Thank you for visiting nature.com. You are using a browser version with limited support for CSS. To obtain the best experience, we recommend you use a more up to date browser (or turn off compatibility mode in Internet Explorer). In the meantime, to ensure continued support, we are displaying the site without styles and JavaScript.
- View all journals
- Explore content
- About the journal
- Publish with us
- Sign up for alerts
- Published: 31 January 2022
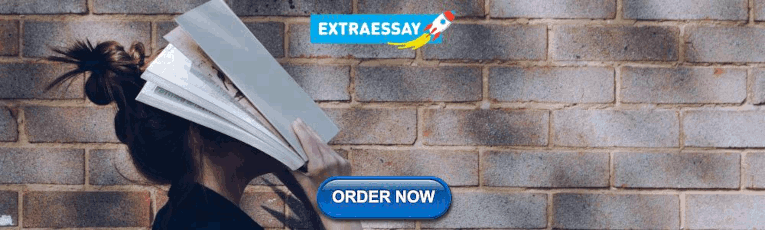
The clinician’s guide to interpreting a regression analysis
- Sofia Bzovsky 1 ,
- Mark R. Phillips ORCID: orcid.org/0000-0003-0923-261X 2 ,
- Robyn H. Guymer ORCID: orcid.org/0000-0002-9441-4356 3 , 4 ,
- Charles C. Wykoff 5 , 6 ,
- Lehana Thabane ORCID: orcid.org/0000-0003-0355-9734 2 , 7 ,
- Mohit Bhandari ORCID: orcid.org/0000-0001-9608-4808 1 , 2 &
- Varun Chaudhary ORCID: orcid.org/0000-0002-9988-4146 1 , 2
on behalf of the R.E.T.I.N.A. study group
Eye volume 36 , pages 1715–1717 ( 2022 ) Cite this article
19k Accesses
9 Citations
1 Altmetric
Metrics details
- Outcomes research
Introduction
When researchers are conducting clinical studies to investigate factors associated with, or treatments for disease and conditions to improve patient care and clinical practice, statistical evaluation of the data is often necessary. Regression analysis is an important statistical method that is commonly used to determine the relationship between several factors and disease outcomes or to identify relevant prognostic factors for diseases [ 1 ].
This editorial will acquaint readers with the basic principles of and an approach to interpreting results from two types of regression analyses widely used in ophthalmology: linear, and logistic regression.
Linear regression analysis
Linear regression is used to quantify a linear relationship or association between a continuous response/outcome variable or dependent variable with at least one independent or explanatory variable by fitting a linear equation to observed data [ 1 ]. The variable that the equation solves for, which is the outcome or response of interest, is called the dependent variable [ 1 ]. The variable that is used to explain the value of the dependent variable is called the predictor, explanatory, or independent variable [ 1 ].
In a linear regression model, the dependent variable must be continuous (e.g. intraocular pressure or visual acuity), whereas, the independent variable may be either continuous (e.g. age), binary (e.g. sex), categorical (e.g. age-related macular degeneration stage or diabetic retinopathy severity scale score), or a combination of these [ 1 ].
When investigating the effect or association of a single independent variable on a continuous dependent variable, this type of analysis is called a simple linear regression [ 2 ]. In many circumstances though, a single independent variable may not be enough to adequately explain the dependent variable. Often it is necessary to control for confounders and in these situations, one can perform a multivariable linear regression to study the effect or association with multiple independent variables on the dependent variable [ 1 , 2 ]. When incorporating numerous independent variables, the regression model estimates the effect or contribution of each independent variable while holding the values of all other independent variables constant [ 3 ].
When interpreting the results of a linear regression, there are a few key outputs for each independent variable included in the model:
Estimated regression coefficient—The estimated regression coefficient indicates the direction and strength of the relationship or association between the independent and dependent variables [ 4 ]. Specifically, the regression coefficient describes the change in the dependent variable for each one-unit change in the independent variable, if continuous [ 4 ]. For instance, if examining the relationship between a continuous predictor variable and intra-ocular pressure (dependent variable), a regression coefficient of 2 means that for every one-unit increase in the predictor, there is a two-unit increase in intra-ocular pressure. If the independent variable is binary or categorical, then the one-unit change represents switching from one category to the reference category [ 4 ]. For instance, if examining the relationship between a binary predictor variable, such as sex, where ‘female’ is set as the reference category, and intra-ocular pressure (dependent variable), a regression coefficient of 2 means that, on average, males have an intra-ocular pressure that is 2 mm Hg higher than females.
Confidence Interval (CI)—The CI, typically set at 95%, is a measure of the precision of the coefficient estimate of the independent variable [ 4 ]. A large CI indicates a low level of precision, whereas a small CI indicates a higher precision [ 5 ].
P value—The p value for the regression coefficient indicates whether the relationship between the independent and dependent variables is statistically significant [ 6 ].
Logistic regression analysis
As with linear regression, logistic regression is used to estimate the association between one or more independent variables with a dependent variable [ 7 ]. However, the distinguishing feature in logistic regression is that the dependent variable (outcome) must be binary (or dichotomous), meaning that the variable can only take two different values or levels, such as ‘1 versus 0’ or ‘yes versus no’ [ 2 , 7 ]. The effect size of predictor variables on the dependent variable is best explained using an odds ratio (OR) [ 2 ]. ORs are used to compare the relative odds of the occurrence of the outcome of interest, given exposure to the variable of interest [ 5 ]. An OR equal to 1 means that the odds of the event in one group are the same as the odds of the event in another group; there is no difference [ 8 ]. An OR > 1 implies that one group has a higher odds of having the event compared with the reference group, whereas an OR < 1 means that one group has a lower odds of having an event compared with the reference group [ 8 ]. When interpreting the results of a logistic regression, the key outputs include the OR, CI, and p-value for each independent variable included in the model.
Clinical example
Sen et al. investigated the association between several factors (independent variables) and visual acuity outcomes (dependent variable) in patients receiving anti-vascular endothelial growth factor therapy for macular oedema (DMO) by means of both linear and logistic regression [ 9 ]. Multivariable linear regression demonstrated that age (Estimate −0.33, 95% CI − 0.48 to −0.19, p < 0.001) was significantly associated with best-corrected visual acuity (BCVA) at 100 weeks at alpha = 0.05 significance level [ 9 ]. The regression coefficient of −0.33 means that the BCVA at 100 weeks decreases by 0.33 with each additional year of older age.
Multivariable logistic regression also demonstrated that age and ellipsoid zone status were statistically significant associated with achieving a BCVA letter score >70 letters at 100 weeks at the alpha = 0.05 significance level. Patients ≥75 years of age were at a decreased odds of achieving a BCVA letter score >70 letters at 100 weeks compared to those <50 years of age, since the OR is less than 1 (OR 0.96, 95% CI 0.94 to 0.98, p = 0.001) [ 9 ]. Similarly, patients between the ages of 50–74 years were also at a decreased odds of achieving a BCVA letter score >70 letters at 100 weeks compared to those <50 years of age, since the OR is less than 1 (OR 0.15, 95% CI 0.04 to 0.48, p = 0.001) [ 9 ]. As well, those with a not intact ellipsoid zone were at a decreased odds of achieving a BCVA letter score >70 letters at 100 weeks compared to those with an intact ellipsoid zone (OR 0.20, 95% CI 0.07 to 0.56; p = 0.002). On the other hand, patients with an ungradable/questionable ellipsoid zone were at an increased odds of achieving a BCVA letter score >70 letters at 100 weeks compared to those with an intact ellipsoid zone, since the OR is greater than 1 (OR 2.26, 95% CI 1.14 to 4.48; p = 0.02) [ 9 ].
The narrower the CI, the more precise the estimate is; and the smaller the p value (relative to alpha = 0.05), the greater the evidence against the null hypothesis of no effect or association.
Simply put, linear and logistic regression are useful tools for appreciating the relationship between predictor/explanatory and outcome variables for continuous and dichotomous outcomes, respectively, that can be applied in clinical practice, such as to gain an understanding of risk factors associated with a disease of interest.
Schneider A, Hommel G, Blettner M. Linear Regression. Anal Dtsch Ärztebl Int. 2010;107:776–82.
Google Scholar
Bender R. Introduction to the use of regression models in epidemiology. In: Verma M, editor. Cancer epidemiology. Methods in molecular biology. Humana Press; 2009:179–95.
Schober P, Vetter TR. Confounding in observational research. Anesth Analg. 2020;130:635.
Article Google Scholar
Schober P, Vetter TR. Linear regression in medical research. Anesth Analg. 2021;132:108–9.
Szumilas M. Explaining odds ratios. J Can Acad Child Adolesc Psychiatry. 2010;19:227–9.
Thiese MS, Ronna B, Ott U. P value interpretations and considerations. J Thorac Dis. 2016;8:E928–31.
Schober P, Vetter TR. Logistic regression in medical research. Anesth Analg. 2021;132:365–6.
Zabor EC, Reddy CA, Tendulkar RD, Patil S. Logistic regression in clinical studies. Int J Radiat Oncol Biol Phys. 2022;112:271–7.
Sen P, Gurudas S, Ramu J, Patrao N, Chandra S, Rasheed R, et al. Predictors of visual acuity outcomes after anti-vascular endothelial growth factor treatment for macular edema secondary to central retinal vein occlusion. Ophthalmol Retin. 2021;5:1115–24.
Download references
R.E.T.I.N.A. study group
Varun Chaudhary 1,2 , Mohit Bhandari 1,2 , Charles C. Wykoff 5,6 , Sobha Sivaprasad 8 , Lehana Thabane 2,7 , Peter Kaiser 9 , David Sarraf 10 , Sophie J. Bakri 11 , Sunir J. Garg 12 , Rishi P. Singh 13,14 , Frank G. Holz 15 , Tien Y. Wong 16,17 , and Robyn H. Guymer 3,4
Author information
Authors and affiliations.
Department of Surgery, McMaster University, Hamilton, ON, Canada
Sofia Bzovsky, Mohit Bhandari & Varun Chaudhary
Department of Health Research Methods, Evidence & Impact, McMaster University, Hamilton, ON, Canada
Mark R. Phillips, Lehana Thabane, Mohit Bhandari & Varun Chaudhary
Centre for Eye Research Australia, Royal Victorian Eye and Ear Hospital, East Melbourne, VIC, Australia
Robyn H. Guymer
Department of Surgery, (Ophthalmology), The University of Melbourne, Melbourne, VIC, Australia
Retina Consultants of Texas (Retina Consultants of America), Houston, TX, USA
Charles C. Wykoff
Blanton Eye Institute, Houston Methodist Hospital, Houston, TX, USA
Biostatistics Unit, St. Joseph’s Healthcare Hamilton, Hamilton, ON, Canada
Lehana Thabane
NIHR Moorfields Biomedical Research Centre, Moorfields Eye Hospital, London, UK
Sobha Sivaprasad
Cole Eye Institute, Cleveland Clinic, Cleveland, OH, USA
Peter Kaiser
Retinal Disorders and Ophthalmic Genetics, Stein Eye Institute, University of California, Los Angeles, CA, USA
David Sarraf
Department of Ophthalmology, Mayo Clinic, Rochester, MN, USA
Sophie J. Bakri
The Retina Service at Wills Eye Hospital, Philadelphia, PA, USA
Sunir J. Garg
Center for Ophthalmic Bioinformatics, Cole Eye Institute, Cleveland Clinic, Cleveland, OH, USA
Rishi P. Singh
Cleveland Clinic Lerner College of Medicine, Cleveland, OH, USA
Department of Ophthalmology, University of Bonn, Bonn, Germany
Frank G. Holz
Singapore Eye Research Institute, Singapore, Singapore
Tien Y. Wong
Singapore National Eye Centre, Duke-NUD Medical School, Singapore, Singapore
You can also search for this author in PubMed Google Scholar
- Varun Chaudhary
- , Mohit Bhandari
- , Charles C. Wykoff
- , Sobha Sivaprasad
- , Lehana Thabane
- , Peter Kaiser
- , David Sarraf
- , Sophie J. Bakri
- , Sunir J. Garg
- , Rishi P. Singh
- , Frank G. Holz
- , Tien Y. Wong
- & Robyn H. Guymer
Contributions
SB was responsible for writing, critical review and feedback on manuscript. MRP was responsible for conception of idea, critical review and feedback on manuscript. RHG was responsible for critical review and feedback on manuscript. CCW was responsible for critical review and feedback on manuscript. LT was responsible for critical review and feedback on manuscript. MB was responsible for conception of idea, critical review and feedback on manuscript. VC was responsible for conception of idea, critical review and feedback on manuscript.
Corresponding author
Correspondence to Varun Chaudhary .
Ethics declarations
Competing interests.
SB: Nothing to disclose. MRP: Nothing to disclose. RHG: Advisory boards: Bayer, Novartis, Apellis, Roche, Genentech Inc.—unrelated to this study. CCW: Consultant: Acuela, Adverum Biotechnologies, Inc, Aerpio, Alimera Sciences, Allegro Ophthalmics, LLC, Allergan, Apellis Pharmaceuticals, Bayer AG, Chengdu Kanghong Pharmaceuticals Group Co, Ltd, Clearside Biomedical, DORC (Dutch Ophthalmic Research Center), EyePoint Pharmaceuticals, Gentech/Roche, GyroscopeTx, IVERIC bio, Kodiak Sciences Inc, Novartis AG, ONL Therapeutics, Oxurion NV, PolyPhotonix, Recens Medical, Regeron Pharmaceuticals, Inc, REGENXBIO Inc, Santen Pharmaceutical Co, Ltd, and Takeda Pharmaceutical Company Limited; Research funds: Adverum Biotechnologies, Inc, Aerie Pharmaceuticals, Inc, Aerpio, Alimera Sciences, Allergan, Apellis Pharmaceuticals, Chengdu Kanghong Pharmaceutical Group Co, Ltd, Clearside Biomedical, Gemini Therapeutics, Genentech/Roche, Graybug Vision, Inc, GyroscopeTx, Ionis Pharmaceuticals, IVERIC bio, Kodiak Sciences Inc, Neurotech LLC, Novartis AG, Opthea, Outlook Therapeutics, Inc, Recens Medical, Regeneron Pharmaceuticals, Inc, REGENXBIO Inc, Samsung Pharm Co, Ltd, Santen Pharmaceutical Co, Ltd, and Xbrane Biopharma AB—unrelated to this study. LT: Nothing to disclose. MB: Research funds: Pendopharm, Bioventus, Acumed—unrelated to this study. VC: Advisory Board Member: Alcon, Roche, Bayer, Novartis; Grants: Bayer, Novartis—unrelated to this study.
Additional information
Publisher’s note Springer Nature remains neutral with regard to jurisdictional claims in published maps and institutional affiliations.
Rights and permissions
Reprints and permissions
About this article
Cite this article.
Bzovsky, S., Phillips, M.R., Guymer, R.H. et al. The clinician’s guide to interpreting a regression analysis. Eye 36 , 1715–1717 (2022). https://doi.org/10.1038/s41433-022-01949-z
Download citation
Received : 08 January 2022
Revised : 17 January 2022
Accepted : 18 January 2022
Published : 31 January 2022
Issue Date : September 2022
DOI : https://doi.org/10.1038/s41433-022-01949-z
Share this article
Anyone you share the following link with will be able to read this content:
Sorry, a shareable link is not currently available for this article.
Provided by the Springer Nature SharedIt content-sharing initiative
This article is cited by
Factors affecting patient satisfaction at a plastic surgery outpatient department at a tertiary centre in south africa.
- Chrysis Sofianos
BMC Health Services Research (2023)
Quick links
- Explore articles by subject
- Guide to authors
- Editorial policies

Click through the PLOS taxonomy to find articles in your field.
For more information about PLOS Subject Areas, click here .
Loading metrics
Open Access
Peer-reviewed
Research Article
Anxiety, Affect, Self-Esteem, and Stress: Mediation and Moderation Effects on Depression
Affiliations Department of Psychology, University of Gothenburg, Gothenburg, Sweden, Network for Empowerment and Well-Being, University of Gothenburg, Gothenburg, Sweden
Affiliation Network for Empowerment and Well-Being, University of Gothenburg, Gothenburg, Sweden
Affiliations Department of Psychology, University of Gothenburg, Gothenburg, Sweden, Network for Empowerment and Well-Being, University of Gothenburg, Gothenburg, Sweden, Department of Psychology, Education and Sport Science, Linneaus University, Kalmar, Sweden
* E-mail: [email protected]
Affiliations Network for Empowerment and Well-Being, University of Gothenburg, Gothenburg, Sweden, Center for Ethics, Law, and Mental Health (CELAM), University of Gothenburg, Gothenburg, Sweden, Institute of Neuroscience and Physiology, The Sahlgrenska Academy, University of Gothenburg, Gothenburg, Sweden
- Ali Al Nima,
- Patricia Rosenberg,
- Trevor Archer,
- Danilo Garcia
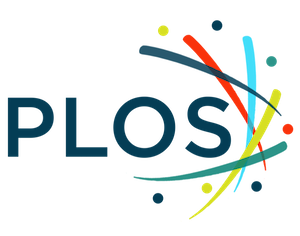
- Published: September 9, 2013
- https://doi.org/10.1371/journal.pone.0073265
- Reader Comments
23 Sep 2013: Nima AA, Rosenberg P, Archer T, Garcia D (2013) Correction: Anxiety, Affect, Self-Esteem, and Stress: Mediation and Moderation Effects on Depression. PLOS ONE 8(9): 10.1371/annotation/49e2c5c8-e8a8-4011-80fc-02c6724b2acc. https://doi.org/10.1371/annotation/49e2c5c8-e8a8-4011-80fc-02c6724b2acc View correction
Mediation analysis investigates whether a variable (i.e., mediator) changes in regard to an independent variable, in turn, affecting a dependent variable. Moderation analysis, on the other hand, investigates whether the statistical interaction between independent variables predict a dependent variable. Although this difference between these two types of analysis is explicit in current literature, there is still confusion with regard to the mediating and moderating effects of different variables on depression. The purpose of this study was to assess the mediating and moderating effects of anxiety, stress, positive affect, and negative affect on depression.
Two hundred and two university students (males = 93, females = 113) completed questionnaires assessing anxiety, stress, self-esteem, positive and negative affect, and depression. Mediation and moderation analyses were conducted using techniques based on standard multiple regression and hierarchical regression analyses.
Main Findings
The results indicated that (i) anxiety partially mediated the effects of both stress and self-esteem upon depression, (ii) that stress partially mediated the effects of anxiety and positive affect upon depression, (iii) that stress completely mediated the effects of self-esteem on depression, and (iv) that there was a significant interaction between stress and negative affect, and between positive affect and negative affect upon depression.
The study highlights different research questions that can be investigated depending on whether researchers decide to use the same variables as mediators and/or moderators.
Citation: Nima AA, Rosenberg P, Archer T, Garcia D (2013) Anxiety, Affect, Self-Esteem, and Stress: Mediation and Moderation Effects on Depression. PLoS ONE 8(9): e73265. https://doi.org/10.1371/journal.pone.0073265
Editor: Ben J. Harrison, The University of Melbourne, Australia
Received: February 21, 2013; Accepted: July 22, 2013; Published: September 9, 2013
Copyright: © 2013 Nima et al. This is an open-access article distributed under the terms of the Creative Commons Attribution License, which permits unrestricted use, distribution, and reproduction in any medium, provided the original author and source are credited.
Funding: The authors have no support or funding to report.
Competing interests: The authors have declared that no competing interests exist.
Introduction
Mediation refers to the covariance relationships among three variables: an independent variable (1), an assumed mediating variable (2), and a dependent variable (3). Mediation analysis investigates whether the mediating variable accounts for a significant amount of the shared variance between the independent and the dependent variables–the mediator changes in regard to the independent variable, in turn, affecting the dependent one [1] , [2] . On the other hand, moderation refers to the examination of the statistical interaction between independent variables in predicting a dependent variable [1] , [3] . In contrast to the mediator, the moderator is not expected to be correlated with both the independent and the dependent variable–Baron and Kenny [1] actually recommend that it is best if the moderator is not correlated with the independent variable and if the moderator is relatively stable, like a demographic variable (e.g., gender, socio-economic status) or a personality trait (e.g., affectivity).
Although both types of analysis lead to different conclusions [3] and the distinction between statistical procedures is part of the current literature [2] , there is still confusion about the use of moderation and mediation analyses using data pertaining to the prediction of depression. There are, for example, contradictions among studies that investigate mediating and moderating effects of anxiety, stress, self-esteem, and affect on depression. Depression, anxiety and stress are suggested to influence individuals' social relations and activities, work, and studies, as well as compromising decision-making and coping strategies [4] , [5] , [6] . Successfully coping with anxiety, depressiveness, and stressful situations may contribute to high levels of self-esteem and self-confidence, in addition increasing well-being, and psychological and physical health [6] . Thus, it is important to disentangle how these variables are related to each other. However, while some researchers perform mediation analysis with some of the variables mentioned here, other researchers conduct moderation analysis with the same variables. Seldom are both moderation and mediation performed on the same dataset. Before disentangling mediation and moderation effects on depression in the current literature, we briefly present the methodology behind the analysis performed in this study.
Mediation and moderation
Baron and Kenny [1] postulated several criteria for the analysis of a mediating effect: a significant correlation between the independent and the dependent variable, the independent variable must be significantly associated with the mediator, the mediator predicts the dependent variable even when the independent variable is controlled for, and the correlation between the independent and the dependent variable must be eliminated or reduced when the mediator is controlled for. All the criteria is then tested using the Sobel test which shows whether indirect effects are significant or not [1] , [7] . A complete mediating effect occurs when the correlation between the independent and the dependent variable are eliminated when the mediator is controlled for [8] . Analyses of mediation can, for example, help researchers to move beyond answering if high levels of stress lead to high levels of depression. With mediation analysis researchers might instead answer how stress is related to depression.
In contrast to mediation, moderation investigates the unique conditions under which two variables are related [3] . The third variable here, the moderator, is not an intermediate variable in the causal sequence from the independent to the dependent variable. For the analysis of moderation effects, the relation between the independent and dependent variable must be different at different levels of the moderator [3] . Moderators are included in the statistical analysis as an interaction term [1] . When analyzing moderating effects the variables should first be centered (i.e., calculating the mean to become 0 and the standard deviation to become 1) in order to avoid problems with multi-colinearity [8] . Moderating effects can be calculated using multiple hierarchical linear regressions whereby main effects are presented in the first step and interactions in the second step [1] . Analysis of moderation, for example, helps researchers to answer when or under which conditions stress is related to depression.
Mediation and moderation effects on depression
Cognitive vulnerability models suggest that maladaptive self-schema mirroring helplessness and low self-esteem explain the development and maintenance of depression (for a review see [9] ). These cognitive vulnerability factors become activated by negative life events or negative moods [10] and are suggested to interact with environmental stressors to increase risk for depression and other emotional disorders [11] , [10] . In this line of thinking, the experience of stress, low self-esteem, and negative emotions can cause depression, but also be used to explain how (i.e., mediation) and under which conditions (i.e., moderation) specific variables influence depression.
Using mediational analyses to investigate how cognitive therapy intervations reduced depression, researchers have showed that the intervention reduced anxiety, which in turn was responsible for 91% of the reduction in depression [12] . In the same study, reductions in depression, by the intervention, accounted only for 6% of the reduction in anxiety. Thus, anxiety seems to affect depression more than depression affects anxiety and, together with stress, is both a cause of and a powerful mediator influencing depression (See also [13] ). Indeed, there are positive relationships between depression, anxiety and stress in different cultures [14] . Moreover, while some studies show that stress (independent variable) increases anxiety (mediator), which in turn increased depression (dependent variable) [14] , other studies show that stress (moderator) interacts with maladaptive self-schemata (dependent variable) to increase depression (independent variable) [15] , [16] .
The present study
In order to illustrate how mediation and moderation can be used to address different research questions we first focus our attention to anxiety and stress as mediators of different variables that earlier have been shown to be related to depression. Secondly, we use all variables to find which of these variables moderate the effects on depression.
The specific aims of the present study were:
- To investigate if anxiety mediated the effect of stress, self-esteem, and affect on depression.
- To investigate if stress mediated the effects of anxiety, self-esteem, and affect on depression.
- To examine moderation effects between anxiety, stress, self-esteem, and affect on depression.
Ethics statement
This research protocol was approved by the Ethics Committee of the University of Gothenburg and written informed consent was obtained from all the study participants.
Participants
The present study was based upon a sample of 206 participants (males = 93, females = 113). All the participants were first year students in different disciplines at two universities in South Sweden. The mean age for the male students was 25.93 years ( SD = 6.66), and 25.30 years ( SD = 5.83) for the female students.
In total, 206 questionnaires were distributed to the students. Together 202 questionnaires were responded to leaving a total dropout of 1.94%. This dropout concerned three sections that the participants chose not to respond to at all, and one section that was completed incorrectly. None of these four questionnaires was included in the analyses.
Instruments
Hospital anxiety and depression scale [17] ..
The Swedish translation of this instrument [18] was used to measure anxiety and depression. The instrument consists of 14 statements (7 of which measure depression and 7 measure anxiety) to which participants are asked to respond grade of agreement on a Likert scale (0 to 3). The utility, reliability and validity of the instrument has been shown in multiple studies (e.g., [19] ).
Perceived Stress Scale [20] .
The Swedish version [21] of this instrument was used to measures individuals' experience of stress. The instrument consist of 14 statements to which participants rate on a Likert scale (0 = never , 4 = very often ). High values indicate that the individual expresses a high degree of stress.
Rosenberg's Self-Esteem Scale [22] .
The Rosenberg's Self-Esteem Scale (Swedish version by Lindwall [23] ) consists of 10 statements focusing on general feelings toward the self. Participants are asked to report grade of agreement in a four-point Likert scale (1 = agree not at all, 4 = agree completely ). This is the most widely used instrument for estimation of self-esteem with high levels of reliability and validity (e.g., [24] , [25] ).
Positive Affect and Negative Affect Schedule [26] .
This is a widely applied instrument for measuring individuals' self-reported mood and feelings. The Swedish version has been used among participants of different ages and occupations (e.g., [27] , [28] , [29] ). The instrument consists of 20 adjectives, 10 positive affect (e.g., proud, strong) and 10 negative affect (e.g., afraid, irritable). The adjectives are rated on a five-point Likert scale (1 = not at all , 5 = very much ). The instrument is a reliable, valid, and effective self-report instrument for estimating these two important and independent aspects of mood [26] .
Questionnaires were distributed to the participants on several different locations within the university, including the library and lecture halls. Participants were asked to complete the questionnaire after being informed about the purpose and duration (10–15 minutes) of the study. Participants were also ensured complete anonymity and informed that they could end their participation whenever they liked.
Correlational analysis
Depression showed positive, significant relationships with anxiety, stress and negative affect. Table 1 presents the correlation coefficients, mean values and standard deviations ( sd ), as well as Cronbach ' s α for all the variables in the study.
- PPT PowerPoint slide
- PNG larger image
- TIFF original image
https://doi.org/10.1371/journal.pone.0073265.t001
Mediation analysis
Regression analyses were performed in order to investigate if anxiety mediated the effect of stress, self-esteem, and affect on depression (aim 1). The first regression showed that stress ( B = .03, 95% CI [.02,.05], β = .36, t = 4.32, p <.001), self-esteem ( B = −.03, 95% CI [−.05, −.01], β = −.24, t = −3.20, p <.001), and positive affect ( B = −.02, 95% CI [−.05, −.01], β = −.19, t = −2.93, p = .004) had each an unique effect on depression. Surprisingly, negative affect did not predict depression ( p = 0.77) and was therefore removed from the mediation model, thus not included in further analysis.
The second regression tested whether stress, self-esteem and positive affect uniquely predicted the mediator (i.e., anxiety). Stress was found to be positively associated ( B = .21, 95% CI [.15,.27], β = .47, t = 7.35, p <.001), whereas self-esteem was negatively associated ( B = −.29, 95% CI [−.38, −.21], β = −.42, t = −6.48, p <.001) to anxiety. Positive affect, however, was not associated to anxiety ( p = .50) and was therefore removed from further analysis.
A hierarchical regression analysis using depression as the outcome variable was performed using stress and self-esteem as predictors in the first step, and anxiety as predictor in the second step. This analysis allows the examination of whether stress and self-esteem predict depression and if this relation is weaken in the presence of anxiety as the mediator. The result indicated that, in the first step, both stress ( B = .04, 95% CI [.03,.05], β = .45, t = 6.43, p <.001) and self-esteem ( B = .04, 95% CI [.03,.05], β = .45, t = 6.43, p <.001) predicted depression. When anxiety (i.e., the mediator) was controlled for predictability was reduced somewhat but was still significant for stress ( B = .03, 95% CI [.02,.04], β = .33, t = 4.29, p <.001) and for self-esteem ( B = −.03, 95% CI [−.05, −.01], β = −.20, t = −2.62, p = .009). Anxiety, as a mediator, predicted depression even when both stress and self-esteem were controlled for ( B = .05, 95% CI [.02,.08], β = .26, t = 3.17, p = .002). Anxiety improved the prediction of depression over-and-above the independent variables (i.e., stress and self-esteem) (Δ R 2 = .03, F (1, 198) = 10.06, p = .002). See Table 2 for the details.
https://doi.org/10.1371/journal.pone.0073265.t002
A Sobel test was conducted to test the mediating criteria and to assess whether indirect effects were significant or not. The result showed that the complete pathway from stress (independent variable) to anxiety (mediator) to depression (dependent variable) was significant ( z = 2.89, p = .003). The complete pathway from self-esteem (independent variable) to anxiety (mediator) to depression (dependent variable) was also significant ( z = 2.82, p = .004). Thus, indicating that anxiety partially mediates the effects of both stress and self-esteem on depression. This result may indicate also that both stress and self-esteem contribute directly to explain the variation in depression and indirectly via experienced level of anxiety (see Figure 1 ).
Changes in Beta weights when the mediator is present are highlighted in red.
https://doi.org/10.1371/journal.pone.0073265.g001
For the second aim, regression analyses were performed in order to test if stress mediated the effect of anxiety, self-esteem, and affect on depression. The first regression showed that anxiety ( B = .07, 95% CI [.04,.10], β = .37, t = 4.57, p <.001), self-esteem ( B = −.02, 95% CI [−.05, −.01], β = −.18, t = −2.23, p = .03), and positive affect ( B = −.03, 95% CI [−.04, −.02], β = −.27, t = −4.35, p <.001) predicted depression independently of each other. Negative affect did not predict depression ( p = 0.74) and was therefore removed from further analysis.
The second regression investigated if anxiety, self-esteem and positive affect uniquely predicted the mediator (i.e., stress). Stress was positively associated to anxiety ( B = 1.01, 95% CI [.75, 1.30], β = .46, t = 7.35, p <.001), negatively associated to self-esteem ( B = −.30, 95% CI [−.50, −.01], β = −.19, t = −2.90, p = .004), and a negatively associated to positive affect ( B = −.33, 95% CI [−.46, −.20], β = −.27, t = −5.02, p <.001).
A hierarchical regression analysis using depression as the outcome and anxiety, self-esteem, and positive affect as the predictors in the first step, and stress as the predictor in the second step, allowed the examination of whether anxiety, self-esteem and positive affect predicted depression and if this association would weaken when stress (i.e., the mediator) was present. In the first step of the regression anxiety ( B = .07, 95% CI [.05,.10], β = .38, t = 5.31, p = .02), self-esteem ( B = −.03, 95% CI [−.05, −.01], β = −.18, t = −2.41, p = .02), and positive affect ( B = −.03, 95% CI [−.04, −.02], β = −.27, t = −4.36, p <.001) significantly explained depression. When stress (i.e., the mediator) was controlled for, predictability was reduced somewhat but was still significant for anxiety ( B = .05, 95% CI [.02,.08], β = .05, t = 4.29, p <.001) and for positive affect ( B = −.02, 95% CI [−.04, −.01], β = −.20, t = −3.16, p = .002), whereas self-esteem did not reach significance ( p < = .08). In the second step, the mediator (i.e., stress) predicted depression even when anxiety, self-esteem, and positive affect were controlled for ( B = .02, 95% CI [.08,.04], β = .25, t = 3.07, p = .002). Stress improved the prediction of depression over-and-above the independent variables (i.e., anxiety, self-esteem and positive affect) (Δ R 2 = .02, F (1, 197) = 9.40, p = .002). See Table 3 for the details.
https://doi.org/10.1371/journal.pone.0073265.t003
Furthermore, the Sobel test indicated that the complete pathways from the independent variables (anxiety: z = 2.81, p = .004; self-esteem: z = 2.05, p = .04; positive affect: z = 2.58, p <.01) to the mediator (i.e., stress), to the outcome (i.e., depression) were significant. These specific results might be explained on the basis that stress partially mediated the effects of both anxiety and positive affect on depression while stress completely mediated the effects of self-esteem on depression. In other words, anxiety and positive affect contributed directly to explain the variation in depression and indirectly via the experienced level of stress. Self-esteem contributed only indirectly via the experienced level of stress to explain the variation in depression. In other words, stress effects on depression originate from “its own power” and explained more of the variation in depression than self-esteem (see Figure 2 ).
https://doi.org/10.1371/journal.pone.0073265.g002
Moderation analysis
Multiple linear regression analyses were used in order to examine moderation effects between anxiety, stress, self-esteem and affect on depression. The analysis indicated that about 52% of the variation in the dependent variable (i.e., depression) could be explained by the main effects and the interaction effects ( R 2 = .55, adjusted R 2 = .51, F (55, 186) = 14.87, p <.001). When the variables (dependent and independent) were standardized, both the standardized regression coefficients beta (β) and the unstandardized regression coefficients beta (B) became the same value with regard to the main effects. Three of the main effects were significant and contributed uniquely to high levels of depression: anxiety ( B = .26, t = 3.12, p = .002), stress ( B = .25, t = 2.86, p = .005), and self-esteem ( B = −.17, t = −2.17, p = .03). The main effect of positive affect was also significant and contributed to low levels of depression ( B = −.16, t = −2.027, p = .02) (see Figure 3 ). Furthermore, the results indicated that two moderator effects were significant. These were the interaction between stress and negative affect ( B = −.28, β = −.39, t = −2.36, p = .02) (see Figure 4 ) and the interaction between positive affect and negative affect ( B = −.21, β = −.29, t = −2.30, p = .02) ( Figure 5 ).
https://doi.org/10.1371/journal.pone.0073265.g003
Low stress and low negative affect leads to lower levels of depression compared to high stress and high negative affect.
https://doi.org/10.1371/journal.pone.0073265.g004
High positive affect and low negative affect lead to lower levels of depression compared to low positive affect and high negative affect.
https://doi.org/10.1371/journal.pone.0073265.g005
The results in the present study show that (i) anxiety partially mediated the effects of both stress and self-esteem on depression, (ii) that stress partially mediated the effects of anxiety and positive affect on depression, (iii) that stress completely mediated the effects of self-esteem on depression, and (iv) that there was a significant interaction between stress and negative affect, and positive affect and negative affect on depression.
Mediating effects
The study suggests that anxiety contributes directly to explaining the variance in depression while stress and self-esteem might contribute directly to explaining the variance in depression and indirectly by increasing feelings of anxiety. Indeed, individuals who experience stress over a long period of time are susceptible to increased anxiety and depression [30] , [31] and previous research shows that high self-esteem seems to buffer against anxiety and depression [32] , [33] . The study also showed that stress partially mediated the effects of both anxiety and positive affect on depression and that stress completely mediated the effects of self-esteem on depression. Anxiety and positive affect contributed directly to explain the variation in depression and indirectly to the experienced level of stress. Self-esteem contributed only indirectly via the experienced level of stress to explain the variation in depression, i.e. stress affects depression on the basis of ‘its own power’ and explains much more of the variation in depressive experiences than self-esteem. In general, individuals who experience low anxiety and frequently experience positive affect seem to experience low stress, which might reduce their levels of depression. Academic stress, for instance, may increase the risk for experiencing depression among students [34] . Although self-esteem did not emerged as an important variable here, under circumstances in which difficulties in life become chronic, some researchers suggest that low self-esteem facilitates the experience of stress [35] .
Moderator effects/interaction effects
The present study showed that the interaction between stress and negative affect and between positive and negative affect influenced self-reported depression symptoms. Moderation effects between stress and negative affect imply that the students experiencing low levels of stress and low negative affect reported lower levels of depression than those who experience high levels of stress and high negative affect. This result confirms earlier findings that underline the strong positive association between negative affect and both stress and depression [36] , [37] . Nevertheless, negative affect by itself did not predicted depression. In this regard, it is important to point out that the absence of positive emotions is a better predictor of morbidity than the presence of negative emotions [38] , [39] . A modification to this statement, as illustrated by the results discussed next, could be that the presence of negative emotions in conjunction with the absence of positive emotions increases morbidity.
The moderating effects between positive and negative affect on the experience of depression imply that the students experiencing high levels of positive affect and low levels of negative affect reported lower levels of depression than those who experience low levels of positive affect and high levels of negative affect. This result fits previous observations indicating that different combinations of these affect dimensions are related to different measures of physical and mental health and well-being, such as, blood pressure, depression, quality of sleep, anxiety, life satisfaction, psychological well-being, and self-regulation [40] – [51] .
Limitations
The result indicated a relatively low mean value for depression ( M = 3.69), perhaps because the studied population was university students. These might limit the generalization power of the results and might also explain why negative affect, commonly associated to depression, was not related to depression in the present study. Moreover, there is a potential influence of single source/single method variance on the findings, especially given the high correlation between all the variables under examination.
Conclusions
The present study highlights different results that could be arrived depending on whether researchers decide to use variables as mediators or moderators. For example, when using meditational analyses, anxiety and stress seem to be important factors that explain how the different variables used here influence depression–increases in anxiety and stress by any other factor seem to lead to increases in depression. In contrast, when moderation analyses were used, the interaction of stress and affect predicted depression and the interaction of both affectivity dimensions (i.e., positive and negative affect) also predicted depression–stress might increase depression under the condition that the individual is high in negative affectivity, in turn, negative affectivity might increase depression under the condition that the individual experiences low positive affectivity.
Acknowledgments
The authors would like to thank the reviewers for their openness and suggestions, which significantly improved the article.
Author Contributions
Conceived and designed the experiments: AAN TA. Performed the experiments: AAN. Analyzed the data: AAN DG. Contributed reagents/materials/analysis tools: AAN TA DG. Wrote the paper: AAN PR TA DG.
- View Article
- Google Scholar
- 3. MacKinnon DP, Luecken LJ (2008) How and for Whom? Mediation and Moderation in Health Psychology. Health Psychol 27 (2 Suppl.): s99–s102.
- 4. Aaroe R (2006) Vinn över din depression [Defeat depression]. Stockholm: Liber.
- 5. Agerberg M (1998) Ut ur mörkret [Out from the Darkness]. Stockholm: Nordstedt.
- 6. Gilbert P (2005) Hantera din depression [Cope with your Depression]. Stockholm: Bokförlaget Prisma.
- 8. Tabachnick BG, Fidell LS (2007) Using Multivariate Statistics, Fifth Edition. Boston: Pearson Education, Inc.
- 10. Beck AT (1967) Depression: Causes and treatment. Philadelphia: University of Pennsylvania Press.
- 21. Eskin M, Parr D (1996) Introducing a Swedish version of an instrument measuring mental stress. Stockholm: Psykologiska institutionen Stockholms Universitet.
- 22. Rosenberg M (1965) Society and the Adolescent Self-Image. Princeton, NJ: Princeton University Press.
- 23. Lindwall M (2011) Självkänsla – Bortom populärpsykologi & enkla sanningar [Self-Esteem – Beyond Popular Psychology and Simple Truths]. Lund:Studentlitteratur.
- 25. Blascovich J, Tomaka J (1991) Measures of self-esteem. In: Robinson JP, Shaver PR, Wrightsman LS (Red.) Measures of personality and social psychological attitudes San Diego: Academic Press. 161–194.
- 30. Eysenck M (Ed.) (2000) Psychology: an integrated approach. New York: Oxford University Press.
- 31. Lazarus RS, Folkman S (1984) Stress, Appraisal, and Coping. New York: Springer.
- 32. Johnson M (2003) Självkänsla och anpassning [Self-esteem and Adaptation]. Lund: Studentlitteratur.
- 33. Cullberg Weston M (2005) Ditt inre centrum – Om självkänsla, självbild och konturen av ditt själv [Your Inner Centre – About Self-esteem, Self-image and the Contours of Yourself]. Stockholm: Natur och Kultur.
- 34. Lindén M (1997) Studentens livssituation. Frihet, sårbarhet, kris och utveckling [Students' Life Situation. Freedom, Vulnerability, Crisis and Development]. Uppsala: Studenthälsan.
- 35. Williams S (1995) Press utan stress ger maximal prestation [Pressure without Stress gives Maximal Performance]. Malmö: Richters förlag.
- 37. Garcia D, Kerekes N, Andersson-Arntén A–C, Archer T (2012) Temperament, Character, and Adolescents' Depressive Symptoms: Focusing on Affect. Depress Res Treat. DOI:10.1155/2012/925372.
- 40. Garcia D, Ghiabi B, Moradi S, Siddiqui A, Archer T (2013) The Happy Personality: A Tale of Two Philosophies. In Morris EF, Jackson M-A editors. Psychology of Personality. New York: Nova Science Publishers. 41–59.
- 41. Schütz E, Nima AA, Sailer U, Andersson-Arntén A–C, Archer T, Garcia D (2013) The affective profiles in the USA: Happiness, depression, life satisfaction, and happiness-increasing strategies. In press.
- 43. Garcia D, Nima AA, Archer T (2013) Temperament and Character's Relationship to Subjective Well- Being in Salvadorian Adolescents and Young Adults. In press.
- 44. Garcia D (2013) La vie en Rose: High Levels of Well-Being and Events Inside and Outside Autobiographical Memory. J Happiness Stud. DOI: 10.1007/s10902-013-9443-x.
- 48. Adrianson L, Djumaludin A, Neila R, Archer T (2013) Cultural influences upon health, affect, self-esteem and impulsiveness: An Indonesian-Swedish comparison. Int J Res Stud Psychol. DOI: 10.5861/ijrsp.2013.228.

- SUGGESTED TOPICS
- The Magazine
- Newsletters
- Managing Yourself
- Managing Teams
- Work-life Balance
- The Big Idea
- Data & Visuals
- Reading Lists
- Case Selections
- HBR Learning
- Topic Feeds
- Account Settings
- Email Preferences
A Refresher on Regression Analysis

Understanding one of the most important types of data analysis.
You probably know by now that whenever possible you should be making data-driven decisions at work . But do you know how to parse through all the data available to you? The good news is that you probably don’t need to do the number crunching yourself (hallelujah!) but you do need to correctly understand and interpret the analysis created by your colleagues. One of the most important types of data analysis is called regression analysis.
- Amy Gallo is a contributing editor at Harvard Business Review, cohost of the Women at Work podcast , and the author of two books: Getting Along: How to Work with Anyone (Even Difficult People) and the HBR Guide to Dealing with Conflict . She writes and speaks about workplace dynamics. Watch her TEDx talk on conflict and follow her on LinkedIn . amyegallo

Partner Center

An official website of the United States government
The .gov means it’s official. Federal government websites often end in .gov or .mil. Before sharing sensitive information, make sure you’re on a federal government site.
The site is secure. The https:// ensures that you are connecting to the official website and that any information you provide is encrypted and transmitted securely.
- Publications
- Account settings
Preview improvements coming to the PMC website in October 2024. Learn More or Try it out now .
- Advanced Search
- Journal List

Review of guidance papers on regression modeling in statistical series of medical journals
Christine wallisch.
1 Institute of Biometry and Clinical Epidemiology, Corporate Member of Freie Universität Berlin and Humboldt-Universität zu Berlin, Charité—Universitätsmedizin Berlin, Berlin, Germany
2 Center for Medical Statistics, Informatics and Intelligent Systems, Section for Clinical Biometrics, Medical University of Vienna, Vienna, Austria
3 School of Business and Economics, Emmy Noether Group in Statistics and Data Science, Humboldt-Universität zu Berlin, Berlin, Germany
Lorena Hafermann
Nadja klein, willi sauerbrei.
4 Faculty of Medicine and Medical Center, Institute of Medical Biometry and Statistics, University of Freiburg, Freiburg, Germany
Ewout W. Steyerberg
5 Department of Biomedical Data Sciences, Leiden University Medical Center, Leiden, The Netherlands
Georg Heinze
Geraldine rauch, associated data.
The data was collected within the review and is available as supporting information S6.
Although regression models play a central role in the analysis of medical research projects, there still exist many misconceptions on various aspects of modeling leading to faulty analyses. Indeed, the rapidly developing statistical methodology and its recent advances in regression modeling do not seem to be adequately reflected in many medical publications. This problem of knowledge transfer from statistical research to application was identified by some medical journals, which have published series of statistical tutorials and (shorter) papers mainly addressing medical researchers. The aim of this review was to assess the current level of knowledge with regard to regression modeling contained in such statistical papers. We searched for target series by a request to international statistical experts. We identified 23 series including 57 topic-relevant articles. Within each article, two independent raters analyzed the content by investigating 44 predefined aspects on regression modeling. We assessed to what extent the aspects were explained and if examples, software advices, and recommendations for or against specific methods were given. Most series (21/23) included at least one article on multivariable regression. Logistic regression was the most frequently described regression type (19/23), followed by linear regression (18/23), Cox regression and survival models (12/23) and Poisson regression (3/23). Most general aspects on regression modeling, e.g. model assumptions, reporting and interpretation of regression results, were covered. We did not find many misconceptions or misleading recommendations, but we identified relevant gaps, in particular with respect to addressing nonlinear effects of continuous predictors, model specification and variable selection. Specific recommendations on software were rarely given. Statistical guidance should be developed for nonlinear effects, model specification and variable selection to better support medical researchers who perform or interpret regression analyses.
Introduction
Knowledge transfer from the rapidly growing body of methodological research in statistics to application in medical research does not always work as it should [ 1 ]. Possible reasons for this problem are the lack of guidance and that not all statistical analyses are conducted by statistical experts but often by medical researchers who may or may not have a solid statistical background. Applied researchers cannot be aware of all statistical pitfalls and the most recent developments in statistical methodology. Keeping up is already challenging for a professional biostatistical researcher, who is often restricted to an area of main interest. Moreover, articles on statistical methodology are often written in a rather technical style making knowledge transfer even more difficult. Therefore, there is a need for statistical guidance documents and tutorials written in more informal language, explaining difficult concepts intuitively and with illustrative educative examples. The international STRengthening Analytical Thinking for Observational Studies (STRATOS) initiative ( http://stratos-initiative.org ) aims to provide accessible and accurate guidance documents for relevant topics in the design and analysis of observational studies [ 1 ]. Guidance is intended for applied statisticians and other medical researchers with varying levels of statistical education, experience and interest. Some medical journals are aware of this situation and regularly publish isolated statistical tutorials and shorter articles or even whole series of articles with the intention to provide some methodological guidance to their readership. Such articles and series can have a high visibility among medical researchers. Although some of the articles are short notes or rather introductory texts, we will use the phrase ‘statistical tutorial’ for all articles in our review.
Regression modeling plays a central role in the analysis of many medical studies, in particular, of observational studies. More specifically, regression model building involves aspects such as selection of a model type that matches the type of outcome variable, selection of explanatory variables to include in a model, choosing an adequate coding of the variables, deciding on how flexibly the association of continuous variables with the outcome should be modeled, planning and performing model diagnostics, model validation and model revision, reporting of a model and describing how well differences in the outcome can be explained by differences in the covariates. Some of the choices made during model building will strongly depend on the aim of modeling. Shmueli (2010) [ 2 ] distinguished between three conceptual modeling approaches: descriptive, predictive and explanatory modeling. In practice these aims are still often not well clarified, leading to confusion about which specific approach is useful in a modeling problem at hand. This confusion, and an ever-growing body of literature in regression modeling may explain why a common state-of-the-art is still difficult to define [ 3 ]. However, not all studies require an analysis with the most advanced techniques and there is the need for guidance for researchers without a strong background in statistical methodology, who might be “medical students or residents, or epidemiologists who completed only a few basic courses in applied statistics” according to the definition of level-1 researchers by the STRATOS initiative [ 1 ].
If suitable guidance for level-1 researchers in peer-reviewed journals was available, many misconceptions about regression model building could be avoided [ 4 – 6 ]. The researchers need to be informed about methods that are easily implemented, and they need to know about strengths and weaknesses of common approaches [ 3 ]. Suitable guidance should also point to possible pitfalls, elaborate on dos and don’ts in regression analyses, and provide software recommendations and understandable code for different methods and aspects. In this review, we focused on low-dimensional regression models where the sample size exceeds the number of candidate predictors. Moreover, we will not specifically address the field of causal inference, which goes beyond classical regression modeling.
So far, it is unclear what aspects of regression modeling have already been well-covered by related tutorials and where gaps still exist. Furthermore, suitable tutorial papers may be published but they are unknown to (nearly all) clinicians and therefore widely ignored in their analyses.
The objective of this review was to provide an evidence-based information basis assessing the extent to which regression modeling has been covered by series of statistical tutorials published in medical journals. Specifically, we sought to define a catalogue of important aspects on regression modeling, to identify series of statistical tutorials in medical journals, and to evaluate which aspects were treated in the identified articles and at which level of sophistication. Thereby, we put an intended focus on the choice of the regression model type, on variable selection and for continuous variables on the functional form. Furthermore, this paper will provide an overview, which helps to inform a broad audience of medical researchers about the availability of suitable papers written in English.
The remainder of this review is organized as follows: In the next section, the review protocol is described. Subsequently, we summarize the results of the review by means of descriptive measures. Finally, we discuss implications of our results suggesting potential topics for future tutorials or entire series.
Material and methods
The protocol of this review describing the detailed design was already published by Bach et al. (2020) [ 7 ]. In here, we summarize its main characteristics.
Eligibility criteria
First, we identified series of statistical tutorials and papers published in medical journals with a target audience mainly or exclusively consisting of medical researchers or practitioners. Second, we searched for topic-relevant articles on regression modeling within these series. Journals with a target audience of pure theoretical, methodological or statistical focus were not considered. We included medical journals if they were available in English language since this implies high international impact and broad visibility. Moreover, the series had to comprise at least five or more articles including at least one topic-relevant article. We focused on statistical series only since we believed that entire series have higher impact and visibility than isolated articles.
Sources of information & search strategy
After conducting a pilot study for a systematic search for series of statistical tutorials, we had to adapt our search strategy since sensitive keywords to identify statistical series could not be found. Therefore, we consulted more than 20 members of the STRATOS initiative via email in spring 2018 for suggestions on statistical series addressing medical researchers. We also asked them to forward this request to colleagues, which resembles snowball sampling [ 8 , 9 ]. This call was repeated at two international STRATOS meetings in summer 2018 and in 2019. The search was closed on June 30 st , 2019. Our approach also included elements of respondent-driven sampling [ 10 ] by offering collaboration and co-authorship in case of relevant contribution to the review. In addition, we included several series that were additionally proposed by a reviewer during the peer-review process of this manuscript, and which were published by the end of June, 2019 to be consistent with the original request.
Data management & selection process
The list of all resulting statistical series suggested is available as S1 File .
Two independent raters selected relevant statistical series from the pool of candidate series by applying the inclusion criteria outlined above.
An article within a series was considered to be topic-relevant if the title included one of the following keywords: regression , linear , logistic , Cox , survival , Poisson , multivariable , multivariate , or if the title suggested that the main topic of the article was statistical regression modeling . Both raters decided on the topic-relevance of an article independently and resolved discrepancies by discussion. To facilitate the selection of relevant statistical series, we designed a report form called inclusion form ( S2 File ).
Data collection process
After the identification of relevant series and topic-relevant articles, a content analysis was performed on all topic-relevant articles using an article content form ( S3 File ). The article content form was filled-in for every identified topic-relevant article by the two raters independently and again discrepancies were resolved by discussion. The results of completed article content forms were copied into a data base for further quantitative analysis.
In total 44 aspects of regression modeling were examined in the article content form ( S3 File ), which were related to four areas: type of regression model , general aspects of regression modeling , functional form of continuous predictors , and selection of variables . The 44 aspects cover topics of different complexity. Some aspects can be considered basic, others are more advanced. This was also commented in the S3 File for orientation. We mainly focused on predictive and descriptive models and did not consider particular aspects attributed to ethological models.
For each aspect, we evaluated whether it was mentioned at all, and if yes, the extent of explanation (short = one sentence only / medium = more than one sentence to one paragraph / long = more than one paragraph) [ 7 ]. We recorded whether examples and software commands were provided, and if recommendations or warnings were given with respect to each aspect. A box for comments provided space to note recommendations, warnings and other issues. In the article content form, it was also possible to add further aspects to each area. A manual for raters was created to support an objective evaluation of the aspects ( S4 File ).
Summary measures & synthesis of results
This review was designed as an explorative study and uses descriptive statistics to summarize results. We calculated absolute and relative frequencies to analyze the 44 statistical aspects. We used stacked bar charts to describe the ordinal variable extent of explanation for each aspect. To structure the analysis, we grouped the aspects into the afore mentioned areas: type of regression model , general aspects of regression modeling , determination of functional form for continuous predictors and selection of variables .
We conducted the above analyses both article-wise and series-wise. In the article-wise analysis, each article was considered individually. For the series-wise analysis, the results from all articles in a series were pooled and each series was considered as the unit of observation. This means, if an aspect was explained in at least one article, this also counted for the entire series.
Risk of bias
The risk of bias by missing a series was addressed extensively in the protocol of this study [ 7 , 11 , 12 ]. Moreover, bias could result from the inclusion criterion of series, which was the requirement of at least five articles in a series. This may have led to a less representative set of series. We set this inclusion criterion to identify highly visible series. Bias could also result from the specific choice of aspects of regression modeling to be screened. We tried to minimize this bias by the possibility for free text entries that could later be combined into additional aspects.
This review has been written according to the PRISMA reporting guideline [ 13 , 14 ], compare S1 Checklist . This review does not include patients or humans. The data that were collected within the review are available in S1 Data .
Selection of series and articles
The initial query revealed 47 series of statistical tutorials ( Fig 1 and S1 File ). Out of these 47 series, two series were not published in a medical journal and five series did not target an audience with low statistical knowledge. Therefore, these series were excluded. Five and ten series were excluded because they were not written in English or they did not comprise at least five articles, respectively. Further, we excluded three series because they did not contain any topic-relevant article. The list of the series and the reason for each excluded series is found in S1 File . Finally, we included 23 series with 57 topic-relevant articles.

Characteristics of the series
Each series contained between one to nine topic-relevant articles (two on average, Table 1 ). The variability of the average number of article pages per series illustrates that the extent of the articles was very different (1 to 10.3 pages). Whereas the series Statistics Notes in the BMJ typically used a single page to discuss a topic, hence pointing only to the most relevant issues, there were longer papers with a length of up to 16 pages [ 15 , 16 ]. The series in the BMJ is also the one spanning over the longest time period (1994–2018). Beside of the series in the BMJ , only the Archives of Disease in Childhood and the Nutrition series started publishing papers already in the last century. Fig 2 shows that most series were published only during a short period, perhaps paralleling terms of office of an Editor.

We considered 44 aspects, see S3 File .
The most informative series with respect to our pre-specified list of aspects was published in Revista Española de Cardiologia , which mentioned 35 aspects in two articles on regression modeling ( Table 1 ). Similarly, Circulation and Archives of Disease in Childhood covered 31 and 30 aspects in three article each. The number of articles and the years of publication varied across the series ( Fig 2 ). Some series comprised only five articles whereas Statistics Notes of the BMJ published 68 short articles, which was very successful with some articles that were cited about 2000 times. Almost all series covered multivariable regression in at least one article. The range of regression types varied across series. Most statistical series were published with the intention to improve the knowledge of their readership about how to apply appropriate methodology in data analyses and how to critically appraise published research [ 17 – 19 ].
Characteristics of articles
The top three articles that covered the highest number of aspects (27 to 34 out of 44 aspects) on six to seven pages were published in Revista Española de Cardiologia , Deutsches Ärzteblatt International , and in European Journal of Cardio-Thoracic Surgery [ 20 – 22 ]. The article of Nuñez et al. [ 22 ] published in Revista Española de Cardiologia covered the most popular regression types (linear, logistic and Cox regression) and explained not only general aspect but also gave insights into non-linear modeling and variable selection. Schneider et al. [ 20 ] covered all regression types that we considered in our review in their publication in Deutsches Ärzteblatt International . The top-ranked article in European Journal of Cardio-Thoracic Surgery [ 21 ] particularly focused on the development and validation of prediction models.
Explanation of aspects in the series
Almost all statistical series included at least one article that mentioned or explained multivariable regression ( Table 1 ). Logistic regression was the most frequently described regression type in 19 out of 23 series (83%), followed by linear regression (78%). Cox regression/survival model (including proportional hazards regression) was mentioned in twelve series (52%) and was less extensively described than linear and logistic regression. Poisson regression was covered by three series (13%). Each of the considered general aspects of regression modeling were mentioned in at least four series (17%) ( Fig 3 ) except for random effect models , which were treated in only one series (4%). Interpretation of regression coefficients , model assumptions , and different purposes of regression mode were covered in 19 series (83%). The aspect different purposes of regression models comprised at least one statement in an article concerning purposes of regression models, which could be identified by keywords like prediction, description, explanation, etiology, or confounding. More than one sentence was used for the explanation of different purposes in 15 series (65%). In 18 series (78%), reporting of regression results and regression diagnostics were described, which was done extensively in most series. Aspects like treatment of binary covariates , missing values , measurement error , and adjusted coefficient of determination were rather infrequently mentioned and found in four to seven series each (25–30%).

Extent of explanation of general aspects of regression modeling in statistical series: One sentence only (light grey), more than one sentence to one paragraph (grey) and more than one paragraph (black).
At least one aspect of functional forms of continuous predictors , was mentioned in 17 series (74%), but details were hardly ever given ( Fig 4 ). The possibility of non-linear relation and non-linear transformations were raised in 16 (70%) and eleven series (48%), respectively. Dichotomization of continuous covariates was found in eight series (35%) and it was extensively discussed in two (9%). More advanced techniques like the use of splines or fractional polynomials were mentioned in some series but detailed information for splines was not provided. Generalized additive models were never mentioned.

Extent of explanation of aspects of functional forms of continuous predictors in statistical series: One sentence only (light grey), more than one sentence to one paragraph (grey) and more than one paragraph (black).
Selection of variables was mentioned in 15 series (65%) and described extensively in ten series (43%) ( Fig 5 ). However, specific variable selection methods were rarely described in detail. Backward elimination , selection based on background knowledge , forward selection , and stepwise selection were the most frequently described selection methods in seven to eleven series (30–48%). Univariate screening , which is still popular in medical research, was only described in three series (13%) in up to one paragraph. Other aspects of variable selection were hardly ever mentioned. Selection based on AIC/BIC , relating to best subset selection or stepwise selection based on these information criteria, and the choice of the significance level were found in 2 series only (9%). Relative frequencies of aspects mentioned in articles are detailed in Figs Figs1 1 – 3 in S5 File .

Extent of explanation of aspects of selection of variables in statistical series: One sentence only (light grey), more than one sentence to one paragraph (grey) and more than one paragraph (black).
We found general recommendations for software in nine articles of nine different series. Authors mentioned R, Nanostat, GLIM package, SAS and SPSS [ 75 – 78 ]. SAS as well as R were recommended in three articles. In only one article the authors referred to a specific package in R. Detailed code examples were provided in two articles only [ 16 , 58 ]. In the article of Curran-Everett [ 58 ], the R script file was provided as appendix and in the article of Obuchowski [ 16 ], code chunks were included throughout the text directly showing how to derive the reported results. In all, software recommendations were rare and mostly not detailed.
Recommendations and warnings in the series
Recommendations and warnings were given on many aspects of our list. All statements are listed in S5 File : Table 1 and some frequent statements across articles are summarized below.
Statements on general aspects
We found numerous recommendations and warnings on general aspects as described in the following. Concerning data preparation, some authors recommended to impute missing values in multivariable models, e.g. by multiple imputation [ 20 – 22 , 31 ]. Steyerberg et al. [ 31 ] and Grant et al. [ 21 ] discouraged from using a complete case analysis to handle missing values. As an aspect of model development, number of observations/events per variable was a disputed topic in several articles [ 79 – 81 ]. In seven articles, we found explicit recommendations for the number of observations (in linear models) or the events per variable (in logistic and Cox/survival models), varying between at least ten to 20 observations/events per variable [ 16 , 20 , 22 , 25 , 31 , 33 , 55 ]. Several recommendations and warnings were given on model assumptions and model diagnostics . Many series authors recommended to check assumptions graphically [ 24 , 27 , 44 , 58 , 72 ] and they warned that models may be inappropriate if the assumptions are not met [ 20 , 24 , 31 , 33 , 52 , 55 , 56 , 62 ]. In the context of Cox proportional hazards model, authors especially mentioned the proportional hazards assumption [ 24 , 44 , 49 , 56 , 62 ]. Concerning reporting of results, some authors warned to not confuse odds ratios with relative risks or hazard ratios [ 25 , 44 , 59 ]. Several warnings could also be found on reporting performance of a model. Most authors did not recommend to report the coefficient of determination R 2 [ 20 , 27 , 51 , 61 ] and indicated that the pitfall of R 2 is that its value increases with increasing number of covariates in the model [ 15 ]. Schneider et al. [ 20 ] and Richardson et al. [ 61 ] recommended to use the adjusted coefficient of determination instead. We also found many recommendations and statements about model validation for prediction models. Authors of the evaluated articles recommended cross-validation or bootstrap validation instead of split sample validation if internal validation is performed [ 21 , 22 , 31 , 70 , 72 ]. It was also suggested that internal validation is not sufficient for the model to be used in clinical practice and an external validation should be executed as well [ 21 ]. In several articles, we found that authors warned about applying the Hosmer-Lemeshow test because of potential pitfalls [ 31 , 60 , 61 ]. For reporting regression results , in two articles the guideline for Transparent Reporting of multivariable prediction models for Individual Prognosis or Diagnosis (TRIPOD) was mentioned [ 21 , 71 , 82 ].
Statements on functional form of continuous predictors
Dichotomization of continuous predictors is an aspect of functional forms of continuous predictors that was frequently discussed. Many authors argued against categorization of continuous variables because it may lead to loss of power, to increased risk of false positive results, to underestimation of variation, and to concealment of non-linearities [ 21 , 26 , 31 , 69 ]. However, other authors advised to categorize continuous variables if the relation to the outcome is non-linear [ 24 , 25 , 59 ].
Statements on variable selection
We also found recommendations in favor of or against specific variable selection methods. Four articles explicitly recommended to take advantage of background knowledge to select variables [ 15 , 20 , 48 , 59 ]. Univariate screening was advised against by one article [ 19 ]. Comparing stepwise selection methods, Grant et al. [ 21 ] preferred backward elimination over forward selection. Authors warned about consequences of stepwise methods such as unstable selection and overfitting [ 21 , 31 ]. It was also pointed out that selected models must be interpreted with greatest caution and implications should be checked on new data [ 28 , 53 ].
Methodological gaps in the series
This descriptive analysis of contents gives rise to some observations on important gaps and possibly misleading recommendations. First, we found that one general type of regression models, Poisson regression, was not treated in most series. This omission is probably due to the fact that Poisson regression is less frequently applied in medical research because most outcomes are binary or time-to-event and, therefore, logistic and Cox regression are more frequent. Second, several series introduced the possibility of non-linear relations of continuous covariates with the outcome. However, only few statements on how to deal with non-linearities by specifying flexible functional forms in multiple regression were available. Third, we did not find very detailed information on advantages and disadvantages of data-driven variable selection methods in any of the series. Finally, tutorials on statistical software and on specific code examples were hardly found in the reviewed series.
Misleading recommendations in the series
Quality assessment of recommendations would have been controversial and we did not intend doing it. Nevertheless, here we mention two issues that we consider as severely misleading. Although univariate screening as a method for variable selection was never recommended in any of the series, one article showed an example with the application of this procedure to pre-filter the explanatory variables based on their associations with the outcome variable [ 47 ]. It is known since long that univariate screening should be avoided because it has the potential to wrongly reject important variables [ 83 ]. In another article it was suggested that a model can be considered robust if results from both backward elimination and forward selection agree [ 20 ]. Such agreement does not support robustness of stepwise methods: relying on agreement is a poor strategy [ 84 , 85 ].
Series and articles recommended to read
Depending on the aim of the planned study, as well as the focus and knowledge level of the reader, different series and articles might be recommended. The series in Circulation comprised three papers about multiple linear and logistic regression [ 24 – 26 ], which provide basics and describe many essential aspects of univariable and multivariable regression modeling. For more advanced researchers, we recommend the article of Nuñ ez et al. in Revista Española de Cardiologia [ 22 ], which gives a quick overview of aspects and existing methods including functional forms and variable selection. The Nature Methods series published short articles focusing on few, specific aspects of regression modeling [ 34 – 42 ]. This series might be of interest if one likes to spent more time on learning about regression modeling. If someone is especially interested in prediction models, we recommend a concise publication in the European Heart Journal [ 31 ], which provides details on model development and validation for predictive purposes. For the same topic we can also recommend the paper by Grant et al. [ 21 ]. We consider all series and articles recommended in this paragraph as suitable reading for medical researchers but this does not imply that we agree to all explanations, statements and aspects discussed.
Summary and consequences for future work
This review summarizes the knowledge about regression modeling that is transferred through statistical tutorials published in medical journals. A total of 23 series with 57 topic-relevant articles were identified and evaluated for coverage of 44 aspects of regression modeling. We found that almost all aspects of regression modeling were at least mentioned in any of the series. Several aspects of regression modeling, in particular most general aspects, were covered. However, detailed descriptions and explanations of non-linear relations and variable selection in multivariable models were lacking. Only few papers provided suitable methods and software guidance for analysts with a relatively weak statistical background and limited practical experience as recommended by the STRATOS initiative [ 1 ]. However, we confess that currently there is no agreement on state of the art methodology [ 3 ].
Nevertheless, readers of statistical tutorials should not only be informed about the possibility of non-linear relations of continuous predictors with the outcome but they should also be given a brief overview about which methods are generally available and may be suitable. This could be achieved by tutorials that introduce readers to methods like fractional polynomials or splines, explaining similarities and differences between these approaches, e.g., by comparative, commented analyses of realistic data sets. Such documents could also show how alternative analyses (considering/ignoring potential non-linearities) may result in conflicting results and explain the reasons for such discrepancies.
Detailed tutorials on variable selection could aim at describing the mechanism of different variable selection methods, which can easily be applied with standard statistical software, and should state in what situations variable selection methods are needed and could be used. For example, if sufficient background knowledge is available, prefiltering or even the selection of variables should be based on this information rather than using data-driven methods on the entire data set. Such tutorials should provide comparisons and interpretation of the results of various variable selection methods and suggest adequate methods for different data settings.
Generally, the articles also lacked details on software to perform statistical analysis and usually did not provide code chunks, descriptions of specific functions, an appendix with commented code or references to software packages. Future work should also focus on filling this gap by recommendations of software as well as providing well commented and documented code for different statistical methods in a format that is accessible by non-experts. We recommend that software, packages and functions therein to apply certain methods should be reported in every statistical tutorial article. The respective code to derive analysis results could be provided in an appendix or directly in the manuscript text, if not too lengthy. Any provided code in the appendix should be well-structured and lavishly commented referring to the particular method and describing all defined parameter settings. This will encourage medical researchers to increase the reproducibility of their research by also publishing their statistical code, e.g., in electronic appendices to their publications. For example, worked examples with openly accessible data sets and commented code allowing fully reproducible results have a high potential to guide researchers in their own statistical tasks. On the contrary, we discourage from using point-and-click software programs, which sometimes output far more analysis results than requested. Users may pick inadequate methods or report wrong results inadvertently, which could debilitate their research work.
Generally, our review may stimulate the development of targeted gap-filling guidance and tutorial papers in the field of regression modeling, which should support medical researchers in several ways: 1) by explaining how to interpret published results correctly, 2) by guiding them how to critically appraise the methodology used in a published article, 3) by enabling them to plan, perform basic statistical analyses and report results in a proper way and 4) by helping them to identify situations in which the advice of a statistical expert is required. In S3 File : CRF article screening we commented which aspects should usually be addressed by an expert and which aspects are considered basic.
Strengths and limitations
According to our knowledge this is the first review on series of statistical tutorials in the medical field with the focus on regression modeling. Our review followed a pre-specified and published protocol to which many experienced researchers in the field of applied regression modeling contributed. One aspect of this contribution was the collection of series of statistical tutorials that could not be identified by common keyword searches.
We standardized the selection process by designing an inclusion checklist for series of statistical tutorials and by providing a manual for the content form with which we extracted the actual information of the article and series. Another strength is that the data collection process was performed objectively since each article was analyzed by two out of three independent raters. Discrepancies were discussed among all three of them to find a consent. This procedure avoided that single opinions were transferred to the output of this review. This review is informative for many clinical colleagues who are interested in statistical issues in regression modeling and search for suitable literature.
This review also has limitations. An automated, systematic search was not possible because series could not be identified by common keywords neither on the series’ title level nor on the article’s title level. Thus, not all available series may have been found. To enrich our initial query, we also searched on certain journals’ webpages and requested our expert panel from the STRATOS initiative to complement our list with other series they were aware of. We also included series that were suggested by one reviewer during the peer-review procedure of this manuscript. This selection strategy may impose a bias towards higher-quality journals since series of less prestigious journals might not be known to the experts. However, the higher-quality journals can be considered as the primary source of information for researchers seeking advice on statistical methodology.
We considered only series with at least five articles. This boundary is of course to a certain extend arbitrary. It was motivated by the fact that we intended to do analyses on the series level, which is only reasonable if a series covers an adequate number of articles. We also assumed that larger series are more visible and well-known to researchers.
We also might have missed or excluded some important aspects of regression modeling in our catalogue. The catalogue of aspects was developed and discussed by several experienced researchers of the STRATOS initiative working in the field of regression modeling. After submission of the protocol paper some more aspects were added on request of its reviewers [ 7 ]. However, further important aspects such as meta-regression, diagnostic models, causal inference, reproducibility or open data and open software code were not addressed. We encourage researchers to repeat similar reviews on these related fields.
A third limitation is that we only searched for series whereas there might be other educational papers on regression modeling that were published as single articles. However, we believe that the average visibility of an entire series and thereby its educational impact is much higher than for isolated articles. This does not negate that there could be excellent isolated articles, which can have a high impact for training medical researchers. While working on the final version of this paper we became aware of the series Big-data Clinical Trial Column in the Annals of Translational Medicine . Until 1 January 2019 they had published 36 papers and the series would have been eligible for our review. Obviously, we might have overseen further series, but it is unlikely that it has a larger effect on the results of our review.
Moreover, there are many introductory textbooks, educational workshops and online video tutorials, some of them with excellent quality, which were not considered here. A detailed review of such sources clearly was out of our scope.
Despite many series of statistical tutorials being available to guide medical researchers on various aspects of regression modeling, several methodological gaps still persist, specifically on addressing nonlinear effects, model specification and variable selection. Furthermore, papers are published in a large number of different journals and are therefore likely unknown to many medical researchers. This review fills the latter gap, but many more steps are needed to improve the quality and interpretation of medical research. More detailed statistical guidance and tutorials with a low technical level on regression modeling and other topics are needed to better support medical researchers who perform or interpret regression analyses.
Supporting information
S1 checklist, acknowledgments.
When this article was written, topic group 2 of STRATOS consisted of the following members: Georg Heinze (Co-chair, [email protected] ), Medical University of Vienna, Austria; Willi Sauerbrei (co-chair, ed.grubierf-inu.ibmi@sfw ), University of Freiburg, Germany; Aris Perperoglou (co-chair, [email protected] ), AstraZeneca, London, Great Britain; Michal Abrahamowicz, Royal Victoria Hospital, Montreal, Canada; Heiko Becher, Medical University Center Hamburg, Eppendorf, Hamburg, Germany; Harald Binder, University of Freiburg, Germany; Daniela Dunkler, Medical University of Vienna, Austria; Rolf Groenwold, Leiden University, Leiden, Netherlands; Frank Harrell, Vanderbilt University School of Medicine, Nashville TN, USA; Nadja Klein, Humboldt Universität, Berlin, Germany; Geraldine Rauch, Charité–Universitätsmedizin Berlin, Germany; Patrick Royston, University College London, Great Britain; Matthias Schmid, University of Bonn, Germany.
We thank Edith Motschall (Freiburg) for her important support in the pilot study where we tried to define keywords for identifying statistical series within medical journals. We thank several members of the STRATOS initiative for proposing a high number of candidate series and we thank Frank Konietschke for English language editing in our protocol.
Funding Statement
CW: I-4739-B Austrian Science Fund, https://www.fwf.ac.at/en/ LH: RA 2347/8-1, German Research Foundation, https://www.dfg.de/en/ WS: SA 580/10-1, German Research Foundation, https://www.dfg.de/en/ All funders had no role in study design, data collection and analysis, decision to publish, or preparation of the manuscript.
Data Availability
- Open access
- Published: 20 April 2024
Specific mortality in patients with diffuse large B-cell lymphoma: a retrospective analysis based on the surveillance, epidemiology, and end results database
- Rong Yan 2 ,
- Chunmei Ye 1 ,
- Jun Li 1 &
European Journal of Medical Research volume 29 , Article number: 241 ( 2024 ) Cite this article
100 Accesses
Metrics details
The full potential of competing risk modeling approaches in the context of diffuse large B-cell lymphoma (DLBCL) patients has yet to be fully harnessed. This study aims to address this gap by developing a sophisticated competing risk model specifically designed to predict specific mortality in DLBCL patients.
We extracted DLBCL patients’ data from the SEER (Surveillance, Epidemiology, and End Results) database. To identify relevant variables, we conducted a two-step screening process using univariate and multivariate Fine and Gray regression analyses. Subsequently, a nomogram was constructed based on the results. The model’s consistency index (C-index) was calculated to assess its performance. Additionally, calibration curves and receiver operator characteristic (ROC) curves were generated to validate the model’s effectiveness.
This study enrolled a total of 24,402 patients. The feature selection analysis identified 13 variables that were statistically significant and therefore included in the model. The model validation results demonstrated that the area under the receiver operating characteristic (ROC) curve (AUC) for predicting 6-month, 1-year, and 3-year DLBCL-specific mortality was 0.748, 0.718, and 0.698, respectively, in the training cohort. In the validation cohort, the AUC values were 0.747, 0.721, and 0.697. The calibration curves indicated good consistency between the training and validation cohorts.
The most significant predictor of DLBCL-specific mortality is the age of the patient, followed by the Ann Arbor stage and the administration of chemotherapy. This predictive model has the potential to facilitate the identification of high-risk DLBCL patients by clinicians, ultimately leading to improved prognosis.
Introduction
DLBCL, known as diffuse large B-cell lymphoma, is a highly heterogeneous disease and is the most common type of non-Hodgkin’s lymphoma, accounting for approximately 30–40% of all lymphoma cases [ 1 ]. While there have been significant advancements in the diagnosis and treatment of DLBCL in recent years, it is disheartening to note that 40–50% of patients with DLBCL still remain incurable [ 2 ]. For patients who experience a relapse or have refractory DLBCL, the prognosis is generally poor [ 3 ]. Hence, it becomes imperative to identify highly specific and sensitive prognostic markers that can effectively identify high-risk patients, thereby enabling improved treatment decisions and ultimately enhancing patient survival.
Several studies have examined prognostic factors in patients with DLBCL [ 2 , 4 , 5 , 6 , 7 ]. However, many of these studies have relied on the conventional Cox proportional hazards model [ 7 , 8 ]. It is important to note that competing mortality events frequently arise during the analysis of survival data. Yet, the traditional Cox regression often fails to consider the occurrence of these competing mortality events, leading to potential misjudgment of patient prognosis, irrespective of the independence between such events. If a patient dies from causes other than DLBCL, and the Cox regression fails to account for these competing mortality events, it introduces bias into the analysis results. The Fine and Gray model enables us to analyze data while taking into account competing risks. Similar to the Cox model, the Fine and Gray model utilizes a risk set function, but it also incorporates the concept of competition between different types of events. This model estimates the probability of each event by comparing the event-specific risk set function with the overall risk set function, while accounting for the impact of other event types. Competing risk models, specifically the Fine and Gray proportional hazards model, demonstrate excellent capability in addressing the correlation between cancer outcomes and competing events, ultimately leading to a remarkable enhancement in the accuracy of prognostic analysis [ 9 ]. Despite its potential, this methodology remains largely underutilized. Leveraging the SEER database, a comprehensive and extensive multi-center database with credible data sources, this study aims to establish a competing risk model based on DLBCL patients. The objective is to investigate the factors that influence cause-specific mortality in DLBCL patients.
Study cohort
We extracted data from the SEER database [Incidence—SEER Research Plus Data, 17 Registries, Nov 2021 Sub (2000–2019)] using SEER Stat (Version 8.4.1) software. The data pertain to patients diagnosed with DLBCL between 2000 and 2015. To ensure data quality, patients with less than 1 month of follow-up and those with one or more missing variables were excluded from the analysis. The collected data encompassed demographic information such as sex, race, age, marital status, median household income, and place of residence. It also included tumor characteristics such as site, primary site, presence of B symptoms, number of malignant tumors, and whether it was the first primary tumor. Additionally, the data recorded the Ann Arbor Stage, surgical and chemoradiotherapy information including surgery, radiation, chemotherapy, the sequence of systemic therapy and surgery, and treatment timing. Furthermore, the cause of death and follow-up information were documented. The diagnosis of diffuse large B-cell lymphoma (DLBCL) was made based on the criteria outlined in the International Classification of Diseases for Oncology, 3rd Edition (ICD-O-3). The staging of lymphoma was determined using the Ann Arbor stage system (AASS). Regarding the analysis of continuous variables, the subjects were categorized into different groups based on their treatment timing (the interval between the diagnosis and initiation of treatment): more than 1 month and 1 month or less. The subjects were also divided into 5 age groups: 0–19 years, 20–39 years, 40–59 years, 60–79 years, and 80–100 years. Furthermore, based on the median annual household income, the subjects were divided into 3 groups: less than $50,000, $50,000–$74,999, and greater than $75,000.
Statistical analysis
The study cohort was divided into a training cohort and a validation cohort in a ratio of 7:3. The purpose of this division was to use the training cohort to train the model and the validation cohort to test the model. All patient features were divided into the training and validation cohorts, and the balance of the data was assessed by comparing the differences between the two groups. Categorical variables were presented as frequencies and percentages (25%), and chi-square tests were used to compare the differences between the two groups. Normally distributed continuous variables were displayed as means and standard deviations [Mean (S.E.)], and t -tests were used to compare the differences. Non-normally distributed continuous variables were presented as medians and quartiles (median [IQR]), and rank sum tests were used to compare the differences. In the competing risk model, the outcome event of interest was death from DLBCL, and death from other causes was treated as a competing event. Variables were screened in two steps using univariate and multivariate Fine and Gray regression analyses. Variables that were statistically significant in the univariate analysis were included in the multivariate analysis. The variables that remained statistically significant in the multivariate analysis were used to construct a competing risk model and develop a corresponding nomogram. The model’s C-index was calculated, and its predictions were compared with the observed actual values. Calibration curves and ROC curves were plotted to assess the consistency and accuracy of the model. All statistical analyses were performed using R 4.2.1 ( https://www.r-project.org/ ). The Fine and Gray regression analysis and competing risk modeling were conducted using the riskRegression (2021.10.10) software package. The pmsampsize (1.1.3) package was used to calculate the sample size and plot ROC and calibration curves, while the rms package was used for nomogram plotting.
Patient features
A total of 117,171 patients diagnosed with diffuse large B-cell lymphoma (DLBCL) were identified from the dataset titled “Incidence—SEER Research Plus Data, 17 Registries, Nov 2021 Sub (2000–2019)” that was submitted to the SEER database in 2021. Patients who had less than 1 month of follow-up ( N = 9758), patients without Ann Arbor Stage data ( N = 29,974), and patients with one or more missing variables ( N = 53,037) were excluded from the study (Fig. 1 ). Eventually, a total of 24,402 patients were included in this study. Among them, 6459 died from DLBCL and 4076 died from other causes. The median survival time for patients in the entire study cohort was 58 months (IQR: [16.00, 83.00]). The majority of patients were between the ages of 60 and 79 years (48.0%), and there was a higher proportion of men compared to women (57.1%). The characteristics of patients in both the training cohort and the validation cohort are described in Table 1 . There were no statistically significant differences in each variable between the two cohorts ( P > 0.05), indicating a balanced distribution of data.
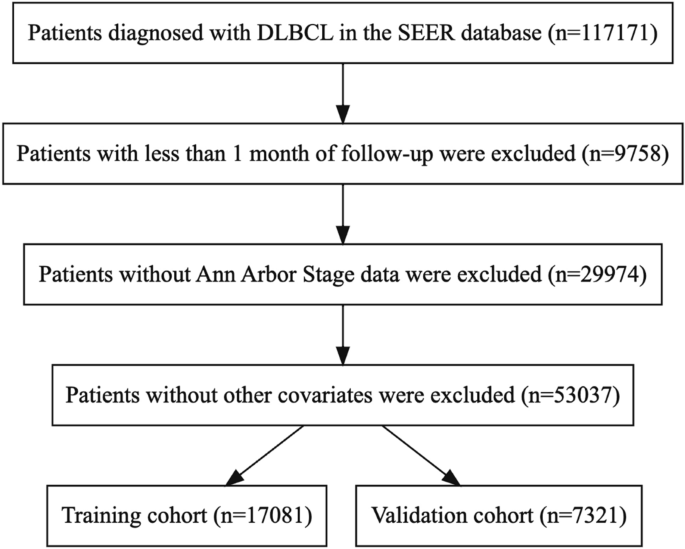
Patient selection flowchart
Feature selection
The selection of features was carried out using univariate and multivariate Fine and Gray regression analyses. In the univariate analyses, only variables that showed statistical significance were included in the multivariate analyses. Similarly, in the multivariate analyses, only variables that showed statistical significance were included in the final model. The univariate analysis revealed that 14 variables were found to be statistically significant and were, therefore, considered potential risk factors for cause-specific mortality in DLBCL patients. These variables included race, tumor site (extranodal or nodal), primary site, Ann Arbor stage, whether surgery was performed, whether radiation therapy was administered, whether chemotherapy was administered, sequence of systemic therapy and surgery, treatment timing, presence of B symptoms, whether it was the first primary tumor, age, marital status, and median annual household income. Upon conducting a multivariate analysis with the above variables included in the adjusted model, it was revealed that 13 variables remained statistically significant and were identified as independent risk factors for cause-specific mortality in DLBCL patients (Additional file 1 : Table S1). These variables included race, tumor site (extranodal or nodal), Ann Arbor stage, whether surgery was performed, whether radiation therapy was administered, whether chemotherapy was administered, sequence of systemic therapy and surgery, treatment timing, presence of B symptoms, whether it was the first primary tumor, age, marital status, and median annual household income. These 13 variables were further included in the competing risk model (Table 2 ). Furthermore, to effectively compare the disparities between Fine and Gray regression and Cox regression, we incorporated the aforementioned variables into the multivariate Cox regression analysis. The findings revealed that age, Ann Arbor Stage, b symptoms, absence of chemotherapy, absence of radiation, absence of surgery, the sequence of systemic therapy and surgery, and treatment timing exerted a more prominent influence on the risk of all-cause mortality (in the Cox proportional risk model) compared to the risk of DLBCL-specific mortality (in the Competing Risk Model) (refer to Table 3 ).
Model development and validation
The competing risk model incorporated 13 independent risk factors, achieving a C-statistic of 0.709 (± 0.002). To facilitate the application of this model, a corresponding nomogram, as shown in Fig. 2 , was constructed. The points assigned to each individual variable were determined based on the patients’ classification, and the sum of these points yielded the Total Points. By matching the Total Points with the corresponding predictor, the cause-specific survival probability of patients could be estimated. The performance of the model was further evaluated through ROC curve analysis. The area under the curve (AUC) for 6-month, 1-year, and 3-year mortality in DLBCL patients was 0.748 (95% confidence interval [CI] [0.736, 0.759]), 0.718 (95% CI [0.708, 0.728]), and 0.698 (95% CI [0.689, 0.707]) in the training cohort, respectively. In the validation cohort, the AUC values were 0.747 (95% CI [0.729, 0.765]), 0.721 (95% CI [0.706, 0.737]), and 0.697 (95% CI [0.683, 0.711]), as depicted in Fig. 3 . Moreover, the calibration curve analysis demonstrated that the model’s predicted results aligned closely with the actual values, as illustrated in Fig. 4 . This further confirms the reliability and accuracy of the model in predicting outcomes.
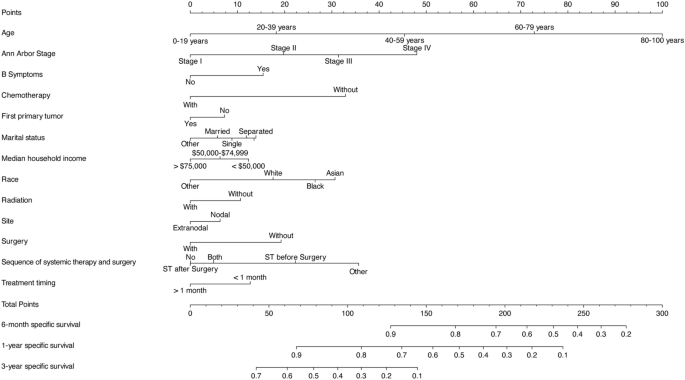
The nomogram of the competing risk model
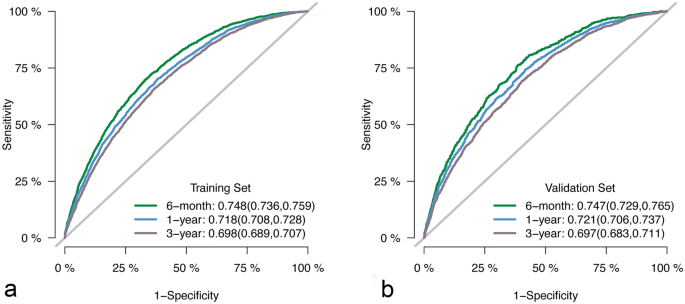
a The results of ROC curve analysis in the training cohort. b The results of ROC curve analysis in the validation cohort
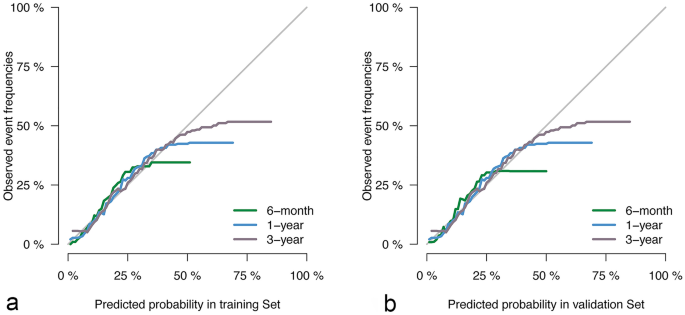
a The results of calibration curve analysis in the training cohort. b The results of calibration curve analysis in the validation cohort
We have devised a competing risk model in this research to forecast cause-specific mortality among DLBCL patients, which is then represented by a graphical nomogram. The model demonstrated favorable predictive accuracy and can offer reliable prognostic insights. This, in turn, may enhance clinicians’ comprehension of DLBCL and facilitate the provision of targeted clinical assistance to individuals at high risk.
The results of the feature selection demonstrated that there are 13 variables that serve as independent predictors of cause-specific mortality in DLBCL patients. These variables include race, tumor site (extranodal or nodal), Ann Arbor stage, surgery, radiation therapy, chemotherapy, sequence of systemic therapy and surgery, treatment timing, B Symptoms, whether it was the first primary tumor, age, marital status, and median annual household income. According to the results obtained from the nomogram, patient age was identified as the most accurate predictor, followed by Ann Arbor stage and chemotherapy. With regard to treatment, our study revealed that the absence of surgery, radiation therapy, chemotherapy, and systemic therapy was associated with a poorer prognosis for patients. It is widely acknowledged that chemotherapy is the primary treatment for DLBCL, and its efficacy has been supported by numerous studies [ 10 , 11 ]. Radiation therapy is often used in conjunction with chemotherapy and has been shown to improve clinical symptoms in relapsed or refractory DLBCL patients following chemotherapy [ 12 ]. For the majority of lymphoma patients, chemotherapeutic agents are deemed more effective, thus surgical treatment is generally not recommended [ 13 ]. In fact, one study has shown that surgical treatment for lymphoma does not improve patient prognosis [ 14 ]. Nevertheless, there are certain specific cases where surgical intervention is necessary. For example, patients with primary gastrointestinal lymphoma may present with intestinal obstruction or splenomegaly alongside symptoms of compression [ 15 ]. In terms of demographic information, our findings suggest that Asians have a significantly higher mortality rate among DLBCL patients as compared to whites, and divorced patients exhibit a higher mortality rate than married patients. Furthermore, age [ 16 ], Ann Arbor stage [ 17 ], and B symptoms [ 18 ] have all been identified as predictors for cause-specific mortality in DLBCL patients, which is consistent with our study’s findings.
We utilized both the Fine and Gray model and the Cox proportional risk model to evaluate the influence of various variables on the outcome. To determine the impact of each variable, we computed the hazard ratio (HR). Table 3 illustrates the disparities between the variables in the two models. Considering the independent risk factors, we observed that age, Ann Arbor Stage, presence of b symptoms, absence of chemotherapy, absence of radiation, absence of surgery, and the sequence of systemic therapy and surgery significantly affected the risk of all-cause mortality in comparison to DLBCL-specific mortality. Regarding the independent protective factors, we found that the presence of a first primary tumor, marital status, median household income, and timing of treatment exerted a more pronounced influence on the risk of all-cause mortality compared to the risk of DLBCL-specific mortality. However, the effect of race (White) on the risk of all-cause mortality was relatively smaller.
Several studies have been conducted to assess the prognosis of patients with DLBCL using the SEER database. One particular study focused on the risk of developing second primary malignancies in DLBCL patients and revealed that the oral cavity and pharynx were the most vulnerable regions for malignant tumor development [ 19 ]. Other studies, encompassing diverse populations with DLBCL, investigated the prognosis of patients [ 20 , 21 , 22 , 23 ]. However, it is worth noting that the majority of these studies relied on the conventional Cox proportional risk model. In contrast, our study adopts a competing risk model, which takes into account both DLBCL-specific mortality events and the influence of competing events on the analysis outcomes. Most prognostic studies commonly utilize the traditional Kaplan–Meier method and Cox regression model to analyze survival patterns and identify significant prognostic indicators [ 24 ]. Nevertheless, real-world medical studies often involve the occurrence of multiple competing outcome events rather than a single event. Consequently, it becomes imperative to employ a competing risk model to mitigate the bias resulting from the presence of these competing risk events [ 25 , 26 ]. The Competitive Risk Model, also known as the Fine and Gray model, was proposed by Fine and Gray in 1999 to address proportional risk situations in which competing risks are present. Unlike traditional survival models, this model focuses on modeling the subdistribution hazard function instead of the risk function for survival time. The subdistribution hazard function calculates the conditional risk of a specific event occurring before a certain point in time, considering the occurrence of competing events. This model is particularly useful when the endpoint event of a study, such as disease recurrence, can be “competed for” by other types of events, such as patient death from other causes. In such cases, traditional survival analysis methods may not provide accurate results. Using competing risk models, researchers can obtain more precise risk estimates and evaluate and compare the risk of specific events while accounting for the influence of other risk events.
The nomogram, a visual representation of models [ 27 ], has been widely recognized for its ability to depict complex relationships. Numerous studies have shown that machine learning models, such as random forests, neural networks, and support vector machines, can effectively capture nonlinear patterns in the data, thereby enhancing their predictive power [ 28 , 29 , 30 , 31 ]. However, one drawback of these models is their “black box” nature, which limits our understanding of the underlying computational process and the importance of each feature. In contrast, the nomogram offers a simple and intuitive graphical interface that allows for the quantification of the risk associated with each feature, making it particularly valuable for clinical applications [ 32 ]. The SEER database, maintained by the National Cancer Institute (NCI) [ 33 ], is a comprehensive and diverse collection of cancer incidence and survival data for specific populations in the United States. It serves as a valuable resource for researchers and healthcare professionals in understanding and analyzing cancer trends. With its large sample size and inclusion of multiple centers and racial backgrounds, the SEER database ensures that statistical findings derived from it are generally representative and reliable. This database offers detailed information on various aspects of cancer cases, such as patient demographics, cancer type, onset time, treatment approaches, and follow-up outcomes. Researchers can utilize this wealth of data to gain insights into the impact of cancer and develop effective strategies for diagnosis, treatment, and prevention.
In summary, an extensive dataset was utilized to develop a competing risk model for the prediction of cause-specific mortality in DLBCL patients. The model was effectively visualized as a nomogram and displayed favorable predictive performance, offering valuable information. However, it is crucial to acknowledge certain limitations within this study. Firstly, although the model exhibited satisfactory performance within both the training and validation cohorts, external validation remains necessary and is planned for the subsequent phase of our research. Secondly, due to constraints imposed by public databases, certain variables of interest were regrettably excluded from this investigation, including the specific chemotherapy agents administered to the patients. Furthermore, the lack of clarity in the categorization of certain variables within the database hinders the interpretation of their clinical significance. One such instance is the subcategory labeled as “Other”. Additionally, the potential impact of small subcategorical sample sizes on the model’s performance should be taken into consideration. However, it should be noted that the large sample sizes in this study mitigated this concern.
Based on the SEER database, we have successfully developed a competing risk model for predicting the specific prognosis of DLBCL patients. The model has shown excellent performance in terms of its predictive accuracy. Among the various predictors evaluated, patient age emerges as the most crucial independent factor associated with DLBCL-specific mortality. Moreover, Ann Arbor stage and chemotherapy also demonstrate significant importance in predicting the prognosis. The clinical implications of our model are noteworthy as it aids clinicians in promptly identifying high-risk DLBCL patients. Consequently, this would facilitate the implementation of targeted clinical interventions and ultimately lead to improved patient outcomes.
Availability of data and materials
On reasonable request, the corresponding author will provide the information supporting the study’s conclusions.
Arber DA, Orazi A, Hasserjian R, Thiele J, Borowitz MJ, Le Beau MM, Bloomfield CD, Cazzola M, Vardiman JW. The 2016 revision to the World Health Organization classification of myeloid neoplasms and acute leukemia. Blood. 2016;127(20):2391–405.
Article CAS PubMed Google Scholar
Liang XJ, Song XY, Wu JL, Liu D, Lin BY, Zhou HS, Wang L. Advances in multi-omics study of prognostic biomarkers of diffuse large B-cell lymphoma. Int J Biol Sci. 2022;18(4):1313–27.
Article CAS PubMed PubMed Central Google Scholar
Zheng W, Lin Q, Issah MA, Liao Z, Shen J. Identification of PLA2G7 as a novel biomarker of diffuse large B cell lymphoma. BMC Cancer. 2021;21(1):927.
Zhu YH, Meng WJ, He LH, Jia YS, Tong ZS. Prognosis analysis of primary breast diffuse large B cell lymphoma. Zhonghua zhong liu za zhi [Chin J Oncol]. 2019;41(3):235–40.
CAS PubMed Google Scholar
Gao F, Wang ZF, Tian L, Dong F, Wang J, Jing HM, Ke XY. A prognostic model of gastrointestinal diffuse large B cell lymphoma. Med Sci Monit Int Med J Exp Clin Res. 2021;27: e929898.
Google Scholar
Huo YJ, Xu PP, Fu D, Yi HM, Huang YH, Wang L, Wang N, Ji MM, Liu QX, Shi Q, et al. Molecular heterogeneity of CD30+ diffuse large B-cell lymphoma with prognostic significance and therapeutic implication. Blood Cancer J. 2022;12(3):48.
Article PubMed PubMed Central Google Scholar
He J, Chen Z, Xue Q, Sun P, Wang Y, Zhu C, Shi W. Identification of molecular subtypes and a novel prognostic model of diffuse large B-cell lymphoma based on a metabolism-associated gene signature. J Transl Med. 2022;20(1):186.
Xu H, Li Y, Jiang Y, Wang J, Sun H, Wu W, Lv Y, Liu S, Zhai Y, Tian L, et al. A novel defined super-enhancer associated gene signature to predict prognosis in patients with diffuse large B-cell lymphoma. Front Genet. 2022;13: 827840.
Austin PC, Lee DS, Fine JP. Introduction to the analysis of survival data in the presence of competing risks. Circulation. 2016;133(6):601–9.
Mondello P, Mian M. Frontline treatment of diffuse large B-cell lymphoma: beyond R-CHOP. Hematol Oncol. 2019;37(4):333–44.
Article PubMed Google Scholar
He MY, Kridel R. Treatment resistance in diffuse large B-cell lymphoma. Leukemia. 2021;35(8):2151–65.
Ng AK, Yahalom J, Goda JS, Constine LS, Pinnix CC, Kelsey CR, Hoppe B, Oguchi M, Suh CO, Wirth A, et al. Role of radiation therapy in patients with relapsed/refractory diffuse large B-cell lymphoma: guidelines from the international lymphoma radiation oncology group. Int J Radiat Oncol Biol Phys. 2018;100(3):652–69.
Ollila TA, Olszewski AJ. Extranodal diffuse large B cell lymphoma: molecular features, prognosis, and risk of central nervous system recurrence. Curr Treat Options Oncol. 2018;19(8):38.
Ayub A, Santana-Rodríguez N, Raad W, Bhora FY. Primary appendiceal lymphoma: clinical characteristics and outcomes of 116 patients. J Surg Res. 2017;207:174–80.
Abbott S, Nikolousis E, Badger I. Intestinal lymphoma—a review of the management of emergency presentations to the general surgeon. Int J Colorectal Dis. 2015;30(2):151–7.
Zhao P, Zhu L, Li L, Zhou S, Qiu L, Qian Z, Xu W, Zhang H. A modified prognostic model in patients with diffuse large B-cell lymphoma treated with immunochemotherapy. Oncol Lett. 2021;21(3):218.
Shen Z, Zhang S, Jiao Y, Shi Y, Zhang H, Wang F, Wang L, Zhu T, Miao Y, Sang W, et al. LASSO model better predicted the prognosis of DLBCL than random forest model: a retrospective multicenter analysis of HHLWG. J Oncol. 2022;2022:1618272.
Yin T, Qi L, Zhou Y, Kong F, Wang S, Yu M, Li F. CD5+ diffuse large B-cell lymphoma has heterogeneous clinical features and poor prognosis: a single-center retrospective study in China. J Int Med Res. 2022;50(9):3000605221110075.
Jiang S, Zhen H, Jiang H. Second primary malignancy in diffuse large B-cell lymphoma patients: a SEER database analysis. Curr Probl Cancer. 2020;44(1): 100502.
Yang S, Chang W, Zhang B, Shang P. What factors are associated with the prognosis of primary testicular diffuse large B-cell lymphoma? A study based on the SEER database. J Cancer Res Clin Oncol. 2023;149(12):10269–78.
Du Y, Wang Y, Li Q, Chang X, Zhang H, Xiao M, Xing S. Risk and outcome of acute myeloid leukaemia among survivors of primary diffuse large B-cell lymphoma: a retrospective observational study based on SEER database. BMJ Open. 2022;12(9): e061699.
Kuczmarski TM, Tramontano AC, Mozessohn L, LaCasce AS, Roemer L, Abel GA, Odejide OO. Mental health disorders and survival among older patients with diffuse large B-cell lymphoma in the USA: a population-based study. Lancet Haematol. 2023;10(7):e530–8.
Liu P-P, Xia Y, Bi X-W, Wang Y, Sun P, Yang H, Li Z-M, Jiang W-Q. Trends in survival of patients with primary gastric diffuse large B-cell lymphoma: an analysis of 7051 cases in the SEER database. Dis Markers. 2018. https://doi.org/10.1155/2018/7473935 .
Shao W, Wang T, Huang Z, Han Z, Zhang J, Huang K. Weakly supervised deep ordinal cox model for survival prediction from whole-slide pathological images. IEEE Trans Med Imaging. 2021;40(12):3739–47.
Southern DA, Faris PD, Brant R, Galbraith PD, Norris CM, Knudtson ML, Ghali WA. Kaplan–Meier methods yielded misleading results in competing risk scenarios. J Clin Epidemiol. 2006;59(10):1110–4.
Johnstone PAS, Spiess PE, Giuliano AR. New directions in penile cancer. Lancet Oncol. 2019;20(1):16–7.
Yang Y, Wang Y, Deng H, Tan C, Li Q, He Z, Wei W, Zhou E, Liu Q, Liu J. Development and validation of nomograms predicting survival in Chinese patients with triple negative breast cancer. BMC Cancer. 2019;19(1):541.
Moll M, Qiao D, Regan EA, Hunninghake GM, Make BJ, Tal-Singer R, McGeachie MJ, Castaldi PJ, SanJoseEstepar R, Washko GR, et al. Machine learning and prediction of all-cause mortality in COPD. Chest. 2020;158(3):952–64.
Poirion OB, Jing Z, Chaudhary K, Huang S, Garmire LX. DeepProg: an ensemble of deep-learning and machine-learning models for prognosis prediction using multi-omics data. Genome Med. 2021;13(1):112.
Ambale-Venkatesh B, Yang X, Wu CO, Liu K, Hundley WG, McClelland R, Gomes AS, Folsom AR, Shea S, Guallar E, et al. Cardiovascular event prediction by machine learning: the multi-ethnic study of atherosclerosis. Circ Res. 2017;121(9):1092–101.
Khera R, Haimovich J, Hurley NC, McNamara R, Spertus JA, Desai N, Rumsfeld JS, Masoudi FA, Huang C, Normand SL, et al. Use of Machine learning models to predict death after acute myocardial infarction. JAMA Cardiol. 2021;6(6):633–41.
Balachandran VP, Gonen M, Smith JJ, DeMatteo RP. Nomograms in oncology: more than meets the eye. Lancet Oncol. 2015;16(4):e173-180.
Doll KM, Rademaker A, Sosa JA. Practical guide to surgical data sets: surveillance, epidemiology, and end results (SEER) database. JAMA Surg. 2018;153(6):588–9.
Download references
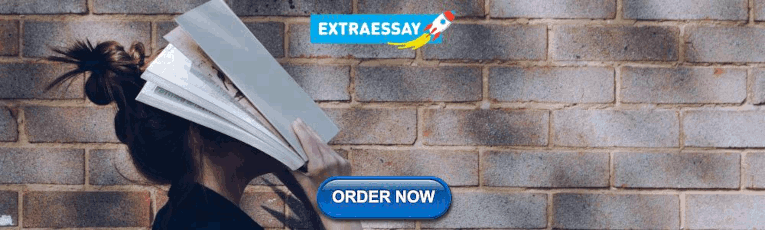
Acknowledgements
Not applicable.
The authors declare that they did not receive any funding from any source.
Author information
Authors and affiliations.
Department of Hematology, Taixing People’s Hospital, No. 98, Runtai South Road, Taixing, 225400, Jiangsu, China
Hui Xu, Chunmei Ye, Jun Li & Guo Ji
Taixing People’s Hospital, Taixing, Jiangsu, China
You can also search for this author in PubMed Google Scholar
Contributions
Conception and design: Hui Xu and Guo Ji were responsible for the conception and design of the study. Jun Li and Hui Xu provided administrative support. Provision of study materials or patients: Rong Yan was responsible for providing the study materials or patients. Collection and assembly of data: Hui Xu, Rong Yan, and Chunmei Ye collected and assembled the data. Data analysis and interpretation: Hui Xu and Rong Yan conducted the data analysis and interpretation. Manuscript writing: all authors contributed to writing the manuscript. Final approval of manuscript: all authors gave their final approval of the manuscript.
Corresponding author
Correspondence to Guo Ji .
Ethics declarations
Ethics approval and consent to participate, consent for publication, competing interests.
The authors have no conflicts of interest to declare.
Additional information
Publisher's note.
Springer Nature remains neutral with regard to jurisdictional claims in published maps and institutional affiliations.
Supplementary Information
Additional file 1: table s1..
The results of univariate and multivariate analysis in competing risk model.
Rights and permissions
Open Access This article is licensed under a Creative Commons Attribution 4.0 International License, which permits use, sharing, adaptation, distribution and reproduction in any medium or format, as long as you give appropriate credit to the original author(s) and the source, provide a link to the Creative Commons licence, and indicate if changes were made. The images or other third party material in this article are included in the article's Creative Commons licence, unless indicated otherwise in a credit line to the material. If material is not included in the article's Creative Commons licence and your intended use is not permitted by statutory regulation or exceeds the permitted use, you will need to obtain permission directly from the copyright holder. To view a copy of this licence, visit http://creativecommons.org/licenses/by/4.0/ . The Creative Commons Public Domain Dedication waiver ( http://creativecommons.org/publicdomain/zero/1.0/ ) applies to the data made available in this article, unless otherwise stated in a credit line to the data.
Reprints and permissions
About this article
Cite this article.
Xu, H., Yan, R., Ye, C. et al. Specific mortality in patients with diffuse large B-cell lymphoma: a retrospective analysis based on the surveillance, epidemiology, and end results database. Eur J Med Res 29 , 241 (2024). https://doi.org/10.1186/s40001-024-01833-4
Download citation
Received : 17 January 2024
Accepted : 06 April 2024
Published : 20 April 2024
DOI : https://doi.org/10.1186/s40001-024-01833-4
Share this article
Anyone you share the following link with will be able to read this content:
Sorry, a shareable link is not currently available for this article.
Provided by the Springer Nature SharedIt content-sharing initiative
- Diffuse large B-cell lymphoma
- Competing risk model
- Fine and Gray regression
- SEER database
European Journal of Medical Research
ISSN: 2047-783X
- Submission enquiries: [email protected]
- General enquiries: [email protected]
- Research article
- Open access
- Published: 20 April 2024
Relationship between lipid metabolism, coagulation and other blood indices and etiology and staging of non-traumatic femoral head necrosis: a multivariate logistic regression-based analysis
- Ximing Yu 1 , 2 na1 ,
- Shilu Dou 2 na1 ,
- Liaodong Lu 2 ,
- Meng Wang 2 ,
- Zhongfeng Li 2 &
- Dongwei Wang 1 , 2
Journal of Orthopaedic Surgery and Research volume 19 , Article number: 251 ( 2024 ) Cite this article
59 Accesses
Metrics details
To analyze the relationship between lipid metabolism, coagulation function, and bone metabolism and the contributing factor and staging of non-traumatic femoral head necrosis, and to further investigate the factors influencing the blood indicators related to the staging of non-traumatic femoral head necrosis.
The medical records of patients with femoral head necrosis were retrieved from the inpatient medical record management system, and the lipid metabolism, bone metabolism, and coagulation indices of non-traumatic femoral head necrosis (including alcoholic, hormonal, and idiopathic group) were obtained according to the inclusion and exclusion criteria, including Low-Density Lipoprotein Cholesterol, Triglycerides, Non-High-Density Lipoprotein Cholesterol, Apolipoprotein A1, Apolipoprotein (B), Apolipoprotein (E), Uric Acid, Alkaline Phosphatase, Bone-specific Alkaline Phosphatase, Activated Partial Thromboplastin Time, Prothrombin Time, D-dimer, Platelet count. The relationship between these blood indices and the different stages under different causative factors was compared, and the factors influencing the stages of non-traumatic femoral head necrosis were analyzed using multivariate logistic regression.
(i) Gender, Age and BMI stratification, Low-density Lipoprotein Cholesterol, Triglycerides, Non-High-density Lipoprotein Cholesterol, Apolipoprotein (B), Apolipoprotein (E), Uric Acid, Bone-specific Alkaline Phosphatase, Activated Partial Thromboplastin Time, Plasminogen Time, D-dimer, and Platelet count of the alcohol group were statistically different when compared among the different ARCO staging groups; (ii) The differences in Age and BMI stratification, Triglycerides, Non-High-density Lipoprotein Cholesterol, Apolipoprotein A1, Apolipoprotein B, Apolipoprotein E, Uric Acid, Bone-specific Alkaline Phosphatase, Activated Partial Thromboplastin Time, Plasminogen Time, D-dimer, and Platelet count were statistically significant when compared among the different phases in the hormone group ( P < 0.05); (iii) The differences in Age and BMI stratification, Non-High-Density Lipoprotein Cholesterol, Apolipoprotein A1, Apolipoprotein (B), Apolipoprotein (E), Uric Acid, Activated Partial Thromboplastin Time, D-dimer, and Platelet count were statistically significant when compared among the different stages in the idiopathic group ( P < 0.05); (v) Statistically significant indicators were included in the multivariate logistic regression analysis, excluding the highly correlated bone-specific alkaline phosphatase, and the results showed that Low-density lipoprotein was negatively correlated with changes in the course of ARCO, and Non-High-Density Lipoprotein cholesterol, Apo B, Activated Partial Thromboplastin Time, and Platelet count were significantly and positively correlated with disease progression.
An abnormal hypercoagulable state as well as an abnormal hyperlipidemic state are risk factors for the progression of non-traumatic femoral head necrosis under various exposure factors, as indicated by Non-High-Density Lipoprotein Cholesterol, Apolipoprotein B, Activated Fractional Thromboplastin Time, and Platelet Counts.
Introduction
Osteonecrosis of the femoral head (ONFH) is a common clinical disease with a complex etiology, in which insufficient blood supply to the femoral head is the key causative factor for its development [ 1 ]. When it is found in the clinic, it is usually characterized by structural changes of the femoral head and different degrees of collapse, accompanied by pain and activity limitation of the affected side of the hip joint, which ultimately leads to the loss of hip function and seriously affects the quality of life [ 2 ]. Non-traumatic necrosis of the femoral head (NONFH) has a high incidence in middle and young-aged people, and a study in the late 1990s showed that 10,000–20,000 new patients were diagnosed with NONFH in the United States each year [ 3 ], and according to a recent epidemiologic statistic [ 4 ], the number of cases of NONFH in the Chinese population aged 15 years and older is forecasted to be 8.12 million. Therefore, clarifying the pathogenesis and targeting interventional therapy to slow down the progression of the disease is a key concern for clinicians. Currently, the etiology of NONFH has been considered to include coagulation disorders, abnormal lipid metabolism, oxidative stress, fat embolism, vascular endothelial disorders as well as thrombosis, diabetes mellitus, and genetic variation [ 5 , 6 ], and the main risk factors and estimated frequencies include Steroid-induced necrosis of the femoral head (SONFH) with long-term use of steroids (35–40%), alcoholic osteonecrosis of the femoral head(AONFH) with chronic heavy alcohol use (20–40%) and idiopathic osteonecrosis of the femoral head(IONFH)(20–40%) [ 7 ]. In addition, due to the rapid progression of NONFH, the clinical diagnosis is mainly made by patients’ symptomatic complaints and imaging manifestations, and there is no accurate method or index for the clinical detection of each stage of NONFH. Studies have shown that some blood indicators can be used as clinical indicators to monitor the progression of NONFH [ 8 ], and the model is stable by constructing a prediction model to analyze indicators such as total cholesterol level, triglyceride level, white blood cell count, gender and platelet count, which is used for early screening and diagnosis of NONFH. Currently, Some studies have investigated the correlation between lipid metabolism and coagulation abnormalities and the development of femoral head necrosis, but there are no reports on the relationship between lipid metabolism, coagulation function, and bone metabolism-related indexes and the etiology of NONFH and its staging, so it is necessary to further investigate this situation, to clarify the impact of lipid metabolism, bone metabolism and coagulation disorders on the process of NONFH. The study is necessary to further investigate this situation to clarify the effects of lipid metabolism, bone metabolism, and coagulation disorders on the progression of NONFH.
Participants
This study investigated the medical records of 1052 NONFH patients admitted to the Department of Bone and Joint of Liaocheng City Hospital of Traditional Chinese Medicine affiliated Shandong University of Traditional Chinese Medicine(SDUTCM) from June 2019 to June 2023, and the case inclusion criteria were referred to the diagnostic criteria of NONFH in the Guidelines for Integrative Diagnosis and Treatment of NONFH in Western and Eastern Medicine issued by the Chinese Society of Traditional Chinese Medicine in 2023 [ 9 ], (1) the clinical features were characterized by predominantly pain in the hip, buttock or groin area, occasionally accompanied by knee pain and limited hip internal rotation; (2) diagnosis of NONFH by X-ray, MRI and CT manifestations; and (3) absence of direct trauma, cardiovascular and cerebrovascular diseases, ankylosing spondylitis, metabolic disorders or bone metastases. In addition to this according to ZHAO [ 4 ]’s description of the pathogenesis of NONFH, according to the history of alcohol overdrinking(alcohol overdrinking [ 10 ] were defined as drinking ≥ 40 g of alcohol per day for men and ≥ 20 g for women in the past 12 months), hormone overdos(hormone overdos [ 11 ] was defined as ≥ 2 g per day of systemic steroids including prednisone or other isotonic drugs in the past 3 months), and no specific history of the disease were splited into the alcohol group, hormone group, and idiopathic group. The exclusion criteria were (1) incomplete medical records or not the first diagnosis of NONFH; (2) incomplete clinical data; (3) involved serious diseases of vital organs or accompanied by malignant tumors; (4) previous medication for severe hepatic impairment or steatohepatitis due to heavy alcohol consumption and hormone overdose. 260 cases in the alcohol group, 283 cases in the hormone group, and 252 cases in the idiopathic group were obtained, and the combination of Association Research Circulation Osseous (ARCO) 2019 ONFH Combined with the ARCO 2019 ONFH staging criteria [ 12 ], we finally obtained 44 cases of ARCO stage II, 111 cases of stage III, and 105 cases of stage IV in the alcohol group; 63 cases of ARCO stage II, 132 cases of stage III, and 88 cases of stage IV in the hormone group; and 42 cases of ARCO stage II, 119 cases of stage III, and 91 cases of stage IV in the idiopathic group.
Clinical data
This retrospective, single-center observational study extracted general information from the inpatient medical record management system for all patients on admission, including age, sex, region, BMI [kg/m2], and history of smoking and alcohol consumption; and extracted all blood laboratory markers after fasting for at least 10 h, including Low-density Lipoprotein Cholesterol (LDL), Triglycerides (TG), Non-High-density Lipoprotein Cholesterol (serum Total Cholesterol-High-density Lipoprotein cholesterol) (Non-HDL), Apolipoprotein A1 (ApoA1), Apolipoprotein B (ApoB), Apolipoprotein E (ApoE), Uric Acid (UA), Alkaline Phosphatase (ALP), Bone-specific Alkaline Phosphatase (b-ALP), Activated Artial Thromboplastin Time (APTT), Prothrombin Time (PT), D-dimer (D-D), and Platelet count (PLT). Blood laboratory parameters of patients with different ARCO stages in each etiological group were analyzed, and further logistic regression analysis was performed to analyze the independent risk factors for the course of NONFN.
Ethical aspects
This study strictly adhered to the Declaration of Helsinki and was approved by the Ethics Committee of Liaocheng City Hospital of Traditional Chinese Medicine (No. LLW2024003). All patients gave informed consent to participate in this study, and all experimental data were used only by the investigators for disease research, and any public reporting of the results of this study will not reveal any private information.
Statistical analysis
IBM SPSS Statistics version 26.0 was used for statistical analysis. Shapiro-Wilk was used to test the normality of the data.Kruskal-Wallis H test was used to compare continuous data in multiple groups.Two-way comparisons were analyzed and evaluated using the Mann-Whitney U test. Categorical variables were analyzed using the chi-squared test.All statistical analyses were performed using two-tailed tests, and differences were considered significant at P < 0.05.Normally distributed measures are expressed as mean ± SD, and non-normally distributed measures are expressed as median and quartiles [M(Q1, Q3)].All statistically significant indicators were included in Spearman’s correlation analysis, and after excluding highly correlated laboratory indicators, the remaining results were subjected to multivariate logistic regression to analyze the correlates of NONFH, and the level of the test was set at α = 0.05, which ultimately yielded the risk factors for the course of NONFH.
Demographic characteristics of the study participants
A total of 795 cases of NONFH patients were included, including 260 cases in the alcohol group, 283 cases in the hormone group and 252 cases in the idiopathic group, and the following results were obtained by analyzing the gender, age and BMI of different ARCO stages among the groups: there were more male patients than female patients in different stages in the alcohol group, and the difference was statistically significant ( P < 0.05), With regard to age, the majority of people aged 45–59 years in ARCO stages III and IV and the majority of people under 45 years in stage II, the difference was significant ( P < 0.001), ARCO stage II and III with BMI < 25 kg/m2 majority, ARCO stage IV BMI ≥ 25 kg/m2 accounted for 58.1%, the difference was significant ( P < 0.001); hormone group and the idiopathic group of the different ARCO staging between the sexes compared were not statistically significant ( P = 0.05); hormone group and the idiopathic group of the different ARCO staging of gender comparison of the differences are not statistically In the hormone group, the age groups with different stages were 60 years old and above (47.6%), 45 years old and above (38.6%), and 45–59 years old and above (81.8%), while the age groups with different stages in the idiopathic group were 45 years old and above (50%), 60 years old and above (38.4%), and 60 years old and above (38.4%), and in the idiopathic group were 60 years old and above (38.4%). (38.4%), stage IV 45–59 years old (85.7%), the difference between the two groups is significant ( P < 0.001); BMI hormone group III, IV stage BMI 25 kg/m2 or more accounted for more ( P < 0.001), the special group II, III stage BMI25kg/m2 or less accounted for more ( P < 0.001). (Table 1 )
Grouped single factor analysis of laboratory indicators
Only TG in the alcohol group conformed to a normal distribution for the whole group, the rest were continuous variables and did not conform to the normal distribution, analyzed by Kurskai-Wallis H-test, and LDL, N-HDL, TG, ApoB, UA, ApoE, b-ALP, APTT, PT, D-D, and PLT factors were compared between different ARCO staging groups The difference was statistically significant (Table 2 ). LDL and PLT in the hormone group conformed to normal distribution, the rest did not, and the differences between N-HDL, TG, ApoB, ApoA1, UA, ApoE, b-ALP, APTT, PT, D-D, and PLT were tested to be statistically significant when compared among different staging groups ( P < 0.01) (Table 3 ). Only PLT in the idiopathic group conformed to normal distribution, and the analysis revealed statistically significant differences in N-HDL, ApoB, ApoA1, UA, ApoE, APTT, D-D, and PLT when comparing them between different ARCO staging periods (Table 4 ).
Multivariate Logistic Regression Analysis
ARCO stage II was used as a control, and age, gender, and BMI were assigned to illustrate age, etiology, body mass, and gender: age (1 = < 45 years, 2 = 45–59 years, 3 = ≥ 60 years), etiology (1 = alcoholic, 2 = hormonal, and 3 = idiopathic), gender (male = 1, female = 0), and defining a BMI of ≥ 25 kg/m2 as overweight (yes = 0, no = 1 ), Spearman’s correlation analysis was performed for the indicators that were statistically different ( P < 0.05) in the univariate analysis. b-ALP showed a high correlation with other indicators at the 0.05 level, so b-ALP was excluded, and LDL, N-HDL, TG, ApoB, ApoA1, UA, ApoE, and APTT were included. pt, D-D, and PLT were analyzed by multivariate Logistic regression analysis. The results suggested that the model chi-square value was 1433.064, with a significance of < 0.001; the three pseudo-R2 values of Cox and Snell, Nagelkerke, and Mc Fadden were 0.836, 0.955, and 0.868, which indicated a good model fit, and the model had the highest accuracy in predicting the stage III course of ARCO, which amounted to 98.1%, and the overall prediction accuracy was 97.4%.
Comparing ARCO stage III with stage II, LDL, TG, and ApoA1 were negatively correlated with ARCO stage III onset, suggesting that the risk of ARCO stage III onset was lower than that of stage II against the background of these indexes ( P < 0.05), with the corresponding OR and 95% CL of 0.153 (95% CL: 0.09–0.259, P < 0.001), 0.87 (95% CL. 0.611–1.239, P = 0.44), 0.654 (95% CL: 0.481–0.889, P = 0.007); N-HDL, ApoB, UA, APTT, PT, PLT, and age 45–59 years were positively correlated with the onset of Stage III, with an increased probability of onset, corresponding to an OR and 95% CL of 5.000 (95% CL. 3.445–7.257, P < 0.001), 5.009 (95% CL: 2.572–9.754, P < 0.001), 1.008 (95% CL: 1.000-1.016, P = 0.048), 1.336 (95% CL: 1.063–1.679, P = 0.013), 1.738 (95% CL: 1.143–2.642, P = 0.01), 1.024 (95% CL: 1.009–1.039, P = 0.002), and 50.406 (95% CL: 8.941-284.172, P < 0.001).ARCO stage IV vs. stage II, LDL and BMI < 25 kg/m2 suggested an association with reduced risk of morbidity ( P < 0.05), N-HDL, ApoB, APTT, D-D, and age 45–59 years wererelated to the increased risk of morbidity, and their OR and 95% CL were 16.592 (95% CL:10.886–25.287, P < 0.001), 16.63 (95% CL:7.731–35.776, P < 0.001) respectively, 1.335 (95% CL: 1.061–1.678, P = 0.013), 3.227 (95% CL: 2.221–4.668, P < 0.001), 571.533 (95% CL: 84.785-3852.674, P < 0.001). (Table 5 )
At present, NONFH is still recognized as a refractory disease in the world, with young and middle-aged people in the majority, and about 80% of them involve bilateral hip joints [ 9 ], and most of the patients suffer from recurrent pain and severe dysfunction for a long period, even losing their mobility, and eventually have to undergo total hip arthroplasty.In the younger age group, it is important to identify risk factors for morbidity and cautiously pursue hip-conserving surgical treatments to alleviate symptoms [ 13 ]. At present, ARCO staging changes and imaging changes of femoral head collapse are mainly used to evaluate the progression of femoral head necrosis [ 14 ], and several studies have reported that the risk factors influencing the occurrence of NONFH endpoints include: The location, extent, and morphology of necrotic areas of the femoral head, the signal characteristics of necrotic areas, and the strength of the femoral neck [ 15 , 16 ], and there are no articles on whether there is a relationship between clinical laboratory indicators, such as coagulation indicators, lipid metabolism indicators, and bone metabolism indicators, and the progression of NONFH. There are no articles to indicate whether there is a relationship between clinical laboratory indicators, such as coagulation indicators, lipid metabolism indicators, and bone metabolism indicators, and the course of NONFH, and this study focuses on the above indicators to explore the risk factors for the development of NONFH other than imaging factors.
The results of this study showed that elevated LDL was negatively correlated with NONFH disease progression, and in the alcohol group, LDL levels were higher in ARCO stage IV than in ARCO stage II ( P < 0.05);N-HDL and ApoB in the alcohol group, hormone group, and idiopathic group increased significantly with disease progression ( P < 0.001), and the results of multivariate logistic regression analysis showed that for every 1-unit increase in N-HDL, while other indicators remained unchanged, the probability of ARCO stage III and IV was 5-fold and 16. 592-fold higher than that of stage II, and that of ApoB was 5.009-fold and 16.63-fold higher, respectively, indicating that N-HDL and ApoB are risk factors for disease progression in NONFH.Currently, there are more views that hyperlipidemia is a risk factor for the induction of femoral head necrosis in people who abuse hormones and long-term alcoholism, but in the present study, although it was observed that the levels of LDL and TG in the alcohol and hormone groups were elevated higher than normal with the progression of the disease, there was no positive correlation with the actual disease progression ( P > 0.05),In general, elevated serum LDL will be deposited in the arterial walls of blood vessels, gradually forming atherosclerotic plaques and affecting the flow rate of blood to the femoral head, but the factors affecting elevated serum LDL and TG are complex, It has been suggested that the congenital presence of hyperlipidemia may not increase the risk of osteonecrosis in rabbits and that a diet rich in lanolin may also be a protective factor [ 17 ].Another cause of abnormally elevated lipid metabolism markers may be the patient’s comorbidities with varying degrees of liver dysfunction or fatty liver. It has been previously reported [ 18 , 19 ] that liver dysfunction or definite alcoholic/non-alcoholic fatty liver was observed in patients with alcohol-induced NONFH and steroid-induced NONFH, suggesting that any hepatic impairment (including fatty liver as well as oxidative stress, etc.) is a risk factor for NONFH. ) is a risk factor for NONFH, and this was supported by the fact that the abnormal elevation of lipid metabolism indices such as LDL and TG was observed to be higher in the alcohol and hormone groups than in the idiopathic group. This is also supported by the fact that the alcohol and hormone groups had higher LDL and TG levels than the idiopathic group.In addition, the present study observed the changes of N-HDL in different etiologic groups in different ARCO stages, which is consistent with the results of a cross-sectional study [ 4 ]that N-HDL is a reliable indicator in lipid metabolism metrics for assessing the progression of NONFH, N-HDL is also a major risk factor for atherosclerotic disease and supports the increased risk of secondary coronary heart disease in NONFH patients.In conclusion, the relationship between changes in various indices of lipid metabolism and the progression of NONFH remains controversial, and further studies of dyslipidemia and obesity and comorbidities between different etiologies are needed.
In terms of bone metabolism, this study found that alkaline phosphatase generally tended to increase with disease progression in the different etiologic groups, but the difference between the groups was not statistically significant ( P > 0.05);In contrast, elevated bone-specific alkaline phosphatase, one of the phenotypic markers of osteoblasts, is generally seen in metabolic bone diseases with high turnove [ 20 ].A bone histopathologic study of a rabbit model of SONFH [ 21 ] found that serum b-ALP levels began to increase from the 4th week of modeling and decreased by the 8th week, while the number of osteoblasts decreased significantly from the 4th week.In the present study, it was observed that b-ALP levels in the alcohol and hormone groups were significantly higher in ARCO stage III than in the other two stages, and serum b-ALP levels were lowest in stage IV ( P < 0.05), and there was also a trend in the idiopathic group, but the difference between the different stages was not significant ( P = 0.545). Decreased NBAP implies increased apoptosis of periarticular osteoblasts, and prolonged exposure to such an inflammatory environment severely affects hip angiogenesis as well as bone repair and regeneration [ 22 ]. Taken together with the results of the present study, this suggests that with the progression of NONFH, the destruction of the patient’s local bone tissues, as well as the continuous erosion of inflammatory factors, there will be a transient enhancement of bone remodeling in stage III, followed by a further increase in bone resorption.
The present study again validated PLT as a risk factor for disease progression in NONFH. Multiple Logistic regression analysis showed that for every 1-unit decrease in PLT, the odds of ARCO stage progression to stages III and IV were 1.024 and 1.011 times higher, respectively.In different groups, PLT levels were elevated in ARCO stage III compared to ARCO stage II, whereas PLT was significantly lower in ARCO stage IV than in stage II ( P < 0.05).The main functions of platelets include coagulation and repair damaged blood vessels [ 23 ], analyzed with the results, this situation may be because when NONFH progresses, the blood supply to the femoral head is damaged, and at this time, platelets are stimulated to start the vascular repair, The surface viscosity increases and coagulates into a cluster, and at the same time participates in coagulation, forming clots with the blood cells, so it may be possible to have a transient increase in ARCO III stage and then decrease in the Therefore, there may be a transient increase in ARCO III and then decrease in ARCO III.Platelet-rich plasma (PRP) has been widely used in recent years in the biologic treatment of musculoskeletal disorders with the ability to promote injury repair and healing [ 24 ], and the application of PRP arthroplasty injections, alone or in combination, to the treatment of NONFH is currently at the stage of exploratory trials.In addition to PLT, this study also found that APTT was positively correlated with NONFH disease progression, and among the different groups, the reduction in APTT was smaller in ARCO stage III than in stage II ( P < 0.05),The APTT is commonly used as a clinical screening test for the coagulation activity of the endogenous coagulation system, and a shortening usually indicates increased coagulation factor VIII activity as well as a hypercoagulable state of the blood, etc. The results indicate that this stage is weaker than stage II hypercoagulability, and there is a rich perfusion of blood flow; Previous studies have shown that thrombus is a risk factor for NONFH and that perfusion in the necrotic area of the femoral head significantly influences the progression of osteonecrosis, with ARCO stage III being faster than stage II [ 25 ]. PT also showed that ARCO stage IV was shorter than stages II and III and stage II was shorter than stage III in different groups, indicating that stage IV was more prone to hypercoagulable and thrombotic states compared to the first two stages.Another indicator of hypercoagulability in the body, D-dimer, was found to be 3.227 times more likely to progress to ARCO stage IV than stage II for every 1-unit increase in D-dimer in this study, and the results once again demonstrated that the progression of femoral head necrosis is closely related to the hypercoagulable state of the blood. A study using matrix-assisted laser-resolved ionization time-of-flight mass spectrometry and gene sequencing to analyze 146 patients with femoral head necrosis [ 26 ] showed that the rs6020 G-to-A polymorphism was detected in 88.9% of the patients, and 87.6% of the patients had an abnormal hypercoagulable state, Cause analysis revealed that when patients with the rs6020 polymorphism were exposed to risk factors such as alcohol and hormone abuse, it would leads to abnormal hypercoagulation, which in turn would lead to thromboembolism of the femoral head. This shows that changes in some coagulation function indices are closely related to the progression of NONFH.
There are some limitations in this study, first of all, the study retrospectively analyzed the medical records of patients who visited the hospital in the past 4 years, and most of the patients came to the clinic mainly because of obvious hip symptoms, and according to ARCO staging, most of them were in stage III or above, which resulted in unequal number of cases in each staging; Second, with exposure factors such as hormones and alcohol, The patients were mostly comorbid with other underlying diseases or had varying degrees of liver injury, which may have affected the results of this study, and this study did not stratify whether the liver injury present in each group was alcoholic or hormonal.In future studies, we plan to combine imaging data from patients with different exposure factors for further analysis, as well as to increase the sample size and control for the influence of underlying disease on the findings, in order to further analyze the risk factors for progression of NONFH disease.
In the present study, it was observed that N-HDL, ApoB, APTT, and PLT in lipid metabolism and coagulation indices in different etiologic groups were risk factors for disease progression of NONFH, whereas LDL might be a protective factor for NONFH progression.NONFH patients with abnormal blood hypercoagulability and dyslipidemia should be taken seriously in clinical practice and disease progression should be considered. The bone turnover index b-ALP was specific in different ARCO stages in the alcohol and hormone groups, but the correlation with disease progression in NONFH needs further study.
Data availability
Data is provided within the manuscript or supplementary information files.
Zhao D, Zhang F, ,Wang B, et al. Guidelines for clinical diagnosis and treatment of osteonecrosis of the femoral head in adults (2019 version)[J]. J Orthop Translation. 2020;21:100–10.
Article Google Scholar
Liu Y, Zhao D, ,Wang W et al. Efficacy of Core Decompression for treatment of canine femoral Head Osteonecrosis Induced by arterial ischaemia and venous Congestion[J].HIP International,2017,27(4):406–11.
Mont MA, Hungerford DS. Non-traumatic avascular necrosis of the femoral head. J Bone Joint Surg Am. 1995;77(3):459–74.
Article CAS PubMed Google Scholar
Zhao DYM, ,Hu K, et al. Prevalence of nontraumatic osteonecrosis of the femoral head and its Associated Risk factors in the Chinese Population: results from a nationally Representative Survey[J]. Chin Med J. 2015;128(21):2843–50.
Article CAS PubMed PubMed Central Google Scholar
Nagasawa K, Tada Y, Koarada S, et al. Very early development of steroid-associated osteonecrosis of femoral head in systemic lupus erythematosus: prospective study by MRI. Lupus. 2005;14(5):385–90.
Huang C, Wen Z, Niu J, et al. Steroid-Induced Osteonecrosis of the femoral head: Novel Insight into the roles of Bone endothelial cells in Pathogenesis and Treatment. Front Cell Dev Biol. 2021;9:777697. https://doi.org/10.3389/fcell.2021.777697 .
Article PubMed PubMed Central Google Scholar
Bradway JK, Morrey BF. The natural history of the silent hip in bilateral atraumatic osteonecrosis. J Arthroplasty. 1993;8(4):383–7.
Xu Q, Chen H, Chen S, et al. Development and validation of a nomogram for predicting the probability of nontraumatic osteonecrosis of the femoral head in Chinese population. Sci Rep. 2020;10(1):20660. https://doi.org/10.1038/s41598-020-77693-9 .
China Association of Chinese Medicine. Guidelines for the diagnosis and treatment of non-traumatic osteonecrosis of the femoral head with integrated Chinese and Western medicine,2023. www.cacm.org.cn .
Holmes J, Sasso A, Alava MH, et al. The distribution of alcohol consumption and heavy episodic drinking across British drinking occasions in 2019: a cross-sectional, latent, class analysis of event-level drinking diary data. Lancet. 2022;400(Suppl 1):S50. https://doi.org/10.1016/S0140-6736(22)02260-7 .
Mont MA, Jones LC,Hungerford DS. Nontraumatic osteonecrosis of the femoral head: ten years later. J Bone Joint Surg Am. 2006;88(5):1117–32. https://doi.org/10.2106/JBJS.E.01041 .
Article PubMed Google Scholar
Yoon BH, Mont MA, Koo KH, et al. The 2019 Revised Version of Association Research Circulation Osseous Staging System of Osteonecrosis of the femoral head. J Arthroplasty. 2020;35(4):933–40. https://doi.org/10.1016/j.arth.2019.11.029 .
Migliorini F, Maffulli N, Baroncini A, et al. Prognostic factors in the management of osteonecrosis of the femoral head: a systematic review. Surgeon. 2022. https://doi.org/10.1016/j.surge.2021.12.004 .
Huang Z, Li T, Lin N, et al. Evaluation of Radiographic outcomes after Core Decompression for Osteonecrosis of the femoral head: the Beijing University of Chinese Medicine X-ray Evaluation Method. J Bone Joint Surg Am. 2021. https://doi.org/10.2106/JBJS.20.00478 .
Li TX, Huang ZQ, Li Y, et al. Prediction of Collapse using patient-specific finite element analysis of osteonecrosis of the femoral head. Orthop Surg. 2019;11(5):794–800. https://doi.org/10.1111/os.12520 .
Lin T, Yang P, Cai K, et al. [Predictive effect of femoral neck strength composite indexes on femoral head collapse in non-traumatic osteonecrosis of femoral head]. Zhongguo Xiu Fu Chong Jian Wai Ke Za Zhi. 2021;35(8):967–72. https://doi.org/10.7507/1002-1892.202103168 .
Zhao G, Yamamoto T, Motomura G, et al. Cholesterol- and lanolin-rich diets may protect against steroid-induced osteonecrosis in rabbits. Acta Orthop. 2013;84(6):593–7. https://doi.org/10.3109/17453674.2013.859421 .
Breitkopf K, Nagy LE, Beier JI, et al. Current experimental perspectives on the clinical progression of alcoholic liver disease. Alcohol Clin Exp Res. 2009;33(10):1647–55. https://doi.org/10.1111/j.1530-0277.2009.01015.x .
Lin X, Zhu D, Wang K, et al. Activation of aldehyde dehydrogenase 2 protects ethanol-induced osteonecrosis of the femoral head in rat model. Cell Prolif. 2022;55(6):e13252. https://doi.org/10.1111/cpr.13252 .
Sahin Ersoy G, Giray B, Subas S, et al. Interpregnancy interval as a risk factor for postmenopausal osteoporosis. Maturitas. 2015;82(2):236–40. https://doi.org/10.1016/j.maturitas.2015.07.014 .
Yang WQ, Jiang ZG, Huang CL. Bone tissue function and pathological changes of hormone-induced avascular necrosis of the femoral head in rabbits. Zhongguo Zuzhi Gongcheng Yanjiu Yu Linchuang Kangfu. 2011;15(50):9319–22.
CAS Google Scholar
Migliorini F, Maffulli N, Eschweiler J, et al. Core decompression isolated or combined with bone marrow-derived cell therapies for femoral head osteonecrosis. Expert Opin Biol Ther. 2021;21(3):423–30. https://doi.org/10.1080/14712598.2021.1862790 .
Migliorini F, La Padula G, Oliva F, et al. Operative management of avascular necrosis of the femoral head in skeletally immature patients: a systematic review. Life (Basel). 2022;12(2). https://doi.org/10.3390/life12020179 .
Luan S, Wang S, Lin C, et al. Comparisons of Ultrasound-guided platelet-rich plasma intra-articular injection and extracorporeal shock Wave Therapy in Treating ARCO I-III symptomatic non-traumatic femoral head necrosis: a Randomized Controlled Clinical Trial. J Pain Res. 2022;15:341–54. https://doi.org/10.2147/JPR.S347961 .
Yan J, Wei QS, Tian YY, et al. The value of ultrasonography in the perfusion of blood flow in femoral head necrosis. Chin J Med Ultrasound (Electronic Edition). 2021;18(3):301–6. https://doi.org/10.3877/cma.j.issn.1672-6448.2021.03.011 .
Peng KT, Huang KC, Huang TW, et al. Single nucleotide polymorphisms other than factor V Leiden are associated with coagulopathy and osteonecrosis of the femoral head in Chinese patients. PLoS ONE. 2014;9(8):e104461. https://doi.org/10.1371/journal.pone.0104461 .
Download references
Acknowledgements
This study was supported by a grant from the Science and Technology Program of Traditional Chinese Medicine of Shandong Province (No. M-2023270).
Author information
Ximing Yu and Shilu Dou are co-first authors.
Authors and Affiliations
The First Clinical College of Shandong University of Traditional Chinese Medicine, Jinan, 252000, China
Ximing Yu & Dongwei Wang
Department of Orthopedics, Liaocheng City Hospital of Traditional Chinese Medicine, Liaocheng, 252000, China
Ximing Yu, Shilu Dou, Liaodong Lu, Meng Wang, Zhongfeng Li & Dongwei Wang
You can also search for this author in PubMed Google Scholar
Contributions
XY: responsible for experimental design, data analysis, manuscript writing, and revision. SD: responsible for literature research, data analysis, and manuscript revision. LL: responsible for literature research, and data collection. MW: responsible for study design and data review. ZL: responsible for data collection. DW: guarantor of integrity of the entire study, definition of intellectual content, manuscript review, and manuscript editing. All authors read and approved the final manuscript.
Corresponding author
Correspondence to Dongwei Wang .
Ethics declarations
Ethics approval and consent to participate.
This study was approved by the Ethics Committee of Liaocheng City Hospital of Traditional Chinese Medicine.
Consent for publication
All patients were informed in detail about the study and signed an informed consent form.
Competing interests
The authors declare no competing interests.
Additional information
Publisher’s note.
Springer Nature remains neutral with regard to jurisdictional claims in published maps and institutional affiliations.
Electronic supplementary material
Below is the link to the electronic supplementary material.
Supplementary Material 1
Rights and permissions.
Open Access This article is licensed under a Creative Commons Attribution 4.0 International License, which permits use, sharing, adaptation, distribution and reproduction in any medium or format, as long as you give appropriate credit to the original author(s) and the source, provide a link to the Creative Commons licence, and indicate if changes were made. The images or other third party material in this article are included in the article’s Creative Commons licence, unless indicated otherwise in a credit line to the material. If material is not included in the article’s Creative Commons licence and your intended use is not permitted by statutory regulation or exceeds the permitted use, you will need to obtain permission directly from the copyright holder. To view a copy of this licence, visit http://creativecommons.org/licenses/by/4.0/ . The Creative Commons Public Domain Dedication waiver ( http://creativecommons.org/publicdomain/zero/1.0/ ) applies to the data made available in this article, unless otherwise stated in a credit line to the data.
Reprints and permissions
About this article
Cite this article.
Yu, X., Dou, S., Lu, L. et al. Relationship between lipid metabolism, coagulation and other blood indices and etiology and staging of non-traumatic femoral head necrosis: a multivariate logistic regression-based analysis. J Orthop Surg Res 19 , 251 (2024). https://doi.org/10.1186/s13018-024-04715-x
Download citation
Received : 15 March 2024
Accepted : 05 April 2024
Published : 20 April 2024
DOI : https://doi.org/10.1186/s13018-024-04715-x
Share this article
Anyone you share the following link with will be able to read this content:
Sorry, a shareable link is not currently available for this article.
Provided by the Springer Nature SharedIt content-sharing initiative
- Non-traumatic necrosis of the femoral head
- Risk factor
- Coagulation indicators
- Biochemical indicators
Journal of Orthopaedic Surgery and Research
ISSN: 1749-799X
- Submission enquiries: [email protected]
- Introduction
- Conclusions
- Article Information
The size of the box representing the point estimate for each study in the forest plot is proportional to the contribution of that study’s weight estimate to the summary estimate. Error bars indicate the 95% CIs. The diamond represents the pooled odds ratio (OR); the lateral tips of the diamond represent the associated 95% CIs. SB indicates suicidal behavior; SI suicidal ideation.
The middle line indicates the overall effect of the meta-analysis, while the 2 lines on either side represent the 95% CIs.
eAppendix 1. Search Terms
eAppendix 2. Quality Assessment According to the Newcastle-Ottawa Scale
eFigure 1. Drapery Plot—Suicidal Behavior
eFigure 2. Drapery Plot—Suicidal Ideation and Death by Suicide
eFigure 3. Model-Averaged Plot of Predictive Factor Importance—Suicidal Behavior
eFigure 4. Model-Averaged Plot of Predictive Factor Importance—Suicidal Ideation
eFigure 5. Graphic Display of Heterogeneity (GOSH) Plot
eFigure 6. Funnel Plots for Suicidal Ideation and Suicide Death
eTable 1. Covariates for Meta-Regression
eTable 2. Effect Moderators for Studies on Suicidal Behavior
eTable 3. Subgroup Analysis on Study Participants’ Mean Age
eTable 4. Association Between Visual Impairment and Suicidality Risk After Exclusion of Potential Outliers
eReferences
Data Sharing Statement
See More About
Sign up for emails based on your interests, select your interests.
Customize your JAMA Network experience by selecting one or more topics from the list below.
- Academic Medicine
- Acid Base, Electrolytes, Fluids
- Allergy and Clinical Immunology
- American Indian or Alaska Natives
- Anesthesiology
- Anticoagulation
- Art and Images in Psychiatry
- Artificial Intelligence
- Assisted Reproduction
- Bleeding and Transfusion
- Caring for the Critically Ill Patient
- Challenges in Clinical Electrocardiography
- Climate and Health
- Climate Change
- Clinical Challenge
- Clinical Decision Support
- Clinical Implications of Basic Neuroscience
- Clinical Pharmacy and Pharmacology
- Complementary and Alternative Medicine
- Consensus Statements
- Coronavirus (COVID-19)
- Critical Care Medicine
- Cultural Competency
- Dental Medicine
- Dermatology
- Diabetes and Endocrinology
- Diagnostic Test Interpretation
- Drug Development
- Electronic Health Records
- Emergency Medicine
- End of Life, Hospice, Palliative Care
- Environmental Health
- Equity, Diversity, and Inclusion
- Facial Plastic Surgery
- Gastroenterology and Hepatology
- Genetics and Genomics
- Genomics and Precision Health
- Global Health
- Guide to Statistics and Methods
- Hair Disorders
- Health Care Delivery Models
- Health Care Economics, Insurance, Payment
- Health Care Quality
- Health Care Reform
- Health Care Safety
- Health Care Workforce
- Health Disparities
- Health Inequities
- Health Policy
- Health Systems Science
- History of Medicine
- Hypertension
- Images in Neurology
- Implementation Science
- Infectious Diseases
- Innovations in Health Care Delivery
- JAMA Infographic
- Law and Medicine
- Leading Change
- Less is More
- LGBTQIA Medicine
- Lifestyle Behaviors
- Medical Coding
- Medical Devices and Equipment
- Medical Education
- Medical Education and Training
- Medical Journals and Publishing
- Mobile Health and Telemedicine
- Narrative Medicine
- Neuroscience and Psychiatry
- Notable Notes
- Nutrition, Obesity, Exercise
- Obstetrics and Gynecology
- Occupational Health
- Ophthalmology
- Orthopedics
- Otolaryngology
- Pain Medicine
- Palliative Care
- Pathology and Laboratory Medicine
- Patient Care
- Patient Information
- Performance Improvement
- Performance Measures
- Perioperative Care and Consultation
- Pharmacoeconomics
- Pharmacoepidemiology
- Pharmacogenetics
- Pharmacy and Clinical Pharmacology
- Physical Medicine and Rehabilitation
- Physical Therapy
- Physician Leadership
- Population Health
- Primary Care
- Professional Well-being
- Professionalism
- Psychiatry and Behavioral Health
- Public Health
- Pulmonary Medicine
- Regulatory Agencies
- Reproductive Health
- Research, Methods, Statistics
- Resuscitation
- Rheumatology
- Risk Management
- Scientific Discovery and the Future of Medicine
- Shared Decision Making and Communication
- Sleep Medicine
- Sports Medicine
- Stem Cell Transplantation
- Substance Use and Addiction Medicine
- Surgical Innovation
- Surgical Pearls
- Teachable Moment
- Technology and Finance
- The Art of JAMA
- The Arts and Medicine
- The Rational Clinical Examination
- Tobacco and e-Cigarettes
- Translational Medicine
- Trauma and Injury
- Treatment Adherence
- Ultrasonography
- Users' Guide to the Medical Literature
- Vaccination
- Venous Thromboembolism
- Veterans Health
- Women's Health
- Workflow and Process
- Wound Care, Infection, Healing
Get the latest research based on your areas of interest.
Others also liked.
- Download PDF
- X Facebook More LinkedIn
Kim CY , Ha A , Shim SR , Hong IH , Chang IB , Kim YK. Visual Impairment and Suicide Risk : A Systematic Review and Meta-Analysis . JAMA Netw Open. 2024;7(4):e247026. doi:10.1001/jamanetworkopen.2024.7026
Manage citations:
© 2024
- Permissions
Visual Impairment and Suicide Risk : A Systematic Review and Meta-Analysis
- 1 Department of Ophthalmology, Seoul National University Hospital, Seoul, Korea
- 2 Department of Ophthalmology, Seoul National University College of Medicine, Seoul, Korea
- 3 Department of Ophthalmology, Jeju National University Hospital, Jeju-si, Korea
- 4 Department of Ophthalmology, Jeju National University College of Medicine, Jeju-si, Korea
- 5 Department of Health and Medical Informatics, Kyungnam University College of Health Sciences, Changwon, Korea
- 6 Department of Ophthalmology, Dongtan Sacred Heart Hospital, Hwaseong, Korea
- 7 Hallym University Medical Center, Hwaseong, Korea
- 8 Seoul ON Eye Clinic, Seoul, Korea
- 9 EyeLight Data Science Laboratory, Seoul National University College of Medicine, Seoul, Korea
- 10 Trinity Biomedical Sciences Institute, Trinity College Dublin, Dublin, Ireland
Question Is visual impairment associated with suicide risk, and if so, what factors contribute to the association?
Findings In this systematic review and meta-analysis including 31 studies and 5 692 769 unique individuals, visual impairment was associated with an increased risk of suicide, including suicidal ideation, suicidal behavior, and suicide death. This elevated risk was particularly pronounced among adolescents with visual impairment.
Meaning These findings suggest an association between visual impairment and an elevated risk of suicidal tendencies, with variations in risk observed across age groups and a particularly pronounced risk among adolescents.
Importance Suicide is a substantial public health concern that involves various recognized contributing factors. Sensory impairments, specifically visual impairment, are deemed potential risk factors. Nonetheless, comprehensive information about associated risk levels and underlying determinants remains limited.
Objective To investigate the association between visual impairment and different aspects of suicide, including the assessment of risk levels and exploration of potential contributing factors.
Data Sources An electronic search was performed in the PubMed, EMBASE, Scopus, and Cochrane Library databases from their inception to February 8, 2024.
Study Selection All published studies were considered without restrictions on study design, publication date, or language.
Data Extraction and Synthesis Two independent reviewers extracted the published data using a standardized procedure in accordance with the Meta-analysis of Observational Studies in Epidemiology ( MOOSE ) and Preferred Reporting Items for Systematic Reviews and Meta-analyses ( PRISMA ) reporting guidelines. Random-effects meta-analyses were used to estimate pooled effect sizes. Multiple meta-regression analyses were conducted to identify potential factors contributing to the association between visual impairment and the risk of suicide.
Main Outcomes and Measures The primary outcome measure was the odds ratio (OR) of suicidal behavior (including suicide attempt and suicide death) for individuals with visual impairment compared with those without. The secondary outcome measures were the pooled ORs of suicidal ideation and suicide death, respectively.
Results A total of 31 population-based studies with 5 692 769 unique individuals (mean [SD] age, 48.4 [8.5] years; 2 965 933 females [52%]) were included. For 17 studies (5 602 285 individuals) that evaluated suicidal behavior, the pooled OR was 2.49 (95% CI, 1.71-3.63). For 21 studies (611 899 individuals) that assessed suicidal ideation, the pooled OR was 2.01 (95% CI, 1.62-2.50). For 8 studies (5 067 113 individuals) investigating the association between visual impairment and suicide death, the pooled OR was 1.89 (95% CI, 1.32-2.71). The multiple meta-regression model identified age group as a predictive factor associated with suicidal behavior, with the studies included suggesting that adolescents were at the highest risk. While this analysis showed moderate heterogeneity for suicide death, high heterogeneity was observed for suicidal behavior and suicidal ideation.
Conclusions and Relevance The findings of this systematic review and meta-analysis support the association between visual impairment and increased risk of suicidal tendencies. The risk differed by age group, with a pronounced risk observed among adolescents.
Suicide, which has an estimated annual death toll of nearly 1 million lives worldwide, 1 is a significant and urgent global public health challenge. Although a growing body of literature has addressed the topic of suicide, its comprehension and synthesis can be complex due to the various phenotypes that fall within the spectrum of suicide. These phenotypes encompass suicidal ideation, which is characterized by thoughts of ending one’s own life in an active form (with a specific plan) or in a passive form (with a mere desire to die but lacking a concrete plan), suicide attempt, and death by suicide. 2
Risk factors for suicidal ideation differ from those for the transition to suicidal behavior, which includes suicide attempt or completed suicide. 3 Family history of suicide or suicide attempt, mental disorders, chronic physical illness, and sociodemographic factors contribute to increased risk of suicide. 4 , 5 Among older populations, additional risk factors include sleep disorders, reduced mobility, compromised quality of life, and significant functional impairment. 6 , 7
More than 500 million individuals are blind or have significant visual impairment worldwide. 8 Visual impairment is linked to challenges such as decreased independence, social skills, and personal income. 9 It also raises the risk of mental health issues such as depression, stress, and a decline in overall quality of life. 10 Accordingly, previous studies have suggested a plausible association between visual impairment and increased risk of suicide. 4 , 11 , 12 Nonetheless, the consistency and magnitude of this association exhibit variability among studies, posing a challenge for assessments of the precise nature of the association and the extent of the associated risk. The objective of this systematic review and meta-analysis was to consolidate the available literature on the association between visual impairment and diverse aspects of suicide, with the intention of illuminating both the extent of this association and the potential risk factors.
This study was exempt from institutional review board approval and the need for informed consent, as it exclusively used previously published data and did not qualify as human participant research according to the Seoul National University Hospital Institutional Review Board guidelines. The study followed the Meta-Analysis of Observational Studies in Epidemiology ( MOOSE ) and Preferred Reporting Items for Systematic Reviews and Meta-Analyses ( PRISMA ) reporting guidelines. The study protocol was prospectively registered with PROSPERO (CRD 42022325106 ) and has been published. 13
With the assistance of an academic librarian, we conducted a systematic literature search of key databases, including PubMed, EMBASE, Scopus, and the Cochrane Library, to identify relevant studies from their inception to February 8, 2024. Our search strategy used a combination of medical subject headings and text words related to visual impairment and suicide. The search terms encompassed concepts such as visual impairment , low vision , blindness , suicide , suicidal ideation , suicide attempt , suicidal behavior , and association. No restrictions were imposed in terms of the study design, publication date, or language. Additionally, we manually searched the reference lists of published articles to identify any relevant studies missed in the electronic searches. The complete search strategy is outlined in eAppendix 1 in Supplement 1 .
Two independent reviewers (C.Y.K. and A.H.) rigorously screened the titles and abstracts of the identified studies in accordance with predefined inclusion criteria. Subsequently, the full-text articles of the potentially eligible studies underwent meticulous evaluation for inclusion by the reviewers. Any discrepancies or disagreements during the screening process were resolved through consultation with a third investigator (Y.K.K.). In cases where multiple publications reported findings from the same study population, we included only the most comprehensive report with the largest sample size after verifying for duplicates.
We included studies that met the following criteria: (1) population-based; (2) reporting visual impairment as a covariate; (3) incorporating suicide death, suicidal ideation, or suicide attempts as outcome measures; and (4) providing odds ratios (ORs) or relative risks (RRs) with corresponding 95% CIs as measures of association or allowing for computation of these measures based on count data reported in the article. We excluded studies that (1) focused solely on pediatric populations; (2) constituted narrative and/or systematic reviews, case reports, commentaries, editorials, or conference abstracts; and (3) lacked a clear definition of visual impairment or a detailed description of the assessment of suicide.
For each included study, data extraction was performed independently by 2 reviewers (C.Y.K. and A.H.) using a standardized data collection form available in Microsoft Access 2016 (Microsoft Corp). Conflicting data entries were identified by algorithm. The extracted information was as follows: (1) study identification details, such as name of first author and publication year; (2) baseline study year; (3) country of study; (4) total number of participants; (5) race and ethnicity of participants, since disparities in suicide rates among populations based on race and ethnicity are well known; (6) age and sex distribution of participants; (7) details on visual impairment assessment; (8) aspects of suicide measured; (9) measures of association (ORs or RRs) with accompanying 95% CIs; and (10) adjustment for confounding factors, if applicable.
Regarding visual impairment, we extracted information pertaining to (1) the operational definition of visual impairment used in the study and (2) the specific method used for visual impairment assessment. For suicide-related outcomes, we extracted (1) the precise definitions of suicidal ideation and suicide attempts as stipulated within the study and (2) the method used to confirm instances of suicide death. In instances where specific details were not readily available within the published article, attempts were made to contact the corresponding author and solicit supplementary information.
The primary outcome measure was the pooled OR of suicidal behavior among individuals with visual impairment compared with those without, and the secondary outcome measures were the pooled ORs of suicidal ideation and suicide death. We conducted a meta-analysis for exposure (visual impairment) and each outcome combination (suicidal behavior, suicidal ideation, and suicide death) using inverse variance–weighted random-effects models to combine the study-specific measures of association. We included both unadjusted and adjusted estimates of increased risk, giving priority to adjusted estimates for our analysis. When count data were available, we calculated unadjusted measures of association. In cases where neither count data nor risk estimates were provided, we calculated the standardized mortality ratio based on the reference-population statistics provided in the respective studies. 14 The quantification of between-study outcome variation (ie, heterogeneity) was conducted using the I 2 statistic. This metric illustrates the percentage of variation across studies attributed to heterogeneity rather than to chance, irrespective of the treatment effect metric used. 15 Drapery plots were created to illustrate, as curves, the P value function for each individual study and pooled estimates in each meta-analysis, along with the prediction range for a single future study. 16
Due to the discrepancies in definitions of the degree of visual impairment (which included both low vision and blindness) 17 across studies, it was impractical to combine multiple study findings by visual impairment severity. Consequently, we consolidated multiple ORs provided by a study based on visual impairment severity categories to derive a single OR value per study. In cases where individual studies presented different ratio measures of association (eg, OR and/or RR), we considered these estimates to be reasonably similar given the rarity of suicide occurrences. 18
We also conducted meta-regression analyses to investigate possible causes of heterogeneity across studies. 19 These analyses aimed to explore differences in study characteristics and populations that potentially could have altered the association with visual impairment on the risk of suicide. The 8 covariates included were as follows: (1) publication year, (2) main ethnicity of study participants, (3) mean (SD) age of study participants, (4) total sample size, (5) vision assessment method, (6) consideration of potential confounding factors, (7) continents where study was conducted, and (8) studies conducted in low-income country. Definitions of each of the covariates are provided in eTable 1 in Supplement 1 .
In multiple meta-regression analysis, one can try to model all possible combinations of predictive factors associated with outcomes (ie, covariates) in a procedure termed multimodel inference . This allows for determination of which possible covariate combination provides the best fit and which are the most important overall. 20 We modeled all possible combinations of 8 covariates (2 8 = 256) and determined the model that performed best with a lowest value of Akaike information criterion corrected.
Additionally, graphic display of heterogeneity (GOSH) plot analysis, 21 a sophisticated means of exploring the patterns of effect sizes and heterogeneity in our data, was performed. This method uses 3 clustering algorithms: K -means clustering, 22 density-based spatial clustering of applications with noise, 23 and Gaussian mixture models. 24 For those plots, we fit the same meta-analysis model to all possible subsets of our included studies. Then, sensitivity analysis was applied to test the effect of rerunning the meta-analysis after removing studies potentially contributing to cluster imbalance. All statistical analyses were conducted using R, version 4.0.4 (R Project for Statistical Computing). Two-sided P < .05 was considered statistically significant.
To assess the methodological quality of the included studies, we used the Newcastle-Ottawa Scale, a validated tool for evaluating the quality of cross-sectional, case-control, and cohort studies (eAppendix 2 in Supplement 1 ). 25 Our qualitative assessment for publication bias involved the use of funnel plots. 26
A total of 3239 studies were initially identified through a systematic search. Following a process of duplicate exclusion and abstract screening, 101 studies were considered potentially relevant and subjected to a thorough full-text review. Ultimately, 31 studies were included, 27 - 57 encompassing a combined study population of 5 692 769 individuals (mean [SD] age, 48.4 [8.5] years; 2 965 933 female [52%] and 2 726 836 male [48%]). The stepwise selection process is depicted in Figure 1 .
The publication timeline of the studies varied, with 2 studies published in the 1990s, 27 , 28 5 in the 2000s, 29 - 33 12 in the 2010s, 34 , 36 - 46 and 12 in the 2020s. 35 , 47 - 57 Geographically, the studies covered a diverse range of regions, with 8 studies conducted in Europe, 28 - 30 , 33 , 38 , 44 , 45 , 50 10 in Australia and Asia, 27 , 31 , 39 - 43 , 46 , 56 , 57 11 in North America, 32 , 34 , 36 , 37 , 47 , 48 , 51 - 55 1 in the Middle East, 35 and 1 involving multiple countries. 49 The detailed characteristics of each included study can be found in Table 1 . The definitions and criteria of the methods used to assess visual impairment and risk of suicide are provided in Table 2 .
The association between visual impairment and suicidal behavior was investigated in 17 studies 29 , 30 , 32 , 34 - 37 , 40 , 44 , 46 , 48 , 50 , 53 - 57 with a total of 5 602 285 participants. Our summary estimate of visual impairment as a risk factor for suicidal behavior was an OR of 2.49 (95% CI, 1.71-3.63), with heterogeneity of I 2 = 92.8% ( P < .001) ( Figure 2 A). A total of 21 studies 27 , 28 , 31 , 33 , 35 , 38 - 43 , 45 , 47 - 55 assessed suicidal ideation, encompassing 611 899 participants. The pooled OR for the association between visual impairment and suicidal ideation was 2.01 (95% CI, 1.62-2.50), with heterogeneity of I 2 = 89.0% ( P < .001) ( Figure 2 B). Eight studies 29 , 30 , 32 , 37 , 44 , 46 , 56 , 57 involving a total of 5 067 113 participants examined the association between visual impairment and the risk of suicide death. The pooled OR was 1.89 (95% CI, 1.32-2.71), with heterogeneity of I 2 = 73.6% ( P = .002) ( Figure 2 C). Drapery plots in eFigures 1 and 2 in Supplement 1 show the different meta-analytic results by P value functions.
Meta-regression analyses were undertaken to establish the association between individual moderators (predictive factors) and the pooled effect size (ie, risk of suicidal behavior). Within the group of 8 moderators, the mean age of study participants was a notable risk factor for suicidal behavior. Specifically, 71.3% of the variance in true effect sizes could be attributed to age. The Test of Moderators also showed significance ( P < .001) (eTable 2 in Supplement 1 ). This signifies that the predictive factor—namely, the mean age of study participants—indeed was associated with the effect sizes of the studies.
Random-effects meta-regression analyses showed that participant age was a possible risk factor. Thus, we performed a subgroup analysis comparing effect sizes according to mean age (eTable 3 in Supplement 1 ). The pooled OR for studies that had included adolescent patients was 9.85 (95% CI, 4.39-22.10), representing the highest value among the various age groups. The second-highest OR was observed among individuals older than 65 years at 6.66 (95% CI, 2.95-15.00).
Multiple meta-regression analyses were performed to identify the blend of multiple moderators predictive of the pooled effect size, while also considering interactions among moderators. The most optimal model for estimating risk of suicidal behavior in patients with visual impairment was the blend of moderators (Akaike information criterion corrected, 29.7), encompassing mean age of study participants (model importance, 0.99), consideration of potential confounding factors in a study (model importance, 0.27), and country where the study was conducted (model importance, 0.21). A model-averaged plot of predictive factor importance displays the significance of each factor across all of the models (eFigure 3 in Supplement 1 ). The results for predictive factors associated with suicidal ideation risk in patients with visual impairment are plotted in eFigure 4 in Supplement 1 .
Our results showed 2 peaks suggestive of the effect size heterogeneity patterns for the association between visual impairment and risk of suicidal behavior (eFigure 5 in Supplement 1 ). The 3 clustering algorithms for detection of different clusters in a GOSH plot were applied and detected 2 studies 34 , 35 that might have contributed to cluster imbalance. After excluding these 2 potential outliers, we again performed the meta-analysis and obtained 1.83 (95% CI, 1.48-2.28) as the pooled OR for visual impairment and risk of suicidal behavior (eTable 4 in Supplement 1 ).
Figure 3 shows a funnel plot illustrating the potential publication bias. The studies are distributed around the pooled effect size (indicated by the vertical line at the center), both within and outside the funnel’s contours. This suggests a reduced likelihood of significant bias in smaller studies, which are more susceptible to yielding nonsignificant results and thus potentially going unnoticed. Funnel plots for suicidal ideation and suicide death are shown in eFigure 6 in Supplement 1 .
Our comprehensive meta-analysis of 31 population-based studies revealed an association between visual impairment and elevated risk of suicide encompassing suicidal behavior, suicidal ideation, and suicide death. Notably, through multiple meta-regression analyses, we uncovered a particularly pronounced risk of suicide associated with visual impairment among adolescents.
For individuals with visual impairment, the underlying causes of suicidal behavior may be complex and multifactorial. A nationwide survey conducted in the US found that approximately 88% of respondents regarded eye health as a critical component of their overall well-being, with blindness being ranked as the most severe conceivable health outcome. 60 Indeed, visual impairment has implications that extend beyond the confines of clinical ophthalmology. Systematic examination of and consultation with patients with visual impairment consistently reveal a concerning level of compromised quality of life, reduced physical activity, social isolation, decline in autonomy, diminished personal income, and substantial prevalence of depression. 9 , 61 , 62 Notably, these factors are widely recognized as significant risk factors for suicide. 63
In the literature, 2 meta-analyses have investigated the potential association between visual impairment and suicide. Rajeshkannan et al 64 identified a correlation between suicidal ideation (OR, 1.53 [95% CI, 1.30-1.79]) or suicide attempt (OR, 4.55 [95% CI, 2.39-8.67]) and visual impairment based on 6 relevant studies. Palbo et al, 65 having integrated 8 studies into a quantitative analysis, also posited elevated risks of suicide death (OR, 7.00 [95% CI, 2.30-21.40]), suicide attempt (OR, 2.62 [95% CI, 1.29-5.31]), and suicidal ideation (OR, 1.83 [95% CI, 1.40-2.40]) among individuals with visual impairment. However, the restricted number of studies integrated into their analysis impeded Palbo et al 65 from achieving precise measurements of risk magnitude and constrained their potential to conduct additional analyses. Our report encompasses 31 studies identified through meticulous literature searches, thus allowing for comprehensive summary estimates regarding the association between visual impairment and risk of suicide and facilitating the execution of meta-regression analyses.
In our results, studies focusing on adolescents with visual impairment demonstrated the highest risk of suicidal behavior. Adolescence is a complex stage of life in which both physiological and psychological changes begin. In this period, individuals cultivate independence and build social networks by acquiring new skills and knowledge and navigating educational and interpersonal ups and downs. 66 In the studies scrutinized, symptoms related to anxiety, tension, and general distress were significantly higher in adolescents with visual impairment than in those without. 67 Through interviews with adolescents with visual impairment, Rainey et al 68 demonstrated that these individuals had significant concerns about their future lives. They voiced worries about facing potential prejudice from future employers, managing independent living in unfamiliar surroundings, and shouldering the sole responsibility for household matters, including finances.
This study has some limitations. First, heterogeneities between the examined studies warrant attention. The study population differed to a certain degree; for example, some studies focused on elderly individuals, 1 included homeless individuals, 2 focused on patients with vision-related diagnoses, and others had a broader demographic focus. Although we performed a rigorous sensitivity analysis to validate the results, a potential association between study heterogeneities and the pooled effect remains. Second, methods used to assess the outcome varied among the studies. Parameters related to suicide were evaluated using questions in different phraseology, language, and time frames of interest. Third, variations in the definition, classification, and assessment methods for visual impairment in each study could potentially confound the association between visual impairment and suicide risk. Specifically, objective assessment of vision might better reflect associated ocular diseases and/or physiological decline. Conversely, self-reported vision may be a marker of functional performance in activities of daily living and may be exacerbated by psychological distress such as depression and social isolation. Our model-averaged plots of predictive factor importance, which illustrate the significance of each factor across all models, revealed that the vision assessment methods did not emerge as a significant factor. This suggests that the association between visual impairment and suicide risk might be independent of the types of vision assessment methods used in the studies. However, we believe that it is crucial to acknowledge the discrepancies in definitions and assessments of the degree of visual impairment across studies as significant factors in interpreting the results. Fourth, although most of the studies included in the analysis adjusted for depression and other important risk factors, the potential confounding of additional risk factors cannot be ruled out. Since the etiology of suicidal behavior is complex, and because diverse risk factors are associated with different individual and cultural contexts, further research is warranted to determine which factors may modulate the risk of suicide in patients with visual impairment.
In this systematic review and meta-analysis of visual impairment and suicide risk, an association between visual impairment and an increased risk of suicide was identified. This finding emphasizes the importance of eye health to overall mental well-being. It is recommended that clinicians remain attentive to the elevated risk and be ready to implement suitable suicide prevention measures when required, especially when dealing with adolescents. In addition, the limited number of studies addressing adolescents with visual impairment and suicide highlights the importance of conducting additional research in this area.
Accepted for Publication: February 19, 2024.
Published: April 17, 2024. doi:10.1001/jamanetworkopen.2024.7026
Open Access: This is an open access article distributed under the terms of the CC-BY License . © 2024 Kim CY et al. JAMA Network Open .
Corresponding Authors: Young Kook Kim, MD, PhD, Department of Ophthalmology, Seoul National University College of Medicine, 101 Daehak-ro, Jongno-gu, Seoul 03080, Korea ( [email protected] ); In Boem Chang, MD, PhD, Seoul ON Eye Clinic, 750 Tongil-ro, Eunpyeong-gu, Seoul 03355, Korea ( [email protected] ).
Author Contributions: Prof Y. K. Kim had full access to all of the data in the study and takes responsibility for the integrity of the data and the accuracy of the data analysis. Dr C. Y. Kim and Prof Ha contributed equally to the study as co–first authors.
Concept and design: Ha, Chang, Y. K. Kim.
Acquisition, analysis, or interpretation of data: C. Y, Kim, Ha, Shim, Hong, Y. K. Kim.
Drafting of the manuscript: C. Y. Kim, Ha, Y. K. Kim.
Critical review of the manuscript for important intellectual content: C. Y. Kim, Shim, Hong, Chang, Y. K. Kim.
Statistical analysis: C. Y. Kim, Shim, Y. K. Kim.
Obtained funding: Y. K. Kim.
Administrative, technical, or material support: C. Y. Kim, Ha, Y. K. Kim.
Supervision: Ha, Hong, Y. K. Kim.
Conflict of Interest Disclosures: None reported.
Funding/Support: This study was supported by grant NRF-2021R1F1A1062503 from the National Research Foundation of Korea.
Role of the Funder/Sponsor: The funder had no role in the design and conduct of the study; collection, management, analysis, and interpretation of the data; preparation, review, or approval of the manuscript; and decision to submit the manuscript for publication. The study and researchers are independent of the funder.
Meeting Presentation: This study was presented as a poster to the 2022 American Academy of Ophthalmology Annual Meeting; October 1, 2022; Chicago, Illinois.
Data Sharing Statement: See Supplement 2 .
- Register for email alerts with links to free full-text articles
- Access PDFs of free articles
- Manage your interests
- Save searches and receive search alerts
ORIGINAL RESEARCH article
Mortality prediction and influencing factors for intensive care unit patients with acute tubular necrosis: random survival forest and cox regression analysis.

- 1 Hangzhou Normal University, Hangzhou, Zhejiang Province, China
- 2 School of Public Administration, Hangzhou Normal University, Hangzhou, Zhejiang Province, China
The final, formatted version of the article will be published soon.
Select one of your emails
You have multiple emails registered with Frontiers:
Notify me on publication
Please enter your email address:
If you already have an account, please login
You don't have a Frontiers account ? You can register here
Background: Patients with acute tubular necrosis (ATN) not only have severe renal failure, but also have many comorbidities, which can be life-threatening and require timely treatment. Identifying the influencing factors of ATN and taking appropriate interventions can effectively shorten the duration of the disease to reduce mortality and improve patient prognosis. Methods: Mortality prediction models were constructed by using the random survival forest (RSF) algorithm and the Cox regression. Next, the performance of both models was assessed by the out-of-bag (OOB) error rate, the integrated brier score, the prediction error curve, and area under the curve (AUC) at 30, 60 and 90 days. Finally, the optimal prediction model was selected and the decision curve analysis and nomogram were established. Results: RSF model was constructed under the optimal combination of parameters. Vasopressors, international normalized ratio (INR)_min, chloride_max, and metastatic solid tumor were identified as risk factors that had strong influence on mortality in ATN patients. Uni-variate and multivariate regression analyses were used to establish the Cox regression model. Nor-epinephrine, vasopressors, INR_min, severe liver disease, and metastatic solid tumor were identified as important risk factors. The discrimination and calibration ability of both predictive models were demonstrated by the OOB error rate and the integrated brier score. However, the prediction error curve of Cox regression model was consistently lower than that of RSF model, indicating that Cox regression model was more stable and reliable. Then, Cox regression model was also more accurate in predicting mortality of ATN patients based on the AUC at different time points (30, 60 and 90 days). The analysis of decision curve analysis shows that the net benefit range of Cox regression model at different time points is large, indicating that the model has good clinical effectiveness. Finally, a nomogram predicting the risk of death was created based on Cox model. Conclusion: The Cox regression model is superior to the RSF algorithm model in predicting mortality of patients with ATN. Moreover, the model has certain clinical utility, which can provide clinicians with some reference basis in the treatment of ATN and contribute to improve patient prognosis.
Keywords: acute tubular necrosis, Random survival forest, Cox regression, nomogram, Risk factors
Received: 27 Dec 2023; Accepted: 22 Apr 2024.
Copyright: © 2024 Zeng, Zhang, Du, Han, Song, Duan, Yang and Wu. This is an open-access article distributed under the terms of the Creative Commons Attribution License (CC BY) . The use, distribution or reproduction in other forums is permitted, provided the original author(s) or licensor are credited and that the original publication in this journal is cited, in accordance with accepted academic practice. No use, distribution or reproduction is permitted which does not comply with these terms.
* Correspondence: Jun Yang, School of Public Administration, Hangzhou Normal University, Hangzhou, 311121, Zhejiang Province, China Yinyin Wu, Hangzhou Normal University, Hangzhou, Zhejiang Province, China
Disclaimer: All claims expressed in this article are solely those of the authors and do not necessarily represent those of their affiliated organizations, or those of the publisher, the editors and the reviewers. Any product that may be evaluated in this article or claim that may be made by its manufacturer is not guaranteed or endorsed by the publisher.
Advertisement
Regression analysis of student academic performance using deep learning
- Published: 27 July 2020
- Volume 26 , pages 783–798, ( 2021 )
Cite this article
- Sadiq Hussain ORCID: orcid.org/0000-0002-9840-4796 1 ,
- Silvia Gaftandzhieva 2 ,
- Md. Maniruzzaman 3 ,
- Rositsa Doneva 2 &
- Zahraa Fadhil Muhsin 4
2241 Accesses
18 Citations
Explore all metrics
Educational data mining helps the educational institutions to perform effectively and efficiently by exploiting the data related to all its stakeholders. It can help the at-risk students, develop recommendation systems and alert the students at different levels. It is beneficial to the students, educators and authorities as a whole. Deep learning has gained momentum in various domains especially image processing with a large dataset. We devise a regression model for analyzing the academic performance of the students using deep learning. We have applied regression using deep learning and linear regression on the dataset. For such models with smaller datasets, to tackle the issue of overfitting is critical. Hence, the parameters can be tuned to deal with such issues. The deep learning model records a mean absolute score (mae) of 1.61 and loss 4.7 with the value of k = 3. While the linear regression model yields a loss of 6.7 and mae score of 1.97. The deep learning model outperforms the linear regression model. The model may be successfully extended to other programmes to mine and predict the performance of the learners.
This is a preview of subscription content, log in via an institution to check access.
Access this article
Price includes VAT (Russian Federation)
Instant access to the full article PDF.
Rent this article via DeepDyve
Institutional subscriptions
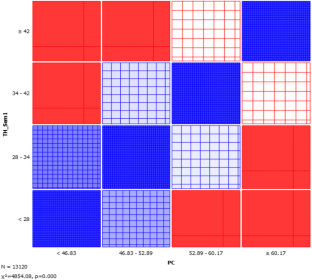
Similar content being viewed by others
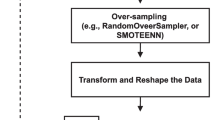
An automatic prediction of students’ performance to support the university education system: a deep learning approach
Yazn Alshamaila, Hamad Alsawalqah, … Bilal Abu Salih
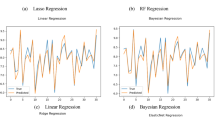
A comparative study of machine learning and deep learning algorithms for predicting student’s academic performance
Megha Bhushan, Satyam Vyas, … Arun Negi
Performance Evaluation for Four Types of Machine Learning Algorithms Using Educational Open Data
Abhinav, K., Subramanian, V., Dubey, A., Bhat, P., & Venkat, A. D. (2018). LeCoRe: A framework for modeling Learner’s preference. In EDM .
Abu Tair, M. M., & El-Halees, A. M. (2012). Mining educational data to improve students’ performance: A case study. Mining Educational Data to Improve Students’ Performance: A Case Study , 2 (2).
Adekitan, A. I., & Salau, O. (2019). The impact of engineering students’ performance in the first three years on their graduation result using educational data mining. Heliyon, 5 (2), e01250.
Article Google Scholar
Ahmed, A. B. E. D., & Elaraby, I. S. (2014). Data mining: A prediction for student’s performance using classification method. World Journal of Computer Application and Technology, 2 (2), 43–47.
Google Scholar
Algarni, A. (2016). Data mining in education. International Journal of Advanced Computer Science and Applications, 7 (6), 456–461.
Al-Radaideh, Q. A., Al-Shawakfa, E. M., & Al-Najjar, M. I. (2006, December). Mining student data using decision trees. In International Arab Conference on Information Technology (ACIT’2006) , Yarmouk University, Jordan.
Baradwaj, B. K., & Pal, S. (2012). Mining educational data to analyze students’ performance. arXiv preprint arXiv:1201.3417.
Ben-Zadok, G., Hershkovitz, A., Mintz, E., & Nachmias, R. (2009). Examining online learning processes based on log files analysis: A case study. In 5th International Conference on Multimedia and ICT in Education (m-ICTE’09) .
Bhise, R. B., Thorat, S. S., & Supekar, A. K. (2013). Importance of data mining in higher education system. IOSR Journal Of Humanities And Social Science (IOSR-JHSS), 6 (6), 18–21.
Campbell, C. M., & Cabrera, A. F. (2014). Making the mark: Are grades and deep learning related? Research in Higher Education, 55 (5), 494–507.
Carmona, C., Castillo, G., & Millán, E. (2007, September). Discovering student preferences in e-learning. In Proceedings of the international workshop on applying data mining in e-learning (pp. 33–42).
Deng, L. (2014). A tutorial survey of architectures, algorithms, and applications for deep learning. APSIPA Transactions on Signal and Information Processing, 3 .
Gadhavi, M., & Patel, C. (2017). Student final grade prediction based on linear regression. Indian Journal of Computer Science and EngineeringIndian Journal of Computer Science and Engineering, 8 (3), 274–279.
Goyal, M., & Vohra, R. (2012). Applications of data mining in higher education. International Journal of Computer Science Issues (IJCSI), 9 (2), 113.
Guo, B., Zhang, R., Xu, G., Shi, C., & Yang, L. (2015, July). Predicting students performance in educational data mining. In 2015 International Symposium on Educational Technology (ISET) (pp. 125–128). IEEE.
Hernández-Blanco, A., Herrera-Flores, B., Tomás, D., & Navarro-Colorado, B. (2019). A systematic review of deep learning approaches to educational data mining. Complexity, 2019 , 1–22.
Hijaz, S. T., & Naqvi, S. R. (2006). Factors affecting students’ performance: A case of private colleges in Bangladesh. Journal of Sociology, 3 (1), 44–45.
Hussain, S., Muhsion, Z. F., Salal, Y. K., Theodoru, P., KurtoÄŸlu, F., & Hazarika, G. C. (2019). Prediction model on student performance based on internal assessment using deep learning. International Journal of Emerging Technologies in Learning (iJET), 14 (08), 4–22.
Jiawei, H., & Kamber, M. (2011) Data mining: Concepts and techniques, (the Morgan Kaufmann series in data management systems), vol. 2.
Kaur, H., & Bathla, E. G. (2018). Student performance prediction using educational data mining techniques. International Journal on Future Revolution in Computer Science & Communication Engineering, 4 (12), 93–97.
Kim, B. H., Vizitei, E., & Ganapathi, V. (2018). GritNet: Student performance prediction with deep learning. arXiv preprint arXiv:1804.07405.
Laxman, S., & Sastry, P. S. (2006). A survey of temporal data mining. Sadhana, 31 (2), 173–198.
Article MathSciNet Google Scholar
Mannila, H. (1996, June). Data mining: Machine learning, statistics, and databases. In Proceedings of 8th International Conference on Scientific and Statistical Data Base Management (pp. 2–9). IEEE.
Mardikyan, S., & Badur, B. (2011). Analyzing teaching performance of instructors using data mining techniques. Informatics in Education, 10 (2), 245–257.
Mihăescu, M. C. (2011, September). Classification of learners using linear regression. In 2011 Federated Conference on Computer Science and Information Systems (FedCSIS) (pp. 717–721). IEEE.
Montgomery, D. C., Peck, E. A., & Vining, G. G. (2012). Introduction to linear regression analysis (Vol. 821). Hoboken: John Wiley & Sons.
MATH Google Scholar
Nichat, A. A., & Raut, D. A. B. (2017). Predicting and analysis of student performance using decision tree technique. International Journal, 5 , 7319–7328.
Oyerinde, O. D., & Chia, P. A. (2017). Predicting students’ academic performances–a learning analytics approach using multiple linear regression.
Padhy, N., Mishra, D., & Panigrahi, R. (2012). The survey of data mining applications and feature scope. arXiv preprint arXiv:1211.5723.
Pandey, U. K., & Pal, S. (2011). Data mining: A prediction of performer or underperformer using classification. arXiv preprint arXiv:1104.4163.
Piad, K. C., Dumlao, M., Ballera, M. A., & Ambat, S. C. (2016, July). Predicting IT employability using data mining techniques. In 2016 Third International Conference on Digital Information Processing, Data Mining, and Wireless Communications (DIPDMWC) (pp. 26–30). IEEE.
Priya, K. S., & Kumar, A. S. (2013). Improving the student’s performance using educational data mining. International Journal of Advanced Networking and Applications, 4 (4), 1806.
Ramesh, V. A. M. A. N. A. N., Parkavi, P., & Ramar, K. (2013). Predicting student performance: A statistical and data mining approach. International Journal of Computer Applications, 63 (8), 35–39.
Romero, C., & Ventura, S. (2010). Educational data mining: A review of the state of the art. IEEE Transactions on Systems, Man, and Cybernetics, Part C (Applications and Reviews), 40 (6), 601–618.
Salas-Rueda, R. A. (2016). The impact of usable system for regression analysis in higher education. International Journal of Educational Technology in Higher Education, 13 (1), 14.
Shahiri, A. M., & Husain, W. (2015). A review on predicting student's performance using data mining techniques. Procedia Computer Science, 72 , 414–422.
Shih, Y. C., Huang, P. R., Hsu, Y. C., & Chen, S. Y. (2012). A complete understanding of disorientation problems in web-based learning. Turkish Online Journal of Educational Technology-TOJET, 11 (3), 1–13.
Srimani, P. K., & Patil, M. M. (2014). Regression model for Edu-data in technical education system: A linear approach. In ICT and Critical Infrastructure: Proceedings of the 48th Annual Convention of Computer Society of India, vol II (pp. 785–793). Springer, Cham.
Sultana, J., Rani, M. U., & Farquad, M. A. H. (2009). Student’s performance prediction using deep learning and data mining methods. International Journal of Recent Technology and Engineering, 8 (1S4), 1–4.
Suthar, V., & Tarmizi, R. (2010). Effects of students’ beliefs on mathematics and achievement of university students: Regression analysis approach. Journal of social sciences, 6 (2), 146–152.
Thomas, E. H., & Galambos, N. (2004). What satisfies students? Mining student-opinion data with regression and decision tree analysis. Research in Higher Education, 45 (3), 251–269.
Vora, D. R., & Iyer, K. (2018). EDM–survey of performance factors and algorithms applied. International Journal of Engineering & Technology, 7 (2.6), 93–97.
Wang, L., Sy, A., Liu, L., & Piech, C. (2017). Learning to represent student knowledge on programming exercises using deep learning. International Educational Data Mining Society.
Xing, W., & Du, D. (2019). Dropout prediction in MOOCs: Using deep learning for personalized intervention. Journal of Educational Computing Research, 57 (3), 547–570.
Yadav, S. K., Bharadwaj, B., & Pal, S. (2012a). Data mining applications: A comparative study for predicting student’s performance. arXiv preprint arXiv:1202.4815.
Yadav, S. K., Bharadwaj, B., & Pal, S. (2012b). Mining education data to predict student’s retention: A comparative study. arXiv preprint arXiv:1203.2987.
Zhou, Q., Quan, W., Zhong, Y., Xiao, W., Mou, C., & Wang, Y. (2018). Predicting high-risk students using internet access logs. Knowledge and Information Systems, 55 (2), 393–413.
Download references
Availability of data and material
Available on request.
Author information
Authors and affiliations.
Dibrugarh University, Dibrugarh, Assam, India
Sadiq Hussain
University of Plovdiv “PaisiiHilendarski”, Plovdiv, Bulgaria
Silvia Gaftandzhieva & Rositsa Doneva
Statistics Discipline, Khulna University, Khulna, Bangladesh
Md. Maniruzzaman
Science College, Baghdad University, Baghdad, Iraq
Zahraa Fadhil Muhsin
You can also search for this author in PubMed Google Scholar
Corresponding author
Correspondence to Sadiq Hussain .
Ethics declarations
Conflicts of interest/competing interests.
Not applicable.
Additional information
Publisher’s note.
Springer Nature remains neutral with regard to jurisdictional claims in published maps and institutional affiliations.
Rights and permissions
Reprints and permissions
About this article
Hussain, S., Gaftandzhieva, S., Maniruzzaman, M. et al. Regression analysis of student academic performance using deep learning. Educ Inf Technol 26 , 783–798 (2021). https://doi.org/10.1007/s10639-020-10241-0
Download citation
Received : 03 April 2020
Accepted : 29 May 2020
Published : 27 July 2020
Issue Date : January 2021
DOI : https://doi.org/10.1007/s10639-020-10241-0
Share this article
Anyone you share the following link with will be able to read this content:
Sorry, a shareable link is not currently available for this article.
Provided by the Springer Nature SharedIt content-sharing initiative
- Data mining
- Educational data mining
- Mean absolute error
- Deep learning
- Find a journal
- Publish with us
- Track your research
This paper is in the following e-collection/theme issue:
Published on 23.4.2024 in Vol 26 (2024)
Electronic Media Use and Sleep Quality: Updated Systematic Review and Meta-Analysis
Authors of this article:

- Xiaoning Han * , PhD ;
- Enze Zhou * , MA ;
- Dong Liu * , PhD
School of Journalism and Communication, Renmin University of China, Beijing, China
*all authors contributed equally
Corresponding Author:
Dong Liu, PhD
School of Journalism and Communication
Renmin University of China
No. 59 Zhongguancun Street, Haidian District
Beijing, 100872
Phone: 86 13693388506
Email: [email protected]
Background: This paper explores the widely discussed relationship between electronic media use and sleep quality, indicating negative effects due to various factors. However, existing meta-analyses on the topic have some limitations.
Objective: The study aims to analyze and compare the impacts of different digital media types, such as smartphones, online games, and social media, on sleep quality.
Methods: Adhering to Preferred Reporting Items for Systematic Reviews and Meta-Analyses (PRISMA) guidelines, the study performed a systematic meta-analysis of literature across multiple databases, including Web of Science, MEDLINE, PsycINFO, PubMed, Science Direct, Scopus, and Google Scholar, from January 2018 to October 2023. Two trained coders coded the study characteristics independently. The effect sizes were calculated using the correlation coefficient as a standardized measure of the relationship between electronic media use and sleep quality across studies. The Comprehensive Meta-Analysis software (version 3.0) was used to perform the meta-analysis. Statistical methods such as funnel plots were used to assess the presence of asymmetry and a p -curve test to test the p -hacking problem, which can indicate publication bias.
Results: Following a thorough screening process, the study involved 55 papers (56 items) with 41,716 participants from over 20 countries, classifying electronic media use into “general use” and “problematic use.” The meta-analysis revealed that electronic media use was significantly linked with decreased sleep quality and increased sleep problems with varying effect sizes across subgroups. A significant cultural difference was also observed in these effects. General use was associated with a significant decrease in sleep quality ( P <.001). The pooled effect size was 0.28 (95% CI 0.21-0.35; k =20). Problematic use was associated with a significant increase in sleep problems ( P ≤.001). The pooled effect size was 0.33 (95% CI 0.28-0.38; k =36). The subgroup analysis indicated that the effect of general smartphone use and sleep problems was r =0.33 (95% CI 0.27-0.40), which was the highest among the general group. The effect of problematic internet use and sleep problems was r =0.51 (95% CI 0.43-0.59), which was the highest among the problematic groups. There were significant differences among these subgroups (general: Q between =14.46, P =.001; problematic: Q between =27.37, P <.001). The results of the meta-regression analysis using age, gender, and culture as moderators indicated that only cultural difference in the relationship between Eastern and Western culture was significant ( Q between =6.69; P =.01). All funnel plots and p -curve analyses showed no evidence of publication and selection bias.
Conclusions: Despite some variability, the study overall confirms the correlation between increased electronic media use and poorer sleep outcomes, which is notably more significant in Eastern cultures.
Introduction
Sleep is vital to our health. Research has shown that high sleep quality can lead to improvements in a series of health outcomes, such as an improved immune system, better mood and mental health, enhanced physical performance, lower risk of chronic diseases, and a longer life span [ 1 - 5 ].
Electronic media refers to forms of media or communication that use electronic devices or technology to create, distribute, and display content. This can include various forms of digital media such as smartphones, tablets, instant messaging, phone calls, social media, online games, short video platforms, etc. Electronic media has permeated every aspect of our lives [ 6 ]. Many prefer to use smartphones or tablets before sleep, which can negatively affect sleep in many aspects, including delayed sleep onset, disrupted sleep patterns, shortened sleep duration, and poor sleep quality [ 7 - 10 ]. Furthermore, problematic use occurs when the behavior surpasses a certain limit. In this study, problematic use of electronic media is not solely determined by the amount of time spent on these platforms, but rather by behavioral indicators that suggest an unhealthy or harmful relationship with them.
Smartphones or tablet use can affect sleep quality in many ways. At first, the use of these devices may directly displace, delay, or interrupt sleep time, resulting in inadequate sleep quantity [ 11 ]. The sound of notifications and vibrations of these devices may interrupt sleep. Second, the screens of smartphones and tablets emit blue light, which can suppress the production of melatonin, the hormone responsible for regulating sleep-wake cycles [ 12 ]. Third, consuming emotionally charged content, such as news, suspenseful movies, or engaging in online arguments, can increase emotional arousal, making it harder to relax and fall asleep. This emotional arousal can also lead to disrupted sleep and nightmares [ 13 ]. Finally, the use of electronic devices before bedtime can lead to a delay in bedtime and a shortened sleep duration, as individuals may lose track of time while engaging with their devices. This can result in a disrupted sleep routine and decreased sleep quality [ 14 ].
Some studies have conducted meta-analyses on screen media use and sleep outcomes in 2016, 2019, and 2021 [ 15 - 17 ]. However, these studies had their own limitations. First, the sample size included in their meta-analyses was small (around 10). Second, these studies only focused on 1 aspect of the effect of digital media on sleep quality. For example, Carter et al [ 16 ] focused only on adolescents, and both Alimoradi et al [ 15 ] and Kristensen et al [ 17 ] only reviewed the relationship between problematic use of digital media or devices and sleep quality. Despite of the high heterogeneity found in the meta-analyses, none have compared the effects of different digital media or devices. This study aims to clarify and compare the effects of these different channels.
Literature Search
The research adhered to Preferred Reporting Items for Systematic Reviews and Meta-Analyses (PRISMA) guidelines ( Multimedia Appendix 1 ) and followed a predetermined protocol [ 18 , 19 ]. As the idea and scope of this study evolved over time, the meta-analysis was not preregistered. However, the methodology was defined a priori and strictly followed to reduce biases, and the possible influence of post hoc decisions was minimized. All relevant studies in English, published from January 1, 2018, to October 9, 2023, were searched. We searched the following databases: Web of Science, MEDLINE, PsycINFO, PubMed, Science Direct, Scopus, and Google Scholar. The abstracts were examined manually. The keywords used to search were the combination of the following words: “sleep” OR “sleep duration” OR “sleep quality” OR “sleep problems” AND “electronic media” OR “smartphone” OR “tablet” OR “social media” OR “Facebook” OR “Twitter” OR “online gaming” OR “internet” OR “addiction” OR “problematic” ( Multimedia Appendix 2 ). Additionally, the reference lists of relevant studies were examined.
Two trained coders independently screened the titles and abstracts of the identified papers for eligibility, followed by a full-text review of the selected studies. Discrepancies between the coders were resolved through discussion until a consensus was reached. The reference lists of the included studies were also manually screened to identify any additional relevant studies. Through this rigorous process, we ensured a comprehensive and replicable literature search that could contribute to the robustness of our meta-analysis findings.
Inclusion or Exclusion Criteria
Titles and abstracts from search results were scrutinized for relevance, with duplicates removed. Full texts of pertinent papers were obtained, and their eligibility for inclusion was evaluated. We mainly included correlational studies that used both continuous measures of time spent using electronic media use and sleep quality. Studies must have been available in English. Four criteria were used to screen studies: (1) only peer-reviewed empirical studies, published in English, were considered for inclusion in the meta-analysis; (2) the studies should report quantitative statistics on electronic media use and sleep quality, including sample size and essential information to calculate the effect size, and review papers, qualitative studies, case studies, and conference abstracts were excluded; (3) studies on both general use and problematic use of electronic media or devices should be included; and (4) only studies that used correlation, regression, or odds ratio were included to ensure consistency.
Study Coding
Two trained coders were used to code the characteristics of the studies independently. Discrepancies were discussed with the first author of the paper to resolve. Sample size and characteristics of participants were coded: country, female ratio, average age, publication year, and electronic types. Effect sizes were either extracted directly from the original publications or manually calculated. If a study reported multiple dependent effects, the effects were merged into one. If a study reported multiple independent effects from different samples, the effects were included separately. Additionally, to evaluate the study quality, the papers were classified into 3 tiers (high, middle, and low) according to Journal Citation Reports 2022 , a ranking of journals based on their impact factor as reported in the Web of Science. The few unindexed papers were rated based on their citation counts as reported in Google Scholar.
Meta-Analysis and Moderator Analyses
The effect sizes were calculated using the correlation coefficient ( r ) as a standardized measure of the relationship between electronic media or device use and sleep quality across studies. When studies reported multiple effect sizes, we selected the one that best represented the overall association between electronic media use and sleep quality. If studies did not provide correlation coefficients, we converted other reported statistics (eg, standardized regression coefficients) into correlation coefficients using established formulas. Once calculated, the correlation coefficients were transformed into Fisher z scores to stabilize the variance and normalize the distribution.
Previous meta-studies have shown high levels of heterogeneity. Hence, the random effects model was adopted for all analyses. To explore potential factors contributing to the heterogeneity and to further understand the relationship between electronic media use and sleep quality, we conducted moderator analyses. The following categorical and continuous moderators were examined: media types (online gaming, social media, smartphone, or intent), participants’ average age, culture, female ratio, and sleep quality assessment method. For categorical moderators, subgroup analyses were performed, while for continuous moderators, meta-regression analyses were conducted. All analyses were completed in the Comprehensive Meta-Analysis software (version 3.0; Biostat, Inc).
Publication Bias
We used statistical methods such as funnel plots to assess the presence of asymmetry and a p -curve test to test the p -hacking problem, which may indicate publication bias. In case of detected asymmetry, we applied techniques such as the trim-and-fill method to adjust the effect size estimates.
By addressing publication bias, we aimed to provide a more accurate and reliable synthesis of the available evidence, enhancing the validity and generalizability of our meta-analytic findings. Nevertheless, it is essential for readers to interpret the results cautiously, considering the potential limitations imposed by publication bias and other methodological concerns.
Search Findings
A total of 98,806 studies were identified from databases, especially Scopus (n=49,643), Google Scholar (n=18,600), Science Direct (n=15,084), and Web of Science (n=11,689). Upon removing duplicate records and excluding studies that did not meet the inclusion criteria, 754 studies remained for the screening phase. After screening titles, abstracts, and full texts, 703 studies were excluded. A total of 4 additional studies were identified from the references of relevant reviews. Finally, 55 studies [ 20 - 74 ] were included in the meta-analysis. The flow diagram of the selection is shown in Figure 1 .

Characteristics of Included Studies
In 20 studies, 21,594 participants were included in the analysis of the general use of electronic media and sleep quality. The average age of the sample ranged from 9.9 to 44 years. The category of general online gaming and sleep quality included 4 studies, with 14,837 participants; the category of general smartphone use and sleep quality included 10 studies, with 5011 participants; and the category of general social media use and sleep quality included 6 studies, with 1746 participants.
These studies came from the following countries or areas: Germany, Serbia, Indonesia, India, China, Italy, Saudi Arabia, New Zealand, the United Kingdom, the United States, Spain, Qatar, Egypt, Argentina, and Portugal. The most frequently used measure of electronic media use was the time spent on it. The most frequently used measure of sleep was the Pittsburgh Sleep Quality Index.
In 35 studies, 20,122 participants were included in the analysis of the problematic use of electronic media and sleep quality. The average age of the sample ranged from 14.76 to 65.62 years. The category of problematic online gaming and sleep quality included 5 studies, with 1874 participants; the category of problematic internet use and sleep quality included 2 studies, with 774 participants; the category of problematic smartphone use and sleep quality included 18 studies, with 12,204 participants; and the category of problematic social media use and sleep quality included 11 studies, with 5270 participants. There was a study that focused on both social media and online gaming, which led to its inclusion in the analysis. These studies came from 14 countries or areas: Turkey, the United States, Indonesia, China, France, Taiwan, India, South Korea, Hong Kong, Iran, Poland, Israel, Hungary, and Saudi Arabia. The most frequently used measures of problematic electronic media use were the Internet Gaming Disorder Scale-Short Form, Smartphone Addiction Scale-Short Form, and Bergen Social Media Addiction Scale.
With respect to study quality, the 56 papers were published in 50 journals, 41 of which were indexed in Journal Citation Reports 2022 , while the remaining 9 journals were rated based on their citation counts as reported in Google Scholar. As a result, of the 56 papers included in the study, 22 papers were assigned a high rating, 18 papers were assigned a middle rating, and 16 papers were assigned a low rating. More information about the included studies is listed in Multimedia Appendix 3 [ 20 - 74 ].
Meta-Analysis
The results of the meta-analysis of the relationship between general electronic media use and sleep quality showed that electronic media use was associated with a significant decrease in sleep quality ( P <.001). The pooled effect size was 0.28 (95% CI 0.21-0.35; k =20), indicating that individuals who used electronic media more frequently were generally associated with more sleeping problems.
The second meta-analysis showed that problematic electronic media use was associated with a significant increase in sleep problems ( P ≤.001). The pooled effect size was 0.33 (95% CI 0.28-0.38; k =36), indicating that participants who used electronic media more frequently were more likely to have more sleep problems.
Moderator Analyses
At first, we conducted subgroup analyses for different media or devices. The results are shown in Tables 1 and 2 . The effect of the relationship between general online gaming and sleep problems was r =0.14 (95% CI 0.06-0.22); the effect of the relationship between general smartphone use and sleep problems was r =0.33 (95% CI 0.27-0.40); and the effect of the relationship between general social media use and sleep problems was r =0.28 (95% CI 0.21-0.34). There are significant differences among these groups ( Q between =14.46; P =.001).
The effect of the relationship between problematic gaming and sleep problems was r =0.49, 95% CI 0.23-0.69; the effect of the relationship between problematic internet use and sleep problems was r =0.51 (95% CI 0.43-0.59); the effect of the relationship between problematic smartphone use and sleep problems was r =0.25 (95% CI 0.20-0.30); and the effect of the relationship between problematic social media use and sleep problems was r =0.35 (95% CI 0.29-0.40). There are significant differences among these groups ( Q between =27.37; P <.001).
We also used age, gender, and culture as moderators to conduct meta-regression analyses. The results are shown in Tables 3 and 4 . Only cultural difference in the relationship between Eastern and Western culture was significant ( Q between =6.694; P =.01). All other analyses were not significant.
a Not applicable.
All funnel plots of the analyses were symmetrical, showing no evidence of publication bias ( Figures 2 - 5 ). We also conducted p -curve analyses to see whether there were any selection biases. The results also showed that there were no biases.

Principal Findings
This study indicated that electronic media use was significantly linked with decreased sleep quality and increased sleep problems with varying effect sizes across subgroups. General use was associated with a significant decrease in sleep quality. Problematic use was associated with a significant increase in sleep problems. A significant cultural difference was also observed by the meta-regression analysis.
First, there is a distinction in the impact on sleep quality between problematic use and general use, with the former exhibiting a higher correlation strength. However, both have a positive correlation, suggesting that the deeper the level of use, the more sleep-related issues are observed. In addressing this research question, the way in which electronic media use is conceptualized and operationalized may have a bearing on the ultimate outcomes. Problematic use is measured through addiction scales, while general use is predominantly assessed by duration of use (time), leading to divergent results stemming from these distinct approaches. The key takeaway is that each measurement possesses unique strengths and weaknesses, and the pathways affecting sleep quality differ. Consequently, the selection of a measurement approach should be tailored to the specific research question at hand. The duration of general use reflects an individual’s comprehensive involvement with electronic media, and its impact on sleep quality is evident in factors such as an extended time to fall asleep and reduced sleep duration. The addiction scale for problematic use illuminates an individual’s preferences, dependencies, and other associations with electronic media. Its impact on sleep quality is evident through physiological and psychological responses, including anxiety, stress, and emotional reactions.
Second, notable variations exist in how different types of electronic media affect sleep quality. In general, the positive predictive effects of smartphone, social media, and online gaming use durations on sleep problems gradually decrease. In the problematic context, the intensity of addiction to the internet and online gaming has the most significant positive impact on sleep problems, followed by social media, while smartphones exert the least influence. On one hand, longitudinal comparisons within the same context reveal that the content and format of electronic media can have varying degrees of negative impact on sleep quality, irrespective of whether it involves general or problematic use. On the other hand, cross-context comparisons suggest that both general and problematic use play a role in moderating the impact of electronic media types on sleep quality. As an illustration, problematic use reinforces the positive impact of online gaming and social media on sleep problems, while mitigating the influence of smartphones. Considering smartphones as electronic media, an extended duration of general use is associated with lower sleep quality. However, during problematic use, smartphones serve as the platform for other electronic media such as games and social media, resulting in a weakened predictive effect on sleep quality. Put differently, in the context of problematic use, the specific type of electronic media an individual consumes on their smartphones becomes increasingly pivotal in shaping sleep quality.
Third, cultural differences were found to be significant moderators of the relationship between electronic media use and sleep problems in both our study and Carter et al [ 16 ]. Kristensen et al [ 17 ], however, did not specifically address the role of cultural differences but revealed that there was a strong and consistent association between bedtime media device use and sleep outcomes across the studies included. Our findings showed that the association between problematic social media use was significantly larger in Eastern culture. We speculate that the difference may be attributed to cultural differences in social media use patterns, perceptions of social norms and expectations, variations in bedtime routines and habits, and diverse coping mechanisms for stress. These speculations warrant further investigation to understand better the underlying factors contributing to the observed cultural differences in the relationship between social media use and sleep quality.
Fourth, it was observed that gender and age had no significant impact on sleep quality. The negative effects of electronic media use are not only confined to the sleep quality of adults, and the association with gender differences remains unclear. Recent studies point out that electronic media use among preschoolers may result in a “time-shifting” process, disrupting their sleep patterns [ 75 ]. Similarly, children and adolescent sleep patterns have been reported to be adversely affected by electronic media use [ 76 - 78 ]. These findings underscore the necessity of considering age group variations in future research, as electronic media use may differently impact sleep quality across age demographics.
In conclusion, our study, Carter et al [ 16 ], and Kristensen et al [ 17 ] collectively emphasize the importance of understanding and addressing the negative impact of electronic media use, particularly problematic online gaming and smartphone use, on sleep quality and related issues. Further research is warranted to explore the underlying mechanisms and specific factors contributing to the relationship between electronic media use and sleep problems.
Strengths and Limitations
Our study, supplemented with research by Carter et al [ 16 ] and Kristensen et al [ 17 ], contributes to the growing evidence supporting a connection between electronic media use and sleep quality. We found that both general and problematic use of electronic media correlates with sleep issues, with the strength of the correlation varying based on the type of electronic media and cultural factors, with no significant relationship observed with age or gender.
Despite the vast amount of research on the relationship between electronic media use and sleep, several gaps and limitations still exist.
First, the inclusion criteria were restricted to English-language, peer-reviewed empirical studies published between January 2018 and October 2023. This may have led to the exclusion of relevant studies published in other languages or before 2018, potentially limiting the generalizability of our findings. Furthermore, the exclusion of non–peer-reviewed studies and conference abstracts may have introduced publication bias, as significant results are more likely to be published in peer-reviewed journals.
Second, although we used a comprehensive search strategy, the possibility remains that some relevant studies may have been missed. Additionally, the search strategies were not linked with Medical Subject Headings headers and may not have captured all possible electronic media types, resulting in an incomplete representation of the effects of electronic media use on sleep quality.
Third, the studies included in our meta-analysis exhibited considerable heterogeneity in sample characteristics, electronic media types, and measures of sleep quality. This heterogeneity might have contributed to the variability in effect sizes observed across studies. Although we conducted moderator analyses to explore potential sources of heterogeneity, other unexamined factors may still have influenced the relationship between electronic media use and sleep quality.
Fourth, our meta-analysis relied on the correlation coefficient ( r ) as the primary effect size measure, which may not fully capture the complex relationships between electronic media use and sleep quality. Moreover, the conversion of other reported statistics into correlation coefficients could introduce additional sources of error. The correlational nature of the included studies limited our ability to draw causal inferences between electronic media use and sleep quality. Experimental and longitudinal research designs would provide stronger evidence for the directionality of this relationship.
Given these limitations, future research should aim to include a more diverse range of studies, examine additional potential moderators, and use more robust research designs to better understand the complex relationship between electronic media use and sleep quality.
Conclusions
In conclusion, our updated meta-analysis affirms the consistent negative impact of electronic media use on sleep outcomes, with problematic online gaming and smartphone use being particularly impactful. Notably, the negative effect of problematic social media use on sleep quality appears more pronounced in Eastern cultures. This research emphasizes the need for public health initiatives to increase awareness of these impacts, particularly for adolescents. Further research, including experimental and longitudinal studies, is necessary to delve deeper into the complex relationship between electronic media use and sleep quality, considering potential moderators like cultural differences.
Acknowledgments
This research was supported by the Journalism and Marxism Research Center, Renmin University of China (MXG202215), and by funds for building world-class universities (disciplines) of Renmin University of China (23RXW195).
A statement on the use of ChatGPT in the process of writing this paper can be found in Multimedia Appendix 4.
Data Availability
The data sets analyzed during this study are available from the corresponding author on reasonable request.
Conflicts of Interest
None declared.
PRISMA (Preferred Reporting Items for Systematic Reviews and Meta-Analyses) 2020 checklist.
Search strategies.
Characteristics of included studies.
Large language model statement.
- Brink-Kjaer A, Leary EB, Sun H, Westover MB, Stone KL, Peppard PE, et al. Age estimation from sleep studies using deep learning predicts life expectancy. NPJ Digit Med. 2022;5(1):103. [ FREE Full text ] [ CrossRef ] [ Medline ]
- Killgore WDS. Effects of sleep deprivation on cognition. Prog Brain Res. 2010;185:105-129. [ CrossRef ] [ Medline ]
- Lee S, Mu CX, Wallace ML, Andel R, Almeida DM, Buxton OM, et al. Sleep health composites are associated with the risk of heart disease across sex and race. Sci Rep. 2022;12(1):2023. [ FREE Full text ] [ CrossRef ] [ Medline ]
- Prather AA. Sleep, stress, and immunity. In: Grandner MA, editor. Sleep and Health, 1st Edition. Cambridge. Academic Press; 2019;319-330.
- Scott AJ, Webb TL, Martyn-St James M, Rowse G, Weich S. Improving sleep quality leads to better mental health: a meta-analysis of randomised controlled trials. Sleep Med Rev. Dec 2021;60:101556. [ FREE Full text ] [ CrossRef ] [ Medline ]
- Guttmann A. Statista. 2023. URL: https://www.statista.com/topics/1536/media-use/#topicOverview [accessed 2023-06-10]
- Hysing M, Pallesen S, Stormark KM, Jakobsen R, Lundervold AJ, Sivertsen B. Sleep and use of electronic devices in adolescence: results from a large population-based study. BMJ Open. Feb 02, 2015;5(1):e006748. [ FREE Full text ] [ CrossRef ] [ Medline ]
- Lavender RM. Electronic media use and sleep quality. Undergrad J Psychol. 2015;28(1):55-62. [ FREE Full text ]
- Exelmans L, Van den Bulck J. Bedtime mobile phone use and sleep in adults. Soc Sci Med. 2016;148:93-101. [ CrossRef ] [ Medline ]
- Twenge JM, Krizan Z, Hisler G. Decreases in self-reported sleep duration among U.S. adolescents 2009-2015 and association with new media screen time. Sleep Med. 2017;39:47-53. [ CrossRef ] [ Medline ]
- Exelmans L. Electronic media use and sleep: a self-control perspective. Curr Sleep Med Rep. 2019;5:135-140. [ CrossRef ]
- Jniene A, Errguig L, El Hangouche AJ, Rkain H, Aboudrar S, El Ftouh M, et al. Perception of sleep disturbances due to bedtime use of blue light-emitting devices and its impact on habits and sleep quality among young medical students. Biomed Res Int. 2019;2019:7012350. [ FREE Full text ] [ CrossRef ] [ Medline ]
- Munezawa T, Kaneita Y, Osaki Y, Kanda H, Minowa M, Suzuki K, et al. The association between use of mobile phones after lights out and sleep disturbances among Japanese adolescents: a nationwide cross-sectional survey. Sleep. 2011;34(8):1013-1020. [ FREE Full text ] [ CrossRef ] [ Medline ]
- Smith LJ, Gradisar M, King DL, Short M. Intrinsic and extrinsic predictors of video-gaming behaviour and adolescent bedtimes: the relationship between flow states, self-perceived risk-taking, device accessibility, parental regulation of media and bedtime. Sleep Med. 2017;30:64-70. [ CrossRef ] [ Medline ]
- Alimoradi Z, Lin CY, Broström A, Bülow PH, Bajalan Z, Griffiths MD, et al. Internet addiction and sleep problems: a systematic review and meta-analysis. Sleep Med Rev. 2019;47:51-61. [ CrossRef ] [ Medline ]
- Carter B, Rees P, Hale L, Bhattacharjee D, Paradkar MS. Association between portable screen-based media device access or use and sleep outcomes: a systematic review and meta-analysis. JAMA Pediatr. 2016;170(12):1202-1208. [ FREE Full text ] [ CrossRef ] [ Medline ]
- Kristensen JH, Pallesen S, King DL, Hysing M, Erevik EK. Problematic gaming and sleep: a systematic review and meta-analysis. Front Psychiatry. 2021;12:675237. [ FREE Full text ] [ CrossRef ] [ Medline ]
- Moher D, Liberati A, Tetzlaff J, Altman DG, PRISMA Group. Preferred reporting items for systematic reviews and meta-analyses: the PRISMA statement. PLoS Med. 2009;6(7):e1000097. [ FREE Full text ] [ CrossRef ] [ Medline ]
- Page MJ, McKenzie JE, Bossuyt PM, Boutron I, Hoffmann TC, Mulrow CD, et al. The PRISMA 2020 statement: an updated guideline for reporting systematic reviews. BMJ. 2021;372:n71. [ FREE Full text ] [ CrossRef ] [ Medline ]
- Akçay D, Akçay BD. The effect of computer game playing habits of university students on their sleep states. Perspect Psychiatr Care. 2020;56(4):820-826. [ CrossRef ] [ Medline ]
- Alahdal WM, Alsaedi AA, Garrni AS, Alharbi FS. The impact of smartphone addiction on sleep quality among high school students in Makkah, Saudi Arabia. Cureus. 2023;15(6):e40759. [ FREE Full text ] [ CrossRef ] [ Medline ]
- Alam A, Alshakhsi S, Al-Thani D, Ali R. The role of objectively recorded smartphone usage and personality traits in sleep quality. PeerJ Comput Sci. 2023;9:e1261. [ FREE Full text ] [ CrossRef ] [ Medline ]
- Almeida F, Marques DR, Gomes AA. A preliminary study on the association between social media at night and sleep quality: the relevance of FOMO, cognitive pre-sleep arousal, and maladaptive cognitive emotion regulation. Scand J Psychol. 2023;64(2):123-132. [ FREE Full text ] [ CrossRef ] [ Medline ]
- Alshobaili FA, AlYousefi NA. The effect of smartphone usage at bedtime on sleep quality among Saudi non-medical staff at King Saud University Medical City. J Family Med Prim Care. 2019;8(6):1953-1957. [ FREE Full text ] [ CrossRef ] [ Medline ]
- Alsulami A, Bakhsh D, Baik M, Merdad M, Aboalfaraj N. Assessment of sleep quality and its relationship to social media use among medical students. Med Sci Educ. 2019;29(1):157-161. [ FREE Full text ] [ CrossRef ] [ Medline ]
- Altintas E, Karaca Y, Hullaert T, Tassi P. Sleep quality and video game playing: effect of intensity of video game playing and mental health. Psychiatry Res. 2019;273:487-492. [ CrossRef ] [ Medline ]
- Asbee J, Slavish D, Taylor DJ, Dietch JR. Using a frequentist and Bayesian approach to examine video game usage, substance use, and sleep among college students. J Sleep Res. 2023;32(4):e13844. [ CrossRef ] [ Medline ]
- Bae ES, Kang HS, Lee HN. The mediating effect of sleep quality in the relationship between academic stress and social network service addiction tendency among adolescents. J Korean Acad Community Health Nurs. 2020;31(3):290-299. [ FREE Full text ] [ CrossRef ]
- Chatterjee S, Kar SK. Smartphone addiction and quality of sleep among Indian medical students. Psychiatry. 2021;84(2):182-191. [ CrossRef ] [ Medline ]
- Chung JE, Choi SA, Kim KT, Yee J, Kim JH, Seong JW, et al. Smartphone addiction risk and daytime sleepiness in Korean adolescents. J Paediatr Child Health. 2018;54(7):800-806. [ CrossRef ] [ Medline ]
- Demir YP, Sumer MM. Effects of smartphone overuse on headache, sleep and quality of life in migraine patients. Neurosciences (Riyadh). 2019;24(2):115-121. [ FREE Full text ] [ CrossRef ] [ Medline ]
- Dewi RK, Efendi F, Has EMM, Gunawan J. Adolescents' smartphone use at night, sleep disturbance and depressive symptoms. Int J Adolesc Med Health. 2018;33(2):20180095. [ CrossRef ] [ Medline ]
- Eden A, Ellithorpe ME, Meshi D, Ulusoy E, Grady SM. All night long: problematic media use is differentially associated with sleep quality and depression by medium. Commun Res Rep. 2021;38(3):143-149. [ CrossRef ]
- Ellithorpe ME, Meshi D, Tham SM. Problematic video gaming is associated with poor sleep quality, diet quality, and personal hygiene. Psychol Pop Media. 2023;12(2):248-253. [ CrossRef ]
- Elsheikh AA, Elsharkawy SA, Ahmed DS. Impact of smartphone use at bedtime on sleep quality and academic activities among medical students at Al -Azhar University at Cairo. J Public Health (Berl.). Jun 15, 2023.:1-10. [ FREE Full text ] [ CrossRef ]
- Gaya AR, Brum R, Brites K, Gaya A, de Borba Schneiders L, Duarte Junior MA, et al. Electronic device and social network use and sleep outcomes among adolescents: the EHDLA study. BMC Public Health. 2023;23(1):919. [ FREE Full text ] [ CrossRef ] [ Medline ]
- Gezgin DM. Understanding patterns for smartphone addiction: age, sleep duration, social network use and fear of missing out. Cypriot J Educ Sci. 2018;13(2):166-177. [ CrossRef ]
- Graham S, Mason A, Riordan B, Winter T, Scarf D. Taking a break from social media improves wellbeing through sleep quality. Cyberpsychol Behav Soc Netw. 2021;24(6):421-425. [ CrossRef ] [ Medline ]
- Guerrero MD, Barnes JD, Chaput JP, Tremblay MS. Screen time and problem behaviors in children: exploring the mediating role of sleep duration. Int J Behav Nutr Phys Act. 2019;16(1):105. [ FREE Full text ] [ CrossRef ] [ Medline ]
- Hamvai C, Kiss H, Vörös H, Fitzpatrick KM, Vargha A, Pikó BF. Association between impulsivity and cognitive capacity decrease is mediated by smartphone addiction, academic procrastination, bedtime procrastination, sleep insufficiency and daytime fatigue among medical students: a path analysis. BMC Med Educ. 2023;23(1):537. [ FREE Full text ] [ CrossRef ] [ Medline ]
- Herlache AD, Lang KM, Krizan Z. Withdrawn and wired: problematic internet use accounts for the link of neurotic withdrawal to sleep disturbances. Sleep Sci. 2018;11(2):69-73. [ FREE Full text ] [ CrossRef ] [ Medline ]
- Huang Q, Li Y, Huang S, Qi J, Shao T, Chen X, et al. Smartphone use and sleep quality in chinese college students: a preliminary study. Front Psychiatry. 2020;11:352. [ FREE Full text ] [ CrossRef ] [ Medline ]
- Hussain Z, Griffiths MD. The associations between problematic social networking site use and sleep quality, attention-deficit hyperactivity disorder, depression, anxiety and stress. Int J Ment Health Addict. 2021;19:686-700. [ FREE Full text ] [ CrossRef ]
- Imani V, Ahorsu DK, Taghizadeh N, Parsapour Z, Nejati B, Chen HP, et al. The mediating roles of anxiety, depression, sleepiness, insomnia, and sleep quality in the association between problematic social media use and quality of life among patients with cancer. Healthcare (Basel). 2022;10(9):1745. [ FREE Full text ] [ CrossRef ] [ Medline ]
- Jeong CY, Seo YS, Cho EH. The effect of SNS addiction tendency on trait-anxiety and quality of sleep in university students'. J Korean Clin Health Sci. 2018;6(2):1147-1155. [ CrossRef ]
- Karaş H, Küçükparlak İ, Özbek MG, Yılmaz T. Addictive smartphone use in the elderly: relationship with depression, anxiety and sleep quality. Psychogeriatrics. 2023;23(1):116-125. [ CrossRef ] [ Medline ]
- Kater MJ, Schlarb AA. Smartphone usage in adolescents: motives and link to sleep disturbances, stress and sleep reactivity. Somnologie. 2020;24(4):245-252. [ CrossRef ]
- Kharisma AC, Fitryasari R, Rahmawati PD. Online games addiction and the decline in sleep quality of college student gamers in the online game communities in Surabaya, Indonesia. Int J Psychosoc Rehabil. 2020;24(7):8987-8993. [ FREE Full text ] [ CrossRef ]
- Kumar VA, Chandrasekaran V, Brahadeeswari H. Prevalence of smartphone addiction and its effects on sleep quality: a cross-sectional study among medical students. Ind Psychiatry J. 2019;28(1):82-85. [ FREE Full text ] [ CrossRef ] [ Medline ]
- Lee Y, Blebea J, Janssen F, Domoff SE. The impact of smartphone and social media use on adolescent sleep quality and mental health during the COVID-19 pandemic. Hum Behav Emerg Technol. 2023;2023:3277040. [ FREE Full text ] [ CrossRef ]
- Li L, Griffiths MD, Mei S, Niu Z. Fear of missing out and smartphone addiction mediates the relationship between positive and negative affect and sleep quality among Chinese university students. Front Psychiatry. 2020;11:877. [ FREE Full text ] [ CrossRef ] [ Medline ]
- Li Y, Mu W, Sun C, Kwok SYCL. Surrounded by smartphones: relationship between peer phubbing, psychological distress, problematic smartphone use, daytime sleepiness, and subjective sleep quality. Appl Res Qual Life. 2023;18:1099-1114. [ CrossRef ]
- Luo X, Hu C. Loneliness and sleep disturbance among first-year college students: the sequential mediating effect of attachment anxiety and mobile social media dependence. Psychol Sch. 2022;59(9):1776-1789. [ CrossRef ]
- Luqman A, Masood A, Shahzad F, Shahbaz M, Feng Y. Untangling the adverse effects of late-night usage of smartphone-based SNS among university students. Behav Inf Technol. 2021;40(15):1671-1687. [ CrossRef ]
- Makhfudli, Aulia A, Pratiwi A. Relationship intensity of social media use with quality of sleep, social interaction, and self-esteem in urban adolescents in Surabaya. Sys Rev Pharm. 2020;11(5):783-788. [ CrossRef ]
- Ozcan B, Acimis NM. Sleep quality in Pamukkale university students and its relationship with smartphone addiction. Pak J Med Sci. 2021;37(1):206-211. [ FREE Full text ] [ CrossRef ] [ Medline ]
- Peltz JS, Bodenlos JS, Kingery JN, Abar C. Psychological processes linking problematic smartphone use to sleep disturbance in young adults. Sleep Health. 2023;9(4):524-531. [ CrossRef ] [ Medline ]
- Pérez-Chada D, Bioch SA, Schönfeld D, Gozal D, Perez-Lloret S, Sleep in Adolescents Collaborative Study Group. Screen use, sleep duration, daytime somnolence, and academic failure in school-aged adolescents. PLoS One. 2023;18(2):e0281379. [ FREE Full text ] [ CrossRef ] [ Medline ]
- Przepiorka A, Blachnio A. The role of Facebook intrusion, depression, and future time perspective in sleep problems among adolescents. J Res Adolesc. 2020;30(2):559-569. [ CrossRef ] [ Medline ]
- Rudolf K, Bickmann P, Froböse I, Tholl C, Wechsler K, Grieben C. Demographics and health behavior of video game and eSports players in Germany: the eSports study 2019. Int J Environ Res Public Health. 2020;17(6):1870. [ FREE Full text ] [ CrossRef ] [ Medline ]
- Sami H, Danielle L, Lihi D, Elena S. The effect of sleep disturbances and internet addiction on suicidal ideation among adolescents in the presence of depressive symptoms. Psychiatry Res. 2018;267:327-332. [ CrossRef ] [ Medline ]
- Scott H, Woods HC. Fear of missing out and sleep: cognitive behavioural factors in adolescents' nighttime social media use. J Adolesc. 2018;68:61-65. [ CrossRef ] [ Medline ]
- Spagnoli P, Balducci C, Fabbri M, Molinaro D, Barbato G. Workaholism, intensive smartphone use, and the sleep-wake cycle: a multiple mediation analysis. Int J Environ Res Public Health. 2019;16(19):3517. [ FREE Full text ] [ CrossRef ] [ Medline ]
- Stanković M, Nešić M, Čičević S, Shi Z. Association of smartphone use with depression, anxiety, stress, sleep quality, and internet addiction. empirical evidence from a smartphone application. Pers Individ Differ. Jan 2021;168:110342. [ CrossRef ]
- Tandon A, Kaur P, Dhir A, Mäntymäki M. Sleepless due to social media? investigating problematic sleep due to social media and social media sleep hygiene. Comput Hum Behav. Dec 2020;113:106487. [ FREE Full text ] [ CrossRef ]
- Wang PY, Chen KL, Yang SY, Lin PH. Relationship of sleep quality, smartphone dependence, and health-related behaviors in female junior college students. PLoS One. 2019;14(4):e0214769. [ FREE Full text ] [ CrossRef ] [ Medline ]
- Wang Q, Zhong Y, Zhao G, Song R, Zeng C. Relationship among content type of smartphone use, technostress, and sleep difficulty: a study of university students in China. Educ Inf Technol. Aug 02, 2022;28(2):1697-1714. [ CrossRef ]
- Wong HY, Mo HY, Potenza MN, Chan MNM, Lau WM, Chui TK, et al. Relationships between severity of internet gaming disorder, severity of problematic social media use, sleep quality and psychological distress. Int J Environ Res Public Health. 2020;17(6):1879. [ FREE Full text ] [ CrossRef ] [ Medline ]
- Xie X, Dong Y, Wang J. Sleep quality as a mediator of problematic smartphone use and clinical health symptoms. J Behav Addict. 2018;7(2):466-472. [ FREE Full text ] [ CrossRef ] [ Medline ]
- Yang SY, Chen KL, Lin PH, Wang PY. Relationships among health-related behaviors, smartphone dependence, and sleep duration in female junior college students. Soc Health Behav. 2019;2(1):26-31. [ FREE Full text ] [ CrossRef ]
- Yıldırım M, Öztürk A, Solmaz F. Fear of COVID-19 and sleep problems in Turkish young adults: mediating roles of happiness and problematic social networking sites use. Psihologija. 2023;56(4):497-515. [ FREE Full text ] [ CrossRef ]
- Zhai X, Ye M, Wang C, Gu Q, Huang T, Wang K, et al. Associations among physical activity and smartphone use with perceived stress and sleep quality of Chinese college students. Mental Health and Physical Activity. Mar 2020;18:100323. [ CrossRef ]
- Zhang MX, Wu AMS. Effects of smartphone addiction on sleep quality among Chinese university students: the mediating role of self-regulation and bedtime procrastination. Addict Behav. 2020;111:106552. [ CrossRef ] [ Medline ]
- Zhang MX, Zhou H, Yang HM, Wu AMS. The prospective effect of problematic smartphone use and fear of missing out on sleep among Chinese adolescents. Curr Psychol. May 24, 2021;42(7):5297-5305. [ CrossRef ]
- Beyens I, Nathanson AI. Electronic media use and sleep among preschoolers: evidence for time-shifted and less consolidated sleep. Health Commun. 2019;34(5):537-544. [ FREE Full text ] [ CrossRef ] [ Medline ]
- Mazurek MO, Engelhardt CR, Hilgard J, Sohl K. Bedtime electronic media use and sleep in children with autism spectrum disorder. J Dev Behav Pediatr. 2016;37(7):525-531. [ CrossRef ] [ Medline ]
- King DL, Delfabbro PH, Zwaans T, Kaptsis D. Sleep interference effects of pathological electronic media use during adolescence. Int J Ment Health Addict. 2014;12:21-35. [ CrossRef ]
- Kubiszewski V, Fontaine R, Rusch E, Hazouard E. Association between electronic media use and sleep habits: an eight-day follow-up study. Int J Adolesc Youth. 2013;19(3):395-407. [ FREE Full text ] [ CrossRef ]
Abbreviations
Edited by G Eysenbach, T Leung; submitted 20.04.23; peer-reviewed by M Behzadifar, F Estévez-López, R Prieto-Moreno; comments to author 18.05.23; revised version received 15.06.23; accepted 26.03.24; published 23.04.24.
©Xiaoning Han, Enze Zhou, Dong Liu. Originally published in the Journal of Medical Internet Research (https://www.jmir.org), 23.04.2024.
This is an open-access article distributed under the terms of the Creative Commons Attribution License (https://creativecommons.org/licenses/by/4.0/), which permits unrestricted use, distribution, and reproduction in any medium, provided the original work, first published in the Journal of Medical Internet Research, is properly cited. The complete bibliographic information, a link to the original publication on https://www.jmir.org/, as well as this copyright and license information must be included.
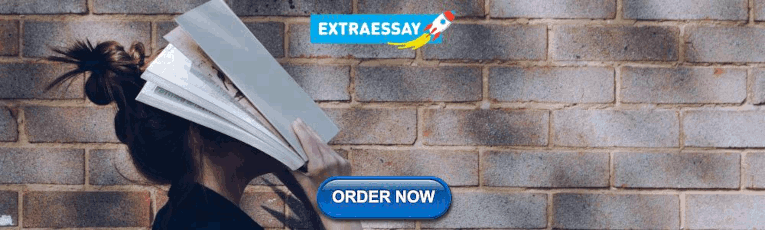
IMAGES
VIDEO
COMMENTS
About the journal; Publish with us; ... Regression analysis is an important statistical method that is commonly used to determine ... Vetter TR. Linear regression in medical research. Anesth Analg ...
Regression analysis allows researchers to understand the relationship between two or more variables by estimating the mathematical relationship between them (Sarstedt & Mooi, 2014). In this case ...
The aim of linear regression analysis is to estimate the coefficients of the regression equation b 0 and b k (k∈K) so that the sum of the squared residuals (i.e., the sum over all squared differences between the observed values of the i th observation of y i and the corresponding predicted values \( {\hat{y}}_i \)) is minimized.The lower part of Fig. 1 illustrates this approach, which is ...
The theory is briefly explained, and the interpretation of statistical parameters is illustrated with examples. The methods of regression analysis are comprehensively discussed in many standard textbooks (1- 3). Cox regression will be discussed in a later article in this journal.
Linear regression analysis involves examining the relationship between one independent and dependent variable. Statistically, the relationship between one independent variable (x) and a dependent variable (y) is expressed as: y= β 0 + β 1 x+ε. In this equation, β 0 is the y intercept and refers to the estimated value of y when x is equal to 0.
Regression analysis is a statistical technique for determining the relationship between a single dependent (criterion) variable and one or more independent (predictor) variables. The analysis yields a predicted value for the criterion resulting from a linear combination of the predictors. According to Pedhazur, 15 regression analysis has 2 uses ...
Although regression models play a central role in the analysis of medical research projects, there still exist many misconceptions on various aspects of modeling leading to faulty analyses. Indeed, the rapidly developing statistical methodology and its recent advances in regression modeling do not seem to be adequately reflected in many medical publications. This problem of knowledge transfer ...
For general information on our other products and services or for technical support, please contact our Customer Care Department within the United States at (800) 762-2974, outside the United States at (317) 572-3993 or fax (317) 572-4002. Wiley also publishes its books in a variety of electronic formats.
Regression analysis is a technique that permits one to study and measure the relation between two or more variables. Starting from data registered in a sample, regression analysis seeks to determine an estimate of a mathematical relation between two or more variables.The goal is to estimate the value of one variable as a function of one or more other variables.
Multiple regression analysis is one of the most widely used statistical procedures for both scholarly and applied marketing research. Yet, correlated predictor variables—and potential collinearity effects—are a common concern in interpretation of regression estimates.
Linear regression is used to quantify the relationship between ≥1 independent (predictor) variables and a continuous dependent (outcome) variable. In this issue of Anesthesia & Analgesia, Müller-Wirtz et al 1 report results of a study in which they used linear regression to assess the relationship in a rat model between tissue propofol ...
Journal portfolios in each of our subject areas. ... Multivariable regression analysis is widely used in transplantation research to address the problem of confounding—where the association between an exposure (independent) variable and an outcome of interest is distorted by a third variable influencing both the exposure and outcome. ...
Linear regression is an extremely versatile technique that can be used to address a variety of research questions and study aims. Researchers may want to test whether there is evidence for a relationship between a categorical (grouping) variable (eg, treatment group or patient sex) and a quantitative outcome (eg, blood pressure).
Pieter Bastiaan Ober. This is already the fifth edition of 'Introduction to Linear Regression Analysis'. With 672 pages, it is a very comprehensive introduction to linear regression analysis, with room for more than just the fundamentals. In fact, the book may be more than some would need, in particular given its rather steep price.
A hierarchical regression analysis using depression as the outcome variable was performed using stress and self-esteem as predictors in the first step, and anxiety as predictor in the second step. This analysis allows the examination of whether stress and self-esteem predict depression and if this relation is weaken in the presence of anxiety ...
A great deal of literature has been established for regression analysis of longitudinal data and in particular, many methods have been proposed for the situation where there exist some change points. However, most of these methods only apply to continuous response and focus on the situations where the change point only occurs on the response or ...
The multiple linear regression is the most widely used multivariate technique in non-laboratory sciences such as social sciences for examining the assumed causal relationships between a set of independent variables and a dependent variable. The dependent variable is a phenomenon which we seek to explain. And an independent variable is a phenomenon which we assume as a causal factor, a thing ...
In this study, data for multilinear regression analysis is occur from Sakarya University Education Faculty student's lesson (measurement and evaluation, educational psychology, program development, counseling and instructional techniques) scores and their 2012- KPSS score. Assumptions of multilinear regression analysis- normality, linearity, no ...
Linear regression analysis involves examining the rela-tionship between one independent and dependent vari-able. Statistically, the relationship between one inde-pendent variable (x) and a dependent variable (y) is expressed as: y= β 0+ β 1x+ε. In this equation, β. 0 is the y intercept and refers to the estimated value of y when x is equal ...
A Refresher on Regression Analysis. Understanding one of the most important types of data analysis. by. Amy Gallo. November 04, 2015. uptonpark/iStock/Getty Images. You probably know by now that ...
Abstract. Although regression models play a central role in the analysis of medical research projects, there still exist many misconceptions on various aspects of modeling leading to faulty analyses. Indeed, the rapidly developing statistical methodology and its recent advances in regression modeling do not seem to be adequately reflected in ...
The Fine and Gray regression analysis and competing risk modeling were conducted using the riskRegression (2021.10.10) software package. The pmsampsize (1.1.3) package was used to calculate the sample size and plot ROC and calibration curves, while the rms package was used for nomogram plotting.
Grouped single factor analysis of laboratory indicators. Only TG in the alcohol group conformed to a normal distribution for the whole group, the rest were continuous variables and did not conform to the normal distribution, analyzed by Kurskai-Wallis H-test, and LDL, N-HDL, TG, ApoB, UA, ApoE, b-ALP, APTT, PT, D-D, and PLT factors were compared between different ARCO staging groups The ...
Random-effects meta-regression analyses showed that participant age was a possible risk factor. Thus, we performed a subgroup analysis comparing effect sizes according to mean age (eTable 3 in Supplement 1). The pooled OR for studies that had included adolescent patients was 9.85 (95% CI, 4.39-22.10), representing the highest value among the ...
Testing the principle assumptions of regression analysis is a process. As such, the presentation of this process in a systems framework provides a comprehensive plan with step-by-step guidelines to help determine the optimal statistical model for a particular data set. ... Western Journal of Nursing Research. Feb 1984. Restricted access ...
Then, Cox regression model was also more accurate in predicting mortality of ATN patients based on the AUC at different time points (30, 60 and 90 days). The analysis of decision curve analysis shows that the net benefit range of Cox regression model at different time points is large, indicating that the model has good clinical effectiveness.
Thomas and Galambos used regression analysis and decision trees with the Chi-square analysis automatic detection algorithm to identify the academic satisfaction of students in academic experiences, social integration and campus services-facilities. The results obtained using the decision tree revealed that social integration is a determining ...
This article presents a comprehensive evaluation of traditional machine learning and deep learning models in analyzing sentiment trends within the SENT-COVID Twitter corpus, curated during the COVID-19 pandemic. The corpus, filtered by COVID-19 related keywords and manually annotated for polarity, is a pivotal resource for conducting sentiment analysis experiments. Our study investigates ...
The results of the meta-regression analysis using age, gender, and culture as moderators indicated that only cultural difference in the relationship between Eastern and Western culture was significant (Qbetween=6.69; P=.01). ... Journal of Medical Internet Research 8315 articles
The paper sampled data from 102 Saudi non-financial listed firms from 2012 to 2021. The data was analyzed using a fixed effect regression, while the generalized method of moments approach (GMM) was employed for robustness check. The research finding strongly suggests that the audit committee size may increase audit report lag.