Academia.edu no longer supports Internet Explorer.
To browse Academia.edu and the wider internet faster and more securely, please take a few seconds to upgrade your browser .
Enter the email address you signed up with and we'll email you a reset link.
- We're Hiring!
- Help Center
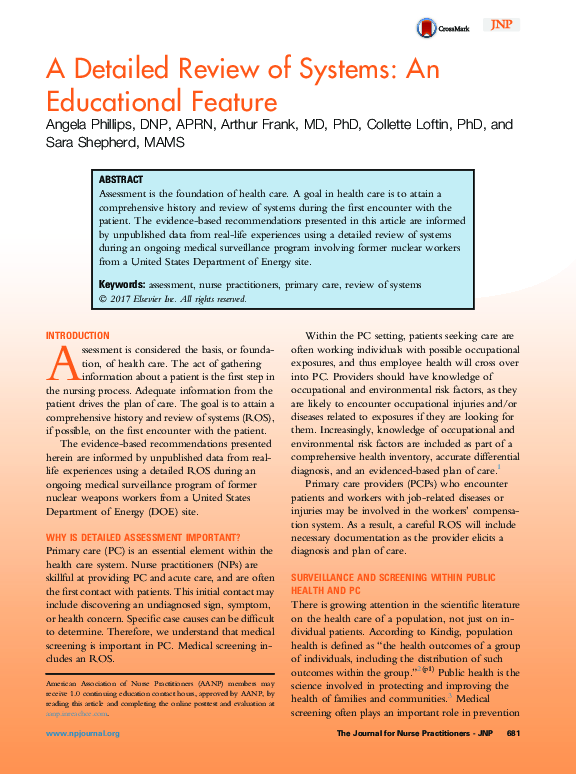
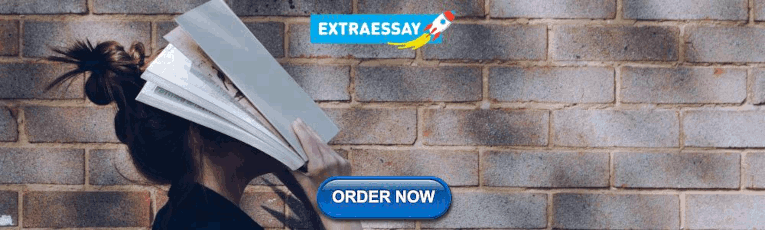
A Detailed Review of Systems: An Educational Feature

2017, The Journal for Nurse Practitioners
Related Papers
The American Journal of Medicine
Jason Szostek
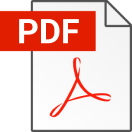
The Journal of Emergency Medicine
Robert Rodriguéz
Contemporary Systems Thinking
albert vlug
American Journal of Industrial Medicine
BMJ Quality & Safety
Sidney Dekker
Nawal Khattabi
World Healthcare Organization identifies patient Safety as a global public health concern. Despite concerted efforts made to improve the healthcare system, incidents continues to happen at same rate (Braithwaite, Wears, & Hollnagel, 2015). The most contemporary approaches to safety such as System thinking, and Safety II remain underutilized in healthcare where learning from incidents is mainly conducted using RCA. In the complexity of healthcare organization as dynamic socio-technical system, the effect of applying system thinking and learning from success during incident review is still unknown. This thesis aims at exploring how system-focused incident reviews that embed the new view of human errors and Safety II could have any impact in bridging the gap between “work as imagined” and “work as done”. The study focuses on recommendations related to policies/procedures/guidelines and how those are addressed by policy owner and perceived by the frontline staff. The recommendations at ...
Gillian Ray-Barruel
Susan Burnett
International Journal of Medical Informatics
Jeremy C Wyatt
Mukta Panda , Cecelia Wigal , Norman Desbiens
RELATED PAPERS
Walid Denguezli
Studies in Computational Intelligence
Bjorn Stenger
Actualizaciones en Sida e Infectología
Carlos Sebastián Nardi
Daniel Igbini
Acta Amazonica
Fernanda Nascimento
Humanities and Social Sciences Communications
Archives of Cardiovascular Diseases Supplements
sofien kamoun
Frontiers in Psychology
Applied Research in Quality of Life
JORNADA INTERNACIONAL DE INDIA
CIENCIA ergo sum
Daniel Gonzalez Olivares
Psicologia Escolar e Educacional
Poliana Santos Camargo
Proceedings of the 1st International Conference on Advanced Multidisciplinary Research (ICAMR 2018)
Bida Purnamasari
Trends in Biotechnology
Steffen B Petersen
RSC Advances
Konstantins Jefimovs
Human Body Interaction
Mirko Daneluzzo
Terra Latinoamericana
ENRIQUE PALACIOS VELEZ
The Visva Bharati Quarterly
Mausumi Sen Bhattacharjee
American Journal of Physiology-Endocrinology and Metabolism
Jeff Trimmer
UCLA文凭证书 加州大学洛杉矶分校文凭证书 klhjkgh
Preventive Veterinary Medicine
jerome despres
Santé Publique
Henri Chriqui
Alexandria Journal of Veterinary Sciences
Hend A. Elbarbary
Alaaddin Yorulmaz
RELATED TOPICS
- We're Hiring!
- Help Center
- Find new research papers in:
- Health Sciences
- Earth Sciences
- Cognitive Science
- Mathematics
- Computer Science
- Academia ©2024

Want to create or adapt books like this? Learn more about how Pressbooks supports open publishing practices.
Review of Systems (ROS)
The review of systems is a list of closed ended questions intended to uncover any recent symptoms that haven’t already come up. In the clinic, patients often complete the ROS on paper or online before the visit, and the provider simply reviews it. For a new patient in the hospital, physicians typically ask at least one or two of the most important questions about each organ system, but they would rarely ask all the questions on the checklist below.
A complete review of systems is important when a patient’s presenting problem is unusual or their diagnosis is very unclear. Uncovering another symptom, which the patient might not recognize as important or related, can be very helpful in narrowing the differential diagnosis
As a beginning medical student, practicing the complete ROS can help you remember which other symptoms to ask about related to your patient’s chief concern. Once you identify the organ system or systems that may be causing your patient’s problem, you’d ask all of the questions in those sections of the ROS. The presence or absence of each of these symptoms is included in the HPI.
For other systems, practice asking at least a few questions from each organ system. We encourage you NOT to use the checklist to tick off every question as you interview your patient, though you can use it to double check that you’ve asked all the pertinent questions. You’ll need to remember both the lay language that you might use with patients and the medical terms you might use in communicating with other clinicians.
Example: Review of systems
REVIEW OF SYSTEMS – FCM CHECKLIST
Download FCM ROS Checklist as a PDF
Use the middle column prior to your interview to make note of how you might ask about the particular system or symptom. In the “Notes” column, note the presence or absence of the symptom and any additional details.
The Foundations of Clinical Medicine Copyright © by Karen McDonough. All Rights Reserved.
Advertisement
How can education systems improve? A systematic literature review
- Published: 07 April 2022
- Volume 24 , pages 479–499, ( 2023 )
Cite this article
- Ignacio Barrenechea ORCID: orcid.org/0000-0002-4673-3862 1 ,
- Jason Beech 2 &
- Axel Rivas 1
1986 Accesses
8 Citations
10 Altmetric
Explore all metrics
Understanding what contributes to improving a system will help us tackle the problems in education systems that usually fail disproportionately in providing quality education for all, especially for the most disadvantage sectors of the population. This paper presents the results of a qualitative systematic literature review aimed at providing a comprehensive overview of what education research can say about the factors that promote education systems’ improvement. This literature is emerging as a topic of empirical research that merges comparative education and school effectiveness studies as standardized assessments make it possible to compare results across systems and time. To examine and synthesize the papers included in this review we followed a thematic analysis approach. We identify, analyze, and report patterns in the papers included in this systematic review. From the coding process, four drivers for system improvement emerged: (1) system-wide approaches; (2) human capital; (3) governance and macro–micro level bridges; and (4) availability of resources.
This is a preview of subscription content, log in via an institution to check access.
Access this article
Price includes VAT (Russian Federation)
Instant access to the full article PDF.
Rent this article via DeepDyve
Institutional subscriptions
Similar content being viewed by others
Ethical Considerations of Conducting Systematic Reviews in Educational Research
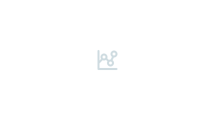
Inclusive education: Developments and challenges in South Africa
Global Comparison of Education Systems
For example, Improving schools https://journals.sagepub.com/aims-scope/IMP .
School effectiveness and school improvement https://www.tandfonline.com/action/journalInformation?show=aimsScope&journalCode=nses20 .
For example, International Congress for School Effectiveness and Improvement https://www.icsei.net/about-icsei/ .
Our search countries were Albania, Qatar, Estonia, Portugal, Poland, Peru, Ireland, Russia, Israel, and Slovenia.
Addey, C., Sellar, S., Steiner-Khamsi, G., Lingard, B., & Verger, A. (2017). The rise of international large-scale assessments and rationales for participation. Compare: A Journal of Comparative and International Education , 47 (3), 434–452.
Alves, F. (2008). Educational policies and school performance in the Brazilian capitals of states. Cadernos De Pesquisa, 38 (34), 413–440.
Article Google Scholar
Arnove, R. F. (2015). Comparative education: Dimensions and trends: A contribution to the 50th anniversary celebration of the Japan comparative education society. Comparative Education, 2015 (50), 168–177.
Auld, E., & Morris, P. (2016). PISA, policy and persuasion: Translating complex conditions into education' best practice'. Comparative Education , 52 (2), 202–229. https://doi.org/10.1080/03050068.2016.1143278 .
Barber, M., Kihn, P., & Moffit, A. (2011). Deliverology 101: A field guide for educational leaders . Corwin Press.
Barber, M., & Mourshed, M. (2007). How the world’s best-performing school systems come out on top . McKinsey & Company.
Becker, G. S. (1976). The economic approach to human behavior . University of Chicago Press.
Beech, J., & Lista, E. (2012). Flowing Discourses and Border Crossing: The Slogan of ‘Respect for Diversity’in Latin America. In World Yearbook of Education 2012 (pp. 391–410). Routledge.
Beech, J., & Rizvi, F. (2017). Revisiting Jullien in an era of globalisation. Compare: A Journal of Comparative and International Education , 47 (3), 374–387.
Betts, J. R., Zau, A., & King, K. (2005). From blueprint to reality: San Diego’s education reforms . Public Policy Institute of California.
Bin Mahfooz, S., & Hovde, K. (2010). Successful education reform: lessons from Poland. ECA Knowledge Brief , 34 (11).
Boman, B. (2020). What makes Estonia and Singapore so good? Globalisation, Societies and Education, 18 (2), 181–193.
Booth, A., Papaioannou, D., & Sutton, A. (2012). Systematic approaches to a successful literature review . Sage Publications.
Campbell, C. (2020). Educational equity in Canada: The case of Ontario’s strategies and actions to advance excellence and equity for students. School Leadership & Management , 1–20.
Carvalho, L. M., Costa, E., & Gonçalves, C. (2017). Fifteen years looking at the mirror: On the presence of PISA in education policy processes (Portugal, 2000–2016). European Journal of Education, 52 (2), 154–166.
Coffield, F. (2012). Why the McKinsey reports will not improve school systems. Journal of Education Policy, 27 (1), 131–149.
Coffield, F., & Williamson, B. (2011). From Exam Factories to Communities of Discovery. Adults Learning, 23 (2), 24–25.
Cogan, L. S., Schmidt, W. H., & Wiley, D. E. (2001). Who takes what math and in which track? Using TIMSS to characterize US students’ eighth-grade mathematics learning opportunities. Educational Evaluation and Policy Analysis, 23 (4), 323–341.
Cohen, D. K., & Spillane, J. P. (1992). Chapter 1: Policy and practice: The relations between governance and instruction. Review of research in education , 18 (1), 3–49. American Educational Research Association.
Cox, C. (2004). Innovation and reform to improve the quality of primary education: Chile. Unpublished manuscript, Ministry of Education, Santiago .
Crato, N. (2021). Setting up the Scene: Lessons Learned from PISA 2018 Statistics and Other International Student Assessments. In Improving a Country’s Education (pp. 1–24). Springer, Cham.
Cueto, S., Miranda, A., León, J., & Vásquez, M. C. (2016b). Education trajectories: From early childhood to early adulthood in Peru.
Cueto, S., León, J., & Muñoz, I. G. (2016a). Conductas, estrategias y rendimiento en lectura en PISA: análisis para el Perú. REICE: Revista Iberoamericana sobre Calidad, Eficacia y Cambio en Educación , 14 (3), 5–31.
David, J. L., & Talbert, J. E. (2012). Turning around a high-poverty school district: Learning from Sanger Unified’s success. SH Cowell Foundation .
Deaton, A. (2020). Randomization in the tropics revisited: A theme and eleven variations . Working Paper No. 27600. National Bureau Of Economic Research.
Dhaliwal, I., Duflo, E., Glennerster, R., & Tulloch, C. (2013). Comparative costeffectiveness analysis to inform policy in developing countries: a general framework with applications for education. Education policy in developing countries, 17 , 285–338.
Dinham, S., Crowther, F., Robinson, V. M., McNaughton, S., & Timperley, H. (2011). Building capacity in a self‐managing schooling system: The New Zealand experience. Journal of Educational Administration .
Dykstra, T. (2006). High performance and success in education in Flemish Belgium and the Netherlands . National Center on Education and the Economy.
Edwards, D. B., Jr. (2018). Global education policy, impact evaluations, and alternatives: The political economy of knowledge production . Springer.
Elmore, R. (2007). Educational improvement in Victoria. Unpublished internal communication.
Elmore, R. F., & Burney, D. (1998). Continuous improvement in community district# 2 . University of Pittsburgh, HPLC Project, Learning Research, and Development Center.
Fazlagić, J., & Erkol, A. (2015). Knowledge mobilisation in the Polish education system. Journal of Education for Teaching, 41 (5), 541–554.
Feniger, Y., & Lefstein, A. (2014). How not to reason with PISA data: An ironic investigation. Journal of Education Policy, 29 (6), 845–855.
Fernandez Cano, A. (2016). A methodological critique of the PISA evaluations. Relieve, 22 (1), 1–16. Disponible en: https://www.uv.es/RELIEVE/v22n1/RELIEVEv22n1_M15eng.pdf .
Fleisch, B. (2016). System-wide improvement at the instructional core: Changing reading teaching in South Africa. Journal of Educational Change, 17 (4), 437–451.
Fuhrman, S. (1993). Designing coherent education policy: Improving the system . Jossey-Bass.
Fullan, M., & Rincon-Gallardo, S. (2016). Developing high-quality public education in Canada: The case of Ontario. In Global Education Reform (pp. 169–193). Routledge.
Fullan, M. (2016). The elusive nature of whole system improvement in education. Journal of Educational Change, 17 (4), 539–544.
Glaser, B. G., & Strauss, A. (1967). The discovery of grounded theory . Aldine.
Gómez, R. L., & Suárez, A. M. (2020). Do inquiry-based teaching and school climate influence science achievement and critical thinking? Evidence from PISA 2015. International Journal of STEM Education, 7 (1), 1–11.
Graczewski, C., Knudson, J., & Holtzman, D. J. (2009). Instructional leadership in practice: What does it look like, and what influence does it have? Journal of Education for Students Placed at Risk, 14 (1), 72–96.
Hallinger, P., & Heck, R. H. (2011). Collaborative leadership and school improvement: Understanding the impact on school capacity and student learning. In International handbook of leadership for learning (pp. 469–485). Springer.
Hanushek, E. A. & Woessmann, L. (2007). Calidad de la educación y crecimiento económico . En Documento N° 39. PREAL.
Harris, A. (2010). Leading system transformation. School Leadership and Management, 30 (3), 197–207.
Harris, A., & Jones, M. S. (2017). Professional learning communities: A strategy for school and system improvement? Wales Journal of Education, 19 (1), 16–38.
Hood, C. (1991). A public management for all seasons? Public Administration, 69 (1), 3–19.
Hopfenbeck, T. N., Lenkeit, J., El Masri, Y., Cantrell, K., Ryan, J., & Baird, J. A. (2018). Lessons learned from PISA: A systematic review of peer-reviewed articles on the programme for international student assessment. Scandinavian Journal of Educational Research, 62 (3), 333–353.
Hopkins, D. (2007). Every school a great school: Realizing the potential of system leadership . McGraw-Hill Education.
Hopkins, D., Ahtaridou, E., Matthews, P., Posner, C., & Toledo, F. D. (2007). An analysis of the Mexican school system in light of PISA 2006 . London Centre for Leadership in Learning, University of London.
Hussen, T. (1994). Problems of Educational Reforms in a Changing Society. En A. Yogev y V. Rust (Eds.), International perspectives on education and society . Jai Press.
Jakubowski, M. (2015). Opening up opportunities: Education reforms in Poland. IBS Policy Paper, 1 , 2015.
Google Scholar
Jessop, B. (1998). The narrative of enterprise and the enterprise of narrative: Place marketing and the entrepreneurial city. En The entrepreneurial city: Geographies of politics, regime and representation . John Wiley.
Lapping, M. B. (2004). Education in a restoration democracy: The case of Estonia. Citizenship, Social and Economics Education, 6 (2), 101–115.
Levin, B. (2012). System-wide improvement in education. Education Policy Series, 13 , 1–38.
Lindblad, S., Pettersson, D., & Popkewitz, T. S. (2015). International comparisons of school results: A systematic review of research on large-scale assessments in education . Swedish Research Council.
LLECE-UNESCO. (2013). Análisis del clima escolar: ¿Poderoso factor que explica el aprendizaje en América Latina y el Caribe? OREALC-UNESCO Santiago.
Masino, S., & Niño-Zarazúa, M. (2016). What works to improve the quality of student learning in developing countries? International Journal of Educational Development, 48 , 53–65.
McAleavy, T., & Elwick, A. (2016). School improvement in London: A global perspective . Education Development Trust. Highbridge House, 16–18 Duke Street, Reading Berkshire, England RG1 4RU, United Kingdom.
McEwan, P. J. (2015). Improving learning in primary schools of developing countries: A meta-analysis of randomized experiments. Review of Educational Research, 85 (3), 353–394.
Mikk, J. (2015). Explaining the difference between PISA 2009 reading scores in Finland and Estonia. Educational Research and Evaluation, 21 (4), 324–342.
Morris, P. (2015). Comparative education, PISA, politics and educational reform: A cautionary note. Compare: A Journal of Comparative and International Education , 45 (3), 470–474.
Morris, P. (1996). Asia’s four little tigers: A comparison of the role of education in their development. Comparative Education, 32 (1), 95–110.
Mourshed, M., Chijioke, C., & Barber, M. (2010). How the world’s most improved school systems keep getting better. McKinsey & Company (En español: Mourshed, M., Chijioke, C. y Barber, M. (2012). Cómo continúan mejorando los sistemas educativos de mayor progreso en el mundo. Documento N° 61. PREAL).
Murnane, R. J., & Ganimian, A. (2014). Improving educational outcomes in developing countries: Lessons from rigorous impact evaluations . Working Paper No. 20284. NBER.
Murphy, J., & Hallinger, P. (1988). Characteristics of instructionally effective school districts. The Journal of Educational Research, 81 (3), 175–181.
Nguyen, X. T., Roemmele, D., & Peel, D. (2013). Education reform in Vietnam: A critical analysis of inclusion and management discourses. Journal of Asian Critical Education , 2 .
Noah, H. J., & Eckstein, M. A. (1969). Toward a science of comparative education . Macmillan.
Nóvoa, A., & Yariv-Mashal, T. (2014). Comparative research in education: A model of governance or a historical journey. En T. Fenwick, E. Mangez y J. Ozga (Eds.), World yearbook of education 2014: Governing knowledge comparison, knowledge-based technologies and expertise in the regulation of education. Routledge.
O’Day, J. A., & Smith, M. S. (2016). Quality and equality in American education: Systemic problems, systemic solutions. In The dynamics of opportunity in America (pp. 297–358). Springer.
O’Day, J., & Quick, H. E. (2009). Assessing instructional reform in San Diego: A theory-based approach. Journal of Education for Students Placed at Risk, 14 (1), 1–16.
OECD. (2019). PISA 2018 assessment and analytical framework . PISA, OECD Publishing. https://doi.org/10.1787/b25efab8-en .
Osborne, D., & Gaebler, T. (1992). Reinventing government . Reading, MA: Addison-Wesley.
Osmond-Johnson, P., & Campbell, C. (2018). Transforming an education system through professional learning: Developing educational change at scale in Ontario. Educational Research for Policy and Practice, 17 (3), 241–256.
Pang, N. S. K., & Miao, Z. (2017). The roles of teacher leadership in Shanghai education success. Bulgarian Comparative Education Society .
Paterson, G. D. (2019). Improving student learning through professional learning communities: Employing a system-wide approach. Canadian Journal for New Scholars in Education/Revue canadienne des jeunes chercheures et chercheurs en éducation , 10 (1).
Porter, C. (2002). Measuring the content of instruction: Uses in research and practice. In 2002 Presidential address . University of Wisconsin.
Quick, H. E., Holtzman, D. J., & Chaney, K. R. (2009). Professional development and instructional practice: Conceptions and evidence of effectiveness. Journal of Education for Students Placed at Risk, 14 (1), 45–71.
Rindermann, H., & Ceci, S. J. (2009). Educational policy and country outcomes in international cognitive competence studies. Perspectives on Psychological Science, 4 (6), 551–568.
Rivas, A. (2015). América Latina después de PISA: Lecciones aprendidas sobre la educación en siete países . CIPPEC, Natura e Instituto Natura.
Rivas, A. et al. (2020): Las llaves de la educación. Estudio comparado sobre la mejora de los sistemas educativos subnacionales en América Latina, Fundación Santillana, Madrid.
Rivas, A., & Scasso, M. G. (2021). Low stakes, high risks: The problem of intertemporal validity of PISA in Latin America. Journal of Education Policy, 36 (2), 279–302.
Rizvi, F., & Beech, J. (2017). Global mobilities and the possibilities of a cosmopolitan curriculum. Curriculum Inquiry, 47 (1), 125–134.
Sahlberg, P. (2011). The fourth way of Finland. Journal of Educational Change, 12 (2), 173–185.
Sam, C., & Riggan, M. (2013). Building district capacity for system-wide instructional improvement in Cincinnati public schools. Working Paper. GE Foundation" Developing Futures "™ in Education Evaluation Series. Consortium for Policy Research in Education .
Schleicher, A. (2012). Preparing teachers and developing school leaders for the 21st century: Lessons from around the world . OECD Publishing. 2, rue Andre Pascal, F-75775 Paris Cedex 16, France.
Schleicher, A. (2018). How to build a 21st-century school system . OECD Publishing.
Schmidt, W. H., & Prawat, R. S. (2006). Curriculum coherence and national control of education: Issue or non-issue? Journal of Curriculum Studies, 38 (6), 641–658.
Schmidt, W. H., Wang, H. C., & McKnight, C. C. (2005). Curriculum coherence: An examination of US mathematics and science content standards from an international perspective. Journal of Curriculum Studies, 37 , 525–559.
Schneider, B. R., Estarellas, P. C., & Bruns, B. (2019). The politics of transforming education in Ecuador: Confrontation and continuity, 2006–2017. Comparative Education Review, 63 (2), 259–280.
Sellar, S., & Lingard, B. (2013). The OECD and the expansion of PISA: New global modes of governance in education. British Educational Research Journal, 40 (6), 917–936.
Snilstveit, B., Stevenson, J., Menon, R., Phillips, D., Gallagher, E., Geleen, M., et al. (2016). The impact of education programmes on learning and school participation in low-and middle-income countries.
Snipes, J., Doolittle, F., & Herlihy, C. (2002). Foundations for success: Case studies of how urban school systems improve student achievement. Council of the Great City Schools.
Snyder, H. (2019). Literature review as a research methodology: An overview and guidelines. Journal of Business Research, 104 , 333–339.
Steiner-Khamsi, G. (2019a). Randomized controlled trials: League leader in the hierarchy of evidence?. En R. Gorur y S. Sellar (Eds.), World yearbook of education 2019a: Comparative methodology in the era of big data and global networks . Routledge.
Steiner-Khamsi, G. (2019b). Conclusions: What policy-makers do with PISA. Understanding PISA’s attractiveness: Critical analyses in comparative policy studies , 233.
Steiner-Khamsi, G. (2016). New directions in policy borrowing research. Asia Pacific Education Review, 17 (3), 381–390.
Tan, C. (2019). Parental responses to education reform in Singapore, Shanghai and Hong Kong. Asia Pacific Education Review, 20 (1), 91–99.
Trace, A. (1961) What Ivan knows that Johnny doesn’t: A comparison of Soviet and American school programs. Harper.
Tucker, M. (Ed.). (2011). Surpassing Shanghai. An agenda for American education built on the world’s leading systems . Harvard Education Press.
Tyack, D. B. and Cuban, L. (1995). Tinkering toward utopia . Harvard University Press.
Valverde, G. A. (2014). Educational quality: global politics, comparative inquiry, and opportunities to learn. Comparative Education Review, 58 (4), 575–589.
Verger, A., Novelli, M., & Altinyelken, H. K. (2012). Global education policy and international development: An introductory framework. Global education policy and international development: New agendas, issues and policies , 3–32.
Wenger, E., McDermott, R. A., & Snyder, W. (2002). Cultivating communities of practice: A guide to managing knowledge . Harvard Business Press.
Zavadsky, H. (2016). Bringing effective instructional practice to scale in American schools: Lessons from the Long Beach Unified School District. Journal of Educational Change, 17 (4), 505–527.
Download references
Author information
Authors and affiliations.
Universidad de San Andrés, Victoria, Argentina
Ignacio Barrenechea & Axel Rivas
Monash University, Clayton, VIC, Australia
Jason Beech
You can also search for this author in PubMed Google Scholar
Corresponding author
Correspondence to Ignacio Barrenechea .
Additional information
Publisher's note.
Springer Nature remains neutral with regard to jurisdictional claims in published maps and institutional affiliations.
Rights and permissions
Reprints and permissions
About this article
Barrenechea, I., Beech, J. & Rivas, A. How can education systems improve? A systematic literature review. J Educ Change 24 , 479–499 (2023). https://doi.org/10.1007/s10833-022-09453-7
Download citation
Accepted : 03 March 2022
Published : 07 April 2022
Issue Date : September 2023
DOI : https://doi.org/10.1007/s10833-022-09453-7
Share this article
Anyone you share the following link with will be able to read this content:
Sorry, a shareable link is not currently available for this article.
Provided by the Springer Nature SharedIt content-sharing initiative
- Educational change
- System-wide improvement
- Comparative education
- International education
- Find a journal
- Publish with us
- Track your research
Systems thinking to transform schools: Identifying levers that lift educational quality
- Download the full policy brief
Subscribe to the Center for Universal Education Bulletin
Bruce fuller and bruce fuller sociologist - university of california, berkeley, author - "when schools work" (2021) hoyun kim hoyun kim ph.d. student - berkeley school of education.
September 12, 2022
The United Nations has set forth an ambitious vision for education systems around the globe: cultivating lifelong learning from early childhood through an individual’s civic and work life. Schools must support children and youth in basic learning—including crucial socio-emotional, literacy, and numeracy competencies—to contribute to sustainable societies. State-run education systems and their communities must now engage these global goals by 2030.
But in the wake of the global pandemic, virtually every country in the world is far behind. Prior to the pandemic, a severe learning crisis held back hundreds of millions of children. Analysts project that 9 out of 10 children in low-income countries and 5 out of 10 in middle-income countries will not develop core secondary education skills in literacy and numeracy by 2030. The pandemic has only deepened the learning crisis and widened achievement gaps.
By crisply defining what systems entail in the education sector and which levers yield organizational change, education leaders and their partners can do better in rethinking the aims of schooling and raising student achievement.
Specific country cases remain distressing: More than half of fifth grade students in India are not proficient in second-grade literacy. In Nigeria, just 1 in 10 girls completing grade six can read a single sentence in their native language. In the United States, children of African-American or Latino heritage attending fourth grade read at two grade levels below white peers on average.
Beyond deepening inequality in foundational learning, calls to rethink the underlying aims of education grow louder and more urgent. The next generation’s future—marked by global warming, fragile economic sustainability, and worsening inequality—requires new skills and wider awareness, too often poorly addressed in classrooms around the globe. The digital revolution has already shifted what and how children learn and explore and the knowledge pathways they maneuver—a radical change that many education systems fail to harness to advance learning.
Related Content
The Hon. Minister David Sengeh, Rebecca Winthrop
June 23, 2022
Rebecca Winthrop, The Hon. Minister David Sengeh
Hayin Kimner, Stacey Campo, Abe Fernández
August 15, 2022
Against this evolving backdrop, the global education community—catalyzed by the U.N. Secretary General’s Transforming Education Summit (September 2022)—is looking past recovering from the pandemic to consider full-scale system transformation. This blossoming policy discourse is replete with hopes for radically improving and transforming education systems. But how to define educational systems and then reshape them remains poorly defined. We cannot merely utter this ambitious goal without precisely defining how to surround the system, identify potent levers for change, and rethink the aims and means of human learning on a fragile planet.
INFORMING POLICY DEBATES
This brief informs these discussions of system transformation by reviewing the historical roots of systems thinking and what they contribute to education reform. It draws primarily on the intellectual traditions and literature in the Global North but also illustrates how these ideas have traveled to the Global South, in part through the work of organizations such as the World Bank. We recognize how elements of education systems may unfold quite differently across diverse societies—for example, rethinking how teachers are prepared and motivated to recast what students are expected to learn—and can draw from a range of cultural traditions. Our goals are simply to lift up how we think about systems and harness their strengths to rethink what children should learn and how classrooms and communities can better motivate achievement and civic engagement.
The brief is arranged in the following four sections:
- A short history of systems thinking, emphasizing the pressure points or organizational levers inside the education institution that touch classrooms.
- How systems thinking moved into the education sector (from biology and mechanics), along with how differing versions of systems reform take root and are conceptualized.
- Clarifying the various concepts and pathways associated with systems thinking in the education sector.
- Concluding reflections, informing how education leaders might interrogate system improvement and transformation efforts.
We summarize cases around the globe where systems thinking has yielded discernible gains for students and teachers. By crisply defining what systems entail in the education sector and which levers yield organizational change, education leaders and their partners can do better in rethinking the aims of schooling and raising student achievement.
Download the full brief»
Global Education K-12 Education
Global Economy and Development
Center for Universal Education
Thinley Choden
May 3, 2024
Ghulam Omar Qargha, Rachel Dyl, Sreehari Ravindranath, Nariman Moustafa, Erika Faz de la Paz
Kathy Hirsh-Pasek, Rebecca Winthrop, Sweta Shah
May 2, 2024

An official website of the United States government
The .gov means it’s official. Federal government websites often end in .gov or .mil. Before sharing sensitive information, make sure you’re on a federal government site.
The site is secure. The https:// ensures that you are connecting to the official website and that any information you provide is encrypted and transmitted securely.
- Publications
- Account settings
Preview improvements coming to the PMC website in October 2024. Learn More or Try it out now .
- Advanced Search
- Journal List
- Springer Nature - PMC COVID-19 Collection

A systematic literature review on educational recommender systems for teaching and learning: research trends, limitations and opportunities
Felipe leite da silva.
1 Centro de Estudos Interdisciplinares em Novas Tecnologias da Educação, Universidade Federal do Rio Grande do Sul, Porto Alegre, Rio Grande do Sul Brazil
Bruna Kin Slodkowski
Ketia kellen araújo da silva, sílvio césar cazella.
2 Departamento de Ciências Exatas e Sociais Aplicadas, Universidade Federal de Ciências da Saúde de Porto Alegre, Porto Alegre, Rio Grande do Sul Brazil
Associated Data
The datasets generated during the current study correspond to the papers identified through the systematic literature review and the quality evaluation results (refer to Section 3.4 in paper). They are available from the corresponding author on reasonable request.
Recommender systems have become one of the main tools for personalized content filtering in the educational domain. Those who support teaching and learning activities, particularly, have gained increasing attention in the past years. This growing interest has motivated the emergence of new approaches and models in the field, in spite of it, there is a gap in literature about the current trends on how recommendations have been produced, how recommenders have been evaluated as well as what are the research limitations and opportunities for advancement in the field. In this regard, this paper reports the main findings of a systematic literature review covering these four dimensions. The study is based on the analysis of a set of primary studies ( N = 16 out of 756, published from 2015 to 2020) included according to defined criteria. Results indicate that the hybrid approach has been the leading strategy for recommendation production. Concerning the purpose of the evaluation, the recommenders were evaluated mainly regarding the quality of accuracy and a reduced number of studies were found that investigated their pedagogical effectiveness. This evidence points to a potential research opportunity for the development of multidimensional evaluation frameworks that effectively support the verification of the impact of recommendations on the teaching and learning process. Also, we identify and discuss main limitations to clarify current difficulties that demand attention for future research.
Supplementary Information
The online version contains supplementary material available at 10.1007/s10639-022-11341-9.
Introduction
Digital technologies are increasingly integrated into different application domains. Particularly in education, there is a vast interest in using them as mediators of the teaching and learning process. In such a task, the computational apparatus serves as an instrument to support human knowledge acquisition from different educational methodologies and pedagogical practices (Becker, 1993 ).
In this sense, Educational Recommender Systems (ERS) play an important role for both educators and students (Maria et al., 2019 ). For instructors, these systems can contribute to their pedagogical practices through recommendations that improve their planning and assist in educational resources filtering. As for the learners, through preferences and educational constraints recognition, recommenders can contribute for their academic performance and motivation by indicating personalized learning content (Garcia-Martinez & Hamou-Lhadj, 2013 ).
Despite the benefits, there are known issues upon the usage of the recommender system in the educational domain. One of the main challenges is to find an appropriate correspondence between the expectations of users and the recommendations (Cazella et al., 2014 ). Difficulties arise from differences in learner’s educational interests and needs (Verbert et al., 2012 ). The variety of student’s individual factors that can influence the learning process (Buder & Schwind, 2012 ) is one of the challenging matters that makes it complex to be overcome. On a recommender standpoint, this reflects an input diversity with potential to tune recommendations for users.
In another perspective, from a technological and artificial intelligence standpoint, the ERS are likely to suffer from already known issues noted on the general-purpose ones, such as the cold start and data sparsity problems (Garcia-Martinez & Hamou-Lhadj, 2013 ). Furthermore, problems are related to the approach used to generate recommendations. For instance, the overspecialization is inherently associated with the way that content-based recommender systems handle data (Iaquinta et al., 2008 ; Khusro et al., 2016 ). These issues pose difficulties to design recommenders that best suit the user’s learning needs and that distance themselves from user’s dissatisfaction in the short and long term.
From an educational point of view, issues emerge on how to evaluate ERS effectiveness. A usual strategy to measure the quality of educational recommenders is to apply the traditional recommender’s evaluation methods (Erdt et al., 2015 ). This approach determines system quality based on performance properties, such as its precision and prediction accuracy. Nevertheless, in the educational domain, system effectiveness needs to take into account the students’ learning performance. This dimension brings new complexities on how to successfully evaluate ERS.
As ERS topic has gradually increased in attraction for scientific community (Zhong et al., 2019 ), extensive research have been carried out in recent years to address these issues (Manouselis et al. 2010 ; Manouselis et al., 2014 ; Tarus et al., 2018 ; George & Lal, 2019 ). ERS has become a field of application and combination of different computational techniques, such as data mining, information filtering and machine learning, among others (Tarus et al., 2018 ). This scenario indicates a diversity in the design and evaluation of recommender systems that support teaching and learning activities. Nonetheless, research is dispersed in literature and there is no recent study that encompasses the current scientific efforts in the field that reveals how such issues are addressed in current research. Reviewing evidence, and synthesizing findings of current approaches in how ERS produce recommendations, how ERS are evaluated and what are research limitations and opportunities can provide a panoramic perspective of the research topic and support practitioners and researchers for implementation and future research directions.
From the aforementioned perspective, this work aims to investigate and summarize the main trends and research opportunities on ERS topic through a Systematic Literature Review (SLR). The study was conducted based on the last six years publications, particularly, regarding to recommenders that support teaching and learning process.
Main trends referrer to recent research direction on the ERS field. They are analyzed in regard to how recommender systems produce recommendations and how they are evaluated. As mentioned above, these are significant dimensions related to current issues of the area. Specifically for the recommendation production, this paper provides a three-axis-based analysis centered on systems underlying techniques, input data and results presentation.
Additionally, research opportunities in the field of ERS as well as their main limitations are highlighted. Because current comprehension of these aspects is fragmented in literature, such an analysis can shed light for future studies.
The SLR was carried out using Kitchenham and Charters ( 2007 ) guidelines. The SLR is the main method for summarizing evidence related to a topic or a research question (Kitchenham et al., 2009 ). Kitchenham and Charters ( 2007 ) guidelines, in turn, are one of the leading orientations for reviews on information technology in education (Dermeval et al., 2020 ).
The remainder of this paper is structured as follows. In Section 2 , the related works are presented. Section 3 details the methodology used in carrying out the SLR. Section 4 covers the SLR results and related discussion. Section 5 presents the conclusion.
Related works
In the field of education, there is a growing interest in technologies that support teaching and learning activities. For this purpose, ERS are strategic solutions to provide a personalized educational experience. Research in this sense has attracted the attention of the scientific community and there has been an effort to map and summarize different aspects of the field in the last 6 years.
In Drachsler et al. ( 2015 ) a comprehensive review of technology enhanced learning recommender systems was carried out. The authors analyzed 82 papers published from 2000 to 2014 and provided an overview of the area. Different aspects were analyzed about recommenders’ approach, source of information and evaluation. Additionally, a categorization framework is presented and the study includes the classification of selected papers according to it.
Klašnja-Milićević et al. ( 2015 ) conducted a review on recommendation systems for e-learning environments. The study focuses on requirements, challenges, (dis)advantages of techniques in the design of this type of ERS. An analysis on collaborative tagging systems and their integration in e-learning platform recommenders is also discussed.
Ferreira et al. ( 2017 ) investigated particularities of research on ERS in Brazil. Papers published between 2012 and 2016 in three Brazilian scientific vehicles were analyzed. Rivera et al. ( 2018 ) presented a big picture of the ERS area through a systematic mapping. The study covered a larger set of papers and aimed to detect global characteristics in ERS research. Aiming at the same focus, however, setting different questions and repositories combination, Pinho, Barwaldt, Espíndola, Torres, Pias, Topin, Borba and Oliveira (2019) performed a systematic review on ERS. In these works, it is observed the common concern of providing insights about the systems evaluation methods and the main techniques adopted in the recommendation process.
Nascimento et al. ( 2017 ) carried out a SLR covering learning objects recommender systems based on the user’s learning styles. Learning objects metadata standards, learning style theoretical models, e-learning systems used to provide recommendations and the techniques used by the ERS were investigated.
Tarus et al ( 2018 ) and George and Lal ( 2019 ) concentrated their reviews on ontology-based ERS. Tarus et al. ( 2018 ) examined research distribution in a period from 2005 to 2014 according to their years of publication. Furthermore, the authors summarized the techniques, knowledge representation, ontology types and ontology representations covered in the papers. George and Lal ( 2019 ), in turn, update the contributions of Tarus et al. ( 2018 ), investigating papers published between 2010 and 2019. The authors also discuss how ontology-based ERS can be used to address recommender systems traditional issues, such as cold start problem and rating sparsity.
Ashraf et al. ( 2021 ) directed their attention to investigate course recommendation systems. Through a comprehensive review, the study summarized the techniques and parameters used by this type of ERS. Additionally, a taxonomy of the factors taken into account in the course recommendation process was defined. Salazar et al. ( 2021 ), on the other hand, conducted a review on affectivity-based ERS. Authors presented a macro analysis, identifying the main authors and research trends, and summarized different recommender systems aspects, such as the techniques used in affectivity analysis, the source of affectivity data collection and how to model emotions.
Khanal et al. ( 2019 ) reviewed e-learning recommendation systems based on machine learning algorithms. A total of 10 papers from two scientific vehicles and published between 2016 and 2018 were examined. The study focal point was to investigate four categories of recommenders: those based on collaborative filtering, content-based filtering, knowledge and a hybrid strategy. The dimensions analyzed were the machine learning algorithms used, the recommenders’ evaluation process, inputs and outputs characterization and recommenders’ challenges addressed.
Related works gaps and contribution of this study
The studies presented in the previous section have a diversity of scope and dimensions of analysis, however, in general, they can be classified into two distinct groups. The first, focus on specific subjects of ERS field, such as similar methods of recommendations (George & Lal, 2019 ; Khanal et al., 2019 ; Salazar et al., 2021 ; Tarus et al., 2018 ) and same kind of recommendable resources (Ashraf et al., 2021 ; Nascimento et al., 2017 ). This type of research scrutinizes the particularities of the recommenders and highlights aspects that are difficult to be identified in reviews with a broader scope. Despite that, most of the reviews concentrate on analyses of recommenders’ operational features and have limited discussion on crosswise issues, such as ERS evaluation and presentation approaches. Khanal et al. ( 2019 ), specifically, makes contributions regarding evaluation, but the analysis is limited to four types of recommender systems.
The second group is composed of wider scope reviews and include recommendation models based on a diversity of methods, inputs and outputs strategies (Drachsler et al., 2015 ; Ferreira et al., 2017 ; Klašnja-Milićević et al., 2015 ; Pinho et al., 2019 ; Rivera et al., 2018 ). Due to the very nature of systematic mappings, the research conducted by Ferreira et al. ( 2017 ) and Rivera et al. ( 2018 ) do not reach in depth some topics, for example, the data synthesized on the evaluations of the ERS are delimited to indicate only the methods used. Ferreira et al. ( 2017 ), in particular, aims to investigate only Brazilian recommendation systems, offering partial contributions to an understanding of the state of the art of the area. In Pinho et al. ( 2019 ) it is noted the same limitation of the systematic mappings. The review was reported with a restricted number of pages, making it difficult to detail the findings. On the other hand, Drachsler et al. ( 2015 ) and, Klašnja-Milićević et al. ( 2015 ) carried out comprehensive reviews that summarizes specific and macro dimensions of the area. However, the papers included in their reviews were published until 2014 and there is a gap on the visto que advances and trends in the field in the last 6 years.
Given the above, as far as the authors are aware, there is no wide scope secondary study that aggregate the research achievements on recommendation systems that support teaching and learning in recent years. Moreover, a review in this sense is necessary since personalization has become an important feature in the teaching and learning context and ERS are one of main tools to deal with different educational needs and preferences that affect individuals’ learning process.
In order to widen the frontiers of knowledge in the field of research, this review aims to contribute to the area by presenting a detailed analysis of the following dimensions: how recommendations are produced and presented, how recommender systems are evaluated and what are the studies limitations and research opportunities. Specifically, to summarize the current knowledge, a SLR was conducted based on four research questions (Section 3.1 ). The review focused on papers published from 2015 to 2020 in scientific journals. A quality assessment was performed to select the most mature systems. The data found on the investigated topics are summarized and discussed in Section 4 .
Methodology
This study is based on the SLR methodology for gathering evidences related to the research topic investigated. As stated by Kitchenham and Charters ( 2007 ) and Kitchenham et al. ( 2009 ), this method provides the means for aggregate evidences from current research prioritizing the impartiality and reproducibility of the review. Therefore, a SLR is based on a process that entails the development of a review protocol that guides the selection of relevant studies and the subsequent extraction of data for analysis.
Guidelines for SLR are widely described in literature and the method can be applied for gathering evidences in different domains, such as, medicine and social science (Khan et al., 2003 ; Pai et al., 2004 ; Petticrew & Roberts, 2006 ; Moher et al., 2015 ). Particularly for informatics in education area, Kitchenham and Charters ( 2007 ) guidelines have been reported as one of the main orientations (Dermeval et al, 2020 ). Their approach appears in several studies (Petri & Gresse von Wangenheim, 2017 ; Medeiros et al., 2019 ; Herpich et al, 2019 ) including mappings and reviews on ERS field (Rivera et al., 2018 ; Tarus et al., 2018 ).
As mentioned in Section 1 , Kitchenham and Charters ( 2007 ) guidelines were used in the conducted SLR. They are based on three main stages: the first for planning the review, the second for conducting it and the last for the results report. Following these orientations, the review was structured in three phases with seven main activities distributed among them as depicted in Fig. 1 .

Systematic literature review phases and activities
The first was the planning phase. The identification of the need for a SLR about teaching and learning support recommenders and the development of the review protocol occurred on this stage. In activity 1, the search for SLR with the intended scope of this study was performed. The result did not return compatible papers with this review scope. Papers identified are described in Section 2 . In activity 2, the review process was defined. The protocol was elaborated through rounds of discussion by the authors until consensus was reached. The activity 2 output were the research questions, search strategy, papers selection strategy and the data extraction method.
The next was the conducting phase. At this point, activities for relevant papers identification (activity 3) and selection (activities 4) were executed. In Activity 3, searches were carried out in seven repositories indicated by Dermeval et al. ( 2020 ) as relevant to the area of informatics in education. Authors applied the search string into these repositories search engines, however, due to the large number of returned research, the authors established the limit of 600 to 800 papers that would be analyzed. Thus, three repositories whose sum of search results was within the established limits were chosen. The list of potential repositories considered for this review and the selected ones is listed in Section 3.1 . The search string used is also shown in Section 3.1 .
In activity 4, studies were selected through two steps. In the first, inclusion and exclusion criteria were applied to each identified paper. Accepted papers had they quality assessed in the second step. Parsifal 1 was used to manage planning and conducting phase data. Parsifal is a web system, adhering to Kitchenham and Charters ( 2007 ) guidelines, that helps in SLR conduction. At the end of this step, relevant data were extracted (activity 5) and registered in a spreadsheet. Finally, in the reporting phase, the extracted data were analyzed in order to answer the SLR research questions (activity 6) and the results were recorded in this paper (activity 7).
Research question, search string and repositories
Teaching and learning support recommender systems have particularities of configuration, design and evaluation method. Therefore, the following research questions (Table (Table1) 1 ) were elaborated in an effort to synthesize these knowledge as well as the main limitations and research opportunities in the field from the perspective of the most recent studies:
SLR research questions
Regarding the search strategy, papers were selected from three digital repositories (Table (Table2). 2 ). For the search, “Education” and “Recommender system” were defined as the keywords and synonyms were derived from them as secondary terms (Table (Table3). 3 ). From these words, the following search string was elaborated:
- ("Education" OR "Educational" OR "E-learning" OR "Learning" OR "Learn") AND ("Recommender system" OR "Recommender systems" OR "Recommendation system" OR "Recommendation systems" OR "Recommending system" OR "Recommending systems")
Repositories considered for the SLR
Keywords and their synonyms used in the search string
Inclusion and exclusion criteria
The first step for the selection of papers was performed through the application of objective criteria, thus a set of inclusion and exclusion criteria was defined. The approved papers formed a group that comprises the primary studies with potential relevance for the scope of the SLR. Table Table4 4 lists the defined criteria. In the description column of Table Table4, 4 , the criteria are informed and in the id column they are identified with a code. The latter was defined appending an abbreviation of the respective kind of criteria (IC for Inclusion Criteria and EC for Exclusion Criteria) with an index following the sequence of the list. The Id is used for referencing its corresponding criterion in the rest of this document.
Inclusion and exclusion criteria of the SLR
Since the focus of this review is on the analysis of recent ERS publications, only studies from the past 6 years (2015–2020) were screened (see IC1). Targeting mature recommender systems, only full papers from scientific journals that present the recommendation system evaluation were considered (see IC2, IC4 and IC7). Also, solely works written in English language were selected, because they are the most expressive in quantity and are within the reading ability of the authors (see IC3). Search string was verified on papers’ title, abstract and keywords to ensure only studies related to the ERS field were screened (see IC5). The IC6, specifically, delimited the subject of selected papers and aligned it to the scope of the review. Additionally, it prevented the selection of secondary studies in process (e.g., others reviews or systematic mappings). Conversely, exclusion criteria were defined to clarify that papers contrasting with the inclusion criteria should be excluded from review (see EC1 to EC8). Finally, duplicate searches were marked and, when all criteria were met, only the latest was selected.
Quality evaluation
The second step in studies selection activity was the quality evaluation of the papers. A set of questions were defined with answers of different weights to estimate the quality of the studies. The objective of this phase was to filter researches with higher: (i) validity; (ii) details of the context and implications of the research; and (iii) description of the proposed recommenders. Research that detailed the configuration of the experiment and carried out an external validation of the ERS obtained higher weight in the quality assessment. Hence, the questions related to recommender evaluation (QA8 and QA9) ranged from 0 to 3, while the others, from 0 to 2. The questions and their respective answers are presented in Table Table7 7 (see Appendix). Each paper evaluated had a total weight calculated according to Formula 1 :
Quality evaluation questions and answers
Papers total weight range from 0 to 10. Only works that reached the minimum weight of 7 were accepted.
Screening process
Papers screening process occurred as shown in Fig. 2 . Initially, three authors carried out the identification of the studies. In this activity, the search string was applied into search engines of the repositories along with the inclusion and exclusion criteria through filtering settings. Two searches were undertaken on the three repositories at distinct moments, one in November 2020 and another in January 2021. The second one was performed to ensure that all 2020 published papers in the repositories were counted. A number of 756 preliminary primary studies were returned and their metadata were registered in Parsifal.

Flow of papers search and selection
Following the protocol, the selection activity was initiated. At the start, the duplicity verification feature of Parsifal was used. A total of 5 duplicate papers were returned and the oldest copies were ignored. Afterwards, papers were divided into groups and distributed among the authors. Inclusion and exclusion criteria were applied through titles and abstracts reading. In cases which were not possible to determine the eligibility of the papers based on these two fields, the body of text was read until it was possible to apply all criteria accurately. Finally, 41 studies remained for the next step. Once more, papers were divided into three groups and each set of works was evaluated by one author. Studies were read in full and weighted according to each quality assessment question. At any stage of this process, when questions arose, the authors defined a solution through consensus. As a final result of the selection activity, 16 papers were approved for data extraction.
Procedure for data analysis
Data from selected papers were extracted in a data collection form that registered general information and specific information. The general information extracted was: reviewer identification, date of data extraction and title, authors and origin of the paper. General information was used to manage the data extraction activity. The specific information was: recommendation approach, recommendation techniques, input parameters, data collection strategy, method for data collection, evaluation methodology, evaluation settings, evaluation approaches, evaluation metrics. This information was used to answer the research questions. Tabulated records were interpreted and a descriptive summary with the findings was prepared.
Results and discussion
In this section, the SLR results are presented. Firstly, an overview of the selected papers is introduced. Next, the finds are analyzed from the perspective of each research question in a respective subsection.
Selected papers overview
Each selected paper presents a distinct recommendation approach that advances the ERS field. Following, an overview of these studies is provided.
Sergis and Sampson ( 2016 ) present a recommendation system that supports educators’ teaching practices through the selection of learning objects from educational repositories. It generates recommendations based on the level of instructors’ proficiency on ICT Competences. In Tarus et al. ( 2017 ), the recommendations are targeted at students. The study proposes an e-learning resource recommender based on both user and item information mapped through ontologies.
Nafea et al. ( 2019 ) propose three recommendation approaches. They combine item ratings with student’s learning styles for learning objects recommendation. Klašnja-Milićević et al. ( 2018 ) present a recommender of learning materials based on tags defined by the learners. The recommender is incorporated in Protus e-learning system.
In Wan and Niu ( 2016 ), a recommender based on mixed concept mapping and immunological algorithms is proposed. It produces sequences of learning objects for students. In a different approach, the same authors incorporate the self-organization theory into ERS. Wan and Niu ( 2018 ) deals with the notion of self-organizing learning objects. In this research, resources behave as individuals who can move towards learners. This movement results in recommendations and is triggered based on students’ learning attributes and actions. Wan and Niu ( 2020 ), in turn, self-organization refers to the approach of students motivated by their learning needs. The authors propose an ERS that recommends self-organized cliques of learners and, based on these, recommend learning objects.
Zapata et al. ( 2015 ) developed a learning object recommendation strategy for teachers. The study describes a methodology based on collaborative methodology and voting aggregation strategies for the group recommendations. This approach is implemented in the Delphos recommender system. In a similar research line, Rahman and Abdullah ( 2018 ) show an ERS that recommends Google results tailored to students’ academic profile. The proposed system classifies learners into groups and, according to the similarity of their members, indicates web pages related to shared interests.
Wu et al. ( 2015 ) propose a recommendation system for e-learning environments. In this study, complexity and uncertainties related to user profile data and learning activities is modeled through tree structures combined with fuzzy logic. Recommendations are produced from matches of these structures. Ismail et al. ( 2019 ) developed a recommender to support informal learning. It suggests Wikipedia content taking into account unstructured textual platform data and user behavior.
Huang et al. ( 2019 ) present a system for recommending optional courses. The system indications rely on the student’s curriculum time constraints and similarity of academic performance between him and senior students. The time that individuals dedicate for learning is also a relevant factor in Nabizadeh et al. ( 2020 ). In this research, a learning path recommender that includes lessons and learning objects is proposed. Such a system estimates the learner’s good performance score and, based on that, produces a learning path that satisfies their time constraints. The recommendation approach also provides indication of auxiliary resources for those who do not reach the estimated performance.
Fernandez-Garcia et al. ( 2020 ) deals with recommendations of disciplines through a dataset with few instances and sparse. The authors developed a model based on several techniques of data mining and machine learning to support students’ decision in choosing subjects. Wu et al. ( 2020 ) create a recommender that captures students’ mastery of a topic and produces a list of exercises with a level of difficulty adapted to them. Yanes et al. ( 2020 ) developed a recommendation system, based on different machine learning algorithms, that provides appropriate actions to assist teachers to improve the quality of teaching strategies.
How teaching and learning support recommender systems produce recommendations?
The process of generating recommendations is analyzed based on two axes. Underlying techniques of recommender systems are discussed first then input parameters are covered. Studies details are provided in Table Table5 5 .
Summary of ERS techniques and input parameters used in the selected papers
Techniques approaches
Through selected papers analysis is observed that hybrid recommendation systems are predominant in selected papers. Such recommenders are characterized by computing predictions through a set of two or more algorithms in order to mitigate or avoid the limitations of pure recommendation systems (Isinkaye et al., 2015 ). From sixteen analyzed papers, thirteen (p = 81,25%) are based on hybridization. This tendency seems to be related with the support that hybrid approach provides for development of recommender systems that must meet multiple educational needs of users. For example, Sergis and Sampson ( 2016 ) proposed a recommender based on two main techniques: fuzzy set to deal with uncertainty about teacher competence level and Collaborative Filtering (CF) to select learning objects based on neighbors who may have competences similarities. In Tarus et al. ( 2017 ) students and learning resources profiles are represented as ontologies. The system calculates predictions based on them and recommends learning items through a mechanism that applies collaborative filtering followed by a sequential pattern mining algorithm.
Moreover, the hybrid approach that combines CF and Content-Based Filtering (CBF), although a traditional technique (Bobadilla, Ortega, Hernando and Gutiérrez, 2013), it seems to be not popular in teaching and learning support recommender systems research. From the selected papers, only Nafea et al. ( 2019 ) has a proposal in this regard. Additionally, the extracted data indicates that a significant number of hybrid recommendation systems (p = 53.85%, n = 7) have been built based on the combination of methods of treatment or representation of data, such as the use of ontologies and fuzzy sets, with methods to generate recommendation. For example, Wu et al. ( 2015 ) structure users profile data and learning activities through fuzzy trees. In such structures the values assigned to their nodes are represented by fuzzy sets. The fuzzy tree data model and users’ ratings feed a tree structured data matching method and a CF algorithm for similarities calculation.
Collaborative filtering recommendation paradigm, in turn, plays an important role in research. Nearly a third of the studies (p = 30.77%, n = 4) that propose hybrid recommenders includes a CF-based strategy. In fact, this is the most frequent pure technique on the research set. A total of 31.25%( n = 5) are based on a CF adapted version or combine it with other approaches. CBF-based recommenders, in contrast, have not shared the same popularity. This technique is an established recommendation approach that produces results based on the similarity between items known to the user and others recommendable items (Bobadilla et al., 2013 ). Only Nafea et al. ( 2019 ) propose a CBF-based recommendation system.
Also, CF user-based variant is widely used in analyzed research. In this version, predictions are calculated by similarity between users, as opposed to the item-based version where predictions are based on item similarities (Isinkaye et al., 2015 ). All CF-based recommendation systems identified, whether pure or combined with other techniques, use this variant.
The above finds seem to be related to the growing perception, in the education domain, of the relevance of a student-centered teaching and learning process (Krahenbuhl, 2016 ; Mccombs, 2013 ). Recommendation approaches that are based on users’ profile, such as interests, needs, and capabilities, naturally fit this notion and are more widely used than those based on other information such as the characteristics of the recommended items.
Input parameters approaches
In regard to the inputs consumed in the recommendation process, collected data shows that the main parameters are attributes related to users’ educational profile. Examples are ICT competences (Sergis & Sampson, 2016 ); learning objectives (Wan & Niu, 2018 ; Wu et al., 2015 ), learning styles (Nafea et al., 2019 ), learning levels (Tarus et al., 2017 ) and different academic data (Yanes et al., 2020 ; Fernández-García et al., 2020). Only 25% ( n = 4) of the systems apply item-related information in the recommendation process. Furthermore, with the exception of the Nafea et al. ( 2019 ) CBF-based recommendation, the others are based on a combination of items and users’ information. A complete list of the identified input parameters is provided in Table Table5 5 .
Academic information and learning styles, compared to others parameters, features highly on research. They appear, respectively, in 37.5% ( n = 6) and 31.25% ( n = 5) papers. Student’s scores (Huang et al., 2019 ), academic background (Yanes et al., 2020 ), learning categories (Wu et al., 2015 ) and subjects taken (Fernández-García et al.,2020) are some of the academic data used. Learning styles, in turn, are predominantly based on Felder ( 1988 ) theory. Wan and Niu ( 2016 ), exceptionally, combine Felder ( 1988 ), Kolb et al. ( 2001 ) and Betoret ( 2007 ) to build a specific notion of learning styles. This is also used in two other researchers, carried out by the same authors, and has a questionnaire also developed by them (Wan & Niu, 2018 , 2020 ).
Regarding the way inputs are captured, it was observed that explicit feedback is prioritized over others data collection strategies. In this approach, users have to directly provide the information that will be used in the process of preparing recommendations (Isinkaye et al., 2015 ). Half of analyzed studies are based only on explicit feedback. The use of graphical interface components (Klašnja-Milićević et al., 2018 ), questionnaires (Wan & Niu, 2016 ) and manual entry of datasets (Wu et al., 2020 ; Yanes et al., 2020 ) are the main methods identified.
Only 18.75%( n = 3) ERS rely solely on gathering information through implicit feedback, that is, when inputs are inferred by the system (Isinkaye et al., 2015 ). This type of data collection appears to be more popular when applied with an explicit feedback method for enhancing the prediction tasks. Recommenders that combine both approaches occur in 31.25%( n = 5) of the studies. Implicit data collection methods identified are user’s data usage tracking, as access, browsing and rating history (Rahman & Abdullah, 2018 ; Sergis & Sampson, 2016 ; Wan & Niu, 2018 ), data extraction from another system (Ismail et al., 2019 ), users data session monitoring (Rahman & Abdullah, 2018 ) and data estimation (Nabizadeh et al., 2020 ).
The aforementioned results indicate that, in the context of the teaching and learning support recommender systems, the implicit collection of data has usually been explored in a complementary way to the explicit one. A possible rationale is that the inference of information is noisy and less accurate (Isinkaye et al., 2015 ) and, therefore, the recommendations produced from it involve greater complexity to be adjusted to the users’ expectations (Nichols, 1998 ). This aspect makes it difficult to apply the strategy in isolation and can be a factor that produces greater user dissatisfaction when compared to the disadvantage of the acquisition load of the explicit strategy inputs.
How teaching and learning support recommender systems present recommendations?
From the analyzed paper, two approaches for presenting recommendations are identified. The majority of the proposed ERS are based on a listing of ranked items according to a per-user prediction calculation (p = 87.5%, n = 14). This strategy is applied in all cases where the supported task is to find good items that assist users in teaching and learning tasks (Ricci et al., 2015 ; Drachsler et al., 2015 ). The second one, is based on a learning pathway generation. In this case, recommendations are displayed through a series of linked items tied by some prerequisites. Only 2 recommenders use this approach. In them, the sequence is established by learning objects association attributes (Wan & Niu, 2016 ) and by a combination of prior knowledge of the user, the time he has available and a learning score (Nabizadeh et al., 2020 ). These ERS are associated with the item sequence recommendation task and are intended to guide users who wish to achieve a specific knowledge (Drachsler et al., 2015 ).
In a further examination, it is observed that more than a half (62.5%, n = 10) do not present details of how recommendations list is presented to the end user. In Huang et al. ( 2019 ), for example, there is a vague description of a production of predicted scores for students and a list of the top-n optional courses and it is not specified how this list is displayed. This may be related to the fact that most of these recommenders do not report an integration into another system (e.g., learning management systems) or the purpose of making it available as a standalone tool (e.g., web or mobile recommendation system). The absence of such requirements mitigates the need for the development of a refined presentation interface. Only Tarus et al. ( 2017 ), Wan and Niu ( 2018 ) and Nafea et al. ( 2019 ) propose recommenders incorporated in an e-learning system and do not detail the way in which the results are exhibited. In the six papers that provide insights about recommendation presentation, a few of them (33.33%, n = 2), have a graphical interface that explicitly seeks to capture the attention of the user who may be performing another task in the system. This approach highlights recommendations and is common in commercial systems (Beel, Langer and Genzmehr, 2013). In Rahman and Abdullah ( 2018 ), a panel entitled “recommendations for you” is used. In Ismail et al. ( 2019 ), a pop-up box with suggestions is displayed to the user. The other part of the studies exhibits organic recommendations, i.e., naturally arranged items for user interaction (Beel et al., 2013 ).
In Zapata et al. ( 2015 ), after the user defines some parameters, a list of recommended learning objects that are returned similarly to a search engine result. As for the aggregation methods, another item recommended by the system, only the strategy that fits better to the interests of the group is recommended. The result is visualized through a five-star Likert scale that represents the users’ consensus rating. In Klašnja-Milićević et al. ( 2018 ) and Wu et al. ( 2015 ), the recommenders’ results are listed in the main area of the system. In Nabizadeh et al. ( 2020 ) the learning path occupies a panel on the screen and the items associated with it are displayed as the user progresses through the steps. The view of the auxiliary learning objects is not described in the paper. These three last recommenders do not include filtering settings and distance themselves from the archetype of a search engine.
Also, a significant number of researches are centralized on learning objects recommendations (p = 56.25%, n = 9). Other researches recommendable items identified are learning activities (Wu et al., 2015 ), pedagogical actions (Yanes et al., 2020 ), web pages (Ismail et al., 2019 ; Rahman & Abdullah, 2018 ), exercises (Wu et al., 2020 ), aggregation methods (Zapata et al., 2015 ), lessons (Nabizadeh et al., 2020 ) and subjects (Fernández-García et al., 2020). None of the study relates the way of displaying results to the recommended item. This is a topic that needs further investigation to answer whether there are more appropriate ways to present specific types of items to the user.
How teaching and learning support recommender systems are evaluated?
In ERS, there are three main evaluation methodologies (Manouselis et al., 2013 ). One of them is the offline experiment, which is based on the use of pre-collected or simulated data to test recommenders’ prediction quality (Shani & Gunawardana, 2010 ). User study is the second approach. It takes place in a controlled environment where information related to real interactions of users are collected (Shani & Gunawardana, 2010 ). This type of evaluation can be conducted, for example, through a questionnaire and A/B tests (Shani & Gunawardana, 2010 ). Finally, the online experiment, also called real life testing, is one in which recommenders are used under real conditions by the intended users (Shani & Gunawardana, 2010 ).
In view of these definitions, the analyzed researches comprise only user studies and offline experiments in reported experiments. Each of these methods were identified in 68.75% ( n = 11) papers respectively. Note that they are not exclusive for all cases and therefore the sum of the percentages is greater than 100%. For example, Klašnja-Milićević et al. ( 2018 ) and Nafea et al. ( 2019 ) assessed the quality of ERS predictions from datasets analysis and also asked users to use the systems to investigate their attractiveness. Both evaluation methods are carried out jointly in 37.5%( n = 6) papers. When comparing with methods exclusive usage, each one is conducted at 31.25% ( n = 5). Therefore, the two methods seem to have a balanced popularity. Real-life tests, on the contrary, although they are the ones that best demonstrate the quality of a recommender (Shani & Gunawardana, 2010 ), are the most avoided, probably due to the high cost and complexity of execution.
An interesting finding concerns user study methods used in research. When associated with offline experiments, the user satisfaction assessment is the most common ( p = 80%, n = 5). Of these, only Nabizadeh et al. ( 2020 ) performed an in-depth evaluation combining a satisfaction questionnaire with an experiment to verify the pedagogical effectiveness of their recommender. Wu et al. ( 2015 ), in particular, does not include a satisfaction survey. They conducted a qualitative investigation of user interactions and experiences.
Although questionnaires assist in identification of users’ valuables information, it is sensitive to respondents’ intentions and can be biased with erroneous answers (Shani & Gunawardana, 2010 ). Papers that present only user studies, in contrast, have a higher rate of experiments that results in direct evidence about the recommender’s effectiveness in teaching and learning. All papers in this group have some investigation in this sense. Wan and Niu ( 2018 ), for example, verified whether the recommender influenced the academic score of students and their time to reach a learning objective. Rahman and Abdullah ( 2018 ) investigated whether the recommender impacted the time students took to complete a task.
Regarding the purpose of the evaluations, ten distinct research goals were identified. Through Fig. 3 , it is observed that the occurrence of accuracy investigation excelled the others. Only 1 study did not carry out experiments in this regard. Different traditional metrics were identified for measuring the accuracy of recommenders. The Mean Absolute Error (MAE), in particular, has the higher frequency. Table Table6 6 lists the main metrics identified.

Evaluation purpose of recommender systems in selected papers
Summary of ERS evaluation settings, approaches and metrics in selected papers
The system attractiveness analysis, through the verification of user satisfaction, has the second highest occurrence. It is present in 62.5% ( n = 10) studies. The pedagogical effectiveness evaluation of the ERS has a reduced participation in the studies and occurs in only 37.5% ( n = 6). Experiments to examine recommendations diversity, user’s profile elicitation accuracy, evolution process, user’s experience and interactions, entropy, novelty and perceived usefulness and easiness were also identified, albeit to a lesser extent.
Also, 81.25% ( n = 13) papers presented experiments to achieve multiple purposes. For example, in Wan and Niu ( 2020 ) an evaluation is carried out to investigate recommenders’ pedagogical effectiveness, student satisfaction, accuracy, diversity of recommendations and entropy. Only in Huang et al. ( 2019 ), Fernandez-Garcia et al. ( 2020 ) and Yanes et al. ( 2020 ) evaluated a single recommender system dimension.
The upper evidence suggests an engagement of the scientific community in demonstrating the quality of the recommender systems developed through multidimensional analysis. However, offline experiments and user studies, particularly those based on questionnaires, are mostly adopted and can lead to incomplete or biased interpretations. Thus, such data also signalize the need for a greater effort to conduct real life tests and experiments that lead to an understanding of the real impact of recommenders on the teaching and learning process. Researches that synthesize and discuss the empirical possibilities of evaluating the pedagogical effectiveness of ERS can help to increase the popularity of these experiments.
Through papers analysis is also find that the results of offline experiments are usually based on a greater amount of data compared to user studies. In this group, 63.64% ( n = 7) of evaluation datasets have records of more than 100 users. User studies, on the other hand, predominate sets of up to 100 participants in the experiments (72.72%, n = 8). In general, offline assessments that have smaller datasets are those that occur in association with a user study. This is because the data for both experiments usually come from the same subjects (Nafea et al., 2019 ; Tarus et al., 2017 ). The cost (e.g., time and money) related to surveying participants for the experiment is possibly a determining factor in defining appropriate samples.
Furthermore, it is also verified that the greater parcel of offline experiments has a 70/30% division approach for training and testing data. Nguyen et al. ( 2021 ) give some insights in this sense arguing that this is the most suitable ratio for training and validating machine learning models. Further details on recommendation systems evaluation approaches and metrics are presented in Table Table6 6 .
What are the limitations and research opportunities related to the teaching and learning support recommender systems field?
The main limitations observed in selected papers are presented below. They are based on articles’ explicit statements and on authors’ formulations. In this section, only those that are transverse to the majority of the studies are listed. Next, a set of research opportunities for future investigations are pointed out.
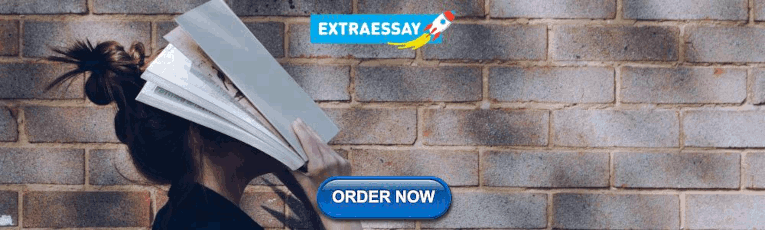
Research limitations
Research limitations are factors that hinders current progress in the ERS field. Knowing these factors can assist researchers to attempt coping with them on their study and mitigate the possibility of the area stagnation, that is, when new proposed recommenders does not truly generate better outcomes than the baselines (Anelli et al., 2021 ; Dacrema et al., 2021 ). As a result of this SLR, research limitations were identified in three strands that are presented below.
Reproducibility restriction
The majority of the papers report a specifically collected dataset to evaluate the proposed ERS. The main reason for this is the scarcity of public datasets suited to the research’s needs, as highlighted by some authors (Nabizadeh et al., 2020 ; Tarus et al., 2017 ; Wan & Niu, 2018 ; Wu et al., 2015 ; Yanes et al., 2020 ). Such approach restricts the feasibility of experiment reproduction and makes it difficult to compare recommenders. In fact, this is an old issue in the ERS field. Verbert et al. ( 2011 ) observed, in the beginning of the last decade, the necessity to improve reproducibility and comparison on ERS in order to provide stronger conclusions about their validity and generalizability. Although there was an effort in this direction in the following years based on a broad educational dataset sharing, currently, most of the known ones (Çano & Morisio, 2015 ; Drachsler et al., 2015 ) are retired, and the remaining, proved not to be sufficient to meet current research demands. Of the analyzed studies, only Wu et al. ( 2020 ) use public educational datasets.
Due to the fact that datasets sharing play an important role for recommenders’ model reproduction and comparison in the same conditions, this finding highlight the need of a research community effort for the creation of means to supply this need (e.g., development of public repositories) in order to mitigate current reproducibility limitation.
Dataset size / No of subjects
As can be observed on Table Table6, 6 , a few experimental results are based on a large amount of data. Only five studies have information from 1000 or more users. In particular, the offline evaluation conducted by Wu et al. ( 2015 ), despite having an extensive dataset, uses MovieLens records and is not based on real information related to teaching and learning. Another limitation concerns where data comes from, it is usually from a single origin (e.g., class of a college).
Although experiments based on small datasets can reveal the relevance of an ERS, an evaluation based on a large-scale dataset should provide stronger conclusions on recommendation effectiveness (Verbert et al., 2011 ). Experiments based on larger and more diverse data (e.g., users from different areas and domains) would contribute to most generalizable results. On another hand, scarcity of public dataset may be impairing the quantity and diversity of data used on scientific experiments in the ERS field. As reported by Nabizadeh et al. ( 2020 ), the increasement of the size of the experiment is costly in different aspects. If more public dataset were available, researchers would be more likely to find the ones that could be aligned to their needs and, naturally, increasing the size of their experiment. In this sense, they could be favored by reducing data acquisition difficulty and cost. Furthermore, the scientific community would access users’ data out of their surrounding context and could base their experiments on diversified data.
Lack of in-depth investigation of the impact of known issues in the recommendation system field
Cold start, overspecialization and sparsity are some known challenges in the field of recommender systems (Khusro et al., 2016 ). They are mainly related to a reduced and unequally distributed number of users’ feedback or item description used for generating recommendations (Kunaver & Požrl, 2017 ). These issues also permeate the ERS Field. For instance, in Cechinel et al. ( 2011 ) is reported that on a sample of more than 6000 learning objects from Merlot repository was observed a reduced number of users ratings over items. Cechinel et al. ( 2013 ), in turn, observed, in a dataset from the same repository, a pattern of few users rating several resources while the vast number of them rating 5 or less. Since such issues directly impact the quality of recommendations, teaching and learning support recommenders should be evaluated considering such issues to clarify in which extent they can be effective in real life situations. Conversely, in this SLR, we detected an expressive number of papers (43.75%, n = 7) that do not analyze or discuss how the recommenders behave or handle, at least partially, these issues. Studies that rely on experiments to examine such aspects would elucidate more details of the quality of the proposed systems.
Research opportunities
From the analyzed papers, a set of research opportunities were identified. They are based on gaps related to the subjects explored through the research questions of this SLR. The identified opportunities provide insights of under-explored topics that need further investigation taking into account their potential to contribute to the advancement of the ERS field. Research opportunities were identified in three strands that are presented below.
Study of the potential of overlooked user’s attributes
The papers examined present ERS based on a variety of inputs. Preferences, prior knowledge, learning style, and learning objectives are some examples (Table (Table5 5 has the complete list). Actually, as reported by Chen and Wang ( 2021 ), this is aligned with a current research trend of investigating the relationships between individual differences and personalized learning. Nevertheless, one evidence that rises from this SLR also confirms that “some essential individual differences are neglected in existing works” (Chen & Wang, 2021 ). The papers sample suggests a lack of studies that incorporate, in recommendation model, others notably relevant information, such as emotional state and cultural context of students (Maravanyika & Dlodlo, 2018 ; Salazar et al., 2021 ; Yanes et al., 2020 ). This indicates that further investigation is needed in order to clarify the true contributions and existing complexities of collect, measure and apply these other parameters. In this sense, an open research opportunity refers to the investigation of these other users’ attributes in order to explore the impact of such characteristics on the quality of ERS results.
Increase studies on the application of ERS in informal learning situations
Informal learning refers to a type of learning that, typically, occurs out of an education institution (Pöntinen et al., 2017 ). In it, learners do not follow a structured curriculum or have a domain expert to guide him (Pöntinen et al., 2017 ; Santos & Ali, 2012 ). Such aspects influence how ERS can support users. For instance, in informal settings, content can come from multiple providers, as a consequence, it can be delivered without taking into account a proper pedagogical sequence. ERS targeting this scenario, in turn, should concentrate on organizing and sequencing recommendations guiding users’ learning process (Drachsler et al., 2009 ).
Although literature highlight the existence of significative differences on the design of educational recommenders that involves formal or informal learning circumstance (Drachsler et al., 2009 ;Okoye et al, 2012 ; Manouselis et al., 2013 ; Harrathi & Braham, 2021 ), through this SLR was observed that current studies tend to not be explicit in reporting this characteristic. This scenario makes it difficult to obtain a clear landscape of the current field situation in this dimension. Nonetheless, through the characteristics of the proposed ERS, it was observed that current research seems to be concentrated on the formal learning context. This is because recommenders from analyzed papers usually use data that are maintained by institutional learning systems. Moreover, recommendations, predominantly, do not provide a pedagogical sequencing to support self-directed and self-paced learning (e.g., recommendations that build a learning path to lead to specific knowledge). Conversely, informal learning has increasingly gained attention of the scientific community with the emergence of the coronavirus pandemic (Watkins & Marsick, 2020 ).
In view of this, the lack of studies of ERS targeting informal learning settings open a research opportunity. Specifically, further investigation focused on the design and evaluation of recommenders that take into consideration different contexts (ex. location or used device) and that guide users through a learning sequence to achieve a specific knowledge would figure prominently in this context considering the less structured format informal learning circumstances has in terms of learning objectives and learning support.
Studies on the development of multidimensional evaluation frameworks
Evidence from this study shows that the main purpose of ERS evaluation has been to assess recommender’s accuracy and users’ satisfaction (Section 4.4 ). This result, connected with Erdt et al. ( 2015 ) reveals a two decade of evaluation predominantly based on these two goals. Even though others evaluation purposes had a reduced participation in research, they are also critical for measuring the success of ERS. Moubayed et al. ( 2018 ), for example, highlights two e-learning systems evaluation aspects, one is concerned with how to properly evaluate the student performance, the other refers to measuring learners’ learning gains through systems usage. Tahereh et al. ( 2013 ) identifies that stakeholder and indicators associated with technological quality are relevant to consider in educational system assessment. From the perspective of recommender systems field, there are also important aspects to be analyzed in the context of its application in the educational domain such as novelty and diversity (Pu et al., 2011 ; Cremonesi et al., 2013 ; Erdt et al., 2015 ).
Upon this context, it is noted that, although evaluating recommender's accuracy and users’ satisfaction give insights about the value of the ERS, they are not sufficient to fully indicate the quality of the system in supporting the learning process. Other different factors reported in literature are relevant to take in consideration. However, to the best of our knowledge, there is no framework that identifies and organizes these factors to be considered in an ERS evaluation, leading to difficulties for the scientific community to be aware of them and incorporate them in studies.
Because the evaluation of ERS needs to be a joint effort between computer scientists and experts from other domains (Erdt et al., 2015 ), further investigation should be carried out seeking the development of a multidimensional evaluation framework that encompass evaluation requirements based on a multidisciplinary perspective. Such studies would clarify the different dimensions that have the potential to contribute to better ERS evaluation and could even identify which one should be prioritized to truly assess learning impact with reduced cost.
In recent years, there has been an extensive scientific effort to develop recommenders that meet different educational needs; however, research is dispersed in literature and there is no recent study that encompasses the current scientific efforts in the field.
Given this context, this paper presents an SLR that aims to analyze and synthesize the main trends, limitations and research opportunities related to the teaching and learning support recommender systems area. Specifically, this study contributes to the field providing a summary and an analysis of the current available information about the teaching and learning support recommender systems topic in four dimensions: (i) how the recommendations are produced (ii) how the recommendations are presented to the users (iii) how the recommender systems are evaluated and (iv) what are the limitations and opportunities for research in the area.
Evidences are based on primary studies published from 2015 to 2020 from three repositories. Through this review, it is provided an overarching perspective of current evidence-based practice in ERS in order to support practitioners and researchers for implementation and future research directions. Also, research limitations and opportunities are summarized in light of current studies.
The findings, in terms of current trends, shows that hybrid techniques are the most used in teaching and learning support recommender systems field. Furthermore, it is noted that approaches that naturally fit a user centered design (e.g., techniques that allow to represent students’ educational constraints) have been prioritized over that based on other aspects, like item characteristics (e.g., CBF Technique). Results show that these approaches have been recognized as the main means to support users with recommendations in their teaching and learning process and provide directions for practitioners and researchers who seek to base their activities and investigations on evidence from current studies. On the other hand, this study also reveals that highly featured techniques in the major topic of general recommender systems, such as the bandit-based and the deep learning ones (Barraza-Urbina & Glowacka, 2020 ; Zhang et al., 2020 ), have been underexplored, implying a mismatch between the areas. Therefore, the result of this systematic review indicates that a greater scientific effort should be employed to investigate the potential of these uncovered approaches.
With respect to recommendation presentation, the organic display is the most used strategy. However, most of the researches have the tendency to not show details of the used approach making it difficult to understand the state of the art of this dimension. Furthermore, among other results, it is observed that the majority of the ERS evaluation are based on the accuracy of recommenders and user's satisfaction analysis. Such a find open research opportunity scientific community for the development of multidimensional evaluation frameworks that effectively support the verification of the impact of recommendations on the teaching and learning process.
Lastly, the limitations identified indicate that difficulties related to obtaining data to carry out evaluations of ERS is a reality that extends for more than a decade (Verbert et al., 2011 ) and call for scientific community attention for the treatment of this situation. Likewise, the lack of in-depth investigation of the impact of known issues in the recommendation system field, another limitation identified, points to the importance of aspects that must be considered in the design and evaluation of these systems in order to provide a better elucidation of their potential application in a real scenario.
With regard to research limitations and opportunities, some of this study findings indicate the need for a greater effort in the conduction of evaluations that provide direct evidence of the systems pedagogical effectiveness and the development of a multidimensional evaluation frameworks for ERS is suggested as a research opportunity. Also, it was observed a scarcity of public dataset usage on current studies that leads to limitation in terms of reproducibility and comparison of recommenders. This seems to be related to a restricted number of public datasets currently available, and such aspect can also be affecting the size of experiments conducted by researchers.
In terms of limitations of this study, the first refers to the number of datasources used for paper selection. Only the repositories mentioned in Section 3.1 were considered. Thus, the scope of this work is restricted to evidence from publications indexed by these platforms. Furthermore, only publications written in English were examined, thus, results of papers written in other languages are beyond the scope of this work. Also, the research limitations and opportunities presented on Section 4.5 were identified based on the extracted data used to answer this SLR research questions, therefore they are limited to their scope. As a consequence, limitations and opportunities of the ERS field that surpass this context were not identified nor discussed in this study. Finally, the SLR was directed to papers published in scientific journals and, due to this, the results obtained do not reflect the state of the area from the perspective of conference publications. In future research, it is intended to address such limitations.
Electronic supplementary material
Below is the link to the electronic supplementary material.
Table Table7 7
Author contribution
Felipe Leite da Silva: Conceptualization, Methodology approach, Data curation, Writing – original draft. Bruna Kin Slodkowski: Data curation, Writing – original draft. Ketia Kellen Araújo da Silva: Data curation, Writing – original draft. Sílvio César Cazella: Supervision and Monitoring of the research; Writing – review & editing.
Data availability statement
Informed consent.
This research does not involve human participation as research subject, therefore research subject consent does not apply.
Authors consent with the content presented in the submitted manuscript.
Financial and non-financial interests
The authors have no relevant financial or non-financial interests to disclose.
Research involving human participants and/or animals
This research does not involve an experiment with human or animal participation.
Competing interests
The authors have no competing interests to declare that are relevant to the content of this article.
1 http://parsif.al/
Publisher's note
Springer Nature remains neutral with regard to jurisdictional claims in published maps and institutional affiliations.
- Anelli, V. W., Bellogín, A., Di Noia, T., & Pomo, C. (2021). Revisioning the comparison between neural collaborative filtering and matrix factorization. Proceedings of the Fifteenth ACM Conference on Recommender Systems , 521–529. 10.1145/3460231.3475944
- Ashraf E, Manickam S, Karuppayah S. A comprehensive review of curse recommender systems in e-learning. Journal of Educators Online. 2021; 18 :23–35. [ Google Scholar ]
- Barraza-Urbina, A., & Glowacka, D. (2020). Introduction to Bandits in Recommender Systems. Proceedings of the Fourteenth ACM Conference on Recommender Systems , 748–750. 10.1145/3383313.3411547
- Becker F. Teacher epistemology: The daily life of the school. 1. Editora Vozes; 1993. [ Google Scholar ]
- Beel J, Langer S, Genzmehr M. Sponsored vs. Organic (Research Paper) Recommendations and the Impact of Labeling. In: Aalberg T, Papatheodorou C, Dobreva M, Tsakonas G, Farrugia CJ, editors. Research and Advanced Technology for Digital Libraries. Springer Berlin Heidelberg; 2013. pp. 391–395. [ Google Scholar ]
- Betoret F. The influence of students’ and teachers’ thinking styles on student course satisfaction and on their learning process. Educational Psychology. 2007; 27 (2):219–234. doi: 10.1080/01443410601066701. [ CrossRef ] [ Google Scholar ]
- Bobadilla J, Serradilla F, Hernando A. Collaborative filtering adapted to recommender systems of e-learning. Knowledge-Based Systems. 2009; 22 (4):261–265. doi: 10.1016/j.knosys.2009.01.008. [ CrossRef ] [ Google Scholar ]
- Bobadilla J, Ortega F, Hernando A, Gutiérrez A. Recommender systems survey. Knowledge-Based Systems. 2013; 46 :109–132. doi: 10.1016/j.knosys.2013.03.012. [ CrossRef ] [ Google Scholar ]
- Buder J, Schwind C. Learning with personalized recommender systems: A psychological view. Computers in Human Behavior. 2012; 28 (1):207–216. doi: 10.1016/j.chb.2011.09.002. [ CrossRef ] [ Google Scholar ]
- Çano, E., & Morisio, M. (2015). Characterization of public datasets for Recommender Systems. (2015 IEEE 1 st ) International Forum on Research and Technologies for Society and Industry Leveraging a better tomorrow (RTSI) , 249–257.10.1109/RTSI.2015.7325106
- Cazella SC, Behar PA, Schneider D, Silva KKd, Freitas R. Developing a learning objects recommender system based on competences to education: Experience report. New Perspectives in Information Systems and Technologies. 2014; 2 :217–226. doi: 10.1007/978-3-319-05948-8_21. [ CrossRef ] [ Google Scholar ]
- Cechinel C, Sánchez-Alonso S, García-Barriocanal E. Statistical profiles of highly-rated learning objects. Computers & Education. 2011; 57 (1):1255–1269. doi: 10.1016/j.compedu.2011.01.012. [ CrossRef ] [ Google Scholar ]
- Cechinel C, Sicilia M-Á, Sánchez-Alonso S, García-Barriocanal E. Evaluating collaborative filtering recommendations inside large learning object repositories. Information Processing & Management. 2013; 49 (1):34–50. doi: 10.1016/j.ipm.2012.07.004. [ CrossRef ] [ Google Scholar ]
- Chen SY, Wang J-H. Individual differences and personalized learning: A review and appraisal. Universal Access in the Information Society. 2021; 20 (4):833–849. doi: 10.1007/s10209-020-00753-4. [ CrossRef ] [ Google Scholar ]
- Cremonesi P, Garzotto F, Turrin R. User-centric vs. system-centric evaluation of recommender systems. In: Kotzé P, Marsden G, Lindgaard G, Wesson J, Winckler M, editors. Human-Computer Interaction – INTERACT 2013, 334–351. Springer Berlin Heidelberg; 2013. [ Google Scholar ]
- Dacrema MF, Boglio S, Cremonesi P, Jannach D. A troubling analysis of reproducibility and progress in recommender systems research. ACM Transactions on Information Systems. 2021; 39 (2):1–49. doi: 10.1145/3434185. [ CrossRef ] [ Google Scholar ]
- Dermeval, D., Coelho, J.A.P.d.M., & Bittencourt, I.I. (2020). Mapeamento Sistemático e Revisão Sistemática da Literatura em Informática na Educação. Metodologia de Pesquisa Científica em Informática na Educação: Abordagem Quantitativa . Porto Alegre. https://jodi-ojs-tdl.tdl.org/jodi/article/view/442
- Drachsler H, Hummel HGK, Koper R. Identifying the goal, user model and conditions of recommender systems for formal and informal learning. Journal of Digital Information. 2009; 10 (2):1–17. [ Google Scholar ]
- Drachsler H, Verbert K, Santos OC, Manouselis N. Panorama of Recommender Systems to Support Learning. In: Ricci F, Rokach L, Shapira B, editors. Recommender Systems Handbook. Springer; 2015. pp. 421–451. [ Google Scholar ]
- Erdt M, Fernández A, Rensing C. Evaluating recommender systems for technology enhanced learning: A quantitative survey. IEEE Transactions on Learning Technologies. 2015; 8 (4):326–344. doi: 10.1109/TLT.2015.2438867. [ CrossRef ] [ Google Scholar ]
- Felder R. Learning and teaching styles in engineering education. Journal of Engineering Education. 1988; 78 :674–681. [ Google Scholar ]
- Fernandez-Garcia AJ, Rodriguez-Echeverria R, Preciado JC, Manzano JMC, Sanchez-Figueroa F. Creating a recommender system to support higher education students in the subject enrollment decision. IEEE Access. 2020; 8 :189069–189088. doi: 10.1109/ACCESS.2020.3031572. [ CrossRef ] [ Google Scholar ]
- Ferreira, V., Vasconcelos, G., & França, R. (2017). Mapeamento Sistemático sobre Sistemas de Recomendações Educacionais. Proceedings of the XXVIII Brazilian Symposium on Computers in Education , 253-262. 10.5753/cbie.sbie.2017.253
- Garcia-Martinez S, Hamou-Lhadj A. Educational recommender systems: A pedagogical-focused perspective. Multimedia Services in Intelligent Environments. Smart Innovation, Systems and Technologies. 2013; 25 :113–124. doi: 10.1007/978-3-319-00375-7_8. [ CrossRef ] [ Google Scholar ]
- George G, Lal AM. Review of ontology-based recommender systems in e-learning. Computers & Education. 2019; 142 :103642–103659. doi: 10.1016/j.compedu.2019.103642. [ CrossRef ] [ Google Scholar ]
- Harrathi M, Braham R. Recommenders in improving students’ engagement in large scale open learning. Procedia Computer Science. 2021; 192 :1121–1131. doi: 10.1016/j.procs.2021.08.115. [ CrossRef ] [ Google Scholar ]
- Herpich F, Nunes F, Petri G, Tarouco L. How Mobile augmented reality is applied in education? A systematic literature review. Creative Education. 2019; 10 :1589–1627. doi: 10.4236/ce.2019.107115. [ CrossRef ] [ Google Scholar ]
- Huang L, Wang C-D, Chao H-Y, Lai J-H, Yu PS. A score prediction approach for optional course recommendation via cross-user-domain collaborative filtering. IEEE Access. 2019; 7 :19550–19563. doi: 10.1109/ACCESS.2019.2897979. [ CrossRef ] [ Google Scholar ]
- Iaquinta, L., Gemmis, M. de,Lops, P., Semeraro, G., Filannino, M.& Molino, P. (2008). Introducing serendipity in a content-based recommender system. Proceedings of the Eighth International Conference on Hybrid Intelligent Systems , 168-173, 10.1109/HIS.2008.25
- Isinkaye FO, Folajimi YO, Ojokoh BA. Recommendation systems: Principles, methods and evaluation. Egyptian Informatics Journal. 2015; 16 (3):261–273. doi: 10.1016/j.eij.2015.06.005. [ CrossRef ] [ Google Scholar ]
- Ismail HM, Belkhouche B, Harous S. Framework for personalized content recommendations to support informal learning in massively diverse information Wikis. IEEE Access. 2019; 7 :172752–172773. doi: 10.1109/ACCESS.2019.2956284. [ CrossRef ] [ Google Scholar ]
- Khan KS, Kunz R, Kleijnen J, Antes G. Five steps to conducting a systematic review. Journal of the Royal Society of Medicine. 2003; 96 (3):118–121. doi: 10.1258/jrsm.96.3.118. [ PMC free article ] [ PubMed ] [ CrossRef ] [ Google Scholar ]
- Khanal SS, Prasad PWC, Alsadoon A, Maag A. A systematic review: Machine learning based recommendation systems for e-learning. Education and Information Technologies. 2019; 25 (4):2635–2664. doi: 10.1007/s10639-019-10063-9. [ CrossRef ] [ Google Scholar ]
- Khusro S, Ali Z, Ullah I. Recommender Systems: Issues, Challenges, and Research Opportunities. In: Kim K, Joukov N, editors. Lecture Notes in Electrical Engineering. Springer; 2016. pp. 1179–1189. [ Google Scholar ]
- Kitchenham, B. A., & Charters, S. (2007). Guidelines for performing Systematic Literature Reviews in Software Engineering. Technical Report EBSE 2007–001 . Keele University and Durham University Joint Report. https://www.elsevier.com/data/promis_misc/525444systematicreviewsguide.pdf .
- Kitchenham B, Pearl Brereton O, Budgen D, Turner M, Bailey J, Linkman S. Systematic literature reviews in software engineering – A systematic literature review. Information and Software Technology. 2009; 51 (1):7–15. doi: 10.1016/j.infsof.2008.09.009. [ CrossRef ] [ Google Scholar ]
- Klašnja-Milićević A, Ivanović M, Nanopoulos A. Recommender systems in e-learning environments: A survey of the state-of-the-art and possible extensions. Artificial Intelligence Review. 2015; 44 (4):571–604. doi: 10.1007/s10462-015-9440-z. [ CrossRef ] [ Google Scholar ]
- Klašnja-Milićević A, Vesin B, Ivanović M. Social tagging strategy for enhancing e-learning experience. Computers & Education. 2018; 118 :166–181. doi: 10.1016/j.compedu.2017.12.002. [ CrossRef ] [ Google Scholar ]
- Kolb, D., Boyatzis, R., Mainemelis, C., (2001). Experiential Learning Theory: Previous Research and New Directions Perspectives on Thinking, Learning and Cognitive Styles , 227–247.
- Krahenbuhl KS. Student-centered Education and Constructivism: Challenges, Concerns, and Clarity for Teachers. The Clearing House: A Journal of Educational Strategies, Issues and Ideas. 2016; 89 (3):97–105. doi: 10.1080/00098655.2016.1191311. [ CrossRef ] [ Google Scholar ]
- Kunaver M, Požrl T. Diversity in recommender systems – A survey. Knowledge-Based Systems. 2017; 123 :154–162. doi: 10.1016/j.knosys.2017.02.009. [ CrossRef ] [ Google Scholar ]
- Manouselis N, Drachsler H, Vuorikari R, Hummel H, Koper R. Recommender systems in technology enhanced learning. In: Ricci F, Rokach L, Shapira B, Kantor P, editors. Recommender Systems Handbook. Springer; 2010. pp. 387–415. [ Google Scholar ]
- Manouselis N, Drachsler H, Verbert K, Santos OC. Recommender systems for technology enhanced learning. Springer; 2014. [ Google Scholar ]
- Manouselis, N., Drachsler, H., Verbert, K., & Duval, E. (2013). Challenges and Outlook. Recommender Systems for Learning , 63–76. 10.1007/978-1-4614-4361-2
- Maravanyika M, Dlodlo N. An adaptive framework for recommender-based learning management systems. Open Innovations Conference (OI) 2018; 2018 :203–212. doi: 10.1109/OI.2018.8535816. [ CrossRef ] [ Google Scholar ]
- Maria, S. A. A., Cazella, S. C., & Behar, P. A. (2019). Sistemas de Recomendação: conceitos e técnicas de aplicação. Recomendação Pedagógica em Educação a Distância , 19–47, Penso.
- McCombs, B. L. (2013). The Learner-Centered Model: Implications for Research Approaches. In Cornelius-White, J., Motschnig-Pitrik, R. & Lux, M. (eds), Interdisciplinary Handbook of the Person-Centered Approach , 335–352. 10.1007/ 978-1-4614-7141-7_23
- Medeiros RP, Ramalho GL, Falcao TP. A systematic literature review on teaching and learning introductory programming in higher education. IEEE Transactions on Education. 2019; 62 (2):77–90. doi: 10.1109/te.2018.2864133. [ CrossRef ] [ Google Scholar ]
- Moher D, Shamseer L, Clarke M, Ghersi D, Liberati A, Petticrew M, Shekelle P, Stewart LA, PRISMA-P Group Preferred reporting items for systematic review and meta-analysis protocols (PRISMA-P) 2015 statement. Systematic Reviews. 2015; 4 (1):1. doi: 10.1186/2046-4053-4-1. [ PMC free article ] [ PubMed ] [ CrossRef ] [ Google Scholar ]
- Moubayed A, Injadat M, Nassif AB, Lutfiyya H, Shami A. E-Learning: Challenges and research opportunities using machine learning & data analytics. IEEE Access. 2018; 6 :39117–39138. doi: 10.1109/access.2018.2851790. [ CrossRef ] [ Google Scholar ]
- Nabizadeh AH, Gonçalves D, Gama S, Jorge J, Rafsanjani HN. Adaptive learning path recommender approach using auxiliary learning objects. Computers & Education. 2020; 147 :103777–103793. doi: 10.1016/j.compedu.2019.103777. [ CrossRef ] [ Google Scholar ]
- Nafea SM, Siewe F, He Y. On Recommendation of learning objects using Felder-Silverman learning style model. IEEE Access. 2019; 7 :163034–163048. doi: 10.1109/ACCESS.2019.2935417. [ CrossRef ] [ Google Scholar ]
- Nascimento PD, Barreto R, Primo T, Gusmão T, Oliveira E. Recomendação de Objetos de Aprendizagem baseada em Modelos de Estilos de Aprendizagem: Uma Revisão Sistemática da Literatura. Proceedings of XXVIII Brazilian Symposium on Computers in Education- SBIE. 2017; 2017 :213–222. doi: 10.5753/cbie.sbie.2017.213. [ CrossRef ] [ Google Scholar ]
- Nguyen QH, Ly H-B, Ho LS, Al-Ansari N, Le HV, Tran VQ, Prakash I, Pham BT. Influence of data splitting on performance of machine learning models in prediction of shear strength of soil. Mathematical Problems in Engineering. 2021; 2021 :1–15. doi: 10.1155/2021/4832864. [ CrossRef ] [ Google Scholar ]
- Nichols, D. M. (1998). Implicit rating and filtering. Proceedings of the Fifth Delos Workshop: Filtering and Collaborative Filtering , 31–36.
- Okoye, I., Maull, K., Foster, J., & Sumner, T. (2012). Educational recommendation in an informal intentional learning system. Educational Recommender Systems and Technologies , 1–23. 10.4018/978-1-61350-489-5.ch001
- Pai M, McCulloch M, Gorman JD, Pai N, Enanoria W, Kennedy G, Tharyan P, Colford JM., Jr Systematic reviews and meta-analyses: An illustrated, step-by-step guide. The National Medical Journal of India. 2004; 17 (2):86–95. [ PubMed ] [ Google Scholar ]
- Petri G, Gresse von Wangenheim C. How games for computing education are evaluated? A systematic literature review. Computers & Education. 2017; 107 :68–90. doi: 10.1016/j.compedu.2017.01.00. [ CrossRef ] [ Google Scholar ]
- Petticrew M, Roberts H. Systematic reviews in the social sciences a practical guide. Blackwell Publishing. 2006 doi: 10.1002/9780470754887. [ CrossRef ] [ Google Scholar ]
- Pinho PCR, Barwaldt R, Espindola D, Torres M, Pias M, Topin L, Borba A, Oliveira M. Developments in educational recommendation systems: a systematic review. Proceedings of 2019 IEEE Frontiers in Education Conference (FIE) 2019 doi: 10.1109/FIE43999.2019.9028466. [ CrossRef ] [ Google Scholar ]
- Pöntinen S, Dillon P, Väisänen P. Student teachers’ discourse about digital technologies and transitions between formal and informal learning contexts. Education and Information Technologies. 2017; 22 (1):317–335. doi: 10.1007/s10639-015-9450-0. [ CrossRef ] [ Google Scholar ]
- Pu, P., Chen, L., & Hu, R. (2011). A user-centric evaluation framework for recommender systems. Proceedings of the fifth ACM conference on Recommender systems , 157–164. 10.1145/2043932.2043962
- Rahman MM, Abdullah NA. A personalized group-based recommendation approach for web search in E-Learning. IEEE Access. 2018; 6 :34166–34178. doi: 10.1109/ACCESS.2018.2850376. [ CrossRef ] [ Google Scholar ]
- Ricci, F., Rokach, L., & Shapira, B. (2015). Recommender Systems: Introduction and Challenges. I Ricci, F., Rokach, L., Shapira, B. (eds), Recommender Systems Handbook , 1–34. 10.1007/978-1-4899-7637-6_1
- Rivera, A. C., Tapia-Leon, M., & Lujan-Mora, S. (2018). Recommendation Systems in Education: A Systematic Mapping Study. Proceedings of the International Conference on Information Technology & Systems (ICITS 2018) , 937–947. 10.1007/978-3-319-73450-7_89
- Salazar C, Aguilar J, Monsalve-Pulido J, Montoya E. Affective recommender systems in the educational field. A systematic literature review. Computer Science Review. 2021; 40 :100377. doi: 10.1016/j.cosrev.2021.100377. [ CrossRef ] [ Google Scholar ]
- Santos IM, Ali N. Exploring the uses of mobile phones to support informal learning. Education and Information Technologies. 2012; 17 (2):187–203. doi: 10.1007/s10639-011-9151-2. [ CrossRef ] [ Google Scholar ]
- Sergis S, Sampson DG. Learning object recommendations for teachers based on elicited ICT competence profiles. IEEE Transactions on Learning Technologies. 2016; 9 (1):67–80. doi: 10.1109/TLT.2015.2434824. [ CrossRef ] [ Google Scholar ]
- Shani G, Gunawardana A. Evaluating recommendation systems. In: Ricci F, Rokach L, Shapira B, Kantor P, editors. Recommender Systems Handbook. Springer; 2010. pp. 257–297. [ Google Scholar ]
- Tahereh, M., Maryam, T. M., Mahdiyeh, M., & Mahmood, K. (2013). Multi dimensional framework for qualitative evaluation in e-learning. 4th International Conference on e-Learning and e-Teaching (ICELET 2013), 69–75. 10.1109/icelet.2013.6681648
- Tarus JK, Niu Z, Yousif A. A hybrid knowledge-based recommender system for e-learning based on ontology and sequential pattern mining. Future Generation Computer Systems. 2017; 72 :37–48. doi: 10.1016/j.future.2017.02.049. [ CrossRef ] [ Google Scholar ]
- Tarus JK, Niu Z, Mustafa G. Knowledge-based recommendation: A review of ontology-based recommender systems for e-learning. Artificial Intelligence Review. 2018; 50 (1):21–48. doi: 10.1007/s10462-017-9539-5. [ CrossRef ] [ Google Scholar ]
- Verbert K, Manouselis N, Ochoa X, Wolpers M, Drachsler H, Bosnic I, Duval E. Context-aware recommender systems for learning: A survey and future challenges. IEEE Transactions on Learning Technologies. 2012; 5 (4):318–335. doi: 10.1109/TLT.2012.11. [ CrossRef ] [ Google Scholar ]
- Verbert, K., Drachsler, H., Manouselis, N., Wolpers, M., Vuorikari, R., & Duval, E. (2011). Dataset-Driven Research for Improving Recommender Systems for Learning. Proceedings of the 1st International Conference on Learning Analytics and Knowledge , 44–53. 10.1145/2090116.2090122
- Wan S, Niu Z. A learner oriented learning recommendation approach based on mixed concept mapping and immune algorithm. Knowledge-Based Systems. 2016; 103 :28–40. doi: 10.1016/j.knosys.2016.03.022. [ CrossRef ] [ Google Scholar ]
- Wan S, Niu Z. An e-learning recommendation approach based on the self-organization of learning resource. Knowledge-Based Systems. 2018; 160 :71–87. doi: 10.1016/j.knosys.2018.06.014. [ CrossRef ] [ Google Scholar ]
- Wan S, Niu Z. A hybrid E-Learning recommendation approach based on learners’ influence propagation. IEEE Transactions on Knowledge and Data Engineering. 2020; 32 (5):827–840. doi: 10.1109/TKDE.2019.2895033. [ CrossRef ] [ Google Scholar ]
- Watkins KE, Marsick VJ. Informal and incidental learning in the time of COVID-19. Advances in Developing Human Resources. 2020; 23 (1):88–96. doi: 10.1177/1523422320973656. [ CrossRef ] [ Google Scholar ]
- Wu D, Lu J, Zhang G. A Fuzzy Tree Matching-based personalized E-Learning recommender system. IEEE Transactions on Fuzzy Systems. 2015; 23 (6):2412–2426. doi: 10.1109/TFUZZ.2015.2426201. [ CrossRef ] [ Google Scholar ]
- Wu Z, Li M, Tang Y, Liang Q. Exercise recommendation based on knowledge concept prediction. Knowledge-Based Systems. 2020; 210 :106481–106492. doi: 10.1016/j.knosys.2020.106481. [ CrossRef ] [ Google Scholar ]
- Yanes N, Mostafa AM, Ezz M, Almuayqil SN. A machine learning-based recommender system for improving students learning experiences. IEEE Access. 2020; 8 :201218–201235. doi: 10.1109/ACCESS.2020.3036336. [ CrossRef ] [ Google Scholar ]
- Zapata A, Menéndez VH, Prieto ME, Romero C. Evaluation and selection of group recommendation strategies for collaborative searching of learning objects. International Journal of Human-Computer Studies. 2015; 76 :22–39. doi: 10.1016/j.ijhcs.2014.12.002. [ CrossRef ] [ Google Scholar ]
- Zhang S, Yao L, Sun A, Tay Y. Deep learning based recommender system. ACM Computing Surveys. 2020; 52 (1):1–38. doi: 10.1145/3285029. [ CrossRef ] [ Google Scholar ]
- Zhong J, Xie H, Wang FL. The research trends in recommender systems for e-learning: A systematic review of SSCI journal articles from 2014 to 2018. Asian Association of Open Universities Journal. 2019; 14 (1):12–27. doi: 10.1108/AAOUJ-03-2019-0015. [ CrossRef ] [ Google Scholar ]
- Open access
- Published: 14 May 2024
Comparative analysis of GoPro and digital cameras in head and neck flap harvesting surgery video documentation: an innovative and efficient method for surgical education
- Xin-Yue Huang 1 na1 ,
- Zhe Shao 1 , 2 na1 ,
- Nian-Nian Zhong 1 ,
- Yuan-Hao Wen 1 ,
- Tian-Fu Wu 1 , 2 ,
- Bing Liu 1 , 2 ,
- Si-Rui Ma 1 , 2 &
- Lin-Lin Bu 1 , 2
BMC Medical Education volume 24 , Article number: 531 ( 2024 ) Cite this article
Metrics details
An urgent need exists for innovative surgical video recording techniques in head and neck reconstructive surgeries, particularly in low- and middle-income countries where a surge in surgical procedures necessitates more skilled surgeons. This demand, significantly intensified by the COVID-19 pandemic, highlights the critical role of surgical videos in medical education. We aimed to identify a straightforward, high-quality approach to recording surgical videos at a low economic cost in the operating room, thereby contributing to enhanced patient care.
The recording was comprised of six head and neck flap harvesting surgeries using GoPro or two types of digital cameras. Data were extracted from the recorded videos and their subsequent editing process. Some of the participants were subsequently interviewed.
Both cameras, set at 4 K resolution and 30 frames per second (fps), produced satisfactory results. The GoPro, worn on the surgeon’s head, moves in sync with the surgeon, offering a unique first-person perspective of the operation without needing an additional assistant. Though cost-effective and efficient, it lacks a zoom feature essential for close-up views. In contrast, while requiring occasional repositioning, the digital camera captures finer anatomical details due to its superior image quality and zoom capabilities.
Merging these two systems could significantly advance the field of surgical video recording. This innovation holds promise for enhancing technical communication and bolstering video-based medical education, potentially addressing the global shortage of specialized surgeons.
1) The GoPro camera offers stable vision and does not require an assistant for operation.
2) A digital camera provides images of higher quality and better anatomical detail.
3) Combining the two could result in a highly efficient and innovative method.
4) Trainees provided positive feedback on the educational impact of the videos.
Peer Review reports
Introduction
Innovation often occurs when knowledge from different disciplines converges and new ideas emerge or merge to foster progress [ 1 ]. Technological advancements have introduced innovations and tools that have entered head and neck surgical practice, ranging from the operating microscope and robotic, imaging-based navigation to computer-assisted design and perfusion monitoring technologies, providing precision care and better patient prognoses [ 1 , 2 , 3 , 4 ]. The combination of video recording and streaming with head and neck reconstructive surgery enables recording the surgeon’s view, allowing others to see exactly what the surgeon observes and does. Video recording technology can also be beneficial in various areas, such as technical communication, research, case data backup, and clinical education. As the saying goes, “A picture is worth a thousand words,” but video holds more convincing power than pictures alone. In the field of head and neck surgery, medical students and junior surgical trainees often do not acquire the full range of surgical skills during their operating room clerkships [ 5 ]. Simultaneously, the global shortage and uneven distribution of the surgical workforce are gaining recognition, with low- and middle-income countries (LMICs) in dire need of skilled surgeons [ 6 ]. There is significant demand for surgical videos in surgical education and surgeon training, especially as COVID-19 ravaged the world, affecting many residents’ clinical practice schedules to varying degrees [ 7 , 8 ]. Consequently, teaching surgical skills has become more challenging.
Digital video capture during surgical procedures is an essential technology in modern-day surgical education [ 9 , 10 , 11 , 12 ]. The advent of fifth-generation mobile technology (5G) has facilitated the distribution of video formats, making it as effortless as sharing text and picture formats in the past, no longer constrained by mobile devices or network bandwidth. Recording surgeries in video format is employed across various domains, such as open surgery, microsurgery, laryngoscopy, and laparoscopy, yielding excellent outcomes regarding video quality and educational purposes [ 13 , 14 , 15 , 16 , 17 ]. Its benefits include 1) assisting medical students in their training, 2) enhancing comprehension of the surgical procedure and the patient’s clinical condition, 3) visualizing crucial routine manual operations, such as flap harvesting, 4) aiding in the preservation of legal evidence, and 5) providing a more precise anatomical description of body regions [ 18 ]. These critical aspects are challenging to convey effectively through descriptions, even with the support of photographs and other media.
The high construction costs associated with a dedicated medical recording system in the operating room can be prohibitive for some hospitals and medical institutions in LMICs and developed countries. Fortunately, due to the rapid advancement of technological innovation in recent years, personal digital video technologies have become more affordable and offer good image quality. Previous studies have also demonstrated that these technologies when applied to surgical video recording, can yield positive results [ 19 , 20 ]. However, few studies have compared different types of camera systems for surgical recordings.
Our study compared the GoPro (Hero 8 Black), a low-cost commercially available action camera, with two higher-priced commercial digital cameras (Canon EOS R5 and EOS 850D). We preliminarily explored other types of surgical video recording (Figure S1), as flap harvesting is a crucial operation in head and neck reconstructive surgery with significant teaching values. Our research focused on comparing the video recording outcomes of these two camera systems during flap harvesting procedures. This study aimed to identify a straightforward, high-quality approach to recording surgical videos at a low economic cost in the operating room, thereby contributing to enhanced patient care.
Materials and methods
The recordings were taken in the Department of Oral & Maxillofacial—Head Neck Oncology at the Hospital of Stomatology, Wuhan University, from November to December 2021. A total of six operations were prospectively recorded. All patients signed informed consent forms before surgery, and the recordings did not involve any parts of the patients’ bodies outside the operative areas.
GoPro is a brand of action camera that can be attached to the body with simple accessories, enabling hands-free recording and first-person perspectives, especially in extreme sports. The GoPro HERO 8 Black (GoPro Inc, San Mateo, CA), used in this study, is currently a widely recognized product. This camera is exceptionally compact and portable, measuring 62*33.7*44.6 mm and weighing 450 g. The GoPro 8 supports stabilized 4 K video recording at 30 or 60 frames per second (fps) and slow-motion 1080P video at 240 fps. It is equipped with the HyperSmooth system, which stabilizes the video image without the need for external stabilizers, even when the surgeon wearing the device is moving. It can also connect to a smart device via a wireless network during filming to monitor the shot or even broadcast live using the GoPro Quik app. The fixed focus setting on this device maintains consistent focus, regardless of whether the subject gets closer or moves further away within a certain distance.
Generally, the term “digital camera” may also refer to the camera systems integrated into smartphones (such as an iPhone). However, surgical videos require precise documentation of operations on delicate anatomical structures, and our previous pilot study found that the images captured by smartphones (iPhone X) did not meet the requirements for teaching or technical communication. Therefore, the “digital camera” referenced in this article pertains specifically to professional digital cameras. We utilized two relatively recent models on the market, the EOS R5, Canon’s flagship product, and the EOS 850D, its entry-level counterpart.
A total of six operations were prospectively studied, involving three surgeons, seven circulating nurses, and ten surgical residents.
The surgeon wore the GoPro 8 camera attached to a unique headband (Fig. 1 ), with no additional loupes or head-mounted lighting systems to physically interfere with the camera. An iPad, connected to the GoPro and equipped with the GoPro Quik app, served as a viewfinder and remote control for recording the six operations.
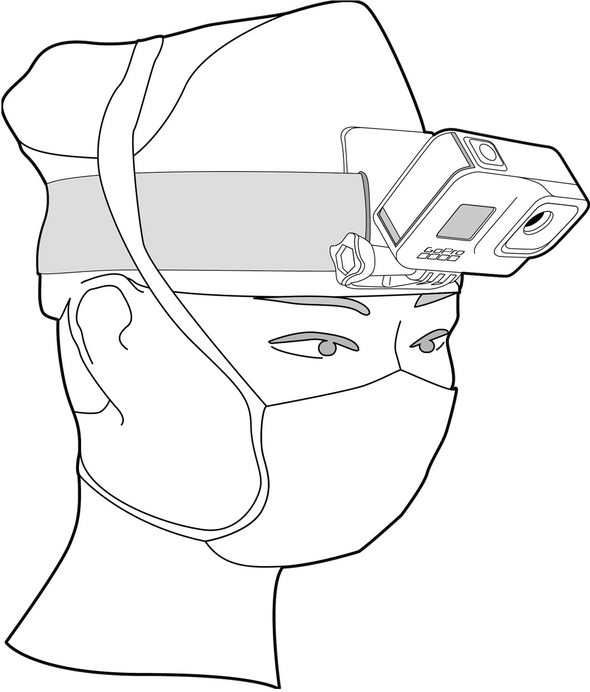
The surgeon with the head-mounted camera in place to record the surgery
The digital cameras were mounted on an external tripod for recording and were set to manual mode with manual focus. The first three recordings observed that the surgical team members occasionally obscured the surgical area. Therefore, the tripod setup was modified in the subsequent three recordings, drawing on previous studies’ methods to attain a better field of view (FOV) (Fig. 2 ) [ 21 ]. The sixth surgery was recorded using an EOS 850D, while the others were documented with an EOS R5.
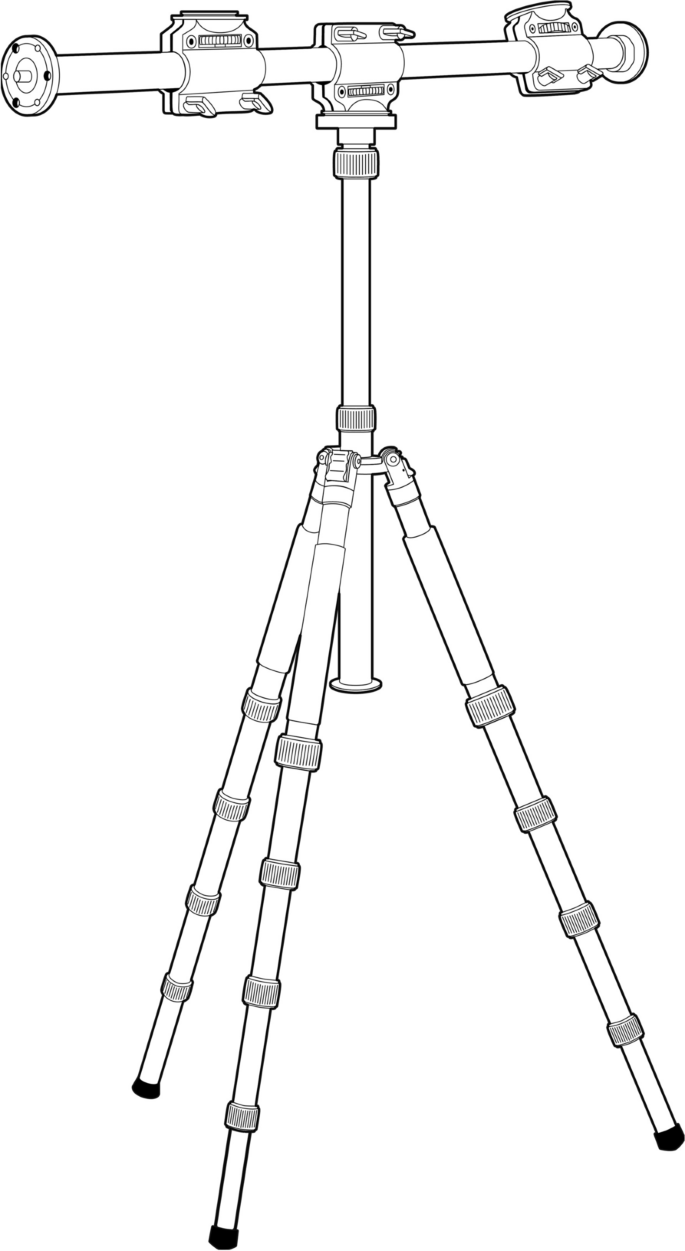
Post-assembly view of the modified tripod
Characterization of videos
Data for this study were extracted from the recorded videos and their subsequent editing process. Selected variables were entered into a Microsoft Excel database. The evaluated variables included: 1) the total recording time for each device during all surgeries; 2) the duration of all surgical procedures; 3) the duration of unavailable video (including obscured surgical areas, inaccurate focus, and overexposure) and their percentage of the entire surgical procedure; and 4) the file size of the original videos.
Educational impact analysis
To assess the quality of the recorded surgical videos and their applicability in teaching, we designed a questionnaire to gather opinions from three surgeons and nine resident physicians (students) on the edited videos. The surgeons’ questionnaire primarily assessed whether the videos clearly conveyed the surgeon’s operational concepts and specific details. Trainees were queried whether they could clearly view the procedures and derive educational benefits from it.
To further analyze the educational impact of the surgical videos, we established an Expert Review Panel (ERP) comprising five experts with over ten years of experience in clinical surgery and medical student education. We also created an assessment table for evaluating surgical education video (Table 1 ). The ERP reviewed six edited surgical videos and evaluated their instructional quality, clarity, stability, and effectiveness in conveying surgical techniques. Subsequently, the table was completed to categorize the overall quality of each video.
It took approximately 10 min to prepare the video equipment before surgery, including setting up the tripod, determining the recording settings, and adjusting the camera’s position. All surgeons reported that the head-mounted recording device did not interfere with the operation. The operations involved different types of flap harvesting, including ilium flap harvesting ( n = 3), fibula flap harvesting ( n = 1), anterolateral thigh flap harvesting ( n = 1), and forearm flap harvesting ( n = 1). The average duration of the operations was one and a half hours. Six surgical procedures were recorded simultaneously using both the GoPro and digital cameras. The characteristics of each surgical video are shown in Table 2 . The technical details of the two types of cameras used in the study (GoPro HERO 8 Black, EOS R5, and EOS 850D) are summarized in Table 3 . A video of the sample can be found in the Supplementary Video.
Video settings
While filming surgeries, we consulted previous studies for camera settings. Graves et al. conducted research using a GoPro camera in the operating room [ 20 ]. They selected an earlier type—the GoPro HERO 3 + Black—and concluded that with a narrow FOV, automatic white balance, 1080P resolution, and 48 fps, one could achieve high-quality, low-cost video recordings of surgical procedures. In our study, we initially tried a 1080P resolution with a narrow field and obtained relatively good results (Fig. 3 A). However, a 1080P resolution HD video consists of two million pixels (1920 × 1080), whereas a 4 K (Ultra HD) video comprises over eight million pixels (3840 × 2160). Thus, 4 K video produces a sharper image with four times the resolution of 1080P. Given that the GoPro does not support narrow-field shooting at 4 K resolution, we discovered that setting it to a “linear” FOV with 4 K resolution provided more precise and crisper imagery (Fig. 3 B). In most of our recordings, we used 4 K resolution and a frame rate of 30 fps for both the GoPro and digital cameras.
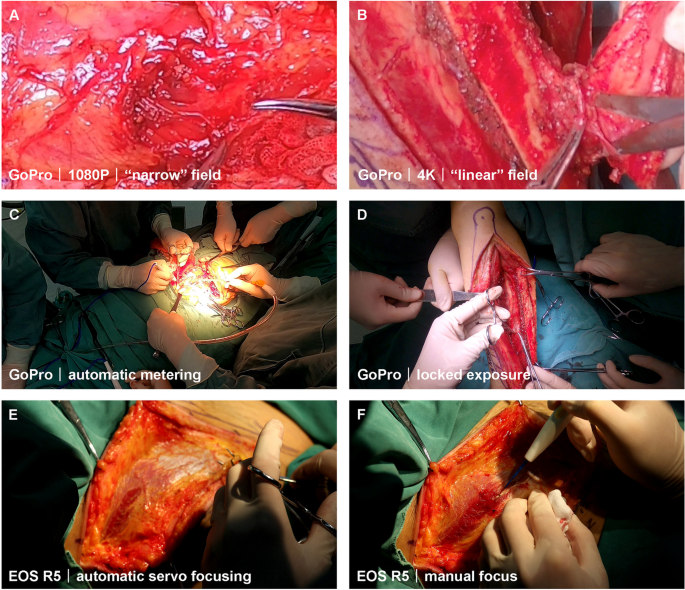
Image quality comparison of video screenshots with the magnification of 500% obtained from ( A ) 1080P narrow field and ( B ) 4K linear field. C When set to automatic metering, the intraoperative area was overexposed, and ( D ) the area was normally exposed after locked exposure. E In automatic servo focusing mode, the focal plane is not in the area but in the operator’s hand. F Manual focus keeps the focus on the area, even if the operator’s hand is covered
Light metering involves determining the necessary exposure based on environmental conditions, which can be manually adjusted by the photographer with different exposure settings or automatically by the camera’s program. The light environment of the operating room is complex, as the surgical field illuminated by a shadowless lamp is typically brighter than the surrounding area. When recording the surgical area with a digital camera that permits manual operation, it is recommended to use a smaller aperture (an opening that allows light to reach a lens) to reduce light intake and increase the depth of field (DOF), which is the range within which objects appear sharp. Although the GoPro was set to automatic metering mode due to the difficulty of manual operation, its FOV shifted with the surgeon’s head movements. As bright lights continually focused on the surgical area, rapid changes in the FOV easily caused overexposure in the operating area (Fig. 3 C). This issue was later addressed by locking the exposure before recording (Fig. 3 D).
The first recorded video revealed that the digital camera’s automatic servo focusing caused instability in the focal plane within the operational area due to various instruments and the surgeon’s hands in the surgical field (Fig. 3 E). This issue was addressed by manually focusing and locking the focal plane before recording (Fig. 3 F). However, when the position changed during the procedure, an assistant without surgical hand disinfection was required to adjust the camera promptly.
Quality of videos
The image quality of videos from three cameras was sufficient for depicting static and moving objects. However, the operating room is a unique environment where multiple factors influence the cameras’ effectiveness. These factors include the distance from the operating area, obstruction by surgical team members [ 22 ], the lens’s FOV, light overexposure, and reflection from metal instruments.
To more clearly compare the video quality of the three devices across six different head and neck reconstructive procedures, we extracted images from the video files of all devices and assessed their clarity at magnifications of 100% and 300%. Fig. 4 A-C show 100% images alongside detailed 300% magnified images captured from videos recorded by the GoPro8, EOS 850D, and EOS R5, respectively. All three devices provided precise and reliable output under various circumstances and lighting conditions.
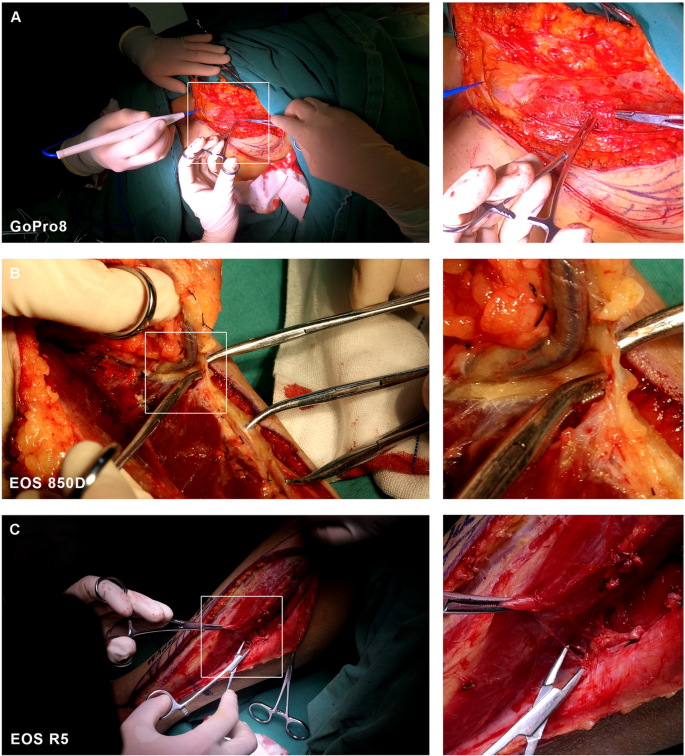
A 100% image from uncompressed file video of GoPro8, compared with magnified 300% video image in detail. B 100% image from uncompressed file video of EOS 850D, compared with magnified 300% video image in detail. C 100% image from uncompressed file video of EOS R5, compared with magnified 300% video image in detail
Positioning in the operative room
Digital cameras capture high-definition images during surgery with accurate focus. However, there were instances when the lens was obscured by the bodies of surgical team members or instruments, missing critical moments. As shown in Table 2 , videos recorded with a digital camera placed on an unmodified tripod had a higher rate of unavailable video duration, primarily due to obscuration. The tripod was modified to position the camera’s FOV more perpendicular to the surgical area. Consequently, the average duration of unusable video recorded by the digital camera using the modified tripod was 11.3%, a significant decrease from the 37.55% average without modification.
Figure 5 depicts the positioning of the modified tripod, head-mounted camera, and surgical field used for the recording.
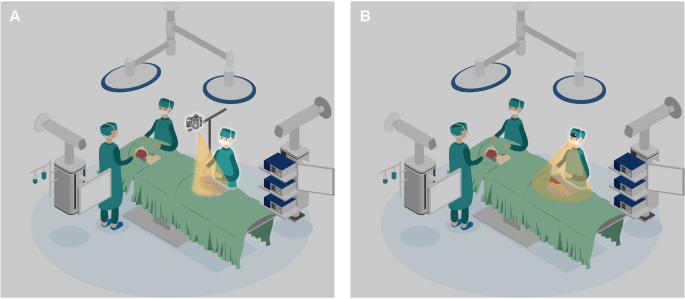
The position used for the recording with the surgical field and surgeon. A Ilium flap harvesting with digital camera. B Ilium flap harvesting with GoPro
Field of view
In the recordings of Anterolateral thigh flap harvesting, Ilium flap harvesting 2, and Fibula flap harvesting surgeries, the use of the GoPro resulted in a lower duration of unavailable video (1.9%, 2.5%, and 2.0%, respectively) compared to digital cameras (29.5%, 14.7%, and 5.5%), even with the tripod modified for the latter two recordings. This outcome is primarily because the surgeon’s hand often blocked the digital camera, positioned for a third-person perspective. In contrast, the GoPro, attached to the surgeon’s head, offered a viewpoint closer to the surgeon’s own eyes, thereby capturing a better visual field. The surgeon’s perspective is arguably the most advantageous, as corroborated by many previous studies that placed the camera on the surgeon’s forehead for procedural recording [ 13 , 20 , 23 ]. Rafael et al. reported that the head camera position was well-received by volunteers [ 24 ]. Though we obtained valuable images this way, there were limitations. The angle from the eye to the target point varies with the surgical techniques. Digital cameras can easily shift focus by adjusting the tripod’s position and angle to maintain the view of the surgical area; however, the GoPro’s FOV and focus are fixed upon installation, allowing horizontal adjustments, occasionally resulting in the surgical area going out of frame. Nevertheless, the GoPro’s wide FOV in both “wide” and “linear” modes generally ensures that the area remains within the shot without continuous monitoring.
Based on the images extracted from the videos, the digital camera can achieve a more detailed view of the surgical area with its zoom capabilities compared to the GoPro’s wider FOV. Although the GoPro’s images reveal clear anatomical structures upon magnification, they are not as sharp as those from the digital camera. This limitation, however, had unexpected benefits, as it could record the surgeon’s hand movements between the patient’s tissues and the instruments, providing insights into surgical hand positioning and instrument ergonomics that are crucial for training but often overlooked [ 23 ]. Experienced surgeons efficiently organize their workspace, holding instruments currently in use while preparing others for subsequent steps. On-site trainees, focusing primarily on the operative site, may miss these subtle ergonomic maneuvers. When used in education, surgical recordings simultaneously displaying the operative site and hand positioning can offer learners vital insights previously unnoticed [ 25 ].
Connectivity
All three devices possess the capability for wireless connectivity via Wi-Fi or Bluetooth systems. Video captured by these devices can be streamed in real-time to nearby mobile devices or monitors and can even be broadcast online. This feature forms the foundation for remote tele-proctoring and education purposes in surgery, a method proven to be innovative for enhancing surgical education in high-resource settings [ 26 ]. Fig. 6 illustrates the connectivity scheme, which includes a wireless link between the cameras and mobile devices through Wi-Fi or Bluetooth, facilitating further dissemination by these devices.
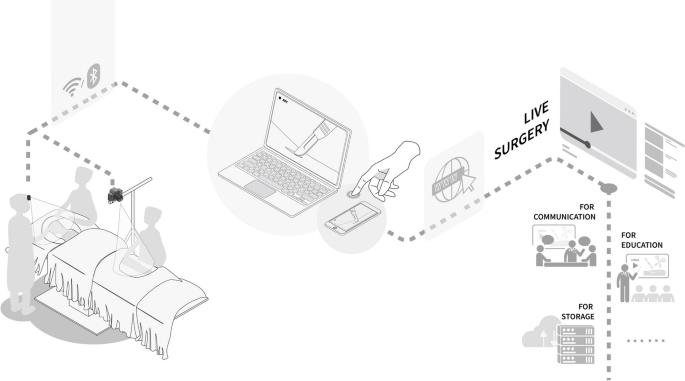
The camera can be connected to a mobile phone or laptop via Wi-Fi or Bluetooth or even broadcast live via the Internet for more purposes
In addition, the GoPro device itself comes equipped with a Livestream function in full 1080P HD mode. However, the video quality of the Webcast is not as high as the recordings due to limitations imposed by wireless connection speeds and bandwidth. The "choppy" nature of the video presentation during streaming can be mitigated by using a direct cable for live broadcasts, allowing direct streaming onto a monitor for local presentation or broader live broadcasts, and offering a quality superior to Wi-Fi or Bluetooth options. The downside is the cumbersome nature of the required cables.
Editing of video
Benefiting from the high resolution of 4K video, high definition is maintained even after the original video clip is magnified. Structural details are well-preserved, and the clarity of the operation remains evident in the magnified version, which can be further saved or shared. GoPro Quik, an application developed by the GoPro company, facilitates customized video editing. It can be used to edit original clips shot by the GoPro camera, re-adjust the field of interest, and conveniently export the video in the appropriate format. High-resolution video has its pros and cons. The extensive data involved makes storing and editing raw video files challenging. Future technologies should enable surgeons to ensure real-time recording of the area of interest, allowing for more manageable data acquisition without the need for zooming or cropping post-capture.
Videos for education
The results of the questionnaire were as follows. In the surgeons’ group, 100% ( n = 3) confirmed that the videos well represented the details of their operations. In the students’ group, 66.7% of respondents ( n = 6) rated the image quality with GoPro as excellent, and 33.3% ( n = 3) found it fine, while for the digital camera, 88.9% of respondents ( n = 8) rated it as excellent and 11.1% ( n = 1) as fine (Fig. 7 ). All respondents ( n = 9) positively affirmed that they could learn professional skills from the videos. In the evaluation conducted by the Expert Review Panel, of the six videos, four were considered suitable for clinical teaching applications, one was also suitable but required a better replacement, and one was deemed unsuitable for clinical teaching applications. However, these results must be interpreted with caution due to the small sample size.
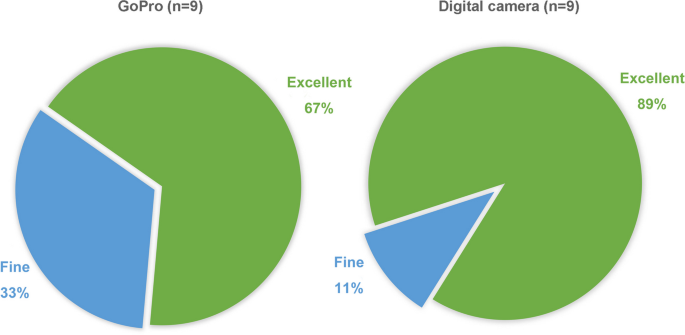
Trainees reported satisfaction degrees from surgical videos, according to whether they can see the procedure clearly and learn from it—comparison of GoPro versus digital camera
With the increasing demand for technical communication, medical teaching, surgical procedure recording, and so on, surgical video has become a popular multimedia mode. It is a powerful medium that can enhance patient safety in several ways: education, real-time consultation, research, process improvement, and workflow coordination [ 27 ]. Operation videos can be transmitted through the internet in real-time, providing a platform for communication and cooperation between hospitals. Experienced surgeons can assess trainees’ surgical competency in an unbiased fashion through the trainees’ intraoperative video [ 28 ]. Experienced individual surgeons hope to share their professional knowledge and skills through surgical videos and achieve the purpose of self-publicity. Regarding privacy protection, Turnbull et al. emphasized that video documentation has significant ethical and legal considerations as it contains personal information and infringes on patients’ privacy [ 29 ]. The patient’s privacy should be carefully considered to avoid potential ethical and legal conflicts brought about by filming operations.
Pros and cons of two camera systems
The introduction of video technology into surgical procedures is becoming more common, and high-resolution camera technology has been integrated into surgical instrumentation for laparoscopic and minimally invasive procedures [ 30 ]. Although technology continuously evolves, leading to the adoption of many new technologies in intraoperative video recording, there are still limitations in devices for capturing open surgery. Due to economic conditions and space constraints, operating rooms are not routinely equipped with video recording equipment, making personal recording equipment a more viable solution. This study compared two technologies (GoPro and digital camera) used for intraoperative video capture in open surgeries and summarized their advantages and disadvantages (Table 4 ).
GoPro cameras are designed for extreme sports, featuring high resolution, high frame rates, small image sensors, and a lack of complete manual control. They are light and portable enough to be worn on a surgeon’s head, providing an image that approximates the natural field of vision without hindering the operation. Simultaneously, their built-in stabilizer function ensures the output image remains stable and visible. Being waterproof, they can be soaked in povidone-iodine for disinfection, facilitating hand-held shooting [ 31 ]. Existing studies confirm that this disinfection method does not compromise asepsis [ 32 ]. The built-in Wi-Fi and Bluetooth allow for remote monitoring and real-time transmission of intraoperative video. The affordability of GoPro enables doctors wanting to record surgeries to do so cost-effectively, making them accessible to surgeons from LMICs. However, the downsides are clear: users in the operating room are more likely to obtain a narrower FOV aimed at the surgical area, but the GoPro, as an action camera, is designed to capture as comprehensive a panoramic view as possible. Due to the absence of manual controls, it does not adapt well to frequent brightness changes caused by bright overhead operating room lights. Additionally, the battery capacity of the GoPro lasts approximately 60 min and may shut down if its body temperature reaches an upper limit during prolonged sessions. For extened recording times, spare batteries are necessary, and consideration of the device temperature is essential.
Digital cameras, due to their optimal optical performance and excellent zoom capabilities, can capture specific areas of interest in high quality. They are typically more durable and are generally equipped with larger image sensors, better adapting to unfavorable lighting conditions. Their robust maneuverability makes them suitable for the complex operating room environment. Digital cameras were not widely used for surgical video recording due to their high cost. However, this study shows that even inexpensive digital cameras, such as the EOS 850D, can produce adequate surgical videos. The picture quality is not significantly different from the much pricier EOS R5 when using the same 4 K 30 fps model. Of course, more expensive cameras like the EOS R5, which supports 8 K quality video, allow for a better representation of delicate anatomy.
Nevertheless, these cameras’ drawback is that they always require supports like tripods or rocker arms for steady recording. The positioning, height, and relationship with the surgical team determine the final video quality. Furthermore, an additional assistant is needed to adjust camera positions and video settings to maintain the appropriate shooting angle during the procedure. This camera operator might need to direct the surgeon to stop and start at different points throughout the surgery, potentially interfering with the surgical team. The risk of breaking sterility should also be considered when introducing an extra individual into the operating room. This cumbersome and time-consuming shooting method does not lend itself to daily, routine intraoperative videotaping.
Using either a GoPro or a digital camera is a commendable choice. According to our research, the GoPro is a highly efficient option that is better suited for personal recording and can be operated easily without an assistant. Digital cameras, though requiring additional assistance, deliver higher output quality. If the two are innovatively combined, images from different fields of vision can be captured to produce rich, comprehensive, and high-quality videos.
Application of surgical video in education and other aspects
Surgical video holds broad application prospects in medical teaching, technical communication, patient safety, workflow coordination, case data backup, research, real-time consulting, and skill improvement. With the advancement of communication facilities, real-time video recording during surgery presents extensive development prospects akin to digital twin technology [ 33 , 34 ]. Mentoring through this medium can enhance quality and patient safety throughout a medical student’s career. Future developments may involve coaching sessions or honing non-technical skills, such as optimizing teamwork in the operating room to elevate patient care.
Medical students’ journey to becoming surgeons critically requires specific technical feedback while developing foundational skills during their internships. Despite the importance of targeted feedback, medical students often endure inconsistent, fragmented, and stressful experiences in the operating room [ 5 ]. Compounding these challenges, a study on oral and maxillofacial surgery trainees in the United States revealed that the COVID-19 pandemic disrupted the scheduling of non-urgent and elective operations [ 35 ]. With approximately 2.28 million more skilled medical professionals needed to meet the global demand for surgical procedures [ 6 ], training a substantial cohort of future surgeons is a pressing, worldwide challenge.
In addition to traditional book learning and clinical practice, watching surgical videos can help medical students acquire technical details related to surgical operations more precisely, and some critical but fleeting points can be repeated during video playback. Video-based interventions to enhance surgical skills are gaining attention for their educational applications and related research [ 12 ]. The use of video technology in teaching is relatively common in other fields, including sports. In head and neck surgery, some advantages of utilizing high-quality surgical recordings as educational tools are as follows: 1) They provide clear, sharp images that depict fine anatomical structure; 2) Learning through videos offers a more intuitive experience, as viewing surgery footage from a first-person perspective affords residents a more immersive sensation, encouraging them to conceptualize the surgery from the surgeon’s viewpoint; 3) Video recordings of resident physicians’ operations facilitate the assessment of their skill levels, paving the way for enhanced performance; 4) Essential intraoperative findings can be documented and elucidated; 5) The zoom feature enables close-up, detailed recording of surgical procedures and anatomical nuances.
Leveraging the Wi-Fi and Bluetooth capabilities of recording devices, real-time videos can be streamed to mobile phones or laptops or even broadcast live over the internet for tele-proctoring. This emerging technology allows instructors to provide real-time guidance and technical support through audio and video interactions from various geographical locations. This method effectively circumvents the additional logistical costs, time constraints, and challenges posed by distance that are inherent when instructors physically travel to the field [ 36 ]. McCullough et al. [ 26 ] previously explored the feasibility of wearable recording technology in expanding the reach and availability of specialized surgical training in LMICs, using Mozambique as a case study. Their research suggests that this educational model connects surgeons globally and fosters advanced mentoring in regions where surgical trainees have limited opportunities.
Limitations
The findings of this study must be considered within the context of certain limitations. The research was single-centered with a limited number of surgeons involved, and only a single brand of digital camera was selected, which may lead to a lack of diversity and overlook ergonomic differences between types of surgeries and the subtle imaging details between different camera manufacturers. The assessment of the impact of video on teaching also had a small sample size, so potential biases in questionnaire feedback should be considered. Furthermore, there is a persistent need for objective and repeatable metrics to conclusively demonstrate the efficacy of camera technology in clinical education, continuous performance improvement, and quality enhancement initiatives.
Considering that the primary aim of this study was to compare and recommend a high-quality approach for recording surgical videos, future research will focus on conducting multi-centered studies with larger sample sizes and emphasis on the diversity of surgical specialties and camera brands. It is also essential to assess its application more effectively in a learning experience in surgical education, not only in head and neck surgery but also in other surgical areas. Future studies will improve the evaluation of skill levels through practical techniques and written exams, study learning curves in relation to surgical timing, analyze cost-effectiveness, and gather evaluations from the trainer’s perspective.
The field of head and neck surgery has consistently welcomed innovation, embracing the introduction of new techniques into surgical practice. There is a substantial demand and room for development in the domain of open surgical recordings. Surgical video recording serves the purpose of technical communication and accomplishes the objective of medical education through real-time connectivity, addressing the current global shortage of specialized surgeons. The two systems examined in this study, the GoPro and the digital camera, each have distinct features and advantages. The GoPro, an affordable and physician-independent solution, offers a stable and continuous view of the surgical area, though it lacks a medical-specific design and a zoom function. On the other hand, despite requiring periodic repositioning and potentially distracting the surgical team, the digital camera delivers superior visibility of anatomical details and higher image quality.
Availability of data and materials
The data supporting the findings of this study are available within the article and its supplementary materials.
Suchyta M, Mardini S. Innovations and future directions in head and neck microsurgical reconstruction. Clin Plast Surg. 2017;44(2):325–44. https://doi.org/10.1016/j.cps.2016.11.009 .
Article Google Scholar
Tarsitano A, Battaglia S, Ciocca L, Scotti R, Cipriani R, Marchetti C. Surgical reconstruction of maxillary defects using a computer-assisted design/computer-assisted manufacturing-produced titanium mesh supporting a free flap. J Craniomaxillofac Surg. 2016;44(9):1320–6. https://doi.org/10.1016/j.jcms.2016.07.013 .
Rana M, Essig H, Eckardt AM, et al. Advances and innovations in computer-assisted head and neck oncologic surgery. J Craniofac Surg. 2012;23(1):272–8. https://doi.org/10.1097/SCS.0b013e318241bac7 .
Poon H, Li C, Gao W, Ren H, Lim CM. Evolution of robotic systems for transoral head and neck surgery. Oral Oncol. 2018;87:82–8. https://doi.org/10.1016/j.oraloncology.2018.10.020 .
Alameddine MB, Englesbe MJ, Waits SA. A video-based coaching intervention to improve surgical skill in fourth-year medical students. J Surg Educ. 2018;75(6):1475–9. https://doi.org/10.1016/j.jsurg.2018.04.003 .
Meara JG, Leather AJM, Hagander L, et al. Global surgery 2030: evidence and solutions for achieving health, welfare, and economic development. Lancet. 2015;386(9993):569–624. https://doi.org/10.1016/s0140-6736(15)60160-x .
Huntley RE, Ludwig DC, Dillon JK. Early effects of COVID-19 on oral and maxillofacial surgery residency training-results from a national survey. J Oral Maxillofac Surg. 2020;78(8):1257–67. https://doi.org/10.1016/j.joms.2020.05.026 .
Shah JP. The impact of COVID-19 on head and neck surgery, education, and training. Head Neck. 2020;42(6):1344–7. https://doi.org/10.1002/hed.26188 .
Augestad KM, Lindsetmo RO. Overcoming distance: video-conferencing as a clinical and educational tool among surgeons. World J Surg. 2009;33(7):1356–65. https://doi.org/10.1007/s00268-009-0036-0 .
Hu YY, Mazer LM, Yule SJ, et al. Complementing operating room teaching with video-based coaching. JAMA Surg. 2017;152(4):318–25. https://doi.org/10.1001/jamasurg.2016.4619 .
Augestad KM, Butt K, Ignjatovic D, Keller DS, Kiran R. Video-based coaching in surgical education: a systematic review and meta-analysis. Surg Endosc. 2020;34(2):521–35. https://doi.org/10.1007/s00464-019-07265-0 .
Greenberg CC, Dombrowski J, Dimick JB. Video-based surgical coaching: an emerging approach to performance improvement. JAMA Surg. 2016;151(3):282–3. https://doi.org/10.1001/jamasurg.2015.4442 .
Bizzotto N, Sandri A, Lavini F, Dall’Oca C, Regis D. Video in operating room: GoPro HERO3 camera on surgeon’s head to film operations–a test. Surg Innov. 2014;21(3):338–40. https://doi.org/10.1177/1553350613513514 .
Matsumoto S, Sekine K, Yamazaki M, et al. Digital video recording in trauma surgery using commercially available equipment. Scand J Trauma Resusc Emerg Med. 2013;21:27. https://doi.org/10.1186/1757-7241-21-27 .
Knight H, Gajendragadkar P, Bokhari A. Wearable technology: using Google Glass as a teaching tool. BMJ Case Rep. 2015;2015:bcr2014208768. https://doi.org/10.1136/bcr-2014-208768 .
Liao CH, Ooyang CH, Chen CC, et al. Video coaching improving contemporary technical and nontechnical ability in laparoscopic education. J Surg Educ. 2020;77(3):652–60. https://doi.org/10.1016/j.jsurg.2019.11.012 .
Volz S, Stevens TP, Dadiz R. A randomized controlled trial: does coaching using video during direct laryngoscopy improve residents’ success in neonatal intubations? J Perinatol. 2018;38(8):1074–80. https://doi.org/10.1038/s41372-018-0134-7 .
Giusto G, Caramello V, Comino F, Gandini M. The surgeon’s view: comparison of two digital video recording systems in veterinary surgery. J Vet Med Educ. 2015;42(2):161–5. https://doi.org/10.3138/jvme.0814-088R1 .
Silberthau KR, Chao TN, Newman JG. Innovating surgical education using video in the otolaryngology operating room. JAMA Otolaryngol Head Neck Surg. 2020;146(4):321–2. https://doi.org/10.1001/jamaoto.2019.4862 .
Graves SN, Shenaq DS, Langerman AJ, Song DH. Video capture of plastic surgery procedures using the GoPro HERO 3+. Plast Reconstr Surg Glob Open. 2015;3(2):e312. https://doi.org/10.1097/gox.0000000000000242 .
Kapi E. Surgeon-manipulated live surgery video recording apparatuses: personal experience and review of literature. Aesthetic Plast Surg. 2017;41(3):738–46. https://doi.org/10.1007/s00266-017-0826-y .
Kajita H, Takatsume Y, Shimizu T, Saito H, Kishi K. Overhead multiview camera system for recording open surgery. Plastic and reconstructive surgery Global open. 2020;8(4):e2765. https://doi.org/10.1097/GOX.0000000000002765 .
Warrian KJ, Ashenhurst M, Gooi A, Gooi P. A novel combination point-of-view (POV) action camera recording to capture the surgical field and instrument ergonomics in oculoplastic surgery. Ophthalmic Plast Reconstr Surg. 2015;31(4):321–2. https://doi.org/10.1097/iop.0000000000000465 .
Chaves RO, de Oliveira PAV, Rocha LC, et al. An innovative streaming video system with a point-of-view head camera transmission of surgeries to smartphones and tablets: an educational utility. Surg Innov. 2017;24(5):462–70. https://doi.org/10.1177/1553350617715162 .
Wentzell D, Dort J, Gooi A, Gooi P, Warrian K. Surgeon and assistant point of view simultaneous video recording. Studies in health technology and informatics. 2019;257:489–93.
Google Scholar
McCullough MC, Kulber L, Sammons P, Santos P, Kulber DA. Google glass for remote surgical tele-proctoring in low- and middle-income countries: a feasibility study from Mozambique. Plastic and reconstructive surgery Global open. 2018;6(12):e1999. https://doi.org/10.1097/GOX.0000000000001999 .
Xiao Y, Schimpff S, Mackenzie C, et al. Video technology to advance safety in the operating room and perioperative environment. Surg Innov. 2007;14(1):52–61. https://doi.org/10.1177/1553350607299777 .
Berger AJ, Gaster RS, Lee GK. Development of an affordable system for personalized video-documented surgical skill analysis for surgical residency training. Ann Plast Surg. 2013;70(4):442–6. https://doi.org/10.1097/SAP.0b013e31827e513c .
Turnbull AM, Emsley ES. Video recording of ophthalmic surgery–ethical and legal considerations. Surv Ophthalmol. 2014;59(5):553–8. https://doi.org/10.1016/j.survophthal.2014.01.006 .
Rassweiler JJ, Teber D. Advances in laparoscopic surgery in urology. Nat Rev Urol. 2016;13(7):387–99. https://doi.org/10.1038/nrurol.2016.70 .
Navia A, Parada L, Urbina G, Vidal C, Morovic CG. Optimizing intraoral surgery video recording for residents’ training during the COVID-19 pandemic: Comparison of 3 point of views using a GoPro. J Plast Reconstr Aesthet Surg. 2021;74(5):1101–60. https://doi.org/10.1016/j.bjps.2020.10.068 .
Purnell CA, Alkureishi LWT, Koranda C, Patel PK. Use of a waterproof camera immersed in povidone-iodine to improve intraoperative photography. Plastic and reconstructive surgery. 2019;143(3):962–5. https://doi.org/10.1097/prs.0000000000005327 .
Ahmed H, Devoto L. The potential of a digital twin in surgery. Surg Innov. 2021;28(4):509–10. https://doi.org/10.1177/1553350620975896 .
Laaki H, Miche Y, Tammi K. Prototyping a digital twin for real time remote control over mobile networks: application of remote surgery. IEEE Access. 2019;7:20325–36. https://doi.org/10.1109/access.2019.2897018 .
Hope C, Reilly JJ, Griffiths G, Lund J, Humes D. The impact of COVID-19 on surgical training: a systematic review. Tech Coloproctol. 2021;25(5):505–20. https://doi.org/10.1007/s10151-020-02404-5 .
Ereso AQ, Garcia P, Tseng E, et al. Live transference of surgical subspecialty skills using telerobotic proctoring to remote general surgeons. J Am Coll Surg. 2010;211(3):400–11. https://doi.org/10.1016/j.jamcollsurg.2010.05.014 .
Download references
Acknowledgements
The authors express their gratitude to the surgeons and students who participated in this study. Special thanks are also extended to Dr. Jun Jia and Dr. Kun Lv from the Department of Oral and Maxillofacial Surgery at the School & Hospital of Stomatology, Wuhan University, for their assistance with the surgical cases.
This study was supported by Wuhan University Undergraduate Education Quality Improvement and Comprehensive Reform Project (1607–413200072), Fundamental Research Funds for the Central Universities (Wuhan University, Clinical Medicine + X) (2042024YXB017), Postdoctoral Science Foundation of China (2018M630883& 2019T120688), Hubei Province Chinese Medicine Research Project (ZY20230015), Natural Science Foundation of Hubei Province (2023AFB665), and Medical Young Talents Program of Hubei Province, and Wuhan Young Medical Talents Training Project to L.-L. Bu.
Author information
Xin-Yue Huang and Zhe Shao contributed equally to this work.
Authors and Affiliations
State Key Laboratory of Oral & Maxillofacial Reconstruction and Regeneration, Key Laboratory of Oral Biomedicine Ministry of Education, Hubei Key Laboratory of Stomatology, School & Hospital of Stomatology, Wuhan University, Wuhan, China
Xin-Yue Huang, Zhe Shao, Nian-Nian Zhong, Yuan-Hao Wen, Tian-Fu Wu, Bing Liu, Si-Rui Ma & Lin-Lin Bu
Department of Oral & Maxillofacial - Head Neck Oncology, School & Hospital of Stomatology, Wuhan University, Wuhan, China
Zhe Shao, Tian-Fu Wu, Bing Liu, Si-Rui Ma & Lin-Lin Bu
You can also search for this author in PubMed Google Scholar
Contributions
XYH: Methodology, Investigation, Visualization, Writing—Original Draft, Writing—Review & Editing. ZS: Conceptualization, Investigation, Writing—Original Draft. NNZ: Methodology, Investigation, Writing—Review & Editing. YHW: Visualization, Writing—Review & Editing. TFW: Investigation, Writing—Review & Editing. BL: Writing—Review & Editing, Supervision, Funding acquisition. SRM: Conceptualization, Writing—Review & Editing, Supervision. LLB: Conceptualization, Methodology, Writing—Review & Editing, Supervision, Funding acquisition. All authors have reviewed and approved the final version of this manuscript for publication. Each author agrees to be accountable for all aspects of the work in ensuring that questions related to the accuracy or integrity of any part of the work are appropriately investigated and resolved.
Corresponding authors
Correspondence to Si-Rui Ma or Lin-Lin Bu .
Ethics declarations
Ethics approval and consent to participate.
This study was approved by the Ethics Committee of the School of Stomatology of Wuhan University (Approval No. 2022B11), and followed the guidelines of the Declaration of Helsinki of the World Medical Association. Informed consent was obtained fom all subjects involved in the study.
Consent for publication
Not applicable.
Competing interests
The authors declare no competing interests.
Additional information
Publisher’s note.
Springer Nature remains neutral with regard to jurisdictional claims in published maps and institutional affiliations.
Supplementary Information
Supplementary material 1..
Supplementary Material 2.
Rights and permissions
Open Access This article is licensed under a Creative Commons Attribution 4.0 International License, which permits use, sharing, adaptation, distribution and reproduction in any medium or format, as long as you give appropriate credit to the original author(s) and the source, provide a link to the Creative Commons licence, and indicate if changes were made. The images or other third party material in this article are included in the article's Creative Commons licence, unless indicated otherwise in a credit line to the material. If material is not included in the article's Creative Commons licence and your intended use is not permitted by statutory regulation or exceeds the permitted use, you will need to obtain permission directly from the copyright holder. To view a copy of this licence, visit http://creativecommons.org/licenses/by/4.0/ . The Creative Commons Public Domain Dedication waiver ( http://creativecommons.org/publicdomain/zero/1.0/ ) applies to the data made available in this article, unless otherwise stated in a credit line to the data.
Reprints and permissions
About this article
Cite this article.
Huang, XY., Shao, Z., Zhong, NN. et al. Comparative analysis of GoPro and digital cameras in head and neck flap harvesting surgery video documentation: an innovative and efficient method for surgical education. BMC Med Educ 24 , 531 (2024). https://doi.org/10.1186/s12909-024-05510-2
Download citation
Received : 18 November 2023
Accepted : 02 May 2024
Published : 14 May 2024
DOI : https://doi.org/10.1186/s12909-024-05510-2
Share this article
Anyone you share the following link with will be able to read this content:
Sorry, a shareable link is not currently available for this article.
Provided by the Springer Nature SharedIt content-sharing initiative
- Head and neck surgery
- Surgery video recording
- Video-based education
- Medical education
BMC Medical Education
ISSN: 1472-6920
- Submission enquiries: [email protected]
- General enquiries: [email protected]
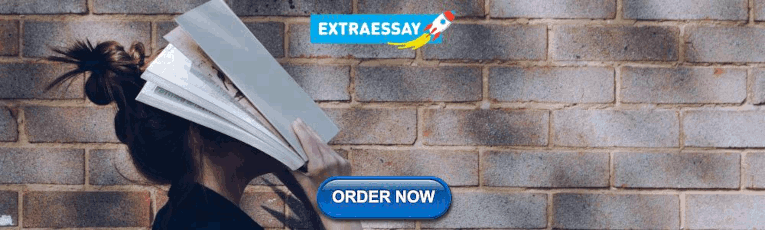
IMAGES
VIDEO
COMMENTS
Assessment is the foundation of health care. A goal in health care is to attain a comprehensive history and review of systems during the first encounter with the patient. The evidence-based recommendations presented in this article are informed by unpublished data from real-life experiences using a detailed review of systems during an ongoing medical surveillance program involving former ...
A Detailed Review of Systems: An Educational Feature @article{Phillips2017ADR, title={A Detailed Review of Systems: An Educational Feature}, author={Angela Phillips and A. La Sorte Frank and Collette Loftin and Sara E. Shepherd}, journal={The Journal for Nurse Practitioners}, year={2017}, volume={13}, pages={681-686}, url={https://api ...
A Detailed Review of Systems: An Educational Feature Angela Phillips, DNP, APRN, Arthur Frank, MD, PhD, Collette Loftin, PhD, and Sara Shepherd, MAMS ABSTRACT Assessment is the foundation of health care. A goal in health care is to attain a comprehensive history and review of systems during the first encounter with the patient.
The goal is to attain a comprehensive history and review of systems (ROS), if possible, on the first encounter with the patient. The evidence-based recommendations presented herein are informed by unpublished data from real-life experiences using a detailed ROS during an ongoing medical surveillance program of former nuclear weapons workers ...
Request PDF | A Detailed Review of Systems: An Educational Feature | Assessment is the foundation of health care. A goal in health care is to attain a comprehensive history and review of systems ...
A Detailed Review of Systems: An Educational Feature Angela Phillips, DNP, APRN, Arthur Frank, MD, PhD, Collette Loftin, PhD, and Sara Shepherd, MAMS. ABSTRACT Assessment is the foundation of health care. A goal in health care is to attain a comprehensive history and review of systems during thefirst encounter with the patient.
Review of Systems: The Backbone of Practice October 3, 2018 Angela Phillips, DNP, APRN, West Texas A&M University ... •Participants who qualify for CE will receive a detailed email on how to obtain CE credit using the AANP CE Center ... A detailed review of systems: an educational feature. The Journal for Nurse Practitioners, 13(10), 681-686 ...
The review of systems is a list of closed ended questions intended to uncover any recent symptoms that haven't already come up. In the clinic, patients often complete the ROS on paper or online before the visit, and the provider simply reviews it. For a new patient in the hospital, physicians typically ask at least one or two of the most ...
Highlights. •. Assessment is the foundation of health care. •. A detailed review of systems is an essential element in each patient encounter. •. A review of systems is crucial in gaining optimal understanding of a patient. •. New problems can be discovered during a detailed review of systems.
Over the past two decades, policy mandates in science education and real-world complex systems research have spurred an interest in helping students learn about complex systems at the K-12 level. This systematic review was aimed at understanding how well the CSSE research reflects three central features of educational interventions.
Assessment is the foundation of health care. A goal in health care is to attain a comprehensive history and review of systems during the first encounter with the patient. The evidence-based recommendations presented in this article are informed by unpublished data from real-life experiences using a detailed review of systems during an ongoing medical surveillance program involving former ...
Phillips A., Frank A., Loftin C., Shepherd S. A detailed review of systems: an educational feature. The Journal of Nurse Practitioners, 13(10): 681-686, 2017. Assessment is the foundation of health care. A goal in health care is to attain a comprehensive history and review of systems during the first encounter with the patient.
An education system includes four kinds of elements: (1) inputs: pupils, administration, teachers, material for formal or informal education; (2) processes: formal or informal education process; (3) outputs: people who have attained educational objectives, such as grades and abilities; (4) and an environment: formal learning venues (e.g., schools) and informal learning venues (e.g., home ...
Understanding what contributes to improving a system will help us tackle the problems in education systems that usually fail disproportionately in providing quality education for all, especially for the most disadvantage sectors of the population. This paper presents the results of a qualitative systematic literature review aimed at providing a comprehensive overview of what education research ...
This is one of a series of working papers from "RISE"—the large-scale education systems research programme supported by funding from the United Kingdom's Foreign, Commonwealth and Development Office (FCDO), the Australian Government's Department of Foreign Affairs and Trade (DFAT), and the Bill and Melinda Gates Foundation.
Meta-analysis comprises a powerful tool for synthesising prior research and empirically validating theoretical frameworks. Using this tool and two recent multilevel models of educational effectiveness as guiding frameworks, this paper synthesises the results of 195 studies investigating the association between system-level characteristics and student learning outcomes.
A short history of systems thinking, emphasizing the pressure points or organizational levers inside the education institution that touch classrooms. How systems thinking moved into the education ...
My Research and Language Selection Sign into My Research Create My Research Account English; Help and support. Support Center Find answers to questions about products, access, use, setup, and administration.; Contact Us Have a question, idea, or some feedback? We want to hear from you.
A full systems review is not necessary for every patient.You should only review systems relevant to the presenting complaint with the aim of identifying/excluding significant associated features. For example, for left iliac fossa pain, a complete history will include questions about gastrointestinal, urological and, if female, obstetric and gynaecological systems.
A systematic literature review on educational recommender systems for teaching and learning: research trends, limitations and opportunities ... this review aims to contribute to the area by presenting a detailed analysis of the following dimensions: how recommendations are produced and presented, how recommender systems are evaluated and what ...
Explore millions of resources from scholarly journals, books, newspapers, videos and more, on the ProQuest Platform.
Merging these two systems could significantly advance the field of surgical video recording. ... The zoom feature enables close-up, detailed recording of surgical procedures and anatomical nuances. ... Keller DS, Kiran R. Video-based coaching in surgical education: a systematic review and meta-analysis. Surg Endosc. 2020;34(2):521-35. https ...