Data Security and Privacy: Concepts, Approaches, and Research Directions
Ieee account.
- Change Username/Password
- Update Address
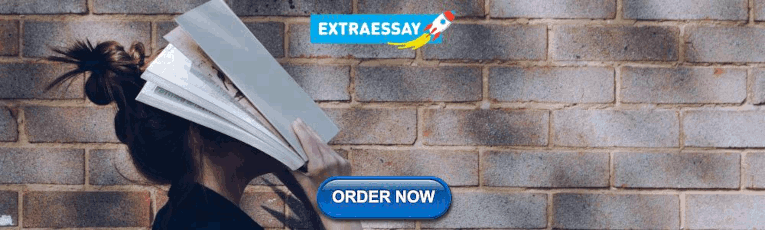
Purchase Details
- Payment Options
- Order History
- View Purchased Documents
Profile Information
- Communications Preferences
- Profession and Education
- Technical Interests
- US & Canada: +1 800 678 4333
- Worldwide: +1 732 981 0060
- Contact & Support
- About IEEE Xplore
- Accessibility
- Terms of Use
- Nondiscrimination Policy
- Privacy & Opting Out of Cookies
A not-for-profit organization, IEEE is the world's largest technical professional organization dedicated to advancing technology for the benefit of humanity. © Copyright 2024 IEEE - All rights reserved. Use of this web site signifies your agreement to the terms and conditions.
- Survey paper
- Open access
- Published: 26 November 2016
Big data privacy: a technological perspective and review
- Priyank Jain ORCID: orcid.org/0000-0001-7988-1338 1 ,
- Manasi Gyanchandani 1 &
- Nilay Khare 1
Journal of Big Data volume 3 , Article number: 25 ( 2016 ) Cite this article
126k Accesses
200 Citations
36 Altmetric
Metrics details
Big data is a term used for very large data sets that have more varied and complex structure. These characteristics usually correlate with additional difficulties in storing, analyzing and applying further procedures or extracting results. Big data analytics is the term used to describe the process of researching massive amounts of complex data in order to reveal hidden patterns or identify secret correlations. However, there is an obvious contradiction between the security and privacy of big data and the widespread use of big data. This paper focuses on privacy and security concerns in big data, differentiates between privacy and security and privacy requirements in big data. This paper covers uses of privacy by taking existing methods such as HybrEx, k-anonymity, T-closeness and L-diversity and its implementation in business. There have been a number of privacy-preserving mechanisms developed for privacy protection at different stages (for example, data generation, data storage, and data processing) of a big data life cycle. The goal of this paper is to provide a major review of the privacy preservation mechanisms in big data and present the challenges for existing mechanisms. This paper also presents recent techniques of privacy preserving in big data like hiding a needle in a haystack, identity based anonymization, differential privacy, privacy-preserving big data publishing and fast anonymization of big data streams. This paper refer privacy and security aspects healthcare in big data. Comparative study between various recent techniques of big data privacy is also done as well.
Big data [ 1 , 2 ] specifically refers to data sets that are so large or complex that traditional data processing applications are not sufficient. It’s the large volume of data—both structured and unstructured—that inundates a business on a day-to-day basis. Due to recent technological development, the amount of data generated by internet, social networking sites, sensor networks, healthcare applications, and many other companies, is drastically increasing day by day. All the enormous measure of data produced from various sources in multiple formats with very high speed [ 3 ] is referred as big data. The term big data [ 4 , 5 ] is defined as “a new generation of technologies and architectures, designed to economically separate value from very large volumes of a wide variety of data, by enabling high-velocity capture, discovery and analysis”. On the premise of this definition, the properties of big data are reflected by 3V’s, which are, volume, velocity and variety. Later studies pointed out that the definition of 3Vs is insufficient to explain the big data we face now. Thus, veracity, validity, value, variability, venue, vocabulary, and vagueness were added to make some complement explanation of big data [ 6 ]. A common theme of big data is that the data are diverse, i.e., they may contain text, audio, image, or video etc. This differing qualities of data is signified by variety. In order to ensure big data privacy, various mechanisms have been developed in recent years. These mechanisms can be grouped based on the stages of big data life cycle [ 7 ] Fig. 1 , i.e., data generation, storage, and processing. In data generation phase, for the protection of privacy, access restriction as well as falsifying data techniques are used. The approaches to privacy protection in data storage phase are chiefly based on encryption procedures. Encryption based techniques can be further divided into Identity Based Encryption (IBE), Attribute Based Encryption (ABE) and storage path encryption. In addition, to protect the sensitive information, hybrid clouds are utilized where sensitive data are stored in private cloud. The data processing phase incorporates Privacy Preserving Data Publishing (PPDP) and knowledge extraction from the data. In PPDP, anonymization techniques such as generalization and suppression are utilized to protect the privacy of data. These mechanisms can be further divided into clustering, classification and association rule mining based techniques. While clustering and classification split the input data into various groups, association rule mining based techniques find the useful relationships and trends in the input data [ 8 ]. To handle diverse measurements of big data in terms of volume, velocity, and variety, there is need to design efficient and effective frameworks to process expansive measure of data arriving at very high speed from various sources. Big data needs to experience multiple phases during its life cycle.
Big data life cycle stages of big data life cycle, i.e., data generation, storage, and processing are shown
As of 2012, 2.5 quintillion bytes of data are created daily. The volumes of data are vast, the generation speed of data is fast and the data/information space is global [ 9 ]. Lightweight incremental algorithms should be considered that are capable of achieving robustness, high accuracy and minimum pre-processing latency. Like, in case of mining, lightweight feature selection method by using Swarm Search and Accelerated PSO can be used in place of the traditional classification methods [ 10 ]. Further ahead, Internet of Things (IoT) would lead to connection of all of the things that people care about in the world due to which much more data would be produced than nowadays [ 11 ]. Indeed, IoT is one of the major driving forces for big data analytics [ 9 ].
In today’s digital world, where lots of information is stored in big data’s, the analysis of the databases can provide the opportunities to solve big problems of society like healthcare and others. Smart energy big data analytics is also a very complex and challenging topic that share many common issues with the generic big data analytics. Smart energy big data involve extensively with physical processes where data intelligence can have a huge impact to the safe operation of the systems in real-time [ 12 ]. This can also be useful for marketing and other commercial companies to grow their business. As the database contains the personal information, it is vulnerable to provide the direct access to researchers and analysts. Since in this case, the privacy of individuals is leaked, it can cause threat and it is also illegal. The paper is based on research not ranging to a specific timeline. As the references suggest, research papers range from as old as 1998 to papers published in 2016. Also, the number of papers that were retrieved from the keyword-based search can be verified from the presence of references based on the keywords. “ Privacy and security concerns ” section discusses of privacy and security concerns in big data and “ Privacy requirements in big data ” section covers the Privacy requirement in big data. “ Big data privacy in data generation phase ”, “ Big data privacy in data storage phase ” and “ Big data privacy preserving in data processing ” sections discusses about big data privacy in data generation, data storage, and data processing Phase. “ Privacy Preserving Methods in Big Data ” section covers the privacy preserving techniques using big data. “ Recent Techniques of Privacy Preserving in Big Data ” section presents some recent techniques of big data privacy and comparative study between these techniques.
Privacy and security concerns in big data
Privacy and security concerns.
Privacy and security in terms of big data is an important issue. Big data security model is not suggested in the event of complex applications due to which it gets disabled by default. However, in its absence, data can always be compromised easily. As such, this section focuses on the privacy and security issues.
Privacy Information privacy is the privilege to have some control over how the personal information is collected and used. Information privacy is the capacity of an individual or group to stop information about themselves from becoming known to people other than those they give the information to. One serious user privacy issue is the identification of personal information during transmission over the Internet [ 13 ].
Security Security is the practice of defending information and information assets through the use of technology, processes and training from:-Unauthorized access, Disclosure, Disruption, Modification, Inspection, Recording, and Destruction.
Privacy vs. security Data privacy is focused on the use and governance of individual data—things like setting up policies in place to ensure that consumers’ personal information is being collected, shared and utilized in appropriate ways. Security concentrates more on protecting data from malicious attacks and the misuse of stolen data for profit [ 14 ]. While security is fundamental for protecting data, it’s not sufficient for addressing privacy. Table 1 focuses on additional difference between privacy and security.
Privacy requirements in big data
Big data analytics draw in various organizations; a hefty portion of them decide not to utilize these services because of the absence of standard security and privacy protection tools. These sections analyse possible strategies to upgrade big data platforms with the help of privacy protection capabilities. The foundations and development strategies of a framework that supports:
The specification of privacy policies managing the access to data stored into target big data platforms,
The generation of productive enforcement monitors for these policies, and
The integration of the generated monitors into the target analytics platforms. Enforcement techniques proposed for traditional DBMSs appear inadequate for the big data context due to the strict execution necessities needed to handle large data volumes, the heterogeneity of the data, and the speed at which data must be analysed.
Businesses and government agencies are generating and continuously collecting large amounts of data. The current increased focus on substantial sums of data will undoubtedly create opportunities and avenues to understand the processing of such data over numerous varying domains. But, the potential of big data come with a price; the users’ privacy is frequently at danger. Ensures conformance to privacy terms and regulations are constrained in current big data analytics and mining practices. Developers should be able to verify that their applications conform to privacy agreements and that sensitive information is kept private regardless of changes in the applications and/or privacy regulations. To address these challenges, identify a need for new contributions in the areas of formal methods and testing procedures. New paradigms for privacy conformance testing to the four areas of the ETL (Extract, Transform, and Load) process as shown in Fig. 2 [ 15 , 16 ].
Big data architecture and testing area new paradigms for privacy conformance testing to the four areas of the ETL (Extract, Transform, and Load) processes are shown here
Pre‐hadoop process validation This step does the representation of the data loading process. At this step, the privacy specifications characterize the sensitive pieces of data that can uniquely identify a user or an entity. Privacy terms can likewise indicate which pieces of data can be stored and for how long. At this step, schema restrictions can take place as well.
Map‐reduce process validation This process changes big data assets to effectively react to a query. Privacy terms can tell the minimum number of returned records required to cover individual values, in addition to constraints on data sharing between various processes.
ETL process validation Similar to step (2), warehousing rationale should be confirmed at this step for compliance with privacy terms. Some data values may be aggregated anonymously or excluded in the warehouse if that indicates high probability of identifying individuals.
Reports testing reports are another form of questions, conceivably with higher visibility and wider audience. Privacy terms that characterize ‘purpose’ are fundamental to check that sensitive data is not reported with the exception of specified uses.
Big data privacy in data generation phase
Data generation can be classified into active data generation and passive data generation. By active data generation, we mean that the data owner will give the data to a third party [ 17 ], while passive data generation refers to the circumstances that the data are produced by data owner’s online actions (e.g., browsing) and the data owner may not know about that the data are being gathered by a third party. Minimization of the risk of privacy violation amid data generation by either restricting the access or by falsifying data.
Access restriction If the data owner thinks that the data may uncover sensitive information which is not supposed to be shared, it refuse to provide such data. If the data owner is giving the data passively, a few measures could be taken to ensure privacy, such as anti-tracking extensions, advertisement or script blockers and encryption tools.
Falsifying data In some circumstances, it is unrealistic to counteract access of sensitive data. In that case, data can be distorted using certain tools prior to the data gotten by some third party. If the data are distorted, the true information cannot be easily revealed. The following techniques are utilized by the data owner to falsify the data:
A tool Socketpuppet is utilized to hide online identity of individual by deception. By utilizing multiple Socketpuppets, the data belonging to one specific individual will be regarded as having a place with various people. In that way the data collector will not have enough knowledge to relate different socketpuppets to one individual.
Certain security tools can be used to mask individual’s identity, such as Mask Me. This is especially useful when the data owner needs to give the credit card details amid online shopping.
Big data privacy in data storage phase
Storing high volume data is not a major challenge due to the advancement in data storage technologies, for example, the boom in cloud computing [ 18 ]. If the big data storage system is compromised, it can be exceptionally destructive as individuals’ personal information can be disclosed [ 19 ]. In distributed environment, an application may need several datasets from various data centres and therefore confront the challenge of privacy protection.
The conventional security mechanisms to protect data can be divided into four categories. They are file level data security schemes, database level data security schemes, media level security schemes and application level encryption schemes [ 20 ]. Responding to the 3V’s nature of the big data analytics, the storage infrastructure ought to be scalable. It should have the ability to be configured dynamically to accommodate various applications. One promising technology to address these requirements is storage virtualization, empowered by the emerging cloud computing paradigm [ 21 ]. Storage virtualization is process in which numerous network storage devices are combined into what gives off an impression of being a single storage device. SecCloud is one of the models for data security in the cloud that jointly considers both of data storage security and computation auditing security in the cloud [ 22 ]. Therefore, there is a limited discussion in case of privacy of data when stored on cloud.
Approaches to privacy preservation storage on cloud
When data are stored on cloud, data security predominantly has three dimensions, confidentiality, integrity and availability [ 23 ]. The first two are directly related to privacy of the data i.e., if data confidentiality or integrity is breached it will have a direct effect on users privacy. Availability of information refers to ensuring that authorized parties are able to access the information when needed. A basic requirement for big data storage system is to protect the privacy of an individual. There are some existing mechanisms to fulfil that requirement. For example, a sender can encrypt his data using pubic key encryption (PKE) in a manner that only the valid recipient can decrypt the data. The approaches to safeguard the privacy of the user when data are stored on the cloud are as follows [ 7 ]:
Attribute based encryption Access control is based on the identity of a user complete access over all resources.
Homomorphic encryption Can be deployed in IBE or ABE scheme settings updating cipher text receiver is possible.
Storage path encryption It secures storage of big data on clouds.
Usage of Hybrid clouds Hybrid cloud is a cloud computing environment which utilizes a blend of on-premises, private cloud and third-party, public cloud services with organization between the two platforms.
Integrity verification of big data storage
At the point when cloud computing is used for big data storage, data owner loses control over data. The outsourced data are at risk as cloud server may not be completely trusted. The data owner should be firmly convinced that the cloud is storing data properly according to the service level contract. To ensure privacy to the cloud user is to provide the system with the mechanism to allow data owner verify that his data stored on the cloud is intact [ 24 , 25 ]. The integrity of data storage in traditional systems can be verified through number of ways i.e., Reed-Solomon code, checksums, trapdoor hash functions, message authentication code (MAC), and digital signatures etc. Therefore data integrity verification is of critical importance. It compares different integrity verification schemes discussed [ 24 , 26 ]. To verify the integrity of the data stored on cloud, straight forward approach is to retrieve all the data from the cloud. To verify the integrity of data without having to retrieve the data from cloud [ 25 , 26 ]. In integrity verification scheme, the cloud server can only provide the substantial evidence of integrity of data when all the data are intact. It is highly prescribed that the integrity verification should be conducted regularly to provide highest level of data protection [ 26 ].
Big data privacy preserving in data processing
Big data processing paradigm categorizes systems into batch, stream, graph, and machine learning processing [ 27 , 28 ]. For privacy protection in data processing part, division can be done into two phases. In the first phase, the goal is to safeguard information from unsolicited disclosure since the collected data might contain sensitive information of the data owner. In the second phase, the aim is to extract meaningful information from the data without violating the privacy.
Privacy preserving methods in big data
Few traditional methods for privacy preserving in big data is described in brief here. These methods being used traditionally provide privacy to a certain amount but their demerits led to the advent of newer methods.
De-identification
De-identification [ 29 , 30 ] is a traditional technique for privacy-preserving data mining, where in order to protect individual privacy, data should be first sanitized with generalization (replacing quasi-identifiers with less particular but semantically consistent values) and suppression (not releasing some values at all) before the release for data mining. Mitigate the threats from re-identification; the concepts of k-anonymity [ 29 , 31 , 32 ], l-diversity [ 30 , 31 , 33 ] and t-closeness [ 29 , 33 ] have been introduced to enhance traditional privacy-preserving data mining. De-identification is a crucial tool in privacy protection, and can be migrated to privacy preserving big data analytics. Nonetheless, as an attacker can possibly get more external information assistance for de-identification in the big data, we have to be aware that big data can also increase the risk of re-identification. As a result, de-identification is not sufficient for protecting big data privacy.
Privacy-preserving big data analytics is still challenging due to either the issues of flexibility along with effectiveness or the de-identification risks.
De-identification is more feasible for privacy-preserving big data analytics if develop efficient privacy-preserving algorithms to help mitigate the risk of re-identification.
There are three -privacy-preserving methods of De-identification, namely, K-anonymity, L-diversity and T-closeness. There are some common terms used in the privacy field of these methods:
Identifier attributes include information that uniquely and directly distinguish individuals such as full name, driver license, social security number.
Quasi - identifier attributes means a set of information, for example, gender, age, date of birth, zip code. That can be combined with other external data in order to re-identify individuals.
Sensitive attributes are private and personal information. Examples include, sickness, salary, etc.
Insensitive attributes are the general and the innocuous information.
Equivalence classes are sets of all records that consists of the same values on the quasi-identifiers.
K-anonymity
A release of data is said to have the k -anonymity [ 29 , 31 ] property if the information for each person contained in the release cannot be perceived from at least k-1 individuals whose information show up in the release. In the context of k -anonymization problems, a database is a table which consists of n rows and m columns, where each row of the table represents a record relating to a particular individual from a populace and the entries in the different rows need not be unique. The values in the different columns are the values of attributes connected with the members of the population. Table 2 is a non-anonymized database comprising of the patient records of some fictitious hospital in Hyderabad.
There are six attributes along with ten records in this data. There are two regular techniques for accomplishing k -anonymity for some value of k .
Suppression In this method, certain values of the attributes are supplanted by an asterisk ‘*’. All or some of the values of a column may be replaced by ‘*’. In the anonymized Table 3 , replaced all the values in the ‘Name’ attribute and each of the values in the ‘Religion’ attribute by a ‘*’.
Generalization In this method, individual values of attributes are replaced with a broader category. For instance, the value ‘19’ of the attribute ‘Age’ may be supplanted by ‘ ≤20’, the value ‘23’ by ‘20 < age ≤ 30’, etc.
Table 3 has 2-anonymity with respect to the attributes ‘Age’, ‘Gender’ and ‘State of domicile’ since for any blend of these attributes found in any row of the table there are always no less than two rows with those exact attributes. The attributes that are available to an adversary are called “quasi-identifiers”. Each “quasi-identifier” tuple occurs in at least k records for a dataset with k-anonymity. K-anonymous data can still be helpless against attacks like unsorted matching attack, temporal attack, and complementary release attack [ 33 , 34 ]. On the positive side, it will present a greedy O(k log k)-approximation algorithm for optimal k-anonymity via suppression of entries. The complexity of rendering relations of private records k-anonymous, while minimizing the amount of information that is not released and simultaneously ensure the anonymity of individuals up to a group of size k, and withhold a minimum amount of information to achieve this privacy level and this optimization problem is NP-hard. In general, a further restriction of the problem where attributes are suppressed instead of individual entries is also NP-hard [ 35 ]. Therefore we move towards L-diversity strategy of data anonymization.
L-diversity
It is a form of group based anonymization that is utilized to safeguard privacy in data sets by reducing the granularity of data representation. This decrease is a trade-off that results outcomes in some loss of viability of data management or mining algorithms for gaining some privacy. The l -diversity model (Distinct, Entropy, Recursive) [ 29 , 31 , 34 ] is an extension of the k -anonymity model which diminishes the granularity of data representation utilizing methods including generalization and suppression in a way that any given record maps onto at least k different records in the data. The l -diversity model handles a few of the weaknesses in the k -anonymity model in which protected identities to the level of k -individuals is not equal to protecting the corresponding sensitive values that were generalized or suppressed, particularly when the sensitive values in a group exhibit homogeneity. The l -diversity model includes the promotion of intra-group diversity for sensitive values in the anonymization mechanism. The problem with this method is that it depends upon the range of sensitive attribute. If want to make data L-diverse though sensitive attribute has not as much as different values, fictitious data to be inserted. This fictitious data will improve the security but may result in problems amid analysis. Also L-diversity method is subject to skewness and similarity attack [ 34 ] and thus can’t prevent attribute disclosure.
T-closeness
It is a further improvement of l -diversity group based anonymization that is used to preserve privacy in data sets by decreasing the granularity of a data representation. This reduction is a trade-off that results in some loss of adequacy of data management or mining algorithms in order to gain some privacy. The t -closeness model(Equal/Hierarchical distance) [ 29 , 33 ] extends the l -diversity model by treating the values of an attribute distinctly by taking into account the distribution of data values for that attribute.
An equivalence class is said to have t -closeness if the distance between the conveyance of a sensitive attribute in this class and the distribution of the attribute in the whole table is less than a threshold t . A table is said to have t -closeness if all equivalence classes have t -closeness. The main advantage of t-closeness is that it intercepts attribute disclosure. The problem lies in t-closeness is that as size and variety of data increases, the odds of re-identification too increases. The brute-force approach that examines each possible partition of the table to find the optimal solution takes \({\text{n}}^{{{\text{O}}({\text{n}})}} {\text{m}}^{{{\text{O}}( 1)}} = 2^{{{\text{O}}({\text{nlogn}})}} {\text{m}}^{{{\text{O}}( 1)}}\) time. We first improve this bound to single exponential in n (Note that it cannot be improved to polynomial unless P = NP) [ 36 ].
Comparative analysis of de-identification privacy methods
Advanced data analytics can extricate valuable information from big data but at the same time it poses a big risk to the users’ privacy [ 32 ]. There have been numerous proposed approaches to preserve privacy before, during, and after analytics process on the big data. This paper discusses three privacy methods such as K-anonymity, L-diversity, and T-closeness. As consumer’s data continues to grow rapidly and technologies are unremittingly improving, the trade-off between privacy breaching and preserving will turn out to be more intense. Table 4 presents existing De-identification preserving privacy measures and its limitations in big data.
Hybrid execution model [ 37 ] is a model for confidentiality and privacy in cloud computing. It executes public clouds only for operations which are safe while integrating an organization’s private cloud, i.e., it utilizes public clouds only for non-sensitive data and computation of an organization classified as public, whereas for an organization’s sensitive, private, data and computation, the model utilizes their private cloud. It considers data sensitivity before a job’s execution. It provides integration with safety.
The four categories in which HybrEx MapReduce enables new kinds of applications that utilize both public and private clouds are as follows-
Map hybrid The map phase is executed in both the public and the private clouds while the reduce phase is executed in only one of the clouds as shown in Fig. 3 a.
HybrEx methods: a map hybrid b vertical partitioning c horizontal partitioning d hybrid. The four categories in which HybrEx MapReduce enables new kinds of applications that utilize both public and private clouds are shown
Vertical partitioning It is shown in Fig. 3 b. Map and reduce tasks are executed in the public cloud using public data as the input, shuffle intermediate data amongst them, and store the result in the public cloud. The same work is done in the private cloud with private data. The jobs are processed in isolation.
Horizontal partitioning The Map phase is executed at public clouds only while the reduce phase is executed at a private cloud as can be seen in Fig. 3 c.
Hybrid As in the figure shown in Fig. 3 d, the map phase and the reduce phase are executed on both public and private clouds. Data transmission among the clouds is also possible.
Integrity check models of full integrity and quick integrity checking are suggested as well. The problem with HybridEx is that it does not deal with the key that is generated at public and private clouds in the map phase and that it deals with only cloud as an adversary.
Privacy-preserving aggregation
Privacy-preserving aggregation [ 38 ] is built on homomorphic encryption used as a popular data collecting technique for event statistics. Given a homomorphic public key encryption algorithm, different sources can use the same public key to encrypt their individual data into cipher texts [ 39 ]. These cipher texts can be aggregated, and the aggregated result can be recovered with the corresponding private key. But, aggregation is purpose-specific. So, privacy- preserving aggregation can protect individual privacy in the phases of big data collecting and storing. Because of its inflexibility, it cannot run complex data mining to exploit new knowledge. As such, privacy-preserving aggregation is insufficient for big data analytics.
Operations over encrypted data
Motivated by searching over encrypted data [ 38 ], operations can be run over encrypted data to protect individual privacy in big data analytics. Since, operations over encrypted data are mostly complex along with being time-consuming and big data is high-volume and needs us to mine new knowledge in a reasonable timeframe, running operations over encrypted data can be termed as inefficient in the case of big data analytics.
Recent techniques of privacy preserving in big data
Differential privacy.
Differential Privacy [ 40 ] is a technology that provides researchers and database analysts a facility to obtain the useful information from the databases that contain personal information of people without revealing the personal identities of the individuals. This is done by introducing a minimum distraction in the information provided by the database system. The distraction introduced is large enough so that they protect the privacy and at the same time small enough so that the information provided to analyst is still useful. Earlier some techniques have been used to protect the privacy, but proved to be unsuccessful.
In mid-90s when the Commonwealth of Massachusetts Group Insurance Commission (GIC) released the anonymous health record of its clients for research to benefit the society [ 32 ]. GIC hides some information like name, street address etc. so as to protect their privacy. Latanya Sweeney (then a PhD student in MIT) using the publicly available voter database and database released by GIC, successfully identified the health record by just comparing and co-relating them. Thus hiding some information cannot assures the protection of individual identity.
Differential Privacy (DP) deals to provide the solution to this problem as shown Fig. 4 . In DP analyst are not provided the direct access to the database containing personal information. An intermediary piece of software is introduced between the database and the analyst to protect the privacy. This intermediary software is also called as the privacy guard.
Differential privacy big data differential privacy (DP) as a solution to privacy-preserving in big data is shown
Step 1 The analyst can make a query to the database through this intermediary privacy guard.
Step 2 The privacy guard takes the query from the analyst and evaluates this query and other earlier queries for the privacy risk. After evaluation of privacy risk.
Step 3 The privacy guard then gets the answer from the database.
Step 4 Add some distortion to it according to the evaluated privacy risk and finally provide it to the analyst.
The amount of distortion added to the pure data is proportional to the evaluated privacy risk. If the privacy risk is low, distortion added is small enough so that it do not affect the quality of answer, but large enough that they protect the individual privacy of database. But if the privacy risk is high then more distortion is added.
Identity based anonymization
These techniques encountered issues when successfully combined anonymization, privacy protection, and big data techniques [ 41 ] to analyse usage data while protecting the identities of users. Intel Human Factors Engineering team wanted to use web page access logs and big data tools to enhance convenience of Intel’s heavily used internal web portal. To protect Intel employees’ privacy, they were required to remove personally identifying information (PII) from the portal’s usage log repository but in a way that did not influence the utilization of big data tools to do analysis or the ability to re-identify a log entry in order to investigate unusual behaviour. Cloud computing is a type of large-scale distributed computing paradigms which has become a driving force for Information and Communications Technology over the past several years, due to its innovative and promising vision. It provides the possibility of improving IT systems management and is changing the way in which hardware and software are designed, purchased, and utilized. Cloud storage service brings significant benefits to data owners, say, (1) reducing cloud users’ burden of storage management and equipment maintenance, (2) avoiding investing a large amount of hardware and software, (3) enabling the data access independent of geographical position, (4) accessing data at any time and from anywhere [ 42 ].
To meet these objectives, Intel created an open architecture for anonymization [ 41 ] that allowed a variety of tools to be utilized for both de-identifying and re-identifying web log records. In the process of implementing architecture, found that enterprise data has properties different from the standard examples in anonymization literature [ 43 ]. This concept showed that big data techniques could yield benefits in the enterprise environment even when working on anonymized data. Intel also found that despite masking obvious Personal Identification Information like usernames and IP addresses, the anonymized data was defenceless against correlation attacks. They explored the trade-offs of correcting these vulnerabilities and found that User Agent (Browser/OS) information strongly correlates to individual users. This is a case study of anonymization implementation in an enterprise, describing requirements, implementation, and experiences encountered when utilizing anonymization to protect privacy in enterprise data analysed using big data techniques. This investigation of the quality of anonymization used k-anonymity based metrics. Intel used Hadoop to analyse the anonymized data and acquire valuable results for the Human Factors analysts [ 44 , 45 ]. At the same time, learned that anonymization needs to be more than simply masking or generalizing certain fields—anonymized datasets need to be carefully analysed to determine whether they are vulnerable to attack.
Privacy preserving Apriori algorithm in MapReduce framework
Hiding a needle in a haystack [ 46 ].
Existing privacy-preserving association rule algorithms modify original transaction data through the noise addition. However, this work maintained the original transaction in the noised transaction in light of the fact that the goal is to prevent data utility deterioration while prevention the privacy violation. Therefore, the possibility that an untrusted cloud service provider infers the real frequent item set remains in the method [ 47 ]. Despite the risk of association rule leakage, provide enough privacy protection because this privacy-preserving algorithm is based on “hiding a needle in a haystack” [ 46 ] concept. This concept is based on the idea that detecting a rare class of data, such as the needles, is hard to find in a haystack, such as a large size of data, as shown in Fig. 5 . Existing techniques [ 48 ] cannot add noise haphazardly because of the need to consider privacy-data utility trade-off. Instead, this technique incurs additional computation cost in adding noise that will make the “haystack” to hide the “needle.” Therefore, ought to consider a trade-off between problems would be easier to resolve with the use of the Hadoop framework in a cloud environment. In Fig. 5 , the dark diamond dots are original association rule and the empty circles are noised association rule. Original rules are hard to be revealed because there are too many noised association rules [ 46 ]
Hiding a needle in a haystack Mechanism of hiding a needle in a haystack is shown
In Fig. 6 , the service provider adds a dummy item as noise to the original transaction data collected by the data provider. Subsequently, a unique code is assigned to the dummy and the original items. The service provider maintains the code information to filter out the dummy item after the extraction of frequent item set by an external cloud platform. Apriori algorithm is performed by the external cloud platform using data which is sent by the service provider. The external cloud platform returns the frequent item set and support value to the service provider. The service provider filters the frequent item set that is affected by the dummy item using a code to extract the correct association rule using frequent item set without the dummy item. The process of extraction association rule is not a burden to the service provider, considering that the amount of calculation required for extracting the association rule is not much.
Overview of the process of association rule mining the service provider adds a dummy item as noise to the original transaction data collected by the data provider
Privacy-preserving big data publishing
The publication and dissemination of raw data are crucial components in commercial, academic, and medical applications with an increasing number of open platforms, such as social networks and mobile devices from which data might be gathered, the volume of such data has also increased over time [ 49 ]. Privacy-preserving models broadly fall into two different settings, which are referred to as input and output privacy. In input privacy, the primary concern is publishing anonymized data with models such as k-anonymity and l-diversity. In output privacy, generally interest is in problems such as association rule hiding and query auditing where the output of different data mining algorithms is perturbed or audited in order to preserve privacy. Much of the work in privacy has been focused on the quality of privacy preservation (vulnerability quantification) and the utility of the published data. The solution is to just divide the data into smaller parts (fragments) and anonymize each part independently [ 50 ].
Despite the fact that k-anonymity can prevent identity attacks, it fails to protect from attribute disclosure attacks because of the lack of diversity in the sensitive attribute within the equivalence class. The l-diversity model mandates that each equivalence class must have at least l well-represented sensitive values. It is common for large data sets to be processed with distributed platforms such as the MapReduce framework [ 51 , 52 ] in order to distribute a costly process among multiple nodes and accomplish considerable performance improvement. Therefore, in order to resolve the inefficiency, improvements of privacy models are introduced.
Trust evaluation plays an important role in trust management. It is a technical approach of representing trust for digital processing, in which the factors influencing trust are evaluated based on evidence data to get a continuous or discrete number, referred to as a trust value. It propose two schemes to preserve privacy in trust evaluation. To reduce the communication and computation costs, propose to introduce two servers to realize the privacy preservation and evaluation result sharing among various requestors. Consider a scenario with two independent service parties that do not collude with each other due to their business incentives. One is an authorized proxy (AP) that is responsible for access control and management of aggregated evidence to enhance the privacy of entities being evaluated. The other is an evaluation party (EP) (e.g., offered by a cloud service provider) that processes the data collected from a number of trust evidence providers. The EP processes the collected data in an encrypted form and produces an encrypted trust pre-evaluation result. When a user requests the pre-evaluation result from EP, the EP first checks the user’s access eligibility with AP. If the check is positive, the AP re-encrypts the pre evaluation result that can be decrypted by the requester (Scheme 1) or there is an additional step involving the EP that prevents the AP from obtaining the plain pre-evaluation result while still allowing decryption of the pre-evaluation result by the requester (Scheme 2) [ 53 ].
Improvement of k-anonymity and l-diversity privacy model
Mapreduce-based anonymization.
For efficient data processing MapReduce framework is proposed. Larger data sets are handled with large and distributed MapReduce like frameworks. The data is split into equal sized chunks which are then fed to separate mapper. The mappers process its chunks and provide pairs as outputs. The pairs having the same key are transferred by the framework to one reducer. The reducer output sets are then used to produce the final result [ 32 , 34 ].
K-anonymity with MapReduce
Since the data is automatically split by the MapReduce framework, the k-anonymization algorithm must be insensitive to data distribution across mappers. Our MapReduce based algorithm is reminiscent of the Mondrian algorithm. For better generality and more importantly, reducing the required iterations, each equivalence class is split into (at most) q equivalence classes in each iteration, rather than only two [ 50 ].
MapReduce-based l-diversity
The extension of the privacy model from k-anonymity to l-diversity requires the integration of sensitive values into either the output keys or values of the mapper. Thus, pairs which are generated by mappers and combiners need to be appropriately modified. Unlike the mapper in k-anonymity, the mapper in l-diversity, receives both quasi-identifiers and the sensitive attribute as input [ 50 ].
Fast anonymization of big data streams
Big data associated with time stamp is called big data stream. Sensor data, call centre records, click streams, and health- care data are examples of big data streams. Quality of service (QoS) parameters such as end-to-end delay, accuracy, and real-time processing are some constraints of big data stream processing. The most pre-requirement of big data stream mining in applications such as health-care is privacy preserving [ 54 ]. One of the common approaches to anonymize static data is k-anonymity. This approach is not directly applicable for the big data streams anonymization. The reasons are as follows [ 55 ]:
Unlike static data, data streams need real-time processing and the existing k-anonymity approaches are NP-hard, as proved.
For the existing static k-anonymization algorithms to reduce information loss, data must be repeatedly scanned during the anonymization procedure. The same process is impossible in data streams processing.
The scales of data streams that need to be anonymized in some applications are increasing tremendously.
Data streams have become so large that anonymizing them is becoming a challenge for existing anonymization algorithms.
To cope with the first and second aforementioned challenges, FADS algorithm was chosen. This algorithm is the best choice for data stream anonymization. But it has two main drawbacks:
The FADS algorithm handles tuples sequentially so is not suitable for big data stream.
Some tuples may remain in the system for quite a while and are discharged when a specified threshold comes to an end.
This work provided three contributions. First, utilizing parallelism to expand the effectiveness of FADS algorithm and make it applicable for big data stream anonymization. Second, proposal of a simple proactive heuristic estimated round-time to prevent publishing of a tuple after its expiration. Third, illustrating (through experimental results) that FAST is more efficient and effective over FADS and other existing algorithm while it noticeably diminishes the information loss and cost metric during anonymization process.
Proactive heuristic
In FADS, a new parameter is considered that represented the maximum delay that is tolerable for an application. This parameter is called expiration-time. To avert a tuple be published when its expiration-time passed, a simple heuristic estimated-round-time is defined. In FADS, there is no check for whether a tuple can remain more in the system or not. As a result, some tuples are published after expiration. This issue is violated the real time condition of a data stream application and also increase cost metric notably.
Privacy and security aspects healthcare in big data
The new wave of digitizing medical records has seen a paradigm shift in the healthcare industry. As a result, healthcare industry is witnessing an increase in sheer volume of data in terms of complexity, diversity and timeliness [ 56 – 58 ]. The term “big data” refers to the agglomeration of large and complex data sets, which exceeds existing computational, storage and communication capabilities of conventional methods or systems. In healthcare, several factors provide the necessary impetus to harness the power of big data [ 59 ]. The harnessing the power of big data analysis and genomic research with real-time access to patient records could allow doctors to make informed decisions on treatments [ 60 ]. Big data will compel insurers to reassess their predictive models. The real-time remote monitoring of vital signs through embedded sensors (attached to patients) allows health care providers to be alerted in case of an anomaly. Healthcare digitization with integrated analytics is one of the next big waves in healthcare Information Technology (IT) with Electronic Health Records (EHRs) being a crucial building block for this vision. With the introduction of HER incentive programs [ 61 ], healthcare organizations recognized EHR’s value proposition to facilitate better access to complete, accurate and sharable healthcare data, that eventually lead to improved patient care. With the ever-changing risk environment and introduction of new emerging threats and vulnerabilities, security violations are expected to grow in the coming years [ 62 ].
Big data presented a comprehensive survey of different tools and techniques used in Pervasive healthcare in a disease-specific manner. It covered the major diseases and disorders that can be quickly detected and treated with the use of technology, such as fatal and non-fatal falls, Parkinson’s disease, cardio-vascular disorders, stress, etc. We have discussed different pervasive healthcare techniques available to address those diseases and many other permanent handicaps, like blindness, motor disabilities, paralysis, etc. Moreover, a plethora of commercially available pervasive healthcare products. It provides understanding of the various aspects of pervasive healthcare with respect to different diseases [ 63 ].
Adoption of big data in healthcare significantly increases security and patient privacy concerns. At the outset, patient information is stored in data centres with varying levels of security. Traditional security solutions cannot be directly applied to large and inherently diverse data sets. With the increase in popularity of healthcare cloud solutions, complexity in securing massive distributed Software as a Service (SaaS) solutions increases with varying data sources and formats. Hence, big data governance is necessary prior to exposing data to analytics.
Data governance
As the healthcare industry moves towards a value-based business model leveraging healthcare analytics, data governance will be the first step in regulating and managing healthcare data.
The goal is to have a common data representation that encompasses industry standards and local and regional standards.
Data generated by BSN is diverse in nature and would require normalization, standardization and governance prior to analysis.
Real-time security analytics
Analysing security risks and predicting threat sources in real-time is of utmost need in the burgeoning healthcare industry.
Healthcare industry is witnessing a deluge of sophisticated attacks ranging from Distributed Denial of Service (DDoS) to stealthy malware.
Healthcare industry leverages on emerging big data technologies to make better-informed decisions, security analytics will be at the core of any design for the cloud based SaaS solution hosting protected health information (PHI) [ 64 ].
Privacy-preserving analytics
Invasion of patient privacy is a growing concern in the domain of big data analytics.
Privacy-preserving encryption schemes that allow running prediction algorithms on encrypted data while protecting the identity of a patient is essential for driving healthcare analytics [ 65 ].
Data quality
Health data is usually collected from different sources with totally different set-ups and database designs which makes the data complex, dirty, with a lot of missing data, and different coding standards for the same fields.
Problematic handwritings are no more applicable in EHR systems, the data collected via these systems are not mainly gathered for analytical purposes and contain many issues—missing data, incorrectness, miscoding—due to clinicians’ workloads, not user friendly user interfaces, and no validity checks by humans [ 66 ].
Data sharing and privacy
The health data contains personal health information (PHI), there will be legal difficulties in accessing the data due to the risk of invading the privacy.
Health data can be anonymized using masking and de-identification techniques, and be disclosed to the researchers based on a legal data sharing agreement [ 67 ].
The data gets anonymized so much with the aim of protecting the privacy, on the other hand it will lose its quality and would not be useful for analysis anymore And coming up with a balance between the privacy-protection elements (anonymization, sharing agreement, and security controls) is essential to be able to access a data that is usable for analytics.
Relying on predictive models
It should not be unrealistic expectations from the constructed data mining models. Every model has an accuracy.
It is important to consider that it would be dangerous to only rely on the predictive models when making critical decisions that directly affects the patient’s life, and this should not even be expected from the predictive model.
Variety of methods and complex math’s
The underlying math of almost all data mining techniques is complex and not very easily understandable for non-technical fellows, thus, clinicians and epidemiologists have usually preferred to continue working with traditional statistics methods.
It is essential for the data analyst to be familiar with the different techniques, and also the different accuracy measurements to apply multiple techniques when analysing a specific dataset.
Summary on recent approaches used in big data privacy
In this section, a summary on recent approaches used in big data privacy is done. Table 5 is presented here comprising of different papers, the methods introduced, their focus and demerits. It presents an overview of the work done till now in the field of big data privacy.
Conclusion and future work
Big data [ 2 , 68 ] is analysed for bits of knowledge that leads to better decisions and strategic moves for overpowering businesses. Yet only a small percentage of data is actually analysed. In this paper, we have investigated the privacy challenges in big data by first identifying big data privacy requirements and then discussing whether existing privacy-preserving techniques are sufficient for big data processing. Privacy challenges in each phase of big data life cycle [ 7 ] are presented along with the advantages and disadvantages of existing privacy-preserving technologies in the context of big data applications. This paper also presents traditional as well as recent techniques of privacy preserving in big data. Hiding a needle in a haystack [ 46 ] is one such example in which privacy preserving is used by association rule mining. Concepts of identity based anonymization [ 41 ] and differential privacy [ 40 ] and comparative study between various recent techniques of big data privacy are also discussed. It presents scalable anonymization methods [ 69 ] within the MapReduce framework. It can be easily scaled up by increasing the number of mappers and reducers. As our future direction, perspectives are needed to achieve effective solutions to the scalability problem [ 70 ] of privacy and security in the era of big data and especially to the problem of reconciling security and privacy models by exploiting the map reduce framework. In terms of healthcare services [ 59 , 64 – 67 ] as well, more efficient privacy techniques need to be developed. Differential privacy is one such sphere which has got much of hidden potential to be utilized further. Also with the rapid development of IoT, there are lots of challenges when IoT and big data come; the quantity of data is big but the quality is low and the data are various from different data sources inherently possessing a great many different types and representation forms, and the data is heterogeneous, as-structured, semi structured, and even entirely unstructured [ 71 ]. This poses new privacy challenges and open research issues. So, different methods of privacy preserving mining may be studied and implemented in future. As such, there exists a huge scope for further research in privacy preserving methods in big data.
Abadi DJ, Carney D, Cetintemel U, Cherniack M, Convey C, Lee S, Stone-braker M, Tatbul N, Zdonik SB. Aurora: a new model and architecture for data stream manag ement. VLDB J. 2003;12(2):120–39.
Article Google Scholar
Kolomvatsos K, Anagnostopoulos C, Hadjiefthymiades S. An efficient time optimized scheme for progressive analytics in big data. Big Data Res. 2015;2(4):155–65.
Big data at the speed of business, [online]. http://www-01.ibm.com/soft-ware/data/bigdata/2012 .
Manyika J, Chui M, Brown B, Bughin J, Dobbs R, Roxburgh C, Byers A. Big data: the next frontier for innovation, competition, and productivity. New York: Mickensy Global Institute; 2011. p. 1–137.
Google Scholar
Gantz J, Reinsel D. Extracting value from chaos. In: Proc on IDC IView. 2011. p. 1–12.
Tsai C-W, Lai C-F, Chao H-C, Vasilakos AV. Big data analytics: a survey. J Big Data Springer Open J. 2015.
Mehmood A, Natgunanathan I, Xiang Y, Hua G, Guo S. Protection of big data privacy. In: IEEE translations and content mining are permitted for academic research. 2016.
Jain P, Pathak N, Tapashetti P, Umesh AS. Privacy preserving processing of data decision tree based on sample selection and singular value decomposition. In: 39th international conference on information assurance and security (lAS). 2013.
Qin Y, et al. When things matter: a survey on data-centric internet of things. J Netw Comp Appl. 2016;64:137–53.
Fong S, Wong R, Vasilakos AV. Accelerated PSO swarm search feature selection for data stream mining big data. In: IEEE transactions on services computing, vol. 9, no. 1. 2016.
Middleton P, Kjeldsen P, Tully J. Forecast: the internet of things, worldwide. Stamford: Gartner; 2013.
Hu J, Vasilakos AV. Energy Big data analytics and security: challenges and opportunities. IEEE Trans Smart Grid. 2016;7(5):2423–36.
Porambage P, et al. The quest for privacy in the internet of things. IEEE Cloud Comp. 2016;3(2):36–45.
Jing Q, et al. Security of the internet of things: perspectives and challenges. Wirel Netw. 2014;20(8):2481–501.
Han J, Ishii M, Makino H. A hadoop performance model for multi-rack clusters. In: IEEE 5th international conference on computer science and information technology (CSIT). 2013. p. 265–74.
Gudipati M, Rao S, Mohan ND, Gajja NK. Big data: testing approach to overcome quality challenges. Data Eng. 2012:23–31.
Xu L, Jiang C, Wang J, Yuan J, Ren Y. Information security in big data: privacy and data mining. IEEE Access. 2014;2:1149–76.
Liu S. Exploring the future of computing. IT Prof. 2011;15(1):2–3.
Sokolova M, Matwin S. Personal privacy protection in time of big data. Berlin: Springer; 2015.
Cheng H, Rong C, Hwang K, Wang W, Li Y. Secure big data storage and sharing scheme for cloud tenants. China Commun. 2015;12(6):106–15.
Mell P, Grance T. The NIST definition of cloud computing. Natl Inst Stand Technol. 2009;53(6):50.
Wei L, Zhu H, Cao Z, Dong X, Jia W, Chen Y, Vasilakos AV. Security and privacy for storage and computation in cloud computing. Inf Sci. 2014;258:371–86.
Xiao Z, Xiao Y. Security and privacy in cloud computing. In: IEEE Trans on communications surveys and tutorials, vol 15, no. 2, 2013. p. 843–59.
Wang C, Wang Q, Ren K, Lou W. Privacy-preserving public auditing for data storage security in cloud computing. In: Proc. of IEEE Int. Conf. on INFOCOM. 2010. p. 1–9.
Liu C, Ranjan R, Zhang X, Yang C, Georgakopoulos D, Chen J. Public auditing for big data storage in cloud computing—a survey. In: Proc. of IEEE Int. Conf. on computational science and engineering. 2013. p. 1128–35.
Liu C, Chen J, Yang LT, Zhang X, Yang C, Ranjan R, Rao K. Authorized public auditing of dynamic big data storage on cloud with efficient verifiable fine-grained updates. In: IEEE trans. on parallel and distributed systems, vol 25, no. 9. 2014. p. 2234–44
Xu K, et al. Privacy-preserving machine learning algorithms for big data systems. In: Distributed computing systems (ICDCS) IEEE 35th international conference; 2015.
Zhang Y, Cao T, Li S, Tian X, Yuan L, Jia H, Vasilakos AV. Parallel processing systems for big data: a survey. In: Proceedings of the IEEE. 2016.
Li N, et al. t-Closeness: privacy beyond k -anonymity and L -diversity. In: Data engineering (ICDE) IEEE 23rd international conference; 2007.
Machanavajjhala A, Gehrke J, Kifer D, Venkitasubramaniam M. L-diversity: privacy beyond k - anonymity. In: Proc. 22nd international conference data engineering (ICDE); 2006. p. 24.
Ton A, Saravanan M. Ericsson research. [Online]. http://www.ericsson.com/research-blog/data-knowledge/big-data-privacy-preservation/2015 .
Samarati P. Protecting respondent’s privacy in microdata release. IEEE Trans Knowl Data Eng. 2001;13(6):1010–27.
Samarati P, Sweeney L. Protecting privacy when disclosing information: k-anonymity and its enforcement through generalization and suppression. Technical Report SRI-CSL-98-04, SRI Computer Science Laboratory; 1998.
Sweeney L. K-anonymity: a model for protecting privacy. Int J Uncertain Fuzz. 2002;10(5):557–70.
Article MathSciNet MATH Google Scholar
Meyerson A, Williams R. On the complexity of optimal k-anonymity. In: Proc. of the ACM Symp. on principles of database systems. 2004.
Bredereck R, Nichterlein A, Niedermeier R, Philip G. The effect of homogeneity on the complexity of k-anonymity. In: FCT; 2011. p. 53–64.
Ko SY, Jeon K, Morales R. The HybrEx model for confidentiality and privacy in cloud computing. In: 3rd USENIX workshop on hot topics in cloud computing, HotCloud’11, Portland; 2011.
Lu R, Zhu H, Liu X, Liu JK, Shao J. Toward efficient and privacy-preserving computing in big data era. IEEE Netw. 2014;28:46–50.
Paillier P. Public-key cryptosystems based on composite degree residuosity classes. In: EUROCRYPT. 1999. p. 223–38.
Microsoft differential privacy for everyone, [online]. 2015. http://download.microsoft.com/…/Differential_Privacy_for_Everyone.pdf .
Sedayao J, Bhardwaj R. Making big data, privacy, and anonymization work together in the enterprise: experiences and issues. Big Data Congress; 2014.
Yong Yu, et al. Cloud data integrity checking with an identity-based auditing mechanism from RSA. Future Gener Comp Syst. 2016;62:85–91.
Oracle Big Data for the Enterprise, 2012. [online]. http://www.oracle.com/ca-en/technoloqies/biq-doto .
Hadoop Tutorials. 2012. https://developer.yahoo.com/hadoop/tutorial .
Fair Scheduler Guide. 2013. http://hadoop.apache.org/docs/r0.20.2/fair_scheduler.html ,
Jung K, Park S, Park S. Hiding a needle in a haystack: privacy preserving Apriori algorithm in MapReduce framework PSBD’14, Shanghai; 2014. p. 11–17.
Ateniese G, Johns RB, Curtmola R, Herring J, Kissner L, Peterson Z, Song D. Provable data possession at untrusted stores. In: Proc. of int. conf. of ACM on computer and communications security. 2007. p. 598–609.
Verma A, Cherkasova L, Campbell RH. Play it again, SimMR!. In: Proc. IEEE Int’l conf. cluster computing (Cluster’11); 2011.
Feng Z, et al. TRAC: Truthful auction for location-aware collaborative sensing in mobile crowd sourcing INFOCOM. Piscataway: IEEE; 2014. p. 1231–39.
HessamZakerdah CC, Aggarwal KB. Privacy-preserving big data publishing. La Jolla: ACM; 2015.
Dean J, Ghemawat S. Map reduce: simplied data processing on large clusters. OSDI; 2004.
Lammel R. Google’s MapReduce programming model-revisited. Sci Comput Progr. 2008;70(1):1–30.
Yan Z, et al. Two schemes of privacy-preserving trust evaluation. Future Gener Comp Syst. 2016;62:175–89.
Zhang Y, Fong S, Fiaidhi S, Mohammed S. Real-time clinical decision support system with data stream mining. J Biomed Biotechnol. 2012;2012:8.
Mohammadian E, Noferesti M, Jalili R. FAST: fast anonymization of big data streams. In: ACM proceedings of the 2014 international conference on big data science and computing, article 1. 2014.
Haferlach T, Kohlmann A, Wieczorek L, Basso G, Kronnie GT, Bene M-C, De Vos J, Hernandez JM, Hofmann W-K, Mills KI, Gilkes A, Chiaretti S, Shurtleff SA, Kipps TJ, Rassenti LZ, Yeoh AE, Papenhausen PR, Liu WM, Williams PM, Fo R. Clinical utility of microarray-based gene expression profiling in the diagnosis and sub classification of leukemia: report from the international microarray innovations in leukemia study group. J Clin Oncol. 2010;28(15):2529–37.
Salazar R, Roepman P, Capella G, Moreno V, Simon I, Dreezen C, Lopez-Doriga A, Santos C, Marijnen C, Westerga J, Bruin S, Kerr D, Kuppen P, van de Velde C, Morreau H, Van Velthuysen L, Glas AM, Tollenaar R. Gene expression signature to improve prognosis prediction of stage II and III colorectal cancer. J Clin Oncol. 2011;29(1):17–24.
Golub TR, Slonim DK, Tamayo P, Huard C, Gaasenbeek M, Mesirov JP, Coller H, Loh ML, Downing JR, Caligiuri MA, Bloomfield CD, Lander ES. Molecular classification of cancer: class discovery and class prediction by gene expression monitoring. Science. 1999;286(5439):531–7.
Groves P, Kayyali B, Knott D, Kuiken SV. The ‘big data’ revolution in healthcare. New York: McKinsey & Company; 2013.
Public Law 111–148—Patient Protection and Affordable Care Act. U.S. Government Printing Office (GPO); 2013.
EHR incentive programs. 2014. [Online]. https://www.cms.gov/Regulations-and Guidance/Legislation/EHRIncentivePrograms/index.html.
First things first—highmark makes healthcare-fraud prevention top priority with SAS. SAS; 2006.
Acampora G, et al. Data analytics for pervasive health. In: Healthcare data analytics. ISSN:533-576. 2015.
Haselton MG, Nettle D, Andrews PW. The evolution of cognitive bias. In: The handbook of evolutionary psychology. Hoboken: Wiley; 2005. p. 724–46.
Hill K. How target figured out a teen girl was pregnant before her father did. New York: Forbes, Inc.; 2012. [Online]. http://www.forbes.com/sites/kashmirhill/2012/02/16/howtarget - figured-out-a-teen-girl-was-pregnant-before-herfather- did/.
Violán C, Foguet-Boreu Q, Hermosilla-Pérez E, Valderas JM, Bolíbar B, Fàbregas-Escurriola M, Brugulat-Guiteras P, Muñoz-Pérez MÁ. Comparison of the information provided by electronic health records data and a population health survey to estimate prevalence of selected health conditions and multi morbidity. BMC Public Health. 2013;13(1):251.
Emam KE. Guide to the de-identification of personal health information. Boca Raton: CRC Press; 2013.
Book Google Scholar
Wu X. Data mining with big data. IEEE Trans Knowl Data Eng. 2014;26(1):97–107.
Zhang X, Yang T, Liu C, Chen J. A scalable two-phase top-down specialization approach for data anonymization using systems, in MapReduce on cloud. IEEE Trans Parallel Distrib. 2014;25(2):363–73.
Zhang X, Dou W, Pei J, Nepal S, Yang C, Liu C, Chen J. Proximity-aware local-recoding anonymization with MapReduce for scalable big data privacy preservation in cloud. In: IEEE transactions on computers, vol. 64, no. 8, 2015.
Chen F, et al. Data mining for the internet of things: literature review and challenges. Int J Distrib Sens Netw. 2015;501:431047.
Fei H, et al. Robust cyber-physical systems: concept, models, and implementation. Future Gener Comp Syst. 2016;56:449–75.
Sweeney L. k-anonymity: a model for protecting privacy. Int J Uncertain Fuzziness Knowl Based Syst. 2002;10(5):557–70.
Dou W, et al. Hiresome-II: towards privacy-aware cross-cloud service composition for big data applications. IEEE Trans Parallel Distrib Syst. 2014;26(2):455–66.
Liang K, Susilo W, Liu JK. Privacy-preserving ciphertext for big data storage. In: IEEE transactions on informatics and forensics security. vol 10, no. 8. 2015.
Xu K, Yue H, Guo Y, Fang Y. Privacy-preserving machine learning algorithms for big data systems. In: IEEE 35th international conference on distributed systems. 2015.
Yan Z, Ding W, Xixun Yu, Zhu H, Deng RH. Deduplication on encrypted big data in cloud. IEEE Trans Big Data. 2016;2(2):138–50.
Download references
Authors’ contributions
PJ performed the primary literature review and analysis for this manuscript work. MG worked with PJ to develop the article framework and focus, and MG also drafted the manuscript. NK introduced this topic to PJ. MG and NK revised the manuscript for important intellectual content and have given final approval of the version to be published. All authors read and approved the final manuscript.
Competing interests
The authors declare that they have no competing interests.
Author information
Authors and affiliations.
Computer Science Department, MANIT, Bhopal, India
Priyank Jain, Manasi Gyanchandani & Nilay Khare
You can also search for this author in PubMed Google Scholar
Corresponding author
Correspondence to Priyank Jain .
Rights and permissions
Open Access This article is distributed under the terms of the Creative Commons Attribution 4.0 International License ( http://creativecommons.org/licenses/by/4.0/ ), which permits unrestricted use, distribution, and reproduction in any medium, provided you give appropriate credit to the original author(s) and the source, provide a link to the Creative Commons license, and indicate if changes were made.
Reprints and permissions
About this article
Cite this article.
Jain, P., Gyanchandani, M. & Khare, N. Big data privacy: a technological perspective and review. J Big Data 3 , 25 (2016). https://doi.org/10.1186/s40537-016-0059-y
Download citation
Received : 27 July 2016
Accepted : 05 November 2016
Published : 26 November 2016
DOI : https://doi.org/10.1186/s40537-016-0059-y
Share this article
Anyone you share the following link with will be able to read this content:
Sorry, a shareable link is not currently available for this article.
Provided by the Springer Nature SharedIt content-sharing initiative
- Privacy and security
- Privacy preserving: k-anonymity: T-closeness, L-diversity
Editorial: Introduction to Data Security and Privacy
- Open access
- Published: 30 September 2016
- Volume 1 , pages 125–126, ( 2016 )
Cite this article
You have full access to this open access article
- Elisa Bertino 1
6365 Accesses
7 Citations
Explore all metrics
Avoid common mistakes on your manuscript.
Issues around data security [ 1 ], trustworthiness [ 2 ], and privacy [ 3 ] are today under greater focus than ever before. Technological advances, such as sensors, smart mobile devices, Internet of things (IoTs), and novel systems, and applications, such as cloud systems, cyber–physical systems, social networks, and smart and connected health care, are making possible to capture, process, share, and use huge amounts of data from everywhere and at every time, and to extract knowledge and predict trends from these data [ 3 ]. The widespread and intensive use of data for many different tasks makes, however, data security, trustworthiness, and privacy increasingly critical requirements. For example, the availability of multiple datasets, which can be easily combined and analyzed, makes it very easy to infer sensitive information. Such issue may make data sharing more difficult, if at all possible. Pervasive data gathering from multiple devices, such as smart phones, smart power meters, and personal well-being devices, further exacerbates the problem of data security and privacy. The use of cloud as a platform for storing, retrieving, and processing data introduces another party in the already complex data ecosystem. Malicious actors may compromise cloud systems and cloud applications in order to gain access to private data as well as remove or tamper the data, so to undermine the trust of users toward the data.
Research has been very active in designing techniques for data protection over the past 20 years. As a result, many such techniques have been developed ranging from encryption techniques supporting privacy-preserving searches over encrypted data [ 4 ] and access control systems supporting the specification and enforcement of access control policies for data sharing [ 5 ], to techniques for trustworthiness assessment of data [ 6 ] and integrity techniques for complex data [ 7 ]. However, despite such large number of research efforts, the problem of data protection in the era of big data and IoT [ 8 ] is challenging. We need to develop novel access control models tailored to no-SQL data management systems. Also we need approaches to merge heterogeneous data access control policies when dealing with data originating from multiple sources—a common situation in many big data applications. We need efficient privacy-preserving protocols to assure the confidentiality of data stored in the cloud. In this respect, it is important to notice that protocols have to be developed that are tailored to specific usage of data. Data trustworthiness is also an area where extensive research is needed. We need solutions for the many different contexts and platforms involved in collecting, managing, and delivering data, such as sensor networks and cloud.
This issue of the journal is devoted to recent advances in data security, trustworthiness, and privacy that address relevant challenges. The papers, all invited, provide a broad perspective about the variety of researches that can contribute to the development of effective and efficient data protection technology. P. Colombo and E. Ferrari in “Fine-grained Access Control within NoSQL Document-Oriented Datastores” present an overview of the many challenges related to the design of fine-grained access control models for relational database systems that do not use SQL. The development of such models is critical as today there are several data management systems that for performance reason do not use SQL. This paper is an excellent starting point for everyone interested in advances in access control models. F. Akeel, A. S. Fathabadi, F. Paci, A. Gravell, and G. Wills in “Formal Modelling of Data Integration Systems Security Policies” address the challenging problem of assuring data confidentiality, privacy, and trust in the context of data integration systems. The paper, after providing a comprehensive set of system requirements toward addressing such problem, presents formal methods for the verification of security policies specified for the integrated data. This paper is an excellent reference for anyone interested in exploring data security in the context of data integration systems. J. Kim and S. Nepal in “Cryptographically Enforced Access Control with a Flexible User Revocation on Untrusted Cloud Storage” focus on the challenging problem of revoking user authorizations for access to encrypted data stored in the cloud. Extensive experimental results reported in the paper show that their approach is efficient. S. Badsha, X. Yi, and I. Khalil in “A Practical Privacy-Preserving Recommender System” show a cryptographic approach by which one can build recommender systems that preserve the privacy of data used for deriving the recommendations. J. Wang and X. Chen in “Efficient and Secure Storage for Outsourced Data: A Survey” also focus on security for data stored in the cloud. Their paper, however, focuses on the challenging issue of data integrity. The paper presents a comprehensive survey of key integrity techniques designed specifically for data outsourcing platforms and also discusses integrity techniques in the context of data deduplication—a technique widely used to reduce storage costs when outsourcing data. Finally, C. Wang, W. Zheng, and E. Bertino in “Provenance for Wireless Sensor Networks: A Survey” provide a comprehensive discussion on state-of-the-art data provenance techniques. Such techniques are a critical factor for assessing data trustworthiness in unprotected and large-scale distributed systems of small devices, such as sensors and IoT devices. Future issues of DSE will include additional invited papers and special issues focusing on novel challenging research topics concerning data security, trustworthiness, and privacy.
I hope you will enjoy this issue and find interesting research results and directions from the papers in the issue.
Bertino E (2013) Data security—challenges and research opportunities. Secure data management—10th VLDB workshop, SDM 2013, Trento, Italy, August 30, 2013, proceedings. LNCS 8425
Bertino E (2014) Data trustworthiness—approaches and research challenges. Data privacy management, autonomous spontaneous security, and security assurance—9th international workshop, DPM 2014, 7th international workshop, SETOP 2014, and 3rd international workshop, QASA 2014, Wroclaw, Poland, September 10–11, 2014. Revised selected papers
Bertino E (2015) Big data—security and privacy. 2015 IEEE international congress on big data, New York City, NY, USA, June 27–July 2, 2015
Yi X, Paulet R, Bertino E (2014) Homomorphic encryption and applications. Springer briefs in computer science. Springer, pp 1–126. ISBN 978-3-319-12228-1
Bertino E, Ghinita G, Kamra A (2011) Access control for databases: concepts and systems. Found Trends Databases 3(1–2):1–148
MATH Google Scholar
Rezvani M, Ignjatovic A, Bertino E, Jha S (2015) Secure data aggregation technique for wireless sensor networks in the presence of collusion attacks. IEEE Trans Dependable Secure Comput 12(1):98–110
Article Google Scholar
Kundu A, Bertino E (2008) Structural signatures for tree data structures. In: Proceedings of the 34th international conference on very large databases (VLDB’08), Auckland, New Zealand, August 23–28, 2008 (also in PVLDB 1(1):138–150)
Bertino E (2016) Data security and privacy in the IoT, summary of EDBT 2016 keynote talk. In: Proceedings of the 19th international conference on extending database technology, EDBT 2016, Bordeaux, France, March 15–16, 2016, Bordeaux, France, March 15–16, 2016
Download references
Author information
Authors and affiliations.
Purdue University, West Lafayette, IN, USA
Elisa Bertino
You can also search for this author in PubMed Google Scholar
Corresponding author
Correspondence to Elisa Bertino .
Rights and permissions
Open Access This article is distributed under the terms of the Creative Commons Attribution 4.0 International License ( http://creativecommons.org/licenses/by/4.0/ ), which permits unrestricted use, distribution, and reproduction in any medium, provided you give appropriate credit to the original author(s) and the source, provide a link to the Creative Commons license, and indicate if changes were made.
Reprints and permissions
About this article
Bertino, E. Editorial: Introduction to Data Security and Privacy . Data Sci. Eng. 1 , 125–126 (2016). https://doi.org/10.1007/s41019-016-0021-1
Download citation
Received : 12 September 2016
Accepted : 14 September 2016
Published : 30 September 2016
Issue Date : September 2016
DOI : https://doi.org/10.1007/s41019-016-0021-1
Share this article
Anyone you share the following link with will be able to read this content:
Sorry, a shareable link is not currently available for this article.
Provided by the Springer Nature SharedIt content-sharing initiative
- Access Control
- Recommender System
- Data Security
- Access Control Policy
- Access Control Model
- Find a journal
- Publish with us
- Track your research
- Follow us on Facebook
- Follow us on Twitter
- Criminal Justice
- Environment
- Politics & Government
- Race & Gender
Expert Commentary
Data security: Research on privacy in the digital age
Research on consumer attitudes toward digital privacy and the practices of tech companies that shape data collection and use policies.
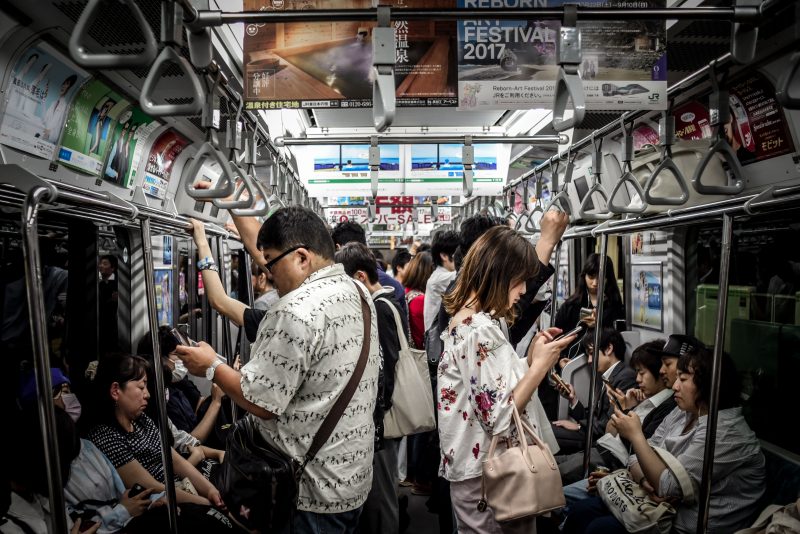
Republish this article

This work is licensed under a Creative Commons Attribution-NoDerivatives 4.0 International License .
by Chloe Reichel, The Journalist's Resource April 12, 2018
This <a target="_blank" href="https://journalistsresource.org/economics/data-digital-privacy-cambridge-analytica/">article</a> first appeared on <a target="_blank" href="https://journalistsresource.org">The Journalist's Resource</a> and is republished here under a Creative Commons license.<img src="https://journalistsresource.org/wp-content/uploads/2020/11/cropped-jr-favicon-150x150.png" style="width:1em;height:1em;margin-left:10px;">
On your smartphone, you’re not much more than a data machine, generating reams of valuable information that tech companies can mine for insights, sell to advertisers and use to optimize their products.
The Cambridge Analytica scandal, which involves a third-party Facebook app that harvested data well beyond the scope of the 270,000 users who initially consented to its terms of service for use in political campaigns (including Donald Trump’s 2016 bid for the presidency), highlights anew the vulnerability of consumer data in this digital age.
But it’s easy to forget these risks to personal privacy and security while tapping out messages to friends or scrolling endlessly through the web. The distraction machines at our fingertips ask for access and we give it up quickly, hastily agreeing to unread privacy policies and terms of service in exchange for a fresh jolt of content.
Studies highlight this “digital privacy paradox,” in which people express concerns over their privacy but then act in ways that undermine these beliefs , for example, offering up personal data for a small incentive. This review features research on this topic — consumer attitudes toward digital privacy — as well as studies of the supply-side — that is, research on the practices of app developers and other tech companies that shape data collection and use policies.
“ Artificial Intelligence and Consumer Privacy ” Jin, Ginger Zhe. National Bureau of Economic Research working paper, 2018. DOI: 10.3386/w24253.
Summary: This paper looks at the risks big data poses to consumer privacy. The author describes the causes and consequences of data breaches and the ways in which technological tools can be used for data misuse. She then explores the interaction between privacy risks and the U.S. market. For example, the author highlights the “self-conflicting” views consumers hold about their privacy, citing literature in which consumers give away personal data for small incentives despite attitudes that might indicate otherwise. On the supply side, similar paradoxes exist — for example, despite an awareness of cyber risks, firms “tend to deploy new technology… before adopting security measures to protect them.” The author discusses how market forces might motivate firms to strengthen privacy settings in response to consumer concerns, but also mentions how market mechanisms can have the opposite effect, using the example of password policies and consumers’ demand for convenience (in the form of weaker password requirements). The author then describes how artificial intelligence might be used to mitigate data security and privacy risks. Lastly, she provides an overview of U.S. policy on consumer privacy and data security and describes future challenges in the field.
“ The Digital Privacy Paradox: Small Money, Small Costs, Small Talk ” Athey, Susan; Catalini, Christian; Tucker, Catherine. National Bureau of Economic Research working paper, 2017. DOI: 10.3386/w23488.
Abstract: “‘Notice and Choice’ has been a mainstay of policies designed to safeguard consumer privacy. This paper investigates distortions in consumer behavior when faced with notice and choice which may limit the ability of consumers to safeguard their privacy using field experiment data from the MIT digital currency experiment. There are three findings. First, the effect small incentives have on disclosure may explain the privacy paradox: Whereas people say they care about privacy, they are willing to relinquish private data quite easily when incentivized to do so. Second, small navigation costs have a tangible effect on how privacy-protective consumers’ choices are, often in sharp contrast with individual stated preferences about privacy. Third, the introduction of irrelevant, but reassuring information about privacy protection makes consumers less likely to avoid surveillance, regardless of their stated preferences towards privacy.”
“ Mobile Applications and Access to Private Data: The Supply Side of the Android Ecosystem ” Kesler, Reinhold; Kummer, Michael E.; Schulte, Patrick. SSRN Electronic Journal , 2017. DOI: 10.2139/ssrn.3106571.
Summary: This paper looks at strategies mobile app developers use to collect data, which apps are most likely to practice intrusive data collection, and what factors predict problematic personal data usage. By examining the variations in data collection strategies of different apps created by the same developers over a period of four years, the researchers uncover three trends. 1) With time and experience, developers adopt more intrusive data collection tactics. 2) Apps with intrusive data collection strategies most commonly target adolescents. 3) Apps that request “critical and atypical permissions” (i.e., access to various data sources) are linked with an increased risk of problematic data practices later on.
“ Consumer Privacy Choice in Online Advertising: Who Opts Out and at What Cost to Industry? ” Johnson, Garrett A.; Shriver, Scott; Du, Shaoyin. SSRN Electronic Journal , 2017. DOI: 10.2139/ssrn.3020503.
Abstract: “We study consumer privacy choice in the context of online display advertising, where advertisers track consumers’ browsing to improve ad targeting. In 2010, the American ad industry implemented a self-regulation mechanism that overlaid ‘AdChoices’ icons on ads, which consumers could click to opt out of online behavioral advertising. We examine the real-world uptake of AdChoices using transaction data from an ad exchange. Though consumers express strong privacy concerns in surveys, we find that only 0.23 percent of American ad impressions arise from users who opted out of online behavioral advertising. We also find that opt-out user ads fetch 59.2 percent less revenue on the exchange than do comparable ads for users who allow behavioral targeting. These findings are broadly consistent with evidence from the European Union and Canada, where industry subsequently implemented the AdChoices program. We calculate an upper bound for the industry’s value of the average opt-out user’s browsing information to be $8 per capita annually in the US. We find that opt-out users tend to be more technologically sophisticated, though opt-out rates are higher in American states with lower income. These results inform the privacy policy discussion by illuminating the real-world consequences of an opt-out privacy mechanism.”
“ The Economics of Privacy ” Acquisti, Alessandro; Taylor, Curtis R.; Wagman, Liad. Journal of Economic Literature , 2016. DOI: 10.2139/ssrn.2580411.
Abstract: “This article summarizes and draws connections among diverse streams of theoretical and empirical research on the economics of privacy. We focus on the economic value and consequences of protecting and disclosing personal information, and on consumers’ understanding and decisions regarding the trade-offs associated with the privacy and the sharing of personal data. We highlight how the economic analysis of privacy evolved over time, as advancements in information technology raised increasingly nuanced and complex issues associated with the protection and sharing of personal information. We find and highlight three themes that connect diverse insights from the literature. First, characterizing a single unifying economic theory of privacy is hard, because privacy issues of economic relevance arise in widely diverse contexts. Second, there are theoretical and empirical situations where the protection of privacy can both enhance, and detract from, individual and societal welfare. Third, in digital economies, consumers’ ability to make informed decisions about their privacy is severely hindered, because consumers are often in a position of imperfect or asymmetric information regarding when their data is collected, for what purposes, and with what consequences. We conclude the article by highlighting some of the ongoing issues in the privacy debate of interest to economists.”
About The Author
Chloe Reichel
Read our research on: Abortion | Podcasts | Election 2024
Regions & Countries
How americans view data privacy, the role of technology companies, ai and regulation – plus personal experiences with data breaches, passwords, cybersecurity and privacy policies.
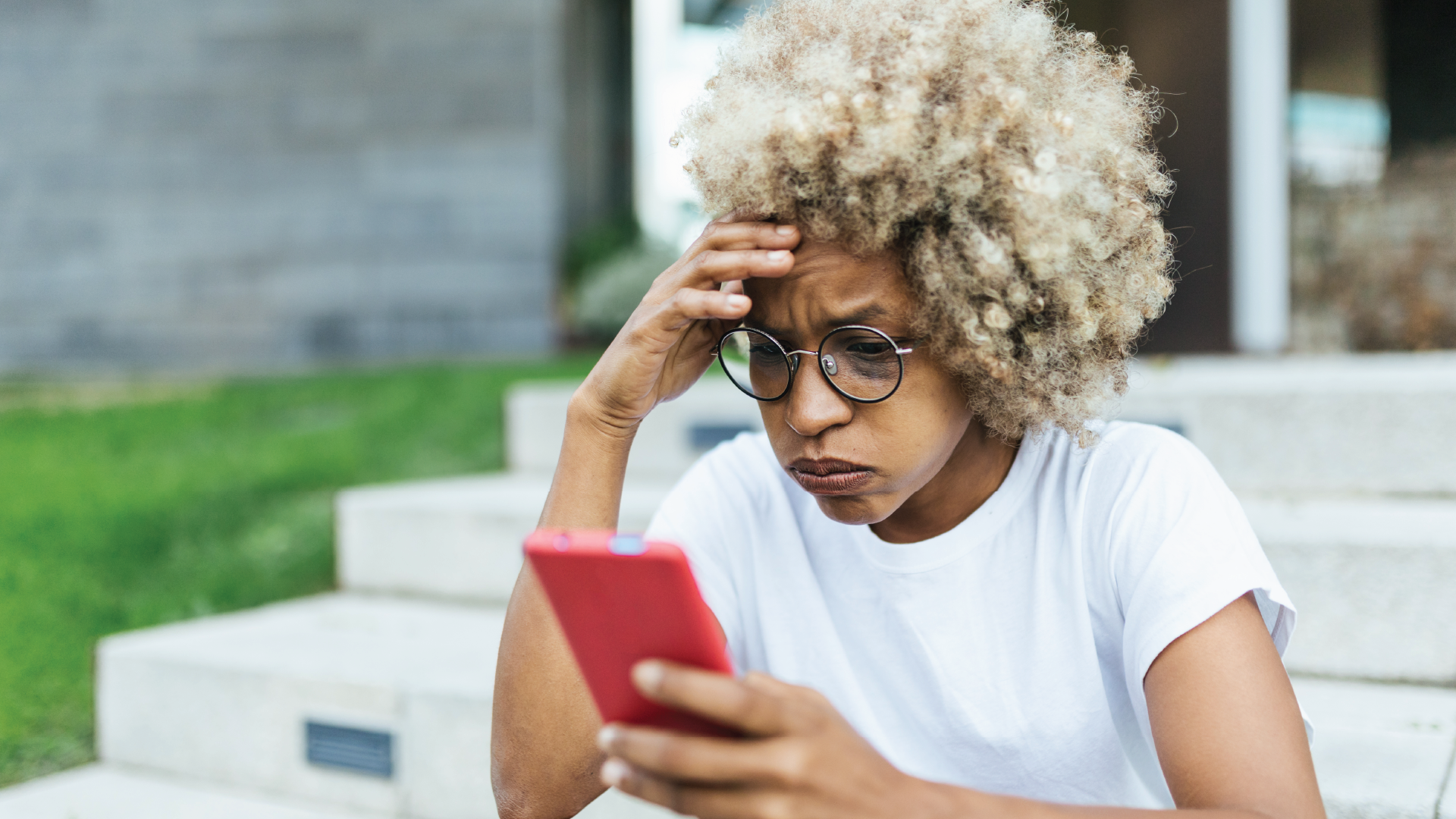
Pew Research Center has a long record of studying Americans’ views of privacy and their personal data, as well as their online habits. This study sought to understand how people think about each of these things – and what, if anything, they do to manage their privacy online.
This survey was conducted among 5,101 U.S. adults from May 15 to 21, 2023. Everyone who took part in the survey is a member of the Center’s American Trends Panel (ATP), an online survey panel that is recruited through national, random sampling of residential addresses. This way nearly all U.S. adults have a chance of selection. The survey is weighted to be representative of the U.S. adult population by gender, race and ethnicity, partisan affiliation, education and other categories. Read more about the ATP’s methodology .
Here are the questions used for this analysis , along with responses, and its methodology .
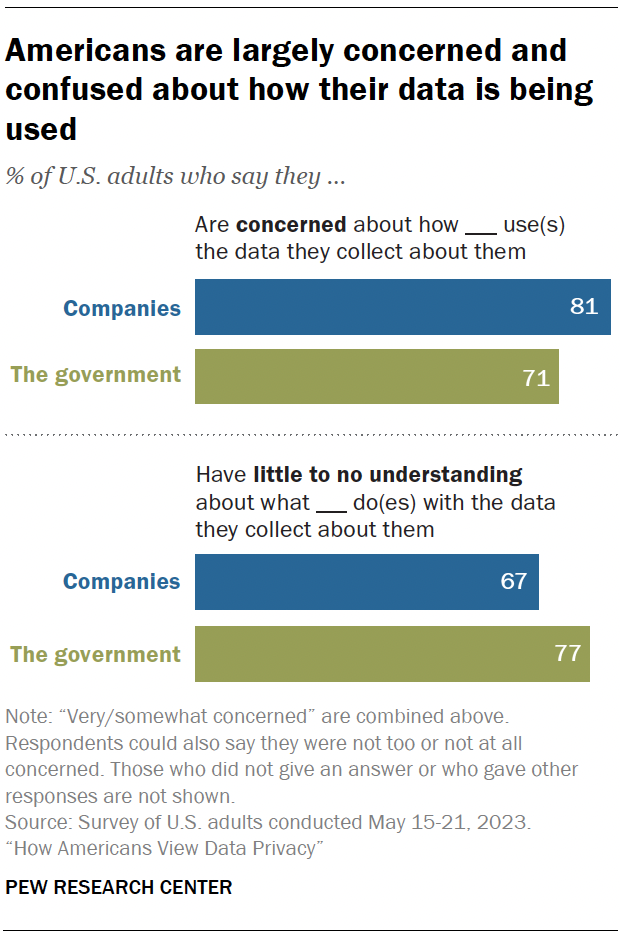
In an era where every click, tap or keystroke leaves a digital trail, Americans remain uneasy and uncertain about their personal data and feel they have little control over how it’s used.
This wariness is even ticking up in some areas like government data collection, according to a new Pew Research Center survey of U.S. adults conducted May 15-21, 2023.
Today, as in the past, most Americans are concerned about how companies and the government use their information. But there have been some changes in recent years:
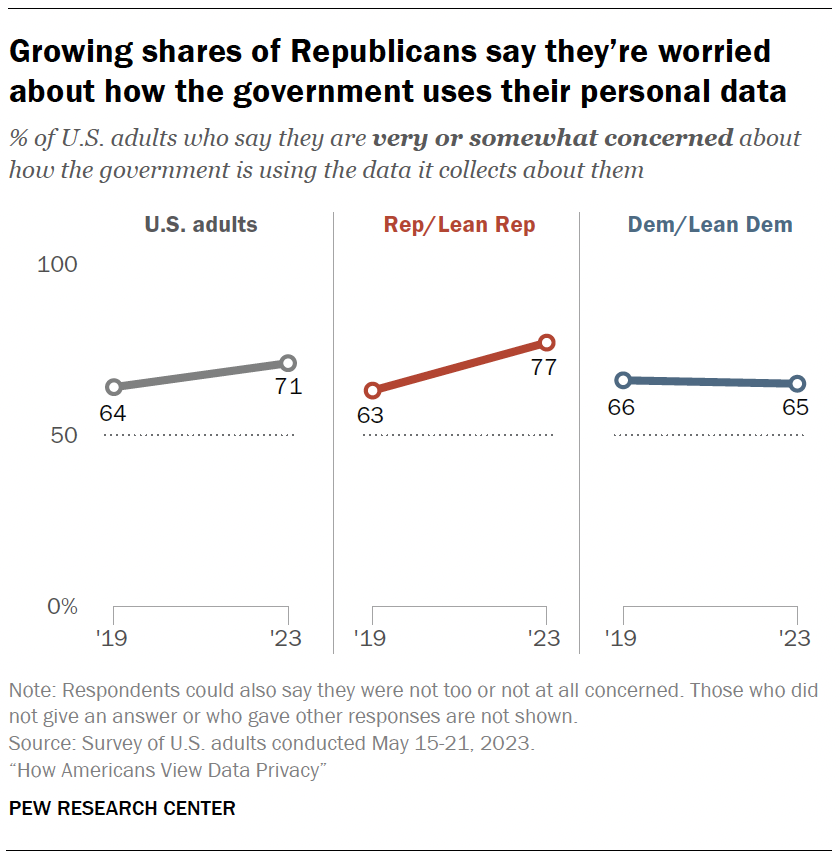
Americans – particularly Republicans – have grown more concerned about how the government uses their data. The share who say they are worried about government use of people’s data has increased from 64% in 2019 to 71% today. That reflects rising concern among Republicans (from 63% to 77%), while Democrats’ concern has held steady. (Each group includes those who lean toward the respective party.)
The public increasingly says they don’t understand what companies are doing with their data. Some 67% say they understand little to nothing about what companies are doing with their personal data, up from 59%.
Most believe they have little to no control over what companies or the government do with their data. While these shares have ticked down compared with 2019 , vast majorities feel this way about data collected by companies (73%) and the government (79%).
We’ve studied Americans’ views on data privacy for years. The topic remains in the national spotlight today, and it’s particularly relevant given the policy debates ranging from regulating AI to protecting kids on social media . But these are far from abstract concepts. They play out in the day-to-day lives of Americans in the passwords they choose, the privacy policies they agree to and the tactics they take – or not – to secure their personal information. We surveyed 5,101 U.S. adults using Pew Research Center’s American Trends Panel to give voice to people’s views and experiences on these topics.
In addition to the key findings covered on this page, the three chapters of this report provide more detail on:
- Views of data privacy risks, personal data and digital privacy laws (Chapter 1) . Concerns, feelings and trust, plus children’s online privacy, social media companies and views of law enforcement.
- How Americans protect their online data (Chapter 2) . Data breaches and hacks, passwords, cybersecurity and privacy policies.
- A deep dive into online privacy choices (Chapter 3) . How knowledge, confidence and concern relate to online privacy choices.
Role of social media, tech companies and government regulation
Trust in social media ceos.
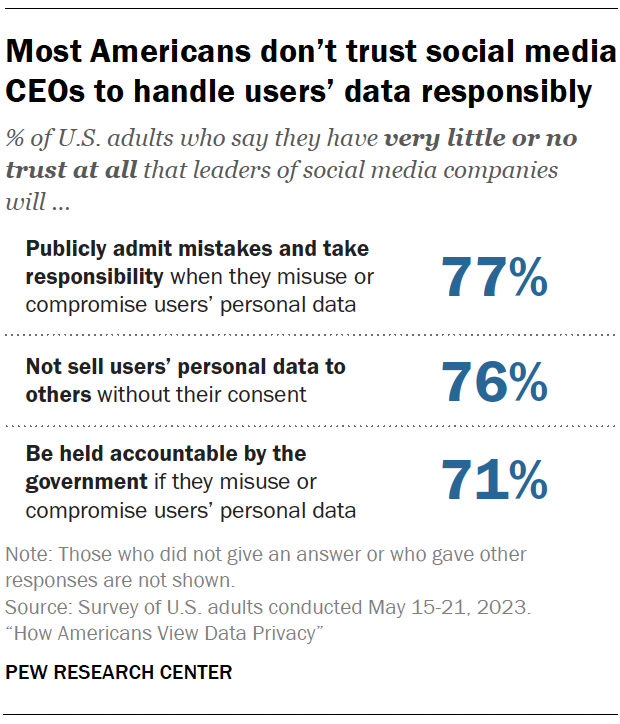
Americans have little faith that social media executives will responsibly handle user privacy.
Some 77% of Americans have little or no trust in leaders of social media companies to publicly admit mistakes and take responsibility for data misuse.
And they are no more optimistic about the government’s ability to rein them in: 71% have little to no trust that these tech leaders will be held accountable by the government for data missteps.
Artificial intelligence
People’s views on artificial intelligence (AI) are marked with distrust and worry about their data.
As AI raises new frontiers in how people’s data is being used, unease is high. Among those who’ve heard about AI, 70% have little to no trust in companies to make responsible decisions about how they use it in their products.
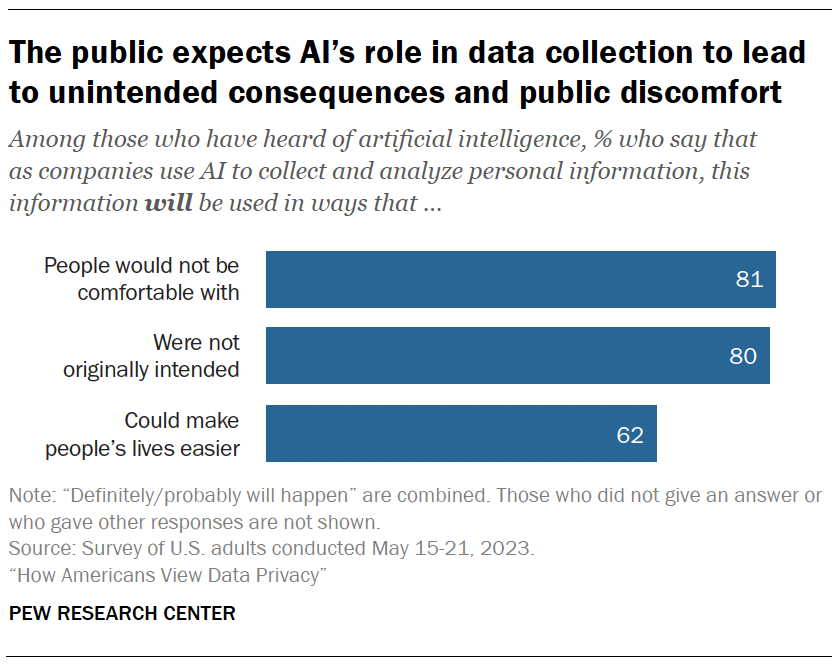
And about eight-in-ten of those familiar with AI say its use by companies will lead to people’s personal information being used in ways they won’t be comfortable with (81%) or that weren’t originally intended (80%).
Still, there’s some positivity: 62% of Americans who’ve heard of AI think that as companies use it, people’s information will be used to make life easier.
Children’s online privacy
Americans worry about kids’ online privacy – but largely expect parents to take responsibility. Some 89% are very or somewhat concerned about social media platforms knowing personal information about kids. Large shares also worry about advertisers and online games or gaming apps using kids’ data. And while most Americans (85%) say parents hold a great deal of responsibility for protecting kids’ online privacy, 59% also say this about tech companies and 46% about the government.
Government regulation
There is bipartisan support for more regulation of what companies can do with people’s data. Some 72% of Americans say there should be more regulation than there is now; just 7% say there should be less. Support for more regulation reaches across the political aisle, with 78% of Democrats and 68% of Republicans taking this stance.
Americans’ day-to-day experiences with online privacy
Americans’ day-to-day experiences reflect the difficulty of managing your privacy, even amid widespread concern. Some people are overwhelmed navigating the options tech companies provide or skeptical these steps will make a difference. And at times, people fail to take steps to safeguard their data.
Feelings about managing online privacy
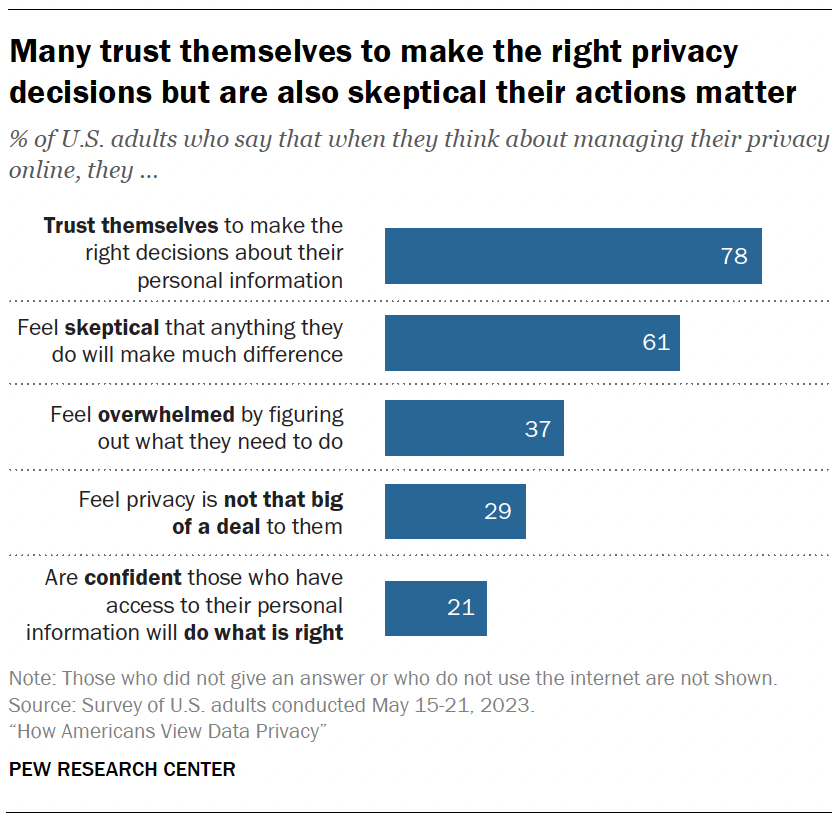
Americans’ feelings about managing their online privacy range from confident to overwhelmed. Most Americans (78%) trust themselves to make the right decisions about their personal information.
But a majority say they’re skeptical anything they do will make much difference. And only about one-in-five are confident that those who have their personal information will treat it responsibly.
How people approach privacy policies
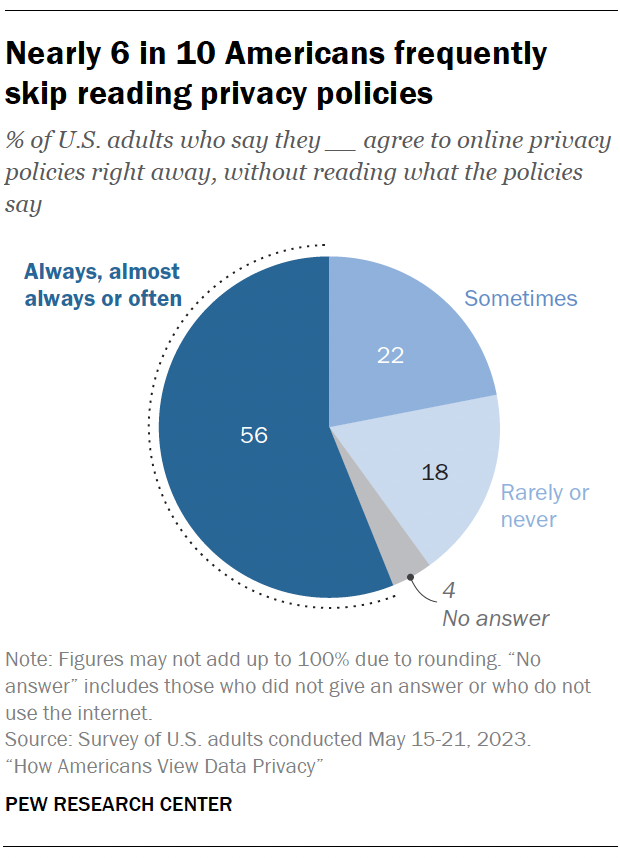
Privacy policies used by apps, websites and other online services allow users to review and consent to what is being done with their data.
But many say privacy policies’ long and technical nature can limit their usefulness – and that consumers lack meaningful choices .
Our survey finds that a majority of Americans ignore privacy policies altogether: 56% frequently click “agree” without actually reading their content.
People are also largely skeptical that privacy policies do what they’re intended to do. Some 61% think they’re ineffective at explaining how companies use people’s data. And 69% say they view these policies as just something to get past.
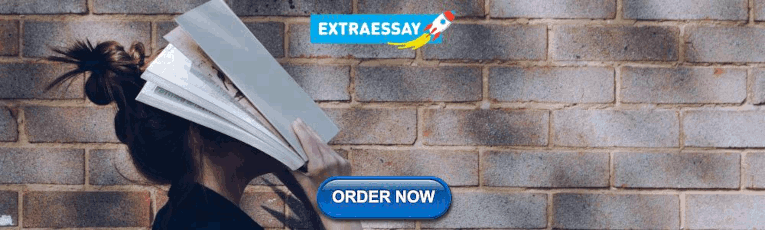
Password overload
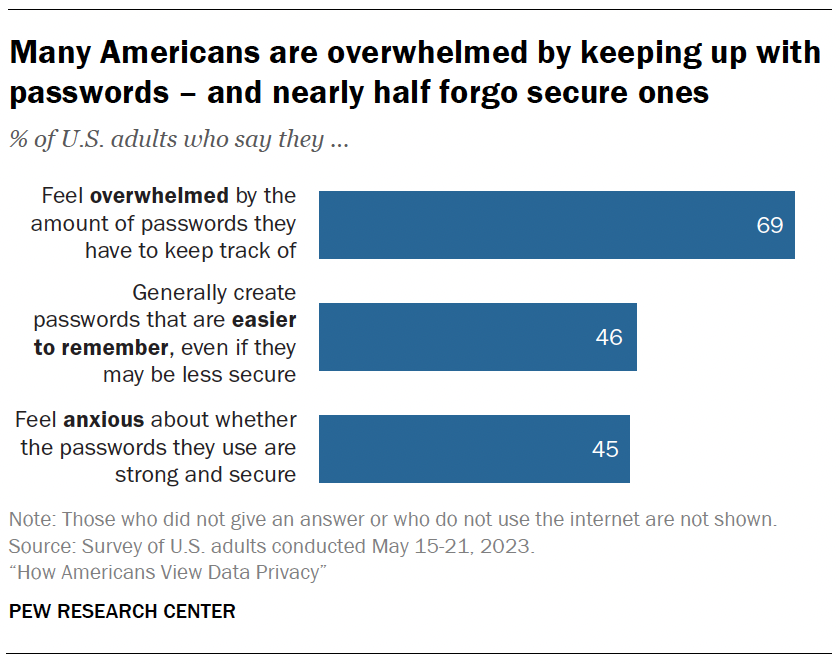
From social media accounts to mobile banking and streaming services, Americans must keep track of numerous passwords. This can leave many feeling fatigued, resigned and even anxious.
This survey finds about seven-in-ten Americans (69%) are overwhelmed by the number of passwords they have to keep track of. And nearly half (45%) report feeling anxious about whether their passwords are strong and secure.
But despite these concerns, only half of adults say they typically choose passwords that are more secure, even if they are harder to remember. A slightly smaller share opts for passwords that are easier to remember, even if they are less secure.
Password management
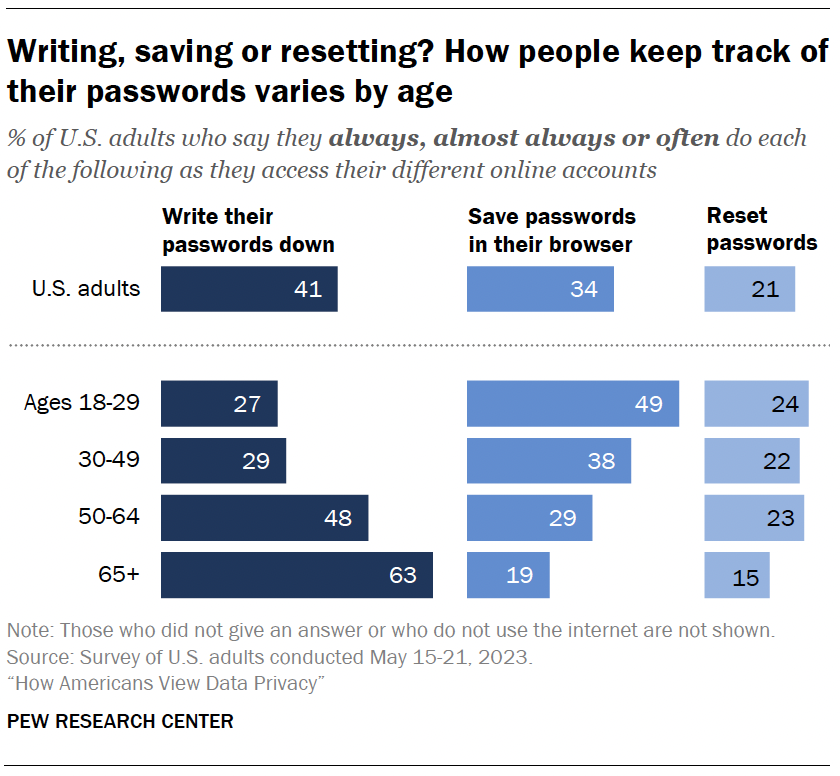
The public is adopting a range of strategies for managing their passwords.
Some 41% of Americans say they always, almost always or often write down their passwords. A slightly smaller share (34%) save their passwords in their browser with the same frequency. And 21% regularly reset the passwords to their online accounts.
These tactics vary across age groups. Some 63% of Americans ages 65 and older regularly write their passwords down. By contrast, 49% of adults under 30 say the same about saving their passwords in their browser.
One recommended approach to password management is becoming more common: More Americans are turning to password managers for help.
The share who say they use a password manager has risen from 20% in 2019 to 32% today. And roughly half of those ages 18 to 29 (49%) say they use these tools.
Smartphone security
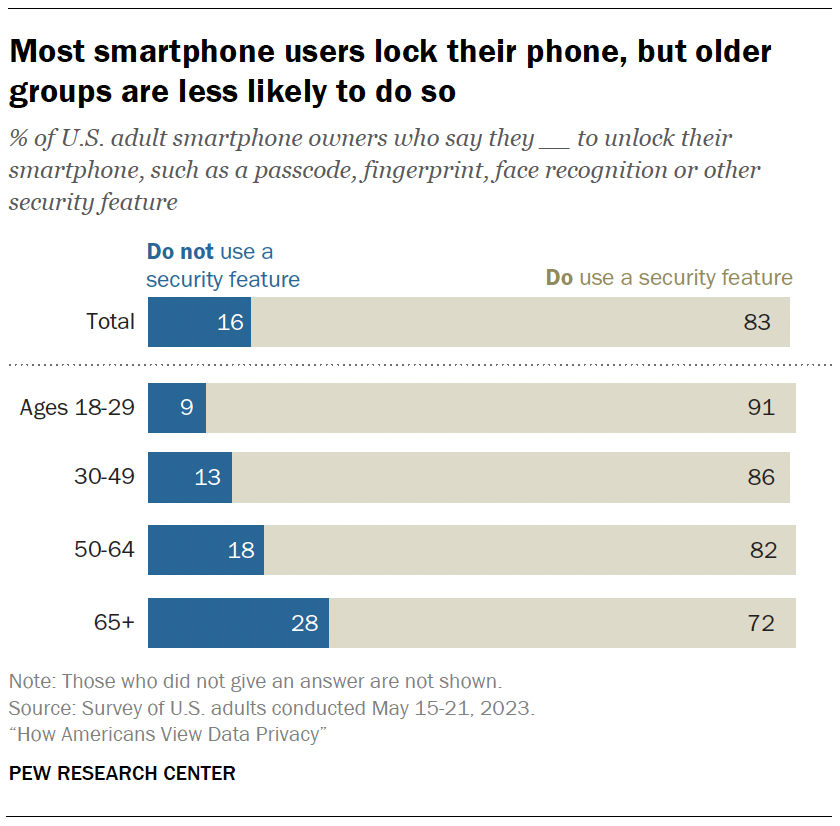
Even so, some riskier privacy habits linger. Notably, 16% of smartphone users say they do not use a security feature – like a passcode, fingerprint or face recognition – to unlock their phone.
And this is more common among older smartphone users. Those ages 65 and older are more likely than adults under 30 to say they do not use a security feature to unlock their mobile devices (28% vs. 9%).
Still, most users across age groups do take this security precaution.
Data breaches and hacks
Today’s data environment also comes with tangible risks: Some Americans’ personal information has fallen into the wrong hands.
Roughly one-quarter of Americans (26%) say someone has put fraudulent charges on their debit or credit card in the last 12 months. And 11% have had their email or social media accounts taken over without permission, while 7% have had someone attempt to open a line of credit or apply for a loan in their name.
All told, 34% have experienced at least one of these things in the past year.
Sign up for our Internet, Science and Tech newsletter
New findings, delivered monthly
Report Materials
Table of contents, what americans know about ai, cybersecurity and big tech, quiz: test your knowledge of digital topics, majority of americans say tiktok is a threat to national security, as ai spreads, experts predict the best and worst changes in digital life by 2035, how black americans view the use of face recognition technology by police, most popular.
About Pew Research Center Pew Research Center is a nonpartisan fact tank that informs the public about the issues, attitudes and trends shaping the world. It conducts public opinion polling, demographic research, media content analysis and other empirical social science research. Pew Research Center does not take policy positions. It is a subsidiary of The Pew Charitable Trusts .

An official website of the United States government
The .gov means it’s official. Federal government websites often end in .gov or .mil. Before sharing sensitive information, make sure you’re on a federal government site.
The site is secure. The https:// ensures that you are connecting to the official website and that any information you provide is encrypted and transmitted securely.
- Publications
- Account settings
Preview improvements coming to the PMC website in October 2024. Learn More or Try it out now .
- Advanced Search
- Journal List
- Elsevier - PMC COVID-19 Collection
- PMC10160179

A systematic review of privacy-preserving methods deployed with blockchain and federated learning for the telemedicine
Madhuri hiwale.
a Symbiosis Institute of Technology, Symbiosis International (Deemed University), Pune 412115, India
Rahee Walambe
b Symbiosis Centre for Applied Artificial Intelligence (SCAAI), Symbiosis International (Deemed University), Pune 412115, India
Vidyasagar Potdar
c Blockchain R&D Lab, School of Management and Marketing, Curtin University, Perth 6107, Australia
Ketan Kotecha
Associated data.
No data was used for the research described in the article
The unexpected and rapid spread of the COVID-19 pandemic has amplified the acceptance of remote healthcare systems such as telemedicine. Telemedicine effectively provides remote communication, better treatment recommendation, and personalized treatment on demand. It has emerged as the possible future of medicine. From a privacy perspective, secure storage, preservation, and controlled access to health data with consent are the main challenges to the effective deployment of telemedicine. It is paramount to fully overcome these challenges to integrate the telemedicine system into healthcare. In this regard, emerging technologies such as blockchain and federated learning have enormous potential to strengthen the telemedicine system. These technologies help enhance the overall healthcare standard when applied in an integrated way. The primary aim of this study is to perform a systematic literature review of previous research on privacy-preserving methods deployed with blockchain and federated learning for telemedicine. This study provides an in-depth qualitative analysis of relevant studies based on the architecture, privacy mechanisms, and machine learning methods used for data storage, access, and analytics. The survey allows the integration of blockchain and federated learning technologies with suitable privacy techniques to design a secure, trustworthy, and accurate telemedicine model with a privacy guarantee.
1. Introduction
The healthcare sector is crucial for the overall development of any nation around the globe. Historically, the primary goal of healthcare was to cure patients using medical aids. As a result, there was no emphasis on data privacy and security [1] . However, in the digital era, data privacy, secure data storage, exchange, and controlled accessibility of sensitive health data have become the primary concerns for the current healthcare system. As a result, of late, traditional healthcare systems have shown radical growth and eagerness to adopt new and advanced technologies in the pursuit of transferring to modern healthcare systems. Even with the acceptance of the latest technologies, healthcare systems still face numerous challenges related to data ownership, privacy, security, and integrity.
Since 2020, the COVID-19 pandemic has started a new virtual healthcare era. One promising technology in this regard is telemedicine or a remote healthcare system. The speedy global outbreak of COVID-19 has accelerated the demand for telemedicine technology-based solutions to a new height that too on an urgent basis [2] . Telemedicine or a remote healthcare system enhances the ability of all stakeholders to treat multiple patients without face-to-face communication. It establishes a healthier environment to boost the overall standard of healthcare [3] . In the case of infectious disease, telemedicine plays a vital role in improving the health outcomes of patient adherence, hospital readmission, and mortality [4] . Telemedicine effectively eliminates the geographical distance barrier to facilitate proper medical treatment and clinical health care at a low cost to remote patients [5] .
Despite the many potential advantages, there are still issues with the widespread adoption of the telemedicine system. Currently, all the stakeholders involved in the telemedicine system rely on centralized storage to exchange health data. Centralized data storage causes many problems, such as data breaches, lack of transparency, and trust, high cost, lack of patient-centric approach, and data privacy [6] . Therefore, any cloud-based solution must address data privacy and security concerns effectively.
One of the core concerns telemedicine faces is ensuring data privacy and security of exchanged data. A set of legal, economic, ethical, and technical problems are also related to health data privacy. Due to privacy concerns, stringent laws and regulations such as Health Insurance Portability and Accountability Act (HIPAA) [7] and General Data Protection Regulation (GDPR) [8] restrict hospitals from sharing sensitive data across healthcare institutes from building data analytics models. Due to these data privacy concerns, novel technologies such as the Internet of Things (IoT), Blockchain, Artificial Intelligence (AI), Machine Learning (ML), Big Data, and newer technology such as Federated learning are incorporated into healthcare to provide appropriate solutions [9] .
Since 2018, tremendous growth has been observed in adopting blockchain in healthcare [10] . The blockchain is an immutable, append-only distributed data structure. The acceptance of blockchain has increased as it provides a convenient means to overcome the current challenges of remote healthcare applications and enhance health data transparency, security, trustworthiness, integrity, and authenticity [11] . Estonia’s public healthcare system is one of the best examples demonstrating the potential of blockchain application in the healthcare industry. Since 2011, Estonia has deployed Guardtime blockchain technology for the complete implementation of its public digital health infrastructure [12] . Transactional data privacy is one of the challenging issues associated with blockchain technology [1] . Blockchain technology alone cannot guarantee advanced data privacy protection [13] . Thus, it is necessary to incorporate appropriate privacy-preserving mechanisms with blockchain technology to enhance its adaptability in the healthcare industry [14] , [15] .
There exists a good amount of literature on the blockchain. In [16] , the authors have highlighted the need for exhaustive work to understand the effectiveness of the blockchain in healthcare. The authors in [17] have covered the blockchain’s privacy challenges and privacy-preserving mechanisms. Similarly, in [18] , the authors have discussed the challenges and provided a detailed research plan for using blockchain in healthcare. Finally, in [19] , the authors have discussed blockchain-based use cases and their current status and open issues. This study categorized privacy challenges and provided the taxonomy of existing privacy mechanisms employed with blockchain.
Due to stringent privacy regulations, healthcare organizations are often unwilling to share sensitive data with other entities. However, cross-institute health data is necessary to develop a highly generalized global model. Another emerging technology that can play a vital role in healthcare data analytics in such a situation is Federated Learning (FL) [20] . Google introduced the federated learning approach in 2016. In this approach, the client never uploads the raw data to the coordinating or central server. The model updates are uploaded to the coordinating server [21] . Federated learning facilitates entities to create a collaborative global model without transferring raw data to third parties [22] , [23] . In global health emergencies, collaborative research and cooperation between multiple healthcare organizations and research institutes are vital to improving health outcomes. Federated learning can make this research collaboration and cooperation possible [24] . However, naive federated learning systems are susceptible to accountability and privacy threats, such as model poisoning attacks [25] , [26] .
Few studies have discussed the recent progress and challenges of federated learning technology for healthcare informatics [27] . In [20] , the authors have outlined future directions and challenges in federated learning. In [28] , the authors provide detailed categorization of federated learning according to different aspects such as data distribution, privacy mechanisms, architectures, and machine learning models. In [29] , the authors provide a detailed classification of security and privacy threats in federated learning. They discussed the trade-off between various privacy-preserving approaches and identified ways to enhance privacy in federated learning.
Federated learning and blockchain techniques are promising in health data analytics and management. Fig. 1 highlights the benefits of integrating blockchain and federated learning for different use cases. Concerning the potential of these technologies, many studies have integrated these technologies for various use cases. However, very few research studies have performed a systematic literature review on this research area [30] . In [31] , the authors systematically discussed the challenges and benefits of incorporating blockchain and federated learning. They have focused on three challenges, i.e., incentivization, decentralization, and membership selection. In [32] , the authors have discussed the challenges and opportunities in designing a blockchain-enabled federated framework for the mobile-edge computing domain. In [33] , the authors presented a systematic review that explored the current state and the future research opportunity to integrate Artificial Intelligence (AI) and blockchain. The authors have expressed how integration may create product innovation and economic value in industries. In [34] , the authors have presented an overview of combining machine learning and blockchain for healthcare.

Benefits of integrating blockchain with federated learning for different usecases.
Despite the scholarly contribution in the form of the existing studies, there is still a need to provide an in-depth analysis of the literature that focuses on integrating blockchain and federated learning to develop trustworthy and privacy-oriented remote healthcare applications. In the current remote healthcare era, integrating these emerging technologies into the telemedicine system could facilitate secure storage, exchange, and utilization of patient data, predict patient outcomes in a trustworthy manner and improve overall care quality [34] , [35] . However, there is a need to understand the current state-of-the-art research to develop reliable and privacy-oriented healthcare applications in alliance with federated learning and blockchain technology. For this reason, this survey discusses the potential benefits of blockchain and federated learning in improving the overall care quality of the telemedicine system. Data privacy is a core concern in remote healthcare applications. In this regard, this survey emphasizes privacy issues/attacks associated with blockchain and federated learning. The main focus is to review various privacy-preserving mechanisms deployed with these technologies to develop privacy-preserving healthcare applications.
In summary, the main contribution of this research study is threefold.
- • To comprehensively review the relevant literature on blockchain and federated learning for developing trustworthy healthcare applications.
- • To conduct an investigation and comparative analysis of various privacy-preserving mechanisms deployed on a blockchain and federated learning to develop privacy-oriented data models in the future.
- • To discuss and analyze the previous studies about the convergence of blockchain and federated learning techniques for the privacy-preservation of healthcare systems.
The rest of the survey is structured as follows: Section 2 describes the research methodology used to retrieve the relevant literature. Section 3 includes a comprehensive literature analysis that discusses the overview of telemedicine, blockchain, and federated learning. It covers blockchain-based telemedicine models, privacy-preserving methods used with blockchain, and federated learning for healthcare scenarios. Section 4 provides the discussion and main findings of our study. Finally, Section 5 discusses the future opportunity to integrate blockchain and federated learning technologies for developing robust healthcare applications. Fig. 2 depicts the thematic structure of the paper.

Thematic structure of paper.
2. Research methodology
This section represents the adopted research methodology to identify and analyze relevant literature regarding our research objectives and the topic. We have adopted Kitchenham and Charters method to perform a systematic literature review [36] , [37] . The Kitchenham and Charters guidelines are composed of three parts. The first part is planning the review; the main aim of this part is to define the research objectives of the Systematic Literature Review (SLR). The second part is to identify well-defined research questions. The main objective of this part is to refine the research questions into specific search queries to facilitate subject analysis and pinpoint the further research direction. The last part is responsible for reporting the results of the review.
This method helps define the research objectives, formulate the topic-specific research questions, and define topic-specific inclusion and exclusion selection criteria. These criteria help to find the documents necessary to develop privacy-preserving healthcare applications integrating blockchain and federated learning. Table 1 highlights the objective-based research questions.
Proposed research questions and main research objectives.
2.1. Search criteria
This study used academic data repositories such as Scopus, Web of Science, ScienceDirect, ArXiv, and IEEE Xplore to retrieve and analyze the relevant literature to include a wide range of publications. These documents helped us understand the state-of-the-art ongoing research in the respective research area. We have used well-defined search queries/keywords to systematically search the relevant research papers to address our formulated research questions. We have used the keywords such as (‘Blockchain AND Healthcare’), (‘Blockchain AND Telemedicine’), (‘Federated learning AND Healthcare’), (‘Federated learning AND Telemedicine’), (‘Blockchain AND Federated learning’), (‘Blockchain AND Federated learning AND Healthcare’), and (‘Blockchain AND Federated learning AND Telemedicine’) to get the basic idea about federated learning and blockchain for developing privacy-preserving healthcare applications such as telemedicine. There was considerable overlap in the research studies found using the initial level search queries. Therefore, this review used several analytical functions and filters provided by databases to extract valuable insight from the data.
2.2. Data collection
In the initial search, we included only journal articles, conference papers, and early access articles written in English. The role of federated machine learning and blockchain in remote healthcare is still emerging, and it is one of the fastest-growing research fields. Due to this, we have included early access articles and ArXiv preprints in our search, as the latest research results may be available as preprints that are valuable to review. The blockchain was launched by Satoshi Nakamoto in 2009, so we have included the documents published after 2009 for blockchain, and for federated learning, we reviewed 2017 onwards documents.
2.3. Inclusion and exclusion criteria
After retrieving the documents from the respective databases based on our search criteria, the next step was screening the relevant documents. For this study, we have screened only journal articles, conference papers, and early-access articles for further in-depth qualitative analysis. The documents were screened based on the title and abstract, and the irrelevant and duplicate copies were removed. Afterward, all the relevant documents were selected, and the references of those documents were also scanned to identify additional significant documents (Forward and Reverse Snowballing). This study excluded the research studies that were found irrelevant and were not peer-reviewed. Several research studies were also excluded from the qualitative analysis process because they were not related to the healthcare domain.
Overall, this review includes the relevant research papers to address our key research questions and excludes those that did not fit our research questions. As a result, 158 research studies were selected for further in-depth analysis. Table 2 depicts the search criteria used for inclusion and exclusion.
Search criteria for inclusion and exclusion.
2.4. Quality assessment criteria
Quality assessment criteria help evaluate significant research works to answer objective-based research questions. The research works satisfying the desired criteria were selected for further qualitative analysis. Table 3 depicts the quality assessment criteria.
Quality assessment criteria.
2.5. Data extraction
The data from selected research studies and reports which meet our inclusion criteria were considered for qualitative analysis. Based on the thematic map, the content was categorized and summarized in the following sections and subsections.
3. Comprehensive analysis of the literature
This section provides the analysis of formulated research questions. This section reviews the research papers that show the applicability of blockchain and federated learning for telemedicine systems.
3.1. RQ1: Overview and challenges of telemedicine
Telemedicine is a remote healthcare service. According to the World Health Organization (WHO), telemedicine facilitates the exchange of medical information between geographically separate locations by healthcare providers using information and communication technologies (ICT) for better treatment, prevention, and diagnosis of diseases [3] . This virtual platform establishes remote coordination between patients and healthcare providers and has excellent potential to increase data access and care quality [38] . It creates a safe environment to provide on-demand and personalized treatment quickly. Fig. 3 shows the classic architecture of a telemedicine system.

Architecture of cloud-based telemedicine system.
During the COVID-19 crisis, the adoption of telemedicine systems has shown exponential growth around the globe [39] . According to the global telemedicine market report, a telemedicine system is an efficient solution that facilitates the effective management of COVID-19 [40] . For example- The Italian government launched family-centered telemedicine to control the spread of coronavirus. This developed system provided immediate telemedicine support to children and their families to reduce the risk of psychological burnout and emotional distress during the lockdown phase [41] .
Currently, the telemedicine system depends on the Cloud Service Provider (CSP) to gather and transfer healthcare data [42] . Few state-of-art systems rely on the cloud server, such as cyber–physical systems [43] and health monitoring systems designed for stroke management [44] . These system collects health information using medical sensors and stores it on the cloud server [45] , [46] . This centralized health data storage may result in data breaches, and patients may not trust the telehealth system. Centralized storage allows cybercriminals to launch attacks targeting data integrity, privacy, and confidentiality. Due to this, the patient may feel insecure about storing their personal health information on the cloud server. So, privacy and security are challenging issues in cloud-based service platforms [6] . Another issue in remote healthcare is the secure and authorized control of health data between multiple healthcare providers [47] . Once the data is collected in the cloud, the patient loses control over their data. The patient is unaware of who is accessing and sharing their health records. There is a lack of a data ownership approach and patient-centric access control mechanisms [48] . In such cases, security and easy accessibility of data become critical concerns. Adopting advanced technologies with telemedicine systems is essential to enhance their adaptability in healthcare. Fig. 4 depicts the current challenges faced by the cloud-based telemedicine system.

Challenges in telemedicine system.
3.2. RQ2: Blockchain-based healthcare solutions
The blockchain is an immutable, append-only distributed data structure. Initially, its applicability was mostly limited to financial sectors in the form of Bitcoin (a cryptocurrency) application [49] . Recently, owing to its inherited potential, it has shown substantial adaptability in various other sectors [12] . In healthcare, blockchain has a huge potential to create a technological revolution [50] . The decentralized and immutable nature of blockchain helps to create a transparent healthcare workflow that allows patients to know how their health data is shared and accessed in the network [16] , [51] . In addition, blockchain uses a cryptographic algorithm to ensure data security [52] . From the healthcare perspective, blockchain’s data provenances, accountability, availability, and robustness are notable benefits to facilitating effective health record management [53] . Blockchain enables efficient, immutable, and scalable data sharing from various sources, for example, EHR, clinical trials, genomic databases, and IoT data from multiple sensors [54] .
3.2.1. Advantages and challenges of blockchain in healthcare
Few research studies have explored blockchain’s key advantages and challenges to healthcare applications [55] . Table 4 highlights the advantages and problems associated with blockchain technology.
Advantages and challenges of blockchain.
• Advantages of blockchain: Blockchain is a highly effective technology for storing and retrieving health data [56] . One of the fascinating features of blockchain is that it eliminates the dependency on centralized third-party. Blockchain offers a viable data transmission and storage solution owing to its immutability, decentralized, and transparency features. The decentralization quality helps create a transparent healthcare workflow that lets patients know how their health data is shared and accessed in the network. Blockchain technology facilitates secure storage and workflow of health data. It provides authorized data access and ensures data integrity and confidentiality.
The blockchain-based system is resilient against health data corruption and data losses. With the transparency and data availability feature, blockchain creates a trustworthy atmosphere for distributed healthcare applications. The health data saved on the blockchain are time-stamped, cryptographically encrypted, and appended chronologically. It helps ensure health data security [16] . With well-designed smart contracts, blockchain facilitates health data ownership [57] .
• Challenges of blockchain: A few specific challenges make healthcare organizations hesitant to adopt blockchain technology. These challenges mainly include scalability, advanced-level privacy, and interoperability issues [58] . Scalability and interoperability are highly discussed technical threats faced by blockchain technology. Scalability is the core problem with the current blockchain implementation [15] . To solve the scalability problem in [59] , the authors have used off-chain storage protocols such as IPFS (InterPlanetary File system). This protocol is the content-addressed, peer-to-peer distributed file structure that helps to access and store health data easily. Integrating IPFS with blockchain helps protect health data and build a robust healthcare system [60] .
In the case of the Proof of Work (POW)-based public blockchain, slow transaction speed, high energy consumption, and potential privacy are noticeable threats. Initial installation costs and the lack of essential technical skills by health stakeholders to operate blockchain technology are other issues identified in the previous studies [61] . Writing efficient smart contracts is also a challenging task. Another challenge is the usability of handling complicated healthcare systems. Since health professionals are not technically sound as IT professionals to manage complex healthcare systems. These are the hurdles that blockchain technology needs to overcome before being significantly adopted in healthcare applications.
Recently, several research studies have developed blockchain-based healthcare models to improve the current EHR system [62] , [63] , [64] . Few research studies focus on patient-centric data transfer among multiple healthcare stakeholders [65] , [66] , [67] . Some studies focus on developing a blockchain-based model to overcome privacy and security issues in digital healthcare systems [68] , [69] , [70] , [71] . In the following subsection, we have divided the existing studies based on the issues they handled and how blockchain helps deal with them. Table 5 shows the summarization of relevant studies that implemented blockchain-based models.
Summarization of relevant studies implementing blockchain-based healthcare solutions.
• Single point of failure: Traditional healthcare systems depend on a centralized authority to store and access health data. The centralized data storage raises issues such as single point of failure and data breaches. To address the existing problems of the traditional healthcare system, many authors have highlighted the benefits of blockchain-based architectures for effective data management [80] , [81] , [82] . In [65] , the authors have proposed a novel blockchain-based decentralized framework that eliminates the need to depend on any third party to facilitate secure and patient-centric communication between patients and hospitals. In [80] , the authors have described the potential features of blockchain in alliance with personalized mobile-based applications to facilitate trustworthy data exchange.
• Lack of patient-centric approach: Few researchers implemented owner-centric blockchains in healthcare applications. They have developed a blockchain-based patient-centric EHR exchange framework to improvise the current healthcare workflow [66] . For example, In [66] , the authors have developed a blockchain-based patient-centric data-sharing model for diabetes patients. They have created multi-signature contracts to control and share access to health data. In [67] , the authors have proposed a patient-centric blockchain model. In this architecture, patient-centric smart contracts are designed to grant clinicians consent to use patient data. The EHR data is in the local database, whereas the blockchain contains metadata. Similarly, in [95] , the authors have developed dual blockchain platforms: one permissioned blockchain owned by the patient and another consortium blockchain by the health authority.
• Insecure data sharing and management: The framework designed by [47] provides a blockchain-based method to protect medical data exchange. The developed framework guarantees the integrity and trustworthiness of Medical Resonance Imaging (MRI) distributed through various hospital networks. In [84] , the authors have proposed BlockHR, a blockchain-based health data management framework to provide better data management and access between patients and healthcare providers. The data retrieval is 20 times faster for BlockHR than the client–server approach. In this regard, the authors in [96] have proposed Hyperledger Fabric and NDN, i.e., Naming Data Networking Protocols, to provide secure health monitoring.
• Lack of access control mechanism: In current healthcare services, EHR is always present in multiple hospitals and accessed by a centralized authority. There is a need to build access control frameworks to protect and secure EHR sharing. In [74] , the authors have proposed a public and private blockchain-based framework. Blockchain maintains the interaction between external and internal entities. To solve the issues related to access control, the authors [89] have designed a blockchain-based architecture that uses a Genetic algorithm and Discrete Wavelet Transform to ensure authorized access control and optimize the system performance.
• Interoperability: In [90] , the authors have addressed the interoperability and regulatory compliance issues in home-based healthcare applications. They have incorporated a blockchain and edge computing platform called CORD (Central Office Rearchitected as a Data center) to enable authorized communication between patients and home-based applications. In [58] , the authors have emphasized the blockchain’s significance in overcoming security and interoperability issues of EHR management in eHealth. Similarly, in [92] , to improve interoperability and reliability, authors have built a consortium blockchain-based health data sharing architecture called SHAREChain. This architecture incorporates two standards: Cross-Enterprise Document Sharing (XDS) and another is Fast Healthcare Interoperable Resource (FHIR). Similarly, in [91] , the authors used the FHIR standard to manage health data in an interoperable manner. They have proposed permissioned blockchain-based architecture with Proof of Authority (PoA) technology that facilitates patient-centric data exchange.
• Limited data provenance: Traceability, transparency, and immutability are vital features of blockchain that make it appealing in various applications. Due to these features, In [93] , The authors have incorporated blockchain within the public healthcare system to enhance accountability and transparency in public healthcare. In [94] , the authors have presented a blockchain-based architecture to improve drug traceability in a decentralized manner. With smart contract logic, blockchain guarantees data provenance and provides a robust end-to-end trace system for the drug supply chain [75] .
3.2.2. Blockchain-based telemedicine solutions
Few research studies have developed a blockchain-based telemedicine model to build robust and patient-centric systems for reliable communication between patients and healthcare stakeholders [77] , [97] . In [98] , the authors have designed a blockchain-based patient-centric platform for telemedicine called HapiChain. The framework secures the workflow between patients and doctors for teleconsultation services. In [99] , the authors have proposed a blockchain-based telemedical laboratory. They have used the Internet of Medical Things (IoMT) and the cloud to provide better treatment. The article [80] focused on patient location privacy to design a telecare medical information system. The authors have proposed a blockchain-based scheme for protecting patient locations using a Merkle tree and Order-Preserving Encryption (OPE). Merkel tree uses a one-way hash function to construct a binary tree, verifying data integrity.
One of the fascinating applications of telemedicine is telesurgery. In the case of telesurgery or remote surgery, uninterrupted and authorized data access is a crucial part. For this purpose, in [100] , the authors have proposed blockchain-based telesurgery. This system uses Interplanetary File System (IPFS) to resolve data storage cost issues and provide higher throughput and lower latency for data distribution.
Similarly, in [101] , the authors have proposed a telesurgery framework that uses public blockchain-based smart contracts to develop trust between various entities, such as patients and surgeons. This framework uses IPFS for data storage cost-effectiveness. They have incorporated artificial learning techniques to train the surgical robot. In [102] , the authors have developed an interoperable telesurgery framework. This framework uses a permissioned blockchain to design trusted digital agreements to facilitating secure coordination between surgeons, patients, and caregivers. Each surgeon has a copy of complete surgical procedure information executed by all surgeons this transparent and trustworthy.
Teledermatology is considered the well-known application of telemedicine. In [103] , the authors have applied a blockchain-based approach to a teledermatology e-health platform. This platform includes smart contracts that manage communication and access between multiple stakeholders. Table 6 summarizes the relevant research studies implementing blockchain-based telemedicine solutions.
Summarization of relevant studies implementing blockchain-based telemedicine solutions.
3.3. RQ3: Blockchain-based privacy-preserving mechanisms
There are various privacy-preserving mechanisms deployed on the blockchain that helps to ensure data privacy and secure accessibility [110] . This section covers encryption schemes, cryptography, smart contracts, and data anonymization methods.
3.3.1. Privacy issues in blockchain
Privacy is a primary concern for the integration of blockchain in healthcare applications. Even the Bitcoin blockchain has proven illusory in guaranteeing strong privacy as it fails to provide complete anonymity [17] , [111] . Data privacy and confidentiality are challenging issues for blockchain. In a blockchain, private keys are employed to sign transactions; therefore, these keys are critical for the user’s privacy [17] . Several mechanisms, such as encryption-based or anonymization, can improve blockchain privacy and data confidentiality. Most blockchains are publicly accessible databases exposed to various potential privacy challenges, such as on-chain data privacy, transaction likability, malicious smart contracts, and privacy regulation compliance. These potential privacy issues may hinder the wide adaptability of blockchain in the healthcare industry [112] . There are two issues of privacy: identity privacy and transactional privacy [113] . Identity privacy means maintaining the patient’s private identity and not mixing it with the transactions. Transactional privacy is a challenging issue faced by blockchain. Some measures, such as pseudonyms, are insufficient to ensure transactional privacy [19] . Therefore, several mechanisms, such as zero-knowledge proof and mixing, were proposed to improve privacy.
3.3.2. Privacy-preserving mechanisms in blockchain
Recently, privacy-preserving data exchange has gained tremendous attention in healthcare scenarios, especially for effective data analytics. For this reason, in previous research studies, several privacy methods have been used with blockchain to strengthen privacy. Fig. 5 shows well-known privacy-preserving mechanisms deployed on the blockchain. Encryption schemes such as proxy re-encryption and attribute-based encryption were the well-known cryptographic methods used to develop privacy-preserving data exchange. The permissioned blockchain-based smart contract is an efficient solution for fine-grained access control policies [114] . In the following subsection, we have explored several well-known privacy mechanisms to gain insightful information.

Privacy-preserving mechanisms deployed on blockchain.
- • Encryption Scheme: Encryption is a well-known method used in blockchain. Encryption methods are integrated with blockchain technology to meet data ownership and security requirements [115] . In the symmetric encryption method, the same key is accessible by both sender and the receiver. In the asymmetric scheme, the private key and the public facilitate the decryption and encryption of the data. Several data encryption methods provide access control and security over the network, for example- attribute-based encryption, identity-based encryption [116] , and proxy re-encryption [117] . These techniques help securely transfer data from the data owner to the requester. Several past research studies integrated these methods with the blockchain platform. In [118] , the authors created Health-chain, a privacy-preserving framework for health data. The hash value of health data stored in IPFS ensures privacy preservation while reducing the computational overhead.
- • Identity-based Encryption: Shamir first introduced the novel idea of a public-key cryptography scheme in 1984 [116] . Since then, researchers have proposed several Identity-based Encryption (IBE) proposals [112] . The IBE scheme uses bilinear maps theory. In the article [112] , the authors integrated the IBE scheme with permissioned blockchain to provide a privacy protection scheme. This scheme was better than traditional Public Key Infrastructure (PKI), as it avoids complicated certificate management and prevents passive attacks. The IBE scheme uses a unique identity ID to generate the user’s public key. With a unique identity ID, any user who wants to join the permissioned blockchain can obtain the encrypted key. In the article [119] , the authors used blockchain and identity-based encryption schemes to provide a decentralized and privacy-preserving exchange of Internet of Things (IoT) data. Blockchain provides access control policies, and identity-based encryption facilitates cryptographic access control policies. In the article [120] , the authors have developed medical information exchange platform based on blockchain and identity-based encryption to guarantee medical data privacy and confidentiality.
- • Proxy Re-encryption: Proxy re-encryption (PRE) is a feasible cryptographic public-key encryption method proposed by Blaze et al. [121] and Mambo and Okamoto [117] . This method permits third parties or proxies to securely transform the ciphertexts or encrypted data from one public key to another. The proxy or cloud service providers cannot acquire any details about the original message [122] . It is a feasible solution to provide secure access delegation. Integrating PRE with blockchain smart contracts provides a fast and efficient platform for sharing and storing data [34] , [82] . In [123] , [124] , the authors have proposed a blockchain-based model to protect EHR. This model uses the proxy re-encryption method to transfer patient data without revealing the private key. In [125] , the authors have designed a proxy re-encryption and blockchain-based distributed secure file storage and sharing system. In [126] , the authors have designed a blockchain-based cloud-assisted framework that combines proxy-re encryption and searchable encryption technology to ensure data privacy for EHR sharing.
- • Attribute-based Encryption (ABE): This is one of the promising techniques of a public-key-based scheme. It is an efficient technique that guarantees fine-grained access policies [127] . ABE allows only specific users with certain attributes to view or access the data, depending on the access control policies. In [128] , the authors have designed an ABE-based blockchain model for IoT applications. With ABE, only the user with a specific attribute can access the data. This proposed model achieves higher privacy with minimum computational overhead. Few other encryption approaches ensure health data protection, for example- Cipher-Policy Attribute-based Encryption (CP-ABE) [129] and Key-Policy Attribute-based Encryption (CP-ABE) [127] . Only the user with valid attributes (user key) can decrypt the data to achieve authenticity with these methods. With CP-ABE, users can define the access control structure of their health data [107] . The authors of the article [130] have designed blockchain-based privacy-oriented architecture. In this article, the patient specifies the access policies and the time duration to access his data. The architecture used a voting-based Practical Byzantine Fault Tolerance (PBFT) method and encrypted the user’s data, ensuring confidentiality.
- • Blockchain-based Smart Contracts: Nick Sazabo introduced the Smart Contracts (SC) term in 1997 [131] . Smart contracts are digital contractual conditions of agreements written in various computer programming languages. The smart contract executes automatically once the predefined conditions written in the contracts are satisfied [52] . A smart contract is implemented on the topmost level of the blockchain to guarantee proper access control. Various programming languages such as Golang, Kotlin, Solidity, Java, and JavaScript are used to write down smart contracts depending on the blockchain platform. However, contract correctness, designing careful control flow, improving execution efficiency, proliferation, and redeployment are challenging issues associated with smart contracts [57] . Permissioned blockchain platforms such as Hyperledger Fabric (HF) have been used in previous studies to achieve privacy and confidentiality [130] , [132] . Hyperledger Fabric or an enterprise blockchain is feasible to protect private health data. In [124] , the authors have utilized HF and public key infrastructure features to provide patient-centric authorized access to health records. In [133] , the authors have proposed HF-based modular architecture that adopts fundamental concepts of HF, such as modularity. In [134] , the authors have proposed Ancile ; this blockchain-based model applies smart contracts to maintain the cryptographic hashes of medical records and proxy-re encryption privacy-preservation efficient access control.
- • Attribute-based Signature: Attribute-based Signature (ABS) is beneficial in attribute-based messaging and anonymous authentication systems [135] . In an attribute-based signature, an authority issues the set of attributes to a valid user or signer. A signer signs the message or document with a predicate satisfied by the singer’s set of attributes. In this scheme, the signature hides any identifying detail about the signer and issued attributes. In [130] , the authors have used an attribute-based signature method to propose a privacy-preserving blockchain-based framework. The users, such as doctors and nurses, invoked the Attribute-based signature to upload and access the EHR stored on the blockchain. KUNodes is a node selection algorithm that helps to achieve attribute revocation. Similarly, in [136] , the authors have integrated the Attribute-based Multi-Signature (ABMS) method and ABE scheme with Hyperledger Fabric and Hyperledger Ursa library. This architecture helps authenticate patients anonymously and encrypt EHR to facilitate efficient EHR management.
- • Ring Signature: Ring signature is another encryption technique for data privacy protection. This digital signature scheme is developed by Rivest et al. [137] . The ring signature transaction contains only a specific group of members (ring members). Any member can produce a digital signature by randomly selecting multiple member’s public keys, a random value, a signer’s secret key, and other techniques. It is computationally infeasible to revoke the anonymity of the actual singer. It is an elegant way to solve issues with multiparty computations. In [138] , the authors have proposed a ring signature-based blockchain framework to build a privacy-preserving data storage model. This model ensures data privacy.
- • Data Anonymization: In the blockchain, differential privacy is a simple anonymization method applied to protect data privacy. For instance, in [139] , the authors have employed differential privacy to prevent an adversary who can gather sensitive personal data when implementing federated learning using blockchain to note crowdsourcing activities. In [110] , [140] , the authors have integrated blockchain and differential privacy for the privacy preservation of data. In [141] , a differential privacy technique protects personal information while performing federated learning.
Mixing is another anonymization method [142] used in blockchain to conceal the history of transactions. This method makes it difficult to correlate the transaction history [17] . Thus, it helps to make the address non-correctable in the transaction history. In [143] , the authors have used a mixing scheme with the Bitcoin blockchain to protect private information. The Zero-Knowledge Proof (ZKP) is a cryptographic method in which the prover attempts to convince the verifier of his knowledge without revealing any other information apart from its gained knowledge [144] . It keeps the sender and receiver transaction detail hidden. Due to this feature, ZKP ensures authentication [82] , secure communication, and privacy [145] .
3.4. RQ4: Federated learning for privacy-preservation
Machine Learning-based (ML) models have emerged as an efficient approach to achieving robust and accurate health data analytics in the last two decades. However, to take full advantage of the machine learning approach, a large amount of health data is needed to build effective predictive models. For this reason, the collaboration between multiple health organizations must collect and share health data [27] . The recent COVID-19 pandemic has also highlighted the need to effectively share health data, resources, and knowledge globally [146] , [147] . In practice, the sensitive nature of health data and stringent privacy regulation laws such as HIPAA and GDPR restrict hospitals from sharing their raw health data with other entities. Thus, there is a trade-off between data privacy and better predictive data analytics [148] . The Federated Learning (FL) approach is relevant to remove this trade-off as it eliminates the need to share raw data to train a global machine learning model. FL is an emerging technology that can guarantee data privacy and train a collaborative model from multiple data providers [149] . The main advantage of FL over the traditional centralized machine learning methods is the ability to provide decentralized collaborative learning for implementing ML algorithms. There is no need to collect or process data at data centers; instead, the ML model is trained at the local node. Another advantage of FL is compliance with GDPR, as data never leaves the local node, and only model updates are shared [150] .
There are three main steps involved in FL implementation. In the first step, the central or coordinating server initiates the process and shares the global model, i.e., the initial model parameter, with all the federated users/clients. In the second step, all the clients/users train their respective local models using the initial model parameter and their data. Afterward, the client sent trained local model updates to the coordinating server. In the third step, the coordinating server aggregates all the local updates and generates a new global model. Finally, the new global model was shared with all the clients. This iterative process repeats till the model achieves a certain level of accuracy [29] .
Generally, categorizing a federated learning system depends on the data distribution characteristics and the client’s participation in the FL environment. The three types of FL are horizontal FL, vertical FL, and last one is federated transfer learning. In horizontal FL, datasets own by different clients share similar feature spaces but with different sample spaces. In vertical FL, clients have similar sample spaces with different feature spaces. Federated transfer learning is the hybrid of horizontal FL and vertical FL [151] . FL is a rapidly evolving technology. Google and WeBank have launched open-source FL platforms such as TensorFlow Federated [152] and Federated AI Technology Enabler (FATE) to increase FL implementation [153] . In the recent past, FL has become a promising technique that plays a vital role in various applications to offer low-latency decisions with privacy guarantees. Some well-known applications that highlight the potential of FL are- virtual keyboards, self-driving cars, healthcare, robotics, Unmanned Arial Vehicles (UAV), and supply chain finance [154] .
One of the highly influential sectors of FL is the healthcare industry. FL addresses data privacy and governance issues, usually in health data aggregation [27] . In healthcare, FL allows hospitals to collaboratively train a global model without sharing raw data with other entities. In the FL mechanism, multiple healthcare organizations share locally calculated model updates to a coordinating server. These model updates generate a global predictive model without revealing private datasets [155] . Thus, it protects privacy and ensures legal and ethical compliance between hospitals [156] . More recently, in the COVID pandemic, the Standford Institute of Human-Centered AI created a federated learning-based in-home system to monitor persons for coronavirus symptoms. In addition, some research works proposed FL-based solutions to detect coronavirus infections while preserving patient data privacy [146] , [157] . Similarly, NVIDIA Clara is a healthcare service platform that uses federated learning to protect patient data privacy in healthcare and medical institutions. Fig. 6 shows a typical model of federated learning.

Architecture of federated learning in healthcare scenario.
3.4.1. Advantages and privacy issues in federated learning
Compared to the traditional ML approach, FL naturally provides a privacy guarantee. Multiple hospitals train models collaboratively in federated learning scenarios without a centralized dataset. Hospitals only shared updated models with the coordinating servers. Thus, FL avoids collecting massive health data at any centralized repository. FL reduced the training time and cost and improved data security. Table 7 shows the advantages and challenges of federated learning.
Advantages and challenges in federated learning.
3.4.2. Privacy attacks/threats in federated learning
FL offers initial privacy protection but is still susceptible to potential privacy attacks. Despite the significant research on FL schemes, existing FL schemes are still vulnerable to potential privacy attacks. FL schemes cannot meet the advanced security requirements for applications. Keeping this in view, we have discussed the attacks in FL and reviewed several privacy-preserving techniques incorporated in FL.
According to prior research, sharing local model updates to the collaborator server in FL can leak sensitive information [21] . For example, in [158] , the authors have discussed the model training process. In that process, adversaries can partially extract each client’s training data based on their uploaded model parameter. A recent work depicts how those attackers can extract training data from model parameters in a few iterations. Such issues make FL vulnerable to privacy attacks such as Poisoning attacks [159] and Inference attacks that include membership inference attacks [160] , model inversion attacks [161] , and reconstruction attacks. The current FL system faces two types of attacks. The first is an insider attack launched by the FL server. The clients in the FL system fall under the insider attacks category- for example, Sybil Attacks [25] and Byzantine Attacks,162 . The second is outsider attacks launched by the final users of FL systems and by intruders.
- • Membership Inference Attack: The main aim of membership inference attacks is to determine whether the input samples to the learning model come from the training dataset or not. In the FL approach, the adversary aims to determine if a specific input sample belongs to the personal training data of only a single party or if it belongs to any party [162] . In the FL, attackers can conduct passive and active membership inference attacks. In passive attacks, attackers perform the inference by observing updated model parameters without changing anything in the global or local training process. Inactive attacks, attackers can carry out stronger attacks against other clients by tampering with the FL model [163] .
- • Poisoning Attack: In the FL framework, the coordinating server is susceptible to inspecting the original data or training process at the local node. Thus, this situation prohibits the transparency of the model training process. Moreover, it imposes model poising attacks. Throughout the training phase, the poisoning attacks can be carried out on the model called model poisoning and on data called data poisoning attacks [164] . Model poisoning attacks target model parameters or insert the backdoor attacks into the global model before sending them to the coordinating server [25] . At a very high level, both the data and model poisoning attacks try to change the behavior of the trained model.
Membership inference attacks and poisoning attacks show the need to protect model parameters in the FL training process. Therefore, there is a need to use privacy mechanisms to protect client data effectively in the FL system [150] .
3.5. RQ5: Privacy-preserving mechanisms in federated learning
Recent work on FL has demonstrated that FL may fail to provide a strong privacy guarantee. Therefore, for designing a robust FL system, there is a need to use privacy-preserving techniques to protect privacy at two-level- first at the training dataset and another at the exchange of local model parameters [150] . The various privacy-preserving methods have integrated with FL to deal with privacy threats/attacks [165] .
- • Differential Privacy (DP): DP was proposed in 2006 by Dwork et al. [166] . DP is the most widely used privacy technique due to its algorithmic simplicity and small system overhead. Communication between a coordinating server and clients is the trickiest element in the FL scheme. In such a situation, to provide effective communication between the central server and clients DP mechanism is commonly used before sharing model parameters. In the DP mechanism, a certain amount of random noise is added to data or the model updates before being exchanged to the central server [167] . DP mechanism is also used before sharing the algorithm updates or computational results [168] . DP upsurges the level of privacy in the FL framework [169] ; still, it often yields lower data utility or substantial loss in global model performance [170] . Furthermore, owing to the random noise present in the training process, the FL system may develop less accurate data models. Still, a trade-off exists between data utility and privacy guarantee [166] .
- • Homomorphic Encryption: It is an attractive privacy-preserving cryptographic method adopted in many FL systems that can perform specialized calculations (e.g., addition) on encrypted data or ciphertext without decrypting it first [171] . Based on the computational operations and encryption, HE consists of different types, such as partial HE, fully homomorphic encryption [172] , and somewhat HE. In the FL system, the HE technique paillier helps securely aggregate model parameters [173] . In addition, some research studies have used the additive property of partial HE to prevent attacks on locally computed models [174] , [175] . HE provides potential privacy for cross-silos FL by performing easy and complex computation operations (e.g., exponentiation, modular multiplication). However, the computation of complex functions is expensive to compute. In HE, performing complex operations increases significant computational overhead [150] . The authors in [176] have designed privacy-preserving FL architecture that protects the data privacy and integrity of the global model. They have used Trusted Execution Environment (TTE) to create a training-integrity protocol to detect causative attacks.
- • Secure Multi-party Computation: It is also known as secure/privacy-preserving computation or Multi-Party Computation (MPC). It was proposed in 1986 by Yao [177] . With SMPC, multiple parties can perform distributed computing tasks in a protected manner. Parties can jointly perform computation tasks on their private input data. A single party cannot learn the personal data of the other party. In SMPC, after computation, each party can only obtain the final output and its inputs [150] . The SMPC protects the model parameters in the FL system before exchanging them to a central server. The FL system only encrypts the model parameters, as there is no need to encrypt all input data [29] . SMPC helps to eliminate the trade-off between privacy and data usability. But still, it is susceptible to inference attacks. Another concern in SMPC implementation is computational overhead that leads to longer training time. However, there exists a trade-off between data privacy and efficiency. In SMPC, the continuous transfer of encrypted and decrypted data between multiple parties may have a higher communication overhead [178] .
3.6. RQ6: The convergence of federated learning and blockchain
Many researchers have integrated blockchain technology and a federated learning approach for different use cases. In Table 8 , we have summarized the relevant research studies that have integrated blockchain and federated learning for various use cases. In [179] , the authors have designed a blockchain-based protocol for secure data sharing in federated learning. They have developed secure communication between the FL client and the FL server. In [180] , the authors have discussed the application of blockchain and FL in vehicular (IoT) networks. Integrating federated learning in vehicular networks makes offloading the trained models to vehicles more secure and reliable. In [181] , the authors have used a blockchain network as a coordinating server to exchange local model updates between devices. In [182] , the authors proposed a Galaxy Federated Learning (GFL) architecture incorporating the Ethereum blockchain and federated learning. In addition, they have developed a ring-decentralized federated learning algorithm to improve network robustness and bandwidth utilization. In recent research [183] , the authors have developed a blockchain-enabled asynchronous FL-based IoT anomaly detection model. This work devised a DP-GAN algorithm (Generative Adversarial Nets) to preserve the model parameter privacy.
Summarization of relevant studies implementing blockchain and federated learning.
3.6.1. Federated learning and blockchain for healthcare scenario
The adoption of federated learning techniques and blockchain in the medical sector carries enormous benefits and promises. These technologies ensure secure storage, exchange, and utilization of health data. In the COVID-19 era, distributing accurate and trustworthy information is very important; for this purpose, few authors applied FL and blockchain to design robust models to share COVID-19 patient information in a privacy-preserving manner [192] , [200] , [201] . In [202] , the authors have proposed a patient-centric blockchain and AI-based model to fight against COVID-19. In [203] , the authors have proposed a blockchain and federated learning framework to collect and share COVID-19 patient data among several hospitals while maintaining data privacy. Few authors focused on designing a trustworthy healthcare model based on FL and blockchain. These architectures focused on the privacy protection of IoHT, i.e. (Internet of Health Things) data [204] , [205] , and designing IoMT solutions [164] . Similarly, in [206] , the authors have proposed a blockchain-empowered FL architecture to enhance fairness in FL tasks and enable accountability. They have presented the data sampler algorithm to increase the model accuracy.
By understanding the enormous potential of these technologies, we have integrated them into the telemedicine system. In Fig. 7 , our proposed architecture shows the merging of blockchain and federated learning for the telemedicine system. This study adopted a private blockchain platform, i.e., Hyperledger Fabric blockchain. In this diagram, hospitals A is primary care clinic, and hospitals B and C are remote specialist hospitals. Hospital C acts as a miner or coordinator hospital. The primary care clinician collects the patient’s health data at the initial level. Health data is stored in a hospital’s database using a proper privacy-preserving mechanism. Patient-centric smart contract logic is applied before sharing data with remote hospitals to achieve secure data transmission. With federated learning, decentralized and collaborative learning is performed. Global models update is shared in a trustworthy and safe manner. By integrating blockchain and federated learning, health data is securely transmitted and stored and can be used to perform effective data analytics. Algorithm 1 illustrates the detailed workflow of our proposed blockchain and FL-based telemedicine system.

The proposed telemedicine architecture integrating blockchain and federated learning. The remote hospitals can access the patient clinical data and global model updates via blockchain.

4. Discussion and future scope
This survey helps to highlight the benefits and issues in the current remote healthcare system. It explores the significance of promising technologies such as blockchain and federated learning to strengthen the remote healthcare system. Compared to the previous studies, this survey discussed several privacy-preserving methods incorporated with blockchain and federated learning to design a privacy-centric telemedicine system. The main objective is to highlight the issues faced while designing patient-centric privacy-preserving telemedicine systems and how blockchain and federated learning have the potential to overcome those issues. This survey discussed the privacy issues/attacks in federated learning and explored the existing privacy-preserving techniques that facilitate achieving potential privacy.
4.1. Summarization of research questions
- • RQ1: In the COVID-19 pandemic, tremendous growth is observed in adopting the telemedicine system. Telemedicine has become the latest efficient way of communicating and accessing healthcare. Despite the advancement in telemedicine, it still faces challenges that need to be handled urgently. Telemedicine is still in its development phase. Unauthorized data access, data privacy, lack of trust, data breach, and lack of a patient-centric approach are the hurdles that need to be considered to integrate telemedicine systems in healthcare fully. There is a need for a trustworthy, robust telemedicine platform that ensures data privacy and easy availability or accessibility of data. In this regard, it is essential to adopt advanced technologies with telemedicine systems to design reliable systems. Blockchain and telemedicine share a mutual vision to develop a decentralized and reliable system. The blockchain facilitates an efficient way to store and share electronic health records in a decentralized manner. Similarly, the adoption of federated learning in telemedicine is a hot research topic. It helps to design a collaborative and accurate diagnosis model that aids in achieving precision medicine. However, the sustained use of blockchain and federated learning in telemedicine systems is still under development. In the future, much more research and the maturation of these technologies will be necessary before the framework can be used securely and safely across the globe.
- • RQ2: Blockchain has several fascinating features that can be valuable for healthcare applications. The decentralized nature of blockchain helps create applications without needing to depend on any centralized authority. Decentralization helps create a transparent healthcare workflow that lets patients know how their health data is shared and accessed in the network. Immutability helps to ensure the validity and integrity of sensitive health records. Traceability, transparency, and data availability are key features of blockchain that enhance its applicability to developing trustworthy healthcare applications. Regarding data privacy, despite the encryption mechanism employed with the blockchain, there is a concern that it is possible in public blockchain to reveal the patient’s identity by linking sufficient information related to that patient. In addition, a privacy leakage can occur in blockchain even though users only perform transactions with their private and public keys. The private keys in the blockchain are also susceptible to potential compromise, resulting in a lack of authorized access to health data. Due to this, patient data privacy is a core concern when integrating blockchain into the healthcare industry. In the future, the challenge of data privacy will be an open research issue to enhance the health stakeholder’s confidence to boost the adoption of blockchain in healthcare applications.
- • RQ3: This study highlights the privacy-related challenges in the blockchain and explores several privacy-preserving mechanisms deployed with blockchain to ensure privacy guarantees. It becomes possible to achieve a strong privacy guarantee by deploying different encryption schemes (e.g., Attribute-based encryption, proxy re-encryption, and identity encryption) and other mechanisms like homomorphic encryption and ZKP with blockchain. Identity-based encryption improves key distribution. In this mechanism, the user’s identity is used to generate a public key; thus, there is no need to obtain a user public key to transfer encrypted data. Proxy Re-encryption is suitable for data access control and is a feasible solution to provide secure access delegation. Attribute-based encryption guarantees fine-grained access policies that help to define authorized users. Blockchain-based smart contracts help to guarantee proper access control. Attribute-based Signature (ABS) benefits attribute-based messaging and anonymous authentication systems. Ring signature is a feasible solution to solve the issues with multiparty computations. Mixing makes it challenging to correlate the transaction history. In addition, some other privacy-preserving mechanisms, such as differential privacy, zero-knowledge proof, and homomorphic encryption, are also deployed with blockchain to provide a strong privacy guarantee. However, no privacy-preserving mechanism is free from vulnerabilities. Each privacy-preserving method has its shortcomings. The drawback of attribute-based encryption is that the data owner must use each authorized user’s public key to encrypt the data. This mechanism is not suitable for some real-world applications. The high cost required for data encryption and decryption is another challenge the encryption schemes face. High computational complexity is the main challenge in adopting zero-knowledge proof and homomorphic encryption. In some use cases, zero-knowledge proof depends on a third party, while homomorphic encryption requires an extended processing time. The challenging issues with smart contracts are contract correctness, designing careful control flow, improving execution efficiency, proliferation, and redeployment of contracts that need to consider for better privacy preservation. Thus, there is a need to consider the current benefits and issues with these privacy-preserving mechanisms before deploying them with blockchain for healthcare applications.
- • RQ4: The main reason behind the widespread adoption of federated learning is that it allows collaborative learning that facilitates efficient machine learning while ensuring legal compliance and privacy between multiple hospitals. FL addresses data privacy and data governance issues that usually exist in health data aggregation. With the FL technique, machine learning models run in a distributed and heterogeneous manner while minimizing the risk of data transfer. Unlike centralized learning, FL has few valuable features, such as ensuring privacy since the hospitals never share their private datasets. It possesses lower latency as hospitals can make predictions locally and less power consumption since models run on local hospital data. Thus, FL offers large datasets to build a better predictive global model through multi-site collaboration and provides easy scalability. Furthermore, minimum resources are required in the FL to aggregate models; thus, deployment becomes more economical. However, the core challenges of FL, such as lack of coordinating side trust, potential privacy, and traceability, must be addressed before adopting FL in the healthcare domain.
- • RQ5: According to the latest research studies, federated learning is susceptible to several privacy threats, such as membership attacks, inference attacks, and poisoning attacks. Several privacy methods were integrated with FL, such as SMPC, Homomorphic encryption, and Differential privacy for potential privacy. Most recent studies primarily focused on differential privacy and SMPC for privacy-preserving FL. Each method might resolve the privacy issues, but they still have shortcomings. For example, differential privacy suffers accuracy issues, and SMPC and HE lead to higher communication and computational overheads. However, these existing privacy-preserving methods, such as Differential privacy, impose lower data utility, Secure Multi-party Computation, impose a high computational overhead, and are susceptible to inference attacks. With these methods, FL achieves strong privacy, but it loses efficiency as well as accuracy. Thus, there is a need to consider the current issues with DP, SMPC, and HE before designing robust FL systems. Therefore, further research is still required to eliminate the trade-off between data privacy and accuracy. Developing a practical FL system with a potential privacy guarantee is still very challenging. In the future, even combining various privacy techniques with blockchain and FL may lead to a potential privacy guarantee. The ultimate goal is to design a secure, efficient, and accurate federated learning system with a privacy guarantee. Privacy-preserving FL is still a challenging research area.
- • RQ6: More recently, blockchain and federated learning technology are independently making a tremendous technological revolution in healthcare industries. The ethical challenges in a medical setting are data privacy, which means preserving patient identity; data transparency means the patient must know how their data are accessed; and consent means patients have the right to control their data. In this regard, blockchain is mainly used in healthcare to facilitate decentralized, secure storage and controlled access to health data. On the other hand, federated learning allows gaining insights from data across isolated hospitals and medical institutes to generate a collaborative model with a privacy guarantee. Integrating blockchain and federated learning is very beneficial in addressing challenges present in the medical context. Furthermore, blockchain ensures auditability and verifiability in federated learning to improve the correctness and efficiency of training procedures. These two technologies are complementary to each other and have the potential to resolve traditional healthcare’s core challenges. With this convergence, it is possible to securely share and analyze data and models in a trustworthy manner. As the convergence of these two technologies is still under development, there is a need further to explore this promising research field in an integrated way. Despite this, federated learning is vulnerable to inference attacks, and it is not easy to achieve strong privacy. Blockchain also fails to achieve a complete privacy guarantee. Blockchain and naïve federated learning systems are susceptible to advanced data privacy threats. Thus, to design a privacy-centric healthcare architecture, it is necessary to incorporate a suitable privacy-preserving mechanism with these technologies to enhance its adaptability in healthcare. However, blockchain and federated learning offer an initial level of privacy, but it offers a stronger privacy guarantee in combination with various privacy methods. In the near future, there is a need to explore these two emerging technologies to produce reliable modern healthcare services to improve care quality. The exciting future research topic is integrating federated learning and blockchain technology with appropriate privacy-preserving mechanisms in telemedicine systems. This integration helps develop a cost-effective, reliable, and trustworthy decision-making platform. Furthermore, designing a blockchain-based federated learning framework is a promising way to boost the overall standard of healthcare.
4.2. Limitation
Our SLR has recognized a few limitations. First, this SLR analyzes the research studies published in IEEE Xplore, Web of Science, Science Direct, ArXiv, and Scopus, which may result in the incompleteness of relevant literature. However, as stated earlier, we only focused on journal articles and conference papers, which meant we could not retrieve the more relevant information published as book chapters or lecture notes. As a result, this study may miss a few relevant research works that have not been searched in the grey literature.
5. Conclusion
The COVID-19 pandemic has taught us a few very important lessons in a hard way. On one side, it has exposed vulnerabilities of the existing healthcare systems; on the other side, it has also shown the potential of available technologies, such as telemedicine, to rapidly achieve universal healthcare coverage that too at a fraction of the cost compared to that of the brick-and-mortar model of healthcare. The use of an online web-based portal CoWIN, to coordinate and administer more than two billion vaccine doses by India is one such example, which clearly demonstrates how digital technologies could be game changers for healthcare. This indicates how the digital divide between developed and developing nations is rapidly reducing. It would not be an exaggeration to suggest that the stage has been set for digital technologies to take the next big step in healthcare.
The new digital dawn, however, is not without issues. This new digital age has its own set of problems. Given the sensitive nature of data generated and processed in the healthcare domain, it is crucial to diligently deal with all the issues pretending to be data in this domain. We found from the literature that for effective deployment of telemedicine systems, secure storage, privacy-preservation, and authorized access to health data with the consent of patients are identified as the main data-related challenges. Several approaches have been proposed to solve these issues; however, it is not possible to solve these issues using conventional telemedicine architectures, which were proposed decades ago. Moreover, the rapid rate of technological development and ever-increasing computation power, with quantum computing being just in the corner, also makes it futile to solve these issues using old methods.
Blockchain and Federated learning are emerging technologies and are actively pursued by researchers across the globe. In recent years, both federated learning and blockchain advancements have gained enormous attention, and they have shown to be path-breaking technologies in their own regard. Integrating these two powerful technologies could provide an excellent opportunity to build a highly secure and accurate collaborative model in various domains, especially in healthcare.
In this review, we have provided a systematic and in-depth overview of telemedicine, blockchain, and federated learning in the healthcare domain using well-defined research queries. This review systematically explores the benefits and limitations of blockchain and federated learning. We have also discussed the privacy-preserving issues in blockchain and federated learning and have reviewed the several privacy-preserving methods incorporated with these technologies to design privacy-centric applications. Finally, we have proposed a generic framework for merging the blockchain and federated learning-based approaches for telemedicine-based applications. To summarize, this research survey highlights the future opportunity to integrate blockchain and federated-based technologies with suitable privacy techniques in healthcare to create a highly secure and accurate collaborative model.
Declaration of Competing Interest
The authors declare that they have no known competing financial interests or personal relationships that could have appeared to influence the work reported in this paper.
Data availability
- Introduction
- Conclusions
- Article Information
a The 4 privacy protections were consent, data transparency, oversight, and data deletion.
b Participants rated each scenario on a 5-point Likert scale assessing their willingness to share their information, with 1 indicating least willingness to share and 5 most willingness.
eMethods. Conjoint Survey Instrument
eTable. Main Effects + Second Order Interactions
Data Sharing Statement
See More About
Sign up for emails based on your interests, select your interests.
Customize your JAMA Network experience by selecting one or more topics from the list below.
- Academic Medicine
- Acid Base, Electrolytes, Fluids
- Allergy and Clinical Immunology
- American Indian or Alaska Natives
- Anesthesiology
- Anticoagulation
- Art and Images in Psychiatry
- Artificial Intelligence
- Assisted Reproduction
- Bleeding and Transfusion
- Caring for the Critically Ill Patient
- Challenges in Clinical Electrocardiography
- Climate and Health
- Climate Change
- Clinical Challenge
- Clinical Decision Support
- Clinical Implications of Basic Neuroscience
- Clinical Pharmacy and Pharmacology
- Complementary and Alternative Medicine
- Consensus Statements
- Coronavirus (COVID-19)
- Critical Care Medicine
- Cultural Competency
- Dental Medicine
- Dermatology
- Diabetes and Endocrinology
- Diagnostic Test Interpretation
- Drug Development
- Electronic Health Records
- Emergency Medicine
- End of Life, Hospice, Palliative Care
- Environmental Health
- Equity, Diversity, and Inclusion
- Facial Plastic Surgery
- Gastroenterology and Hepatology
- Genetics and Genomics
- Genomics and Precision Health
- Global Health
- Guide to Statistics and Methods
- Hair Disorders
- Health Care Delivery Models
- Health Care Economics, Insurance, Payment
- Health Care Quality
- Health Care Reform
- Health Care Safety
- Health Care Workforce
- Health Disparities
- Health Inequities
- Health Policy
- Health Systems Science
- History of Medicine
- Hypertension
- Images in Neurology
- Implementation Science
- Infectious Diseases
- Innovations in Health Care Delivery
- JAMA Infographic
- Law and Medicine
- Leading Change
- Less is More
- LGBTQIA Medicine
- Lifestyle Behaviors
- Medical Coding
- Medical Devices and Equipment
- Medical Education
- Medical Education and Training
- Medical Journals and Publishing
- Mobile Health and Telemedicine
- Narrative Medicine
- Neuroscience and Psychiatry
- Notable Notes
- Nutrition, Obesity, Exercise
- Obstetrics and Gynecology
- Occupational Health
- Ophthalmology
- Orthopedics
- Otolaryngology
- Pain Medicine
- Palliative Care
- Pathology and Laboratory Medicine
- Patient Care
- Patient Information
- Performance Improvement
- Performance Measures
- Perioperative Care and Consultation
- Pharmacoeconomics
- Pharmacoepidemiology
- Pharmacogenetics
- Pharmacy and Clinical Pharmacology
- Physical Medicine and Rehabilitation
- Physical Therapy
- Physician Leadership
- Population Health
- Primary Care
- Professional Well-being
- Professionalism
- Psychiatry and Behavioral Health
- Public Health
- Pulmonary Medicine
- Regulatory Agencies
- Reproductive Health
- Research, Methods, Statistics
- Resuscitation
- Rheumatology
- Risk Management
- Scientific Discovery and the Future of Medicine
- Shared Decision Making and Communication
- Sleep Medicine
- Sports Medicine
- Stem Cell Transplantation
- Substance Use and Addiction Medicine
- Surgical Innovation
- Surgical Pearls
- Teachable Moment
- Technology and Finance
- The Art of JAMA
- The Arts and Medicine
- The Rational Clinical Examination
- Tobacco and e-Cigarettes
- Translational Medicine
- Trauma and Injury
- Treatment Adherence
- Ultrasonography
- Users' Guide to the Medical Literature
- Vaccination
- Venous Thromboembolism
- Veterans Health
- Women's Health
- Workflow and Process
- Wound Care, Infection, Healing
Get the latest research based on your areas of interest.
Others also liked.
- Download PDF
- X Facebook More LinkedIn
Gupta R , Iyengar R , Sharma M, et al. Consumer Views on Privacy Protections and Sharing of Personal Digital Health Information. JAMA Netw Open. 2023;6(3):e231305. doi:10.1001/jamanetworkopen.2023.1305
Manage citations:
© 2024
- Permissions
Consumer Views on Privacy Protections and Sharing of Personal Digital Health Information
- 1 Johns Hopkins University School of Medicine, Baltimore, Maryland
- 2 Hopkins Business of Health Initiative, Johns Hopkins University, Baltimore, Maryland
- 3 Center for Health Services and Outcomes Research, Johns Hopkins Bloomberg School of Public Health, Baltimore, Maryland
- 4 Wharton School, University of Pennsylvania, Philadelphia
- 5 Perelman School of Medicine, Division of General Internal Medicine, Department of Medicine, University of Pennsylvania, Philadelphia
- 6 Perelman School of Medicine, Department of Family Medicine and Community Health, University of Pennsylvania, Philadelphia
- 7 Leonard Davis Institute of Health Economics, University of Pennsylvania, Philadelphia
- 8 Perelman School of Medicine, Department of Emergency Medicine, University of Pennsylvania, Philadelphia
- 9 Perelman School of Medicine, Department of Biostatistics, Epidemiology and Informatics, University of Pennsylvania, Philadelphia
Question Are privacy protections, including consent, transparency of collected data with the consumer, regulatory oversight over data use, and ability to delete data, associated with consumers’ willingness to share their digital health information?
Findings In this survey study of 3539 US adults, conjoint analyses revealed that a combination of privacy protections including consent, consumer access to data collected from them, ethical and regulatory oversight, and ability to delete data together were associated with higher consumer willingness to share their digital health data.
Meaning Results of this study suggest that strengthening consent as a primary privacy protection and adding protections including data transparency, regulatory oversight, and ability to delete data may increase consumer trust and thereby support socially beneficial uses of digital health data.
Importance Digital health information has many potential health applications, but privacy is a growing concern among consumers and policy makers. Consent alone is increasingly seen as inadequate to safeguard privacy.
Objective To determine whether different privacy protections are associated with consumers’ willingness to share their digital health information for research, marketing, or clinical uses.
Design, Setting, and Participants This 2020 national survey with an embedded conjoint experiment recruited US adults from a nationally representative sample with oversampling of Black and Hispanic individuals. Willingness to share digital information across 192 different scenarios reflecting the product of 4 possible privacy protections, 3 uses of information, 2 users of information, and 2 sources of digital information was evaluated. Each participant was randomly assigned 9 scenarios. The survey was administrated between July 10 and July 31, 2020, in Spanish and English. Analysis for this study was conducted between May 2021 and July 2022.
Main Outcomes and Measures Participants rated each conjoint profile on a 5-point Likert scale measuring their willingness to share their personal digital information (with 5 indicating the most willingness to share). Results are reported as adjusted mean differences.
Results Of the 6284 potential participants, 3539 (56%) responded to the conjoint scenarios. A total of 1858 participants (53%) were female, 758 (21%) identified as Black, 833 (24%) identified as Hispanic, 1149 (33%) had an annual income less than $50 000, and 1274 (36%) were 60 years or older. Participants were more willing to share health information with the presence of each individual privacy protection, including consent (difference, 0.32; 95% CI, 0.29-0.35; P < .001), followed by data deletion (difference, 0.16; 95% CI, 0.13-0.18; P < .001), oversight (difference, 0.13; 95% CI, 0.10-0.15; P < .001), and transparency of data collected (difference, 0.08; 95% CI, 0.05-0.10; P < .001). The relative importance (importance weight on a 0%-100% scale) was greatest for the purpose of use (29.9%) but when considered collectively, the 4 privacy protections together were the most important (51.5%) factor in the conjoint experiment. When the 4 privacy protections were considered separately, consent was the most important (23.9%).
Conclusions and Relevance In this survey study of a nationally representative sample of US adults, consumers’ willingness to share personal digital health information for health purposes was associated with the presence of specific privacy protections beyond consent alone. Additional protections, including data transparency, oversight, and data deletion may strengthen consumer confidence in sharing their personal digital health information.
Interactions with the health care system and use of wearable devices, social media, telephone apps, and retail generate vast amounts of digital data reflecting personal health. These data can lead to meaningful social benefits, such as identifying individuals’ mental health concerns through social media, 1 - 3 building algorithms to estimate risk of developing conditions such as dementia 4 and cardiovascular disease, 5 and tracking COVID-19 infections. 6 The growing collection of digital health information and blurred lines between health and nonhealth data also raise privacy and security concerns that are in tension with benefits. The Supreme Court’s decision in Dobbs v Jackson Women’s Health , 7 for example, has elevated concerns that digital health information from menstrual period tracker apps and website purchases may reveal sensitive reproductive health data. 8 The 1996 Health Insurance Portability and Accountability Act (HIPAA) offers privacy protections only for health data and for certain health entities, which excludes most internet data and large technology firms. 9 , 10
Protection of consumer privacy has relied heavily on a model of consent. Prior literature 11 , 12 has demonstrated the shortcomings of consent in research protocols and clinical care given the complexity of understanding required from patients to make informed decisions, particularly with the growing involvement of large technology companies. 13 Several reasons explain this inadequacy, including the inability to estimate future uses of data at the time of collection; dense and convoluted company privacy policies that can change without consumer notice; abrogation of companies’ responsibility after attaining consent; and shifting of an impossible burden onto individuals to understand the policies, make choices, and oversee the continued use of their personal data. 14 In other cases, such as health data sharing with third parties, consent may be absent altogether. 15
The proliferation of health data from consumer digital interactions and sophisticated data science methods thus requires new approaches to health information privacy beyond consent. 13 , 16 , 17 To our knowledge, no studies have systematically examined how privacy protections increase consumer willingness to share their digital health information. We studied a nationally representative population to determine consumer perceptions of the relative importance of specific privacy protections derived from the fair information practice principles and approaches in other nations, 18 - 20 including consent, data transparency, regulatory oversight, and ability to delete previously collected personal data in various uses of digital health data.
We used the web-enabled Ipsos KnowledgePanel to recruit participants for this cross-sectional survey study, as previously described. 21 Ipsos is a probability-based panel designed to be representative of the US population, with participants recruited using address-based sampling methods. 22 At the time of joining the panel, participants were asked to complete a general informed consent process followed by participants self-reporting key demographic characteristics including race and ethnicity using the US Census Bureau categories. We assessed race and ethnicity in this study given known racial and ethnic differences in concerns about privacy and historical distrust in biomedical research, 23 - 25 with oversampling of Black and Hispanic individuals. We also ascertained participants’ political ideology, given that political views have been associated with trust in various uses of consumer digital data. 26
The survey was administrated between July 10 and July 31, 2020, in Spanish and English. Analysis for this study was conducted between May 2021 and July 2022. All data received by the study team were deidentified. This study was reviewed and deemed exempt from the need for informed consent by the University of Pennsylvania Institutional Review Board based on the minimal risk of the research and use of deidentified data. This study followed reporting guidelines and ethical conducts of public opinion as well as survey research as defined by American Association for Public Opinion Research ( AAPOR ) reporting guideline.
We used conjoint analysis to measure consumer willingness to share their digital health information. Conjoint analysis is widely used in marketing to assess consumer preferences. 27 Participants rate descriptions of items or circumstances that vary along established dimensions felt to be important (eg, color, price, quality) and statistical models are used to identify the relative contributions of each dimension to the overall rating. In our context, we evaluated 4 digital information use attributes in the scenarios: the information being used (information type), who is using it (user), the purpose of use (use), and the privacy protections present (privacy protection). The experimental design included 192 possible scenarios reflecting a full factorial design of 2 users, 2 information types, 3 uses, and the absence or presence of 4 different privacy protections ( Table 1 ). The survey instrument was adapted from a prior instrument using conjoint analysis to assess consumer privacy preferences (eMethods in Supplement 1 ). 28
The conjoint attributes and levels were selected based on qualitative interviews with consumers and subject matter experts. 29 , 30 We conducted cognitive interviews 31 to evaluate the survey instrument for clarity and participant comprehension prior to administration. Participants were asked to evaluate 9 scenarios (ie, profiles) randomly selected from the 192 total. The scenarios were presented in the context of diabetes care, meaning that all scenarios reflected reusing data for the purposes of reducing the risk of diabetes. Participants rated each scenario on a 5-point Likert scale assessing their willingness to share their information of 1 (definitely would share) to 5 (definitely would not share). We reversed the scale in analyses, with 1 indicating least willingness to share and 5 most willingness to ease interpretation.
Scenarios were constructed using 2 different information types chosen to reflect those relevant for consumers’ health: information about places people visit from apps or software on their telephone and health information from electronic health records. There were 2 different users of the participant’s data: a university hospital and a digital technology company. The 3 possible uses of data included research (published results in a medical journal to help doctors improve diabetes care), clinical (help patients improve their diabetes care), and marketing (develop a marketing campaign to double the number of people taking a diabetes medication).
The scenarios included 4 different privacy protections based on the fair information practice principles originating from 1967 work by Westin 18 and refined by the Federal Trade Commission in 2000 reflecting consumers’ preferences on the most important protections. 19 The first was whether the person was asked permission for their data to be used (consent). The other nonconsent privacy protections included whether people could view the data collected from them (transparency), a group of experts determined that personal privacy would be well-protected (oversight), and people could request that their data be erased at any time (deletion).
Conjoint analysis uses information on how consumers assess trade-offs across attributes to determine the attributes’ relative importance, termed part-worths. In this study, the part-worth utilities for each level of each conjoint attribute were computed using a generalized estimating equation model to account for correlation of responses within participants, under a gaussian distribution and identity link and assuming an independent working correlation structure with robust, empirical standard errors. In these models, positive differences represent more favored levels and negative differences represent less favored levels relative to a baseline level for each attribute. For each attribute, the difference between the maximum and minimum part-worth utilities reflects how important that attribute is in determining the profiles’ attractiveness. Each attribute’s range is normalized by the sum of the ranges across attributes to allow a comparison of the importance across attributes.
Poststratification weights provided by Ipsos were used across the participant sample to account for differential rates of nonresponse and oversampling to reflect the US population. All statistical tests were 2-tailed and with a significance level of .05.
The main attribute of interest was the relative importance of each privacy protection in consumers’ willingness to share their digital health information for additional uses. We conducted additional analyses for second order interactions between combinations of each privacy protection. For 16 representative conjoint scenarios, we determined part-worth utilities. We also assessed interaction effect sizes between each privacy protection and consumer sociodemographic characteristics including race and ethnicity, political ideology, household income, and age. We used Stata version 16 (StataCorp LP) to conduct all analyses.
Of the 6284 potential participants, 3539 (56%) responded; a total of 1858 participants (53%) were female, 758 (21%) identified as Black, 833 (24%) identified as Hispanic, 1149 (33%) had an annual income less than $50 000, and 1274 (36%) were 60 years or older ( Table 2 ). The participant political ideologies were nearly evenly split among liberal, moderate, and conservative points of view.
Table 3 presents main interaction effect sizes results from the conjoint experiment. Model coefficients represent differences in consumers’ willingness to share their digital health information. The relative importance (importance weight on a 0%-100% scale) was greatest for the purpose of use (29.9%) but when considered collectively, the 4 privacy protections together were the most important (51.5%). When privacy protections were considered separately, consent was the most important among the 4 protections (23.9%).
Participants were most willing to share health information with the presence of each individual privacy protection, including consent (difference, 0.32; 95% CI, 0.29-0.35; P < .001), followed by data deletion (difference, 0.16; 95% CI, 0.13-0.18; P < .001), regulatory oversight (difference, 0.13; 95% CI, 0.10-0.15; P < .001), and data transparency (difference, 0.08; 95% CI, 0.05-0.10; P < .001). We tested second-order interactions between privacy protections, which were generally not significant or small with negative effect sizes (−0.06 or less) with the exception of consent and data deletion (difference, −0.10; 95% CI, −0.15 to −0.05; P < .001) (eTable in Supplement 1 ). Among 16 representative scenarios, compared with a baseline of data being used by university hospitals for research purposes in the absence of any privacy protections, the greatest willingness to share digital health information was when data were used by university hospitals for research purposes in the presence of all 4 privacy protections (3.81; 95% CI, 3.76-3.87; P < .001) ( Figure ). The lowest willingness to share was when the data were used by digital technology companies for marketing purposes in the absence of any privacy protections (2.56; 95% CI, 2.51-2.60; P < .001).
In comparison with information about places visited from telephone apps, participants were slightly more willing to share health information from personal electronic health records (difference, 0.08; 95% CI, 0.05-0.10; P < .001). Compared with a university hospital, participants were less willing to share health information with digital technology companies (difference, −0.17; 95% CI, −0.20 to −0.14; P < .001). Relative to digital health information being used for diabetes care research, participants were less willing to share health information for clinical purposes (difference, −0.09; 95% CI, −0.12 to −0.06; P < .001) and even less willing for marketing purposes to increase prescriptions of a diabetes medication (difference, −0.40; 95% CI, −0.44 to −0.37; P < .001).
In the main interaction effect size model, there were no differences between Black and White respondents in willingness to share health information. Compared with Non-Hispanic respondents, Hispanic respondents were more willing to share health information (difference, 0.12; 95% CI, 0.04-0.19; P = .001). Willingness to share health information decreased as age increased. Compared with respondents who self-identified as being liberal, those who self-identified as conservative were less willing to share health information (difference, −0.26; 95% CI, −0.33 to −0.19; P < .001). A single model was used to test interactions of each privacy protection and demographic characteristics including race and ethnicity, political ideology, household income, and age ( Table 4 ). Interactions between each privacy protection and demographic characteristics were generally nonsignificant, though requiring consent was a greater factor for non-Hispanic respondents and those earning greater than $100 000 in their willingness to share health information (differences, 0.13 [95% CI, 0.06-0.20] and 0.18 [95% CI, 0.09-0.28], respectively).
This study has 3 main findings. First, consumers’ willingness to share personal health information varied considerably by contextual factors. The purpose of data use mattered most to consumers compared with any single privacy protection. Compared with research uses of their information, consumers were less willing to share data for clinical purposes and even less so for marketing purposes. Consumers seemed to be less sensitive to the particular entity using the data although less willing to share data with digital technology companies compared with university hospitals. These findings confirm prior literature on the importance of contextual factors and consumers’ preference for sharing health data for research purposes and clinicians. 32 - 35
Second, consumers viewed consent as the most important privacy protection. The central role of consent may reflect the value placed by consumers on preserving autonomy and the ability to choose whether and how their personal data are used. 36 These results affirm the importance of establishing consent as a baseline model of promoting digital data privacy. Moreover, each of the nonconsent protections, including ability to delete data, regulatory oversight, and transparency, was associated with an increased willingness to share data. Whereas none of the nonconsent protections were individually more important than consent, together the nonconsent protections were at least as important as consent alone, suggesting that a combination of consent with all the other nonconsent protections may maximize utility and consumer willingness to share their data. We did not find any evidence that combining protections was more than additive in their association with consumer utility. These results are consistent with a prior study 37 that found fewer consumer privacy concerns when protections were viewed as being stronger. The present findings add to this literature by providing evidence on the relative importance of specific privacy protections beyond consent.
Third, willingness to share differed by sociodemographic characteristics. However, the importance of privacy protections varied little across subgroups. Overall, older and more conservative respondents were less willing to share health data. These findings are consistent with prior research 23 demonstrating that older individuals are less likely to feel they have control over their digital information and less likely to believe they benefit from data governments collect from them. In contrast, political ideology has varying associations with digital privacy views and appears to be issue-dependent. 26 For instance, people with conservative views expressed greater support relative to people with liberal views for domestic security purposes 38 but were less supportive of using digital tools to reduce COVID-19 transmission. 21 , 39 In addition, we found that although willingness to share was consistent across different racial groups, consent was more important to high-income White respondents. In addition, Hispanic compared with non-Hispanic respondents were more willing to share health data. In a prior study, 40 minority groups, including Hispanic adults, have expressed greater concern about online privacy and security, but also have reported greater control over their health information from the internet relative to White respondents. 23 Differences by ethnicity in the present findings may be due to the specific conjoint scenario, though further exploration is needed to better understand the reasons.
A key finding from this study, that many consumers would rather not share their digital health information when privacy protections are lacking but are more willing to share when more comprehensive privacy protections are established, points to the need to update and fill gaps in US privacy law. For example, the recent US Department of Health and Human Services guidance to protect patient privacy given the Dobbs v Jackson Women’s Health decision continues to rely on an outdated HIPAA framework without acknowledging the sensitive data generated from non–health related sources and without mentioning privacy protections. 41 Ensuring comprehensive privacy protections may also benefit the users of the digital data by addressing consumers’ concerns and encouraging further data sharing. Although the European Union enacted digital privacy regulations in 2018 and in 2021 encompassing health and consumer digital information, the US has not. The California Consumer Privacy Act of 2020 42 strengthened some aspects of consent, including allowing consumers the ability to opt out of data uses, but it continues to rely primarily on consent as a privacy protection.
Notably, consumers’ preferences in this study showing a low willingness to share digital health information for marketing purposes contrasts with their actual behavior as they frequently click through companies’ privacy agreements with limited privacy protections. 14 Several potential reasons explain this contradiction, including that the agreements are often cumbersome to read and privacy protections difficult to understand; the desired product or service is more appealing than the potentially lost privacy; and the responses in our study based on hypothetical scenarios may not be trustworthy. There is also evidence that consumers care about their data privacy but simultaneously carry a sense of resignation about their control over use of their data. 43 The reasons for these differences require more investigation, though the inconsistency also points to the need for rectifying the current model of obtaining consent and strengthening privacy protections.
Given the growing complexities of data sharing, unpredictable future uses of data, and the infeasibility of repeatedly acquiring consent for new uses, one approach to protecting consumer privacy is to implement a combination of individualized and early consent with collective and ongoing governance. 44 , 45 Such a model would reduce individual burden while maintaining protections. Moreover, transparency and comprehensibility must apply both to the specific data being shared as well as how the data were collected and used. 16 Ensuring a frictionless and efficient combination of privacy protections is vital to affirming cross-sectoral protection of consumer data, advancing regulation to meet twenty-first century needs, and leading to social progress by continually learning from data generated in a responsible manner.
This study has limitations. First, we included only 4 privacy protections, and others may be important to consumers. However, the inclusion of these specific protections was based on well-established privacy principles. 18 Second, we did not ascertain whether respondents had a history of diabetes or provided care for someone with diabetes, which may affect their perceptions of data use for diabetes care. Third, our findings rely on ratings of hypothetical scenarios vs actual decisions, which may have resulted in different responses. However, conjoint analysis is a rigorous and well-established approach to measure preferences and individuals’ assessments of trade-offs and to estimate consumer decisions. 46 , 47 Fourth, this study uses a cross-sectional design, and thus the findings reflect a particular moment in time when the survey was conducted in July 2020. The increase in use of digital platforms to reduce public harm from COVID-19, for example, may have affected findings. Fifth, similar to all survey findings, there may be important differences between responders and nonresponders. However, our survey had a relatively high response rate, and the conjoint experimental design allows for strong internal validity.
In this national survey study using conjoint analysis, consumers’ willingness to share personal digital health information for health purposes was associated with the presence of specific privacy protections beyond consent alone. Additional protections, including data transparency, oversight, and data deletion may strengthen consumer trust and support socially beneficial uses of digital health data.
Accepted for Publication: January 17, 2023.
Published: March 2, 2023. doi:10.1001/jamanetworkopen.2023.1305
Open Access: This is an open access article distributed under the terms of the CC-BY License . © 2023 Gupta R et al. JAMA Network Open .
Corresponding Author: Ravi Gupta, MD, Assistant Professor of Medicine, Johns Hopkins University School of Medicine, 1830 E Monument St, Baltimore, MD 21205 ( [email protected] ).
Author Contributions: Drs Grande and Gupta had full access to all of the data in this study and take responsibility for the integrity of the data and the accuracy of the data analysis.
Concept and design: Gupta, Iyengar, Cannuscio, Asch, Grande.
Acquisition, analysis, or interpretation of data: Gupta, Iyengar, Sharma, Cannuscio, Merchant, Mitra, Grande.
Drafting of the manuscript: Gupta, Iyengar, Sharma.
Critical revision of the manuscript for important intellectual content: Iyengar, Cannuscio, Merchant, Asch, Mitra, Grande.
Statistical analysis: Gupta, Iyengar, Mitra, Grande.
Obtained funding: Cannuscio, Grande.
Administrative, technical, or material support: Sharma, Merchant, Grande.
Supervision: Grande.
Conflict of Interest Disclosures: Dr Sharma reported receiving grants from the National Institutes of Health (NIH) during the conduct of the study. Dr Cannuscio reported receiving grants from NIH R01 HG009655-01 during the conduct of the study. Dr Merchant reported receiving grants from NIH K24 HL157621 and NIH R01 NHLBI 141844 141844 during the conduct of the study. Dr Asch reported receiving grants from NIH during the conduct of the study; personal fees from VAL Health; and being a partner and part owner of VAL Health outside the submitted work. Dr Grande reported receiving grants from NIH during the conduct of the study. No other disclosures were reported.
Funding/Support: This research was supported by the National Human Genome Research Institute/NIH (5R01HG009655-04).
Role of the Funder/Sponsor: The funding organization had no role in the design and conduct of the study; collection, management, analysis, and interpretation of the data; preparation, review, or approval of the manuscript; and decision to submit the manuscript for publication.
Data Sharing Statement: See Supplement 2 .
- Register for email alerts with links to free full-text articles
- Access PDFs of free articles
- Manage your interests
- Save searches and receive search alerts
The University of Chicago The Law School
Whose judicial data is it, anyway, in his latest research project, aziz huq focuses on making public data from courts more accessible.
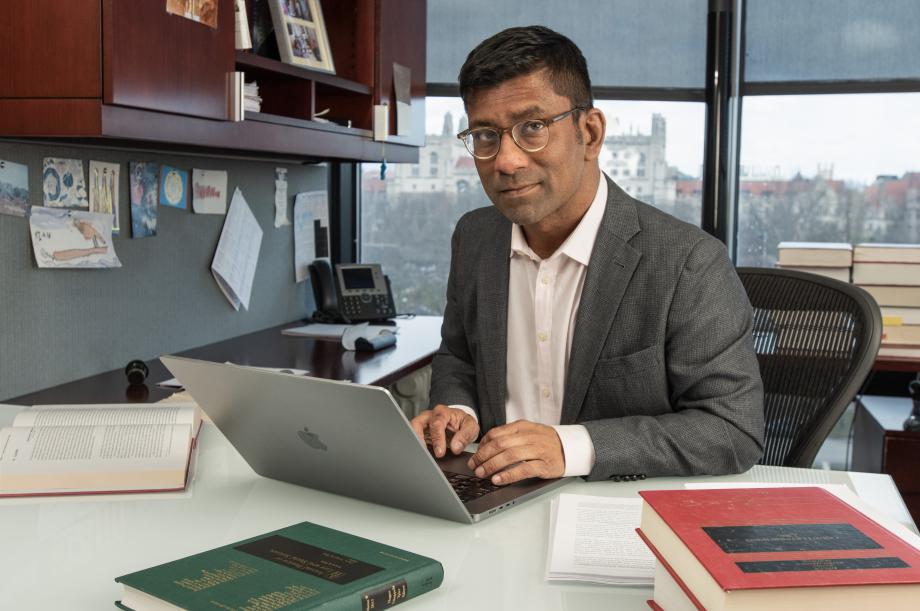
Editor’s Note: This story is the first in an occasional series on research projects currently in the works at the Law School.
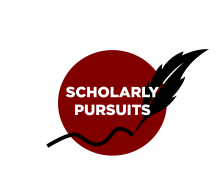
Every court case and judicial proceeding generates an enormous amount of data, some of which is either non-public or difficult to access.
What to do with that data is a question that Aziz Z. Huq, the Frank and Bernice J. Greenberg Professor at the Law School has been pondering lately. Huq is coauthoring a paper with Northwestern Law School Professor (and former Chicago Law School Public Fellow) Zachary D. Clopton that they hope will begin a thoughtful discussion of who should control this judicial data and who should have access to it.
If currently hidden data were made accessible and affordable, Huq explains, attorneys and researchers could use it to help find answers to a wide range of constitutional and public policy questions. For example:
- W hen is the provision of legal counsel effective, unnecessary, or sorely needed?
- When and where is litigation arising and what are the barriers to court access?
- Are judges consistent when they determine in forma pauperis status?
- Do judges ’ sentencing decisions reflect defendants ’ observed race, ethnicity, or gender?
- Are any state and local governments infringing on civil rights though their policing or municipal court systems?
According to Huq and Clopton, judicial data could be used to help clarify the law in ways that advance legality and judicial access, reveal shortfalls in judicial practice, and enable the provision of cheaper and better access to justice.
That potential has increased dramatically with the advent of AI and large language models (LLMs), such as ChatGPT.
“I had been writing about public law and technology, especially AI, for about five years. I became curious recently about why, of all the branches of government, only courts have been left largely to their own devices when it comes to collecting, archiving, and releasing information about its work,” said Huq.
While the legislative and executive branches have an extensive body of constitutional, statutory, and regulatory provisions channeling Congress and executive branch information—and countless public debates about transparency and opacity in and around both elected branches—the federal judiciary still relies on ad hoc procedures to determine what data to collect, preserve, and make available.
As a result, Huq and Clopton believe that “a lot of valuable data is either lost or stored in a way that makes it hard to use for the public good.”
Meanwhile, the authors note that large commercial firms such as Westlaw (owned by the Thomson Reuters Corporation), Lexis (owned by the RELX Group), and Bloomberg are moving to become the de facto data managers and gatekeepers who decide on the public flow of this information and who capture much of its value.
“At minimum, these developments should be the subject of more public discussion and scholarly debate,” said Huq. “Until now, however, one of the biggest obstacles to having that discussion is a lack of information about what data is at stake. It became apparent that we didn’t know why we knew what we knew, and we didn’t know what we didn’t know.”
The Scope of the Data
There were no studies about the full scope and depth of judicial data currently being preserved by the various courts’ disparate procedures—and no certainty about what other data could be preserved if there was a concerted effort to do so.
To fill that gap, Huq and Clopton drew on primary sources and previous scholarship, and then supplement ed that research with anonymized interviews with selected judicial staff and judges.
They quickly discovered that, with no regulatory framework to guide them, institutional practices varied widely among federal courts. Different courts save different types of data, organize it differently, and make different types available to the public.
Even significant judicial data that has been collected is often kept just out of reach. For example, the cover sheets that are filed in every civil case contain a treasure trove of useful information, such as the court’s basis of jurisdiction, the type of relief sought, and the nature of the suit .
“A comprehensive database of civil cover sheets,” the authors write, “would be an extremely valuable source of insight into the timing, cyclicality, substance, and distribution of civil litigation in federal courts.”
Defective Delivery of Data
While federal courts make some data available via the Public Access to Court Electronic Records (PACER) database, that archive is neither comprehensive nor easy to use, and with a 10 cents per page public access fee, expensive, especially for large research projects. Moreover, its search capabilities are limited; PACER does not allow the user to search by judge and does not permit full-text or natural-language searches.
The Federal Judicial Center ’ s Integrated Database suffers from similar defects, as do the courts’ various statistical reports.
Huq and Clopton’s paper demonstrates how these database design choices — kludgy interfaces, limited search options, requiring downloads to proceed page-by-page and at a fee — have the effect of partly privatizing this info by driving the public to commercial firms, who then get to decide what data they want to make available and at what price.
Data Should Be Open, Not Opaque
In the authors’ view, openness and transparency are critical ingredients for making an institution that all Americans would recognize as a true “court.”
“To be clear,” Huq said, “we are not saying the courts must disclose everything. We recognize that there are privacy and other interests at stake and there needs to be some balance and debate around them. But we do believe there are some things we could all agree that the courts could be required to do now. So, our article focuses on that low-hanging fruit and seeks to provoke a conversation rather than partisanship.”
Huq and Clopton’s article will be published this summer by the Stanford Law Review .
Charles Williams is a freelance writer based in South Bend, Indiana.
Help | Advanced Search
Computer Science > Cryptography and Security
Title: homomorphic wisards: efficient weightless neural network training over encrypted data.
Abstract: The widespread application of machine learning algorithms is a matter of increasing concern for the data privacy research community, and many have sought to develop privacy-preserving techniques for it. Among existing approaches, the homomorphic evaluation of ML algorithms stands out by performing operations directly over encrypted data, enabling strong guarantees of confidentiality. The homomorphic evaluation of inference algorithms is practical even for relatively deep Convolution Neural Networks (CNNs). However, training is still a major challenge, with current solutions often resorting to lightweight algorithms that can be unfit for solving more complex problems, such as image recognition. This work introduces the homomorphic evaluation of Wilkie, Stonham, and Aleksander's Recognition Device (WiSARD) and subsequent Weightless Neural Networks (WNNs) for training and inference on encrypted data. Compared to CNNs, WNNs offer better performance with a relatively small accuracy drop. We develop a complete framework for it, including several building blocks that can be of independent interest. Our framework achieves 91.7% accuracy on the MNIST dataset after only 3.5 minutes of encrypted training (multi-threaded), going up to 93.8% in 3.5 hours. For the HAM10000 dataset, we achieve 67.9% accuracy in just 1.5 minutes, going up to 69.9% after 1 hour. Compared to the state of the art on the HE evaluation of CNN training, Glyph (Lou et al., NeurIPS 2020), these results represent a speedup of up to 1200 times with an accuracy loss of at most 5.4%. For HAM10000, we even achieved a 0.65% accuracy improvement while being 60 times faster than Glyph. We also provide solutions for small-scale encrypted training. In a single thread on a desktop machine using less than 200MB of memory, we train over 1000 MNIST images in 12 minutes or over the entire Wisconsin Breast Cancer dataset in just 11 seconds.
Submission history
Access paper:.
- Other Formats

References & Citations
- Google Scholar
- Semantic Scholar
BibTeX formatted citation

Bibliographic and Citation Tools
Code, data and media associated with this article, recommenders and search tools.
- Institution
arXivLabs: experimental projects with community collaborators
arXivLabs is a framework that allows collaborators to develop and share new arXiv features directly on our website.
Both individuals and organizations that work with arXivLabs have embraced and accepted our values of openness, community, excellence, and user data privacy. arXiv is committed to these values and only works with partners that adhere to them.
Have an idea for a project that will add value for arXiv's community? Learn more about arXivLabs .

Personalize Your Experience
Log in or create an account for a personalized experience based on your selected interests.
Already have an account? Log In
Free standard shipping is valid on orders of $45 or more (after promotions and discounts are applied, regular shipping rates do not qualify as part of the $45 or more) shipped to US addresses only. Not valid on previous purchases or when combined with any other promotional offers.
Register for an enhanced, personalized experience.
Receive free access to exclusive content, a personalized homepage based on your interests, and a weekly newsletter with topics of your choice.
Home / Healthy Aging / AI in healthcare: The future of patient care and health management
AI in healthcare: The future of patient care and health management
Curious about artificial intelligence? Whether you're cautious or can't wait, there is a lot to consider when AI is used in a healthcare setting.
Please login to bookmark

With the widespread media coverage in recent months, it’s likely that you’ve heard about artificial intelligence (AI) — technology that enables computers to do things that would otherwise require a human’s brain. In other words, machines can be given access to large amounts of information, and trained to solve problems, spot patterns and make recommendations. Common examples of AI in everyday life are virtual assistants like Alexa and Siri.
What you might not know is that AI has been and is being used for a variety of healthcare applications. Here’s a look at how AI can be helpful in healthcare, and what to watch for as it evolves.
What can AI technology in healthcare do for me?
A report from the National Academy of Medicine identified three potential benefits of AI in healthcare: improving outcomes for both patients and clinical teams, lowering healthcare costs, and benefitting population health.
From preventive screenings to diagnosis and treatment, AI is being used throughout the continuum of care today. Here are two examples:
Preventive care
Cancer screenings that use radiology , like a mammogram or lung cancer screening, can leverage AI to help produce results faster.
For example, in polycystic kidney disease (PKD), researchers discovered that the size of the kidneys — specifically, an attribute known as total kidney volume — correlated with how rapidly kidney function was going to decline in the future.
But assessing total kidney volume, though incredibly informative, involves analyzing dozens of kidney images, one slide after another — a laborious process that can take about 45 minutes per patient. With the innovations developed at the PKD Center at Mayo Clinic, researchers now use artificial intelligence (AI) to automate the process, generating results in a matter of seconds.
Bradley J. Erickson, M.D., Ph.D., director of Mayo Clinic’s Radiology Informatics Lab, says that AI can complete time-consuming or mundane work for radiology professionals , like tracing tumors and structures, or measuring amounts of fat and muscle. “If a computer can do that first pass, that can help us a lot,” says Dr. Erickson.
Risk assessment
In a Mayo Clinic cardiolog y study , AI successfully identified people at risk of left ventricular dysfunction, which is the medical name for a weak heart pump , even though the individuals had no noticeable symptoms. And that’s far from the only intersection of cardiology and AI.
“We have an AI model now that can incidentally say , ‘Hey, you’ve got a lot of coronary artery calcium, and you’re at high risk for a heart attack or a stroke in five or 10 years,’ ” says Bhavik Patel, M.D., M.B.A., the chief artificial intelligence officer at Mayo Clinic in Arizona.
How can AI technology advance medicine and public health?
When it comes to supporting the overall health of a population, AI can help people manage chronic illnesses themselves — think asthma, diabetes and high blood pressure — by connecting certain people with relevant screening and therapy, and reminding them to take steps in their care, such as take medication.
AI also can help promote information on disease prevention online, reaching large numbers of people quickly, and even analyze text on social media to predict outbreaks. Considering the example of a widespread public health crisis, think of how these examples might have supported people during the early stages of COVID-19. For example, a study found that internet searches for terms related to COVID-19 were correlated with actual COVID-19 cases. Here, AI could have been used to predict where an outbreak would happen, and then help officials know how to best communicate and make decisions to help stop the spread.
How can AI solutions assist in providing superior patient care?
You might think that healthcare from a computer isn’t equal to what a human can provide. That’s true in many situations, but it isn’t always the case.
Studies have shown that in some situations, AI can do a more accurate job than humans. For example, AI has done a more accurate job than current pathology methods in predicting who will survive malignant mesothelioma , which is a type of cancer that impacts the internal organs. AI is used to identify colon polyps and has been shown to improve colonoscopy accuracy and diagnose colorectal cancer as accurately as skilled endoscopists can.
In a study of a social media forum, most people asking healthcare questions preferred responses from an AI-powered chatbot over those from physicians, ranking the chatbot’s answers higher in quality and empathy. However, the researchers conducting this study emphasize that their results only suggest the value of such chatbots in answering patients’ questions, and recommend it be followed up with a more convincing study.
How can physicians use AI and machine learning in healthcare?
One of the key things that AI may be able to do to help healthcare professionals is save them time . For example:
- Keeping up with current advances. When physicians are actively participating in caring for people and other clinical duties, it can be challenging for them to keep pace with evolving technological advances that support care. AI can work with huge volumes of information — from medical journals to healthcare records — and highlight the most relevant pieces.
- Taking care of tedious work. When a healthcare professional must complete tasks like writing clinical notes or filling out forms , AI could potentially complete the task faster than traditional methods, even if revision was needed to refine the first pass AI makes.
Despite the potential for AI to save time for healthcare professionals, AI isn’t intended to replace humans . The American Medical Association commonly refers to “augmented intelligence,” which stresses the importance of AI assisting, rather than replacing, healthcare professionals. In the case of current AI applications and technology, healthcare professionals are still needed to provide:
- Clinical context for the algorithms that train AI.
- Accurate and relevant information for AI to analyze.
- Translation of AI findings to be meaningful for patients.
A helpful comparison to reiterate the collaborative nature needed between AI and humans for healthcare is that in most cases, a human pilot is still needed to fly a plane. Although technology has enabled quite a bit of automation in flying today, people are needed to make adjustments, interpret the equipment’s data, and take over in cases of emergency.
What are the drawbacks of AI in healthcare?
Despite the many exciting possibilities for AI in healthcare, there are some risks to weigh:
- If not properly trained, AI can lead to bias and discrimination. For example, if AI is trained on electronic health records, it is building only on people that can access healthcare and is perpetuating any human bias captured within the records.
- AI chatbots can generate medical advice that is misleading or false, which is why there’s a need for effectively regulating their use.
Where can AI solutions take the healthcare industry next?
As AI continues to evolve and play a more prominent role in healthcare, the need for effective regulation and use becomes more critical. That’s why Mayo Clinic is a member of Health AI Partnership, which is focused on helping healthcare organizations evaluate and implement AI effectively, equitably and safely.
In terms of the possibilities for healthcare professionals to further integrate AI, Mark D. Stegall, M.D., a transplant surgeon and researcher at Mayo Clinic in Minnesota says, “I predict AI also will become an important decision-making tool for physicians.”
Mayo Clinic hopes that AI could help create new ways to diagnose, treat, predict, prevent and cure disease. This might be achieved by:
- Selecting and matching patients with the most promising clinical trials.
- Developing and setting up remote health-monitoring devices.
- Detecting currently imperceptible conditions.
- Anticipating disease-risk years in advance.

Relevant reading
Mayo Clinic Guide to Arthritis, Second Edition
If you have joint pain, you know it can lead to frustrating limitations in daily life. In fact, arthritis is the most common cause of disability in the United States. This complex group of joint diseases, which includes osteoarthritis and rheumatoid arthritis among others, affects at least 54 million Americans…

Mayo Clinic Guide to Better Vision, Third Edition
Mayo Clinic Guide to Better Vision, Sophie J. Bakri, M.D., walks readers through the diagnoses and treatment options associated with common eye issues, as well as preventive measures for protecting your eyes.

Discover more Healthy Aging content from articles, podcasts, to videos.
You May Also Enjoy

Privacy Policy
We've made some updates to our Privacy Policy. Please take a moment to review.
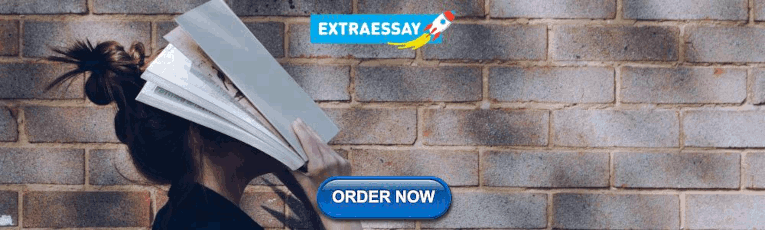
IMAGES
VIDEO
COMMENTS
This chapter explores the concepts and challenges of privacy and data protection in the context of contemporary data-driven economies. It examines the legal frameworks and principles that regulate ...
Five major streams of research inform our work in this paper: (1) technology adoption model (TAM), (2) consumer privacy paradox, (3) service failure, (4) protection motivation theory (PMT), and (5) trust. ... Third, most research on data breaches has focused mainly on post-breach analysis, that is, the impact of data breach. ... Consumer data ...
Data are today an asset more critical than ever for all organizations we may think of. Recent advances and trends, such as sensor systems, IoT, cloud computing, and data analytics, are making possible to pervasively, efficiently, and effectively collect data. However for data to be used to their full power, data security and privacy are critical. Even though data security and privacy have been ...
This paper focuses on privacy and security concerns in Big Data. This paper also covers the encryption techniques by taking existing methods such as differential privacy, k-anonymity, T-closeness, and L-diversity.Several privacy-preserving techniques have been created to safeguard privacy at various phases of a large data life cycle.
Introduction. This paper aims to critically assess the information duties set out in the General Data Protection Regulation (GDPR) and national adaptations when the purpose of processing is scientific research. Due to the peculiarities of the legal regime applicable to the research context, information about the processing plays a crucial role ...
But the same data can also be easily accessed or even hacked when a breach of security occurs. Because of the ubiquity of online data-sharing, most research around privacy concerns has tended to focus on users' attitudes about how their personal information is acquired, stored, and used by companies and organizations (Wang et al., Citation ...
Big data is a term used for very large data sets that have more varied and complex structure. These characteristics usually correlate with additional difficulties in storing, analyzing and applying further procedures or extracting results. Big data analytics is the term used to describe the process of researching massive amounts of complex data in order to reveal hidden patterns or identify ...
This research contributes to marketing theory by applying a structuration theoretical approach to a marketing data privacy context. Structuration theory (Giddens, 1984) overcomes some limitations of prior systems theories that overemphasize the role of either structure or action in social processes and interactions; its theoretical insights instead reflect their interplay.
In this paper, we identify and review key challenges to bridge the knowledge-gap between SME's, companies, organisations, businesses, government institutions and the general public in adopting, promoting and utilising Blockchain technology. The challenges indicated are Cybersecurity and Data privacy in this instance. Additional challenges are set out supported by literature, in researching ...
The relevance of data privacy and security in smart tourism research was further reported in Table 5, which was charted by publication year. Most articles addressed data privacy and security issues as a dimension or variable of their studies without in-depth explanation (n = 23, 60.53%).
Three strategies are summarized in this section. The first is for clinical data and provides a practical user access rating system, and the second is majority for genomic data and designs a network architecture to address both security access and potential risk of privacy disclosure and reidentification.
This issue of the journal is devoted to recent advances in data security, trustworthiness, and privacy that address relevant challenges. The papers, all invited, provide a broad perspective about the variety of researches that can contribute to the development of effective and efficient data protection technology. P.
the privacy of individuals in the digital age has raised national concerns over legal protections of Americans' electronic data. The current legislative paradigms governing cybersecurity and data privacy are complex and technical and lack uniformity at the federal level. This In Focus provides an introduction to data protection laws and an
Collects users' location data. Papers did not discuss its privacy violence clearly but for sure it has been mentioned as one of the applications that has privacy problems. ... A limitation of future research on an ultimate dependent variable is the adoption of COVID-19 applications recommendations for pre- and post-testing in future studies.
In addition, this paper presents recent research trends and open challenges for each component. During the last five years, many survey papers focusing on the security and privacy of e-health data have been published; however, there has been no comprehensive survey of an overall e-healthcare system, such as e-health data, medical devices ...
This paper describes privacy issues in big data analysis and elaborates on two case studies (government-funded projects2,3) in order to elucidate how legal privacy requirements can be met in research projects working on big data and highly sensitive personal information. Finally, it discusses resulted impacts on ...
With the continuous advancement in technology and massive increase in internet usage, the concepts of data privacy and data protection is a hugely debated topic. This is because, the service providers who manage the websites, applications and social media platforms often collect and store user's personal data with the objective of providing ...
National Bureau of Economic Research working paper, 2018. DOI: 10.3386/w24253. Summary: This paper looks at the risks big data poses to consumer privacy. The author describes the causes and consequences of data breaches and the ways in which technological tools can be used for data misuse. She then explores the interaction between privacy risks ...
More recently, the focus of papers has shifted to the broader implications of the growth in "big data" and the rise in analytics and data science. "Market Research and the Ethics of Big Data" (Nunan & Di Domenico, 2013) highlights the many new ethical issues occurring with the growth in big data and weaknesses in existing ethics codes ...
We surveyed 5,101 U.S. adults using Pew Research Center's American Trends Panel to give voice to people's views and experiences on these topics. In addition to the key findings covered on this page, the three chapters of this report provide more detail on: Views of data privacy risks, personal data and digital privacy laws (Chapter 1).
Data infrastructure and AI oversight can assist in multiple goals, including: maintaining data privacy and data integrity; increasing cybersecurity; and guarding against information warfare threats. This Essay concludes that conceptualizing data infrastructure as a form of critical infrastructure can reinforce domestic national security strategies.
Overall, this review includes the relevant research papers to address our key research questions and excludes those that did not fit our research questions. As a result, 158 research studies were selected for further in-depth analysis. ... the challenge of data privacy will be an open research issue to enhance the health stakeholder's ...
Among 16 representative scenarios, compared with a baseline of data being used by university hospitals for research purposes in the absence of any privacy protections, the greatest willingness to share digital health information was when data were used by university hospitals for research purposes in the presence of all 4 privacy protections (3 ...
Editor's Note: This story is the first in an occasional series on research projects currently in the works at the Law School. Every court case and judicial proceeding generates an enormous amount of data, some of which is either non-public or difficult to access. What to do with that data is a question that Aziz Z. Huq, the Frank and Bernice J. Greenberg Professor at the Law School has been ...
The widespread application of machine learning algorithms is a matter of increasing concern for the data privacy research community, and many have sought to develop privacy-preserving techniques for it. Among existing approaches, the homomorphic evaluation of ML algorithms stands out by performing operations directly over encrypted data, enabling strong guarantees of confidentiality. The ...
If you are a Mayo Clinic patient, this could include protected health information. If we combine this information with your protected health information, we will treat all of that information as protected health information and will only use or disclose that information as set forth in our notice of privacy practices.