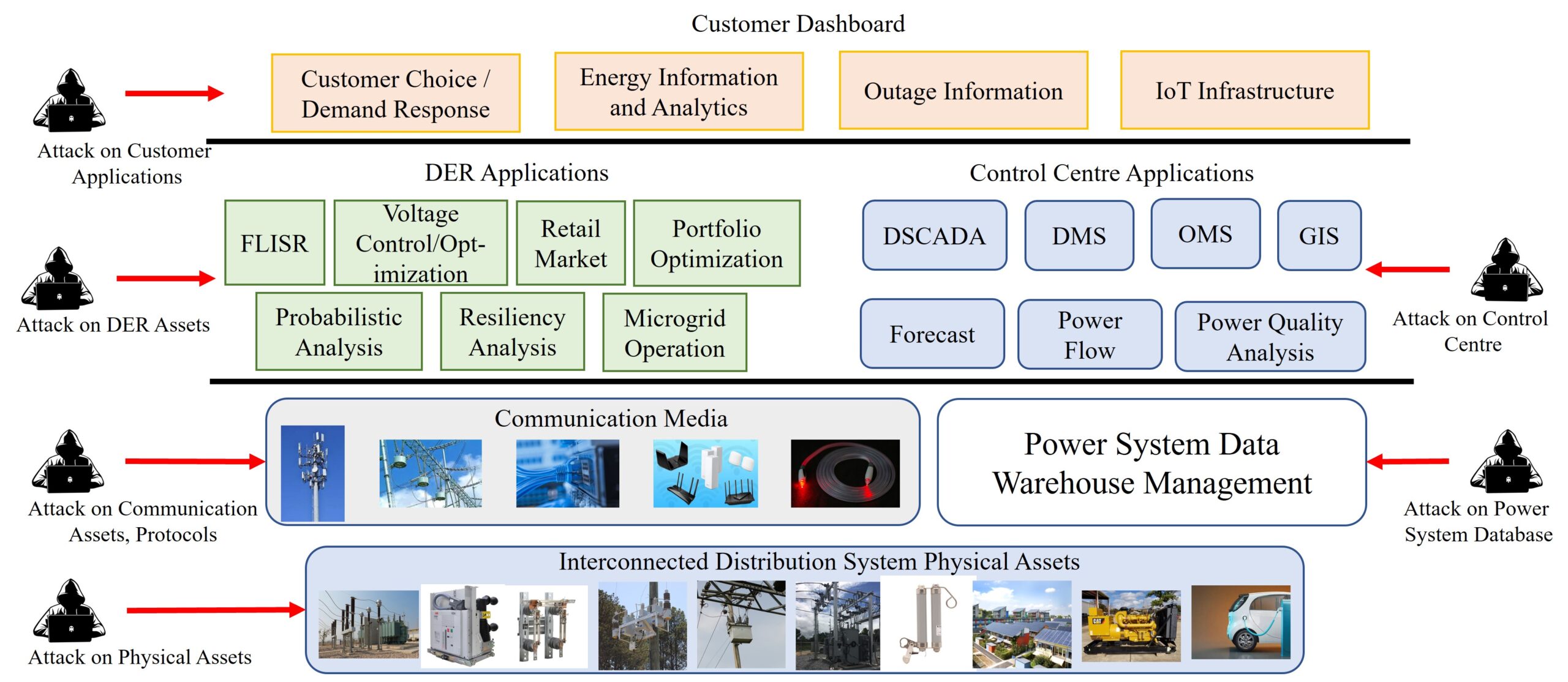
On the Cyber-Physical Needs of DER-Based Voltage Control/Optimization Algorithms in Active Distribution Network
Submission guidelines.
© 2024 IEEE - All rights reserved. Use of this website signifies your agreement to the IEEE TERMS AND CONDITIONS.
A not-for-profit organization, IEEE is the world’s largest technical professional organization dedicated to advancing technology for the benefit of humanity.
AWARD RULES:
NO PURCHASE NECESSARY TO ENTER OR WIN. A PURCHASE WILL NOT INCREASE YOUR CHANCES OF WINNING.
These rules apply to the “2024 IEEE Access Best Video Award Part 1″ (the “Award”).
- Sponsor: The Sponsor of the Award is The Institute of Electrical and Electronics Engineers, Incorporated (“IEEE”) on behalf of IEEE Access , 445 Hoes Lane, Piscataway, NJ 08854-4141 USA (“Sponsor”).
- Eligibility: Award is open to residents of the United States of America and other countries, where permitted by local law, who are the age of eighteen (18) and older. Employees of Sponsor, its agents, affiliates and their immediate families are not eligible to enter Award. The Award is subject to all applicable state, local, federal and national laws and regulations. Entrants may be subject to rules imposed by their institution or employer relative to their participation in Awards and should check with their institution or employer for any relevant policies. Void in locations and countries where prohibited by law.
- Agreement to Official Rules : By participating in this Award, entrants agree to abide by the terms and conditions thereof as established by Sponsor. Sponsor reserves the right to alter any of these Official Rules at any time and for any reason. All decisions made by Sponsor concerning the Award including, but not limited to the cancellation of the Award, shall be final and at its sole discretion.
- How to Enter: This Award opens on January 1, 2024 at 12:00 AM ET and all entries must be received by 11:59 PM ET on June 30, 2024 (“Promotional Period”).
Entrant must submit a video with an article submission to IEEE Access . The video submission must clearly be relevant to the submitted manuscript. Only videos that accompany an article that is accepted for publication in IEEE Access will qualify. The video may be simulations, demonstrations, or interviews with other experts, for example. Your video file should not exceed 100 MB.
Entrants can enter the Award during Promotional Period through the following method:
- The IEEE Author Portal : Entrants can upload their video entries while submitting their article through the IEEE Author Portal submission site .
- Review and Complete the Terms and Conditions: After submitting your manuscript and video through the IEEE Author Portal, entrants should then review and sign the Terms and Conditions .
Entrants who have already submitted a manuscript to IEEE Access without a video can still submit a video for inclusion in this Award so long as the video is submitted within 7 days of the article submission date. The video can be submitted via email to the article administrator. All videos must undergo peer review and be accepted along with the article submission. Videos may not be submitted after an article has already been accepted for publication.
The criteria for an article to be accepted for publication in IEEE Access are:
- The article must be original writing that enhances the existing body of knowledge in the given subject area. Original review articles and surveys are acceptable even if new data/concepts are not presented.
- Results reported must not have been submitted or published elsewhere (although expanded versions of conference publications are eligible for submission).
- Experiments, statistics, and other analyses must be performed to a high technical standard and are described in sufficient detail.
- Conclusions must be presented in an appropriate fashion and are supported by the data.
- The article must be written in standard English with correct grammar.
- Appropriate references to related prior published works must be included.
- The article must fall within the scope of IEEE Access
- Must be in compliance with the IEEE PSPB Operations Manual.
- Completion of the required IEEE intellectual property documents for publication.
- At the discretion of the IEEE Access Editor-in-Chief.
- Disqualification: The following items will disqualify a video from being considered a valid submission:
- The video is not original work.
- A video that is not accompanied with an article submission.
- The article and/or video is rejected during the peer review process.
- The article and/or video topic does not fit into the scope of IEEE Access .
- The article and/or do not follow the criteria for publication in IEEE Access .
- Videos posted in a comment on IEEE Xplore .
- Content is off-topic, offensive, obscene, indecent, abusive or threatening to others.
- Infringes the copyright, trademark or other right of any third party.
- Uploads viruses or other contaminating or destructive features.
- Is in violation of any applicable laws or regulations.
- Is not in English.
- Is not provided within the designated submission time.
- Entrant does not agree and sign the Terms and Conditions document.
Entries must be original. Entries that copy other entries, or the intellectual property of anyone other than the Entrant, may be removed by Sponsor and the Entrant may be disqualified. Sponsor reserves the right to remove any entry and disqualify any Entrant if the entry is deemed, in Sponsor’s sole discretion, to be inappropriate.
- Entrant’s Warranty and Authorization to Sponsor: By entering the Award, entrants warrant and represent that the Award Entry has been created and submitted by the Entrant. Entrant certifies that they have the ability to use any image, text, video, or other intellectual property they may upload and that Entrant has obtained all necessary permissions. IEEE shall not indemnify Entrant for any infringement, violation of publicity rights, or other civil or criminal violations. Entrant agrees to hold IEEE harmless for all actions related to the submission of an Entry. Entrants further represent and warrant, if they reside outside of the United States of America, that their participation in this Award and acceptance of a prize will not violate their local laws.
- Intellectual Property Rights: Entrant grants Sponsor an irrevocable, worldwide, royalty free license to use, reproduce, distribute, and display the Entry for any lawful purpose in all media whether now known or hereinafter created. This may include, but is not limited to, the IEEE A ccess website, the IEEE Access YouTube channel, the IEEE Access IEEE TV channel, IEEE Access social media sites (LinkedIn, Facebook, Twitter, IEEE Access Collabratec Community), and the IEEE Access Xplore page. Facebook/Twitter/Microsite usernames will not be used in any promotional and advertising materials without the Entrants’ expressed approval.
- Number of Prizes Available, Prizes, Approximate Retail Value and Odds of winning Prizes: Two (2) promotional prizes of $350 USD Amazon gift cards. One (1) grand prize of a $500 USD Amazon gift card. Prizes will be distributed to the winners after the selection of winners is announced. Odds of winning a prize depend on the number of eligible entries received during the Promotional Period. Only the corresponding author of the submitted manuscript will receive the prize.
The grand prize winner may, at Sponsor’ discretion, have his/her article and video highlighted in media such as the IEEE Access Xplore page and the IEEE Access social media sites.
The prize(s) for the Award are being sponsored by IEEE. No cash in lieu of prize or substitution of prize permitted, except that Sponsor reserves the right to substitute a prize or prize component of equal or greater value in its sole discretion for any reason at time of award. Sponsor shall not be responsible for service obligations or warranty (if any) in relation to the prize(s). Prize may not be transferred prior to award. All other expenses associated with use of the prize, including, but not limited to local, state, or federal taxes on the Prize, are the sole responsibility of the winner. Winner(s) understand that delivery of a prize may be void where prohibited by law and agrees that Sponsor shall have no obligation to substitute an alternate prize when so prohibited. Amazon is not a sponsor or affiliated with this Award.
- Selection of Winners: Promotional prize winners will be selected based on entries received during the Promotional Period. The sponsor will utilize an Editorial Panel to vote on the best video submissions. Editorial Panel members are not eligible to participate in the Award. Entries will be ranked based on three (3) criteria:
- Presentation of Technical Content
- Quality of Video
Upon selecting a winner, the Sponsor will notify the winner via email. All potential winners will be notified via their email provided to the sponsor. Potential winners will have five (5) business days to respond after receiving initial prize notification or the prize may be forfeited and awarded to an alternate winner. Potential winners may be required to sign an affidavit of eligibility, a liability release, and a publicity release. If requested, these documents must be completed, signed, and returned within ten (10) business days from the date of issuance or the prize will be forfeited and may be awarded to an alternate winner. If prize or prize notification is returned as undeliverable or in the event of noncompliance with these Official Rules, prize will be forfeited and may be awarded to an alternate winner.
- General Prize Restrictions: No prize substitutions or transfer of prize permitted, except by the Sponsor. Import/Export taxes, VAT and country taxes on prizes are the sole responsibility of winners. Acceptance of a prize constitutes permission for the Sponsor and its designees to use winner’s name and likeness for advertising, promotional and other purposes in any and all media now and hereafter known without additional compensation unless prohibited by law. Winner acknowledges that neither Sponsor, Award Entities nor their directors, employees, or agents, have made nor are in any manner responsible or liable for any warranty, representation, or guarantee, express or implied, in fact or in law, relative to any prize, including but not limited to its quality, mechanical condition or fitness for a particular purpose. Any and all warranties and/or guarantees on a prize (if any) are subject to the respective manufacturers’ terms therefor, and winners agree to look solely to such manufacturers for any such warranty and/or guarantee.
11.Release, Publicity, and Privacy : By receipt of the Prize and/or, if requested, by signing an affidavit of eligibility and liability/publicity release, the Prize Winner consents to the use of his or her name, likeness, business name and address by Sponsor for advertising and promotional purposes, including but not limited to on Sponsor’s social media pages, without any additional compensation, except where prohibited. No entries will be returned. All entries become the property of Sponsor. The Prize Winner agrees to release and hold harmless Sponsor and its officers, directors, employees, affiliated companies, agents, successors and assigns from and against any claim or cause of action arising out of participation in the Award.
Sponsor assumes no responsibility for computer system, hardware, software or program malfunctions or other errors, failures, delayed computer transactions or network connections that are human or technical in nature, or for damaged, lost, late, illegible or misdirected entries; technical, hardware, software, electronic or telephone failures of any kind; lost or unavailable network connections; fraudulent, incomplete, garbled or delayed computer transmissions whether caused by Sponsor, the users, or by any of the equipment or programming associated with or utilized in this Award; or by any technical or human error that may occur in the processing of submissions or downloading, that may limit, delay or prevent an entrant’s ability to participate in the Award.
Sponsor reserves the right, in its sole discretion, to cancel or suspend this Award and award a prize from entries received up to the time of termination or suspension should virus, bugs or other causes beyond Sponsor’s control, unauthorized human intervention, malfunction, computer problems, phone line or network hardware or software malfunction, which, in the sole opinion of Sponsor, corrupt, compromise or materially affect the administration, fairness, security or proper play of the Award or proper submission of entries. Sponsor is not liable for any loss, injury or damage caused, whether directly or indirectly, in whole or in part, from downloading data or otherwise participating in this Award.
Representations and Warranties Regarding Entries: By submitting an Entry, you represent and warrant that your Entry does not and shall not comprise, contain, or describe, as determined in Sponsor’s sole discretion: (A) false statements or any misrepresentations of your affiliation with a person or entity; (B) personally identifying information about you or any other person; (C) statements or other content that is false, deceptive, misleading, scandalous, indecent, obscene, unlawful, defamatory, libelous, fraudulent, tortious, threatening, harassing, hateful, degrading, intimidating, or racially or ethnically offensive; (D) conduct that could be considered a criminal offense, could give rise to criminal or civil liability, or could violate any law; (E) any advertising, promotion or other solicitation, or any third party brand name or trademark; or (F) any virus, worm, Trojan horse, or other harmful code or component. By submitting an Entry, you represent and warrant that you own the full rights to the Entry and have obtained any and all necessary consents, permissions, approvals and licenses to submit the Entry and comply with all of these Official Rules, and that the submitted Entry is your sole original work, has not been previously published, released or distributed, and does not infringe any third-party rights or violate any laws or regulations.
12.Disputes: EACH ENTRANT AGREES THAT: (1) ANY AND ALL DISPUTES, CLAIMS, AND CAUSES OF ACTION ARISING OUT OF OR IN CONNECTION WITH THIS AWARD, OR ANY PRIZES AWARDED, SHALL BE RESOLVED INDIVIDUALLY, WITHOUT RESORTING TO ANY FORM OF CLASS ACTION, PURSUANT TO ARBITRATION CONDUCTED UNDER THE COMMERCIAL ARBITRATION RULES OF THE AMERICAN ARBITRATION ASSOCIATION THEN IN EFFECT, (2) ANY AND ALL CLAIMS, JUDGMENTS AND AWARDS SHALL BE LIMITED TO ACTUAL OUT-OF-POCKET COSTS INCURRED, INCLUDING COSTS ASSOCIATED WITH ENTERING THIS AWARD, BUT IN NO EVENT ATTORNEYS’ FEES; AND (3) UNDER NO CIRCUMSTANCES WILL ANY ENTRANT BE PERMITTED TO OBTAIN AWARDS FOR, AND ENTRANT HEREBY WAIVES ALL RIGHTS TO CLAIM, PUNITIVE, INCIDENTAL, AND CONSEQUENTIAL DAMAGES, AND ANY OTHER DAMAGES, OTHER THAN FOR ACTUAL OUT-OF-POCKET EXPENSES, AND ANY AND ALL RIGHTS TO HAVE DAMAGES MULTIPLIED OR OTHERWISE INCREASED. ALL ISSUES AND QUESTIONS CONCERNING THE CONSTRUCTION, VALIDITY, INTERPRETATION AND ENFORCEABILITY OF THESE OFFICIAL RULES, OR THE RIGHTS AND OBLIGATIONS OF ENTRANT AND SPONSOR IN CONNECTION WITH THE AWARD, SHALL BE GOVERNED BY, AND CONSTRUED IN ACCORDANCE WITH, THE LAWS OF THE STATE OF NEW JERSEY, WITHOUT GIVING EFFECT TO ANY CHOICE OF LAW OR CONFLICT OF LAW, RULES OR PROVISIONS (WHETHER OF THE STATE OF NEW JERSEY OR ANY OTHER JURISDICTION) THAT WOULD CAUSE THE APPLICATION OF THE LAWS OF ANY JURISDICTION OTHER THAN THE STATE OF NEW JERSEY. SPONSOR IS NOT RESPONSIBLE FOR ANY TYPOGRAPHICAL OR OTHER ERROR IN THE PRINTING OF THE OFFER OR ADMINISTRATION OF THE AWARD OR IN THE ANNOUNCEMENT OF THE PRIZES.
- Limitation of Liability: The Sponsor, Award Entities and their respective parents, affiliates, divisions, licensees, subsidiaries, and advertising and promotion agencies, and each of the foregoing entities’ respective employees, officers, directors, shareholders and agents (the “Released Parties”) are not responsible for incorrect or inaccurate transfer of entry information, human error, technical malfunction, lost/delayed data transmissions, omission, interruption, deletion, defect, line failures of any telephone network, computer equipment, software or any combination thereof, inability to access web sites, damage to a user’s computer system (hardware and/or software) due to participation in this Award or any other problem or error that may occur. By entering, participants agree to release and hold harmless the Released Parties from and against any and all claims, actions and/or liability for injuries, loss or damage of any kind arising from or in connection with participation in and/or liability for injuries, loss or damage of any kind, to person or property, arising from or in connection with participation in and/or entry into this Award, participation is any Award-related activity or use of any prize won. Entry materials that have been tampered with or altered are void. If for any reason this Award is not capable of running as planned, or if this Award or any website associated therewith (or any portion thereof) becomes corrupted or does not allow the proper playing of this Award and processing of entries per these rules, or if infection by computer virus, bugs, tampering, unauthorized intervention, affect the administration, security, fairness, integrity, or proper conduct of this Award, Sponsor reserves the right, at its sole discretion, to disqualify any individual implicated in such action, and/or to cancel, terminate, modify or suspend this Award or any portion thereof, or to amend these rules without notice. In the event of a dispute as to who submitted an online entry, the entry will be deemed submitted by the authorized account holder the email address submitted at the time of entry. “Authorized Account Holder” is defined as the person assigned to an email address by an Internet access provider, online service provider or other organization responsible for assigning email addresses for the domain associated with the email address in question. Any attempt by an entrant or any other individual to deliberately damage any web site or undermine the legitimate operation of the Award is a violation of criminal and civil laws and should such an attempt be made, the Sponsor reserves the right to seek damages and other remedies from any such person to the fullest extent permitted by law. This Award is governed by the laws of the State of New Jersey and all entrants hereby submit to the exclusive jurisdiction of federal or state courts located in the State of New Jersey for the resolution of all claims and disputes. Facebook, LinkedIn, Twitter, G+, YouTube, IEEE Xplore , and IEEE TV are not sponsors nor affiliated with this Award.
- Award Results and Official Rules: To obtain the identity of the prize winner and/or a copy of these Official Rules, send a self-addressed stamped envelope to Kimberly Rybczynski, IEEE, 445 Hoes Lane, Piscataway, NJ 08854-4141 USA.
- IEEE Xplore Digital Library
- IEEE Standards
- IEEE Spectrum

Publications
IEEE Talks Big Data - Check out our new Q&A article series with big Data experts!
Call for Papers - Check out the many opportunities to submit your own paper. This is a great way to get published, and to share your research in a leading IEEE magazine!
Publications - See the list of various IEEE publications related to big data and analytics here.
Call for Blog Writers!
IEEE Cloud Computing Community is a key platform for researchers, academicians and industry practitioners to share and exchange ideas regarding cloud computing technologies and services, as well as identify the emerging trends and research topics that are defining the future direction of cloud computing. Come be part of this revolution as we invite blog posts in this regard and not limited to the list provided below:
- Cloud Deployment Frameworks
- Cloud Architecture
- Cloud Native Design Patterns
- Testing Services and Frameworks
- Storage Architectures
- Big Data and Analytics
- Internet of Things
- Virtualization techniques
- Legacy Modernization
- Security and Compliance
- Pricing Methodologies
- Service Oriented Architecture
- Microservices
- Container Technology
- Cloud Computing Impact and Trends shaping today’s business
- High availability and reliability
Call for Papers
No call for papers at this time.
IEEE Publications on Big Data

Read more at IEEE Computer Society.
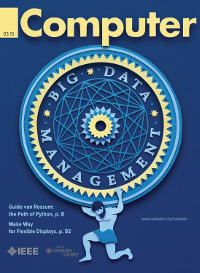
IEEE Computer Magazine Special Issue on Big Data Management
- Big Data: Promises and Problems
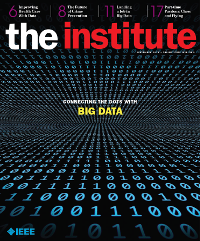
Connecting the Dots With Big Data
- Better Health Care Through Data
- The Future of Crime Prevention
- Census and Sensibility
- Landing a Job in Big Data
Read more at The Institute.
Download full issue. (PDF, 5 MB)
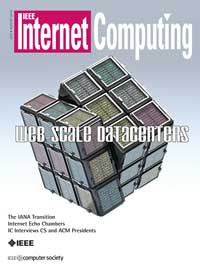
IEEE Internet Computing July/August 2014
Web-Scale Datacenters
This issue of Internet Computing surveys issues surrounding Web-scale datacenters, particularly in the areas of cloud provisioning as well as networking optimization and configuration. They include workload isolation, recovery from transient server availability, network configuration, virtual networking, and content distribution.
Read more at IEEE Computer Society .
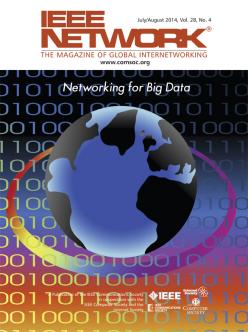
Networking for Big Data
The most current information for communications professionals involved with the interconnection of computing systems, this bimonthly magazine covers all aspects of data and computer communications.
Read more at IEEE Communications Society .
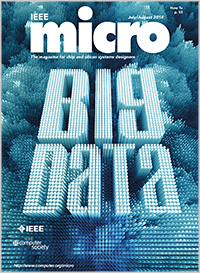
Special Issue on Big Data
Big data is transforming our lives, but it is also placing an unprecedented burden on our compute infrastructure. As data expansion rates outpace Moore's law and supply voltage scaling grinds to a halt, the IT industry is being challenged in its ability to effectively store, process, and serve the growing volumes of data. Delivering on the premise of big data in the postDennard era calls for specialization and tight integration across the system stack, with the aim of maximizing energy efficiency, performance scalability, resilience, and security.
data science Recently Published Documents
Total documents.
- Latest Documents
- Most Cited Documents
- Contributed Authors
- Related Sources
- Related Keywords
Assessing the effects of fuel energy consumption, foreign direct investment and GDP on CO2 emission: New data science evidence from Europe & Central Asia
Documentation matters: human-centered ai system to assist data science code documentation in computational notebooks.
Computational notebooks allow data scientists to express their ideas through a combination of code and documentation. However, data scientists often pay attention only to the code, and neglect creating or updating their documentation during quick iterations. Inspired by human documentation practices learned from 80 highly-voted Kaggle notebooks, we design and implement Themisto, an automated documentation generation system to explore how human-centered AI systems can support human data scientists in the machine learning code documentation scenario. Themisto facilitates the creation of documentation via three approaches: a deep-learning-based approach to generate documentation for source code, a query-based approach to retrieve online API documentation for source code, and a user prompt approach to nudge users to write documentation. We evaluated Themisto in a within-subjects experiment with 24 data science practitioners, and found that automated documentation generation techniques reduced the time for writing documentation, reminded participants to document code they would have ignored, and improved participants’ satisfaction with their computational notebook.
Data science in the business environment: Insight management for an Executive MBA
Adventures in financial data science, gecoagent: a conversational agent for empowering genomic data extraction and analysis.
With the availability of reliable and low-cost DNA sequencing, human genomics is relevant to a growing number of end-users, including biologists and clinicians. Typical interactions require applying comparative data analysis to huge repositories of genomic information for building new knowledge, taking advantage of the latest findings in applied genomics for healthcare. Powerful technology for data extraction and analysis is available, but broad use of the technology is hampered by the complexity of accessing such methods and tools. This work presents GeCoAgent, a big-data service for clinicians and biologists. GeCoAgent uses a dialogic interface, animated by a chatbot, for supporting the end-users’ interaction with computational tools accompanied by multi-modal support. While the dialogue progresses, the user is accompanied in extracting the relevant data from repositories and then performing data analysis, which often requires the use of statistical methods or machine learning. Results are returned using simple representations (spreadsheets and graphics), while at the end of a session the dialogue is summarized in textual format. The innovation presented in this article is concerned with not only the delivery of a new tool but also our novel approach to conversational technologies, potentially extensible to other healthcare domains or to general data science.
Differentially Private Medical Texts Generation Using Generative Neural Networks
Technological advancements in data science have offered us affordable storage and efficient algorithms to query a large volume of data. Our health records are a significant part of this data, which is pivotal for healthcare providers and can be utilized in our well-being. The clinical note in electronic health records is one such category that collects a patient’s complete medical information during different timesteps of patient care available in the form of free-texts. Thus, these unstructured textual notes contain events from a patient’s admission to discharge, which can prove to be significant for future medical decisions. However, since these texts also contain sensitive information about the patient and the attending medical professionals, such notes cannot be shared publicly. This privacy issue has thwarted timely discoveries on this plethora of untapped information. Therefore, in this work, we intend to generate synthetic medical texts from a private or sanitized (de-identified) clinical text corpus and analyze their utility rigorously in different metrics and levels. Experimental results promote the applicability of our generated data as it achieves more than 80\% accuracy in different pragmatic classification problems and matches (or outperforms) the original text data.
Impact on Stock Market across Covid-19 Outbreak
Abstract: This paper analysis the impact of pandemic over the global stock exchange. The stock listing values are determined by variety of factors including the seasonal changes, catastrophic calamities, pandemic, fiscal year change and many more. This paper significantly provides analysis on the variation of listing price over the world-wide outbreak of novel corona virus. The key reason to imply upon this outbreak was to provide notion on underlying regulation of stock exchanges. Daily closing prices of the stock indices from January 2017 to January 2022 has been utilized for the analysis. The predominant feature of the research is to analyse the fact that does global economy downfall impacts the financial stock exchange. Keywords: Stock Exchange, Matplotlib, Streamlit, Data Science, Web scrapping.
Information Resilience: the nexus of responsible and agile approaches to information use
AbstractThe appetite for effective use of information assets has been steadily rising in both public and private sector organisations. However, whether the information is used for social good or commercial gain, there is a growing recognition of the complex socio-technical challenges associated with balancing the diverse demands of regulatory compliance and data privacy, social expectations and ethical use, business process agility and value creation, and scarcity of data science talent. In this vision paper, we present a series of case studies that highlight these interconnected challenges, across a range of application areas. We use the insights from the case studies to introduce Information Resilience, as a scaffold within which the competing requirements of responsible and agile approaches to information use can be positioned. The aim of this paper is to develop and present a manifesto for Information Resilience that can serve as a reference for future research and development in relevant areas of responsible data management.
qEEG Analysis in the Diagnosis of Alzheimers Disease; a Comparison of Functional Connectivity and Spectral Analysis
Alzheimers disease (AD) is a brain disorder that is mainly characterized by a progressive degeneration of neurons in the brain, causing a decline in cognitive abilities and difficulties in engaging in day-to-day activities. This study compares an FFT-based spectral analysis against a functional connectivity analysis based on phase synchronization, for finding known differences between AD patients and Healthy Control (HC) subjects. Both of these quantitative analysis methods were applied on a dataset comprising bipolar EEG montages values from 20 diagnosed AD patients and 20 age-matched HC subjects. Additionally, an attempt was made to localize the identified AD-induced brain activity effects in AD patients. The obtained results showed the advantage of the functional connectivity analysis method compared to a simple spectral analysis. Specifically, while spectral analysis could not find any significant differences between the AD and HC groups, the functional connectivity analysis showed statistically higher synchronization levels in the AD group in the lower frequency bands (delta and theta), suggesting that the AD patients brains are in a phase-locked state. Further comparison of functional connectivity between the homotopic regions confirmed that the traits of AD were localized in the centro-parietal and centro-temporal areas in the theta frequency band (4-8 Hz). The contribution of this study is that it applies a neural metric for Alzheimers detection from a data science perspective rather than from a neuroscience one. The study shows that the combination of bipolar derivations with phase synchronization yields similar results to comparable studies employing alternative analysis methods.
Big Data Analytics for Long-Term Meteorological Observations at Hanford Site
A growing number of physical objects with embedded sensors with typically high volume and frequently updated data sets has accentuated the need to develop methodologies to extract useful information from big data for supporting decision making. This study applies a suite of data analytics and core principles of data science to characterize near real-time meteorological data with a focus on extreme weather events. To highlight the applicability of this work and make it more accessible from a risk management perspective, a foundation for a software platform with an intuitive Graphical User Interface (GUI) was developed to access and analyze data from a decommissioned nuclear production complex operated by the U.S. Department of Energy (DOE, Richland, USA). Exploratory data analysis (EDA), involving classical non-parametric statistics, and machine learning (ML) techniques, were used to develop statistical summaries and learn characteristic features of key weather patterns and signatures. The new approach and GUI provide key insights into using big data and ML to assist site operation related to safety management strategies for extreme weather events. Specifically, this work offers a practical guide to analyzing long-term meteorological data and highlights the integration of ML and classical statistics to applied risk and decision science.
Export Citation Format
Share document.

For IEEE Members
Ieee spectrum, follow ieee spectrum, support ieee spectrum, enjoy more free content and benefits by creating an account, saving articles to read later requires an ieee spectrum account, the institute content is only available for members, downloading full pdf issues is exclusive for ieee members, downloading this e-book is exclusive for ieee members, access to spectrum 's digital edition is exclusive for ieee members, following topics is a feature exclusive for ieee members, adding your response to an article requires an ieee spectrum account, create an account to access more content and features on ieee spectrum , including the ability to save articles to read later, download spectrum collections, and participate in conversations with readers and editors. for more exclusive content and features, consider joining ieee ., join the world’s largest professional organization devoted to engineering and applied sciences and get access to all of spectrum’s articles, archives, pdf downloads, and other benefits. learn more about ieee →, join the world’s largest professional organization devoted to engineering and applied sciences and get access to this e-book plus all of ieee spectrum’s articles, archives, pdf downloads, and other benefits. learn more about ieee →, access thousands of articles — completely free, create an account and get exclusive content and features: save articles, download collections, and talk to tech insiders — all free for full access and benefits, join ieee as a paying member., data science news & articles.
Showing 14 posts that have the tag “data-science”
This Startup Is Building the Internet of Underwater Things
Wsense’s innovative networking systems are transforming how we explore ocean environments, deploying data science and ai to fight wildlife trafficking, nyu tandon’s juliana freire is leading a team aimed at using data science to bring down criminals trafficking humans and exotic animals, pfizer’s edge in the covid-19 vaccine race: data science, a year in the life of the data scientists who helped bring pfizer's covid-19 vaccine to the public in record time, engineering bias out of ai, machines that learn the worst human impulses can still relearn, robotics news in your inbox, weekly.

Fully Open Access Topical Journals
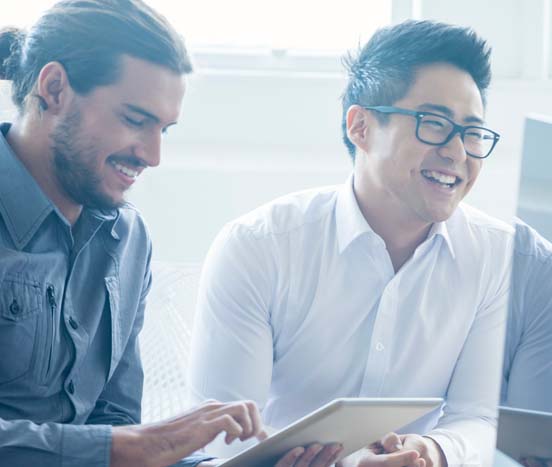
A Growing Collection of Gold Fully Open Access (OA) Options
IEEE offers more options than ever to authors with the launch of new gold fully open access journals spanning a wide range of technologies. These journals are significant additions to IEEE’s well-known and respected portfolio of fully open access journals. In addition, many of the journals featured here target an accelerated publication time frame of 10 weeks for most accepted papers to help get your research exposed faster. Visit the publication home page of each title for details.
The fully open access journals are accepting submissions. Please see each journal’s description below for more details. All of the titles are fully compliant with funder mandates including Plan S. All IEEE Open Access titles, current and new, will be hosted on the IEEE Xplore ® platform.
Call for Papers
Submit a paper to an ieee fully open access journal.
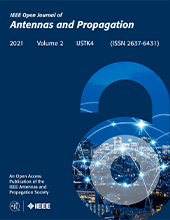
IEEE Open Journal of Antennas and Propagation
High-quality, peer reviewed research covering antennas, including analysis, design, development, measurement, standards, and testing; radiation, propagation, and the interaction of electromagnetic waves with discrete and continuous media.
This fully open access journal publishes high-quality, peer reviewed papers covering antennas, including analysis, design, development, measurement, standards, and testing; radiation, propagation, and the interaction of electromagnetic waves with discrete and continuous media; and applications and systems pertinent to antennas, propagation, and sensing, such as applied optics, millimeter-and sub-millimeter-wave techniques, antenna signal processing and control, radio astronomy, and propagation and radiation aspects of terrestrial and space-based communication, including wireless, mobile, satellite, and telecommunications at all frequencies. The journal peer-review process targets a publication period of 10 weeks from submission to online publication.
Editor-in-Chief: Konstantina (Nantia) Nikita Professor National Technical University of Athens, Greece
Learn More and Submit a Paper
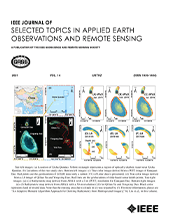
IEEE Journal of Selected Topics in Applied Earth Observations and Remote Sensing
Addresses the growing field of applications in Earth observations and remote sensing and provides a venue for the rapidly expanding special issues that are being sponsored by the IEEE Geosciences and Remote Sensing Society.
The IEEE Journal of Selected Topics in Applied Earth Observations and Remote Sensing addresses the growing field of applications in Earth observations and remote sensing, and also provides a venue for the rapidly expanding special issues that are being sponsored by the IEEE Geosciences and Remote Sensing Society. The journal draws upon the experience of the highly successful “IEEE Transactions on Geoscience and Remote Sensing” and provide a complementary medium for the wide range of topics in applied earth observations. Papers should address current issues and techniques in applied remote and in situ sensing, their integration, and applied modeling and information creation for understanding the Earth. Applications are for the Earth, oceans and atmosphere. Topics can include observations, derived information such as forecast data, simulated information, data assimilation and Earth information techniques to address science and engineering issues of the Earth system. The technical content of papers must be both new and significant.
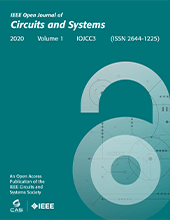
IEEE Open Journal of Circuits and Systems
Featuring high-quality peer reviewed research covering the theory, analysis, design, tools, and implementation of circuits and systems.
This fully open access journal publishes high-quality, peer-reviewed papers covering the theory, analysis, design, tools, and implementation of circuits and systems. This includes their theoretical foundations, applications, and architectures, as well as circuits and systems implementation of algorithms for signal and information processing. The journal peer-review process targets a publication period of 10 weeks from submission to online publication.
Editor-in-Chief: Gabriele Manganaro, Ph.D., FIEEE Technology Director Analog Devices, Inc., USA
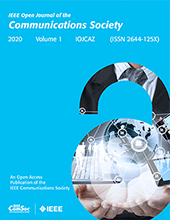
IEEE Open Journal of the Communications Society
Featuring high-quality peer reviewed research covering science, technology, applications and standards for information organization, collection and transfer using electronic, optical and wireless channels and networks.
As a fully open access journal publishing high-quality peer reviewed papers, IEEE Open Journal of the Communications Society covers science, technology, applications and standards for information organization, collection and transfer using electronic, optical and wireless channels and networks, including but not limited to: Systems and network architecture, control and management; Protocols, software and middleware; Quality of service, reliability and security; Modulation, detection, coding, and signaling; Switching and routing; Mobile and portable communications; Terminals and other end-user devices; Networks for content distribution and distributed computing; and Communications-based distributed resources control. The journal peer-review process targets a publication period of 10 weeks from submission to online publication.
Editor-in-Chief: Octavia A. Dobre, Dipl.-Ing., Ph.D. Professor and Research Chair Memorial University, Canada
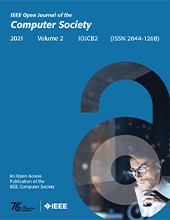
IEEE Open Journal of the Computer Society
Forum for rapid publication of open access articles describing high-impact results in all aspects of theory, design, practice, and application relating to computer and information processing science and technology.
The IEEE Open Journal of the Computer Society (OJ-CS) is a rigorously peer-reviewed forum for rapid publication of open access articles describing high-impact results in all areas of interest to the IEEE Computer Society. This new fully open access journal complements existing IEEE Computer Society publications by providing a rapid review cycle and a thorough review of technical articles. It is dedicated to publishing articles on the latest emerging topics and trends in all aspects of computing with a scope that encompasses all aspects of theory, design, practice, and application relating to computer and information processing science and technology. The journal peer-review process targets a publication period of 10 weeks from submission to online publication.
Editor-in-Chief: Dr. Song Guo Department of Computing The Hong Kong Polytechnic University
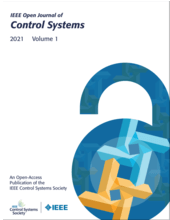
IEEE Open Journal of Control Systems
Publication of the IEEE Control Systems Society, this journal aims to publish high-quality papers on the theory, design, optimization, and applications of dynamic systems and control.
The IEEE Open Journal of Control Systems covers the theory, design, optimization, and applications of dynamic systems and control. The field integrates elements of sensing, communication, decision and actuation components, as relevant for the analysis, design and operation of dynamic systems and control. The systems considered include: technological, physical, biological, economic, organizational and other entities, and combinations thereof. The journal peer-review process targets a publication period of 10 weeks from submission to online publication.
Editor-in-Chief: Sonia Martínez University of California, San Diego United States
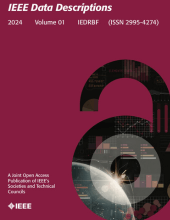
IEEE Data Descriptions
Now Accepting Submissions! This new publication is a peer-reviewed journal that publishes short articles on all aspects of data: data descriptors, data collections, and metadata.
IEEE Data Descriptions is a peer-reviewed journal that publishes short articles on all aspects of data: data descriptors, data collections, and metadata. Its overarching purpose is to promote publicly available datasets (open access or subscription-based access) in support of reproducible science while at the same time bringing insights into the associated dataset, data collection methods, and data quality. The metadata collected provides enhanced dataset discoverability and creates a foundation for future data science tools such as auto-discovery and mashups.
Datasets described in IEEE Data Descriptions must be findable, accessible, interoperable, and reusable. The dataset needs to be of a quality high enough that other researchers can use it for their research experimentation and have some permanence. Articles describing datasets must be comprehensive and follow the outlined sections listed in Author Information. The preference is for data to be stored within IEEE DataPort, however, IEEE Data Descriptions accepts submissions where data is stored at other persistent/permanent locations.
Editor-in-Chief: Stephen Makonin Simon Fraser University Vancouver, Canada
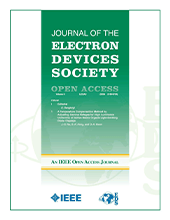
IEEE Journal of the Electron Devices Society
Featuring high quality research in the field of electron and ion devices ranging from fundamentals to applied research.
Featuring high-quality research in the field of electron and ion devices ranging from fundamentals to applied research, this journal provides authors an affordable outlet for rapid publishing and universal access, coupled with superior technical quality.
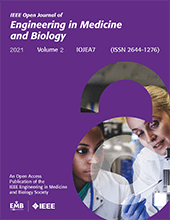
IEEE Open Journal of Engineering in Medicine and Biology
High-quality research covering the development and application of engineering concepts and methods to biology, medicine and health sciences.
As a fully open access journal publishing high-quality peer reviewed papers, IEEE Open Journal of Engineering in Medicine and Biology covers the development and application of engineering concepts and methods to biology, medicine and health sciences to provide effective solutions to biological, medical and healthcare problems. It encompasses the development of mathematical theories, physical, biological and chemical principles, computational models and algorithms, devices and systems for clinical, industrial and educational applications. The journal peer-review process targets a publication period of 10 weeks from submission to online publication.
Editor-in-Chief: Paolo Bonato Associate Professor Harvard University, USA
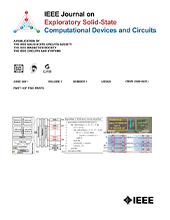
IEEE Journal on Exploratory Solid-State Computational Devices and Circuits
Multi-disciplinary research in solid-state circuits using exploratory materials and devices for novel energy efficient computation beyond standard CMOS (Complementary Metal Oxide Semiconductor) technology.
Multi-disciplinary research in solid-state circuits using exploratory materials and devices for novel energy efficient computation beyond standard CMOS (Complementary Metal Oxide Semiconductor) technology. Focus is on the exploration of materials, devices and computation circuits to enable Moore’s Law to continue for computation beyond a 10 to 15 year horizon (beyond end of the roadmap for CMOS technologies) with the associated density scaling and improvement in energy efficiency.
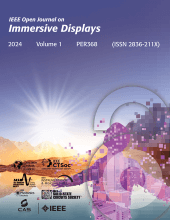
IEEE Open Journal on Immersive Displays
Now Accepting Submissions! New publication will be home to publications in display science and applications.
The IEEE Open Journal on Immersive Displays (OJID) will be home to publications in display science and applications. The field of displays is diverse, ranging from the science and engineering of materials and devices to their application in high definition, form-factor-independent displays featuring interactivity, virtual and augmented reality, and 3D content. Submissions on advanced fabrication processing, thin film active and passive devices, and lifetime and reliability evaluation are welcome when display is the focus or where there is a direct relationship to the nature of the display system. Tutorial and review papers extending the frontiers of immersive display technologies and novel applications are also published.
Editor-in-Chief: Arokia Nathan University of Cambridge Hertfordshire, U.K.
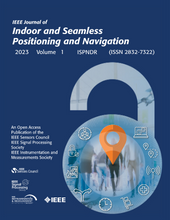
IEEE Journal of Indoor and Seamless Positioning and Navigation
Publishes original research in the fields of localization and tracking of people, robots, and objects.
IEEE Journal of Indoor and Seamless Positioning and Navigation (J-ISPIN) publishes original research in the fields of localization and tracking of people, robots, and objects. It covers all aspects of localization systems, including sensing, communications, location-based services, mapping, protocols, human interfaces and standards. The scope includes methods and systems addressing indoor environments as well as those enabling seamless transition between heterogeneous indoor contexts or between indoor and outdoor environments, for example where Global Navigation Satellites Systems are underperforming or unavailable.
Editor-in-Chief: Valérie Renaudin Senior Researcher University Gustave Eiffel, France
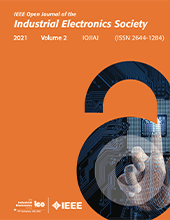
IEEE Open Journal of the Industrial Electronics Society
Featuring high quality research covering the theory and applications of electronics, controls, communications, instrumentation and computational intelligence to industrial and manufacturing systems and processes.
This fully open access journal publishes high-quality, peer-reviewed papers covering the theory and applications of electronics, controls, communications, instrumentation and computational intelligence to industrial and manufacturing systems and processes. The journal peer-review process targets a publication period of 10 weeks from submission to online publication.
Editor-in-Chief: Dr. Leopoldo Garcia Franquelo Professor, Electronics Engineering Universidad de Sevilla, Spain
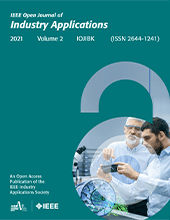
IEEE Open Journal of Industry Applications
Covering the advancement of the theory and practice of electrical and electronic engineering in the development, design, manufacture and application of electrical systems, apparatus, devices, and controls to the processes and equipment of industry and commerce.
As a fully open access journal publishing high-quality peer reviewed papers, IEEE Open Journal of Industry Applications covers the advancement of the theory and practice of electrical and electronic engineering in the development, design, manufacture and application of electrical systems, apparatus, devices, and controls to the processes and equipment of industry and commerce; the promotion of safe, reliable, and economic installations; industry leadership in energy conservation and environmental, health, and safety issues; the creation of voluntary engineering standards and recommended practices; and the professional development of its readers. The journal peer-review process targets a publication period of 10 weeks from submission to online publication.
Editor-in-Chief: Professor Pericle Zanchetta Fellow IEEE Faculty of Engineering University of Nottingham, UK
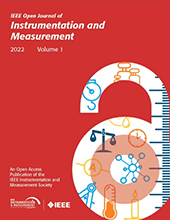
IEEE Open Journal of Instrumentation and Measurement
Publication of the Instrumentation and Measurement Society, this journal publishes papers on the science, technology, and application of instrumentation and measurement.
The IEEE Open Journal of Instrumentation and Measurement publishes papers on the science, technology, and application of instrumentation and measurement. Instrumentation and measurement, in the current context of the IEEE IMS community, consists of methods, instruments, systems, and applications for measurement, detection, tracking, monitoring, characterization, identification, sensing, estimation, recognition, or diagnosis of a physical phenomenon; or metrology and measurement theory including measurement uncertainty, instrument precision, calibration, etc.
Editor-in-Chief: Shervin Shirmohammadi University of Ottawa Canada
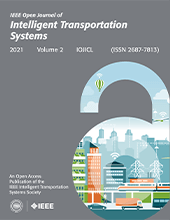
IEEE Open Journal of Intelligent Transportation Systems
Featuring high-quality research covering the theoretical, experimental and operational aspects of electrical and electronics engineering and information technologies as applied to Intelligent Transportation Systems (ITS).
As a fully open access journal publishing high-quality peer reviewed papers, IEEE Open Journal of Intelligent Transportation Systems covers theoretical, experimental and operational aspects of electrical and electronics engineering and information technologies as applied to Intelligent Transportation Systems (ITS), defined as those systems utilizing synergistic technologies and systems engineering concepts to develop and improve transportation systems of all kinds. The journal peer-review process targets a publication period of 10 weeks from submission to online publication.
Editor-in-Chief: Dr. Bart van Arem Full Professor of Transport Modelling Delft University of Technology, The Netherlands
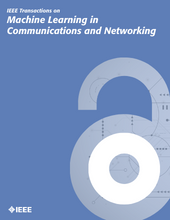
IEEE Transactions on Machine Learning in Communications and Networking
Featuring high-quality manuscripts on advances in machine learning methods for and applications to communications and networking.
The IEEE Transactions on Machine Learning in Communications and Networking publishes high-quality manuscripts on advances in machine learning methods for and applications to communications and networking. Furthermore, articles developing novel communication and networking techniques for distributed machine learning algorithms are of interest. Both theoretical contributions (including new theories, techniques, concepts, algorithms, and analyses) and practical contributions (including system experiments, prototypes, and new applications) are encouraged.
Editor-in-Chief: Walid Saad Professor Virginia Tech Research Center – Arlington, USA
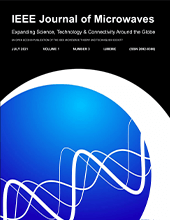
IEEE Journal of Microwaves
Covering articles on the theory, techniques and applications of guided wave and wireless technologies and spanning the electromagnetic spectrum from RF/microwave through millimeter-waves and terahertz.
The IEEE Journal of Microwaves is a fully open access publication covering the complete scope of the Microwave Theory and Techniques Society which includes articles on the theory, techniques and applications of guided wave and wireless technologies and spanning the electromagnetic spectrum from RF/microwave through millimeter-waves and terahertz, covering the aspects of materials, components, devices, circuits, modules, and systems which involve the generation, modulation, demodulation, control, transmission, sensing and effects of electromagnetic signals.
Editor-in-Chief: Peter H. Siege THz Global, NASA Jet Propulsion Laboratory, California Institute of Technology Pasadena, California
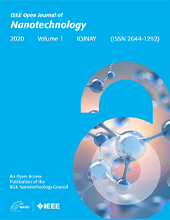
IEEE Open Journal of Nanotechnology
Featuring high-quality, peer reviewed research covering the theory, design, and development of nanotechnology and its scientific, engineering, and industrial applications.
As a fully open access journal publishing high-quality peer reviewed papers, IEEE Open Journal of Nanotechnology covers the theory, design, and development of nanotechnology and its scientific, engineering, and industrial applications. The journal peer-review process targets a publication period of 10 weeks from submission to online publication.
Co-Editors-in-Chief: Professor Wen J. Li Chair Professor of Biomedical Engineering Associate Provost City University of Hong Kong, Hong Kong
Professor Jin-Woo Kim Professor of Biological Engineering and Nanoscience & Engineering University of Arkansas, USA
Professor Seiji Samukawa Director of Innovative Energy Research Center, Institute of Fluid Science (IFS) Principal Investigator of Advance Institute for Materials Research (AIMR) Tohoku University, Japan
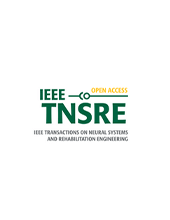
IEEE Transactions on Neural Systems and Rehabilitation Engineering
Covering the rehabilitative and neural aspects of biomedical engineering, including functional electrical stimulation, acoustic dynamics, human performance measurement, and more.
Rehabilitative and neural aspects of biomedical engineering, including functional electrical stimulation, acoustic dynamics, human performance measurement and analysis, nerve stimulation, electromyography, motor control and stimulation; and hardware and software applications for rehabilitation engineering and assistive devices.
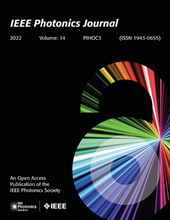
IEEE Photonics Journal
Dedicated to the rapid disclosure of research at the forefront of all areas of photonics and addressing issues ranging from fundamental understanding to emerging technologies.
Breakthroughs in the generation of light and its control and utilization have given rise to the field of Photonics: a rapidly expanding area of science and technology with major technological and economic impact. IEEE Photonics Journal is an online-only journal dedicated to the rapid disclosure of top-quality peer-reviewed research at the forefront of all areas of photonics. Contributions addressing issues ranging from fundamental understanding to emerging technologies and applications are within the scope of the Journal.
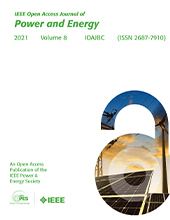
IEEE Open Access Journal of Power and Energy
High-quality, peer reviewed research covering the development, planning, design, construction, maintenance, installation, and operation of equipment, structures, power systems and usage of electric energy, including its measurement and control.
As a fully open access journal publishing high-quality peer reviewed papers, the IEEE Open Access Journal of Power and Energy publishes articles focused on the development, planning, design, construction, maintenance, installation, and operation of equipment, structures, and power systems for the safe, sustainable, economic, and reliable conversion, generation, transmission, distribution, storage, and usage of electric energy, including its measurement and control. The journal peer-review process targets a publication period of 10 weeks from submission to online publication.
Editor-in-Chief: Fangxing “Fran” Li The University of Tennessee Knoxville, TN 37996 USA [email protected]
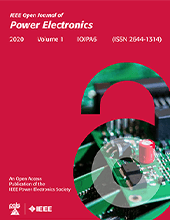
IEEE Open Journal of Power Electronics
Covering the development and application of power electronic systems and technologies, which encompass the effective use of electronic components, the application of circuit theory and design techniques and the development of analytical methods and tools.
The IEEE Open Journal of Power Electronics covers the development and application of power electronic systems and technologies, which encompass the effective use of electronic components, the application of circuit theory and design techniques and the development of analytical methods and tools toward efficient electronic conversion, control and conditioning of electric power to enable the sustainable use of energy. As a fully open access journal publishing high-quality peer reviewed papers, the Society’s aim is to publish novel developments as well as tutorial and survey articles including those of value to both the R&D and practicing professionals in the field. The journal peer-review process targets a publication period of 10 weeks from submission to online publication.
Editor-in-Chief: Alan Mantooth, Ph.D., P.E., FIEEE Distinguished Professor of Electrical Engineering University of Arkansas, USA
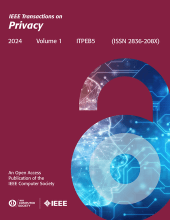
IEEE Transactions on Privacy
Now Accepting Submissions! New publication will provide a multidisciplinary forum for theoretical, methodological, engineering, and applications aspects of privacy and data protection, including specification, design, implementation, testing, and validation.
The IEEE Transactions on Privacy provides a multidisciplinary forum for theoretical, methodological, engineering, and applications aspects of privacy and data protection, including specification, design, implementation, testing, and validation. Privacy, in this context, is defined as the freedom from unauthorized intrusion in its broadest sense, arising from any activity in information collection, information processing, information dissemination or invasion. The transactions publishes articles reporting significant advances in theoretical models and formalization as well as engineering tools supporting the above activities, design frameworks and languages, architectures, infrastructures, model-based approaches, study cases, and standards.
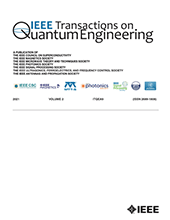
IEEE Transactions on Quantum Engineering
Publishing regular, review, and tutorial articles based on the engineering applications of quantum phenomena, including quantum computation, information, communication, software, hardware, devices, and metrology.
Publishes regular, review, and tutorial articles based on the engineering applications of quantum phenomena, including quantum computation, information, communication, software, hardware, devices, and metrology. Articles also address quantum-engineering aspects of superconductivity, magnetics, microwave techniques, photonics, and signal processing.
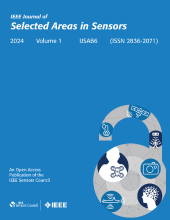
IEEE Journal of Selected Areas in Sensors
Now Accepting Submissions! New publication of the IEEE Sensors Council, this journal publishes papers in all areas of the field of interest of the IEEE Sensors Council.
The IEEE Journal of Selected Areas in Sensors publishes papers in all areas of the field of interest of the IEEE Sensors Council, i.e., the theory, design, simulation, fabrication, manufacturing and application of devices for sensing and transducing physical, chemical, and biological phenomena, with emphasis on the electronics, physics and reliability aspects of sensors and integrated sensor-actuators. The Journal is built exclusively from papers on selected topics of current interest to the Sensors community.
Editor-in-Chief: Chonggang Wang InterDigital, Inc. USA
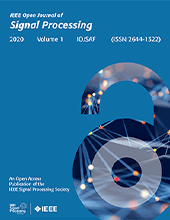
IEEE Open Journal of Signal Processing
High-quality, peer reviewed research covering the enabling technology for the generation, transformation, extraction, and interpretation of information.
This fully open access journal publishes high-quality, peer-reviewed papers covering the enabling technology for the generation, transformation, extraction, and interpretation of information. It comprises the theory, algorithms with associated architectures and implementations, and applications related to processing information contained in many different formats broadly designated as signals. Signal processing uses mathematical, statistical, computational, heuristic, and/or linguistic representations, formalisms, modeling techniques and algorithms for generating, transforming, transmitting, and learning from signals. The journal peer-review process targets a publication period of 10 weeks from submission to online publication.
Editor-in-Chief: Brendt Wohlberg Los Alamos National Laboratory, USA
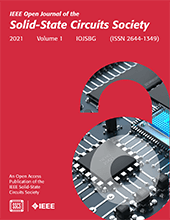
IEEE Open Journal of the Solid-State Circuits Society
High-quality, peer reviewed research covering the design, implementation, and application of solid-state integrated circuits.
As a fully open access journal publishing high-quality peer reviewed papers, IEEE Open Journal of the Solid-State Circuits Society covers design, implementation and application of solid-state integrated circuits. The journal peer-review process targets a publication period of 10 weeks from submission to online publication.
Editor-in-Chief: Jan Craninckx Distinguished Member of Technical Staff IMEC, Belgium
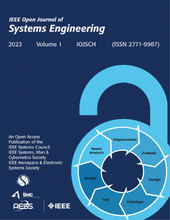
IEEE Open Journal of Systems Engineering
Provides a forum for practitioners, scientists, academics, and researchers engaged in the discipline of Systems Engineering.
The IEEE Open Journal of Systems Engineering (OJSE) is an open access journal that is sponsored by the consortium of IEEE Aerospace and Electronic Systems Society, IEEE Systems, Man, and Cybernetics Society, and the IEEE Systems Council. OJSE provides a forum for practitioners, scientists, academics, and researchers engaged in the discipline of Systems Engineering. Multidisciplinary aspects of systems engineering is a focus of this journal. Methodologies, tools, principles, and applied engineering aspects of the process of systems engineering for complex systems are of interest. The methodologies, tools, and principles include such elements as model-based systems engineering; digital thread; requirements generation, flowdown, tracking, needs analysis, validation/verification; integration and test; and full life cycle of the target system. OJSE deals primarily with the science, methodology, and tools of systems engineering, rather than the results of the application of systems engineering that is the focus of other IEEE journals.
Editor-in-Chief: W. Dale Blair Principal Research Engineer Georgia Tech Research Institute, USA
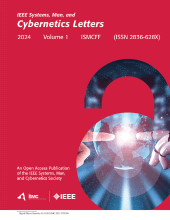
IEEE Systems, Man, and Cybernetics Letters
Now Accepting Submissions! New publication of the IEEE Systems, Man, and Cybernetics Society (SMC), this journal covers the ultimate aims and key strategies of the SMC society towards the next generation of symbiotic human and machine intelligence systems.
IEEE Systems, Man, and Cybernetics Letter (SMC-L) will cover the ultimate aims and key strategies of the SMC society towards the next generation of symbiotic human and machine intelligence systems. The feature of SMC-L is highlighted by its rapid publication of peer-reviewed short articles within 5 pages, which provide a timely and concise account of innovative research ideas, novel application results, and significant theoretical findings, as well as analyses of emerging trends and groundbreakingly work in SMC fields. SMC-L will provide a new means for members and readers to complement established SMC transactions.
Editor-in-Chief: Prof. Yingxu Wang Editor-In-Chief Univ. of Calgary, Canada
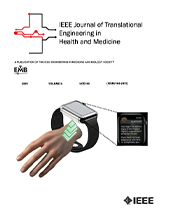
IEEE Journal of Translational Engineering in Health and Medicine
Bridges the engineering and clinical worlds, focusing on detailed descriptions of advanced technical solutions to a clinical need along with clinical results and healthcare relevance.
This journal bridges the engineering and clinical worlds, focusing on detailed descriptions of advanced technical solutions to a clinical need along with clinical results and healthcare relevance. Its aim is to provide a platform for state-of-the-art technology directions in the interdisciplinary field of biomedical engineering, embracing engineering, life sciences and medicine. The journal provides an active forum for clinical research and relevant state-of-the-art technology for members of all the IEEE societies that have an interest in biomedical engineering as well as reaching out directly to physicians and the medical community through the American Medical Association (AMA) and other clinical societies.
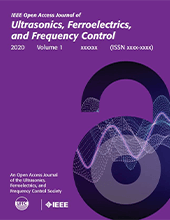
IEEE Open Journal of Ultrasonics, Ferroelectrics, and Frequency Control
Covering high-quality, peer reviewed research theory, technology, materials, and applications relating to the generation, transmission, and detection of ultrasonic waves and related phenomena.
OJ-UFFC covers theory, technology, materials, and applications relating to: the generation, transmission, and detection of ultrasonic waves and related phenomena; medical ultrasound, and associated technologies; ferroelectric, piezoelectric, and piezomagnetic materials; frequency generation and control, timing, and time coordination and distribution. This interest ranges from fundamental studies to the design and/or applications of devices, sensors, systems and manufacturing technologies within the general scope defined above. The journal peer-review process targets a publication period of 10 weeks from submission to online publication.
Editor-in-Chief: Steven Freear University of Leeds, School of Electronic and Electrical Engineering Leeds, United Kingdom
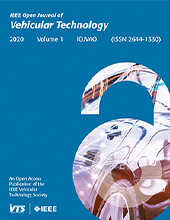
IEEE Open Journal of Vehicular Technology
Featuring high-quality, peer reviewed research on the theoretical, experimental and operational aspects of electrical and electronics engineering in mobile radio, motor vehicles and land transportation.
This fully open access journal publishes high-quality, peer-reviewed papers covering the theoretical, experimental and operational aspects of electrical and electronics engineering in mobile radio, motor vehicles and land transportation. (a) Mobile radio shall include all terrestrial mobile services. (b) Motor vehicles shall include the components and systems and motive power for propulsion and auxiliary functions. (c) Land transportation shall include the components and systems used in both automated and non-automated facets of ground transport technology. The journal peer-review process targets a publication period of 10 weeks from submission to online publication.
Editor-in-Chief: Dr. Sumei Sun Fellow of the IEEE Principal Scientist, Institute for Infocomm Research Adjunct Professor, National University of Singapore, Singapore

Sign up for IEEE open access news
Sign Up Now

Institutions
Learn about open access options

Answers to common questions on open access

Society Members
APC discounts now available
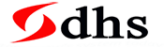
IEEE 2024-2025 : Data Science Projects
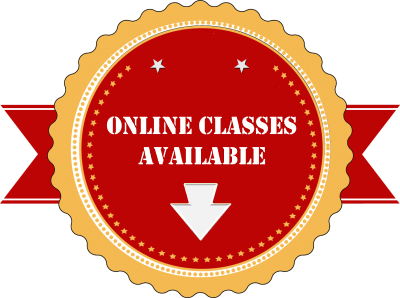
For Outstation Students, we are having online project classes both technical and coding using net-meeting software
For details, call: 9886692401/9845166723.
DHS Informatics providing latest 2024-2025 IEEE projects on Data science for the final year engineering students. DHS Informatics trains all students to develop their project with good idea what they need to submit in college to get good marks. DHS Informatics offers placement training in Bangalore and the program name is OJT – On Job Training , job seekers as well as final year college students can join in this placement training program and job opportunities in their dream IT companies. We are providing IEEE projects for B.E / B.TECH, M.TECH, MCA, BCA, DIPLOMA students from more than two decades.
Python Final year CSE projects in Bangalore
- Python 2024 – 2025 IEEE PYTHON PROJECTS CSE | ECE | ISE
- Python 2024 – 2025 IEEE PYTHON MACHINE LEARNING PROJECTS
- Python 2024 – 2025 IEEE PYTHON IMAGE PROCESSING PROJECTS
- Python 2024 – 2025 IEEE IOT PYTHON RASPBERRY PI PROJECTS
DATA SCIENCE PROJECTS
A data mining based model for detection of fraudulent behaviour in water consumption.
Abstract: Fraudulent behavior in drinking water consumption is a significant problem facing water supplying companies and agencies. This behavior results in a massive loss of income and forms the highest percentage of non-technical loss. Finding efficient measurements for detecting fraudulent activities has been an active research area in recent years. Intelligent data mining techniques can help water supplying companies to detect these fraudulent activities to reduce such losses. This research explores the use of two classification techniques (SVM and KNN) to detect suspicious fraud water customers. The main motivation of this research is to assist Yarmouk Water Company (YWC) in Irbid city of Jordan to overcome its profit loss. The SVM based approach uses customer load profile attributes to expose abnormal behavior that is known to be correlated with non-technical loss activities. The data has been collected from the historical data of the company billing system. The accuracy of the generated model hit a rate of over 74% which is better than the current manual prediction procedures taken by the YWC. To deploy the model, a decision tool has been built using the generated model. The system will help the company to predict suspicious water customers to be inspected on site.
Correlated Matrix Factorization for Recommendation with Implicit Feedback
Abstract: As a typical latent factor model, Matrix Factorization (MF) has demonstrated its great effectiveness in recommender systems. Users and items are represented in a shared low-dimensional space so that the user preference can be modeled by linearly combining the item factor vector V using the user-specific coefficients U. From a generative model perspective, U and V are drawn from two independent Gaussian distributions, which is not so faithful to the reality. Items are produced to maximally meet users’ requirements, which makes U and V strongly correlated. Meanwhile, the linear combination between U and V forces a bisection (one-to-one mapping), which thereby neglects the mutual correlation between the latent factors. In this paper, we address the upper drawbacks, and propose a new model, named Correlated Matrix Factorization (CMF). Technically, we apply Canonical Correlation Analysis (CCA) to map U and V into a new semantic space. Besides achieving the optimal fitting on the rating matrix, one component in each vector (U or V) is also tightly correlated with every single component in the other. We derive efficient inference and learning algorithms based on variational EM methods. The effectiveness of our proposed model is comprehensively verified on four public data sets. Experimental results show that our approach achieves competitive performance on both prediction accuracy and efficiency compared with the current state of the art.
Heterogeneous Information Network Embedding for Recommendation
Abstract: Due to the flexibility in modelling data heterogeneity, heterogeneous information network (HIN) has been adopted to characterize complex and heterogeneous auxiliary data in recommended systems, called HIN based recommendation. It is challenging to develop effective methods for HIN based recommendation in both extraction and exploitation of the information from HINs. Most of HIN based recommendation methods rely on path based similarity, which cannot fully mine latent structure features of users and items. In this paper, we propose a novel heterogeneous network embedding based approach for HIN based recommendation, called HERec. To embed HINs, we design a meta-path based random walk strategy to generate meaningful node sequences for network embedding. The learned node embeddings are first transformed by a set of fusion functions, and subsequently integrated into an extended matrix factorization (MF) model. The extended MF model together with fusion functions are jointly optimized for the rating prediction task. Extensive experiments on three real-world datasets demonstrate the effectiveness of the HERec model. Moreover, we show the capability of the HERec model for the cold-start problem, and reveal that the transformed embedding information from HINs can improve the recommendation performance.
NetSpam: A Network-Based Spam Detection Framework for Reviews in Online Social Media
Abstract: Nowadays, a big part of people rely on available content in social media in their decisions (e.g., reviews and feedback on a topic or product). The possibility that anybody can leave a review provides a golden opportunity for spammers to write spam reviews about products and services for different interests. Identifying these spammers and the spam content is a hot topic of research, and although a considerable number of studies have been done recently toward this end, but so far the methodologies put forth still barely detect spam reviews, and none of them show the importance of each extracted feature type. In this paper, we propose a novel framework, named NetSpam, which utilizes spam features for modeling review data sets as heterogeneous information networks to map spam detection procedure into a classification problem in such networks. Using the importance of spam features helps us to obtain better results in terms of different metrics experimented on real-world review data sets from Yelp and Amazon Web sites. The results show that NetSpam outperforms the existing methods and among four categories of features, including review-behavioral, user-behavioral, review-linguistic, and user-linguistic, the first type of features performs better than the other categories.
Comparative Study to Identify the Heart Disease Using Machine Learning Algorithms
Abstract: Nowadays, heart disease is a common and frequently present disease in the human body and it’s also hunted lots of humans from this world. Especially in the USA, every year mass people are affected by this disease after that in India also. Doctor and clinical research said that heart disease is not a suddenly happen disease it’s the cause of continuing irregular lifestyle and different body’s activity for a long period after then it’s appeared in sudden with symptoms. After appearing those symptoms people seek for a treat in hospital for taken different test and therapy but these are a little expensive. So awareness before getting appeared in this disease people can get an idea about the patient condition from this research result. This research collected data from different sources and split that data into two parts like 80% for the training dataset and the rest 20% for the test dataset. Using different classifier algorithms tried to get better accuracy and then summarize that accuracy. These algorithms are namely Random Forest Classifier, Decision Tree Classifier, Support Vector Machine, k-nearest neighbor, Logistic Regression, and Naive Bayes. SVM, Logistic Regression, and KNN gave the same and better accuracy as other algorithms. This paper proposes a development that which factor is vulnerable to heart disease given basic prefix like sex, glucose, Blood pressure, Heart rate, etc. The future direction of this paper is using different devices and clinical trials for the real-life experiment.
A machine learning approach for opinion mining online customer reviews
Abstract :This study was conducted to apply supervised machine learning methods in opinion mining online customer reviews. First, the study automatically collected 39,976 traveler reviews on hotels in Vietnam on Agoda.com website, then conducted the training with machine learning models to find out which model is most compatible with the training dataset and apply this model to forecast opinions for the collected dataset. The results showed that Logistic Regression (LR), Support Vector Machines (SVM) and Neural Network (NN) methods have the best performance in opinion mining in Vietnamese language. This study is valuable as a reference for applications of opinion mining in the field of business.
Hybrid Machine Learning Classification Technique for Improve Accuracy of Heart Disease
Abstract: The area of medical science has attracted great attention from researchers. Several causes for human early mortality have been identified by a decent number of investigators. The related literature has confirmed that diseases are caused by different reasons and one such cause is heart-based sicknesses. Many researchers proposed idiosyncratic methods to preserve human life and help health care experts to recognize, prevent and manage heart disease. Some of the convenient methodologies facilitate the expert’s decision but every successful scheme has its own restrictions. The proposed approach robustly analyze an act of Hidden Markov Model (HMM), Artificial Neural Network (ANN), Support Vector Machine (SVM), and Decision Tree J48 along with the two different feature selection methods such as Correlation Based Feature Selection (CFS) and Gain Ratio. The Gain Ratio accompanies the Ranker method over a different group of statistics. After analyzing the procedure the intended method smartly builds Naive Bayes processing that utilizes the operation of two most appropriate processes with suitable layered design. Initially, the intention is to select the most appropriate method and analyzing the act of available schemes executed with different features for examining the statistics.
Novel Supervised Machine Learning Classification Technique for Improve Accuracy of Multi-Valued Datasets in Agriculture
Abstract: In the modern era, many reasons for agricultural plant disease due to unfavorable weather conditions. Many reasons that influence disease in agricultural plants include variety/hybrid genetics, the lifetime of plants at the time of infection, environment(soil, climate), weather (temperature, wind, rain, hail, etc), single versus mixed infections, and genetics of the pathogen populations. Due to these factors, diagnosis of plant diseases at the early stages can be a difficult task. Machine Learning (ML) classification techniques such as Naïve Bayes (NB) and Neural Network (NN) techniques were compared to develop a novel technique to improve the level of accuracy
Machine Learning and Deep Learning Approaches for Brain Disease Diagnosis: Principles and Recent Advances
Abstract: Brain is the controlling center of our body. With the advent of time, newer and newer brain diseases are being discovered. Thus, because of the variability of brain diseases, existing diagnosis or detection systems are becoming challenging and are still an open problem for research. Detection of brain diseases at an early stage can make a huge difference in attempting to cure them. In recent years, the use of artificial intelligence (AI) is surging through all spheres of science, and no doubt, it is revolutionizing the field of neurology. Application of AI in medical science has made brain disease prediction and detection more accurate and precise. In this study, we present a review on recent machine learning and deep learning approaches in detecting four brain diseases such as Alzheimer’s disease (AD), brain tumor, epilepsy, and Parkinson’s disease. 147 recent articles on four brain diseases are reviewed considering diverse machine learning and deep learning approaches, modalities, datasets etc. Twenty-two datasets are discussed which are used most frequently in the reviewed articles as a primary source of brain disease data. Moreover, a brief overview of different feature extraction techniques that are used in diagnosing brain diseases is provided. Finally, key findings from the reviewed articles are summarized and a number of major issues related to machine learning/deep learning-based brain disease diagnostic approaches are discussed. Through this study, we aim at finding the most accurate technique for detecting different brain diseases which can be employed for future betterment.
Prediction of Chronic Kidney Disease - A Machine Learning Perspective
Abstract: Chronic Kidney Disease is one of the most critical illness nowadays and proper diagnosis is required as soon as possible. Machine learning technique has become reliable for medical treatment. With the help of a machine learning classifier algorithms, the doctor can detect the disease on time. For this perspective, Chronic Kidney Disease prediction has been discussed in this article. Chronic Kidney Disease dataset has been taken from the UCI repository. Seven classifier algorithms have been applied in this research such as artificial neural network, C5.0, Chi-square Automatic interaction detector, logistic regression, linear support vector machine with penalty L1 & with penalty L2 and random tree. The important feature selection technique was also applied to the dataset. For each classifier, the results have been computed based on (i) full features, (ii) correlation-based feature selection, (iii) Wrapper method feature selection, (iv) Least absolute shrinkage and selection operator regression, (v) synthetic minority over-sampling technique with least absolute shrinkage and selection operator regression selected features, (vi) synthetic minority over-sampling technique with full features. From the results, it is marked that LSVM with penalty L2 is giving the highest accuracy of 98.86% in synthetic minority over-sampling technique with full features. Along with accuracy, precision, recall, F-measure, area under the curve and GINI coefficient have been computed and compared results of various algorithms have been shown in the graph. Least absolute shrinkage and selection operator regression selected features with synthetic minority over-sampling technique gave the best after synthetic minority over-sampling technique with full features. In the synthetic minority over-sampling technique with least absolute shrinkage and selection operator selected features, again linear support vector machine gave the highest accuracy of 98.46%. Along with machine learning models one deep neural network has been applied on the same dataset and it has been noted that deep neural network achieved the highest accuracy of 99.6%
Potato Disease Detection Using Machine Learning
Abstract: In Bangladesh potato is one of the major crops. Potato cultivation has been very popular in Bangladesh for the last few decades. But potato production is being hampered due to some diseases which are increasing the cost of farmers in potato production. However, some potato diseases are hampering potato production that is increasing the cost of farmers. Which is disrupting the life of the farmer. An automated and rapid disease detection process to increase potato production and digitize the system. Our main goal is to diagnose potato disease using leaf pictures that we are going to do through advanced machine learning technology. This paper offers a picture that is processing and machine learning based automated systems potato leaf diseases will be identified and classified. Image processing is the best solution for detecting and analyzing these diseases. In this analysis, picture division is done more than 2034 pictures of unhealthy potato and potato’s leaf, which is taken from openly accessible plant town information base and a few pre-prepared models are utilized for acknowledgment and characterization of sick and sound leaves. Among them, the program predicts with an accuracy of 99.23% in testing with 25% test data and 75% train data. Our output has shown that machine learning exceeds all existing tasks in potato disease detection.
A Comparative Evaluation of Traditional Machine Learning and Deep Learning Classification Techniques for Sentiment Analysis
Abstract :With the technological advancement in the field of digital transformation, the use of the internet and social media has increased immensely. Many people use these platforms to share their views, opinions and experiences. Analyzing such information is significant for any organization as it apprises the organization to understand the need of their customers. Sentiment analysis is an intelligible way to interpret the emotions from the textual information and it helps to determine whether that emotion is positive or negative. This paper outlines the data cleaning and data preparation process for sentiment analysis and presents experimental findings that demonstrates the comparative performance analysis of various classification algorithms. In this context, we have analyzed various machine learning techniques (Support Vector Machine, and Multinomial Naive Bayes) and deep learning techniques (Bidirectional Encoder Representations from Transformers, and Long Short-Term Memory) for sentiment analysis
A Comprehensive Review on Email Spam Classification using Machine Learning Algorithms
Abstract: Email is the most used source of official communication method for business purposes. The usage of the email continuously increases despite of other methods of communications. Automated management of emails is important in the today’s context as the volume of emails grows day by day. Out of the total emails, more than 55 percent is identified as spam. This shows that these spams consume email user time and resources generating no useful output. The spammers use developed and creative methods in order to fulfil their criminal activities using spam emails, Therefore, it is vital to understand different spam email classification techniques and their mechanism. This paper mainly focuses on the spam classification approached using machine learning algorithms. Furthermore, this study provides a comprehensive analysis and review of research done on different machine learning techniques and email features used in different Machine Learning approaches. Also provides future research directions and the challenges in the spam classification field that can be useful for future researchers.
Heart Disease Prediction using Hybrid machine Learning Model
Abstract: Heart disease causes a significant mortality rate around the world, and it has become a health threat for many people. Early prediction of heart disease may save many lives; detecting cardiovascular diseases like heart attacks, coronary artery diseases etc., is a critical challenge by the regular clinical data analysis. Machine learning (ML) can bring an effective solution for decision making and accurate predictions. The medical industry is showing enormous development in using machine learning techniques. In the proposed work, a novel machine learning approach is proposed to predict heart disease. The proposed study used the Cleveland heart disease dataset, and data mining techniques such as regression and classification are used. Machine learning techniques Random Forest and Decision Tree are applied. The novel technique of the machine learning model is designed. In implementation, 3 machine learning algorithms are used, they are 1. Random Forest, 2. Decision Tree and 3. Hybrid model (Hybrid of random forest and decision tree). Experimental results show an accuracy level of 88.7% through the heart disease prediction model with the hybrid model. The interface is designed to get the user’s input parameter to predict the heart disease, for which we used a hybrid model of Decision Tree and Random Forest
Heart Failure Prediction by Feature Ranking Analysis in Machine Learning
Abstract: Heart disease is one of the major cause of mortality in the world today. Prediction of cardiovascular disease is a critical challenge in the field of clinical data analysis. With the advanced development in machine learning (ML), artificial intelligence (AI) and data science has been shown to be effective in assisting in decision making and predictions from the large quantity of data produced by the healthcare industry. ML approaches has brought lot of improvements and broadens the study in medical field which recognizes patterns in the human body by using various algorithms and correlation techniques. One such reality is coronary heart disease, various studies gives impression into predicting heart disease with ML techniques. Initially ML was used to find degree of heart failure, but also used to identify significant features that affects the heart disease by using correlation techniques. There are many features/factors that lead to heart disease like age, blood pressure, sodium creatinine, ejection fraction etc. In this paper we propose a method to finding important features by applying machine learning techniques. The work is to design and develop prediction of heart disease by feature ranking machine learning. Hence ML has huge impact in saving lives and helping the doctors, widening the scope of research in actionable insights, drive complex decisions and to create innovative products for businesses to achieve key goals.
Design of face detection and recognition system to monitor students during online examinations using Machine Learning algorithms
Abstract: Today’s pandemic situation has transformed the way of educating a student. Education is undertaken remotely through online platforms. In addition to the way the online course contents and online teaching, it has also changed the way of assessments. In online education, monitoring the attendance of the students is very important as the presence of students is part of a good assessment for teaching and learning. Educational institutions have adopting online examination portals for the assessments of the students. These portals make use of face recognition techniques to monitor the activities of the students and identify the malpractice done by them. This is done by capturing the students’ activities through a web camera and analyzing their gestures and postures. Image processing algorithms are widely used in the literature to perform face recognition. Despite the progress made to improve the performance of face detection systems, there are issues such as variations in human facial appearance like varying lighting condition, noise in face images, scale, pose etc., that blocks the progress to reach human level accuracy. The aim of this study is to increase the accuracy of the existing face recognition systems by making use of SVM and Eigenface algorithms. In this project, an approach similar to Eigenface is used for extracting facial features through facial vectors and the datasets are trained using Support Vector Machine (SVM) algorithm to perform face classification and detection. This ensures that the face recognition can be faster and be used for online exam monitoring.
IEEE DATA SCIENCE PROJECTS (2024-2025)
1. | IEEE : Deep Air Learning: Interpolation, Prediction, and Feature Analysis of Fine-grained Air Quality | |||
2. | IEEE : Classification Of A Bank Data Set On Various Data Mining Platforms Bir Banka Müşteri Verilerinin Farklı Veri Madenciliği Platformlarında Sınıflandırılması | |||
3. | IEEE : A Data Mining based Model for Detection of Fraudulent Behaviour in Water Consumption | |||
4. | IEEE : Collaborative Filtering Algorithm Based on Rating Difference and User Interest | |||
5. | IEEE : A Framework for Real-Time Spam Detection in Twitter | |||
6. | IEEE : Serendipitous Recommendation in E-Commerce Using Innovator-Based Collaborative Filtering | |||
7. | IEEE : Review Spam Detection using Machine Learning | |||
8. | IEEE : NetSpam: a Network-based Spam Detection Framework for Reviews in Online Social Media | |||
9. | IEEE : SociRank: Identifying and Ranking Prevalent News Topics Using Social Media Factors |
DHS Informatics believes in students’ stratification, we first brief the students about the technologies and type of Data Science projects and other domain projects. After complete concept explanation of the IEEE Data Science projects, students are allowed to choose more than one IEEE Data Science projects for functionality details. Even students can pick one project topic from Data Science and another two from other domains like Data Science,Data mining, image process, information forensic, big data, Data Mining, block chain etc. DHS Informatics is a pioneer institute in Bangalore / Bengaluru; we are supporting project works for other institute all over India. We are the leading final year project centre in Bangalore / Bengaluru and having office in five different main locations Jayanagar, Yelahanka, Vijayanagar, RT Nagar & Indiranagar.
We allow the ECE, CSE, ISE final year students to use the lab and assist them in project development work; even we encourage students to get their own idea to develop their final year projects for their college submission.
DHS Informatics first train students on project related topics then students are entering into practical sessions. We have well equipped lab set-up, experienced faculties those who are working in our client projects and friendly student coordinator to assist the students in their college project works.
We appreciated by students for our Latest IEEE projects & concepts on final year Data Mining projects for ECE, CSE, and ISE departments.
Latest IEEE 2024-2025 projects on Data Mining with real time concepts which are implemented using Java, MATLAB, and NS2 with innovative ideas. Final year students of computer Data Mining, computer science, information science, electronics and communication can contact our corporate office located at Jayanagar, Bangalore for Data Science project details.
DATA SCIENCE
Data Science is mining knowledge from data, Involving methods at the intersection of machine learning, statistics, and database systems. Its the powerful new technology with great potential to help companies focus on the most important information in their data warehouses. We have the best in class infrastructure, lab set up , Training facilities, And experienced research and development team for both educational and corporate sectors.
Data Science is the process of searching huge amount of data from different aspects and summarize it to useful information. Data Science is logical than physical subset. Our concerns usually implicate mining and text based classification on Data Science projects for Students.
The usages of variety of tools associated to data analysis for identifying relationships in data are the process for Data Science. Our concern support data mining projects for IT and CSE students to carry out their academic research projects.
Data Science is the process of searching huge amount of data from different aspects and summarize it to useful information. Data Science is logical than physical subset. Our concerns usually implicate mining and text based classification on data Science projects for Students. The usages of variety of tools associated to data analysis for identifying relationships in data are the process for data Science. Our concern support data Science projects for IT and CSE students to carry out their academic research projects.
Relational Statics
The popularity of the term “data science” has exploded in business environments and academia, as indicated by a jump in job openings. However, many critical academics and journalists see no distinction between data science and statistics. Writing in Forbes, Gil Press argues that data science is a buzzword without a clear definition and has simply replaced “business analytics” in contexts such as graduate degree programs.In the question-and-answer section of his keynote address at the Joint Statistical Meetings of American Statistical Association, noted applied statistician Nate Silver said, “I think data-scientist is a sexed up term for a statistician….Statistics is a branch of science. Data scientist is slightly redundant in some way and people shouldn’t berate the term statistician.”Similarly, in business sector, multiple researchers and analysts state that data scientists alone are far from being sufficient in granting companies a real competitive advantage and consider data scientists as only one of the four greater job families companies require to leverage big data effectively, namely: data analysts, data scientists, big data developers and big data engineers.
On the other hand, responses to criticism are as numerous. In a 2014 Wall Street Journal article, Irving Wladawsky-Berger compares the data science enthusiasm with the dawn of computer science. He argues data science, like any other interdisciplinary field, employs methodologies and practices from across the academia and industry, but then it will morph them into a new discipline. He brings to attention the sharp criticisms computer science, now a well respected academic discipline, had to once face.Likewise, NYU Stern’s Vasant Dhar, as do many other academic proponents of data science,argues more specifically in December 2013 that data science is different from the existing practice of data analysis across all disciplines, which focuses only on explaining data sets. Data science seeks actionable and consistent pattern for predictive uses.This practical engineering goal takes data science beyond traditional analytics. Now the data in those disciplines and applied fields that lacked solid theories, like health science and social science, could be sought and utilized to generate powerful predictive models.
Java Final year CSE projects in Bangalore
- Java Information Forensic / Block Chain B.E Projects
- Java Cloud Computing B.E Projects
- Java Big Data with Hadoop B.E Projects
- Java Networking & Network Security B.E Pr ojects
- Java Data Mining / Web Mining / Cyber Secu rity B.E Projects
- Java DataScience / Machine Learning B.E Projects
- Java Artificaial Inteligence B.E Projects
- Java Wireless Sensor Network B.E Projects
- Java Distributed & Parallel Networking B.E Projects
- Java Mobile Computing B.E Projects
Android Final year CSE projects in Bangalore
- Android GPS, GSM, Bluetooth & GPRS B.E Projects
- Android Embedded System Application Projetcs for B.E
- Android Database Applications Projects for B.E Students
- Android Cloud Computing Projects for Final Year B.E Students
- Android Surveillance Applications B.E Projects
- Android Medical Applications Projects for B.E
Embedded Final year CSE projects in Bangalore
- Embedded Robotics Projects for M.tech Final Year Students
- Embedded IEEE Internet of Things Projects for B.E Students
- Embedded Raspberry PI Projects for B.E Final Year Students
- Embedded Automotive Projects for Final Year B.E Students
- Embedded Biomedical Projects for B.E Final Year Students
- Embedded Biometric Projects for B.E Final Year Students
- Embedded Security Projects for B.E Final Year
MatLab Final year CSE projects in Bangalore
- Matlab Image Processing Projects for B.E Students
- MatLab Wireless Communication B.E Projects
- MatLab Communication Systems B.E Projects
- MatLab Power Electronics Projects for B.E Students
- MatLab Signal Processing Projects for B.E
- MatLab Geo Science & Remote Sensors B.E Projects
- MatLab Biomedical Projects for B.E Students
DATA SCIENCE IEEE PAPERS AND PROJECTS-2020
Data science is an inter-disciplinary field that uses scientific methods, processes, algorithms and systems to extract knowledge and insights from structured and unstructured data. Data science is related to data mining and big data.
FREE IEEE PAPER AND PROJECTS
Ieee projects 2022, seminar reports, free ieee projects ieee papers.
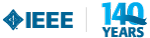
is Mainsite
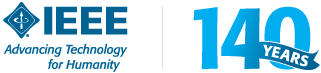
- Search all IEEE websites
- Mission and vision
- IEEE at a glance
- IEEE Strategic Plan
- Organization of IEEE
- Diversity, Equity, & Inclusion
- Organizational Ethics
- Annual Report
- History of IEEE
- Volunteer resources
- IEEE Corporate Awards Program
- Financials and Statistics
- IEEE Future Directions
- IEEE for Industry (Corporations, Government, Individuals)
IEEE Climate Change
- Humanitarian and Philanthropic Opportunities
- Select an option
- Get the latest news
- Access volunteer resources (Code of Ethics, financial forms, tools and templates, and more)
- Find IEEE locations
- Get help from the IEEE Support Center
- Recover your IEEE Account username and password
- Learn about the IEEE Awards program and submit nomination
- View IEEE's organizational structure and leadership
- Apply for jobs at IEEE
- See the history of IEEE
- Learn more about Diversity, Equity & Inclusion at IEEE
- Join an IEEE Society
- Renew your membership
- Member benefits
- IEEE Contact Center
- Connect locally
- Memberships and Subscriptions Catalog
- Member insurance and discounts
- Member Grade Elevation
- Get your company engaged
- Access your Account
- Learn about membership dues
- Learn about Women in Engineering (WIE)
- Access IEEE member email
- Find information on IEEE Fellows
- Access the IEEE member directory
- Learn about the Member-Get-a-Member program
- Learn about IEEE Potentials magazine
- Learn about Student membership
- Affinity groups
- IEEE Societies
- Technical Councils
- Technical Communities
- Geographic Activities
- Working groups
- IEEE Regions
- IEEE Collabratec®
- IEEE Resource Centers
IEEE DataPort
- See the IEEE Regions
- View the MGA Operations Manual
- Find information on IEEE Technical Activities
- Get IEEE Chapter resources
- Find IEEE Sections, Chapters, Student Branches, and other communities
- Learn how to create an IEEE Student Chapter
- Upcoming conferences
- IEEE Meetings, Conferences & Events (MCE)
- IEEE Conference Application
- IEEE Conference Organizer Education Program
- See benefits of authoring a conference paper
- Search for 2025 conferences
- Search for 2024 conferences
- Find conference organizer resources
- Register a conference
- Publish conference papers
- Manage conference finances
- Learn about IEEE Meetings, Conferences & Events (MCE)
- Visit the IEEE SA site
- Become a member of the IEEE SA
- Find information on the IEEE Registration Authority
- Obtain a MAC, OUI, or Ethernet address
- Access the IEEE 802.11™ WLAN standard
- Purchase standards
- Get free select IEEE standards
- Purchase standards subscriptions on IEEE Xplore®
- Get involved with standards development
- Find a working group
- Find information on IEEE 802.11™
- Access the National Electrical Safety Code® (NESC®)
- Find MAC, OUI, and Ethernet addresses from Registration Authority (regauth)
- Get free IEEE standards
- Learn more about the IEEE Standards Association
- View Software and Systems Engineering Standards
- IEEE Xplore® Digital Library
- Subscription options
- IEEE Spectrum
- The Institute
Proceedings of the IEEE
- IEEE Access®
- Author resources
- Get an IEEE Xplore Digital Library trial for IEEE members
- Review impact factors of IEEE journals
- Request access to the IEEE Thesaurus and Taxonomy
- Access the IEEE copyright form
- Find article templates in Word and LaTeX formats
- Get author education resources
- Visit the IEEE Xplore digital library
- Find Author Digital Tools for IEEE paper submission
- Review the IEEE plagiarism policy
- Get information about all stages of publishing with IEEE
- IEEE Learning Network (ILN)
- IEEE Credentialing Program
- Pre-university
- IEEE-Eta Kappa Nu
- Accreditation
- Access continuing education courses on the IEEE Learning Network
- Find STEM education resources on TryEngineering.org
- Learn about the TryEngineering Summer Institute for high school students
- Explore university education program resources
- Access pre-university STEM education resources
- Learn about IEEE certificates and how to offer them
- Find information about the IEEE-Eta Kappa Nu honor society
- Learn about resources for final-year engineering projects
- Access career resources
Publications
Ieee provides a wide range of quality publications that make the exchange of technical knowledge and information possible among technology professionals..
Expand All | Collapse All
- > Get an IEEE Xplore Digital Library trial for IEEE members
- > Review impact factors of IEEE journals
- > Access the IEEE thesaurus and taxonomy
- > Find article templates in Word and LaTeX formats
- > Get author education resources
- > Visit the IEEE Xplore Digital Library
- > Learn more about IEEE author tools
- > Review the IEEE plagiarism policy
- > Get information about all stages of publishing with IEEE

Why choose IEEE publications?
IEEE publishes the leading journals, transactions, letters, and magazines in electrical engineering, computing, biotechnology, telecommunications, power and energy, and dozens of other technologies.
In addition, IEEE publishes more than 1,800 leading-edge conference proceedings every year, which are recognized by academia and industry worldwide as the most vital collection of consolidated published papers in electrical engineering, computer science, and related fields.
Spotlight on IEEE publications
Ieee xplore ®.
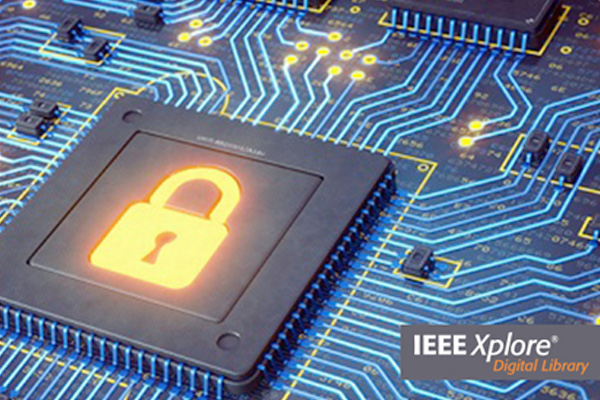
- About IEEE Xplore
- Visit the IEEE Xplore Digital Library
- See how to purchase articles and standards
- Find support and training
- Browse popular content
- Sign up for a free trial
IEEE Spectrum Magazine
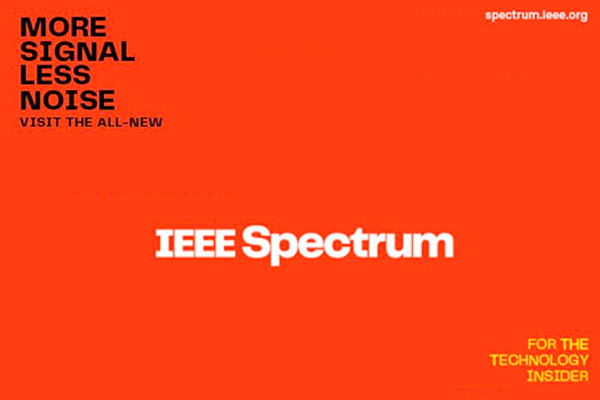
- Visit the IEEE Spectrum website
- Visit the Institute for IEEE member news
IEEE Access
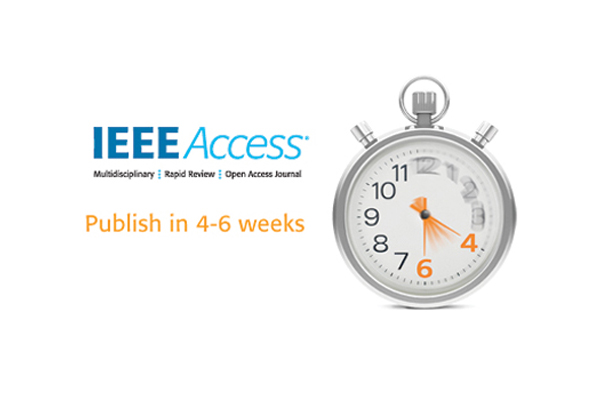
- Visit IEEE Access
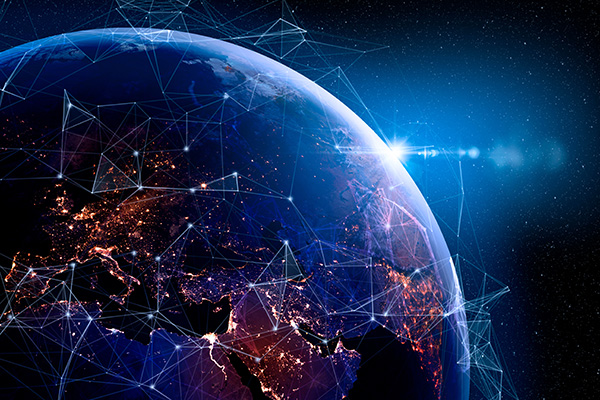
- See recent issues
Benefits of publishing
Authors: why publish with ieee.
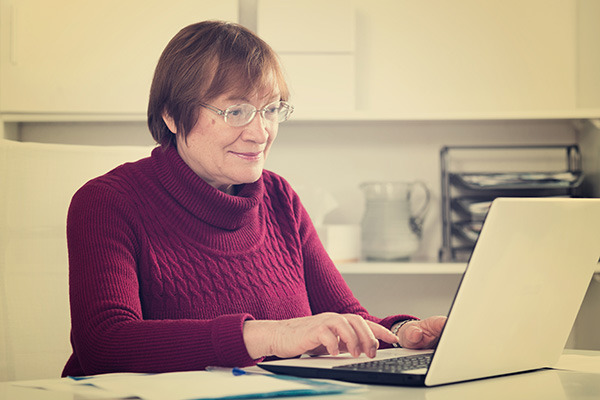
- PSPB Accomplishments in 2023 (PDF, 228 KB)
- IEEE statement of support for Open Science
- IEEE signs San Francisco Declaration on Research Assessment (DORA)
- Read about how IEEE journals maintain top citation rankings
Open Access Solutions
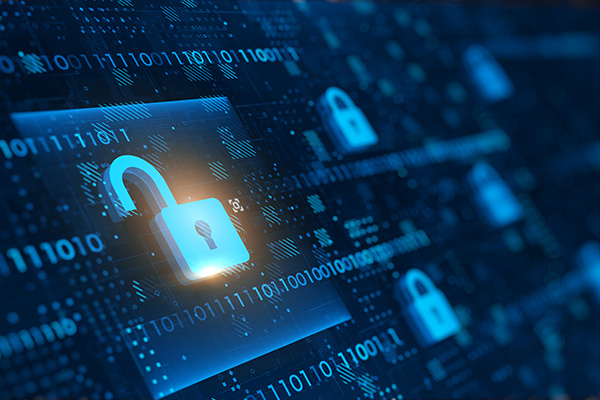
- Visit IEEE Open
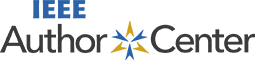
Visit the IEEE Author Center
Find author resources >
- > IEEE Collabratec ®
- > Choosing a journal
- > Writing
- > Author Tools
- > How to Publish with IEEE (English) (PPT, 3 MB)
- > How to Publish with IEEE (Chinese) (PPT, 3 MB)
- > Benefits of Publishing with IEEE (PPT, 7 MB)
- > View author tutorial videos
- Read the IEEE statement on appropriate use of bibliometric indicators
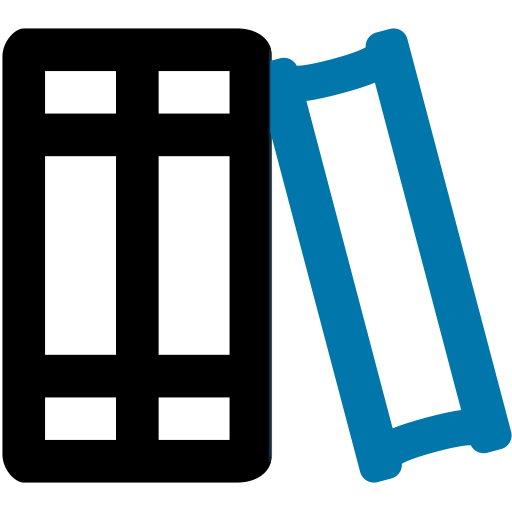
Publication types and subscription options
- Journal and magazine subscriptions
- Digital library subscriptions
- Buy individual articles from IEEE Xplore
For organizations:
- Browse IEEE subscriptions
- Get institutional access
- Subscribe through your local IEEE account manager
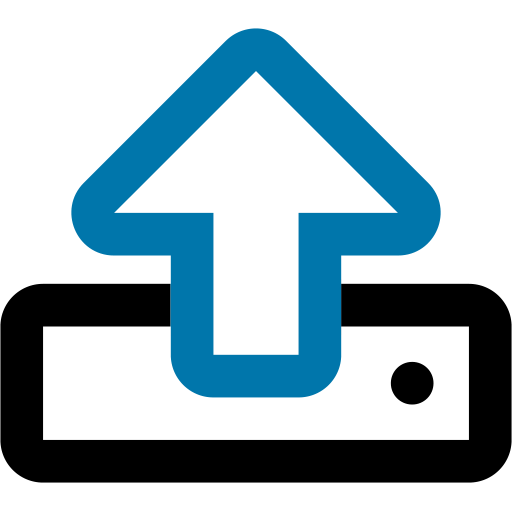
Publishing information
IEEE publishing makes the exchange of technical knowledge possible with the highest quality and the greatest impact.
- Open access publishing options
- Intellectual Property Rights (IPR)
- Reprints of articles
- Services for IEEE organizations
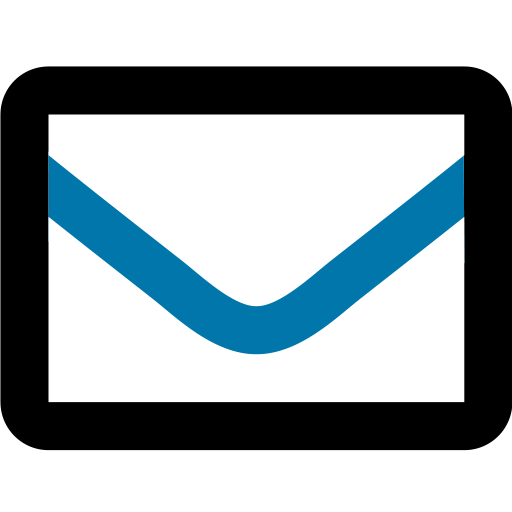
Contact information
- Contact IEEE Publications
- About the Publication Services & Products Board
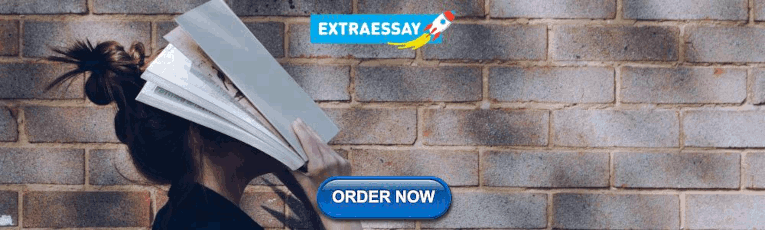
Related Information >
Network. collaborate. create with ieee collabratec®..
All within one central hub—with exclusive features for IEEE members.
- Experience IEEE Collabratec
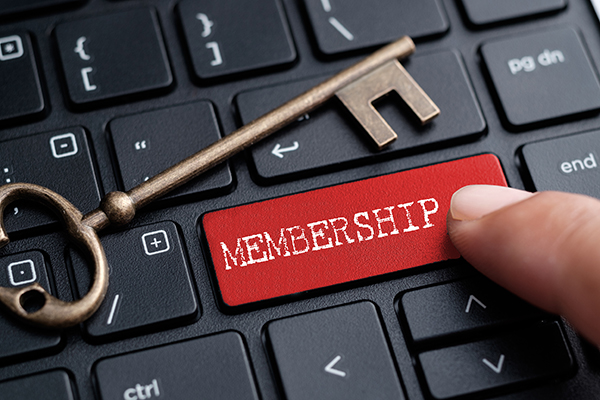
Join/Renew IEEE or a Society
Receive member access to select content, product discounts, and more.
- Review all member benefits
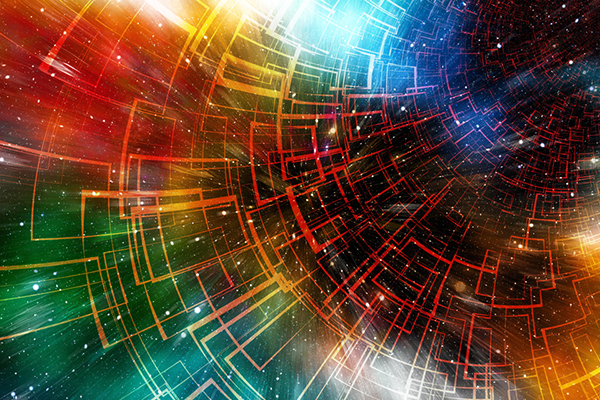
Try this easy-to-use, globally accessible data repository that provides significant benefits to researchers, data analysts, and the global technical community.
- Start learning today
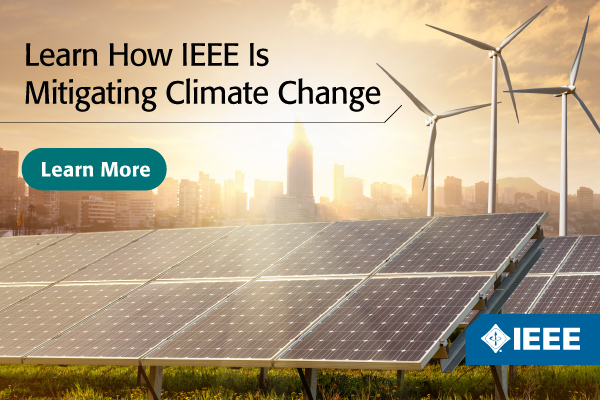
IEEE is committed to helping combat and mitigate the effects of climate change.
- See what's new on the IEEE Climate Change site
- DOI: 10.1109/SiPhotonics60897.2024.10543636
- Corpus ID: 270397456
Data Throughput for Efficient Photonic Neural Network Accelerators
- R. L. T. Schwartz , B. Jahannia , +2 authors V. Sorger
- Published in IEEE Silicon Photonics… 15 April 2024
- Engineering, Computer Science, Physics
- 2024 IEEE Silicon Photonics Conference (SiPhotonics)
Figures from this paper
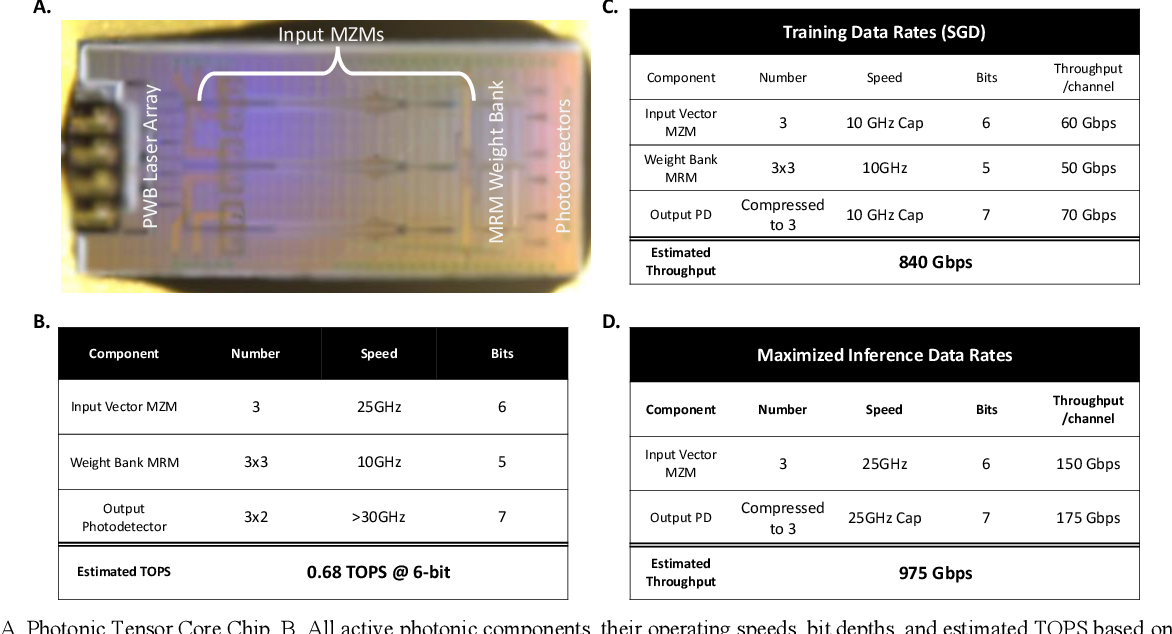
6 References
Integrated photonic tensor processing unit for a matrix multiply: a review, photonics for artificial intelligence and neuromorphic computing, high-density integrated photonic tensor processing unit with a matrix multiply compile, high density photonic tensor core for matrix-vector multiplication, related papers.
Showing 1 through 3 of 0 Related Papers
SF IEEE STB20132
- Core Members
Data Science
As we all know that, when the world has entered the era of big data, the question raised was “Where to store this huge amount of data”. It was the concern and big challenge to the enterprise and many other industries until 2010. The only solution to this problem was to build frameworks that can store this data. Then Hadoop and other frameworks came into the picture and so the problem of storing large data was solved. Now the problem was how to process this large amount of data. Data Science is the secret sauce here.
Therefore it is important to understand what Data Science is and why people are crazy after Data Science.
Data Science in simple words is the study of data. Data Science is the process through which you can convert raw data into knowledge to support decision making. It involves developing methods of storing and analyzing data effectively. Then through this data, you
can extract useful information from scientific methods, processes, algorithms, and systems. The main goal of data science is to gain insights and knowledge data that can be both structured and unstructured.
Why Data Science?
➢ Industries require accurate data to help them and make careful decisions.
➢ Data Science churns raw data into meaningful insights and provides it to
organizations.
➢ Companies use data or meaningful insights to analyze their marketing strategies and make better advertisements.
➢ Improves business, society or performance by gaining knowledge from data.
➢ Take real-time decisions.
➢ Make each dollar count and increase the return of investment.
➢ Builds confidence in business decisions.
Life Cycle of Data Science
Phase 1: Define Problem
Data Scientists do not start with the Data. They start with the problem. You should well- defined problem which contains its solution within it and it makes the problem easier to solve. If you define a problem effectively then it will help you in saving your time and your resources.
Phase 2: Collect Data
Data Collection is the process of gathering information on variables which are data requirements. You have to emphasis on ensuring accurate and honest collection of data such that the related decisions are valid. You should gather and scrap the data which is necessary for your project.
Phase 3: Data Cleaning
The data collected may be incomplete and may contain errors. The duplicate values should be discarded from the data. For data cleaning, you should fix the inconsistencies within the data and handle the missing values. Data cleaning helps you in making the data effective and accurate.
Phase 4: Analyze Data
You can analyze data through various techniques. The analyzed data will help you to understand and interpret the data efficiently. Through analyzed data, you can derive conclusions that will be required in further phases. The best way to analyze data is through data visualization which can be in the graphical or in chart format. Statistical data models can also be used which includes regression analysis and correlation. It includes methods like logistic regression, decision trees, random forest, and neural networks.
Phase 5: Interpret Result
The results which came through analyzing data are to be reported in a particular format as required by the industry person. The interpreted results can be in the form of data visualization through charts and graphs. Through these charts and graphs, you can get insights and will help you in marketing strategies.
What skills need to be a DATA SCIENTIST?
➢ Strong knowledge of Python, SAS, R.
➢ Hands-on experience in SQL database coding.
➢ Knowledge of machine learning.
➢ Ability to work with unstructured data from various sources like video and social media.
➢ Understand multiple analytical functions.
Data Scientist is a person who is a part analyst who makes the use of his technical and analytical abilities to extract meaning and insights from massive data sets.
Data Scientist helps in increasing data accuracy, reducing costs and developing strategies.
Python VS R for Data Science
Python is great for Machine Learning and Deep Learning. R is good for statistical analysis of data.
The fact is that learning both tools Python and R, and using them for their respective strengths can only improve you as a data scientist. Versatility and flexibility are traits of a data scientist in their field. The python vs. R debate makes you stick to one programming language. You should look beyond it and learn both tools for their respective strengths. Using more tools will only make you better as a data scientist.
Top Application Areas
➢ Digital advertisements
➢ Internet Research
➢ Real-Time Predictive Analytics
➢ Recommendation Engines
➢ Cyber Security
In the end, it won’t be wrong to say that the future belongs to the Data Scientists. It was predicted that there will be a need of around one million Data Scientists. Working on more and more data will provide you with opportunities to solve problems and make accurate decisions. And hence Data Science will fulfill all your dreams once you become a successful data scientist.
Written By: Mansi Mahajan
IEEE Member No: 96171462

50+ IEEE Projects For CSE [Updated 2024]
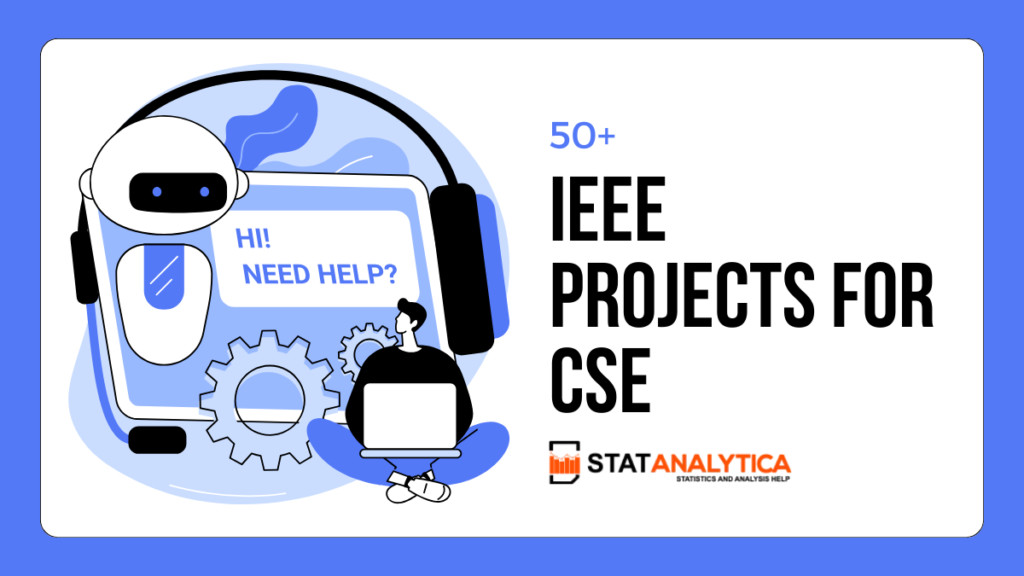
In the dynamic realm of Computer Science Engineering (CSE), staying updated about the latest developments is essential for students to thrive in their academic and professional journeys. One key avenue for this exploration is engaging in IEEE (Institute of Electrical and Electronics Engineers) projects tailored for CSE students. This blog aims to provide a comprehensive guide on IEEE projects for CSE, helping students understand the importance of choosing the right projects and navigating the complexities of implementation.
What are IEEE Standards?
Table of Contents
IEEE, as a globally recognized authority in technology standards, plays a pivotal role in shaping the landscape of CSE projects. Its standards not only ensure the quality of projects but also contribute to the seamless integration of technological advancements.
By adhering to IEEE standards, CSE students can enhance the credibility and reliability of their projects, making them valuable assets in the academic and professional spheres.
How to Select the Right IEEE Project?
Selecting the right IEEE project is a critical step in a student’s academic and professional journey. Here’s a step-by-step guide to help you navigate this process:
- Identify Your Interests and Strengths:
- Consider your passions within the vast field of Computer Science.
- Assess your skills and strengths to determine areas where you excel.
- Stay Updated on Industry Trends:
- Keep abreast of current trends and emerging technologies in Computer Science Engineering.
- Choose a project that aligns with the latest advancements in the industry.
- Evaluate Project Relevance:
- Assess the practicality and relevance of potential projects in real-world scenarios.
- Opt for projects that address current challenges or contribute to industry needs.
- Understand Project Scope and Complexity:
- Gauge the complexity of projects and ensure it aligns with your skill level.
- Consider the time and resources required to complete the project successfully.
- Explore IEEE Project Databases:
Utilize IEEE databases and resources to explore a variety of project options.
Narrow down projects that match your interests and align with your academic goals.
- Consult with Mentors and Peers:
- Seek guidance from professors, mentors, or peers who can provide valuable insights.
- Discuss your interests and goals to receive recommendations tailored to your profile.
- Consider Personal and Academic Goals:
- Align the chosen project with your academic objectives and career aspirations.
- Ensure the project contributes to your skill development and overall growth.
- Evaluate Resource Availability:
- Assess the availability of resources, including hardware, software, and expertise.
- Choose a project that can be feasibly implemented with the resources at your disposal.
- Assess Project Impact:
- Consider the potential impact of the project on your academic and professional portfolio.
- Choose projects that showcase your abilities and contribute meaningfully to your field.
- Plan for Continuous Learning:
- Opt for projects that offer opportunities for continuous learning and skill enhancement.
- Embrace challenges that push you to expand your knowledge and capabilities.
Remember, the right IEEE project for CSE students should align with their interests, match their skill level, contribute to their academic and career goals, and be feasible within the available resources. By following these steps, you can make an informed decision and embark on a rewarding project journey.
50+ IEEE Projects for CSE [Category Wise]
Machine learning and ai projects.
- Image Recognition using Convolutional Neural Networks (CNN)
- Natural Language Processing (NLP) for Sentiment Analysis
- Predictive Analytics for Stock Market Trends
- Autonomous Vehicle Navigation using Reinforcement Learning
Data Science and Big Data Projects
- Predictive Analytics for Disease Outbreaks
- Fraud Detection in Financial Transactions
- Social Media Analytics for User Behavior Prediction
- Large-scale Data Processing with Hadoop and Spark
Cyber Security Projects
- Intrusion Detection System using Machine Learning
- Blockchain-Based Secure Healthcare Records
- Biometric Authentication Systems
- Network Security Monitoring and Analysis
Internet of Things (IoT) Projects
- Smart Home Automation System
- Industrial IoT for Predictive Maintenance
- Healthcare Monitoring using IoT Devices
- Energy Management in Smart Cities
Cloud Computing Projects
- Cloud-Based E-Learning System
- Resource Allocation in Cloud Computing
- Cloud Security and Encryption
- IoT Integration with Cloud Services
Blockchain Projects
- Supply Chain Transparency using Blockchain
- Decentralized Voting System
- Blockchain-Based Identity Management
- Smart Contracts for Legal Processes
Mobile App Development Projects
- Health and Fitness Tracking App
- Augmented Reality (AR) Gaming Application
- Location-Based Services for Tourism
- Secure Messaging App with End-to-End Encryption
Computer Vision Projects
- Human Activity Recognition using Computer Vision
- Object Detection and Tracking in Video Streams
- Facial Recognition for Access Control
- Augmented Reality Applications
Web Development Projects
- Content Recommendation System for Websites
- E-Commerce Platform with Personalized Shopping
- Online Learning Management System
- Social Networking Platform with Advanced Features
Networking Projects
- Software-Defined Networking (SDN) for Improved Network Management
- Quality of Service (QoS) in Wireless Networks
- IoT Communication Protocols
- Network Function Virtualization (NFV) Implementation
Edge Computing Projects
- Real-time Video Analytics at the Edge
- Edge-based Health Monitoring for Remote Areas
- Intelligent Traffic Management using Edge Devices
- Edge Computing for IoT Security
Biomedical Engineering Projects
- Wearable Devices for Continuous Health Monitoring
- Computer-Aided Diagnosis System for Medical Images
- Brain-Computer Interface for Assistive Technology
- Predictive Modeling for Disease Outbreaks in Healthcare
Human-Computer Interaction (HCI) Projects
- Gesture Recognition System for Human-Computer Interaction
- Voice User Interface (VUI) for Smart Assistants
- Augmented Reality (AR) for Enhancing User Experience
- Accessibility Features for Software Applications
Methodology for Implementing IEEE Projects
Implementing IEEE projects in Computer Science Engineering involves a systematic methodology to ensure successful execution. Below is a step-by-step guide that outlines the key phases and considerations in the implementation process:
- Project Selection and Definition:
- Define Clear Objectives: Clearly outline the goals and objectives of the project.
- Choose a Methodology: Select a development methodology (e.g., Waterfall, Agile) based on the project’s nature.
- Literature Review and Research:
- Review Existing Work: Explore relevant literature and existing projects in the chosen domain.
- Identify Gaps and Challenges: Determine gaps in current research and challenges that the project aims to address.
- Requirement Analysis:
- Define User Requirements: Gather and document user requirements comprehensively.
- Create a Functional Specification: Develop a detailed specification document outlining the system’s functionalities.
- Design Phase:
- Architectural Design: Create a high-level architecture and design the system’s structure.
- Detailed Design: Develop detailed designs for each module or component of the project.
- Development:
- Coding: Write code according to the design specifications.
- Use Version Control: Implement version control systems (e.g., Git) to manage code changes.
- Unit Testing: Test individual components to ensure they function as intended.
- Integration Testing: Verify that components work seamlessly together.
- System Testing: Evaluate the system as a whole against defined requirements.
- Documentation:
- Technical Documentation: Create detailed documentation for code, algorithms, and system architecture.
- User Documentation: Develop user manuals and guides for easy system understanding.
- Deployment:
- Prepare for Deployment: Ensure all dependencies are met and system requirements are fulfilled.
- Deploy in Staging Environment: Test the project in a controlled environment before deployment to production.
- Evaluation and Validation:
- User Acceptance Testing (UAT): Have end-users validate the system against their requirements.
- Performance Testing: Evaluate the system’s performance under various conditions.
- Feedback and Iteration:
- Gather Feedback: Collect feedback from users, stakeholders, and testing teams.
- Iterate and Improve: Implement necessary changes based on feedback to enhance the project.
- Final Documentation and Presentation:
- Compile Final Documentation: Update documentation to reflect the final state of the project.
- Prepare for Presentation: Create presentations summarizing the project’s objectives, methodology, and outcomes.
- Knowledge Transfer and Maintenance:
- Knowledge Sharing: Conduct knowledge transfer sessions to share insights with team members or successors.
- Maintenance Plan: Develop a plan for ongoing maintenance and updates, if necessary.
- Publication and Dissemination (Optional):
- Prepare Research Papers: If applicable, document the research findings for publication.
- Present at Conferences: Share project outcomes at relevant conferences or forums.
- Reflect and Learn:
- Post-Implementation Review: Conduct a post-implementation review to identify lessons learned.
- Reflect on Challenges: Assess challenges faced during implementation for future improvement.
By following this comprehensive methodology, you can streamline the implementation process of IEEE projects, ensuring a structured and successful outcome.
Each phase is crucial, and attention to detail in planning, development, testing, and documentation is key to the project’s overall success.
Challenges and Solutions
Embarking on an IEEE project journey is not without its challenges. This section identifies common obstacles that students may encounter during the execution of their projects and offers strategies to overcome them.
Real-life examples of successful project execution serve as inspirations, demonstrating that challenges can be surmounted with perseverance, creativity, and strategic problem-solving.
In conclusion, navigating the world of IEEE projects for CSE offers students a pathway to not only enhance their academic knowledge but also to contribute meaningfully to the ever-evolving field of technology.
By understanding IEEE standards, choosing the right projects, overcoming challenges, and embracing the benefits, students can position themselves as leaders in the dynamic and exciting realm of Computer Science Engineering.
The future holds limitless possibilities, and IEEE projects serve as a gateway to unlocking the potential of aspiring CSE professionals.
Related Posts
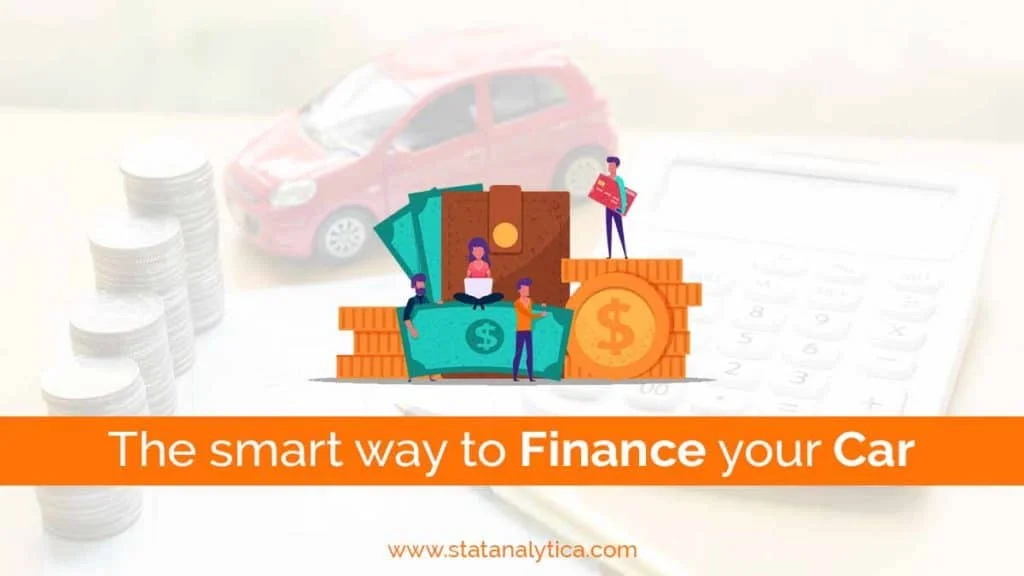
Step by Step Guide on The Best Way to Finance Car
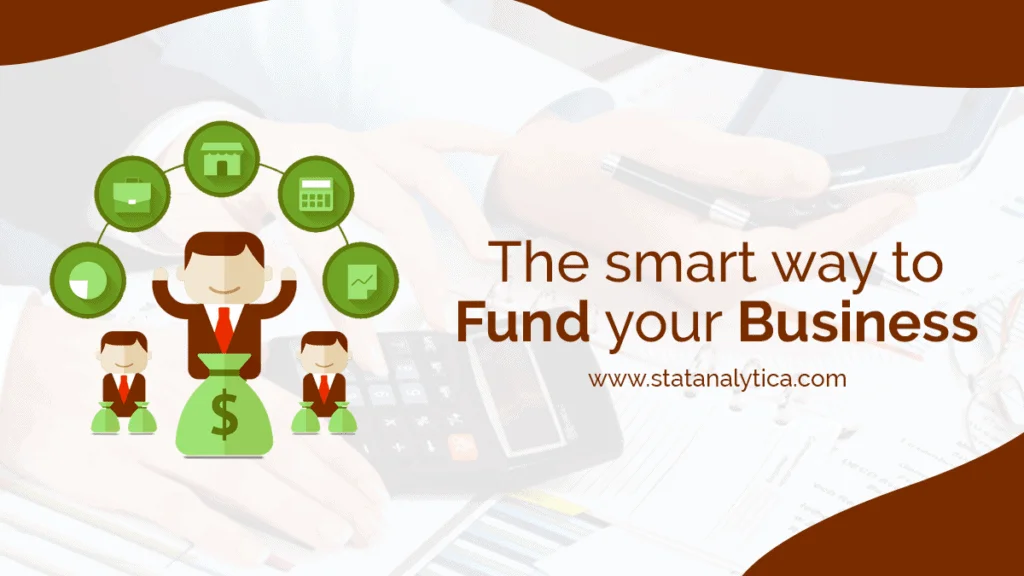
The Best Way on How to Get Fund For Business to Grow it Efficiently
Thank you for visiting nature.com. You are using a browser version with limited support for CSS. To obtain the best experience, we recommend you use a more up to date browser (or turn off compatibility mode in Internet Explorer). In the meantime, to ensure continued support, we are displaying the site without styles and JavaScript.
- View all journals
- Explore content
- About the journal
- Publish with us
- Sign up for alerts
- Open access
- Published: 19 June 2024
Detecting hallucinations in large language models using semantic entropy
- Sebastian Farquhar ORCID: orcid.org/0000-0002-9185-6415 1 na1 ,
- Jannik Kossen 1 na1 ,
- Lorenz Kuhn 1 na1 &
- Yarin Gal ORCID: orcid.org/0000-0002-2733-2078 1
Nature volume 630 , pages 625–630 ( 2024 ) Cite this article
72k Accesses
1 Citations
1459 Altmetric
Metrics details
- Computer science
- Information technology
Large language model (LLM) systems, such as ChatGPT 1 or Gemini 2 , can show impressive reasoning and question-answering capabilities but often ‘hallucinate’ false outputs and unsubstantiated answers 3 , 4 . Answering unreliably or without the necessary information prevents adoption in diverse fields, with problems including fabrication of legal precedents 5 or untrue facts in news articles 6 and even posing a risk to human life in medical domains such as radiology 7 . Encouraging truthfulness through supervision or reinforcement has been only partially successful 8 . Researchers need a general method for detecting hallucinations in LLMs that works even with new and unseen questions to which humans might not know the answer. Here we develop new methods grounded in statistics, proposing entropy-based uncertainty estimators for LLMs to detect a subset of hallucinations—confabulations—which are arbitrary and incorrect generations. Our method addresses the fact that one idea can be expressed in many ways by computing uncertainty at the level of meaning rather than specific sequences of words. Our method works across datasets and tasks without a priori knowledge of the task, requires no task-specific data and robustly generalizes to new tasks not seen before. By detecting when a prompt is likely to produce a confabulation, our method helps users understand when they must take extra care with LLMs and opens up new possibilities for using LLMs that are otherwise prevented by their unreliability.
Similar content being viewed by others
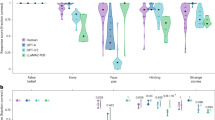
Testing theory of mind in large language models and humans
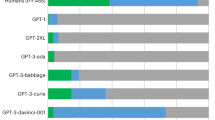
Human-like intuitive behavior and reasoning biases emerged in large language models but disappeared in ChatGPT
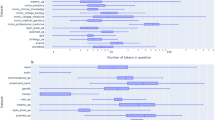
ThoughtSource: A central hub for large language model reasoning data
‘Hallucinations’ are a critical problem 9 for natural language generation systems using large language models (LLMs), such as ChatGPT 1 or Gemini 2 , because users cannot trust that any given output is correct.
Hallucinations are often defined as LLMs generating “content that is nonsensical or unfaithful to the provided source content” 9 , 10 , 11 but they have come to include a vast array of failures of faithfulness and factuality. We focus on a subset of hallucinations which we call ‘confabulations’ 12 for which LLMs fluently make claims that are both wrong and arbitrary—by which we mean that the answer is sensitive to irrelevant details such as random seed. For example, when asked a medical question “What is the target of Sotorasib?” an LLM confabulates by sometimes answering KRASG12 ‘C’ (correct) and other times KRASG12 ‘D’ (incorrect) despite identical instructions. We distinguish this from cases in which a similar ‘symptom’ is caused by the following different mechanisms: when LLMs are consistently wrong as a result of being trained on erroneous data such as common misconceptions 13 ; when the LLM ‘lies’ in pursuit of a reward 14 ; or systematic failures of reasoning or generalization. We believe that combining these distinct mechanisms in the broad category hallucination is unhelpful. Our method makes progress on a portion of the problem of providing scalable oversight 15 by detecting confabulations that people might otherwise find plausible. However, it does not guarantee factuality because it does not help when LLM outputs are systematically bad. Nevertheless, we significantly improve question-answering accuracy for state-of-the-art LLMs, revealing that confabulations are a great source of error at present.
We show how to detect confabulations by developing a quantitative measure of when an input is likely to cause an LLM to generate arbitrary and ungrounded answers. Detecting confabulations allows systems built on LLMs to avoid answering questions likely to cause confabulations, to make users aware of the unreliability of answers to a question or to supplement the LLM with more grounded search or retrieval. This is essential for the critical emerging field of free-form generation in which naive approaches, suited to closed vocabulary and multiple choice, fail. Past work on uncertainty for LLMs has focused on simpler settings, such as classifiers 16 , 17 and regressors 18 , 19 , whereas the most exciting applications of LLMs relate to free-form generations.
The term hallucination in the context of machine learning originally comes from filling in ungrounded details, either as a deliberate strategy 20 or as a reliability problem 4 . The appropriateness of the metaphor has been questioned as promoting undue anthropomorphism 21 . Although we agree that metaphor must be used carefully with LLMs 22 , the widespread adoption of the term hallucination reflects the fact that it points to an important phenomenon. This work represents a step towards making that phenomenon more precise.
To detect confabulations, we use probabilistic tools to define and then measure the ‘semantic’ entropy of the generations of an LLM—an entropy that is computed over meanings of sentences. High entropy corresponds to high uncertainty 23 , 24 , 25 —so semantic entropy is one way to estimate semantic uncertainties. Semantic uncertainty, the broader category of measures we introduce, could be operationalized with other measures of uncertainty, such as mutual information, instead. Entropy in free-form generation is normally hard to measure because answers might mean the same thing (be semantically equivalent) despite being expressed differently (being syntactically or lexically distinct). This causes naive estimates of entropy or other lexical variation scores 26 to be misleadingly high when the same correct answer might be written in many ways without changing its meaning.
By contrast, our semantic entropy moves towards estimating the entropy of the distribution of meanings of free-form answers to questions, insofar as that is possible, rather than the distribution over the ‘tokens’ (words or word-pieces) which LLMs natively represent. This can be seen as a kind of semantic consistency check 27 for random seed variation. An overview of our approach is provided in Fig. 1 and a worked example in Supplementary Table 1 .
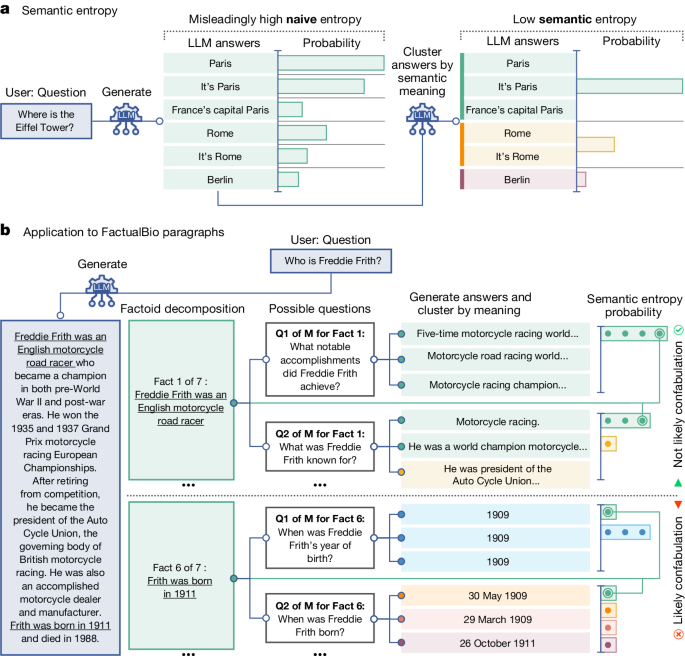
a , Naive entropy-based uncertainty measures variation in the exact answers, treating ‘Paris’, ‘It’s Paris’ and ‘France’s capital Paris’ as different. But this is unsuitable for language tasks for which sometimes different answers mean the same things. Our semantic entropy clusters answers which share meanings before computing the entropy. A low semantic entropy shows that the LLM is confident about the meaning. b , Semantic entropy can also detect confabulations in longer passages. We automatically decompose a long generated answer into factoids. For each factoid, an LLM generates questions to which that factoid might have been the answer. The original LLM then samples M possible answers to these questions. Finally, we compute the semantic entropy over the answers to each specific question, including the original factoid. Confabulations are indicated by high average semantic entropy for questions associated with that factoid. Here, semantic entropy classifies Fact 1 as probably not a confabulation because generations often mean the same thing, despite very different wordings, which a naive entropy would have missed.
Intuitively, our method works by sampling several possible answers to each question and clustering them algorithmically into answers that have similar meanings, which we determine on the basis of whether answers in the same cluster entail each other bidirectionally 28 . That is, if sentence A entails that sentence B is true and vice versa, then we consider them to be in the same semantic cluster. We measure entailment using both general-purpose LLMs and natural language inference (NLI) tools developed specifically for detecting entailment for which we show direct evaluations in Supplementary Tables 2 and 3 and Supplementary Fig. 1 . Textual entailment has previously been shown to correlate with faithfulness 10 in the context of factual consistency 29 as well as being used to measure factuality in abstractive summarization 30 , especially when applied at the right granularity 31 .
Semantic entropy detects confabulations in free-form text generation across a range of language models and domains, without previous domain knowledge. Our evaluations cover question answering in trivia knowledge (TriviaQA 32 ), general knowledge (SQuAD 1.1; ref. 33 ), life sciences (BioASQ 34 ) and open-domain natural questions (NQ-Open 35 ) derived from actual queries to Google Search 36 . In addition, semantic entropy detects confabulations in mathematical word problems (SVAMP 37 ) and in a biography-generation dataset, FactualBio, accompanying this paper.
Our results for TriviaQA, SQuAD, BioASQ, NQ-Open and SVAMP are all evaluated context-free and involve sentence-length answers (96 ± 70 characters, mean ± s.d.) and use LLaMA 2 Chat (7B, 13B and 70B parameters) 38 , Falcon Instruct (7B and 40B) 39 and Mistral Instruct (7B) 40 . In the Supplementary Information , we further consider short-phrase-length answers. Results for FactualBio (442 ± 122 characters) use GPT-4 (ref. 1 ). At the time of writing, GPT-4 (ref. 1 ) did not expose output probabilities 41 or hidden states, although it does now. As a result, we propose a discrete approximation of our estimator for semantic entropy which allows us to run experiments without access to output probabilities, which we use for all GPT-4 results in this paper and which performs similarly well.
Our confabulation detection with semantic entropy is more robust to user inputs from previously unseen domains than methods which aim to ‘learn’ how to detect confabulations from a set of example demonstrations. Our method is unsupervised, meaning that we do not need labelled examples of confabulations. By contrast, supervised methods detect confabulations by learning patterns behind examples of confabulations, assuming that future questions preserve these patterns. But this assumption is often untrue in new situations or with confabulations that human overseers are unable to identify (compare Fig. 17 of ref. 24 ). As a strong supervised baseline, we compare to an embedding regression method inspired by ref. 24 which trains a logistic regression classifier to predict whether the model correctly answered a question on the basis of the final ‘embedding’ (hidden state) of the LLM. We also use the P (True) method 24 which looks at the probability with which an LLM predicts that the next token is ‘True’ when few-shot prompted to compare a main answer with ‘brainstormed’ alternatives.
Confabulations contribute substantially to incorrect answers given by language models. We show that semantic entropy can be used to predict many incorrect model answers and to improve question-answering accuracy by refusing to answer those questions the model is uncertain about. Corresponding to these two uses, we evaluate two main metrics. First, the widely used area under the receiver operating characteristic (AUROC) curve for the binary event that a given answer is incorrect. This measure captures both precision and recall and ranges from 0 to 1, with 1 representing a perfect classifier and 0.5 representing an un-informative classifier. We also show a new measure, the area under the ‘rejection accuracy’ curve (AURAC). This studies the case in which the confabulation detection score is used to refuse to answer the questions judged most likely to cause confabulations. Rejection accuracy is the accuracy of the answers of the model on the remaining questions and the area under this curve is a summary statistic over many thresholds (representative threshold accuracies are provided in Supplementary Material ). The AURAC captures the accuracy improvement which users would experience if semantic entropy was used to filter out questions causing the highest entropy.
Detecting confabulations in QA and math
In Fig. 2 , we show that both semantic entropy and its discrete approximation outperform our best baselines for sentence-length generations. These results are averaged across datasets and provide the actual scores on the held-out evaluation dataset. We report the raw average score across held-out evaluation datasets without standard error because the distributional characteristics are more a property of the models and datasets selected than the method. Consistency of relative results across different datasets is a stronger indicator of variation in this case.
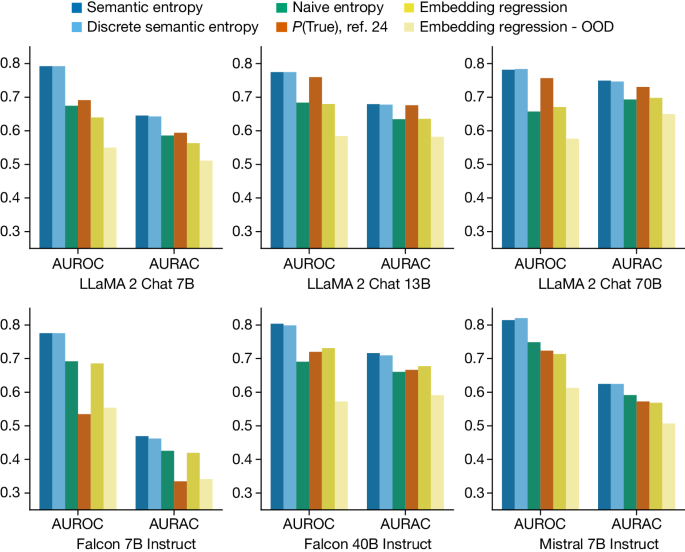
Semantic entropy outperforms leading baselines and naive entropy. AUROC (scored on the y -axes) measures how well methods predict LLM mistakes, which correlate with confabulations. AURAC (likewise scored on the y -axes) measures the performance improvement of a system that refuses to answer questions which are judged likely to cause confabulations. Results are an average over five datasets, with individual metrics provided in the Supplementary Information .
Semantic entropy greatly outperforms the naive estimation of uncertainty using entropy: computing the entropy of the length-normalized joint probability of the token sequences. Naive entropy estimation ignores the fact that token probabilities also express the uncertainty of the model over phrasings that do not change the meaning of an output.
Our methods also outperform the supervised embedding regression method both in- and out-of-distribution. In pale-yellow bars we show that embedding regression performance deteriorates when its training data do not match the deployment distribution—which mirrors the common real-world case in which there is a distribution shift between training and deployment 42 —the plotted value is the average metric for embedding regression trained on one of the four ‘off-distribution’ datasets for that evaluation. This is critical because reliable uncertainty is most important when the data distribution shifts. Semantic entropy also outperforms P (True) which is supervised ‘in-context’; that is, it is adapted to the deployment task with a few training examples provided in the LLM prompt itself. The discrete variant of semantic entropy performs similarly to our standard estimator, despite not requiring exact output probabilities.
Averaged across the 30 combinations of tasks and models we study, semantic entropy achieves the best AUROC value of 0.790 whereas naive entropy (0.691), P (True) (0.698) and the embedding regression baseline (0.687) lag behind it. Semantic entropy performs well consistently, with stable performance (between 0.78 and 0.81 AUROC) across the different model families (LLaMA, Falcon and Mistral) and scales (from 7B to 70B parameters) which we study (we report summary statistics for each dataset and model as before). Although semantic entropy outperforms the baselines across all model sizes, P (True) seems to improve with model size, suggesting that it might become more competitive for very capable honest models in settings that the model understands well (which are, however, not the most important cases to have good uncertainty). We use ten generations to compute entropy, selected using analysis in Supplementary Fig. 2 . Further results for short-phrase generations are described in Supplementary Figs. 7 – 10 .
The results in Fig. 2 offer a lower bound on the effectiveness of semantic entropy at detecting confabulations. These evaluations determine whether semantic entropy and baseline methods can detect when the answers of the model are incorrect (which we validate against human correctness evaluations in Supplementary Table 4 ). In addition to errors from confabulations (arbitrary incorrectness), this also includes other types of mistakes for which semantic entropy is not suited, such as consistent errors learned from the training data. The fact that methods such as embedding regression are able to spot other kinds of errors, not just confabulations, but still are outperformed by semantic entropy, suggests that confabulations are a principal category of errors for actual generations.
Examples of questions and answers from TriviaQA, SQuAD and BioASQ, for LLaMA 2 Chat 70B, are shown in Table 1 . These illustrate how only semantic entropy detects when the meaning is constant but the form varies (the first row of the table) whereas semantic entropy and naive entropy both correctly predict the presence of confabulations when the form and meaning vary together (second row) and predict the absence of confabulations when the form and meaning are both constant across several resampled generations (third row). In the final row, we give an example in which semantic entropy is erroneously high as a result of overly sensitive semantic clustering relative to the reference answer. Our clustering method distinguishes the answers which provide a precise date from those which only provide a year. For some contexts that would have been correct but in this context the distinction between the specific day and the year is probably irrelevant. This highlights the importance of context and judgement in clustering, especially in subtle cases, as well as the shortcomings of evaluating against fixed reference answers which do not capture the open-ended flexibility of conversational deployments of LLMs.
Detecting confabulations in biographies
Semantic entropy is most natural for sentences that express a single proposition but the idea of semantic equivalence is trickier to apply to longer passages which express many propositions which might only agree partially 43 . Nevertheless, we can use semantic entropy to detect confabulations in longer generations, such as entire paragraphs of text. To show this, we develop a dataset of biographical generations from GPT-4 (v.0613) for 21 individuals notable enough to have their own Wikipedia page but without extensive online biographies. From each biography generated by GPT-4, we automatically extract propositional factual claims about the individual (150 factual claims in total), which we manually label as true or false.
Applying semantic entropy to this problem is challenging. Naively, one might simply regenerate each sentence (conditioned on the text so far) and then compute semantic entropy over these regenerations. However, the resampled sentences often target different aspects of the biography: for example, one time describing family and the next time profession. This is analogous to the original problem semantic entropy was designed to resolve: the model is uncertain about the right ordering of facts, not about the facts themselves. To address this, we break down the entire paragraph into factual claims and reconstruct questions which might have been answered by those claims. Only then do we apply semantic entropy (Fig. 1 ) by generating three new answers to each question (selected with analysis in Supplementary Figs. 3 and 4 ) and computing the semantic entropy over those generations plus the original factual claim. We aggregate these by averaging the semantic entropy over all the questions to get an uncertainty score for each proposition, which we use to detect confabulations. Unaggregated results are shown in Supplementary Figs. 5 and 6 .
As GPT-4 did not allow access to the probability of the generation at the time of writing, we use a discrete variant of semantic entropy which makes the further approximation that we can infer a discrete empirical distribution over semantic meaning clusters from only the generations ( Methods ). This allows us to compute semantic entropy using only the black-box outputs of an LLM. However, we were unable to compute the naive entropy baseline, the standard semantic entropy estimator or the embedding regression baseline for GPT-4 without output probabilities and embeddings.
In Fig. 3 we show that the discrete variant of semantic entropy effectively detects confabulations on this dataset. Its AUROC and AURAC are higher than either a simple ‘self-check’ baseline—which just asks the LLM whether the factoid is likely to be true—or a variant of P (True) which has been adapted to work for the paragraph-length setting. Discrete semantic entropy has better rejection accuracy performance until 20% of the questions have been rejected at which point P (True) has a narrow edge. This indicates that the questions predicted to cause confabulations are indeed more likely to be wrong.
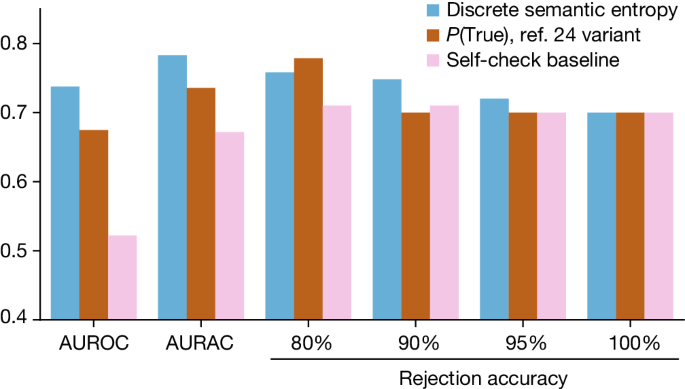
The discrete variant of our semantic entropy estimator outperforms baselines both when measured by AUROC and AURAC metrics (scored on the y -axis). The AUROC and AURAC are substantially higher than for both baselines. At above 80% of questions being answered, semantic entropy has the highest accuracy. Only when the top 20% of answers judged most likely to be confabulations are rejected does the answer accuracy on the remainder for the P (True) baseline exceed semantic entropy.
Our probabilistic approach, accounting for semantic equivalence, detects an important class of hallucinations: those that are caused by a lack of LLM knowledge. These are a substantial portion of the failures at present and will continue even as models grow in capabilities because situations and cases that humans cannot reliably supervise will persist. Confabulations are a particularly noteworthy failure mode for question answering but appear in other domains too. Semantic entropy needs no previous domain knowledge and we expect that algorithmic adaptations to other problems will allow similar advances in, for example, abstractive summarization. In addition, extensions to alternative input variations such as rephrasing or counterfactual scenarios would allow a similar method to act as a form of cross-examination 44 for scalable oversight through debate 45 .
The success of semantic entropy at detecting errors suggests that LLMs are even better at “knowing what they don’t know” than was argued by ref. 24 —they just don’t know they know what they don’t know. Our method explicitly does not directly address situations in which LLMs are confidently wrong because they have been trained with objectives that systematically produce dangerous behaviour, cause systematic reasoning errors or are systematically misleading the user. We believe that these represent different underlying mechanisms—despite similar ‘symptoms’—and need to be handled separately.
One exciting aspect of our approach is the way it makes use of classical probabilistic machine learning methods and adapts them to the unique properties of modern LLMs and free-form language generation. We hope to inspire a fruitful exchange of well-studied methods and emerging new problems by highlighting the importance of meaning when addressing language-based machine learning problems.
Semantic entropy as a strategy for overcoming confabulation builds on probabilistic tools for uncertainty estimation. It can be applied directly to any LLM or similar foundation model without requiring any modifications to the architecture. Our ‘discrete’ variant of semantic uncertainty can be applied even when the predicted probabilities for the generations are not available, for example, because access to the internals of the model is limited.
In this section we introduce background on probabilistic methods and uncertainty in machine learning, discuss how it applies to language models and then discuss our contribution, semantic entropy, in detail.
Uncertainty and machine learning
We aim to detect confabulations in LLMs, using the principle that the model will be uncertain about generations for which its output is going to be arbitrary.
One measure of uncertainty is the predictive entropy of the output distribution, which measures the information one has about the output given the input 25 . The predictive entropy (PE) for an input sentence x is the conditional entropy ( H ) of the output random variable Y with realization y given x ,
A low predictive entropy indicates an output distribution which is heavily concentrated whereas a high predictive entropy indicates that many possible outputs are similarly likely.
Aleatoric and epistemic uncertainty
We do not distinguish between aleatoric and epistemic uncertainty in our analysis. Researchers sometimes separate aleatoric uncertainty (uncertainty in the underlying data distribution) from epistemic uncertainty (caused by having only limited information) 46 . Further advances in uncertainty estimation which separate these kinds of uncertainty would enhance the potential for our semantic uncertainty approach by allowing extensions beyond entropy.
Joint probabilities of sequences of tokens
Generative LLMs produce strings of text by selecting tokens in sequence. Each token is a wordpiece that often represents three or four characters (though especially common sequences and important words such as numbers typically get their own token). To compute entropies, we need access to the probabilities the LLM assigns to the generated sequence of tokens. The probability of the entire sequence, s , conditioned on the context, x , is the product of the conditional probabilities of new tokens given past tokens, whose resulting log-probability is \(\log P({\bf{s}}| {\boldsymbol{x}})={\sum }_{i}\log P({s}_{i}| {{\bf{s}}}_{ < i},{\boldsymbol{x}})\) , where s i is the i th output token and s < i denotes the set of previous tokens.
Length normalization
When comparing the log-probabilities of generated sequences, we use ‘length normalization’, that is, we use an arithmetic mean log-probability, \(\frac{1}{N}{\sum }_{i}^{N}\log P({s}_{i}| {{\bf{s}}}_{ < i},{\boldsymbol{x}})\) , instead of the sum. In expectation, longer sequences have lower joint likelihoods because of the conditional independence of the token probabilities 47 . The joint likelihood of a sequence of length N shrinks exponentially in N . Its negative log-probability therefore grows linearly in N , so longer sentences tend to contribute more to entropy. We therefore interpret length-normalizing the log-probabilities when estimating the entropy as asserting that the expected uncertainty of generations is independent of sentence length. Length normalization has some empirical success 48 , including in our own preliminary experiments, but little theoretical justification in the literature.
Principles of semantic uncertainty
If we naively calculate the predictive entropy directly from the probabilities of the generated sequence of tokens, we conflate the uncertainty of the model over the meaning of its answer with the uncertainty over the exact tokens used to express that meaning. For example, even if the model is confident in the meaning of a generation, there are still usually many different ways for phrasing that generation without changing its meaning. For the purposes of detecting confabulations, the uncertainty of the LLM over meanings is more important than the uncertainty over the exact tokens used to express those meanings.
Our semantic uncertainty method therefore seeks to estimate only the uncertainty the LLM has over the meaning of its generation, not the choice of words. To do this, we introduce an algorithm that clusters model generations by meaning and subsequently calculates semantic uncertainty. At a high level this involves three steps:
Generation: sample output sequences of tokens from the predictive distribution of a LLM given a context x .
Clustering: cluster sequences by their meaning using our clustering algorithm based on bidirectional entailment.
Entropy estimation: estimate semantic entropy by summing probabilities of sequences that share a meaning following equation ( 2 ) and compute their entropy.
Generating a set of answers from the model
Given some context x as input to the LLM, we sample M sequences, { s (1) , …, s ( M ) } and record their token probabilities, { P ( s (1) ∣ x ), …, P ( s ( M ) ∣ x )}. We sample all our generations from a single model, varying only the random seed used for sampling from the token probabilities. We do not observe the method to be particularly sensitive to details of the sampling scheme. In our implementation, we sample at temperature 1 using nucleus sampling ( P = 0.9) (ref. 49 ) and top- K sampling ( K = 50) (ref. 50 ). We also sample a single generation at low temperature (0.1) as an estimate of the ‘best generation’ of the model to the context, which we use to assess the accuracy of the model. (A lower sampling temperature increases the probability of sampling the most likely tokens).
Clustering by semantic equivalence
To estimate semantic entropy we need to cluster generated outputs from the model into groups of outputs that mean the same thing as each other.
This can be described using ‘semantic equivalence’ which is the relation that holds between two sentences when they mean the same thing. We can formalize semantic equivalence mathematically. Let the space of tokens in a language be \({\mathcal{T}}\) . The space of all possible sequences of tokens of length N is then \({{\mathcal{S}}}_{N}\equiv {{\mathcal{T}}}^{N}\) . Note that N can be made arbitrarily large to accommodate whatever size of sentence one can imagine and one of the tokens can be a ‘padding’ token which occurs with certainty for each token after the end-of-sequence token. For some sentence \({\bf{s}}\in {{\mathcal{S}}}_{N}\) , composed of a sequence of tokens, \({s}_{i}\in {\mathcal{T}}\) , there is an associated meaning. Theories of meaning are contested 51 . However, for specific models and deployment contexts many considerations can be set aside. Care should be taken comparing very different models and contexts.
Let us introduce a semantic equivalence relation, E ( ⋅ , ⋅ ), which holds for any two sentences that mean the same thing—we will operationalize this presently. Recall that an equivalence relation is any reflexive, symmetric and transitive relation and that any equivalence relation on a set corresponds to a set of equivalence classes. Each semantic equivalence class captures outputs that can be considered to express the same meaning. That is, for the space of semantic equivalence classes \({\mathcal{C}}\) the sentences in the set \(c\in {\mathcal{C}}\) can be regarded in many settings as expressing a similar meaning such that \(\forall {\bf{s}},{{\bf{s}}}^{{\prime} }\in c:E({\bf{s}},{{\bf{s}}}^{{\prime} })\) . So we can build up these classes of semantically equivalent sentences by checking if new sentences share a meaning with any sentences we have already clustered and, if so, adding them into that class.
We operationalize E ( ⋅ , ⋅ ) using the idea of bidirectional entailment, which has a long history in linguistics 52 and natural language processing 28 , 53 , 54 . A sequence, s , means the same thing as a second sequence, s ′, only if the sequences entail (that is, logically imply) each other. For example, ‘The capital of France is Paris’ entails ‘Paris is the capital of France’ and vice versa because they mean the same thing. (See later for a discussion of soft equivalence and cases in which bidirectional entailment does not guarantee equivalent meanings).
Importantly, we require that the sequences mean the same thing with respect to the context—key meaning is sometimes contained in the context. For example, ‘Paris’ does not entail ‘The capital of France is Paris’ because ‘Paris’ is not a declarative sentence without context. But in the context of the question ‘What is the capital of France?’, the one-word answer does entail the longer answer.
Detecting entailment has been the object of study of a great deal of research in NLI 55 . We rely on language models to predict entailment, such as DeBERTa-Large-MNLI 56 , which has been trained to predict entailment, or general-purpose LLMs such as GPT-3.5 (ref. 57 ), which can predict entailment given suitable prompts.
We then cluster sentences according to whether they bidirectionally entail each other using the algorithm presented in Extended Data Fig. 1 . Note that, to check if a sequence should be added to an existing cluster, it is sufficient to check if the sequence bidirectionally entails any of the existing sequences in that cluster (we arbitrarily pick the first one), given the transitivity of semantic equivalence. If a sequence does not share meaning with any existing cluster, we assign it its own cluster.
Computing the semantic entropy
Having determined the classes of generated sequences that mean the same thing, we can estimate the likelihood that a sequence generated by the LLM belongs to a given class by computing the sum of the probabilities of all the possible sequences of tokens which can be considered to express the same meaning as
Formally, this treats the output as a random variable whose event-space is the space of all possible meaning-classes, C , a sub- σ -algebra of the standard event-space S . We can then estimate the semantic entropy (SE) as the entropy over the meaning-distribution,
There is a complication which prevents direct computation: we do not have access to every possible meaning-class c . Instead, we can only sample c from the sequence-generating distribution induced by the model. To handle this, we estimate the expectation in equation ( 3 ) using a Rao–Blackwellized Monte Carlo integration over the semantic equivalence classes C ,
where \(P({C}_{i}| {\boldsymbol{x}})=\frac{P({c}_{i}| {\boldsymbol{x}})}{{\sum }_{c}P(c| {\boldsymbol{x}})}\) estimates a categorical distribution over the cluster meanings, that is, ∑ i P ( C i ∣ x ) = 1. Without this normalization step cluster ‘probabilities’ could exceed one because of length normalization, resulting in degeneracies. Equation ( 5 ) is the estimator giving our main method that we refer to as semantic entropy throughout the text.
For scenarios in which the sequence probabilities are not available, we propose a variant of semantic entropy which we call ‘discrete’ semantic entropy. Discrete semantic entropy approximates P ( C i ∣ x ) directly from the number of generations in each cluster, disregarding the token probabilities. That is, we approximate P ( C i ∣ x ) as \({\sum }_{1}^{M}\frac{{I}_{c={C}_{i}}}{M}\) , the proportion of all the sampled answers which belong to that cluster. Effectively, this just assumes that each output that was actually generated was equally probable—estimating the underlying distribution as the categorical empirical distribution. In the limit of M the estimator converges to equation ( 5 ) by the law of large numbers. We find that discrete semantic entropy results in similar performance empirically.
We provide a worked example of the computation of semantic entropy in Supplementary Note 1 .
Semantic entropy is designed to detect confabulations, that is, model outputs with arbitrary meaning. In our experiments, we use semantic uncertainty to predict model accuracy, demonstrating that confabulations make up a notable fraction of model mistakes. We further show that semantic uncertainty can be used to improve model accuracy by refusing to answer questions when semantic uncertainty is high. Last, semantic uncertainty can be used to give users a way to know when model generations are probably unreliable.
We use the datasets BioASQ 34 , SQuAD 33 , TriviaQA 32 , SVAMP 37 and NQ-Open 35 . BioASQ is a life-sciences question-answering dataset based on the annual challenge of the same name. The specific dataset we use is based on the QA dataset from Task B of the 2023 BioASQ challenge (11B). SQuAD is a reading comprehension dataset whose context passages are drawn from Wikipedia and for which the answers to questions can be found in these passages. We use SQuAD 1.1 which excludes the unanswerable questions added in v.2.0 that are deliberately constructed to induce mistakes so they do not in practice cause confabulations to occur. TriviaQA is a trivia question-answering dataset. SVAMP is a word-problem maths dataset containing elementary-school mathematical reasoning tasks. NQ-Open is a dataset of realistic questions aggregated from Google Search which have been chosen to be answerable without reference to a source text. For each dataset, we use 400 train examples and 400 test examples randomly sampled from the original larger dataset. Note that only some of the methods require training, for example semantic entropy does not use the training data. If the datasets themselves are already split into train and test (or validation) samples, we sample our examples from within the corresponding split.
All these datasets are free-form, rather than multiple choice, because this better captures the opportunities created by LLMs to produce free-form sentences as answers. We refer to this default scenario as our ‘sentence-length’ experiments. In Supplementary Note 7 , we also present results for confabulation detection in a ‘short-phrase’ scenario, in which we constrain model answers on these datasets to be as concise as possible.
To make the problems more difficult and induce confabulations, we do not provide the context passages for any of the datasets. When the context passages are provided, the accuracy rate is too high for these datasets for the latest generations of models to meaningfully study confabulations.
For sentence-length generations we use: Falcon 39 Instruct (7B and 40B), LLaMA 2 Chat 38 (7B, 13B and 70B) and Mistral 40 Instruct (7B).
In addition to reporting results for semantic entropy, discrete semantic entropy and naive entropy, we consider two strong baselines.
Embedding regression is a supervised baseline inspired by the P (IK) method 24 . In that paper, the authors fine-tune their proprietary LLM on a dataset of questions to predict whether the model would have been correct. This requires access to a dataset of ground-truth answers to the questions. Rather than fine-tuning the entire LLM in this way, we simply take the final hidden units and train a logistic regression classifier to make the same prediction. By contrast to their method, this is much simpler because it does not require fine-tuning the entire language model, as well as being more reproducible because the solution to the logistic regression optimization problem is not as seed-dependent as the fine-tuning procedure. As expected, this supervised approach performs well in-distribution but fails when the distribution of questions is different from that on which the classifier is trained.
The second baseline we consider is the P (True) method 24 , in which the model first samples M answers (identically to our semantic entropy approach) and then is prompted with the list of all answers generated followed by the highest probability answer and a question whether this answer is “(a) True” or “(b) False”. The confidence score is then taken to be the probability with which the LLM responds with ‘a’ to the multiple-choice question. The performance of this method is boosted with a few-shot prompt, in which up to 20 examples from the training set are randomly chosen, filled in as above, but then provided with the actual ground truth of whether the proposed answer was true or false. In this way, the method can be considered as supervised ‘in-context’ because it makes use of some ground-truth training labels but can be used without retraining the model. Because of context-size constraints, this method cannot fit a full 20 few-shot examples in the context when input questions are long or large numbers of generations are used. As a result, we sometimes have to reduce the number of few-shot examples to suit the context size and we note this in the Supplementary Material .
Entailment estimator
Any NLI classification system could be used for our bidirectional entailment clustering algorithm. We consider two different kinds of entailment detector.
One option is to use an instruction-tuned LLM such as LLaMA 2, GPT-3.5 (Turbo 1106) or GPT-4 to predict entailment between generations. We use the following prompt:
We are evaluating answers to the question {question} Here are two possible answers: Possible Answer 1: {text1} Possible Answer 2: {text2} Does Possible Answer 1 semantically entail Possible Answer 2? Respond with entailment, contradiction, or neutral.
Alternatively, we consider using a language model trained for entailment prediction, specifically the DeBERTa-large model 56 fine-tuned on the NLI dataset MNLI 58 . This builds on past work towards paraphrase identification based on embedding similarity 59 , 60 and BERT-style models 61 , 62 . We template more simply, checking if DeBERTa predicts entailment between the concatenation of the question and one answer and the concatenation of the question and another answer. Note that DeBERTa-large is a relatively lightweight model with only 1.5B parameters which is much less powerful than most of the LLMs under study.
In Supplementary Note 2 , we carefully evaluate the benefits and drawbacks of these methods for entailment prediction. We settle on using GPT-3.5 with the above prompt, as its entailment predictions agree well with human raters and lead to good confabulation detection performance.
In Supplementary Note 3 , we provide a discussion of the computational cost and choosing the number of generations for reliable clustering.
Prompting templates
We use a simple generation template for all sentence-length answer datasets:
Answer the following question in a single brief but complete sentence. Question: {question} Answer:
Metrics and accuracy measurements
We use three main metrics to evaluate our method: AUROC, rejection accuracy and AURAC. Each of these is grounded in an automated factuality estimation measurement relative to the reference answers provided by the datasets that we use.
AUROC, rejection accuracy and AURAC
First, we use the AUROC curve, which measures the reliability of a classifier accounting for both precision and recall. The AUROC can be interpreted as the probability that a randomly chosen correct answer has been assigned a higher confidence score than a randomly chosen incorrect answer. For a perfect classifier, this is 1.
Second, we compute the ‘rejection accuracy at X %’, which is the question-answering accuracy of the model on the most-confident X % of the inputs as identified by the respective uncertainty method. If an uncertainty method works well, predictions on the confident subset should be more accurate than predictions on the excluded subset and the rejection accuracy should increase as we reject more inputs.
To summarize this statistic we compute the AURAC—the total area enclosed by the accuracies at all cut-off percentages X %. This should increase towards 1 as given uncertainty method becomes more accurate and better at detecting likely-inaccurate responses but it is more sensitive to the overall accuracy of the model than the AUROC metric.
In Supplementary Note 5 , we provide the unaggregated rejection accuracies for sentence-length generations.
Assessing accuracy
For the short-phrase-length generation setting presented in Supplementary Note 7 , we simply assess the accuracy of the generations by checking if the F1 score of the commonly used SQuAD metric exceeds 0.5. There are limitations to such simple scoring rules 63 but this method is widely used in practice and its error is comparatively small on these standard datasets.
For our default scenario, the longer sentence-length generations, this measure fails, as the overlap between the short reference answer and our long model answer is invariably too small. For sentence-length generations, we therefore automatically determine whether an answer to the question is correct or incorrect by using GPT-4 to compare the given answer to the reference answer. We use the template:
We are assessing the quality of answers to the following question: {question} The expected answer is: {reference answer} The proposed answer is: {predicted answer} Within the context of the question, does the proposed answer mean the same as the expected answer? Respond only with yes or no.
We make a small modification for datasets with several reference answers: line two becomes “The following are expected answers to this question:” and the final line asks “does the proposed answer mean the same as any of the expected answers?”.
In Supplementary Note 6 , we check the quality of our automated ground-truth evaluations against human judgement by hand. We find that GPT-4 gives the best results for determining model accuracy and thus use it in all our sentence-length experiments.
In this section we describe the application of semantic entropy to confabulation detection in longer model generations, specifically paragraph-length biographies.
We introduce a biography-generation dataset—FactualBio—available alongside this paper. FactualBio is a collection of biographies of individuals who are notable enough to have Wikipedia pages but not notable enough to have large amounts of detailed coverage, generated by GPT-4 (v.0613). To generate the dataset, we randomly sampled 21 individuals from the WikiBio dataset 64 . For each biography, we generated a list of factual claims contained in each biography using GPT-4, with 150 total factual claims (the total number is only coincidentally a round number). For each of these factual claims, we manually determined whether the claim was correct or incorrect. Out of 150 claims, 45 were incorrect. As before, we apply confabulation detection to detect incorrect model predictions, even though there may be model errors which are not confabulations.
Prompting and generation
Given a paragraph-length piece of LLM-generated text, we apply the following sequence of steps:
Automatically decompose the paragraph into specific factual claims using an LLM (not necessarily the same as the original).
For each factual claim, use an LLM to automatically construct Q questions which might have produced that claim.
For each question, prompt the original LLM to generate M answers.
For each question, compute the semantic entropy of the answers, including the original factual claim.
Average the semantic entropies over the questions to arrive at a score for the original factual claim.
We pursue this slightly indirect way of generating answers because we find that simply resampling each sentence creates variation unrelated to the uncertainty of the model about the factual claim, such as differences in paragraph structure.
We decompose the paragraph into factual claims using the following prompt:
Please list the specific factual propositions included in the answer above. Be complete and do not leave any factual claims out. Provide each claim as a separate sentence in a separate bullet point.
We found that we agreed with the decompositions in all cases in the dataset.
We then generate six questions for each of the facts from the decomposition. We generate these questions by prompting the model twice with the following:
Following this text: {text so far} You see the sentence: {proposition} Generate a list of three questions, that might have generated the sentence in the context of the preceding original text, as well as their answers. Please do not use specific facts that appear in the follow-up sentence when formulating the question. Make the questions and answers diverse. Avoid yes-no questions. The answers should not be a full sentence and as short as possible, e.g. only a name, place, or thing. Use the format “1. {question} – {answer}”.
These questions are not necessarily well-targeted and the difficulty of this step is the main source of errors in the procedure. We generate three questions with each prompt, as this encourages diversity of the questions, each question targeting a different aspect of the fact. However, we observed that the generated questions will sometimes miss obvious aspects of the fact. Executing the above prompt twice (for a total of six questions) can improve coverage. We also ask for brief answers because the current version of GPT-4 tends to give long, convoluted and highly hedged answers unless explicitly told not to.
Then, for each question, we generate three new answers using the following prompt:
We are writing an answer to the question “{user question}”. So far we have written: {text so far} The next sentence should be the answer to the following question: {question} Please answer this question. Do not answer in a full sentence. Answer with as few words as possible, e.g. only a name, place, or thing.
We then compute the semantic entropy over these answers plus the original factual claim. Including the original fact ensures that the estimator remains grounded in the original claim and helps detect situations in which the question has been interpreted completely differently from the original context. We make a small modification to handle the fact that GPT-4 generations often include refusals to answer questions. These refusals were not something we commonly observe in our experiments with LLaMA 2, Falcon or Mistral models. If more than half of the answers include one of the strings ‘not available’, ‘not provided’, ‘unknown’ or ‘unclear’ then we treat the semantic uncertainty as maximal.
We then average the semantic entropies for each question corresponding to the factual claim to get an entropy for this factual claim.
Despite the extra assumptions and complexity, we find that this method greatly outperforms the baselines.
To compute semantic entailment between the original claim and regenerated answers, we rely on the DeBERTa entailment prediction model as we find empirically that DeBERTa predictions result in higher train-set AUROC than other methods. Because DeBERTa has slightly lower recall than GPT-3.5/4, we use a modified set-up for which we say the answers mean the same as each other if at least one of them entails the other and neither is seen to contradict the other—a kind of ‘non-defeating’ bidirectional entailment check rather than true bidirectional entailment. The good performance of DeBERTa in this scenario is not surprising as both factual claims and regenerated answers are relatively short. We refer to Supplementary Notes 2 and 3 for ablations and experiments regarding our choice of entailment estimator for paragraph-length generations.
We implement two baselines. First, we implement a variant of the P (True) method, which is adapted to the new setting. For each factoid, we generate a question with answers in the same way as for semantic entropy. We then use the following prompt:
Question: {question} Here are some brainstormed ideas: {list of regenerated answers} Possible answer: {original answer} Is the possible answer true? Respond with “yes” or “no”.
As we cannot access the probabilities GPT-4 assigns to predicting ‘yes’ and ‘no’ as the next token, we approximate this using Monte Carlo samples. Concretely, we execute the above prompt ten times (at temperature 1) and then take the fraction of answers which was ‘yes’ as our unbiased Monte Carlo estimate of the token probability GPT-4 assigns to ‘yes’.
As a second, simpler, baseline we check if the model thinks the answer is true. We simply ask:
Following this text: {text so far} You see this statement: {proposition} Is it likely that the statement is true? Respond with ‘yes’ or ‘no’.
It is interesting that this method ought to perform very well if we think that the model has good ‘self-knowledge’ (that is, if “models mostly know what they don’t know” 24 ) but in fact semantic entropy is much better at detecting confabulations.
Data availability
The data used for the short-phrase and sentence-length generations are publicly available and the released code details how to access it. We release a public version of the FactualBio dataset as part of the code base for reproducing the paragraph-length experiments.
Code availability
We release all code used to produce the main experiments. The code for short-phrase and sentence-length experiments can be found at github.com/jlko/semantic_uncertainty and https://doi.org/10.5281/zenodo.10964366 (ref. 65 ). The code for paragraph-length experiments can be found at github.com/jlko/long_hallucinations and https://doi.org/10.5281/zenodo.10964366 (ref. 65 ).
GPT-4 technical report. Preprint at https://arxiv.org/abs/2303.08774 (2023).
Gemini: a family of highly capable multimodal models. Preprint at https://arxiv.org/abs/2312.11805 (2023).
Xiao, Y. & Wang, W. Y. On hallucination and predictive uncertainty in conditional language generation. In Proc. 16th Conference of the European Chapter of the Association for Computational Linguistics 2734–2744 (Association for Computational Linguistics, 2021).
Rohrbach, A., Hendricks, L. A., Burns, K., Darrell, T. & Saenko, K. Object hallucination in image captioning. In Proc. 2018 Conference on Empirical Methods in Natural Language Processing (eds Riloff, E., Chiang, D., Hockenmaier, J. & Tsujii, J.) 4035–4045 (Association for Computational Linguistics, 2018).
Weiser, B. Lawyer who used ChatGPT faces penalty for made up citations. The New York Times (8 Jun 2023).
Opdahl, A. L. et al. Trustworthy journalism through AI. Data Knowl. Eng . 146 , 102182 (2023).
Shen, Y. et al. ChatGPT and other large language models are double-edged swords. Radiology 307 , e230163 (2023).
Article PubMed Google Scholar
Schulman, J. Reinforcement learning from human feedback: progress and challenges. Presented at the Berkeley EECS Colloquium. YouTube www.youtube.com/watch?v=hhiLw5Q_UFg (2023).
Ji, Z. et al. Survey of hallucination in natural language generation. ACM Comput. Surv. 55 , 248 (2023).
Maynez, J., Narayan, S., Bohnet, B. & McDonald, R. On faithfulness and factuality in abstractive summarization. In Proc. 58th Annual Meeting of the Association for Computational Linguistics (eds Jurafsky, D., Chai, J., Schluter, N. & Tetreault, J.) 1906–1919 (Association for Computational Linguistics, 2020).
Filippova, K. Controlled hallucinations: learning to generate faithfully from noisy data. In Findings of the Association for Computational Linguistics: EMNLP 2020 (eds Webber, B., Cohn, T., He, Y. & Liu, Y.) 864–870 (Association for Computational Linguistics, 2020).
Berrios, G. Confabulations: a conceptual history. J. Hist. Neurosci. 7 , 225–241 (1998).
Article CAS PubMed Google Scholar
Lin, S., Hilton, J. & Evans, O. Teaching models to express their uncertainty in words. Transact. Mach. Learn. Res. (2022).
Evans, O. et al. Truthful AI: developing and governing AI that does not lie. Preprint at https://arxiv.org/abs/2110.06674 (2021).
Amodei, D. et al. Concrete problems in AI safety. Preprint at https://arxiv.org/abs/1606.06565 (2016).
Jiang, Z., Araki, J., Ding, H. & Neubig, G. How can we know when language models know? On the calibration of language models for question answering. Transact. Assoc. Comput. Linguist. 9 , 962–977 (2021).
Article Google Scholar
Desai, S. & Durrett, G. Calibration of pre-trained transformers. In Proc. 2020 Conference on Empirical Methods in Natural Language Processing (EMNLP) (eds Webber, B., Cohn, T., He, Y. & Liu, Y.) 295–302 (Association for Computational Linguistics, 2020).
Glushkova, T., Zerva, C., Rei, R. & Martins, A. F. Uncertainty-aware machine translation evaluation. In Findings of the Association for Computational Linguistics: EMNLP 2021 (eds Moens, M-F., Huang, X., Specia, L. & Yih, S.) 3920–3938 (Association for Computational Linguistics, 2021).
Wang, Y., Beck, D., Baldwin, T. & Verspoor, K. Uncertainty estimation and reduction of pre-trained models for text regression. Transact. Assoc. Comput. Linguist. 10 , 680–696 (2022).
Baker, S. & Kanade, T. Hallucinating faces. In Proc. Fourth IEEE International Conference on Automatic Face and Gesture Recognition . 83–88 (IEEE, Catalogue no PR00580, 2002).
Eliot, L. AI ethics lucidly questioning this whole hallucinating AI popularized trend that has got to stop. Forbes Magazine (24 August 2022).
Shanahan, M. Talking about large language models. Commun. Assoc. Comp. Machinery 67 , 68–79 (2024).
MacKay, D. J. C. Information-based objective functions for active data selection. Neural Comput. 4 , 590–604 (1992).
Kadavath, S. et al. Language models (mostly) know what they know. Preprint at https://arxiv.org/abs/2207.05221 (2022).
Lindley, D. V. On a measure of the information provided by an experiment. Ann. Math. Stat. 27 , 986–1005 (1956).
Article MathSciNet Google Scholar
Xiao, T. Z., Gomez, A. N. & Gal, Y. Wat zei je? Detecting out-of-distribution translations with variational transformers. In Workshop on Bayesian Deep Learning at the Conference on Neural Information Processing Systems (NeurIPS, Vancouver, 2019).
Christiano, P., Cotra, A. & Xu, M. Eliciting Latent Knowledge (Alignment Research Center, 2021); https://docs.google.com/document/d/1WwsnJQstPq91_Yh-Ch2XRL8H_EpsnjrC1dwZXR37PC8/edit .
Negri, M., Bentivogli, L., Mehdad, Y., Giampiccolo, D. & Marchetti, A. Divide and conquer: crowdsourcing the creation of cross-lingual textual entailment corpora. In Proc. 2011 Conference on Empirical Methods in Natural Language Processing 670–679 (Association for Computational Linguistics, 2011).
Honovich, O. et al. TRUE: Re-evaluating factual consistency evaluation. In Proc. Second DialDoc Workshop on Document-grounded Dialogue and Conversational Question Answering 161–175 (Association for Computational Linguistics, 2022).
Falke, T., Ribeiro, L. F. R., Utama, P. A., Dagan, I. & Gurevych, I. Ranking generated summaries by correctness: an interesting but challenging application for natural language inference. In Proc. 57th Annual Meeting of the Association for Computational Linguistics 2214–2220 (Association for Computational Linguistics, 2019).
Laban, P., Schnabel, T., Bennett, P. N. & Hearst, M. A. SummaC: re-visiting NLI-based models for inconsistency detection in summarization. Trans. Assoc. Comput. Linguist. 10 , 163–177 (2022).
Joshi, M., Choi, E., Weld, D. S. & Zettlemoyer, L. TriviaQA: a large scale distantly supervised challenge dataset for reading comprehension. In Proc. 55th Annual Meeting of the Association for Computational Linguistics 1601–1611 (Association for Computational Linguistics. 2017).
Rajpurkar, P., Zhang, J., Lopyrev, K. & Liang, P. SQuAD: 100,000+ questions for machine compression of text. In Proc. 2016 Conference on Empirical Methods in Natural Language Processing (eds Su, J., Duh, K. & Carreras, X.) 2383–2392 (Association for Computational Linguistics, 2016).
Tsatsaronis, G. et al. An overview of the BIOASQ large-scale biomedical semantic indexing and question answering competition. BMC Bioinformatics 16 , 138 (2015).
Article PubMed PubMed Central Google Scholar
Lee, K., Chang, M.-W. & Toutanova, K. Latent retrieval for weakly supervised open domain question answering. In Proc. 57th Annual Meeting of the Association for Computational Linguistics 6086–6096 (Association for Computational Linguistics, 2019).
Kwiatkowski, T. et al. Natural questions: a benchmark for question answering research. Transact. Assoc. Comput. Linguist. 7 , 452–466 (2019).
Patel, A., Bhattamishra, S. & Goyal, N. Are NLP models really able to solve simple math word problems? In Proc. 2021 Conference of the North American Chapter of the Association for Computational Linguistics: Human Language Technologies (eds Toutanova, K. et al.) 2080–2094 (Assoc. Comp. Linguistics, 2021).
Touvron, H. et al. Llama 2: open foundation and fine-tuned chat models. Preprint at https://arxiv.org/abs/2307.09288 (2023).
Penedo, G. et al. The RefinedWeb dataset for Falcon LLM: outperforming curated corpora with web data, and web data only. In Proc. 36th Conference on Neural Information Processing Systems (eds Oh, A. et al.) 79155–79172 (Curran Associates, 2023)
Jiang, A. Q. et al. Mistral 7B. Preprint at https://arxiv.org/abs/2310.06825 (2023).
Manakul, P., Liusie, A. & Gales, M. J. F. SelfCheckGPT: Zero-Resource Black-Box hallucination detection for generative large language models. In Findings of the Association for Computational Linguistics: EMNLP 2023 (eds Bouamor, H., Pino, J. & Bali, K.) 9004–9017 (Assoc. Comp. Linguistics, 2023).
Mukhoti, J., Kirsch, A., van Amersfoort, J., Torr, P. H. & Gal, Y. Deep deterministic uncertainty: a new simple baseline. In IEEE/CVF Conference on Computer Vision and Pattern Recognition 24384–24394 (Computer Vision Foundation, 2023).
Schuster, T., Chen, S., Buthpitiya, S., Fabrikant, A. & Metzler, D. Stretching sentence-pair NLI models to reason over long documents and clusters. In Findings of the Association for Computational Linguistics: EMNLP 2022 (eds Goldberg, Y. et al.) 394–412 (Association for Computational Linguistics, 2022).
Barnes, B. & Christiano, P. Progress on AI Safety via Debate. AI Alignment Forum www.alignmentforum.org/posts/Br4xDbYu4Frwrb64a/writeup-progress-on-ai-safety-via-debate-1 (2020).
Irving, G., Christiano, P. & Amodei, D. AI safety via debate. Preprint at https://arxiv.org/abs/1805.00899 (2018).
Der Kiureghian, A. & Ditlevsen, O. Aleatory or epistemic? Does it matter? Struct. Saf. 31 , 105–112 (2009).
Malinin, A. & Gales, M. Uncertainty estimation in autoregressive structured prediction. In Proceedings of the International Conference on Learning Representations https://openreview.net/forum?id=jN5y-zb5Q7m (2021).
Murray, K. & Chiang, D. Correcting length bias in neural machine translation. In Proc. Third Conference on Machine Translation (eds Bojar, O. et al.) 212–223 (Assoc. Comp. Linguistics, 2018).
Holtzman, A., Buys, J., Du, L., Forbes, M. & Choi, Y. The curious case of neural text degeneration. In Proceedings of the International Conference on Learning Representations https://openreview.net/forum?id=rygGQyrFvH (2020).
Fan, A., Lewis, M. & Dauphin, Y. Hierarchical neural story generation. In Proc. 56th Annual Meeting of the Association for Computational Linguistics (eds Gurevych, I. & Miyao, Y.) 889–898 (Association for Computational Linguistics, 2018).
Speaks, J. in The Stanford Encyclopedia of Philosophy (ed. Zalta, E. N.) (Metaphysics Research Lab, Stanford Univ., 2021).
Culicover, P. W. Paraphrase generation and information retrieval from stored text. Mech. Transl. Comput. Linguist. 11 , 78–88 (1968).
Google Scholar
Padó, S., Cer, D., Galley, M., Jurafsky, D. & Manning, C. D. Measuring machine translation quality as semantic equivalence: a metric based on entailment features. Mach. Transl. 23 , 181–193 (2009).
Androutsopoulos, I. & Malakasiotis, P. A survey of paraphrasing and textual entailment methods. J. Artif. Intell. Res. 38 , 135–187 (2010).
MacCartney, B. Natural Language Inference (Stanford Univ., 2009).
He, P., Liu, X., Gao, J. & Chen, W. Deberta: decoding-enhanced BERT with disentangled attention. In International Conference on Learning Representations https://openreview.net/forum?id=XPZIaotutsD (2021).
Brown, T. et al. Language models are few-shot learners. Adv. Neural Inf. Process. Syst. 33 , 1877–1901 (2020).
Williams, A., Nangia, N. & Bowman, S. R. A broad-coverage challenge corpus for sentence understanding through inference. In Proc. 2018 Conference of the North American Chapter of the Association for Computational Linguistics: Human Language Technologies (eds Walker, M. et al.) 1112–1122 (Assoc. Comp. Linguistics, 2018).
Yu, L., Hermann, K. M., Blunsom, P. & Pulman, S. Deep learning for answer sentence selection. Preprint at https://arxiv.org/abs/1412.1632 (2014).
Socher, R., Huang, E., Pennin, J., Manning, C. D. & Ng, A. Dynamic pooling and unfolding recursive autoencoders for paraphrase detection. In Proceedings of the 24th Conference on Neural Information Processing Systems (eds Shawe-Taylor, J. et al.) (2011)
He, R., Ravula, A., Kanagal, B. & Ainslie, J. Realformer: Transformer likes residual attention. In Findings of the Association for Computational Linguistics: ACL-IJCNLP 2021 (eds Zhong, C., et al.) 929–943 (Assoc. Comp. Linguistics, 2021).
Tay, Y. et al. Charformer: fast character transformers via gradient-based subword tokenization. In Proceedings of the International Conference on Learning Representations https://openreview.net/forum?id=JtBRnrlOEFN (2022).
Kane, H., Kocyigit, Y., Abdalla, A., Ajanoh, P. & Coulibali, M. Towards neural similarity evaluators. In Workshop on Document Intelligence at the 32nd conference on Neural Information Processing (2019).
Lebret, R., Grangier, D. & Auli, M. Neural text generation from structured data with application to the biography domain. In Proc. 2016 Conference on Empirical Methods in Natural Language Processing (eds Su, J. et al.) 1203–1213 (Association for Computational Linguistics, 2016).
Kossen, J., jlko/semantic_uncertainty: Initial release v.1.0.0. Zenodo https://doi.org/10.5281/zenodo.10964366 (2024).
Download references
Acknowledgements
We thank G. Irving, K. Perlin, J. Richens, L. Rimell and M. Turpin for their comments or discussion related to this work. We thank K. Handa for his help with the human evaluation of our automated accuracy assessment. We thank F. Bickford Smith and L. Melo for their code review. Y.G. is supported by a Turing AI Fellowship funded by the UK government’s Office for AI, through UK Research and Innovation (grant reference EP/V030302/1), and delivered by the Alan Turing Institute.
Author information
These authors contributed equally: Sebastian Farquhar, Jannik Kossen, Lorenz Kuhn
Authors and Affiliations
OATML, Department of Computer Science, University of Oxford, Oxford, UK
Sebastian Farquhar, Jannik Kossen, Lorenz Kuhn & Yarin Gal
You can also search for this author in PubMed Google Scholar
Contributions
S.F. led the work from conception to completion and proposed using bidirectional entailment to cluster generations as a way of computing entropy in LLMs. He wrote the main text, most of the Methods and Supplementary Information and prepared most of the figures. J.K. improved the mathematical formalization of semantic entropy; led the extension of semantic entropy to sentence- and paragraph-length generations; wrote the code for, and carried out, all the experiments and evaluations; wrote much of the Methods and Supplementary Information and prepared drafts of many figures; and gave critical feedback on the main text. L.K. developed the initial mathematical formalization of semantic entropy; wrote code for, and carried out, the initial experiments around semantic entropy and its variants which demonstrated the promise of the idea and helped narrow down possible research avenues to explore; and gave critical feedback on the main text. Y.G. ideated the project, proposing the idea to differentiate semantic and syntactic diversity as a tool for detecting hallucinations, provided high-level guidance on the research and gave critical feedback on the main text; he runs the research laboratory in which the work was carried out.
Corresponding author
Correspondence to Sebastian Farquhar .
Ethics declarations
Competing interests.
S.F. is currently employed by Google DeepMind and L.K. by OpenAI. For both, this paper was written under their University of Oxford affiliation. The remaining authors declare no competing interests.
Peer review
Peer review information.
Nature thanks Mirella Lapata and the other, anonymous, reviewer(s) for their contribution to the peer review of this work.
Additional information
Publisher’s note Springer Nature remains neutral with regard to jurisdictional claims in published maps and institutional affiliations.
Extended data figures and tables
Extended data fig. 1 algorithm outline for bidirectional entailment clustering..
Given a set of outputs in response to a context, the bidirectional entailment answer returns a set of sets of outputs which have been classified as sharing a meaning.
Supplementary information
Supplementary information.
Supplementary Notes 1–7, Figs. 1–10, Tables 1–4 and references. Includes, worked example for semantic entropy calculation, discussion of limitations and computational cost of entailment clustering, ablation of entailment prediction and clustering methods, discussion of automated accuracy assessment, unaggregated results for sentence-length generations and further results for short-phrase generations.
Rights and permissions
Open Access This article is licensed under a Creative Commons Attribution 4.0 International License, which permits use, sharing, adaptation, distribution and reproduction in any medium or format, as long as you give appropriate credit to the original author(s) and the source, provide a link to the Creative Commons licence, and indicate if changes were made. The images or other third party material in this article are included in the article’s Creative Commons licence, unless indicated otherwise in a credit line to the material. If material is not included in the article’s Creative Commons licence and your intended use is not permitted by statutory regulation or exceeds the permitted use, you will need to obtain permission directly from the copyright holder. To view a copy of this licence, visit http://creativecommons.org/licenses/by/4.0/ .
Reprints and permissions
About this article
Cite this article.
Farquhar, S., Kossen, J., Kuhn, L. et al. Detecting hallucinations in large language models using semantic entropy. Nature 630 , 625–630 (2024). https://doi.org/10.1038/s41586-024-07421-0
Download citation
Received : 17 July 2023
Accepted : 12 April 2024
Published : 19 June 2024
Issue Date : 20 June 2024
DOI : https://doi.org/10.1038/s41586-024-07421-0
Share this article
Anyone you share the following link with will be able to read this content:
Sorry, a shareable link is not currently available for this article.
Provided by the Springer Nature SharedIt content-sharing initiative
By submitting a comment you agree to abide by our Terms and Community Guidelines . If you find something abusive or that does not comply with our terms or guidelines please flag it as inappropriate.
Quick links
- Explore articles by subject
- Guide to authors
- Editorial policies
Sign up for the Nature Briefing: AI and Robotics newsletter — what matters in AI and robotics research, free to your inbox weekly.

- IEEE CS Standards
- Career Center
- Subscribe to Newsletter
- IEEE Standards
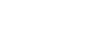
- For Industry Professionals
- For Students
- Launch a New Career
- Membership FAQ
- Membership FAQs
- Membership Grades
- Special Circumstances
- Discounts & Payments
- Distinguished Contributor Recognition
- Grant Programs
- Find a Local Chapter
- Find a Distinguished Visitor
- About Distinguished Visitors Program
- Find a Speaker on Early Career Topics
- Technical Communities
- Collabratec (Discussion Forum)
- My Subscriptions
- My Referrals
- Computer Magazine
- ComputingEdge Magazine
- Let us help make your event a success. EXPLORE PLANNING SERVICES
- Events Calendar
- Calls for Papers
- Conference Proceedings
- Conference Highlights
- Top 2024 Conferences
- Conference Sponsorship Options
- Conference Planning Services
- Conference Organizer Resources
- Virtual Conference Guide
- Get a Quote
- CPS Dashboard
- CPS Author FAQ
- CPS Organizer FAQ
- Find the latest in advanced computing research. VISIT THE DIGITAL LIBRARY
- Open Access
- Tech News Blog
- Author Guidelines
- Reviewer Information
- Guest Editor Information
- Editor Information
- Editor-in-Chief Information
- Volunteer Opportunities
- Video Library
- Member Benefits
- Institutional Library Subscriptions
- Advertising and Sponsorship
- Code of Ethics
- Educational Webinars
- Online Education
- Certifications
- Industry Webinars & Whitepapers
- Research Reports
- Bodies of Knowledge
- CS for Industry Professionals
- Resource Library
- Newsletters
- Women in Computing
- Digital Library Access
- Organize a Conference
- Run a Publication
- Become a Distinguished Speaker
- Participate in Standards Activities
- Peer Review Content
- Author Resources
- Publish Open Access
- Society Leadership
- Boards & Committees
- Local Chapters
- Governance Resources
- Conference Publishing Services
- Chapter Resources
- About the Board of Governors
- Board of Governors Members
- Diversity & Inclusion
- Open Volunteer Opportunities
- Award Recipients
- Student Scholarships & Awards
- Nominate an Election Candidate
- Nominate a Colleague
- Corporate Partnerships
- Conference Sponsorships & Exhibits
- Advertising
- Recruitment
- Publications
- Education & Career
CVPR 2024 Announces Best Paper Award Winners
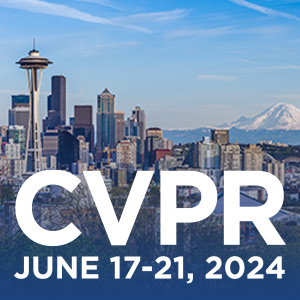
This year, from more than 11,500 paper submissions, the CVPR 2024 Awards Committee selected the following 10 winners for the honor of Best Papers during the Awards Program at CVPR 2024, taking place now through 21 June at the Seattle Convention Center in Seattle, Wash., U.S.A.
Best Papers
- “ Generative Image Dynamics ” Authors: Zhengqi Li, Richard Tucker, Noah Snavely, Aleksander Holynski The paper presents a new approach for modeling natural oscillation dynamics from a single still picture. This approach produces photo-realistic animations from a single picture and significantly outperforms prior baselines. It also demonstrates potential to enable several downstream applications such as creating seamlessly looping or interactive image dynamics.
- “ Rich Human Feedback for Text-to-Image Generation ” Authors: Youwei Liang, Junfeng He, Gang Li, Peizhao Li, Arseniy Klimovskiy, Nicholas Carolan, Jiao Sun, Jordi Pont-Tuset, Sarah Young, Feng Yang, Junjie Ke, Krishnamurthy Dj Dvijotham, Katherine M. Collins, Yiwen Luo, Yang Li, Kai J. Kohlhoff, Deepak Ramachandran, and Vidhya Navalpakkam This paper highlights the first rich human feedback dataset for image generation. Authors designed and trained a multimodal Transformer to predict the rich human feedback and demonstrated some instances to improve image generation.
Honorable mention papers included, “ EventPS: Real-Time Photometric Stereo Using an Event Camera ” and “ pixelSplat: 3D Gaussian Splats from Image Pairs for Scalable Generalizable 3D Reconstruction. ”
Best Student Papers
- “ Mip-Splatting: Alias-free 3D Gaussian Splatting ” Authors: Zehao Yu, Anpei Chen, Binbin Huang, Torsten Sattler, Andreas Geiger This paper introduces Mip-Splatting, a technique improving 3D Gaussian Splatting (3DGS) with a 3D smoothing filter and a 2D Mip filter for alias-free rendering at any scale. This approach significantly outperforms state-of-the-art methods in out-of-distribution scenarios, when testing at sampling rates different from training, resulting in better generalization to out-of-distribution camera poses and zoom factors.
- “ BioCLIP: A Vision Foundation Model for the Tree of Life ” Authors: Samuel Stevens, Jiaman Wu, Matthew J. Thompson, Elizabeth G. Campolongo, Chan Hee Song, David Edward Carlyn, Li Dong, Wasila M. Dahdul, Charles Stewart, Tanya Berger-Wolf, Wei-Lun Chao, and Yu Su This paper offers TREEOFLIFE-10M and BIOCLIP, a large-scale diverse biology image dataset and a foundation model for the tree of life, respectively. This work shows BIOCLIP is a strong fine-grained classifier for biology in both zero- and few-shot settings.
There also were four honorable mentions in this category this year: “ SpiderMatch: 3D Shape Matching with Global Optimality and Geometric Consistency ”; “ Image Processing GNN: Breaking Rigidity in Super-Resolution; Objects as Volumes: A Stochastic Geometry View of Opaque Solids ;” and “ Comparing the Decision-Making Mechanisms by Transformers and CNNs via Explanation Methods. ”
“We are honored to recognize the CVPR 2024 Best Paper Awards winners,” said David Crandall, Professor of Computer Science at Indiana University, Bloomington, Ind., U.S.A., and CVPR 2024 Program Co-Chair. “The 10 papers selected this year – double the number awarded in 2023 – are a testament to the continued growth of CVPR and the field, and to all of the advances that await.”
Additionally, the IEEE Computer Society (CS), a CVPR organizing sponsor, announced the Technical Community on Pattern Analysis and Machine Intelligence (TCPAMI) Awards at this year’s conference. The following were recognized for their achievements:
- 2024 Recipient : “ Rich Feature Hierarchies for Accurate Object Detection and Semantic Segmentation ” Authors: Ross Girshick, Jeff Donahue, Trevor Darrell, Jitendra Malik
- 2024 Recipient : Angjoo Kanazawa, Carl Vondrick
- 2024 Recipient : Andrea Vedaldi
“The TCPAMI Awards demonstrate the lasting impact and influence of CVPR research and researchers,” said Walter J. Scheirer, University of Notre Dame, Notre Dame, Ind., U.S.A., and CVPR 2024 General Chair. “The contributions of these leaders have helped to shape and drive forward continued advancements in the field. We are proud to recognize these achievements and congratulate them on their success.”
About the CVPR 2024 The Computer Vision and Pattern Recognition Conference (CVPR) is the preeminent computer vision event for new research in support of artificial intelligence (AI), machine learning (ML), augmented, virtual and mixed reality (AR/VR/MR), deep learning, and much more. Sponsored by the IEEE Computer Society (CS) and the Computer Vision Foundation (CVF), CVPR delivers the important advances in all areas of computer vision and pattern recognition and the various fields and industries they impact. With a first-in-class technical program, including tutorials and workshops, a leading-edge expo, and robust networking opportunities, CVPR, which is annually attended by more than 10,000 scientists and engineers, creates a one-of-a-kind opportunity for networking, recruiting, inspiration, and motivation.
CVPR 2024 takes place 17-21 June at the Seattle Convention Center in Seattle, Wash., U.S.A., and participants may also access sessions virtually. For more information about CVPR 2024, visit cvpr.thecvf.com .
About the Computer Vision Foundation The Computer Vision Foundation (CVF) is a non-profit organization whose purpose is to foster and support research on all aspects of computer vision. Together with the IEEE Computer Society, it co-sponsors the two largest computer vision conferences, CVPR and the International Conference on Computer Vision (ICCV). Visit thecvf.com for more information.
About the IEEE Computer Society Engaging computer engineers, scientists, academia, and industry professionals from all areas and levels of computing, the IEEE Computer Society (CS) serves as the world’s largest and most established professional organization of its type. IEEE CS sets the standard for the education and engagement that fuels continued global technological advancement. Through conferences, publications, and programs that inspire dialogue, debate, and collaboration, IEEE CS empowers, shapes, and guides the future of not only its 375,000+ community members, but the greater industry, enabling new opportunities to better serve our world. Visit computer.org for more information.
Recommended by IEEE Computer Society
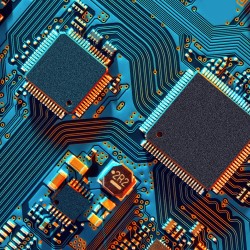
The IEEE International Roadmap for Devices and Systems (IRDS) Emerges as a Global Leader for Chips Acts Visions and Programs
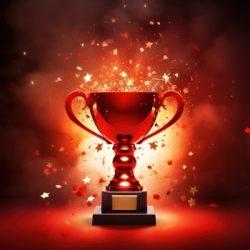
IEEE Computer Society Announces 2024 Class of Fellow
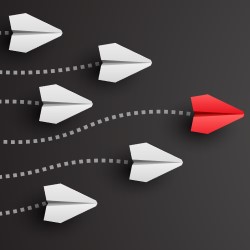
IEEE CS Releases 20 in their 20s List, Identifying Emerging Leaders in Computer Science and Engineering
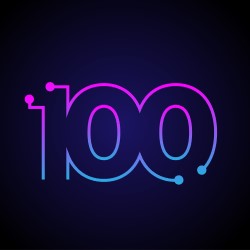
IEEE CS Authors, Speakers, and Leaders Named to Inaugural TIME100 Most Influential People in Artificial Intelligence List
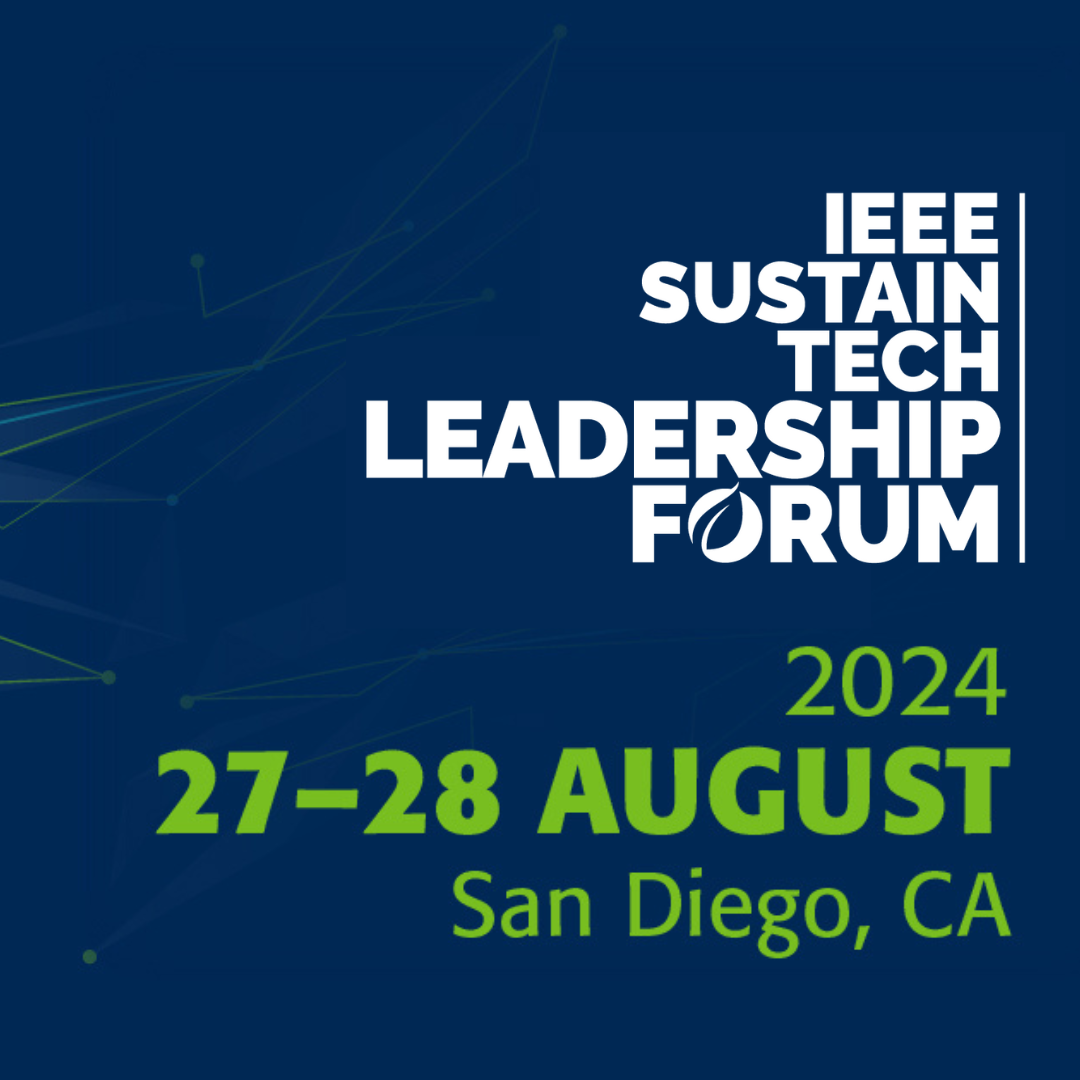
IEEE SustainTech Leadership Forum 2024: Unlocking the Future of Sustainable Technology for Buildings and Factories in the Built Environment
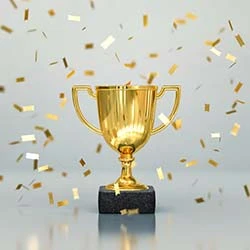
J. Gregory Pauloski and Rohan Basu Roy Named Recipients of 2023 ACM/IEEE CS George Michael Memorial HPC Fellowships
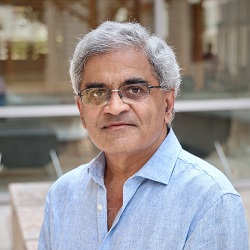
Keshav Pingali Selected to Receive ACM-IEEE CS Ken Kennedy Award
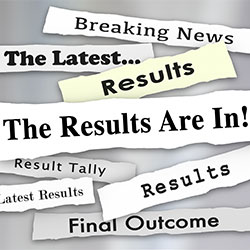
Hironori Washizaki Elected IEEE Computer Society 2025 President
Main Navigation
- Contact NeurIPS
- Code of Ethics
- Code of Conduct
- Create Profile
- Journal To Conference Track
- Diversity & Inclusion
- Proceedings
- Future Meetings
- Exhibitor Information
- Privacy Policy
NeurIPS 2024, the Thirty-eighth Annual Conference on Neural Information Processing Systems, will be held at the Vancouver Convention Center
Monday Dec 9 through Sunday Dec 15. Monday is an industry expo.

Registration
Pricing » Registration 2024 Registration Cancellation Policy » Certificate of Attendance
Our Hotel Reservation page is currently under construction and will be released shortly. NeurIPS has contracted Hotel guest rooms for the Conference at group pricing, requiring reservations only through this page. Please do not make room reservations through any other channel, as it only impedes us from putting on the best Conference for you. We thank you for your assistance in helping us protect the NeurIPS conference.
Announcements
- The call for High School Projects has been released
- The Call For Papers has been released
- See the Visa Information page for changes to the visa process for 2024.
Latest NeurIPS Blog Entries [ All Entries ]
Jun 19, 2024 | |
Jun 04, 2024 | |
May 17, 2024 | |
May 07, 2024 | |
Apr 17, 2024 | |
Apr 15, 2024 | |
Mar 03, 2024 | |
Dec 11, 2023 | |
Dec 10, 2023 | |
Dec 09, 2023 |
Important Dates
Mar 15 '24 11:46 AM PDT * | ||
Apr 05 '24 (Anywhere on Earth) | ||
Apr 21 '24 (Anywhere on Earth) | ||
Main Conference Paper Submission Deadline | May 22 '24 01:00 PM PDT * | |
May 22 '24 01:00 PM PDT * | ||
Jun 14 '24 (Anywhere on Earth) | ||
Jun 27 '24 01:00 PM PDT * | ||
Aug 02 '24 06:00 PM PDT * | ||
Sep 05 '24 (Anywhere on Earth) | ||
Main Conference Author Notification | Sep 25 '24 06:00 PM PDT * | |
Datasets and Benchmarks - Author Notification | Sep 26 '24 (Anywhere on Earth) | |
Workshop Accept/Reject Notification Date | Sep 29 '24 (Anywhere on Earth) | |
Oct 30 '24 (Anywhere on Earth) | ||
Nov 15 '24 11:00 PM PST * | ||
Timezone: |
If you have questions about supporting the conference, please contact us .
View NeurIPS 2024 exhibitors » Become an 2024 Exhibitor Exhibitor Info »
Organizing Committee
General chair, program chair, workshop chair, workshop chair assistant, tutorial chair, competition chair, data and benchmark chair, affinity chair, diversity, inclusion and accessibility chair, ethics review chair, communication chair, social chair, journal chair, creative ai chair, workflow manager, logistics and it, mission statement.
The Neural Information Processing Systems Foundation is a non-profit corporation whose purpose is to foster the exchange of research advances in Artificial Intelligence and Machine Learning, principally by hosting an annual interdisciplinary academic conference with the highest ethical standards for a diverse and inclusive community.
About the Conference
The conference was founded in 1987 and is now a multi-track interdisciplinary annual meeting that includes invited talks, demonstrations, symposia, and oral and poster presentations of refereed papers. Along with the conference is a professional exposition focusing on machine learning in practice, a series of tutorials, and topical workshops that provide a less formal setting for the exchange of ideas.
More about the Neural Information Processing Systems foundation »
NeurIPS uses cookies to remember that you are logged in. By using our websites, you agree to the placement of cookies. |
share this!
June 24, 2024 report
This article has been reviewed according to Science X's editorial process and policies . Editors have highlighted the following attributes while ensuring the content's credibility:
fact-checked
peer-reviewed publication
trusted source
Analysis of data suggests homosexual behavior in other animals is far more common than previously thought
by Bob Yirka , Phys.org
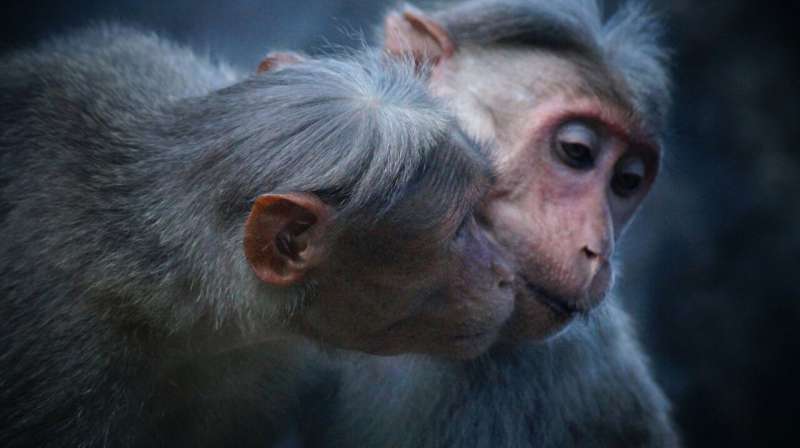
A team of anthropologists and biologists from Canada, Poland, and the U.S., working with researchers at the American Museum of Natural History, in New York, has found via meta-analysis of data from prior research efforts that homosexual behavior is far more common in other animals than previously thought. The paper is published in PLOS ONE .
For many years, the biology community has accepted the notion that homosexuality is less common in animals than in humans, despite a lack of research on the topic. In this new effort, the researchers sought to find out if such assumptions are true.
The work involved study of 65 studies into the behavior of multiple species of animals, mostly mammals, such as elephants, squirrels, monkeys, rats and racoons.
The researchers found that 76% of the studies mentioned observations of homosexual behavior, though they also noted that only 46% had collected data surrounding such behavior—and only 18.5% of those who had mentioned such behavior in their papers had focused their efforts on it to the extent of publishing work with homosexuality as it core topic.
They noted that homosexual behavior observed in other species included mounting, intromission and oral contact—and that researchers who identified as LGBTQ+ were no more or less likely to study the topic than other researchers.
The researchers point to a hesitancy in the biological community to study homosexuality in other species , and thus, little research has been conducted. They further suggest that some of the reluctance has been due to the belief that such behavior is too rare to warrant further study.
The research team suggests that homosexuality is far more common in the animal kingdom than has been reported—they further suggest more work is required regarding homosexual behaviors in other animals to dispel the myth of rarity.
Journal information: PLoS ONE
© 2024 Science X Network
Explore further
Feedback to editors

The Milky Way's eROSITA bubbles are large and distant
Jun 29, 2024

Saturday Citations: Armadillos are everywhere; Neanderthals still surprising anthropologists; kids are egalitarian

NASA astronauts will stay at the space station longer for more troubleshooting of Boeing capsule

The beginnings of fashion: Paleolithic eyed needles and the evolution of dress
Jun 28, 2024

Analysis of NASA InSight data suggests Mars hit by meteoroids more often than thought

New computational microscopy technique provides more direct route to crisp images

A harmless asteroid will whiz past Earth Saturday. Here's how to spot it

Tiny bright objects discovered at dawn of universe baffle scientists

New method for generating monochromatic light in storage rings

Soft, stretchy electrode simulates touch sensations using electrical signals
Relevant physicsforums posts, who chooses official designations for individual dolphins, such as fb15, f153, f286.
Jun 26, 2024
Color Recognition: What we see vs animals with a larger color range
Jun 25, 2024
Innovative ideas and technologies to help folks with disabilities
Jun 24, 2024
Is meat broth really nutritious?
Covid virus lives longer with higher co2 in the air.
Jun 22, 2024
Periodical Cicada Life Cycle
Jun 21, 2024
More from Biology and Medical
Related Stories

How, and why, did homosexual behavior evolve in humans and other animals?
Oct 12, 2023

Male rhesus macaques often have sex with each other, a trait they have inherited in part from their parents
Jul 15, 2023

Same-gender sexual behavior found to be widespread across mammal species and to have multiple origins
Oct 4, 2023

Stop calling it a choice: Biological factors drive homosexuality
Sep 4, 2019
Clinicians' personal religious beliefs may impact treatment provided to patients who are homosexual
Oct 23, 2017

Study shows same-sex sexual behavior is widespread and heritable in macaque monkeys
Jul 10, 2023
Recommended for you

Do vertebrate populations really decline so much? Calculations indicating severe declines might be wrong, says study
Jun 27, 2024

Under pressure: How comb jellies have adapted to life at the bottom of the ocean

Predicting chronic wasting disease in counties could prevent spread

Three new extinct walnut species discovered in high Arctic mummified forest

Unlocking biodiversity insights from the tropical Andes

Pacific cod can't rely on coastal safe havens for protection during marine heat waves, study finds
Let us know if there is a problem with our content.
Use this form if you have come across a typo, inaccuracy or would like to send an edit request for the content on this page. For general inquiries, please use our contact form . For general feedback, use the public comments section below (please adhere to guidelines ).
Please select the most appropriate category to facilitate processing of your request
Thank you for taking time to provide your feedback to the editors.
Your feedback is important to us. However, we do not guarantee individual replies due to the high volume of messages.
E-mail the story
Your email address is used only to let the recipient know who sent the email. Neither your address nor the recipient's address will be used for any other purpose. The information you enter will appear in your e-mail message and is not retained by Phys.org in any form.
Newsletter sign up
Get weekly and/or daily updates delivered to your inbox. You can unsubscribe at any time and we'll never share your details to third parties.
More information Privacy policy
Donate and enjoy an ad-free experience
We keep our content available to everyone. Consider supporting Science X's mission by getting a premium account.
E-mail newsletter
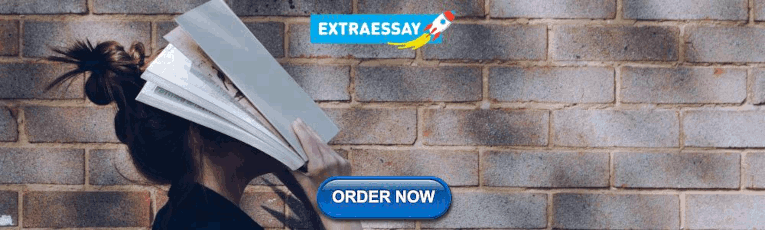
IMAGES
VIDEO
COMMENTS
The articles in this special section are dedicated to the application of artificial intelligence AI), machine learning (ML), and data analytics to address different problems of communication systems, presenting new trends, approaches, methods, frameworks, systems for efficiently managing and optimizing networks related operations. Even though AI/ML is considered a key technology for next ...
This paper illustrates What is Data Science, How it processes, and also its Applications. Section II of this paper consists of the different review regarding data science. Section III of this paper illustrates about the complete process of data science. Section IV describes all the related research issues for data science.
This paper seeks to explain the different aspects of data science related to modern needs and future significance. Data science is explored in detail with emphasis on the background, history, and concepts of data management. ... Date Added to IEEE Xplore: 05 July 2021 ISBN Information: Electronic ISBN: 978-1-6654-0428- Print ...
Read all the papers in 2021 IEEE 8th International Conference on Data Science and Advanced Analytics (DSAA) | IEEE Conference | IEEE Xplore
Data Science has proved to be one of the sexiest jobs of 21st Century where humans more rely on the observations or results calculated by the computers and humans can focus more on creativity and to implement the strategies on how to solve the real-world problems. Data Science is extremely used in E-healthcare and has been proved for predicting the diseases and curing them at a primary stage ...
Data Science study utilized for gathering information as data, taking out data from other systems, accumulating information, signifying and safeguarding data collected are used by organizations for marketing purposes and in high-tech implementations. Name of data science denotes a combination of databases and software engineering as well as a number of types of qualitative, quantitative ...
AI systems cannot exist without data. Now that AI models (data science and AI) have matured and are readily available to apply in practice, most organizations struggle with the data infrastructure to do so. There is a growing need for data engineers that know how to prepare data for AI systems or that can setup enterprise-wide data architectures for analytical projects. But until now, the data ...
This paper explores some of the challenges related to developing a "data science" approach in the research university setting. Despite these hurdles, with improved data governance and availability, a team with the right skills and outlook, and the support of senior leadership, the transition from a more traditional institutional research function to one representing a data science perspective ...
Data Science. Knowledge Discovery. Neuroinformatics. OCEANS 2024 - Halifax. IGARSS 2025 - 2025 IEEE International Geoscience and Remote Sensing Symposium. 2023 IEEE International Solid- State Circuits Conference (ISSCC) 2023 IEEE Applied Power Electronics Conference and Exposition (APEC)
data science research activities, along the implications of dif-ferent methods for executing industry and business projects. At present, data science is a young field and conveys the impres-Preprint submitted to Big Data Research - Elsevier January 6, 2020 arXiv:2106.07287v2 [cs.LG] 14 Jan 2022
In this paper, an intelligent identification method for rail vehicle running state is proposed based on Tiny Machine Learning (TinyML) technology, and an IoT system is developed with small size and low energy consumption. The system uses a Micro-Electro-Mechanical System (MEMS) sensor to collect acceleration data for machine learning training.
Publications. IEEE Talks Big Data - Check out our new Q&A article series with big Data experts!. Call for Papers - Check out the many opportunities to submit your own paper. This is a great way to get published, and to share your research in a leading IEEE magazine! Publications - See the list of various IEEE publications related to big data and analytics here.
Assessing the effects of fuel energy consumption, foreign direct investment and GDP on CO2 emission: New data science evidence from Europe & Central Asia. Fuel . 10.1016/j.fuel.2021.123098 . 2022 . Vol 314 . pp. 123098. Author (s): Muhammad Mohsin . Sobia Naseem .
All the latest data science news, videos, and more from the world's leading engineering magazine. data science - IEEE Spectrum IEEE.org IEEE Xplore Digital Library IEEE Standards More Sites
These journals are significant additions to IEEE's well-known and respected portfolio of fully open access journals. In addition, many of the journals featured here target an accelerated publication time frame of 10 weeks for most accepted papers to help get your research exposed faster. Visit the publication home page of each title for details.
For details, Call: 9886692401/9845166723. DHS Informatics providing latest 2024-2025 IEEE projects on Data science for the final year engineering students. DHS Informatics trains all students to develop their project with good idea what they need to submit in college to get good marks. DHS Informatics offers placement training in Bangalore and ...
In the 21st century, emerging fields in computer science are Data Science & Machine Learning. Data Science analyse the given data using statistical analysis and identifies hidden patterns among ...
Fig 4. Sensitivity Analysis of hybrid setup - "Data Science Applications in Renewable Energy: Leveraging Big Data for Sustainable Solutions" ... The research paper provides a number of case studies and examples of real-world applications of data-driven approaches in the field of renewable energy, showing how smart analysis of big data can ...
The promise of modern data science to advance research has generated palpable excitement in the scientific ... (2019). Software engineering for machine learning: A case study. In Proceedings - 2019 IEEE/ACM 41st . Harvard Data Science Review • Issue 6.2, Spring 2024 In the Academy, Data Science Is Lonely: Barriers to Adopting Data ...
DATA SCIENCE-2020-RESEARCH TECHNOLOGIES IEEE PROJECTS PAPERS . ENGPAPER.COM - IEEE PAPER. CSE ECE EEE IEEE PROJECT. ... The data science tools for research of emigratio n processes in Ukraine free download The process of world globalization, labor, and academic mobility, the visa-free regime with the EU countries have caused a significant ...
IEEE publishes the leading journals, transactions, letters, and magazines in electrical engineering, computing, biotechnology, telecommunications, power and energy, and dozens of other technologies. In addition, IEEE publishes more than 1,800 leading-edge conference proceedings every year, which are recognized by academia and industry worldwide ...
An analysis of the required data bandwidths, reaching nearly 1 Tbps for a single chip and necessitating the use of high bandwidth memory, is provided. Machine Learning has become a dominant technology, spurring the invention of photonic systems to implement neural network tasks. These photonic systems offer high throughput operations with low power but require large bandwidths of data to ...
Data Science in simple words is the study of data. Data Science is the process through which you can convert raw data into knowledge to support decision making. It involves developing methods of storing and analyzing data effectively. Then through this data, you. can extract useful information from scientific methods, processes, algorithms, and ...
In this paper, we substantiate our premise that statistics is one of the most important disciplines to provide tools and methods. to find structure in and to give deeper insight into data, and ...
Data Science and Big Data Projects. ... Implementing IEEE projects in Computer Science Engineering involves a systematic methodology to ensure successful execution. Below is a step-by-step guide that outlines the key phases and considerations in the implementation process: ... Prepare Research Papers: If applicable, document the research ...
Snowflake for data science was created from the ground. up to serve applications driven b y machine learning and AI. In addition to being tightly integrated with Spark, R, Python, and Qubole ...
Hallucinations (confabulations) in large language model systems can be tackled by measuring uncertainty about the meanings of generated responses rather than the text itself to improve ...
SEATTLE, 19 June 2024 - Today, during the 2024 Computer Vision and Pattern Recognition (CVPR) Conference opening session, the CVPR Awards Committee announced the winners of its prestigious Best Paper Awards, which annually recognize top research in computer vision, artificial intelligence (AI), machine learning (ML), augmented, virtual and mixed reality (AR/VR/MR), deep learning, and much more.
The Neural Information Processing Systems Foundation is a non-profit corporation whose purpose is to foster the exchange of research advances in Artificial Intelligence and Machine Learning, principally by hosting an annual interdisciplinary academic conference with the highest ethical standards for a diverse and inclusive community.
The paper is published in PLOS ONE. ... This article has been reviewed according to Science X's ... has found via meta-analysis of data from prior research efforts that homosexual behavior is far ...