The PRISMA 2020 statement: An updated guideline for reporting systematic reviews
Affiliations.
- 1 School of Public Health and Preventive Medicine, Monash University, Melbourne, Australia.
- 2 Department of Clinical Epidemiology, Biostatistics and Bioinformatics, Amsterdam University Medical Centres, University of Amsterdam, Amsterdam, Netherlands.
- 3 Université de Paris, Centre of Epidemiology and Statistics (CRESS), Inserm, Paris, France.
- 4 Institute for Evidence-Based Healthcare, Faculty of Health Sciences and Medicine, Bond University, Gold Coast, Australia.
- 5 University of Texas Health Science Center at San Antonio, San Antonio, Texas, United States of America; Annals of Internal Medicine.
- 6 Knowledge Translation Program, Li Ka Shing Knowledge Institute, Toronto, Canada; School of Epidemiology and Public Health, Faculty of Medicine, University of Ottawa, Ottawa, Canada.
- 7 Evidence Partners, Ottawa, Canada.
- 8 Clinical Research Institute, American University of Beirut, Beirut, Lebanon; Department of Health Research Methods, Evidence, and Impact, McMaster University, Hamilton, Ontario, Canada.
- 9 Department of Medical Informatics and Clinical Epidemiology, Oregon Health & Science University, Portland, Oregon, United States of America.
- 10 York Health Economics Consortium (YHEC Ltd), University of York, York, United Kingdom.
- 11 Clinical Epidemiology Program, Ottawa Hospital Research Institute, Ottawa, Canada; School of Epidemiology and Public Health, University of Ottawa, Ottawa, Canada; Department of Medicine, University of Ottawa, Ottawa, Canada.
- 12 Centre for Evidence-Based Medicine Odense (CEBMO) and Cochrane Denmark, Department of Clinical Research, University of Southern Denmark, Odense, Denmark; Open Patient data Exploratory Network (OPEN), Odense University Hospital, Odense, Denmark.
- 13 Department of Anesthesiology and Pain Medicine, The Ottawa Hospital, Ottawa, Canada; Clinical Epidemiology Program, Blueprint Translational Research Group, Ottawa Hospital Research Institute, Ottawa, Canada; Regenerative Medicine Program, Ottawa Hospital Research Institute, Ottawa, Canada.
- 14 Department of Ophthalmology, School of Medicine, University of Colorado Denver, Denver, Colorado, United States; Department of Epidemiology, Johns Hopkins Bloomberg School of Public Health, Baltimore, Maryland, United States of America.
- 15 Division of Headache, Department of Neurology, Brigham and Women's Hospital, Harvard Medical School, Boston, Massachusetts, USA; Head of Research, The BMJ, London, United Kingdom.
- 16 Department of Epidemiology and Biostatistics, Indiana University School of Public Health-Bloomington, Bloomington, Indiana, United States of America.
- 17 Population Health Sciences, Bristol Medical School, University of Bristol, Bristol, United Kingdom.
- 18 Centre for Reviews and Dissemination, University of York, York, United Kingdom.
- 19 EPPI-Centre, UCL Social Research Institute, University College London, London, United Kingdom.
- 20 Li Ka Shing Knowledge Institute of St. Michael's Hospital, Unity Health Toronto, Toronto, Canada; Epidemiology Division of the Dalla Lana School of Public Health and the Institute of Health Management, Policy, and Evaluation, University of Toronto, Toronto, Canada; Queen's Collaboration for Health Care Quality Joanna Briggs Institute Centre of Excellence, Queen's University, Kingston, Canada.
- 21 Methods Centre, Bruyère Research Institute, Ottawa, Ontario, Canada; School of Epidemiology and Public Health, Faculty of Medicine, University of Ottawa, Ottawa, Canada.
- 22 Centre for Journalology, Clinical Epidemiology Program, Ottawa Hospital Research Institute, Ottawa, Canada; School of Epidemiology and Public Health, Faculty of Medicine, University of Ottawa, Ottawa, Canada.
- PMID: 33780438
- PMCID: PMC8007028
- DOI: 10.1371/journal.pmed.1003583
Matthew Page and co-authors describe PRISMA 2020, an updated reporting guideline for systematic reviews and meta-analyses.
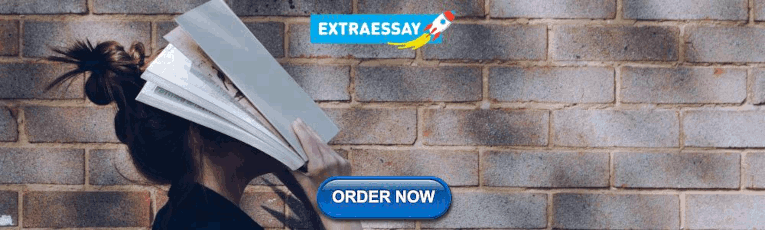
Publication types
- Research Support, N.I.H., Extramural
- Research Support, Non-U.S. Gov't
- Evidence-Based Medicine / standards*
- Publishing / standards*
- Systematic Reviews as Topic* / methods
- Systematic Reviews as Topic* / standards
Grants and funding
- UG1 EY020522/EY/NEI NIH HHS/United States
- DRF-2018-11-ST2-048/DH_/Department of Health/United Kingdom
- Open access
- Published: 26 January 2021
PRISMA-S: an extension to the PRISMA Statement for Reporting Literature Searches in Systematic Reviews
- Melissa L. Rethlefsen ORCID: orcid.org/0000-0001-5322-9368 1 ,
- Shona Kirtley ORCID: orcid.org/0000-0002-7801-5777 2 ,
- Siw Waffenschmidt ORCID: orcid.org/0000-0001-6860-6699 3 ,
- Ana Patricia Ayala ORCID: orcid.org/0000-0002-3613-2270 4 ,
- David Moher ORCID: orcid.org/0000-0003-2434-4206 5 ,
- Matthew J. Page ORCID: orcid.org/0000-0002-4242-7526 6 ,
- Jonathan B. Koffel ORCID: orcid.org/0000-0003-1723-5087 7 &
PRISMA-S Group
Systematic Reviews volume 10 , Article number: 39 ( 2021 ) Cite this article
147k Accesses
937 Citations
356 Altmetric
Metrics details
Literature searches underlie the foundations of systematic reviews and related review types. Yet, the literature searching component of systematic reviews and related review types is often poorly reported. Guidance for literature search reporting has been diverse, and, in many cases, does not offer enough detail to authors who need more specific information about reporting search methods and information sources in a clear, reproducible way. This document presents the PRISMA-S (Preferred Reporting Items for Systematic reviews and Meta-Analyses literature search extension) checklist, and explanation and elaboration.
The checklist was developed using a 3-stage Delphi survey process, followed by a consensus conference and public review process.
The final checklist includes 16 reporting items, each of which is detailed with exemplar reporting and rationale.
Conclusions
The intent of PRISMA-S is to complement the PRISMA Statement and its extensions by providing a checklist that could be used by interdisciplinary authors, editors, and peer reviewers to verify that each component of a search is completely reported and therefore reproducible.
Peer Review reports
Introduction
One crucial component of a systematic review is the literature search. The literature search, or information retrieval process, not only informs the results of a systematic review; it is the underlying process that establishes the data available for analysis. Additional components of the systematic review process such as screening, data extraction, and qualitative or quantitative synthesis procedures are dependent on the identification of eligible studies. As such, the literature search must be designed to be both robust and reproducible to ensure the minimization of bias.
Guidelines exist for both the conduct of literature searches (Table 2 ) for systematic reviews and their reporting [ 2 , 3 , 4 , 5 , 6 , 7 ]. Problematically, however, the many guidelines for reporting systematic review searches share few common reporting elements. In fact, Sampson et al. discovered that of the eleven instruments designed to help authors report literature searches well, only one item appeared in all eleven instruments [ 8 ]. Though Sampson et al.’s study was conducted in 2007, the problem has only been compounded as new checklists and tools have continued to be developed. The most commonly used reporting guidance for systematic reviews, which covers the literature search component, is the Preferred Reporting Items for Systematic reviews and Meta-Analyses Statement, or PRISMA Statement [ 9 ]. The 2009 PRISMA Statement checklist included three items related to literature search reporting, items 7, 8, and 17:
Item 7: Describe all information sources (e.g., databases with dates of coverage, contact with study authors to identify additional studies) in the search and date last searched.
Item 8: Present full electronic search strategy for at least one database, including any limits used, such that it could be repeated.
Item 17: Give numbers of studies screened, assessed for eligibility, and included in the review, with reasons for exclusions at each stage, ideally with a flow diagram.
Despite wide usage of the PRISMA Statement [ 10 ], compliance with its items regarding literature search reporting is low [ 11 , 12 , 13 , 14 ]. Even for those studies which explicitly reference PRISMA, there is only slight, statistically non-significant evidence of improved reporting, as found by Page et al. [ 15 ]. Part of the challenge may be the multifactorial nature of each of the PRISMA items relating to searches; authors may feel if they completed one of the components of the item that they can check off that item altogether. Another part of the challenge may be that many systematic reviews do not include librarians or information specialists as members of the systematic review team or as authors on the final manuscript [ 11 , 16 , 17 , 18 ]. Preliminary research suggests that librarian or information specialist involvement is correlated with reproducibility of searches [ 16 , 17 , 18 ], likely due to their expertise surrounding search development and documentation. However, reviews where librarians are authors still include reproducible searches only 64% of the time [ 17 ].
A larger issue may be that, even amongst librarians and information specialists, debate exists as to what constitutes a reproducible search and how best to report the details of the search. Researchers assessing the reproducibility of the search have used varying methods to determine what constitutes a reproducible search [ 11 , 17 , 19 , 20 ]. Post-publication peer review of search methods, even amongst Cochrane reviews, which generally have superior reporting compared to non-Cochrane reviews [ 15 ], has shown that reporting that appears complete may still pose challenges for those wishing to reproduce searches [ 20 , 21 , 22 , 23 , 24 ]. Furthermore, little guidance on how to report searches using information sources or methods other than literature databases, such as searching web sites or study registries, exists [ 25 , 26 ].
Incomplete reporting of the literature search methods can introduce doubt and diminish trust in the final systematic review conclusions. If researchers are unable to understand or reproduce how information was gathered for a systematic review, they may suspect the authors of having introduced bias into their review by not conducting a thorough or pre-specified literature search. After observing the high number of systematic reviews with poorly reported literature searches, we sought to create an extension to the PRISMA statement. Our aims were four-fold:
To provide extensive guidance on reporting the literature search components of a systematic review.
To create a checklist that could be used by authors, editors, and peer reviewers to verify that each component of a search was completely reported and therefore reproducible.
To develop an interdisciplinary checklist applicable to all method-driven literature searches for evidence synthesis.
To complement the PRISMA Statement and its extensions.
Because we intend the checklist to be used in all fields and disciplines, we use “systematic reviews” throughout this document as a representative name for the entire family of evidence syntheses [ 27 ]. This includes, but is not limited to, scoping reviews, rapid reviews, realist reviews, metanarrative reviews, mixed methods reviews, umbrella reviews, and evidence maps [ 28 ]. We use the term “literature search” or “search” throughout to encompass the full range of possible search methods and information sources.
Part 1: Developing the Checklist
After consultation with members of the PRISMA Statement steering group (D.M. and D.G.A.), we formed an executive committee (M.L.R, J.K., S.K.) and developed a protocol [ 29 ] according to the steps outlined in the “Guidance for Developers of Health Research Reporting Guidelines [ 30 ].” The protocol was registered on the EQUATOR Network [ 29 ]. We identified 405 potential items relevant to reporting searches in systematic reviews from 61 sources (see Additional file 1 ) located through a search of MEDLINE via Ovid, Embase via Embase.com , and LISTA via EBSCOhost, in addition to reviewing all of the sources identified by the EQUATOR Network relating to systematic reviews. We also searched our personal files and examined references of included documents for additional sources. Details of the search are available in Additional file 1 . Sources included both explicit reporting guidelines and studies assessing reproducibility of search strategies. The 405 items were reviewed for overlap and consolidated into 123 remaining items for potential inclusion in a checklist.
To narrow the list into a usable checklist, we then used a three-step Delphi survey process [ 31 ]. The first survey included the initially identified 123 items and asked respondents to rate each item on a 4-point Likert-type scale. Items that 70% of experts rated as 3 or 4 (4 being “essential” and 1 “not important”) and that received a mean score of at least 3.25 were retained for rating in the second round of the Delphi process. Respondents to the first survey were invited to participate in the second and third rounds. The second round asked respondents to pick the 25 most essential items out of the remaining 53 potential items; the third round was identical, except respondents also selected the most appropriate location for reporting their selected items (e.g., in the main text, or a supplementary file). The items were ranked and categorized by general theme for discussion at an in-person consensus conference.
We created a list of one hundred and sixty-three international experts, including librarian and information specialists with expertise in systematic reviews, researchers who had written about systematic review reporting, journal editors, and systematic review methodologists, to whom we sent our initial Delphi survey. The list of experts was created using a combination of publications, mailing lists, conference proceedings, and knowledge of the authors to represent research groups and experts in 23 countries. We received 52 responses (32% response rate) to the first survey, and of these, 35 (67% response rate) completed both surveys two and three. This study was declared exempt by the University of Utah Institutional Review Board (IRB_00088425).
The results of the Delphi process were reported at a consensus conference meeting that took place in May 2016 concurrently with Mosaic ‘16, the joint meeting of the Medical Library Association, Canadian Health Libraries Association/Association des bibliothèques de la santé du Canada, and the International Clinical Librarian Conference (ICLC). 38 individuals attended the consensus conference, 14 (37%) of whom had participated in the Delphi surveys. At the consensus conference, the grouped and ranked remaining items were distributed to small groups who were asked to discuss, consolidate, remove, or add missing critical items under the guidance of a group leader. After two rounds of discussion, the group leaders presented the discussion and proposed list items from their small groups for consideration by the whole group of experts.
Upon completion of the consensus conference, 30 items remained from those identified during the Delphi process, with an additional three items that had been excluded during the Delphi process added back to the draft checklist because meeting attendees considered them critical to the guideline. The list was then consolidated and reviewed by executive committee members, including two new information specialist members (S.W. and A.P.A). The draft checklist and explanation and elaboration document was released to the public on March 20, 2019, along with all data and study materials [ 32 ]. All participants in the Delphi process and/or consensus conference were contacted via email with instructions on how to provide feedback on the draft checklist items and/or elaboration and explanation document by commenting directly on the explanation and elaboration draft using a private commenting system, Hypothesis [ 33 ], or if preferred, via email. Comments from other interested individuals were solicited via Twitter, conference presentations, and personal contacts. Comments were collected from the private Hypothesis group, the public Hypothesis comments, and via email. All comments were combined into a single document. Executive committee members reviewed each comment in duplicate to indicate what type of feedback was received (i.e., linguistic, major substantive, minor substantive, or unclear) and, for substantive comments, whether change was recommended or required further discussion.
During the draft and revision process (March 20–June 15, 2019), 358 separate comments were received from 22 individuals and organizations. Based upon the extensive feedback received, the executive team revised the checklist and developed the next iteration, which was released on December 6, 2019, to coincide with the 2019 Virtual Cochrane Colloquium Santiago. Additional feedback from this release was incorporated into the final checklist. Throughout the draft and revision process, several teleconferences were held with the lead of the PRISMA 2020 statement (M.J.P), as an update of the 2009 PRISMA statement was in development, to ensure that the content on search methods was consistent between the PRISMA 2020 and PRISMA-S guidelines [ 34 , 35 ].
Part 2: Checklist
PRISMA-S is a 16-item checklist that covers multiple aspects of the search process for systematic reviews. It is intended to guide reporting, not conduct, of the search. The checklist should be read in conjunction with the Explanation and Elaboration (Part 3), which provides more detail about each item. We also include two boxes, one a glossary of terms (see Table 2 ) and the other, guidance on depositing search data and method descriptions in online repositories (see Table 3 ).
The Explanation and Elaboration also includes examples of good reporting for each item. Each exemplar is drawn from published systematic reviews. For clarity, some exemplars are edited to match the style of this document, including any original citations, and abbreviations are spelled out to aid comprehension. Any other edits to the text are noted with square brackets. A description of the rationale behind the item is explained, followed by additional suggestions for clear reporting and a suggested location(s) for reporting the item.
Not every systematic review will make use of all of the items in the Information Sources and Methods section of the checklist, depending on the research question and the methods chosen by the authors. The checklist provides a framework for the current most common and recommended types of information sources and methods for systematic reviews, but authors should use and report those items relevant and appropriate to their review. The checklist may also be used for systematic review protocols to fully document the planned search, in conjunction with the PRISMA-P reporting guideline [ 36 ] (Table 1 ).
Part 3: Explanation and Elaboration
Item 1. database name.
Name each individual database searched, stating the platform for each.
“The following electronic databases were searched: MEDLINE (Ovid), CINAHL (EBSCOhost), PsycINFO (Ovid), Cochrane Central Register of Controlled Trials (Ovid), SPORTDiscus (EBSCOhost), EMBASE (Ovid) and ProQuest Dissertations and Theses Global (ProQuest).” [ 38 ]
Explanation
Databases are the most commonly used tool to locate studies to include in systematic reviews and meta-analyses [ 6 , 39 ]. There is no single database that is able to provide a complete and accurate list of all studies that meet systematic review criteria due to the differences in the articles included and the indexing methods used between databases (Table 2 ). These differences have led to recommendations that systematic review teams search multiple databases to maximize the likelihood of finding relevant studies [ 6 , 39 , 40 ]. This may include using broad disciplinary databases (e.g., MEDLINE [ 41 ], Embase [ 42 ], Scopus [ 43 ]), specialized databases (e.g., PsycINFO [ 44 ] or EconLit [ 45 ]), or regional databases (e.g., LILACS [ 46 ] or African Index Medicus [ 47 ]).
Many of these literature databases are available through multiple different search platforms (Table 2 ). For example, the MEDLINE database is available through at least 10 different platforms, including Ovid, EBSCOhost, Web of Science, and PubMed. Each platform offers different ways of searching the databases, such as platform-specific field codes (Table 2 ), phrase searching, truncation, or searching full-text versus abstract and keyword only [ 48 ]. Different platforms may contain additional data that are not available in the original database, such as times cited, social media impact, or additional keywords. These differences between the platforms can have a meaningful impact on the results provided [ 48 , 49 , 50 ].
Authors should identify which specific literature databases were searched to locate studies included in the systematic review. It is important that authors indicate not only the database, but the platform through which the database was searched. This helps readers to evaluate the quality and comprehensiveness of the search and supports reproducibility and updating (Table 2 ) in the future by allowing the strategy to be copied and pasted as recommended in Item 8, below.
The distinctions between database and platform may not always be clear to authors, especially when the database is the only one available through a platform (e.g., Scopus [ 43 ]). In these cases, authors may choose to include the web address of the database in the text or the bibliography to provide clarity for their readers.
Suggested location for reporting
Report each database name and platform in the methods section and any supplementary materials (Table 2 ). If space permits, report key database names in the abstract.
Item 2. Multi-database searching
If databases were searched simultaneously on a single platform, state the name of the platform, listing all of the databases searched.
“The MEDLINE and Embase strategies were run simultaneously as a multi-file search in Ovid and the results de-duplicated using the Ovid de-duplication tool.” [ 51 ]
“A systematic literature search was performed in Web of Knowledge™ (including KCI Korean Journal Database, MEDLINE, Russian Science Citation Index, and SciELO Citation Index)….” [ 52 ]
Authors may choose to search multiple databases at once through a single search platform to increase efficiency. Along with the name of the platform, it is necessary to list the names of each of the individual databases included as part of the search. Including information about using this approach in the text of the manuscript helps readers immediately understand how the search was constructed and executed. This helps readers determine how effective the search strategy (Table 2 ) will be for each database [ 1 ].
Report any multi-database search (Table 2 ) in the methods section and any supplementary materials. If space permits, report key individual database names in the abstract, even if run through a multi-database search.
Item 3. Study registries
List any study registries searched.
“[We] searched several clinical trial registries ( ClinicalTrials.gov , Current Controlled Trials ( www.controlled-trials.com ), Australian New Zealand Clinical Trials Registry ( www.actr.org.au ), and University Hospital Medical Information Network Clinical Trials Registry ( www.umin.ac.jp/ctr )) to identify ongoing trials.” [ 53 ]
Study registries are a key source of information for systematic reviews and meta-analyses in the health sciences and increasingly in other disciplines. In the health sciences, study registries (Table 2 ) allow researchers to locate ongoing clinical trials and studies that may have gone unpublished [ 54 , 55 , 56 ]. Some funders, including the National Institutes of Health, require principal investigators to share their data on study registries within a certain time frame after grant completion [ 57 ]. This data may not have been published in any other location, making study registries a critical component of an information strategy, though timely reporting remains a challenge [ 58 , 59 ]. Different countries have their own study registries, as do many pharmaceutical companies.
Outside the health sciences, study registries are becoming increasingly important as many disciplines adopt study pre-registration as a tactic for improving the rigor of research. Though not yet as established as in the health sciences, these study registries are continually expanding and will serve as key sources for finding unpublished studies in fields in the social sciences and beyond.
To fully describe the study registries searched, list the name of each study registry searched, and include a citation or link to the study registry.
Report any study registries searched in the methods section and any supplementary materials.
Item 4. Online resources and browsing
Describe any online or print source purposefully searched or browsed (e.g., tables of contents, print conference proceedings, web sites), and how this was done.
“ We also searched the grey literature using the search string: “public attitudes” AND “sharing” AND “health data” on Google (in June 2017). The first 20 results were selected and screened.” [ 60 ]
“The grey literature search was conducted in October 2015 and included targeted, iterative hand searching of 22 government and/or research organization websites that were suggested during the expert consultation and are listed in S1 Protocol. Twenty two additional citations were added to the review from the grey literature search.” [ 61 ]
“To locate unpublished studies, we searched Embase [via Embase.com ] for conference proceedings since 2000 and hand-searched meeting abstracts of the Canadian Conference on Physician Health and the International Conference on Physician Health (2012 to 2016).” [ 62 ]
Systematic reviews were developed to remove as much bias as possible from the literature review process. One of the most important ways they achieve this reduction in bias is by searching beyond literature databases, which are skewed towards English-language publications with positive results [ 63 , 64 ]. To achieve a fuller picture of what the research on a specific topic looks like, systematic reviewers could seek out research that may be in progress and research that was never published [ 6 ]. Using other methods of finding research also helps identify research that may have been indexed in literature databases, but went undiscovered when searching those sources [ 40 ]. Seeking out this research often involves a complex strategy, drawing on a wealth of online and print resources as well as personal contacts.
Web search engines and specific web sites
Searching general internet search engines and searching the contents of specific websites is a key component of many systematic reviews [ 26 , 65 ]. Government, non-profit organization, and pharmaceutical company websites, for example, contain a wealth of information not published elsewhere [ 6 , 66 ]. Though searching a general search engine like Google or using a general search engine to search a specific website may introduce some bias into the search methodology through the personalization algorithms inherent in many of these tools [ 67 , 68 ], it is still important to fully document how web searches were conducted [ 65 ].
Authors should list all websites searched, along with their corresponding web address. Readers should be able to clearly understand if researchers used a website’s native search interface or advanced search techniques within a general search engine. If authors used a general search engine, authors should declare whether steps were taken to reduce personalization bias (e.g., using “incognito” mode in a browser). Authors may choose whether to detail the websites searched within the text (i.e., Google ( http://www.google.com )), by citing the websites in the bibliography, or by listing the website with corresponding web address in supplementary material, as shown in the examples above.
Review teams may occasionally set an artificial limit to the number of items they will screen from a given search or source [ 65 ]. This is because searching web search engines and individual websites will often lead to an unmanageable number of results, the search engine itself may only display a restricted number of results (e.g., Google will only display 1000 results), or the team has a finite budget or timeline to complete the review. Thus, many systematic review teams utilizing web search engines will often pre-designate a limit to the number of results they review. If review teams choose to review a limited set of results, it should be noted in the text, along with the rationale.
Conference proceedings
Studies show that large percentages of research presented as papers and posters at conferences never make their way into the published literature, particularly if the study’s results were statistically negative [ 63 , 69 ]. Conference proceedings are often the only way to locate these studies. Including conference proceedings in a systematic review search helps minimize bias [ 70 ]. The introduction of online conference proceedings has been a boon to researchers and reduced the need to review printed abstract books. Additionally, some databases either include conference proceedings along with journal articles (i.e., Embase [ 42 ]) or contain only conference proceedings (i.e., ProceedingsFirst [ 71 ] or Directory of Published Papers [ 72 ]). Some conferences have made their abstracts available in a single database (i.e., International AIDS Society’s Abstract Archive [ 73 ]). When using these types of databases to search conference proceedings, authors can treat them as above in Item 1.
Individual conferences’ online proceedings may be password-protected for association members or conference attendees [ 74 ]. When reporting on conference proceedings searched or browsed (Table 2 ) via a conference or association’s online or print proceedings, authors must specify the conference names, the dates of conferences included, and the method used to search the proceedings (i.e., browsing print abstract books or using an online source). If the conference proceedings are searched online, authors should specify the web address(es) for the conference proceedings and the date(s) of the conferences. If the conference proceedings are published in a journal, the authors should cite the journal. If the proceedings are a standalone publication, authors may choose to cite them using the same methods used to cite a book or by providing the full information about the conference (name, location, dates, etc.) in a supplementary file.
General browsing
Authors also commonly browse print or online tables of contents, full contents of journals, or other sources that are the most likely to contain research on the topic sought. When purposefully browsing, describe any method used, the name of the journal or other source, and the time frame covered by the search, if applicable.
Report online information sources (Table 2 ) searched or browsed in the methods section and in any supplementary materials. Systematic reviews using several of these methods, or using multiple information sources for each method, may need to report their methods briefly in the methods section, but should fully report all necessary information to describe their approaches in supplementary materials.
Item 5. Citation searching
Indicate whether cited references or citing references were examined, and describe any methods used for locating cited/citing references (e.g., browsing reference lists, using a citation index, setting up email alerts for references citing included studies).
“Reference lists of included articles were manually screened to identify additional studies.” [ 75 ]
“[W]e used all shared decision making measurement instruments that were identified in Gärtner et al’s recent systematic review (Appendix A). We then performed a systematic citation search, collecting all articles that cited the original papers reporting on the development, validation, or translation of any the observational and/or self-reported shared decision making measurement instruments identified in that review. An experienced librarian (P.J.E.) searched Web of Science [Science Citation Index] and Scopus for articles published between January 2012 and February 2018.” [ 76 ]
“We [conducted] citation tracking of included studies in Web of Science Core Collection on an ongoing basis, using citation alerts in Web of Science Core Collection.” [ 77 ]
One of the most common search methods is reviewing the references or bibliographies of included studies [ 11 , 17 ]. This type of citation searching (looking for cited references) can be additive to other cited reference searching methods, such as examining bibliographies of relevant systematic reviews. In addition, researchers may choose to look for articles that cite specified studies [ 78 ]. This may include looking beyond one level forwards and backwards (e.g., examining the bibliographies of articles cited by specified articles) [ 78 ]. Looking at bibliographies of included articles or other specified articles is often conducted by examining full-text articles, but it can also be accomplished using online tools called citation indexes (Table 2 ).
The use of these methods can be complicated to describe, but the explanation should clearly state the database used, if applicable (i.e., Scopus, Google Scholar, Science Citation Index) and describe any other methods used. Authors also must cite the “base” article(s) that citation searching was performed upon, either for examining cited or citing articles (Table 2 ). If the same database is used for both a topical search as well as citation searching, describe each use separately. For manually checking the reference lists for included articles, a simple statement as in the first example is sufficient.
Report citation searching details in the methods section and in any supplementary materials.
Item 6. Contacts
Indicate whether additional studies or data were sought by contacting authors, experts, manufacturers, or others.
“We contacted representatives from the manufacturers of erythropoietin-receptor agonists (Amgen, Ortho-Biotech, Roche), corresponding or first authors of all included trials and subject-area experts for information about ongoing studies.” [ 79 ]
“We also sought data via expert requests. We requested data on the epidemiology of injecting drug use and blood-borne viruses in October, 2016, via an email distribution process and social media. This process consisted of initial emails sent to more than 2000 key experts and organisations, including contacts in the global, regional, and country offices of WHO, UNAIDS, Global Fund, and UNODC (appendix p 61). Staff in those agencies also forwarded the request to their colleagues and other relevant contacts. One member of the research team (SL) posted a request for data on Twitter, which was delivered to 5525 individual feeds (appendix p 62).” [ 80 ]
Contacting manufacturers (e.g., pharmaceutical companies), or reaching out to authors or experts directly or through organizations, is a key method to locate unpublished and ongoing studies [ 6 ]. Contacting authors or manufacturers may also be necessary when publications, conference proceedings, or clinical trials registry records do not provide the complete information needed [ 63 , 81 ]. Contacting manufacturers or regulating agencies might be required to acquire complete trial data from the clinical study reports [ 82 , 83 ]. More broad calls for evidence may also be conducted when no specific groups or individuals are targeted.
Contact methods may vary widely, depending on the context, and may include personal contact, web forms, email mailing lists, mailed letters, social media contacts, or other methods. As these strategies are inherently difficult to reproduce, researchers should attempt to give as much detail as possible on what data or information was sought, who or what group(s) provided data or information, and how the individuals or groups were identified.
Report information about contacts to solicit additional information in the methods section and in any supplementary materials. Systematic reviews using elaborate calls for evidence or making extensive use of contacts as an information source may need to report their methods briefly in the methods section, but should fully report all necessary information to describe their approaches in supplementary materials.
Item 7. Other methods
Describe any additional information sources or search methods used.
“We also searched… our personal files.” [ 84 ]
“PubMed’s related articles search was performed on all included articles.” [ 85 ]
A thorough systematic review may utilize many additional methods of locating studies beyond database searching, many of which may not be reproducible methods. A key example is searching personal files. Another is using databases’ built in tools, such as PubMed’s Related Articles feature [ 86 ] or Clarivate Analytics’ Web of Science’s Related Records feature [ 87 ], to locate relevant articles based on commonalities with a starting article. Because these tools are often proprietary and their algorithms opaque, researchers may not be able to replicate the exact results at a later date. To attempt to be as transparent as possible, researchers should both note the tool that was used and cite any articles these operations were run upon. For all “other” methods, it is still important to declare that the method was used, even if it may not be fully replicable.
Report information about any other additional information sources or search methods used in the methods section and in any supplementary materials.
Item 8. Full search strategies
Include the search strategies for each database and information source, copied and pasted exactly as run.
Database search. Methods section description . “The reproducible searches for all databases are available at DOI:10.7302/Z2VH5M1H.” [ 88 ]
Database search. One of the full search strategies from supplemental materials in online repository . “ Embase.com (692 on Jan 19, 2017) 1. 'social media'/exp OR (social NEAR/2 (media* OR medium* OR network*)):ti OR twitter:ti OR youtube:ti OR facebook:ti OR linkedin:ti OR pinterest:ti OR microblog*:ti OR blog:ti OR blogging:ti OR tweeting:ti OR 'web 2.0':ti 2. 'professionalism'/exp OR 'ethics'/exp OR 'professional standard'/de OR 'professional misconduct'/de OR ethic*:ab,ti OR unprofessional*:ab,ti OR professionalism:ab,ti OR (professional* NEAR/3 (standard* OR misconduct)):ab,ti OR ((professional OR responsib*) NEAR/3 (behavi* OR act OR conduct*)):ab,ti 3. #1 AND #2 AND [english]/lim NOT ('conference abstract':it OR 'conference paper':it) [ 88 ]
Online resources and browsing. Methods section description . “The approach to study identification from this systematic review is transparently reported in the Electronic Supplementary Material Appendix S1.” [ 89 ]
Online resources and browsing. One of the full online resource search strategies reported in supplement . “Date: 12/01/16. Portal/URL: Google. https://www.google.co.uk/webhp?hl=en . Search terms: ((Physical training) and (man or men or male or males) and (female or females or women or woman) and (military)). Notes: First 5 pages screened on title (n=50 records).” [ 89 ]
Systematic reviews and related review types rely on thorough and complex search strategies to identify literature on a given topic. The search strategies used to conduct this data gathering are essential to the transparency and reproducibility of any systematic review. Without being able to assess the quality of the search strategies used, readers are unable to assess the quality of the systematic review [ 9 , 11 , 17 ].
When space was at a premium in publications, complete reporting of search strategies was a challenge. Because it was necessary to balance the need for transparency with publication restrictions, previous PRISMA guidelines recommended including the complete search strategy from a minimum of one database searched [ 9 ]. Many systematic reviews therefore reported only the minimum necessary. However, reporting only selected search strategies can contribute to the observed irreproducibility of many systematic reviews [ 11 , 17 ].
The prior versions of PRISMA did not elaborate on methods for reporting search strategies outside of literature databases. Subsequent to its publication, many groups have begun identifying the challenges of fully documenting other types of search methods [ 90 , 91 ]. Now recommended is the explicit documentation of all of the details of all search strategies undertaken [ 91 , 92 ]. These should be reported to ensure transparency and maximum reproducibility, including searches and purposeful browsing activities undertaken in web search engines, websites, conference proceeding databases, electronic journals, and study registries.
Journal restrictions vary, but many journals now allow authors to publish supplementary materials with their manuscripts. At minimum, all search strategies, including search strategies for web search engines, websites, conference proceedings databases, electronic journals, and study registries, should be submitted as a supplement for publication. Though most supplements are appropriately accessible on journal publishers’ web sites as submitted, supplements may disappear [ 17 ]. In addition, many supplements are only available to journal subscribers [ 93 ]. Similarly, manuscripts available on public access systems like PubMed Central [ 94 ] may not have the corresponding supplemental materials properly linked. For optimal accessibility, authors should upload complete documentation to a data repository (Table 2 ), an institutional repository, or other secure and permanent online archive instead of relying on journal publication (see Table 3 for additional information).
It is important to document and report the search strategy exactly as run, typically by copying and pasting the search strategy directly as entered into the search platform. This is to ensure that information such as the fields searched, term truncation, and combinations of terms (i.e., Boolean logic or phrases) are accurately recorded. Many times, the copied and pasted version of a search strategy will also include key information such as limits (see Item 9; Table 2 ) used, databases searched within a multi-database search, and other database-specific detail that will enable more accurate reporting and greater reproducibility. This documentation must also repeat the database or resource name, database platform or web address, and other details necessary to clearly describe the resource.
Report the full search strategy in supplementary materials as described above. Describe and link to the location of the supplementary materials in the methods section.
Item 9: Limits and restrictions
Specify that no limits were used, or describe any limits or restrictions applied to a search (e.g., date or time period, language, study design) and provide justification for their use.
No limits . “We imposed no language or other restrictions on any of the searches.” [ 95 ]
Limits described without justification . “The search was limited to the English language and to human studies.” [ 96 ]
“The following search limits were then applied: randomized clinical trials (RCTs) of humans 18 years or older, systematic reviews, and meta-analyses.” [ 97 ]
Limits described with justification . “The search was limited to publications from 2000 to 2018 given that more contemporary studies included patient cohorts that are most reflective of current co-morbidities and patient characteristics as a result of the evolving obesity epidemic.” [ 98 ]
Limits described, one with justification . “Excluded publication types were comments, editorials, patient education handouts, newspaper articles, biographies, autobiographies, and case reports. All languages were included in the search result; non-English results were removed during the review process…. To improve specificity, the updated search was limited to human participants.” [ 99 ]
Many databases have features that allow searchers to quickly restrict a search using limits. What limits are available in a database are unique to both the database and the platform used to search it. Limits are dependent on the accuracy of the indexer, the timeliness of indexing, and the quality of any publisher-supplied data. For instance, using database limits to restrict searches to randomized controlled trials will only find records identified by the indexer as randomized controlled trials. Since the indexing may take 6 months or more to complete for any given article, searchers risk missing new articles when using database limits.
Using database-provided limit features should not be confused with using filters (see Item 10; Table 2 ) or inclusion criteria for the systematic review. For example, systematic review teams may choose to only include English-language randomized controlled trials. This can be done using limits, a combination of a filter (see Item 10) and screening, or screening alone. It should be clear to the reader which approach is used. For instance, in the “ Limits described, with one justification ” example above, the authors used database limits to restrict their search by publication type, but they did not use database limits to restrict by language, even though that was a component of their eligibility criteria. They also used database limits to restrict to human participants in their search update.
It is important for transparency and reproducibility that any database limits applied when running the search are reported accurately, as their use has high potential for introducing bias into a search [ 1 , 64 , 100 , 101 ]. Database limits are not recommended for use in systematic reviews, due to their fallibility [ 39 , 100 ]. If used, review teams should include a statement of justification for each use of a database limit in the methods section, the limitations section, or both [ 102 , 103 ]. In the examples above, only the last two examples provide some justification in the methods section (“to improve specificity” [ 99 ] and “contemporary studies included patient cohorts that are most reflective of current co-morbidities and patient characteristics as a result of the evolving obesity epidemic” [ 98 ]).
Report any limits or restrictions used or that no limits were used in the abstract, methods section, and in any supplementary materials, including the full search strategies (Item 8). Report the justification for any limits used within the methods section and/or in the limitations section.
Item 10. Search filters
Indicate whether published search filters were used (as originally designed or modified), and if so, cite the filter(s) used.
“For our MEDLINE search we added a highly sensitive filter for identifying randomised trials developed by the Cochrane Collaboration [38]. For Embase we used the filter for randomised trials proposed by the Scottish Intercollegiate Guidelines Network [ 104 ].” [ 105 ]
Filters are a predefined combination of search terms developed to identify references with a specific content, such as a particular type of study design (e.g., randomized controlled trials) [ 106 ], populations (e.g., the elderly), or a topic (e.g., heart failure) [ 107 ]. They often consist of a combination of subject headings, free-text terms, and publication types [ 107 ]. For systematic reviews, filters are generally recommended for use instead of limits built into databases, as discussed in Item 9, because they provide the much higher sensitivity (Table 2 ) required for a comprehensive search [ 108 ].
Any filters used as part of the search strategy should be cited, whether published in a journal article or other source. This enables readers to assess the quality of the filter(s) used, as most published search filters are validated and/or peer reviewed [ 106 , 107 ]. Many commonly used filters are published on the InterTASC Information Specialists’ Sub-Group [ 109 ], in the Cochrane Handbook [ 4 , 39 ], and through the Health Information Research Unit of McMaster University [ 110 ].
Cite any search filter used in the methods section and describe adaptations made to any filter. Include the copied and pasted details of any search filter used or adapted for use as part of the full search strategy (Item 8).
Item 11. Prior work
Indicate when search strategies from other literature reviews were adapted or reused for a substantive part or all of the search, citing the previous review(s).
“We included [search strategies] used in other systematic reviews for research design [ 111 ], setting [ 112 , 113 ], physical activity and healthy eating [ 114 , 115 , 116 ], obesity [ 111 ], tobacco use prevention [ 117 ], and alcohol misuse [ 118 ]. We also used a search [strategy] for intervention (implementation strategies) that had been employed in previous Cochrane Reviews [ 119 , 120 ], and which was originally developed based on common terms in implementation and dissemination research.” [ 121 ]
Many authors may also examine previously published search strategies to develop the search strategies for their review. Sometimes, authors adapt or reuse these searches for different systematic reviews [ 122 ]. When basing a new search strategy on a published search strategy, it is appropriate to cite the original publication(s) consulted.
Search strategies differ from filters (Item 10) because search strategies are often developed for a specific project, not necessarily designed to be repeatedly used. Filters, on the other hand, are developed with the express purpose of reuse. Filters are often objectively derived, tested, and validated, whereas most search strategies published as part of systematic review or other evidence synthesis are “best guess,” relying on the expertise of the searcher and review team [ 107 ].
As in the example above, researchers may rely on multiple prior published searches to construct a new search for a novel review. Many times, researchers will use the same searches from a published systematic review to update the existing systematic review. In either case, it is helpful to the readers to understand whether major portions of a search are being adapted or reused.
Report any prior work consulted, adapted, or reused in the methods section. Include the copied and pasted search strategies used, including portions or the entirety of any prior work used or adapted for use, in the full search strategy (Item 8).
Item 12. Updates
Report the methods used to update the search(es) (e.g., rerunning searches, email alerts).
“Ovid Auto Alerts were set up to provide weekly updates of new literature until July 09, 2012.” [ 123 ]
“ Two consecutive searches were conducted and limited by publication type and by date, first from January 1, 1990, to November 30, 2012, and again from December 1, 2012, to July 31, 2015, in an updated search…. The original search strategy was used to model the updated search from December 1, 2012, to July 31, 2015. The updated search strategy was consistent with the original search; however, changes were required in the ERIC database search because of a change in the ERIC search algorithm. Excluded publication types were identical to the initial search. To improve specificity, the updated search was limited to human participants.” [ 99 ]
The literature search is usually conducted at the initial stage of the production of a systematic review. As a consequence, the results of a search may be outdated before the review is published [ 124 , 125 , 126 ]. The last search in a review should be conducted ideally less than 6 months before publication [ 90 , 92 , 125 ]. For this reason, authors often update searches by rerunning (Table 2 ) the same search(es) or otherwise updating searches before the planned publication date. Updating searches differs from updating a systematic review, i.e., when the same or different authors or groups decide to redo a published systematic review to bring its findings up to date. If authors are updating a published systematic review, either authored by the same review team or another, Item 11 contains relevant guidance.
When reporting search updates, the extent of reporting depends on methods used and any changes that were made while updating the searches. If there are no changes in information sources and/or search syntax (Table 2 ), it is sufficient to indicate the date the last search was run in the methods section and in the supplementary materials. If there are any changes in information sources and/or search syntax, the changes should be indicated (e.g., different set of databases, changes in search syntax, date restrictions) in the methods section. Authors should explain why these changes were made. When there were changes in the search strategy syntax, the original and the updated searches should both be reported as described in Item 8.
If authors use email alerts or other methods to update searches, these methods can be briefly described by indicating the method used, the frequency of any updates, the name of the database(s) used, or other relevant information that will help readers understand how the authors conducted search updates. If deduplication methods are used as part of the search update process, these methods can be described using guidance in Item 16.
Report the methods used to update the searches in the methods section and the supplementary materials, as described above.
Item 13. Dates of searches
For each search strategy, provide the date when the last search occurred.
“A comprehensive literature search was initially run on 26 February 2017 and then rerun on 5 February 2018….” [ 127 ]
Most literature databases are regularly updated with new citations as articles are published. Citations already in the database may also be updated once new information (such as indexing terms or citing articles) is available. As an example, MEDLINE added over 900,000 indexed citations (Table 2 ) in fiscal year 2018 [ 41 ]. In addition, the information gathered by databases (such as author affiliations in MEDLINE) can change over time. Because new citations are regularly being added, systematic review guidelines recommend updating searches throughout the writing process to ensure that all relevant articles are retrieved [ 6 , 92 ].
It is necessary for authors to document the date when searches were executed, either the date the initial search was conducted, if only searched once, or the most recent date the search was rerun. This allows readers to evaluate the currency of each search and understand what literature the search could have potentially identified [ 125 ]. In addition, it supports reproducibility and updating by allowing other researchers to use date limits to view the same “slice” of the database that the original authors used or to update a systematic review by searching from the last time point searched.
Report the date of the last search of the primary information sources used in the abstract for optimal clarity for readers [ 128 ]. Report the time frame during which searches were conducted, the initial search date(s), and/or the last update search date(s) in the methods section. Report the initial and/or last update search date with each complete search strategy in the supplementary materials, as in the examples for Item 8.
Item 14. Peer review
Describe any search peer review process.
“The strategies were peer reviewed by another senior information specialist prior to execution using the PRESS Checklist [ 1 ].” [ 129 ]
Peer reviewing search strategies is an increasingly valued component of search strategy development for systematic reviews. Expert guidance recommends taking this step to help increase the robustness of the search strategy [ 6 , 74 ]. Peer reviewing (Table 2 ) searches is useful to help to guide and improve electronic search strategies. One of peer review’s main benefits is the reduction of errors [ 23 , 130 ]. Peer review may also increase the number of relevant records found for inclusion in reviews, thus improving the overall quality of the systematic review [ 131 ].
Authors should consider using the Peer Review of Electronic Search Strategies (PRESS) Guideline statement, a practice guideline for literature search peer review outlining the major components important to review and the benefits of peer reviewing searches [ 1 ]. Authors should strongly consider having the search strategy peer reviewed by an experienced searcher, information specialist, or librarian [ 1 , 131 ]. Though peer review may be conducted generally with publication of a protocol, for example, this item is designed to document search-specific peer review.
Describe the use of peer review in the methods section.
Item 15. Total records
Document the total number of records identified from each database and other information sources.
Methods section . “A total of 3251 citations were retrieved from the six databases and four grey literature websites.” [ 133 ] Flow diagram . Fig. 1 . Fig. 1 “Figure 1. PRISMA 2009 flow diagram” [ 132 ] Full size image
Recording the flow of citations through the systematic review process is a key component of the PRISMA Statement [ 9 , 35 ]. It is helpful to identify how many records (Table 2 ) were identified within each database and additional source. Readers can use this information to see whether databases or expert contacts constituted the majority of the records reviewed, for example. Knowing the number of records from each source also helps with reproducibility. If a reader tries to duplicate a search from a systematic review, one would expect to retrieve nearly the same results when limiting to the timeframe in the original review. If instead, the searcher locates a drastically different number of results than reported in the original review, this can be indicative of errors in the published search [ 23 ] or major changes to a database, both of which might be reasons to update a systematic review or view the systematic review’s results with skepticism.
Report the total number of references retrieved from all sources, including updates, in the results section. Report the total number of references from each database and information source in the supplementary materials. If space permits, report the total number of references from each database in the PRISMA flow diagram [ 35 ].
Item 16. Deduplication
Describe the processes and any software used to deduplicate records from multiple database searches and other information sources.
“Duplicates were removed by the librarians (LP, PJE), using EndNote's duplicate identification strategy and then manually.” [ 134 ]
Databases contain significant overlap in content. When searching in multiple databases and additional information sources, as is necessary for a systematic review, authors often employ a variety of techniques to reduce the number of duplicates within their results prior to screening [ 135 , 136 , 137 , 138 ]. Techniques vary in their efficacy, sensitivity, and specificity (Table 2 ) [ 136 , 138 ]. Knowing which method is used enables readers to evaluate the process and understand to what extent these techniques may have removed false positive duplicates [ 138 ]. Authors should describe and cite any software or technique used, when applicable. If duplicates were removed manually, authors should include a description.
Report any deduplication method used in the methods section. The total number of references after deduplication should be reported in the PRISMA flow diagram [ 35 ].
Part 5. Discussion and conclusions
The PRISMA-S extension is designed to be used in conjunction with PRISMA 2020 [ 35 ] and PRISMA extensions including PRISMA-P for protocols [ 36 ], PRISMA-ScR for scoping reviews [ 139 ], the PRISMA Network Meta-analyses statement [ 140 ], and PRISMA-IPD for systematic reviews using individual patient data [ 141 ]. It may also be used with other reporting guidelines that relate to systematic reviews and related review types, such as RepOrting standards for Systematic Evidence Syntheses (ROSES) [ 142 ]. It provides additional guidance for systematic review teams, information specialists, librarians, and other researchers whose work contains a literature search as a component of the research methods. Though its origins are in the biomedical fields, PRISMA-S is flexible enough to be applied in all disciplines that use method-driven literature searching. Ultimately, PRISMA-S attempts to give systematic review teams a framework that helps ensure transparency and maximum reproducibility of the search component of their review.
PRISMA-S is intended to capture and provide specific guidance for reporting the most common methods used in systematic reviews today. As new methods and information sources are adopted, authors may need to adjust their reporting methods to accommodate new processes. Currently, PRISMA-S does not address using text mining or text analysis methods to create the search, for example, though this is an increasingly common way for information specialists to develop robust and objective search strategies [ 143 , 144 , 145 ]. Likewise, PRISMA-S does not require that decisions about the rationale behind choices in search terms and search construction be recorded, though this provides readers a great deal of insight. In the future, methods and rationales used to create search strategies may become more important for reproducibility.
PRISMA-S offers extensive guidance for many different types of information source and methods, many of them not described in detail in other reporting guidelines relating to literature searching. This includes detailed information on reporting study registry searches, web searches, multi-database searches, and updates. PRISMA-S can help authors report all components of their search, hopefully making the reporting process easier. As a note, PRISMA-S provides guidance on transparent reporting to authors and is not intended as a tool to either guide conduct of a systematic review or to evaluate the quality of a search or a systematic review.
The PRISMA-S checklist is available for download in Word and PDF formats from the PRISMA Statement web site [ 37 ]. The checklist should be used together with its Explanation & Elaboration documentation to provide authors with guidance for the complexities of different types of information sources and methods.
We intend to work with systematic review and information specialist organizations to broadly disseminate PRISMA-S and encourage its adoption by journals. In addition, we plan to host a series of webinars discussing how to use PRISMA-S most effectively. These webinars will also be available for later viewing and will serve as a community resource.
We hope that journal editors will recommend authors of systematic reviews and other related reviews to use PRISMA-S and submit a PRISMA-S checklist with their manuscripts. We also hope that journal editors will encourage more stringent peer review of systematic review searches to ensure greater transparency and reproducibility within the review literature.
Availability of data and materials
All data is available via the PRISMA-S PRISMA Search Reporting Extension OSF site ( https://doi.org/10.17605/OSF.IO/YGN9W ) [ 32 ]. This includes all data relating to item development, survey instruments, data from the Delphi surveys, and consent documents.
Abbreviations
Digital object identifier
Peer Review of Electronic Search Strategies
Preferred Reporting Items for Systematic reviews and Meta-Analyses
PRISMA for individual patient data
PRISMA for systematic review protocols
PRISMA for scoping reviews
RepOrting standards for Systematic Evidence Syntheses
McGowan J, Sampson M, Salzwedel DM, Cogo E, Foerster V, Lefebvre C. PRESS Peer Review of Electronic Search Strategies: 2015 guideline statement. J Clin Epidemiol. 2016;75:40–6.
Article PubMed Google Scholar
Lefebvre C, Glanville J, Briscoe S, et al. Searching for and selecting studies. In: Higgins J, Thomas J, Chandler J, et al, eds. Cochrane Handbook for Systematic Reviews of Interventions: version 6.0. 2019. https://training.cochrane.org/handbook/current/chapter-04 .
Centre for Reviews and Dissemination. Systematic reviews: CRD’s guidance for undertaking reviews in health care. 1.3 Undertaking the review. 2009; https://www.york.ac.uk/media/crd/Systematic_Reviews.pdf . Accessed 31 Jan, 2020.
Lefebvre C, Glanville J, Briscoe S, Littlewood A, Marshall C, Metzendorf MI. Technical supplement to chapter 4: searching for and selecting studies. In: Higgins JPT, Thomas J, Chandler J, Cumpston M, Li T, Page MJ, editors. Cochrane Handbook for Systematic Reviews of Interventions: version 6.0. 2019. https://training.cochrane.org/handbook .
Relevo R, Balshem H. Finding evidence for comparing medical interventions: AHRQ and the Effective Health Care Program. J Clin Epidemiol. 2011;64(11):1168–77.
Institute of Medicine. Finding What Works in Health Care : Standards for Systematic Reviews. Washington, D.C.: National Academies Press; 2011. https://doi.org/10.17226/13059 .
European Network for Health Technology Assessment. Process of information retrieval for systematic reviews and health technology assessments on clinical effectiveness: guideline; version 2.0. 2019; https://eunethta.eu/wp-content/uploads/2020/01/EUnetHTA_Guideline_Information_Retrieval_v2-0.pdf . Accessed 31 Jan, 2020.
Sampson M, McGowan J, Tetzlaff J, Cogo E, Moher D. No consensus exists on search reporting methods for systematic reviews. J Clin Epidemiol. 2008;61(8):748–54.
Liberati A, Altman DG, Tetzlaff J, et al. The PRISMA statement for reporting systematic reviews and meta-analyses of studies that evaluate health care interventions: explanation and elaboration. PLoS Med. 2009;6(7):e1000100.
Article PubMed PubMed Central Google Scholar
Page MJ, Moher D. Evaluations of the uptake and impact of the Preferred Reporting Items for Systematic reviews and Meta-Analyses (PRISMA) Statement and extensions: a scoping review. Syst Rev. 2017;6(1):263.
Koffel JB, Rethlefsen ML. Reproducibility of search strategies is poor in systematic reviews published in high-impact pediatrics, cardiology and surgery journals: a cross-sectional study. PLoS One. 2016;11(9):e0163309.
Article PubMed PubMed Central CAS Google Scholar
Faggion CM Jr, Wu YC, Tu YK, Wasiak J. Quality of search strategies reported in systematic reviews published in stereotactic radiosurgery. Br J Radiol. 2016;89(1062):20150878.
Layton D. A critical review of search strategies used in recent systematic reviews published in selected prosthodontic and implant-related journals: are systematic reviews actually systematic? Int J Prosthodont. 2017;30(1):13–21.
Yaylali IE, Alacam T. Critical assessment of search strategies in systematic reviews in endodontics. J Endod. 2016;42(6):854–60.
Page MJ, Shamseer L, Altman DG, et al. Epidemiology and reporting characteristics of systematic reviews of biomedical research: a cross-sectional study. PLoS Med. 2016;13(5):e1002028.
Koffel JB. Use of recommended search strategies in systematic reviews and the impact of librarian involvement: a cross-sectional survey of recent authors. PLoS One. 2015;10(5):e0125931.
Rethlefsen ML, Farrell AM, Osterhaus Trzasko LC, Brigham TJ. Librarian co-authors correlated with higher quality reported search strategies in general internal medicine systematic reviews. J Clin Epidemiol. 2015;68(6):617–26.
Meert D, Torabi N, Costella J. Impact of librarians on reporting of the literature searching component of pediatric systematic reviews. J Med Libr Assoc. 2016;104(4):267–77.
Maggio LA, Tannery NH, Kanter SL. Reproducibility of literature search reporting in medical education reviews. Acad Med. 2011;86(8):1049–54.
Yoshii A, Plaut DA, McGraw KA, Anderson MJ, Wellik KE. Analysis of the reporting of search strategies in Cochrane systematic reviews. J Med Libr Assoc. 2009;97(1):21–9.
Franco JVA, Garrote VL, Escobar Liquitay CM, Vietto V. Identification of problems in search strategies in Cochrane Reviews. Res Synth Methods. 2018;9(3):408–16.
Salvador-Oliván JA, Marco-Cuenca G, Arquero-Avilés R. Errors in search strategies used in systematic reviews and their effects on information retrieval. J Med Libr Assoc. 2019;107(2):210–21.
Sampson M, McGowan J. Errors in search strategies were identified by type and frequency. J Clin Epidemiol. 2006;59(10):1057–63.
Mullins MM, DeLuca JB, Crepaz N, Lyles CM. Reporting quality of search methods in systematic reviews of HIV behavioral interventions (2000-2010): are the searches clearly explained, systematic and reproducible? Res Synth Methods. 2014;5(2):116–30.
Rader T, Mann M, Stansfield C, Cooper C, Sampson M. Methods for documenting systematic review searches: a discussion of common issues. Res Synth Methods. 2014;5(2):98–115.
Briscoe S. Web searching for systematic reviews: a case study of reporting standards in the UK Health Technology Assessment programme. BMC Res Note. 2015;8:153.
Article Google Scholar
Moher D, Stewart L, Shekelle P. All in the family: systematic reviews, rapid reviews, scoping reviews, realist reviews, and more. Syst Rev. 2015;4:183.
Grant MJ, Booth A. A typology of reviews: an analysis of 14 review types and associated methodologies. Health Info Libr . 2009;26(2):91–108.
Rethlefsen ML, Koffel JB, Kirtley S. PRISMA-Search (PRISMA-S) extension to PRISMA development protocol. 2016; https://www.equator-network.org/wp-content/uploads/2009/02/Protocol-PRISMA-S-Delphi.pdf . Accessed 16 Jan, 2020.
Moher D, Schulz KF, Simera I, Altman DG. Guidance for developers of health research reporting guidelines. PLoS Med. 2010;7(2):e1000217.
Hsu C, Sandford BA. The Delphi technique: making sense of consensus. Pract Assess Res Eval. 2007;12:10. https://doi.org/10.7275/pdz9-th90 .
Rethlefsen ML, Koffel JB, Kirtley S, Ayala AP, Waffenschmidt S. PRISMA-S: PRISMA Search Reporting Extension. 2019; https://doi.org/10.17605/OSF.IO/YGN9W . Accessed 5 Feb, 2020.
Hypothesis. 2020; https://web.hypothes.is/ . Accessed 3 Jan, 2020.
Page MJ, McKenzie JE, Bossuyt PM, et al. Updating the PRISMA reporting guideline for systematic reviews and meta-analyses: study protocol. 2018; http://osf.io/2v7mk . Accessed 13 Feb, 2020.
Page MJ, McKenzie JE, Bossuyt PM, et al. The PRISMA 2020 statement: an updated guideline for reporting systematic reviews. MetaArXiv Preprints 2020; https://doi.org/10.31222/osf.io/v7gm2 . Accessed 25 Oct 2020.
Shamseer L, Moher D, Clarke M, et al. Preferred reporting items for systematic review and meta-analysis protocols (PRISMA-P) 2015: elaboration and explanation. BMJ. 2015;350:g7647.
PRISMA: Transparent reporting of systematic reviews and meta-analyses. 2015; http://prisma-statement.org/ . Accessed 13 Feb, 2020.
Schneider KJ, Leddy JJ, Guskiewicz KM, et al. Rest and treatment/rehabilitation following sport-related concussion: a systematic review. Br J Sports Med. 2017;51(12):930–4.
Lefebvre C, Manheimer E, Glanville J. Searching for studies. 2011; http://handbook.cochrane.org/chapter_6/6_searching_for_studies.htm . Accessed 25 Nov, 2014.
Bramer WM, Rethlefsen ML, Kleijnen J, Franco OH. Optimal database combinations for literature searches in systematic reviews: a prospective exploratory study. Syst Rev. 2017;6(1):245.
National Library of Medicine. MEDLINE®: description of the database 2019; https://www.nlm.nih.gov/bsd/medline.html . Accessed 1 Feb, 2020.
Embase content. 2019; https://www.elsevier.com/solutions/embase-biomedical-research/embase-coverage-and-content . Accessed 28 February, 2019.
Scopus. 2020; http://www.scopus.com . Accessed 1 Feb, 2020.
PsycINFO. 2020; https://www.apa.org/pubs/databases/psycinfo . Accessed 1 Feb, 2020.
EconLit. 2020; https://www.aeaweb.org/econlit/ . Accessed 1 Feb, 2020.
BIREME - PAHO - WHO Latin American and Caribbean Center on Health Sciences Information. LILACS. 2020; http://lilacs.bvsalud.org/en/ . Accessed 1 Feb, 2020.
World Health Organization. African Index Medicus. 2020; http://indexmedicus.afro.who.int/ . Accessed 1 Feb, 2020.
Craven J, Jefferies J, Kendrick J, Nicholls D, Boynton J, Frankish R. A comparison of searching the Cochrane library databases via CRD, Ovid and Wiley: implications for systematic searching and information services. Health Info Libr J. 2014;31(1):54–63.
Bramer WM, Giustini D, Kleijnen J, Franco OH. Searching Embase and MEDLINE by using only major descriptors or title and abstract fields: a prospective exploratory study. Syst Rev. 2018;7(1):200.
Younger P, Boddy K. When is a search not a search? A comparison of searching the AMED complementary health database via EBSCOhost, OVID and DIALOG. Health Info Libr J. 2009;26(2):126–35.
Fraser C, Murray A, Burr J. Identifying observational studies of surgical interventions in MEDLINE and EMBASE. BMC Med Res Methodol. 2006;6:41.
De Cagna F, Fusar-Poli L, Damiani S, et al. The role of intranasal oxytocin in anxiety and depressive disorders: a systematic review of randomized controlled trials. Clin Psychopharmacol Neurosci. 2019;17(1):1–11.
Rutjes AW, Juni P, da Costa BR, Trelle S, Nuesch E, Reichenbach S. Viscosupplementation for osteoarthritis of the knee: a systematic review and meta-analysis. Ann Intern Med. 2012;157(3):180–91.
Potthast R, Vervolgyi V, McGauran N, Kerekes MF, Wieseler B, Kaiser T. Impact of inclusion of industry trial results registries as an information source for systematic reviews. PLoS One. 2014;9(4):e92067.
Turner EH, Matthews AM, Linardatos E, Tell RA, Rosenthal R. Selective publication of antidepressant trials and its influence on apparent efficacy. N Engl J Med. 2008;358(3):252–60.
Article CAS PubMed Google Scholar
Baudard M, Yavchitz A, Ravaud P, Perrodeau E, Boutron I. Impact of searching clinical trial registries in systematic reviews of pharmaceutical treatments: methodological systematic review and reanalysis of meta-analyses. BMJ. 2017;356:j448.
Zarin DA, Tse T, Williams RJ, Carr S. Trial reporting in ClinicalTrials.gov - the final rule. N Engl J Med 2016;375(20):1998-2004.
Anderson ML, Chiswell K, Peterson ED, Tasneem A, Topping J, Califf RM. Compliance with results reporting at ClinicalTrials.gov. N Engl J Med. 2015;372(11):1031–9.
Article CAS PubMed PubMed Central Google Scholar
DeVito NJ, Bacon S, Goldacre B. Compliance with legal requirement to report clinical trial results on ClinicalTrials.gov: a cohort study. Lancet. 2020;395(10221):361–9.
Stockdale J, Cassell J, Ford E. “Giving something back”: a systematic review and ethical enquiry into public views on the use of patient data for research in the United Kingdom and the Republic of Ireland. Wellcome Open Res. 2018;3:6.
Mascarenhas M, Garasia S, Berthiaume P, et al. A scoping review of published literature on chikungunya virus. PLoS One. 2018;13(11):e0207554.
Gates M, Wingert A, Featherstone R, Samuels C, Simon C, Dyson MP. Impact of fatigue and insufficient sleep on physician and patient outcomes: a systematic review. BMJ Open. 2018;8(9):e021967.
Song F, Parekh-Bhurke S, Hooper L, et al. Extent of publication bias in different categories of research cohorts: a meta-analysis of empirical studies. BMC Med Res Methodol. 2009;9:79.
Egger M, Zellweger-Zahner T, Schneider M, Junker C, Lengeler C, Antes G. Language bias in randomised controlled trials published in English and German. Lancet. 1997;350(9074):326–9.
Stansfield C, Dickson K, Bangpan M. Exploring issues in the conduct of website searching and other online sources for systematic reviews: how can we be systematic? Syst Rev. 2016;5(1):191.
Farrah K, Mierzwinski-Urban M. Almost half of references in reports on new and emerging nondrug health technologies are grey literature. J Med Libr Assoc. 2019;107(1):43–8.
Piasecki J, Waligora M, Dranseika V. Google search as an additional source in systematic reviews. Sci Eng Ethics. 2018;24(2):809–10.
PubMed Google Scholar
Dax the duck. Measuring the “filter bubble”: how Google is influencing what you click. DuckDuckGo Blog 2018; https://spreadprivacy.com/google-filter-bubble-study/ .
Scherer RW, Meerpohl JJ, Pfeifer N, Schmucker C, Schwarzer G, von Elm E. Full publication of results initially presented in abstracts. Cochrane Database Syst Rev. 2018;11:MR000005.
McAuley L, Pham B, Tugwell P, Moher D. Does the inclusion of grey literature influence estimates of intervention effectiveness reported in meta-analyses? Lancet. 2000;356(9237):1228–31.
ProceedingsFirst. 2018; https://help.oclc.org/Discovery_and_Reference/FirstSearch/FirstSearch_databases/ProceedingsFirst . Accessed 28 February, 2019.
Directory of Published Papers. InterDok Media Services LLC; 2019. http://www.interdok.com/search_paper.php .
Abstract Archive. International AIDS Society; 2019. http://www.abstract-archive.org/ .
Foster MJ, Jewell ST. Assembling the pieces of a systematic review: guide for librarians. Lanham, MD: Rowman & Littlefield; 2017.
Google Scholar
Stephens RJ, Dettmer MR, Roberts BW, et al. Practice patterns and outcomes associated with early sedation depth in mechanically ventilated patients: a systematic review and meta-analysis. Crit Care Med. 2018;46(3):471–9.
Kunneman M, Gionfriddo MR, Toloza FJK, et al. Humanistic communication in the evaluation of shared decision making: a systematic review. Patient Educ Couns. 2018;102(3):452–66.
Spurling GK, Del Mar CB, Dooley L, Foxlee R, Farley R. Delayed antibiotic prescriptions for respiratory infections. Cochrane Database Syst Rev. 2017;9:CD004417.
Wright K, Golder S, Rodriguez-Lopez R. Citation searching: a systematic review case study of multiple risk behaviour interventions. BMC Med Res Methodol. 2014;14:73.
Zarychanski R, Turgeon AF, McIntyre L, Fergusson DA. Erythropoietin-receptor agonists in critically ill patients: a meta-analysis of randomized controlled trials. CMAJ. 2007;177(7):725–34.
Degenhardt L, Peacock A, Colledge S, et al. Global prevalence of injecting drug use and sociodemographic characteristics and prevalence of HIV, HBV, and HCV in people who inject drugs: a multistage systematic review. Lancet Glob Health. 2017;5(12):e1192–207.
Kirkham JJ, Dwan KM, Altman DG, et al. The impact of outcome reporting bias in randomised controlled trials on a cohort of systematic reviews. BMJ. 2010;340:c365.
Hodkinson A, Dietz KC, Lefebvre C, et al. The use of clinical study reports to enhance the quality of systematic reviews: a survey of systematic review authors. Syst Rev. 2018;7(1):117.
Musini VM, Lawrence KA, Fortin PM, Bassett K, Wright JM. Blood pressure lowering efficacy of renin inhibitors for primary hypertension. Cochrane Database Syst Rev. 2017;4:CD007066.
Samarasekera N, Smith C, Al-Shahi SR. The association between cerebral amyloid angiopathy and intracerebral haemorrhage: systematic review and meta-analysis. J Neurol Neurosurg Psychiatry. 2012;83(3):275–81.
Tham T, Machado R, Khaymovich J, Costantino P. Detection of HPV16, HPV18, p16, and E6/E7 MRNA in nasopharyngeal cancer: a systematic review and meta-analysis. bioRxiv 2018:401554. https://www.biorxiv.org/content/biorxiv/early/2018/08/27/401554.full.pdf .
Lin J, Wilbur WJ. PubMed related articles: a probabilistic topic-based model for content similarity. BMC Bioinformatics. 2007;8:423.
Clarivate Analytics. Related records. Web of Science Core Collection Help 2018; https://images.webofknowledge.com/images/help/WOS/hp_related_records.html . Accessed 1 Feb, 2020.
Bennett KG, Berlin NL, MacEachern MP, Buchman SR, Preminger BA, Vercler CJ. The ethical and professional use of social media in surgery: a systematic review of the literature. Plast Reconstr Surg. 2018;142(3):388e–98e.
Varley-Campbell J, Cooper C, Wilkerson D, Wardle S, Greeves J, Lorenc T. Sex-specific changes in physical performance following military training: a systematic review. Sports Med. 2018;48(11):2623–40.
Chandler J, Churchill R, Higgins J, Lasserson T, Tovey D. Methodological standards for the conduct of new Cochrane Intervention Reviews: version 2.1. 2011; https://sti.cochrane.org/sites/sti.cochrane.org/files/public/uploads/Methodological%20standards%20for%20the%20conduct%20of%20Cochrane%20Intervention%20Reviews.PDF . Accessed 1 Feb, 2020.
CADTH. Grey Matters: a practical tool for searching health-related grey literature. 2019; https://www.cadth.ca/resources/finding-evidence/grey-matters . Accessed 1 Feb, 2020.
Higgins J, Lasserson T, Chandler J, Tovey D, Churchill R. Methodological Expectations of Cochrane Intervention Reviews. 2019; https://community.cochrane.org/mecir-manual . Accessed 3 Jan 2020.
Kim Y. Fostering scientists’ data sharing behaviors via data repositories, journal supplements, and personal communication methods. Inform Process Manag. 2017;53(4):871–85.
National Center for Biotechnology Information. PubMed Central. 2020; https://www.ncbi.nlm.nih.gov/pmc/ . Accessed 9 Jan 2020.
Thomas LH, Coupe J, Cross LD, Tan AL, Watkins CL. Interventions for treating urinary incontinence after stroke in adults. Cochrane Database Syst Rev. 2019;2:CD004462.
Speer K, Upton D, Semple S, McKune A. Systemic low-grade inflammation in post-traumatic stress disorder: a systematic review. J Inflamm Res. 2018;11:111–21.
Rudmik L, Soler ZM. Medical therapies for adult chronic sinusitis: a systematic review. JAMA. 2015;314(9):926–39.
Joseph MS, Tincopa MA, Walden P, Jackson E, Conte ML, Rubenfire M. The impact of structured exercise programs on metabolic syndrome and its components: a systematic review. Diabetes Metab Syndr Obes. 2019;12:2395–404.
Lumba-Brown A, Yeates KO, Sarmiento K, et al. Diagnosis and management of mild traumatic brain injury in children: a systematic review. JAMA Pediatr. 2018;172(11):e182847.
Kugley S, Wade A, Thomas J, et al. Searching for studies: a guide to information retrieval for Campbell systematic reviews. Campbell Syst Rev. 2017;13(1):1–73.
Iansavichene AE, Sampson M, McGowan J, Ajiferuke IS. Should systematic reviewers search for randomized, controlled trials published as letters? Ann Intern Med. 2008;148(9):714–5.
Cooper C, Booth A, Varley-Campbell J, Britten N, Garside R. Defining the process to literature searching in systematic reviews: a literature review of guidance and supporting studies. BMC Med Res Methodol. 2018;18(1):85.
Craven J, Levay P. Recording database searches for systematic reviews - what is the value of adding a narrative to peer-review checklists? A case study of NICE interventional procedures guidance. Evid Based Libr Inf Pract. 2011;6(4):72–87.
Scottish Intercollegiate Guidelines Network (SIGN). Search filters. 2011; https://www.sign.ac.uk/search-filters.html .
Karagiannis T, Paschos P, Paletas K, Matthews DR, Tsapas A. Dipeptidyl peptidase-4 inhibitors for treatment of type 2 diabetes mellitus in the clinical setting: systematic review and meta-analysis. BMJ. 2012;344:e1369.
Article PubMed CAS Google Scholar
Lefebvre C, Glanville J, Beale S, et al. Assessing the performance of methodological search filters to improve the efficiency of evidence information retrieval: five literature reviews and a qualitative study. Health Technol Assess. 2017;21(69):1–148.
Damarell RA, May N, Hammond S, Sladek RM, Tieman JJ. Topic search filters: a systematic scoping review. Health Info Libr J. 2019;36(1):4–40.
McKibbon KA, Wilczynski NL, Haynes RB, Hedges T. Retrieving randomized controlled trials from medline: a comparison of 38 published search filters. Health Info Libr J. 2009;26(3):187–202.
InterTASC Information Specialists’ Sub-Group. The InterTASC Information Specialists’ Sub-Group Search Filter Resource. 2020; https://sites.google.com/a/york.ac.uk/issg-search-filters-resource . Accessed 1 Feb, 2020.
Health Information Research Unit. Search filters for MEDLINE in Ovid syntax and the PubMed translation. 2016; http://hiru.mcmaster.ca/hiru/HIRU_Hedges_MEDLINE_Strategies.aspx . Accessed 1 Feb, 2020.
Waters E, de Silva-Sanigorski A, Hall BJ, et al. Interventions for preventing obesity in children. Cochrane Database Syst Rev. 2011;(12):Cd00187.
Cahill K, Lancaster T. Workplace interventions for smoking cessation. Cochrane Database Syst Rev. 2014;(2):Cd003440.
Freak-Poli RL, Cumpston M, Peeters A, Clemes SA. Workplace pedometer interventions for increasing physical activity. Cochrane Database Syst Rev. 2013;(4):Cd009209.
Dobbins M, Husson H, DeCorby K, LaRocca RL. School-based physical activity programs for promoting physical activity and fitness in children and adolescents aged 6 to 18. Cochrane Database Syst Rev. 2013;(2):Cd007651.
Guerra PH, Nobre MR, da Silveira JA, Taddei JA. School-based physical activity and nutritional education interventions on body mass index: a meta-analysis of randomised community trials - project PANE. Prev Med. 2014;61:81–9.
Jaime PC, Lock K. Do school based food and nutrition policies improve diet and reduce obesity? Prev Med. 2009;48(1):45–53.
Thomas RE, McLellan J, Perera R. School-based programmes for preventing smoking. Cochrane Database Syst Rev. 2013;(4):Cd001293.
Foxcroft D, Ireland D, Lowe G, Breen R. Primary prevention for alcohol misuse in young people. Cochrane Database Syst Rev. 2011;(9):Cd003024.
Wolfenden L, Jones J, Williams CM, et al. Strategies to improve the implementation of healthy eating, physical activity and obesity prevention policies, practices or programmes within childcare services. Cochrane Database Syst Rev. 2016;10:Cd011779.
Wolfenden L, Nathan NK, Sutherland R, et al. Strategies for enhancing the implementation of school-based policies or practices targeting risk factors for chronic disease. Cochrane Database Syst Rev. 2017;11:Cd011677.
Wolfenden L, Goldman S, Stacey FG, et al. Strategies to improve the implementation of workplace-based policies or practices targeting tobacco, alcohol, diet, physical activity and obesity. Cochrane Database Syst Rev. 2018;11:CD012439.
White CM, Ip S, McPheeters M, et al. Using existing systematic reviews to replace de novo processes in conducting comparative effectiveness reviews. In: Methods Guide for Effectiveness and Comparative Effectiveness Reviews. Rockville (MD); 2008.
Lopez-Olivo MA, Tayar JH, Martinez-Lopez JA, et al. Risk of malignancies in patients with rheumatoid arthritis treated with biologic therapy: a meta-analysis. JAMA. 2012;308(9):898–908.
Sampson M, Shojania KG, Garritty C, Horsley T, Ocampo M, Moher D. Systematic reviews can be produced and published faster. J Clin Epidemiol. 2008;61(6):531–6.
Shojania KG, Sampson M, Ansari MT, Ji J, Doucette S, Moher D. How quickly do systematic reviews go out of date? A survival analysis. Ann Intern Med. 2007;147(4):224–33.
Shojania KG, Sampson M, Ansari MT, et al. Updating systematic reviews: AHRQ publication no 07-0087. AHRQ Technical Reviews 2007; 16: http://www.ncbi.nlm.nih.gov/books/NBK44099/pdf/TOC.pdf . Accessed 1 Feb, 2020.
Bhaskar V, Chan HL, MacEachern M, Kripfgans OD. Updates on ultrasound research in implant dentistry: a systematic review of potential clinical indications. Dentomaxillofac Radiol. 2018;47(6):20180076.
Beller EM, Chen JK, Wang UL, Glasziou PP. Are systematic reviews up-to-date at the time of publication? Syst Rev. 2013;2:36.
Velez MP, Hamel C, Hutton B, et al. Care plans for women pregnant using assisted reproductive technologies: a systematic review. Reprod Health. 2019;16(1):9.
Relevo R, Paynter R. Peer review of search strategies. AHRQ Methods for Effective Health Care 2012; https://www.ncbi.nlm.nih.gov/books/NBK98353/ .
Spry C, Mierzwinski-Urban M. The impact of the peer review of literature search strategies in support of rapid review reports. Res Synth Methods. 2018;9(4):521–6.
Banno M, Harada Y, Taniguchi M, et al. Exercise can improve sleep quality: a systematic review and meta-analysis. PeerJ. 2018;6:e5172.
Tsamalaidze L, Stauffer JA, Brigham T, Asbun HJ. Postsplenectomy thrombosis of splenic, mesenteric, and portal vein (PST-SMPv): a single institutional series, comprehensive systematic review of a literature and suggested classification. Am J Surg. 2018;216(6):1192–204.
Barakat S, Boehmer K, Abdelrahim M, et al. Does health coaching grow capacity in cancer survivors? A systematic review. Popul Health Manag. 2018;21(1):63–81.
Qi X, Yang M, Ren W, et al. Find duplicates among the PubMed, EMBASE, and Cochrane Library Databases in systematic review. PLoS One. 2013;8(8):e71838.
Bramer WM, Giustini D, de Jonge GB, Holland L, Bekhuis T. De-duplication of database search results for systematic reviews in EndNote. J Med Libr Assoc. 2016;104(3):240–3.
Rathbone J, Carter M, Hoffmann T, Glasziou P. Better duplicate detection for systematic reviewers: evaluation of Systematic Review Assistant-Deduplication Module. Syst Rev. 2015;4:6.
Kwon Y, Lemieux M, McTavish J, Wathen N. Identifying and removing duplicate records from systematic review searches. J Med Libr Assoc. 2015;103(4):184–8.
Tricco AC, Lillie E, Zarin W, et al. PRISMA extension for Scoping Reviews (PRISMA-ScR): checklist and explanation. Ann Intern Med. 2018;169(7):467–73.
Hutton B, Salanti G, Caldwell DM, et al. The PRISMA extension statement for reporting of systematic reviews incorporating network meta-analyses of health care interventions: checklist and explanations. Ann Intern Med. 2015;162(11):777–84.
Stewart LA, Clarke M, Rovers M, et al. Preferred Reporting Items for Systematic Review and Meta-Analyses of individual participant data: the PRISMA-IPD Statement. JAMA. 2015;313(16):1657–65.
Haddaway NR, Macura B, Whaley P, Pullin AS. ROSES RepOrting standards for Systematic Evidence Syntheses: pro forma, flow-diagram and descriptive summary of the plan and conduct of environmental systematic reviews and systematic maps. Environ Evid. 2018;7(1):7.
Stansfield C, O'Mara-Eves A, Thomas J. Text mining for search term development in systematic reviewing: a discussion of some methods and challenges. Res Synth Methods. 2017;8(3):355–65.
Hausner E, Guddat C, Hermanns T, Lampert U, Waffenschmidt S. Prospective comparison of search strategies for systematic reviews: an objective approach yielded higher sensitivity than a conceptual one. J Clin Epidemiol. 2016;77:118–24.
Paynter R, Banez LL, Berliner E, et al. EPC Methods: an exploration of the use of text-mining software in systematic reviews. 2016; https://www.ncbi.nlm.nih.gov/books/NBK362044/ . Accessed 3 Feb,2020.
Download references
Acknowledgements
We would like to thank all of the members of the PRISMA-S Group, which is comprised of participants in the Delphi process, consensus conference, or both. PRISMA-S Group members include Heather Blunt (Dartmouth College), Tara Brigham (Mayo Clinic in Florida), Steven Chang (La Trobe University), Justin Clark (Bond University), Aislinn Conway (BORN Ontario and CHEO Research Institute), Rachel Couban (McMaster University), Shelley de Kock (Kleijnen Systematic Reviews Ltd), Kelly Farrah (Canadian Agency for Drugs and Technologies in Health (CADTH)), Paul Fehrmann (Kent State University), Margaret Foster (Texas A & M University), Susan A. Fowler (Washington University in St. Louis), Julie Glanville (University of York), Elizabeth Harris (La Trobe University), Lilian Hoffecker (University of Colorado Denver), Jaana Isojarvi (Tampere University), David Kaunelis (Canadian Agency for Drugs and Technologies in Health (CADTH)), Hans Ket (VU Amsterdam), Paul Levay (National Institute for Health and Care Excellence (NICE)), Jennifer Lyon, Jessie McGowan (uOttawa), M. Hassan Murad (Mayo Clinic), Joey Nicholson (NYU Langone Health), Virginia Pannabecker (Virginia Tech), Robin Paynter (VA Portland Health Care System), Rachel Pinotti (Icahn School of Medicine at Mount Sinai), Amanda Ross-White (Queens University), Margaret Sampson (CHEO), Tracy Shields (Naval Medical Center Portsmouth), Adrienne Stevens (Ottawa Hospital Research Institute), Anthea Sutton (University of Sheffield), Elizabeth Weinfurter (University of Minnesota), Kath Wright (University of York), and Sarah Young (Carnegie Mellon University). We would also like to thank Kate Nyhan (Yale University), Katharina Gronostay (IQWiG), the many others who contributed to the PRISMA-S project anonymously or as draft reviewers, and our peer reviewers. We would like to give special thanks to the late Douglas G. Altman (D.G.A.; University of Oxford) for his support and guidance, and the co-chairs of the Medical Library Association’s Systematic Reviews SIG in 2016, Margaret Foster (Texas A & M University) and Susan Fowler (Washington University in St. Louis), for allowing us to use one of their meeting times for the consensus conference.
Melissa Rethlefsen was funded in part by the University of Utah’s Center for Clinical and Translational Science under the National Center for Advancing Translational Sciences of the National Institutes of Health Award Number UL1TR002538 in 2017–2018 . The content is solely the responsibility of the authors and does not necessarily represent the official views of the National Institutes of Health.
Shona Kirtley was funded by the Cancer Research UK (grant C49297/A27294). The funder had no role in the study design, data collection and analysis, decision to publish, or preparation of the manuscript. The views expressed are those of the authors and not necessarily those of the Cancer Research UK.
Matthew Page is supported by an Australian Research Council Discovery Early Career Researcher Award (DE200101618).
David Moher is supported by a University Research Chair, University of Ottawa, Ottawa, Canada.
The consensus conference was sponsored by the Systematic Reviews SIG of the Medical Library Association. There was no specific funding associated with this event.
Author information
Authors and affiliations.
Health Science Center Libraries, George A. Smathers Libraries, University of Florida, Gainesville, USA
Melissa L. Rethlefsen
UK EQUATOR Centre, Centre for Statistics in Medicine (CSM), Nuffield Department of Orthopaedics, Rheumatology and Musculoskeletal Sciences (NDORMS), Botnar Research Centre, University of Oxford, Windmill Road, Oxford, OX3 7LD, UK
Shona Kirtley
Institute for Quality and Efficiency in Health Care, Cologne, Germany
Siw Waffenschmidt
Gerstein Science Information Centre, University of Toronto, Toronto, Canada
Ana Patricia Ayala
Centre for Journalology, Clinical Epidemiology Program, Ottawa Hospital Research Institute, The Ottawa Hospital, General Campus, Centre for Practice Changing Research Building, 501 Smyth Road, PO BOX 201B, Ottawa, Ontario, K1H 8L6, Canada
David Moher
School of Public Health and Preventive Medicine, Monash University, Melbourne, Australia
Matthew J. Page
University of Minnesota, Minneapolis, USA
Jonathan B. Koffel
You can also search for this author in PubMed Google Scholar
- Heather Blunt
- , Tara Brigham
- , Steven Chang
- , Justin Clark
- , Aislinn Conway
- , Rachel Couban
- , Shelley de Kock
- , Kelly Farrah
- , Paul Fehrmann
- , Margaret Foster
- , Susan A. Fowler
- , Julie Glanville
- , Elizabeth Harris
- , Lilian Hoffecker
- , Jaana Isojarvi
- , David Kaunelis
- , Paul Levay
- , Jennifer Lyon
- , Jessie McGowan
- , M. Hassan Murad
- , Joey Nicholson
- , Virginia Pannabecker
- , Robin Paynter
- , Rachel Pinotti
- , Amanda Ross-White
- , Margaret Sampson
- , Tracy Shields
- , Adrienne Stevens
- , Anthea Sutton
- , Elizabeth Weinfurter
- , Kath Wright
- & Sarah Young
Contributions
M.L.R. conceived and designed the study, conducted the thematic and quantitative analyses, curated the data, drafted the manuscript, and reviewed and edited the manuscript. M.L.R. is the guarantor. J.B.K. and S.K. contributed to the design of the study, developed the literature search strategies, contributed to the thematic content analyses, drafted a portion of the Elaboration & Explanation, and reviewed and edited the manuscript. J.B.K. developed the survey instrument. M.L.R., J.B.K., and S.K. hosted and organized the consensus conference. S.W. and A.P.A. contributed to the thematic content analysis, drafted a portion of the Elaboration & Explanation, and reviewed and edited the manuscript. S.W. supervised the draft revision documentation. D.M. helped conceive and design the study. M.J.P. provided substantive review and editing of the checklist, Explanation & Elaboration, and final manuscript. The author (s) read and approved the final manuscript.
Corresponding author
Correspondence to Melissa L. Rethlefsen .
Ethics declarations
Ethics approval and consent to participate.
This study was declared exempt by the University of Utah Institutional Review Board (IRB_00088425). Consent was received from all survey participants.
Consent for publication
Not applicable
Competing interests
The authors declare no competing interests. MJP and DM are leading the PRISMA 2020 update.
Additional information
Publisher’s note.
Springer Nature remains neutral with regard to jurisdictional claims in published maps and institutional affiliations.
Supplementary Information
Additional file 1., rights and permissions.
Open Access This article is licensed under a Creative Commons Attribution 4.0 International License, which permits use, sharing, adaptation, distribution and reproduction in any medium or format, as long as you give appropriate credit to the original author(s) and the source, provide a link to the Creative Commons licence, and indicate if changes were made. The images or other third party material in this article are included in the article's Creative Commons licence, unless indicated otherwise in a credit line to the material. If material is not included in the article's Creative Commons licence and your intended use is not permitted by statutory regulation or exceeds the permitted use, you will need to obtain permission directly from the copyright holder. To view a copy of this licence, visit http://creativecommons.org/licenses/by/4.0/ . The Creative Commons Public Domain Dedication waiver ( http://creativecommons.org/publicdomain/zero/1.0/ ) applies to the data made available in this article, unless otherwise stated in a credit line to the data.
Reprints and permissions
About this article
Cite this article.
Rethlefsen, M.L., Kirtley, S., Waffenschmidt, S. et al. PRISMA-S: an extension to the PRISMA Statement for Reporting Literature Searches in Systematic Reviews. Syst Rev 10 , 39 (2021). https://doi.org/10.1186/s13643-020-01542-z
Download citation
Received : 28 February 2020
Accepted : 23 November 2020
Published : 26 January 2021
DOI : https://doi.org/10.1186/s13643-020-01542-z
Share this article
Anyone you share the following link with will be able to read this content:
Sorry, a shareable link is not currently available for this article.
Provided by the Springer Nature SharedIt content-sharing initiative
- Systematic reviews
- Reporting guidelines
- Search strategies
- Literature search
- Information retrieval
- Reproducibility
Systematic Reviews
ISSN: 2046-4053
- Submission enquiries: Access here and click Contact Us
- General enquiries: [email protected]

An official website of the United States government
The .gov means it’s official. Federal government websites often end in .gov or .mil. Before sharing sensitive information, make sure you’re on a federal government site.
The site is secure. The https:// ensures that you are connecting to the official website and that any information you provide is encrypted and transmitted securely.
- Publications
- Account settings
Preview improvements coming to the PMC website in October 2024. Learn More or Try it out now .
- Advanced Search
- Journal List
- v.372; 2021
The PRISMA 2020 statement: an updated guideline for reporting systematic reviews
Matthew j page.
1 School of Public Health and Preventive Medicine, Monash University, Melbourne, Australia
Joanne E McKenzie
Patrick m bossuyt.
2 Department of Clinical Epidemiology, Biostatistics and Bioinformatics, Amsterdam University Medical Centres, University of Amsterdam, Amsterdam, Netherlands
Isabelle Boutron
3 Université de Paris, Centre of Epidemiology and Statistics (CRESS), Inserm, F 75004 Paris, France
Tammy C Hoffmann
4 Institute for Evidence-Based Healthcare, Faculty of Health Sciences and Medicine, Bond University, Gold Coast, Australia
Cynthia D Mulrow
5 University of Texas Health Science Center at San Antonio, San Antonio, Texas, USA; Annals of Internal Medicine
Larissa Shamseer
6 Knowledge Translation Program, Li Ka Shing Knowledge Institute, Toronto, Canada; School of Epidemiology and Public Health, Faculty of Medicine, University of Ottawa, Ottawa, Canada
Jennifer M Tetzlaff
7 Evidence Partners, Ottawa, Canada
8 Clinical Research Institute, American University of Beirut, Beirut, Lebanon; Department of Health Research Methods, Evidence, and Impact, McMaster University, Hamilton, Ontario, Canada
Sue E Brennan
9 Department of Medical Informatics and Clinical Epidemiology, Oregon Health & Science University, Portland, Oregon, USA
Julie Glanville
10 York Health Economics Consortium (YHEC Ltd), University of York, York, UK
Jeremy M Grimshaw
11 Clinical Epidemiology Program, Ottawa Hospital Research Institute, Ottawa, Canada; School of Epidemiology and Public Health, University of Ottawa, Ottawa, Canada; Department of Medicine, University of Ottawa, Ottawa, Canada
Asbjørn Hróbjartsson
12 Centre for Evidence-Based Medicine Odense (CEBMO) and Cochrane Denmark, Department of Clinical Research, University of Southern Denmark, Odense, Denmark; Open Patient data Exploratory Network (OPEN), Odense University Hospital, Odense, Denmark
Manoj M Lalu
13 Department of Anesthesiology and Pain Medicine, The Ottawa Hospital, Ottawa, Canada; Clinical Epidemiology Program, Blueprint Translational Research Group, Ottawa Hospital Research Institute, Ottawa, Canada; Regenerative Medicine Program, Ottawa Hospital Research Institute, Ottawa, Canada
Tianjing Li
14 Department of Ophthalmology, School of Medicine, University of Colorado Denver, Denver, Colorado, United States; Department of Epidemiology, Johns Hopkins Bloomberg School of Public Health, Baltimore, Maryland, USA
Elizabeth W Loder
15 Division of Headache, Department of Neurology, Brigham and Women's Hospital, Harvard Medical School, Boston, Massachusetts, USA; Head of Research, The BMJ , London, UK
Evan Mayo-Wilson
16 Department of Epidemiology and Biostatistics, Indiana University School of Public Health-Bloomington, Bloomington, Indiana, USA
Steve McDonald
Luke a mcguinness.
17 Population Health Sciences, Bristol Medical School, University of Bristol, Bristol, UK
Lesley A Stewart
18 Centre for Reviews and Dissemination, University of York, York, UK
James Thomas
19 EPPI-Centre, UCL Social Research Institute, University College London, London, UK
Andrea C Tricco
20 Li Ka Shing Knowledge Institute of St. Michael's Hospital, Unity Health Toronto, Toronto, Canada; Epidemiology Division of the Dalla Lana School of Public Health and the Institute of Health Management, Policy, and Evaluation, University of Toronto, Toronto, Canada; Queen's Collaboration for Health Care Quality Joanna Briggs Institute Centre of Excellence, Queen's University, Kingston, Canada
Vivian A Welch
21 Methods Centre, Bruyère Research Institute, Ottawa, Ontario, Canada; School of Epidemiology and Public Health, Faculty of Medicine, University of Ottawa, Ottawa, Canada
Penny Whiting
David moher.
22 Centre for Journalology, Clinical Epidemiology Program, Ottawa Hospital Research Institute, Ottawa, Canada; School of Epidemiology and Public Health, Faculty of Medicine, University of Ottawa, Ottawa, Canada
Associated Data
The Preferred Reporting Items for Systematic reviews and Meta-Analyses (PRISMA) statement, published in 2009, was designed to help systematic reviewers transparently report why the review was done, what the authors did, and what they found. Over the past decade, advances in systematic review methodology and terminology have necessitated an update to the guideline. The PRISMA 2020 statement replaces the 2009 statement and includes new reporting guidance that reflects advances in methods to identify, select, appraise, and synthesise studies. The structure and presentation of the items have been modified to facilitate implementation. In this article, we present the PRISMA 2020 27-item checklist, an expanded checklist that details reporting recommendations for each item, the PRISMA 2020 abstract checklist, and the revised flow diagrams for original and updated reviews.
Systematic reviews serve many critical roles. They can provide syntheses of the state of knowledge in a field, from which future research priorities can be identified; they can address questions that otherwise could not be answered by individual studies; they can identify problems in primary research that should be rectified in future studies; and they can generate or evaluate theories about how or why phenomena occur. Systematic reviews therefore generate various types of knowledge for different users of reviews (such as patients, healthcare providers, researchers, and policy makers). 1 2 To ensure a systematic review is valuable to users, authors should prepare a transparent, complete, and accurate account of why the review was done, what they did (such as how studies were identified and selected) and what they found (such as characteristics of contributing studies and results of meta-analyses). Up-to-date reporting guidance facilitates authors achieving this. 3
The Preferred Reporting Items for Systematic reviews and Meta-Analyses (PRISMA) statement published in 2009 (hereafter referred to as PRISMA 2009) 4 5 6 7 8 9 10 is a reporting guideline designed to address poor reporting of systematic reviews. 11 The PRISMA 2009 statement comprised a checklist of 27 items recommended for reporting in systematic reviews and an “explanation and elaboration” paper 12 13 14 15 16 providing additional reporting guidance for each item, along with exemplars of reporting. The recommendations have been widely endorsed and adopted, as evidenced by its co-publication in multiple journals, citation in over 60 000 reports (Scopus, August 2020), endorsement from almost 200 journals and systematic review organisations, and adoption in various disciplines. Evidence from observational studies suggests that use of the PRISMA 2009 statement is associated with more complete reporting of systematic reviews, 17 18 19 20 although more could be done to improve adherence to the guideline. 21
Many innovations in the conduct of systematic reviews have occurred since publication of the PRISMA 2009 statement. For example, technological advances have enabled the use of natural language processing and machine learning to identify relevant evidence, 22 23 24 methods have been proposed to synthesise and present findings when meta-analysis is not possible or appropriate, 25 26 27 and new methods have been developed to assess the risk of bias in results of included studies. 28 29 Evidence on sources of bias in systematic reviews has accrued, culminating in the development of new tools to appraise the conduct of systematic reviews. 30 31 Terminology used to describe particular review processes has also evolved, as in the shift from assessing “quality” to assessing “certainty” in the body of evidence. 32 In addition, the publishing landscape has transformed, with multiple avenues now available for registering and disseminating systematic review protocols, 33 34 disseminating reports of systematic reviews, and sharing data and materials, such as preprint servers and publicly accessible repositories. To capture these advances in the reporting of systematic reviews necessitated an update to the PRISMA 2009 statement.
Summary points
- To ensure a systematic review is valuable to users, authors should prepare a transparent, complete, and accurate account of why the review was done, what they did, and what they found
- The PRISMA 2020 statement provides updated reporting guidance for systematic reviews that reflects advances in methods to identify, select, appraise, and synthesise studies
- The PRISMA 2020 statement consists of a 27-item checklist, an expanded checklist that details reporting recommendations for each item, the PRISMA 2020 abstract checklist, and revised flow diagrams for original and updated reviews
- We anticipate that the PRISMA 2020 statement will benefit authors, editors, and peer reviewers of systematic reviews, and different users of reviews, including guideline developers, policy makers, healthcare providers, patients, and other stakeholders
Development of PRISMA 2020
A complete description of the methods used to develop PRISMA 2020 is available elsewhere. 35 We identified PRISMA 2009 items that were often reported incompletely by examining the results of studies investigating the transparency of reporting of published reviews. 17 21 36 37 We identified possible modifications to the PRISMA 2009 statement by reviewing 60 documents providing reporting guidance for systematic reviews (including reporting guidelines, handbooks, tools, and meta-research studies). 38 These reviews of the literature were used to inform the content of a survey with suggested possible modifications to the 27 items in PRISMA 2009 and possible additional items. Respondents were asked whether they believed we should keep each PRISMA 2009 item as is, modify it, or remove it, and whether we should add each additional item. Systematic review methodologists and journal editors were invited to complete the online survey (110 of 220 invited responded). We discussed proposed content and wording of the PRISMA 2020 statement, as informed by the review and survey results, at a 21-member, two-day, in-person meeting in September 2018 in Edinburgh, Scotland. Throughout 2019 and 2020, we circulated an initial draft and five revisions of the checklist and explanation and elaboration paper to co-authors for feedback. In April 2020, we invited 22 systematic reviewers who had expressed interest in providing feedback on the PRISMA 2020 checklist to share their views (via an online survey) on the layout and terminology used in a preliminary version of the checklist. Feedback was received from 15 individuals and considered by the first author, and any revisions deemed necessary were incorporated before the final version was approved and endorsed by all co-authors.
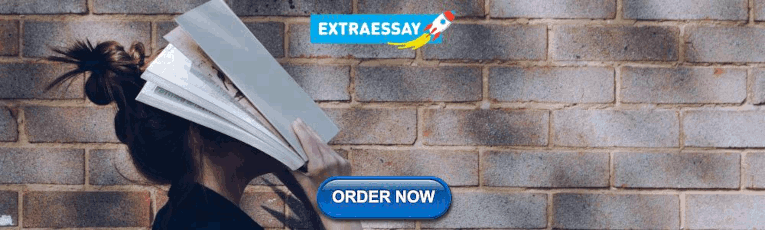
The PRISMA 2020 statement
Scope of the guideline.
The PRISMA 2020 statement has been designed primarily for systematic reviews of studies that evaluate the effects of health interventions, irrespective of the design of the included studies. However, the checklist items are applicable to reports of systematic reviews evaluating other interventions (such as social or educational interventions), and many items are applicable to systematic reviews with objectives other than evaluating interventions (such as evaluating aetiology, prevalence, or prognosis). PRISMA 2020 is intended for use in systematic reviews that include synthesis (such as pairwise meta-analysis or other statistical synthesis methods) or do not include synthesis (for example, because only one eligible study is identified). The PRISMA 2020 items are relevant for mixed-methods systematic reviews (which include quantitative and qualitative studies), but reporting guidelines addressing the presentation and synthesis of qualitative data should also be consulted. 39 40 PRISMA 2020 can be used for original systematic reviews, updated systematic reviews, or continually updated (“living”) systematic reviews. However, for updated and living systematic reviews, there may be some additional considerations that need to be addressed. Where there is relevant content from other reporting guidelines, we reference these guidelines within the items in the explanation and elaboration paper 41 (such as PRISMA-Search 42 in items 6 and 7, Synthesis without meta-analysis (SWiM) reporting guideline 27 in item 13d). Box 1 includes a glossary of terms used throughout the PRISMA 2020 statement.
Glossary of terms
- Systematic review —A review that uses explicit, systematic methods to collate and synthesise findings of studies that address a clearly formulated question 43
- Statistical synthesis —The combination of quantitative results of two or more studies. This encompasses meta-analysis of effect estimates (described below) and other methods, such as combining P values, calculating the range and distribution of observed effects, and vote counting based on the direction of effect (see McKenzie and Brennan 25 for a description of each method)
- Meta-analysis of effect estimates —A statistical technique used to synthesise results when study effect estimates and their variances are available, yielding a quantitative summary of results 25
- Outcome —An event or measurement collected for participants in a study (such as quality of life, mortality)
- Result —The combination of a point estimate (such as a mean difference, risk ratio, or proportion) and a measure of its precision (such as a confidence/credible interval) for a particular outcome
- Report —A document (paper or electronic) supplying information about a particular study. It could be a journal article, preprint, conference abstract, study register entry, clinical study report, dissertation, unpublished manuscript, government report, or any other document providing relevant information
- Record —The title or abstract (or both) of a report indexed in a database or website (such as a title or abstract for an article indexed in Medline). Records that refer to the same report (such as the same journal article) are “duplicates”; however, records that refer to reports that are merely similar (such as a similar abstract submitted to two different conferences) should be considered unique.
- Study —An investigation, such as a clinical trial, that includes a defined group of participants and one or more interventions and outcomes. A “study” might have multiple reports. For example, reports could include the protocol, statistical analysis plan, baseline characteristics, results for the primary outcome, results for harms, results for secondary outcomes, and results for additional mediator and moderator analyses
PRISMA 2020 is not intended to guide systematic review conduct, for which comprehensive resources are available. 43 44 45 46 However, familiarity with PRISMA 2020 is useful when planning and conducting systematic reviews to ensure that all recommended information is captured. PRISMA 2020 should not be used to assess the conduct or methodological quality of systematic reviews; other tools exist for this purpose. 30 31 Furthermore, PRISMA 2020 is not intended to inform the reporting of systematic review protocols, for which a separate statement is available (PRISMA for Protocols (PRISMA-P) 2015 statement 47 48 ). Finally, extensions to the PRISMA 2009 statement have been developed to guide reporting of network meta-analyses, 49 meta-analyses of individual participant data, 50 systematic reviews of harms, 51 systematic reviews of diagnostic test accuracy studies, 52 and scoping reviews 53 ; for these types of reviews we recommend authors report their review in accordance with the recommendations in PRISMA 2020 along with the guidance specific to the extension.
How to use PRISMA 2020
The PRISMA 2020 statement (including the checklists, explanation and elaboration, and flow diagram) replaces the PRISMA 2009 statement, which should no longer be used. Box 2 summarises noteworthy changes from the PRISMA 2009 statement. The PRISMA 2020 checklist includes seven sections with 27 items, some of which include sub-items ( table 1 ). A checklist for journal and conference abstracts for systematic reviews is included in PRISMA 2020. This abstract checklist is an update of the 2013 PRISMA for Abstracts statement, 54 reflecting new and modified content in PRISMA 2020 ( table 2 ). A template PRISMA flow diagram is provided, which can be modified depending on whether the systematic review is original or updated ( fig 1 ).
Noteworthy changes to the PRISMA 2009 statement
- Inclusion of the abstract reporting checklist within PRISMA 2020 (see item #2 and table 2 ).
- Movement of the ‘Protocol and registration’ item from the start of the Methods section of the checklist to a new Other section, with addition of a sub-item recommending authors describe amendments to information provided at registration or in the protocol (see item #24a-24c).
- Modification of the ‘Search’ item to recommend authors present full search strategies for all databases, registers and websites searched, not just at least one database (see item #7).
- Modification of the ‘Study selection’ item in the Methods section to emphasise the reporting of how many reviewers screened each record and each report retrieved, whether they worked independently, and if applicable, details of automation tools used in the process (see item #8).
- Addition of a sub-item to the ‘Data items’ item recommending authors report how outcomes were defined, which results were sought, and methods for selecting a subset of results from included studies (see item #10a).
- Splitting of the ‘Synthesis of results’ item in the Methods section into six sub-items recommending authors describe: the processes used to decide which studies were eligible for each synthesis; any methods required to prepare the data for synthesis; any methods used to tabulate or visually display results of individual studies and syntheses; any methods used to synthesise results; any methods used to explore possible causes of heterogeneity among study results (such as subgroup analysis, meta-regression); and any sensitivity analyses used to assess robustness of the synthesised results (see item #13a-13f).
- Addition of a sub-item to the ‘Study selection’ item in the Results section recommending authors cite studies that might appear to meet the inclusion criteria, but which were excluded, and explain why they were excluded (see item #16b).
- Splitting of the ‘Synthesis of results’ item in the Results section into four sub-items recommending authors: briefly summarise the characteristics and risk of bias among studies contributing to the synthesis; present results of all statistical syntheses conducted; present results of any investigations of possible causes of heterogeneity among study results; and present results of any sensitivity analyses (see item #20a-20d).
- Addition of new items recommending authors report methods for and results of an assessment of certainty (or confidence) in the body of evidence for an outcome (see items #15 and #22).
- Addition of a new item recommending authors declare any competing interests (see item #26).
- Addition of a new item recommending authors indicate whether data, analytic code and other materials used in the review are publicly available and if so, where they can be found (see item #27).
PRISMA 2020 item checklist
PRISMA 2020 for Abstracts checklist*

PRISMA 2020 flow diagram template for systematic reviews. The new design is adapted from flow diagrams proposed by Boers, 55 Mayo-Wilson et al. 56 and Stovold et al. 57 The boxes in grey should only be completed if applicable; otherwise they should be removed from the flow diagram. Note that a “report” could be a journal article, preprint, conference abstract, study register entry, clinical study report, dissertation, unpublished manuscript, government report or any other document providing relevant information.
We recommend authors refer to PRISMA 2020 early in the writing process, because prospective consideration of the items may help to ensure that all the items are addressed. To help keep track of which items have been reported, the PRISMA statement website ( http://www.prisma-statement.org/ ) includes fillable templates of the checklists to download and complete (also available in the data supplement on bmj.com). We have also created a web application that allows users to complete the checklist via a user-friendly interface 58 (available at https://prisma.shinyapps.io/checklist/ and adapted from the Transparency Checklist app 59 ). The completed checklist can be exported to Word or PDF. Editable templates of the flow diagram can also be downloaded from the PRISMA statement website.
We have prepared an updated explanation and elaboration paper, in which we explain why reporting of each item is recommended and present bullet points that detail the reporting recommendations (which we refer to as elements). 41 The bullet-point structure is new to PRISMA 2020 and has been adopted to facilitate implementation of the guidance. 60 61 An expanded checklist, which comprises an abridged version of the elements presented in the explanation and elaboration paper, with references and some examples removed, is available in the data supplement on bmj.com. Consulting the explanation and elaboration paper is recommended if further clarity or information is required.
Journals and publishers might impose word and section limits, and limits on the number of tables and figures allowed in the main report. In such cases, if the relevant information for some items already appears in a publicly accessible review protocol, referring to the protocol may suffice. Alternatively, placing detailed descriptions of the methods used or additional results (such as for less critical outcomes) in supplementary files is recommended. Ideally, supplementary files should be deposited to a general-purpose or institutional open-access repository that provides free and permanent access to the material (such as Open Science Framework, Dryad, figshare). A reference or link to the additional information should be included in the main report. Finally, although PRISMA 2020 provides a template for where information might be located, the suggested location should not be seen as prescriptive; the guiding principle is to ensure the information is reported.
Use of PRISMA 2020 has the potential to benefit many stakeholders. Complete reporting allows readers to assess the appropriateness of the methods, and therefore the trustworthiness of the findings. Presenting and summarising characteristics of studies contributing to a synthesis allows healthcare providers and policy makers to evaluate the applicability of the findings to their setting. Describing the certainty in the body of evidence for an outcome and the implications of findings should help policy makers, managers, and other decision makers formulate appropriate recommendations for practice or policy. Complete reporting of all PRISMA 2020 items also facilitates replication and review updates, as well as inclusion of systematic reviews in overviews (of systematic reviews) and guidelines, so teams can leverage work that is already done and decrease research waste. 36 62 63
We updated the PRISMA 2009 statement by adapting the EQUATOR Network’s guidance for developing health research reporting guidelines. 64 We evaluated the reporting completeness of published systematic reviews, 17 21 36 37 reviewed the items included in other documents providing guidance for systematic reviews, 38 surveyed systematic review methodologists and journal editors for their views on how to revise the original PRISMA statement, 35 discussed the findings at an in-person meeting, and prepared this document through an iterative process. Our recommendations are informed by the reviews and survey conducted before the in-person meeting, theoretical considerations about which items facilitate replication and help users assess the risk of bias and applicability of systematic reviews, and co-authors’ experience with authoring and using systematic reviews.
Various strategies to increase the use of reporting guidelines and improve reporting have been proposed. They include educators introducing reporting guidelines into graduate curricula to promote good reporting habits of early career scientists 65 ; journal editors and regulators endorsing use of reporting guidelines 18 ; peer reviewers evaluating adherence to reporting guidelines 61 66 ; journals requiring authors to indicate where in their manuscript they have adhered to each reporting item 67 ; and authors using online writing tools that prompt complete reporting at the writing stage. 60 Multi-pronged interventions, where more than one of these strategies are combined, may be more effective (such as completion of checklists coupled with editorial checks). 68 However, of 31 interventions proposed to increase adherence to reporting guidelines, the effects of only 11 have been evaluated, mostly in observational studies at high risk of bias due to confounding. 69 It is therefore unclear which strategies should be used. Future research might explore barriers and facilitators to the use of PRISMA 2020 by authors, editors, and peer reviewers, designing interventions that address the identified barriers, and evaluating those interventions using randomised trials. To inform possible revisions to the guideline, it would also be valuable to conduct think-aloud studies 70 to understand how systematic reviewers interpret the items, and reliability studies to identify items where there is varied interpretation of the items.
We encourage readers to submit evidence that informs any of the recommendations in PRISMA 2020 (via the PRISMA statement website: http://www.prisma-statement.org/ ). To enhance accessibility of PRISMA 2020, several translations of the guideline are under way (see available translations at the PRISMA statement website). We encourage journal editors and publishers to raise awareness of PRISMA 2020 (for example, by referring to it in journal “Instructions to authors”), endorsing its use, advising editors and peer reviewers to evaluate submitted systematic reviews against the PRISMA 2020 checklists, and making changes to journal policies to accommodate the new reporting recommendations. We recommend existing PRISMA extensions 47 49 50 51 52 53 71 72 be updated to reflect PRISMA 2020 and advise developers of new PRISMA extensions to use PRISMA 2020 as the foundation document.
We anticipate that the PRISMA 2020 statement will benefit authors, editors, and peer reviewers of systematic reviews, and different users of reviews, including guideline developers, policy makers, healthcare providers, patients, and other stakeholders. Ultimately, we hope that uptake of the guideline will lead to more transparent, complete, and accurate reporting of systematic reviews, thus facilitating evidence based decision making.
Acknowledgments
We dedicate this paper to the late Douglas G Altman and Alessandro Liberati, whose contributions were fundamental to the development and implementation of the original PRISMA statement.
We thank the following contributors who completed the survey to inform discussions at the development meeting: Xavier Armoiry, Edoardo Aromataris, Ana Patricia Ayala, Ethan M Balk, Virginia Barbour, Elaine Beller, Jesse A Berlin, Lisa Bero, Zhao-Xiang Bian, Jean Joel Bigna, Ferrán Catalá-López, Anna Chaimani, Mike Clarke, Tammy Clifford, Ioana A Cristea, Miranda Cumpston, Sofia Dias, Corinna Dressler, Ivan D Florez, Joel J Gagnier, Chantelle Garritty, Long Ge, Davina Ghersi, Sean Grant, Gordon Guyatt, Neal R Haddaway, Julian PT Higgins, Sally Hopewell, Brian Hutton, Jamie J Kirkham, Jos Kleijnen, Julia Koricheva, Joey SW Kwong, Toby J Lasserson, Julia H Littell, Yoon K Loke, Malcolm R Macleod, Chris G Maher, Ana Marušic, Dimitris Mavridis, Jessie McGowan, Matthew DF McInnes, Philippa Middleton, Karel G Moons, Zachary Munn, Jane Noyes, Barbara Nußbaumer-Streit, Donald L Patrick, Tatiana Pereira-Cenci, Ba’ Pham, Bob Phillips, Dawid Pieper, Michelle Pollock, Daniel S Quintana, Drummond Rennie, Melissa L Rethlefsen, Hannah R Rothstein, Maroeska M Rovers, Rebecca Ryan, Georgia Salanti, Ian J Saldanha, Margaret Sampson, Nancy Santesso, Rafael Sarkis-Onofre, Jelena Savović, Christopher H Schmid, Kenneth F Schulz, Guido Schwarzer, Beverley J Shea, Paul G Shekelle, Farhad Shokraneh, Mark Simmonds, Nicole Skoetz, Sharon E Straus, Anneliese Synnot, Emily E Tanner-Smith, Brett D Thombs, Hilary Thomson, Alexander Tsertsvadze, Peter Tugwell, Tari Turner, Lesley Uttley, Jeffrey C Valentine, Matt Vassar, Areti Angeliki Veroniki, Meera Viswanathan, Cole Wayant, Paul Whaley, and Kehu Yang. We thank the following contributors who provided feedback on a preliminary version of the PRISMA 2020 checklist: Jo Abbott, Fionn Büttner, Patricia Correia-Santos, Victoria Freeman, Emily A Hennessy, Rakibul Islam, Amalia (Emily) Karahalios, Kasper Krommes, Andreas Lundh, Dafne Port Nascimento, Davina Robson, Catherine Schenck-Yglesias, Mary M Scott, Sarah Tanveer and Pavel Zhelnov. We thank Abigail H Goben, Melissa L Rethlefsen, Tanja Rombey, Anna Scott, and Farhad Shokraneh for their helpful comments on the preprints of the PRISMA 2020 papers. We thank Edoardo Aromataris, Stephanie Chang, Toby Lasserson and David Schriger for their helpful peer review comments on the PRISMA 2020 papers.
Web Extra.
Extra material supplied by the author
PRISMA 2020 checklist
PRISMA 2020 expanded checklist
Contributors: JEM and DM are joint senior authors. MJP, JEM, PMB, IB, TCH, CDM, LS, and DM conceived this paper and designed the literature review and survey conducted to inform the guideline content. MJP conducted the literature review, administered the survey and analysed the data for both. MJP prepared all materials for the development meeting. MJP and JEM presented proposals at the development meeting. All authors except for TCH, JMT, EAA, SEB, and LAM attended the development meeting. MJP and JEM took and consolidated notes from the development meeting. MJP and JEM led the drafting and editing of the article. JEM, PMB, IB, TCH, LS, JMT, EAA, SEB, RC, JG, AH, TL, EMW, SM, LAM, LAS, JT, ACT, PW, and DM drafted particular sections of the article. All authors were involved in revising the article critically for important intellectual content. All authors approved the final version of the article. MJP is the guarantor of this work. The corresponding author attests that all listed authors meet authorship criteria and that no others meeting the criteria have been omitted.
Funding: There was no direct funding for this research. MJP is supported by an Australian Research Council Discovery Early Career Researcher Award (DE200101618) and was previously supported by an Australian National Health and Medical Research Council (NHMRC) Early Career Fellowship (1088535) during the conduct of this research. JEM is supported by an Australian NHMRC Career Development Fellowship (1143429). TCH is supported by an Australian NHMRC Senior Research Fellowship (1154607). JMT is supported by Evidence Partners Inc. JMG is supported by a Tier 1 Canada Research Chair in Health Knowledge Transfer and Uptake. MML is supported by The Ottawa Hospital Anaesthesia Alternate Funds Association and a Faculty of Medicine Junior Research Chair. TL is supported by funding from the National Eye Institute (UG1EY020522), National Institutes of Health, United States. LAM is supported by a National Institute for Health Research Doctoral Research Fellowship (DRF-2018-11-ST2-048). ACT is supported by a Tier 2 Canada Research Chair in Knowledge Synthesis. DM is supported in part by a University Research Chair, University of Ottawa. The funders had no role in considering the study design or in the collection, analysis, interpretation of data, writing of the report, or decision to submit the article for publication.
Competing interests: All authors have completed the ICMJE uniform disclosure form at http://www.icmje.org/conflicts-of-interest/ and declare: EL is head of research for the BMJ ; MJP is an editorial board member for PLOS Medicine ; ACT is an associate editor and MJP, TL, EMW, and DM are editorial board members for the Journal of Clinical Epidemiology ; DM and LAS were editors in chief, LS, JMT, and ACT are associate editors, and JG is an editorial board member for Systematic Reviews . None of these authors were involved in the peer review process or decision to publish. TCH has received personal fees from Elsevier outside the submitted work. EMW has received personal fees from the American Journal for Public Health , for which he is the editor for systematic reviews. VW is editor in chief of the Campbell Collaboration, which produces systematic reviews, and co-convenor of the Campbell and Cochrane equity methods group. DM is chair of the EQUATOR Network, IB is adjunct director of the French EQUATOR Centre and TCH is co-director of the Australasian EQUATOR Centre, which advocates for the use of reporting guidelines to improve the quality of reporting in research articles. JMT received salary from Evidence Partners, creator of DistillerSR software for systematic reviews; Evidence Partners was not involved in the design or outcomes of the statement, and the views expressed solely represent those of the author.
Provenance and peer review: Not commissioned; externally peer reviewed.
Patient and public involvement: Patients and the public were not involved in this methodological research. We plan to disseminate the research widely, including to community participants in evidence synthesis organisations.

Literature Reviews: systematic searching at various levels
- for assignments
- for dissertations / theses
- Search strategy and searching
- Boolean Operators
- Search strategy template
- Screening & critiquing
- Citation Searching
- Google Scholar (with Lean Library)
- Resources for literature reviews
- Adding a referencing style to EndNote
- Exporting from different databases
PRISMA Flow Diagram
- Grey Literature
- What is the PRISMA Flow Diagram?
- How should I use it?
- When should I use it?
- PRISMA Links
The PRISMA Flow Diagram is a tool that can be used to record different stages of the literature search process--across multiple resources--and clearly show how a researcher went from, 'These are the databases I searched for my terms', to, 'These are the papers I'm going to talk about'.
PRISMA is not inflexible; it can be modified to suit the research needs of different people and, indeed, if you did a Google images search for the flow diagram you would see many different versions of the diagram being used. It's a good idea to have a look at a couple of those examples, and also to have a look at a couple of the articles on the PRISMA website to see how it has--and can--be used.
The PRISMA 2020 Statement was published in 2021. It consists of a checklist and a flow diagram , and is intended to be accompanied by the PRISMA 2020 Explanation and Elaboration document.
In order to encourage dissemination of the PRISMA 2020 Statement, it has been published in several journals.
- How to use the PRISMA Flow Diagram for literature reviews A PDF [3.81MB] of the PowerPoint used to create the video. Each slide that has notes has a callout icon on the top right of the page which can be toggled on or off to make the notes visible.
There is also a PowerPoint version of the document but the file size is too large to upload here.
If you would like a copy, please email the Academic Librarians' mailbox from your university account to ask for it to be sent to you.
This is an example of how you could fill in the PRISMA flow diagram when conducting a new review. It is not a hard and fast rule but it should give you an idea of how you can use it.
For more detailed information, please have a look at this article:
Page, M.J., McKenzie, J.E., Bossuyt, P.M., Boutron, I., Hoffmann, T.C., Mulrow, C.D., Shamseer, L., Tetzlaff, J.M., Akl, E.A., Brennan, S.E., Chou, R., Glanville, J., Grimshaw, J.M., Hróbjartsson, A., Lalu, M.M., Li, T., Loder, E.W., Mayo-Wilson, E., McDonald, S., McGuinness, L.A., Stewart, L.A., Thomas, J., Tricco, A.C., Welch, V.A., Whiting,P. & Moher, D. (2021) 'The PRISMA 2020 statement: an updated guideline for reporting systematic reviews', BMJ 372:(71). doi: 10.1136/bmj.n71 .
- Example of PRISMA 2020 diagram This is an example of *one* of the PRISMA 2020 flow diagrams you can use when reporting on your research process. There is more than one form that you can use so for other forms and advice please look at the PRISMA website for full details.
Start using the flow diagram as you start searching the databases you've decided upon.
Make sure that you record the number of results that you found per database (before removing any duplicates) as per the filled in example. You can also do a Google images search for the PRISMA flow diagram to see the different ways in which people have used them to express their search processes.
- Preferred Reporting Items for Systematic Reviews and Meta-Analyses (PRISMA) PRISMA is an evidence-based minimum set of items for reporting in systematic reviews and meta-analyses. PRISMA focuses on the reporting of reviews evaluating randomized trials, but can also be used as a basis for reporting systematic reviews of other types of research, particularly evaluations of interventions.
- Prisma Flow Diagram This link will take you to downloadable Word and PDF copies of the flow diagram. These are modifiable and act as a starting point for you to record the process you engaged in from first search to the papers you ultimately discuss in your work. more... less... Do an image search on the internet for the flow diagram and you will be able to see all the different ways that people have modified the diagram to suit their personal research needs.
You can access the various checklists via the Equator website and the articles explaining PRISMA and its various extensions are available via PubMed.
Page, M.J., McKenzie, J.E., Bossuyt, P.M., Boutron, I., Hoffmann, T.C., Mulrow, C.D., Shamseer, L., Tetzlaff, J.M., Akl, E.A., Brennan, S.E., Chou, R., Glanville, J., Grimshaw, J.M., Hróbjartsson, A., Lalu, M.M., Li, T., Loder, E.W., Mayo-Wilson, E., McDonald, S., McGuinness, L.A., Stewart, L.A., Thomas, J., Tricco, A.C., Welch, V.A., Whiting, P., & Moher, D. (2021) ' The PRISMA 2020 statement: an updated guideline for reporting systematic reviews,' BMJ . Mar 29; 372:n71. doi: 10.1136/bmj.n71 .
Page, M.J., Moher, D., Bossuyt, P.M., Boutron, I., Hoffmann, T.C., Mulrow, C.D., Shamseer, L., Tetzlaff, J.M., Akl, E.A., Brennan, S.E., Chou, R., Glanville, J., Grimshaw, J.M., Hróbjartsson, A., Lalu, M.M., Li, T., Loder, E.W., Mayo-Wilson, E., McDonald, S., McGuinness, L.A., Stewart, L.A., Thomas, J., Tricco, A.C., Welch, V.A., Whiting, P., & McKenzie, J.E. (2021) 'PRISMA 2020 explanation and elaboration: updated guidance and exemplars for reporting systematic reviews', BMJ, Mar 29; 372:n160. doi: 10.1136/bmj.n160 .
Page, M.J., McKenzie, J.E., Bossuyt, P.M., Boutron, I., Hoffmann, T.C., Mulrow, C.D., Shamseer, L., Tetzlaff, J.M., Akl, E.A., Brennan, S.E., Chou, R., Glanville, J., Grimshaw, J.M., Hróbjartsson, A., Lalu, M.M., Li, T., Loder, E.W., Mayo-Wilson, E., McDonald, S., McGuinness, L.A., Stewart, L.A., Thomas, J., Tricco, A.C., Welch, V.A., Whiting, P., & Moher, D. (2021) ' The PRISMA 2020 statement: An updated guideline for reporting systematic reviews,' Journal of Clinical Epidemiology, June; 134:178-189. doi: 10.1016/j.jclinepi.2021.03.001 .
- << Previous: Exporting from different databases
- Next: Grey Literature >>
- Last Updated: Apr 12, 2024 11:57 AM
- URL: https://libguides.derby.ac.uk/literature-reviews
- UNC Libraries
- HSL Academic Process
- Creating a PRISMA flow diagram
- PRISMA 2020
Creating a PRISMA flow diagram: PRISMA 2020
Created by health science librarians.

What is PRISMA?
Which prisma 2020 flow diagram should i use, step-by-step: prisma 2020 flow diagram, using the covidence prisma diagram, documenting your grey literature search, updating a systematic review with prisma 2020, citing prisma 2020, for more information, prisma 2020 checklist.
- PRISMA 2020 Checklist (.doc)
- PRISMA 2020 Checklist (.pdf)
- PRISMA 2020 Expanded Checklist
PRISMA 2020 Flow Diagram Templates
- PRISMA 2020 V1- New Reviews with Databases and Registers only PRISMA 2020 flow diagram for new systematic reviews which included searches of databases and registers only
- PRISMA 2020 V2 - New Reviews with Databases, Registers, and Other Sources PRISMA 2020 flow diagram for new systematic reviews which included searches of databases, registers and other sources
The format of the PRISMA Step-By-Step was first developed by Glasgow Caledonian University https://www.gcu.ac.uk/library

"PRISMA stands for Preferred Reporting Items for Systematic Reviews and Meta-Analyses.
It is an evidence-based minimum set of items for reporting in systematic reviews and meta-analyses.
The aim of the PRISMA Statement is to help authors improve the reporting of systematic reviews and meta-analyses. We have focused on randomized trials, but PRISMA can also be used as a basis for reporting systematic reviews of other types of research, particularly evaluations of interventions. PRISMA may also be useful for critical appraisal of published systematic reviews, although it is not a quality assessment instrument to gauge the quality of a systematic review. The PRISMA Statement consists of a 27-item checklist and a four-phase flow diagram ."
"The PRISMA Explanation and Elaboration document explains and illustrates the principles underlying the PRISMA Statement. It is strongly recommended that it be used in conjunction with the PRISMA Statement.
PRISMA is part of a broader effort, to improve the reporting of different types of health research, and in turn to improve the quality of research used in decision-making in healthcare."
From prisma-statement.org
Liberati A, Altman DG, Tetzlaff J, et al. The PRISMA statement for reporting systematic reviews and meta-analyses of studies that evaluate health care interventions: explanation and elaboration. J Clin Epidemiol . 2009;62(10):e1-e34. doi:10.1016/j.jclinepi.2009.06.006
Page MJ, Moher D, Bossuyt P, et al. PRISMA 2020 explanation and elaboration: updated guidance and exemplars for reporting systematic reviews. doi:10.31222/osf.io/gwdhk.
Rethlefsen M, Kirtley S, Waffenschmidt S, et al. PRISMA-S: An Extension to the PRISMA Statement for Reporting Literature Searches in Systematic Reviews. doi:10.31219/osf.io/sfc38.
In PRISMA 2020, there are now expanded options depending on where you search and whether you are updating a review. Version 1 of PRISMA 2020 includes databases and clinical trial or preprint registers. Version 2 includes additional sections for elaborating on your grey literature search, such as searches on websites or in citation lists. Both versions are available for new and updated reviews from the Equator Network's PRISMA Flow Diagram page .
Templates for New Reviews

Step 1: Preparation To complete the the PRISMA diagram, save a copy of the diagram to use alongside your searches. It can be downloaded from the PRISMA website .
Step 2: Doing the Database Search Run the search for each database individually, including ALL your search terms, any MeSH or other subject headings, truncation (like hemipleg * ), and/or wildcards (like sul ? ur). Apply all your limits (such as years of search, English language only, and so on). Once all search terms have been combined and you have applied all relevant limits, you should have a final number of records or articles for each database. Enter this information in the top left box of the PRISMA flow chart. You should add the total number of combined results from all databases (including duplicates) after the equal sign where it says Databases (n=) . Many researchers also add notations in the box for the number of results from each database search, for example, Pubmed (n=335), Embase (n= 600), and so on. If you search trial registers, such as ClinicalTrials.gov , CENTRAL , ICTRP , or others, you should enter that number after the equal sign in Registers (n=) .
NOTE: Some citation managers automatically remove duplicates with each file you import. Be sure to capture the number of articles from your database searches before any duplicates are removed.

Step 3: Remove All Duplicates To avoid reviewing duplicate articles, you need to remove any articles that appear more than once in your results. You may want to export the entire list of articles from each database to a citation manager such as EndNote, Sciwheel, Zotero, or Mendeley (including both citation and abstract in your file) and remove the duplicates there. If you are using Covidence for your review, you should also add the duplicate articles identified in Covidence to the citation manager number. Enter the number of records removed as duplicates in the second box on your PRISMA template. If you are using automation tools to help evaluate the relevance of citations in your results, you would also enter that number here.

NOTE: If you are using Covidence to screen your articles , you can copy the numbers from the PRISMA diagram in your Covidence review into the boxes mentioned below. Covidence does not include the number of results from each database, so you will need to keep track of that number yourself.
Step 4: Records Screened- Title/Abstract Screening The next step is to add the number of articles that you will screen. This should be the number of records identified minus the number from the duplicates removed box.

Step 5: Records Excluded- Title/Abstract Screening You will need to screen the titles and abstracts for articles which are relevant to your research question. Any articles that appear to help you provide an answer to your research question should be included. Record the number of articles excluded through title/abstract screening in the box to the right titled "Records excluded." You can optionally add exclusion reasons at this level, but they are not required until full text screening.

Step 6: Reports Sought for Retrieval This is the number of articles you obtain in preparation for full text screening. Subtract the number of excluded records (Step 5) from the total number screened (Step 4) and this will be your number sought for retrieval.

Step 7: Reports Not Retrieved List the number of articles for which you are unable to find the full text. Remember to use Find@UNC and Interlibrary Loan to request articles to see if we can order them from other libraries before automatically excluding them.

Step 8: Reports Assessed for Eligibility- Full Text Screening This should be the number of reports sought for retrieval (Step 6) minus the number of reports not retrieved (Step 7). Review the full text for these articles to assess their eligibility for inclusion in your systematic review.

Step 9: Reports Excluded After reviewing all articles in the full-text screening stage for eligibility, enter the total number of articles you exclude in the box titled "Reports excluded," and then list your reasons for excluding the articles as well as the number of records excluded for each reason. Examples include wrong setting, wrong patient population, wrong intervention, wrong dosage, etc. You should only count an excluded article once in your list even if if meets multiple exclusion criteria.

Step 10: Included Studies The final step is to subtract the number of records excluded during the eligibility review of full-texts (Step 9) from the total number of articles reviewed for eligibility (Step 8). Enter this number in the box labeled "Studies included in review," combining numbers with your grey literature search results in this box if needed. You have now completed your PRISMA flow diagram, unless you have also performed searches in non-database sources.

To view the PRISMA diagram created after using Covidence to screen references for your review, click the PRISMA button on the main menu of your review in Covidence.

If you listed your sources when importing citations, your PRISMA diagram will include the list of databases you used and the number of references from each.

If you imported references from a citation manager, your PRISMA diagram starts with duplicate removal. To have a complete PRISMA diagram, you will need to add the number of results from each database you searched, as well as the number of additional sources you found.
Once you have finished title/abstract and full text screening (and data extraction or quality assessment if applicable), click Download DOCX to download your flow diagram as a Word document, or click View as text to copy and paste the PRISMA data or into an editable template for PRISMA and fill in the numbers.

There are many places articles can get lost in the review process. Remember to make sure your PRISMA numbers add up correctly!

Step 6: Included Studies The final step is to subtract the number of excluded articles or records during the eligibility review of full-texts from the total number of articles reviewed for eligibility. Enter this number in the box labeled "Studies included in review," combining numbers with your database search results in this box if needed. You have now completed your PRISMA flow diagram, which you can now include in the results section of your article or assignment.

If you are updating an existing review, use one of these PRISMA 2020 Updated Review templates, which feature an additional box for the number of studies and reports of studies included in the previous search iterations.
- PRISMA 2020 flow diagram for updated systematic reviews- databases and registers only
- PRISMA 2020 flow diagram for updated systematic reviews- databases, registers and other sources
When referring to PRISMA 2020, The Equator Network recommends using journal article citations (such as those in our For More Information box ) rather than referring to the PRISMA website. If you are not already using a journal article citation, they recommend that you cite one of the original publications of the PRISMA Statement or PRISMA Explanation and Elaboration .
Related HSL Guides
- Systematic Reviews
Additional Readings
- Page MJ, McKenzie JE, Bossuyt PM, et al. Updating guidance for reporting systematic reviews: development of the PRISMA 2020 statement . J Clin Epidemiol. 2021;134:103-112.
- Page MJ, Moher D, Bossuyt PM, et al. PRISMA 2020 explanation and elaboration: updated guidance and exemplars for reporting systematic reviews . Bmj. 2021;372:n160.
- Radua J. PRISMA 2020 - An updated checklist for systematic reviews and meta-analyses. Neurosci Biobehav Rev. 2021;124:324-325.
- Sarkis-Onofre R, Catalá-López F, Aromataris E, Lockwood C. How to properly use the PRISMA Statement . Systematic reviews. 2021;10(1):117-117.
- Sohrabi C, Franchi T, Mathew G, et al. PRISMA 2020 statement: What's new and the importance of reporting guidelines. Int J Surg. 2021;88:105918.
- Page MJ, McKenzie JE, Bossuyt PM, et al. The PRISMA 2020 statement: An updated guideline for reporting systematic reviews . Int J Surg. 2021;88:105906.
- Page MJ, McKenzie JE, Bossuyt PM, et al. The PRISMA 2020 statement: An updated guideline for reporting systematic reviews. J Clin Epidemiol. 2021.
- Page MJ, McKenzie JE, Bossuyt PM, et al. The PRISMA 2020 statement: an updated guideline for reporting systematic reviews . Bmj. 2021;372:n71.
- Page MJ, McKenzie JE, Bossuyt PM, et al. The PRISMA 2020 statement: An updated guideline for reporting systematic reviews . PLoS Med. 2021;18(3):e1003583.
- Page MJ, McKenzie JE, Bossuyt PM, et al. The PRISMA 2020 statement: an updated guideline for reporting systematic reviews . Syst Rev. 2021;10(1):89.
- Last Updated: May 14, 2024 12:49 PM
- URL: https://guides.lib.unc.edu/prisma
SYSTEMATIC REVIEW article
Utility of artificial intelligence in the diagnosis and management of keratoconus: a systematic review.
- Bascom Palmer Eye Institute, Miller School of Medicine, University of Miami, Miami, FL, United States
Introduction: The application of artificial intelligence (AI) systems in ophthalmology is rapidly expanding. Early detection and management of keratoconus is important for preventing disease progression and the need for corneal transplant. We review studies regarding the utility of AI in the diagnosis and management of keratoconus and other corneal ectasias.
Methods: We conducted a systematic search for relevant original, English-language research studies in the PubMed, Web of Science, Embase, and Cochrane databases from inception to October 31, 2023, using a combination of the following keywords: artificial intelligence, deep learning, machine learning, keratoconus, and corneal ectasia. Case reports, literature reviews, conference proceedings, and editorials were excluded. We extracted the following data from each eligible study: type of AI, input used for training, output, ground truth or reference, dataset size, availability of algorithm/model, availability of dataset, and major study findings.
Results: Ninety-three original research studies were included in this review, with the date of publication ranging from 1994 to 2023. The majority of studies were regarding the use of AI in detecting keratoconus or subclinical keratoconus (n=61). Among studies regarding keratoconus diagnosis, the most common inputs were corneal topography, Scheimpflug-based corneal tomography, and anterior segment-optical coherence tomography. This review also summarized 16 original research studies regarding AI-based assessment of severity and clinical features, 7 studies regarding the prediction of disease progression, and 6 studies regarding the characterization of treatment response. There were only three studies regarding the use of AI in identifying susceptibility genes involved in the etiology and pathogenesis of keratoconus.
Discussion: Algorithms trained on Scheimpflug-based tomography seem promising tools for the early diagnosis of keratoconus that can be particularly applied in low-resource communities. Future studies could investigate the application of AI models trained on multimodal patient information for staging keratoconus severity and tracking disease progression.
1 Introduction
1.1 keratoconus.
Keratoconus is a progressive corneal ectasia characterized by stromal thinning and corneal steepening, ranging in severity from asymptomatic subclinical disease to severe corneal scarring requiring corneal transplantation for visual rehabilitation ( 1 ). As the condition progresses, patients may experience decreased visual acuity, photophobia, and image distortion ( 1 ). A meta-analysis study reported that younger patients and patients with a baseline maximum keratometry steeper than 55 D were more likely to experience disease progression ( 2 ). Patients with a medical history involving allergic eye disease or atopic conditions were also more likely to experience keratoconus progression ( 3 ). The pathophysiology of keratoconus involves reduced keratocyte density, loss of stromal lamellae with fibroblast degradation, redistribution of collagen, and increased proteolysis leading to a breakdown in structural integrity ( 4 ). This condition most commonly occurs between the second and third decade of life and has an estimated global prevalence of 1.38 per 1000 individuals ( 5 , 6 ). The global prevalence of keratoconus varies across populations but has been previously estimated to range between 0.2-4790 per 100,000 individuals, with the lowest reported in Russia ( 5 ). The highest prevalence rates have been reported in Asian and Middle Eastern communities ( 5 ).
Multiple ancillary testing modalities have been used in conjunction with clinical examination for the diagnosis of keratoconus. Placido-based videokeratoscopy and ultrasonic central pachymetry were previously used in the diagnosis and severity staging of keratoconus ( 7 ). Modern Placido disc-based corneal topography devices are still popular, as they provide information about corneal curvature, surface irregularities, and aberrations by generating color-coded maps ( 1 , 8 ). More recently, Scheimpflug-based corneal tomography imaging has allowed for greater analysis of the cornea by generating three-dimensional representations of the anterior segment to provide information about corneal thickness as well as the anterior and posterior cornea ( 7 , 9 ). Corneal tomography is now the gold standard method for corneal ectasia diagnosis, and the Pentacam® (OCULUS, Arlington, WA, USA) is one of the most common Scheimpflug-based corneal tomography devices ( 9 , 10 ). Another adjunct imaging technology used for evaluation of corneal ectasias is anterior-segment optical coherence tomography (AS-OCT), which generates cross-sectional corneal images that can be used to identify asymmetry in corneal thinning, posterior curvature, and epithelial/total corneal thickness via epithelial mapping ( 1 ). Corneal biomechanics, including corneal hysteresis and deformation amplitude, can also be used to diagnose early stages of keratoconus through an association with central corneal thickness ( 11 ).
Early keratoconus diagnosis is important for successful management and prevention of disease progression ( 12 ). Corneal cross-linking (CXL) is a procedure developed in 2003 that promotes bond formation between corneal collagen fibrils using riboflavin and ultraviolet-A light, which has demonstrated excellent long-term efficacy in reducing progression of keratoconus but has a threshold of procedural safety for the thinnest pachymetry value that can be treated ( 12 ). As CXL alone does not significantly improve visual outcomes, early detection and disease management can also allow for enhanced visual rehabilitation of patients without requiring keratoplasty, including options of hard contact lenses, intracorneal ring segment implantation, intraocular collamer lens implantation, and CXL combined with laser ablative procedures (e.g. photorefractive keratectomy) ( 12 ).
1.2 Models of artificial intelligence
The application of artificial intelligence (AI) in the diagnosis and management of ophthalmic diseases has been rapidly increasing since the 1970s ( 13 ). AI associates a particular outcome with variables of different weights, and trained models can be applied in establishing disease diagnosis, determining management, and predicting the prognosis of patients ( 14 ). Machine learning (ML) is a subset of AI that uses a training dataset for tasks but does not require programming ( 15 ). Features from the input data, which may include imaging and patient demographic information, are used to form a feature vector which then serves as the starting point for the ML model ( 16 ). Supervised ML is trained on a set of inputs with correct outcome labels available ( 17 ). In contrast, unsupervised ML models receive inputs without outcome labels ( 18 ). ML techniques include logistic regression, decision tree, random forest, support vector machine, and multilayer perceptron ( Table 1 ) ( 15 , 16 ).
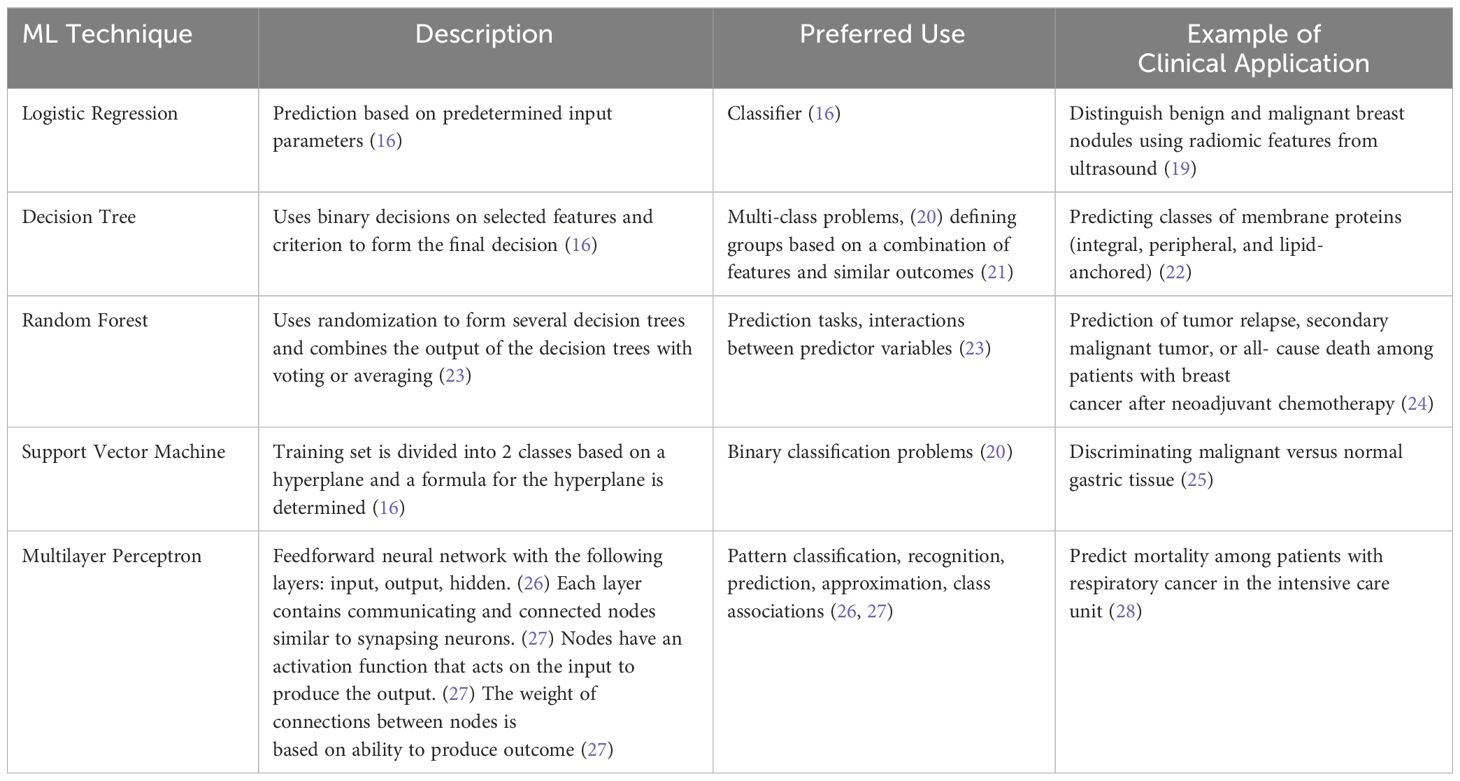
Table 1 Description, use, and examples of machine learning techniques in medical literature.
Deep learning (DL) is a subset of machine learning that includes convolutional neural networks and does not require manual feature extraction from the research team ( 15 , 29 ). DL models are composed of neural network layers that represent operations, and the output of one layer serves as the input of the next layer ( 30 ). Convolutional neural networks consist of convolution layers, pooling layers, and a fully connected network layer ( 16 ). Back propagation, normalization of input, dropout, and residual networks may be used to reduce error, reduce overfitting, and improve training ( 16 ).
The application of AI models is evaluated through several measures, which most commonly include accuracy, sensitivity, specificity, and area under the receiver operating curve (AUC or AUROC). The output of AI models in the majority of included studies is a class prediction (i.e. keratoconus versus healthy). Accuracy is the proportion of predictions that are true positives and negatives among all predictions. Sensitivity of a class is the proportion of true positives among all predictions of that class. Specificity describes the rate of true negatives ( 31 ). AUC ranges from 0 to 1 and is the area under the curve of the function modeled by sensitivity and 1-specificity. This provides a measure of diagnostic accuracy, with higher scores (closer to 1) representing greater accuracy ( 32 ).
1.3 Ophthalmic applications of artificial intelligence
The application of AI models in ophthalmology has been widely studied, particularly for posterior segment diseases including diabetic retinopathy, glaucoma, age-related macular degeneration, and retinopathy of prematurity (ROP) ( 33 , 34 ). Ng et al. reported that AI algorithms for diabetic retinopathy may be closest to application in clinical medicine ( 33 ). DL systems are able to detect diabetic retinopathy, predict disease progression, and predict diabetic macular edema using fundus images ( 33 ). There also exist DL algorithms to detect glaucoma and predict progression using fundus photographs, optical coherence tomography, and Humphrey visual fields ( 33 ). AI systems are particularly useful for detecting ROP from fundus imaging given that there is grading variation among experts, and some low-resource countries have heavy disease burden with limited access to specialist care ( 33 ). DL algorithms have demonstrated high AUC, sensitivity, and specificity for identifying retinopathy of prematurity requiring further management ( 33 ).
There has also been an increase in reports evaluating the application of AI systems in detecting anterior segment diseases, including keratoconus, infectious keratitis, cataract, and pterygium using anterior segment photographs and AS-OCT images ( 35 ). AI has also been used to screen patients for post-corneal transplant rejection as well as to grade cataracts ( 35 ). However, several studies regarding the application of AI in anterior segment disease diagnosis and management were limited by a small sample size with low heterogeneity ( 35 ).
As shown, clinical utilization of AI models in ophthalmology has rapidly increased in the past 7 years since the first reports of DL systems trained for screening of retinal pathologies ( 36 , 37 ). Due to the greater variety of anterior segment imaging and variable use in evaluating different conditions, widespread adoption of ML/DL algorithms for corneal pathology has not yet occurred. However, as imaging-based evaluation of keratoconus has now become standard of care due to improved technology, this study aims to provide a systematic review on the current state of utilizing AI and ML/DL platforms in the diagnosis, evaluation, management, and prognosis of keratoconus and corneal ectasias.
2 Methods of literature search
2.1 search strategy.
Using the Preferred Reporting Items for Systematic reviews and Meta-Analyses (PRISMA) framework as a guide, a systematic review technique was used to evaluate studies describing artificial intelligence and keratoconus. The search strategy was created based on the population, interventions, comparators, outcomes, and study design (PICOS) architecture, resulting in the study question: “Is artificial intelligence a sensitive and specific tool for the diagnosis and management of keratoconus or other corneal ectasias compared to clinical diagnosis and management led by ophthalmologists?” The systematic search was conducted using the PubMed, Web of Science, Embase, and Cochrane databases from inception to October 31, 2023, to select full-length, English articles in peer-reviewed journals. The MeSH keywords included in the search strategy were keratoconus, corneal ectasia, artificial intelligence, machine learning, and deep learning, with all combinations of these terms searched.
2.2 Inclusion and exclusion criteria
Original, English-language research articles published in peer-reviewed journals regarding the use of any AI, ML or DL model in the evaluation of the diagnosis, pathophysiology, severity, clinical progression, or evaluation of the response to management of keratoconus and other corneal ectasias were included in this study. Case reports, editorials, commentaries, conference abstracts, and literature reviews were excluded. Non-English-language articles were also excluded. Publications were not restricted by year. Figure 1 displays PRISMA diagram of the study selection methodology ( 38 ).
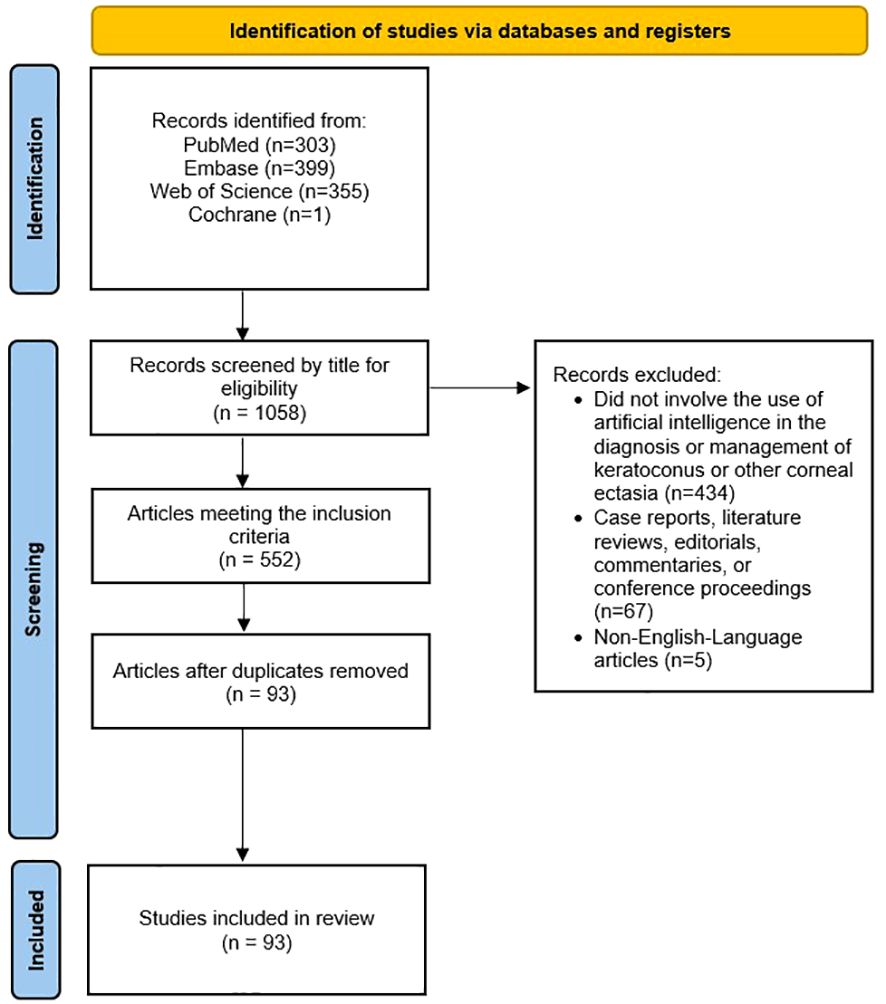
Figure 1 Preferred Reporting Items for Systematic Reviews and Meta-Analyses (PRISMA) diagram of the study selection methodology for this review.
2.3 Data extraction
The following variables were collected for each included study:
● Type of artificial intelligence
● Input used for training
● Output
● Ground truth or reference standard
● Dataset size (number of images, eyes, and patients, when applicable and available)
● Availability of algorithm/model
● Source and availability of dataset
● Major study findings
2.4 Quality assessment
The Quality Assessment of Diagnostic Accuracy Studies-2 (QUADAS-2) tool was used to assess the risk of bias and applicability concerns of studies regarding the diagnosis, severity, and clinical grading of keratoconus and other corneal ectasias ( 39 ). The Quality Assessment of Prognostic Accuracy Studies (QUAPAS) tool was used to assess the risk of bias and applicability concerns of studies regarding the prediction of disease progression and response to treatment/management ( 40 ). A quality assessment for studies regarding the etiology and pathogenesis was not completed due to the absence of a validated assessment tool for this type of study.
The search strategy yielded 93 original research studies. These studies are summarized in Table 2 , and we discuss some key studies in the following Results section. There were no concerns regarding the applicability of any studies given the broad nature of our review question and strict application of the inclusion and exclusion criteria.
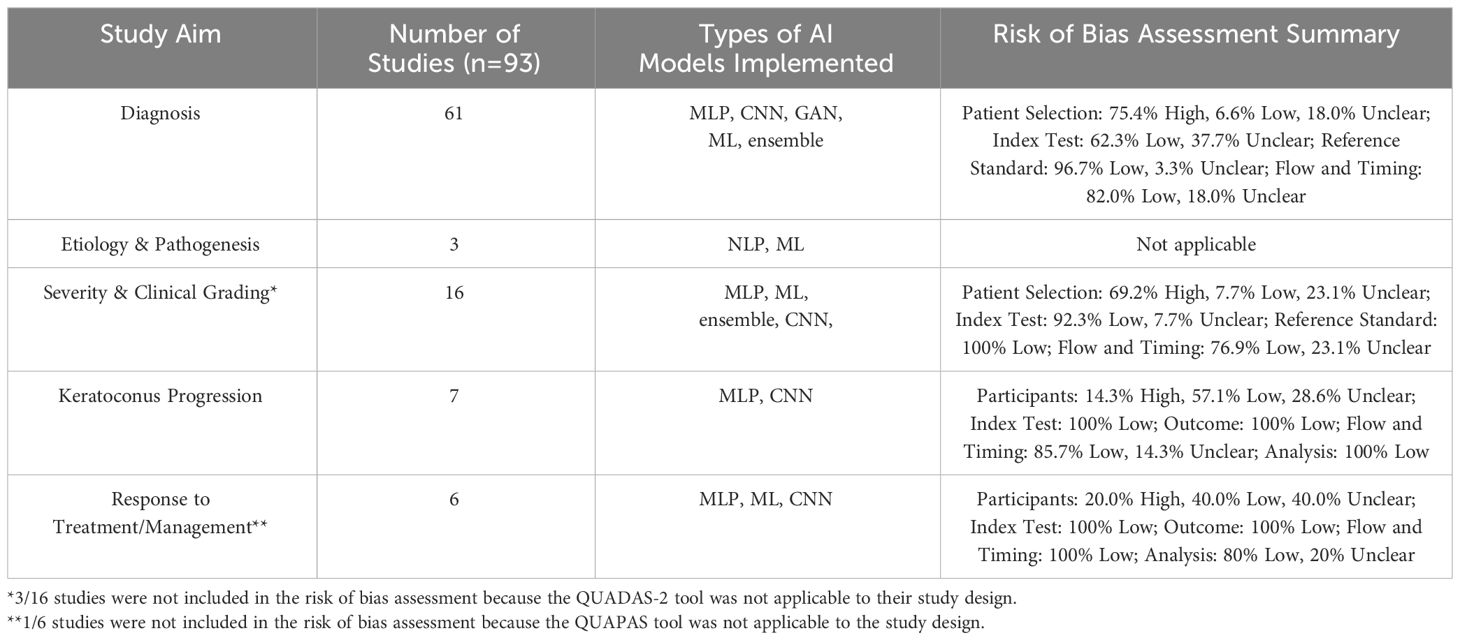
Table 2 Overview of the number of studies, types of AI models implemented for each study aim, and quality assessment of studies included in this review. The types of AI models include multilayer perceptron (MLP), convolutional neural network (CNN), generative adversarial network (GAN), traditional machine learning (ML), ensemble, and natural language processing (NLP).
3.1 Diagnosis of keratoconus
Among the included studies, AI was most frequently used for the diagnosis of keratoconus and other ectasias, particularly in subclinical cases (n = 61, Supplementary Table 1 ) ( 31 , 41 – 100 ). The earliest study using artificial intelligence to detect keratoconus was published in 1994 by Maeda et al. ( 65 ) This study used eight indices from TMS-1 videokeratoscope data (Computed Anatomy Inc., New York City, NY, USA) to detect keratoconus among a set of eyes with normal corneas and corneas with various diagnoses ( 65 ). The linear discriminant function generated a Keratoconus Prediction Index value that was used to classify eyes as “keratoconus” or “nonkeratoconus” based on a cutoff value ( 65 , 100 ). The model demonstrated sensitivities of 100% and 89% with three false-positives and one false-positive in the training and validation set, respectively ( 65 ). More recently, Silverman et al. evaluated the use of stepwise linear discriminant analysis and neural networks in detecting keratoconus using corneal epithelial and stromal thickness maps from Artemis 1® (StarFish Medical, Victoria, BC, Canada) very high frequency ultrasound arc-scans ( 96 ). Eyes with keratoconus were reported to have focal epithelial and stromal thinning with a surrounding ring of epithelial thickening ( 96 , 101 ).
The majority of the included studies regarding keratoconus or corneal ectasia diagnosis with AI used data from corneal topography ( 47 , 51 – 53 , 56 , 57 , 67 , 73 , 74 , 77 , 78 , 83 , 91 , 92 , 94 , 100 ), Scheimpflug-based tomography ( 43 , 45 , 49 , 54 , 55 , 59 , 60 , 62 , 64 , 66 , 70 , 76 , 79 , 80 , 84 , 86 – 88 , 90 , 95 , 99 ), or optical coherence tomography (OCT) ( 31 , 46 , 50 , 53 , 68 , 69 , 72 , 80 , 84 ) as the input. For example, de Almeida et al. utilized 52 parameters from Pentacam® (OCULUS, Arlington, WA, USA) tomography to generate the Corneal Tomography Multivariate Index (CTMVI) using paraconsistent feature engineering and a support vector machine classifier ( 90 ). CTMVI was then used to discriminate between very asymmetric ectasia with normal topography and healthy corneas. When using these 52 features, CTMVI demonstrated a sensitivity, specificity, and AUC of 0.844, 0.874, and 0.926, respectively ( 90 ). After combining CTMVI and the Pentacam Random Forest Index, the model demonstrated a sensitivity and specificity of 0.99 and 0.84, respectively. Other input for AI-based keratoconus or corneal ectasia diagnosis included data from corneal deformation videos ( 42 , 55 , 71 , 97 ), air-puff tonometry ( 50 ), corneal endothelial images from specular microscopy ( 81 ), and lateral segment photographs ( 93 ).
Beyond diagnosing subclinical keratoconus or keratoconus, Ahn et al. demonstrated that AI can be used to screen patients in a primary care setting that may need further evaluation with corneal topography for keratoconus. Using input parameters of subjective visual impairment (based on a patient survey), visual acuity, intraocular pressure, and autokeratometry parameters, they compared the performance of five previously-reported AI models ( 102 – 106 ). The ensemble model with soft voting method demonstrated superior performance with this task with a sensitivity of 90.5% and 96.4% in the internal and external test datasets, respectively ( 44 ). Intraocular pressure and mean corneal power were the most highly ranked in the feature importance analysis ( 44 ). A soft voting ensemble classifier has been previously used to predict major adverse cardiovascular events among patients with acute coronary system ( 107 ).
In the absence of a large dataset of eyes with keratoconus, Lavric et al. and Subramanian et al. used SyntEyes KTC to generate a sufficiently large set of corneal topography images for model training ( 57 , 97 ). The SyntEyes KTC model was developed by Rozema et al. using Scheimpflug tomography, ocular biometry, and wavefront data of 145 eyes with keratoconus ( 108 ). Abdelmotaal et al. used a conditional generative adversarial network (CGAN) called pix2pix to produce images of keratoconus eyes ( 95 ). The pix2pix CGAN is composed of a Generator that uses the input image to form the output image, and a Discriminator that determines the similarity of the generated image to an image from the original dataset or an image from the Generator ( 95 ). The authors reported that the model produced subjectively and objectively plausible images of keratoconus, early keratoconus, and normal eyes ( 95 ). They trained a VGG-16 deep convolutional neural network on combinations of original images and images synthesized by the pix2pix CGAN. The VGG-16 model trained with all original images and synthesized images demonstrated the highest accuracy of 99.56% in discriminating early keratoconus, keratoconus, and normal eyes ( 95 ).
3.2 Etiology & pathogenesis of keratoconus
Only three studies have investigated the utility of AI in exploring the genetic etiology and mechanical pathogenesis of keratoconus ( Supplementary Table 2 ) ( 109 – 111 ). Hosoda et al. conducted a genome-wide association study (GWAS) of central corneal thickness using IBM’s Watson for Drug Discovery AI technology. They found the STON2 rs2371597 and SMAD3 rs12913547 loci to be involved in keratoconus development. STON2 and SMAD3 have roles in extracellular matrix (ECM) remodeling, so these variants may contribute to the stromal ECM changes described in keratoconus ( 109 , 112 ). The authors noted that additional GWAS may be used to identify pathways driving keratoconus development ( 109 ). Wang et al. identified 8 differentially expressed genes (AREG, BBC3, DUSP2, MAP3K8, SMAD7, CDKN1A, JUN, and LIF) between patients with and without keratoconus using the random forest model, support vector machine model, and generalized linear model ( 110 ). These genes may affect cell mitosis (AREG), macrophage dysfunction (BBC3), cell cycle arrest (CDKN1A), apoptosis (DUSP2, CDKN1A), and proliferation, differentiation, and death (JUN) ( 110 ). The authors concluded that abnormal cell proliferation, differentiation, and autophagy pathways may be involved in keratoconus development ( 110 ).
Given the reported association between mechanical eye rubbing and pathogenesis of keratoconus, Nokas et al. built a wrist-mounted sensor that used an accelerometer, gyroscope, and machine learning algorithms to detect eye rubbing activity and remind the user to cease such activity ( 111 ). With limited AI-based studies on this topic, future research could further investigate susceptibility genes and utility of behavior modification in keratoconus pathogenesis.
3.3 Severity & clinical grading of keratoconus
Sixteen original research articles identified in this review assessed severity or other clinical features of patients with keratoconus ( Supplementary Table 3 ) ( 104 , 113 – 127 ). Among studies evaluating keratoconus severity, the ground truth included staging based on topography findings ( 104 , 113 , 118 , 120 , 125 , 127 ), tomography findings ( 116 ), the Ectasia Screening Index ( 114 ), Keratoconus Severity Index ( 119 ), and Amsler-Krumeich criteria ( 120 , 121 , 126 ). Chen et al. compared six convolutional neural network models trained with one or a combination of four color-coded corneal tomography maps (axial, anterior elevation, posterior elevation, and pachymetry) as well as a majority voting strategy model to predict the presence and stage of keratoconus ( 121 ). The model trained with all four maps demonstrated the best AUC in distinguishing healthy from keratoconus eyes ( 121 ). Interestingly, the majority voting model and the model using the back elevation map demonstrated the highest AUC for discriminating healthy and stage 1 keratoconus eyes, as well as stage 1 and stage 2 keratoconus eyes, respectively ( 121 ).
Dong et al. and Dos Santos et al. both used deep learning models to segment corneal OCT scans ( 122 , 123 ). Dong et al. used a corneal segmentation algorithm to measure the thickness of epithelial and stromal tissue with an error of less than 4 microns ( 123 ). The OCT images were taken by the Optovue RTVUE 100 device (Optovue, Inc., Fremont, CA, USA). They reported that as keratoconus progressed, total corneal thickness decreased, particularly temporal and inferior to the pupil center ( 123 ). Epithelial thickness also decreased as keratoconus severity increased ( 123 ). However, keratoconic eyes with stromal scarring demonstrated a larger epithelial thickness with irregular variations ( 123 ). Dos Santos et al. developed CorneaNet, a neural network to segment OCT images of eyes with and without keratoconus and produce thickness maps of the epithelium, Bowman layer, and stroma ( 122 ). The image scans were acquired on a custom-built, ultra-high resolution OCT system ( 128 ). CorneaNet achieved an accuracy of 99.56% with this task, but the authors noted that accuracy may be limited by network architecture, image noise, as well as insufficient and incorrect training data ( 122 ).
3.4 Keratoconus progression
Seven studies investigated AI-based prediction of disease progression in eyes with keratoconus ( Supplementary Table 4 ) ( 129 – 135 ). The majority of these studies only provided binary categorization of keratoconus eyes as progressive or nonprogressive. Kundu et al. developed two AI models, based on either tomographic changes or clinical risk factors, to identify risk factors underlying keratoconus progression and label patients with either likely disease “progression” or “no progression.” ( 129 ) They found that elevated serum immunoglobin E (IgE), systemic allergies, eye rubbing, and serum vitamin D level were important characteristics in the evaluation for the risk of keratoconus progression ( 129 ). Patients categorized into the progression group had a significantly higher serum IgE compared to those categorized into the no progression group ( 129 ). Eye rubbing may also be due to ocular irritation, fatigue, and stress ( 129 ). Eye rubbing reduces keratocyte density and modifies intraocular pressure, which can contribute to keratoconus development ( 129 ). The results of this study were limited in their generalizability given that the study was based only on an Asian Indian group of patients ( 129 ).
Alternatively, Kamiya et al. used a deep learning model to predict keratoconus progression with color-coded maps from AS-OCT ( 133 ). They reported that after adjusting for age, the accuracy of their algorithm improved from 0.794 to 0.849 ( 133 ). However, this study was limited by the absence of external validation and confirmation of repeatability, small sample size, possibly inaccurate keratoconus diagnosis, and the effect of contact lenses ( 133 ).
3.5 Response to treatment/management
Six studies featured the use of AI in characterizing the response to different treatment modalities, such as by predicting postoperative outcomes or the need for future intervention ( Supplementary Table 5 ) ( 136 – 141 ). Both Valdés-Mas et al. and Lyra et al. employed machine learning to predict postoperative refractive outcomes after intracorneal ring implantation, including corneal curvature, astigmatism, asphericity, and keratometry ( 138 , 140 ). Liu et al. utilized machine learning to predict postoperative visual acuity and keratometry two years after corneal crosslinking ( 139 ). While these studies demonstrate the potential of AI models to aid in disease prognostication after different treatments, validation studies are necessary prior to their widespread generalization and adoption.
4 Discussion
Keratoconus is a corneal ectatic disease that can be diagnosed through several imaging modalities, including corneal topography and tomography ( 1 ). Furthermore, early detection of keratoconus and other corneal ectasias with prompt management can help slow disease progression and reduce the risk for vision loss or need for a corneal transplant ( 12 ). Therefore, AI systems that can process images and generate predictions may be used to reduce the rate of missed or delayed diagnoses, thereby improving patient outcomes. We conducted a systematic review to evaluate the state of AI systems that have been applied in the diagnosis and management of keratoconus and other corneal ectasias among adult patients.
We found that the majority of original research studies involving the clinical application of AI algorithms among patients with keratoconus are based on disease diagnosis. The most current and highest impact studies in the field include Al-Timemy et al., Tan et al., Ambrosio et al., Kuo et al., Kamiya et al., and Chen et al. ( 42 , 52 , 72 , 82 , 88 , 121 ) The majority of these studies used a convolutional neural network AI model, which is a DL method that does not require manual feature extraction from the research team unlike ML algorithms ( 15 , 29 ). Most of these studies also used Scheimpflug-based corneal tomography scans or indices as their model’s input. This may be explained by the ability of Scheimpflug-based corneal tomography to provide additional information about the posterior cornea, which has been shown to exhibit ectatic changes earlier than the anterior cornea in keratoconus, allowing for earlier diagnosis ( 142 ). Additionally, the Belin ABCD classification system (A: anterior radius of curvature, B: back (posterior) radius of curvature, C: minimal corneal thickness, and D: best spectacle distance visual acuity) was developed to monitor disease progression evident on the posterior corneal surface in the absence of anterior corneal surface changes ( 142 ). Given that the aforementioned studies demonstrated relatively high diagnostic accuracy of DL algorithms trained on tomography input, further refinement and validation of AI systems evaluating information from Scheimpflug-based tomography may be adopted in clinical practice to streamline the identification of patients with subclinical keratoconus that may benefit from close monitoring for progression to clinical disease. Additionally, a mobile-based application with this technology can be used to develop a screening program for subclinical keratoconus and other corneal ectasia in low-resource countries.
AI models have been less commonly used in other contexts, such as identifying genetic susceptibility and predicting disease progression. Unlike disease diagnosis, these contexts may rely on model input that involves more subjective elements, such as demographic, environmental risk factors, and other clinical factors. For example, Kundu et al. recently developed an AI model using the random forest algorithm to predict progressive keratoconus using clinical and ocular surface risk factors determined from a patient questionnaire ( 129 ). Their system demonstrated an AUC of 0.81, and 76% of cases classified as progressive by an AI model trained on tomographic changes were also classified as progressive by the clinical risk factors AI model ( 129 ). Within each nondiagnostic context, the AI systems demonstrated relative variability in their performance in completing classification or prediction tasks, and further research is needed to draw conclusions regarding the application of AI models in nondiagnostic contexts for keratoconus. In the future, validated AI models trained on environmental and clinical risk factors could be particularly useful in predicting disease progression to identify patients at risk for severe disease. These identified patients may potentially benefit from prophylactic corneal crosslinking to strengthen corneal integrity and reduce risk of progression.
Some studies included in this review were limited by their design. For example, only one specialist determined the presence of keratoconus for the reference standard of some diagnostic accuracy studies, including Almeida Jr. et al., Lucena et al., Lopes et al., Chandapura et al., Cohen et al., Zéboulon et al., Mosa et al., and Zaki et al. ( 45 , 47 , 49 , 53 , 54 , 75 , 87 , 93 ) This could have resulted in biased classification of eyes. Additionally, some studies included a relatively low sample size of study groups, which reduced the power of the study. Ahn et al. included only 69, 39, and 43 patients in the subclinical keratoconus study group in the training, internal, and external datasets, respectively ( 44 ). Other studies, such as Cohen et al., do not include parameters for demographic information, such as age, sex, or race/ethnicity which can affect the baseline corneal curvature and thickness ( 54 , 143 – 145 ).
The strengths of this study lie in the comprehensiveness of clinical contexts included in this systematic review. While previously published reviews regarding the application of AI in keratoconus and other corneal ectasia focus on diagnostic accuracy ( 146 – 149 ), we also included studies regarding grading disease severity, predicting disease progression, understanding etiology and pathogenesis, and predicting response to treatment or management. However, our review has several limitations. To begin with, except for nine studies published prior to 2013, the majority of studies included in our review were published within the last decade. This may be explained by the fact that the application of ML/DL in medicine, including ophthalmology, has grown in popularity more recently ( 150 ). Furthermore, we searched a limited number of medical databases, including PubMed, Embase, Web of Science, and Cochrane. Studies meeting our eligibility criteria but not indexed in these databases may have been excluded from this review. While we completed a qualitative data extraction among included studies, we were unable to conduct a meta-analysis with statistical methodology given the contextual and methodological variation between studies and their AI systems. Lastly, some studies included in this review were based on specific patient populations with low diversity, which decreases the generalizability of this review’s findings.
With the incorporation of ML/DL AI algorithms and advancements in corneal imaging technology, the potential ability of clinicians to detect and treat keratoconus at earlier stages to prevent disease progression is promising. AI can also be used to determine ectasia severity, identify susceptibility genes, categorize the keratoconus as progressive or non-progressive, and predict response to surgical management. These applications are particularly important for low-resource nations which may have a scarcity of cornea specialists. In these underserved areas, the incorporation of validated AI models could help reduce the rate of missed or delayed corneal ectasia diagnoses. For example, validated smartphone applications could allow for longitudinal at-home screening of keratoconus among patients with risk factors for the condition. Looking ahead, some clinical applications of AI include using multimodal patient data (a combination of corneal images, demographic information, and environmental risk factors) as the input to determine the keratoconus stage and track disease progression.
Additionally, AI can be used in the surgical planning of some therapeutic options for keratoconus that are growing in popularity, such as ray-tracing-guided transepithelial photorefractive keratectomy with accelerated crosslinking, which may help reduce refractive overcorrection and stromal tissue ablation ( 151 ). Mazzotta et al. used a tissue-preservation algorithm involving ray tracing among 38 patients with stable keratoconus undergoing this procedure ( 151 ). They reported that the algorithm and surgical treatment significantly improved visual outcomes in their cohort ( 151 ). This demonstrates that AI-based algorithms could personalize surgical planning to improve postoperative outcomes, so the implementation of these algorithms for other surgical procedures for visual rehabilitation in keratoconus patients should be investigated.
Data availability statement
The original contributions presented in the study are included in the article/ Supplementary Material . Further inquiries can be directed to the corresponding author.
Author contributions
DG: Investigation, Writing – original draft, Writing – review & editing. AYZ: Conceptualization, Methodology, Supervision, Writing – original draft, Writing – review & editing.
The author(s) declare that no financial support was received for the research, authorship, and/or publication of this article.
Conflict of interest
The authors declare that the research was conducted in the absence of any commercial or financial relationships that could be construed as a potential conflict of interest.
Publisher’s note
All claims expressed in this article are solely those of the authors and do not necessarily represent those of their affiliated organizations, or those of the publisher, the editors and the reviewers. Any product that may be evaluated in this article, or claim that may be made by its manufacturer, is not guaranteed or endorsed by the publisher.
Supplementary material
The Supplementary Material for this article can be found online at: https://www.frontiersin.org/articles/10.3389/fopht.2024.1380701/full#supplementary-material
1. Asimellis G, Kaufman EJ. Keratoconus . Treasure Island (FL: StatPearls Publishing Copyright © 2023, StatPearls Publishing LLC (2023).
Google Scholar
2. Ferdi AC, Nguyen V, Gore DM, Allan BD, Rozema JJ, Watson SL. Keratoconus natural progression: A systematic review and meta-analysis of 11 529 eyes. Ophthalmology . (2019) 126:935–45. doi: 10.1016/j.ophtha.2019.02.029
PubMed Abstract | CrossRef Full Text | Google Scholar
3. Antunes-Foschini R, Doná H, de Mello PHSA, Pereira RB, Marqueis IM, Rocha EM, et al. Natural history and predictors for progression in pediatric keratoconus. Sci Rep . (2023) 13:4940. doi: 10.1038/s41598-023-32176-5
4. Mas Tur V, MacGregor C, Jayaswal R, O’Brart D, Maycock N. A review of keratoconus: Diagnosis, pathophysiology, and genetics. Survey Ophthalmol . (2017) 62:770–83. doi: 10.1016/j.survophthal.2017.06.009
CrossRef Full Text | Google Scholar
5. Santodomingo-Rubido J, Carracedo G, Suzaki A, Villa-Collar C, Vincent SJ, Wolffsohn JS. Keratoconus: An updated review. Contact Lens Anterior Eye . (2022) 45:101559. doi: 10.1016/j.clae.2021.101559
6. Hashemi H, Heydarian S, Hooshmand E, Saatchi M, Yekta A, Aghamirsalim M, et al. The prevalence and risk factors for keratoconus: A systematic review and meta-analysis. Cornea . (2020) 39:263–70. doi: 10.1097/ICO.0000000000002150
7. Belin MW, Ambrósio R. Scheimpflug imaging for keratoconus and ectatic disease. Indian J Ophthalmol . (2013) 61:401–6. doi: 10.4103/0301-4738.116059
8. Cavas-Martínez F, de la Cruz Sánchez E, Nieto Martínez J, Fernández Cañavate FJ, Fernández-Pacheco DG. Corneal topography in keratoconus: state of the art. Eye Vis (Lond) . (2016) 3:5. doi: 10.1186/s40662-016-0036-8
9. Hashemi H, Mehravaran S. Day to day clinically relevant corneal elevation, thickness, and curvature parameters using the orbscan II scanning slit topographer and the pentacam scheimpflug imaging device. Middle East Afr J Ophthalmol . (2010) 17:44–55. doi: 10.4103/0974-9233.61216
10. Alghamdi A, Khan MS, Dakhil TA. Understanding corneal epithelial thickness mapping. Middle East Afr J Ophthalmol . (2022) 29:147–55. doi: 10.4103/meajo.meajo_207_22
11. Masiwa LE, Moodley V. A review of corneal imaging methods for the early diagnosis of pre-clinical Keratoconus. J Optom . (2020) 13:269–75. doi: 10.1016/j.optom.2019.11.001
12. Bui AD, Truong A, Pasricha ND, Indaram M. Keratoconus diagnosis and treatment: recent advances and future directions. Clin Ophthalmol . (2023) 17:2705–18. doi: 10.2147/OPTH.S392665
13. Honavar SG. Artificial intelligence in ophthalmology - Machines think! Indian J Ophthalmol . (2022) 70:1075–9. doi: 10.4103/ijo.IJO_644_22
14. Siddiqui AA, Ladas JG, Lee JK. Artificial intelligence in cornea, refractive, and cataract surgery. Curr Opin Ophthalmol . (2020) 31:253–60. doi: 10.1097/ICU.0000000000000673
15. Rampat R, Deshmukh R, Chen X, Ting DSW, Said DG, Dua HS, et al. Artificial intelligence in cornea, refractive surgery, and cataract: basic principles, clinical applications, and future directions. Asia Pac J Ophthalmol (Phila) . (2021) 10:268–81. doi: 10.1097/APO.0000000000000394
16. Erickson BJ. Basic artificial intelligence techniques: machine learning and deep learning. Radiol Clin North Am . (2021) 59:933–40. doi: 10.1016/j.rcl.2021.06.004
17. Sidey-Gibbons JAM, Sidey-Gibbons CJ. Machine learning in medicine: a practical introduction. BMC Med Res Methodol . (2019) 19:64. doi: 10.1186/s12874-019-0681-4
18. Singh Pathania Y, Budania A. Artificial intelligence in dermatology: “unsupervised” versus “supervised” machine learning. Int J Dermatol . (2021) 60:e28–e9. doi: 10.1111/ijd.15288
19. Shi S, An X, Li Y. Ultrasound radiomics-based logistic regression model to differentiate between benign and Malignant breast nodules. J Ultrasound Med . (2023) 42:869–79. doi: 10.1002/jum.16078
20. Baştanlar Y, Ozuysal M. Introduction to machine learning. Methods Mol Biol . (2014) 1107:105–28. doi: 10.1007/978-1-62703-748-8_7
21. Venkatasubramaniam A, Wolfson J, Mitchell N, Barnes T, JaKa M, French S. Decision trees in epidemiological research. Emerg Themes Epidemiol . (2017) 14:11. doi: 10.1186/s12982-017-0064-4
22. Sankari ES, Manimegalai D. Predicting membrane protein types using various decision tree classifiers based on various modes of general PseAAC for imbalanced datasets. J Theor Biol . (2017) 435:208–17. doi: 10.1016/j.jtbi.2017.09.018
23. Rigatti SJ. Random forest. J Insur Med . (2017) 47:31–9. doi: 10.17849/insm-47-01-31-39.1
24. Jin Y, Lan A, Dai Y, Jiang L, Liu S. Development and testing of a random forest-based machine learning model for predicting events among breast cancer patients with a poor response to neoadjuvant chemotherapy. Eur J Med Res . (2023) 28:394. doi: 10.1186/s40001-023-01361-7
25. Zhang S, Li Z, Xin XG. [Support vector machine?assisted diagnosis of human Malignant gastric tissues based on dielectric properties]. Nan Fang Yi Ke Da Xue Xue Bao . (2017) 37:1637–42. doi: 10.3969/j.issn.1673-4254.2017.12.13
26. Abirami S, Chitra P. Chapter Fourteen - Energy-efficient edge based real-time healthcare support system. In: Raj P, Evangeline P, editors. Advances in computers , vol. 117 . Cambridge, Massachusetts: Elsevier (2020). p. 339–68.
27. Choi RY, Coyner AS, Kalpathy-Cramer J, Chiang MF, Campbell JP. Introduction to machine learning, neural networks, and deep learning. Transl Vis Sci Technol . (2020) 9:14. doi: 10.1167/tvst.9.2.14
28. Nistal-Nuño B. Outcome prediction for critical care patients with respiratory neoplasms using a multilayer perceptron neural network. Einstein (Sao Paulo) . (2023) 21:eAO0071. doi: 10.31744/einstein_journal/2023AO0071
29. Sarker IH. Deep learning: A comprehensive overview on techniques, taxonomy, applications and research directions. SN Comput Sci . (2021) 2:420. doi: 10.1007/s42979-021-00815-1
30. Heilbroner SP, Miotto R. Deep learning in medicine. Clin J Am Soc Nephrol . (2023) 18:397–9. doi: 10.2215/CJN.0000000000000080
31. Elsawy A, Abdel-Mottaleb M. A novel network with parallel resolution encoders for the diagnosis of corneal diseases. IEEE Trans BioMed Eng . (2021) 68:3671–80. doi: 10.1109/TBME.2021.3082152
32. Zou KH, O’Malley AJ, Mauri L. Receiver-operating characteristic analysis for evaluating diagnostic tests and predictive models. Circulation . (2007) 115:654–7. doi: 10.1161/CIRCULATIONAHA.105.594929
33. Ng Wei Y, Zhang S, Wang Z, Ong Charles Jit T, Gunasekeran Dinesh V, Lim Gilbert Yong S, et al. Updates in deep learning research in ophthalmology. Clin Sci . (2021) 135:2357–76. doi: 10.1042/CS20210207
34. Ting DSW, Pasquale LR, Peng L, Campbell JP, Lee AY, Raman R, et al. Artificial intelligence and deep learning in ophthalmology. Br J Ophthalmol . (2019) 103:167–75. doi: 10.1136/bjophthalmol-2018-313173
35. Ting DSJ, Foo VH, Yang LWY, Sia JT, Ang M, Lin H, et al. Artificial intelligence for anterior segment diseases: Emerging applications in ophthalmology. Br J Ophthalmol . (2021) 105:158–68. doi: 10.1136/bjophthalmol-2019-315651
36. Gulshan V, Peng L, Coram M, Stumpe MC, Wu D, Narayanaswamy A, et al. Development and validation of a deep learning algorithm for detection of diabetic retinopathy in retinal fundus photographs. Jama . (2016) 316:2402–10. doi: 10.1001/jama.2016.17216
37. Ting DSW, Cheung CY, Lim G, Tan GSW, Quang ND, Gan A, et al. Development and validation of a deep learning system for diabetic retinopathy and related eye diseases using retinal images from multiethnic populations with diabetes. Jama . (2017) 318:2211–23. doi: 10.1001/jama.2017.18152
38. Page MJ, Moher D, Bossuyt PM, Boutron I, Hoffmann TC, Mulrow CD, et al. PRISMA 2020 explanation and elaboration: updated guidance and exemplars for reporting systematic reviews. BMJ . (2021) 372:n160. doi: 10.1136/bmj.n160
39. Whiting PF, Rutjes AW, Westwood ME, Mallett S, Deeks JJ, Reitsma JB, et al. QUADAS-2: a revised tool for the quality assessment of diagnostic accuracy studies. Ann Intern Med . (2011) 155:529–36. doi: 10.7326/0003-4819-155-8-201110180-00009
40. Lee J, Mulder F, Leeflang M, Wolff R, Whiting P, Bossuyt PM. QUAPAS: an adaptation of the QUADAS-2 tool to assess prognostic accuracy studies. Ann Intern Med . (2022) 175:1010–8. doi: 10.7326/M22-0276
41. Mohammadpour M, Heidari Z, Hashemi H, Yaseri M, Fotouhi A. Comparison of artificial intelligence-based machine learning classifiers for early detection of keratoconus. Eur J Ophthalmol . (2022) 32:1352–60. doi: 10.1177/11206721211073442
42. Tan Z, Chen X, Li K, Liu Y, Cao H, Li J, et al. Artificial intelligence-based diagnostic model for detecting keratoconus using videos of corneal force deformation. Transl Vis Sci Technol . (2022) 11:32. doi: 10.1167/tvst.11.9.32
43. Xu Z, Feng R, Jin X, Hu H, Ni S, Xu W, et al. Evaluation of artificial intelligence models for the detection of asymmetric keratoconus eyes using Scheimpflug tomography. Clin Exp Ophthalmol . (2022) 50:714–23. doi: 10.1111/ceo.14126
44. Ahn H, Kim NE, Chung JL, Kim YJ, Jun I, Kim TI, et al. Patient selection for corneal topographic evaluation of keratoconus: A screening approach using artificial intelligence. Front Med (Lausanne) . (2022) 9:934865. doi: 10.3389/fmed.2022.934865
45. Almeida GC Jr., Guido RC, Balarin Silva HM, Brandão CC, de Mattos LC, Lopes BT, et al. New artificial intelligence index based on Scheimpflug corneal tomography to distinguish subclinical keratoconus from healthy corneas. J Cataract Refract Surg . (2022) 48:1168–74. doi: 10.1097/j.jcrs.0000000000000946
46. Kundu G, Shetty R, Khamar P, Mullick R, Gupta S, Nuijts R, et al. Universal architecture of corneal segmental tomography biomarkers for artificial intelligence-driven diagnosis of early keratoconus. Br J Ophthalmol . (2023) 107:635–43. doi: 10.1136/bjophthalmol-2021-319309
47. Lucena AR, Araújo MO, Carneiro RFL, Cavalcante TDS, Ribeiro ABN, Anselmo FJM. Development of an application for providing corneal topography reports based on artificial intelligence. Arq Bras Oftalmol . (2021) 85:351–8. doi: 10.5935/0004-2749.20220051
48. Issarti I, Consejo A, Jiménez-García M, Hershko S, Koppen C, Rozema JJ. Computer aided diagnosis for suspect keratoconus detection. Comput Biol Med . (2019) 109:33–42. doi: 10.1016/j.compbiomed.2019.04.024
49. Lopes BT, Ramos IC, Salomão MQ, Guerra FP, Schallhorn SC, Schallhorn JM, et al. Enhanced tomographic assessment to detect corneal ectasia based on artificial intelligence. Am J Ophthalmol . (2018) 195:223–32. doi: 10.1016/j.ajo.2018.08.005
50. Lu NJ, Elsheikh A, Rozema JJ, Hafezi N, Aslanides IM, Hillen M, et al. Combining spectral-domain OCT and air-puff tonometry analysis to diagnose keratoconus. J Refract Surg . (2022) 38:374–80. doi: 10.3928/1081597X-20220414-02
51. Lavric A, Valentin P. KeratoDetect: keratoconus detection algorithm using convolutional neural networks. Comput Intell Neurosci . (2019) 2019:8162567. doi: 10.1109/ECAI46879.2019
52. Kuo BI, Chang WY, Liao TS, Liu FY, Liu HY, Chu HS, et al. Keratoconus screening based on deep learning approach of corneal topography. Transl Vis Sci Technol . (2020) 9:53. doi: 10.1167/tvst.9.2.53
53. Chandapura R, Salomao MQ, Ambrosio R Jr., Swarup R, Shetty R, Sinha Roy A. Bowman’s topography for improved detection of early ectasia. J Biophotonics . (2019) 12:e201900126. doi: 10.1002/jbio.201900126
54. Cohen E, Bank D, Sorkin N, Giryes R, Varssano D. Use of machine learning to achieve keratoconus detection skills of a corneal expert. Int Ophthalmol . (2022) 42:3837–47. doi: 10.1007/s10792-022-02404-4
55. Ambrósio R Jr., Lopes BT, Faria-Correia F, Salomão MQ, Bühren J, Roberts CJ, et al. Integration of scheimpflug-based corneal tomography and biomechanical assessments for enhancing ectasia detection. J Refract Surg . (2017) 33:434–43. doi: 10.3928/1081597X-20170426-02
56. Gao HB, Pan ZG, Shen MX, Lu F, Li H, Zhang XQ. KeratoScreen: early keratoconus classification with zernike polynomial using deep learning. Cornea . (2022) 41:1158–65. doi: 10.1097/ICO.0000000000003038
57. Subramanian P, Ramesh GP. Keratoconus classification with convolutional neural networks using segmentation and index quantification of eye topography images by particle swarm optimisation. BioMed Res Int . (2022) 2022:8119685. doi: 10.1155/2022/8119685
58. Lu NJ, Koppen C, Hafezi F, Ni Dhubhghaill S, Aslanides IM, Wang QM, et al. Combinations of Scheimpflug tomography, ocular coherence tomography and air-puff tonometry improve the detection of keratoconus. Cont Lens Anterior Eye . (2023) 46:101840. doi: 10.1016/j.clae.2023.101840
59. Cao K, Verspoor K, Sahebjada S, Baird PN. Evaluating the performance of various machine learning algorithms to detect subclinical keratoconus. Transl Vis Sci Technol . (2020) 9:24. doi: 10.1167/tvst.9.2.24
60. Smadja D, Touboul D, Cohen A, Doveh E, Santhiago MR, Mello GR, et al. Detection of subclinical keratoconus using an automated decision tree classification. Am J Ophthalmol . (2013) 156:237–46 e1. doi: 10.1016/j.ajo.2013.03.034
61. Karimi A, Meimani N, Razaghi R, Rahmati SM, Jadidi K, Rostami M. Biomechanics of the healthy and keratoconic corneas: A combination of the clinical data, finite element analysis, and artificial neural network. Curr Pharm Des . (2018) 24:4474–83. doi: 10.2174/1381612825666181224123939
62. Ruiz Hidalgo I, Rozema JJ, Saad A, Gatinel D, Rodriguez P, Zakaria N, et al. Validation of an objective keratoconus detection system implemented in a scheimpflug tomographer and comparison with other methods. Cornea . (2017) 36:689–95. doi: 10.1097/ICO.0000000000001194
63. Maeda N, Klyce SD, Smolek MK. Comparison of methods for detecting keratoconus using videokeratography. Arch Ophthalmol . (1995) 113:870–4. doi: 10.1001/archopht.1995.01100070044023
64. Cao K, Verspoor K, Chan E, Daniell M, Sahebjada S, Baird PN. Machine learning with a reduced dimensionality representation of comprehensive Pentacam tomography parameters to identify subclinical keratoconus. Comput Biol Med . (2021) 138:104884. doi: 10.1016/j.compbiomed.2021.104884
65. Maeda N, Klyce SD, Smolek MK, Thompson HW. Automated keratoconus screening with corneal topography analysis. Invest Ophthalmol Vis Sci . (1994) 35:2749–57.
PubMed Abstract | Google Scholar
66. Feng R, Xu Z, Zheng X, Hu H, Jin X, Chen DZ, et al. KerNet: A novel deep learning approach for keratoconus and sub-clinical keratoconus detection based on raw data of the pentacam HR system. IEEE J BioMed Health Inform . (2021) 25:3898–910. doi: 10.1109/JBHI.2021.3079430
67. Al-Timemy AH, Mosa ZM, Alyasseri Z, Lavric A, Lui MM, Hazarbassanov RM, et al. A hybrid deep learning construct for detecting keratoconus from corneal maps. Transl Vis Sci Technol . (2021) 10:16. doi: 10.1167/tvst.10.14.16
68. Elsawy A, Eleiwa T, Chase C, Ozcan E, Tolba M, Feuer W, et al. Multidisease deep learning neural network for the diagnosis of corneal diseases. Am J Ophthalmol . (2021) 226:252–61. doi: 10.1016/j.ajo.2021.01.018
69. Fassbind B, Langenbucher A, Streich A. Automated cornea diagnosis using deep convolutional neural networks based on cornea topography maps. Sci Rep . (2023) 13:6566. doi: 10.1038/s41598-023-33793-w
70. Kovacs I, Mihaltz K, Kranitz K, Juhasz E, Takacs A, Dienes L, et al. Accuracy of machine learning classifiers using bilateral data from a Scheimpflug camera for identifying eyes with preclinical signs of keratoconus. J Cataract Refract Surg . (2016) 42:275–83. doi: 10.1016/j.jcrs.2015.09.020
71. Leao E, Ing Ren T, Lyra JM, MaChado A, Koprowski R, Lopes B, et al. Corneal deformation amplitude analysis for keratoconus detection through compensation for intraocular pressure and integration with horizontal thickness profile. Comput Biol Med . (2019) 109:263–71. doi: 10.1016/j.compbiomed.2019.04.019
72. Kamiya K, Ayatsuka Y, Kato Y, Fujimura F, Takahashi M, Shoji N, et al. Keratoconus detection using deep learning of colour-coded maps with anterior segment optical coherence tomography: a diagnostic accuracy study. BMJ Open . (2019) 9:e031313. doi: 10.1136/bmjopen-2019-031313
73. Maeda N, Klyce SD, Smolek MK. Neural network classification of corneal topography. Preliminary demonstration. Invest Ophthalmol Vis Sci . (1995) 36:1327–35.
74. Prakash G, Perera C, Jhanji V. Comparison of machine learning-based algorithms using corneal asymmetry vs. single-metric parameters for keratoconus detection. Graefes Arch Clin Exp Ophthalmol . (2023) 261:2335–42. doi: 10.1007/s00417-023-06049-6
75. Zeboulon P, Debellemaniere G, Bouvet M, Gatinel D. Corneal topography raw data classification using a convolutional neural network. Am J Ophthalmol . (2020) 219:33–9. doi: 10.1016/j.ajo.2020.06.005
76. Ruiz Hidalgo I, Rodriguez P, Rozema JJ, ND S, Zakaria N, Tassignon MJ, et al. Evaluation of a machine-learning classifier for keratoconus detection based on scheimpflug tomography. Cornea . (2016) 35:827–32. doi: 10.1097/ICO.0000000000000834
77. Souza MB, Medeiros FW, Souza DB, Garcia R, Alves MR. Evaluation of machine learning classifiers in keratoconus detection from orbscan II examinations. Clinics (Sao Paulo) . (2010) 65:1223–8. doi: 10.1590/S1807-59322010001200002
78. Accardo PA, Pensiero S. Neural network-based system for early keratoconus detection from corneal topography. J BioMed Inform . (2002) 35:151–9. doi: 10.1016/S1532-0464(02)00513-0
79. Castro-Luna G, Jimenez-Rodriguez D, Castano-Fernandez AB, Perez-Rueda A. Diagnosis of subclinical keratoconus based on machine learning techniques. J Clin Med . (2021) 10:4281. doi: 10.3390/jcm10184281
80. Shi C, Wang M, Zhu T, Zhang Y, Ye Y, Jiang J, et al. Machine learning helps improve diagnostic ability of subclinical keratoconus using Scheimpflug and OCT imaging modalities. Eye Vis (Lond) . (2020) 7:48. doi: 10.1186/s40662-020-00213-3
81. Wan Q, Wei R, Ma K, Yin H, Deng YP, Tang J. Deep learning-based automatic diagnosis of keratoconus with corneal endothelium image. Ophthalmol Ther . (2023) 12:3047–65. doi: 10.1007/s40123-023-00795-w
82. Al-Timemy AH, Alzubaidi L, Mosa ZM, Abdelmotaal H, Ghaeb NH, Lavric A, et al. A deep feature fusion of improved suspected keratoconus detection with deep learning. Diagnostics (Basel) . (2023) 13:1689. doi: 10.3390/diagnostics13101689
83. Castro-Luna GM, Martinez-Finkelshtein A, Ramos-Lopez D. Robust keratoconus detection with Bayesian network classifier for Placido-based corneal indices. Cont Lens Anterior Eye . (2020) 43:366–72. doi: 10.1016/j.clae.2019.12.006
84. Abdelmotaal H, Mostafa MM, Mostafa ANR, Mohamed AA, Abdelazeem K. Classification of color-coded scheimpflug camera corneal tomography images using deep learning. Transl Vis Sci Technol . (2020) 9:30. doi: 10.1167/tvst.9.13.30
85. Twa MD, Parthasarathy S, Roberts C, Mahmoud AM, Raasch TW, Bullimore MA. Automated decision tree classification of corneal shape. Optom Vis Sci . (2005) 82:1038–46. doi: 10.1097/01.opx.0000192350.01045.6f
86. Fırat M, Çankaya C, Çınar A, Tuncer T. Automatic detection of keratoconus on Pentacam images using feature selection based on deep learning. Int J Imaging Syst Technol . (2022) 32:1548–60. doi: 10.1002/ima.22717
87. Mosa ZM, Ghaeb NH, Ali AH. Detecting keratoconus by using SVM and decision tree classifiers with the aid of image processing. Baghdad Sci J . (2019) 16:1022–9. doi: 10.21123/bsj.2019.16.4(Suppl.)
88. Ambrosio R Jr., MaChado AP, Leao E, Lyra JMG, Salomao MQ, Esporcatte LGP, et al. Optimized artificial intelligence for enhanced ectasia detection using scheimpflug-based corneal tomography and biomechanical data. Am J Ophthalmol . (2023) 251:126–42. doi: 10.1016/j.ajo.2022.12.016
89. Lavric A, Popa V, Takahashi H, Yousefi S. Detecting keratoconus from corneal imaging data using machine learning. IEEE Access . (2020) 8:149113–21. doi: 10.1109/Access.6287639
90. de Almeida GC Jr., Guido RC, Neto JS, Rosa JM, Castiglioni L, de Mattos LC, et al. Corneal Tomography Multivariate Index (CTMVI) effectively distinguishes healthy corneas from those susceptible to ectasia. Biomed Signal Process Control . (2021) 70. doi: 10.1016/j.bspc.2021.102995
91. Zeboulon P, Debellemaniere G, Gatinel D. Unsupervised learning for large-scale corneal topography clustering. Sci Rep . (2020) 10:16973. doi: 10.1038/s41598-020-73902-7
92. Al-Timemy AH, Ghaeb NH, Mosa ZM, Escudero J. Deep transfer learning for improved detection of keratoconus using corneal topographic maps. Cogn Computation . (2021) 14:1627–42. doi: 10.1007/s12559-021-09880-3
93. Zaki WMD W, Mat Daud M, Saad AH, Hussain A. Abdul mutalib H. A mobile solution for lateral segment photographed images based deep keratoconus screening method. Int J Integrated Eng . (2021) 13:18–27. doi: 10.30880/ijie.2021.013.05.003
94. Alshammari G, Hamad AA, Abdullah ZM, Alshareef AM, Alhebaishi N, Alshammari A, et al. Applications of deep learning on topographic images to improve the diagnosis for dynamic systems and unconstrained optimization. Wireless Commun Mobile Computing . (2021) 2021:1–7. doi: 10.1155/2021/4672688
95. Abdelmotaal H, Abdou AA, Omar AF, El-Sebaity DM, Abdelazeem K. Pix2pix conditional generative adversarial networks for scheimpflug camera color-coded corneal tomography image generation. Transl Vis Sci Technol . (2021) 10:21. doi: 10.1167/tvst.10.7.21
96. Silverman RH, Urs R, Roychoudhury A, Archer TJ, Gobbe M, Reinstein DZ. Epithelial remodeling as basis for machine-based identification of keratoconus. Invest Ophthalmol Vis Sci . (2014) 55:1580–7. doi: 10.1167/iovs.13-12578
97. Abdelmotaal H, Hazarbassanov RM, Salouti R, Nowroozzadeh MH, Taneri S, Al-Timemy AH, et al. Keratoconus detection-based on dynamic corneal deformation videos using deep learning. Ophthalmol Sci . (2024) 4:100380. doi: 10.1016/j.xops.2023.100380
98. Francis M, Shetty R, Padmanabhan P, Vinciguerra R, Vinciguerra P, Lippera M, et al. New simulation software to predict postoperative corneal stiffness before laser vision correction. J Cataract Refract Surg . (2023) 49:620–7. doi: 10.1097/j.jcrs.0000000000001169
99. Velazquez-Blazquez JS, Bolarin JM, Cavas-Martinez F, Alio JL. EMKLAS: A new automatic scoring system for early and mild keratoconus detection. Transl Vis Sci Technol . (2020) 9:30. doi: 10.1167/tvst.9.2.30
100. Santhiago MR, Araujo DC, Stival LR, Smadja D, Veloso AA. Ectasia risk model: A novel method without cut-off point based on artificial intelligence improves detection of higher-risk eyes. J Refract Surg . (2022) 38:716–24. doi: 10.3928/1081597X-20221018-01
101. Reinstein DZ, Archer TJ, Gobbe M. Corneal epithelial thickness profile in the diagnosis of keratoconus. J Refract Surg . (2009) 25:604–10. doi: 10.3928/1081597X-20090610-06
102. Li Y, Li M, Li C, Liu Z. Forest aboveground biomass estimation using Landsat 8 and Sentinel-1A data with machine learning algorithms. Sci Rep . (2020) 10:9952. doi: 10.1038/s41598-020-67024-3
103. Arik SÖ, Pfister T. TabNet: attentive interpretable tabular learning. ArXiv . (2019). doi: 10.48550/arXiv.1908.07442
104. Ghaderi M, Sharifi A, Jafarzadeh Pour E. Proposing an ensemble learning model based on neural network and fuzzy system for keratoconus diagnosis based on Pentacam measurements. Int Ophthalmol . (2021) 41:3935–48. doi: 10.1007/s10792-021-01963-2
105. Saikia MJ, Kuanar S, Mahapatra D, Faghani S. Multi-modal ensemble deep learning in head and neck cancer HPV sub-typing. Bioengineering (Basel) . (2023) 11:13. doi: 10.3390/bioengineering11010013
106. Xie X, Xie M, Moshayedi AJ, Noori Skandari MH. A hybrid improved neural networks algorithm based on L2 and dropout regularization. Math Problems Engineering . (2022) 2022:8220453. doi: 10.1155/2022/8220453
107. Sherazi SWA, Bae J-W, Lee JY. A soft voting ensemble classifier for early prediction and diagnosis of occurrences of major adverse cardiovascular events for STEMI and NSTEMI during 2-year follow-up in patients with acute coronary syndrome. PloS One . (2021) 16:e0249338. doi: 10.1371/journal.pone.0249338
108. Rozema JJ, Rodriguez P, Ruiz Hidalgo I, Navarro R, Tassignon MJ, Koppen C. SyntEyes KTC: higher order statistical eye model for developing keratoconus. Ophthalmic Physiol Opt . (2017) 37:358–65. doi: 10.1111/opo.12369
109. Hosoda Y, Miyake M, Meguro A, Tabara Y, Iwai S, Ueda-Arakawa N, et al. Keratoconus-susceptibility gene identification by corneal thickness genome-wide association study and artificial intelligence IBM Watson. Commun Biol . (2020) 3:410. doi: 10.1038/s42003-020-01137-3
110. Wang L, Wang Y, Liu J, Zhao W. Identification of important genes of keratoconus and construction of the diagnostic model. Genet Res (Camb) . (2022) 2022:5878460. doi: 10.1155/2022/5878460
111. Nokas G, Kotsilieris T. Preventing keratoconus through eye rubbing activity detection: A machine learning approach. Electronics . (2023) 12:1028. doi: 10.3390/electronics12041028
112. Kenney MC, Nesburn AB, Burgeson RE, Butkowski RJ, Ljubimov AV. Abnormalities of the extracellular matrix in keratoconus corneas. Cornea . (1997) 16:345–51. doi: 10.1097/00003226-199705000-00016
113. Herber R, Pillunat LE, Raiskup F. Development of a classification system based on corneal biomechanical properties using artificial intelligence predicting keratoconus severity. Eye Vis (Lond) . (2021) 8:21. doi: 10.1186/s40662-021-00244-4
114. Yousefi S, Yousefi E, Takahashi H, Hayashi T, Tampo H, Inoda S, et al. Keratoconus severity identification using unsupervised machine learning. PloS One . (2018) 13:e0205998. doi: 10.1371/journal.pone.0205998
115. Mahmoud HAH, Mengash HA. Automated keratoconus detection by 3D corneal images reconstruction. Sensors (Basel) . (2021) 21:2326. doi: 10.3390/s21072326
116. Issarti I, Consejo A, Jiménez-García M, Kreps EO, Koppen C, Rozema JJ. Logistic index for keratoconus detection and severity scoring (Logik). Comput Biol Med . (2020) 122:103809. doi: 10.1016/j.compbiomed.2020.103809
117. Aatila M, Lachgar M, Hamid H, Kartit A. Keratoconus severity classification using features selection and machine learning algorithms. Comput Math Methods Med . (2021) 2021:9979560. doi: 10.1155/2021/9979560
118. Malyugin B, Sakhnov S, Izmailova S, Boiko E, Pozdeyeva N, Axenova L, et al. Keratoconus diagnostic and treatment algorithms based on machine-learning methods. Diagnostics (Basel) . (2021) 11:1933. doi: 10.3390/diagnostics11101933
119. Tai HY, Lin JJ, Huang YH, Shih PJ, Wang IJ, Yen JY. Correlation between corneal dynamic responses and keratoconus topographic parameters. J Int Med Res . (2022) 50:3000605221108100. doi: 10.1177/03000605221108100
120. Kamiya K, Ayatsuka Y, Kato Y, Shoji N, Mori Y, Miyata K. Diagnosability of keratoconus using deep learning with placido disk-based corneal topography. Front Med (Lausanne) . (2021) 8:724902. doi: 10.3389/fmed.2021.724902
121. Chen X, Zhao J, Iselin KC, Borroni D, Romano D, Gokul A, et al. Keratoconus detection of changes using deep learning of colour-coded maps. BMJ Open Ophthalmol . (2021) 6:e000824. doi: 10.1136/bmjophth-2021-000824
122. Dos Santos VA, Schmetterer L, Stegmann H, Pfister M, Messner A, Schmidinger G, et al. CorneaNet: fast segmentation of cornea OCT scans of healthy and keratoconic eyes using deep learning. BioMed Opt Express . (2019) 10:622–41. doi: 10.1364/BOE.10.000622
123. Dong Y, Li D, Guo Z, Liu Y, Lin P, Lv B, et al. Dissecting the profile of corneal thickness with keratoconus progression based on anterior segment optical coherence tomography. Front Neurosci . (2021) 15:804273. doi: 10.3389/fnins.2021.804273
124. Lavric A, Anchidin L, Popa V, Al-Timemy AH, Alyasseri Z, Takahashi H, et al. Keratoconus severity detection from elevation, topography and pachymetry raw data using a machine learning approach. IEEE Access . (2021) 9:84344–55. doi: 10.1109/ACCESS.2021.3086021
125. Bolarín JM, Cavas F, Velázquez JS, Alió JL. A machine-learning model based on morphogeometric parameters for RETICS disease classification and GUI development. Appl Sci . (2020) 10:1874. doi: 10.3390/app10051874
126. Zorto AD, Sharif MS, Wall J, Brahma A, Alzahrani AI, Alalwan N. An innovative approach based on machine learning to evaluate the risk factors importance in diagnosing keratoconus. Inf Med Unlocked . (2023) 38. doi: 10.1016/j.imu.2023.101208
127. Smolek MK, Klyce SD. Current keratoconus detection methods compared with a neural network approach. Invest Ophthalmol Vis Sci . (1997) 38:2290–9.
128. Werkmeister RM, Sapeta S, Schmidl D, Garhöfer G, Schmidinger G, Aranha Dos Santos V, et al. Ultrahigh-resolution OCT imaging of the human cornea. BioMed Opt Express . (2017) 8:1221–39. doi: 10.1364/BOE.8.001221
129. Kundu G, Shetty N, Shetty R, Khamar P, D’Souza S, Meda TR, et al. Artificial intelligence-based stratification of demographic, ocular surface high-risk factors in progression of keratoconus. Indian J Ophthalmol . (2023) 71:1882–8. doi: 10.4103/IJO.IJO_2651_22
130. Shetty R, Kundu G, Narasimhan R, Khamar P, Gupta K, Singh N, et al. Artificial intelligence efficiently identifies regional differences in the progression of tomographic parameters of keratoconic corneas. J Refract Surg . (2021) 37:240–8. doi: 10.3928/1081597X-20210120-01
131. Reddy JC, Bhamidipati P, Dwivedi S, Dhara KK, Joshi V, Hasnat Ali M, et al. KEDOP: Keratoconus early detection of progression using tomography images. Eur J Ophthalmol . (2022) 32:2554–64. doi: 10.1177/11206721221087566
132. Jiménez-García M, Issarti I, Kreps EO, ND S, Koppen C, Varssano D, et al. Forecasting progressive trends in keratoconus by means of a time delay neural network. J Clin Med . (2021) 10:3238. doi: 10.3390/jcm10153238
133. Kamiya K, Ayatsuka Y, Kato Y, Shoji N, Miyai T, Ishii H, et al. Prediction of keratoconus progression using deep learning of anterior segment optical coherence tomography maps. Ann Transl Med . (2021) 9:1287. doi: 10.21037/atm
134. Kato N, Masumoto H, Tanabe M, Sakai C, Negishi K, Torii H, et al. Predicting keratoconus progression and need for corneal crosslinking using deep learning. J Clin Med . (2021) 10:844. doi: 10.3390/jcm10040844
135. Cao K, Verspoor K, Chan E, Daniell M, Sahebjada S, Baird PN. Stratification of keratoconus progression using unsupervised machine learning analysis of tomographical parameters. Intelligence-Based Med . (2023) 7:100095. doi: 10.1016/j.ibmed.2023.100095
136. Fariselli C, Vega-Estrada A, Arnalich-Montiel F, Alio JL. Artificial neural network to guide intracorneal ring segments implantation for keratoconus treatment: a pilot study. Eye Vis (Lond) . (2020) 7:20. doi: 10.1186/s40662-020-00184-5
137. Yousefi S, Takahashi H, Hayashi T, Tampo H, Inoda S, Arai Y, et al. Predicting the likelihood of need for future keratoplasty intervention using artificial intelligence. Ocul Surf . (2020) 18:320–5. doi: 10.1016/j.jtos.2020.02.008
138. Valdés-Mas MA, Martín-Guerrero JD, Rupérez MJ, Pastor F, Dualde C, Monserrat C, et al. A new approach based on Machine Learning for predicting corneal curvature (K1) and astigmatism in patients with keratoconus after intracorneal ring implantation. Comput Methods Programs Biomed . (2014) 116:39–47. doi: 10.1016/j.cmpb.2014.04.003
139. Liu Y, Shen D, Wang HY, Qi MY, Zeng QY. Development and validation to predict visual acuity and keratometry two years after corneal crosslinking with progressive keratoconus by machine learning. Front Med (Lausanne) . (2023) 10:1146529. doi: 10.3389/fmed.2023.1146529
140. Lyra D, Ribeiro G, Torquetti L, Ferrara P, MaChado A, Lyra JM. Computational models for optimization of the intrastromal corneal ring choice in patients with keratoconus using corneal tomography data. J Refract Surg . (2018) 34:547–50. doi: 10.3928/1081597X-20180615-01
141. Firat M, Cinar A, Cankaya C, Firat IT, Tuncer T. Prediction of Pentacam image after corneal cross-linking by linear interpolation technique and U-NET based 2D regression model. Comput Biol Med . (2022) 146:105541. doi: 10.1016/j.compbiomed.2022.105541
142. Belin MW. Chapter 15 - scheimpflug imaging for keratoconus and ectatic disease. In: Izquierdo L, Henriquez M, Mannis M, editors. Keratoconus . Elsevier, New Delhi (2023). p. 203–20.
143. Hashemi H, Asgari S, Emamian MH, Mehravaran S, Fotouhi A. Age-related changes in corneal curvature and shape: the shahroud eye cohort study. Cornea . (2015) 34:1456–8. doi: 10.1097/ICO.0000000000000595
144. Baboolal SO, Smit DP. South African Eye Study (SAES): ethnic differences in central corneal thickness and intraocular pressure. Eye . (2018) 32:749–56. doi: 10.1038/eye.2017.291
145. Alsaqr AM, Fagehi R, Sharha AA, Alkhudair M, Alshabrami A, Muammar AB, et al. Ethnic differences of corneal parameters: A cross-sectional study. Open Ophthalmol J . (2021) 15:13–20. doi: 10.2174/1874364102115010013
146. Pagano L, Posarelli M, Giannaccare G, Coco G, Scorcia V, Romano V, et al. Artificial intelligence in cornea and ocular surface diseases. Saudi J Ophthalmol . (2023) 37:179–84. doi: 10.4103/sjopt.sjopt_52_23
147. Shanthi S, Aruljyothi L, Balasundaram MB, Janakiraman A, Nirmaladevi K, Pyingkodi M. Artificial intelligence applications in different imaging modalities for corneal topography. Surv Ophthalmol . (2022) 67:801–16. doi: 10.1016/j.survophthal.2021.08.004
148. Zhang Z, Wang Y, Zhang H, Samusak A, Rao H, Xiao C, et al. Artificial intelligence-assisted diagnosis of ocular surface diseases. Front Cell Dev Biol . (2023) 11. doi: 10.3389/fcell.2023.1133680
149. Hashemi H, Doroodgar F, Niazi S, Khabazkhoob M, Heidari Z. Comparison of different corneal imaging modalities using artificial intelligence for diagnosis of keratoconus: a systematic review and meta-analysis. Graefes Arch Clin Exp Ophthalmol . (2023) 11:1133680. doi: 10.1007/s00417-023-06154-6
150. Li Z, Wang L, Wu X, Jiang J, Qiang W, Xie H, et al. Artificial intelligence in ophthalmology: The path to the real-world clinic. Cell Rep Med . (2023) 4:101095. doi: 10.1016/j.xcrm.2023.101095
151. Mazzotta C, Stojanovic A, Romano V, Addabbo G, Borroni D, Balamoun AA, et al. Ray-tracing transepithelial excimer laser central corneal remodeling plus pachymetry-guided accelerated corneal crosslinking for keratoconus. Cornea . (2024) 43:285–94. doi: 10.1097/ICO.0000000000003380
Keywords: keratoconus, corneal ectasia, artificial intelligence, machine learning, deep learning
Citation: Goodman D and Zhu AY (2024) Utility of artificial intelligence in the diagnosis and management of keratoconus: a systematic review. Front. Ophthalmol. 4:1380701. doi: 10.3389/fopht.2024.1380701
Received: 02 February 2024; Accepted: 23 April 2024; Published: 17 May 2024.
Reviewed by:
Copyright © 2024 Goodman and Zhu. This is an open-access article distributed under the terms of the Creative Commons Attribution License (CC BY) . The use, distribution or reproduction in other forums is permitted, provided the original author(s) and the copyright owner(s) are credited and that the original publication in this journal is cited, in accordance with accepted academic practice. No use, distribution or reproduction is permitted which does not comply with these terms.
*Correspondence: Angela Y. Zhu, [email protected]
† These authors have contributed equally to this work
Disclaimer: All claims expressed in this article are solely those of the authors and do not necessarily represent those of their affiliated organizations, or those of the publisher, the editors and the reviewers. Any product that may be evaluated in this article or claim that may be made by its manufacturer is not guaranteed or endorsed by the publisher.

Identifying the practice patterns of optometrists in providing falls prevention management: A mixed-methods systematic review protocol
- Find this author on Google Scholar
- Find this author on PubMed
- Search for this author on this site
- ORCID record for Si Ye Lee
- For correspondence: [email protected]
- ORCID record for Khyber Alam
- ORCID record for Jason Charng
- ORCID record for Hamed Niyazmand
- ORCID record for Jingyi Chen
- ORCID record for Anne-Marie Hill
- Info/History
- Preview PDF
Objective The objective of this systematic review is to synthesise the best available evidence for optometrists practice patterns in providing falls prevention management. Introduction Falls remain the main cause of injury related hospitalisation and mortality in Australia and worldwide, significantly affecting older adults. The increased risk of comorbidities, including visual impairment in this cohort is linked to a higher incidence of falls. Despite being primary eye care practitioners, community optometrists may not consistently integrate falls prevention strategies into their practice. Furthermore, the extent to which they adhere to evidence-based recommendations for falls management remains unclear. Inclusion criteria The review will include optometrists, in regions where optometry is a regulated profession, and report their understanding and practice patterns in delivering falls prevention management to older community dwelling adults. Qualitative, quantitative, and mixed methods studies will be eligible for inclusion. It is envisioned that most studies will be qualitative. Studies published in English and those published from 1980 onwards will be eligible for inclusion since published evidence for falls prevention began to increase sharply around this time. Methods The review will follow the JBI guidelines for mixed methods systematic reviews and will be developed and reported in accordance with PRISMA P guidelines. Databases that will be searched are Excerpta Medica Database (Embase), Scopus, Cumulative Index to Nursing and Allied Health Literature (CINAHL) Complete, and OVID MEDLINE. Grey literature will be searched through Conference Proceedings Citation Index (Web of Science), Google Scholar, and ProQuest Dissertations databases. Two reviewers will independently conduct all screening and critical appraisal. The reviewers will screen all articles titles and abstracts retrieved from the searches to determine potential eligibility. All full-text articles considered relevant will then be assessed for final eligibility for inclusion. The final included articles will be assessed for methodological rigour using the JBI SUMARI critical appraisal tools, subsequently, all relevant data will be extracted. Discrepancies at any stage of the procedures will be resolved through discussion and consensus with a third senior researcher. A convergent integrated approach to synthesising and integrating the quantitative and qualitative data will be followed. Review registration CRD42024539668 Keywords Accidental falls, Optometry, Practice Patterns, Aged
Competing Interest Statement
The authors have declared no competing interest.
Funding Statement
Anne-Marie Hill is supported by a National Health and Medical Research Council (NHMRC) of Australia Investigator (EL2) award (GNT1174179) and the Royal Perth Hospital Research Foundation. Si Ye Lee is conducting this research with the support of an Australian Government Research Training Program Fees Offset scholarship and is a recipient of a Perth Eye Foundation scholarship through the University of Western Australia.
Author Declarations
I confirm all relevant ethical guidelines have been followed, and any necessary IRB and/or ethics committee approvals have been obtained.
I confirm that all necessary patient/participant consent has been obtained and the appropriate institutional forms have been archived, and that any patient/participant/sample identifiers included were not known to anyone (e.g., hospital staff, patients or participants themselves) outside the research group so cannot be used to identify individuals.
I understand that all clinical trials and any other prospective interventional studies must be registered with an ICMJE-approved registry, such as ClinicalTrials.gov. I confirm that any such study reported in the manuscript has been registered and the trial registration ID is provided (note: if posting a prospective study registered retrospectively, please provide a statement in the trial ID field explaining why the study was not registered in advance).
I have followed all appropriate research reporting guidelines, such as any relevant EQUATOR Network research reporting checklist(s) and other pertinent material, if applicable.
Data Availability
All data produced in the present work are contained in the manuscript.
View the discussion thread.
Thank you for your interest in spreading the word about medRxiv.
NOTE: Your email address is requested solely to identify you as the sender of this article.

Citation Manager Formats
- EndNote (tagged)
- EndNote 8 (xml)
- RefWorks Tagged
- Ref Manager
- Tweet Widget
- Facebook Like
- Google Plus One
- Addiction Medicine (324)
- Allergy and Immunology (627)
- Anesthesia (163)
- Cardiovascular Medicine (2371)
- Dentistry and Oral Medicine (289)
- Dermatology (206)
- Emergency Medicine (379)
- Endocrinology (including Diabetes Mellitus and Metabolic Disease) (836)
- Epidemiology (11768)
- Forensic Medicine (10)
- Gastroenterology (702)
- Genetic and Genomic Medicine (3736)
- Geriatric Medicine (350)
- Health Economics (633)
- Health Informatics (2395)
- Health Policy (932)
- Health Systems and Quality Improvement (896)
- Hematology (341)
- HIV/AIDS (782)
- Infectious Diseases (except HIV/AIDS) (13308)
- Intensive Care and Critical Care Medicine (767)
- Medical Education (365)
- Medical Ethics (104)
- Nephrology (398)
- Neurology (3501)
- Nursing (198)
- Nutrition (524)
- Obstetrics and Gynecology (674)
- Occupational and Environmental Health (663)
- Oncology (1823)
- Ophthalmology (537)
- Orthopedics (218)
- Otolaryngology (287)
- Pain Medicine (232)
- Palliative Medicine (66)
- Pathology (446)
- Pediatrics (1033)
- Pharmacology and Therapeutics (426)
- Primary Care Research (420)
- Psychiatry and Clinical Psychology (3175)
- Public and Global Health (6138)
- Radiology and Imaging (1280)
- Rehabilitation Medicine and Physical Therapy (747)
- Respiratory Medicine (826)
- Rheumatology (379)
- Sexual and Reproductive Health (372)
- Sports Medicine (323)
- Surgery (402)
- Toxicology (50)
- Transplantation (172)
- Urology (145)
Proceedings of the International Conference on Economic Management, Accounting and Tourism (ICEMAT 2023)
A Systematic Literature Review on Entrepreneurial Intention: PRISMA method
Several studies use Entrepreneurial Intention as a theoretical framework. Thus, it requires a more extension of Entrepreneurial Intention research to be investigated. The Systematic Literature Review (SLR) is one of the methods to find the research gaps. However, none of the researchers comprehensively conducted a Systematic Literature Review on Entrepreneurial Intention. Therefore, this study aims to review the main finding, database, citation, study setting, methodology, unit of analysis, field of study analysis, and underpinning theory of previous studies on Entrepreneurial Intention. A total of 152 articles addressing Entrepreneurial Intention published between 2000 and 2021 have been analyzed. The present study identifies Self-Efficacy as the most often used by authors as an antecedent of Entrepreneurial Intention. Most of the Entrepreneurial Intention research was conducted in a quantitative method specified in the survey method and most of the researchers used Structural Equation Modelling and Multiple Regression for data analysis. Most study has been done in multi-country, dominated by students as a unit of analysis and management, education, business, and economics are the fields of study that frequently use Entrepreneurial Intention as the topic of research. Almost half of the Entrepreneurial Intention research uses the Theory of Planned Behaviour (TPB) as the underpinning theory of the research.
Download article (PDF)
Cite this article
Click through the PLOS taxonomy to find articles in your field.
For more information about PLOS Subject Areas, click here .
Loading metrics
Open Access
Peer-reviewed
Research Article
Effect of topical emollient oil application on weight of preterm newborns: A systematic review and meta-analysis
Roles Conceptualization, Formal analysis, Methodology, Software, Writing – original draft
* E-mail: [email protected] , [email protected]
Affiliation College of Medicine and Health Sciences, Wollo University, Dessie, Ethiopia

Roles Data curation, Methodology, Writing – review & editing
Roles Conceptualization, Methodology, Visualization, Writing – original draft
Roles Data curation, Formal analysis, Methodology, Writing – review & editing
Roles Data curation, Methodology, Software, Writing – review & editing
Roles Conceptualization, Data curation, Formal analysis, Methodology, Software, Writing – original draft
Roles Conceptualization, Methodology, Writing – review & editing
Roles Data curation, Methodology, Writing – original draft
Affiliation College of Health Sciences, Addis Ababa University, Addis Ababa, Ethiopia
Roles Formal analysis, Software, Writing – review & editing
Affiliation College of Health Sciences, Debre Tabor University, Debre Tabor, Ethiopia
Affiliation College of Health Sciences, Dilla University, Dilla, Ethiopia
Roles Conceptualization, Formal analysis, Methodology, Software, Writing – review & editing
Affiliation College of Health Sciences, St. Paul’s Hospital Millennium Medical College, Addis Ababa, Ethiopia
Roles Conceptualization, Formal analysis, Software, Writing – original draft, Writing – review & editing
- Fekadeselassie Belege Getaneh,
- Anissa Mohammed,
- Alemu Gedefie Belete,
- Amare Muche,
- Aznamariyam Ayres,
- Yibeltal Asmamaw,
- Zemen Mengesha,
- Asrat Dimtse,
- Natnael Moges Misganaw,
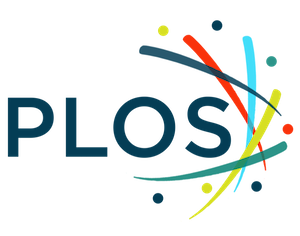
- Published: May 14, 2024
- https://doi.org/10.1371/journal.pone.0302969
- Peer Review
- Reader Comments
Synthesizing current evidence on interventions to improve survival outcomes in preterm infants is crucial for informing programs and policies. The objective of this study is to investigate the impact of topical emollient oil application on the weight of preterm infants.
A systematic review and meta-analysis of randomized controlled trials (RCTs) was conducted. To identify relevant studies, comprehensive searches were conducted across multiple databases, including PubMed, Cochrane, Scopus, Clinical trials, ProQuest Central, Epistemonikos, and gray literature sources. The inclusion criteria were based on the PICO (Population, Intervention, Comparison, and Outcomes) format. Study quality was assessed using the Cochrane risk of bias tool for randomized trials (RoB 2.0). Data analysis was performed using StataCrop MP V.17 software, which included evaluating heterogeneity, conducting subgroup analysis, sensitivity analysis, and meta-regression. The findings were reported in accordance with the PRISMA checklist, and the review was registered with PROSPERO (CRD42023413770).
Out of the initial pool of 2734 articles, a total of 18 studies involving 1454 preterm neonates were included in the final analysis. Fourteen of these studies provided data that contributed to the calculation of the pooled difference in mean weight gain in preterm neonates. The random effects meta-analysis revealed a significant pooled difference in mean weight gain of 52.15 grams (95% CI: 45.96, 58.35), albeit with high heterogeneity (I 2 > 93.24%, p 0.000). Subgroup analyses were conducted, revealing that preterm infants who received massages three times daily with either sunflower oil or coconut oil exhibited greater mean differences in weight gain. Meta-regression analysis indicated that the type of emollient oil, duration of therapy, and frequency of application significantly contributed to the observed heterogeneity. A sensitivity analysis was performed, excluding two outlier studies, resulting in a pooled mean weight difference of 78.57grams (95% CI: 52.46, 104.68). Among the nine studies that reported adverse events, only two mentioned occurrences of rash and accidental slippage in the intervention groups.
The available evidence suggests that the application of topical emollient oil in preterm neonates is likely to be effective in promoting weight gain, with a moderate-to-high level of certainty. Based on these findings, it is recommended that local policymakers and health planners prioritize the routine use of emollient oils in newborn care for preterm infants. By incorporating emollient oils into standard care protocols, healthcare providers can provide additional support to promote optimal growth and development in preterm infants.
Citation: Getaneh FB, Mohammed A, Belete AG, Muche A, Ayres A, Asmamaw Y, et al. (2024) Effect of topical emollient oil application on weight of preterm newborns: A systematic review and meta-analysis. PLoS ONE 19(5): e0302969. https://doi.org/10.1371/journal.pone.0302969
Editor: Rehana Abdus Salam, The University of Sydney, AUSTRALIA
Received: February 16, 2024; Accepted: April 16, 2024; Published: May 14, 2024
Copyright: © 2024 Getaneh et al. This is an open access article distributed under the terms of the Creative Commons Attribution License , which permits unrestricted use, distribution, and reproduction in any medium, provided the original author and source are credited.
Data Availability: All relevant data are within the manuscript and its Supporting Information files.
Funding: The author(s) received no specific funding for this work.
Competing interests: The authors have declared that no competing interests exist.
Abbreviations: Aes, Assess adverse events; AHRQ, Agency for Healthcare Research and Quality; CI, Confidence intervals; DECIDE, Developing and Evaluating Communication strategies to support Informed Decisions and practice based on Evidence; LBW, Low birth weight; MCT, Medium chain triglycerides; MD, Mean difference; NICU, Neonatal intensive care unit; PRISMA, Preferred Reporting Items for Systematic Reviews and Meta-Analysis; RCTs, Randomized control trials; RR, Risk ratio; UNICEF, United Nations International Children’s Emergency Fund; WHO, World Health Organization
Introduction
Preterm births refer to neonatal births occurring before the completion of 37 weeks of gestation [ 1 ]. It is estimated that Asia and sub-Saharan Africa account for 81.1% of preterm births and over 80% of all newborn mortality among preterm neonates [ 2 ]. One of the challenges faced by preterm newborns is their underdeveloped skin, which lacks an adequate epidermal barrier. The stratum corneum, responsible for regulating the function of the epidermal barrier, typically reaches functional maturity in normal development around 32 to 34 weeks of gestation [ 3 ]. However, in preterm infants, this skin barrier is often compromised, leading to high rates of trans-epidermal water loss and simultaneous losses of fluid and heat [ 4 – 6 ].
In 2014, the World Health Organization (WHO) and UNICEF introduced Every Newborn Action Plan (ENAP) as a global roadmap to reduce avoidable newborn mortality and stillbirth by 2035. Among the interventions included in ENAP is skin massage, which is considered a therapeutic-touch technique that can have positive effects on both the bodies and minds of infants [ 7 ]. However, despite the availability of evidence-based strategies for implementation, many nations have overlooked or inadequately implemented these strategies [ 8 ]. This highlights the need for improved efforts to ensure the effective and widespread adoption of interventions such as skin massage.
A systematic review conducted in 2013 found that emollient therapy has several benefits for preterm neonates, including enhanced weight gain, decreased risk of infection, and reduced newborn mortality. The review indicates that utilizing emollient therapy shows potential as an intervention in settings with limited resources [ 9 ].
The use of various types of emollient oils, such as sunflower oil, mustard oil, and vegetable oil, has gained popularity in recent years for skincare purposes. However, studies examining the efficacy of topical emollient oil application have yielded inconsistent results. Some studies have reported advantages of applying emollient oil on the skin of premature infants, including moisturization, reduction of water loss, and preservation of skin integrity [ 10 – 12 ], On the other hand, other studies have found no significant differences in weight gain when comparing the use of emollient oil to no intervention [ 10 , 13 , 14 ]. It is worth noting that oil massage, including the use of emollient oils, is associated with potential side effects. These can include allergic reactions, rash, necrosis, and uremia [ 15 , 16 ].
Given the limited number of trials assessing the impact of emollient oil on preterm newborns, inconsistencies in the findings, and a lack of information regarding the appropriate dosage, frequency, duration, and selection of effective and safe emollient oils, it becomes crucial to address these inconsistencies, generate practical recommendations, and enhance the survival rates of preterm neonates through evidence-based interventions. Therefore, the objective of this study was to examine the effects of emollient oil applications on weight of preterm infants. The findings of this study can serve as valuable input for initiatives aimed at improving the survival outcomes of preterm newborns.
Methods and materials
Protocol registration.
To ensure the avoidance of duplications, an initial search was conducted, encompassing narrative analysis and systematic reviews, along with registered protocols. The protocol for this systematic review and meta-analysis was registered in the International Prospective Register of Systematic Reviews (PROSPERO) under the reference number CRD42023413770. The methodology for the systematic review and meta-analysis was developed in accordance with the PRISMA (Preferred Reporting Items for Systematic Reviews and Meta-Analyses) reporting checklist, providing a comprehensive and standardized approach for reporting the findings ( S1 Appendix ).
Eligibility criteria or inclusion criteria
The inclusion criteria for this study were
- Study designs : Randomized control trials studies
- Publication status : published articles or grey literatures.
- Language : articles that are written in English or those can be translated to English.
- Study quality : this meta-analysis included those studies with a Good, Fair and poor-quality score based on Cochrane Risk of Bias Tool for Randomized Controlled Trials criteria [ 17 ].
- The outcome of interest : all research articles that investigated the effect of emollient oil on preterm neonates’ weights were included.
Information sources
A comprehensive search was conducted on different databases, including PubMed, Cochrane, Scopus, Clinical trials, ProQuest Central, Epistemonikos, CINAHL, HINARI, Global Index Medicus and gray literature sources (Google scholar, Mednar, World cat, dissertation).
Search strategy
Comprehensive searches were conducted in electronic databases and grey literature sources, encompassing all articles related to human subject research prior to the search date. The searches were conducted in the relevant search fields of electronic databases, employing sensitive search strategies that combined text words using Boolean operators and indexing terms (PICO search) to ensure thorough coverage. The search period extended from July 7, 2023, to July 14, 2023 ( S2 Appendix ).
- ♣ Population–’Preterm newborn’
- ♣ Intervention–’Emollient Oil application’
- ♣ Comparison–‘Standard care or massage without oil’
- ♣ Outcomes–‘weight’.
Additionally, cross-reference search was carried out to include other related studies from the final included studies that the database search might have missed.
Study selection process
After conducting electronic database searches, the research articles were imported into Endnote to identify and manage any duplicate publications. The article selection process for this review was carried out in three stages: initially based on titles alone, followed by abstracts, and finally full-text articles, in a sequential manner. To identify articles relevant to the topic of interest, the primary investigator (FSB) and coauthor (YA) independently screened the titles and abstracts using Rayan online software available at ( https://www.rayansoftware.com ). Any discrepancies that arose during this screening process were resolved through discussions between the investigators. Subsequently, a thorough review of the full-text articles was conducted to assess their eligibility based on predefined inclusion criteria. Specifically, studies investigating the impact of applying emollient oil on weight of preterm neonates were identified for further evaluation.
In cases where papers were not freely accessible, we contacted the corresponding authors via email to request access. Unfortunately, six articles were excluded from the final analysis because the corresponding authors did not respond to the emails ( S3 Appendix ).
Data collection process (extraction.
A data extraction form was developed based on the guide from the Cochrane Collaboration for interventional reviews of randomized controlled trials (RCTs). This form served as a standardized tool to extract relevant data from the included studies. The data extraction was performed independently by Mr. FSB and Mr. AG, and subsequently reviewed by Mr. YA to enhance consistency and reliability. The data extraction form captured important details including the last name of the first author, publication year, place of study, frequency of application, dose and type of emollient oil, duration of intervention, sample size, and mean and standard deviation from both the intervention and control groups. To ensure efficient data management, a Microsoft Excel spreadsheet was utilized to record and organize the extracted data.
Outcomes of the study
Primary outcome : Estimate the effect of emollient oil application in weight in preterm newborns (mean differences in grams). Secondary outcome : Assess adverse events ( AEs) following applying topical emollient oil application in preterm newborns.
Missing data management
We made an effort to address missing data in the articles by contacting the respective authors and requesting the relevant data points. However, in cases where it was not possible to obtain the missing data despite our attempts, we made the decision to exclude those particular papers from the analysis. The reasons for their exclusion were documented and provided as part of the description in the analysis.
Study risk of bias assessment
The quality evaluation of the included studies was conducted using the Cochrane Risk of Bias Tool for Randomized Controlled Trials (RoB 2.0). This tool consists of five domains that assess different sources of bias: bias arising from the randomization process, bias due to deviations from intended interventions, bias due to missing outcome data, bias in measurement of the outcome, and bias in selection of the reported result [ 18 ]. Each domain is rated as having a low risk of bias, high risk of bias, or some concerns.
The principal investigator, in collaboration with the coauthor (YA), independently rated each publication using the Cochrane RoB 2.0 tool. Once the quality evaluation was completed, the results were converted into the categories defined by the Agency for Healthcare Research and Quality (AHRQ) for easier interpretation. These AHRQ categories include Good, Fair, and Poor quality, which provide a summary assessment of the overall quality of the studies. ( S4 Appendix ).
Data synthesis and analysis plan
Once the eligible articles with assured quality were identified, their findings were gathered, analyzed, and summarized. The STATA Version 17 software (STATA Corporation, College Station, Texas) was utilized to estimate the pooled effect estimates of emollient oil application on weight of premature babies.
Initially, a narrative overview of the selected articles was provided, highlighting key findings and trends. Subsequently, a summary table was created, which included details such as the author, year of publication, region, primary outcome, sample size, adverse events, quality score, and other significant results. This table served as a comprehensive reference for easy comparison and retrieval of information.
To facilitate comparisons of weight among preterm neonates, a mean difference (MD) along with a corresponding 95% confidence interval (CI) was employed as the effect measure. Forest plots, summary tables, and accompanying text were utilized to present a comprehensive summary of the results derived from the meta-analyses. These graphical and tabular representations provided a clear visualization of the overall findings and effect sizes across the analyzed studies.
Heterogeneity exploration
To assess heterogeneity across the included studies, employed both graphical and statistical methods. Graphical methods included the use of forest plots and Galbraith plots, which provide visual representations of the variability in effect sizes across studies. Statistical tests were also utilized to assess heterogeneity. Cochran’s Q test was employed to determine if the amount of heterogeneity between studies was greater than what would be expected by chance alone. A significance level of P < 0.05 was considered indicative of statistically significant heterogeneity. Additionally, the I 2 statistic was used to estimate the percentage of variability in results across studies that is due to real differences rather than chance. Threshold values of 25%, 50%, and 75% were considered as representing low, medium, and high levels of heterogeneity, respectively [ 19 ]. To further investigate and manage the causes of heterogeneity, random-effects model, subgroup analysis based on factors such as types of emollient oil, duration, dose, frequency of intervention, and study quality were employed. Sensitivity analysis and meta-regression analysis were also conducted to explore potential sources of heterogeneity across studies.
Small study effect diagnosis
Both graphical and objective tests were employed to evaluate the presence of publication bias. Funnel plots, a graphical method, were used to visually assess the symmetry of the distribution of study results. Asymmetry in the plot may indicate the presence of publication bias, specifically the small study effect. In addition to the graphical assessment, Egger’s and Begg’s tests to quantitatively examine publication bias. A statistically significant result (p-value < 0.05) in these tests suggests the presence of a small study effect, which can be indicative of publication bias [ 20 ]. Non-parametric trim and fill analysis employed to address the potential small study effect and publication bias. This method aims to estimate the potential number of missing studies due to publication bias and adjust the effect size accordingly. The analysis provides an adjusted effect size by imputing hypothetical missing studies to create symmetry in the funnel plot [ 21 ].
Study selection
Out of the initial 2,734 articles retrieved, a total of 1,681 articles were excluded as they were identified as duplicates. Additionally, 995 articles were deemed unrelated to the goal of our study based on the screening of titles and abstracts. Consequently, the full texts of the remaining 58 publications were thoroughly reviewed to determine their eligibility. After this assessment, a total of 18 articles met the inclusion criteria and were included in the final analysis. These 18 articles were utilized to evaluate the impact of emollient oil application on weight of preterm newborns ( Fig 1 ).
- PPT PowerPoint slide
- PNG larger image
- TIFF original image
https://doi.org/10.1371/journal.pone.0302969.g001
Characteristics of included studies
This review included 18 randomized controlled trials (RCTs) conducted between 2000 and 2023, encompassing various continents. A total of 1454 preterm neonates participated in these trials, with 723 in the intervention group and 731 in the control group. The studies were conducted in nine different countries across five continents, including Asia [ 11 , 15 , 22 – 32 ], Australia [ 33 ], North and South America [ 34 , 35 ], and Europe [ 36 , 37 ]. The primary focus of these studies was to examine the effects of emollient oil therapy on weight in preterm infants.
Among the 18 included studies, 17 were conducted in institutional settings, while one study was community-based and carried out in a rural environment [ 15 ]. The total study population across the included studies ranged from 25 participants [ 36 ] to 258 participants [ 27 ]. Regarding the types of emollient oils used in the intervention groups, half of the studies employed coconut oil [ 15 , 26 , 27 , 38 ] or sunflower oil [ 22 , 29 , 31 , 32 ] as topical emollient therapies. The remaining studies utilized olive oil [ 25 , 28 , 30 ], Medium-chain triglyceride (MCT) oil [ 11 , 23 , 24 ], Soya oil [ 35 ], iSio4 [ 36 , 37 ], and Vimala [ 34 ] in their intervention groups. The duration of therapy for preterm neonates shows variation, spanning from 5 to 60 days, with an average duration of 18.5 days. However, we encountered difficulties in calculating the exact mean gestational age and baseline weight due to inconsistencies in reporting across the studies included in our analysis.
In the review, it is noted that six out of the eighteen included studies were published within the last five years. The remaining twelve studies were conducted between 2000 and December 2017, providing a range of data from different time periods. To summarize and draw conclusions regarding the effect of topical emollient oil application on weight of preterm newborns, a narrative synthesis was performed. This synthesis involved extracting key findings from the full-text articles and summarizing them ( Table 1 ).
https://doi.org/10.1371/journal.pone.0302969.t001
In terms of adverse events related to topical emollient oil application, a total of nine studies investigated this aspect. Out of these, only two studies reported specific adverse events in the intervention groups, namely rash and accidental slippage [ 15 , 26 ].
Effect of emollient oil on preterm weight
In the meta-analysis, the pooled mean difference was calculated based on data from 14 studies, involving a total of 1067 preterm neonates. Among these, 531 neonates were in the treatment group receiving topical emollient oil, while 536 neonates were in the control group.
The mean difference in weight gain varied across the included studies, ranging from 8.34 grams [ 25 ] to 313.2 gram [ 30 ]. To obtain a summary estimate of the effect of topical emollient oil application on weight of preterm babies, the pooled mean difference was calculated using fixed and random effects models.
However, because to the notable heterogeneity among the included studies (I 2 > 93.24%, p 0:000), the final values were reported using a random effect model. The pooled mean difference was found to be 52.15 grams (95% CI: 45.96, 58.35) by using the DerSimonian and Laird random-effects model ( Fig 2 ). The blue square in the figure represents the mean difference for each study according to the random-effects model, while the length of the segment it lies on represents the confidence interval for each study. The diamond symbol represents the overall combined mean difference for all the studies, which indicates the pooled estimate of the effect size.
https://doi.org/10.1371/journal.pone.0302969.g002
Subgroup analysis effect of emollient oil on preterm weight.
Subgroup analyses were conducted in response to the observed higher levels of heterogeneity among the included studies, which were evident both subjectively (forest plot and Galbraith plot) and quantitatively (I 2 and Q test). The type of emollient oil used, the duration of therapy, dose of therapy, frequency of therapy, studies quality score was taken into consideration when doing these analyses.
Subgroup analyses revealed improvements in heterogeneity when considering types of emollient oil used, the dose of therapy, and the duration of therapy. In terms of the types of emollient oil, preterm newborns who received massages with coconut oil showed a mean difference in weight gain of 51.06 grams (95% CI: 44.86, 57.26). On the other hand, infants who received massages with sunflower oil had a higher mean difference of 133.52 grams (95% CI: 112.24, 154.8) compared to their counterparts. Regarding the dose of therapy, infants who received emollient oil massages three times a day exhibited a significant higher mean weight increment of 130.76 grams (95% CI: 102.88, 158.64) compared to other dosing frequencies ( Table 2 ) .
https://doi.org/10.1371/journal.pone.0302969.t002
Meta regression.
Meta-regression analysis was conducted to determine whether specific covariates could account for some of the heterogeneity observed. The results of the meta-regression analysis indicated that the type of emollient oil used, the duration of therapy, and the frequency of therapy collectively explained a significant proportion of the observed heterogeneity.
Specifically, the emollient oil type accounted for 36.6% of the heterogeneity, the duration of therapy explained 34.39% of the heterogeneity, and the frequency of therapy explained 59.85% of the heterogeneity in the bi-variable regression analysis. On the other hand, variables such as study setting and study quality, as well as multiple regression of covariates, did not significantly influence the heterogeneity observed among the included studies ( Table 3 ).
https://doi.org/10.1371/journal.pone.0302969.t003
Sensitivity analysis of mean weight difference of premature babies.
To evaluate the influence of individual studies on the overall meta-analysis estimate, sensitivity analyses or influential analyses were conducted. These analyses aimed to assess the impact of removing outlier studies from the analysis.
Based on the sensitivity analysis, it was identified that two studies were considered outliers or influential papers. This determination was made by examining the pooled estimate after excluding the individual study’s point estimate, which resulted in the estimate falling outside the confidence interval of the "combined" analysis (95% CI: 45.96, 58.35) ( Fig 3 ).
https://doi.org/10.1371/journal.pone.0302969.g003
The sensitivity analysis identified two specific studies, Saeidi et al. (2009) and Saeidi, Reza (2015), as having a notable impact on the overall estimation in the meta-analysis. The original pooled difference in mean weight gain was estimated to be 52.15 grams (95% CI: 45.96, 58.35). However, after excluding the outlier studies, the pooled difference in mean weight changed to 78.57grams (95% CI: 52.46, 104.68) ( Table 4 ).
https://doi.org/10.1371/journal.pone.0302969.t004
Publication bias assessmen.
To assess the potential presence of publication bias, two methods were employed: funnel plots and Egger’s statistical test. The funnel plot, a graphical test, revealed a slight dispersion of studies, particularly on the right side, indicating the presence of potential publication bias. This suggests that there may be a tendency for small studies with positive outcomes to be published ( Fig 4 ). Additionally, Egger’s test was conducted to statistically evaluate the presence of small study effects (p-value = 0.000).
https://doi.org/10.1371/journal.pone.0302969.g004
To address the potential impact of publication bias and adjust for its influence, a nonparametric Trim-and-fill analysis was performed. The analysis revealed a significant change in the pooled mean difference, shifting from 52.15 grams to 43.3 grams (95% CI: 36.39, 50.22), after imputing six studies using the random effects model ( Fig 5 ).
https://doi.org/10.1371/journal.pone.0302969.g005
In this systematic review and meta-analysis, the primary objective was to assess the effect of emollient oil application on weight gain in preterm neonates. The analysis considered studies conducted in various countries at the global level in order to capture a wide range of evidence. After extensive searching and quality assessment process, a total of eighteen studies were identified for the estimation of weight gain in preterm neonates. However, after further screening and applying inclusion criteria, fourteen studies were included in the final analysis of this meta-analysis. These fourteen studies met the specified criteria and provided relevant data for the analysis of the effect of emollient oil application on weight gain in preterm neonates.
The study’s findings indicate that the pooled difference in mean weight among preterm neonates who received emollient therapy was estimated to be 52.15 grams (95% CI: 45.96, 58.35). This result aligns with evidence generated from developing countries, suggesting a consistent effect of emollient therapy on promoting postnatal growth in preterm infants [ 9 ]. Furthermore, recent emerging evidence also supports the notion that emollient therapy contributes to enhanced postnatal growth [ 9 , 39 ].
Considering the significance of optimal growth and its potential impact on the short- and long-term health outcomes of preterm neonates, a weight gain of 52 grams, although seemingly modest, can be considered clinically relevant. This weight gain has the potential to contribute to improved organ maturation, enhanced thermoregulation, and a decreased risk of complications such as hypoglycemia and respiratory distress syndrome [ 40 , 41 ]. Additionally, it can help reduce the likelihood of long-term growth faltering and associated health complications [ 42 ].
However, it is important to consider the results of the nonparametric trim and fill analysis, which revealed a decline in the pooled difference in mean weight to 43.3 grams (95% CI: 36.39, 50.22). This adjustment may be attributed to publication bias, suggesting that studies with small sample sizes and negative results may be underrepresented in the published literature.
The study further suggests that preterm infants who received massages with sunflower oil and coconut oil exhibited better weight gain compared to control groups. This finding could be explained by the presence of fatty acids, such as linoleic acid, and vitamin D in these oils. These components may enhance the binding to peroxisome proliferator–activated receptor α receptors on keratinocytes, facilitating skin development, maturation, and potentially preventing complications such as hypothermia and apnea [ 12 , 37 , 43 ].
Another significant finding of the study reveals that preterm neonates who received massages three times per day exhibited a notable increase in weight compared to their counterparts. This effect could be attributed to the impact of massage or tactile stimulation on the physiological stability and development of preterm newborns [ 44 ]. The mechanical stimulation provided by oil massage may stimulate the production of proteins and the growth hormone IGF-1, ultimately leading to weight gain [ 45 ]. Moreover, regular oil massage has the potential to enhance blood flow, regulate body temperature, and reduce the energy required to maintain body temperature, thereby supporting healthier weight gain [ 9 ]. Additionally, oil massage improves the skin’s barrier function, reducing trans-epidermal water loss and facilitating weight gain in premature newborns [ 46 ].
Furthermore, the study suggests that the observed weight gain in preterm neonates through oil massage may contribute to a reduced hospital stay. Consistent weight gain is often one of the discharge criteria from the Neonatal Intensive Care Unit (NICU). Therefore, the positive effect of oil massage on weight gain may expedite the hospital discharge process for premature babies.
In conclusion, the moderate-to-high level of certainty regarding the effectiveness of topical emollient oil application in promoting weight gain among preterm neonates provides strong support for its use as a beneficial intervention. This finding indicates a positive impact on weight gain outcomes in this population, highlighting the reliability and confidence in this conclusion.
Limitations of the study
The study encountered notable limitations that affected its conclusions. A primary concern was the scarcity of available primary studies, which restricted the researchers’ ability to estimate the mean difference across different continents and skin colors. The absence of data from Africa and the heterogeneous nature of the available data were also limited the generalizability of the findings. Another important limitation was the insufficient discussion on publication bias and other influential factors, including nutrition and age at enrollment, which could impact weight gain. It is important to consider that weight gain in preterm neonates is a multifactorial event, not solely attributable to body massage with emollient oil. Additionally, the study did not adequately explore the challenges associated with weight gain during the first week of life in preterm neonates, which is a critical period. These aspects should be further explored and discussed in future studies to provide a more comprehensive understanding of the factors influencing weight gain in this population.
Considering the significant global public health concern surrounding premature birth and its associated mortality, it is crucial to implement low-tech and cost-effective interventions to reduce preterm newborn deaths. This systematic review and meta-analysis indicate that the application of sunflower oil and coconut oil during massage resulted in better weight gain for preterm neonates compared to those who did not receive oil massage or only received routine care.
Furthermore, preterm babies who received massage three times a day demonstrated improved weight gain compared to control groups. Additionally, the limited reporting of adverse events highlights the need for further investigation into potential risks associated with emollient oil application.
The significant pooled difference in mean weight gain observed indicates that preterm infants who received emollient oil experienced improved nutrition, reduced infection risk, and potentially better neurodevelopmental outcomes. Therefore, it is essential for local policymakers and health planners to prioritize the implementation of emollient oil use in routine care for preterm infants. Integrating this evidence into national guidelines for NICU settings would greatly contribute to facilitating weight gain and overall well-being.
Supporting information
S1 appendix. prisma checklist..
https://doi.org/10.1371/journal.pone.0302969.s001
S2 Appendix. Search strategies queries results.
https://doi.org/10.1371/journal.pone.0302969.s002
S3 Appendix. List of excluded studies from final analysis.
https://doi.org/10.1371/journal.pone.0302969.s003
S4 Appendix. Quality assessment based on cochrane risk of bias tool for RCTs ‘ROB 2’.
https://doi.org/10.1371/journal.pone.0302969.s004
Acknowledgments
We are thankful to the authors of the original studies included in this systematic review and meta-analysis, and to those who contributed a lot in this work.
- View Article
- PubMed/NCBI
- Google Scholar
- 3. R. KLIEGMAN, J. ST. GEME, B. stanton N schor (eds). Nelson textbook of PEDIATRICS. 21th Edition. 2020. https://doi.org/10.1007/978-3-319-52792-5_1
- 7. World Health Organization, WHO U. Every newborn An Action Plan To End Preventable Deaths. 2014.
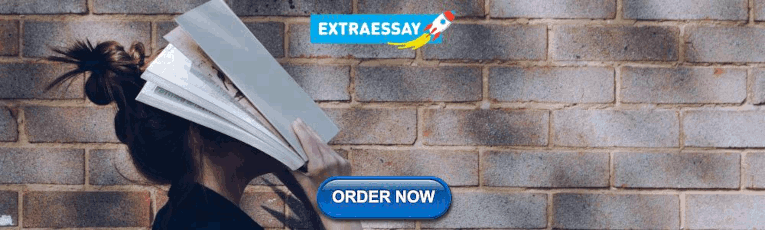
IMAGES
VIDEO
COMMENTS
Here you can access information about the PRISMA reporting guidelines, which are designed to help authors transparently report why their systematic review was done, what methods they used, and what they found. The main PRISMA reporting guideline (the PRISMA 2020 statement) primarily provides guidance for the reporting of systematic reviews ...
It has been more than a decade since the original publication of the Preferred Reporting Items for Systematic Reviews and Meta-Analyses (PRISMA) Statement [], and it has become one of the most cited reporting guidelines in biomedical literature [2, 3].Since its publication, multiple extensions of the PRISMA Statement have been published concomitant with the advancement of knowledge synthesis ...
The Preferred Reporting Items for Systematic reviews and Meta-Analyses (PRISMA) statement, published in 2009, was designed to help systematic reviewers transparently report why the review was done, what the authors did, and what they found. Over the past decade, advances in systematic review methodology and terminology have necessitated an update to the guideline. The PRISMA 2020 statement ...
The PRISMA 2020 items are relevant for mixed-methods systematic reviews (which include quantitative and qualitative studies), but reporting guidelines addressing the presentation and synthesis of qualitative data should also be consulted. 14 15 PRISMA 2020 can be used for original systematic reviews, updated systematic reviews, or continually ...
Over the past decade, advances in systematic review methodology and terminology have necessitated an update to the guideline. The PRISMA 2020 statement replaces the 2009 statement and includes new reporting guidance that reflects advances in methods to identify, select, appraise, and synthesise studies. The structure and presentation of the ...
Over the past decade, advances in systematic review methodology and terminology have necessitated an update to the guideline. The PRISMA 2020 statement replaces the 2009 statement and includes new reporting guidance that reflects advances in methods to identify, select, appraise, and synthesise studies. The structure and presentation of the ...
Using statistical methods for the interpretation of the results implies a systematic review containing meta-analysis ( 6 ). The PRISMA guidelines consist of a four-phase flow diagram and a 27-item checklist. The flow diagram describes the identification, screening, eligibility and inclusion criteria of the reports that fall under the scope of a ...
Matthew Page and co-authors describe PRISMA 2020, an updated reporting guideline for systematic reviews and meta-analyses. PLoS Med . 2021 Mar 29;18(3):e1003583. doi: 10.1371/journal.pmed.1003583.
Systematic reviews and meta-analyses are essential to summarise evidence relating to efficacy and safety of healthcare interventions accurately and reliably. The clarity and transparency of these reports, however, are not optimal. Poor reporting of systematic reviews diminishes their value to clinicians, policy makers, and other users. Since the development of the QUOROM (quality of reporting ...
views and Meta-Analyses (PRISMA) group, which. mainly consists of Cochrane authors, has developed. the PRISMA guidelines in 2009 (6). A systematic. review will extensively scan all reports ...
Many innovations in the conduct of systematic reviews have occurred since publication of the PRISMA 2009 statement. For example, technological advances have enabled the use of natural language processing and machine learning to identify relevant evidence [22,23,24], methods have been proposed to synthesise and present findings when meta-analysis is not possible or appropriate [25,26,27], and ...
Literature searches underlie the foundations of systematic reviews and related review types. Yet, the literature searching component of systematic reviews and related review types is often poorly reported. Guidance for literature search reporting has been diverse, and, in many cases, does not offer enough detail to authors who need more specific information about reporting search methods and ...
The PRISMA 2020 items are relevant for mixed-methods systematic reviews (which include quantitative and qualitative studies), but reporting guidelines addressing the presentation and synthesis of qualitative data should also be consulted. 39 40 PRISMA 2020 can be used for original systematic reviews, updated systematic reviews, or continually ...
The Preferred Reporting Items for Systematic Reviews and Meta-Analysis (PRISMA) is a 27-item checklist used to improve transparency in systematic reviews. These items cover all aspects of the manuscript, including title, abstract, introduction, methods, results, discussion, and funding. The PRISMA checklist can be downloaded in PDF or Word files.
PRISMA is an evidence-based minimum set of items for reporting in systematic reviews and meta-analyses. PRISMA focuses on the reporting of reviews evaluating randomized trials, but can also be used as a basis for reporting systematic reviews of other types of research, particularly evaluations of interventions.
Systematic literature reviews (SRs) are a way of synthesising scientific evidence to answer a particular ... SRs treat the literature review process like a scientific process, and apply concepts of empirical ... PRISMA chart for reporting systematic reviews (Moher et al., 2009) Following this approach, the review process is more transparent and ...
To document your grey literature search, download the flow diagram template version 1 PRISMA 2020 flow diagram for new systematic reviews which included searches of databases, registers and other sources or the version 2 PRISMA 2020 flow diagram for updated systematic reviews which included searches of databases, registers and other sources.
2 Methods of literature search 2.1 Search strategy. Using the Preferred Reporting Items for Systematic reviews and Meta-Analyses (PRISMA) framework as a guide, a systematic review technique was used to evaluate studies describing artificial intelligence and keratoconus. The search strategy was created based on the population, interventions ...
Methods The review will follow the JBI guidelines for mixed methods systematic reviews and will be developed and reported in accordance with PRISMA P guidelines. Databases that will be searched are Excerpta Medica Database (Embase), Scopus, Cumulative Index to Nursing and Allied Health Literature (CINAHL) Complete, and OVID MEDLINE.
Methods: A systematic literature search following Preferred Reporting Items for Systematic Reviews and Meta-Analyses (PRISMA) guidelines was conducted on 22nd of February 2022 using the Ovid MEDLINE and Web of Science databases. Three categories of search terms were used: (1) self-harm, self-injury, self-mutilation, suicide, self-poisoning; (2 ...
Evidence from past research studies indicated that the maturity model had been used to measure safety culture in organisations. Safety Culture Maturity Model (SCMM) was the step taken by numerous organisations to attain better safety practices. This study aimed to review their approach to measure safety culture by the generative systematic literature review (SLR) approach. Guided by PRISMA ...
However, none of the researchers comprehensively conducted a Systematic Literature Review on Entrepreneurial Intention. Therefore, this study aims to review the main finding, database, citation, study setting, methodology, unit of analysis, field of study analysis, and underpinning theory of previous studies on Entrepreneurial Intention.
mixed-methods systematic reviews (which include quantitative and qualitative studies), but reporting guidelines addressing the presentation and synthesis of qualitative data should also be consulted.39 40 PRISMA 2020 can be used for original systematic reviews, updated systematic reviews, or continually updated ("living") systematic reviews.
The literature review was conducted between January 2000 and March 2024, utilizing a rigorous and systematic methodology in accordance with the Preferred Reporting Items for Systematic Reviews and Meta-analyses (PRISMA) guidelines , which is illustrated in the study review progression flowchart .
Background Synthesizing current evidence on interventions to improve survival outcomes in preterm infants is crucial for informing programs and policies. The objective of this study is to investigate the impact of topical emollient oil application on the weight of preterm infants. Methods A systematic review and meta-analysis of randomized controlled trials (RCTs) was conducted. To identify ...