Have a language expert improve your writing
Run a free plagiarism check in 10 minutes, generate accurate citations for free.
- Knowledge Base
Methodology
- Data Collection | Definition, Methods & Examples
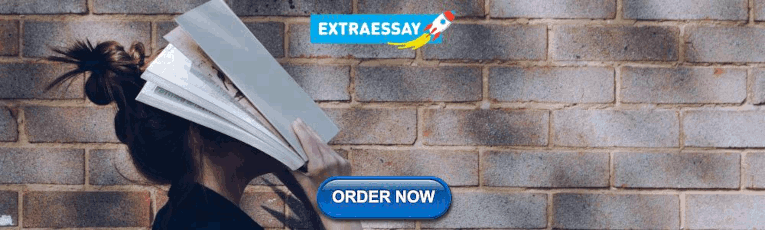
Data Collection | Definition, Methods & Examples
Published on June 5, 2020 by Pritha Bhandari . Revised on June 21, 2023.
Data collection is a systematic process of gathering observations or measurements. Whether you are performing research for business, governmental or academic purposes, data collection allows you to gain first-hand knowledge and original insights into your research problem .
While methods and aims may differ between fields, the overall process of data collection remains largely the same. Before you begin collecting data, you need to consider:
- The aim of the research
- The type of data that you will collect
- The methods and procedures you will use to collect, store, and process the data
To collect high-quality data that is relevant to your purposes, follow these four steps.
Table of contents
Step 1: define the aim of your research, step 2: choose your data collection method, step 3: plan your data collection procedures, step 4: collect the data, other interesting articles, frequently asked questions about data collection.
Before you start the process of data collection, you need to identify exactly what you want to achieve. You can start by writing a problem statement : what is the practical or scientific issue that you want to address and why does it matter?
Next, formulate one or more research questions that precisely define what you want to find out. Depending on your research questions, you might need to collect quantitative or qualitative data :
- Quantitative data is expressed in numbers and graphs and is analyzed through statistical methods .
- Qualitative data is expressed in words and analyzed through interpretations and categorizations.
If your aim is to test a hypothesis , measure something precisely, or gain large-scale statistical insights, collect quantitative data. If your aim is to explore ideas, understand experiences, or gain detailed insights into a specific context, collect qualitative data. If you have several aims, you can use a mixed methods approach that collects both types of data.
- Your first aim is to assess whether there are significant differences in perceptions of managers across different departments and office locations.
- Your second aim is to gather meaningful feedback from employees to explore new ideas for how managers can improve.
Prevent plagiarism. Run a free check.
Based on the data you want to collect, decide which method is best suited for your research.
- Experimental research is primarily a quantitative method.
- Interviews , focus groups , and ethnographies are qualitative methods.
- Surveys , observations, archival research and secondary data collection can be quantitative or qualitative methods.
Carefully consider what method you will use to gather data that helps you directly answer your research questions.
When you know which method(s) you are using, you need to plan exactly how you will implement them. What procedures will you follow to make accurate observations or measurements of the variables you are interested in?
For instance, if you’re conducting surveys or interviews, decide what form the questions will take; if you’re conducting an experiment, make decisions about your experimental design (e.g., determine inclusion and exclusion criteria ).
Operationalization
Sometimes your variables can be measured directly: for example, you can collect data on the average age of employees simply by asking for dates of birth. However, often you’ll be interested in collecting data on more abstract concepts or variables that can’t be directly observed.
Operationalization means turning abstract conceptual ideas into measurable observations. When planning how you will collect data, you need to translate the conceptual definition of what you want to study into the operational definition of what you will actually measure.
- You ask managers to rate their own leadership skills on 5-point scales assessing the ability to delegate, decisiveness and dependability.
- You ask their direct employees to provide anonymous feedback on the managers regarding the same topics.
You may need to develop a sampling plan to obtain data systematically. This involves defining a population , the group you want to draw conclusions about, and a sample, the group you will actually collect data from.
Your sampling method will determine how you recruit participants or obtain measurements for your study. To decide on a sampling method you will need to consider factors like the required sample size, accessibility of the sample, and timeframe of the data collection.
Standardizing procedures
If multiple researchers are involved, write a detailed manual to standardize data collection procedures in your study.
This means laying out specific step-by-step instructions so that everyone in your research team collects data in a consistent way – for example, by conducting experiments under the same conditions and using objective criteria to record and categorize observations. This helps you avoid common research biases like omitted variable bias or information bias .
This helps ensure the reliability of your data, and you can also use it to replicate the study in the future.
Creating a data management plan
Before beginning data collection, you should also decide how you will organize and store your data.
- If you are collecting data from people, you will likely need to anonymize and safeguard the data to prevent leaks of sensitive information (e.g. names or identity numbers).
- If you are collecting data via interviews or pencil-and-paper formats, you will need to perform transcriptions or data entry in systematic ways to minimize distortion.
- You can prevent loss of data by having an organization system that is routinely backed up.
Finally, you can implement your chosen methods to measure or observe the variables you are interested in.
The closed-ended questions ask participants to rate their manager’s leadership skills on scales from 1–5. The data produced is numerical and can be statistically analyzed for averages and patterns.
To ensure that high quality data is recorded in a systematic way, here are some best practices:
- Record all relevant information as and when you obtain data. For example, note down whether or how lab equipment is recalibrated during an experimental study.
- Double-check manual data entry for errors.
- If you collect quantitative data, you can assess the reliability and validity to get an indication of your data quality.
Here's why students love Scribbr's proofreading services
Discover proofreading & editing
If you want to know more about statistics , methodology , or research bias , make sure to check out some of our other articles with explanations and examples.
- Student’s t -distribution
- Normal distribution
- Null and Alternative Hypotheses
- Chi square tests
- Confidence interval
- Cluster sampling
- Stratified sampling
- Data cleansing
- Reproducibility vs Replicability
- Peer review
- Likert scale
Research bias
- Implicit bias
- Framing effect
- Cognitive bias
- Placebo effect
- Hawthorne effect
- Hindsight bias
- Affect heuristic
Data collection is the systematic process by which observations or measurements are gathered in research. It is used in many different contexts by academics, governments, businesses, and other organizations.
When conducting research, collecting original data has significant advantages:
- You can tailor data collection to your specific research aims (e.g. understanding the needs of your consumers or user testing your website)
- You can control and standardize the process for high reliability and validity (e.g. choosing appropriate measurements and sampling methods )
However, there are also some drawbacks: data collection can be time-consuming, labor-intensive and expensive. In some cases, it’s more efficient to use secondary data that has already been collected by someone else, but the data might be less reliable.
Quantitative research deals with numbers and statistics, while qualitative research deals with words and meanings.
Quantitative methods allow you to systematically measure variables and test hypotheses . Qualitative methods allow you to explore concepts and experiences in more detail.
Reliability and validity are both about how well a method measures something:
- Reliability refers to the consistency of a measure (whether the results can be reproduced under the same conditions).
- Validity refers to the accuracy of a measure (whether the results really do represent what they are supposed to measure).
If you are doing experimental research, you also have to consider the internal and external validity of your experiment.
Operationalization means turning abstract conceptual ideas into measurable observations.
For example, the concept of social anxiety isn’t directly observable, but it can be operationally defined in terms of self-rating scores, behavioral avoidance of crowded places, or physical anxiety symptoms in social situations.
Before collecting data , it’s important to consider how you will operationalize the variables that you want to measure.
In mixed methods research , you use both qualitative and quantitative data collection and analysis methods to answer your research question .
Cite this Scribbr article
If you want to cite this source, you can copy and paste the citation or click the “Cite this Scribbr article” button to automatically add the citation to our free Citation Generator.
Bhandari, P. (2023, June 21). Data Collection | Definition, Methods & Examples. Scribbr. Retrieved April 12, 2024, from https://www.scribbr.com/methodology/data-collection/
Is this article helpful?
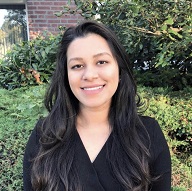
Pritha Bhandari
Other students also liked, qualitative vs. quantitative research | differences, examples & methods, sampling methods | types, techniques & examples, unlimited academic ai-proofreading.
✔ Document error-free in 5minutes ✔ Unlimited document corrections ✔ Specialized in correcting academic texts
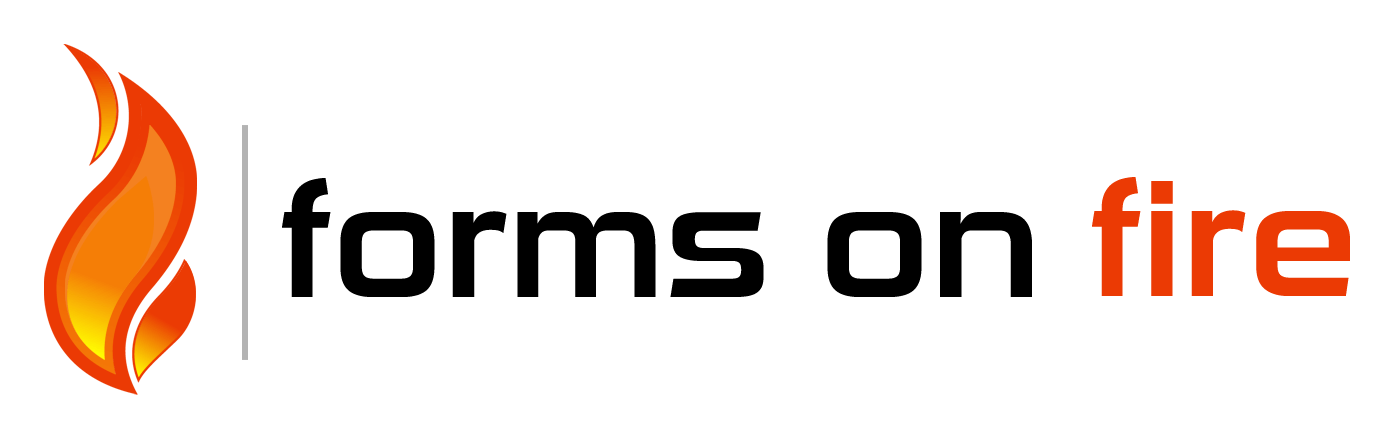
How to Write a Data Collection Plan (Templates and Examples Included)
In a world where data drives decisions, how do you make sure you're gathering the right information? With a clear data collection plan in place, you ensure that the collected data leads to actionable insights.
Effective data collection is key to smart decision-making, grounding strategies in solid evidence rather than guesses. A well-designed data collection plan guarantees that you're collecting not just any data, but the right data, crucial for spotting trends, refining processes, and deeply understanding customer needs in any sector.
By the end of this article, you'll understand the importance of planning your data collection and how to do it effectively.
What is a data collection plan?
A data collection plan is a roadmap for identifying what data you need, the ways in which you'll collect it, and how you'll analyze it. The core purpose is to ensure that your data collection is targeted, efficient, and reliable, providing meaningful insights for your project or study.
Data collection plans should be developed at the start of a project or study, before any data is collected. Typically, this responsibility falls to project leaders, researchers, data analysts, or a designated team member with expertise in data management.
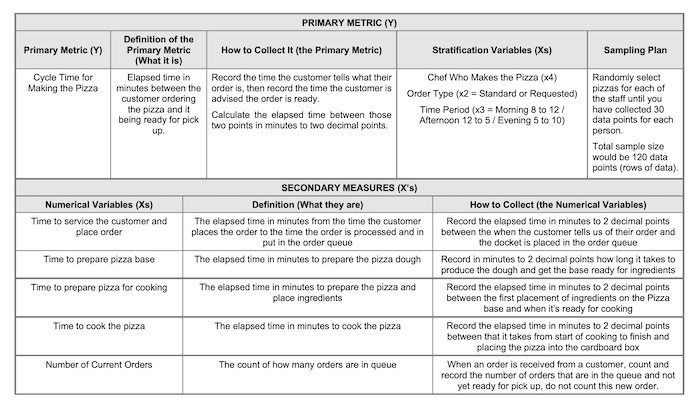
What does a typical data collection plan document cover
From setting clear objectives to establishing robust communication channels, each section of the plan is a stepping stone towards having a thorough data collection strategy:
- Objectives: Start with a specific goal for your data collection. Clearly state why this data is crucial and how it will impact your project or decision-making. This step ensures that every part of your plan aligns with your end goal.
- Data typology: Decide whether you need quantitative (numerical) or qualitative (descriptive) data. Clarify the importance of each data type in the context of your objectives. This clarity helps in selecting the right tools and methods for data collection.
- Collection methodology: Select appropriate methods like surveys, interviews, or analysis of existing data. Prioritize data quality; for surveys, this means clear, unbiased questions; for interviews, standardized interviewing techniques; etc.
- Data management protocols: Plan for the storage, organization, and protection of your data. Address ethical considerations, especially for sensitive information. Include a system for updating and correcting data to maintain its accuracy over time.
- Project timeline : Outline a realistic timeline with start and end dates, including key milestones. Incorporate flexibility for unforeseen delays or challenges.
- Needed resources: Identify the team, tools, and budget required. Clearly define roles and responsibilities to ensure a smooth data collection process.
- Data analysis strategy: Determine how you'll analyze the collected data. Include methods for dealing with unexpected findings, like ambiguous, conflicting, corrupted, or incomplete data.
- Feedback mechanisms: Establish a mechanism for ongoing assessment and adjustment of your data collection methods. This allows you to adapt and refine your approach as needed.
- Communication framework: Decide how and when you'll communicate your findings. Depending on the project, you might need to keep stakeholders updated throughout the process, not just at the end, to maintain engagement and transparency.
Try to meticulously address each of these elements to set the stage for successful data gathering.
Ways to collect data
Collecting data is akin to gathering and sorting the pieces for a puzzle. Each piece, or data point, is critical to form a complete and accurate picture of the subject under study.
To ensure that this picture is as clear and precise as possible, researchers and analysts employ a variety of data collection methods outlined in the image below.
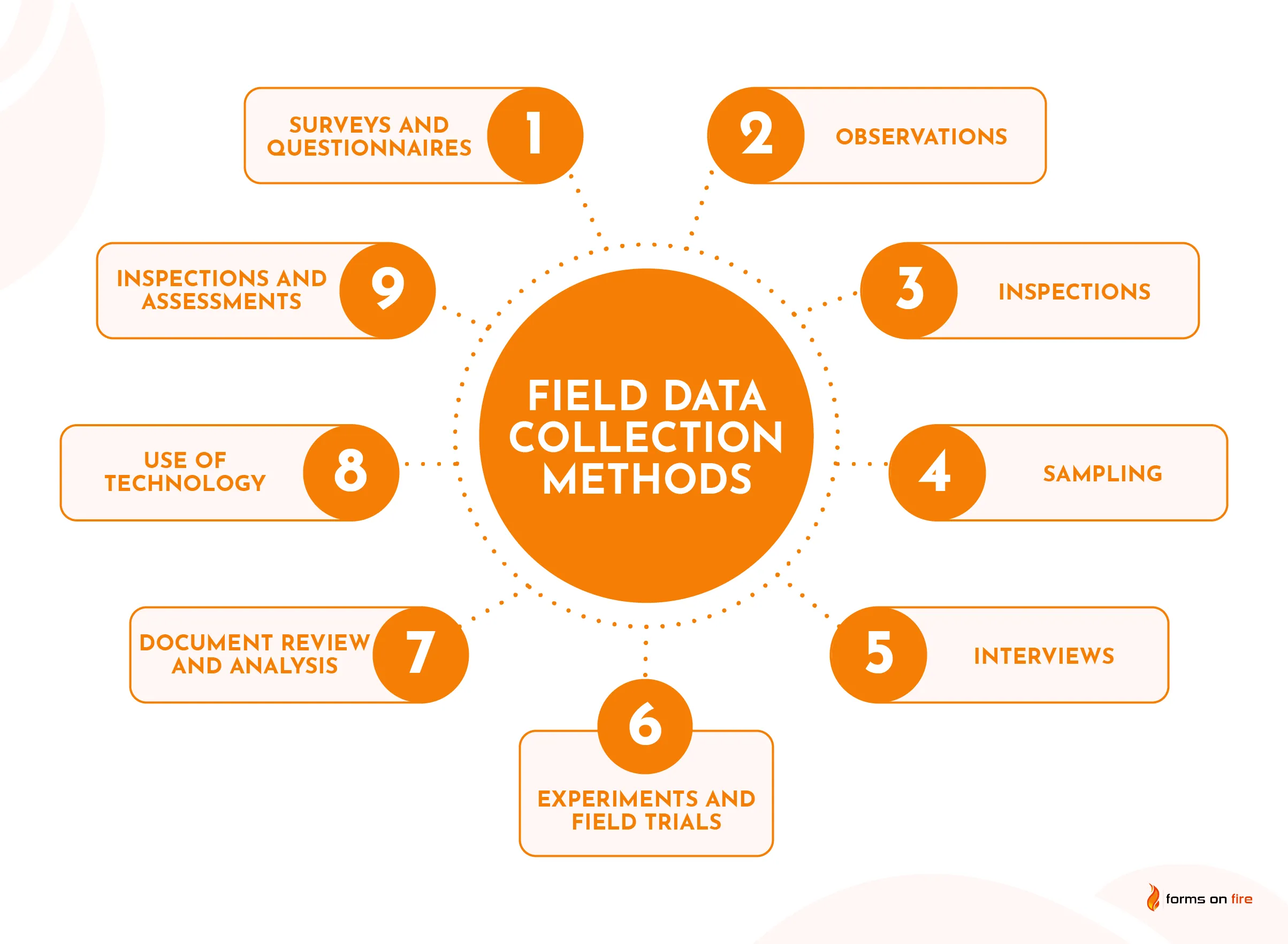
- Surveys and questionnaires: These involve asking structured questions to a large group of people. Consider the timing of your survey distribution — sending out surveys at a time when your target audience is likely to be available and attentive can significantly improve the response quality.
- Interviews: One-on-one conversations that allow for deep dives into subjects' thoughts and experiences. Record interviews (with permission) and note non-verbal cues. These can provide context often lost in written notes, like the respondent's tone or hesitation.
- Focus groups: Small groups of people discuss specific topics, providing qualitative data on opinions and behaviors. Use a skilled moderator who can encourage quieter members to speak up and keep dominant personalities from overtaking the conversation.
- Observations: Watching and recording behavior or events as they naturally occur. If possible, conduct observations at different times or in varied settings. This helps in understanding if the observed behavior is consistent or situation-dependent.
- Inspections and assessments: Examining objects, processes, or places in detail, often using a structured approach supported by pre-made checklists.
- Document review and analysis: Systematically reviewing and interpreting existing documents to extract data. Cross-reference information from different documents for a more comprehensive understanding. This triangulation can validate findings and reveal deeper insights.
Each of these methods offers a unique way to gather data and comes with its own set of pros and cons. Take your time to decide which data collection methods are the best fit for your use case.
Steps for writing an effective data collection plan
With the theory out of the way, let’s see how to write a proper data collection plan, step by step.
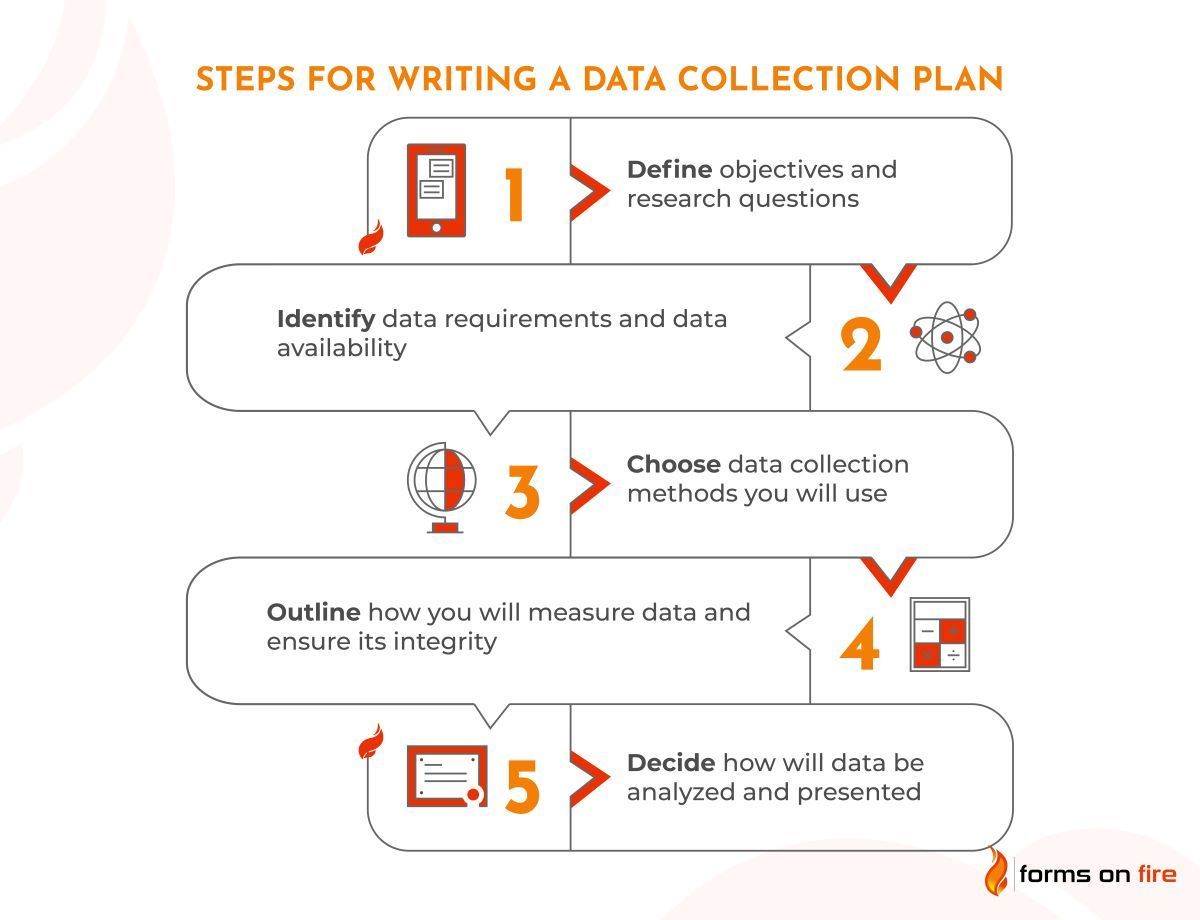
1. Define objectives and research questions
Write down a statement of purpose that explains what you intend to discover, decide, or achieve. This statement will act as the compass for your data collection journey.
Your research questions must be clear, focused, and aligned with your stated objectives. For every objective, draft at least one research question that, when answered, will bring you closer to your goal.
When finalizing your list of research questions, don't overlook the "so what?" factor. For each one, ask yourself what the implications are if the question is answered or the objective is met. How will it change your understanding, decision-making, or actions? This ensures that your plan has practical value and isn't just an academic exercise.
2. Identify data requirements and availability
Identifying your data requirements is a two-part process: you need to understand the type of data you need and assess the data that is already available to you.
Here's how to understand the type of data you need:
- Consider the nature of your research questions: What data will provide the answers? Is it demographic information, behavioral metrics, financial statistics, etc.?
- Determine the data quantity: How much data is enough to make your results reliable? This can depend on the statistical methods you plan to use and the scale of your project.
- Think about the data quality: What level of accuracy is required? Does the data need to be current, historical, or predictive?
Create a data inventory list. For each research question, list the types of data that could potentially answer it. Next to each type, note down the attributes of the data you need (timeframe, demographic details, granularity, etc.).
To assess the data that is already available to you, follow these:
- Look internally first: Does your organization already have some of the data you need? This could be sales records, customer feedback, or past survey results.
- Consider external sources: Is there public data available that fits your needs, such as government databases, research papers, or industry reports?
- Evaluate accessibility: Can you easily access this data, or are there barriers (e.g., paywalls, privacy laws, data sharing agreements) that you need to consider?
For each piece of required data, try to record its source, format, any costs associated with obtaining it, and any potential challenges in accessing it. If data is not available, note down what proxies could be used or whether secondary data collection is necessary.
Completing this step will form the backbone of your data collection strategy, guiding you on where to focus your resources.
3. Choose how you will collect data
Based on your data requirements, select the most suitable collection methods. Will you use surveys, interviews, observations, experiments, or a combination of multiple methods?
Match data collection methods to the type of data you need. For quantitative data, you might use surveys or sensor data. For qualitative data, consider interviews or focus groups. Think about the context of your research — does it call for controlled experiments, or would field studies yield better results?
Once you've selected a method, it's time to think about who will shoulder the task. The 'who' could range from your own team members to external professionals, depending on the expertise required.
Incorporate quality control measures right from the start. This should include when and where data will be collected, the tools or technologies used, and the step-by-step process for gathering the data.
Finally, address ethical considerations, especially if you’re dealing with human subjects or sensitive data. Obtain necessary permissions and ensure you’re compliant with relevant laws and regulations.
4. Outline how you will measure data and ensure its integrity
Clearly specify what you are measuring and how it will be quantified. Are you looking at frequencies, averages, percentages, or growth rates? Ensure that the chosen metrics align directly with your research questions and objectives.
Develop and document standardized procedures for data measurement: define operational terms, detail measurement techniques, and specify the equipment or software used.
For each variable, write down a clear operational definition, which is a detailed description of the procedures used to measure it . For example, if you're measuring customer satisfaction, define what constitutes satisfaction and the scale you're using (e.g., 1-5 likert scale ).
To ensure data integrity, team members tasked with collecting and analyzing data really need to know what they’re doing. If you’re using instruments or software, ensure they are calibrated and tested before data collection begins. Consider running a pilot study or trial to test your measurement processes and make adjustments where necessary. This helps you catch potential issues before you roll out large-scale data collection.
Create a data log that records when and by whom data was collected, entered, and verified. Make sure to regularly check a sample of data entries against the original data to ensure accuracy. If you’re using mobile forms or other digital tools to collect data, most of this can be automated.
Lastly, decide in advance how you will deal with missing data or outliers. Will you use imputation methods , or will you exclude it? Make sure your approach is consistent and documented.
5. Decide how will data be analyzed and presented
Outlines each step of your analysis process: the methods you'll use, the required tools, and the sequence of analysis.
Choose analysis methods that align with your data types and objectives. For analyzing quantitative data , statistical methods like regression analysis, ANOVA, or cluster analysis might be appropriate. For analyzing qualitative data , try content analysis, thematic analysis, or discourse analysis.
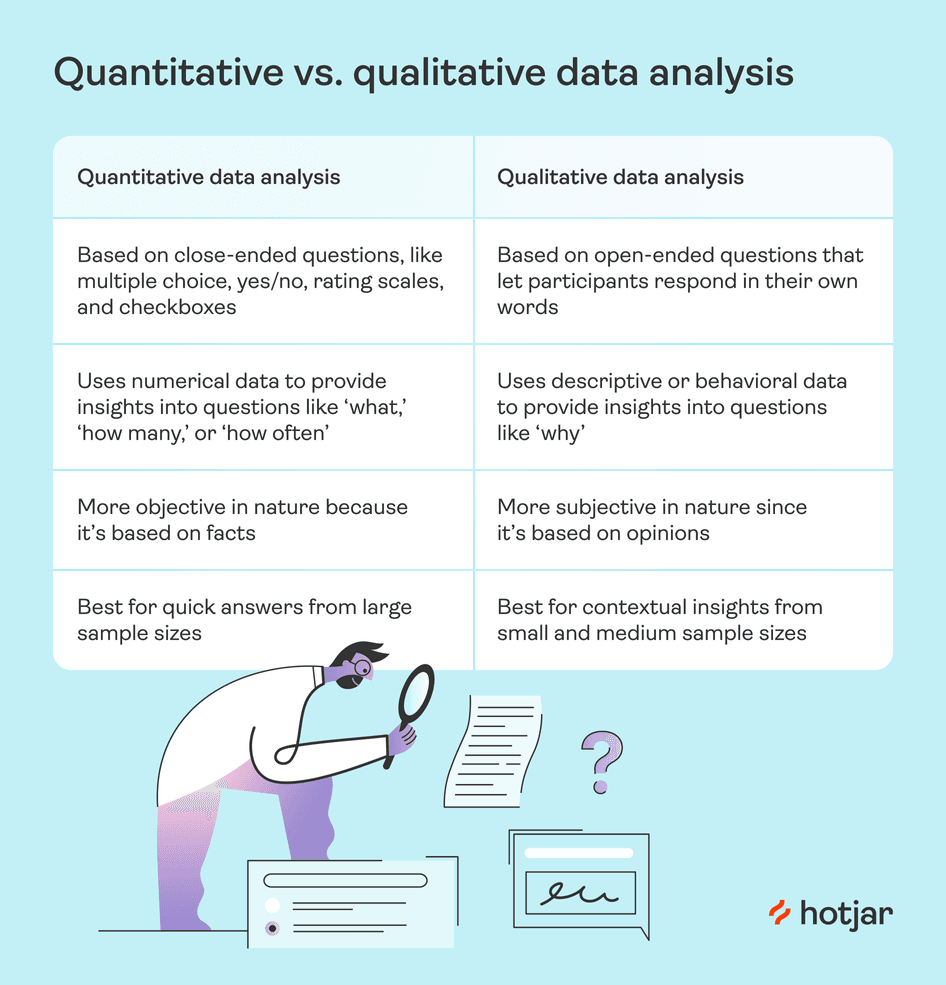
If you have a complex project and plan to use specific software to analyze data, decide which one that is going to be. Options could range from statistical software like SPSS or R for quantitative analysis to software like NVivo for qualitative data analysis.
Think about how you will present your data. This could be in the form of reports, infographics, dashboards, or presentations. Choose the format with your audience in mind — what format will be most clear and persuasive to them?
Try sketching out a draft of your final report or presentation early in the planning process. This helps you visualize the end product and ensure that your data collection and analysis will support this outcome.
Data collection plan examples and templates
Below are four different examples and templates you can use to build your own data collection plans.
Streamline data collection process with Forms On Fire
Forms on Fire offers a flexible, accessible, and efficient platform for automating and simplifying data collection and management:
- Customizable forms and mobile accessibility: Design forms specific to your needs and capture data on the go, even offline, with mobile device support.
- Automated workflows and integration capabilities: Automate processes like notifications and data analysis upon form submission, while seamlessly integrating with over 1,500 systems.
- Real-time data access and reporting: Access and analyze data instantly for timely decision-making, trend spotting, and addressing issues quickly.
- Cloud-based data storage: Securely store all data in the cloud, ensuring safety and availability from any location at any time.
- User-friendly interface: Enjoy an intuitive platform that requires no coding skills, making it easy for anyone in your organization to create and use forms.
Ready to transform your data collection process? Start a free trial below or schedule a product demo and see how our solution can answer your data management needs!
+1 (425) 214-1920
10900 NE 4th Street, Suite 2300
Bellevue, WA 98004
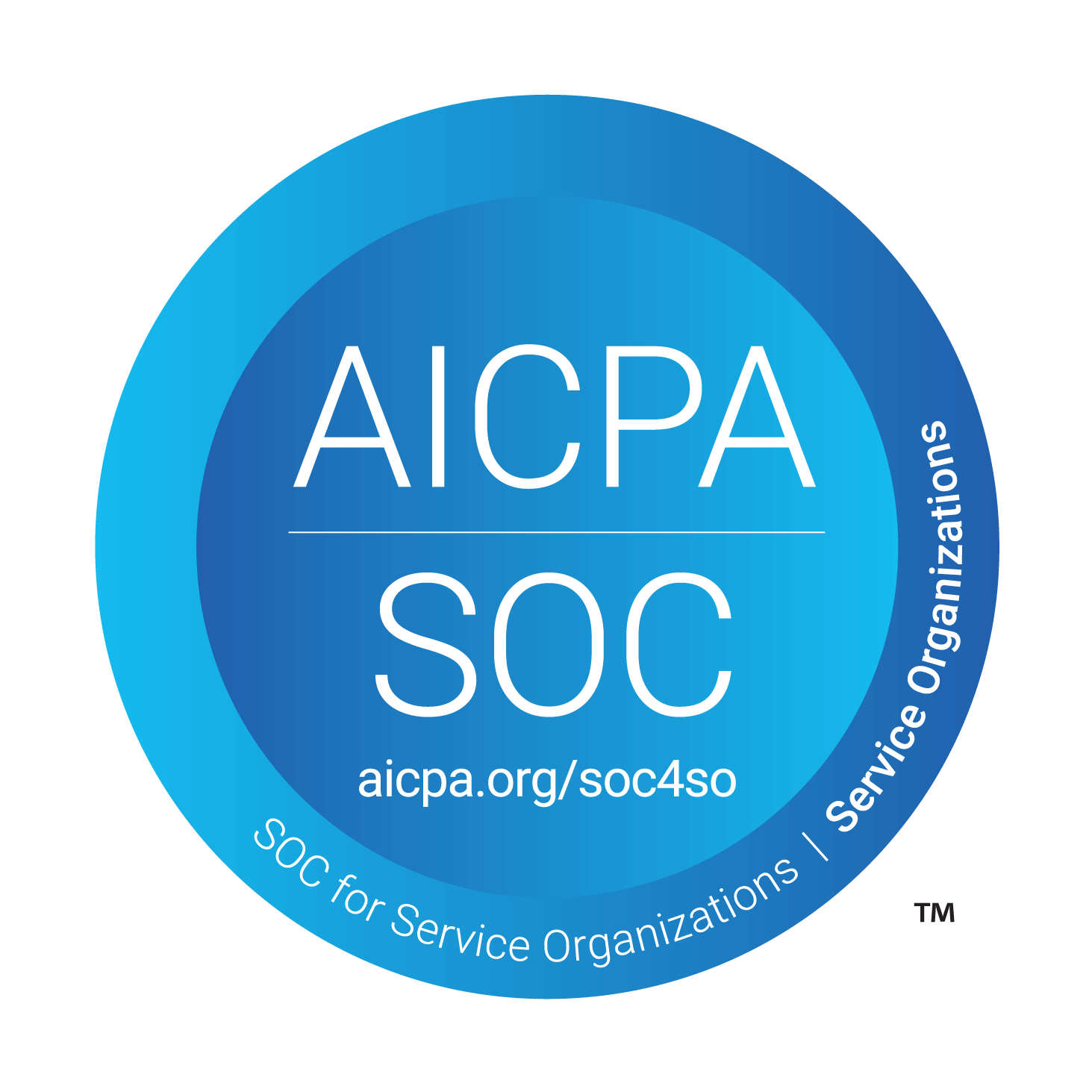
© 2023 Forms On Fire, All Rights Reserved
Policy Center | Terms of Service | Privacy
- Skip to main content
- Skip to primary sidebar
- Skip to footer
- QuestionPro

- Solutions Industries Gaming Automotive Sports and events Education Government Travel & Hospitality Financial Services Healthcare Cannabis Technology Use Case NPS+ Communities Audience Contactless surveys Mobile LivePolls Member Experience GDPR Positive People Science 360 Feedback Surveys
- Resources Blog eBooks Survey Templates Case Studies Training Help center

Home Market Research
Data Collection Plan: What it is + Steps to Do It
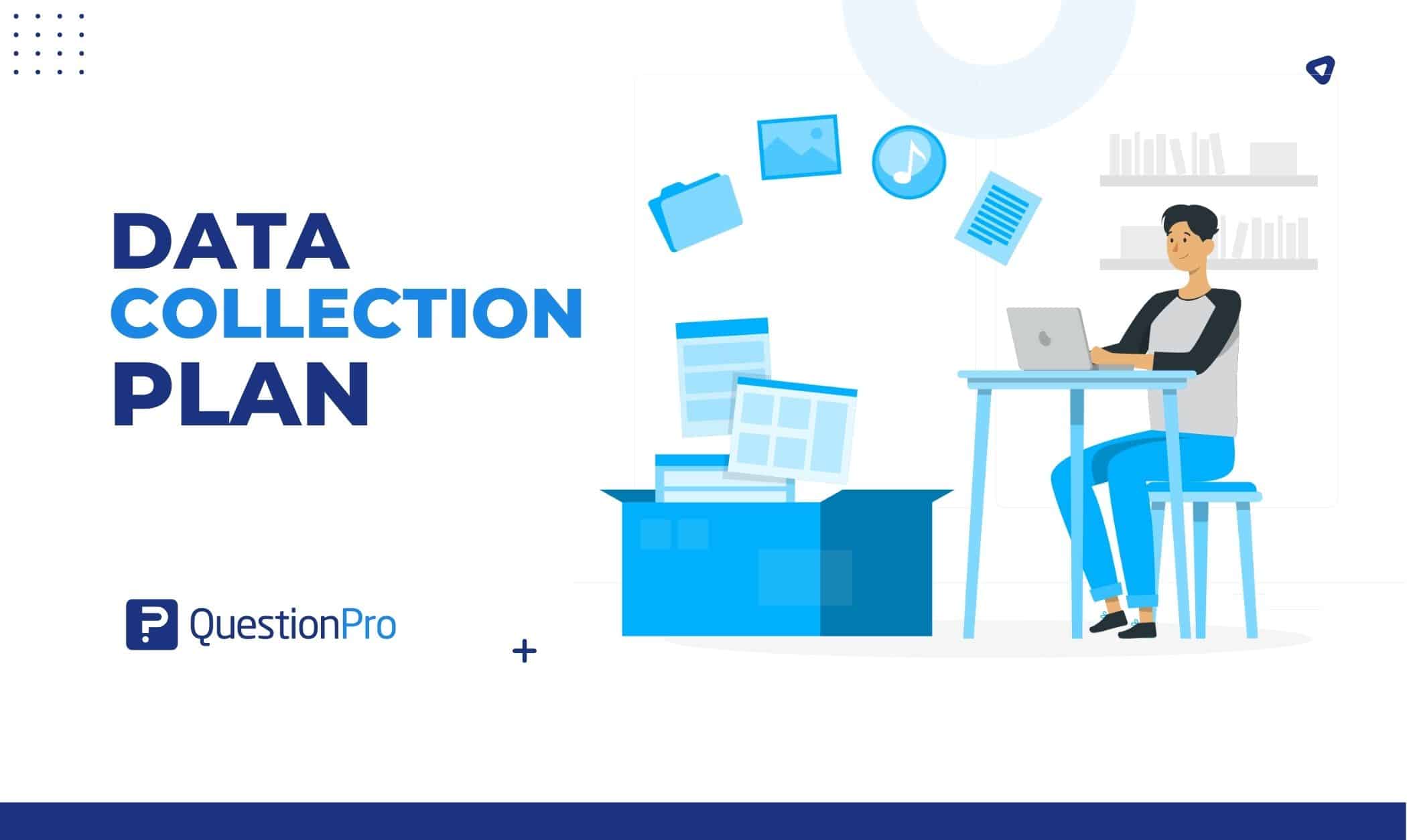
Several plans work together to create a robust, comprehensive, and overall successful market research initiative, and one of the most important pieces is a data collection plan.
LEARN ABOUT: Research Process Steps
A data collection plan describes how your organization’s data will flow from its source to actionable insights. The process of creating this plan will reveal where your data comes from, who has access to it, and how it is collected and stored.
Below, we explain a data collection plan, why you need one, and how you will use it. We also go over the key steps to creating a data-collecting plan to ensure your data is on track to produce actionable insights that drive your business forward.
LEARN ABOUT: Pareto Chart
What is a data collection plan?
A data collection plan is a detailed document outlining the exact steps and sequences to collect data for a project. It is a statistical analysis plan to achieve breakthrough improvement by reducing variation and defects.
A data-collecting plan ensures that data is accurately sent to the organization’s key stakeholders, who will assist them in meeting their data needs. The plan aims to ensure that the data gathered are valid and meaningful.
We need a data-collecting plan to avoid wasting resources on irrelevant or unusable data. By making a plan for collecting data, we can focus on answering specific business-related questions.
Why Do You Need a Data Collection Plan?
Gathering a lot of different kinds of data and looking at them won’t help much if you can’t figure out what they mean. A good plan for collecting data helps save money and time since gathering data without a plan can take a long time. Also, it may not be possible to get all the data when it is needed.
And these are the most important reasons your business needs a plan for collecting data. By making a plan for collecting data, a business can focus on answering specific questions important to the business.
When and how to utilize a data collection plan?
A comprehensive data-collecting plan ensures that the gathered data is useful and well-organized. The plan is utilized to evaluate the existing state of a process or to enhance a project or process. In addition, it is useful during the last phase of a project when generating new metrics and the necessary evaluation procedures.
A proper data collecting plan means taking a systematic approach, including:
- Identify the data to collect.
- How the data will be collected
- Collecting the data
Steps to create a data collection plan
Now, we will explore the steps of a data collection plan to explain how to make one. The plan for gathering data involves 8 steps:
- Identify the questions
The first step in making a data collection plan is to decide what questions we want to answer. Our information has to be useful for the project. These questions should be based on what our process is really like in its current state.
The best way to gather data is to use the SIPOC diagram as a guide. We also have to decide what measurements or metrics we want to use.
- Identify the accessible data
The second step in developing a data collecting plan is to determine what types of data can be collected. A specific piece of data may occasionally provide us with many solutions. Make sure you list all the data points you need to answer the questions on which the project is based.
- Determine how much data is required
A data collection plan’s third step is determining the required data. Write down how much data is required for each bit on the list. Our goal is to gather enough information to do appropriate data analysis and identify patterns and trends.
- Decide how to measure the data
The fourth step in developing a data collection plan is determining how we measure the data. Data can be measured in various ways, such as check sheets, survey responses, etc. The type of data we seek will determine how we measure it.
Contact QuestionPro if you want to measure your data by conducting surveys. QuestionPro is survey software with a wide variety of survey features.
- Determine who will collect the data
The fifth step in developing a data collecting plan is determining who will collect the data. Data can now be collected using automated software. We may need to communicate with the person in charge of the software to ensure that the data is in the proper format.
- Choose the data source
The sixth step is to determine the data sources. Choosing a location or data source is what it signifies. Location does not always mean a physical place. It is the place where the process is at. The data collection plan must specifically state where data must be collected throughout the process.
- Choose whether to measure a sample or the entire population
The seventh step is deciding whether or not to sample the data. It is often impractical to measure a whole data Pre-population . In this situation, we then collect a sample of data. The project team may need to investigate the following question: What should our sampling method and sample size be to produce statistically accurate judgments?
- Determine data display format
The eighth step is to decide how to display the data. We can show data in many ways, such as Pareto Diagrams, Scatter Diagrams, etc.
LEARN ABOUT: Best Data Collection Tools
We gained a strong understanding of the data collection plan, why you need it for your business, and how you use it from the talk above. Additionally, we discussed steps that will assist you in creating your own.
The data collecting plan states what kinds of data need to be collected, how, where, how often, and who is responsible for collecting the data. This document ensures that all relevant employees understand the requirements for data collection.
Now you know how to develop a data collection plan for your business. If you need help making your plan, you can contact QuestionPro. QuestionPro is a survey software and data management software that assists in data management and provides real-time data and business insight. To learn more, ask for a free trial now!
LEARN MORE FREE TRIAL
MORE LIKE THIS
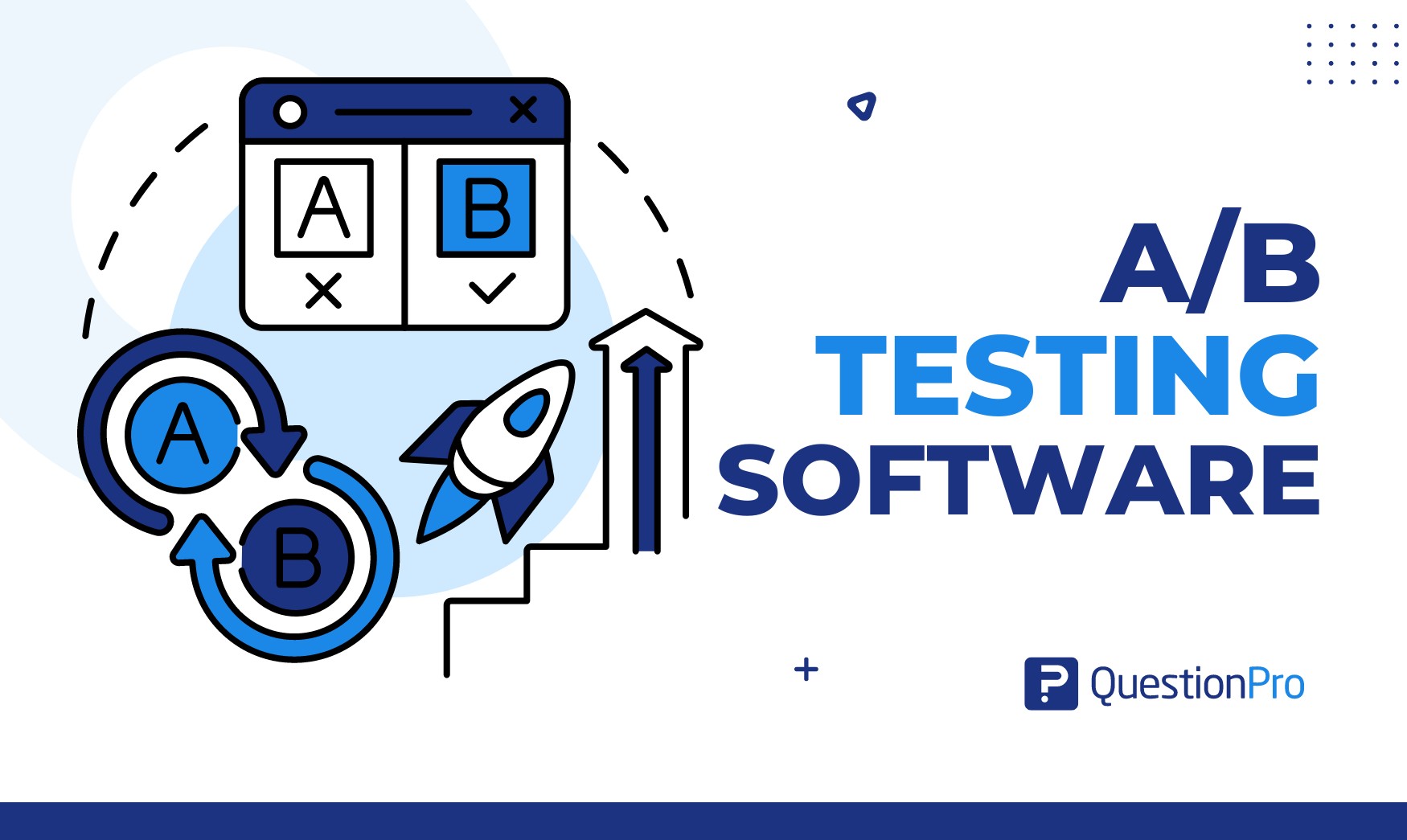
Top 13 A/B Testing Software for Optimizing Your Website
Apr 12, 2024
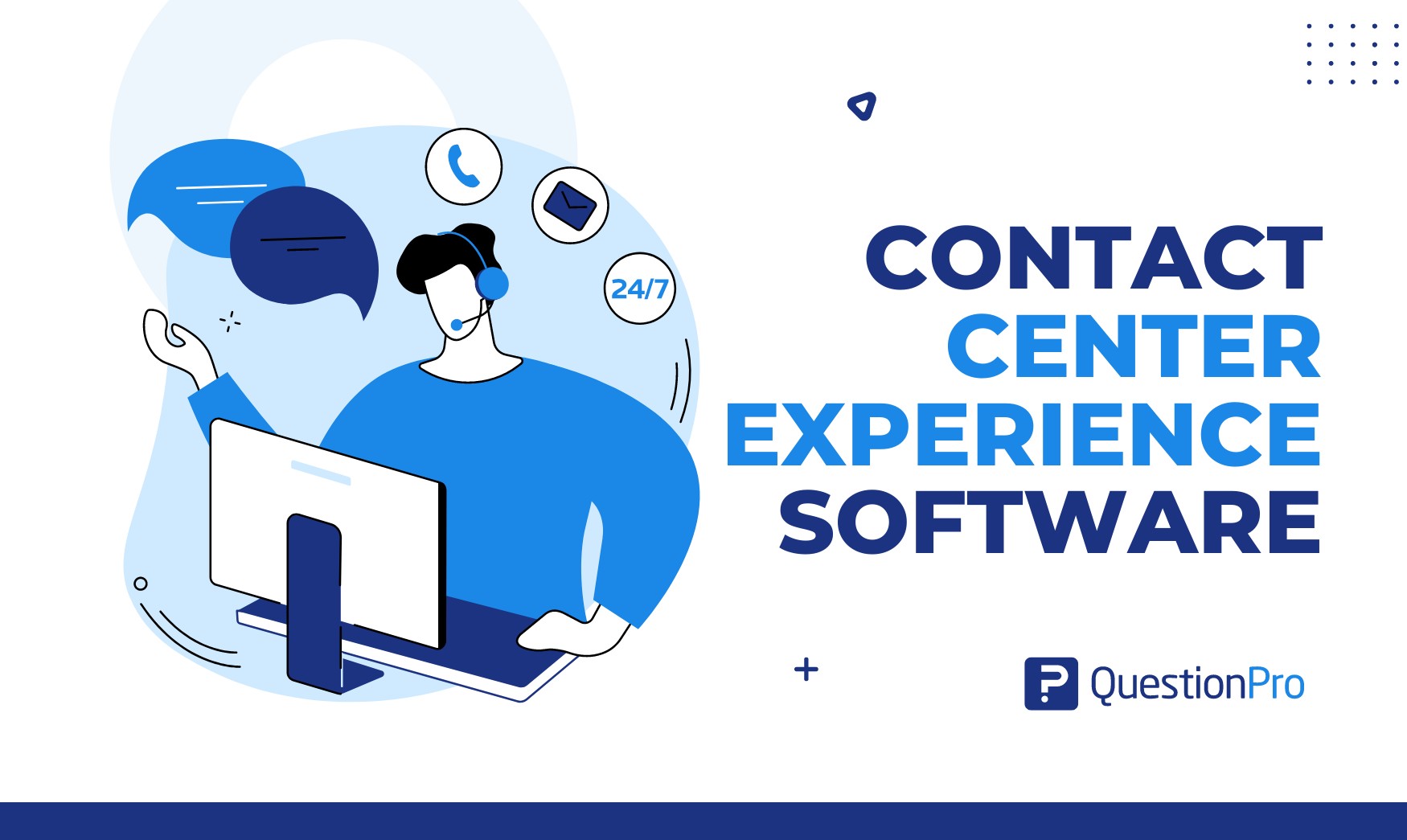
21 Best Contact Center Experience Software in 2024
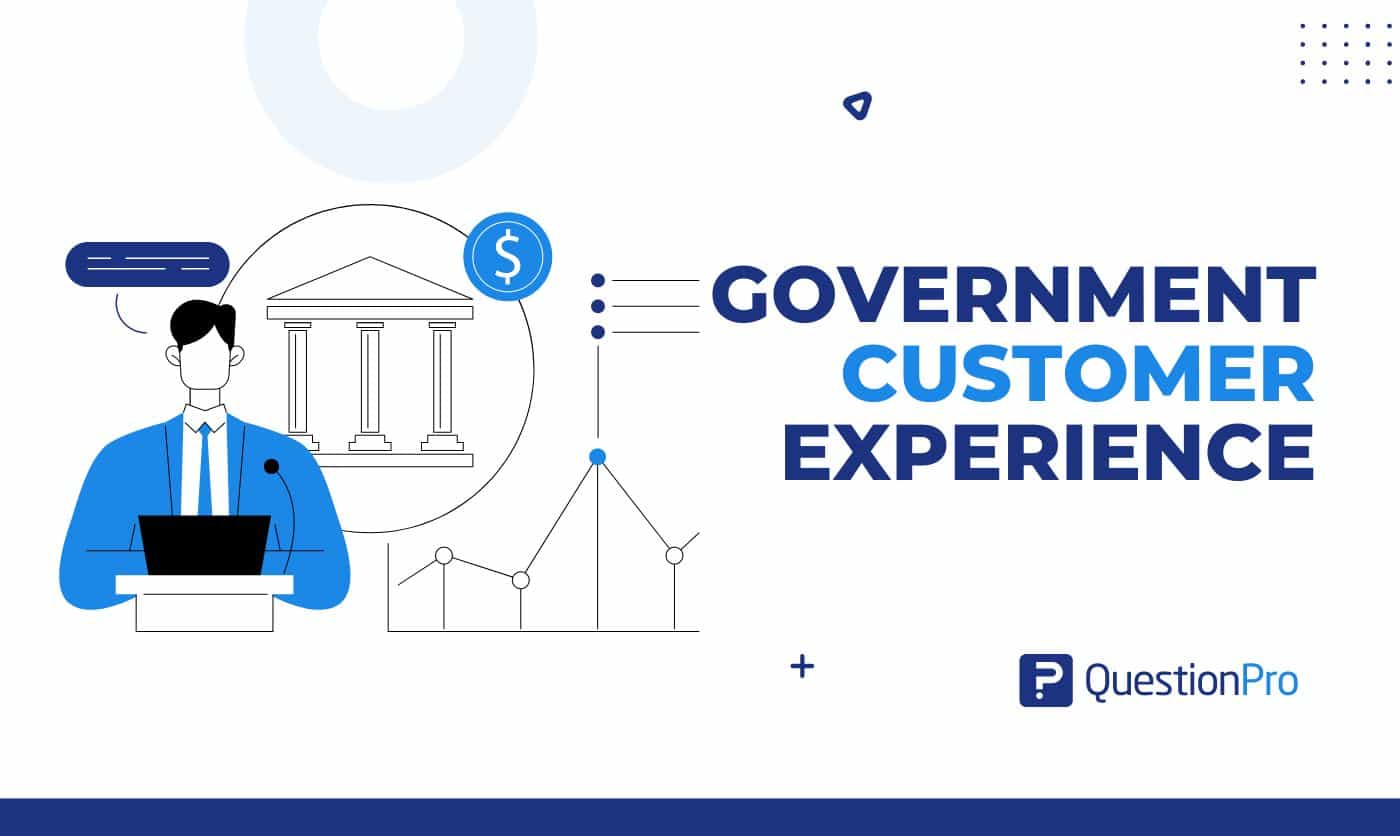
Government Customer Experience: Impact on Government Service
Apr 11, 2024
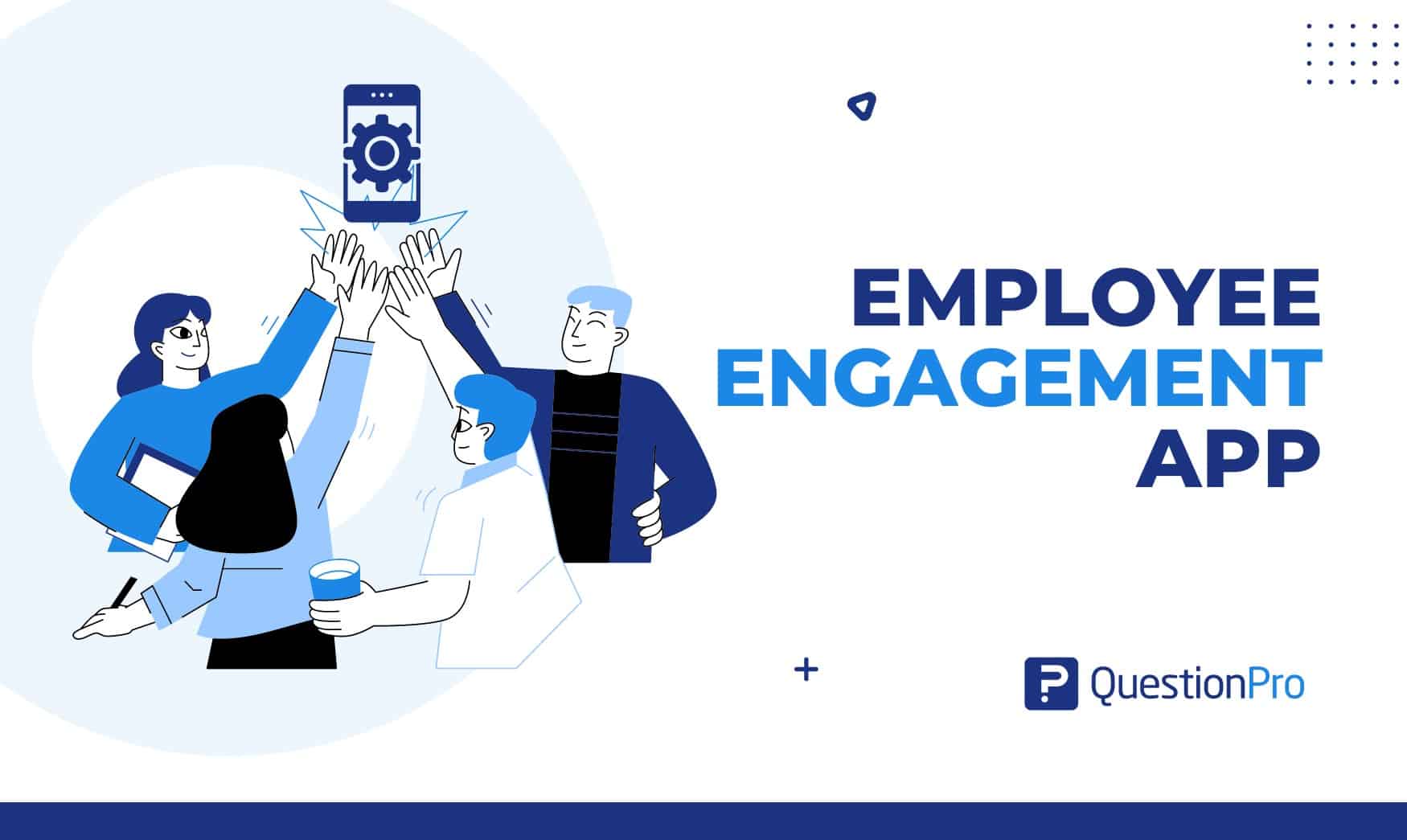
Employee Engagement App: Top 11 For Workforce Improvement
Apr 10, 2024
Other categories
- Academic Research
- Artificial Intelligence
- Assessments
- Brand Awareness
- Case Studies
- Communities
- Consumer Insights
- Customer effort score
- Customer Engagement
- Customer Experience
- Customer Loyalty
- Customer Research
- Customer Satisfaction
- Employee Benefits
- Employee Engagement
- Employee Retention
- Friday Five
- General Data Protection Regulation
- Insights Hub
- Life@QuestionPro
- Market Research
- Mobile diaries
- Mobile Surveys
- New Features
- Online Communities
- Question Types
- Questionnaire
- QuestionPro Products
- Release Notes
- Research Tools and Apps
- Revenue at Risk
- Survey Templates
- Training Tips
- Uncategorized
- Video Learning Series
- What’s Coming Up
- Workforce Intelligence
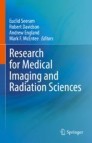
Research for Medical Imaging and Radiation Sciences pp 97–157 Cite as
Data Collection, Analysis, and Interpretation
- Mark F. McEntee 5
- First Online: 03 January 2022
458 Accesses
Often it has been said that proper prior preparation prevents performance. Many of the mistakes made in research have their origins back at the point of data collection. Perhaps it is natural human instinct not to plan; we learn from our experiences. However, it is crucial when it comes to the endeavours of science that we do plan our data collection with analysis and interpretation in mind. In this section on data collection, we will review some fundamental concepts of experimental design, sample size estimation, the assumptions that underlie most statistical processes, and ethical principles.
This is a preview of subscription content, log in via an institution .
Buying options
- Available as PDF
- Read on any device
- Instant download
- Own it forever
- Available as EPUB and PDF
- Compact, lightweight edition
- Dispatched in 3 to 5 business days
- Free shipping worldwide - see info
- Durable hardcover edition
Tax calculation will be finalised at checkout
Purchases are for personal use only
Al-Murshedi, S., Hogg, P., & England, A. (2018). An investigation into the validity of utilising the CDRAD 2.0 phantom for optimisation studies in digital radiography. The British Journal of Radiology . British Institute of Radiology , 91 (1089), 4. https://doi.org/10.1259/bjr.20180317
Article Google Scholar
Alhailiy, A. B., et al. (2019). The associated factors for radiation dose variation in cardiac CT angiography. The British Journal of Radiology . British Institute of Radiology , 92 (1096), 20180793. https://doi.org/10.1259/bjr.20180793
Article PubMed PubMed Central Google Scholar
Armato, S. G., et al. (2011). The Lung Image Database Consortium (LIDC) and Image Database Resource Initiative (IDRI): A completed reference database of lung nodules on CT scans. Medical Physics . John Wiley and Sons Ltd , 38 (2), 915–931. https://doi.org/10.1118/1.3528204
Avison, D. E., et al. (1999). Action research. Communications of the ACM . Association for Computing Machinery (ACM) , 42 (1), 94–97. https://doi.org/10.1145/291469.291479
Båth, M., & Månsson, L. G. (2007). Visual grading characteristics (VGC) analysis: A non-parametric rank-invariant statistical method for image quality evaluation. British Journal of Radiology, 80 (951), 169–176. https://doi.org/10.1259/bjr/35012658
Chakraborty, D. P. (2017). Observer performance methods for diagnostic imaging . CRC Press. https://doi.org/10.1201/9781351228190
Book Google Scholar
Couper, M. P., Traugott, M. W., & Lamias, M. J. (2001). Web survey design and administration. Public Opinion Quarterly . Oxford Academic , 65 (2), 230–253. https://doi.org/10.1086/322199
Article CAS PubMed Google Scholar
European Commission European Guidelines on Quality Criteria for Diagnostic Radiographic Images EUR 16260 EN. (1995).
Google Scholar
Fähling, M., et al. (2017). Understanding and preventing contrast-induced acute kidney injury. Nature Reviews Nephrology . Nature Publishing Group, 169–180. https://doi.org/10.1038/nrneph.2016.196
Faucon, A. L., Bobrie, G., & Clément, O. (2019). Nephrotoxicity of iodinated contrast media: From pathophysiology to prevention strategies. European Journal of Radiology . Elsevier Ireland Ltd, 231–241. https://doi.org/10.1016/j.ejrad.2019.03.008
Fisher, M. J., & Marshall, A. P. (2009). Understanding descriptive statistics. Australian Critical Care . Elsevier , 22 (2), 93–97. https://doi.org/10.1016/j.aucc.2008.11.003
Article PubMed Google Scholar
Fryback, D. G., & Thornbury, J. R. (1991). The efficacy of diagnostic imaging. Medical Decision Making . Sage PublicationsSage CA: Thousand Oaks, CA , 11 (2), 88–94. https://doi.org/10.1177/0272989X9101100203
Ganesan, A., et al. (2018). A review of factors influencing radiologists’ visual search behaviour. Journal of Medical Imaging and Radiation Oncology . Blackwell Publishing , 62 (6), 747–757. https://doi.org/10.1111/1754-9485.12798
Gilligan, L. A., et al. (2020). Risk of acute kidney injury following contrast-enhanced CT in hospitalized pediatric patients: A propensity score analysis. Radiology . Radiological Society of North America Inc. , 294 (3), 548–556. https://doi.org/10.1148/radiol.2020191931
Good, P. I., & Hardin, J. W. (2012). Common errors in statistics (and how to avoid them): Fourth edition . Wiley. https://doi.org/10.1002/9781118360125
Gusterson, H. (2008). Ethnographic research. In Qualitative methods in international relations (pp. 93–113). Palgrave Macmillan UK. https://doi.org/10.1057/9780230584129_7
Chapter Google Scholar
Hansson, J., Månsson, L. G., & Båth, M. (2016). The validity of using ROC software for analysing visual grading characteristics data: An investigation based on the novel software VGC analyzer. Radiation Protection Dosimetry . Oxford University Press , 169 (1–4), 54–59. https://doi.org/10.1093/rpd/ncw035
Home - LUNA16 - Grand Challenge. (n.d.). Available at: https://luna16.grand-challenge.org/ . Accessed 25 Mar 2021.
Huda, W., et al. (1997). Comparison of a photostimulable phosphor system with film for dental radiology. Oral Surgery, Oral Medicine, Oral Pathology, Oral Radiology, and Endodontics . Mosby Inc. , 83 (6), 725–731. https://doi.org/10.1016/S1079-2104(97)90327-9
Iarossi, G. (2006). The power of survey design: A user’s guide for managing surveys, interpreting results, and influencing respondents . Available at: https://books.google.com/books?hl=en&lr=&id=E-8XHVsqoeUC&oi=fnd&pg=PR5&dq=survey+design&ots=fADK9Aznuk&sig=G5DiPgYM18VcoZ-PF05kT7G0OGI . Accessed 21 Mar 2021.
Jang, J. S., et al. (2018). Image quality assessment with dose reduction using high kVp and additional filtration for abdominal digital radiography. Physica Medica . Associazione Italiana di Fisica Medica , 50 , 46–51. https://doi.org/10.1016/j.ejmp.2018.05.007
Jessen, K. A. (2004). Balancing image quality and dose in diagnostic radiology. European Radiology, Supplement . Springer , 14 (1), 9–18. https://doi.org/10.1007/s10406-004-0003-7
King, N., Horrocks, C., & Brooks, J. (2018). Interviews in qualitative research . Available at: https://books.google.com/books?hl=en&lr=&id=ZdB2DwAAQBAJ&oi=fnd&pg=PP1&dq=interviews+in+research&ots=hwRx2cwH3W&sig=_gt8y-4GlHSCnTQAhLfynA3C17E . Accessed: 21 Mar 2021.
Krul, A. J., Daanen, H. A. M., & Choi, H. (2011). Self-reported and measured weight, height and body mass index (BMI) in Italy, the Netherlands and North America. The European Journal of Public Health . Oxford Academic , 21 (4), 414–419. https://doi.org/10.1093/eurpub/ckp228
Kundel, H. L. (1979). Images, image quality and observer performance. New horizons in radiology lecture. Radiology, 132 (2), 265–271. https://doi.org/10.1148/132.2.265
Makary, M. A., & Daniel, M. (2016). Medical error-the third leading cause of death in the US. BMJ (Online) . BMJ Publishing Group , 353 . https://doi.org/10.1136/bmj.i2139
Martin, C. J., Sharp, P. F., & Sutton, D. G. (1999). Measurement of image quality in diagnostic radiology. Applied Radiation and Isotopes . Elsevier Sci Ltd , 50 (1), 21–38. https://doi.org/10.1016/S0969-8043(98)00022-0
Mathematical methods of statistics / by Harald Cramer | National Library of Australia (n.d.). Available at: https://catalogue.nla.gov.au/Record/81100 . Accessed: 22 Mar 2021.
McCollough, C. H., & Schueler, B. A. (2000). Calculation of effective dose. Medical Physics . John Wiley and Sons Ltd , 27 (5), 828–837. https://doi.org/10.1118/1.598948
Meissner, H., et al. (n.d.). Best Practices for Mixed Methods Research in the Health Sciences_the_nature_and_design_of_mixed_methods_research .
Morgan, D. L. (1996). Focus groups. Annual Review of Sociology . Annual Reviews Inc. , 22 , 129–152. https://doi.org/10.1146/annurev.soc.22.1.129
Moses, L. E., Shapiro, D., & Littenberg, B. (1993). Combining independent studies of a diagnostic test into a summary roc curve: Data-analytic approaches and some additional considerations. Statistics in Medicine . John Wiley & Sons, Ltd , 12 (14), 1293–1316. https://doi.org/10.1002/sim.4780121403
Neill Howell 2008 Inferential Statistical Decision Tree – StuDocu . (n.d.). Available at: https://www.studocu.com/en-gb/document/university-of-hertfordshire/using-data-to-address-research-questions/summaries/neill-howell-2008-inferential-statistical-decision-tree/1193346/view . Accessed: 23 Mar 2021.
Neuendorf, K. A., & Kumar, A. (2016). Content analysis. In The international encyclopedia of political communication (pp. 1–10). Wiley. https://doi.org/10.1002/9781118541555.wbiepc065
Nguyen, P. K., et al. (2015). Assessment of the radiation effects of cardiac CT angiography using protein and genetic biomarkers. JACC: Cardiovascular Imaging . Elsevier Inc. , 8 (8), 873–884. https://doi.org/10.1016/j.jcmg.2015.04.016
Noordzij, M., et al. (2010). Sample size calculations: Basic principles and common pitfalls. Nephrology Dialysis Transplantation . Oxford University Press, , 25 (5), 1388–1393. https://doi.org/10.1093/ndt/gfp732
Pisano, E. D., et al. (2005). Diagnostic performance of digital versus film mammography for breast-cancer screening. New England Journal of Medicine . Massachusetts Medical Society , 353 (17), 1773–1783. https://doi.org/10.1056/NEJMoa052911
Article CAS Google Scholar
ROC curve analysis with MedCalc. (n.d.). Available at: https://www.medcalc.org/manual/roc-curves.php . Accessed 30 Mar 2021.
Rudolfer, S. M. (2003). ZHOU, X.-H., OBUCHOWSKI, N. A. and MCCLISH, D. K. statistical methods in diagnostic medicine. Wiley, New York, 2002. xv + 437 pp. $94.95/£70.50. ISBN 0-471-34772-8. Biometrics . Wiley-Blackwell , 59 (1), 203–204. https://doi.org/10.1111/1541-0420.00266
Sudheesh, K., Duggappa, D. R., & Nethra, S. S. (2016). How to write a research proposal? Indian Journal of Anaesthesia . https://doi.org/10.4103/0019-5049.190617
Download references
Author information
Authors and affiliations.
University College Cork, Cork, Ireland
Mark F. McEntee
You can also search for this author in PubMed Google Scholar
Corresponding author
Correspondence to Mark F. McEntee .
Editor information
Editors and affiliations.
Medical Imaging, Faculty of Health, University of Canberra, Burnaby, BC, Canada
Euclid Seeram
Faculty of Health, University of Canberra, Canberra, ACT, Australia
Robert Davidson
Brookfield Health Sciences, University College Cork, Cork, Ireland
Andrew England
Rights and permissions
Reprints and permissions
Copyright information
© 2021 Springer Nature Switzerland AG
About this chapter
Cite this chapter.
McEntee, M.F. (2021). Data Collection, Analysis, and Interpretation. In: Seeram, E., Davidson, R., England, A., McEntee, M.F. (eds) Research for Medical Imaging and Radiation Sciences. Springer, Cham. https://doi.org/10.1007/978-3-030-79956-4_6
Download citation
DOI : https://doi.org/10.1007/978-3-030-79956-4_6
Published : 03 January 2022
Publisher Name : Springer, Cham
Print ISBN : 978-3-030-79955-7
Online ISBN : 978-3-030-79956-4
eBook Packages : Biomedical and Life Sciences Biomedical and Life Sciences (R0)
Share this chapter
Anyone you share the following link with will be able to read this content:
Sorry, a shareable link is not currently available for this article.
Provided by the Springer Nature SharedIt content-sharing initiative
- Publish with us
Policies and ethics
- Find a journal
- Track your research
- Data Management Plans: A Comprehensive Guide for Researchers
This guide outlines the main areas that researchers should consider when preparing a Data Management Plan (DMP). It includes information on the data specification, institutional repositories, long-term preservation services, IT infrastructure requirements and more.

Data Management Plans (DMPs) are documents prepared by researchers as they plan a project and write a grant proposal. These plans provide an outline of the types of data that will be collected, the data format, including data and metadata standards, and how the data will be handled throughout the project lifecycle. Many research funders require a data management plan with a grant proposal, so it is important for researchers to understand the main areas to consider when preparing one. This guide outlines the main areas that researchers should consider when preparing a data management plan.
It includes information on the data specification, including size, file format , number of files, data dictionary, and codebook. It also covers the use of institutional data repositories hosted in university libraries as an open access platform for the dissemination and archiving of university research data. Additionally, it provides information on long-term preservation of digital data files using services such as migration (limited format types), secure backups, bit-level checksums, and maintaining a persistent DOI for data sets. When a project includes data about people and organizations, this affects the design of the necessary IT infrastructure.
It is expected that the full dataset will be accessible after the study and all related publications are completed, and will remain accessible for at least 10 years after the data become publicly available. This section includes a description of the format of your data and how it will be created, collected, maintained and delivered. In addition, information from individuals or entities that own the intellectual property rights to the data should be included in this section. SPARC (the Academic Resources and Academic Publications Coalition) has compiled an excellent resource with information on the data management and data exchange requirements of all federal funding agencies.
ICPSR allows several mediated forms of exchange, where individuals interested in obtaining a less de-identified individual level would sign data use agreements before receiving the data, or would need to use special software to access it directly from ICPSR instead of downloading it, for security reasons. Some demographics may not be shareable on an individual level and would therefore only be provided in aggregate form. Finally, all applicants submitting funding proposals to the MRC must include a Data Management Plan (DMP) as an integral part of the application. Through DRUM policies, unidentified data will be accompanied by appropriate documentation, metadata and code to facilitate reuse and provide the potential for interoperability with similar datasets. The main benefit of DRUM is that everything shared through this repository is public; however, a fully open system is not optimal if any of the data can be identified.
Related Posts
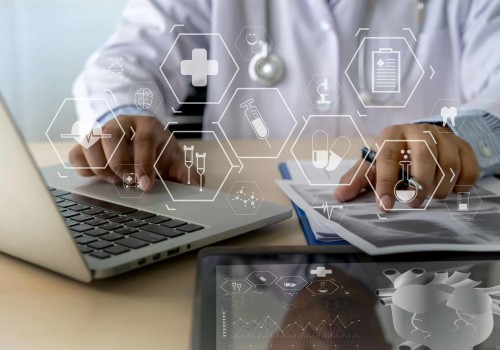
- What are data management skills?
Data management skills are the skills used to effectively manage and use information. Data management skills involve looking for patterns, understanding database design concepts, and being able to participate in the short- and long-term planning of database projects.
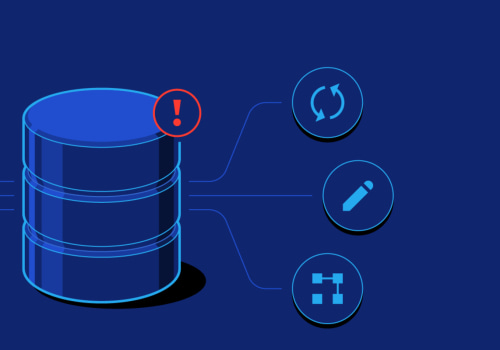
- What software create and manage data base?
Similarly, a database management system (DBMS) is software for creating and managing data in databases, SolarWinds Database Performance Analyzer, DbVisualizer, ManageEngine Applications Manager, Oracle RDBMS, IBM DB2, Microsoft SQL Server, SAP Sybase ASE and Teradata. Database management software or DBMS is used to store, manipulate, and manage data in a database environment.

- Which of the following is api management tool?
Dell Boomi is a 100% cloud-based API solution that allows companies to move, manage and manage data through local or cloud-based applications. It's a single, scalable platform that provides endpoints as a web service.
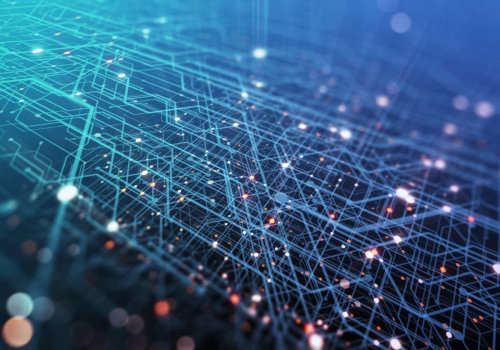
- What are the 5 importance of data processing?
These five areas are (in no particular order of importance): decision making, problem solving, understanding, improving processes, and understanding customers. The importance of data processing includes greater productivity and profits, better decisions, greater accuracy and reliability.
More articles
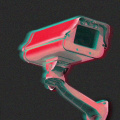
- What Are the Different Types of Management Tools?
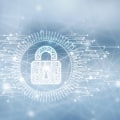
- Four Essential Best Practices for Big Data Governance
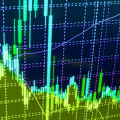
- The Benefits of Understanding Project Data and Processes
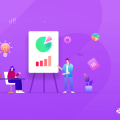
- Data Management Tools: An Expert's Guide
- Data Management Principles: A Comprehensive Guide
- Data Collection Methods: An Expert's Guide
- 9 Best Practices of Master Data Management
- Data Management: Unlocking the Potential of Data
- Data Management: Functions, Storage, Security and More
- What are the 3 dimensions of data governance defined in the active data governance framework?
- Data Management: A Comprehensive Guide to Maximize Benefits
- What is a value in data management?
- Data Management: A Comprehensive Guide
- The Essential Role of Data Management in the Digital Economy
The Benefits of Enterprise Data Management for Businesses
- Understanding Research Data Management Systems
- What is Data Services and How Does it Work?
Why is tools management important?
- What are data standards and interoperability?
- Data Management: What You Need to Know
- Data Management in Research: A Comprehensive Guide
- Data Management: What is it and How to Implement it?
- What are spreadsheet data tools?
- What are the best practices in big data adoption?
- The Benefits of Data Management in Research
- Data Governance vs Data Management: What's the Difference?
- The Ultimate Guide to Enterprise Data Management
- Data Management in Quantitative Research: An Expert's Guide
- What is a data management tool?
- What tools are used in data analysis?
- How do you create a data standard?
- Data Management Softwares: A Comprehensive Guide
- What is Data Management Software and How Does it Work?
- Data Management Services: A Comprehensive Guide
- The Benefits of Data Management: Unlocking the Value of Data
- Who created data management?
- Data Management: Unlocking the Power of Data Analysis
- The Difference Between Enterprise Data Management and Data Management
- 8 Core Principles of Data Management
- What is a data standard?
- How to Effectively Manage Big Data Analytics
- The Essential Role of Data Management in Business
- What is data management framework in d365?
- Data Management: What It Is and Examples
- The Benefits of Data Management: Unlocking the Power of Your Data
- What are the data management principles?
- Data Management: A Comprehensive Guide to Activities Involved
- The Ultimate Guide to Data Management
- What is Data Management and How to Manage it Effectively?
- Unlocking the Secrets of Big Data Analytics: A Comprehensive Guide
- The 10 Principles of Data Management for a Successful Organization
- Six Major Areas for Best Practices in Data Management
- What are the 6 types of data analysis?
- Data Management: A Comprehensive Guide to Unlocking Data Potential
- The Benefits of Enterprise Data Management for Every Enterprise
- What are the 5 basic steps in data analysis?
- A Comprehensive Guide to Writing a Data Management Research Plan
- The Benefits of Research Data Management
- 6 Major Areas for Best Practices in Data Management
- The Essential Role of Enterprise Data Management
- The Benefits of Data Management in Biology
- Data Management Best Practices: A Comprehensive Guide
- Types of Data Management Functions Explained
- A Comprehensive Guide to Database Management Systems
- What is Enterprise Data Management and How Can It Help Your Organization?
- What is data processing and its types?
- Data Management: A Comprehensive Guide for Researchers
- 4 Types of Data Management Explained
- Data Management: An Overview of the 5 Key Functions
- Data Management: A Comprehensive Guide to the Process
- What is a data principle?
- The Benefits of Good Data Management Explained
- How does data governance add value?
- The Benefits of Enterprise Data Management
- What is managing the data?
- Do You Need Math to Become a Data Scientist?
Data Management: Unlocking the Potential of Your Data
- Types of Management Tools: A Comprehensive Guide
- Best Practices for Managing Big Data Analytics Programs
- The Benefits of Data Management in Research: A Comprehensive Guide
- Is sql a database management system?
- Data Processing: Exploring the 3 Main Methods
- 10 Steps to Master Data Management
- Data Management in Research Studies: An Expert's Guide
New Articles
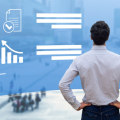
Which cookies do you want to accept?
Have a language expert improve your writing
Run a free plagiarism check in 10 minutes, automatically generate references for free.
- Knowledge Base
- Methodology
- Data Collection Methods | Step-by-Step Guide & Examples
Data Collection Methods | Step-by-Step Guide & Examples
Published on 4 May 2022 by Pritha Bhandari .
Data collection is a systematic process of gathering observations or measurements. Whether you are performing research for business, governmental, or academic purposes, data collection allows you to gain first-hand knowledge and original insights into your research problem .
While methods and aims may differ between fields, the overall process of data collection remains largely the same. Before you begin collecting data, you need to consider:
- The aim of the research
- The type of data that you will collect
- The methods and procedures you will use to collect, store, and process the data
To collect high-quality data that is relevant to your purposes, follow these four steps.
Table of contents
Step 1: define the aim of your research, step 2: choose your data collection method, step 3: plan your data collection procedures, step 4: collect the data, frequently asked questions about data collection.
Before you start the process of data collection, you need to identify exactly what you want to achieve. You can start by writing a problem statement : what is the practical or scientific issue that you want to address, and why does it matter?
Next, formulate one or more research questions that precisely define what you want to find out. Depending on your research questions, you might need to collect quantitative or qualitative data :
- Quantitative data is expressed in numbers and graphs and is analysed through statistical methods .
- Qualitative data is expressed in words and analysed through interpretations and categorisations.
If your aim is to test a hypothesis , measure something precisely, or gain large-scale statistical insights, collect quantitative data. If your aim is to explore ideas, understand experiences, or gain detailed insights into a specific context, collect qualitative data.
If you have several aims, you can use a mixed methods approach that collects both types of data.
- Your first aim is to assess whether there are significant differences in perceptions of managers across different departments and office locations.
- Your second aim is to gather meaningful feedback from employees to explore new ideas for how managers can improve.
Prevent plagiarism, run a free check.
Based on the data you want to collect, decide which method is best suited for your research.
- Experimental research is primarily a quantitative method.
- Interviews , focus groups , and ethnographies are qualitative methods.
- Surveys , observations, archival research, and secondary data collection can be quantitative or qualitative methods.
Carefully consider what method you will use to gather data that helps you directly answer your research questions.
When you know which method(s) you are using, you need to plan exactly how you will implement them. What procedures will you follow to make accurate observations or measurements of the variables you are interested in?
For instance, if you’re conducting surveys or interviews, decide what form the questions will take; if you’re conducting an experiment, make decisions about your experimental design .
Operationalisation
Sometimes your variables can be measured directly: for example, you can collect data on the average age of employees simply by asking for dates of birth. However, often you’ll be interested in collecting data on more abstract concepts or variables that can’t be directly observed.
Operationalisation means turning abstract conceptual ideas into measurable observations. When planning how you will collect data, you need to translate the conceptual definition of what you want to study into the operational definition of what you will actually measure.
- You ask managers to rate their own leadership skills on 5-point scales assessing the ability to delegate, decisiveness, and dependability.
- You ask their direct employees to provide anonymous feedback on the managers regarding the same topics.
You may need to develop a sampling plan to obtain data systematically. This involves defining a population , the group you want to draw conclusions about, and a sample, the group you will actually collect data from.
Your sampling method will determine how you recruit participants or obtain measurements for your study. To decide on a sampling method you will need to consider factors like the required sample size, accessibility of the sample, and time frame of the data collection.
Standardising procedures
If multiple researchers are involved, write a detailed manual to standardise data collection procedures in your study.
This means laying out specific step-by-step instructions so that everyone in your research team collects data in a consistent way – for example, by conducting experiments under the same conditions and using objective criteria to record and categorise observations.
This helps ensure the reliability of your data, and you can also use it to replicate the study in the future.
Creating a data management plan
Before beginning data collection, you should also decide how you will organise and store your data.
- If you are collecting data from people, you will likely need to anonymise and safeguard the data to prevent leaks of sensitive information (e.g. names or identity numbers).
- If you are collecting data via interviews or pencil-and-paper formats, you will need to perform transcriptions or data entry in systematic ways to minimise distortion.
- You can prevent loss of data by having an organisation system that is routinely backed up.
Finally, you can implement your chosen methods to measure or observe the variables you are interested in.
The closed-ended questions ask participants to rate their manager’s leadership skills on scales from 1 to 5. The data produced is numerical and can be statistically analysed for averages and patterns.
To ensure that high-quality data is recorded in a systematic way, here are some best practices:
- Record all relevant information as and when you obtain data. For example, note down whether or how lab equipment is recalibrated during an experimental study.
- Double-check manual data entry for errors.
- If you collect quantitative data, you can assess the reliability and validity to get an indication of your data quality.
Data collection is the systematic process by which observations or measurements are gathered in research. It is used in many different contexts by academics, governments, businesses, and other organisations.
When conducting research, collecting original data has significant advantages:
- You can tailor data collection to your specific research aims (e.g., understanding the needs of your consumers or user testing your website).
- You can control and standardise the process for high reliability and validity (e.g., choosing appropriate measurements and sampling methods ).
However, there are also some drawbacks: data collection can be time-consuming, labour-intensive, and expensive. In some cases, it’s more efficient to use secondary data that has already been collected by someone else, but the data might be less reliable.
Quantitative research deals with numbers and statistics, while qualitative research deals with words and meanings.
Quantitative methods allow you to test a hypothesis by systematically collecting and analysing data, while qualitative methods allow you to explore ideas and experiences in depth.
Reliability and validity are both about how well a method measures something:
- Reliability refers to the consistency of a measure (whether the results can be reproduced under the same conditions).
- Validity refers to the accuracy of a measure (whether the results really do represent what they are supposed to measure).
If you are doing experimental research , you also have to consider the internal and external validity of your experiment.
In mixed methods research , you use both qualitative and quantitative data collection and analysis methods to answer your research question .
Operationalisation means turning abstract conceptual ideas into measurable observations.
For example, the concept of social anxiety isn’t directly observable, but it can be operationally defined in terms of self-rating scores, behavioural avoidance of crowded places, or physical anxiety symptoms in social situations.
Before collecting data , it’s important to consider how you will operationalise the variables that you want to measure.
Cite this Scribbr article
If you want to cite this source, you can copy and paste the citation or click the ‘Cite this Scribbr article’ button to automatically add the citation to our free Reference Generator.
Bhandari, P. (2022, May 04). Data Collection Methods | Step-by-Step Guide & Examples. Scribbr. Retrieved 9 April 2024, from https://www.scribbr.co.uk/research-methods/data-collection-guide/
Is this article helpful?
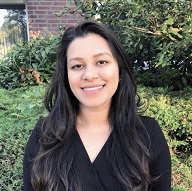
Pritha Bhandari
Other students also liked, qualitative vs quantitative research | examples & methods, triangulation in research | guide, types, examples, what is a conceptual framework | tips & examples.
- Topical Articles =>
- PMP Certification
- CAPM Certification
- Agile Training
- Corporate Training
- Project Management Tools
Home / Six Sigma / Data Collection Plan: Learn to Create It In 8 Steps
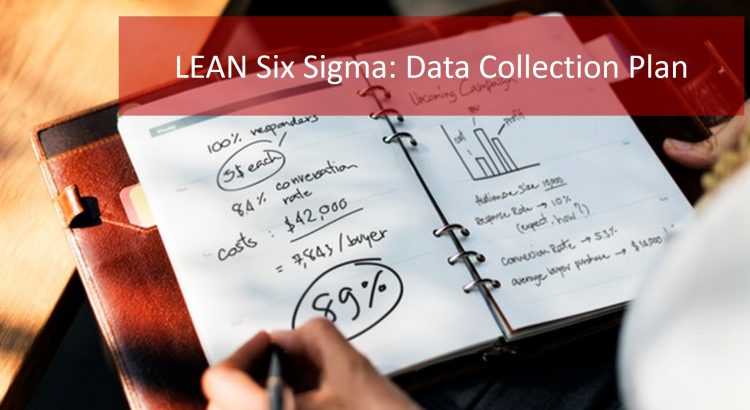
Data Collection Plan: Learn to Create It In 8 Steps
In the DMAIC framework of the Six Sigma Method , a Data Collection Plan is created during the Measure phase . A project manager who already has a Six Sigma Green Belt training will know that it is a useful tool to focus your efforts on. It is a detailed document that describes the exact steps as well as the sequence that needs to be followed in gathering the data for the given Six Sigma project.
Attend our 100% Online & Self-Paced Free Six Sigma Training .

Green Belt training is a good way to learn more about how a Data Collection Plan fits into the DMAIC process outside of what we will be discussing in this article.
- Power BI Course Beginner
- Power BI Course Intermediate
A Data Collection Plan ensures that everyone, working on a Lean Six Sigma project, is on the same page with regard to the data plan. It also ensures that this information is correctly channeled to the right stakeholders in the organization who are going to help us with our data needs. The purpose of the plan is to make sure that the data collected are meaningful and valid and that all relevant data are collected concurrently, the Xs and the Ys.
We need a Data Collection Plan here because we want to be efficient by not wasting resources on collecting data that are irrelevant to the project or not usable. By creating a Data Collection Plan, we can focus our efforts on answering specific questions that have business value. This directed approach with a Data Collection Plan helps us avoid locating and measuring data just for the sake of doing so.
How to Create a Data Collection Plan Six Sigma?
1) Identify the questions that you want to answer
The first step in creating a Data Collection Plan is to identify the questions we want to answer. Our data must be relevant to the project . The entire reason to have a DMAIC project is to improve a process. Hence, these questions should be centered on what the reality of our process is under the current state of affairs or status quo. The best practice is to use the SIPOC diagram as a guide for data collection. We also need to figure out the type of measurements or metrics we want to include.
Check our Six Sigma Training Video
2) Determine the kind of data that is available
The second step in creating a Data Collection Plan is to find out what kind of data is available to collect. What data exists that can give us all the required answers? Sometimes, a particular piece of data can give us multiple answers. Make sure that you make a list of all of the data points that are needed to answer the questions the project is centered on.
3) Determine how much data is needed
The third step in creating a Data Collection Plan is to decide how much data we need. We want to get enough data so that we can see patterns and trends . For each data element on the list, write down how much data is actually needed.
4) Determine how to measure the data
The fourth step in creating a data collection plan is to see how we are going to measure the data. As we all know, data can be measured in different ways : check sheets, survey answers, etc. The way we measure will be dependent upon the type of data we seek.
5) Decide who is going to gather data
The fifth step in creating a data collection plan is to decide who is going to collect the data. Nowadays, the data can also be collected through automated software . We may be required to liaise with the person in charge of the software to ensure the data is available and in the correct format.
6) Determine where the data will be collected from
The sixth step is to check where to collect the data from. It means deciding on the location and/or source of data. The location does not mean any physical location. It is the location within the process. The data collection plan must explicitly specify where in the process data must be collected from.
7) Decide whether to measure a sample or the whole population
The seventh step is to decide whether to sample the data or not . Sometimes it is impractical to measure an entire population of data. In such a case, we then take a sample of data. The question that the project team needs to look into could be: What should be our sampling method and sample size be to make statistically-sound judgments?
8) Determine in what format the data will be displayed
The eighth step is to decide the format of displaying the data. We can display data in many ways such as Pareto Diagrams, Scatter Diagram, etc.
A Sample Plan Template
The typical components of a Data Collection Plan are as follows:
The first thing that needs to be clarified before any effort is done is the purpose. The most common purposes include finding whether a process is stable? Whether a process is capable?
Data Collection Plan/Matrix Introduction
Have a look at the example of a Data Collection Plan or Matrix in the figure below. Let’s try to understand the different elements of the Data Collection Plan here. First, we start with capturing the process name. The name of the process owner is filled in accompanied by their contact information, location and area. The stakeholder who prepares the Data Collection Plan has to sign it off and then the first level, second level, and third level of authorized stakeholders are supposed to sign the off the Data Collection Plan. If the Data Collection Plan runs into multiple pages, you can add the page number. The document number and revision data would need to be mentioned for audit purposes.
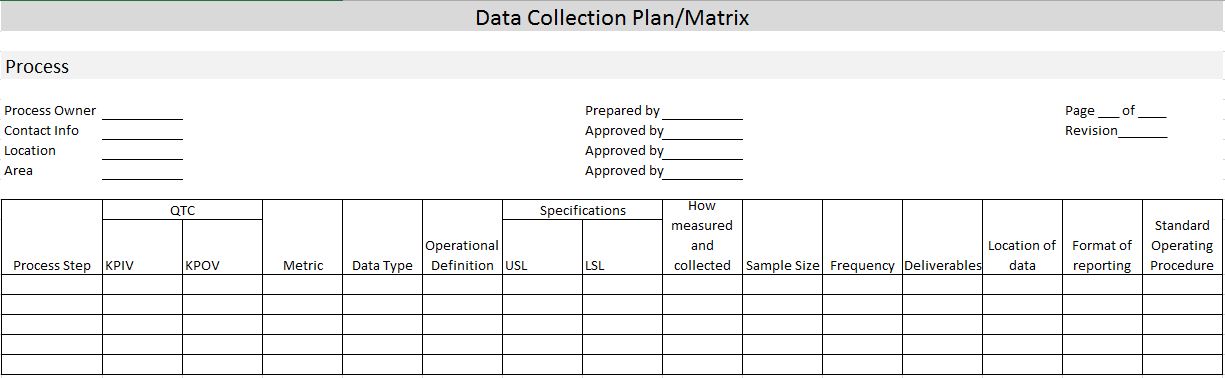
- Process Steps: We have now reached the main section of the Data Collection Plan. The first column of the data collection plan shall capture all process steps which we plan to collect the data for. Remember that we are currently progressing in the Six Sigma MEASURE phase. The objective of the Measure phase is to assess the status quo of a business problem and/or process improvement opportunity with the help of data collection and analysis. In Lean Six Sigma projects, the business problem and/or process improvement opportunity typically relates to the process under consideration. That is why the Data Collection Plan requires us to make a note of relevant process steps in the beginning.
- Critical to Qualities: The second column of the Data Collection Plan talks about two types of CTQ s (Critical to Quality) . The first one is Key Process Input Variables (KPIV) and the second one is Key Process Output Variables (KPOV). A KPIV is a process input that provides a significant impact on the output variation of a process or a system or on the Key Process Output Variable (KPOV) of a product. This means that the KPOV is determined by the KPIV. If the KPIV is held constant, then it would yield a predictable and consistent output. Let’s consider the simple process of preparing a cup of tea. For preparing a cup of tea, hot water, sugar, milk, etc. are the ingredients. The quality and quantity of each of these ingredients for a cup of tea will be the KPIV. The KPOV here would be the taste of tea and/or customer satisfaction after drinking that cup of tea. The CTQ column shall note KPIVs and KPOVs applicable to the respective process step.
- Metrics and data types: The third column is all for capturing all metrics ; applicable to the respective process step. The fourth column shall indicate the data type for the data points collected or to be collected in the context of the relevant process step only.
- Operational Definition: The fifth column records an Operational Definition for each measurement or metric. An Operational Definition is a document that details exactly how a specific metric will be measured.
- Specification limits: The sixth column helps us make a note of specification limits for each process step. The quantified specification limits, in terms of upper and lower specification limits (USL and LSL), are noted in the Data Collection Plan for all relevant process steps.
- The method of data collection: The seventh column records the method of collecting and measuring different pieces of data. We may also need to mention the Unit of Measurement (UOM) for measuring different data types only for relevant process steps.
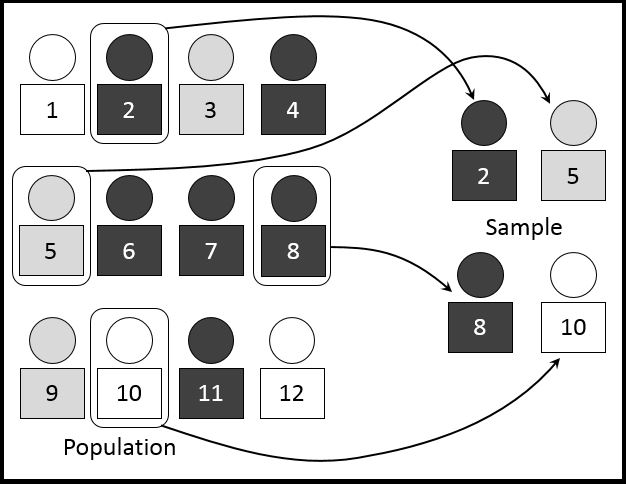
- Sample Size: The eighth column refers to the sample size for collecting different data points. As we all know, sample data represent population data. In order to make it happen in reality, we have to choose the right sampling method followed by the right sample size. If it is practically possible to go ahead with population data, then we must do so. If we plan to make use of only sample data, then we must determine both the sampling method and the sample size for collecting a variety of data as needed. In such a case, we might be required to sort and even prioritize our data collection requirements. The ninth column of the Data Collection Plan refers to the frequency of data collection for instance, daily, weekly, bi-monthly or monthly, etc.
- Process step deliverables: The tenth column has to mention the deliverables for each process step under consideration. It is also important to know who owns those deliverables.
- Source and location of data: The eleventh column of the Plan records the source for the availability of data accompanied by the location of data . The location of data does not refer to the physical location. It mainly refers to the location within the process . The Data Collection Plan must explicitly specify where in the process data must be collected from.
- Reporting format: The twelfth column of the Plan talks about the method or format of reporting to the internal and/or external stakeholders. The data collection plan shall explain the format in which the collected data needs to be displayed to internal and/or external stakeholders. Most probably a graphical method is used because it is intuitively easier to use.
- Standard Operating Procedure: The final column of the Plan shall incorporate Standard Operating Procedure (SOP) document for everybody’s reference
A solid Data Collection Plan will help Lean Six Sigma teams to collect data in the Measure phase of the DMAIC cycle with accuracy, precision, and transparency. With a Data Collection Plan, all the stakeholders will be informed and there will be an opportunity to question some of the Data Collection Plan ideas before the actual data collection begins. Creating a Data Collection Plan avoids teams just jumping in and collecting data at random. A Data Collection Plan is a structured way of stipulating exactly how the project’s data will be collected.
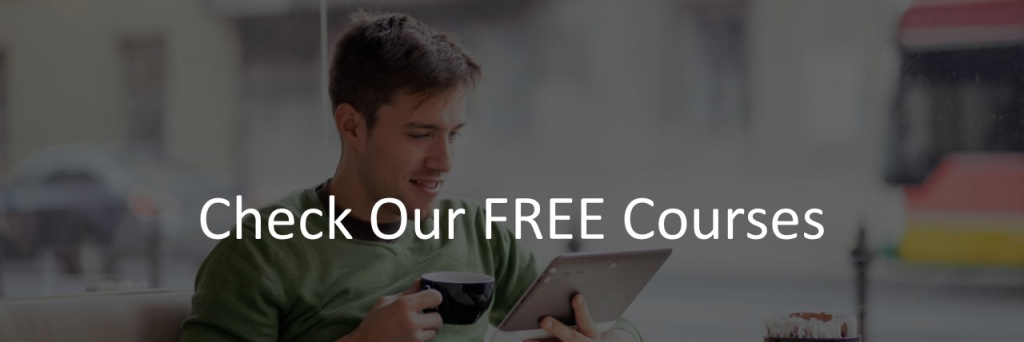
Related Posts
Disclaimer:
- PMP, PMI, PMBOK, CAPM, ACP and PDU are registered marks of the Project Management Institute.
- ITIL® is a [registered] trade mark of AXELOS Limited, used under permission of AXELOS Limited. All rights reserved.
- PRINCE2® is a [registered] trade mark of AXELOS Limited, used under permission of AXELOS Limited. All rights reserved.
- Certified ScrumMaster® (CSM) and Certified Scrum Trainer® (CST) are registered trademarks of SCRUM ALLIANCE®
- Professional Scrum Master is a registered trademark of Scrum.org
- CISA® is a Registered Trade Mark of the Information Systems Audit and Control Association (ISACA) and the IT Governance Institute.
- CISSP® is a registered mark of The International Information Systems Security Certification Consortium ((ISC)2).
Master of Project Promo Codes PMP Articles
PMP Certification Ultimate Guide – 99.6% Pass Rate CAPM Articles

Research Data Management
- Finding Existing Data
- Data Citation
- Research Proposal
- RDM Requirements
- Collaboration
- Data Security
- GDPR & Privacy
- Data Management Plan
- Policies & Regulations
- Data Collection
- Data Storage
- Data Protection
- Data Provenance
- Data Processing
- Data Analysis
- High Performance Computing
- Selecting Data
- Data Documentation
- Selecting an Archive
- Data Publication
- Dataset Registration
Research Proposal / Grant Office
Grant programmes from organisations like NWO, ZonMW and ERC increasingly require you to not only think about the journey of the data in your research project, but also the method of data collection and how to protect or share data during and after the research project. It is important to bear in mind the specific laws and regulations that apply to the kind of data that is collected. If a project involves data on persons and organisations this impacts the design of the necessary IT infrastructure. A more detailed description of this will later be captured in the data management plan.
When writing your research proposal the following items are important:
- Fill in the data management paragraph (see the four questions below)
- Planning: one of the early deliverables will be a detailed data management plan
- Budget: take into account the costs (labour and material) for data storage during and data archiving after your project.
- Writing: Funders that distribute grants like to maximise the effectiveness of this investment. It is therefore highly recommended that the data will be made Findable, Accessible, Interoperable and Re-usable (FAIRdata). This does not mean that the data have to be open: laws, licenses and contracts regarding personal and sensitive data may limit the possibility to share the data publicly.
Research Data Services provides advice and help when writing a data paragraph as part of the Research proposal. The Library also regularly organises workshops to help you get started. Together with the VU Grants Office and project control we are part of the grant support team offering advice and practical aid for your grant. You will be directed to the specific unit during your support trajectory. Make sure to contact the team as early as possible.
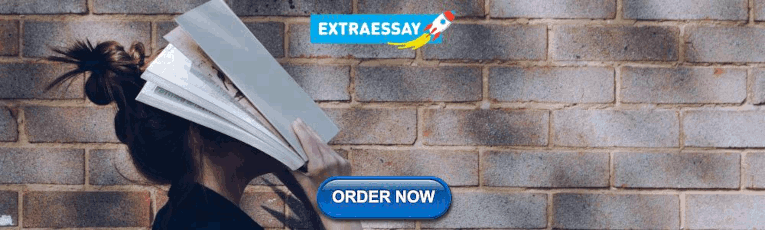
Data Management Paragraph
In order to make data re-usable, funders require researchers to include a data section (= paragraph) in their project proposal, in which they explain whether research data will be collected or generated during the project, and how they plan to structure, archive and share their data. Depending on requirements of the funder, the paragraph can be short or more extensive.
Funders may have different requirements for the Data Management Paragraph in the project proposal. Always check what your funder asks for. For example, in 2016, the NWO formulated four questions that need to be answered in the data paragraph of the research proposal:
- Will data be collected or generated that are suitable for re-use?
- Where will the data be stored during the research?
- After the project has been completed, how will the data be stored for the long term and made available for the use by third parties? To whom will the data be accessible?
- Which facilities (ICT, (secure) archive, refrigerators or legal expertise) do you expect will be needed for the storage of data during the research and after the research? Are these available?
- << Previous: Plan & Design
- Next: RDM Costs >>
- Last Updated: Feb 22, 2024 1:30 PM
- URL: https://libguides.vu.nl/rdm
- Privacy Policy
Buy Me a Coffee

Home » How To Write A Research Proposal – Step-by-Step [Template]
How To Write A Research Proposal – Step-by-Step [Template]
Table of Contents

How To Write a Research Proposal
Writing a Research proposal involves several steps to ensure a well-structured and comprehensive document. Here is an explanation of each step:
1. Title and Abstract
- Choose a concise and descriptive title that reflects the essence of your research.
- Write an abstract summarizing your research question, objectives, methodology, and expected outcomes. It should provide a brief overview of your proposal.
2. Introduction:
- Provide an introduction to your research topic, highlighting its significance and relevance.
- Clearly state the research problem or question you aim to address.
- Discuss the background and context of the study, including previous research in the field.
3. Research Objectives
- Outline the specific objectives or aims of your research. These objectives should be clear, achievable, and aligned with the research problem.
4. Literature Review:
- Conduct a comprehensive review of relevant literature and studies related to your research topic.
- Summarize key findings, identify gaps, and highlight how your research will contribute to the existing knowledge.
5. Methodology:
- Describe the research design and methodology you plan to employ to address your research objectives.
- Explain the data collection methods, instruments, and analysis techniques you will use.
- Justify why the chosen methods are appropriate and suitable for your research.
6. Timeline:
- Create a timeline or schedule that outlines the major milestones and activities of your research project.
- Break down the research process into smaller tasks and estimate the time required for each task.
7. Resources:
- Identify the resources needed for your research, such as access to specific databases, equipment, or funding.
- Explain how you will acquire or utilize these resources to carry out your research effectively.
8. Ethical Considerations:
- Discuss any ethical issues that may arise during your research and explain how you plan to address them.
- If your research involves human subjects, explain how you will ensure their informed consent and privacy.
9. Expected Outcomes and Significance:
- Clearly state the expected outcomes or results of your research.
- Highlight the potential impact and significance of your research in advancing knowledge or addressing practical issues.
10. References:
- Provide a list of all the references cited in your proposal, following a consistent citation style (e.g., APA, MLA).
11. Appendices:
- Include any additional supporting materials, such as survey questionnaires, interview guides, or data analysis plans.
Research Proposal Format
The format of a research proposal may vary depending on the specific requirements of the institution or funding agency. However, the following is a commonly used format for a research proposal:
1. Title Page:
- Include the title of your research proposal, your name, your affiliation or institution, and the date.
2. Abstract:
- Provide a brief summary of your research proposal, highlighting the research problem, objectives, methodology, and expected outcomes.
3. Introduction:
- Introduce the research topic and provide background information.
- State the research problem or question you aim to address.
- Explain the significance and relevance of the research.
- Review relevant literature and studies related to your research topic.
- Summarize key findings and identify gaps in the existing knowledge.
- Explain how your research will contribute to filling those gaps.
5. Research Objectives:
- Clearly state the specific objectives or aims of your research.
- Ensure that the objectives are clear, focused, and aligned with the research problem.
6. Methodology:
- Describe the research design and methodology you plan to use.
- Explain the data collection methods, instruments, and analysis techniques.
- Justify why the chosen methods are appropriate for your research.
7. Timeline:
8. Resources:
- Explain how you will acquire or utilize these resources effectively.
9. Ethical Considerations:
- If applicable, explain how you will ensure informed consent and protect the privacy of research participants.
10. Expected Outcomes and Significance:
11. References:
12. Appendices:
Research Proposal Template
Here’s a template for a research proposal:
1. Introduction:
2. Literature Review:
3. Research Objectives:
4. Methodology:
5. Timeline:
6. Resources:
7. Ethical Considerations:
8. Expected Outcomes and Significance:
9. References:
10. Appendices:
Research Proposal Sample
Title: The Impact of Online Education on Student Learning Outcomes: A Comparative Study
1. Introduction
Online education has gained significant prominence in recent years, especially due to the COVID-19 pandemic. This research proposal aims to investigate the impact of online education on student learning outcomes by comparing them with traditional face-to-face instruction. The study will explore various aspects of online education, such as instructional methods, student engagement, and academic performance, to provide insights into the effectiveness of online learning.
2. Objectives
The main objectives of this research are as follows:
- To compare student learning outcomes between online and traditional face-to-face education.
- To examine the factors influencing student engagement in online learning environments.
- To assess the effectiveness of different instructional methods employed in online education.
- To identify challenges and opportunities associated with online education and suggest recommendations for improvement.
3. Methodology
3.1 Study Design
This research will utilize a mixed-methods approach to gather both quantitative and qualitative data. The study will include the following components:
3.2 Participants
The research will involve undergraduate students from two universities, one offering online education and the other providing face-to-face instruction. A total of 500 students (250 from each university) will be selected randomly to participate in the study.
3.3 Data Collection
The research will employ the following data collection methods:
- Quantitative: Pre- and post-assessments will be conducted to measure students’ learning outcomes. Data on student demographics and academic performance will also be collected from university records.
- Qualitative: Focus group discussions and individual interviews will be conducted with students to gather their perceptions and experiences regarding online education.
3.4 Data Analysis
Quantitative data will be analyzed using statistical software, employing descriptive statistics, t-tests, and regression analysis. Qualitative data will be transcribed, coded, and analyzed thematically to identify recurring patterns and themes.
4. Ethical Considerations
The study will adhere to ethical guidelines, ensuring the privacy and confidentiality of participants. Informed consent will be obtained, and participants will have the right to withdraw from the study at any time.
5. Significance and Expected Outcomes
This research will contribute to the existing literature by providing empirical evidence on the impact of online education on student learning outcomes. The findings will help educational institutions and policymakers make informed decisions about incorporating online learning methods and improving the quality of online education. Moreover, the study will identify potential challenges and opportunities related to online education and offer recommendations for enhancing student engagement and overall learning outcomes.
6. Timeline
The proposed research will be conducted over a period of 12 months, including data collection, analysis, and report writing.
The estimated budget for this research includes expenses related to data collection, software licenses, participant compensation, and research assistance. A detailed budget breakdown will be provided in the final research plan.
8. Conclusion
This research proposal aims to investigate the impact of online education on student learning outcomes through a comparative study with traditional face-to-face instruction. By exploring various dimensions of online education, this research will provide valuable insights into the effectiveness and challenges associated with online learning. The findings will contribute to the ongoing discourse on educational practices and help shape future strategies for maximizing student learning outcomes in online education settings.
About the author
Muhammad Hassan
Researcher, Academic Writer, Web developer
You may also like

How To Write A Proposal – Step By Step Guide...

Grant Proposal – Example, Template and Guide

How To Write A Business Proposal – Step-by-Step...

Business Proposal – Templates, Examples and Guide

Proposal – Types, Examples, and Writing Guide

How to choose an Appropriate Method for Research?
- Data Collection Plan: Definition + Steps to Do It

- Data Collection
Introduction
A data collection plan is a way to get specific information on your audience. You can use it to better understand what they like and dislike, what motivates them, how they respond to different messages, and how they’re using the content you’re providing. A good data collection plan will also allow you to predict future patterns in your audience.
What is a Data Collection Plan?
A data collection plan is a document that outlines how you’ll collect data from your users and customers. It’s a step-by-step guide to collecting and storing information about your customers.
It’s also a way to ensure that all the steps in your process are entirely documented and organized correctly. Data collection plan is also a way to communicate with your team and stakeholders what information you’re trying to collect, why you need it, when you need it, how you’ll get it, and who will get it.
A data collection plan gives you an easy way to organize, categorize, and store information about your customers. This helps you keep track of what’s happening with them so that you can make informed decisions about improving their experience with your products or services.
Another purpose of the data collection plan is to help you understand how your product or service performs, and how to improve it in the future. It’s also helpful for ensuring that your team understands what you’re trying to achieve and how they can help.
Explore: 7 Data Collection Methods & Tools For Research
Why Do You Need a Data Collection Plan?
Data collection plans are a great way to ensure that your research is as accurate and thorough as possible.
A data collection plan is designed to help you collect data from your subjects and then report back their results. You can use it to determine how much data is needed, how to measure it, where the data will be collected from and delivered, who will gather it all, whether to measure a sample or the whole population, and how exactly the information will be presented.
Data collection plans can also help you decide whether you need an online survey or a paper questionnaire. A data collection plan can be used for things like:
- Making decisions about what metrics are important to monitor (e.g., number of purchases vs. number of users)
- Setting goals for collecting information about your client base or customer base (e.g., number of new customers each quarter)
- Collecting data can be time-consuming and costly. If you don’t have a data collection plan, you could miss out on valuable insights that could help your business grow.
- It’s difficult to make changes if you don’t have the right information about what works and what doesn’t work (and why). Having all this information in one place makes it easier for everyone on your team to stay on top of things—and makes it easier for you to make changes when needed.
How To Utilize a Data Collection Plan?
When it comes to running your business, it’s important to have a good understanding of the people you’re trying to reach. It’s also important to understand how they respond to different types of content and messaging.
The best way to do this is through data collection plans. You can use them for anything from improving your understanding of your audience to identifying areas for improvement or expansion and predicting future patterns.
Also, using a data collection plan helps you personalize the content that you’re creating for your audience, which helps keep them engaged and interested in your brand. This can help your business grow faster than ever before.
1. Improving Your Understanding of Your Audience : The first step to a successful data collection plan is to understand your audience . What are they looking for? How can you help them achieve their goals? What kind of content would resonate with them? By answering these questions, you’ll be able to tailor the content you share with your audience and create a plan that will help them reach their goals.
2. Identifying Areas for Improvement or Expansion: After understanding your audience, it’s time to identify areas for improvement or expansion. Are there areas where you’re lacking in knowledge about how people use your product? Or perhaps there’s a feature that could be improved upon and made more user-friendly? By identifying these areas, you’ll be able to think critically about what topics are relevant and where they’re lacking, which will help shape and expand your content strategy moving forward.
3. Predicting Future Patterns: Before you even start collecting data, you need to know what questions you’re going to ask. This is called a “data collection plan,” or DCP. The DCP should be clear and concise, taking into account all aspects of your business and explaining how the information you collect will be used. For example: if you want to know how many people click over from Facebook to your website every day at 4 pm, what questions should you include in your DCP? What time frame do they come from? Are they only coming from a specific country or region? Will they only come during lunch hours on Fridays or Saturdays? You can add as many details as necessary—just make sure they’re clear.
4. Better Personalization of Content and Messaging: A data collection plan can also be used for better personalization of content and messaging. For example, if you know that many people who visit your site are interested in buying products on Amazon, then you could include links in the footer of your site that take them directly there without having them scroll all over your page first (which might make them feel like they’re being sold something).
Steps To Create a Data Collection Plan
- Identify the questions that you want to answer. What are your goals for this project? Are there any specific pieces of information about your company or consumers’ behavior or needs that you’d like to know more about? What kind of data would help answer those questions? Do any other stakeholders have their own questions they’d like answered as well?
- Determine the kind of data that is available. Is any proprietary information being shared with third parties, such as vendors or contractors; if so, can you allow them access to some of this info without compromising your competitive advantage? If not, what kinds of open-source materials are available (like blog posts or social media posts) that might give you insight into your customers’ thoughts and feelings about your services and products?
- Determine the kind of data that can be gathered from those people interviewed and how much time it will take them to complete their interviews. If possible, try not to ask too many questions at once; instead, ask one at a time until everyone has had an opportunity to respond. Once all individuals have responded or if no one else responds within 24 hours after receiving an invitation for participation, move on to the next step.
- Determine how much data is needed; this may require additional questions before moving on to measure your data.
- Decide where the data will be collected from. Whether it’s an online survey, a phone call, or another way altogether.
- Decide whether to measure a sample or the whole population. Some studies only need one person’s opinion, while others might need 100 people’s opinions. And some studies only collect data on one type of person (like gender), while other studies might collect information on many different types of people at once (like age and income).
- Determine in what format the data will be displayed. Do you want it all in text form or do you want it all in charts?
A data collection plan is a detailed outline of the types of questions you want to answer, how much data you need, how you’re going to measure that data and make it available, who will gather that data, where that data will be collected from, and what format it will be displayed in.
It is a great way to ensure that everyone is on the same page when it comes to answering those questions. And this is why you should come up with a detailed plan to collect your data beforehand to avoid errors and to save time.
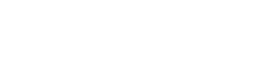
Connect to Formplus, Get Started Now - It's Free!
- data collection methods
- data collection plan
- research tools
- Olayemi Jemimah Aransiola
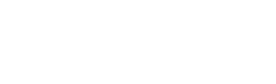
You may also like:
Surveys vs Censuses: Know The Differences
Introduction If you’re collecting data, you’ve probably heard the terms “survey” and “census.” But do you know how they differ? Surveys...

What is Field Research: Meaning, Examples, Pros & Cons
Introduction Field research is a method of research that deals with understanding and interpreting the social interactions of groups of...
What Are Research Repositories?
A research repository is a database that helps organizations to manage, share, and gain access to research data to make product and...
Unit of Analysis: Definition, Types & Examples
Introduction A unit of analysis is the smallest level of analysis for a research project. It’s important to choose the right unit of...
Formplus - For Seamless Data Collection
Collect data the right way with a versatile data collection tool. try formplus and transform your work productivity today..

An official website of the United States government
The .gov means it’s official. Federal government websites often end in .gov or .mil. Before sharing sensitive information, make sure you’re on a federal government site.
The site is secure. The https:// ensures that you are connecting to the official website and that any information you provide is encrypted and transmitted securely.
- Publications
- Account settings
Preview improvements coming to the PMC website in October 2024. Learn More or Try it out now .
- Advanced Search
- Journal List
- Indian J Anaesth
- v.60(9); 2016 Sep
How to write a research proposal?
Department of Anaesthesiology, Bangalore Medical College and Research Institute, Bengaluru, Karnataka, India
Devika Rani Duggappa
Writing the proposal of a research work in the present era is a challenging task due to the constantly evolving trends in the qualitative research design and the need to incorporate medical advances into the methodology. The proposal is a detailed plan or ‘blueprint’ for the intended study, and once it is completed, the research project should flow smoothly. Even today, many of the proposals at post-graduate evaluation committees and application proposals for funding are substandard. A search was conducted with keywords such as research proposal, writing proposal and qualitative using search engines, namely, PubMed and Google Scholar, and an attempt has been made to provide broad guidelines for writing a scientifically appropriate research proposal.
INTRODUCTION
A clean, well-thought-out proposal forms the backbone for the research itself and hence becomes the most important step in the process of conduct of research.[ 1 ] The objective of preparing a research proposal would be to obtain approvals from various committees including ethics committee [details under ‘Research methodology II’ section [ Table 1 ] in this issue of IJA) and to request for grants. However, there are very few universally accepted guidelines for preparation of a good quality research proposal. A search was performed with keywords such as research proposal, funding, qualitative and writing proposals using search engines, namely, PubMed, Google Scholar and Scopus.
Five ‘C’s while writing a literature review

BASIC REQUIREMENTS OF A RESEARCH PROPOSAL
A proposal needs to show how your work fits into what is already known about the topic and what new paradigm will it add to the literature, while specifying the question that the research will answer, establishing its significance, and the implications of the answer.[ 2 ] The proposal must be capable of convincing the evaluation committee about the credibility, achievability, practicality and reproducibility (repeatability) of the research design.[ 3 ] Four categories of audience with different expectations may be present in the evaluation committees, namely academic colleagues, policy-makers, practitioners and lay audiences who evaluate the research proposal. Tips for preparation of a good research proposal include; ‘be practical, be persuasive, make broader links, aim for crystal clarity and plan before you write’. A researcher must be balanced, with a realistic understanding of what can be achieved. Being persuasive implies that researcher must be able to convince other researchers, research funding agencies, educational institutions and supervisors that the research is worth getting approval. The aim of the researcher should be clearly stated in simple language that describes the research in a way that non-specialists can comprehend, without use of jargons. The proposal must not only demonstrate that it is based on an intelligent understanding of the existing literature but also show that the writer has thought about the time needed to conduct each stage of the research.[ 4 , 5 ]
CONTENTS OF A RESEARCH PROPOSAL
The contents or formats of a research proposal vary depending on the requirements of evaluation committee and are generally provided by the evaluation committee or the institution.
In general, a cover page should contain the (i) title of the proposal, (ii) name and affiliation of the researcher (principal investigator) and co-investigators, (iii) institutional affiliation (degree of the investigator and the name of institution where the study will be performed), details of contact such as phone numbers, E-mail id's and lines for signatures of investigators.
The main contents of the proposal may be presented under the following headings: (i) introduction, (ii) review of literature, (iii) aims and objectives, (iv) research design and methods, (v) ethical considerations, (vi) budget, (vii) appendices and (viii) citations.[ 4 ]
Introduction
It is also sometimes termed as ‘need for study’ or ‘abstract’. Introduction is an initial pitch of an idea; it sets the scene and puts the research in context.[ 6 ] The introduction should be designed to create interest in the reader about the topic and proposal. It should convey to the reader, what you want to do, what necessitates the study and your passion for the topic.[ 7 ] Some questions that can be used to assess the significance of the study are: (i) Who has an interest in the domain of inquiry? (ii) What do we already know about the topic? (iii) What has not been answered adequately in previous research and practice? (iv) How will this research add to knowledge, practice and policy in this area? Some of the evaluation committees, expect the last two questions, elaborated under a separate heading of ‘background and significance’.[ 8 ] Introduction should also contain the hypothesis behind the research design. If hypothesis cannot be constructed, the line of inquiry to be used in the research must be indicated.
Review of literature
It refers to all sources of scientific evidence pertaining to the topic in interest. In the present era of digitalisation and easy accessibility, there is an enormous amount of relevant data available, making it a challenge for the researcher to include all of it in his/her review.[ 9 ] It is crucial to structure this section intelligently so that the reader can grasp the argument related to your study in relation to that of other researchers, while still demonstrating to your readers that your work is original and innovative. It is preferable to summarise each article in a paragraph, highlighting the details pertinent to the topic of interest. The progression of review can move from the more general to the more focused studies, or a historical progression can be used to develop the story, without making it exhaustive.[ 1 ] Literature should include supporting data, disagreements and controversies. Five ‘C's may be kept in mind while writing a literature review[ 10 ] [ Table 1 ].
Aims and objectives
The research purpose (or goal or aim) gives a broad indication of what the researcher wishes to achieve in the research. The hypothesis to be tested can be the aim of the study. The objectives related to parameters or tools used to achieve the aim are generally categorised as primary and secondary objectives.
Research design and method
The objective here is to convince the reader that the overall research design and methods of analysis will correctly address the research problem and to impress upon the reader that the methodology/sources chosen are appropriate for the specific topic. It should be unmistakably tied to the specific aims of your study.
In this section, the methods and sources used to conduct the research must be discussed, including specific references to sites, databases, key texts or authors that will be indispensable to the project. There should be specific mention about the methodological approaches to be undertaken to gather information, about the techniques to be used to analyse it and about the tests of external validity to which researcher is committed.[ 10 , 11 ]
The components of this section include the following:[ 4 ]
Population and sample
Population refers to all the elements (individuals, objects or substances) that meet certain criteria for inclusion in a given universe,[ 12 ] and sample refers to subset of population which meets the inclusion criteria for enrolment into the study. The inclusion and exclusion criteria should be clearly defined. The details pertaining to sample size are discussed in the article “Sample size calculation: Basic priniciples” published in this issue of IJA.
Data collection
The researcher is expected to give a detailed account of the methodology adopted for collection of data, which include the time frame required for the research. The methodology should be tested for its validity and ensure that, in pursuit of achieving the results, the participant's life is not jeopardised. The author should anticipate and acknowledge any potential barrier and pitfall in carrying out the research design and explain plans to address them, thereby avoiding lacunae due to incomplete data collection. If the researcher is planning to acquire data through interviews or questionnaires, copy of the questions used for the same should be attached as an annexure with the proposal.
Rigor (soundness of the research)
This addresses the strength of the research with respect to its neutrality, consistency and applicability. Rigor must be reflected throughout the proposal.
It refers to the robustness of a research method against bias. The author should convey the measures taken to avoid bias, viz. blinding and randomisation, in an elaborate way, thus ensuring that the result obtained from the adopted method is purely as chance and not influenced by other confounding variables.
Consistency
Consistency considers whether the findings will be consistent if the inquiry was replicated with the same participants and in a similar context. This can be achieved by adopting standard and universally accepted methods and scales.
Applicability
Applicability refers to the degree to which the findings can be applied to different contexts and groups.[ 13 ]
Data analysis
This section deals with the reduction and reconstruction of data and its analysis including sample size calculation. The researcher is expected to explain the steps adopted for coding and sorting the data obtained. Various tests to be used to analyse the data for its robustness, significance should be clearly stated. Author should also mention the names of statistician and suitable software which will be used in due course of data analysis and their contribution to data analysis and sample calculation.[ 9 ]
Ethical considerations
Medical research introduces special moral and ethical problems that are not usually encountered by other researchers during data collection, and hence, the researcher should take special care in ensuring that ethical standards are met. Ethical considerations refer to the protection of the participants' rights (right to self-determination, right to privacy, right to autonomy and confidentiality, right to fair treatment and right to protection from discomfort and harm), obtaining informed consent and the institutional review process (ethical approval). The researcher needs to provide adequate information on each of these aspects.
Informed consent needs to be obtained from the participants (details discussed in further chapters), as well as the research site and the relevant authorities.
When the researcher prepares a research budget, he/she should predict and cost all aspects of the research and then add an additional allowance for unpredictable disasters, delays and rising costs. All items in the budget should be justified.
Appendices are documents that support the proposal and application. The appendices will be specific for each proposal but documents that are usually required include informed consent form, supporting documents, questionnaires, measurement tools and patient information of the study in layman's language.
As with any scholarly research paper, you must cite the sources you used in composing your proposal. Although the words ‘references and bibliography’ are different, they are used interchangeably. It refers to all references cited in the research proposal.
Successful, qualitative research proposals should communicate the researcher's knowledge of the field and method and convey the emergent nature of the qualitative design. The proposal should follow a discernible logic from the introduction to presentation of the appendices.
Financial support and sponsorship
Conflicts of interest.
There are no conflicts of interest.
An official website of the United States government
Here's how you know
Official websites use .gov A .gov website belongs to an official government organization in the United States.
Secure .gov websites use HTTPS. A lock ( Lock Locked padlock ) or https:// means you've safely connected to the .gov website. Share sensitive information only on official, secure websites.
Data Management Guidance for SBE Directorate Proposals and Awards
Proposals submitted to the U.S. National Science Foundation must include a supplementary document of no more than two pages labeled "Data Management Plan" (DMP). This supplementary document should describe how the proposal will conform to NSF policy on the dissemination and sharing of research results. Proposals that do not include a DMP will not be able to be submitted.
This page provides additional background and guidance on data management for proposals submitted to SBE programs. Some SBE programs have specific requirements in addition to the directorate's requirements. Please check the program's webpage, and direct questions to your program officer.
SBE data management guidance
Originally posted April, 2018
The Data Management Plan (DMP) should be short, no more than two pages, and submitted as a supplementary document. The plan will need to address:
- What data are generated by your research?
- What is your plan for managing the data during and after your project is completed, including are you making your data and/or metadata available to others and how, or why not if you are not making the data available?
This document is meant to provide guidance for investigators within the Social, Behavioral, and Economic (SBE) Sciences as they develop their Data Management Plans. A thoughtful and thorough consideration of data management issues for the SBE sciences is available in a workshop report, Public Access to NSF-Funded Research Data for the Social, Behavioral, and Economic Sciences . NSF’s comprehensive Public Access Plan, Today’s Data, Tomorrow’s Discoveries: Increasing Access to the Results of Research Funded by the National Science Foundation , was published in 2015.
“Data” are defined as the recorded factual material commonly accepted in the scientific community as necessary to validate research findings. This includes original data, but also “metadata” (e.g., experimental protocols, code written for statistical analyses, etc.).
It is acknowledged that there are many variables governing what constitutes “data,” and the management of data. Moreover, each area of science has its own culture regarding data. The data management plan will be evaluated as part of your proposal. Proposals must include sufficient information so that peer reviewers can assess both the data management plan and past performance. The plan should reflect best practices in your area of research and should be appropriate to the data you generate.
The Requirement: Include a Data Management Plan in Proposals
An appropriate data management plan is required as a supplementary document (maximum of two pages) for all full research proposals submitted. This plan is to be included in the Supplementary Documents section of the proposal and is not part of the 15-page limit for the Project Description. The NSF will not accept any proposal submitted that is lacking a DMP. Even if no data are to be produced, (e.g., the research is purely theoretical or is in support of a workshop where there will be no report), a DMP is required. In such a case, the DMP can simply state that no data will be produced.
The plan should describe how the PIs will manage and disseminate data generated by the project, and how will data and/or metadata be stored and made available to the public . The DMP will be considered by NSF and its reviewers during the proposal review process. Strategies and eventual compliance with the proposed DMP will be evaluated not only by proposal peer review, but also through project monitoring by NSF program officers, by Committees of Visitors, and by the National Science Board.
NSF is aware of the need to provide flexibility in assessment of data management plans. In developing a plan, researchers may want to consult with university officials as many universities have explicit data management policies. Some professional organizations also have recommended data management practices and refer PIs to repositories. NSF does not endorse the use of any specific repository.
Contents of the Data Management Plan
The DMP should clearly articulate how “sharing of primary data” is to be implemented. It should outline the rights and obligations of all parties as to their roles and responsibilities in the management and retention of research data. It should also consider changes to roles and responsibilities that will occur should a principal investigator or Co-PI leave the institution or project. Any costs should be explained in the Budget Justification pages. Specific components are listed below.
Expected data. The DMP should describe the types of data, samples, physical collections, software, curriculum materials, or other materials to be produced during the project. It should then describe the expected types of data to be retained.
The Federal government defines ‘data’ in OMB Circular A-110 (now 2 CFR, Ch. II, §215.36(d)(2)(i), and codified in 5 U.S.C. 552(a)(4)(A)) as:
Research data is defined as the recorded factual material commonly accepted in the scientific community as necessary to validate research findings, but not any of the following: Preliminary analyses, drafts of scientific papers, plans for future research, peer reviews, or communications with colleagues. This "recorded" material excludes physical objects (e.g., laboratory samples). Research data also do not include:
- (A) Trade secrets, commercial information, materials necessary to be held confidential by a researcher until they are published, or similar information which is protected under law; and
- (B) Personnel and medical information and similar information the disclosure of which would constitute a clearly unwarranted invasion of personal privacy, such as information that could be used to identify a particular person in a research study.
PIs should use the opportunity of the DMP to give thought to matters such as:
- The types of data that their project might generate and eventually share with others, and under what conditions
- How data are to be managed and maintained until they are shared with others
- Factors that might impinge on their ability to manage data, e.g. legal and ethical restrictions on access to non-aggregated data
- The lowest level of aggregated data that PIs might share
- The mechanism for sharing data and/or making them accessible to others
- Other types of information that should be maintained and shared regarding data, e.g. the way it was generated, analytical and procedural information, and the metadata
Period of data retention. SBE is committed to timely and rapid data distribution. However, it recognizes that types of data can vary widely and that acceptable norms also vary by scientific discipline. It is strongly committed, however, to the underlying principle of timely access, and applicants should address how this will be met in their DMP statement.
Data formats and dissemination. The DMP should describe data formats, media, and dissemination approaches that will be used to make data and metadata available to others. Policies for public access and sharing should be described, including provisions for appropriate protection of privacy, confidentiality, security, intellectual property, or other rights or requirements. Research centers and major partnerships with industry or other user communities must also address how data are to be shared and managed with partners, center members, and other major stakeholders.
Data storage and preservation of access. The DMP should describe physical and cyber resources and facilities that will be used for the effective preservation and storage of research data. These can include third party facilities and repositories.
Additional possible data management requirements. More stringent data management requirements may be specified in NSF solicitations or result from local policies and best practices at the PI’s home institution. Additional requirements will be specified in the program solicitation and award conditions. Principal Investigators to be supported by such programs must discuss how they will meet these additional requirements in their Data Management Plans.
Post-Award Monitoring
After an award is made, data management will be monitored primarily through the normal Annual and Final Report process and through evaluation of subsequent proposals.
Annual Reports. Annual reports, required for all multi-year NSF awards, must provide information on the progress on data management and sharing of the research products. This information could include citations of relevant publications, conference proceedings, and descriptions of other types of data sharing and dissemination of results.
Final Project Reports. Final Project Reports are required for all NSF awards. The Final Project Report must discuss execution and any updating of the original DMP. This discussion should describe:
- Data produced during the award;
- Data to be retained after the award expires;
- Verification that data will be available for sharing;
- Discussion of community standards for data format;
- How data will be disseminated;
- The format that will be used to make data available to others, including any metadata; and
- The archival location of data.
Subsequent proposals. Data management must be reported in subsequent proposals by the PI and Co-PIs under “Results of prior NSF support.”
- Latest Latest
- The West The West
- Sports Sports
- Opinion Opinion
- Magazine Magazine
New federal proposal aims to unveil Big Tech’s data collection practices
Sweeping plan would require new levels of transparency for tech company data collection and new rights for users.
By Art Raymond
Federal lawmakers unveiled a sweeping new plan Sunday aiming to institute new data privacy and collection rules that include requiring tech platforms to reveal their data collection and sharing practices, grant new data ownership and transport rights to individuals and require that privacy policy statements be shared in clear and easy-to-understand language.
The bipartisan “ American Privacy Rights Act ”, proposed by Sen. Maria Cantwell, D-Wash., and Rep. Cathy McMorris Rodgers, R-Wash., comes after years of wrangling and dozens of congressional hearings that have led to little substantive change in federal oversight of troves of individual user data collected, leveraged and distributed by online companies.
The authors say the draft legislation “sets clear, national data privacy rights and protections for Americans, eliminates the existing patchwork of state comprehensive data privacy laws and establishes robust enforcement mechanisms to hold violators accountable, including a private right of action for individuals.”
Provisions of the current proposal, which is being characterized as a “discussion draft” and subject to modifications, would limit the type of data companies can collect, store and use on individuals “beyond what is necessary, proportionate, and limited to provide or maintain” the collectors’ product or service offering. Users would also be granted new rights to request their data files from collecting entities, as well as information about where the data was sourced, how it was collected and where it has been shared. Stipulations also include the right for users to opt out of targeted advertising, delete their data and remove their information from the files of data brokers that function as user information resellers.
“This landmark legislation gives Americans the right to control where their information goes and who can sell it,” Rodgers said in a statement Sunday. “It reins in Big Tech by prohibiting them from tracking, predicting and manipulating people’s behaviors for profit without their knowledge and consent. Americans overwhelmingly want these rights, and they are looking to us, their elected representatives, to act.”
States look for solutions
In the void left by lack of Congressional action on digital data privacy issues, many state legislatures, including Utah’s, have taken action in an attempt to provide some data collection accountability and regulatory framework. Last month, Utah Gov. Spencer Cox signed three bills passed in the 2024 session that include data privacy provisions in the areas of social media use by minors, motor vehicle data collection and third-party access to individual health information.
But do state-level rules on internet-based companies, whose operations span state and national boundaries, represent the best level of government to be engaging in oversight of technology platforms?
In statewide polling conducted last year by the Deseret News in partnership with the University of Utah’s Hinckley Institute of Politics, Utahns answered the question with a resounding “no”.
When it comes to what level of government should determine how technology companies are regulated, the poll found 61% of those surveyed believe it is a federal government responsibility, 18% said regulation should fall to state government and 11% said that government should not play a role in regulating technology companies.
A citizen call for regulation
That said, a plurality of Utah voters who participated in the polling do believe that more regulation is in order when it comes to Big Tech operations.
When asked, “Do you think the government should increase, decrease or not change its regulation of technology companies such as Amazon, Facebook and Google?”, 47% of poll participants said regulation should be increased, 12% believe it should be decreased, 28% said the current level of regulation should not change and 13% of respondents didn’t know.
In a shared statement, Cantwell and Rodgers said they believe their proposal strikes the right balance to earn Congressional approval of a first-ever comprehensive national data rights and privacy standard.
“This bipartisan, bicameral draft legislation is the best opportunity we’ve had in decades to establish a national data privacy and security standard that gives people the right to control their personal information,” Cantwell and Rodgers said. “This landmark legislation represents the sum of years of good faith efforts in both the House and Senate. It strikes a meaningful balance on issues that are critical to moving comprehensive data privacy legislation through Congress.”
Content Search
Request for proposals for data collection firms.
- Methodica Consulting
Methodica Consulting ( https://www.methodicaconsulting.com/ ) is a consulting firm specialised in measuring social change in complex settings. We build tailored M&E and research methodologies to support public and private entities in delivering social change in remote locations, fragile states, and developing countries.
Our complementary expertise in qualitative and quantitative methods has been successfully employed in various assignments: from OECD-DAC-based evaluations and verification exercises to more advanced outcome-focused monitoring systems and research; from descriptive and inferential statistics to country-level econometric assessments.
Our team and network include experienced professionals with a wide range of advisory and on-field experience, as well as relationships with trusted data collection partners across our countries of operation. Methodica Consulting is led by Monica Gazzola, with 12+ years of experience in large-scale, multi-country M&E projects primarily in sub-Saharan Africa, supporting major donors (e.g., UN, EU, UK, US) in a wide range of thematic areas, including stabilisation, peacekeeping, governance, countering violent extremism (CVE), migration, women empowerment and military operations.
Request for Proposals
Methodica Consulting is currently looking to expand its network of local partners in West, East and North Africa to participate in new business proposals. Methodica Consulting is seeking applications for qualified organizations to support data collection (Quantitative and Qualitative), translation and analysis activities. Eligible organizations must be locally registered and based in one or more of any West, East, North African countries.
Scope of potential work:
Typical activities that Methodica would like the local partner to undertake include the below:
- Support the design and translation of qualitative and/or quantitative research tools in West, East and North Africa
- Lead the collection of qualitative and quantitative data through multiple tools provided by Methodica (e.g., surveys, key informant interviews (KII), focus group discussions (FGD), Observations, Checklists, etc.) in insecure or hard-to reach areas
- Liaise, on behalf of Methodica, with local stakeholders (e.g., ministers, local authorities, traditional leaders, vulnerable groups, security personnel, etc.)
- Identify and implement methods that reflect contextual considerations (e.g., remote monitoring methods such as phone/video meetings, mobile phone surveys, virtual site visits, GIS/remote sensing monitoring, etc.)
Application Guidelines:
Proposals are expected to contain the following sections:
- Company Presentation including: Organization Name, Physical location of organization’s main office, Website; Name, Title, number, and email address of the Applicant’s primary contact regarding this proposal
- M&E and Research Experience detailing example of relevant previous work, including previous clients/ donors
- Geographical Reach detailing capabilities in key geographies, especially in remote areas
- Data Collection Capabilities, e.g.quantitative, qualitative, surveys, KIIs, FGDs, site visits
- Language Capabilities
- Overview of Software Capabilities ,especially data collection ones
- Overview of Quality Assurance and Control protocols
- Quotes and Cost Estimations (please provide a quote range for deliverable (e.g., KIIs, FGD, Survey etc) by country and location)
- References (please provide three organizational references who can attest to the quality and timeliness of tasks related to the above scope of work)
How to apply
The issuance of this RFP does not constitute an award commitment on the part of Methodica Consulting, nor does it commit Methodica Consulting to pay for costs incurred in the preparation and submission of an application.
Applicants should submit a complete application no later than April 30th, 2024 at 12:00pm CET. Proposals should be written in English or French and follow instructions provided and contain only requested information.
Questions and Applications should be submitted by email to [email protected]. No questions will be entertained if received by means other than the specified email address.
Latest Updates
Côte d'Ivoire + 1 more
UNICEF Côte d'Ivoire Humanitarian Situation Report No 1 - 01 January - 31 March 2024
Colombia: briefing regional - región del magdalena medio, julio a diciembre de 2023.
PNG + 1 more
Emergency Assistance to the Independent State of Papua New Guinea in Response to the Damage caused by the Flood and Landslide
oPt + 1 more
Under the directives of the UAE President, the UAE supports the “Amalthea Fund” for humanitarian response in Gaza with USD 15 million
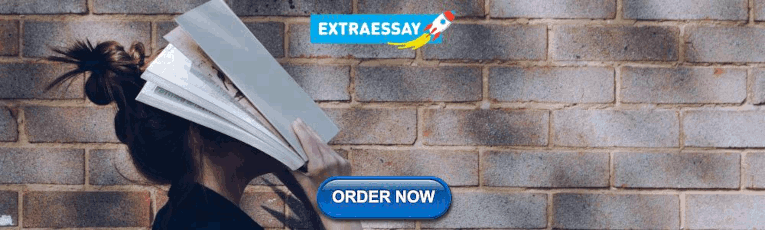
IMAGES
VIDEO
COMMENTS
Data Collection | Definition, Methods & Examples. Published on June 5, 2020 by Pritha Bhandari.Revised on June 21, 2023. Data collection is a systematic process of gathering observations or measurements. Whether you are performing research for business, governmental or academic purposes, data collection allows you to gain first-hand knowledge and original insights into your research problem.
Steps for writing an effective data collection plan. With the theory out of the way, let's see how to write a proper data collection plan, step by step. 1. Define objectives and research questions. Write down a statement of purpose that explains what you intend to discover, decide, or achieve.
The plan for gathering data involves 8 steps: Identify the questions. The first step in making a data collection plan is to decide what questions we want to answer. Our information has to be useful for the project. These questions should be based on what our process is really like in its current state.
PDF | Learn how to choose the best data collection methods and tools for your research project, with examples and tips from ResearchGate experts. | Download and read the full-text PDF.
A clear data collection plan at the proposal stage can alleviate stress and ensure that future researchers can replicate your study. Additionally, a clear data collection plan will help ensure that you obtain the information you need to answer your research questions. Below are some suggestions for creating a solid data collection plan.
Now you need to plan your data collection procedures, especially if you're doing quantitative research! This video will walk you through some key steps for g...
1 and if collecting new data, how it will be collected and by whom. If needed, create a Data Collection Form to assist in the collection of data. 4. Prepare data collection plan: Document the plan for collecting the data identified using a Data Collection Plan. Identify the following information for each metric: name, operational definition ...
6.1.1 Preparation for a Data Collection. A first step in any research project is the research proposal (Sudheesh et al., 2016 ). The research proposal should set out the background to the work, and the reason of the work is necessary. It should set out a hypothesis or a research question.
Clinical Research Center Boston Children's Hospita l. analysis plan: bird's-eye view A good analysis plan is the ultimate demonstration that your whole proposal is well formulated. Specific Aims Hypotheses Study Design Data Collection Analysis Plan Power. what the analysis plan shows
INTRODUCTION. Statistics represent an essential part of a study because, regardless of the study design, investigators need to summarize the collected information for interpretation and presentation to others. It is therefore important for us to heed Mr Twain's concern when creating the data analysis plan. In fact, even before data collection ...
A Data Analysis Plan (DAP) is about putting thoughts into a plan of action. Research questions are often framed broadly and need to be clarified and funnelled down into testable hypotheses and action steps. The DAP provides an opportunity for input from collaborators and provides a platform for training. Having a clear plan of action is also ...
These plans provide an outline of the types of data that will be collected, the data format, including data and metadata standards, and how the data will be handled throughout the project lifecycle. Many research funders require a data management plan with a grant proposal, so it is important for researchers to understand the main areas to ...
Step 2: Choose your data collection method. Based on the data you want to collect, decide which method is best suited for your research. Experimental research is primarily a quantitative method. Interviews, focus groups, and ethnographies are qualitative methods. Surveys, observations, archival research, and secondary data collection can be ...
In this Rip Out we focus on data collection, but in qualitative research, the entire project must be considered. 1, 2 Careful design of the data collection phase requires the following: deciding who will do what, where, when, and how at the different stages of the research process; acknowledging the role of the researcher as an instrument of ...
3) Determine how much data is needed. The third step in creating a Data Collection Plan is to decide how much data we need. We want to get enough data so that we can see patterns and trends. For each data element on the list, write down how much data is actually needed. 4) Determine how to measure the data.
When writing your research proposal the following items are important: Fill in the data management paragraph (see the four questions below) Planning: one of the early deliverables will be a detailed data management plan. Budget: take into account the costs (labour and material) for data storage during and data archiving after your project.
Data collection is the process of gathering and collecting information from various sources to analyze and make informed decisions based on the data collected. This can involve various methods, such as surveys, interviews, experiments, and observation. In order for data collection to be effective, it is important to have a clear understanding ...
3.3 Data Collection. The research will employ the following data collection methods: ... A detailed budget breakdown will be provided in the final research plan. 8. Conclusion. This research proposal aims to investigate the impact of online education on student learning outcomes through a comparative study with traditional face-to-face ...
Part I was published in issue 27(1) of the Western Journal of Nursing Research. The comments provided by the National Institutes of Health reviewers follow the application. Readers may refer to the introductory comments published with Part I of the proposal for further information about this document.
The investigator specifies the maximum discrepancy between the sample and population proportion of ± 5%. To determine the sample size, the investigator would use the formula. n = (z/p)2π(1-π), n = the required sample size. p = the desired maximum discrepancy (i.e. ± 5%) π = the population proportion.
A data collection plan is a document that outlines how you'll collect data from your users and customers. It's a step-by-step guide to collecting and storing information about your customers. It's also a way to ensure that all the steps in your process are entirely documented and organized correctly. Data collection plan is also a way to ...
Writing the proposal of a research work in the present era is a challenging task due to the constantly evolving trends in the qualitative research design and the need to incorporate medical advances into the methodology. The proposal is a detailed plan or 'blueprint' for the intended study, and once it is completed, the research project ...
The Requirement: Include a Data Management Plan in Proposals. An appropriate data management plan is required as a supplementary document (maximum of two pages) for all full research proposals submitted. This plan is to be included in the Supplementary Documents section of the proposal and is not part of the 15-page limit for the Project ...
New federal proposal aims to unveil Big Tech's data collection practices Sweeping plan would require new levels of transparency for tech company data collection and new rights for users. Published: April 8, 2024, ... Provisions of the current proposal, which is being characterized as a "discussion draft" and subject to modifications ...
Lead the collection of qualitative and quantitative data through multiple tools provided by Methodica (e.g., surveys, key informant interviews (KII), focus group discussions (FGD), Observations ...