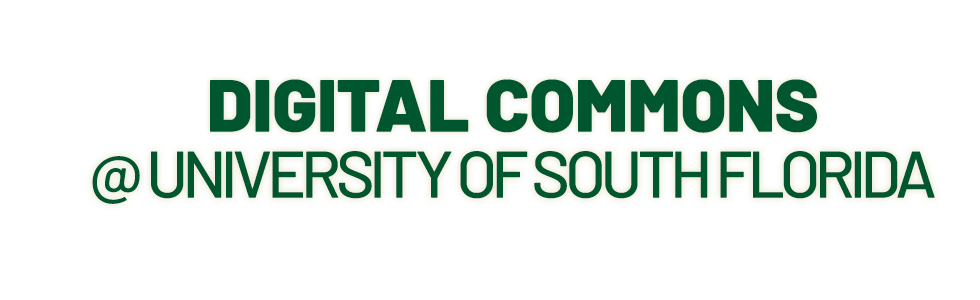
- USF Research
- USF Libraries
Digital Commons @ USF > College of Arts and Sciences > Mathematics and Statistics > Theses and Dissertations
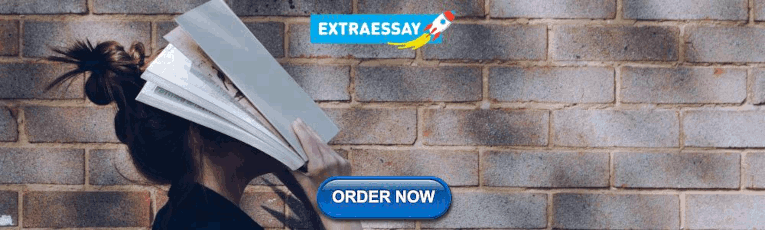
Mathematics and Statistics Theses and Dissertations
Theses/dissertations from 2023 2023.
Classification of Finite Topological Quandles and Shelves via Posets , Hitakshi Lahrani
Applied Analysis for Learning Architectures , Himanshu Singh
Rational Functions of Degree Five That Permute the Projective Line Over a Finite Field , Christopher Sze
Theses/Dissertations from 2022 2022
New Developments in Statistical Optimal Designs for Physical and Computer Experiments , Damola M. Akinlana
Advances and Applications of Optimal Polynomial Approximants , Raymond Centner
Data-Driven Analytical Predictive Modeling for Pancreatic Cancer, Financial & Social Systems , Aditya Chakraborty
On Simultaneous Similarity of d-tuples of Commuting Square Matrices , Corey Connelly
Symbolic Computation of Lump Solutions to a Combined (2+1)-dimensional Nonlinear Evolution Equation , Jingwei He
Boundary behavior of analytic functions and Approximation Theory , Spyros Pasias
Stability Analysis of Delay-Driven Coupled Cantilevers Using the Lambert W-Function , Daniel Siebel-Cortopassi
A Functional Optimization Approach to Stochastic Process Sampling , Ryan Matthew Thurman
Theses/Dissertations from 2021 2021
Riemann-Hilbert Problems for Nonlocal Reverse-Time Nonlinear Second-order and Fourth-order AKNS Systems of Multiple Components and Exact Soliton Solutions , Alle Adjiri
Zeros of Harmonic Polynomials and Related Applications , Azizah Alrajhi
Combination of Time Series Analysis and Sentiment Analysis for Stock Market Forecasting , Hsiao-Chuan Chou
Uncertainty Quantification in Deep and Statistical Learning with applications in Bio-Medical Image Analysis , K. Ruwani M. Fernando
Data-Driven Analytical Modeling of Multiple Myeloma Cancer, U.S. Crop Production and Monitoring Process , Lohuwa Mamudu
Long-time Asymptotics for mKdV Type Reduced Equations of the AKNS Hierarchy in Weighted L 2 Sobolev Spaces , Fudong Wang
Online and Adjusted Human Activities Recognition with Statistical Learning , Yanjia Zhang
Theses/Dissertations from 2020 2020
Bayesian Reliability Analysis of The Power Law Process and Statistical Modeling of Computer and Network Vulnerabilities with Cybersecurity Application , Freeh N. Alenezi
Discrete Models and Algorithms for Analyzing DNA Rearrangements , Jasper Braun
Bayesian Reliability Analysis for Optical Media Using Accelerated Degradation Test Data , Kun Bu
On the p(x)-Laplace equation in Carnot groups , Robert D. Freeman
Clustering methods for gene expression data of Oxytricha trifallax , Kyle Houfek
Gradient Boosting for Survival Analysis with Applications in Oncology , Nam Phuong Nguyen
Global and Stochastic Dynamics of Diffusive Hindmarsh-Rose Equations in Neurodynamics , Chi Phan
Restricted Isometric Projections for Differentiable Manifolds and Applications , Vasile Pop
On Some Problems on Polynomial Interpolation in Several Variables , Brian Jon Tuesink
Numerical Study of Gap Distributions in Determinantal Point Process on Low Dimensional Spheres: L -Ensemble of O ( n ) Model Type for n = 2 and n = 3 , Xiankui Yang
Non-Associative Algebraic Structures in Knot Theory , Emanuele Zappala
Theses/Dissertations from 2019 2019
Field Quantization for Radiative Decay of Plasmons in Finite and Infinite Geometries , Maryam Bagherian
Probabilistic Modeling of Democracy, Corruption, Hemophilia A and Prediabetes Data , A. K. M. Raquibul Bashar
Generalized Derivations of Ternary Lie Algebras and n-BiHom-Lie Algebras , Amine Ben Abdeljelil
Fractional Random Weighted Bootstrapping for Classification on Imbalanced Data with Ensemble Decision Tree Methods , Sean Charles Carter
Hierarchical Self-Assembly and Substitution Rules , Daniel Alejandro Cruz
Statistical Learning of Biomedical Non-Stationary Signals and Quality of Life Modeling , Mahdi Goudarzi
Probabilistic and Statistical Prediction Models for Alzheimer’s Disease and Statistical Analysis of Global Warming , Maryam Ibrahim Habadi
Essays on Time Series and Machine Learning Techniques for Risk Management , Michael Kotarinos
The Systems of Post and Post Algebras: A Demonstration of an Obvious Fact , Daviel Leyva
Reconstruction of Radar Images by Using Spherical Mean and Regular Radon Transforms , Ozan Pirbudak
Analyses of Unorthodox Overlapping Gene Segments in Oxytricha Trifallax , Shannon Stich
An Optimal Medium-Strength Regularity Algorithm for 3-uniform Hypergraphs , John Theado
Power Graphs of Quasigroups , DayVon L. Walker
Theses/Dissertations from 2018 2018
Groups Generated by Automata Arising from Transformations of the Boundaries of Rooted Trees , Elsayed Ahmed
Non-equilibrium Phase Transitions in Interacting Diffusions , Wael Al-Sawai
A Hybrid Dynamic Modeling of Time-to-event Processes and Applications , Emmanuel A. Appiah
Lump Solutions and Riemann-Hilbert Approach to Soliton Equations , Sumayah A. Batwa
Developing a Model to Predict Prevalence of Compulsive Behavior in Individuals with OCD , Lindsay D. Fields
Generalizations of Quandles and their cohomologies , Matthew J. Green
Hamiltonian structures and Riemann-Hilbert problems of integrable systems , Xiang Gu
Optimal Latin Hypercube Designs for Computer Experiments Based on Multiple Objectives , Ruizhe Hou
Human Activity Recognition Based on Transfer Learning , Jinyong Pang
Signal Detection of Adverse Drug Reaction using the Adverse Event Reporting System: Literature Review and Novel Methods , Minh H. Pham
Statistical Analysis and Modeling of Cyber Security and Health Sciences , Nawa Raj Pokhrel
Machine Learning Methods for Network Intrusion Detection and Intrusion Prevention Systems , Zheni Svetoslavova Stefanova
Orthogonal Polynomials With Respect to the Measure Supported Over the Whole Complex Plane , Meng Yang
Theses/Dissertations from 2017 2017
Modeling in Finance and Insurance With Levy-It'o Driven Dynamic Processes under Semi Markov-type Switching Regimes and Time Domains , Patrick Armand Assonken Tonfack
Prevalence of Typical Images in High School Geometry Textbooks , Megan N. Cannon
On Extending Hansel's Theorem to Hypergraphs , Gregory Sutton Churchill
Contributions to Quandle Theory: A Study of f-Quandles, Extensions, and Cohomology , Indu Rasika U. Churchill
Linear Extremal Problems in the Hardy Space H p for 0 p , Robert Christopher Connelly
Statistical Analysis and Modeling of Ovarian and Breast Cancer , Muditha V. Devamitta Perera
Statistical Analysis and Modeling of Stomach Cancer Data , Chao Gao
Structural Analysis of Poloidal and Toroidal Plasmons and Fields of Multilayer Nanorings , Kumar Vijay Garapati
Dynamics of Multicultural Social Networks , Kristina B. Hilton
Cybersecurity: Stochastic Analysis and Modelling of Vulnerabilities to Determine the Network Security and Attackers Behavior , Pubudu Kalpani Kaluarachchi
Generalized D-Kaup-Newell integrable systems and their integrable couplings and Darboux transformations , Morgan Ashley McAnally
Patterns in Words Related to DNA Rearrangements , Lukas Nabergall
Time Series Online Empirical Bayesian Kernel Density Segmentation: Applications in Real Time Activity Recognition Using Smartphone Accelerometer , Shuang Na
Schreier Graphs of Thompson's Group T , Allen Pennington
Cybersecurity: Probabilistic Behavior of Vulnerability and Life Cycle , Sasith Maduranga Rajasooriya
Bayesian Artificial Neural Networks in Health and Cybersecurity , Hansapani Sarasepa Rodrigo
Real-time Classification of Biomedical Signals, Parkinson’s Analytical Model , Abolfazl Saghafi
Lump, complexiton and algebro-geometric solutions to soliton equations , Yuan Zhou
Theses/Dissertations from 2016 2016
A Statistical Analysis of Hurricanes in the Atlantic Basin and Sinkholes in Florida , Joy Marie D'andrea
Statistical Analysis of a Risk Factor in Finance and Environmental Models for Belize , Sherlene Enriquez-Savery
Putnam's Inequality and Analytic Content in the Bergman Space , Matthew Fleeman
On the Number of Colors in Quandle Knot Colorings , Jeremy William Kerr
Statistical Modeling of Carbon Dioxide and Cluster Analysis of Time Dependent Information: Lag Target Time Series Clustering, Multi-Factor Time Series Clustering, and Multi-Level Time Series Clustering , Doo Young Kim
Some Results Concerning Permutation Polynomials over Finite Fields , Stephen Lappano
Hamiltonian Formulations and Symmetry Constraints of Soliton Hierarchies of (1+1)-Dimensional Nonlinear Evolution Equations , Solomon Manukure
Modeling and Survival Analysis of Breast Cancer: A Statistical, Artificial Neural Network, and Decision Tree Approach , Venkateswara Rao Mudunuru
Generalized Phase Retrieval: Isometries in Vector Spaces , Josiah Park
Leonard Systems and their Friends , Jonathan Spiewak
Resonant Solutions to (3+1)-dimensional Bilinear Differential Equations , Yue Sun
Statistical Analysis and Modeling Health Data: A Longitudinal Study , Bhikhari Prasad Tharu
Global Attractors and Random Attractors of Reaction-Diffusion Systems , Junyi Tu
Time Dependent Kernel Density Estimation: A New Parameter Estimation Algorithm, Applications in Time Series Classification and Clustering , Xing Wang
On Spectral Properties of Single Layer Potentials , Seyed Zoalroshd
Theses/Dissertations from 2015 2015
Analysis of Rheumatoid Arthritis Data using Logistic Regression and Penalized Approach , Wei Chen
Active Tile Self-assembly and Simulations of Computational Systems , Daria Karpenko
Nearest Neighbor Foreign Exchange Rate Forecasting with Mahalanobis Distance , Vindya Kumari Pathirana
Statistical Learning with Artificial Neural Network Applied to Health and Environmental Data , Taysseer Sharaf
Radial Versus Othogonal and Minimal Projections onto Hyperplanes in l_4^3 , Richard Alan Warner
Ensemble Learning Method on Machine Maintenance Data , Xiaochuang Zhao
Theses/Dissertations from 2014 2014
Properties of Graphs Used to Model DNA Recombination , Ryan Arredondo
Recursive Methods in Number Theory, Combinatorial Graph Theory, and Probability , Jonathan Burns
On the Classification of Groups Generated by Automata with 4 States over a 2-Letter Alphabet , Louis Caponi
Statistical Analysis, Modeling, and Algorithms for Pharmaceutical and Cancer Systems , Bong-Jin Choi
Topological Data Analysis of Properties of Four-Regular Rigid Vertex Graphs , Grant Mcneil Conine
Trend Analysis and Modeling of Health and Environmental Data: Joinpoint and Functional Approach , Ram C. Kafle
Advanced Search
- Email Notifications and RSS
- All Collections
- USF Faculty Publications
- Open Access Journals
- Conferences and Events
- Theses and Dissertations
- Textbooks Collection
Useful Links
- Mathematics and Statistics Department
- Rights Information
- SelectedWorks
- Submit Research
Home | About | Help | My Account | Accessibility Statement | Language and Diversity Statements
Privacy Copyright
Secondary Menu
- Our Mission
- Diversity, Equity, and Inclusion
- International Recognition
- Department History
- Departmental Awards
- Why Study Statistics?
- Degree Options
- Getting Started with Statistics
- For Current Students
- Frequently Asked Questions
- Summer Session Courses
- For Prospective Students
- Student Governance
- Program Requirements
- MS Focus Areas / Tracks
- Course Help & Tutoring
- MSS Completion Exercise
- Independent Study
- Statistical Science Proseminar
- Primary Faculty
- Secondary Faculty
- Visiting Faculty
- Postdoctoral Fellows
- Ph.D. Students
- M.S. Students
- Theory, Methods, and Computation
- Interdisciplinary Collaborations
- Statistical Consulting Center
- Alumni Profiles
- For Our Alumni
- Alumni Lists and Theses
- Master's Thesis
As an integral component of the Master of Science in Statistical Science program, you can submit and defend a Master's Thesis. Your Master's Committee administers this oral examination. If you choose to defend a thesis, it is advisable to commence your research early, ideally during your second semester or the summer following your first year in the program. It's essential to allocate sufficient time for the thesis writing process. Your thesis advisor, who also serves as the committee chair, must approve both your thesis title and proposal. The final thesis work necessitates approval from all committee members and must adhere to the Master's thesis requirements set forth by the Duke University Graduate School.
Master’s BEST Award
Each second-year Duke Master’s of Statistical Science (MSS) student defending their MSS thesis may be eligible for the Master’s BEST Award . The Statistical Science faculty BEST Award Committee selects the awardee based on the submitted thesis of MSS thesis students, and the award is presented at the departmental graduation ceremony.
Thesis Proposal
All second-year students choosing to do a thesis must submit a proposal (not more than two pages) approved by their thesis advisor to the Master's Director via Qualtrics by November 10th. The thesis proposal should include a title, the thesis advisor, committee members, and a description of your work. The description must introduce the research topic, outline its main objectives, and emphasize the significance of the research and its implications while identifying gaps in existing statistical literature. In addition, it can include some of the preliminary results.
Committee members
MSS Students will have a thesis committee, which includes three faculty members - two must be departmental primary faculty, and the third could be from an external department in an applied area of the student’s interest, which must be a Term Graduate Faculty through the Graduate School or have a secondary appointment with the Department of Statistical Science. All Committee members must be familiar with the Student’s work. The department coordinates Committee approval. The thesis defense committee must be approved at least 30 days before the defense date.
Thesis Timeline and Departmental Process:
Before defense:.
Intent to Graduate: Students must file an Intent to Graduate in ACES, specifying "Thesis Defense" during the application. For graduation deadlines, please refer to https://gradschool.duke.edu/academics/preparing-graduate .
Scheduling Thesis Defense: The student collaborates with the committee to set the date and time for the defense and communicates this information to the department, along with the thesis title. The defense must be scheduled during regular class sessions. Be sure to review the thesis defense and submission deadlines at https://gradschool.duke.edu/academics/theses-and-dissertations/
Room Reservations: The department arranges room reservations and sends confirmation details to the student, who informs committee members of the location.
Defense Announcement: The department prepares a defense announcement, providing a copy to the student and chair. After approval, it is signed by the Master's Director and submitted to the Graduate School. Copies are also posted on department bulletin boards.
Initial Thesis Submission: Two weeks before the defense, the student submits the initial thesis to the committee and the Graduate School. Detailed thesis formatting guidelines can be found at https://gradschool.duke.edu/academics/theses-and-dissertations.
Advisor Notification: The student requests that the advisor email [email protected] , confirming the candidate's readiness for defense. This step should be completed before the exam card appointment.
Format Check Appointment: One week before the defense, the Graduate School contacts the student to schedule a format check appointment. Upon approval, the Graduate School provides the Student Master’s Exam Card, which enables the student to send a revised thesis copy to committee members.
MSS Annual Report Form: The department provides the student with the MSS Annual Report Form to be presented at the defense.
Post Defense:
Communication of Defense Outcome: The committee chair conveys the defense results to the student, including any necessary follow-up actions in case of an unsuccessful defense.
In Case of Failure: If a student does not pass the thesis defense, the committee's decision to fail the student must be accompanied by explicit and clear comments from the chair, specifying deficiencies and areas that require attention for improvement.
Documentation: The student should ensure that the committee signs the Title Page, Abstract Page, and Exam Card.
Annual Report Form: The committee chair completes the Annual Report Form.
Master's Director Approval: The Master's director must provide their approval by signing the Exam Card.
Form Submission: Lastly, the committee chair is responsible for returning all completed and signed forms to the Department.
Final Thesis Submission: The student must meet the Graduate School requirement by submitting the final version of their Thesis to the Graduate School via ProQuest before the specified deadline. For detailed information, visit https://gradschool.duke.edu/academics/preparinggraduate .
- The Stochastic Proximal Distance Algorithm
- Logistic-tree Normal Mixture for Clustering Microbiome Compositions
- Inference for Dynamic Treatment Regimes using Overlapping Sampling Splitting
- Bayesian Modeling for Identifying Selection in B Cell Maturation
- Differentially Private Verification with Survey Weights
- Stable Variable Selection for Sparse Linear Regression in a Non-uniqueness Regime
- A Cost-Sensitive, Semi-Supervised, and Active Learning Approach for Priority Outlier Investigation
- Bayesian Decoupling: A Decision Theory-Based Approach to Bayesian Variable Selection
- A Differentially Private Bayesian Approach to Replication Analysis
- Numerical Approximation of Gaussian-Smoothed Optimal Transport
- Computational Challenges to Bayesian Density Discontinuity Regression
- Hierarchical Signal Propagation for Household Level Sales in Bayesian Dynamic Models
- Logistic Tree Gaussian Processes (LoTgGaP) for Microbiome Dynamics and Treatment Effects
- Bayesian Inference on Ratios Subject to Differentially Private Noise
- Multiple Imputation Inferences for Count Data
- An Euler Characteristic Curve Based Representation of 3D Shapes in Statistical Analysis
- An Investigation Into the Bias & Variance of Almost Matching Exactly Methods
- Comparison of Bayesian Inference Methods for Probit Network Models
- Differentially Private Counts with Additive Constraints
- Multi-Scale Graph Principal Component Analysis for Connectomics
- MCMC Sampling Geospatial Partitions for Linear Models
- Bayesian Dynamic Network Modeling with Censored Flow Data
- An Application of Graph Diffusion for Gesture Classification
- Easy and Efficient Bayesian Infinite Factor Analysis
- Analyzing Amazon CD Reviews with Bayesian Monitoring and Machine Learning Methods
- Missing Data Imputation for Voter Turnout Using Auxiliary Margins
- Generalized and Scalable Optimal Sparse Decision Trees
- Construction of Objective Bayesian Prior from Bertrand’s Paradox and the Principle of Indifference
- Rethinking Non-Linear Instrumental Variables
- Clustering-Enhanced Stochastic Gradient MCMC for Hidden Markov Models
- Optimal Sparse Decision Trees
- Bayesian Density Regression with a Jump Discontinuity at a Given Threshold
- Forecasting the Term Structure of Interest Rates: A Bayesian Dynamic Graphical Modeling Approach
- Testing Between Different Types of Poisson Mixtures with Applications to Neuroscience
- Multiple Imputation of Missing Covariates in Randomized Controlled Trials
- A Bayesian Strategy to the 20 Question Game with Applications to Recommender Systems
- Applied Factor Dynamic Analysis for Macroeconomic Forecasting
- A Theory of Statistical Inference for Ensuring the Robustness of Scientific Results
- Bayesian Inference Via Partitioning Under Differential Privacy
- A Bayesian Forward Simulation Approach to Establishing a Realistic Prior Model for Complex Geometrical Objects
- Two Applications of Summary Statistics: Integrating Information Across Genes and Confidence Intervals with Missing Data
- Past Recipients
- Considering a Statistical Science major at Duke?
- Careers for Statisticians
- Typical Pathways
- Applied Electives for BS
- Interdepartmental Majors
- Minor in Statistical Science
- Student Learning Outcomes
- Study Abroad
- Past Theses
- Research Teams
- Transfer Credit
- Conference Funding for Research
- Statistical Science Majors Union
- Duke Actuarial Society
- Duke Sports Analytics Club
- Trinity Ambassadors
- How to Apply
- Financial Support
- Graduate Placements
- Living in Durham
- Preliminary Examination
- Dissertation
- English Language Requirement
- TA Guidelines
- Progress Toward Completion
- Ph.D. Committees
- Terminal MS Degree
- PhD / Research
- Data Science & Analytics
- Health Data Science
- Finance & Economics
- Marketing Research & Business Analytics
- Social Science & Policy
- Admission Statistics
- Portfolio of Work
- Capstone Project
- Assisting Duke Students
- StatSci Alumni Network
- Ph.D. Student - Alumni Fund
- Our Ph.D. Alums
- Our M.S. Alums
- Our Undergrad Alums
- Our Postdoc Alums
- Skip to main content
We use cookies
Necessary cookies.
Necessary cookies enable core functionality. The website cannot function properly without these cookies, and can only be disabled by changing your browser preferences.
Analytics cookies
Analytical cookies help us improve our website. We use Google Analytics. All data is anonymised.
Hotjar and Clarity
Hotjar and Clarity help us to understand our users’ behaviour by visually representing their clicks, taps and scrolling. All data is anonymised.
Privacy policy
School of Mathematics & Statistics
- Postgraduate research study
- Statistics Thesis Topics
- About the School
- Postgraduate Research Courses
- Mathematics Thesis Topics
Statistics thesis topics
Below are sample topics available for prospective postgraduate research students. These sample topics do not contain every possible project; they are aimed at giving an impression of the breadth of different topics available. Most prospective supervisors would be more than happy to discuss projects not listed below.
Funded projects are projects with project-specific funding. Funding for other projects is usally available on a competitive basis.
Modelling in Space and Time - Example Research Projects
Information about postgraduate research opportunities and how to apply can be found on the Postgraduate Research Study page . Below is a selection of projects that could be undertaken with our group.
Evaluating probabilistic forecasts in high-dimensional settings (PhD)
Supervisors: Jethro Browell Relevant research groups: Modelling in Space and Time , Computational Statistics , Applied Probability and Stochastic Processes
Many decisions are informed by forecasts, and almost all forecasts are uncertain to some degree. Probabilistic forecasts quantify uncertainty to help improve decision-making and are playing an important role in fields including weather forecasting, economics, energy, and public policy. Evaluating the quality of past forecasts is essential to give forecasters and forecast users confidence in their current predictions, and to compare the performance of forecasting systems.
While the principles of probabilistic forecast evaluation have been established over the past 15 years, most notably that of “ sharpness subject to calibration/reliability” , we lack a complete toolkit for applying these principles in many situations, especially those that arise in high-dimensional settings. Furthermore, forecast evaluation must be interpretable by forecast users as well as expert forecasts, and assigning value to marginal improvements in forecast quality remains a challenge in many sectors.
This PhD will develop new statistical methods for probabilistic forecast evaluation considering some of the following issues:
- Verifying probabilistic calibration conditional on relevant covariates
- Skill scores for multivariate probabilistic forecasts where “ideal” performance is unknowable
- Assigning value to marginal forecast improvement though the convolution of utility functions and Murphey Diagrams
- Development of the concept of “anticipated verification” and “predicting the of uncertainty of future forecasts”
- Decomposing forecast misspecification (e.g. into spatial and temporal components)
- Evaluation of Conformal Predictions
Good knowledge of multivariate statistics is essential, prior knowledge of probabilistic forecasting and forecast evaluation would be an advantage.
Adaptive probabilistic forecasting (PhD)
Supervisors: Jethro Browell Relevant research groups: Modelling in Space and Time , Computational Statistics , Applied Probability and Stochastic Processes
Data-driven predictive models depend on the representativeness of data used in model selection and estimation. However, many processes change over time meaning that recent data is more representative than old data. In this situation, predictive models should track these changes, which is the aim of “online” or “adaptive” algorithms. Furthermore, many users of forecasts require probabilistic forecasts, which quantify uncertainty, to inform their decision-making. Existing adaptive methods such as Recursive Least Squares, the Kalman Filter have been very successful for adaptive point forecasting, but adaptive probabilistic forecasting has received little attention. This PhD will develop methods for adaptive probabilistic forecasting from a theoretical perspective and with a view to apply these methods to problems in at least one application area to be determined.
In the context of adaptive probabilistic forecasting, this PhD may consider:
- Online estimation of Generalised Additive Models for Location Scale and Shape
- Online/adaptive (multivariate) time series prediction
- Online aggregation (of experts, or hierarchies)
A good knowledge of methods for time series analysis and regression is essential, familiarity with flexible regression (GAMs) and distributional regression (GAMLSS/quantile regression) would be an advantage.
The evolution of shape (PhD)
Supervisors: Vincent Macaulay Relevant research groups: Bayesian Modelling and Inference , Modelling in Space and Time , Statistical Modelling for Biology, Genetics and *omics
Shapes of objects change in time. Organisms evolve and in the process change form: humans and chimpanzees derive from some common ancestor presumably different from either in shape. Designed objects are no different: an Art Deco tea pot from the 1920s might share some features with one from Ikea in 2010, but they are different. Mathematical models of evolution for certain data types, like the strings of As, Gs , Cs and Ts in our evolving DNA, are quite mature and allow us to learn about the relationships of the objects (their phylogeny or family tree), about the changes that happen to them in time (the evolutionary process) and about the ways objects were configured in the past (the ancestral states), by statistical techniques like phylogenetic analysis. Such techniques for shape data are still in their infancy. This project will develop novel statistical inference approaches (in a Bayesian context) for complex data objects, like functions, surfaces and shapes, using Gaussian-process models, with potential application in fields as diverse as language evolution, morphometrics and industrial design.
New methods for analysis of migratory navigation (PhD)
Supervisors: Janine Illian Relevant research groups: Modelling in Space and Time , Bayesian Modelling and Inference , Computational Statistics , Environmental, Ecological Sciences and Sustainability
Joint project with Dr Urška Demšar (University of St Andrews)
Migratory birds travel annually across vast expanses of oceans and continents to reach their destination with incredible accuracy. How they are able to do this using only locally available cues is still not fully understood. Migratory navigation consists of two processes: birds either identify the direction in which to fly (compass orientation) or the location where they are at a specific moment in time (geographic positioning). One of the possible ways they do this is to use information from the Earth’s magnetic field in the so-called geomagnetic navigation (Mouritsen 2018). While there is substantial evidence (both physiological and behavioural) that they do sense magnetic field (Deutschlander and Beason 2014), we however still do not know exactly which of the components of the field they use for orientation or positioning. We also do not understand how rapid changes in the field affect movement behaviour.
There is a possibility that birds can sense these rapid large changes and that this may affect their navigational process. To study this, we need to link accurate data on Earth’s magnetic field with animal tracking data. This has only become possible very recently through new spatial data science advances: we developed the MagGeo tool, which links contemporaneous geomagnetic data from Swarm satellites of the European Space Agency with animal tracking data (Benitez Paez et al. 2021).
Linking geomagnetic data to animal tracking data however creates a highly-dimensional data set, which is difficult to explore. Typical analyses of contextual environmental information in ecology include representing contextual variables as co-variates in relatively simple statistical models (Brum Bastos et al. 2021), but this is not sufficient for studying detailed navigational behaviour. This project will analyse complex spatio-temporal data using computationally efficient statistical model fitting approches in a Bayesian context.
This project is fully based on open data to support reproducibility and open science. We will test our new methods by annotating publicly available bird tracking data (e.g. from repositories such as Movebank.org), using the open MagGeo tool and implementing our new methods as Free and Open Source Software (R/Python).
Benitez Paez F, Brum Bastos VdS, Beggan CD, Long JA and Demšar U, 2021. Fusion of wildlife tracking and satellite geomagnetic data for the study of animal migration. Movement Ecology , 9:31. https://doi.org/10.1186/s40462-021-00268-4
Brum Bastos VdS, Łos M, Long JA, Nelson T and Demšar U, 2021, Context-aware movement analysis in ecology: a systematic review. International Journal of Geographic Information Science , https://doi.org/10.1080/13658816.2021.1962528
Deutschlander ME and Beason RC, 2014. Avian navigation and geographic positioning. Journal of Field Ornithology , 85(2):111–133. https://doi.org/10.1111/jofo.12055
Integrated spatio-temporal modelling for environmental data (PhD)
Supervisors: Janine Illian Relevant research groups: Modelling in Space and Time , Bayesian Modelling and Inference , Computational Statistics , Environmental, Ecological Sciences and Sustainability
(Jointly supervised by Peter Henrys, CEH)
The last decade has seen a proliferation of environmental data with vast quantities of information available from various sources. This has been due to a number of different factors including: the advent of sensor technologies; the provision of remotely sensed data from both drones and satellites; and the explosion in citizen science initiatives. These data represent a step change in the resolution of available data across space and time - sensors can be streaming data at a resolution of seconds whereas citizen science observations can be in the hundreds of thousands.
Over the same period, the resources available for traditional field surveys have decreased dramatically whilst logistical issues (such as access to sites, ) have increased. This has severely impacted the ability for field survey campaigns to collect data at high spatial and temporal resolutions. It is exactly this sort of information that is required to fit models that can quantify and predict the spread of invasive species, for example.
Whilst we have seen an explosion of data across various sources, there is no single source that provides both the spatial and temporal intensity that may be required when fitting complex spatio-temporal models (cf invasive species example) - each has its own advantages and benefits in terms of information content. There is therefore potentially huge benefit in beginning together data from these different sources within a consistent framework to exploit the benefits each offers and to understand processes at unprecedented resolutions/scales that would be impossible to monitor.
Current approaches to combining data in this way are typically very bespoke and involve complex model structures that are not reusable outside of the particular application area. What is needed is an overarching generic methodological framework and associated software solutions to implement such analyses. Not only would such a framework provide the methodological basis to enable researchers to benefit from this big data revolution, but also the capability to change such analyses from being stand alone research projects in their own right, to more operational, standard analytical routines.
FInally, such dynamic, integrated analyses could feedback into data collection initiatives to ensure optimal allocation of effort for traditional surveys or optimal power management for sensor networks. The major step change being that this optimal allocation of effort is conditional on other data that is available. So, for example, given the coverage and intensity of the citizen science data, where should we optimally send our paid surveyors? The idea is that information is collected at times and locations that provide the greatest benefit in understanding the underpinning stochastic processes. These two major issues - integrated analyses and adaptive sampling - ensure that environmental monitoring is fit for purpose and scientists, policy and industry can benefit from the big data revolution.
This project will develop an integrated statistical modelling strategy that provides a single modelling framework for enabling quantification of ecosystem goods and services while accounting for the fundamental differences in different data streams. Data collected at different spatial resolutions can be used within the same model through projecting it into continuous space and projecting it back into the landscape level of interest. As a result, decisions can be made at the relevant spatial scale and uncertainty is propagated through, facilitating appropriate decision making.
Statistical methodology for assessing the impacts of offshore renewable developments on marine wildlife (PhD)
(jointly supervised by Esther Jones and Adam Butler, BIOSS)
Assessing the impacts of offshore renewable developments on marine wildlife is a critical component of the consenting process. A NERC-funded project, ECOWINGS, will provide a step-change in analysing predator-prey dynamics in the marine environment, collecting data across trophic levels against a backdrop of developing wind farms and climate change. Aerial survey and GPS data from multiple species of seabirds will be collected contemporaneously alongside prey data available over the whole water column from an automated surface vehicle and underwater drone.
These methods of data collection will generate 3D space and time profiles of predators and prey, creating a rich source of information and enormous potential for modelling and interrogation. The data present a unique opportunity for experimental design across a dynamic and changing marine ecosystem, which is heavily influenced by local and global anthropogenic activities. However, these data have complex intrinsic spatio-temporal properties, which are challenging to analyse. Significant statistical methods development could be achieved using this system as a case study, contributing to the scientific knowledge base not only in offshore renewables but more generally in the many circumstances where patchy ecological spatio-temporal data are available.
This PhD project will develop spatio-temporal modelling methodology that will allow user to anaylse these exciting - and complex - data sets and help inform our knowledge on the impact of off-shore renewable on wildlife.
Analysis of spatially correlated functional data objects (PhD)
Supervisors: Surajit Ray Relevant research groups: Modelling in Space and Time , Computational Statistics , Nonparametric and Semi-parametric Statistics , Imaging, Image Processing and Image Analysis
Historically, functional data analysis techniques have widely been used to analyze traditional time series data, albeit from a different perspective. Of late, FDA techniques are increasingly being used in domains such as environmental science, where the data are spatio-temporal in nature and hence is it typical to consider such data as functional data where the functions are correlated in time or space. An example where modeling the dependencies is crucial is in analyzing remotely sensed data observed over a number of years across the surface of the earth, where each year forms a single functional data object. One might be interested in decomposing the overall variation across space and time and attribute it to covariates of interest. Another interesting class of data with dependence structure consists of weather data on several variables collected from balloons where the domain of the functions is a vertical strip in the atmosphere, and the data are spatially correlated. One of the challenges in such type of data is the problem of missingness, to address which one needs develop appropriate spatial smoothing techniques for spatially dependent functional data. There are also interesting design of experiment issues, as well as questions of data calibration to account for the variability in sensing instruments. Inspite of the research initiative in analyzing dependent functional data there are several unresolved problems, which the student will work on:
- robust statistical models for incorporating temporal and spatial dependencies in functional data
- developing reliable prediction and interpolation techniques for dependent functional data
- developing inferential framework for testing hypotheses related to simplified dependent structures
- analysing sparsely observed functional data by borrowing information from neighbours
- visualisation of data summaries associated with dependent functional data
- Clustering of functional data
Estimating the effects of air pollution on human health (PhD)
Supervisors: Duncan Lee Relevant research groups: Modelling in Space and Time , Biostatistics, Epidemiology and Health Applications
The health impact of exposure to air pollution is thought to reduce average life expectancy by six months, with an estimated equivalent health cost of 19 billion each year (from DEFRA). These effects have been estimated using statistical models, which quantify the impact on human health of exposure in both the short and the long term. However, the estimation of such effects is challenging, because individual level measures of health and pollution exposure are not available. Therefore, the majority of studies are conducted at the population level, and the resulting inference can only be made about the effects of pollution on overall population health. However, the data used in such studies are spatially misaligned, as the health data relate to extended areas such as cities or electoral wards, while the pollution concentrations are measured at individual locations. Furthermore, pollution monitors are typically located where concentrations are thought to be highest, known as preferential sampling, which is likely to result in overly high measurements being recorded. This project aims to develop statistical methodology to address these problems, and thus provide a less biased estimate of the effects of pollution on health than are currently produced.
Mapping disease risk in space and time (PhD)
Disease risk varies over space and time, due to similar variation in environmental exposures such as air pollution and risk inducing behaviours such as smoking. Modelling the spatio-temporal pattern in disease risk is known as disease mapping, and the aims are to: quantify the spatial pattern in disease risk to determine the extent of health inequalities, determine whether there has been any increase or reduction in the risk over time, identify the locations of clusters of areas at elevated risk, and quantify the impact of exposures, such as air pollution, on disease risk. I am working on all these related problems at present, and I have PhD projects in all these areas.
Bayesian Mixture Models for Spatio-Temporal Data (PhD)
Supervisors: Craig Anderson Relevant research groups: Modelling in Space and Time , Bayesian Modelling and Inference , Biostatistics, Epidemiology and Health Applications
The prevalence of disease is typically not constant across space – instead the risk tends to vary from one region to another. Some of this variability may be down to environmental conditions, but many of them are driven by socio-economic differences between regions, with poorer regions tending to have worse health than wealthier regions. For example, within the the Greater Glasgow and Clyde region, where the World Health Organisation noted that life expectancy ranges from 54 in Calton to 82 in Lenzie, despite these areas being less than 10 miles apart. There is substantial value to health professionals and policymakers in identifying some of the causes behind these localised health inequalities.
Disease mapping is a field of statistical epidemiology which focuses on estimating the patterns of disease risk across a geographical region. The main goal of such mapping is typically to identify regions of high disease risk so that relevant public health interventions can be made. This project involves the development of statistical models which will enhance our understanding regional differences in the risk of suffering from major diseases by focusing on these localised health inequalities.
Standard Bayesian hierarchical models with a conditional autoregressive prior are frequently used for risk estimation in this context, but these models assume a smooth risk surface which is often not appropriate in practice. In reality, it will often be the case that different regions have vastly different risk profiles and require different data generating functions as a result.
In this work we propose a mixture model based approach which allows different sub-populations to be represented by different underlying statistical distributions within a single modelling framework. By integrating CAR models into mixture models, researchers can simultaneously account for spatial dependencies and identify distinct disease patterns within subpopulations.
Bayesian Modelling and Inference - Example Research Projects
Modelling genetic variation (msc/phd).
Supervisors: Vincent Macaulay Relevant research groups: Bayesian Modelling and Inference , Statistical Modelling for Biology, Genetics and *omics
Variation in the distribution of different DNA sequences across individuals has been shaped by many processes which can be modelled probabilistically, processes such as demographic factors like prehistoric population movements, or natural selection. This project involves developing new techniques for teasing out information on those processes from the wealth of raw data that is now being generated by high-throughput genetic assays, and is likely to involve computationally-intensive sampling techniques to approximate the posterior distribution of parameters of interest. The characterization of the amount of population structure on different geographical scales will influence the design of experiments to identify the genetic variants that increase risk of complex diseases, such as diabetes or heart disease.
The evolution of shape (PhD)
Supervisors: Vincent Macaulay Relevant research groups: Bayesian Modelling and Inference , Modelling in Space and Time , Statistical Modelling for Biology, Genetics and *omics
New methods for analysis of migratory navigation (PhD)
Integrated spatio-temporal modelling for environmental data (phd), statistical methodology for assessing the impacts of offshore renewable developments on marine wildlife (phd).
This PhD project will develop spatio-temporal modelling methodology that will allow user to anaylse these exciting - and complex - data sets and help inform our knowledge on the impact of off-shore renewable on wildlife.
Bayesian variable selection for genetic and genomic studies (PhD)
Supervisors: Mayetri Gupta Relevant research groups: Bayesian Modelling and Inference , Computational Statistics , Statistical Modelling for Biology, Genetics and *omics
An important issue in high-dimensional regression problems is the accurate and efficient estimation of models when, compared to the number of data points, a substantially larger number of potential predictors are present. Further complications arise with correlated predictors, leading to the breakdown of standard statistical models for inference; and the uncertain definition of the outcome variable, which is often a varying composition of several different observable traits. Examples of such problems arise in many scenarios in genomics- in determining expression patterns of genes that may be responsible for a type of cancer; and in determining which genetic mutations lead to higher risks for occurrence of a disease. This project involves developing broad and improved Bayesian methodologies for efficient inference in high-dimensional regression-type problems with complex multivariate outcomes, with a focus on genetic data applications.
The successful candidate should have a strong background in methodological and applied Statistics, expert skills in relevant statistical software or programming languages (such as R, C/C++/Python), and also have a deep interest in developing knowledge in cross-disciplinary topics in genomics. The candidate will be expected to consolidate and master an extensive range of topics in modern Statistical theory and applications during their PhD, including advanced Bayesian modelling and computation, latent variable models, machine learning, and methods for Big Data. The successful candidate will be considered for funding to cover domestic tuition fees, as well as paying a stipend at the Research Council rate for four years.
Bayesian statistical data integration of single-cell and bulk “OMICS” datasets with clinical parameters for accurate prediction of treatment outcomes in Rheumatoid Arthritis (PhD)
Supervisors: Mayetri Gupta Relevant research groups: Bayesian Modelling and Inference , Computational Statistics , Statistical Modelling for Biology, Genetics and *omics , Biostatistics, Epidemiology and Health Applications
In recent years, many different computational methods to analyse biological data have been established: including DNA (Genomics), RNA (Transcriptomics), Proteins (proteomics) and Metabolomics, that captures more dynamic events. These methods were refined by the advent of single cell technology, where it is now possible to capture the transcriptomics profile of single cells, spatial arrangements of cells from flow methods or imaging methods like functional magnetic resonance imaging. At the same time, these OMICS data can be complemented with clinical data – measurement of patients, like age, smoking status, phenotype of disease or drug treatment. It is an interesting and important open statistical question how to combine data from different “modalities” (like transcriptome with clinical data or imaging data) in a statistically valid way, to compare different datasets and make justifiable statistical inferences. This PhD project will be jointly supervised with Dr. Thomas Otto and Prof. Stefan Siebert from the Institute of Infection, Immunity & Inflammation ), you will explore how to combine different datasets using Bayesian latent variable modelling, focusing on clinical datasets from Rheumatoid Arthritis.
Funding Notes
The successful candidate will be considered for funding to cover domestic tuition fees, as well as paying a stipend at the Research Council rate for four years.
Scalable Bayesian models for inferring evolutionary traits of plants (PhD)
Supervisors: Vinny Davies , Richard Reeve Relevant research groups: Bayesian Modelling and Inference , Computational Statistics , Environmental, Ecological Sciences and Sustainability , Statistical Modelling for Biology, Genetics and *omics
The functional traits and environmental preferences of plant species determine how they will react to changes resulting from global warming. The main global biodiversity repositories, such as the Global Biodiversity Information Facility ( GBIF ), contain hundreds of millions of records from hundreds of thousands of species in the plant kingdom alone, and the spatiotemporal data in these records can be associated with soil, climate or other environmental data from other databases. Combining these records allow us to identify environmental preferences, especially for common species where many records exist. Furthermore, in a previous PhD studentship we showed that these traits are highly evolutionarily conserved ( Harris et al., 2022 ), so it is possible to impute the preferences for rare species where little data exists using phylogenetic inference techniques.
The aim of this PhD project is to investigate the application of Bayesian variable selection methods to identify these evolutionarily conserved traits more effectively, and to quantify these traits and their associated uncertainty for all plant species for use in a plant ecosystem digital twin that we are developing separately to forecast the impact of climate change on biodiversity. In another PhD studentship, we previously developed similar methods for trait inference in viral evolution ( Davies et al., 2017 ; Davies et al., 2019 ), but due to the scale of the data here, these methods will need to be significantly enhanced. We therefore propose a project to investigate extensions to methods for phylogenetic trait inference to handle datasets involving hundreds of millions of records in phylogenies with hundreds of thousands of tips, potentially through either sub-sampling ( Quiroz et al, 2018 ) or modelling splitting and recombination ( Nemeth & Sherlock, 2018 ).
Computational Statistics - Example Research Projects
Supervisors: Jethro Browell Relevant research groups: Modelling in Space and Time , Computational Statistics , Applied Probability and Stochastic Processes
Supervisors: Jethro Browell Relevant research groups: Modelling in Space and Time , Computational Statistics , Applied Probability and Stochastic Processes
This project will develop an integrated statistical modelling strategy that provides a single modelling framework for enabling quantification of ecosystem goods and services while accounting for the fundamental differences in different data streams. Data collected at different spatial resolutions can be used within the same model through projecting it into continuous space and projecting it back into the landscape level of interest. As a result, decisions can be made at the relevant spatial scale and uncertainty is propagated through, facilitating appropriate decision making.
Statistical methodology for assessing the impacts of offshore renewable developments on marine wildlife (PhD)
Bayesian variable selection for genetic and genomic studies (phd), bayesian statistical data integration of single-cell and bulk “omics” datasets with clinical parameters for accurate prediction of treatment outcomes in rheumatoid arthritis (phd), scalable bayesian models for inferring evolutionary traits of plants (phd).
The aim of this PhD project is to investigate the application of Bayesian variable selection methods to identify these evolutionarily conserved traits more effectively, and to quantify these traits and their associated uncertainty for all plant species for use in a plant ecosystem digital twin that we are developing separately to forecast the impact of climate change on biodiversity. In another PhD studentship, we previously developed similar methods for trait inference in viral evolution ( Davies et al., 2017 ; Davies et al., 2019 ), but due to the scale of the data here, these methods will need to be significantly enhanced. We therefore propose a project to investigate extensions to methods for phylogenetic trait inference to handle datasets involving hundreds of millions of records in phylogenies with hundreds of thousands of tips, potentially through either sub-sampling ( Quiroz et al, 2018 ) or modelling splitting and recombination ( Nemeth & Sherlock, 2018 ).
Multi objective Bayesian optimisation for in silico to real metabolomics experiments (PhD/MSc)
Supervisors: Vinny Davies , Craig Alexander Relevant research groups: Computational Statistics , Machine Learning and AI , Emulation and Uncertainty Quantification , Statistical Modelling for Biology, Genetics and *omics , Statistics in Chemistry/Physics
Untargeted metabolomics experiments aim to identify the small molecules that make up a particular sample (e.g. , blood), allowing us to identify biomarkers, discover new chemicals, or understand the metabolism ( Smith et al., 2014 ) . Data Dependent Acquisition (DDA) methods are used to collect the information needed to identify the metabolites , and various more advanced DDA methods have recently been designed to improve this process ( Davies et al. (2021) ; McBride et al. (2023) ) . Each of these methods , however, ha s parameters that must be chosen in order to maximise the amount of relevant data (metabolite spectra) that is collected . Our recent work led to the design of a Virtual Metabolomics Mass Spectrometer ( ViMMS ) in which we can run computer simulations of experiments and test different parameter settings ( Wandy et al., 2019 , 2022 ). Previously this has involve d running a pre-determined set of parameters as part of a grid search in ViMMS , and then choosing the best parameter settings based on a single measure of performance. The proposed M . Res . (or Ph . D . ) will extend this appro ach by using multi objective Bayesian Optimisation to adapt simulations and optimise over multiple different measurements of quality . By optimising parameters in this manner, we can help improve real experiments currently underway at the University of Glasgow and beyond.
Analysis of spatially correlated functional data objects (PhD)
Nonparametric and semi-parametric statistics - example research projects, modality of mixtures of distributions (phd).
Supervisors: Surajit Ray Relevant research groups: Nonparametric and Semi-parametric Statistics , Applied Probability and Stochastic Processes , Statistical Modelling for Biology, Genetics and *omics , Biostatistics, Epidemiology and Health Applications
Finite mixtures provide a flexible and powerful tool for fitting univariate and multivariate distributions that cannot be captured by standard statistical distributions. In particular, multivariate mixtures have been widely used to perform modeling and cluster analysis of high-dimensional data in a wide range of applications. Modes of mixture densities have been used with great success for organizing mixture components into homogenous groups. But the results are limited to normal mixtures. Beyond the clustering application existing research in this area has provided fundamental results regarding the upper bound of the number of modes, but they too are limited to normal mixtures. In this project, we wish to explore the modality of non-normal distributions and their application to real life problems.
Applied Probability and Stochastic Processes - Example Research Projects
Modality of mixtures of distributions (phd).
Finite mixtures provide a flexible and powerful tool for fitting univariate and multivariate distributions that cannot be captured by standard statistical distributions. In particular, multivariate mixtures have been widely used to perform modeling and cluster analysis of high-dimensional data in a wide range of applications. Modes of mixture densities have been used with great success for organizing mixture components into homogenous groups. But the results are limited to normal mixtures. Beyond the clustering application existing research in this area has provided fundamental results regarding the upper bound of the number of modes, but they too are limited to normal mixtures. In this project, we wish to explore the modality of non-normal distributions and their application to real life problems.
Machine Learning and AI - Example Research Projects
Estimating false discovery rates in metabolite identification using generative ai (phd).
Supervisors: Vinny Davies , Andrew Elliott , Justin J.J. van der Hooft (Wageningen University) Relevant research groups: Machine Learning and AI , Emulation and Uncertainty Quantification , Statistical Modelling for Biology, Genetics and *omics , Statistics in Chemistry/Physics
Metabolomics is the study field that aims to map all molecules that are part of an organism, which can help us understand its metabolism and how it can be affected by disease, stress, age, or other factors. During metabolomics experiments, mass spectra of the metabolites are collected and then annotated by comparison against spectral databases such as METLIN ( Smith et al., 2005 ) or GNPS ( Wang et al., 2016 ). Generally, however, these spectral databases do not contain the mass spectra of a large proportion of metabolites, so the best matching spectrum from the database is not always the correct identification. Matches can be scored using cosine similarity, or more advanced methods such as Spec2Vec ( Huber et al., 2021 ), but these scores do not provide any statement about the statistical accuracy of the match. Creating decoy spectral libraries, specifically a large database of fake spectra, is one potential way of estimating False Discovery Rates (FDRs), allowing us to quantify the probability of a spectrum match being correct ( Scheubert et al., 2017 ). However, these methods are not widely used, suggesting there is significant scope to improve their performance and ease of use. In this project, we will use the code framework from our recently developed Virtual Metabolomics Mass Spectrometer (ViMMS) ( Wandy et al., 2019 , 2022 ) to systematically evaluate existing methods and identify possible improvements. We will then explore how we can use generative AI, e.g., Generative Adversarial Networks or Variational Autoencoders, to train a deep neural network that can create more realistic decoy spectra, and thus improve our estimation of FDRs.
Medical image segmentation and uncertainty quantification (PhD)
Supervisors: Surajit Ray Relevant research groups: Machine Learning and AI , Imaging, Image Processing and Image Analysis
This project focuses on the application of medical imaging and uncertainty quantification for the detection of tumours. The project aims to provide clinicians with accurate, non-invasive methods for detecting and classifying the presence of malignant and benign tumours. It seeks to combine advanced medical imaging technologies such as ultrasound, computed tomography (CT) and magnetic resonance imaging (MRI) with the latest artificial intelligence algorithms. These methods will automate the detection process and may be used for determining malignancy with a high degree of accuracy. Uncertainty quantification (UQ) techniques will help generate a more precise prediction for tumour malignancy by providing a characterisation of the degree of uncertainty associated with the diagnosis. The combination of medical imaging and UQ will significantly decrease the requirement for performing invasive medical procedures such as biopsies. This will improve the accuracy of the tumour detection process and reduce the duration of diagnosis. The project will also benefit from the development of novel image processing algorithms (e.g. deep learning) and machine learning models. These algorithms and models will help improve the accuracy of the tumour detection process and assist clinicians in making the best treatment decisions.
Generating deep fake left ventricles: a step towards personalised heart treatments (PhD)
Supervisors: Andrew Elliott , Vinny Davies , Hao Gao Relevant research groups: Machine Learning and AI , Emulation and Uncertainty Quantification , Biostatistics, Epidemiology and Health Applications , Imaging, Image Processing and Image Analysis
Personalised medicine is an exciting avenue in the field of cardiac healthcare where an understanding of patient-specific mechanisms can lead to improved treatments ( Gao et al., 2017 ). The use of mathematical models to link the underlying properties of the heart with cardiac imaging offers the possibility of obtaining important parameters of heart function non-invasively ( Gao et al., 2015 ). Unfortunately, current estimation methods rely on complex mathematical forward simulations, resulting in a solution taking hours, a time frame not suitable for real-time treatment decisions. To increase the applicability of these methods, statistical emulation methods have been proposed as an efficient way of estimating the parameters ( Davies et al., 2019 ; Noè et al., 2019 ). In this approach, simulations of the mathematical model are run in advance and then machine learning based methods are used to estimate the relationship between the cardiac imaging and the parameters of interest. These methods are, however, limited by our ability to understand the how cardiac geometry varies across patients which is in term limited by the amount of data available ( Romaszko et al., 2019 ). In this project we will look at AI based methods for generating fake cardiac geometries which can be used to increase the amount of data ( Qiao et al., 2023 ). We will explore different types of AI generation, including Generative Adversarial Networks or Variational Autoencoders, to understand how we can generate better 3D and 4D models of the fake left ventricles and create an improved emulation strategy that can make use of them.
Emulation and Uncertainty Quantification - Example Research Projects
Metabolomics is the study field that aims to map all molecules that are part of an organism, which can help us understand its metabolism and how it can be affected by disease, stress, age, or other factors. During metabolomics experiments, mass spectra of the metabolites are collected and then annotated by comparison against spectral databases such as METLIN ( Smith et al., 2005 ) or GNPS ( Wang et al., 2016 ). Generally, however, these spectral databases do not contain the mass spectra of a large proportion of metabolites, so the best matching spectrum from the database is not always the correct identification. Matches can be scored using cosine similarity, or more advanced methods such as Spec2Vec ( Huber et al., 2021 ), but these scores do not provide any statement about the statistical accuracy of the match. Creating decoy spectral libraries, specifically a large database of fake spectra, is one potential way of estimating False Discovery Rates (FDRs), allowing us to quantify the probability of a spectrum match being correct ( Scheubert et al., 2017 ). However, these methods are not widely used, suggesting there is significant scope to improve their performance and ease of use. In this project, we will use the code framework from our recently developed Virtual Metabolomics Mass Spectrometer (ViMMS) ( Wandy et al., 2019 , 2022 ) to systematically evaluate existing methods and identify possible improvements. We will then explore how we can use generative AI, e.g., Generative Adversarial Networks or Variational Autoencoders, to train a deep neural network that can create more realistic decoy spectra, and thus improve our estimation of FDRs.
Supervisors: Andrew Elliott , Vinny Davies , Hao Gao Relevant research groups: Machine Learning and AI , Emulation and Uncertainty Quantification , Biostatistics, Epidemiology and Health Applications , Imaging, Image Processing and Image Analysis
Environmental, Ecological Sciences and Sustainability - Example Research Projects
Statistical methodology for assessing the impacts of offshore renewable developments on marine wildlife (phd), statistical modelling for biology, genetics and *omics - example research projects, modelling genetic variation (msc/phd).
Supervisors: Vincent Macaulay Relevant research groups: Bayesian Modelling and Inference , Modelling in Space and Time , Statistical Modelling for Biology, Genetics and *omics
Bayesian statistical data integration of single-cell and bulk “OMICS” datasets with clinical parameters for accurate prediction of treatment outcomes in Rheumatoid Arthritis (PhD)
Supervisors: Vinny Davies , Richard Reeve , Claire Harris (BIOSS) Relevant research groups: Bayesian Modelling and Inference , Computational Statistics , Environmental, Ecological Sciences and Sustainability , Statistical Modelling for Biology, Genetics and *omics
Supervisors: Vinny Davies , Andrew Elliott , Justin J.J. van der Hooft (Wageningen University) Relevant research groups: Machine Learning and AI , Emulation and Uncertainty Quantification , Statistical Modelling for Biology, Genetics and *omics , Statistics in Chemistry/Physics
Multi objective Bayesian optimisation for in silico to real metabolomics experiments (PhD/MSc)
Finite mixtures provide a flexible and powerful tool for fitting univariate and multivariate distributions that cannot be captured by standard statistical distributions. In particular, multivariate mixtures have been widely used to perform modeling and cluster analysis of high-dimensional data in a wide range of applications. Modes of mixture densities have been used with great success for organizing mixture components into homogenous groups. But the results are limited to normal mixtures. Beyond the clustering application existing research in this area has provided fundamental results regarding the upper bound of the number of modes, but they too are limited to normal mixtures. In this project, we wish to explore the modality of non-normal distributions and their application to real life problems
Implementing a biology-empowered statistical framework to detect rare varient risk factors for complex diseases in whole genome sequence cohorts (PhD)
Supervisors: Vincent Macaulay , Luísa Pereira (Geneticist, i3s ) Relevant research groups: Statistical Modelling for Biology, Genetics and *omics , Biostatistics, Epidemiology and Health Applications
The traditional genome-wide association studies to detect candidate genetic risk factors for complex diseases/phenotypes (GWAS) recur largely to the microarray technology, genotyping at once thousands or millions of variants regularly spaced across the genome. These microarrays include mostly common variants (minor allele frequency, MAF>5%), missing candidate rare variants which are the more likely to be deleterious [ 1 ]. Currently, the best strategy to genotype low-frequency (1%<MAF<5%) and rare (MAF<1%) variants is through next generation sequencing, and the increasingly availability of whole genome sequences (WGS) places us in the brink of detecting rare variants associated with complex diseases [ 2 ]. Statistically, this detection constitutes a challenge, as the massive number of rare variants in genomes (for example, 64.7M in 150 Iberian WGSs) would imply genotyping millions/billions of individuals to attain statistical power. In the last couple years, several statistical methods have being tested in the context of association of rare variants with complex traits [ 2 , 3 , 4 ], largely testing strategies to aggregate the rare variants. These works have not yet tested the statistical empowerment that can be gained by incorporating reliable biological evidence on the aggregation of rare variants in the most probable functional regions, such as non-coding regulatory regions that control the expression of genes [ 4 ]. In fact, it has been demonstrated that even for common candidate variants, most of these variants (around 88%; [ 5 ]) are located in non-coding regions. If this is true for the common variants detected by the traditional GWAS, it is highly probable to be also true for rare variants.
In this work, we will implement a biology-empowered statistical framework to detect rare variant risk factors for complex diseases in WGS cohorts. We will recur to the 200,000 WGSs from UK Biobank database [ 6 ], that will be available to scientists before the end of 2023. Access to clinical information of these >40 years old UK residents is also provided. We will build our framework around type-2 diabetes (T2D), a common complex disease for which thousands of common variant candidates have been found [ 7 ]. Also, the mapping of regulatory elements is well known for the pancreatic beta cells that play a leading role in T2D [ 8 ]. We will use this mapping in guiding the rare variants’ aggregation and test it against a random aggregation across the genome. Of course, the framework rationale will be appliable to any other complex disease. We will browse literature for aggregation methods available at the beginning of this work, but we already selected the method SKAT (sequence kernel association test; [ 3 ]) to be tested. SKAT fits a random-effects model to the set of variants within a genomic interval or biologically-meaningful region (such as a coding or regulatory region) and computes variant-set level p-values, while permitting correction for covariates (such as the principal components mentioned above that can account for population stratification between cases and controls).
Biostatistics, Epidemiology and Health Applications - Example Research Projects
Bayesian statistical data integration of single-cell and bulk “omics” datasets with clinical parameters for accurate prediction of treatment outcomes in rheumatoid arthritis (phd).
Supervisors: Mayetri Gupta Relevant research groups: Bayesian Modelling and Inference , Computational Statistics , Vincent Macaulay , Biostatistics, Epidemiology and Health Applications
Supervisors: Andrew Elliott , Vinny Davies , Hao Gao Relevant research groups: Machine Learning and AI , Emulation and Uncertainty Quantification , Biostatistics, Epidemiology and Health Applications , Statistical Modelling for Biology, Genetics and *omics
Supervisors: Craig Anderson Relevant research groups: Modelling in Space and Time , Bayesian Modelling and Inference , Biostatistics, Epidemiology and Health Applications
Implementing a biology-empowered statistical framework to detect rare varient risk factors for complex diseases in whole genome sequence cohorts (PhD)
Supervisors: Vincent Macaulay , Luísa Pereira (Geneticist, i3s ) Relevant research groups: Statistical Modelling for Biology, Genetics and *omics , Biostatistics, Epidemiology and Health Applications
The traditional genome-wide association studies to detect candidate genetic risk factors for complex diseases/phenotypes (GWAS) recur largely to the microarray technology, genotyping at once thousands or millions of variants regularly spaced across the genome. These microarrays include mostly common variants (minor allele frequency, MAF>5%), missing candidate rare variants which are the more likely to be deleterious [ 1 ]. Currently, the best strategy to genotype low-frequency (1%<MAF<5%) and rare (MAF<1%) variants is through next generation sequencing, and the increasingly availability of whole genome sequences (WGS) places us in the brink of detecting rare variants associated with complex diseases [ 2 ]. Statistically, this detection constitutes a challenge, as the massive number of rare variants in genomes (for example, 64.7M in 150 Iberian WGSs) would imply genotyping millions/billions of individuals to attain statistical power. In the last couple years, several statistical methods have being tested in the context of association of rare variants with complex traits [ 2 , 3 , 4 ], largely testing strategies to aggregate the rare variants. These works have not yet tested the statistical empowerment that can be gained by incorporating reliable biological evidence on the aggregation of rare variants in the most probable functional regions, such as non-coding regulatory regions that control the expression of genes [ 4 ]. In fact, it has been demonstrated that even for common candidate variants, most of these variants (around 88%; [ 5 ]) are located in non-coding regions. If this is true for the common variants detected by the traditional GWAS, it is highly probable to be also true for rare variants.
Social and Urban Studies - Example Research Projects
Our group has an active PhD student community, and every year we admit new PhD students. We welcome applications from across the world. Further information can be found here .
Imaging, Image Processing and Image Analysis - Example Research Projects
Supervisors: Andrew Elliott , Vinny Davies , Hao Gao Relevant research groups: Machine Learning and AI , Emulation and Uncertainty Quantification , Biostatistics, Epidemiology and Health Applications , Imaging, Image Processing and Image Analysis
Statistics in Chemistry/Physics - Example Research Projects
Statistics and data analytics education - example research projects.
Our group has an active PhD student community, and every year we admit new PhD students. We welcome applications from across the world. Further information can be found here .
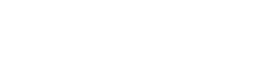
Recent Dissertation Topics
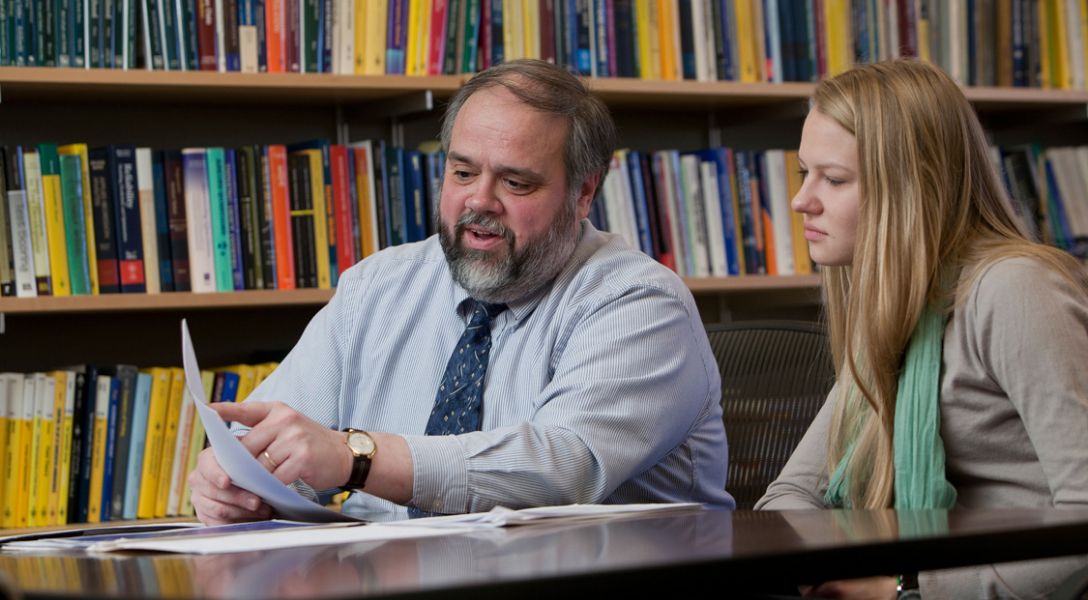
Kerstin Emily Frailey - “PRACTICAL DATA QUALITY FOR MODERN DATA & MODERN USES, WITH APPLICATIONS TO AMERICA’S COVID-19 DATA"
Dissertation Advisor: Martin Wells
Initial job placement: Co-Founder & CEO
David Kent - “Smoothness-Penalized Deconvolution: Rates of Convergence, Choice of Tuning Parameter, and Inference"
Dissertation Advisor: David Ruppert
Initial job placement: VISITING ASSISTANT PROFESSOR - Cornell University
Yuchen Xu - “Dynamic Atomic Column Detection in Transmission Electron Microscopy Videos via Ridge Estimation”
Dissertation Advisor: David Matteson
Initial job placement: Postdoctoral Fellow - UCLA
Siyi Deng - “Optimal and Safe Semi-supervised Estimation and Inference for High-dimensional Linear Regression"
Dissertation Advisor: Yang Ning
Initial job placement: Data Scientist - TikTok
Peter (Haoxuan) Wu - “Advances in adaptive and deep Bayesian state-space models”
Initial job placement: Quantitative Researcher - DRW
Grace Deng - “Generative models and Bayesian spillover graphs for dynamic networks”
Initial job placement: Data Scientist - Research at Google
Samriddha Lahiry - “Some problems of asymptotic quantum statistical inference”
Dissertation Advisor: Michael Nussbaum
Initial job placement: Postdoctoral Fellow - Harvard University
Yaosheng Xu - “WWTA load-balancing for parallel-server systems with heterogeneous servers and multi-scale heavy traffic limits for generalized Jackson networks”
Dissertation Advisor: Jim Dai
Initial job placement: Applied Scientist - Amazon
Seth Strimas-Mackey - “Latent structure in linear prediction and corpora comparison”
Dissertation Advisor: Marten Wegkamp and Florentina Bunea
Initial job placement: Data Scientist at Google
Tao Zhang - “Topics in modern regression modeling”
Dissertation Advisor: David Ruppert and Kengo Kato
Initial job placement: Quantitative Researcher - Point72
Wentian Huang - “Nonparametric and semiparametric approaches to functional data modeling”
Initial job placement: Ernst & Young
Binh Tang - “Deep probabilistic models for sequential prediction”
Initial job placement: Amazon
Yi Su - “Off-policy evaluation and learning for interactive systems"
Dissertation Advisor: Thorsten Joachims
Initial job placement: Berkeley (postdoc)
Ruqi Zhang - “Scalable and reliable inference for probabilistic modeling”
Dissertation Advisor: Christopher De Sa
Jason Sun - “Recent developments on Matrix Completion"
Initial job placement: LinkedIn
Indrayudh Ghosal - “Model combinations and the Infinitesimal Jackknife : how to refine models with boosting and quantify uncertainty”
Dissertation Advisor: Giles Hooker
Benjamin Ryan Baer - “Contributions to fairness and transparency”
Initial job placement: Rochester (postdoc)
Megan Lynne Gelsinger - “Spatial and temporal approaches to analyzing big data”
Dissertation Advisor: David Matteson and Joe Guinness
Initial job placement: Institute for Defense Analysis
Zhengze Zhou - “Statistical inference for machine learning : feature importance, uncertainty quantification and interpretation stability”
Initial job placement: Facebook
Huijie Feng - “Estimation and inference of high-dimensional individualized threshold with binary responses”
Initial job placement: Microsoft
Xiaojie Mao - “Machine learning methods for data-driven decision making : contextual optimization, causal inference, and algorithmic fairness”
Dissertation Advisor: Nathan Kallus and Madeleine Udell
Initial job placement: Tsinghua University, China
Xin Bing - “Structured latent factor models : Identifiability, estimation, inference and prediction”
Initial job placement: Cambridge (postdoc), University of Toronto
Yang Liu - “Nonparametric regression and density estimation on a network"
Dissertation Advisor: David Ruppert and Peter Frazier
Initial job placement: Research Analyst - Cubist Systematic Strategies
Skyler Seto - “Learning from less : improving and understanding model selection in penalized machine learning problems”
Initial job placement: Machine Learning Researcher - Apple
Jiekun Feng - “Markov chain, Markov decision process, and deep reinforcement learning with applications to hospital management and real-time ride-hailing”
Initial job placement:
Wenyu Zhang - “Methods for change point detection in sequential data”
Initial job placement: Research Scientist - Institute for Infocomm Research
Liao Zhu - “The adaptive multi-factor model and the financial market"
Initial job placement: Quantitative Researcher - Two Sigma
Xiaoyun Quan - “Latent Gaussian copula model for high dimensional mixed data, and its applications”
Dissertation Advisor: James Booth and Martin Wells
Praphruetpong (Ben) Athiwaratkun - "Density representations for words and hierarchical data"
Dissertation Advisor: Andrew Wilson
Initial job placement: AI Scientist - AWS AI Labs
Yiming Sun - “High dimensional data analysis with dependency and under limited memory”
Dissertation Advisor: Sumanta Basu and Madeleine Udell
Zi Ye - “Functional single index model and jensen effect"
Dissertation Advisor: Giles Hooker
Initial job placement: Data & Applied Scientist - Microsoft
Hui Fen (Sarah) Tan - “Interpretable approaches to opening up black-box models”
Dissertation Advisor: Giles Hooker and Martin Wells
Daniel E. Gilbert - “Luck, fairness and Bayesian tensor completion”
Yichen zhou - “asymptotics and interpretability of decision trees and decision tree ensemblesg”.
Initial job placement: Data Scientist - Google
Ze Jin - “Measuring statistical dependence and its applications in machine learning”
Initial job placement: Research Scientist, Facebook Integrity Ranking & ML - Facebook
Xiaohan Yan - “Statistical learning for structural patterns with trees”
Dissertation Advisor: Jacob Bien
Initial job placement: Senior Data Scientist - Microsoft
Guo Yu - “High-dimensional structured regression using convex optimization”
Dan kowal - "bayesian methods for functional and time series data".
Dissertation Advisor: David Matteson and David Ruppert
Initial job placement: assistant professor, Department of Statistics, Rice University
Keegan Kang - "Data Dependent Random Projections"
David sinclair - "model selection results for high dimensional graphical models on binary and count data with applications to fmri and genomics", liu, yanning – "statistical issues in the design and analysis of clinical trials".
Dissertation Advisor: Bruce Turnbull
Nicholson, William Bertil – "Tools for Modeling Sparse Vector Autoregressions"
Tupper, laura lindley – "topics in classification and clustering of high-dimensional data", chetelat, didier – "high-dimensional inference by unbiased risk estimation".
Initial Job Placement: Assistant Professor Universite de Montreal, Montreal, Canada
Gaynanova, Irina – "Estimation Of Sparse Low-Dimensional Linear Projections"
Dissertation Advisor: James Booth
Initial Job Placement: Assistant Professor, Texas A&M, College Station, TX
Mentch, Lucas – "Ensemble Trees and CLTS: Statistical Inference in Machine Learning"
Initial Job Placement: Assistant Professor, University of Pittsburgh, Pittsburgh, PA
Risk, Ben – "Topics in Independent Component Analysis, Likelihood Component Analysis, and Spatiotemporal Mixed Modeling"
Dissertation Advisors: David Matteson and David Ruppert
Initial Job Placement: Postdoctoral Fellow, University of North Carolina, Chapel Hill, NC
Zhao, Yue – "Contributions to the Statistical Inference for the Semiparametric Elliptical Copula Model"
Disseration Advisor: Marten Wegkamp
Initial Job Placement: Postoctoral Fellow, McGill University, Montreal, Canada
Chen, Maximillian Gene – "Dimension Reduction and Inferential Procedures for Images"
Dissertation Advisor: Martin Wells
Earls, Cecelia – Bayesian hierarchical Gaussian process models for functional data analysis
Dissertation Advisor: Giles Hooker
Initial Job Placement: Lecturer, Cornell University, Ithaca, NY
Li, James Yi-Wei – "Tensor (Multidimensional Array) Decomposition, Regression, and Software for Statistics and Machine Learning"
Initial Job Placement: Research Scientist, Yahoo Labs
Schneider, Matthew John – "Three Papers on Time Series Forecasting and Data Privacy"
Dissertation Advisor: John Abowd
Initial Job Placement: Assistant Professor, Northwestern University, Evanston, IL
Thorbergsson, Leifur – "Experimental design for partially observed Markov decision processes"
Initial Job Placement: Data Scientist, Memorial Sloan Kettering Cancer Center, New York, NY
Wan, Muting – "Model-Based Classification with Applications to High-Dimensional Data in Bioinformatics"
Initial Job Placement: Senior Associate, 1010 Data, New York, NY
Johnson, Lynn Marie – "Topics in Linear Models: Methods for Clustered, Censored Data and Two-Stage Sampling Designs"
Dissertation Advisor: Robert Strawderman
Initial Job Placement: Statistical Consultant, Cornell, Statistical Consulting Unit, Ithaca, NY
Tecuapetla Gomez, Inder Rafael – "Asymptotic Inference for Locally Stationary Processes"
Initial Job Placement: Postdoctoral Fellow, Georg-August-Universitat Gottigen, Gottigen, Germany.
Bar, Haim – "Parallel Testing, and Variable Selection -- a Mixture-Model Approach with Applications in Biostatistics"
Dissertation Advisor: James Booth
Initial Job Placement: Postdoc, Department of Medicine, Weill Medical Center, New York, NY
Cunningham, Caitlin – "Markov Methods for Identifying ChIP-seq Peaks"
Initial Job Placement: Assistant Professor, Le Moyne College, Syracuse, NY
Ji, Pengsheng – "Selected Topics in Nonparametric Testing and Variable Selection for High Dimensional Data"
Dissertation Advisor: Michael Nussbaum
Initial Job Placement: Assistant Professor, University of Georgia, Athens, GA
Morris, Darcy Steeg – "Methods for Multivariate Longitudinal Count and Duration Models with Applications in Economics"
Dissertation Advisor: Francesca Molinari
Initial Job Placement: Research Mathematical Statistician, Center for Statistical Research and Methodology, U.S. Census Bureau, Washington DC
Narayanan, Rajendran – "Shrinkage Estimation for Penalised Regression, Loss Estimation and Topics on Largest Eigenvalue Distributions"
Initial Job Placement: Visiting Scientist, Indian Statistical Institute, Kolkata, India
Xiao, Luo – "Topics in Bivariate Spline Smoothing"
Dissertation Advisor: David Ruppert
Initial Job Placement: Postdoc, Johns Hopkins University, Baltimore, MD
Zeber, David – "Extremal Properties of Markov Chains and the Conditional Extreme Value Model"
Dissertation Advisor: Sidney Resnick
Initial Job Placement: Data Analyst, Mozilla, San Francisco, CA
Clement, David – "Estimating equation methods for longitudinal and survival data"
Dissertation Advisor: Robert Strawderman
Initial Job Placement: Quantitative Analyst, Smartodds, London UK
Eilertson, Kirsten – "Estimation and inference of random effect models with applications to population genetics and proteomics"
Dissertation Advisor: Carlos Bustamante
Initial Job Placement: Biostatistician, The J. David Gladstone Institutes, San Francisco CA
Grabchak, Michael – "Tempered stable distributions: properties and extensions"
Dissertation Advisor: Gennady Samorodnitsky
Initial Job Placement: Assistant Professor, UNC Charlotte, Charlotte NC
Li, Yingxing – "Aspects of penalized splines"
Initial Job Placement: Assistant Professor, The Wang Yanan Institute for Studies in Economics, Xiamen University
Lopez Oliveros, Luis – "Modeling end-user behavior in data networks"
Dissertation Advisor: Sidney Resnick
Initial Job Placement: Consultant, Murex North America, New York NY
Ma, Xin – "Statistical Methods for Genome Variant Calling and Population Genetic Inference from Next-Generation Sequencing Data"
Initial Job Placement: Postdoc, Stanford University, Stanford CA
Kormaksson, Matthias – "Dynamic path analysis and model based clustering of microarray data"
Dissertation Advisor: James Booth
Initial Job Placement: Postdoc, Department of Public Health, Weill Cornell Medical College, New York NY
Schifano, Elizabeth – "Topics in penalized estimation"
Initial Job Placement: Postdoc, Department of Biostatistics, Harvard University, Boston MA
Hanlon, Bret – "High-dimensional data analysis"
Dissertation Advisor: Anand Vidyashankar
Shaby, Benjamin – "Tools for hard bayesian computations"
Initial Job Placement: Postdoc, SAMSI, Durham NC
Zipunnikov, Vadim – "Topics on generalized linear mixed models"
Initial Job Placement: Postdoc, Department of Biostatistics, Johns Hopkins University, Baltimore MD
Barger, Kathryn Jo-Anne – "Objective bayesian estimation for the number of classes in a population using Jeffreys and reference priors"
Dissertation Advisor: John Bunge
Initial Job Placement: Pfizer Incorporated
Chan, Serena Suewei – "Robust and efficient inference for linear mixed models using skew-normal distributions"
Initial Job Placement: Statistician, Takeda Pharmaceuticles, Deerfield IL
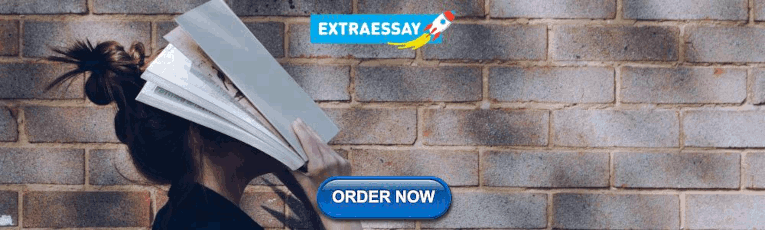
Lin, Haizhi – "Distressed debt prices and recovery rate estimation"
Dissertation Advisor: Martin Wells
Initial Job Placement: Associate, Fixed Income Department, Credit Suisse Securities (USA), New York, NY
Applied Statistics
Possible master's thesis.
Are you interested in writing a Master's thesis within the broad topic of Applied Statistics? On this website we describe what writing a Master's thesis in our group involves and list some projects opportunities.
Course of action
Contact us a few month before you plan to start you Master's thesis. We will then discuss the topic and technicalities with you. Before the work begins, we will provide you with a starting repository in Git containing additional information about available resources, template for the report, guidelines etc. You will fill out a thesis plan agreement where you record the goals of the thesis. Besides the supervision of Reinhard Furrer, you will also be assigned a PhD student who will provide additional help. You will work quite independently, but will have meetings with one of the supervisors approximately every two or three weeks to discuss your questions. There will be an intermediate and a final presentation. The intermediate presentation should be approximately halfway through your project. It serves to check if you are on the right track, and understood the problem, data, and hypotheses; and to train for the final presentation. The final presentation will take place after you have handed in the thesis.
The work of the thesis is tailored to 6 month (as awarded 30 ECTS). Under unforeseen circumstances (TA activities, sickness, ...) it might be possible to get an extension.
The report has to be written in LaTeX with knitr. We will provide you with a template and support you acquiring the necessary skills if you have never used with this report generating system.
Reproducibility
All work has to be done in a reproducible framework. We adhere to these guidelines .
Prerequisites
We strongly recommend the modules "STA402 Likelihood Inference" and "STA121 Statistical Modeling" or similar as prerequisites. In addition, we also recommend that you take the module "STA472 Good Satistical Practice", which teaches very useful technical and communication skills for the Master's thesis.
List of topics
The list below give an idea of prototypic MSc thesis. Depending on the background and the degree they may be further tailored.
Topics in Applied Statistics
Visit this page often as we try to keep the list up to date. Moreover, there are often "now or never" opportunities; we might have additional projects ready when you visit us.
Sample size estimation for mixed models
In animal experimentation often measurements are taken at several time points in the same animal during the study. Typical examples for such longitudinal data include the antibody titer in a vaccine trial, hemodynamic parameter during a clinical trial with different anesthetics, tumor growth at distinct time points in different treatment groups, and cross-over studies. Further complications for sample size estimation might occur due to the presence of additional covariates, the necessity for a baseline adjustment and heteroscedasticity. This type of data requires a statistical analysis which considers the potential clustering within animal, i.e. mixed effects models. A number of R packages have been developed for sample size estimation in the case of mixed models, but information on how to choose the most appropriate approach in specific situations is lacking. The aim of this Master's thesis is to provide guidance on the most appropriate methods for sample size estimation for different types of longitudinal data. Data sets from published studies as well as simulated data sets will be used for the assessment.
Bayesian modeling: model selection in the eggCounts model family
The prevalence of anthelmintic resistance has increased in recent years due to the extensive use of anthelmintic drugs to reduce the infection of parasitic worms in livestock. In order to detect the resistance, the number of parasite eggs in animal feces is counted. Typically a subsample of the diluted feces is examined, and the mean egg counts from both untreated and treated animals are compared. In the past, conventional methods have been extended by rather complex Bayesian hierarchical models that take into account the variabilities introduced by the counting process, different infection levels across animals, or extra zero counts, which arise as a result of the unexposed animals in an infected population or animals. Current practice in Germany for horses relies only very few animals only and the recently introduce methods may not have sufficient statistical power or are not suitable for sequential test procedures.
The goal of this Master thesis is to study and implement model selection guidelines for the eggCounts family.
Stan implementation of a parametric bootstrapping procedure for additive bayesian network analysis
Studying the causes and effects of health and disease conditions is the cornerstone of epidemiology. Classical approaches, such as regression techniques, have been successfully used to model the impact of health determinants on the population. However, recently there is a growing recognition of biological, behavioral factors, at multiple levels that can impact health condition. Those epidemiological data are, by nature, highly complex and correlated. Classical regression techniques have shown a limited ability to embrace high-dimensional epidemiological variables' correlated multivariate nature. Models driven by expert knowledge often fail to efficiently manage epidemiological data's complexity and correlation. Additive Bayesian Networks (ABNs) address those challenges in producing a data selected' set of multivariate models. It is known that overfitting is a limitation of an ABN analysis. Actually there is a well developed bunch of code which script a bootstrapping procedure dedicated to ABN in JAGS. Although, the computation time is typically very long. JAGS and Stan have slightly different strengths and limitations, but Stan is known to be slightly faster.
The goal of this Master thesis is to implement a bootstrapping procedure in Stan, to compare computing performance over simulated examples between a JAGS and a Stan implementation and implement the code as an R function that can be added to existing ABN R package.
Functional data ANOVA
The concept of an Analysis of Variance (ANOVA) for single observations is straightforward and quite intuitive from a statistical or geometrical point of view. However, if we observe an entire function instead, the concept needs to be extended.
The thesis studies and discusses existing approaches and illustrates these according to different datasets we have used in the past.
Topics in Spatial Statistics
We have a pretty long list of topics in spatial (and spatio-temporal) statistics. Most of these can be further tailored to fit the student's background. We recommend the module "STA330 Modeling Dependent Data" for these projects.
A non-exhaustive list is as follows:
Cholesky factorization of sparse matrices
In the setting of multivariate Gaussian random vectors, generating samples or evaluating the likelihood is computationally demanding, especially in large dimensions. An algorithm of choice is the Cholesky factorization of the underlying covariance matrix such that the determinant and the quadratic form of the density can be evaluated relatively fast. The main objective is a (numerical) complexity analysis of the Cholesky factorization implemented in the R package spam and compare the performance to alternative factorizations. Ideally, an existing approximate minimal degree algorithm will embedded in the spam environment.
Gaussian equivalent measures
In the context of a bounded and fixed domain, it is impossible to differentiate between specific covariance models based on data only. More specifically, two different Gaussian measures are equivalent. This fact is used to work with computationally simpler models. The theoretical assumptions for equivalence are explicit, but often practical recommendations still need to be elucidated. The thesis elaborates on the assumptions for specific parameter settings and incorporates a simulation study illustrating the practical aspect of the approximation.
Computational and statistical efficiency of a 32-bit Cholesky factorization
When working with Gaussian likelihoods, one is confronted with calculating the log-determinant of the covariance matrix and solving a linear system based on the covariance matrix. In case of sparse matrices, both steps can be efficiently accomplished after a Cholesky factorization (based on dedicated algorithms).
© Universität Zürich | Jul 3, 2023
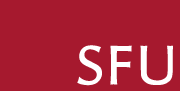
Statistics and Actuarial Science
Graduate theses.
- Statistics Workshop
- Actuarial Science
- Data Science
- Course Information
- Getting Involved
- Accreditation
- EAL and Other Resources
- Actuarial Science Info Session
- Statistics Admission
- Actuarial Science Admission
- Data Science Admission
- Moving to SFU
- Program Information
- Teaching Assistant Positions
- Intranet Grad Students
- Statistics M.Sc.
- Statistics Ph.D.
- Actuarial Science M.Sc.
- Tuition and Financial Support
- Academic Resources
- M.Sc. and Ph.D. Defences
- Statistical Consulting
- Graduate Students
- News and Events
Below is a list of the theses produced by graduate students in the Department of Statistics and Actuarial Science.
Projects and Theses From Previous Years
2015 - 2019 2010 - 2014 2005 - 2009 2000 - 2004 1990's 1980's and prior
Supervisors and topics for master's theses in statistics
As part of his or her master's study, a student should write a thesis. There are two options for the thesis: a long thesis corresponding to one year full time work, and a short thesis corresponding to one semester's full time work. The work on a long thesis typically starts in the second semester of the master's study (as described here ), while the work on a short thesis should be done during the last semester of the master's study. Below we give an overview of possible supervisors and topic for the master's thesis.
Riccardo De Bin
My main field of research is statistical learning ( STK-IN4300 ), with focus on methodological issues related to high-dimensional data. In particular, I am strongly interested in the statistical boosting, a method which combines the powerfulness of a machine-learning approach and the interpretability of a statistical model. In the same context of computational approaches, I am also interested in resampling-based methods for variable selection, data analysis and model averaging. In addition, I have also more theoretical interests, mainly in the field of asymptotic theory, where I am focusing on methods for the treatment of nuisance parameters.
Examples of master's theses that I have supervised:
Vegard Stikbakke. A boosting algorithm to extend first-hitting-time models to a high-dimensional survival setting , Long thesis, 2019.
Jonas Gjesvik. Statistical modelling of Goalkeepers in the Norwegian Tippeliga ,Long thesis, 2019.
Ingrid Kristine Glad
Over several years, my main research interest has been in developing methodology tailored for the analysis of high dimensional data, with links to topics in f.ex. STK-IN4300 .
I am heavily involved in the research for innovation center for big and complex data problems, BigInsight , as researcher and co-director. BigInsight would like to offer master projects within most BigInsight topics. For me it is most actual to supervise master projects related to high dimensional, high frequency sensor data, mainly from the maritime sector, see the BigInsight web pages (Innovation Objective Sensor Systems).
I have also been working for several years with high dimensional genomic data connected to cancer research. I can offer master projects related to statistical analysis of genomic and/or epigenetic data, where the high dimensionality of the data (especially p>n) leads to interesting methodological challenges.
Martin Tveten: Multi-Stream Sequential Change Detection Using Sparsity and Dimension Reduction , long thesis, 2017
Camilla Lingjærde: Tailored Graphical Lasso for Data Integration in Gene Network Reconstruction , long thesis, 2019
Ingrid Hobæk Haff
My main field of research over the past years has been multivariate modelling, in particular copulas, with applications within for instance finance, insurance and climate. I am also involved in BigInsight , as a researcher and co-director, and also supervise master’s theses with topics from this centre. More specifically I work on problems related to personalised fraud detection, i.e. constructing systems for uncovering tax fraud, insurance fraud and money laundering, which involves high-dimensional, imbalanced data. Further, I am engaged in the new convergence environment ImmunoLingo , a transdisciplinarily project, whose aim is to decipher the molecular language of adaptive immunity. I will also propose master thesis topics from this project.
Daniel Piacek: Detecting fraud using information from social networks . Long thesis, 2017
Eirik Lødøen Halsteinlid: Addressing collinearity and class imbalance in logistic regression for statistical fraud detection . Long thesis, 2019.
Nils Lid Hjort
I work in several areas of theoretical and applied statistics, with some key words being model building, model selection and model averaging, confidence distributions, estimation theory, survival analysis, Bayesian nonparametrics, stability and change. I led the research project group FocuStat with several PostDocs, PhDs, and Master level students , from 2014 to 2018, and several of our themes are continued, in partly new directions. Two projects, flowing from FocuStat themes, are From Processes to Models (ways of constructing better models for data) and Stability and Change (theory for finding changes, and conditions for stability, with application to war-and-peace data).
You may check the FocuStat webpage, including blog posts, with various themes that may also lead to Master thesis projects.
The majority of my students are working on the theoretical side of the spectrum, but from time to time I also supervise more applied projects (examples being recommender systems for finn.no; analysis of track and field data; examination of the forensic information used to convict Fredrik Fasting Torgersen for murder in 1958; the keeper's role in football matches; Markov chains for modelling escalation in armed conflicts).
If interested, check the list of Master- and PhD-students at the FocuStat website , which includes brief descriptions of and links to their projects and theses.
Sven Ove Samuelsen
My field is event history analysis (cf. STK4080 ) and in particular I have been interested in theoretical developments and applications in epidemiology. Some of the master’s theses I have supervised have had a focus on developments of case-control studies and similar epidemiological designs with a time to event perspective. Others master’s theses have been more directly connected to analyses of specific epidemiological data sets. The problems and data sets for the master’s theses often turn up in connection with collaborations with researchers at the Norwegian Institute of Public Health where I work part time. I am also involved in the strategic research initiative Pharmatox at the University of Oslo where we will study possible effects of medicines taken during pregnancy on neurodevelopment in childhood.
Morten Madshus: A Match Too Much? - A simulation study on overmatching in nested case-control studies. Long thesis 2019
Lena Johansen: Metoder og metodiske utfordringer for matchede kohortstudier. Long thesis 2018
Simon Lergenmuller: Two-stage predictor substitution for time-to-event data . Long thesis, 2017.
My research themes are often connected to Bayesian modelling and analysis, for example some type of space-time modelling or model diagnostics. I am a participant in the Research-based Innovation (SFI) BigInsight . Projects that are motivated by applications include one where we model the viral spread of products on social networks, a project which is in collaboration with Telenor. Another concerns a new Bayesian recommender system for clicking data. ‘Clicking data’, the history that consumers have of clicking on webpages, reveal their preferences. Such data arise in very many areas in the digital world, including business (e.g. a company selling products online) and entertainment (e.g. Spotify, Netflix, NRK). A recommender system aims at personalised recommendations based on the history of the consumer and other consumers. For this project we collaborate both with NRK and finn.no. More theoretically motivated is my interest in model diagnostics, mainly concerning checking for possible modelling conflicts at the node-level of (Bayesian) hierarchical models. A possible master project could be connected to this field.
Examples of master’s theses that I have supervised:
Jonas Fredrik Schenkel: Collaborative Filtering for Implicit Feedba ck: Investigating how to improve NRK TV's recommender system by including context . Long thesis, 2017.
Jenine Gaspar Corrales: Analyzing and Predicting Demographics of NRK's Digital Users . Long thesis, 2019.
Geir Storvik
My main fields of research are (Bayesian) computational statistics and Monte Carlo methods. In particular, analysis of data that have a dependence structure in time and/or space is central in many of my projects. Such problems impose challenges both at the modelling stage and with respect to computation. I am involved in CELS , a multidisciplinary center for computational inference in evolutionary life science at the University of Oslo. Problems considered are within ecology, evolution but also forensic science. Analysis of big data is also possible through my engagement in BigInsight , a new center for Research-based Innovation (SFI), where huge amounts of multivariate sensor data needs to be analysed. I also have a part time position at Norwegian Computing Center (Norsk Regnesentral) where I currently am involved in projects concerning marine resources (estimation of abundance etc).
Possible master-project
- Useful background: STK4021 , STK4051
Martin Gjesdal Bjørndal: Distance Metrics in Variant Graphs , Long thesis, 2017
Kjersti Moss: The Poisson-Binomial Model for Fish Abundance Estimation: With Applications to Northeast Arctic cod . Long thesis, 2015
- Del på Facebook
- Del på Twitter
- Privacy Policy
Buy Me a Coffee

Home » 500+ Statistics Research Topics
500+ Statistics Research Topics

Statistics is a branch of mathematics that deals with the collection, analysis, interpretation, presentation, and organization of data . It is a fundamental tool used in various fields such as business, social sciences, engineering, healthcare, and many more. As a research topic , statistics can be a fascinating subject to explore, as it allows researchers to investigate patterns, trends, and relationships within data. With the help of statistical methods, researchers can make informed decisions and draw valid conclusions based on empirical evidence. In this post, we will explore some interesting statistics research topics that can be pursued by researchers to further expand our understanding of this field.
Statistics Research Topics
Statistics Research Topics are as follows:
- Analysis of the effectiveness of different marketing strategies on consumer behavior.
- An investigation into the relationship between economic growth and environmental sustainability.
- A study of the effects of social media on mental health and well-being.
- A comparative analysis of the educational outcomes of public and private schools.
- The impact of climate change on agriculture and food security.
- A survey of the prevalence and causes of workplace stress in different industries.
- A statistical analysis of crime rates in urban and rural areas.
- An evaluation of the effectiveness of alternative medicine treatments.
- A study of the relationship between income inequality and health outcomes.
- A comparative analysis of the effectiveness of different weight loss programs.
- An investigation into the factors that affect job satisfaction among employees.
- A statistical analysis of the relationship between poverty and crime.
- A study of the factors that influence the success of small businesses.
- A survey of the prevalence and causes of childhood obesity.
- An evaluation of the effectiveness of drug addiction treatment programs.
- A statistical analysis of the relationship between gender and leadership in organizations.
- A study of the relationship between parental involvement and academic achievement.
- An investigation into the causes and consequences of income inequality.
- A comparative analysis of the effectiveness of different types of therapy for mental health conditions.
- A survey of the prevalence and causes of substance abuse among teenagers.
- An evaluation of the effectiveness of online education compared to traditional classroom learning.
- A statistical analysis of the impact of globalization on different industries.
- A study of the relationship between social media use and political polarization.
- An investigation into the factors that influence customer loyalty in the retail industry.
- A comparative analysis of the effectiveness of different types of advertising.
- A survey of the prevalence and causes of workplace discrimination.
- An evaluation of the effectiveness of different types of employee training programs.
- A statistical analysis of the relationship between air pollution and health outcomes.
- A study of the factors that affect employee turnover rates.
- An investigation into the causes and consequences of income mobility.
- A comparative analysis of the effectiveness of different types of leadership styles.
- A survey of the prevalence and causes of mental health disorders among college students.
- An evaluation of the effectiveness of different types of cancer treatments.
- A statistical analysis of the impact of social media influencers on consumer behavior.
- A study of the factors that influence the adoption of renewable energy sources.
- An investigation into the relationship between alcohol consumption and health outcomes.
- A comparative analysis of the effectiveness of different types of conflict resolution strategies.
- A survey of the prevalence and causes of childhood poverty.
- An evaluation of the effectiveness of different types of diversity training programs.
- A statistical analysis of the relationship between immigration and economic growth.
- A study of the factors that influence customer satisfaction in the service industry.
- An investigation into the causes and consequences of urbanization.
- A comparative analysis of the effectiveness of different types of economic policies.
- A survey of the prevalence and causes of elder abuse.
- An evaluation of the effectiveness of different types of rehabilitation programs for prisoners.
- A statistical analysis of the impact of automation on different industries.
- A study of the factors that influence employee productivity in the workplace.
- An investigation into the causes and consequences of gentrification.
- A comparative analysis of the effectiveness of different types of humanitarian aid.
- A survey of the prevalence and causes of homelessness.
- Exploring the relationship between socioeconomic status and access to healthcare services
- An analysis of the relationship between parental education level and children’s academic performance.
- Exploring the effects of different statistical models on prediction accuracy in machine learning.
- The Impact of Social Media on Consumer Behavior: A Statistical Analysis
- Bayesian hierarchical modeling for network data analysis
- Spatial statistics and modeling for environmental data
- Nonparametric methods for time series analysis
- Bayesian inference for high-dimensional data analysis
- Multivariate analysis for genetic data
- Machine learning methods for predicting financial markets
- Causal inference in observational studies
- Sampling design and estimation for complex surveys
- Robust statistical methods for outlier detection
- Statistical inference for large-scale simulations
- Survival analysis and its applications in medical research
- Mixture models for clustering and classification
- Time-varying coefficient models for longitudinal data
- Multilevel modeling for complex data structures
- Graphical modeling and Bayesian networks
- Experimental design for clinical trials
- Inference for network data using stochastic block models
- Nonlinear regression modeling for data with complex structures
- Statistical learning for social network analysis
- Time series forecasting using deep learning methods
- Model selection and variable importance in high-dimensional data
- Spatial point process modeling for environmental data
- Bayesian spatial modeling for disease mapping
- Functional data analysis for longitudinal studies
- Bayesian network meta-analysis
- Statistical methods for big data analysis
- Mixed-effects models for longitudinal data
- Clustering algorithms for text data
- Bayesian modeling for spatiotemporal data
- Multivariate analysis for ecological data
- Statistical analysis of genomic data
- Bayesian network inference for gene regulatory networks
- Principal component analysis for high-dimensional data
- Time series analysis of financial data
- Multivariate survival analysis for complex outcomes
- Nonparametric estimation of causal effects
- Bayesian network analysis of complex systems
- Statistical inference for multilevel network data
- Generalized linear mixed models for non-normal data
- Bayesian inference for dynamic systems
- Latent variable modeling for categorical data
- Statistical inference for social network data
- Regression models for panel data
- Bayesian spatiotemporal modeling for climate data
- Predictive modeling for customer behavior analysis
- Nonlinear time series analysis for ecological systems
- Statistical modeling for image analysis
- Bayesian hierarchical modeling for longitudinal data
- Network-based clustering for high-dimensional data
- Bayesian spatial modeling for ecological systems.
- Analysis of the Effect of Climate Change on Crop Yields: A Case Study
- Examining the Relationship Between Physical Activity and Mental Health in Young Adults
- A Comparative Study of Crime Rates in Urban and Rural Areas Using Statistical Methods
- Investigating the Effect of Online Learning on Student Performance in Mathematics
- A Statistical Analysis of the Relationship Between Economic Growth and Environmental Sustainability
- Evaluating the Effectiveness of Different Marketing Strategies for E-commerce Businesses
- Identifying the Key Factors Affecting Customer Loyalty in the Hospitality Industry
- An Analysis of the Factors Influencing Student Dropout Rates in Higher Education
- Examining the Impact of Gender on Salary Disparities in the Workplace Using Statistical Methods
- Investigating the Relationship Between Physical Fitness and Academic Performance in High School Students
- Analyzing the Effect of Social Support on Mental Health in Elderly Populations
- A Comparative Study of Different Methods for Forecasting Stock Prices
- Investigating the Effect of Online Reviews on Consumer Purchasing Decisions
- Identifying the Key Factors Affecting Employee Turnover Rates in the Technology Industry
- Analyzing the Effect of Advertising on Brand Awareness and Purchase Intentions
- A Study of the Relationship Between Health Insurance Coverage and Healthcare Utilization
- Examining the Effect of Parental Involvement on Student Achievement in Elementary School
- Investigating the Impact of Social Media on Political Campaigns Using Statistical Methods
- A Comparative Analysis of Different Methods for Detecting Fraud in Financial Transactions
- Analyzing the Relationship Between Entrepreneurial Characteristics and Business Success
- Investigating the Effect of Job Satisfaction on Employee Performance in the Service Industry
- Identifying the Key Factors Affecting the Adoption of Renewable Energy Technologies
- A Study of the Relationship Between Personality Traits and Academic Achievement
- Examining the Impact of Social Media on Body Image and Self-Esteem in Adolescents
- Investigating the Effect of Mobile Advertising on Consumer Behavior
- Analyzing the Relationship Between Healthcare Expenditures and Health Outcomes Using Statistical Methods
- A Comparative Study of Different Methods for Analyzing Customer Satisfaction Data
- Investigating the Impact of Economic Factors on Voter Behavior Using Statistical Methods
- Identifying the Key Factors Affecting Student Retention Rates in Community Colleges
- Analyzing the Relationship Between Workplace Diversity and Organizational Performance
- Investigating the Effect of Gamification on Learning and Motivation in Education
- A Study of the Relationship Between Social Support and Depression in Cancer Patients
- Examining the Impact of Technology on the Travel Industry Using Statistical Methods
- Investigating the Effect of Customer Service Quality on Customer Loyalty in the Retail Industry
- Analyzing the Relationship Between Internet Usage and Social Isolation in Older Adults
- A Comparative Study of Different Methods for Predicting Customer Churn in Telecommunications
- Investigating the Impact of Social Media on Consumer Attitudes Towards Brands Using Statistical Methods
- Identifying the Key Factors Affecting Student Success in Online Learning Environments
- Analyzing the Relationship Between Employee Engagement and Organizational Commitment
- Investigating the Effect of Customer Reviews on Sales in E-commerce Businesses
- A Study of the Relationship Between Political Ideology and Attitudes Towards Climate Change
- Examining the Impact of Technological Innovations on the Manufacturing Industry Using Statistical Methods
- Investigating the Effect of Social Support on Postpartum Depression in New Mothers
- Analyzing the Relationship Between Cultural Intelligence and Cross-Cultural Adaptation
- Investigating the relationship between socioeconomic status and health outcomes using statistical methods.
- Analyzing trends in crime rates and identifying factors that contribute to them using statistical methods.
- Examining the effectiveness of different advertising strategies using statistical analysis of consumer behavior.
- Identifying factors that influence voting behavior and election outcomes using statistical methods.
- Investigating the relationship between employee satisfaction and productivity in the workplace using statistical methods.
- Developing new statistical models to better understand the spread of infectious diseases.
- Analyzing the impact of climate change on global food production using statistical methods.
- Identifying patterns and trends in social media data using statistical methods.
- Investigating the relationship between social networks and mental health using statistical methods.
- Developing new statistical models to predict financial market trends and identify investment opportunities.
- Analyzing the effectiveness of different educational programs and interventions using statistical methods.
- Investigating the impact of environmental factors on public health using statistical methods.
- Developing new statistical models to analyze complex biological systems and identify new drug targets.
- Analyzing trends in consumer spending and identifying factors that influence buying behavior using statistical methods.
- Investigating the relationship between diet and health outcomes using statistical methods.
- Developing new statistical models to analyze gene expression data and identify biomarkers for disease.
- Analyzing patterns in crime data to predict future crime rates and improve law enforcement strategies.
- Investigating the effectiveness of different medical treatments using statistical methods.
- Developing new statistical models to analyze the impact of air pollution on public health.
- Analyzing trends in global migration and identifying factors that influence migration patterns using statistical methods.
- Investigating the impact of automation on the job market using statistical methods.
- Developing new statistical models to analyze climate data and predict future climate trends.
- Analyzing trends in online shopping behavior and identifying factors that influence consumer decisions using statistical methods.
- Investigating the impact of social media on political discourse using statistical methods.
- Developing new statistical models to analyze gene-environment interactions and identify new disease risk factors.
- Analyzing trends in the stock market and identifying factors that influence investment decisions using statistical methods.
- Investigating the impact of early childhood education on long-term academic and social outcomes using statistical methods.
- Developing new statistical models to analyze the relationship between human behavior and the environment.
- Analyzing trends in the use of renewable energy and identifying factors that influence adoption rates using statistical methods.
- Investigating the impact of immigration on labor market outcomes using statistical methods.
- Developing new statistical models to analyze the relationship between social determinants and health outcomes.
- Analyzing patterns in customer churn to predict future customer behavior and improve business strategies.
- Investigating the effectiveness of different marketing strategies using statistical methods.
- Developing new statistical models to analyze the relationship between air pollution and climate change.
- Analyzing trends in global tourism and identifying factors that influence travel behavior using statistical methods.
- Investigating the impact of social media on mental health using statistical methods.
- Developing new statistical models to analyze the impact of transportation on the environment.
- Analyzing trends in global trade and identifying factors that influence trade patterns using statistical methods.
- Investigating the impact of social networks on political participation using statistical methods.
- Developing new statistical models to analyze the relationship between climate change and biodiversity loss.
- Analyzing trends in the use of alternative medicine and identifying factors that influence adoption rates using statistical methods.
- Investigating the impact of technological change on the labor market using statistical methods.
- Developing new statistical models to analyze the impact of climate change on agriculture.
- Investigating the impact of social media on mental health: A longitudinal study.
- A comparison of the effectiveness of different types of teaching methods on student learning outcomes.
- Examining the relationship between sleep duration and productivity among college students.
- A study of the factors that influence employee job satisfaction in the tech industry.
- Analyzing the relationship between income level and health outcomes among low-income populations.
- Investigating the effectiveness of online learning platforms for high school students.
- A study of the factors that contribute to success in online entrepreneurship.
- Analyzing the impact of climate change on agricultural productivity in developing countries.
- A comparison of different statistical models for predicting stock market trends.
- Examining the impact of sports on mental health: A cross-sectional study.
- A study of the factors that influence employee retention in the hospitality industry.
- Analyzing the impact of cultural differences on international business negotiations.
- Investigating the effectiveness of different weight loss interventions for obese individuals.
- A study of the relationship between personality traits and academic achievement.
- Examining the impact of technology on job displacement: A longitudinal study.
- A comparison of the effectiveness of different types of advertising strategies on consumer behavior.
- Analyzing the impact of environmental regulations on corporate profitability.
- Investigating the effectiveness of different types of therapy for treating depression.
- A study of the factors that contribute to success in e-commerce.
- Examining the relationship between social support and mental health in the elderly population.
- A comparison of different statistical methods for analyzing complex survey data.
- Analyzing the impact of employee diversity on organizational performance.
- Investigating the effectiveness of different types of exercise for improving cardiovascular health.
- A study of the relationship between emotional intelligence and job performance.
- Examining the impact of work-life balance on employee well-being.
- A comparison of the effectiveness of different types of financial education programs for low-income populations.
- Analyzing the impact of air pollution on respiratory health in urban areas.
- Investigating the relationship between personality traits and leadership effectiveness.
- A study of the factors that influence consumer behavior in the luxury goods market.
- Examining the impact of social networks on political participation: A cross-sectional study.
- A comparison of different statistical methods for analyzing survival data.
- Analyzing the impact of government policies on income inequality.
- Investigating the effectiveness of different types of counseling for substance abuse.
- A study of the relationship between cultural values and consumer behavior.
- Examining the impact of technology on privacy: A longitudinal study.
- A comparison of the effectiveness of different types of online marketing strategies.
- Analyzing the impact of the gig economy on job satisfaction: A cross-sectional study.
- Investigating the effectiveness of different types of education interventions for improving financial literacy.
- A study of the factors that contribute to success in social entrepreneurship.
- Examining the impact of gender diversity on board performance in publicly-traded companies.
- A comparison of different statistical methods for analyzing panel data.
- Analyzing the impact of employee involvement in decision-making on organizational performance.
- Investigating the effectiveness of different types of treatment for anxiety disorders.
- A study of the relationship between cultural values and entrepreneurial success.
- Examining the impact of technology on the labor market: A longitudinal study.
- A comparison of the effectiveness of different types of direct mail campaigns.
- Analyzing the impact of telecommuting on employee productivity: A cross-sectional study.
- Investigating the effectiveness of different types of retirement planning interventions for low-income individuals.
- Analyzing the effectiveness of different educational interventions in improving student performance
- Investigating the impact of climate change on food production and food security
- Identifying factors that influence employee satisfaction and productivity in the workplace
- Examining the prevalence and causes of mental health disorders in different populations
- Evaluating the effectiveness of different marketing strategies in promoting consumer behavior
- Analyzing the prevalence and consequences of substance abuse in different communities
- Investigating the relationship between social media use and mental health outcomes
- Examining the role of genetics in the development of different diseases
- Identifying factors that contribute to the gender wage gap in different industries
- Analyzing the effectiveness of different policing strategies in reducing crime rates
- Investigating the impact of immigration on economic growth and development
- Examining the prevalence and causes of domestic violence in different populations
- Evaluating the effectiveness of different interventions for treating addiction
- Analyzing the prevalence and impact of childhood obesity on health outcomes
- Investigating the relationship between diet and chronic diseases such as diabetes and heart disease
- Examining the effects of different types of exercise on physical and mental health outcomes
- Identifying factors that influence voter behavior and political participation
- Analyzing the prevalence and impact of sleep disorders on health outcomes
- Investigating the effectiveness of different educational interventions in improving health outcomes
- Examining the impact of environmental pollution on public health outcomes
- Evaluating the effectiveness of different interventions for reducing opioid addiction and overdose rates
- Analyzing the prevalence and causes of homelessness in different communities
- Investigating the relationship between race and health outcomes
- Examining the impact of social support networks on health outcomes
- Identifying factors that contribute to income inequality in different regions
- Analyzing the prevalence and impact of workplace stress on employee health outcomes
- Investigating the relationship between education and income levels in different communities
- Examining the effects of different types of technology on mental health outcomes
- Evaluating the effectiveness of different interventions for reducing healthcare costs
- Analyzing the prevalence and impact of chronic pain on health outcomes
- Investigating the relationship between urbanization and public health outcomes
- Examining the effects of different types of drugs on health outcomes
- Identifying factors that contribute to educational attainment in different populations
- Analyzing the prevalence and causes of food insecurity in different communities
- Investigating the relationship between race and crime rates
- Examining the impact of social media on political participation and engagement
- Evaluating the effectiveness of different interventions for reducing poverty levels
- Analyzing the prevalence and impact of stress on mental health outcomes
- Investigating the relationship between religion and health outcomes
- Examining the effects of different types of parenting styles on child development outcomes
- Identifying factors that contribute to political polarization in different regions
- Analyzing the prevalence and causes of teenage pregnancy in different communities
- Investigating the impact of globalization on economic growth and development
- Examining the prevalence and impact of social isolation on mental health outcomes
- Evaluating the effectiveness of different interventions for reducing gun violence
- Analyzing the prevalence and impact of bullying on mental health outcomes
- Investigating the relationship between immigration and crime rates
- Examining the effects of different types of diets on health outcomes
- Identifying factors that contribute to social inequality in different regions
- Bayesian inference for high-dimensional models
- Analysis of longitudinal data with missing values
- Nonparametric regression with functional predictors
- Estimation and inference for copula models
- Statistical methods for neuroimaging data analysis
- Robust methods for high-dimensional data analysis
- Analysis of spatially correlated data
- Bayesian nonparametric modeling
- Statistical methods for network data
- Optimal experimental design for nonlinear models
- Multivariate time series analysis
- Inference for partially identified models
- Statistical learning for personalized medicine
- Statistical inference for rare events
- High-dimensional mediation analysis
- Analysis of multi-omics data
- Nonparametric regression with mixed types of predictors
- Estimation and inference for graphical models
- Statistical inference for infectious disease dynamics
- Robust methods for high-dimensional covariance matrix estimation
- Analysis of spatio-temporal data
- Bayesian modeling for ecological data
- Multivariate spatial point pattern analysis
- Statistical methods for functional magnetic resonance imaging (fMRI) data
- Nonparametric estimation of conditional distributions
- Statistical methods for spatial econometrics
- Inference for stochastic processes
- Bayesian spatiotemporal modeling
- High-dimensional causal inference
- Analysis of data from complex survey designs
- Bayesian nonparametric survival analysis
- Statistical methods for fMRI connectivity analysis
- Spatial quantile regression
- Statistical modeling for climate data
- Estimation and inference for item response models
- Bayesian model selection and averaging
- High-dimensional principal component analysis
- Analysis of data from clinical trials with noncompliance
- Nonparametric regression with censored data
- Statistical methods for functional data analysis
- Inference for network models
- Bayesian nonparametric clustering
- High-dimensional classification
- Analysis of ecological network data
- Statistical modeling for time-to-event data with multiple events
- Estimation and inference for nonparametric density estimation
- Bayesian nonparametric regression with time-varying coefficients
- Statistical methods for functional magnetic resonance spectroscopy (fMRS) data
About the author
Muhammad Hassan
Researcher, Academic Writer, Web developer
You may also like

200+ Funny Research Topics

500+ Sports Research Topics

300+ American History Research Paper Topics

500+ Cyber Security Research Topics

500+ Environmental Research Topics

500+ Economics Research Topics

Top 99+ Trending Statistics Research Topics for Students
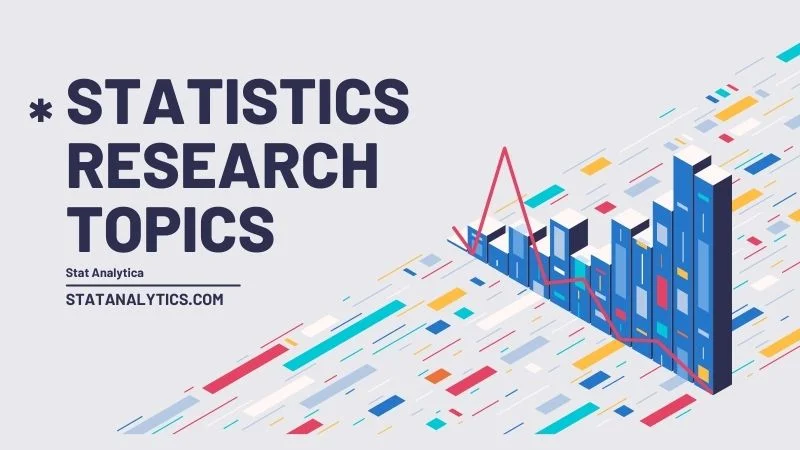
Being a statistics student, finding the best statistics research topics is quite challenging. But not anymore; find the best statistics research topics now!!!
Statistics is one of the tough subjects because it consists of lots of formulas, equations and many more. Therefore the students need to spend their time to understand these concepts. And when it comes to finding the best statistics research project for their topics, statistics students are always looking for someone to help them.
In this blog, we will share with you the most interesting and trending statistics research topics in 2023. It will not just help you to stand out in your class but also help you to explore more about the world.
If you face any problem regarding statistics, then don’t worry. You can get the best statistics assignment help from one of our experts.
As you know, it is always suggested that you should work on interesting topics. That is why we have mentioned the most interesting research topics for college students and high school students. Here in this blog post, we will share with you the list of 99+ awesome statistics research topics.
Why Do We Need to Have Good Statistics Research Topics?
Table of Contents
Having a good research topic will not just help you score good grades, but it will also allow you to finish your project quickly. Because whenever we work on something interesting, our productivity automatically boosts. Thus, you need not invest lots of time and effort, and you can achieve the best with minimal effort and time.
What Are Some Interesting Research Topics?
If we talk about the interesting research topics in statistics, it can vary from student to student. But here are the key topics that are quite interesting for almost every student:-
- Literacy rate in a city.
- Abortion and pregnancy rate in the USA.
- Eating disorders in the citizens.
- Parent role in self-esteem and confidence of the student.
- Uses of AI in our daily life to business corporates.
Top 99+ Trending Statistics Research Topics For 2023
Here in this section, we will tell you more than 99 trending statistics research topics:
Sports Statistics Research Topics
- Statistical analysis for legs and head injuries in Football.
- Statistical analysis for shoulder and knee injuries in MotoGP.
- Deep statistical evaluation for the doping test in sports from the past decade.
- Statistical observation on the performance of athletes in the last Olympics.
- Role and effect of sports in the life of the student.
Psychology Research Topics for Statistics
- Deep statistical analysis of the effect of obesity on the student’s mental health in high school and college students.
- Statistical evolution to find out the suicide reason among students and adults.
- Statistics analysis to find out the effect of divorce on children in a country.
- Psychology affects women because of the gender gap in specific country areas.
- Statistics analysis to find out the cause of online bullying in students’ lives.
- In Psychology, PTSD and descriptive tendencies are discussed.
- The function of researchers in statistical testing and probability.
- Acceptable significance and probability thresholds in clinical Psychology.
- The utilization of hypothesis and the role of P 0.05 for improved comprehension.
- What types of statistical data are typically rejected in psychology?
- The application of basic statistical principles and reasoning in psychological analysis.
- The role of correlation is when several psychological concepts are at risk.
- Actual case study learning and modeling are used to generate statistical reports.
- In psychology, naturalistic observation is used as a research sample.
- How should descriptive statistics be used to represent behavioral data sets?
Applied Statistics Research Topics
- Does education have a deep impact on the financial success of an individual?
- The investment in digital technology is having a meaningful return for corporations?
- The gap of financial wealth between rich and poor in the USA.
- A statistical approach to identify the effects of high-frequency trading in financial markets.
- Statistics analysis to determine the impact of the multi-agent model in financial markets.
Personalized Medicine Statistics Research Topics
- Statistical analysis on the effect of methamphetamine on substance abusers.
- Deep research on the impact of the Corona vaccine on the Omnicrone variant.
- Find out the best cancer treatment approach between orthodox therapies and alternative therapies.
- Statistics analysis to identify the role of genes in the child’s overall immunity.
- What factors help the patients to survive from Coronavirus .
Experimental Design Statistics Research Topics
- Generic vs private education is one of the best for the students and has better financial return.
- Psychology vs physiology: which leads the person not to quit their addictions?
- Effect of breastmilk vs packed milk on the infant child overall development
- Which causes more accidents: male alcoholics vs female alcoholics.
- What causes the student not to reveal the cyberbullying in front of their parents in most cases.
Easy Statistics Research Topics
- Application of statistics in the world of data science
- Statistics for finance: how statistics is helping the company to grow their finance
- Advantages and disadvantages of Radar chart
- Minor marriages in south-east Asia and African countries.
- Discussion of ANOVA and correlation.
- What statistical methods are most effective for active sports?
- When measuring the correctness of college tests, a ranking statistical approach is used.
- Statistics play an important role in Data Mining operations.
- The practical application of heat estimation in engineering fields.
- In the field of speech recognition, statistical analysis is used.
- Estimating probiotics: how much time is necessary for an accurate statistical sample?
- How will the United States population grow in the next twenty years?
- The legislation and statistical reports deal with contentious issues.
- The application of empirical entropy approaches with online grammar checking.
- Transparency in statistical methodology and the reporting system of the United States Census Bureau.
Statistical Research Topics for High School
- Uses of statistics in chemometrics
- Statistics in business analytics and business intelligence
- Importance of statistics in physics.
- Deep discussion about multivariate statistics
- Uses of Statistics in machine learning
Survey Topics for Statistics
- Gather the data of the most qualified professionals in a specific area.
- Survey the time wasted by the students in watching Tvs or Netflix.
- Have a survey the fully vaccinated people in the USA
- Gather information on the effect of a government survey on the life of citizens
- Survey to identify the English speakers in the world.
Statistics Research Paper Topics for Graduates
- Have a deep decision of Bayes theorems
- Discuss the Bayesian hierarchical models
- Analysis of the process of Japanese restaurants.
- Deep analysis of Lévy’s continuity theorem
- Analysis of the principle of maximum entropy
AP Statistics Topics
- Discuss about the importance of econometrics
- Analyze the pros and cons of Probit Model
- Types of probability models and their uses
- Deep discussion of ortho stochastic matrix
- Find out the ways to get an adjacency matrix quickly
Good Statistics Research Topics
- National income and the regulation of cryptocurrency.
- The benefits and drawbacks of regression analysis.
- How can estimate methods be used to correct statistical differences?
- Mathematical prediction models vs observation tactics.
- In sociology research, there is bias in quantitative data analysis.
- Inferential analytical approaches vs. descriptive statistics.
- How reliable are AI-based methods in statistical analysis?
- The internet news reporting and the fluctuations: statistics reports.
- The importance of estimate in modeled statistics and artificial sampling.
Business Statistics Topics
- Role of statistics in business in 2023
- Importance of business statistics and analytics
- What is the role of central tendency and dispersion in statistics
- Best process of sampling business data.
- Importance of statistics in big data.
- The characteristics of business data sampling: benefits and cons of software solutions.
- How may two different business tasks be tackled concurrently using linear regression analysis?
- In economic data relations, index numbers, random probability, and correctness are all important.
- The advantages of a dataset approach to statistics in programming statistics.
- Commercial statistics: how should the data be prepared for maximum accuracy?
Statistical Research Topics for College Students
- Evaluate the role of John Tukey’s contribution to statistics.
- The role of statistics to improve ADHD treatment.
- The uses and timeline of probability in statistics.
- Deep analysis of Gertrude Cox’s experimental design in statistics.
- Discuss about Florence Nightingale in statistics.
- What sorts of music do college students prefer?
- The Main Effect of Different Subjects on Student Performance.
- The Importance of Analytics in Statistics Research.
- The Influence of a Better Student in Class.
- Do extracurricular activities help in the transformation of personalities?
- Backbenchers’ Impact on Class Performance.
- Medication’s Importance in Class Performance.
- Are e-books better than traditional books?
- Choosing aspects of a subject in college
How To Write Good Statistics Research Topics?
So, the main question that arises here is how you can write good statistics research topics. The trick is understanding the methodology that is used to collect and interpret statistical data. However, if you are trying to pick any topic for your statistics project, you must think about it before going any further.
As a result, it will teach you about the data types that will be researched because the sample will be chosen correctly. On the other hand, your basic outline for choosing the correct topics is as follows:
- Introduction of a problem
- Methodology explanation and choice.
- Statistical research itself is in the main part (Body Part).
- Samples deviations and variables.
- Lastly, statistical interpretation is your last part (conclusion).
Note: Always include the sources from which you obtained the statistics data.
Top 3 Tips to Choose Good Statistics Research Topics
It can be quite easy for some students to pick a good statistics research topic without the help of an essay writer. But we know that it is not a common scenario for every student. That is why we will mention some of the best tips that will help you choose good statistics research topics for your next project. Either you are in a hurry or have enough time to explore. These tips will help you in every scenario.
1. Narrow down your research topic
We all start with many topics as we are not sure about our specific interests or niche. The initial step to picking up a good research topic for college or school students is to narrow down the research topic.
For this, you need to categorize the matter first. And then pick a specific category as per your interest. After that, brainstorm about the topic’s content and how you can make the points catchy, focused, directional, clear, and specific.
2. Choose a topic that gives you curiosity
After categorizing the statistics research topics, it is time to pick one from the category. Don’t pick the most common topic because it will not help your grades and knowledge. Instead of it, please choose the best one, in which you have little information, or you are more likely to explore it.
In a statistics research paper, you always can explore something beyond your studies. By doing this, you will be more energetic to work on this project. And you will also feel glad to get them lots of information you were willing to have but didn’t get because of any reasons.
It will also make your professor happy to see your work. Ultimately it will affect your grades with a positive attitude.
3. Choose a manageable topic
Now you have decided on the topic, but you need to make sure that your research topic should be manageable. You will have limited time and resources to complete your project if you pick one of the deep statistics research topics with massive information.
Then you will struggle at the last moment and most probably not going to finish your project on time. Therefore, spend enough time exploring the topic and have a good idea about the time duration and resources you will use for the project.
Statistics research topics are massive in numbers. Because statistics operations can be performed on anything from our psychology to our fitness. Therefore there are lots more statistics research topics to explore. But if you are not finding it challenging, then you can take the help of our statistics experts . They will help you to pick the most interesting and trending statistics research topics for your projects.
With this help, you can also save your precious time to invest it in something else. You can also come up with a plethora of topics of your choice and we will help you to pick the best one among them. Apart from that, if you are working on a project and you are not sure whether that is the topic that excites you to work on it or not. Then we can also help you to clear all your doubts on the statistics research topic.
Frequently Asked Questions
Q1. what are some good topics for the statistics project.
Have a look at some good topics for statistics projects:- 1. Research the average height and physics of basketball players. 2. Birth and death rate in a specific city or country. 3. Study on the obesity rate of children and adults in the USA. 4. The growth rate of China in the past few years 5. Major causes of injury in Football
Q2. What are the topics in statistics?
Statistics has lots of topics. It is hard to cover all of them in a short answer. But here are the major ones: conditional probability, variance, random variable, probability distributions, common discrete, and many more.
Q3. What are the top 10 research topics?
Here are the top 10 research topics that you can try in 2023:
1. Plant Science 2. Mental health 3. Nutritional Immunology 4. Mood disorders 5. Aging brains 6. Infectious disease 7. Music therapy 8. Political misinformation 9. Canine Connection 10. Sustainable agriculture
Related Posts
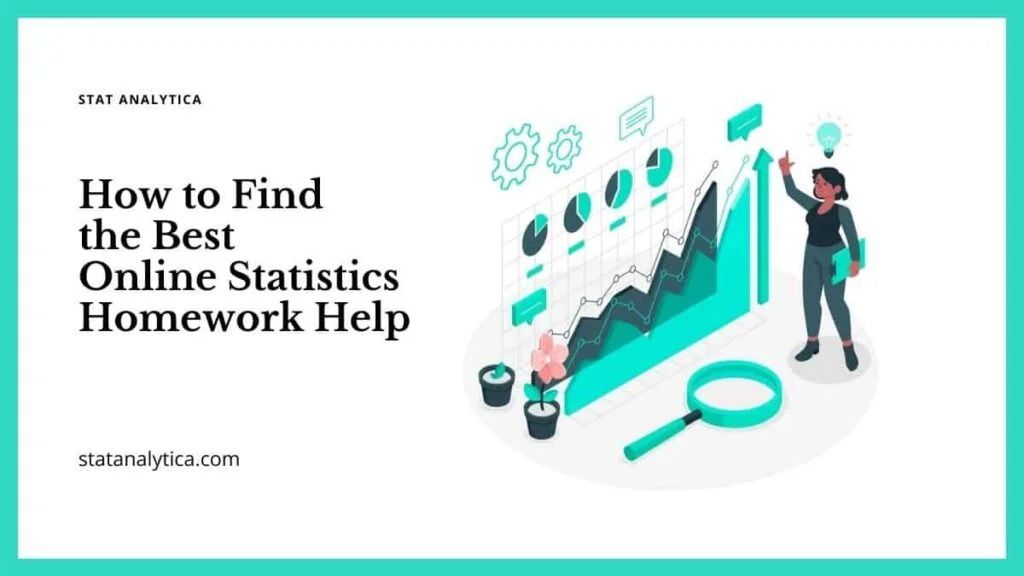
How to Find the Best Online Statistics Homework Help
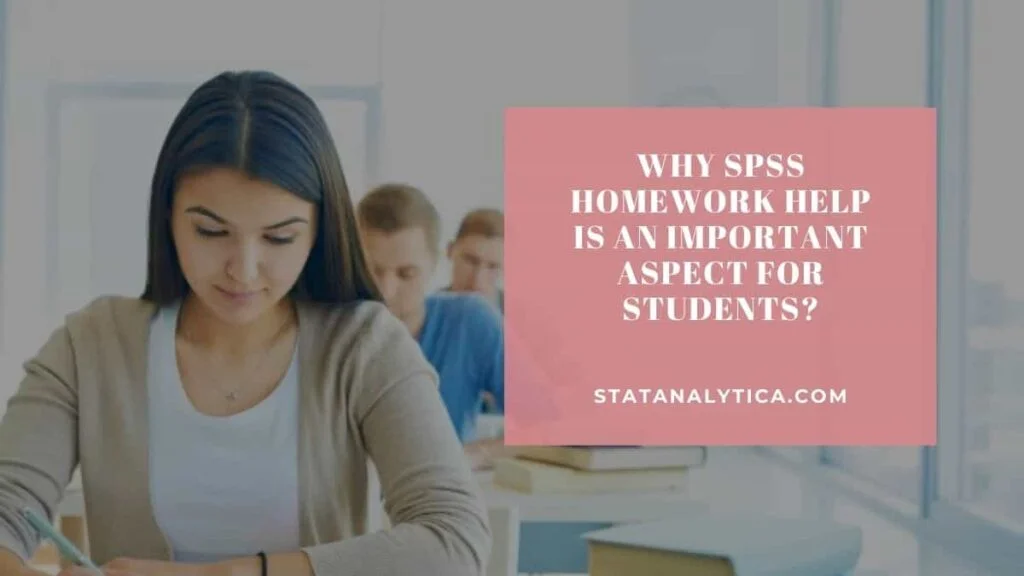
Why SPSS Homework Help Is An Important aspect for Students?
DigitalCommons@University of Nebraska - Lincoln
Home > Statistics > Dissertations, Theses, and Student Work
Statistics, Department of
Department of statistics: dissertations, theses, and student work.
Examining the Effect of Word Embeddings and Preprocessing Methods on Fake News Detection , Jessica Hauschild
Exploring Experimental Design and Multivariate Analysis Techniques for Evaluating Community Structure of Bacteria in Microbiome Data , Kelsey Karnik
Human Perception of Exponentially Increasing Data Displayed on a Log Scale Evaluated Through Experimental Graphics Tasks , Emily Robinson
Factors Influencing Student Outcomes in a Large, Online Simulation-Based Introductory Statistics Course , Ella M. Burnham
Comparing Machine Learning Techniques with State-of-the-Art Parametric Prediction Models for Predicting Soybean Traits , Susweta Ray
Using Stability to Select a Shrinkage Method , Dean Dustin
Statistical Methodology to Establish a Benchmark for Evaluating Antimicrobial Resistance Genes through Real Time PCR assay , Enakshy Dutta
Group Testing Identification: Objective Functions, Implementation, and Multiplex Assays , Brianna D. Hitt
Community Impact on the Home Advantage within NCAA Men's Basketball , Erin O'Donnell
Optimal Design for a Causal Structure , Zaher Kmail
Role of Misclassification Estimates in Estimating Disease Prevalence and a Non-Linear Approach to Study Synchrony Using Heart Rate Variability in Chickens , Dola Pathak
A Characterization of a Value Added Model and a New Multi-Stage Model For Estimating Teacher Effects Within Small School Systems , Julie M. Garai
Methods to Account for Breed Composition in a Bayesian GWAS Method which Utilizes Haplotype Clusters , Danielle F. Wilson-Wells
Beta-Binomial Kriging: A New Approach to Modeling Spatially Correlated Proportions , Aimee Schwab
Simulations of a New Response-Adaptive Biased Coin Design , Aleksandra Stein
MODELING THE DYNAMIC PROCESSES OF CHALLENGE AND RECOVERY (STRESS AND STRAIN) OVER TIME , Fan Yang
A New Approach to Modeling Multivariate Time Series on Multiple Temporal Scales , Tucker Zeleny
A Reduced Bias Method of Estimating Variance Components in Generalized Linear Mixed Models , Elizabeth A. Claassen
NEW STATISTICAL METHODS FOR ANALYSIS OF HISTORICAL DATA FROM WILDLIFE POPULATIONS , Trevor Hefley
Informative Retesting for Hierarchical Group Testing , Michael S. Black
A Test for Detecting Changes in Closed Networks Based on the Number of Communications Between Nodes , Christopher S. Wichman
GROUP TESTING REGRESSION MODELS , Boan Zhang
A Comparison of Spatial Prediction Techniques Using Both Hard and Soft Data , Megan L. Liedtke Tesar
STUDYING THE HANDLING OF HEAT STRESSED CATTLE USING THE ADDITIVE BI-LOGISTIC MODEL TO FIT BODY TEMPERATURE , Fan Yang
Estimating Teacher Effects Using Value-Added Models , Jennifer L. Green
SEQUENCE COMPARISON AND STOCHASTIC MODEL BASED ON MULTI-ORDER MARKOV MODELS , Xiang Fang
DETECTING DIFFERENTIALLY EXPRESSED GENES WHILE CONTROLLING THE FALSE DISCOVERY RATE FOR MICROARRAY DATA , SHUO JIAO
Spatial Clustering Using the Likelihood Function , April Kerby
FULLY EXPONENTIAL LAPLACE APPROXIMATION EM ALGORITHM FOR NONLINEAR MIXED EFFECTS MODELS , Meijian Zhou
Advanced Search
Search Help
- Notify me via email or RSS
- Administrator Resources
- How to Cite Items From This Repository
- Copyright Information
- Collections
- Disciplines
Author Corner
- Guide to Submitting
- Submit your paper or article
- Statistics Website
Home | About | FAQ | My Account | Accessibility Statement
Privacy Copyright
We use cookies on reading.ac.uk to improve your experience, monitor site performance and tailor content to you
Read our cookie policy to find out how to manage your cookie settings
This site may not work correctly on Internet Explorer. We recommend switching to a different browser for a better experience.
Mathematics MSc dissertations
The Department of Mathematics and Statistics was host until 2014 to the MSc course in the Mathematics of Scientific and Industrial Computation (previously known as Numerical Solution of Differential Equations) and the MSc course in Mathematical and Numerical Modelling of the Atmosphere and Oceans. A selection of dissertation titles are listed below, some of which are available online:
2013 2012 2011 2010 2009 2008 2007 2006 2005 2004 2003 2002 2001 2000 1999 1998 1997 1996 1995 1994 1993 1992 1991
2014: Mathematics of Scientific and Industrial Computation
Amanda Hynes - Slow and superfast diffusion of contaminant species through porous media
2014: Applicable and Numerical Mathematics
Emine Akkus - Estimating forecast error covariance matrices with ensembles
Rabindra Gurung - Numerical solution of an ODE system arising in photosynthesis
2013: Mathematics of Scientific and Industrial Computation
Zeinab Zargar - Modelling of Hot Water Flooding as an Enhanced Oil Recovery Method
Siti Mazulianawati Haji Majid - Numerical Approximation of Similarity in Nonlinear Diffusion Equations
2013: Mathematical and Numerical Modelling of the Atmosphere and Oceans
Yu Chau Lam - Drag and Momentum Fluxes Produced by Mountain Waves
Josie Dodd - A Moving Mesh Approach to Modelling the Grounding Line in Glaciology
2012: Mathematics of Scientific and Industrial Computation
Chris Louder - Mathematical Techniques of Image Processing
Jonathan Muir - Flux Modelling of Polynyas
Naomi Withey - Computer Simulations of Dipolar Fluids Using Ewald Summations
2012: Mathematical and Numerical Modelling of the Atmosphere and Oceans
Jean-Francois Vuillaume - Numerical prediction of flood plains using a Lagrangian approach
2011: Mathematics of Scientific and Industrial Computation
Tudor Ciochina - The Closest Point Method
Theodora Eleftheriou - Moving Mesh Methods Using Monitor Functions for the Porous Medium Equation
Melios Michael - Self-Consistent Field Calculations on a Variable Resolution Grid
2011: Mathematical and Numerical Modelling of the Atmosphere and Oceans
Peter Barnet - Rain Drop Growth by Collision and Coalescence
Matthew Edgington - Moving Mesh Methods for Semi-Linear Problems
Samuel Groth - Light Scattering by Penetrable Convex Polygons
Charlotte Kong - Comparison of Approximate Riemann Solvers
Amy Jackson - Estimation of Parameters in Traffic Flow Models Using Data Assimilation
Bruce Main - Solving Richards' Equation Using Fixed and Moving Mesh Schemes
Justin Prince - Fast Diffusion in Porous Media
Carl Svoboda - Reynolds Averaged Radiative Transfer Model
2010: Mathematics of Scientific and Industrial Computation
Tahnia Appasawmy - Wave Reflection and Trapping in a Two Dimensional Duct
Nicholas Bird - Univariate Aspects of Covariance Modelling within Operational Atmospheric Data Assimilation
Michael Conland - Numerical Approximation of a Quenching Problem
Katy Shearer - Mathematical Modelling of the regulation and uptake of dietary fats
Peter Westwood - A Moving Mesh Finite Element Approach for the Cahn-Hilliard Equation
Kam Wong - Accuracy of a Moving Mesh Numerical Method applied to the Self-similar Solution of Nonlinear PDEs
2010: Mathematical and Numerical Modelling of the Atmosphere and Oceans
James Barlow - Computation and Analysis of Baroclinic Rossby Wave Rays in the Atlantic and Pacific Oceans
Martin Conway - Heat Transfer in a Buried Pipe
Simon Driscoll - The Earth's Atmospheric Angular Momentum Budget and its Representation in Reanalysis Observation Datasets and Climate Models
George Fitton - A Comparative Study of Computational Methods in Cosmic Gas Dynamics Continued
Fay Luxford - Skewness of Atmospheric Flow Associated with a Wobbling Jetstream
Jesse Norris - A Semi-Analytic Approach to Baroclinic Instability on the African Easterly Jet
Robert J. Smith - Minimising Time-Stepping Errors in Numerical Models of the Atmosphere and Ocean
Amandeep Virdi - The Influence of the Agulhas Leakage on the Overturning Circulation from Momentum Balances
2009: Mathematics of Scientific and Industrial Computation
Charlotta Howarth - Integral Equation Formulations for Scattering Problems
David Fairbairn - Comparison of the Ensemble Transform Kalman Filter with the Ensemble Transform Kalman Smoother
Mark Payne - Mathematical Modelling of Platelet Signalling Pathways Mesh Generation and its application to Finite Element Methods
Mary Pham - Mesh Generation and its application to Finite Element Methods
Sarah Cole - Blow-up in a Chemotaxis Model Using a Moving Mesh Method
2009: Mathematical and Numerical Modelling of the Atmosphere and Oceans
Danila Volpi - Estimation of parameters in traffic flow models using data assimilation
Dale Partridge - Analysis and Computation of a Simple Glacier Model using Moving Grids
David MacLeod - Evaluation of precipitation over the Middle East and Mediterranean in high resolution climate models
Joanne Pocock - Ensemble Data Assimilation: How Many Members Do We Need?
Neeral Shah - Impact and implications of climate variability and change on glacier mass balance in Kenya
Tomos Roberts - Non-oscillatory interpolation for the Semi-Lagrangian scheme
Zak Kipling - Error growth in medium-range forecasting models
Zoe Gumm - Bragg Resonance by Ripple Beds
2008: Mathematics of Scientific and Industrial Computation
Muhammad Akram - Linear and Quadratic Finite Elements for a Moving Mesh Method
Andrew Ash - Examination of non-Time Harmonic Radio Waves Incident on Plasmas
Cassandra Moran - Harbour modelling and resonances
Elena Panti - Boundary Element Method for Heat Transfer in a Buried Pipe
Juri Parrinello - Modelling water uptake in rice using moving meshes
Ashley Twigger - Blow-up in the Nonlinear Schrodinger Equation Using an Adaptive Mesh Method
Chloe Ward - Numerical Evaluation of Oscillatory Integrals
Christopher Warner - Forward and Inverse Water-Wave Scattering by Topography
2008: Mathematical and Numerical Modelling of the Atmosphere and Oceans
Fawzi Al Busaidi - Fawzi Albusaidi
Christopher Bowden - A First Step Towards the Calculation of a Connectivity Matrix for the Great Barrier Reef
Evangelia-Maria Giannakopoulou - Flood Prediction and Uncertainty
Victoria Heighton - 'Every snowflake is different'
Thomas Jordan - Does Self-Organised Criticality Occur in the Tropical Convective System?
Gillian Morrison - Numerical Modelling of Tidal Bores using a Moving Mesh
Rachel Pritchard - Evaluation of Fractional Dispersion Models
2007: Numerical solution of differential equations
Tamsin Lee - New methods for approximating acoustic wave transmission through ducts (PDF 2.5MB)
Lee Morgan - Anomalous diffusion (PDF-1.5MB)
Keith Pham - Finite element modelling of multi-asset barrier options (PDF-3MB)
Alastair Radcliffe - Finite element modelling of the atmosphere using the shallow water equations (PDF-2.5MB)
Sanita Vetra - The computation of spectral representations for evolution PDE (PDF-3.2MB)
2007: Mathematical and numerical modelling of the atmosphere and oceans
Laura Baker - Properties of the ensemble Kalman filter (PDF-3.8MB)
Alison Brass - A moving mesh method for the discontinuous Galerkin finite element technique (PDF-916KB)
Daniel Lucas - Application of the phase/amplitude method to the study of trapped waves in the atmosphere and oceans (PDF-1.1MB)
Duduzile Nhlengethwa - Petrol or diesel (PDF-1MB)
Rhiannon Roberts - Modelling glacier flow (PDF-406KB)
David Skinner - A moving mesh finite element method for the shallow water equations (PDF-4.3MB)
Jovan Stojsavljevic - Investigation of waiting times in non-linear diffusion equations using a moving mesh method (PDF-538KB)
2006: Numerical solution of differential equations
Bonhi Bhattacharya - A moving finite element method for high order nonlinear diffusion problems
Jonathan Coleman - High frequency boundary element methods for scattering by complex polygons
Rachael England - The use of numerical methods in solving pricing problems for exotic financial derivatives with a stochastic volatility
Stefan King - Best fits with adjustable nodes and scale invariance
Edmund Ridley - Analysis of integral operators from scattering problems
Nicholas Robertson - A moving Lagrangian mesh model of a lava dome volcano and talus slope
2006: Mathematical and numerical modelling of the atmosphere and oceans
Iain Davison - Scale analysis of short term forecast errors
Richard Silveira - Electromagnetic scattering by simple ice crystal shapes
Nicola Stone - Development of a simplified adaptive finite element model of the Gulf Stream
Halina Watson - The behaviour of 4-D Var for a highly nonlinear system
2005: Numerical solution of differential equations
Jonathan Aitken - Data dependent mesh generation for peicewise linear interpolation
Stephen Arden - A collocation method for high frequency scattering by convex polygons
Shaun Benbow - Numerical methods for american options
Stewart Chidlow - Approximations to linear wave scattering by topography using an integral equation approach
Philip McLaughlin - Outdoor sound propagation and the boundary element method
Antonis Neochoritis - Numerical modelling of islands and capture zone size distributions in thin film growth
Kylie Osman - Numerical schemes for a non-linear diffusion problem
Shaun Potticary - Efficient evaluation of highly oscillatory integrals
Martyn Taylor - Investigation into how the reduction of length scales affects the flow of viscoelastic fluid in parallel plate geometries
Aanand Venkatramanan - American spread option pricing
2005: Mathematical and numerical modelling of the atmosphere and oceans
Richard Fruehmann - Ageostrophic wind storms in the central Caspian sea
Gemma Furness - Using optimal estimation theory for improved rainfall rates from polarization radar
Edward Hawkins - Vorticity extremes in numerical simulations of 2-D geostrophic turbulence
Robert Horton - Two dimensional turbulence in the atmosphere and oceans
David Livings - Aspects of the ensemble Kalman filter
David Sproson - Energetics and vertical structure of the thermohaline circulation
2004: Numerical solution of differential equations
Rakhib Ahmed - Numerical schemes applied to the Burgers and Buckley-Leverett equations
James Atkinson - Embedding methods for the numerical solution of convolution equations
Catherine Campbell-Grant - A comparative study of computational methods in cosmic gas dynamics
Paresh Prema - Numerical modelling of Island ripening
Mark Webber - The point source methods in inverse acoustic scattering
2004: Mathematical and numerical modelling of the atmosphere and oceans
Oliver Browne - Improving global glacier modelling by the inclusion of parameterised subgrid hypsometry within a three-dimensional, dynamical ice sheet model
Petros Dalakakis - Radar scattering by ice crystals
Eleanor Gosling - Flow through porous media: recovering permeability data from incomplete information by function fitting .
Sarah Grintzevitch - Heat waves: their climatic and biometeorological nature in two north american reigions
Helen Mansley - Dense water overflows and cascades
Polly Smith - Application of conservation laws with source terms to the shallow water equations and crowd dynamics
Peter Taylor - Application of parameter estimation to meteorology and food processing
2003: Numerical solution of differential equations
Kate Alexander - Investigation of a new macroscopic model of traffic flow
Luke Bennetts - An application of the re-iterated Galerkin approximation in 2-dimensions
Peter Spence - The Position of the free boundary formed between an expanding plasma and an electric field in differing geometries
Daniel Vollmer - Adaptive mesh refinement using subdivision of unstructured elements for conservation laws
2003: Mathematical and numerical modelling of the atmosphere and oceans
Clare Harris - The Valuation of weather derivatives using partial differential equations
Sarah Kew - Development of a 3D fractal cirrus model and its use in investigating the impact of cirrus inhomogeneity on radiation
Emma Quaile - Rotation dominated flow over a ridge
Jemma Shipton - Gravity waves in multilayer systems
2002: Numerical solution of differential equations
Winnie Chung - A Spectral Method for the Black Scholes Equations
Penny Marno - Crowded Macroscopic and Microscopic Models for Pedestrian Dynamics
Malachy McConnell - On the numerical solution of selected integrable non-linear wave equations
Stavri Mylona - An Application of Kepler's Problem to Formation Flying using the Störmer-Verlet Method
2002: Mathematical and numerical modelling of the atmosphere and oceans
Sarah Brodie - Numerical Modelling of Stratospheric Temperature Changes and their Possible Causes
Matt Sayer - Upper Ocean Variability in the Equatorial Pacific on Diurnal to Intra-seasonal Timescales
Laura Stanton - Linearising the Kepler problem for 4D-var Data Assimilation
2001: Numerical solution of differential equations
R.B. Brad - An Implementation of the Box Scheme for use on Transcritical Problems
D. Garwood - A Comparison of two approaches for the Approximating of 2-D Scattered Data, with Applications to Geological Modelling
R. Hawkes - Mesh Movement Algorithms for Non-linear Fisher-type Equations
P. Jelfs - Conjugate Gradients with Rational and Floating Point Arithmetic
M. Maisey - Vorticity Preserving Lax-Wendroff Type Schemes
C.A. Radcliffe - Positive Schemes for the Linear Advection Equation
2000: Numerical solution of differential equations
D. Brown - Two Data Assimilation Techniques for Linear Multi-input Systems.
S. Christodoulou - Finite Differences Applied to Stochastic Problems in Pricing Derivatives.
C. Freshwater - The Muskingum-Cunge Method for Flood Routing.
S.H. Man - Galerkin Methods for Coupled Integral Equations.
A. Laird - A New Method for Solving the 2-D Advection Equation.
T. McDowall - Finite Differences Applied to Joint Boundary Layer and Eigenvalue Problems.
M. Shahrill - Explicit Schemes for Finding Soliton Solutions of the Korteweg-de Vries Equation.
B. Weston - A Marker and Cell Solution of the Incompressible Navier-Stokes Equations for Free Surface Flow.
1999: Numerical solution of differential equations
M. Ariffin - Grid Equidistribution via Various Algorithmic Approaches.
S.J. Fletcher - Numerical Approximations to Bouyancy Advection in the Eddy Model.
N.Fulcher - The Finite Element Approximation of the Natural Frequencies of a Circular Drum.
V. Green - A Financial Model and Application of the Semi-Lagrangian Time-Stepping Scheme.
D.A. Parry - Construction of Symplectic Runge-Kutta Methods and their Potential for Molecular Dynamics Application.
S.C. Smith - The Evolution of Travelling Waves in a Simple Model for an Ionic Autocatalytic System
P. Swain - Numerical Investigations of Vorticity Preserving Lax-Wendroff Type Schemes.
M. Wakefield - Variational Methods for Upscaling.
1998: Numerical solution of differential equations
C.C. Anderson - A dual-porosity model for simulating the preferential movement of water in the unsaturated zone of a chalk aquifer.
K.W. Blake - Contour zoning.
M.R. Garvie - A comparison of cell-mapping techniques for basins of attraction.
W. Gaudin - HYDRA: a 3-d MPP Eulerian hydrocode.
D. Gnandi - Alternating direction implicit method applied to stochastic problems in derivative finance.
J. Hudson - Numerical techniques for conservation laws with source terms. .
H.S. Khela - The boundary integral method.
K. Singh - A comparison of numerical schemes for pricing bond options.
1997: Numerical solution of differential equations
R.V. Egan - Chaotic response of the Duffing equation. A numerical investigation into the dynamics of the non-linear vibration equation.
R.G. Higgs - Nonlinear diffusion in reservoir simulation.
P.B. Horrocks - Fokker-Planck model of stochastic acceleration: a study of finite difference schemes.
M.A. Wlasek - Variational data assimilation: a study.
1996: Numerical solution of differential equations
A. Barnes - Reaction-diffusion waves in an isothermal chemical system with a general order of autocatalysis.
S.J. Leary - Mesh movement and mesh subdivision.
S. McAllister - First and second order complex differential equations.
R.K. Sadhra - Investigating dynamical systems using the cell-to-cell mapping.
J.P. Wilson - A refined numerical model of sediment deposition on saltmarshes.
1995: Numerical solution of differential equations
M. Bishop - The modelling and analysis of the equations of motion of floating bodies on regular waves.
J. Olwoch - Isothermal autocatalytic reactions with an immobilized autocatalyst.
S. Stoke - Eulerian methods with a Lagrangian phase in gas dynamics.
R. Coad - 1-D and 2-D simulations of open channel flows using upwinding schemes.
1994: Numerical solution of differential equations
M. Ali - Application of control techniques to solving linear systems of equations .
M.H. Brookes - An investigation of a dual-porosity model for the simulation of unsaturated flow in a porous medium .
A.J. Crossley - Application of Roe's scheme to the shallow water equations on the sphere .
D.A. Kirkland - Huge singular values and the distance to instability. .
B.M. Neil - An investigation of the dynamics of several equidistribution schemes .
1993: Numerical solution of differential equations
P.A. Burton - Re-iterative methods for integral equations .
J.M. Hobbs - A moving finite element approach to semiconductor process modelling in 1-D. .
L.M. Whitfield - The application of optimal control theory to life cycle strategies .
S.J. Woolnough - A numerical model of sediment deposition on saltmarshes .
1992: Numerical solution of differential equations
I. MacDonald - The numerical solution of free surface/pressurized flow in pipes. .
A.D. Pollard - Preconditioned conjugate gradient methods for serial and parallel computers. .
C.J. Smith - Adaptive finite difference solutions for convection and convection-diffusion problems .
1991: Numerical solution of differential equations
K.J. Neylon - Block iterative methods for three-dimensional groundwater flow models .
Need Help with Developing a Great Topic?
- A great topic is certainly the foundation of a successful research project. If you seek help with, say, a dissertation topic or guidance with developing a relevant & researchable topic , we got you.
- Do you have ideas on the topic you want to research? Maybe you are totally unsure and not even aware of where to start. Our services are comprehensive, and we will guide you through them.
- Our support desk will guide you and we shall develop sample topics for you, each with some background information, and then you choose the best from the same.
Our service Advantage
01. - Develop a Relevant Research Topic.
You need to have a great research idea that addresses a relevant research gap. We are more than willing to support you, let us know.
02. - Defend & have your Topic Approved.
If possible, review or have your topic approved by your supervisor or senior research advisor so that you do not start and later stall midway.
03. - You can now Start your Research Process.
When your topic has finally been approved & is researchable, you can now start the research process : We can help you Step by Step.
Guidelines for Writing an Exceptional Research Project Topic!
Our Service Process
MSc Project Topics | Recent Research Suggestion Topic Ideas
The importance of choosing the right M.Sc project topics cannot be overstated. It is the bedrock upon which the entire research journey is built. By offering our expertise and resources, we aim to simplify this crucial step, ensuring students embark on a research journey that is meaningful, impactful, and aligned with their academic and career goals. In the vast world of academia, having a guiding hand can make all the difference, and we're here to be that unwavering support for every aspiring MSc student.
Most Recent Research Project Topics for MSc Students;
✔ "Developing a sustainable waste management strategy for a local community": Sustainable waste management is an important issue for many communities, and an MSc project that focuses on developing a sustainable waste management strategy for a local community could make a significant impact. This project would involve researching best practices for waste management and developing a customized strategy that takes into account the specific needs and resources of the community. The resulting strategy could help to reduce waste, improve recycling rates, and minimize the environmental impact on the community.
- Utilizing and applying enterprise information portals in the management of knowledge.
- Medical and genetic data warehousing.
- Development of wireless multimedia network.
- Analysis of the robustness of capture-recapture models.
- Using bioinformatics tools to identify key biological processes for cancer progression.
- Effectiveness of PPE in agricultural applications.
- Impact of raw poultry salmonella on public health.
Master project topics with a narrowed and defined focus can be incredibly impactful and valuable. By choosing a specific research question or problem to focus on, students can make a significant contribution to their field of study and produce research that is both rigorous and relevant. Whether you are interested in healthcare, marketing, wellness, or sustainability, there are many potential MSc project topics with a narrowed focus that could be perfect for your interests and skills. You can liaise with our experts if you need more Master of Science research topic ideas, based on your needs/interests and area of specialization.
Creative & Innovative MSc Research Topics- Professional Help
Key factors that indicate good master of science degree research topics;.
- Relevance of the research topic : The relevance of a research topic is of paramount importance. A good research topic should be related to the field of study and should address a current issue, trend, or problem. The topic should have a clear focus, be feasible, and should have a practical application. An appropriate topic can be beneficial for both the student and the community, as it can help to solve real-world problems.
- Originality and authenticity : Originality is a key aspect of a good project topic. A topic that is unique, innovative, and contributes to existing knowledge is highly recommended. The topic should not be repetitive or redundant but should add a new dimension to the field of study. An original topic can enhance the research value and attract the attention of the academic community.
- Feasibility and viability of the topic : Good master of science degree research topics should be feasible, meaning that they should be achievable within the given time frame and resources. The topic should have a clear scope, and methodology, and should be realistic in terms of data collection, analysis, and interpretation. A feasible topic can provide a sense of direction and focus to the research work and can help to avoid unnecessary complications and delays.
- How interesting the topic is : The topic of an MSc research paper should be interesting and engaging. It should be something that the student is passionate about and has a genuine interest in. A topic that the student finds boring or uninteresting can lead to a lack of motivation, enthusiasm, and creativity. On the other hand, an interesting topic can lead to a more profound understanding of the subject, better engagement, and a higher level of achievement.
- Applicability in future career : A good MSc topic should be relevant to the student's future career aspirations. The topic should be aligned with the student's career goals, skills, and interests. It should provide an opportunity to acquire relevant knowledge, skills, and experiences that can be valuable in the future. A relevant research topic can help to build a strong foundation for the student's career and can be beneficial in terms of employability and professional development.
- Collaboration opportunities : Great MSc research project topics should provide an opportunity for collaboration with other students, researchers, or professionals. Collaboration can enhance the quality of research, provide diverse perspectives, and create a sense of community. A topic that allows collaboration can lead to new networks, opportunities, and experiences, and can contribute to personal and professional growth.
Ideally, choosing good MSc research topics for a paper is an essential step in an academic journey. The topic should be relevant, original, feasible, interesting, aligned with future career goals, and provide an opportunity for collaboration. By keeping these key factors in mind, students can make informed decisions and create high-quality thesis work that can make a positive impact on their academic and professional lives.
Different Subject Areas
- B.Ed . | B.A .
- B.Com | BSc
- BSN | DBa
- DNP | Doctoral
- Ed.D | M.Ed | RN
- Masters | MBA
- MBus | MCom
- MEntr | Msc
- MSN | PhD
- Post graduate
- Undergraduate
Client Testimonials
Thanks a lot for the research topic writing Services!
Read More...
Quality & Reliable Services
- Recent Topic Ideas
- Dissertation Project Ideas
- Case Study Topics
- Topic Writing Tips
- Secrets to a Great Topic
- Literature Review Topics
- Urgent Research Ideas Help
- Topic Brief Assistance
- Relevant Research Ideas
- Thesis Title Defence
- Topic Reviewing Aid
- Significance in a Title
- Base Paper Help
Topic Ideas by Paper Type
- Dissertation Topics
- Thesis Topics
- Proposal Topics
- Research Paper Topics
- Capstone Project Topics
Remarkable Help
There are some services that we recommend for related services, they are reliable but subject to your review.
- Literature Review Help
- Research Writing Service
- Custom Writing Help.ca
- Best Editing & Writing
- Personal Documents Help
MSc Thesis Topics – Determining Research Variables
- Identify the suitable research question: The first step in determining the topic variables for your MSc thesis is to identify the research question. What is the problem that you want to investigate? What is the gap in the literature that you want to fill? The research question should be specific, focused, and achievable within the timeframe of the thesis.
- Conduct a literature review: After identifying the research question, the next step is to conduct a literature review. This will help you to identify the existing research on the topic and to determine the variables that have been used in previous studies. A literature review will also help you to identify the gaps in the literature and to determine the research questions that have not been addressed in previous studies.
- Determine the dependent variable: The dependent variable is the variable that you will be measuring in your study. It is the outcome variable, the variable that you are trying to predict or explain. Determining the dependent variable is an important step in the process of specifying the topic variables for your MSc thesis. The dependent variable should be clearly defined and measurable.
- Determine the independent variable(s): The independent variable(s) are the variable(s) that you will be manipulating or measuring in your study. They are the variable(s) that you think may be causing the changes in the dependent variable. The independent variable(s) should be clearly defined and measurable. They should also be relevant to the research question and the dependent variable.
- Determine the moderating variable(s): Moderating variables are variables that affect the relationship between the independent and dependent variables. They are essential to consider in the process of determining the variables for MSc students' thesis topics because they can influence the results of your study. Examples of moderating variables include age, gender, and socioeconomic status.
- Determine the mediating variable(s): Mediating variables are variables that explain the relationship between the independent and dependent variables. They are important to consider in the process of defining the topic variables for your MSc thesis because they can help to provide a deeper understanding of the relationship between the independent and dependent variables. Examples of mediating variables include motivation, self-efficacy, and attitude.
- Consider the ethical implications: Finally, it is important to consider the ethical implications of your MSc thesis when deciding on the topic variables. Will the thesis involve human participants? If so, what are the risks to the participants? Will the participants be informed of their rights? Will their confidentiality be protected? These are all crucial ethical considerations that should be taken into account when determining the topic variables for your MSc thesis.
Identifying the Right Research Ideas for Your MSC Thesis
Finding science-related issues that can be researched in a Master of Science thesis is not an easy process. However, the process can be manageable if you understand what you are required to do so as to find relevant ideas for your forthcoming thesis. Since all research papers are usually written under the supervision of a professor or a lecturer who has vast research experience, you should not assume that you can just use any topic for your thesis. In consultation with your supervisor/lecturer, by reading science publications, accessing science libraries, and outsourcing professional research help, you can be guided into creating a nice case study. If you feel that you dearly need help with topic ideas for an MSc thesis , liaise with Research Topic Help experts. Our researchers have many years of experience in the field of research and they help our clients with unique and new research topic ideas that are applicable to all the Master of Science areas of specialization, such as for MSc in Statistics, MSc in Data Science, MSc in Environmental Engineering, MSc in Nursing , etc.
Developing Good Thesis Topic Ideas for Master of Science Degree
Crafting hot & most current msc dissertation topics.
Choosing a topic for your Master of Science (MSc) dissertation is a big deal. It's like picking the destination for a long journey, and if you pick the right one, the trip can be both exciting and rewarding. This is where we come in, like a trusted travel guide, helping students like you find the most exciting and up-to-date topics for your research. Think of a dissertation as a big story about something you're super curious about. But instead of just any story, you want yours to be fresh and in tune with what's happening right now in the world of science. Now, why is picking the right topic so crucial? Imagine telling a story that no one wants to hear. That’s what it's like when you pick an outdated or overdone topic. But when you choose something hot and current, everyone's ears perk up. It's like suddenly being the talk of the town! And that's the magic we offer. We have a dedicated team who are like detectives, always on the lookout for the latest happenings in science. They ensure you're not talking about yesterday's news, but tomorrow's headlines. Choosing a good topic is also like laying a strong foundation for a house. When the foundation is robust, building the rest of the house becomes easier. Similarly, with a relevant and engaging topic, the rest of your research, like gathering data, writing, and presenting, flows more smoothly. Our team understands this and offers personalized suggestions. It's not a one-size-fits-all approach. We get to know you, understand your interests, and match them with what's trending in the scientific world. This ensures your topic feels right for you and is also something others will find exciting. Another perk of choosing a fresh topic? It makes your work stand out after it's done. When you submit your dissertation, be it to journals, professors, or potential employers, a modern topic makes them take notice. It's like wearing a bright color in a crowd – you're bound to stand out. To wrap things up, think of your MSc dissertation as a golden opportunity to show your passion, intelligence, and hard work. But the first step, choosing your topic, can often be tricky. It's like standing at a crossroads, unsure of which path to take. But with our professional guidance, you won’t be lost. We'll handpick current and exciting topics that align with your interests, ensuring your research journey is not only rewarding but also enjoyable. With our help, your dissertation won’t just be another paper; it'll be a shining beacon of fresh ideas and insights. So, if you’re aiming for academic success and recognition, let us guide you in choosing the perfect starting point for your dissertation journey.
Helpful Ideas for Writing a Master of Science Dissertation Topic
Why obtain help with your msc dissertation topics.
- With expert help create Master of Science dissertation topic ideas that you are passionate about.
- Experts write the MSc dissertation paper topic ideas from scratch.
- Professionals ensure to offer each client customized assistance.
- Get involved in giving input during the service delivery process.
We first choose broad ideas that you are interested in. We then proceed to narrow down and develop more specific ideas. In order to ensure that your research paper ultimately looks good, we use the materials from books and the internet. What makes us different from other sites is that we strictly stick to your instructions. Our main priority is to ensure that our clients are getting the best ideas for their Master's dissertation research . Thus, you do not have to worry because you are in the right place. You can trust us with your work. We also ensure that the work is finished within the stipulated time.
Thesis tOPIC & pROJECT wRITING aSSISTANCE
Essay Topics | Sociolinguistics Research Topics | Creative Writing Topics | Descriptive Essay Topics | English Essay Topics | Informal & Formal Letter Topics | Criminal Law Research Topics | Economics Research Topics For Students | Psychology Research Topics | Management Project Topics | Topics For Persuasive Essays | Environmental Essay Topics | Shodhganga Project Topics
RESEARCH TOPIC HELP.
+1(813)489-6985 | Chat with Us
Reliable & Legit
100% original help.
We do not Plagiarize
We help Edit Plagiarism
Terms & Conditions
Copyright © 2024 Research Topic Help. All Rights Reserved
Let us know how we can help...
- People/Staff
Mathematical Statistics
An MSc (Dissertation) in Mathematical Statistics offers students who have excelled in their undergraduate and honours studies an opportunity for advanced study in Mathematical Statistics. The course prepares students who are keen to learn further statistical techniques to deal with data and undertak
The degree also offers students an opportunity to go on to do a PhD in mathematical statistics on completion.
Past students graduating from the MSc in Mathematical Statistics Programme have been highly successful in professional practice and a number have progressed to PhD studies in a number of statistics topics like Biostatistics, Reliability, Survival modelling, Statistical modelling, etc.
Why study Mathematical Statistics?
A n MSc Mathematical Statistics should:
- Prepare you for doing a PhD in Mathematical Statistics, Applied statistics, Statistical modelling, etc.
- Help you build expertise in analytical approaches and the classical and underpinning theory of mathematical statistics
- Offer options to focus on applied mathematical statistics if that is your interest and intended career path
- Provide experience in conducting and communicating statistical investigations and the use of statistical software
- Prepare you in conducting independent research
Why is this a good programme to do?
This programme teaches you how to handle data, model data, discover patterns in data and make good decisions based on available data. It also gives you the theoretical and applied underpinnings of modern and classical statistical methods
Why study Mathematical Statistics at Wits?
Wits is one of the top universities in South Africa. It has produced some of the best world-renowned academics. Some of the former lecturers in the school of Statistics and Actuarial science are:
- John Edmund Kerrich , first head of the Statistics department. Famous for experiments in probability performed while interned (in Denmark) during the Second World War. He was one of the 12 founders of South African Statistical Association.
- Herbert Sichel developed the Sichel-t estimator for the lognormal distribution 's t-statistic. He also made great leaps in the area of the generalized inverse Gaussian distribution which became known as the Sichel distribution.
- Danie Gerhardus Krige was a South African statistician and mining engineer who pioneered the field of geostatistics. The technique of kriging is named after him. Krige's empirical work to evaluate mineral resources was formalised in the 1960s by French engineer Georges Matheron .
- Gordon V. Kass, developed the Chi-square automatic interaction detection (CHAID ) a a decision tree technique, based on adjusted significance testing ( Bonferroni testing ).
- Frank Beichelt a professor in the School whose research focuses on probability theory and mathematical statistics. He is the author books on stochastic modelling in reliability, maintenance, and safety analysis etc..
- Paul Fatti - Emeritus Professor - University of the Witwatersrand, a former head of school and respected statistician.
What does Wits have to offer?
Wits offers a variety of statistics courses which gives the student a good foundation to excel in a wide range of areas. The statistics staff are versatile, passionate and progressive in their teaching, supervision and research. The environment and culture is supportive and empowering, giving students the confidence to push their boundaries.
Career Opportunities
- Statisticians
- Data Scientists
- Ecologists (environmental monitoring, water quality control, species management)
- Image processing (computer vision, face detection, remote sensing)
- Biostatistician (designing and analysing clinical trials and epidemiological studies)
- Financial analysts (designing investment strategies and managing risks in finance)
- Market researchers (conducting surveys and experiments etc.)
- Econometricians (investigating the economy at all levels from businesses)
- Epidemiologist (examine patterns in health and sickness)
- Operations research and optimization
- Bio-informatician (study DNA data)
- Programmer (statistical computing is a highly sought-after skill)
- Psychometrician (educational measurement or psychological measurement)
- Statistical physicist (use of statistical mechanics to study thermodynamics)
The MSc (Dissertation) requires a student to do research (Dissertation) totalling 180 credits.
The course duration is a minimum of one year for full-time students and two years for part-time students.
Students are required to register and submit a research proposal within three months. All MSc (dissertation) students are expected to start working on their research after registration, and are charged supervision fee in both years of registration for part-time students and full-time students.Once, registered a student is expected to consult lecturers in the school to
Entry Requirements
• A Bachelor of Science Honours in a related field with a minimum weighted average of 65%. • A supervisor from the School must be identified and approached prior to application.
The school considers all applications to the programme, basing on availability of supervisors and minimum academic requirements. These include an Honours degree in Mathematical Statistics, with the Research component contributing at least 25% of the degree (assessed at University of the Witwatersrand equivalence).
The mark requirements are the same as for the MSc (CWRR), but with the additional requirement of a mark of at least 70% for the Statistics Research component of the Honours degree.
In addition to the above, MSc applicants are required to include a 4-5 pages proposal of their proposed research.
University Application Process
- Applications are handled centrally by the Student Enrolment Centre (SEnC) . Once your application is complete in terms of requested documentation, your application will be referred to the relevant School for assessment. Click here to see an overview of the Wits applications process.
- Please apply online . Upload your supporting documents at the time of application, or via the Self Service Portal .
- Applicants can monitor the progress of their applications via the Self Service Portal .
- Selections for programmes that have a limited intake but attract a large number of applications may only finalise the application at the end of the application cycle.
Please note that the Entry Requirements are a guide. Meeting these requirements does not guarantee a place. Final selection is made subject to the availability of places, academic results and other entry requirements where applicable.
International students , please check this section .
For more information, contact the Student Call Centre +27 (0)11 717 1888, or log a query at www.wits.ac.za/askwits .
University Fees and Funding
Click here to see the current average tuition fees. The Fees site also provides information about the payment of fees and closing dates for fees payments. Once you have applied you will be able to access the fees estimator on the student self-service portal.
For information about postgraduate funding opportunities, including the postgraduate merit award, click here . Please also check your School website for bursary opportunities. NRF bursaries: The National Research Foundation (NRF) offers a wide range of opportunities in terms of bursaries and fellowships to students pursuing postgraduate studies. External bursaries portal: The Bursaries South Africa website provides a comprehensive list of bursaries in South Africa.
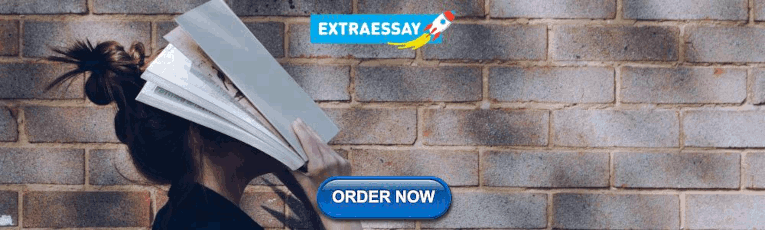
IMAGES
VIDEO
COMMENTS
Digital Commons @ USF > College of Arts and Sciences > Mathematics and Statistics > Theses and Dissertations. Mathematics and Statistics Theses and Dissertations . Follow. Jump to: Theses/Dissertations from 2023 PDF. Classification of Finite Topological Quandles and Shelves via Posets, Hitakshi Lahrani. PDF.
Master's Thesis. As an integral component of the Master of Science in Statistical Science program, you can submit and defend a Master's Thesis. Your Master's Committee administers this oral examination. If you choose to defend a thesis, it is advisable to commence your research early, ideally during your second semester or the summer following ...
Statistics thesis topics. Below are sample topics available for prospective postgraduate research students. These sample topics do not contain every possible project; they are aim
and figures. In Section 4, some notes about the rules of conduct when writing a master's thesis are provided. 2 The Structure of a Master's Thesis A master's thesis is an independent scientific work and is meant to prepare students for future professional or academic work. Largely, the thesis is expected to be similar to papers published in
2015. 2014. 2013. 2012. 2011. 2010. 2009. 2008. This list of recent dissertation topics shows the range of research areas that our students are working on.
In addition, we also recommend that you take the module "STA472 Good Satistical Practice", which teaches very useful technical and communication skills for the Master's thesis. List of topics. The list below give an idea of prototypic MSc thesis. Depending on the background and the degree they may be further tailored.
Below is a list of the theses produced by graduate students in the Department of Statistics and Actuarial Science. ... Student Degree Thesis Supervisor; 2023-3: Payman Nickchi: Ph.D: Linkage fine-mapping on sequences from case-control studies and Goodness-of-fit tests based on empirical distribution function for general likelihood model : 2023 ...
Seminars: In addition to required courses, students register in a research seminar in either actuarial science, biostatistics, mathematics, or statistics (depending on student's specialization). Statistics Courses: Topics may include probability, estimation, hypothesis testing and more. Thesis: Students will be required to submit and defend an ...
Supervisors and topics for master's theses in statistics. As part of his or her master's study, a student should write a thesis. There are two options for the thesis: a long thesis corresponding to one year full time work, and a short thesis corresponding to one semester's full time work. The work on a long thesis typically starts in the second ...
Thesis topics for the master thesis Data Science and Business Analytics Topic 1: Logistic regression for modern data structures Promotor Gerda Claeskens Description Logistic regression is widely used for binary classification. In the classical setting with a fixed number of predictive variables p and a large sample size n, the likelihood ratio test
In the MSc by Research in Statistics, you will investigate a particular project in depth and write a thesis that makes a contribution to the field. It may be possible to change from an MSc by Research and pursue a DPhil in Statistics. You will acquire a wide range of research and transferable skills, as well as in-depth knowledge, understanding ...
500+ Statistics Research Topics. March 25, 2024. by Muhammad Hassan. Statistics is a branch of mathematics that deals with the collection, analysis, interpretation, presentation, and organization of data. It is a fundamental tool used in various fields such as business, social sciences, engineering, healthcare, and many more.
Theses and dissertations. A selection of PhD theses and MSc dissertations are available for you to read: mathematics PhD theses. statistics PhD theses. mathematics MSc dissertations.
If we talk about the interesting research topics in statistics, it can vary from student to student. But here are the key topics that are quite interesting for almost every student:-. Literacy rate in a city. Abortion and pregnancy rate in the USA. Eating disorders in the citizens.
AUTHOR: In each respective box, enter your names (and/or initials) as they appear on the title page of your dissertation or thesis. You are the sole author; your advisor is not considered a co-author. Institution is University of Nebraska-Lincoln (not "at Lincoln" or ", Lincoln"). Do not leave this field blank.
A selection of dissertation titles are listed below, some of which are available online: 2013 2012 2011 2010 2009 2008 2007 2006 2005 2004 2003 2002 2001 2000 1999 1998 1997 1996 1995 1994 1993 1992 1991. 2014: Mathematics of Scientific and Industrial Computation. Amanda Hynes - Slow and superfast diffusion of contaminant species through porous ...
Extreme value analysis is the domain for the project on "Solar flares - Pareto versus lognormal distribution", jointly with the Royal Observatory, described in a separate document. Extreme value analysis. Rather than in mean effects, one is often interested in high quantiles, univariate or multivariate, with or without covariates. Extreme value analysis provides models and inference ...
MSc Thesis Topics - Determining Research Variables. Choosing the right thesis topic is crucial for MSc students as it sets the tone for their academic journey. The topic should not only be interesting but also relevant to their field of study. However, determining the variables that will form the basis of the thesis can be a challenging task.
The degree also offers students an opportunity to go on to do a PhD in mathematical statistics on completion. Past students graduating from the MSc in Mathematical Statistics Programme have been highly successful in professional practice and a number have progressed to PhD studies in a number of statistics topics like Biostatistics, Reliability, Survival modelling, Statistical modelling, etc.
Msc Statistics Thesis Topics - Free download as PDF File (.pdf), Text File (.txt) or read online for free. Scribd is the world's largest social reading and publishing site.
Msc Thesis Topics in Statistics - Free download as PDF File (.pdf), Text File (.txt) or read online for free.
Thesis Topics Ideas For Msc Research Project Topics. A Master of Science degree or diploma is an area of study that has an extensive range of career or professional opportunities, considering that students specialize in different science subjects, and science subjects are very many. Undoubtedly, we can say that research topic ideas for an MSc dissertation are sought by an extremely enormous ...
Thesis Type . 2551 M.Sc 457 M.V.Sc. 322 M.Tech. 57 M.Tech 6 M.B.A. 4 Ph.D 2 M.V.Sc . Search theseType . Submit. Type . 3802 ... Statistics and Management 54 Agricultural Extension 49 Forage Production 48 Agronomy 34 Dairy Economics 32 Food and Nutrition 30 Extension Education 24 Agricultural Extension Education 23 Agricultural Engineering 20 ...