User Preferences
Content preview.
Arcu felis bibendum ut tristique et egestas quis:
- Ut enim ad minim veniam, quis nostrud exercitation ullamco laboris
- Duis aute irure dolor in reprehenderit in voluptate
- Excepteur sint occaecat cupidatat non proident
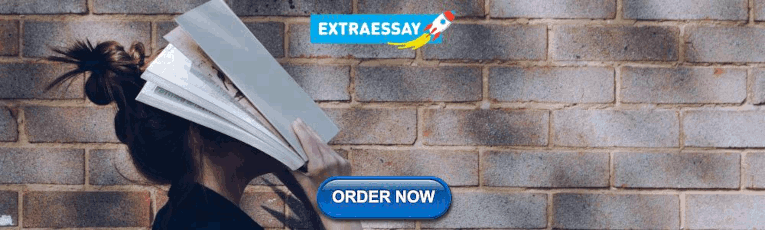
Keyboard Shortcuts
Lesson 1: introduction to design of experiments, overview section .
In this course we will pretty much cover the textbook - all of the concepts and designs included. I think we will have plenty of examples to look at and experience to draw from.
Please note: the main topics listed in the syllabus follow the chapters in the book.
A word of advice regarding the analyses. The prerequisite for this course is STAT 501 - Regression Methods and STAT 502 - Analysis of Variance . However, the focus of the course is on the design and not on the analysis. Thus, one can successfully complete this course without these prerequisites, with just STAT 500 - Applied Statistics for instance, but it will require much more work, and for the analysis less appreciation of the subtleties involved. You might say it is more conceptual than it is math oriented.
Text Reference: Montgomery, D. C. (2019). Design and Analysis of Experiments , 10th Edition, John Wiley & Sons. ISBN 978-1-119-59340-9
What is the Scientific Method? Section
Do you remember learning about this back in high school or junior high even? What were those steps again?
Decide what phenomenon you wish to investigate. Specify how you can manipulate the factor and hold all other conditions fixed, to insure that these extraneous conditions aren't influencing the response you plan to measure.
Then measure your chosen response variable at several (at least two) settings of the factor under study. If changing the factor causes the phenomenon to change, then you conclude that there is indeed a cause-and-effect relationship at work.
How many factors are involved when you do an experiment? Some say two - perhaps this is a comparative experiment? Perhaps there is a treatment group and a control group? If you have a treatment group and a control group then, in this case, you probably only have one factor with two levels.
How many of you have baked a cake? What are the factors involved to ensure a successful cake? Factors might include preheating the oven, baking time, ingredients, amount of moisture, baking temperature, etc.-- what else? You probably follow a recipe so there are many additional factors that control the ingredients - i.e., a mixture. In other words, someone did the experiment in advance! What parts of the recipe did they vary to make the recipe a success? Probably many factors, temperature and moisture, various ratios of ingredients, and presence or absence of many additives. Now, should one keep all the factors involved in the experiment at a constant level and just vary one to see what would happen? This is a strategy that works but is not very efficient. This is one of the concepts that we will address in this course.
- understand the issues and principles of Design of Experiments (DOE),
- understand experimentation is a process,
- list the guidelines for designing experiments, and
- recognize the key historical figures in DOE.
Have a language expert improve your writing
Run a free plagiarism check in 10 minutes, generate accurate citations for free.
- Knowledge Base
Methodology
- Guide to Experimental Design | Overview, Steps, & Examples
Guide to Experimental Design | Overview, 5 steps & Examples
Published on December 3, 2019 by Rebecca Bevans . Revised on June 21, 2023.
Experiments are used to study causal relationships . You manipulate one or more independent variables and measure their effect on one or more dependent variables.
Experimental design create a set of procedures to systematically test a hypothesis . A good experimental design requires a strong understanding of the system you are studying.
There are five key steps in designing an experiment:
- Consider your variables and how they are related
- Write a specific, testable hypothesis
- Design experimental treatments to manipulate your independent variable
- Assign subjects to groups, either between-subjects or within-subjects
- Plan how you will measure your dependent variable
For valid conclusions, you also need to select a representative sample and control any extraneous variables that might influence your results. If random assignment of participants to control and treatment groups is impossible, unethical, or highly difficult, consider an observational study instead. This minimizes several types of research bias, particularly sampling bias , survivorship bias , and attrition bias as time passes.
Table of contents
Step 1: define your variables, step 2: write your hypothesis, step 3: design your experimental treatments, step 4: assign your subjects to treatment groups, step 5: measure your dependent variable, other interesting articles, frequently asked questions about experiments.
You should begin with a specific research question . We will work with two research question examples, one from health sciences and one from ecology:
To translate your research question into an experimental hypothesis, you need to define the main variables and make predictions about how they are related.
Start by simply listing the independent and dependent variables .
Then you need to think about possible extraneous and confounding variables and consider how you might control them in your experiment.
Finally, you can put these variables together into a diagram. Use arrows to show the possible relationships between variables and include signs to show the expected direction of the relationships.
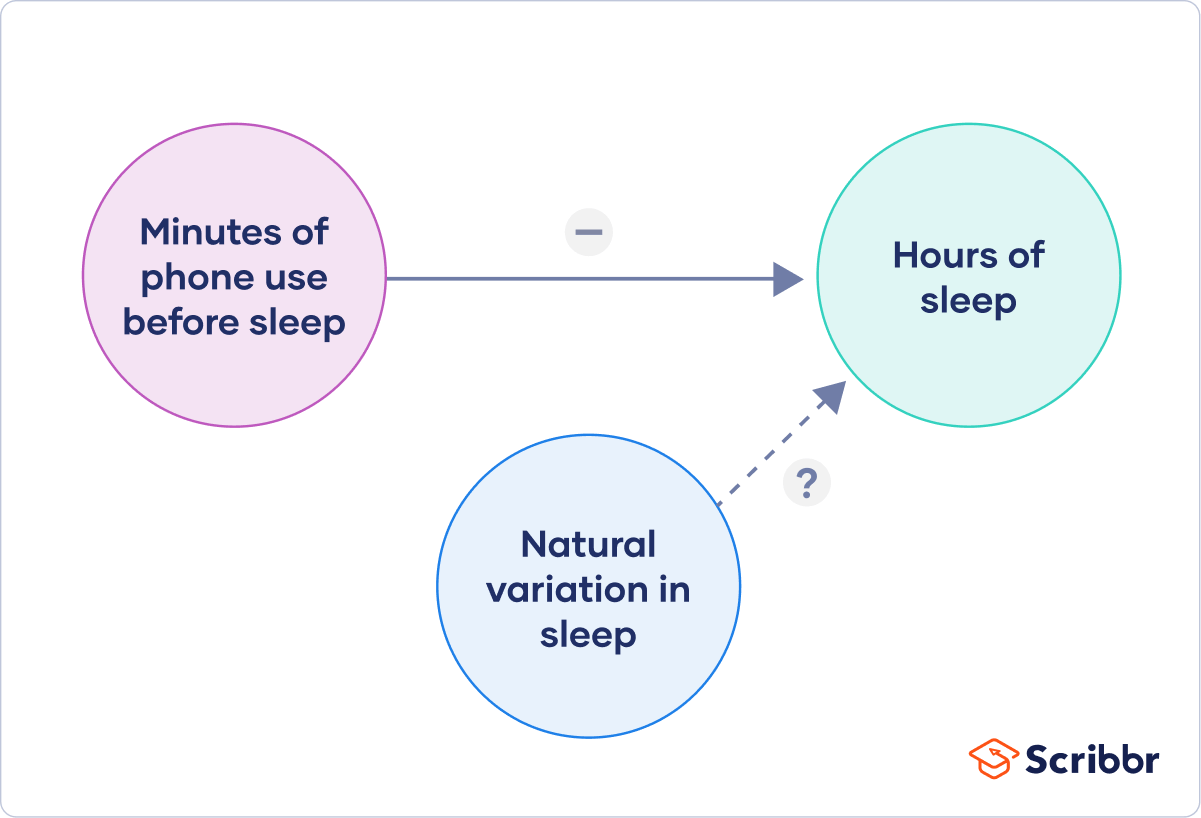
Here we predict that increasing temperature will increase soil respiration and decrease soil moisture, while decreasing soil moisture will lead to decreased soil respiration.
Prevent plagiarism. Run a free check.
Now that you have a strong conceptual understanding of the system you are studying, you should be able to write a specific, testable hypothesis that addresses your research question.
The next steps will describe how to design a controlled experiment . In a controlled experiment, you must be able to:
- Systematically and precisely manipulate the independent variable(s).
- Precisely measure the dependent variable(s).
- Control any potential confounding variables.
If your study system doesn’t match these criteria, there are other types of research you can use to answer your research question.
How you manipulate the independent variable can affect the experiment’s external validity – that is, the extent to which the results can be generalized and applied to the broader world.
First, you may need to decide how widely to vary your independent variable.
- just slightly above the natural range for your study region.
- over a wider range of temperatures to mimic future warming.
- over an extreme range that is beyond any possible natural variation.
Second, you may need to choose how finely to vary your independent variable. Sometimes this choice is made for you by your experimental system, but often you will need to decide, and this will affect how much you can infer from your results.
- a categorical variable : either as binary (yes/no) or as levels of a factor (no phone use, low phone use, high phone use).
- a continuous variable (minutes of phone use measured every night).
How you apply your experimental treatments to your test subjects is crucial for obtaining valid and reliable results.
First, you need to consider the study size : how many individuals will be included in the experiment? In general, the more subjects you include, the greater your experiment’s statistical power , which determines how much confidence you can have in your results.
Then you need to randomly assign your subjects to treatment groups . Each group receives a different level of the treatment (e.g. no phone use, low phone use, high phone use).
You should also include a control group , which receives no treatment. The control group tells us what would have happened to your test subjects without any experimental intervention.
When assigning your subjects to groups, there are two main choices you need to make:
- A completely randomized design vs a randomized block design .
- A between-subjects design vs a within-subjects design .
Randomization
An experiment can be completely randomized or randomized within blocks (aka strata):
- In a completely randomized design , every subject is assigned to a treatment group at random.
- In a randomized block design (aka stratified random design), subjects are first grouped according to a characteristic they share, and then randomly assigned to treatments within those groups.
Sometimes randomization isn’t practical or ethical , so researchers create partially-random or even non-random designs. An experimental design where treatments aren’t randomly assigned is called a quasi-experimental design .
Between-subjects vs. within-subjects
In a between-subjects design (also known as an independent measures design or classic ANOVA design), individuals receive only one of the possible levels of an experimental treatment.
In medical or social research, you might also use matched pairs within your between-subjects design to make sure that each treatment group contains the same variety of test subjects in the same proportions.
In a within-subjects design (also known as a repeated measures design), every individual receives each of the experimental treatments consecutively, and their responses to each treatment are measured.
Within-subjects or repeated measures can also refer to an experimental design where an effect emerges over time, and individual responses are measured over time in order to measure this effect as it emerges.
Counterbalancing (randomizing or reversing the order of treatments among subjects) is often used in within-subjects designs to ensure that the order of treatment application doesn’t influence the results of the experiment.
Here's why students love Scribbr's proofreading services
Discover proofreading & editing
Finally, you need to decide how you’ll collect data on your dependent variable outcomes. You should aim for reliable and valid measurements that minimize research bias or error.
Some variables, like temperature, can be objectively measured with scientific instruments. Others may need to be operationalized to turn them into measurable observations.
- Ask participants to record what time they go to sleep and get up each day.
- Ask participants to wear a sleep tracker.
How precisely you measure your dependent variable also affects the kinds of statistical analysis you can use on your data.
Experiments are always context-dependent, and a good experimental design will take into account all of the unique considerations of your study system to produce information that is both valid and relevant to your research question.
If you want to know more about statistics , methodology , or research bias , make sure to check out some of our other articles with explanations and examples.
- Student’s t -distribution
- Normal distribution
- Null and Alternative Hypotheses
- Chi square tests
- Confidence interval
- Cluster sampling
- Stratified sampling
- Data cleansing
- Reproducibility vs Replicability
- Peer review
- Likert scale
Research bias
- Implicit bias
- Framing effect
- Cognitive bias
- Placebo effect
- Hawthorne effect
- Hindsight bias
- Affect heuristic
Experimental design means planning a set of procedures to investigate a relationship between variables . To design a controlled experiment, you need:
- A testable hypothesis
- At least one independent variable that can be precisely manipulated
- At least one dependent variable that can be precisely measured
When designing the experiment, you decide:
- How you will manipulate the variable(s)
- How you will control for any potential confounding variables
- How many subjects or samples will be included in the study
- How subjects will be assigned to treatment levels
Experimental design is essential to the internal and external validity of your experiment.
The key difference between observational studies and experimental designs is that a well-done observational study does not influence the responses of participants, while experiments do have some sort of treatment condition applied to at least some participants by random assignment .
A confounding variable , also called a confounder or confounding factor, is a third variable in a study examining a potential cause-and-effect relationship.
A confounding variable is related to both the supposed cause and the supposed effect of the study. It can be difficult to separate the true effect of the independent variable from the effect of the confounding variable.
In your research design , it’s important to identify potential confounding variables and plan how you will reduce their impact.
In a between-subjects design , every participant experiences only one condition, and researchers assess group differences between participants in various conditions.
In a within-subjects design , each participant experiences all conditions, and researchers test the same participants repeatedly for differences between conditions.
The word “between” means that you’re comparing different conditions between groups, while the word “within” means you’re comparing different conditions within the same group.
An experimental group, also known as a treatment group, receives the treatment whose effect researchers wish to study, whereas a control group does not. They should be identical in all other ways.
Cite this Scribbr article
If you want to cite this source, you can copy and paste the citation or click the “Cite this Scribbr article” button to automatically add the citation to our free Citation Generator.
Bevans, R. (2023, June 21). Guide to Experimental Design | Overview, 5 steps & Examples. Scribbr. Retrieved April 9, 2024, from https://www.scribbr.com/methodology/experimental-design/
Is this article helpful?
Rebecca Bevans
Other students also liked, random assignment in experiments | introduction & examples, quasi-experimental design | definition, types & examples, how to write a lab report, "i thought ai proofreading was useless but..".
I've been using Scribbr for years now and I know it's a service that won't disappoint. It does a good job spotting mistakes”
Note that this "residual" for the within plot \(subplot\) part of the analysis is actually the sum of squares for the interaction of rows \(w\ hole plots\) with varieties \(subplot treatments\)---as in an RCBD.
- r_k\(i\) ~ N\(0, sigma^2_r\)
- e_ijk ~ N\(0, sigma^2_e\)
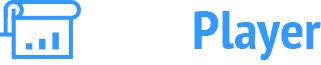
- My presentations
Auth with social network:
Download presentation
We think you have liked this presentation. If you wish to download it, please recommend it to your friends in any social system. Share buttons are a little bit lower. Thank you!
Presentation is loading. Please wait.
Design of Experiments (DOE)
Published by Alfred Fleming Modified over 5 years ago
Similar presentations
Presentation on theme: "Design of Experiments (DOE)"— Presentation transcript:
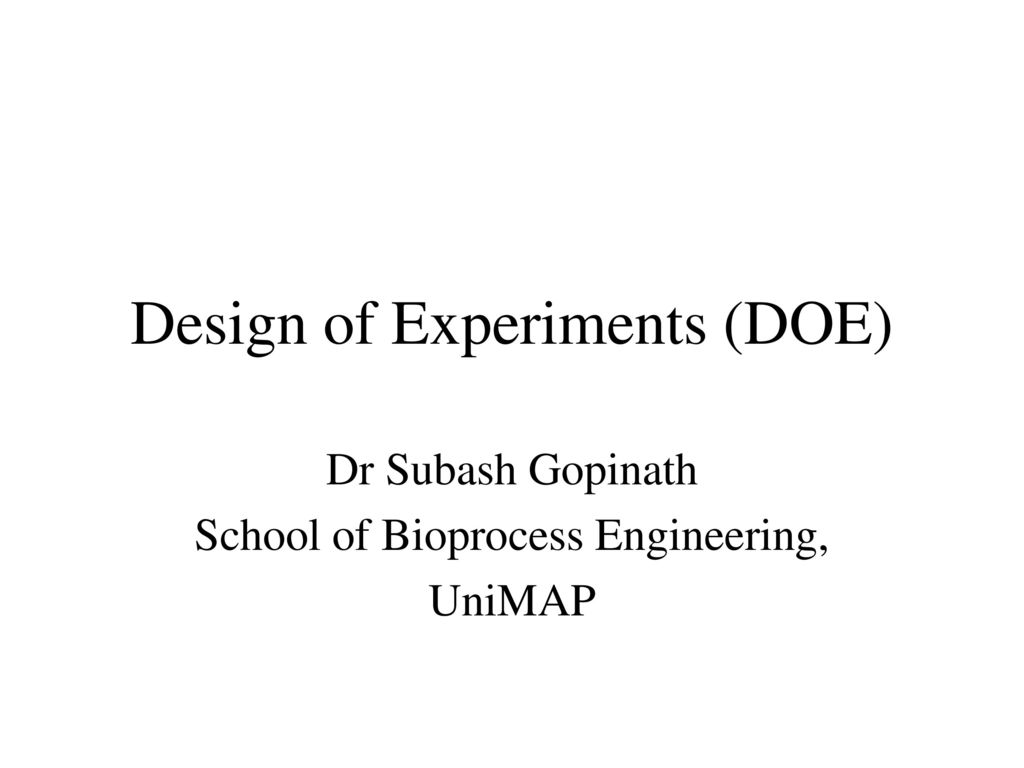
The Robert Gordon University School of Engineering Dr. Mohamed Amish
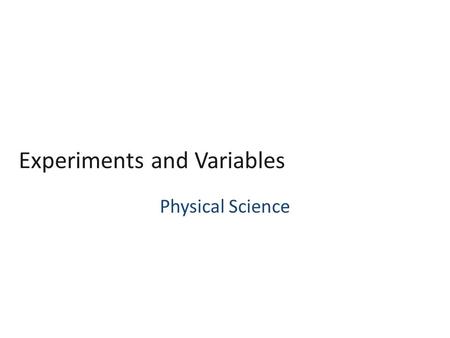
Experiments and Variables
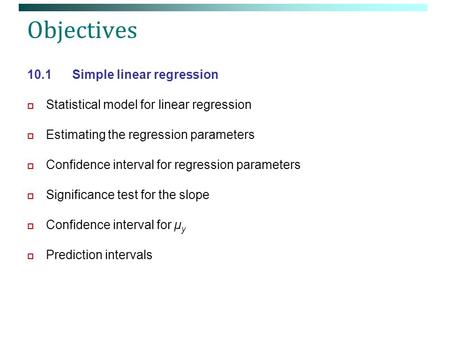
Objectives 10.1 Simple linear regression
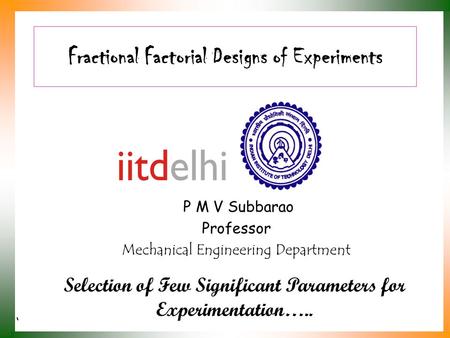
Fractional Factorial Designs of Experiments
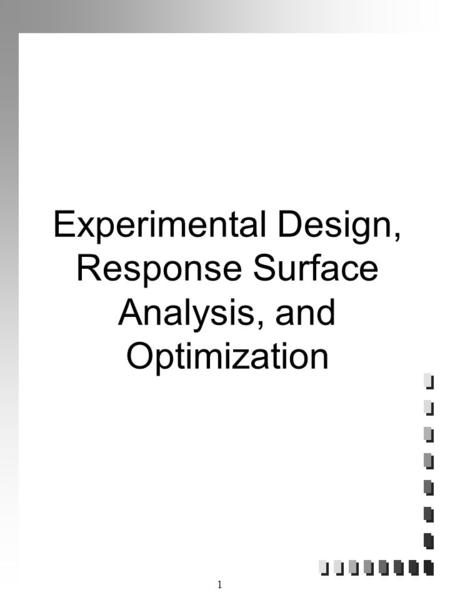
Experimental Design, Response Surface Analysis, and Optimization
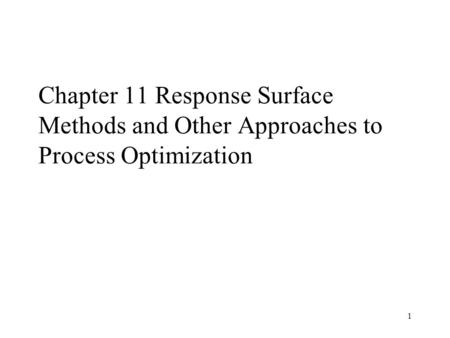
11.1 Introduction to Response Surface Methodology
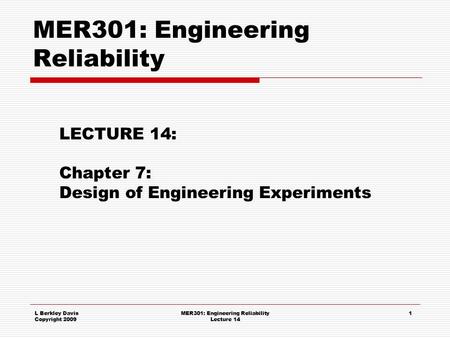
L Berkley Davis Copyright 2009 MER301: Engineering Reliability Lecture 14 1 MER301: Engineering Reliability LECTURE 14: Chapter 7: Design of Engineering.
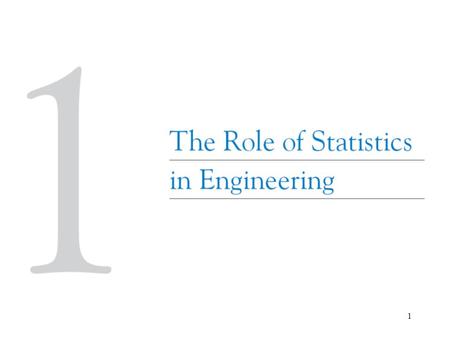
1-1 The Engineering Method and Statistical Thinking Engineers solve problems of interest to society by the efficient application of scientific principles.
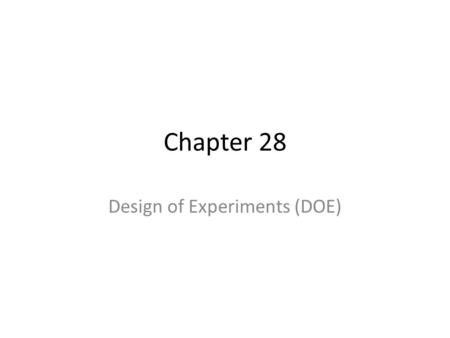
Chapter 28 Design of Experiments (DOE). Objectives Define basic design of experiments (DOE) terminology. Apply DOE principles. Plan, organize, and evaluate.
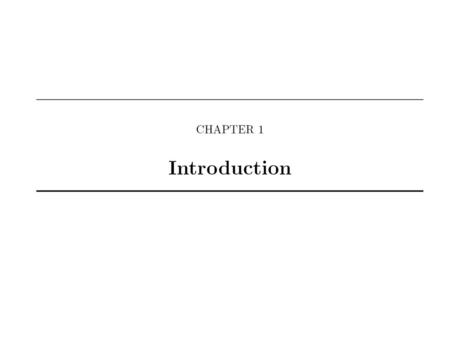
Statistics: The Science of Learning from Data Data Collection Data Analysis Interpretation Prediction Take Action W.E. Deming “The value of statistics.
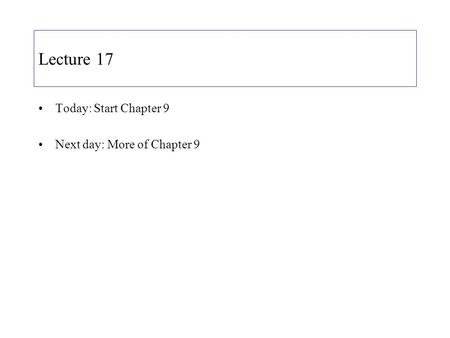
Lecture 17 Today: Start Chapter 9 Next day: More of Chapter 9.
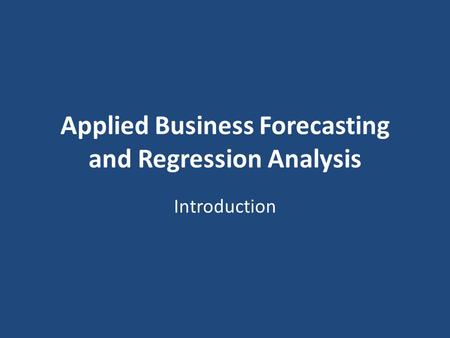
Applied Business Forecasting and Regression Analysis Introduction.
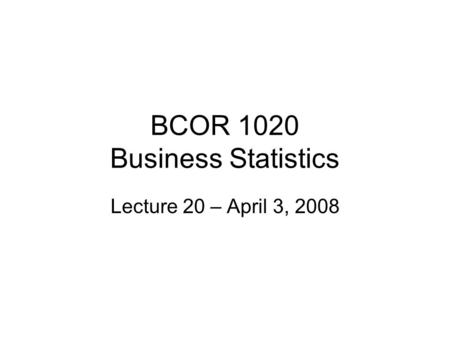
BCOR 1020 Business Statistics Lecture 20 – April 3, 2008.
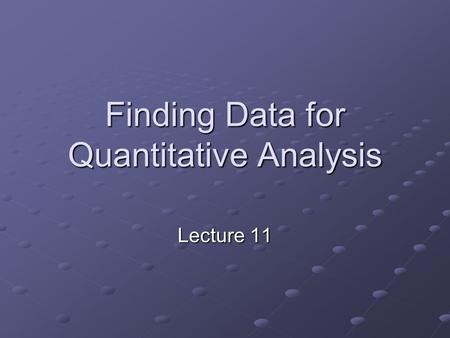
Finding Data for Quantitative Analysis Lecture 11.
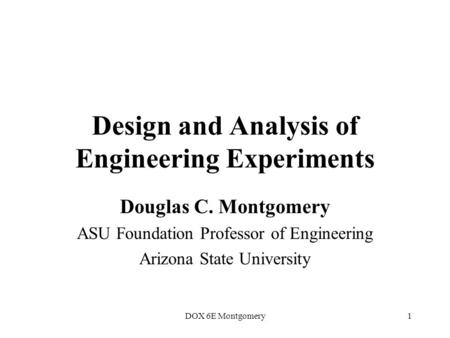
Design and Analysis of Engineering Experiments
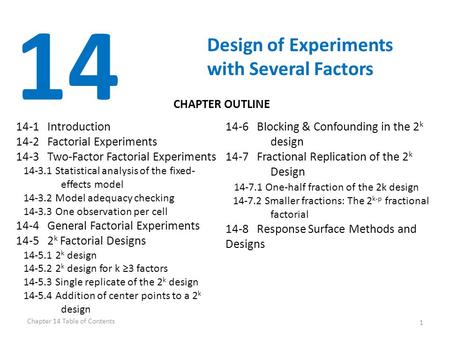
1 14 Design of Experiments with Several Factors 14-1 Introduction 14-2 Factorial Experiments 14-3 Two-Factor Factorial Experiments Statistical analysis.
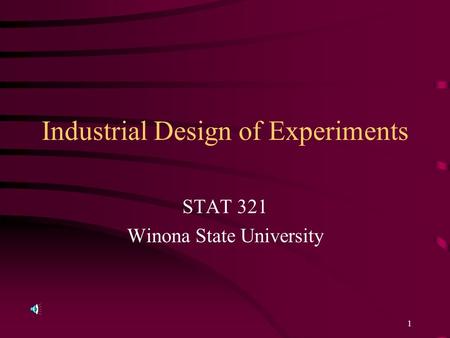
1 Industrial Design of Experiments STAT 321 Winona State University.
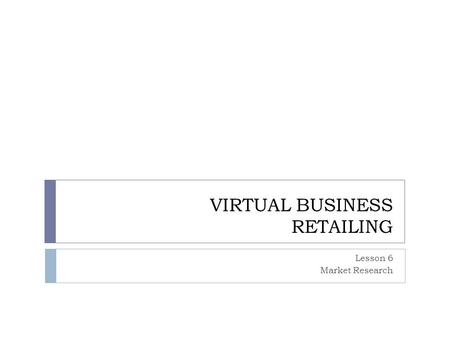
VIRTUAL BUSINESS RETAILING
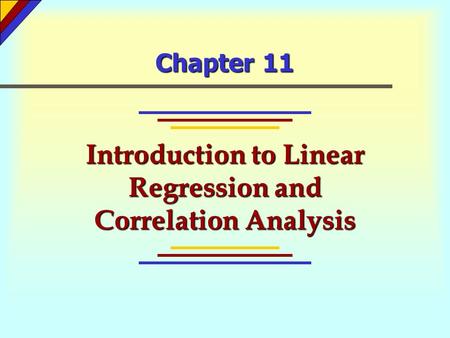
Introduction to Linear Regression and Correlation Analysis
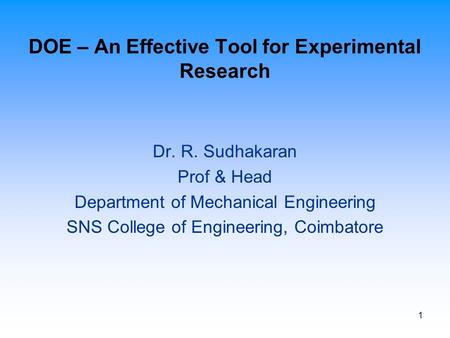
DOE – An Effective Tool for Experimental Research
About project
© 2024 SlidePlayer.com Inc. All rights reserved.
Design of Experiments
Discipline: Innovative Engineering
Presenters:
- Course Materials
- Confirmation of Attendance
- Olivier De Weck's Biography
- NESC Academy Online
- Innovative Engineering Course
Similar Videos
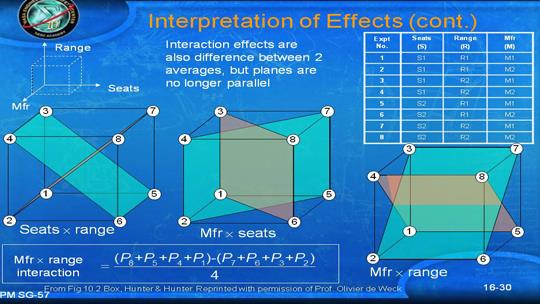
Design Processes
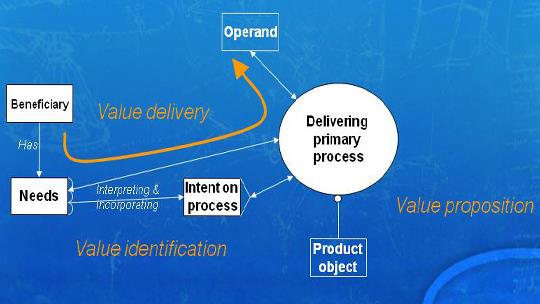
Creativity through Functional Abstraction
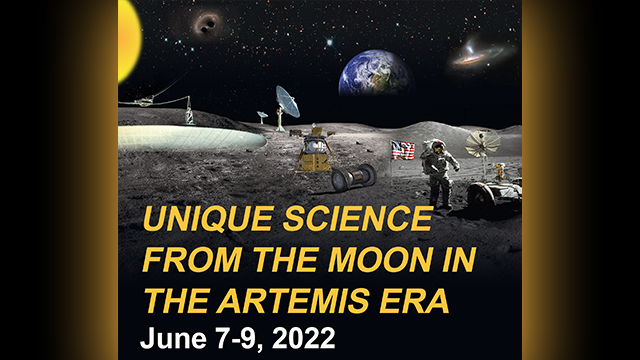
Day 2.2 Power Generational Storage & Human Intervention Challenges with Assembly & Servicing of Scientific Experiments
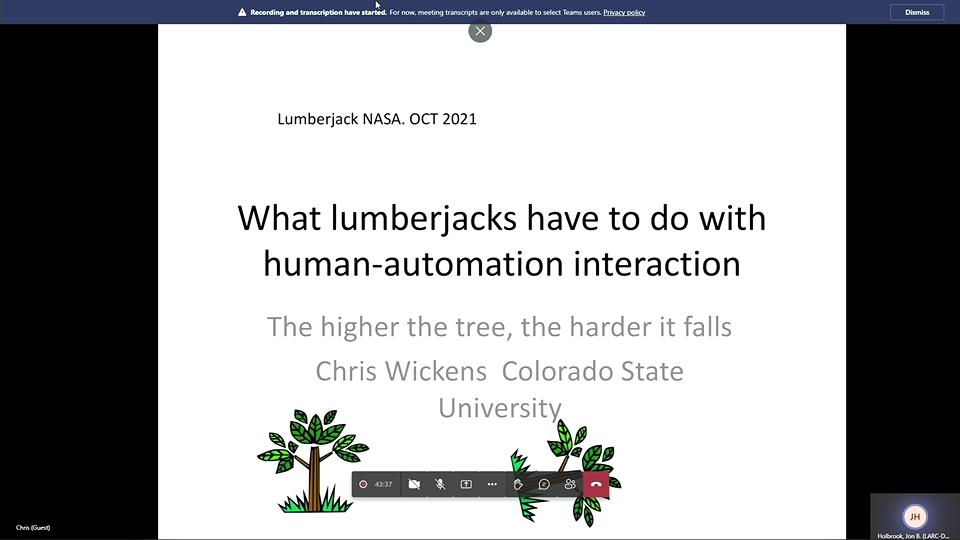
Lumberjack Tradeoff Model: The Degree of Imperfect Automation
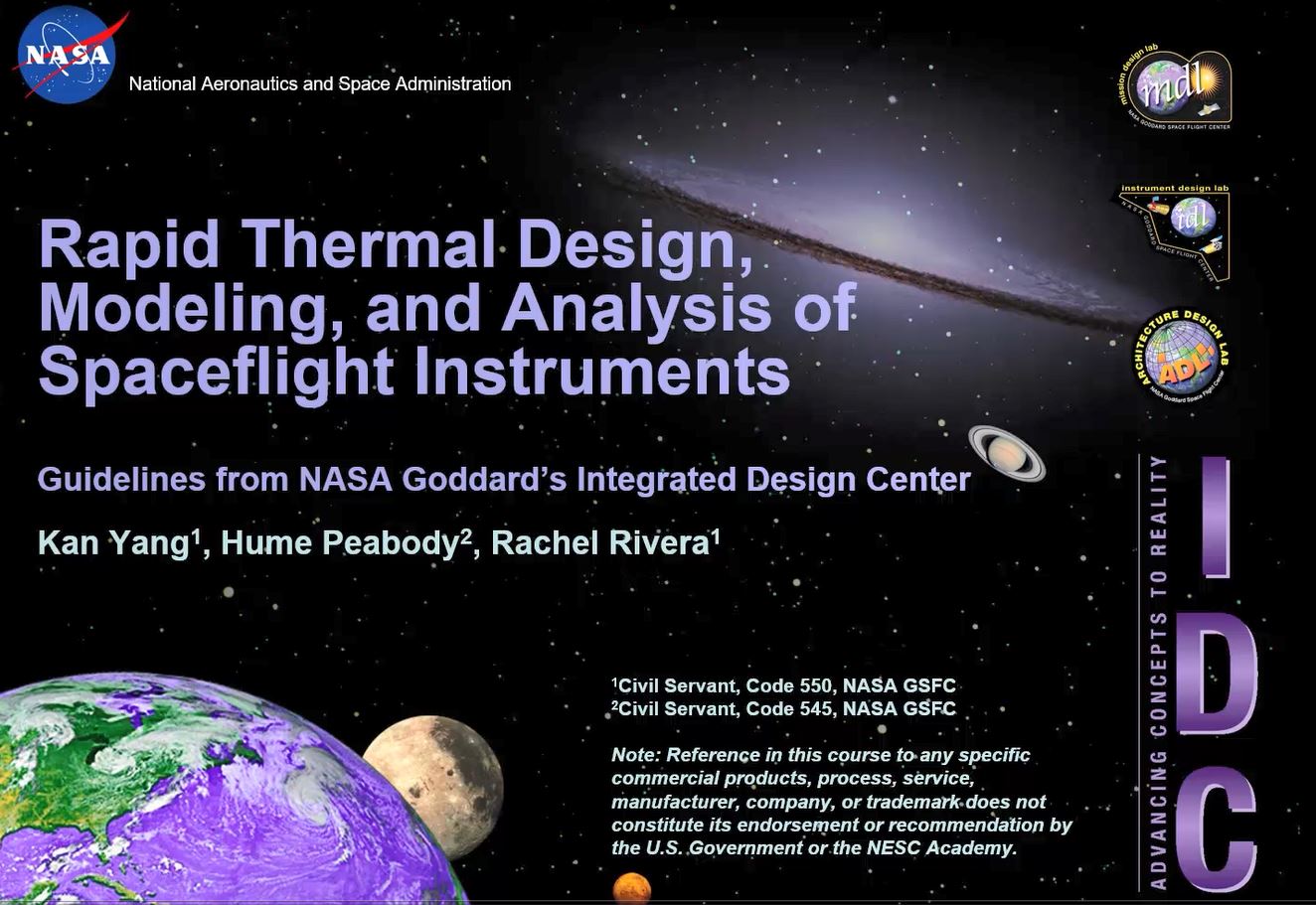
Rapid Thermal Design, Modeling, and Analysis of Spaceflight Instruments, Part 3
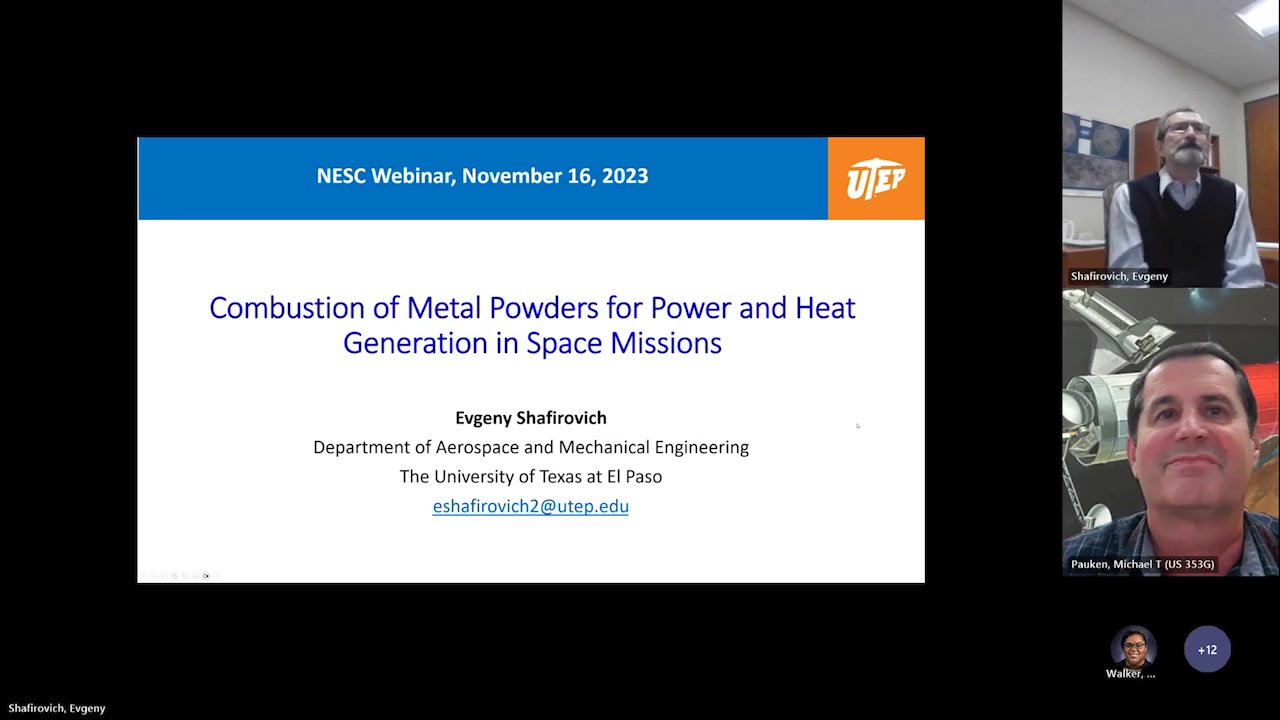
Combustion of Metal Powders for Power and Heat Generation in Space Missions
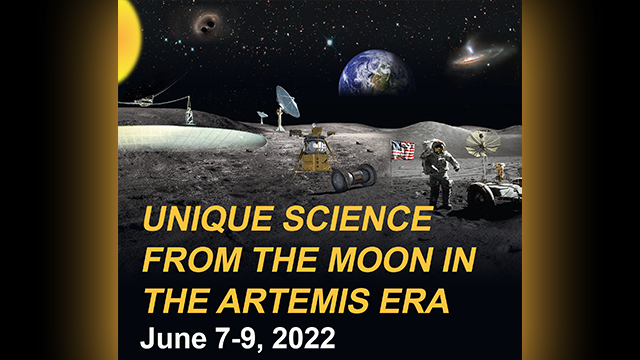
Day 3.1 Overview of LunaNet & Worksite Design and Lighting
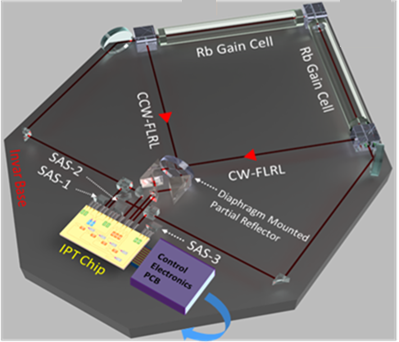
Fast-Light Inertial Sensors
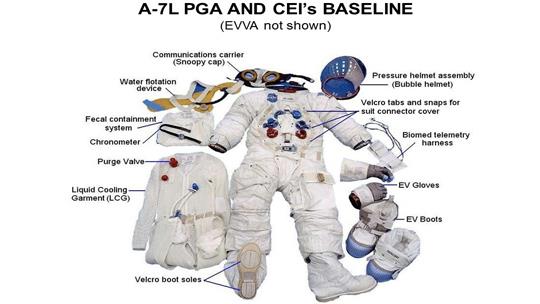
Early Apollo Spacesuit Development, A-7L Suit Requirements, and Design Details
Design of Experiments
Introductory basics, introduction to design of experiments.
The Open Educator Textbook Explanation with Examples and Video Demonstrations
Video Demonstration Only, Click on the Topic Below
What is Design of Experiments DOE?
Hypothesis Testing Basic
Explanation of Factor, Response, dependent, independent, variable
Levels of a Factor
Fixed Factor, Random Factor, and Block
Descriptive Statistics and Inferential Statistics
What is Analysis of Variance ANOVA & Why
p-value & Level of Significance
Errors in Statistical Tests Type 1, Type II, Type III
Hypothesis Testing
How to Choose an Appropriate Statistical Method/Test for Your Design of Experiments or Data Analysis
Single Sample Z Test Application, Data Collection, Analysis, Results Explained in MS Excel & Minitab
Single Sample T Test Application, Data Collection, Analysis, Results Explained in MS Excel & Minitab
Single Proportion Test Application, Data Collection, Analysis, Results Explained MS Excel & Minitab
Two-Sample Z Test Application, Data Collection, Analysis, Results Explained Using MS Excel & Minitab
Two Sample T Test Application, Data Collection, Analysis, Results Explained Using MS Excel & Minitab
Paired T Test Application, Data Collection, Analysis, Results Explained Using MS Excel & Minitab
Two Sample/Population Proportion Test Application, Analysis & Result Explained in MS Excel & Minitab
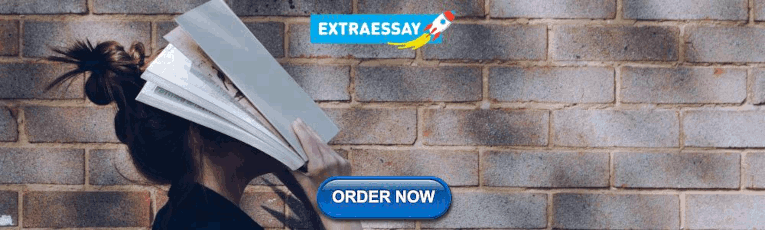
Completely Randomized Design (CRD)
One-way/single factor analysis of variance, anova.
One Way Single Factor Analysis of Variance ANOVA Completely Randomized Design Analysis in MS Excel
One Way Single Factor Analysis of Variance ANOVA Completely Randomized Design Analysis in Minitab
One Way Single Factor Analysis of Variance ANOVA Post Hoc Pairwise Comparison Analysis in MS Excel
Fixed vs Random Effect Model Explained with Examples Using Excel and Minitab
Randomized Complete Block Design
Randomized Complete Block Design of Experiments RCBD Using Minitab 2020
Latin Square Design Using Minitab Updated 2020
Graeco Latin Square Design Updated 2020
Latin Square and Graeco Latin Square Design
Latin Square and Graeco Latin Square Design Analysis using Minitab
Screening the Important Factors/Variables
Factorial design of experiments.
Introduction to Factorial Design and the Main Effect Calculation
C alculate Two Factors Interaction Effect
Regression using the Calculated Effects
Basic Response Surface Methodology RSM Factorial Design
Construct ANOVA Table from the Effect Estimates
2k Factorial Design of Experiments
The Open Educator Textbook Explanation with Examples and Video Demonstrations for All Topics
Introduction to 2K Factorial Design
Contrast, Effect, Sum of Square, Estimate Formula, ANOVA table
Design Layout and Construction of 2K Factorial Design Using MS Excel
Write Treatment Combinations Systematically and Flawlessly
Contrast, Effect, Estimate, and Sum of Square Calculation Using MS Excel
Comparisons between MS Excel, Minitab, SPSS, and SAS in Design and Analysis of Experiments
Blocking and Confounding in 2k Design
Introduction to Blocking and Confounding
Confounding in Factorial and Fractional Factorial
Blocking and Confounding Using -1/+1 Coding System
Blocking and Confounding Using Linear Combination Method
Multiple Blocking and Confounding, How To
Complete vs Partial Confounding and The Appropriate Use of Them
How Many Confounded Treatments are There in a Multiple Confounded Effects
How to Confound Three or More Effects in Eight or More Blocks
Fractional Factorial Design
What is Fractional Factorial Design of Experiments
The One-Half Fraction Explained in 2K Fractional Factorial Design
Introduction to the Primary Basics of the Fractional Factorial Design
Design Resolution Explained
One-Half Fractional Factorial 2k Design Details Explained
How to Design a One-Half Fractional Factorial 2k Design using MS Excel
One-Quarter Fractional Factorial 2k Design
Design a One-Quarter Fractional Factorial 2k Design Using MS Excel
Calculate and Write All Effects in 2k Factorial Design Systematic Flawless
Write Alias Structure in 2K Fractional Factorial Design
Write Alias Structure in 2K Six Factor Quarter Fraction Factorial Design
Design a One-Eighth Fractional Factorial 2k Design Using MS Excel
2K Alias Structure Solution an Example Solution
Fractional Factorial Data Analysis Example Minitab ( Fractional Factorial DOE Data Analysis Example Document )
Design any Fractional Factorial Design with the Lowest Number of Possible Runs Easiest Method in MS Excel
The Easiest Way to Randomize an Experiment in using MS Excel
Plackett-Burman Fractional Factorial Design Using MS Excel
Plackett Burman Fractional Factorial Design of Experiments DOE Using Minitab
Optimize the Important Factors/Variables
Applied regression analysis.
Simple Linear Regression Analysis Using MS Excel and Minitab
Simple Linear Regression Analysis Real Life Example 1
Simple Linear Regression Analysis Real Life Example 2
Simple Linear Regression Analysis Example Cost Estimation
Linear Regression Diagnostics Analysis
Response Surface Methodology
What is Response Surface Methodology RSM and How to Learn it?
Basic Response Surface Methodology RSM Design and Analysis Minitab
Response Surface Basic Central Composite Design
Response Surface Central Composite Design in MS Excel
Response Surface Design Layout Construction Minitab MS Excel
Response Surface Design Analysis Example Minitab
Multiple Response Optimization in Response Surface Methodology RSM
Box Behnken Response Surface Methodology RSM Design and Analysis Explained Example using Minitab
Is Box Behnken Better than the Central Composite Design in the Response Surface Methodology?
Advanced Complex Mixed Factors
Expected mean square, basics to complex models.
Expected Mean Square All Fixed Factors
Expected Mean Square Random Effect Model
Restricted vs Unrestricted Mixed Model Design of Experiments with Fixed and Random Factors
How to Systematically Develop Expected Mean Square Fixed and Random Mixed Effect Model
How to Systematically Develop Expected Mean Square Random, Nested, and Fixed Mixed Effect Model
Restricted vs Unrestricted Mixed Models, How to Choose the Appropriate Model
Nested, & Repeated Measure, Split-Plot Design
Nested Design
Repeated Measure Design
Split Plot Design
Difference between Nested, Split Plot and Repeated Measure Design
Minitab Analysis Nested, Split Plot, and Repeated Measure Design
Analysis & Results Explained for Advanced DOE Partly Nested, Split-Plot, Mixed Fixed Random Models
Approximate F test | Pseudo F Test for Advanced Mixed Models nested, split plot, repeated measure
Taguchi Robust Parameter Design
Files used in the video.
Data Used in the Video for Robust Parameter Taguchi Design
How to Construct Taguchi Orthogonal Arrays Bose Design Generator
How to Construct Taguchi Orthogonal Arrays Plackett-Burman Design Generator
Taguchi Linear Graphs Possible Interactions
Taguchi Interaction Table Development How to
Video Demonstrations
Robust parameter Taguchi Design Terms Explained
Introduction To Robust Parameter Taguchi Design of Experiments Analysis Steps Explained
Robust Parameter Taguchi Design Signal to Noise Ratio Calculation in MS Excel
Robust Parameter Taguchi Design Example in MS Excel
Robust Parameter Taguchi Design Example in Minitab
How to Construct Taguchi Orthogonal Array L8(2^7) in MS Excel
How to Construct Taguchi Orthogonal Array L9(3^4) in MS Excel
How to Construct Taguchi Orthogonal Array L16(4^5) in MS Excel ( MS Excel file for the Design )
How to Construct Taguchi Orthogonal Array L16(2^15) in MS Excel
How to Construct Taguchi Orthogonal Array L32(2^31) in MS Excel
Construct Any (Taguchi) Orthogonal Arrays upto L36(2^35) in MS Excel
Taguchi Linear Graphs Explained and How to Use Them
Taguchi Triangular Interactions Table Explained and How to Use them in the Design of Experiments
Taguchi Interaction Table Construction Design of Experiments How to
Taguchi Linear Graphs, Interactions Table, Design Resolution, Alias Structure, & Fractional Factorial Design of Experiments
How to Create Robust Parameter Taguchi Design in Minitab
How to perform Robust Parameter Taguchi Static Analysis in Minitab
How to perform Robust Parameter Taguchi Dynamic Analysis in Minitab
How to perform Robust Parameter Taguchi Dynamic Analysis in MS Excel
Robust Parameter Taguchi Dynamic Analysis Regress Method in MS Excel and Minitab
Recommended Texts
General design of experiments.
[The order is based on the Use of the Book]
Hinkelmann, K., & Kempthorne, O. (2007). Design and Analysis of Experiments, Introduction to Experimental Design (Volume 1) . John Wiley & Sons. ISBN-13: 978-0471727569; ISBN-10: 0471727563.
Hinkelmann, K., & Kempthorne, O. (2005). Design and Analysis of Experiments, Advanced Experimental Design (Volume 2) . John Wiley & Sons. ISBN-13: 978-0471551775; ISBN-10: 0471551775.
Montgomery, D. C. (2012). Design and analysis of experiments 8 th /E. John Wiley & Sons. ISBN-13: 978-1118146927; ISBN-10: 1118146921
Box, G. E., J. S. Hunter, et al. (2005). Statistics for experimenters: design, discovery and innovation, Wiley-Interscience.
Kempthorne, O. (1952). The design and analysis of experiments, John Wiley & Sons Inc.
Fisher, R. A., Bennett, J. H., Fisher, R. A., & Bennett, J. H. (1990). Statistical methods, experimental design, and scientific inference. Oxford University Press. ISBN-10: 0198522290; ISBN-13: 978-0198522294.
Regression & Response Surface
Kutner, M. H., Nachtsheim, C. J., Neter, J., & Li, W. (2013). Applied linear statistical models .
Myers, R. H., Montgomery, D. C., & Anderson-Cook, C. M. (2019). Response surface methodology: Process and product optimization using designed experiments . Hoboken: Wiley.
Robust Parameter Optimization
Taguchi design of experiments.
Kacker, R. N., Lagergren, E. S., & Filliben, J. J. (1991). Taguchi’s orthogonal arrays are classical designs of experiments. Journal of research of the National Institute of Standards and Technology , 96 (5), 577.
Plackett, R. L., & Burman, J. P. (1946). The design of optimum multifactorial experiments. Biometrika , 305-325. (for Video #11)
Taguchi, G., Chowdhury, S., Wu, Y., Taguchi, S., & Yano, H. (2011). Taguchi's quality engineering handbook. Hoboken, N.J: John Wiley & Sons.
Chowdhury, S., & Taguchi, S. (2016). Robust Optimization: World's Best Practices for Developing Winning Vehicles. John Wiley & Sons.
Random-Effect Models, Mixed Models, Nested, Split-Plot & Repeated Measure Design of Experiments
Quinn, G. P., & Keough, M. J. (2014). Experimental design and data analysis for biologists . Cambridge: Cambridge Univ. Press.

- Medical Device Validation
- Quality Assurance / Quality Management
- Product and Process Validation
- TQM Tools and Techniques
- Risk Management Process

Design of Experiments...
Design of experiments…, information & training presentation..

Information & Training.

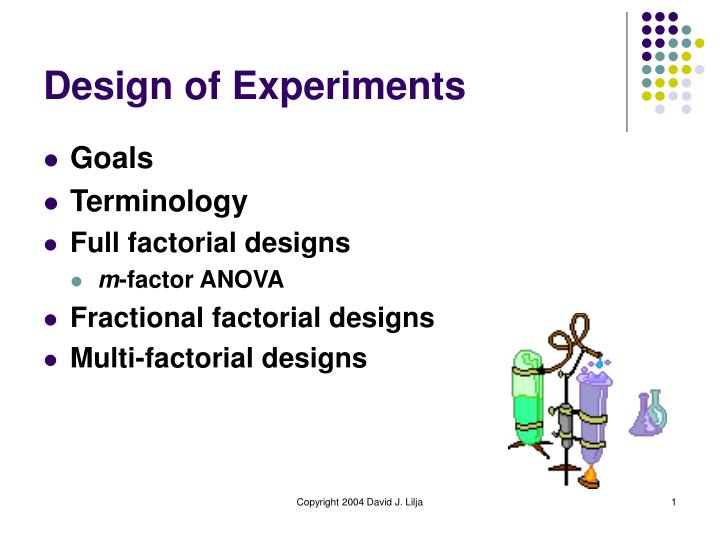
Design of Experiments
Jan 11, 2012
870 likes | 1.36k Views
Design of Experiments. Goals Terminology Full factorial designs m -factor ANOVA Fractional factorial designs Multi-factorial designs. Recall: One-Factor ANOVA. Separates total variation observed in a set of measurements into: Variation within one system Due to random measurement errors
Share Presentation
- ac bc abc copyright
- experimental error copyright
- input factor
- critical f values
- isolate effects
- experimental design

Presentation Transcript
Design of Experiments • Goals • Terminology • Full factorial designs • m-factor ANOVA • Fractional factorial designs • Multi-factorial designs Copyright 2004 David J. Lilja
Recall: One-Factor ANOVA • Separates total variation observed in a set of measurements into: • Variation within one system • Due to random measurement errors • Variation between systems • Due to real differences + random error • Is variation(2) statistically > variation(1)? • One-factor experimental design Copyright 2004 David J. Lilja
ANOVA Summary Copyright 2004 David J. Lilja
Generalized Design of Experiments • Goals • Isolate effects of each input variable. • Determine effects of interactions. • Determine magnitude of experimental error • Obtain maximum information for given effort • Basic idea • Expand 1-factor ANOVA to m factors Copyright 2004 David J. Lilja
Terminology • Response variable • Measured output value • E.g. total execution time • Factors • Input variables that can be changed • E.g. cache size, clock rate, bytes transmitted • Levels • Specific values of factors (inputs) • Continuous (~bytes) or discrete (type of system) Copyright 2004 David J. Lilja
Terminology • Replication • Completely re-run experiment with same input levels • Used to determine impact of measurement error • Interaction • Effect of one input factor depends on level of another input factor Copyright 2004 David J. Lilja
Two-factor Experiments • Two factors (inputs) • A, B • Separate total variation in output values into: • Effect due to A • Effect due to B • Effect due to interaction of A and B (AB) • Experimental error Copyright 2004 David J. Lilja
Example – User Response Time • A = degree of multiprogramming • B = memory size • AB = interaction of memory size and degree of multiprogramming Copyright 2004 David J. Lilja
Two-factor ANOVA • Factor A – a input levels • Factor B – b input levels • n measurements for each input combination • abn total measurements Copyright 2004 David J. Lilja
Two Factors, n Replications n replications Copyright 2004 David J. Lilja
Recall: One-factor ANOVA • Each individual measurement is composition of • Overall mean • Effect of alternatives • Measurement errors Copyright 2004 David J. Lilja
Two-factor ANOVA • Each individual measurement is composition of • Overall mean • Effects • Interactions • Measurement errors Copyright 2004 David J. Lilja
Sum-of-Squares • As before, use sum-of-squares identity SST = SSA + SSB + SSAB + SSE • Degrees of freedom • df(SSA) = a – 1 • df(SSB) = b – 1 • df(SSAB) = (a – 1)(b – 1) • df(SSE) = ab(n – 1) • df(SST) = abn - 1 Copyright 2004 David J. Lilja
Two-Factor ANOVA Copyright 2004 David J. Lilja
Need for Replications • If n=1 • Only one measurement of each configuration • Can then be shown that • SSAB = SST – SSA – SSB • Since • SSE = SST – SSA – SSB – SSAB • We have • SSE = 0 Copyright 2004 David J. Lilja
Need for Replications • Thus, when n=1 • SSE = 0 • → No information about measurement errors • Cannot separate effect due to interactions from measurement noise • Must replicate each experiment at least twice Copyright 2004 David J. Lilja
Example • Output = user response time (seconds) • Want to separate effects due to • A = degree of multiprogramming • B = memory size • AB = interaction • Error • Need replications to separate error Copyright 2004 David J. Lilja
Example Copyright 2004 David J. Lilja
Conclusions From the Example • 77.6% (SSA/SST) of all variation in response time due to degree of multiprogramming • 11.8% (SSB/SST) due to memory size • 9.9% (SSAB/SST) due to interaction • 0.7% due to measurement error • 95% confident that all effects and interactions are statistically significant Copyright 2004 David J. Lilja
Generalized m-factor Experiments Effects for 3 factors: A B C AB AC BC ABC Copyright 2004 David J. Lilja
Degrees of Freedom for m-factor Experiments • df(SSA) = (a-1) • df(SSB) = (b-1) • df(SSC) = (c-1) • df(SSAB) = (a-1)(b-1) • df(SSAC) = (a-1)(c-1) • … • df(SSE) = abc(n-1) • df(SSAB) = abcn-1 Copyright 2004 David J. Lilja
Procedure for Generalized m-factor Experiments • Calculate (2m-1) sum of squares terms (SSx) and SSE • Determine degrees of freedom for each SSx • Calculate mean squares (variances) • Calculate F statistics • Find critical F values from table • If F(computed) > F(table), (1-α) confidence that effect is statistically significant Copyright 2004 David J. Lilja
A Problem • Full factorial design with replication • Measure system response with all possible input combinations • Replicate each measurement n times to determine effect of measurement error • m factors, v levels, n replications → nvm experiments • m = 5 input factors, v = 4 levels, n = 3 • → 3(45) = 3,072 experiments! Copyright 2004 David J. Lilja
Fractional Factorial Designs: n2m Experiments • Special case of generalized m-factor experiments • Restrict each factor to two possible values • High, low • On, off • Find factors that have largest impact • Full factorial design with only those factors Copyright 2004 David J. Lilja
n2m Experiments Copyright 2004 David J. Lilja
Finding Sum of Squares Terms Copyright 2004 David J. Lilja
n2m Contrasts Copyright 2004 David J. Lilja
n2m Sum of Squares Copyright 2004 David J. Lilja
To Summarize --n2m Experiments Copyright 2004 David J. Lilja
Contrasts for n2m with m = 2 factors -- revisited Copyright 2004 David J. Lilja
Contrasts for n2m with m = 3 factors Copyright 2004 David J. Lilja
n2m with m = 3 factors • df(each effect) = 1, since only two levels measured • SST = SSA + SSB + SSC + SSAB + SSAC + SSBC + SSABC • df(SSE) = (n-1)23 • Then perform ANOVA as before • Easily generalizes to m > 3 factors Copyright 2004 David J. Lilja
Important Points • Experimental design is used to • Isolate the effects of each input variable. • Determine the effects of interactions. • Determine the magnitude of the error • Obtain maximum information for given effort • Expand 1-factor ANOVA to m factors • Use n2mdesign to reduce the number of experiments needed • But loses some information Copyright 2004 David J. Lilja
Still Too Many Experiments with n2m! • Plackett and Burman designs (1946) • Multifactorial designs • Effects of main factors only • Logically minimal number of experiments to estimate effects of m input parameters (factors) • Ignores interactions • Requires O(m) experiments • Instead of O(2m) or O(vm) Copyright 2004 David J. Lilja
Plackett and Burman Designs • PB designs exist only in sizes that are multiples of 4 • Requires X experiments for m parameters • X = next multiple of 4 ≥ m • PB design matrix • Rows = configurations • Columns = parameters’ values in each config • High/low = +1/ -1 • First row = from P&B paper • Subsequent rows = circular right shift of preceding row • Last row = all (-1) Copyright 2004 David J. Lilja
PB Design Matrix Copyright 2004 David J. Lilja
PB Design • Only magnitude of effect is important • Sign is meaningless • In example, most → least important effects: • [C, D, E] → F → G → A → B Copyright 2004 David J. Lilja
PB Design Matrix with Foldover • Add X additional rows to matrix • Signs of additional rows are opposite original rows • Provides some additional information about selected interactions Copyright 2004 David J. Lilja
PB Design Matrix with Foldover Copyright 2004 David J. Lilja
Case Study #1 • Determine the most significant parameters in a processor simulator. • [Yi, Lilja, & Hawkins, HPCA, 2003.] Copyright 2004 David J. Lilja
Determine the Most Significant Processor Parameters • Problem • So many parameters in a simulator • How to choose parameter values? • How to decide which parameters are most important? • Approach • Choose reasonable upper/lower bounds. • Rank parameters by impact on total execution time. Copyright 2004 David J. Lilja
Simulation Environment • SimpleScalar simulator • sim-outorder 3.0 • Selected SPEC 2000 Benchmarks • gzip, vpr, gcc, mesa, art, mcf, equake, parser, vortex, bzip2, twolf • MinneSPEC Reduced Input Sets • Compiled with gcc (PISA) at O3 Copyright 2004 David J. Lilja
Functional Unit Values Copyright 2004 David J. Lilja
Memory System Values, Part I Copyright 2004 David J. Lilja
- More by User
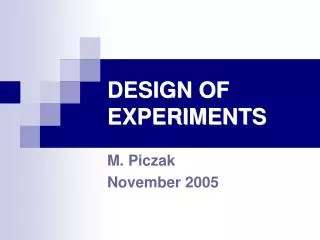
DESIGN OF EXPERIMENTS
DESIGN OF EXPERIMENTS. M. Piczak November 2005. THE ANALYST’S PURPOSES. UNDERSTANDING (a command of general cause and effect relationships associated with a particular phenomenon) EXPLANATION (application of selected relationships to a particular observation)
811 views • 28 slides
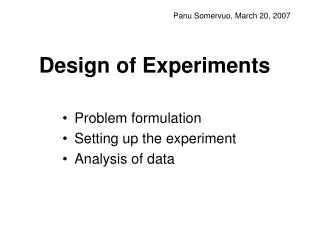
Panu Somervuo, March 20, 2007. Design of Experiments. Problem formulation Setting up the experiment Analysis of data. Problem formulation. what is the biological question? how to answer that? what is already known? what information is missing?
693 views • 36 slides
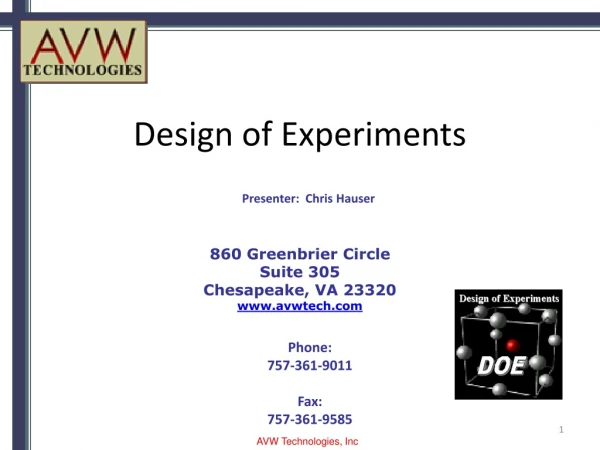
Design of Experiments. Presenter : Chris Hauser. 860 Greenbrier Circle Suite 305 Chesapeake, VA 23320 www.avwtech.com. Phone: 757-361-9011 Fax: 757-361-9585. AVW Technologies, Inc. Why Test?.
518 views • 14 slides
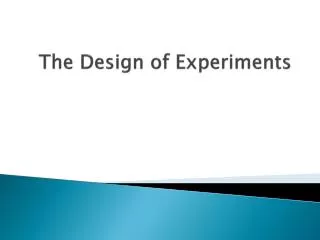
The Design of Experiments
The Design of Experiments.
465 views • 22 slides
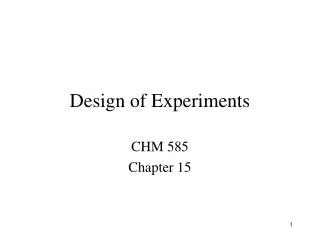
Design of Experiments. CHM 585 Chapter 15. Experimentation is one of the most important methods in the Quality Movement -- the quest for continuous improvement in our products and processes.
271 views • 18 slides
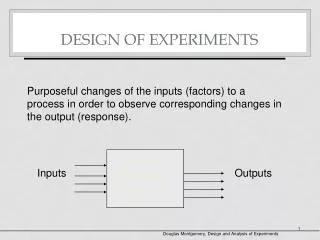
DESIGN OF EXPERIMENTS. Purposeful changes of the inputs (factors) to a process in order to observe corresponding changes in the output (response). Inputs. Outputs. Process. Douglas Montgomery, Design and Analysis of Experiments. Why use DOE ?.
715 views • 24 slides
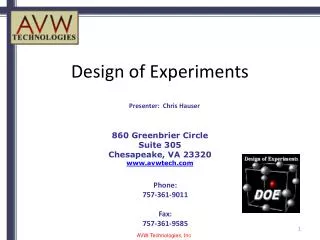
Design of Experiments. Presenter : Chris Hauser. 860 Greenbrier Circle Suite 305 Chesapeake, VA 23320 www.avwtech.com. Phone: 757-361-9011 Fax: 757-361-9585. AVW Technologies, Inc. Why Test? .
211 views • 14 slides
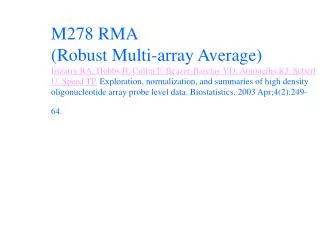
M278 RMA (Robust Multi-array Average) Irizarry RA, Hobbs B, Collin F, Beazer-Barclay YD, Antonellis KJ, Scherf U, Speed TP. Exploration, normalization, and summaries of high density oligonucleotide array probe level data. Biostatistics. 2003 Apr;4(2):249-64. Design of Experiments. 3 data sets
276 views • 19 slides
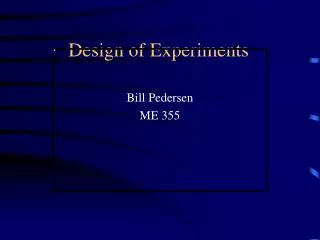
Design of Experiments. Bill Pedersen ME 355. Introduction. DOE Big Picture Concepts and Methodology Applications. What is Design of Experiments?. My Definition: Efficient experimentation methodology. Maximum experimental information for the least expense.
402 views • 21 slides
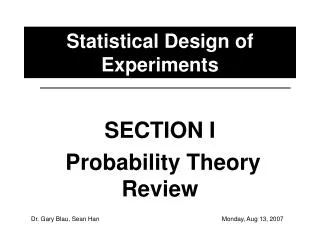
Statistical Design of Experiments
Statistical Design of Experiments. SECTION I Probability Theory Review. PROBABILITY. For any real system or phenomenon, there will be a certain amount of variability associated with data generated by the system.
524 views • 38 slides
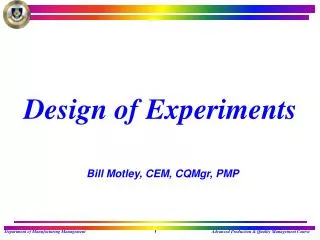
Design of Experiments. Bill Motley, CEM, CQMgr, PMP. Understanding Manufacturing Processes. Theoretical Model -Physical principles understood -Predicts and extrapolates completely -Increased repeatability. Mechanical Model -Empirical
1.28k views • 22 slides
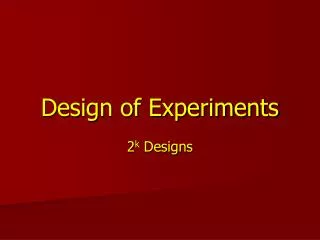
Design of Experiments. 2 k Designs. Catapult Experiment.
954 views • 30 slides
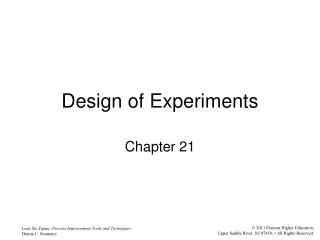
Design of Experiments. Chapter 21. Design Of Experiments. Design of Experiments is a method of experimenting with complex processes with the objective of optimizing the process. Design of Experiments. Dr. Genichi Taguchi (1924- ) Loss Function
2.62k views • 33 slides
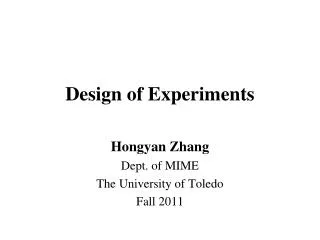
Design of Experiments. Hongyan Zhang Dept. of MIME The University of Toledo Fall 2011. Why is this trip necessary? Goals of the course An abbreviated history of DOX Some basic principles and terminology The strategy of experimentation
232 views • 14 slides
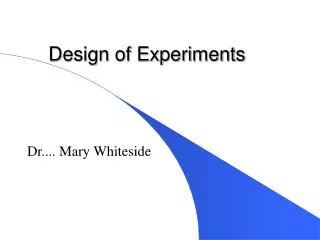
Design of Experiments. Dr.... Mary Whiteside. Experiments. Clinical trials in medicine Taguchi experiments in manufacturing Advertising trials in market research Comparisons of hybrid seeds in agriculture Comparisons of training programs in management Decision making tasks using IS
681 views • 25 slides
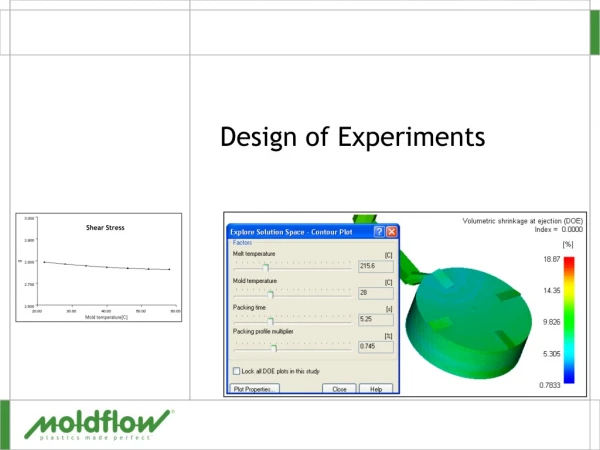
Shear Stress. Design of Experiments. Design of Experiments - DOE. Aim Review theory, setup and interpretation of results for a DOE analysis Why do it Get information on the sensitivity on molding parameters on part quality Overview Setup and run a DOE on a small part to see the process
330 views • 29 slides
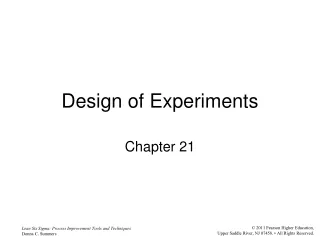
372 views • 33 slides
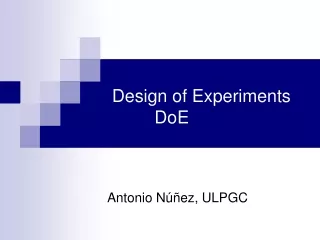
Design of Experiments DoE
Design of Experiments DoE. Antonio Núñez, ULPGC. Objectives of DoE in D&M Processes, Process Investigation, Product and Process Q-improvement, Statistical Inference of KH. Design and conduct engineering experiments involving a single factor with an arbitrary number of levels
127 views • 9 slides
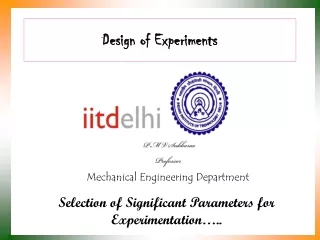
Design of Experiments. P M V Subbarao Professor Mechanical Engineering Department. Selection of Significant Parameters for Experimentation…. Reduced Pressure p R = p/p c. Introductory Remarks. Reduced Temperature T R = T/T c.
473 views • 40 slides
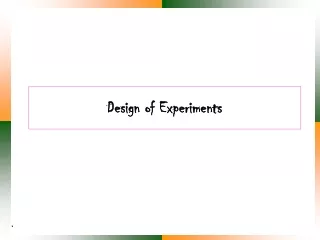
Design of Experiments. Reduced Temperature T R = T/T c. Reduced Pressure p R = p/p c. Introductory Remarks. Many of the thermal experiments involve multi-variable functions. The goal of any experimental activity is to get the maximum realistic information about a system.
446 views • 40 slides
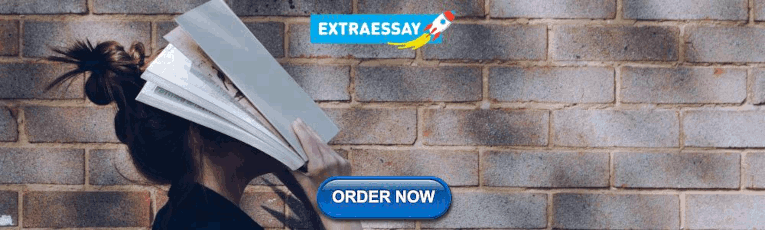
IMAGES
VIDEO
COMMENTS
Upon completion of this lesson, you should be able to: understand the issues and principles of Design of Experiments (DOE), understand experimentation is a process, list the guidelines for designing experiments, and. recognize the key historical figures in DOE. 1.1 - A Quick History of the Design of Experiments (DOE)
Design of experiments. Apr 25, 2017 •. 126 likes • 64,635 views. Upendra K. Approaches to Experimentation What is Design of Experiments Definition of DOE Why DOE History of DOE Basic DOE Example Factors, Levels, Responses General Model of Process or System Interaction, Randomization, Blocking, Replication Experiment Design Process Types of ...
One aspect which is critical to the design is that they be "balanced". A balanced design has an equal number of levels represented for each KPIV. We can confirm this in the design on the right by adding up the number of + and - marks in each column. We see that in each case, they equal 4 + and 4-values, therefore the design is balanced.
Figure 2. Example of the process of research. A designed experiment must satisfy all requirements of the objectives of a study but is also subject to the limitations of available resources. Below we will give examples of how the objective and hypothesis of a study influences the design of an experiment. 1.
The textbook we are using brings an engineering perspective to the design of experiments. We will bring in other contexts and examples from other fields of study including agriculture (where much of the early research was done) education and nutrition. Surprisingly the service industry has begun using design of experiments as well.
Table of contents. Step 1: Define your variables. Step 2: Write your hypothesis. Step 3: Design your experimental treatments. Step 4: Assign your subjects to treatment groups. Step 5: Measure your dependent variable. Other interesting articles. Frequently asked questions about experiments.
Experimental Design Structures. Treatment Structure. Consists of the set of treatments, treatment combinations or populations the experimenter has selected to study and/or compare. Combining the treatment structure and design structure forms an experimental design. The Three R's of Experimental Design. Randomization.
The goal of experimental design is to attain maximum information, precision, and accuracy in the results by efficiently utilizing existing resources. When selecting an experimental design, first and foremost base your choice of design on those designs that will allow you to address your research questions of interest.
Circumscribed (CCC) Designs A i l i t t id th Axial points are outside the design space of a regular full factorial design experiment Advantages: - Highest quality predictions E CCC d i f- Easy to create CCC design from a full factorial experiment Disadvantages: - Axial points may include some unreasonable factor values - E.g. if full ...
The (statistical) design of experiments (DOE) is an efficient procedure for planning experiments so that the data obtained can be analyzed to yield valid and objective DOE begins with determining the objectives of an experiment and selecting the process factors for the study.
8 BASIC STEPS IN DOE Four elements associated with DOE: 1. The design of the experiment, 2. The collection of the data, 3. The statistical analysis of the data, and 4. The conclusions reached and recommendations made as a result of the experiment.
Design of experiments (DOE) is defined as a branch of applied statistics that deals with planning, conducting, analyzing, and interpreting controlled tests to evaluate the factors that control the value of a parameter or group of parameters. DOE is a powerful data collection and analysis tool that can be used in a variety of experimental ...
An experimental design is a detailed plan for collecting and using data to identify causal relationships. Through careful planning, the design of experiments allows your data collection efforts to have a reasonable chance of detecting effects and testing hypotheses that answer your research questions. An experiment is a data collection ...
A Brief Introduction to Design of Experiments Jacqueline K. Telford esign of experiments is a series of tests in which purposeful changes are made to the input variables of a system or pro-cess and the effects on response variables are measured. Design of experiments is applicable to both physical processes and computer simulation models.
The presentations and materials posted to the National Aeronautics and Space Administration Engineering and Safety Center Academy online training are provided for informational purposes only.The use of trademarks, names of manufacturers, or particular items shown or mentioned in the presentations and materials are for identification and example only.
Design. Design: An experimental design consists of specifying the number of experiments, the factor level combinations for each experiment, and the number of replications. In planning an experiment, you have to decide. what measurement to make (the response) what conditions to study. what experimental material to use (the units) Example.
Design and Analysis of Experiments, Introduction to Experimental Design (Volume 1). John Wiley & Sons. John Wiley & Sons. ISBN-13: 978-0471727569; ISBN-10: 0471727563.
Design of Experiments. Shear Stress. Design of Experiments. Design of Experiments - DOE. Aim Review theory, setup and interpretation of results for a DOE analysis Why do it Get information on the sensitivity on molding parameters on part quality Overview Setup and run a DOE on a small part to see the process. 330 views • 29 slides
The Steps in Designing an Experiment • Step 1: Identify the problem or claim to be studied. The statement of the problem needs to be as specific as possible. As your text says, it must "identify the response variable and the population to be studied". • Step 2: Determine the factors affecting the response variable.
Design Of Experiment. Eng. Ibrahim Kuhail. Introduction. Two fundamental approaches to problem solving problems in the discovery of knowledge: Theoretical (physical/mathematical modeling) Experimental measurement ( Most often a combination is used ). Introduction (Cont.).
Presentation Transcript. Design Of Experiments • Design of Experiments is a method of experimenting with complex processes with the objective of optimizing the process. Design Of Experiments • Design of experiments seeks to: • Determine which variables affect the system. • Determine how the magnitude of the variables affects the system.
2) Identify the design centre for each customer need. 3) Measure the current variation from the design centre. 4) Perform Design of Experimentation (DOE) to identify the root causes of variation. 5) Tightly control the root causes. 6) Repeat from step three again, to one order of magnitude improvement. 7) Repeat until you achieve a Cpk of 8 or ...
Virtual reality is a technology with many possible uses and ways to improve various processes, including the presentation of results. This paper deals with the utilization of virtual reality as a tool for assignment presentation. During the classes of manufacturing and assembly systems design, the conventional form of presentation was replaced with immersive virtual reality, where the students ...
870 likes | 1.36k Views. Design of Experiments. Goals Terminology Full factorial designs m -factor ANOVA Fractional factorial designs Multi-factorial designs. Recall: One-Factor ANOVA. Separates total variation observed in a set of measurements into: Variation within one system Due to random measurement errors. Download Presentation.