Researchers work to solve 5G network problems when it matters: right now
- Kelly Izlar
14 Dec 2022
- Share on Facebook
- Share on Twitter
- Copy address link to clipboard
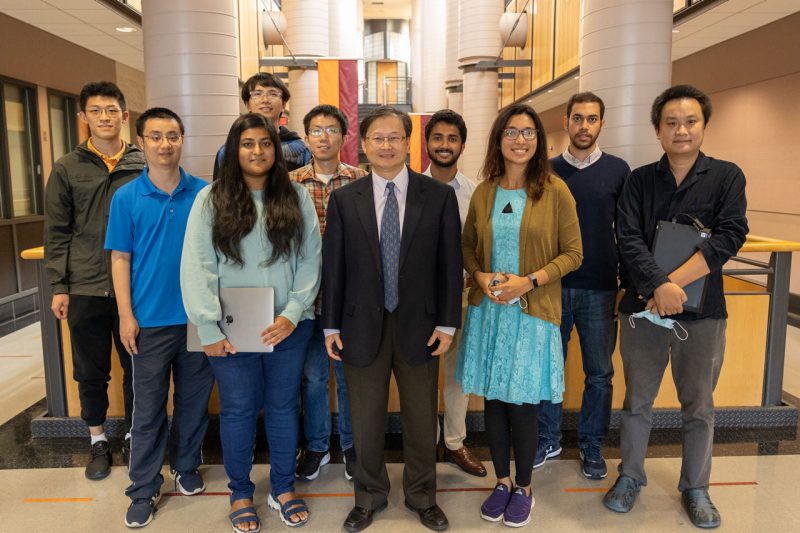
5G can enable smart cities, virtual realities, and self-driving cars — but will these applications be convenient and safe to use if network connection is delayed? Commonwealth Cyber Initiative researchers from Virginia Tech developed a methodology that provides optimal solutions to network problems on the fly and in real time.
The fifth generation of mobile network (5G) is bringing more applications, devices, and users into network operations. But spiking demand can stress local networks, creating bottlenecks that are narrowed by safety-critical or other high-priority tasks that need to happen as soon as possible.
Computer engineers such as Virginia Tech’s Tom Hou have dedicated their careers to fine-tuning network parameters and components to get ever closer to peak performance — an endeavor further complicated by real-time demands.
“The holy grail of my research has always been timing,” said Hou, the Bradley Distinguished Professor of Electrical and Computer Engineering in the College of Engineering.
Timing constraints have forced computer engineers to modify their algorithms to stay within suboptimal network thresholds, which limits functionality and throttles performance.
This changed in 2018, when Hou’s research group hit upon a methodology that pulled real-time into range.
“This was a major breakthrough,” said Hou. “With support from the Commonwealth Cyber Initiative in Southwest Virginia , we elevated network optimization to a whole different level: solving problems in the field in real time.”
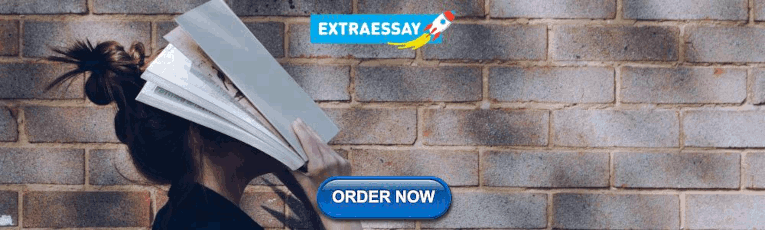
Ultra-high precision for ultra-low latency
In the context of 5G, timing is tied up with the concept of latency. Latency refers to time duration, or how long it takes to complete a certain task or step in a process. Minimizing latency is an attempt to reduce delay. When it comes to 5G, a delay of even a few milliseconds can make a difference to an industrial automation system or a power grid , for instance.
As a delay stretches, not only will user experience degrade, but risk to device, information, or safety increases.
“Think about industrial automation or autonomous driving , which require information to be transported over different systems very quickly to ensure tight synchronization,” said Hou. “Reaction time on the road or in a warehouse is critical to preventing accidents, making latency of utmost importance.”
To deliver that kind of end-to-end latency on the order of millisecond, scheduling from the 5G base station has to be on the same order or even lower.
How it started
In 2018, Hou and his team were designing a system to meet the stringent timing requirements of new radio access technology for the 5G mobile network. To support applications with ultra-low latency, the minimum timing resolution for optimal 5G New Radio performance was capped at 125 microseconds — almost 10 times faster than what was possible with 4G LTE.
Up until then, no one had been able to deliver optimal scheduling in that interval.
The Virginia Tech team proposed a scheduling algorithm that incorporated a graphics processing unit (GPU) — a specialized circuit that uses parallel computing to accelerate workloads in high performance computing.
Parallel computing isn’t a new technology. A supercomputer processes computations in parallel with thousands of central processing units, but it’s expensive, cumbersome, and can’t be accomplished locally — by the time a base station outsources a task to the cloud and receives the results, it’s far too late to meet real time scheduling needs.
Originally designed for graphics rendering, a GPU isn’t in the same league as a supercomputer in terms of processing capability. It wasn’t designed for scientific computation or solving complex optimization problems, but when coupled with Hou’s new scheme, it doesn’t have to be.
Hou and his team developed a multistep methodology that breaks down a big problem into a smaller set of sub problems and then zeros in on the sub problems that are likely to yield the most promising results. For this manageable set of small problems, custom solutions can be developed by a GPU processing in parallel.
“With this technique, even a low-end GPU can find near-optimal solutions within the sub-millisecond time window,” said Hou.
Hou’s team’s innovation rocked the field of wireless network optimization.
“Probably the most important feature of 5G is the ability to communicate with low latency, and Professor Hou’s work makes this feasible,” said Jeff Reed, the Commonwealth Cyber Initiative's chief technology officer and the Willis G. Worcester Professor of electrical and computer engineering at Virginia Tech.
GPU manufacturer Nvidia showcased Hou's work, which was carried out in collaboration with fellow Commonwealth Cyber Initiative researcher Wenjing Lou in computer science. The invention was awarded a U.S. patent as it was applied to 5G schedulers. But this was just the beginning.
“We thought — wait a minute, there’s more than just scheduling for 5G problem,” said Hou. “We identified the key steps, theorized the technique, and implemented it to solve other wireless networking and communications problems with similar mathematical structure.”
Scaling up to secure autonomous vehicles
Armed with a process that brings real-time solutions into reach, Hou’s research group was ready to apply it to complex problems in different domains. With continued support from the Commonwealth Cyber Initiative in Southwest Virginia, the team is tackling radar interference in autonomous vehicles.
In addition to camera and lidar, autonomous vehicles monitor road conditions with radar because it’s not finicky about weather or lighting conditions. Day, night, rain, or snow — radar is robust.
It is, however, susceptible to interference. A radar bounces a signal off nearby objects and then measures the reflected signal to determine what’s on the road and around the vehicle. When there are too many radars on the road, signals bounce around willy-nilly, compromising a radar’s normal capability.
“Such radar-to-radar interference offers a relatively easy way to unleash a cyberattack on an autonomous vehicle and compromise safety,” said Hou.
Hou is applying the methodology to sort through which signals should matter to an autonomous vehicle, mitigating high levels of interference in real time, in-vehicle, and with an affordable GPU.
The application of the scheduling algorithm as applied to radar mitigation will be published through IEEE Radar Conference proceedings in early spring, and a patent has been filed with Virginia Tech Intellectual Properties. The Commonwealth Cyber Initiative provides funds for the translation of research into practice through programs such as “ Innovation: Ideation to Commercialization ” and patent support costs.
Edge computing and elsewhere
Hou and his team also are applying their technique to task-offloading for edge computing, which involves determining which tasks should be processed on a local device and which should be offloaded to the 5G base station.
“Our new real-time optimization methodology has many, many applications. It’s opened up a new life, certainly for me, but also for other researchers doing network optimizations,” said Hou. “We keep seeing new places where this methodology can be applied, and in many cases, finding groundbreaking solutions along the way.”
Lindsey Haugh
- Autonomous Vehicles
- College of Engineering
- Commonwealth Cyber Initiative
- Commonwealth Cyber Initiative, Southwest Virginia Node
- Computer Science
- Cybersecurity
- Electrical and Computer Engineering
- LINK+LICENSE+LAUNCH
- Research Areas
- Research Frontiers
- Security Frontier
Related Content
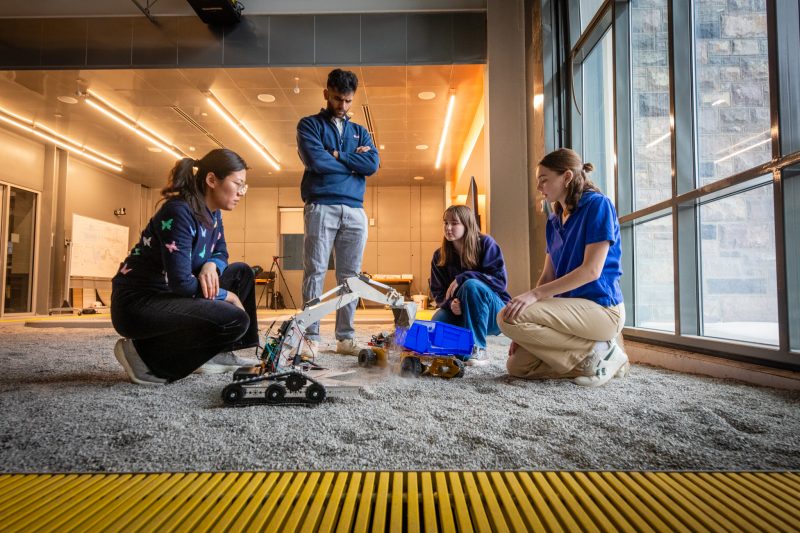
Trending People
trending technology, trending entities.

- Systems & Design
- Low Power - High Performance
- Manufacturing, Packaging & Materials
- Test, Measurement & Analytics
- Auto, Security & Pervasive Computing
- Startup Corner
- Industry Research
- Special Reports
- Business & Startups
- Knowledge Center
- Architectures
- Automotive/ Aerospace
- Communication/Data Movement
- Design & Verification
- Lithography
- Manufacturing
- Optoelectronics / Photonics
- Power & Performance
- Test, Measurement & Analytics
- Transistors
- Z-End Applications
- Newsletters
- Low Power-High Performance
- Technical Papers
Solving 5G’s Thorniest Issues
Incomplete roadmaps and continued uncertainty about millimeter-wave technology make this technology’s future hazy, but solutions are in the works.

5G rollouts are beginning to hit the market, accompanied by a long list of unsolved technical and business issues surrounding this next-generation wireless technology. But progress is being made on some of the key challenges facing this technology, even though not all of those solutions will be in place at launch.
The real challenges are with millimeter-wave implementations of 5G , which operate at significantly higher RF frequencies than existing wireless communication. The first phase of rollouts will be sub-6GHz implementations, which behave much like 4G. Those signals still travel long distances with little disruption.
Millimeter wave is where things get much more interesting and difficult. The technology is capable of transmitting large quantities of data, such as streaming high-resolution video, extremely quickly. But there are some fundamental issues that need to be resolved before that can happen.
Among the top challenges:
- With mmWave technology, arrays of antennas will be required because of the limited reach of mmWave signals. Those antennas likely will be embedded into a package with no exposed leads to test. So far, there is no proven approach for high-volume production testing.
- The effectiveness of those antennas will depend on signal coverage, and so far it isn’t clear how many base stations and small cells will be required to supply adequate coverage in different environments. Numbers may vary greatly, depending on where the cells are located, how many people they need to serve, and how much interference there is between base stations, small cells and people. With mmWave technology, trees and weather can disrupt signals.
- There is no single mmWave spectrum or standard waveform. There are many of them, and they are not the same from one region to the next. That makes it difficult to build devices that will leverage 5G in multiple regions, and there is confusion about what happens with digital and analog technology.
Despite all of that, millimeter-wave technology itself is well understood. It has been used for military applications such as radar for decades. But applying it to billions of consumer devices, many of which are in motion at varying speeds, and maintaining connections is a nightmarish problem.
“It is a realistic technology, but it’s definitely a challenge,” said Neill Mullinger, product manager in the vertical market solutions group at Mentor, a Siemens Business . “The reason that it is worth taking the challenge up is that we have nowhere else to go. We’ve used up all the spectrum, and demand is still skyrocketing. If you look at any city in the world, you test out the performance of your cellular phone at 3 a.m. and it’s pretty good. You try it out at 6 p.m. and it’s lousy because of the sheer volume of users. It’s like being on a congested highway. You can drive freely at night, but you can’t at 6 p.m. It’s the exact same problems, so we have to go there. We have to go into the millimeter wave. But it comes with these really problematic attenuation problems—200 meters and the signal has faded away.”
And this is where things begin to get really complicated. Some of the solutions involve developments in other technology areas, including the edge , which is still a vague concept, and the addition of artificial intelligence, which is in a state of almost constant flux. All of the pieces need to work together seamlessly.
“There is quite a change in the architecture required for 5G,” said Ian Dennison, senior group director for Cadence’s customized IC and PCB Group. “We’ve got all these small cells distributed on lamp poles and in buildings. But we now have to centralize the baseband. And this is where edge compute comes in. You can share baseband processing across a large number of small cells and have a greater efficiency as a result, but the timing needs to be just right for us to be bringing in AI processing to the edge computer, as well, so that AI can assist with the baseband processing. So if I’ve got small cells in different places with different contexts, then AI can learn about how the small cell can best bounce the beams off of local buildings. It sends multiple beams by multiple pathways to the same user to improve the connectivity. If you’re in a strong signal area, it can provide multiple concurrent pathways to the same user via multiple beams. There are a variety of different ways that beamforming is going to have to be intelligent, and AI is arriving at just the right time. That will help a lot with some of the difficulty with 5G.”
Solving many of these issues involves a massive global effort, and it involves a lot of moving pieces— including many that are well beyond the scope of the people working on various pieces of the solution.
“When you have all those mini cells doing this, the 5G solution is not just wireless,” said Peter Zhang, R&D manager in Synopsys ‘ Solutions Group. “The wireless portion of the 5G’s impact on the communication network is only one aspect of that. There is also the gigantic backbone land line networking systems that we’re constructing and working on. We all have to be working together congruently, each with our own 5G wireless portion, so you can access a data network everywhere with a high-performance type of experience.”
A key component of that will be the edge, but so far the edge remains more of a concept than reality.
“There is still a lot of uncertainty about the edge, but some characteristics are starting to come into focus,” said Mike Fitton, senior director of strategic planning at Achronix . “There is a wide variety of devices, there is an increase in the number of devices that are coming, and there is a variety of devices that produce very little data to sensors that produce significant amounts of data, with different characteristics, as well. Classically, we saw all of that data moving from edge devices to the cloud and back, but because of requirements from a latency point of view, we’re starting to push this closer to the edge or edge compute.”
Test and reliability Millimeter wave will play a key role in that paradigm, but one of the big problems today with mmWave is the reliability of data communication. Beamforming, which directs signals from multiple cells around objects, is a proven approach to maintaining a connection. But how well an end device receives and processes that signal depends to a large extent on the antenna array, and testing of an array is a slow process using over-the-air testing.
“We’re starting to see millimeter wave base stations and early testing of the topology, and you’ll see more of those in a year or two,” said Adrian Kwan, senior business development manager at Advantest . “That will be followed by cell phones in 2021. It’s the same base station technology as today, but you need five to six times more pico cells. The wireless has to be in place first before you see customers developing devices to support millimeter wave.”
Kwan said that packaging and testing both have evolved to be able to support testing of the antennas over the air, but so far nothing has been done in high volume. But that’s not the only issue.
“What we’re seeing with multiple carriers is that the waveforms are different,” said Kwan. “There are new standards being defined to deal with this. That all has to be in place before 5G is ready.”

Others report similar challenges.
“To support these bands we have to significantly change the design and testing we do in these environments,” said Ben Thomas, director of technical marketing at Qorvo, in speech at the recent NIWeek. “Combine that with more carrier aggregation combinations, dual uplink, and more complex waveforms and modulation schemes, and quite frankly you’re looking at an exponential impact on the RF section.”
There are other issues on the test side, as well. “The challenge with 5G is that some of this stuff has to get pushed up to the system level,” said Doug Elder, vice president and general manager of the semiconductor business at OptimalPlus . “So you may need to do some of this stuff with system-level test, as opposed to functional test or earlier in the process. If you collect the data that comes out of that test process, you can look at that data and draw some conclusions that you can drive back into your process.”
In addition, just testing a 5G device in the fab or packaging house isn’t sufficient. Because this technology is so new, quality of service is expected to be spotty at first. The early generations of cell phones dropped calls regularly with far lower data rates.
“The current frequencies clearly aren’t going away, so we have to continue to test those in this way that we have done in the past,” said Mentor’s Mullinger. “But then you augment that with new solutions to deal with the expanded frequencies of 5G. The current family of testers allows you to do a lot of that virtually, which means we can set up a test environment for the lab or the field and run the exact same environment.”
That will be critical for improving coverage and quality of service.
“Part of this an expectation-management problem, and we should expect that our 5G systems start to improve over time as the AI systems start to kick in, start to develop better quality of service,” said Cadence’s Dennison. “But straight out the box, it may not be perfect. So testing as it were is going to be a continuous thing, and something that the network operators are going to be relying on to improve the quality of their service. So there is yet another sort of difficulty that is new to us as we move from 4G to 5G.”
Thinking differently So far, there is no consistency in how companies propose to solve the signal attenuation problem for millimeter wave. Still, the solution may not just be blanketing the walls of buildings with small cells. At least part of the answer may be in thinking differently about how a 5G mmWave device behaves, or even what it looks like.
On the behavior side, one possible solution is to use different technology for the uplink and downlink.
“If a mobile device moves relative to the base station, the real problem is the uplink,” said David Hall, principal product marketing manager at National Instruments . “To use beamforming is difficult, so you can use LTE for the uplink and millimeter wave for the downlink. The big questions are about the infrastructure. Most of the end devices have full radio capability, but for the base stations, the choice is whether to use digital versus analog for beamforming. Analog circuits are less efficient, which creates more heat in the base station.”
The 5G standard allows for both and analog and digital, but the beams look different.
“If you using a digital beam, it involves a change of the waveform itself,” said Hall. “You need to adjust the phase to wave carriers.”
Another change involves the end device. The introduction of mmWave technology has much broader ramifications than being able to download more video.
“We shouldn’t get overly focused on 5G just being the next increment over 3G and 4G for the end user with the mobile phone,” said Cadence’s Dennison. “5G is laying claim to a lot of things, and one of them is a very low latency to enable robots, in particular. Robots today are in factories and they’re in cages in factories. If we can get the latency down in communicating with them—imagine you’ve got that massive AI on the edge and it’s talking to robots through 5G and that has a very low latency of communication over milliseconds of order—then you’ve got a chance that those robots can be responsive in real time and not harm human beings that are around them. So 5G opens up a whole new set of opportunities like that. Maybe robots will break out of the factories and the cages, and it could be 5G low latency might be the enabler for that. While these chipsets already are being developed for handsets, that is only one possible device that can take advantage of 5G.”
Along similar lines, there is a lot of concern about relying on 5G for linking cars to the cloud because connectivity is unreliable. But communication to other vehicles is a different story.
“With wireless network, the cars can talk to each other,” said Synopsys’ Zhang. “We can create a link so that they can they can communicate with that, with short latencies, with the highest possible car reliability because they don’t want cars to crash. Those are the things that make 5G more interesting.”
Conclusion Investments in 5G are rising, and so is the industry reliance on this technology for a variety of applications, including fixed structures such as smart buildings and cell phone towers that serve rural areas as well as densely populated areas. There is simply more data being produced and a growing demand for information everywhere.
The global communications business is betting heavily on 5G, even it remains to be seen exactly how that technology will be used and what an 5G end device actually looks like and how it will be served. Nevertheless, progress is being made on some of the most difficult problems in 5G, even if there is a long way to go before all the kinks are worked out and it is fully functioning like 4G LTE.
—Kevin Fogarty contributed to this report.

Ed Sperling
Leave a reply cancel reply.
Name * (Note: This name will be displayed publicly)
Email * (This will not be displayed publicly)
- High-NA EUVL: Automated Defect Inspection Based on SEMI-SuperYOLO-NAS April 10, 2024 by Technical Paper Link
- Chiplet Hardware Security Module To Mitigate Security Vulnerabilities In SiP Systems (Univ. of Florida) April 9, 2024 by Technical Paper Link
- Comparison Of The Meta Modeling Approach With HGLs April 9, 2024 by Technical Paper Link
- Using Palladium To Address Contact Issues Of Buried Oxide Thin Film Transistors April 9, 2024 by Technical Paper Link
- 3-Channel Package-Scale Galvanic Isolation Interface for SiC and GaN Power Switching Converters April 9, 2024 by Technical Paper Link
Knowledge Centers Entities, people and technologies explored
Related articles, money pours into new fabs and facilities, the rising price of power in chips, chiplet ip standards are just the beginning, the future of memory, risc-v micro-architectural verification, backside power delivery gears up for 2nm devices, visa shakeup on tap to help solve worker shortage, silicon photonics manufacturing ramps up, newsletter signup, popular tags, recent comments.
- Rakesh on Timing Library LVF Validation For Production Design Flows
- Mike Cawthorn on What Will That Chip Cost?
- Liz Allan on Early STEM Education Key To Growing Future Chip Workforce
- Rob Pearson - RIT on Early STEM Education Key To Growing Future Chip Workforce
- Maury Wood on Examining The Impact Of Chip Power Reduction On Data Center Economics
- Erik Jan Marinissen on Chiplet IP Standards Are Just The Beginning
- Peter Bennet on Design Tool Think Tank Required
- Dr. Dev Gupta on Chiplet IP Standards Are Just The Beginning
- Jesse on Hunting For Open Defects In Advanced Packages
- Matt on Chip Ecosystem Apprenticeships Help Close The Talent Gap
- Leonard Schaper IEEE-LF on 2.5D Integration: Big Chip Or Small PCB?
- Apex on Nanoimprint Finally Finds Its Footing
- AKC on Gearing Up For Hybrid Bonding
- Allen Rasafar on Backside Power Delivery Gears Up For 2nm Devices
- Nathaniel on Intel, And Others, Inside
- Chris G on Intel, And Others, Inside
- Richard Collins on Too Much Fab And Test Data, Low Utilization
- Jerry Magera on Why Chiplets Are So Critical In Automotive
- Jenn Mullen on Shattered Silos: 2024’s Top Technology Trends
- Valerio Del Vecchio on Security Becoming Core Part Of Chip Design — Finally
- Lucas on Hybrid Bonding Basics: What Is Hybrid Bonding?
- Robin Grindley on Expand Your Semiconductor’s Market With Programmable Data Planes
- V.P.Sampath on RISC-V Micro-Architectural Verification
- Thermal Guy on Is UCIe Really Universal?
- Colt Wright on Shattered Silos: 2024’s Top Technology Trends
- Nicolas Dujarrier on The Future Of Memory
- Tony on Challenges Of Logic BiST In Automotive ICs
- Raymond Meixner's child on Visa Shakeup On Tap To Help Solve Worker Shortage
- Michael Alan Bruzzone on How Is The Chip Industry Really Doing?
- Art Scott on How Is The Chip Industry Really Doing?
- Liz Allan on Rethinking Engineering Education In The U.S.
- Telkom University on Rethinking Engineering Education In The U.S.
- Ramesh Babu Varadharajan on SRAM’s Role In Emerging Memories
- jake_leone on Visa Shakeup On Tap To Help Solve Worker Shortage
- d0x on How Secure Are FPGAs?
- Mike Bradley on RISC-V Micro-Architectural Verification
- Charles E. Bauer ,Ph.D. on Visa Shakeup On Tap To Help Solve Worker Shortage
- AMAN SINGH on Power Aware Intent And Structural Verification Of Low-Power Designs
- Ed Trevis on Visa Shakeup On Tap To Help Solve Worker Shortage
- AMAN SINGH on Get To Know The Gate-Level Power Aware Simulation
- Pitchumani Guruswamy on RISC-V Micro-Architectural Verification
- Manil Vasantha on AI Accelerator Architectures Poised For Big Changes
- Ramachandra on Packaging Demands For RF And Microwave Devices
- garry on New Insights Into IC Process Defectivity
- Brian Bailey on The Good Old Days Of EDA
- Ann Mutschler on AI Accelerator Architectures Poised For Big Changes
- John Derrick on AI Accelerator Architectures Poised For Big Changes
- allan cox on AI Accelerator Architectures Poised For Big Changes
- Madhusudhanan RAVISHANKAR on Curbing Automotive Cybersecurity Attacks
- Eric Cigan on The Good Old Days Of EDA
- Peter Flake on The Good Old Days Of EDA
- Mike Cummings on MEMS: New Materials, Markets And Packaging
- Bill Martin on The Good Old Days Of EDA
- Gretchen Patti on 3D-ICs May Be The Least-Cost Option
- Carlos on An Entangled Heterarchy
- Ann Mutschler on Flipping Processor Design On Its Head
- Gil Russell on Flipping Processor Design On Its Head
- Ed Sperling on China Unveils Memory Plans
- David on The Limits Of AI-Generated Models
- Bill on The Limits Of AI-Generated Models
- Dr. Dev Gupta on Gearing Up For Hybrid Bonding
- Faizan on China Unveils Memory Plans
- Jan Hoppe on Streamlining Failure Analysis Of Chips
- Riko R on Why Curvy Design Now? Manufacturing Is Possible And Scaling Needs It
- Derrick Meyer on Higher Automotive MCU Performance With Interface IP
- Kevin Cameron on Why Silent Data Errors Are So Hard To Find
- Rale on How Secure Are RISC-V Chips?
- Ed Sperling on Patterns And Issues In AI Chip Design
- Chip Greely on Building Better Bridges In Advanced Packaging
- Art Scott on Setting Standards For The Chip Industry
- Muhammet on Higher Creepage And Clearance Make For More Reliable Systems
- Andy Deng on Quantum Plus AI Widens Cyberattack Threat Concerns
- Dr. Rahul Razdan on The Threat Of Supply Chain Insecurity
- Roger on Patterns And Issues In AI Chip Design
- David Leary on Improving Reliability In Chips
- Ann Mutschler on The Threat Of Supply Chain Insecurity
- Cliff Greenberg on Setting Standards For The Chip Industry
- Kevin Parmenter on The Threat Of Supply Chain Insecurity
- Esther soria on Automotive Complexity, Supply Chain Strength Demands Tech Collaboration
- Kumar Venkatramani on Predicting The Future For Semiconductors
- Spike on Is UCIe Really Universal?
- David Sempek on Power Semis Usher In The Silicon Carbide Era
- Dp on Specialization Vs. Generalization In Processors
- Eric on Addressing The ABF Substrate Shortage With In-Line Monitoring
- Karl Stevens Logic Designer on Software-Hardware Co-Design Becomes Real
- Jim Handy on MRAM Getting More Attention At Smallest Nodes
- Nicolas Dujarrier on MRAM Getting More Attention At Smallest Nodes
- Lou Covey on Are In-Person Conferences Sustainable?
- Cas Wonsowicz on AI Transformer Models Enable Machine Vision Object Detection
- Nancy Zavada on Are In-Person Conferences Sustainable?
- Fred Chen on High-NA Lithography Starting To Take Shape
- Dave Taht on Wi-Fi 7 Moves Forward, Adding Yet Another Protocol
- Robert Boissy on Rethinking Engineering Education In The U.S.
- Allen Rasafar on High-NA Lithography Starting To Take Shape
- Mathias Tomandl on Multi-Beam Writers Are Driving EUV Mask Development
- K on High-NA Lithography Starting To Take Shape
- Adibhatla krishna Rao on How Do Robots Navigate?
- Doug L. on Getting Rid Of Heat In Chips
- Ken Rygler on DAC/Semicon West Wednesday
- Mark Camenzind on Why IC Industry Is Great Place To Work
- Peter Bennet on The True Cost Of Software Changes
- ALLEN RASAFAR on Balancing AI And Engineering Expertise In The Fab
- Ron Lavallee on The True Cost Of Software Changes
- Alex Peterson on Welcome To EDA 4.0 And The AI-Driven Revolution
- Allen Rasafar on Managing Yield With EUV Lithography And Stochastics
- Art Scott on Rethinking Engineering Education In The U.S.
- Paul Clifton on Week In Review: Semiconductor Manufacturing, Test
- Mark L Schattenburg on A Highly Wasteful Industry
- Gordon Harling on Rethinking Engineering Education In The U.S.
- Santosh Kurinec on Rethinking Engineering Education In The U.S.
- Brian Bailey on Rethinking Engineering Education In The U.S.
- CdrFrancis Leo on Will There Be Enough Silicon Wafers?
- Riccardo Vincelli on How Safe Is Safe Enough?
- Jem on 3D Structures Challenge Wire Bond Inspection
- Nikolay on Nanoimprint Finally Finds Its Footing
- Ed Korczynski on Thermal Integrity Challenges Grow In 2.5D
- Allen Rasafar on What Data Center Chipmakers Can Learn From Automotive
- Advertising on SemiEng
- Newsletter SignUp
- Low Power-High Perf
- Knowledge Centers
Connect With Us
- Twitter @semiEngineering
Privacy Overview
5G Coverage Problem Analysis and Optimization Scheme Exploration
Ieee account.
- Change Username/Password
- Update Address
Purchase Details
- Payment Options
- Order History
- View Purchased Documents
Profile Information
- Communications Preferences
- Profession and Education
- Technical Interests
- US & Canada: +1 800 678 4333
- Worldwide: +1 732 981 0060
- Contact & Support
- About IEEE Xplore
- Accessibility
- Terms of Use
- Nondiscrimination Policy
- Privacy & Opting Out of Cookies
A not-for-profit organization, IEEE is the world's largest technical professional organization dedicated to advancing technology for the benefit of humanity. © Copyright 2024 IEEE - All rights reserved. Use of this web site signifies your agreement to the terms and conditions.

Global Atlanta
The world is here.
Solving for 5G: How Math Modeling Can Improve Modern Communications
Share this:
- Click to email a link to a friend (Opens in new window)
- Click to print (Opens in new window)
- Click to share on LinkedIn (Opens in new window)
- Click to share on Twitter (Opens in new window)
- Click to share on Facebook (Opens in new window)
- Click to share on WhatsApp (Opens in new window)

Editor’s note: This commentary, written by Assistant Professor Eric Stachura, is published as part of an annual sponsorship of Global Atlanta by Kennesaw State University’s Division of Global Affairs.
Mathematical modeling sounds intimidating and complicated, but also vague. What does it really mean, and more importantly, what does it mean for the world?

According to the Society of Industrial and Applied Mathematics , the process involves using mathematics to represent, analyze, make predictions or otherwise provide insight into real-world phenomena.
Any student who has banged their head on a desk while wrestling with an equation has grasped the need to connect theoretical thinking to solving real-world problems.
It’s easy to get lost in entire courses or abstract disciplines related to math (and some of us do), but at its core there exists a more utilitarian function of our discipline, and mine in particular: Mathematical modeling teaches us how to make assumptions, test predictions, come up with formulas and analyze and assess the solutions.
From COVID to Cell Phones
Modeling entails a particularly useful set of skills today, in light of the current pandemic, for it permits policy makers to foresee the consequences of decisions, as well as educate all of us on the nature of the pandemic and what we can do about it.
But not all mathematicians are epidemiological modelers, and there is a myriad of other big questions toward which we can aim our academic ammunition.
In the modern world, there may be no more vital infrastructure than that of telecommunications, and Swedish companies like Ericsson are world leaders, particularly in the hardware that relays 5G mobile signals, promising exponential increases in mobile Internet speed.
But as many know, questions linger about deployment: particularly how radiofrequency signals will behave in the built environment.
Last June, I was part of a collaborative research project along with Elena Cherkaev ( University of Utah ) and Niklas Wellander (FOI Sweden ) charged with implementing mathematical modeling to ultimately help improve modern communication systems.
Funded in part by an American-Scandinavian Foundation grant , I went to Linköping, Sweden , southwest of the capital in Stockholm , with the particular goal of studying Passive Intermodulation (PIM) from a rigorous mathematical perspective.
Signal Integrity
PIM occurs when multiple signals are active in a passive device (such as a cable) that exhibits a nonlinear response. Frequently, PIM occurs as a result of multiple cell phone providers sharing certain paths in wireless networks. To the everyday user on their cell phone, this can manifest as decreased data speed or even dropped calls.
The focus of our research is the relationship between PIM, temperature effects and rough surfaces. In particular, taking into account the heat generated by radio-frequency signals, we are working to understand how surfaces with sharp corners or angles affect signal integrity.
While the research is ongoing, the impact is clear: understanding the effects of geometry (such as buildings in a large urban environment) on signal integrity is important for the development of improved cellular networks. What we find will affect everyone: From the drivers of future autonomous vehicles to the companies relying on 5G technology to track their products, as well as patients enjoying newly enabled telemedicine sessions.
Indeed, the state of Georgia has already been involved in 5G innovation: the Marine Corps Logistics Base in Albany was selected by the Department of Defense as a testing ground for 5G-enabled warehouses.
To unlock the advantages of these and other technologies, we will need to continue to drive new and collaborative thinking around their usage — perhaps even breaking out a mathematical model every now and then for problems that don’t seem like they can be solved on paper.
About the author:
Dr. Eric Stachura has a B.S. and Ph.D. in Mathematics from the University of Illinois at Chicago and Temple University, respectively. He is currently an Assistant Professor of Mathematics at Kennesaw State University in Atlanta. His research interests include problems at the interface of mathematics and physics, in particular nonlinear electromagnetics and geometric optics. During his studies, he has spent two significant periods abroad studying and conducting research: one at the Eidgenossiche Technische Hochschule (ETH) in Zurich, Switzerland , and one at the Instituto de Ciencias Matematicas (ICMAT) in Madrid, Spain.
In addition to his research, he is interested in innovative teaching techniques. As part of the Silver’19 cohort of the Mathematical Association of America’s Project NExT, he has incorporated numerous active learning strategies into his classes.

GSU-CIBER is the presenting sponsor of Global Atlanta's Education Channel . Subscribe here for monthly Education newsletters.

Atlanta International School is the presenting sponsor of Global Atlanta's Technology Channel. Subscribe here for monthly Tech newsletters.
Leave a comment
You must be logged in to post a comment.
SIGN UP FOR GLOBAL ATLANTA NEWSLETTERS
Join more than 10,000 readers receiving timely updates on the latest international business news in Atlanta.
- IEEE Xplore Digital Library
- IEEE Standards
- IEEE Spectrum
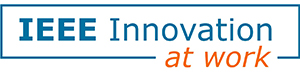
5G is Finally Here, But Questions and Issues Remain
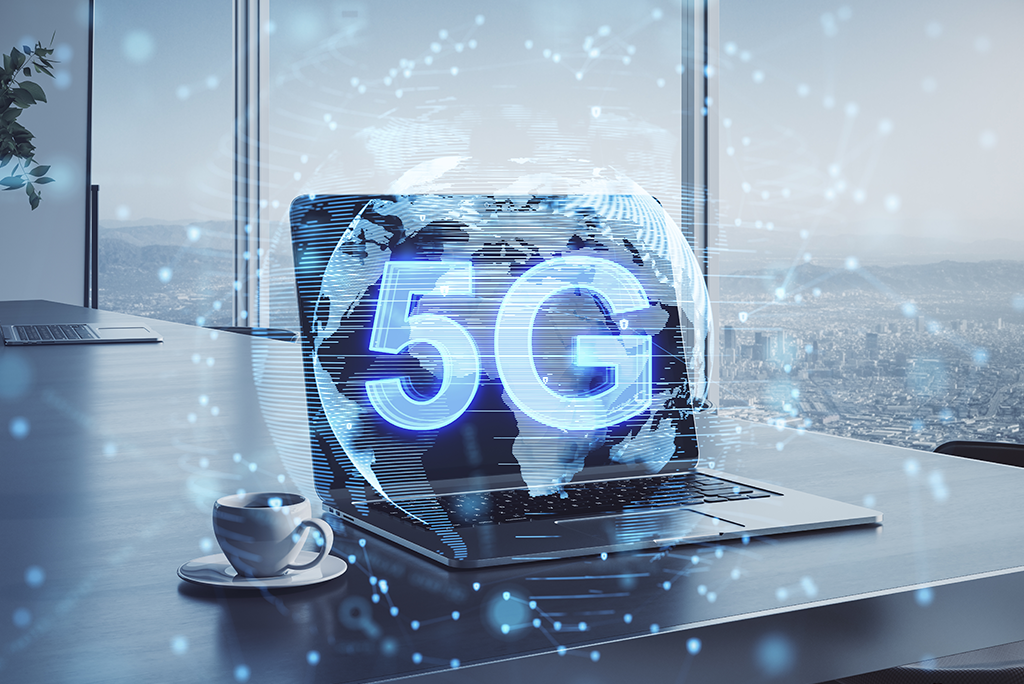
5G has been publicized as a substantial change in mobile networking—promising faster download speeds, real-time data-sharing, and network capacity. As such, the technology is expected to transform mobile networking and create new economic opportunities . Despite delays with the rollout in both the U.S. and parts of Europe, 5G has reached much of the globe. South Korea, the U.S., and China are leading the implementation with around 80-90% population coverage. Although Europe overall is trailing behind, Switzerland has reached 90%. A 2021 report from Ericsson projects 5G will account for nearly half of all mobile subscriptions by 2027 while also becoming mainstream in each of the report’s ten studied regions. Though challenges persist for deployment globally, technology providers are optimistic about the future of 5G.
Forecasts for Industry
While the majority of 5G-supported or enabled applications today are consumer-based (think mobile streaming, augmented reality, virtual reality, and gaming), many experts believe the real money will be in enterprise applications of 5G. In its 5G value report, KPMG estimated the business-to-business case at US$4.3 trillion . Across industries—from factory automation and large-scale video surveillance to remote surgery and connected smart cities— there are a number of potentially groundbreaking use cases.
5G enables fast, secure, and pervasive connectivity across smart networks and Internet of Things (IoT) devices. When combined with artificial intelligence, 5G can enable unparalleled productivity and efficiency. Apart from the global estimate, five industries are poised to see incremental growth : Industrial Manufacturing, Connected Healthcare, Intelligent Transportation, Environmental Monitoring, and Gaming. KPMG estimates the market across the ecosystem for these five industries will be worth more than US$500B by 2023.
Managing Expectations
Before organizations adopt 5G, they should understand the differences between 4G and 5G network architectures to understand how both could affect business operations. Small cell technology enables 5G to provide more cell density and enhance network capacity. While 4G technology also made similar promises, experts anticipate 5G will succeed where its predecessor falls short. However, it is essential to understand that there are still 5G issues, and it may take years to reach its full potential.
Health Concerns Regarding 5G
When you use your phone to communicate with other devices, cellular data is sent through radio frequencies (RFs). “The radiofrequency 5G is higher than the previous iterations of wireless communication, including 4G and 3G”, says Henk De Feyter, Ph.D., an assistant professor of radiology and biomedical imaging at Yale School of Medicine in New Haven, Connecticut. In a world of propaganda and misinformation about 5G, how are policymakers and the public supposed to make sense of any individual claim? (For example, various internet theories have tied 5G technology to cancer and COVID-19.)
Learn more about this topic by watching 5G Demystified: Health and Safety of 5G , an on-demand LinkedIn Live recording where our speakers discuss the science and standards of human health effects from electromagnetic radio waves in 5G communications. Watch now>>
More Ways to Explore Current 5G Issues with IEEE
Depending on what you read, 5G is either a threat to society, the impetus for the next industrial revolution, or a marketing ploy to get us to buy new phones and tablets. Seldom has an emerging technology been so widely known yet so misunderstood. IEEE Future Networks and IEEE Educational Activities have developed a free virtual event series, 5G Demystified , where experts make sense of the technology’s potential.
Check out the events in this series:
- Hype vs. Reality of 5G
- Broadband is Infrastructure?
- Health and Safety of 5G
Plus, check out these online course programs and earn continuing education credits while growing your knowledge of telecommunications technology!
Bridging the 4G/5G Gap: Telecommunications Roadmap for Implementation: This two-part course program provides a historical overview of 4G/5G, explains the legislative and regulatory background, showcases the scientific evidence surrounding wireless facilities’ impact on property value and human health, and offers a roadmap to deploy wireless facilities. Learn more>>
5G Networks: Produced in partnership with Nokia, this online course program provides an in-depth view of performance requirements, future scenarios, and the roadmap to 5G implementation. It also explores the intricacies of 5G standardization by the 3rd Generation Partnership Project (3GPP) and the IEEE 802 LAN/MAN Standards Committee. Learn More>>
Ericsson. (November 2021). Ericsson Mobility Report .
Goss, Michaela. (November 2022). 5G vs. 4G: Learn the key differences between them . TechTarget.
Holt, Alex. (June 2020). The 5G edge computing value opportunity . KPMG.
Laurence, Emily. (31 May 2022). Is 5G Making You Sick? Here’s What Experts Say . Forbes.
Vella, Heidi. (17 February 2022). 5G vs 4G: what is the difference? Raconteur.
5G , 5g health risks , 5g networks , IoT , mobile network , telecommunications
No comments yet.
Leave a reply click here to cancel reply..
Name (required)
Email (will not be published) (required)
Subscribe to get the best articles, infographics, and course news delivered straight to your inbox.
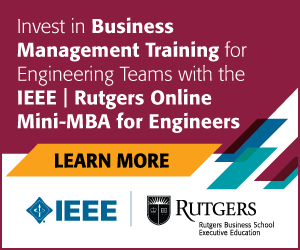
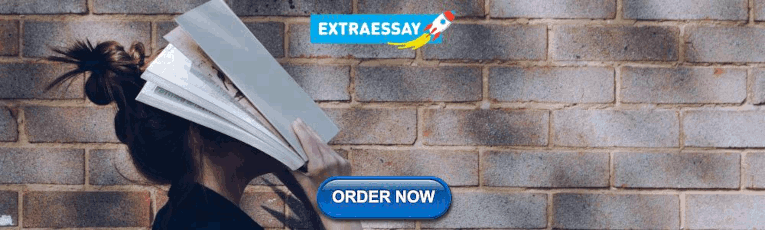
The McKinsey Crossword: 5G | No. 155
Play the interactive version, or if you like putting pen(cil) to paper, download and print when you're ready to engage. Check back each Tuesday for a new puzzle or, better yet, sign up to get an alert each week when the next challenge is ready.
See all puzzles
Related Articles
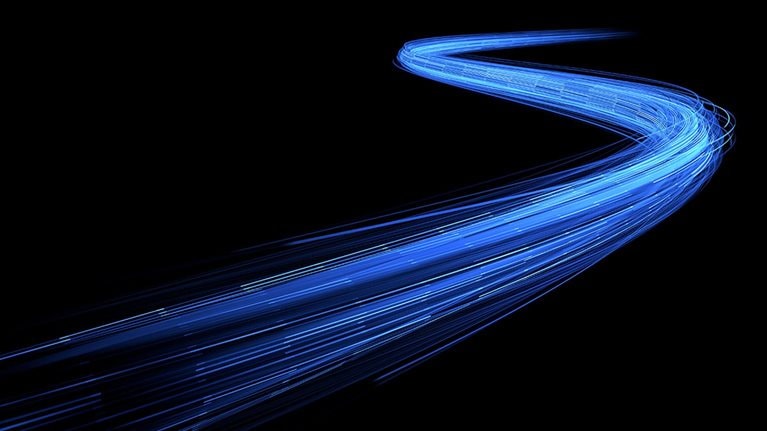
Navigating the three horizons of 5G business building
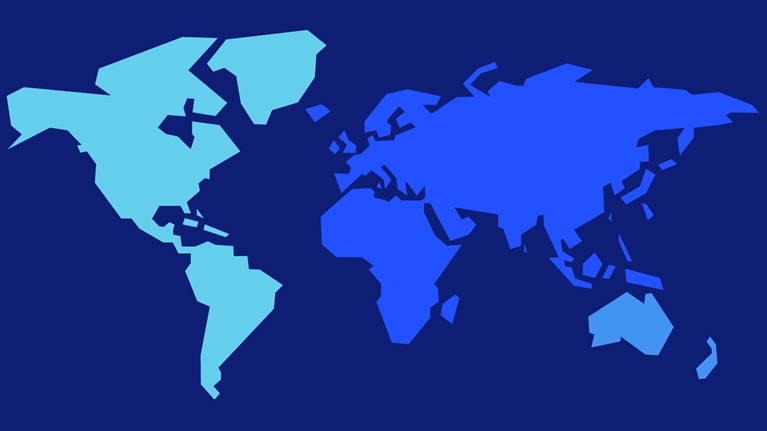
Global trade explorer
This website will offer limited functionality in this browser. We only support the recent versions of major browsers like Chrome, Firefox, Safari, and Edge.
What to expect from the AI/ML in 5G Challenge
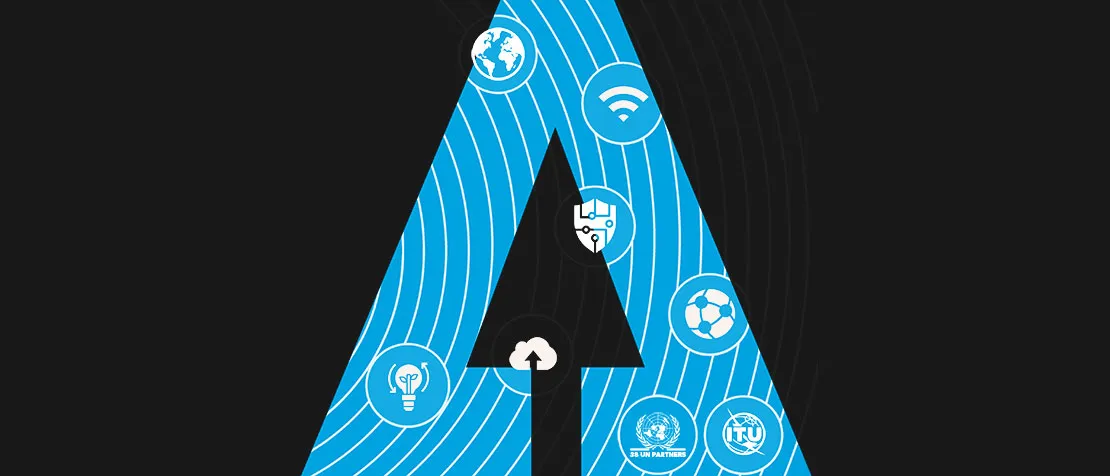
The 2nd Artificial Intelligence/Machine Learning (AI/ML) in 5G Challenge is set to conclude in December, capping a successful debut last year.
Organized by the International Telecommunication Union (ITU) as part of its AI for Good initiative, the competition sees participants around the world solving real-world problems by applying machine learning in communications networks. Reinhard Scholl, Deputy Director at ITU’s Telecommunication Standardization Bureau, shares the journey of the Challenge and what’s coming up next.
This year’s AI/ML in 5G Challenge is the second such competition. What were your learnings from the first year?
Reinhard Scholl: When we started the Challenge, we had no idea where it would be going. It was an adventure and turned out to be such a positive experience. Last year, we had participants from 62 countries.
This year, we have participants from 82 countries, with the Grand Challenge Finale scheduled on 14 December.
We never expected such big numbers. We were also surprised by the large number of problem statements – between 15 and 20 each year – that we were able to offer so far. And we are grateful to this year’s sponsors, Xilinx and the Republic of Korea’s Ministry of Science and ICT.
We also published a special issue on AI and machine learning solutions in 5G and future networks in the ITU Journal Future and Evolving Technologies , with a selection of peer-reviewed papers submitted by Challenge participants.
We are on the lookout for new problem statements for the third Challenge .
One thing we are hoping to offer next year is computing resources for participants who might not have the support of a rich university or company. Training machine-learning models can take a lot of time, and several participants informed us that they don’t have the resources to run meaningful models. So, we are working on that.
How does the Challenge align with the work of the ITU Telecommunication Standardization Sector (ITU-T) and the AI for Good platform?
ITU-T does a lot of technical work related to machine learning in its focus groups – six of which have AI or machine learning in their title – and in its study groups. The specifications of the focus groups are generally turned into ITU standards (“ITU-T Recommendations”).
The most popular standard is the “Architectural framework for machine learning in future networks including IMT-2020” ( ITU-T Y.3172 ), which gives a common nomenclature and primer on how to talk about machine learning in communication networks, so that it can be used by anyone for any network.
Some of the solutions submitted to problem statements in the ITU AI/ML in 5G Challenge reference ITU standards on machine learning. Some have generated contributions to the respective focus groups or study groups – and attracted new ITU-T members. We have run over 50 one-hour, in-depth talks so far – by researchers on machine learning and communication networks – on the AI for Good Discovery Channel , a fabulous resource on what the future of communication networks will look like. We have similar “Discovery Channels” on Trustworthy AI , AI and Health , as well as AI and Climate Science . In January 2022 we are going to launch a Geospatial AI Discovery Channel.
What are the opportunities and challenges you see for AI and ML in the real-world 5G sector?
Network operators have used machine learning for some time, but not at the network level. They have used it to analyse the churn rate or to segment their customers. But applying it at the networking level is complicated. Applying machine learning in communication networks is much more difficult than in computer vision or natural language processing, because time scales in a communication network span many orders of magnitude, ranging from parameters which change on an annual basis, like your subscription to a telecom provider, to milliseconds, like resource block allocations in radio access networks) – for which you then have to retrain your machine learning model on a millisecond basis.
As networks get more and more complicated, machine learning will be essential to make sense of the plethora of data being collected. On the other hand, machine learning could also be useful in the standardization process.
For now, standards are produced by people, who meet, make proposals, negotiate, and agree on a certain outcome. But the resulting protocols are often ambiguous and suboptimal, leading to increasing costs in testing and implementation. Part of this process could be taken on by machine learning, where the algorithm proposes a solution. There have been some attempts to do this, but there’s still quite a long way to go.
What else can we expect?
We are branching into a new Geospatial AI Challenge that draws on location-based data. We have launched a call for problem statements. ITU and the World Health Organization (WHO) meanwhile are working, through their joint focus group, on an incredibly ambitious AI for Health Assessment project. When we take a prescribed medicine or vaccine, there is a sense of trust in the process and in the institutions.
But why would you trust an AI model looking at your X-rays? What does it take to trust a company that has an AI solution to detect skin cancer? The focus group is building a benchmarking framework that allows people to trust in AI health solutions.
The ITU-WHO focus group will come up with a process, guidelines, and best practices to ensure trust in AI solutions.
In addition, it’s developing a platform where a company can submit and test solutions using undisclosed data. A score is generated and published on a leader board, which also allows a regulator to know how good the solution is. You must design a process that allows experts to come to an agreement and then build that into the platform. The prototype will be ready in a matter of weeks. Then it needs to be transformed into a professional platform, which will cost serious money. We are going to start an AI for Good Fund to secure donations for projects like the AI for Health Assessment Platform, along with other work, such as the AI and Road Safety global initiative established in October.
Learn more about the Artificial Intelligence/Machine Learning (AI/ML) in 5G Challenge.
Related content
Itu journal shares research on next-gen performance, energy efficiency, and security, connect with itu standards experts at ofc, world telecommunication/ict indicators database online.
This information is available only to people with TIES access.
Privacy Overview

An official website of the United States government
The .gov means it’s official. Federal government websites often end in .gov or .mil. Before sharing sensitive information, make sure you’re on a federal government site.
The site is secure. The https:// ensures that you are connecting to the official website and that any information you provide is encrypted and transmitted securely.
- Publications
- Account settings
Preview improvements coming to the PMC website in October 2024. Learn More or Try it out now .
- Advanced Search
- Journal List
- Sensors (Basel)

Handover Parameters Optimisation Techniques in 5G Networks
Wasan kadhim saad.
1 Engineering Technical College-Najaf, Al-Furat Al-Awsat Technical University (ATU), Najaf 31001, Iraq; [email protected]
2 Electronics and Communication Engineering Department, Faculty of Electrical and Electronics Engineering, Istanbul Technical University (ITU), Istanbul 34467, Turkey; [email protected]
Ibraheem Shayea
Bashar j. hamza, hafizal mohamad.
3 Faculty of Engineering and Built Environment, Universiti Sains Islam Malaysia, Bandar Baru Nilai, Nilai 71800, Malaysia; ym.ude.misu@lazifah
Yousef Ibrahim Daradkeh
4 Department of Computer Engineering and Networks, College of Engineering at Wadi Addawasir, Prince Sattam Bin Abdulaziz University, Al Kharj 11991, Saudi Arabia; [email protected]
Waheb A. Jabbar
5 Faculty of Electrical & Electronics Engineering Technology, Universiti Malaysia Pahang, Pekan 26600, Malaysia; gro.eeei@behaw
6 Center for Software Development & Integrated Computing, Universiti Malaysia Pahang, Gambang 26300, Malaysia
Associated Data
Not applicable.
The massive growth of mobile users will spread to significant numbers of small cells for the Fifth Generation (5G) mobile network, which will overlap the fourth generation (4G) network. A tremendous increase in handover (HO) scenarios and HO rates will occur. Ensuring stable and reliable connection through the mobility of user equipment (UE) will become a major problem in future mobile networks. This problem will be magnified with the use of suboptimal handover control parameter (HCP) settings, which can be configured manually or automatically. Therefore, the aim of this study is to investigate the impact of different HCP settings on the performance of 5G network. Several system scenarios are proposed and investigated based on different HCP settings and mobile speed scenarios. The different mobile speeds are expected to demonstrate the influence of many proposed system scenarios on 5G network execution. We conducted simulations utilizing MATLAB software and its related tools. Evaluation comparisons were performed in terms of handover probability (HOP), ping-pong handover probability (PPHP) and outage probability (OP). The 5G network framework has been employed to evaluate the proposed system scenarios used. The simulation results reveal that there is a trade-off in the results obtained from various systems. The use of lower HCP settings provides noticeable enhancements compared to higher HCP settings in terms of OP. Simultaneously, the use of lower HCP settings provides noticeable drawbacks compared to higher HCP settings in terms of high PPHP for all scenarios of mobile speed. The simulation results show that medium HCP settings may be the acceptable solution if one of these systems is applied. This study emphasises the application of automatic self-optimisation (ASO) functions as the best solution that considers user experience.
1. Introduction
The explosive growth of mobile communications, the diversity of networks, and three-dimensional (3D) mobile communications (e.g., drones) will radically increase mobile data demands, in which servicing will require a large number of UEs for deploying huge amounts of small and interfering BSs [ 1 , 2 , 3 ]. With the rapid growth of the Internet of Things (IoT), the 3rd Generation Partnership Project (3GPP) proposed a new wireless network generation (5G) to address the numerous challenges faced by existing networks. However, an equally important and additional aspect discussed by 3GPP through the efforts of ongoing standardisation is the aspect of mobility in 5G networks. To become the new wireless standard to exist worldwide, 5G must allow for unrestricted user mobility while effectively managing itself. The methods, as discussed in [ 4 ], aim to provide management efficiency by applying techniques that emphasise requests based on mobility management (MM). An essential component of MM is HO management, since HOs allow users to switch the network anchor point while maintaining service continuity through mobility events in existing cellular networks. Thus, effective HO management will be vital due to the heterogeneous and extremely dense nature of 5G networks [ 5 , 6 , 7 , 8 , 9 , 10 , 11 , 12 , 13 ].
The access of mobile phone users and the resulting traffic load in cellular networks are variable over time, frequently unbalanced and random, making cell loads in the system unequal. In some cells, excessive amounts of UEs are available but overloaded; while, in other cells, fewer UEs are present, and their resources are not fully utilised. The inefficient use of resources can be mitigated through optimised administration as well as network development. The current network planning strategies are far from completely resolved, due to issues such as load balancing (LB) in long-term evolution (LTE) systems [ 8 , 14 , 15 , 16 ]. Generally, converting some UEs at the boundaries of overlapping or adjacent cells from more crowded cells to less crowded cells represent a possible approach for LB; this is often referred to as HO or handoff. By changing the Evolved Node B (eNB) units assigned to UE, the load is balanced, and the system performance is improved at the expense of system overheads, generated by HOs. The HO procedure consumes significant system resources, and the intended UE may experience substantial system delays and performance deterioration. Therefore, HO should not randomly occur [ 17 , 18 ]. Additionally, the use of mm waves in 5G technology is the dominant factor that impacts mobility [ 19 , 20 ]. This is due to higher path loss when using mm-wave frequency bands, thereby decreasing cell coverage. HO probability will significantly increase, leading to an upsurge in the number of mobility issues, such as high HOF, PPHP impact and OP.
The Handover Parameters Optimisation (HPO) is an important function of the self-optimisation network (SON). It was introduced by 3GPP for solving the mobility issues in 4G and 5G mobile phone networks [ 21 , 22 , 23 , 24 ]. Several functions are offered by SON, such as mobility robustness optimisation (MRO) and load balancing optimisation (LBO). Both functions lead to necessary optimisations to achieve various goals throughout the mobility of the utilizer and aims to dynamically enhance HCP values to confront numerous HO problems. MRO was initially proposed in the LTE-Advanced (LTE-A) as part of SON, where it sets HCPs, such as handover margin (HOM) and time to trigger (TTT), to maintain communication links throughout user movements with a minimum number of overlapping operators [ 25 ].
The function of MRO automatically adjusts the values of HCP to preserve the quality of the system. It also automatically optimises HCPs with minimum human overlap. Through adjusting these parameters to appropriate values by the movements of the utilizer within the coverage of the cell, PPHP rates and handover failure (HOF) are adequately reduced, thus improving the quality of service (QoS) [ 26 , 27 ]. HPO functionality was presented as a primary merit in deploying 4G and 5G networks. Its key goal is to automatically adjust HCP settings to maintain the quality of the network. The specific goal of HPO is to detect and correct the impact of both PPHP and OP because of mobility. In another sense, the HPO algorithm adaptively adjusts the settings of HCP when OP or PPHP is detected because of HO is too precocious and HO is too arrear, as shown in Figure 1 . It can also be due to the ineffective usage of the resources of system, caused through unnecessary handover (UHO).

HO problems because of settings of suboptimal HCP [ 34 ].
One of the main methods for improving the performance of 5G network mobility is optimising HCP settings. If HCPs are set to static settings, continuous connection will be adversely influenced, particularly when UE speed is remarkably high. Therefore, HCP settings must be appropriately modified to resolve this deficiency. However, manual modification will complicate the maintenance and management of the system. As a result, the HPO function has been submitted by 3GPP as the primary feature in 4G and 5G network deployment [ 28 , 29 ]. This function automatically estimates the occasion settings of HCP, depending on the conditions of the instantaneous network. Various studies were accomplished to handle this insufficiency [ 11 , 30 , 31 , 32 , 33 ].
In the literature, several algorithms exist to optimally calculate HO parameters, such as TTT and hysteresis value. The algorithms in [ 35 , 36 ] are methods for overcoming the influence of the ping-pong effect due to HO. HO optimisation between femto and macro BS (by exploiting the information of UE, such as velocity, RSSI, etc.) was also discussed in [ 37 ]. Several utilised algorithms have demonstrated ineffective input parameters in their design, resulting in the inaccurate estimation of HCP settings. Currently, mobility with high requirements within 5G networks (such as mm waves and lower latency) has led to the requirement of HPO algorithms that are further progressed. It has become a major development requirement to successfully process the mobility problems found in 5G networks. The available algorithms in the literature [ 11 , 30 , 31 , 32 , 33 ] provide effective optimisation of HCP settings; however, there is no perfect solution, since some suggested algorithms only adjust HCP settings depending on the single parameter (e.g., distance or speed). While many affecting factors must be considered to estimate appropriate HCP settings (e.g., distance, interference, channel state, resource availability, noise and UE speed), these configurations are approximated from a single factor perspective will only cause insufficient HCP settings. Some of these algorithms, such as the Adaptive Handover Algorithm (AHA), are dependent on the distance, speed and Fuzzy Control Algorithm (FCA) [ 38 , 39 , 40 ] where the FCA only sets the HOM level, and the TTT is set to a constant value. This failure diminishes the key aim of the HPO, task all characterised algorithms and optimise per cell, excluding AHA. This may allow for some UEs to execute the HO for other cells, while they do not need to perform HO at that time; thus, unnecessary HOP will increase due to suboptimal HCP settings.
The objective of this paper is to reduce the OP and PPHP occurrences through HO processes while adjusting HCP settings. Several system scenarios have been proposed, such as fixed HOM values and fixed TTT intervals, based on various HCP settings and different mobility speed scenarios, to clarify the effect of these scenarios on 5G network performance. The framework of the 5G network was applied to evaluate the proposed systems scenarios, utilised by comparing the HOP, PPHP and OP with different mobile speeds. The proposed scenarios were then investigated and compared according to the simulation study through utilizing MATLAB 2020a software.
The key contribution of this paper is investigating the effect of various HCP settings on the performance of 5G networks in terms of PPHP and OP. Several system scenarios are proposed, according to various HCP settings with different mobility speed scenarios. The proposed systems in this paper provide noticeable differences in system performance with the use of lower HCP settings, compared to higher HCP settings. The results indicate that medium HCP settings may be a better solution when utilising one of these systems. The consideration of automatic optimisation is another excellent solution that shall be focused on in future investigations and developments.
In summary, this work provides the following listed contributions:
- The impacts of various HCP settings on the system performance of the 5G network are studied based on three key performance metrics: HOP, PPHP and OP.
- Various HCP system settings, HOM and TTT are proposed and investigated for the 5G network with various scenarios for the speed of mobile.
- The suggested systems are validated to ensure their efficiency in the 5G network.
The rest of this paper is organised as follows: Section 2 presents the related works of this study. Section 3 briefly discusses the main HO performance evaluation metrics. Section 4 highlights the system model and the proposed solution of the simulation scenario used in this paper. Section 5 discusses the simulation and performance evaluation analysis. Finally, Section 6 presents the conclusions of this paper.
2. Related Works
A major challenge in wireless networks is the use of numerous algorithms, suggested in the literature for optimising HCP settings [ 41 , 42 , 43 , 44 , 45 , 46 , 47 , 48 ]. Several methodologies have applied these algorithms and examined them in different environments. The authors in [ 41 ] proposed the machine learning and data mining (MLDM) technique to optimise HO parameters that have been evaluated in the long-term evolution (LTE) system within the a building environment. In addition, a high-mobility SON function has been offered in [ 42 ] to shorten the multi-layer time that performs the optimisation process in the LTE system. It estimates the behaviours of user mobility according to data measurements, previously collected through utilizers. Furthermore, the authors in [ 44 ] presented an algorithm to adaptively adjust HOM, based on the user’s position in the cell, whereby, the HOM further decreases the closer the utilizer is to the edge of the cell. The researchers in [ 43 ] presented an adaptive algorithm that specifies various HOM values and load balancing (LB) to every UE in HetNets. The decision of HO in this suggested algorithm is based on the signal to interference noise ratio (SINR) instead of the strength index of the received signal, which is subsequently utilised to compute the HOM actual level. The authors in [ 44 ] also introduced an Enhanced Mobility Status Estimate (EMSE) for optimising HCPs according to HO types and user velocity by estimating the state of mobility. In this paper, the authors updated TTT by solely depending on user speed with limited TTT refresh values. This method did not completely optimise the performance of HO because the gap between the refresh values was too large, and the three fixed values were only chosen according to the UE speed. The authors in [ 45 , 46 ] discussed several executions of LTE HO frequencies, while the researchers in [ 47 , 48 ] considered the optimisation of the inter-system HO parameter.
Various studies have suggested several algorithms for solving and addressing HO problems. The authors in [ 49 ] described the strategy of HO decision and the estimation scheme of the mobility state to avoid service failure and unnecessary HOs (UHOs) in HetNets. The suggested model uses the HOs number, as well as the measurements of residence time, to appreciate the speed of UEs. Simulation results indicated that the suggested model’s mobility state estimation reduces service failures and UHO number; however, its execution in relation to other performance metrics of HO were not discussed, such as Outage Probability (OP), PPHP and delay.
The authors in [ 32 , 33 ] suggested an HO optimisation technique, according to the weighted function of the conveyor assembly. The suggested algorithm automatically modifies the HOM values, based on three functions: speed, traffic load and SINR. The simulation results revealed that the suggested algorithm would boosts execution of the system in terms of OP and spectral efficiency at the edge of the cell.
The authors in [ 50 ] proposed a method to compare the intersections of cell boundaries and the implementation of HO to optimise the performance of the overall network.
The researchers in [ 51 ] adopted a model for HOM optimisation according to fuzzy logic for HetNets, where the fuzzy logic consisting of two inputs, the LB index and the call drop rate, both of which consist of HOM adaptations for small and macro cells.
The authors in [ 11 , 30 , 52 ] proposed several algorithms for investigating and evaluating the mobility management problem in various mobile phone velocity scenarios. Three types of HO have been considered for conditioning HCPs: very late, very early and HO for wrong cells. The simulation results revealed that the modified HCP providers reduce the HOP, OP and HPPP rates.
However, the proposed HO-SON algorithms in the aforementioned papers were found to be inefficient in estimating optimum HCP settings. Although these algorithms contribute to enhancing the performance of HO, but it is neither strong nor ideal in choosing the occasional values of HCP in the 5G system. The current algorithms are inadequate for different reasons. One of the key reasons is that most of these algorithms were developed for 4G technology, which have various requirements and specifications compared to 5G technology. Further investigations are needed to develop the existing algorithms used in previous cellular networks to be effectively executed in 5G networks with different scenarios of mobility and deployment. Another reason is the HCP types considered for optimisation; several current algorithms do not optimise all HCP settings. For instance, algorithms in [ 39 , 53 , 54 ] only optimise one HCP (i.e., HOM) which may cause an increase in HO. Using a static TTT could lead to another HO problem which the Handover Parameter Optimisation (HPO) aims to process. Thus, the HPO algorithms must be developed with high efficiency and should be validated for 5G networks.
In [ 55 ], the threshold approach to the HO procedure has been incorporated into the multi-criteria decision-making (MCDM) process to select the Radio Access Technologies (RATs). The available RATs rankings are ordered through different MCDM algorithms and, depending on the specific threshold of HO, where the decision of whether or not to perform the process of HO is made. The suggested method works to improve the performance of the system, as well as to reduce HO by 13.14%, 19.35% and 8.62% of the RAT amendments for the technique for order preferences by similarity to the ideal solution (TOPSIS), preference ranking organization method for enrichment evaluation (PROMETHEE) and simple additive weighting (SAW) algorithms, respectively.
In [ 7 ], the algorithm for speed-based self-optimization was proposed to modify the HCPs in 4G/5G networks. The suggested algorithm uses the user’s received velocity and power to modify the TTT and HOM as the user navigates in the network. The simulation results show that the suggested algorithm outperforms the other existing algorithms by a rate of more than 70% for all measures of HO performance.
In [ 56 ], the authors proposed a new selection mechanism of context-aware radio access technology (CRAT), which examines user and network contexts in selecting the appropriate RAT for a service. The proposed CRAT performance was tested by using two various scenarios through a smart city environment, namely urban city and shopping centre scenarios by changing the environment parameters for measuring the performance of the proposed mechanism in a near-realistic situation. The results demonstrated that CRAT can help to enhance the utilizer experience through a smart city environment, where it outperforms the traditional A2A4 approach to choose the RATs in terms of number of HOs, throughput, packet delivery ratio and average network delay. Generally, Table 1 briefly summarizes the HO optimisation approaches and displays the utilized data model type and how to take HO decisions.
Summary of the HO optimisation approaches.
Although several studies that focused on HCPs have been conducted in the literature, they are mostly focused on 3G and 4G networks. This means that there is a need for further investigation in 5G networks, with various system scenarios and settings. Moreover, there is no comprehensive study that has considered all of the key performance indicators (KPIs). Thus, in this paper, the impact of different HCP settings has been considered for 5G network performance through various proposed system scenarios with different mobility speeds, all of which are modified depending on the PPHP and OP conducted in the measurement period. Meanwhile, system performance considered various KPIs. The simulation results reveal that the proposed system provides notable improvements with the use of lower HCP settings in terms of OP compared to higher HCP settings. The noticeable drawback of increased PPHP for different mobile speed scenarios is also present. The results prove that medium HCP settings may be the best solution to consider when using one of these systems.
3. The Key HO Performance Evaluation Metrics
Many performance indicators or main performance metrics (MPMs) are frequently defined in wireless networks to determine Quality of Service (QoS). To analyse the proposed HO preparation and signals of failure, HOP, PPHP and OP are the metrics used for evaluation. Employing these metrics is standard practice for assessing new HO strategies. Thus, the proposed algorithm fulfils this criterium and the indicators are compared with previous algorithms using three key MPMs, as follows:
HOP: This is the probability of links exchanged between the source and the target eNBs, where how HO repeatedly occurs between the source and target eNBs is measured. In other words, it is basically handing probability through the served UE from the source to the target eNBs when the quality of the source signal becomes worse than the strength of the target signal by the level of HOM. Thus, HOP can be translated into the HOs average number per call across all UEs served for increasing the accuracy of performance appraisal. The average HOP is computed per simulation period across all UEs served in the network, hence, the HOs average number per UE ( H O P ¯ ) can be mathematically shown as follows [ 7 ]:
where M U E s is the total number of served UEs in the whole simulation through the network, and H O P ( i ) is the HOP for UEi .
PPHP : This is an important measure in HO studies since it calculates the number of UHOs made between two adjacent cells. On other words, the PPHP is the UHO that may occur due to sub-optimal HCP settings. The HO will experience the impact of ping pong if UE-i leaves the eNB-A service to the eNB-B target and then returns to the eNB-A service in a period below the critical period ( T c ) . This represents the critical time required for measuring the UHO between neighbouring cells, which is supposed to be 2 s [ 59 ]. Thus, HPPP can be measured when HO occurs, depending on the following equation:
where the interval time represents the difference between T l (the time at which the UE leaves the serving eNB-A) and T r (the time at which the UE returns to the same serving eNB-A). Thus, the HO is recorded as a ping-pong handover (PPH) if the UE returns to the same serving eNB-A and the interval time is below T c . The number of PPHs is recorded for every UE, while the average PPHP through all service UEs is recorded in each simulation period t to increase performance evaluation accuracy. Therefore, the average HPPP ( P P H P ¯ ) for UE through the simulation period t can be represented as follows [ 7 ]:
where M P P H is the total number of PPH throughout the entire system, and the total number of requested HOs represents the sum of failed HOs ( M F ) ,and non- PPH ( M N P P H ) numbers, respectively.
OP : It is defined as the percentage of the area within the cell that does not meet the minimum power (Pmin) requirements. It is the probability that the immediately received SINR (η) level is less than a certain threshold level. The threshold level ( η th) is the minimum SINR level where performance would be unacceptable below it. Thus, the OP for mobile communication systems is mathematically expressed as follows [ 60 , 61 ]:
The OP is recorded when the serving SINR of the UEi is less than a certain threshold level through the simulation cycle t, where the average OP for all UEs is computed through each simulation cycle to increase the accuracy of results. Thus, the average OP ( O P ¯ ) can be simplified from Equation (4), as follows [ 7 ]:
The sub-optimal settings of HCP can be determined statically or estimated automatically, similar to PPHP , with various directions. Generally, the OP occurs in the static state when the settings of HCP are manually selected at extreme levels. The OP occurs in the automatic state if inconvenient HCP settings are automatically estimated through the HPSO algorithm. Either way, the semi-ideal HCP settings usually cause OP to occur if the settings of HCP are at extreme levels. This causes HO lagging, which may later lead to an increase in the OP in some positions, particularly for mobile utilizers who are at the edges of cell or those who move at high mobile velocities. This will consequently cause an increase in network resource wastage, which will reduce the performance of the network. Thus, it is necessary to reduce the OP as much as possible to conserve network resources.
4. The Simulation Scenario and System Model
The optimised HCPs represent the HCPs that are considered to be automatically estimated (optimised) according to a particular case. The suggested algorithm demonstrates the dynamic estimation of HCP settings considered in this work, which includes the HOM values and TTT intervals, respectively. This dramatically contributes to the estimation of the most suitable HCPs for every UE, according to their independent experiences. The negative effect on other UEs, which do not require any changes in HCP settings, can also be avoided. This will reduce the PPHP and OP, respectively, which will lead to tremendous improvements in providing more stable communications throughout UE mobility.
The HOM represents one of the key parameters that is employed to control the HO decision. Low or high HOM settings may lead to high PPHP, high OP or similar problems that are unsatisfactory in wireless systems. Further adjusting the cell’s HOM settings, where all users within the cell will utilise the same HOM, can also create one of these problems. This situation becomes even more important in 5G networks and beyond because of very small coverage from the application of mm waves. Accordingly, there is a needed to the automated mode to separately estimate HOM settings for every utilizer. However, HOM modification is very sensitive and must be implemented with care. In this work, several system scenarios are proposed according to various HCP settings with different mobility speed scenarios to dynamically and separately estimate the incidental HOM settings for every utilizer based on this presumption. The proposed systems contain two parts: the first part is the HOM threshold level, which is defined as the static value, and the second part is the dynamic and continuous amendment for every individual utilizer. Therefore, the total HOM level can be automatically estimated by the sum of the fixed threshold and the adjusted portion, which is mathematically shown, as follows:
where the fixed threshold is the fixed value calculated as an average HOM setting, representing the half difference between the maximum and minimum HOM values ( H max , H min ) . This was assumed to be 10 dB and 0 dB, respectively [ 62 , 63 ]. The M a p is the adjusted part of HOM .
The TTT interval is another important HCP setting, and takes the range defined by 3GPP in [ 64 ]. The set TTT intervals change from 0 to 5.12 s. Nevertheless, the higher or lower TTT intervals may result in high PPHP or high OP, respectively. Therefore, the best solution would be automatic tuning, according to user and network performances. However, adjusting the TTT for all users in the cell throughout the entire system might pose critical problems for some because they have a variety of different experiences. Some utilizers may have good experiences at the boundaries of cell, while others may have bad experiences. Individually adjusting the TTT for each user would be a good solution; however, the adjustment must be carefully conducted. We further suggest adjusting the TTT up or down by increasing or decreasing the TTT threshold with a fixed period.
The simulation model in this work has been developed to simulate a real 5G network. The network has been designed based on the specifications of LTE-Advanced Pro 3GPP Rel. 16, as shown by 3GPP in [ 65 , 66 ], with the presumption that the network environment will be micro cells, Urban areas and 5G Rel. 16 System. Regarding the network deployment design, each hexagonal cell, constructed with a space between sites, has a cell radius R (m) and one eNB located in its centre. Every hexagonal cell contains three sector antennas, which equip every user with an omni-directional antenna to connect to the service network. However, the number of hexagonal cells can be automatically increased according to the simulation time period specified in the simulation. Figure 2 presents an example of the 5G network deployment scenario used in this work with hexagonal cells, each containing three sectors, where the cross shape represents the UE, while the triangular shape represents the eNB, respectively [ 33 ].

Network deployment of various hexagonal cells, each cell containing three sectors.
To represent the environment of the real 5G network, initially, a number of mobile utilizers were created with random coordinates inside the hexagonal boundaries of each cell. The 200 randomly distributed utilizers are generated throughout every hexagonal cell. The utilizers’ number periodically and randomly changed in each cell during the simulation cycles. This is means that the load traffic for every eNB was periodically and automatically changed to represent this real environment. This is taken into account in the simulation model, developed in order to simulate the arbitrary generation of load traffic during the simulation and to fully enable the acceptable control functions in the target cell during user mobility.
The proposed algorithm has been evaluated and validated through the simulations using the 5G network. The average values taken from 15 users represent all results considered in the measurements throughout this work. The 15 users were randomly generated within cell number 1 in the first simulation period. Initially, every user had various random coordinates in the cell. Each utilizer had a varied path that was parallel to another utilizer’s measure, since user mobility is directed in one direction. This means that all utilizers will move parallel to each other user in one direction. The directional mobility model has been suggested for all mobile device users measured across the network that are permitted to move in one direction only. This will increase the accuracy of the results since performance is measured independently for every utilizer in each simulation period. This is accomplished in 5 s through their mobility inside the cells, matching the movement distance with the periodic interval. Then, the average value was taken for all utilizers, which were measured in each simulation cycle. Therefore, the results for the average values of HOP, PPHP and OP were computed in each simulation cycle. This represents the average values of all 15 users, since they move in parallel through various pathways within cells. The measurement procedure has been accomplished to illustrate wireless network performance according to the proposed HPSO algorithm. In this work, it is assumed that the initial values for HOM and TTT for the applied HPO algorithm are 2 dB and 100 ms, respectively. The simulation began according to parameter settings, shown in Table 2 and in the flowchart in Figure 3 .

Flowchart of the proposed simulation model.
The system simulation parameters [ 62 , 67 ].
The required parameters of the network were first defined and then the entire simulation network environment was built, followed by the mobility model. The directions and positions of users were periodically updated throughout the simulation. The Euclidean distances were computed from the eNB in the network within the distance matrix. The path losses tested on the signal were predetermined from this distance matrix, as well as the Rayleigh fading and log-normal shading in multipath scenarios. In the network, every eNB updates the OP and PPHP report throughout the simulation. The eNB also updates the load report and sends the load information to other eNBs in the network.
Usually, the HO decision algorithm is made according to the received signal, represented by reference signal reception strength (RSRS) or signal-to-interference-plus-noise-ratio (SINR), as well as the conditions of loading for both the service and target BSs.
The application of more practical algorithms for the HO decision [ 68 , 69 ] are mathematically provided as follows:
where RSRSs represents the service BSRS, and RSRSt is the target BSRS. The average received signal level was computed from the UE’s perspective across the carrier by every UE and then it moves to the eNB specific to begin the optimisation operation. In addition, the eNB system implements the modulation scheme selection and coding based on the reports of the received signal level [ 70 ], and the process of self-improvement is then implemented. Generally, the service eNB provides the HO decision based on the reports of the measurement and estimation of HCPs after completing the self-optimisation process. This is achieved by implementing the HO action sequence in 3GPP, where contact with the UE will be preserved if the eNB service offers satisfactory signal quality [ 62 , 71 ].
The simulation model has been applied to the developed algorithm to verify its performance in the system. Then, the results of simulation execution were analysed for the developed algorithm, and the dynamic estimation execution of two HCP settings (HOM and TTT) was compared with various mobile speeds. Throughout the study simulation, six different UE mobile speeds were considered with the following values: {40, 60, 80, 100, 120, and 140} km/h. The effect of various mobile speeds on the performance of the network has been evaluated. The mobile speeds represent the speed properties of vehicles in urban and suburban areas; therefore, they are acceptable in theoretical investigations. As part of the analytical framework, Table 1 presents the values of specific 5G parameters defined in the 3GPP specifications (Rel. 16) that have been taken into account in the simulation.
5. Simulation and Performance Evaluation Analysis
This section introduces the combined results of the simulation study and discusses the performance results of the suggested algorithm by comparing the dynamic estimation of two HCPs settings (HOM and TTT). The studied algorithm was examined using six different mobile speed scenarios, HOM levels and TTT intervals to fully illustrate its performance throughout various conditions. This section displays the HO performance for different fixed HCPs to address HPO, based on mobility scenarios. The performance is quantified using the average values calculated across all UEs in the cells over simulation periods with different UE velocities in 5G networks. The effects of various fixed HOM levels and fixed TTT intervals with various UE speeds on system performance have been investigated. The mobility robustness of the 5G network can be verified using different HOM and TTT settings, selected according to 3GPP [ 15 ] with the following values: HOM = {0, 2, 4, 6, 8, and 10} dB and TTT = {0, 320, 640, 1280, 2560, and 5120}. To analyse the performance of the proposed algorithm, simulations were successfully conducted with the consideration of various UE speeds. The suggested algorithm was then compared with various optimisation algorithms, such as fixed HOM values and fixed TTT intervals. The overall simulation time for the suggested algorithm was assessed utilising three MPMs: HOP, PPHP and OP. The simulation results for the probabilities of HOP, PPHP and OP were acquired by comparing the performance between fixed HOM values and TTT intervals using the MATLAB simulation software. The key parameters used in this simulation are shown in Table 1 .
To analyse the performance of the proposed algorithm with fixed HOM values, simulations with various mobile speeds were performed. Figure 4 presents the average HOP performance of the suggested algorithm for different fixed HOM values under various UE velocities. The suggested algorithm dramatically reduces the average HOP for higher HOM values compared to lower values for all velocities. In this figure, the results indicate that the proposed algorithm with higher HOM (i.e., 10 dB) produces lower HOP, which further increases with time. Nevertheless, with mobile velocity scenarios that are equal to or greater than 60 km/h, the suggested algorithm produced HOPs that were capable of rapidly fluctuating with time for all different HOM values. The outcomes indicate that the suggested algorithm with 10 dB HOM offers remarkable reduction gains in the average HO rate for all mobile speed scenarios. When compared to lower values of HOM (i.e., 0 and 2 dB), the overall average HOP obtained when HOM was 10 dB is 88%. This is 80% less than what was obtained when HOM was 0 dB and 2 dB, respectively. Nevertheless, higher or lower HOP is not always considered as a good or bad indicator. The most important performance indicators are PPHP and OP, as discussed in the following sections.

Handover probability versus UE speeds.
Figure 5 displays the impact of HOPP with different HOP values for a specific time. The suggested algorithm for 10 dB HOM obtained lower HOPP rates than other HOMs because of the proper preparation of HCPs and contact with the better eNB target. Nevertheless, the proposed algorithm and other HOM values also acquired low HOPP rates over a specified period, especially in high HOM scenarios where high HOPP rate caused a significant waste of resource mass from the round-trip switching of UE data. The HOPP impact with 10 and 8 dB HOMs at a time of 3.75 s is high for the proposed algorithm compared to other time scenarios. At low speed, the received signals increasingly fluctuate; therefore, the HOPP rate rises. In the middle and high HOMs, the UE connection to the target eNB is faster, resulting in low HOPP rate. The proposed algorithm achieved a significant decrease in the HOPP rate in low HOM scenarios compared to other HOMs. Thus, the proposed algorithm with 10 dB HOM significantly reduces the HOPP rate compared to other HOMs.

Handover ping-pong probability versus time.
Figure 6 presents the average OP rate of the proposed optimisation algorithm for different HOM levels at varied scenarios of mobile speed. The average OP rate for each HOM level is calculated over all scenarios of mobile phone speed and during the whole simulation time. The OP rate is acquired through the optimisation algorithm, which is significantly reduced for the 40 km/h mobile speed, compared to other mobile speed scenarios. Using an inappropriately modified UE speed for optimising HCPs (i.e., an algorithm based on a mobile phone speed of 140 km/h) may result in high OP rates for all HOM levels. Thus, HCPs must be periodically adapted according to each of the UE’s independent experiences. The impact of the Doppler Effect accompanied by weak connections will further increase the OP rate according to the UE speed. However, at the 6 dB HOM level, the proposed algorithm with 140 km/h mobile speed achieved approximately 8%, 12%, 20%, 22% and 30% average OP rates at 120, 100, 80, 60, and 40 km/h mobile speed scenarios, respectively.

UE outage probability versus HOM level.
Figure 7 illustrates the average HOP at various UE speed scenarios for different fixed TTT intervals. The performance of the proposed algorithm was compared with different TTT intervals. The simulation results reveal that the proposed algorithm reduces the average HOP for all scenarios of mobile velocity when the TTT is equal to 5120 ms, as compared to other TTT intervals. The total average HOPs achieved through the proposed algorithm are approximately 50%, 60%, 87%, 95% and 99.5% lower than those achieved by 2560 ms, 1280 ms, 640 ms, 320 ms and 0 ms, respectively, for 100 km/h mobile speed scenario. A long HO delay will lead to increased HOP rate for various mobile speed scenarios, since many packet transmissions are disabled through vertical HO. The proposed algorithm achieves a low HOP due to effective HCP values according to UE velocity; therefore, the HO delay is greatly reduced.

Figure 8 displays the evaluation of the average PPHP rate for the optimisation algorithm, based on different considered values of TTT intervals, as well as the time of the entire simulation. The PPHP rate obtained for the 0 ms TTT interval is relatively higher than other TTT intervals for all simulation time scenarios. This finding is justified since the improper optimisation of HCPs by the MRO algorithm increases PPHP or UHO. High HOP may further lead to an increase PPHP and HOF, while a large decline in HOP will reduce the PPHP rate. The results indicate that in the initial period of operation, PPHPs were low and gradually increased with time. This case is more apparent in the average PPHP over low TTT scenarios, especially for 0 ms TTT. The operation of the network begins based on the HCP settings initially selected; after that, the HCP settings are automatically optimised and updated through the studied algorithm, resulting in various effects on PPHP, which differ according to the reaction and optimisation strength of the algorithm.

In Figure 9 , the outcomes reveal that the average OP recorded throughout all measured utilizers and scenarios of mobile speed are based on the various TTT intervals that have been taken into account. In this figure, the OPs are served as the average rate for all measured utilizers with different TTT interval scenarios. In general, the results demonstrate that the suggested algorithm continuously interacts with time, and OPs are subject to change over time for all scenarios of mobile speed. However, in Figure 6 , the suggested algorithm with a 40 km/h mobile speed presented a remarkable reduction in the OP rate in comparison with other selected speeds for all TTT intervals. The proposed optimisation algorithm with 140 km/h mobile speed scenario basically caused the highest OP rate as the average over all different TTT interval scenarios. The average reduction gains achieved through the proposed optimisation algorithm with 40 km/h mobile speed scenario and TTT were approximately 32%, 29%, 26%, 17% and 16% lower for 140, 120, 100, 80 and 60 km/h mobile speed scenarios, respectively. This represents a significant achievement for the application of the proposed optimisation algorithm.

UE outage probability versus TTT interval.
The simulation results reveal that the performance of the algorithm with fixed HOM values outperforms the performance of the algorithm with fixed TTT intervals throughout all performance metrics with the scenarios of various speed. In addition, the HOP and PPHP behaviours with different HOM and TTT values correspond to the impact of HOM on both parameters. At low TTT intervals, the HOP and HOPP rates increase and raise the level of signals, while at high TTT periods, both rates reduce and decrease the levels of signals. The high velocity of UE maybe cause OP to rise, since the serving BS or the target BS is not servicing the UE.
In general, this study was performed based on a simulation study only. To the best of our knowledge, most handover management studies are conducted based on simulations. Moreover, it has become very difficult to acquire data from the operator regarding mobility management. Most of the data we received are not useful and cannot be used to study handover management. The acquired data do not consider the handover control parameter settings and it is also not clear which handover decision algorithm was used.
6. Conclusions
This paper verified the effect of different HCP settings on 5G network performance by analysing fixed HCP settings in various scenarios to explain the need for applying more advanced technology in 5G networks. The proposed algorithm in this study was used to assess the HCP settings. These optimisation values were estimated according to the speed of UE’s and the loads of cell. Moreover, the suggested algorithm provides liberty to the service network through independently setting HCP values for all UEs; therefore, all UEs acquire varied HCP settings from other UEs. The MRO adjusts HCPs (such as the HOM and TTT) to maintain connection links throughout user mobility with minimum operator interference. The effects of different fixed HOM values and fixed TTT intervals on system performance at various mobile velocities were examined. The proposed algorithm monitors UE speed through UE mobility and then determines the appropriate HOM and TTT values to meet all requirements for successfully implementing the HO process. The proposed system scenarios were evaluated by analysing various HCP settings. A comparison of three MPMs with different mobile speeds was also included: HOP, PPHP and OP. The influence of utilizer mobility on the performance of system was further investigated, where the average experienced HOP was obtained over various HOM and TTT settings across different scenarios of mobile speed and during different time periods in the 5G network. The simulation results show that the performance of the proposed system provides noticeable improvements for OP with the use of lower HCP settings, as compared to higher HCP settings. However, a noticeable drawback can be seen in terms of increased PPHP for different mobile speed scenarios. These results indicate that medium HCP settings may be the best solution when using one of these proposed systems. The simulation results also revealed that the average rates of PPHPs and OP with fixed HOM values are significantly reduced by the proposed algorithm, compared to that of fixed TTT intervals. For further investigation and development, automatic optimisation should be implemented.
In addition, mobile speed has been considered as a maximum of 140 km/hr, to reflect the real environment for vehicle speeds. To the best of our knowledge, and based on our observations with recommendations from professors, the average maximum movement speed for a car is suggested to be around 140 km/h in a study of this type. Moreover, based on the test drive study conducted in urban areas, on suburban highways, and rural areas [ 72 , 73 ] for a project we performed, the recommended movement speed for the car was 60 km/h to facilitate reliable measurements. Furthermore, we are currently working on different mobile speed scenarios that consider train speed and drones, which will be up to 500 km/hr. The results of these new scenarios will be published in the next research paper.
Abbreviations
The following acronyms are used in this manuscript.
Author Contributions
Conceptualization, W.K.S. and I.S.; methodology W.K.S. and I.S.; software, I.S.; validation, W.K.S. and B.J.H.; formal analysis, I.S. and B.J.H.; investigation, W.K.S. and B.J.H.; resources, W.A.J.; writing—original draft preparation, W.K.S. and B.J.H.; writing—review and editing, W.K.S. and B.J.H.; visualization, W.A.J.; supervision, I.S.; project administration, I.S.; funding acquisition, H.M. and Y.I.D. All authors have read and agreed to the published version of the manuscript.
The authors submit sincere thanks and gratitude to the Ministry of Higher Education & Scientific Research, Al-Furat Al-Awsat Technical University (ATU), Engineering Technical College-Najaf in Iraq, for awarding a Postdoctoral Research Fellowship to work as visiting researchers at Istanbul Technical University (ITU) in Turkey. Meanwhile, this research has been produced, benefiting from the 2232 International Fellowship for Outstanding Researchers Program of TÜBİTAK (Project No: 118C276) conducted at Istanbul Technical University (ITU), and it was also supported in part by the Universiti Sains Islam Malaysia (USIM), Malaysia.
Data Availability Statement
Conflicts of interest.
The authors declare no conflict of interest.
Publisher’s Note: MDPI stays neutral with regard to jurisdictional claims in published maps and institutional affiliations.
- In-Building Tech
- Telco Cloud
- 5G NR Release 17
- Private Networks
- Telco AI Deep Dive
- Cloud RAN Deep Dive
- Sponsored Channels:
- Keysight 5G Solutions
- Qualcomm 5G Insights
- 6G Technology & Testing
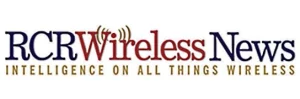
- Advertise/Sponsor
- Editorial Calendar
- 5G Talent Talk
- Wireless Connectivity to Enable Industry 4.0 for the Middleprise
- Well Technically…
- Will 5G Change the World
- Accelerating Industry 4.0 Digitalization
- White Papers
- Analyst Angle
- Network Infrastructure
- Understanding the 5G Advanced and 6G future
- Rohde & Schwarz 6G Technology & Testing
- Telco Cloud & Edge Forum
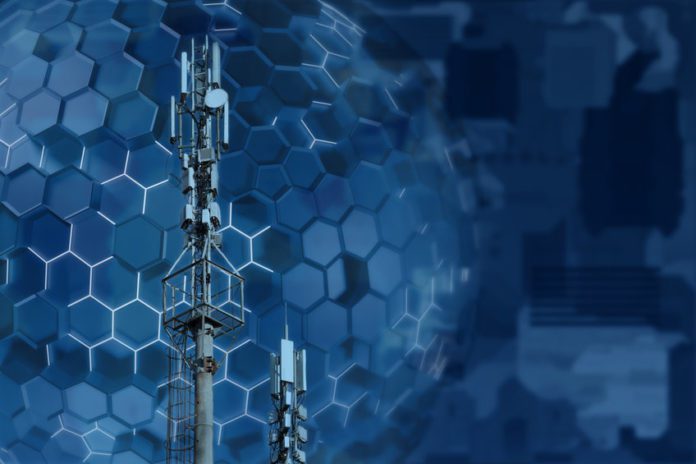
Six operational challenges for 5G

Carriers face a number of operational challenges as 5G continues to evolve and mature, both in terms of deploying and running new 5G networks as well as in managing the relationship of those new networks to existing 4G networks. In a Test and Measurement Forum session , three industry experts laid out some of the primary operational challenges for 5G networks. These include:
– Scale. Dean Brauer, VP of network and field operations for Verizon, said that one of the biggest challenges for network operators is that as 5G is being deployed, the number and types of network nodes are “growing exponentially,” so the ability to both deploy and to manage that network at-scale is crucial.
– Speed of deployment. Not only is 5G being deployed on a broad scale, but it’s being deployed on an aggressive timeline; those represent operational challenges on their own, but they also compound each other. “Service providers really want to deploy a lot of the network very, very fast—so that’s definitely a huge challenge,” says Sophie Legault, director for the transport and datacom businessof test company EXFO.
– Integration . This ties directly to deployment, Brauer notes, in terms of how operators make sure that 5G networks work well with existing 4G networks—which is particularly important because 5G NonStandalone networks depend directly upon LTE as an anchor to carry control plane traffic.
– Testing and support of new 5G technologies. Brauer considers testing to be its own operational challenge in terms of how operators make sure that they can meet the requirements that customers want to see in various 5G services. “We have to be able to measure and monitor the network very well, real-time, at a scale that we haven’t seen before,” he says. Legault frames this in terms of not only new services and related demands, but also new spectrum bands and technological implementations, such as synchronization and high-speed transport.
– Workforce skills. This ties to the new service and technology demands of 5G, but also to the current workforce environment in which 5G is being deployed—a tight labor market, which is also dealing with supply chain issues and where heightened attention and federal/state funding to connectivity services during the global Covid-19 pandemic has meant an influx of dollars that must be spent within a certain time period, so that network operators, system integrators and sub-contractors are competing heatedly for a limited workforce, while also seeking to keep deployment costs down. More is being asked of site technicians: Not just experience with RF testing, but also the ability to test fiber and validate services as well. Sandeep Sharma, VP and global head of Tech Mahindra’s 5G/RAN/ORAN portfolio, also points out that the workforce challenges extend well beyond site deployment. With the increase in virtualization and cloud as well as the emerging role of edge computing in 5G, that changes the set of software skills and familiarity that is needed across the telco industry, Sharma said.
– Network complexity. Sharma went on to add that managing network complexity is one of the most significant operational challenges in 5G. This is compounded by the relatively rapid shift not just from 4G to 5G, but from 5G NonStandalone to Standalone. While the move to 5G SA provides some end-to-end simplification for network management, it doesn’t solve everything—Sharma pointed out that if you look at telco network based on their end-users, the type of 5G devices available, which bands they support, and 5G device penetration still represent issues. There is likely to be a continued lack of continuity across devices, even if there is more continuity across the network. In addition, he said, just as in LTE, the enabling of voice services over the new network (in this case, VoNR) is lagging the support for data services.
Brauer also sees both simplification and increased complexity at work in the move to 5G SA. 5G Standalone, Brauer explains, “will simplify network operation because when you get to a Standalone environment, you no longer have to anchor to your 4G network. So from a performance perspective, and an operational perspective, you get some simplification in how you can operate your network. But the complication comes in because as you introduce 5G, we’re not just introducing a new frequency band … and with Standalone, you can start to slice your network. So the complexity in the operations of the network and the maintenance of the network, and meeting SLAs for various customers goes up exponentially from what we’re used to in our 4G and our 3G networks.”
Legault added, “The network is more dynamic now … and more scalable. So from an operations perspective, you have to be able to understand that and adapt with that change.”
Watch the entirety of this archived session from Test and Measurement Forum, as well as additional forum sessions, on YouTube .
- 5G Fundamentals
- network operations
- Tech Mahindra
ABOUT AUTHOR
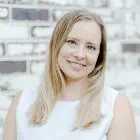
RELATED POSTS
Deals, demos, delays and disappointments in 5g ntn (part 2), radcom extends collaboration with rakuten mobile, o2 telefonica, 5g synergiewerk activate 5g street lights.
Since 1982, RCR Wireless News has been providing wireless and mobile industry news, insights, and analysis to mobile and wireless industry professionals, decision makers, policy makers, analysts and investors.
© 2021-2022 RCR Wireless News
- About RCR Wireless News
- Wireless News Archive
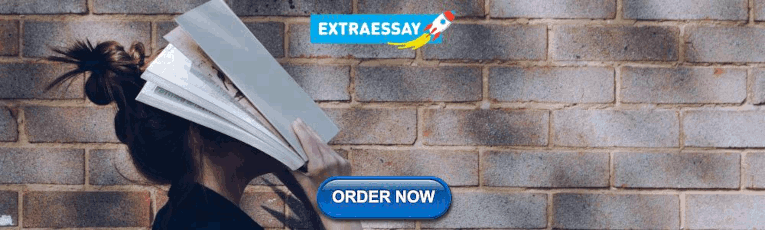
IMAGES
VIDEO
COMMENTS
"This was a major breakthrough," said Hou. "With support from the Commonwealth Cyber Initiative in Southwest Virginia, we elevated network optimization to a whole different level: solving problems in the field in real time." Ultra-high precision for ultra-low latency. In the context of 5G, timing is tied up with the concept of latency.
Published Oct 14, 2023. + Follow. The 5G Method, derived from five Japanese words, offers a structured and holistic way to address and resolve problems. These five "G"s are Gemba, Gembutsu ...
development, examining the proposed development towards 5G and reviewing the industry 'state of the art'. In section III we discuss problem structuring and modelling techniques and their application to our problem. Further, we apply stakeholder mapping and Hierarchical Process Modeling (HPM).
As Cabe Atwell reportsin Fierce Electronics, there are three technologies that can help solve this problem. One is microwave anyhaul, which can deliver data without relying on fiber. Using this technology, air interfaces are strapped to towers and can send radio signals up to 170 GHz over expansive regions. A second potential solution is with ...
Solving 5G's Thorniest Issues. Incomplete roadmaps and continued uncertainty about millimeter-wave technology make this technology's future hazy, but solutions are in the works. August 1st, 2019 - By: Ed Sperling. 5G rollouts are beginning to hit the market, accompanied by a long list of unsolved technical and business issues surrounding ...
Utilizing the "5G Principles" truly took my ability to lead a team during an RCA to another level. The use of 5G in combination with access to a robust root cause analysis tool allowed me to ...
5G Optimization—Future Challenges and Research Issues. 5G key parameters such as improved spectral efficiency, low latency, high data rates, and extended coverage capacity are achieved using the optimization algorithms. There are still some challenges and issues in the implementation of AI-based optimization algorithms that need to be addressed.
Quality of coverage is the key to affect the quality and index of mobile communication, so it is very important to solve the problem of coverage. From the perspective of improving the quality of 5G wireless network, this paper studies the common weak coverage and over coverage problems in 5G wireless network, analyzes the causes, the processing ideas and analysis methods in daily centralized ...
4.6. Optimization Techniques for 5G. Optimization techniques may be applied to capture NP-Complete or NP-Hard problems in 5G technology. This section briefly describes various research works suggested for 5G technology based on optimization techniques. In , Massive MIMO technology is used in 5G mobile network to make it more flexible and ...
Solving for 5G: How Math Modeling Can Improve Modern Communications. by Sponsored Post June 17, 2020. 5G promises exponential increases in data speeds, but questions linger about how to deploy it in urban environments. Editor's note: This commentary, written by Assistant Professor Eric Stachura, is published as part of an annual sponsorship ...
Resource allocation based on artificial intelligence techniques was utilized to resolve issues such as a hybrid problem-solving approach. The main goal behind the study was create a basis on which to select and/or improve a particular technique in the future as a means to allocate the resources of a 5G network.
• The promised speed of 5G is difficult to achieve considering the sub-optimal technological support in many parts of the world. • Many of the old devices that do not support 5G will need to be replaced. 5G could cause unexpected problems for devices relying on the old 3G network. Thus, the transition experience
The added advantage is the 5G approach quashes the division in organizations created by opinions as it is focussed on Data & Facts. Problem solving becomes more structured with revision of ...
5G enables fast, secure, and pervasive connectivity across smart networks and Internet of Things (IoT) devices. When combined with artificial intelligence, 5G can enable unparalleled productivity and efficiency. Apart from the global estimate, five industries are poised to see incremental growth: Industrial Manufacturing, Connected Healthcare ...
Solving the high band 5G handover puzzle. The Ericsson 5G Centralized Neighbor Relations rApp is an automated solution. It enables the user to efficiently search for 4G to 5G cell neighbor candidates in the network and creates the necessary objects for these neighbor relations automatically. It does this by establishing cell-neighbor relations ...
How 5G Changes Communication. With the help of 5G, connections between devices will be faster, substantially more scalable and have much lower latency, albeit not all at the same time. Broadly ...
The problem caused by interference can be solved effectively by a distance protection scheme. ... K Y, and Kz could be extracted by solving the transcendental equation as follows. k x tan (k x a 2) = ... especially in the designing structure of antenna for 5G application. In such techniques, the radiation losses are comparatively lower as in ...
Sharpen your problem-solving skills the McKinsey way, with our weekly crossword. Each puzzle is created with the McKinsey audience in mind, and includes a subtle (and sometimes not-so-subtle) business theme for you to find. Answers that are directionally correct may not cut it if you're looking for a quick win.
News 1 Dec 2021. The 2nd Artificial Intelligence/Machine Learning (AI/ML) in 5G Challenge is set to conclude in December, capping a successful debut last year. Organized by the International Telecommunication Union (ITU) as part of its AI for Good initiative, the competition sees participants around the world solving real-world problems by ...
RF interference impacts the utility of an operator's spectrum investment, and customer experience. He also noted that as channel bandwidths increase, from 5-20 megahertz in 4G to 100-400 megahertz in 5G, the number of resource blocks increases as well. If a resource block is has interference, Lipari explains, a user most likely will not be ...
The skills gap in the wireless industry still exists and is becoming wider with the rollout of 5G. In this episode, Carrie Charles interviews Dr.Rikin Thakker, CTO of The Wireless Infrastructure Association. They discuss workforce challenges and the new solutions and programs that will bring more qualified people into the telecom industry.
The Handover Parameters Optimisation (HPO) is an important function of the self-optimisation network (SON). It was introduced by 3GPP for solving the mobility issues in 4G and 5G mobile phone networks [21,22,23,24]. Several functions are offered by SON, such as mobility robustness optimisation (MRO) and load balancing optimisation (LBO).
-Workforce skills. This ties to the new service and technology demands of 5G, but also to the current workforce environment in which 5G is being deployed—a tight labor market, which is also dealing with supply chain issues and where heightened attention and federal/state funding to connectivity services during the global Covid-19 pandemic has meant an influx of dollars that must be spent ...