Recent Advances in Medical Image Processing
Affiliations.
- 1 Hangzhou Zhiwei Information and Technology Inc., Hangzhou, China.
- 2 Hangzhou Zhiwei Information and Technology Inc., Hangzhou, China, [email protected].
- PMID: 33176311
- DOI: 10.1159/000510992
Background: Application and development of the artificial intelligence technology have generated a profound impact in the field of medical imaging. It helps medical personnel to make an early and more accurate diagnosis. Recently, the deep convolution neural network is emerging as a principal machine learning method in computer vision and has received significant attention in medical imaging. Key Message: In this paper, we will review recent advances in artificial intelligence, machine learning, and deep convolution neural network, focusing on their applications in medical image processing. To illustrate with a concrete example, we discuss in detail the architecture of a convolution neural network through visualization to help understand its internal working mechanism.
Summary: This review discusses several open questions, current trends, and critical challenges faced by medical image processing and artificial intelligence technology.
Keywords: Artificial intelligence; Convolution neural network; Deep learning; Medical imaging.
© 2020 S. Karger AG, Basel.
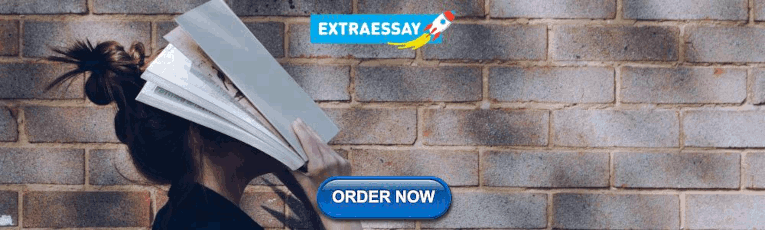
Publication types
- Deep Learning
- Diagnosis, Computer-Assisted*
- Image Interpretation, Computer-Assisted*
- Neural Networks, Computer*
- Predictive Value of Tests
- Reproducibility of Results
- Frontiers in Network Physiology
- Information Theory
- Research Topics
Cutting Edge Advances in Medical Image Analysis and Processing
Total Downloads
Total Views and Downloads
About this Research Topic
Medical Image Processing regards a set of methodologies that have been developed over recent years with the purpose of improving medical image quality, improving medical data visualization, understanding, and assisting medical diagnosis, and so on. Following past years tendency, it is foreseen that these methodologies will increase in complexity and will also have an increasing range of applications. In the last decade, the discipline has undergone a remarkable evolution, with the availability of large volumes of data and the increase in computational power. Deep learning is gaining protagonism and increasing relevance in the medical image processing field and has achieved great success over conventional techniques and is one of the most attractive areas in this field. Despite all the recent advances in medical image analysis in the last decades, there is still a significant uncharted territory and much to be understood in this field mainly due to the daily advances in medical imaging devices. Medical images are typically noisy images due low contrast-to-noise ratio and low spatial and temporal resolution. These characteristics introduce uncertainty in image analysis making it more difficult to quantify information content. Information theory provides theoretical background and tools to quantify information content, and uncertainty, in medical images. This Research Topic aims at presenting the latest advances in medical image processing methodologies and their contribution to the medical field and leveraging research on medical image processing. This Topic intends to cover the development and implementation of new medical image-based algorithms and strategies using biomedical image datasets. The overall aim of this Research Topic is to disclose scientific knowledge on medical image processing and its impacts on the community. This Research Topic welcomes research in the medical image analysis field and intents to promote the dissemination of new research results in the field of medical image processing. We welcome original papers that contribute to medical image understanding through new processing methodologies using image datasets from different medical imaging modalities such as (but not limited to): X-ray Ultrasonography Magnetic resonance (MRI) Computed tomography (CT) Nuclear medicine (PET; SPECT)
Keywords : Segmentation, Image registration, Image denoising, Image visualization, feature extraction and classification, Virtual and augmented reality, Deep Learning, Machine Learning, Network physiology
Important Note : All contributions to this Research Topic must be within the scope of the section and journal to which they are submitted, as defined in their mission statements. Frontiers reserves the right to guide an out-of-scope manuscript to a more suitable section or journal at any stage of peer review.
Topic Editors
Topic coordinators, submission deadlines, participating journals.
Manuscripts can be submitted to this Research Topic via the following journals:
total views
- Demographics
No records found
total views article views downloads topic views
Top countries
Top referring sites, about frontiers research topics.
With their unique mixes of varied contributions from Original Research to Review Articles, Research Topics unify the most influential researchers, the latest key findings and historical advances in a hot research area! Find out more on how to host your own Frontiers Research Topic or contribute to one as an author.
Thank you for visiting nature.com. You are using a browser version with limited support for CSS. To obtain the best experience, we recommend you use a more up to date browser (or turn off compatibility mode in Internet Explorer). In the meantime, to ensure continued support, we are displaying the site without styles and JavaScript.
- View all journals
- Explore content
- About the journal
- Publish with us
- Sign up for alerts
Image processing articles within Scientific Reports
Article 08 April 2024 | Open Access
A novel vector field analysis for quantitative structure changes after macular epiretinal membrane surgery
- Seok Hyun Bae
- , Sojung Go
- & Sang Jun Park
Article 05 April 2024 | Open Access
Advanced disk herniation computer aided diagnosis system
- Maad Ebrahim
- , Mohammad Alsmirat
- & Mahmoud Al-Ayyoub
Article 28 March 2024 | Open Access
Brain temperature and free water increases after mild COVID-19 infection
- Ayushe A. Sharma
- , Rodolphe Nenert
- & Jerzy P. Szaflarski
Article 26 March 2024 | Open Access
High-capacity data hiding for medical images based on the mask-RCNN model
- Hadjer Saidi
- , Okba Tibermacine
- & Ahmed Elhadad
Article 25 March 2024 | Open Access
Integrated image and location analysis for wound classification: a deep learning approach
- , Tirth Shah
- & Zeyun Yu
Article 21 March 2024 | Open Access
A number sense as an emergent property of the manipulating brain
- Neehar Kondapaneni
- & Pietro Perona
Article 16 March 2024 | Open Access
Lesion-conditioning of synthetic MRI-derived subtraction-MIPs of the breast using a latent diffusion model
- Lorenz A. Kapsner
- , Lukas Folle
- & Sebastian Bickelhaupt
Article 14 March 2024 | Open Access
Dual ensemble system for polyp segmentation with submodels adaptive selection ensemble
- , Kefeng Fan
- & Kaijie Jiao
Article 11 March 2024 | Open Access
Generalizable disease detection using model ensemble on chest X-ray images
- Maider Abad
- , Jordi Casas-Roma
- & Ferran Prados
Article 08 March 2024 | Open Access
Segmentation-based cardiomegaly detection based on semi-supervised estimation of cardiothoracic ratio
- Patrick Thiam
- , Christopher Kloth
- & Hans A. Kestler
Article 05 March 2024 | Open Access
Brain volume measured by synthetic magnetic resonance imaging in adult moyamoya disease correlates with cerebral blood flow and brain function
- Kazufumi Kikuchi
- , Osamu Togao
- & Kousei Ishigami
Article 04 March 2024 | Open Access
Critical evaluation of artificial intelligence as a digital twin of pathologists for prostate cancer pathology
- Okyaz Eminaga
- , Mahmoud Abbas
- & Olaf Bettendorf
Computational pathology model to assess acute and chronic transformations of the tubulointerstitial compartment in renal allograft biopsies
- Renaldas Augulis
- , Allan Rasmusson
- & Arvydas Laurinavicius
Opportunistic screening with multiphase contrast-enhanced dual-layer spectral CT for osteoblastic lesions in prostate cancer compared with bone scintigraphy
- Ming-Cheng Liu
- , Chi-Chang Ho
- & Yi-Jui Liu
Article 02 March 2024 | Open Access
Reduction of NIFTI files storage and compression to facilitate telemedicine services based on quantization hiding of downsampling approach
- Ahmed Elhadad
- , Mona Jamjoom
- & Hussein Abulkasim
Article 29 February 2024 | Open Access
Attention-guided jaw bone lesion diagnosis in panoramic radiography using minimal labeling effort
- Minseon Gwak
- , Jong Pil Yun
- & Chena Lee
End-to-end multimodal 3D imaging and machine learning workflow for non-destructive phenotyping of grapevine trunk internal structure
- Romain Fernandez
- , Loïc Le Cunff
- & Cédric Moisy
Article 27 February 2024 | Open Access
An improved V-Net lung nodule segmentation model based on pixel threshold separation and attention mechanism
- , Handing Song
- & Zhan Wang
Article 26 February 2024 | Open Access
Quantifying mangrove carbon assimilation rates using UAV imagery
- Javier Blanco-Sacristán
- , Kasper Johansen
- & Matthew F. McCabe
Article 24 February 2024 | Open Access
Iterative pseudo balancing for stem cell microscopy image classification
- Adam Witmer
- & Bir Bhanu
Article 22 February 2024 | Open Access
Deep learning-based, fully automated, pediatric brain segmentation
- Min-Jee Kim
- , EunPyeong Hong
- & Tae-Sung Ko
Article 21 February 2024 | Open Access
Correction of high-rate motion for photoacoustic microscopy by orthogonal cross-correlation
- , Qiuqin Mao
- & Xiaojun Liu
Article 20 February 2024 | Open Access
ERCP-Net: a channel extension residual structure and adaptive channel attention mechanism for plant leaf disease classification network
- & Yannan Xu
A quality grade classification method for fresh tea leaves based on an improved YOLOv8x-SPPCSPC-CBAM model
- Xiu’yan Zhao
- , Yu’xiang He
- & Kai’xing Zhang
Article 16 February 2024 | Open Access
Stripe noise removal in conductive atomic force microscopy
- , Jan Rieck
- & Michael H. F. Wilkinson
Article 13 February 2024 | Open Access
Automatic enhancement preprocessing for segmentation of low quality cell images
- & Kazuhiro Hotta
Article 09 February 2024 | Open Access
An artificial intelligence based abdominal aortic aneurysm prognosis classifier to predict patient outcomes
- Timothy K. Chung
- , Pete H. Gueldner
- & David A. Vorp
Article 08 February 2024 | Open Access
Application of PET imaging delta radiomics for predicting progression-free survival in rare high-grade glioma
- Shamimeh Ahrari
- , Timothée Zaragori
- & Antoine Verger
Cluster-based histopathology phenotype representation learning by self-supervised multi-class-token hierarchical ViT
- , Shivam Kalra
- & Mohammad Saleh Miri
Article 03 February 2024 | Open Access
YOLOX target detection model can identify and classify several types of tea buds with similar characteristics
- Mengdao Yang
- , Weihao Yuan
- & Gaojian Xu
Phenotypic characterization of liver tissue heterogeneity through a next-generation 3D single-cell atlas
- Dilan Martínez-Torres
- , Valentina Maldonado
- & Fabián Segovia-Miranda
Article 30 January 2024 | Open Access
Machine learning approaches for early detection of non-alcoholic steatohepatitis based on clinical and blood parameters
- Amir Reza Naderi Yaghouti
- , Hamed Zamanian
- & Ahmad Shalbaf
Research on improved black widow algorithm for medical image denoising
- & Lina Zhang
Article 25 January 2024 | Open Access
Methodology of generation of CFD meshes and 4D shape reconstruction of coronary arteries from patient-specific dynamic CT
- Krzysztof Psiuk-Maksymowicz
- , Damian Borys
- & Ryszard A. Bialecki
Article 23 January 2024 | Open Access
Comparison between a deep-learning and a pixel-based approach for the automated quantification of HIV target cells in foreskin tissue
- Zhongtian Shao
- , Lane B. Buchanan
- & Jessica L. Prodger
Task design for crowdsourced glioma cell annotation in microscopy images
- Svea Schwarze
- , Nadine S. Schaadt
- & Friedrich Feuerhake
Article 20 January 2024 | Open Access
Unlocking cardiac motion: assessing software and machine learning for single-cell and cardioid kinematic insights
- Margherita Burattini
- , Francesco Paolo Lo Muzio
- & Michele Miragoli
Article 19 January 2024 | Open Access
Microstructural brain abnormalities, fatigue, and cognitive dysfunction after mild COVID-19
- Lucas Scardua-Silva
- , Beatriz Amorim da Costa
- & Clarissa Lin Yasuda
Article 18 January 2024 | Open Access
Validation of reliability, repeatability and consistency of three-dimensional choroidal vascular index
- , Yifan Bai
- & Qingli Shang
Integrated image and sensor-based food intake detection in free-living
- Tonmoy Ghosh
- & Edward Sazonov
Article 16 January 2024 | Open Access
Early stage black pepper leaf disease prediction based on transfer learning using ConvNets
- Anita S. Kini
- , K. V. Prema
- & Smitha N. Pai
GPU-accelerated lung CT segmentation based on level sets and texture analysis
- Daniel Reska
- & Marek Kretowski
Article 12 January 2024 | Open Access
Accuracy of an AI-based automated plate reading mobile application for the identification of clinical mastitis-causing pathogens in chromogenic culture media
- Breno Luis Nery Garcia
- , Cristian Marlon de Magalhães Rodrigues Martins
- & Marcos Veiga dos Santos
Crowdsourced human-based computational approach for tagging peripheral blood smear sample images from Sickle Cell Disease patients using non-expert users
- José María Buades Rubio
- , Gabriel Moyà-Alcover
- & Nataša Petrović
Article 09 January 2024 | Open Access
Identification of wheel track in the wheat field
- Wanhong Zhang
Article 04 January 2024 | Open Access
Multi scale-aware attention for pyramid convolution network on finger vein recognition
- Huijie Zhang
- , Weizhen Sun
- & Ling Lv
Article 03 January 2024 | Open Access
Rapid artefact removal and H&E-stained tissue segmentation
- B. A. Schreiber
- , J. Denholm
- & E. J. Soilleux
Article 02 January 2024 | Open Access
UNet based on dynamic convolution decomposition and triplet attention
- & Limei Fang
Multi-pose-based convolutional neural network model for diagnosis of patients with central lumbar spinal stenosis
- Seyeon Park
- , Jun-Hoe Kim
- & Chun Kee Chung
Article 21 December 2023 | Open Access
Deep learning framework for automated goblet cell density analysis in in-vivo rabbit conjunctiva
- Seunghyun Jang
- , Seonghan Kim
- & Ki Hean Kim
Browse broader subjects
- Computational biology and bioinformatics
Quick links
- Explore articles by subject
- Guide to authors
- Editorial policies

Advertisement
Convolutional neural networks in medical image understanding: a survey
- Review Article
- Published: 03 January 2021
- Volume 15 , pages 1–22, ( 2022 )
Cite this article
- D. R. Sarvamangala ORCID: orcid.org/0000-0003-2411-3336 1 &
- Raghavendra V. Kulkarni 2
45k Accesses
264 Citations
4 Altmetric
Explore all metrics
Imaging techniques are used to capture anomalies of the human body. The captured images must be understood for diagnosis, prognosis and treatment planning of the anomalies. Medical image understanding is generally performed by skilled medical professionals. However, the scarce availability of human experts and the fatigue and rough estimate procedures involved with them limit the effectiveness of image understanding performed by skilled medical professionals. Convolutional neural networks (CNNs) are effective tools for image understanding. They have outperformed human experts in many image understanding tasks. This article aims to provide a comprehensive survey of applications of CNNs in medical image understanding. The underlying objective is to motivate medical image understanding researchers to extensively apply CNNs in their research and diagnosis. A brief introduction to CNNs has been presented. A discussion on CNN and its various award-winning frameworks have been presented. The major medical image understanding tasks, namely image classification, segmentation, localization and detection have been introduced. Applications of CNN in medical image understanding of the ailments of brain, breast, lung and other organs have been surveyed critically and comprehensively. A critical discussion on some of the challenges is also presented.
Similar content being viewed by others
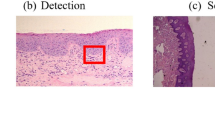
Survey of Supervised Learning for Medical Image Processing
Abeer Aljuaid & Mohd Anwar
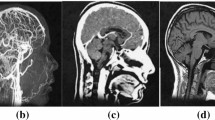
Convolutional Neural Network in Medical Image Analysis: A Review
Sapna Singh Kshatri & Deepak Singh
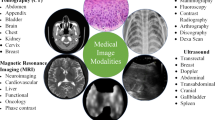
Medical Image Analysis using Convolutional Neural Networks: A Review
Syed Muhammad Anwar, Muhammad Majid, … Muhammad Khurram Khan
Avoid common mistakes on your manuscript.
1 Introduction
Loss of human lives can be prevented or the medical trauma experienced in an injury or a disease can be reduced through the timely diagnosis of medical anomalies. Medical anomalies include glaucoma, diabetic retinopathy, tumors [ 34 ], interstitial lung diseases [ 44 ], heart diseases and tuberculosis. Diagnosis and prognosis involve the understanding of the images of the affected area obtained using X-ray, magnetic resonance imaging (MRI), computed tomography (CT), positron emission tomography (PET), single photon emission computed tomography or ultrasound scanning. Image understanding involves the detection of anomalies, ascertaining their locations and borders, and estimating their sizes and severity. The scarce availability of human experts and their fatigue, high consultation charges and rough estimate procedures limit the effectiveness of image understanding. Further, shapes, locations and structures of the medical anomalies are highly variable [ 55 ]. This makes diagnosis difficult even for specialized physicians [ 4 ]. Therefore, human experts often feel a need for support tools to aid in precise understanding of medical images. This is the motivation for intelligent image understanding systems.
Image understanding systems that exploit machine learning (ML) techniques are fast evolving in recent years. ML techniques include decision tree learning [ 35 ], clustering, support vector machines (SVMs) [ 47 ], k-means nearest neighbor (K-NN), restricted Boltzmann machines (RBMs) [ 42 ] and random forests (RFs) [ 28 ]. The pre-requisite for ML techniques to work efficiently is the extraction of discriminant features. And these features are generally unknown and is also a very challenging task especially for applications involving image understanding and is still a topic of research. A logical step to overcome was to create intelligent machines which could learn features needed for image understanding and extract it on its own. One such intelligent and successful model is the convolutional neural network (CNN) model, which automatically learns the needed features and extracts it for medical image understanding. The CNN model is made of convolutional filters whose primary function is to learn and extract necessary features for efficient medical image understanding. CNN started gaining popularity in the year 2012, due to AlexNet [ 41 ], a CNN model, which defeated all the others models with a record accuracy and low error rate in imageNet challenge 2012. CNN has been used by corporate giants for providing internet services, automatic tagging in images, product recommendations, home feed personalization and autonomous cars [ 59 ]. The major applications of the CNN are in image and signal processing, natural language processing and data analytics. The CNN had a major breakthrough when GoogleNet used it to detect cancer at an accuracy of 89% while human pathologists could achieve the accuracy of only 70% [ 3 ].
1.1 Motivation and purpose
CNNs have contributed significantly in the areas of image understanding. CNN-based approaches are placed in the leader board of the many image understanding challenges, such as Medical Image Computing and Computer Assisted Intervention (MICCAI) biomedical challenge, Brain Tumor segmentation (BRATS) Multimodal Brain Tumor Segmentation challenge [ 48 ], Imagenet classification challenge, challenges of International Conference on Pattern Recognition (ICPR) [ 31 ] and Ischemic Stroke Lesion Segmentation (ISLES) challenge [ 32 ]. CNN has become a powerful choice as a technique for medical image understanding. Researchers have successfully applied CNNs for many medical image understanding applications like detection of tumors and their classification into benign and malignant [ 52 ], detection of skin lesions [ 50 ], detection of optical coherence tomography images [ 39 ], detection of colon cancer [ 71 ], blood cancer, anomalies of the heart [ 40 ], breast [ 36 ], chest, eye etc. Also CNN-based models like CheXNet [ 56 , 58 ], used for classifying 14 different ailments of the chest achieved better results compared to the average performance of human experts.
CNNs have also dominated the area of COVID-19 detection using chest X-rays/CT scans. Research involving CNNs is now a dominant topic at major conferences. In addition, there are special issues reserved in reputed journals for solving challenges using deep learning models. The vast amount of literature available on CNNs is the testimonial of their efficiency and the widespread use. However, various research communities are developing these applications concurrently and the dissemination results are scattered in a wide and diverse range of conference proceedings and journals.
A large number of surveys on deep learning have been published recently. A review of deep learning techniques applied in medical imaging, bioinformatics and pervasive sensing has been presented in [ 60 ]. A thorough review of deep learning techniques for segmentation of MRI images of brain has been presented in [ 2 ]. Survey of deep learning techniques for medical image segmentation, their achievements and challenges involved in medical image segmentation has been presented in [ 27 ] Though literature is replete with many survey papers, most of them concentrate on deep learning models which include CNN, recurrent neural network, generative adversial network or on a particular application. There is also no coverage of the application of CNN in early detection of COVID-19 as well as many other areas.
The survey includes research papers on various applications of CNNs in medical image understanding. The papers for the survey are queried from various journal websites. Additionally, arxiv, conference proceedings of various medical image challenges are also included in the survey. Also the references of these papers are checked. The query used are: “CNN” or “deep learning” or “convolutional neural network” or terms related to medical image understanding. These terms had to be present either in title or abstract to be considered.
The objective of this survey is to offer a comprehensive overview of applications and methodology of CNNs and its variants, in the fields of medical image understanding including the detection of latest global pandemic COVID-19. The survey includes overview tables which can be used for quick reference. The authors leverage experiences of their own and that of the research fraternity on the applications of CNNs to provide an insight into various state of the art CNN models, challenges involved in designing CNN model, overview of research trends in the field, and to motivate medical image understanding researchers and medical professionals to extensively apply CNNs in their research and diagnosis, respectively.
1.2 Contributions and the structure
Primary contributions of this article are as follows:
To briefly introduce medical image understanding and CNN.
To convey that CNN has percolated in the field of medical image understanding.
To identify the various challenges in medical image understanding.
To highlight contributions of CNN to overcome those challenges
The remainder of this article has been organized as follows: Medical image understanding has been briefly introduced in Sect. 2 . A brief introduction of CNN and its architecture has been presented in Sect. 3 . The applications of CNN in medical image understanding have been surveyed comprehensively through Sects. 4 – 7 . Finally, concluding remarks and a projection of the trends in CNN applications in image understanding have been presented in Sect. 8 .
2 Medical image understanding
Medical imaging is necessary for the visualization of internal organs for the detection of abnormalities in their anatomy or functioning. Medical image capturing devices, such as X-ray, CT, MRI, PET and ultrasound scanners capture the anatomy or functioning of the internal organs and present them as images or videos. The images and videos must be understood for the accurate detection of anomalies or the diagnosis of functional abnormalities. If an abnormality is detected, then its exact location, size and shape must be determined. These tasks are traditionally performed by the trained physicians based on their judgment and experience. Intelligent healthcare systems aim to perform these tasks using intelligent medical image understanding. Medical image classification, segmentation, detection and localization are the important tasks in medical image understanding.
2.1 Medical image classification
Medical image classification involves determining and assigning labels to medical images from a fixed set. The task involves the extraction of features from the image, and assigning labels using the extracted features. Let I denote an image made of pixels and \(c_1, c_2, \ldots , c_r\) denote the labels. For each pixel x , a feature vector \(\zeta \) , consisting of values \(f(x_i)\) is extracted from the neighborhood N ( x ) using ( 1 ), where \(x_i \in N(x)\) for \(i = 0, 1, \ldots , k\) .
A label from the list of labels \(c_1, c_2, \ldots , c_r\) is assigned to the image based on \(\zeta \) .
2.2 Medical image segmentation
Medical image segmentation helps in image understanding, feature extraction and recognition, and quantitative assessment of lesions or other abnormalities. It provides valuable information for the analysis of pathologies, and subsequently helps in diagnosis and treatment planning. The objective of segmentation is to divide an image into regions that have strong correlations. Segmentation involves dividing the image I into a finite set of regions \(R_1, R_1, \ldots , R_S\) as expressed in ( 2 ).
2.3 Medical image localization
Automatic localization of pathology in images is quite an important step towards automatic acquisition planning and post imaging analysis tasks, such as segmentation and functional analysis. Localization involves predicting the object in an image, drawing a bounding box around the object and labeling the object.
The localization function f ( I ) on an image I computes \(c, \ l_x, \ l_y, \ l_w, l_h \) , which represent respectively, class label, centroid x and y coordinates, and the proportion of the bounding box with respect to width and height of the image as expressed in ( 3 ).
2.4 Medical image detection
Image detection aims at the classification and the localization of regions of interest by drawing bounding boxes around multiple regions of interest and labeling them. This helps in determining the exact locations of different organs and their orientation. Let I be an image with n objects or regions of interest. Then detection function D ( I ) computes \(c_i, \ x_i, \ y_i, \ w_i, h_i\) and these are respectively the class label, centroid x and y coordinates, proportion of the bounding box with respect to width and height of the image I as given in the ( 4 )
2.5 Evaluation metrics for image understanding
There are many metrics used for the evaluation of performance of medical image understanding algorithms. The confusion matrix, also known as the error matrix, is the table used for visualizing the performance of an algorithm and for calculation of various evaluation metrics. It provides an insight about the types of errors that are made by the classifier. It is a square matrix in which rows represent the instances of actual results and the columns represent the instances of predicted results of the algorithm. The confusion matrix of a binary classifier is shown in Table 1 .
Here, \(T_P\) indicates correctly identified positives, \(T_N\) indicates correctly identified negatives, \(F_P\) indicates incorrectly identified positives and \(F_N\) indicates incorrectly identified negatives. \(F_P\) is also known as false error and \(F_N\) is known as miss. The sum of correct and incorrect predictions is represented as T and expressed as in ( 5 ).
Performance metrics can be determined with the help of confusion matrix and are given in Table 2 .
3 A brief introduction to CNNs
Image understanding by animals is a very fascinating process, and a very simple task for them. But for a machine, to understand an image, there are lot of hidden complexities during the process. What animals feel is the eyes capturing the image, which is processed by the neurons and sent to the brain for interpretation. CNN is a deep learning algorithm inspired by the visual cortex of animal brain [ 30 ] and aims to imitate the visual machinery of animals. CNNs represents a quantum leap in the field of image understanding, involving image classification, segmentation, localization, detection etc. The efficacy of CNNs in image understanding is the main reason of its abundant use. CNNs are made of convolutions having learnable weights and biases similar to neurons (nerve cells) of the animal. Convolutional layers, activation functions, pooling and fully-connected layers are the core building blocks of CNNs, as depicted in Fig. 1 . Very brief introduction to CNNs has been presented in this paper. Detailed discussions on CNNs are presented in [ 9 , 41 ].
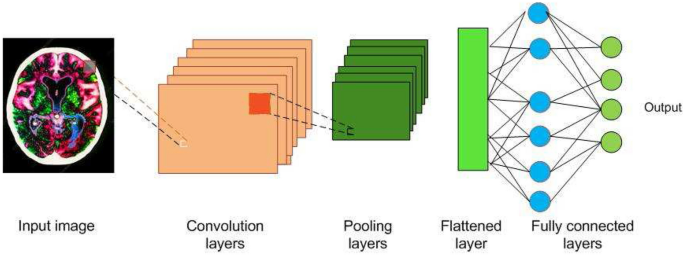
Building blocks of a CNN
3.1 Convolution layers (Conv layers)
The visual cortex of the animal brain is made of neuronal cells which extract features of the images. Each neuronal cell extracts different features, which help in image understanding. The conv layer is modeled over the neuronal cells and its objective is to extract features, such as edges, colors, texture and gradient orientation. Conv layers are made of learnable filters called convolutional filters, or kernels, of size \(n\times m\times d\) , where d is the depth of the image. During the forward pass, the kernels are convolved across the width and height of input volume and dot product is computed between the entries of the filter and the input. Intuitively, the CNN learns filters that gets activated when they come across edge, colors, texture etc. The output of the conv layer is fed into an activation function layer.
3.2 Activation functions or nonlinear functions
Since data in real world is mostly nonlinear, activation functions are used for nonlinear transformation of the data. It is used to ensure that the representation in the input space is mapped to a different output space as per the requirements. The different activation functions are discussed in Sects. 3.2.1 – 3.2.3 .
3.2.1 Sigmoid
It takes a real-valued number x and squashes it into range between 0 and 1. In particular, large negative and positive inputs are placed very close to 0 and unity, respectively. It is expressed as in ( 6 ).
3.2.2 Tan hyperbolic
It takes a real valued number x and squashes it between \(-1\) to 1 as expressed in ( 7 ).
3.2.3 Rectified linear unit (ReLU)
This nonlinear function takes a real valued number x and converts x to 0 if x is negative. ReLU is the most often used nonlinear function for CNN, takes less computation time and hence faster compared to the other two and is expressed in ( 8 ).
3.3 Pooling
Pooling layer performs a nonlinear down sampling of convolved feature. It decreases the computational power required to process the data through dimensionality reduction. It reduces the spatial size by aggregating data over space or feature type, controls overfitting and overcomes translation and rotational variance of images. Pooling operation results in partitioning of its input into a set of rectangle patches. Each patch gets replaced by a single value depending on the type of pooling selected. The different types are maximum pooling and average pooling.
3.4 Fully connected (FC) layer
FC layer is similar to artificial neural network, where each node has incoming connections from all the inputs and all the connections have weights associated with them. The output is sum of all the inputs multiplied by the corresponding weights. FC layer is followed by sigmoid activation function and performs the classifier job.
3.5 Data preprocessing and augmentation
The raw images obtained from imaging modalities need to be preprocessed and augmented before sending to CNN. The raw image data might be skewed, altered by bias distortion [ 55 ], having intensity inhomogeneity during capture, and hence needs to be preprocessed. Multiple data preprocessing methods exist and the preferred methods are mean subtraction and normalization. CNN needs to be trained on a larger dataset to achieve the best performance. Data augmentation increases the existing set of images by horizontal and vertical flips, transformations, scaling, random cropping, color jittering and intensity variations. The preprocessed, augmented image data is then fed into CNN.
3.6 CNN architectures and frameworks
Many CNN architectures have been proposed by researchers depending on kind of task to be performed. A few award-winning architectures are listed in Table 3 . CNN frameworks (toolkits) enable the efficient development and implementation of deep learning methods. Various frameworks used by researchers and developers is listed in Table 4 .
4 CNN applications in medical image classification
4.1 lung diseases.
Interstitial lung disease (ILD) is the disorder of lung parenchyma in which lung tissues get scarred leading to respiratory difficulty. High resolution computed tomography (HRCT) imaging is used to differentiate between different types of ILDs. HRCT images have a high visual variation between different classes and high visual similarity within the same class. Therefore, accurate classification is quite challenging.
4.1.1 Ensemble CNN
Ensemble of rf and overfeat for classification of pulmonary peri fissural nodules of lungs was proposed in [ 14 ]. The complexity of the input was reduced by extracting two-dimensional views from three-dimensional volume. The performance was enhanced by using a combination of overfeat followed by rf. The bagging technique of rf boosted the performance of the model. The proposed model obtained an AUC of \(86.8\%\) .
4.1.2 Small-kernel CNN
Low level textual information and more non linear activations enhances performance of classification was emphasized by [ 4 ]. The authors shrinked the kernel size to \(2\times 2\) to involve more non linear activations. The receptive fields were kept smaller to capture low level textual information. Also, to handle increasing complexity of the structures, the number of kernels were made proportional to the number of receptive field of its neurons. The model classified the lung tissue image into seven classes (a healthy tissue and six different ILD patterns). The results were compared against AlexNet and VGGNet and the ROC curves. The structure took only 20 s to classify the whole lung area in 30 slices of an average size HRCT scan image. AlexNet and VGG-Net took 136 s and 160 s for classification. The model delivered a classification accuracy of \(85\%\) , while the traditional methods delivered an accuracy of \(78\%\) .
4.1.3 Whole image CNN
Smaller image patches to prevent loss of spatial information and different attenuation ranges to enhance better visibility was proposed in [ 18 ]. Since the images were RGB, the proposed CNN model used three lung attenuation ranges namely, lower attenuation, normal attenuation and higher attenuation. To avoid overfitting, the images were augmented by jitter and cropping. A simple Alexnet model with the above variations was implemented and compared against other CNN models implemented to work on image patches. The performance metrics were accuracy and F-score. The model obtained an F-score of \(100\%\) and the average accuracy of \(87.9\%\) .
4.1.4 Multicrop pooling CNN
Limitation of reduced training samples can be overcome by extraction of salient multi scale features. The features were extracted using multicrop pooling for automatic lung nodule malignancy suspicious classification in [ 68 ]. The model was a simple 3 layered CNN architecture but with multicrop pooling and randomized leaky ReLu as activation. The proposed method obtained accuracy and AUC of \(87.4\%\) and \(93\%\) . Fivefold cross validation was used for evaluation.
The CNN applications in lung classification is summarized in Table 5 .
4.2 Coronavirus disease 2019 (COVID-19)
COVID-19 is a global pandemic disease spreading rapidly around the world. Reverse Transcription Polymerase Chain Reaction (RT-PCR) is a commonly employed test for detection of COVID-19 infection. RT-PCR testing is the gold standard for COVID-19 testing, RT-PCR is very complicated, time-consuming and labor-intensive process, sparse availability and not very accurate. Chest X-ray could be used for the initial screening of the COVID-19 in places having shortage of RT-PCR kits and is more accurate at diagnosis. Many researchers have used deep learning to classify if the chest infection is due to COVID-19 or other ailments.
4.2.1 Customized CNN
One of the initial model proposed for detection of COVID-19 was a simple pretrained AlexNet model proposed in [ 45 ] and fine tuned on chest X-ray images. The results were very promising with accuracy of classifying positive and negative patients of around \(95\%\) . Use of pretrained with transfer learning ResNet and InceptionNet CNN models were also proposed. These models demonstrated that the transfer learning models were also efficient and achieved a test accuracy of \(93\%\) .
4.2.2 Bayesian CNN
Uncertainty was explored to enhance the diagnostic performance of classification of COVID-19 datasets in [ 22 ]. The primary aim of proposed method was to avoid COVID-19 misdiagnoses. The method explored Monte-Carlo Dropweights Bayesian CNN to estimate uncertainty in deep learning, to better the diagnostic performance of human-machine decisions. The method showed that there is a strong correlation between classification accuracy and estimated uncertainty in predictions. The proposed method used ResNet50v2 model. The softmax layer was preceded by dropweights. Dropweights were applied as an approximation to the Gaussian Process, which was used to estimate meaningful model uncertainty. The softmax layer finally outputs each possible class label’s probability distribution.
4.2.3 PDCOVIDNET
Use of dilation to detect dominant features in the image was explored in [ 13 ]. The authors proposed parallel dilated CNN model. The dilated module involved skipping of pixels during convolution process. Parallel CNN branches are proposed with different dilation rates. The features obtained from parallel branches were concatenated and input to the next convolution layer. Concatenation-convolution operation was used to explore feature relationship of dilated convolutions so as to detect dominant features for classification. The model also used Grad-CAM and Grad-CAM++ to highlight the regions of class-discriminative saliency maps. The performance metrics used were accuracy, precision, recall, F1-score with ROC/AUC and are \(96.58\%, 95\%, 91\%, 93\%\) and 0.991 respectively.
4.2.4 CVR-Net
To prevent degrading of final prediction and to compensate for lesser number of datasets, multi scale multi encoder ensemble CNN model for classification of COVID-19 was proposed in [ 24 ]. The proposed model ensembled feature maps at different scales obtained from different encoders. To avoid overfitting, geometry based image augmentations and transfer learning was proposed. To overcome vanishing gradients, each encoder consisted of residual and convolutional blocks to allow gradients to pass, like in resNet architecture. Moreover Depth-wise separable convolution was used to create a light weight network. The depth information of feature map was enhanced by concatenating different 2D feature maps of different encoders in channel-wise. The performance metrics for classifying images into positive and negative were recall, precision, f1-score and accuracy. The model showed a very efficient performance with score of nearly \(98\%\) for all the metrics.
4.2.5 Twice transfer learning CNN
A denseNet model trained twice using transfer learning approach was proposed in [ 6 ]. The denseNet201 model was trained initially on imageNet dataset , followed by chest X-ray 14 dataset and then fine tuned on COVID-19 dataset. Various combinations of training the model first with single transfer learning, twice transfer learning, twice transfer learning with output neuron keeping were experimented. The model with twice transfer learning with output neuron keeping achieved the best performance accuracy of \(98.9\%\) over the other models. Transfer learning on chest X-ray 14 dataset enhanced the result, as the model had learnt most of the features related to chest abnormalities.
4.3 Immune response abnormalities
Autoimmune diseases result from an abnormal immune response to a normal body part. The immune system of the body attacks the healthy cells in such diseases. Indirect immunofluorescence (IIF) on human epithelial-2 (HEp-2) cells is used to diagnose an autoimmune disease. Manual identification of these patterns is a time-consuming process.
4.3.1 CUDA ConvNet CNN
Preprocessing using histogram equalization and zero-mean with unit variance increases classification accuracy by an additional \(10\%\) with augmentation was proposed in [ 7 ]. The experiments also demonstrate that pretraining, followed by fine tuning boosts performance. It achieved an average classification accuracy of \(80.3\%\) which was greater than the previous best of \(75.6\%\) . The authors used Caffe library [ 33 ] and CUDA ConvNet model architecture to extract CNN-based features for classification of HEp-2 cells.
4.3.2 Six-layer CNN
Preprocessing and augmentation enhanced the mean classification accuracy of HEp-2 cell images and was shown in [ 21 ]. The framework consisted of three stages of image preprocessing, network training and feature extraction with classification. Mean classification accuracy of \(96.7\%\) on ICPR-2012 dataset was obtained. The CNN approaches for HEp-2 cell classification are summarized in Table 6 .
4.4 Breast tumors
Breast cancer is the most common cancer that affects women across the world. It can be detected by the analysis of mammographs. Two radiologists independently reading the same mammogram has been advocated to overcome any misjudgement.
4.4.1 Stopping monitoring CNN
Stopping monitoring to reduce the computation time is proposed in [ 52 ]. Stopping monitoring was performed using AUC on validation set. The model extracted region of interest (ROI) by cropping. The images were augmented to increase the number of samples and also to prevent overfitting. The CNN model proposed was for classification of breast tumors. The result was compared against the state-of-the-art image descriptors HOG and HOG divergence. The proposed method resulted in AUC of \(82.2\%\) compared to \(78.7\%\) for other methods.
4.4.2 Ensemble CNN
Fine tuning enhanced the performance in the case of limited dataset was proposed in [ 34 ]. The proposed model was similar to AlexNet and was pretrained on imagenet, followed by fine tuning on breast images due to shortage of breast images. The middle and high level features were extracted from different layers of the network and fed into SVM classifiers for training. The model classified the breast masses into malignant and benign. Due to extraction of efficient features by deep network, simple classifier also resulted in accuracy of \(96.7\%\) . The proposed method was compared against bag-of-words, HOG and SIFT and it outperformed all of them.
4.4.3 Semi-supervised CNN
CNN can also be used in scenarios involving sparse labeled data and abundant unlabeled data. To overcome the sparse labeled data problem, a new graph based semi supervised learning techniques for breast cancer diagnosis was proposed in [ 73 ]. For removal of redundancies and feature correlations, dimensionality reduction was employed. The method used four modules, feature extraction to extract 21 features from breast masses, data weighing to minimize the influence of noisy data and division of co-training data labeling followed by the CNN. It involved sub patches extraction of ROIs which were input to three pairs of conv layers, maxpooling and FC layer. Three models CNN, SVM and ANN are compared. The AUC for CNN for mixture of labeled and unlabeled data was \(88\%\) compared to \(85\%\) of SVM and \(84\%\) of ANN and accuracy for CNN with mixed data was \(82\%\) compared to \(80\%\) for SVM and \(80\%\) for ANN. The CNN approaches for breast tumor classification are summarized in Table 7 .
4.5 Heart diseases
Electrocardiogram (ECG) is used for the assessment of the electrical activity of the heart to detect anomalies in the heart.
4.5.1 One-dimensional CNN
ECG classification using CNN model demonstrates superior performance with classification accuracy of \(95\%\) was proposed in [ 40 ]. The model comprised of one-dimensional CNN with three conv layers and two FC layers that fused feature extraction and classification into a single learning body. Once the dedicated CNN was trained for a particular patient, it could be solely used to classify ECG records in a fast and accurate manner.
4.5.2 Fused CNN
Classification of echocardiography videos require both spatial and temporal data. Fused CNN architecture using both spatial and temporal data was proposed in [ 20 ]. It used a two-path CNN, one along the spatial direction and the other along temporal direction. Each individual CNN path executed individually and was fused only after obtaining the final classification scores. The spatial CNN learnt the spatial information automatically from the original normalised echo video images. Temporal CNN learnt from acceleration images along the time direction of the echo videos. The outputs of both CNNs were fused and applied to softmax classifier for the final classification. The proposed model achieved an average accuracy of \(92\%\) compared to \(89.5\%\) for single path CNN, \(87.9\%\) for three-dimensional KAZE, \(73.8\%\) for three-dimensional SIFT. The long time required for initial training was the disadvantage of this approach. The CNN approaches to heart classification are summarized in Table 8 .
4.6 Eye diseases
4.6.1 gaussian initialized cnn.
Initial training time can be reduced by Gaussian initialization, and overfitting can be avoided by weighted class weights. This was proposed for classifying diabetic retinopathy (DR) in fundus imagery in [ 57 ]. The performance was compared with SVM and other methods that required feature extraction prior to classification. The method achieved \(95\%\) specificity but less sensitivity of \(30\%\) . The trained CNN did a quick diagnosis and gave an immediate response to the patient during screening.
4.6.2 Hyper parameter tuning inception-v4
Automated hyper parameter tuning inception-v4 (HPTI-v4) model for DR in color fundus images classification and detection is proposed in [ 67 ]. The images are preprocessed using CLAHE to enhance contrast level, segmented using histogram based segmentation model. Hyper parameter tuning is done using Bayesian optimization method, as Bayesian model has the ability to analyze the previous validation outcome, to create a probabilistic model. Classification is done using HPTI-v4 model followed by multi layer perceptron. The classification is applied on MESSIDOR DR dataset. The CNN model performance was extraordinary with the accuracy, sensitivity, and specificity of \(99.49\%\) , \(98.83\%\) , and \(99.68\%\) respectively.
4.7 Colon cancer
4.7.1 ensemble cnn.
Usage of small patches increased the amount of training data and localized the analysis to small nuclei in images. This enhanced the performance of detecting and classifying nuclei in H&E stained histopathology images of colorectal adenocarcinoma. This was proposed in [ 71 ]. The model also demonstrated locality sensitive deep learning approach with neighboring ensemble predictor (NEP) in conjunction with a standard softmax CNN and eliminated need of segmentation. The model used dropout to avoid overfitting. The model obtained an AUC of \(91.7\%\) and F-score of \(78.4\%\)
The CNN approaches for colon cancer classification are summarized in Table 9 .
4.8 Brain disorders
Alzheimer’s disease causes the destruction of brain cells leading to memory loss. Classification of Alzheimer’s disease (AD) has been challenging since it involves selection of discriminative features.
4.8.1 Fused CNN
Fusion of two-dimensional CNN and three-dimensional CNN achieves better accuracy was demonstrated in [ 19 ]. Information along Z direction acts very crucial for analysis of brain images and three-dimensional CNN was used to retain this information. Since the thickness of brain CT images is thicker than MRI images, geometric normalization of CT images were performed. Output of the last conv layer of two-dimensional CNN was fused with three-dimensional convoluted data to get three classes (Alzheimer’s, lesions, and healthy data). It was compared with two hand-crafted approaches SIFT and KAZE for accuracy and achieved better accuracy of \(86.7\%, 78.9\%\) and \(95.6\%\) for AD, lesion and normal class, respectively.
4.8.2 Input cascaded CNN
Lack of training data can be overcome by extensive augmentation and fine tuning was proposed in [ 62 ]. Multi-grade brain tumor classification was performed by segmenting the tumor regions from an MR image using input cascaded CNN, extensive augmentation and then fine-tuned using data augmented. The performance was compared against state-of-art methods. It resulted in an accuracy of \(94.58\%\) , sensitivity of \(88.41\%\) and specificity of \(96.58\%\) .
The CNN approaches for medical image classification discussed above are summarized in Table 10 .
5 CNN applications in medical image segmentation
CNNs have been applied to implement efficient segmentation of images of brain tumors, hearts, breasts, retina, fetal abdomen, stromal and epithelial tissues.
5.1 Brain tumors
MRI is used to obtain detailed images of the brain to diagnose tumors. Automatic segmentation of a brain tumor is very challenging because it involves the extraction of high level features.
5.1.1 Small kernel CNN
Patch-wise training and use of small filter sizes ( \(3\times 3\) ) was proposed for segmentation of gliomas in [ 54 ]. This provided an advantage of deep architecture, while retaining the same receptive fields. Two separate models were trained for high and low gliomas. High glioma model consisted of eight conv layers and three dense layers. Low glioma model contained four conv layers and three dense layers. Maxpooling was used along with dropout for dense layers. It ranked fourth in the BRATS-2015 challenge. Data augmentation was achieved by rotation which enhanced the performance of segmentation of gliomas.
5.1.2 Fully blown CNN
Fully blown MRI two-dimensional images enhances performance of segmentation of sub-cortical human brain structure. This was shown in [ 66 ]. The proposed model applied Markov random field on CNN output to impose volumetric homogeneity to the final results. It outperformed several state-of-the-art methods.
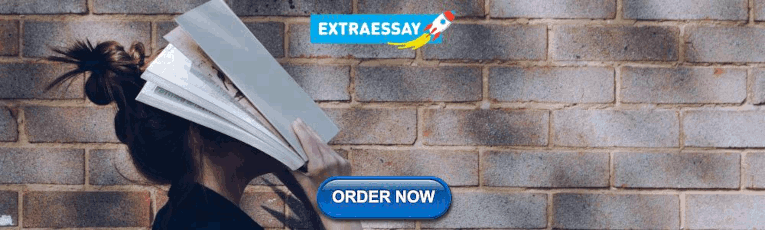
5.1.3 Multipath CNN
Two pathways, one for convolution and the other for deconvolution, enhances segmentation output was shown in [ 8 ]. The model was used for automatic MS lesions segmentation. The model had convolutional pathway consisting of alternating conv, pool layers, and a deconvolutional pathway consisting of alternate deconv layer and unpooling layer. The pretraining was performed by convolutional RBMs (convRBM). Both pretraining and fine training were performed on a highly optimized GPU-accelerated implementation of three-dimensional convRBMs and convolutional encoder networks (CEN). It was compared with five publicly available methods and established as comparison reference points. The model performance was evaluated using evaluation metrics DSC, TPR and FPR. TPR and FPR achieved were comparatively better than the previous models developed. However, it achieved lesser DSC in comparison to other methods.
5.1.4 Cascaded CNN
In case of imbalanced label distributions, two phase training could be used. Global contextual features and local detailed features can be learned simultaneously by two-pathway architecture for brain segmentation and was proposed in [ 25 ]. The advantage of two-pathway was, it could recognize fine details of the tumor at a local scale and correct labels at a global scale to yield a better segmentation. Slice-by-slice segmentation from the axial view due to less resolution in the third dimension was performed. The cascaded CNN achieved better rank than two-pathway CNN and was ranked second at the MICCAI BRATS-2013 challenge. The evaluation metrics used were DSC, specificity and sensitivity and the obtained values were \(79\%, 81\%\) and \(79\%\) . The time taken for segmentation was between 25 s and 3 min.
5.1.5 Multiscale CNN
In case of brain tumor segmentation, a multiscale CNN architecture for extracting both local and global features at different scales was proposed in [ 80 ]. The model performed better due to different features extracted at various resolution. The computation time was reduced by exploiting a two-dimensional CNN instead of a three-dimensional CNN. Three patch sizes \(48\times 48\) , \(28\times 28\) and \(12\times 12\) were input to three CNNs for feature extraction. All the features extracted were input to the FC layer. Evaluation of the model was by DSC and accuracy. The model performance was almost as stable as the best method with an accuracy of nearly \(90\%\) .
5.1.6 Multipath and multiscale CNN
Twopath and multiscale architecture were also explored for brain lesion segmentation by [ 37 ]. The model exploited smaller kernels to get local neighbour information and employed parallel convolutional pathways for multiscale processing. It achieved highest accuracy when applied on patients with severe traumatic brain injuries. It could also segment small and diffused pathologies. Three-dimensional CNN produced accurate segmentation borders. FC three-dimensional CRF imposed regularization constraints on CNN output and produced final hard segmentation labels. Also, due to its generic nature, it cold be applied to different lesion segmentation tasks with slight modifications. It was ranked first in the stroke lesions ISLES-SISS-2015 challenge.
Advantages of multipath and multiscale CNN was exploited for automatic segmentation of analytical brain images in [ 49 ]. The bigger kernel was used for spatial information. A separate network branch was used for each patch size, and only the output layer was shared. Mini batch learning and RMSprop were used to train the network with ReLU and cross entropy as the cost function. Automatic segmentation was evaluated using the DSC and mean surface distance between manual and automatic segmentation. It achieved accurate segmentation in terms of DSC for all tissue classes. The CNN approaches for brain segmentation discussed above are summarized in Table 11 .
5.2 Breast cancer
Breast cancers can be predicted by automatically segmenting breast density and by characterizing mammographic textural patterns.
Redundant computations in conv and max pool layers can be avoided by using ROI segmentation in a fast scanning deep CNN (FCNN). The above technique was applied for segmentation of histopathological breast cancer images and was proposed in [ 72 ]. The proposed work was compared against three texture classification methods namely raw pixel patch with large scale SVM, local binary pattern feature with large scale SVM and texton histogram with logistic booting. The evaluation metrics used were accuracy, efficiency and scalability. Ihe proposed method was robust to intra-class variance. It achieved an F-score of \(85\%\) , whereas the other methods delivered the maximum F-score of \(75\%\) . It took only 2.3 s to segment image of resolution of \(1000\times 1000\) .
5.2.2 Probability map CNN
Probability maps were explored for iterative region merging for shape initialization along with compact nucleus shape repository with selection-based dictionary learning algorithm in [ 77 ]. The model resulted in better automatic nucleus segmentation using CNN. The framework was tested on three types of histopathology images namely, brain tumor, pancreatic neuro endocrine tumor and breast cancer. The parameters for comparison were precision, recall and F-score. It achieved better performance when compared to SVM, RF and DBN, especially for breast cancer images. Pixel-wise segmentation accuracy measured using DSC, HD and MAD resulted in superior performance when compared to other methods.
5.2.3 Patch CNN
Advantages of patch-based CNN was exploited in [ 78 ]. The method also exploited super pixel method to over segment breast cancer H&E images into atomic images. The result was natural boundaries with errors being subtle and less egregious, whereas sliding window methods resulted in zigzag boundaries. Both patch-based CNN and superpixel techniques were combined for segmenting and classifying the stromal and epithelial regions in histopathological images for detection of breast and colorectal cancer. The proposed model outperformed CNN with SVM. The comparison was done against methods using handcrafted features. It achieved \(100\%\) accuracy and Deep CNN-Ncut-SVM had better AUC than other CNN.
5.3 Eye diseases
5.3.1 greedy cnn.
The architecture of conventional CNNs was tweaked by making the filters of the CNN learn sequentially using a greedy approach of boosting instead of backpropagation. Boosting was applied to learn diverse filters to minimize weighted classification error. The ensembling learning was proposed for the automatic segmentation of optic cup and optic disc from retinal fundus images to detect glaucoma in [ 81 ]. The model performed entropy sampling to identify informative points on landmarks such as edges, blood vessels. etc. The weight updates were done considering final classification error instead of back propagation error. The method operated on patches of image taken around a point. A F-score of \(97.3\%\) was obtained which was comparatively better to normal CNN whose best F-score was \(96.7\%\) .
5.3.2 Multi label inference CNN
Retinal blood vessel segmentation was dealt as multi-label inference problem and solved using CNN in [ 16 ]. The model extracted green channel from RGB fundus image, as blood vessels manifest high contrast in green channel. The model was upsampled at the sixth layer to increase spatial dimension for structured output. The output of CNN model was modeled as vector instead of a scalar, due to multiple labels. It achieved precision of \(84.98\%\) , sensitivity of \(76.91\%\) , specificity of \(98.01\%\) , accuracy of \(95.33\%\) and AUC of \(97.44\%\) .
5.4.1 U net
Lung segmentation and bone shadow exclusion techniques for analysis of lung cancer using U-net architecture is proposed in [ 23 ]. The images were preprocessed to eliminate bone shadow and a simple U-net architecture was used to segment the lung ROI. The results obtained were very promising and showed a good speed and precise segmentation. The CNN approaches for medical image segmentation discussed above are summarized in Table 12 .
6 CNN applications in medical image detection
6.1 breast tumors.
A Camelyon grand challenge for automatic detection of metastatic breast cancer in digital whole slide images of sentinel lymph node biopsies is organised by the International Symposium of Biomedical Imaging.
6.1.1 GoogLeNet CNN
The award-winning system with performance very closer to human accuracy was proposed in [ 76 ]. The computation time was reduced by first excluding the white background of digital images using Otsu’s algorithm. The method exploited advantages of patch-based classification to obtain better results. Also the model trained extensively on misclassified image patches, to decrease classification error. The results of the patches were embedded on heatmap image and heatmaps were used to compute evaluation scores. AUC of \(92.5\%\) was obtained, and was the top performer in the challenge. In case of lesion based detection, the system achieved the sensitivity of \(70.51\%\) , whereas the second ranking score was \(57.61\%\) .
6.2 Eye diseases
6.2.1 dynamic cnn.
Random assignment of weights, speeds up the training and improves the performance. This was proposed for hemorrhage detection in fundus eye images in [ 75 ]. Also the samples were dynamically selected at every training epoch from a large pool of medical images. Pre-processing was performed using image contrast using gaussian filters. To prevent overfitting, the images were augmented. For correct classification of hemorrhage, the result was convolved with gaussian filter to smoothen the values. It achieved sensitivity, specificity and ROC of \(93\%\) , \(91.5\%\) and \(98\%\) , whereas non selective sampling obtains sensitivity, specificity and ROC of \(93\%, 93\%\) and \(96.6\%\) for Messidor dataset. AUC was used to monitor overfitting during training and when AUC value reached a stable maximum, the CNN training was stopped.
6.2.2 Ensemble CNN
An ensemble performs better than a single CNN and can be used to achieve higher performance. The ensemble model for detection of retinal vessels in fundus images is proposed in [ 46 ]. The model was an ensemble of twelve CNNs. Each CNN’s output probability was averaged to get the final vessel’s probability of each pixel. The probability was used to discriminate between vessel pixels from non-vessel ones for detection. The performance measures, accuracy and Kappa score were compared with existing state of the art methods. It stood second in terms of accuracy as well as in kappa score. The model obtained a FROC score of 0.928.
6.3 Cell division
6.3.1 lenet cnn.
Augmentation and shifting the centroid of the object enhanced the performance, and was proposed in [ 69 ]. The model was for automatic detection of mitosis (cell divisions) and quantification of mitosis occurring during scratch assay. The positive example training samples was augmented by mirroring and rotating by \(45^{\circ }\) and centering by shifting the centroid of the object to the patch center. A random additional sampling of negative samples was added in the same amount as positive examples. The performance parameters used were sensitivity, specificity, AUC and F-score and compared with SVM. The results indicated significant increase in F-score (for SVM \(78\%\) , for CNN \(89\%\) ). The model concluded that both positive and negative samples are needed for better performance. The CNN applications in medical image detection reviewed in this paper are summarized in Table 13 .
7 CNN applications in medical image localization
7.1 breast tumors, 7.1.1 semi-supervised deep cnn.
To overcome challenges of sparsely labeled data, a model was proposed in [ 73 ]. The unlabeled data was first automatically labeled using labeled data. Newly labeled data and initial labeled data were used to train the deep CNN. The method used semi-supervised deep CNN for breast cancer diagnosis. The performance of CNN was compared with SVM and ANN using different numbers of labeled data like 40, 70 and 100. The model produced comparable results even with sparse labeled data with accuracy of \(83\%\) and AUC of \(88\%\) .
7.2 Heart diseases
7.2.1 pyramid of scales localization.
Pyramid of scales (PoS) localization leads to better performance, specially in cases, where the size of the organ varies between the patients. The size of the heart is not consistent among human, and hence PoS was proposed for localization of left ventricle (LV) in cardiac MRI images in [ 17 ]. The model also exploited patch based training. Evaluation metrics used were accuracy, sensitivity and specificity and were respectively \(98.6\%, 83.9\%\) and \(99.1\%\) . The limitation of the approach was the computing time of 10 s/image.
7.3 Fetal abnormalities
7.3.1 transfer learning cnn.
Transfer learning uses the knowledge of low layers of a base CNN trained on a large cross domain of dataset of images. Transfer learning advantages include saving of training time, and need of less data for training. This reduces overfitting and enhances the classification performance. Domain transferred deep CNN for fetal abdominal standard plane (FASP) localization in fetal ultrasound scanning was proposed in [ 10 ]. The base CNN was trained on 2014 ImageNet detection dataset. The metrics accuracy, precision, recall and F-score were the highest when compared to R-CNN and RVD. The drawback of the system was that it took more time to locate FASP from one ultra sound video. The CNN methods for image localization previewed in this paper are summarized in Table 14 .
The papers reviewed for medical image understanding are summarized in Table 15 .
8 Critical review and conclusion
CNNs have been successfully applied in the areas of medical image understanding and this section provides a critical review of applications of CNNs in medical image understanding. Firstly, the literature contains a vast number of CNN architectures. It is difficult to select the best architecture for a specific task due to high diversity in architectures. Moreover, the same architecture might result in different performance due to inefficient data preprocessing techniques. A prior knowledge of data for applying the correct preprocessing technique is needed. Futhermore, hyper parameters optimization (dropout rate, learning rate, optimizer etc) help in enhancing or declining the performance of a network.
For training, CNNs require exhaustive amounts of data containing the most comprehensive information. Insufficient information or features leads to underfitting of the model. However, augmentation could be applied in such scenarios as it results in translation variance and increases the training dataset, thereby enhancing the CNNs efficiency. Furthermore, transfer learning and fine-tuning could also be used to enhance the efficiency in case of sparse availability of data. These enhance the performance since the low level features are nearly the same for most of the images.
Small-sized kernels could be used to enhance the performance by capturing low-level textual information. However, it is at the cost of increased computational complexity during training. Moreover, multiple pathway architecture could be used to enhance performance of CNN. The performance is enhanced due to simultaneous learning of global contextual features and local detailed features, but this in turn, increases the computational burden on the processor and memory.
One of the challenge involved in medical data is the class imbalance problem, where the positive class is generally under-represented and most of the images belong to the normal class. Designing CNNs to work on imbalanced data is a challenging task. However, researchers have tried to overcome this challenge by applying augmentation of the under-represented data. Denser CNNs could also lead to the vanishing gradient problem which could be overcome by using skip connections as in the inceptionNet architecture.
Furthermore, CNNs’ significant depth and enormous size require huge memory and higher computational resources for training. The deeper CNNs involves millions of training parameters which could lead the model to overfit and also inefficient at generalization, especially in the case of limited dataset. This calls for models which are lightweight and which could also extract critical features like the dense models. Lightweight CNNs could be explored further.
Medical image Understanding would be more efficient in the presence of background context or knowledge about the image to be understood. In this context, CNNs would be more efficient if the data consists of not only images, but also patient history. Hence, the next challenging task would be to build models, which take as input both images and patient history to make a decision and this could be the next research trend.
Interpreting CNNs is challenging due to many layers, millions of parameters, and complex, nonlinear data structures. CNN researchers have been concentrating on building accurate models without quantifying uncertainty in the obtained results. The need for successful utilization of the CNN model in medical diagnosis lies in providing confidence and this confidence needs the ability of the model to ascertain its uncertainty or certainty or explain the results obtained. This field needs further exploration. Although, researchers have proposed heat maps, class activation maps (CAM), grad CAM, grad CAM++ for visualization of CNN outputs, the area of visualization is still a challenge.
The various challenges and methods of overcoming some of the challenges of medical image understanding are summarized in Table 16 . Further, efficient architectures to overcome some of the challenges as per the survey are summarized in Table 17 .
Deep learning includes methods like CNN, recurrent neural network and generative adversial networks. The review of these methods and applications have not been included, as these methods by themselves become topics of research and there is lot of research happening in those areas. Moreover, all the aspects involved in medical image understanding are also not included since it is an ocean and the focus in the paper is only on a few important techniques involved.
9 Conclusion
The heterogeneous nature of medical anomalies in terms of shape, size, appearance, location and symptoms poses challenges for medical anomaly diagnosis and prognosis. Traditional methods of using human specialists involve fatigue, oversight and high cost and also sparse availability. ML-based healthcare systems need efficient feature extraction methods. But efficient features are still unknown and also, the methods available for feature extraction are not very efficient. This calls for intelligent healthcare systems that automatically extract efficient features for medical image understanding that aids diagnosis and prognosis. CNN is a popular technique for solving medical image understanding challenges due to its highly efficient methods of feature extraction and learning low level, mid level and high level discriminant features of an input medical image.
The literature reviewed in this paper underscores that researchers have focused their attention on the use of CNN to overcome many challenges in medical image understanding. Many have accomplished the task successfully. The CNN methods discussed in this paper have been found to either outperform or compliment the existing traditional and ML approaches in terms of accuracy, sensitivity, AUC, DSC, time taken etc. However, their performance is often not the best due to a few factors. A snapshot summary of the quantum of research articles surveyed in this article is presented in the Fig. 2 .
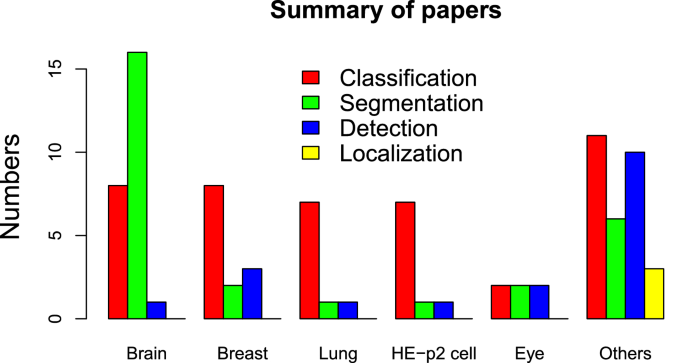
Bar chart summarizing the number of papers surveyed
The challenges in image understanding with respect to medical imaging have been discussed in this paper. Various image understanding tasks have been introduced. In addition, CNN and its various components have been outlined briefly. The approaches used by the researchers to address the various challenges in medical image understanding have been surveyed.
CNN models have been described as black boxes and there is a lot of research happening in terms of analyzing and understanding output at every layer. Since medical images are involved, we need an accountable and efficient prediction system which should also be able to articulate about a decision taken. Researchers are also working on image captioning (textual representations of the image) [ 29 ]. This will enable physicians to understand the perception of the network at both output layer and intermediate levels. Researchers have tried Bayesian deep learning models which calculates the uncertainty estimates [ 38 ]. This would help physicians assess the model. All these could further accelerate medical image understanding using CNNs among physicians.
Abadi M, Agarwal A, Barham P (2016) Tensorflow: large-scale machine learning on heterogeneous distributed systems. CoRR Arxiv: 1603.04467
Akkus Z, Galimzianova A, Hoogi A, Rubin DL, Erickson BJ (2017) Deep learning for brain MRI segmentation: state of the art and future directions. J Digit Imaging 30(4):449–459
Article Google Scholar
Al-Rfou R, Alain G, Almahairi A, Angermueller C, Bahdanau D, Ballas N, Bengio Y (2016) Theano: a python framework for fast computation of mathematical expressions. arXiv:1605.02688
Anthimopoulos M, Christodoulidis S, Ebner L, Christe A, Mougiakakou SG (2016) Lung pattern classification for interstitial lung diseases using a deep convolutional neural network. IEEE Trans Med Imaging 35(5):1207–1216
Apou G, Schaadt NS, Naegel B (2016) Structures in normal breast tissue. Comput Biol Med 74:91–102
Bassi PAS, Attux R (2020) A deep convolutional neural network for COVID-19 detection using chest X-rays
Bayramoglu N, Kannala J, Heikkilä J (2015) Human epithelial type 2 cell classification with convolutional neural networks. In: Proceedings of the 15th IEEE international conference on bioinformatics and bioengineering, BIBE, pp 1–6
Brosch T, Tang LYW, Yoo Y, Li DKB, Traboulsee A, Tam RC (2016) Deep 3-D convolutional encoder networks with shortcuts for multiscale feature integration applied to multiple sclerosis lesion segmentation. IEEE Trans Med Imaging 35(5):1229–1239
CS231N convolutional neural network for visual recogntion (2019). http://cs231n.stanford.edu/ . Accessed 24 June 2019
Chen H, Ni D, Qin J (2015) Standard plane localization in fetal ultrasound via domain transferred deep neural networks. IEEE J Biomed Health Inform 19(5):1627–1636
Chollet F (2018) Keras: the Python deep learning library. https://keras.io/ . Accessed 24 June 2019
Chollet F (2017) Xception: deep learning with depthwise separable convolutions. In: Proceedings of the IEEE conference on computer vision and pattern recognition, (CVPR), pp 1800–1807
Chowdhury NK, Rahman MM, Kabir MA (2020) Pdcovidnet: a parallel-dilated convolutional neural network architecture for detecting COVID-19 from chest X-ray images
Ciompi F, de Hoop B, van Riel SJ (2015) Automatic classification of pulmonary peri-fissural nodules in computed tomography using an ensemble of 2-D views and a convolutional neural network out-of-the-box. Med Image Anal 26(1):195–202. https://doi.org/10.1016/j.media.2015.08.001
Collobert R, Kavukcuoglu K, Farabet C (2011) Torch7: a Matlab-like environment for machine learning. In: BigLearn, NIPS workshop, EPFL-CONF-192376
Dasgupta A, Singh S (2017) A fully convolutional neural network based structured prediction approach towards the retinal vessel segmentation. In: Proceedings of the 14th IEEE international symposium on biomedical imaging (ISBI), pp 248–251
Emad O, Yassine IA, Fahmy AS (2015) Automatic localization of the left ventricle in cardiac MRI images using deep learning. In: Proceedings of the 37th IEEE annual international conference on engineering in medicine and biology society (EMBC), pp 683–686
Gao M, Bagci U, Lu L, Wu A, Buty M (2018) Holistic classification of CT attenuation patterns for interstitial lung diseases via deep convolutional neural networks. CMBBE Imaging Vis 6(1):1–6
Google Scholar
Gao XW, Hui R (2016)A deep learning based approach to classification of CT brain images. In: Proceedings of the SAI computing conference, pp 28–31
Gao XW, Li W, Loomes M, Wang L (2017) A fused deep learning architecture for viewpoint classification of echocardiography. Inf Fusion 36:103–113
Gao Z, Zhang J, Zhou L, Wang L (2014) HEp-2i cell image classification with deep convolutional neural networks. IEEE J Biomed Health Inform 21:416–428
Ghoshal B, Tucker A (2020) Estimating uncertainty and interpretability in deep learning for coronavirus (COVID-19) detection arXiv:2003.10769
Gordienko Y, Gang P, Hui J, Zeng W, Kochura Y, Alienin O, Rokovyi O, Stirenko S (2017) Deep learning with lung segmentation and bone shadow exclusion techniques for chest X-ray analysis of lung cancer. CoRR arXiv:1712.07632
Hasan MK, Alam MA, Elahi MTE, Roy S, Wahid SR (2020) CVR-NET: a deep convolutional neural network for coronavirus recognition from chest radiography images
Havaei M, Davy A, Warde-Farley D (2017) Brain tumor segmentation with deep neural networks. Med Image Anal 35:18–31
He K, Zhang X, Ren S, Sun J (2016) Deep residual learning for image recognition. In: Proceedings of the IEEE conference on computer vision and pattern recognition, (CVPR), pp 770–778
Hesamian M, Jia W, He X (2019) Deep learning techniques for medical image segmentation: achievements and challenges. J Digit Imaging 32:582–596
Ho TK (1995) Random decision forests. In: Proceedings of the 3rd IEEE international conference on document analysis and recognition, vol 1, pp 278–282
Hossain MZ, Sohel F, Shiratuddin MF, Laga H (2018) A comprehensive survey of deep learning for image captioning. CoRR arXiv:1810.04020
Hubel D, Wiesel T (1959) Receptive fields of single neurones in the cat’s striate cortex. J Physiol 148(3):574–591
ICPR 2018 international conference on pattern recognition (2019). http://www.icpr2018.org . Accessed 24 June 2019
ISLES challenge 2018 ischemic stroke lesion segmentation (2018). http://www.isles-challenge.org . Accessed 24 June 2019
Jia, Y., Shelhamer E, Donahue J (2014) Caffe: convolutional architecture for fast feature embedding. In: Proceedings of the 22th ACM international conference on multimedia, MM ’14, pp 675–678. ACM, New York
Jiao Z, Gao X, Wang Y, Li J (2016) A deep feature based framework for breast masses classification. Neurocomputing 197:221–231
Jusman Y, Ng SC, Abu Osman NA (2014) Intelligent screening systems for cervical cancer. Sci World J 2014:810368
Kallenberg M, Petersen K, Nielsen M (2016) Unsupervised deep learning applied to breast density segmentation and mammographic risk scoring. IEEE Trans Med Imaging 35(5):1322–1331
Kamnitsas K, Ferrante E, Parisot S (2016) Deepmedic for brain tumor segmentation. In: Proceedings of the international workshop on brainlesion: glioma, multiple sclerosis, stroke and traumatic brain injuries. Springer, pp 138–149
Kendall A, Gal Y (2017) What uncertainties do we need in bayesian deep learning for computer vision? CoRR arXiv:1703.04977
Kermany DS, Goldbaum M, Cai W, Valentim CC, Liang H, Baxter SL, McKeown A, Yang G, Wu X, Yan F et al (2018) Identifying medical diagnoses and treatable diseases by image-based deep learning. Cell 172(5):1122–1131
Kiranyaz S, Ince T, Gabbouj M (2016) Real-time patient-specific ECG classification by 1-D convolutional neural networks. IEEE Trans Biomed Eng 63(3):664–675
Krizhevsky A, Sutskever I, Hinton GE (2012) Imagenet classification with deep convolutional neural networks. In: Advances in neural information processing systems 25: proceedings of the 26th annual conference on neural information processing systems, pp 1106–1114
Larochelle H, Bengio Y (2008) Classification using discriminative restricted Boltzmann machines. In: Proceedings of the 25th international conference on machine learning, pp 536–543
LeCun Y, Bottou L, Bengio Y, Haffner P (1998) Gradient-based learning applied to document recognition. Proc IEEE 86(11):2278–2324
Li Q, Cai W, Wang X, Zhou Y, Feng DD, Chen M (2014) Medical image classification with convolutional neural network. In: Proceedings of the 13th international conference on control, automation, robotics and vision (ICARCV), pp 844–848
Maghdid HS, Asaad AT, Ghafoor KZ, Sadiq AS, Khan MK (2020)Diagnosing COVID-19 pneumonia from X-ray and CT images using deep learning and transfer learning algorithms. CoRR arXiv:2004.00038
Maji D, Santara A, Mitra P (2016) Ensemble of deep convolutional neural networks for learning to detect retinal vessels in fundus images. arXiv preprint arXiv:1603.04833
Mavroforakis ME, Georgiou HV, Dimitropoulos N (2006) Mammographic masses characterization based on localized texture and dataset fractal analysis using linear, neural and support vector machine classifiers. Artif Intell Med 37(2):145–162
Menze BH, Jakab A, Bauer S (2015) The multimodal brain tumor image segmentation benchmark (BRATS). IEEE Trans Med Imaging 34(10):1993–2024
Moeskops P, Viergever MA, Mendrik AM, de Vries LS, Benders MJNL, Isgum I (2016) Automatic segmentation of MR brain images with a convolutional neural network. IEEE Trans Med Imaging 35(5):1252–1261
Nasr-Esfahani E, Samavi S, Karimi N (2016) Melanoma detection by analysis of clinical images using convolutional neural network. In: Proceedings of the 38th IEEE annual international conference on engineering in medicine and biology society, EMBC, pp 1373–1376
Nervana Systems Inc (2018) neon. http://neon.nervanasys.com/docs/latest/ . Accessed 24 June 2019
Ovalle JEA, González FA, Ramos-Pollán R, Oliveira JL, Guevara-López MÁ (2016) Representation learning for mammography mass lesion classification with convolutional neural networks. Comput Methods Prog Biomed 127:248–257
Paszke A, Gross S, Chintala S, Chanan G (2018) Tensors and dynamic neural networks in Python with strong GPU acceleration. https://pytorch.org/ . Accessed 24 June 2019
Pereira S, Pinto A, Alves V, Silva CA (2015) Deep convolutional neural networks for the segmentation of gliomas in multi-sequence MRI. In: Brainlesion: glioma, multiple sclerosis, stroke and traumatic brain injuries—first international workshop, Brainles 2015, held in conjunction with MICCAI 2015, Munich, Germany, October 5, 2015, revised selected papers, pp 131–143
Pereira S, Pinto A, Alves V, Silva CA (2016) Brain tumor segmentation using convolutional neural networks in MRI images. IEEE Trans Med Imaging 35(5):1240–1251
Phillips NA, Rajpurkar P, Sabini, M, Krishnan R, Zhou, S, Pareek, A, Phu NM, Wang C, Ng AY, Lungren MP (2020) Chexphoto: 10,000+ smartphone photos and synthetic photographic transformations of chest X-rays for benchmarking deep learning robustness. CoRR arXiv:2007.06199
Pratt H, Coenen F, Broadbent DM, Harding SP, Zheng Y (2016) Convolutional neural networks for diabetic retinopathy. In: Proceedings of the 20th conference on medical image understanding and analysis, MIUA, pp 200–205
Rajpurkar P, Irvin J, Zhu K, Yang B, Mehta H, Duan T, Ding DY, Bagul A, Langlotz C, Shpanskaya KS, Lungren MP, Ng AY (2017) Chexnet: radiologist-level pneumonia detection on chest X-rays with deep learning. CoRR arXiv:1711.05225
Ranzato M, Hinton GE, LeCun Y (2015) Guest editorial: deep learning. Int J Comput Vis 113(1):1–2
Article MathSciNet Google Scholar
Ravi D, Wong C, Deligianni F, Berthelot M, Pérez JA, Lo B, Yang G (2017) Deep learning for health informatics. IEEE J Biomed Health Inform 21(1):4–21
Ribeiro E, Uhl A, Häfner M (2016) Colonic polyp classification with convolutional neural networks. In: Proceedings of the 29th IEEE international symposium on computer-based medical systems, (CBMS), pp 253–258
Sajjad M, Khan S, Muhammad K, Wu W, Ullah A, Baik SW (2019) Multi-grade brain tumor classification using deep CNN with extensive data augmentation. J Comput Sci 30:174–182. https://doi.org/10.1016/j.jocs.2018.12.003
Sarraf S, Tofighi G (2016) Classification of Alzheimer’s disease using fMRI data and deep learning convolutional neural networks. Computer Research Repository. arXiv:1603.08631
Seide F, Agarwal A (2016) CNTK: Microsoft’s open-source deep-learning toolkit. In: Proceedings of the 22nd ACM international conference on knowledge discovery and data mining, p 2135
Sermanet P, Eigen D, Zhang X, Mathieu M, Fergus R, LeCun Y (2013) Overfeat: integrated recognition, localization and detection using convolutional networks. Computing Research Repository. arXiv:1312.6229
Shakeri M, Tsogkas S, Ferrante E, Lippe S, Kadoury S, Paragios N, Kokkinos I (2016) Sub-cortical brain structure segmentation using F-CNNs. In: Proceedigns of the IEEE 13th international symposium on biomedical imaging (ISBI). IEEE, pp 269–272
Shankar K, Zhang Y, Liu Y, Wu L, Chen CH (2020) Hyperparameter tuning deep learning for diabetic retinopathy fundus image classification. IEEE Access 8:118164–118173
Shen W, Zhou M (2017) Multi-crop convolutional neural networks for lung nodule malignancy suspiciousness classification. Pattern Recognit 61:663–673
Shkolyar A, Gefen A, Benayahu D, Greenspan H (2015) Automatic detection of cell divisions (mitosis) in live-imaging microscopy images using convolutional neural networks. In: Proceedings of the 37th annual international conference of the IEEE engineering in medicine and biology society (EMBC), pp 743–746
Simonyan K, Zisserman A (2014) Very deep convolutional networks for large-scale image recognition. Computer Research Repository. arXiv:1409.1556
Sirinukunwattana K, Raza SEA, Tsang Y, Snead DRJ, Cree IA, Rajpoot NM (2016) Locality sensitive deep learning for detection and classification of nuclei in routine colon cancer histology images. IEEE Trans Med Imaging 35(5):1196–1206
Su H, Liu F, Xie Y (2015) Region segmentation in histopathological breast cancer images using deep convolutional neural network. In: Proceedings of the 12th IEEE international symposium on biomedical imaging (ISBI), pp 55–58
Sun W, Tseng TB, Zhang J, Qian W (2017) Enhancing deep convolutional neural network scheme for breast cancer diagnosis with unlabeled data. Comput Med Imaging Graph 57:4–9
Szegedy C, Liu W, Jia Y, Sermanet P, Reed SE (2015) Going deeper with convolutions. In: Proceedings of the IEEE conference on computer vision and pattern recognition (CVPR), pp 1–9
van Grinsven MJ, van Ginneken B, Hoyng CB (2016) Fast convolutional neural network training using selective data sampling: application to hemorrhage detection in color fundus images. IEEE Trans Med Imaging 35(5):1273–1284
Wang D, Khosla A, Gargeya R, Irshad H, Beck AH (2016) Deep learning for identifying metastatic breast cancer. Computer Research Repository. arXiv:1606.05718
Xing F, Xie Y, Yang L (2016) An automatic learning-based framework for robust nucleus segmentation. IEEE Trans Med Imaging 35(2):550–566
Xu J, Luo X, Wang G, Gilmore H, Madabhushi A (2016) A deep convolutional neural network for segmenting and classifying epithelial and stromal regions in histopathological images. Neurocomputing 191:214–223
Zeiler MD, Fergus R (2013) Visualizing and understanding convolutional networks. Computer Research Repository. arXiv:1311.2901
Zhao L, Jia K (2016) Multiscale CNNs for brain tumor segmentation and diagnosis. Comput Math Methods Med 2016:8356294:1–8356294:7
Zilly JG, Buhmann JM, Mahapatra D (2017) Glaucoma detection using entropy sampling and ensemble learning for automatic optic cup and disc segmentation. Comput Med Imaging Graph 55:28–41
Zou Y, Li L, Wang Y (2015) Classifying digestive organs in wireless capsule endoscopy images based on deep convolutional neural network. In: Proceedings of the IEEE international conference on digital signal processing, DSP, pp 1274–1278
Zreik M, Leiner T, de Vos BD, van Hamersvelt RW, Viergever MA, Isgum I (2016) Automatic segmentation of the left ventricle in cardiac CT angiography using convolutional neural networks. In: Proceedings of the 13th IEEE international symposium on biomedical imaging (ISBI), pp 40–43
Download references
Acknowledgements
The authors acknowledge with gratitude the support received from REVA University, Bengaluru, and M. S. Ramaiah University of Applied Sciences, Bengaluru, India.
Author information
Authors and affiliations.
REVA University, Bengaluru, India
D. R. Sarvamangala
Ramaiah University of Applied Sciences, Bengaluru, India
Raghavendra V. Kulkarni
You can also search for this author in PubMed Google Scholar
Corresponding author
Correspondence to D. R. Sarvamangala .
Ethics declarations
Conflict of interest.
The authors declare that they have no conflict of interest.
Additional information
Publisher's note.
Springer Nature remains neutral with regard to jurisdictional claims in published maps and institutional affiliations.
Rights and permissions
Reprints and permissions
About this article
Sarvamangala, D.R., Kulkarni, R.V. Convolutional neural networks in medical image understanding: a survey. Evol. Intel. 15 , 1–22 (2022). https://doi.org/10.1007/s12065-020-00540-3
Download citation
Received : 25 March 2020
Revised : 05 October 2020
Accepted : 22 November 2020
Published : 03 January 2021
Issue Date : March 2022
DOI : https://doi.org/10.1007/s12065-020-00540-3
Share this article
Anyone you share the following link with will be able to read this content:
Sorry, a shareable link is not currently available for this article.
Provided by the Springer Nature SharedIt content-sharing initiative
- Classification
- Convolutional neural networks
- Image understanding
- Localization
- Segmentation
- Find a journal
- Publish with us
- Track your research
Medical Imaging 2023: Image Processing
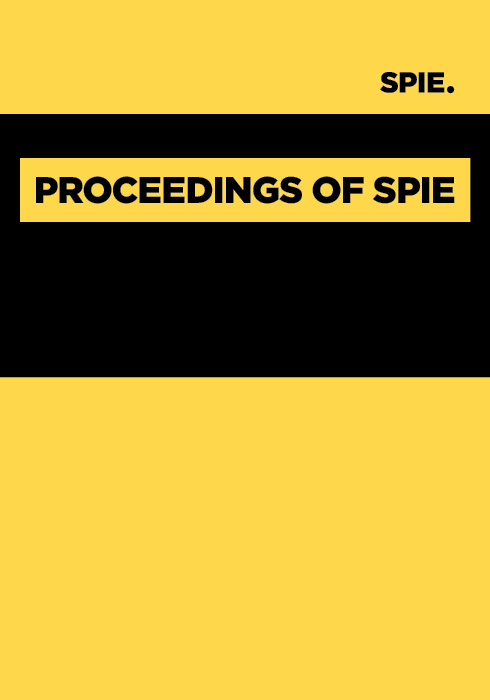
Volume Details
Table of contents.
- Front Matter: Volume 12464
- Registration and Deformable Geometry
- Classification and Segmentation
- Cardiovascular Applications
- Tuesday Morning Keynotes
- Image Synthesis and Generative Models
- Workshop on AI Using Large-Scale Data Warehouses
- Brain Applications
- Image Quality, Harmonization, and Quantitative Analysis
- Deep-Dive Session
- Transformers
- Image Reconstruction, Correction, and Quality
- Segmentation
- Poster Session
- Digital Poster Session
Academia.edu no longer supports Internet Explorer.
To browse Academia.edu and the wider internet faster and more securely, please take a few seconds to upgrade your browser .
- We're Hiring!
- Help Center
Medical Image Processing
- Most Cited Papers
- Most Downloaded Papers
- Newest Papers
- Save to Library
- Last »
- Medical Imaging Follow Following
- Medical Image Analysis Follow Following
- Image Processing Follow Following
- Biomedical Engineering Follow Following
- Biomedical signal and image processing Follow Following
- Computer Vision Follow Following
- Digital Image Processing Follow Following
- Pattern Recognition Follow Following
- Machine Learning Follow Following
- Medical Image Segmentation Follow Following
Enter the email address you signed up with and we'll email you a reset link.
- Academia.edu Publishing
- We're Hiring!
- Help Center
- Find new research papers in:
- Health Sciences
- Earth Sciences
- Cognitive Science
- Mathematics
- Computer Science
- Academia ©2024
- Alzheimer's disease & dementia
- Arthritis & Rheumatism
- Attention deficit disorders
- Autism spectrum disorders
- Biomedical technology
- Diseases, Conditions, Syndromes
- Endocrinology & Metabolism
- Gastroenterology
- Gerontology & Geriatrics
- Health informatics
- Inflammatory disorders
- Medical economics
- Medical research
- Medications
- Neuroscience
- Obstetrics & gynaecology
- Oncology & Cancer
- Ophthalmology
- Overweight & Obesity
- Parkinson's & Movement disorders
- Psychology & Psychiatry
- Radiology & Imaging
- Sleep disorders
- Sports medicine & Kinesiology
- Vaccination
- Breast cancer
- Cardiovascular disease
- Chronic obstructive pulmonary disease
- Colon cancer
- Coronary artery disease
- Heart attack
- Heart disease
- High blood pressure
- Kidney disease
- Lung cancer
- Multiple sclerosis
- Myocardial infarction
- Ovarian cancer
- Post traumatic stress disorder
- Rheumatoid arthritis
- Schizophrenia
- Skin cancer
- Type 2 diabetes
- Full List »
share this!
April 11, 2024
This article has been reviewed according to Science X's editorial process and policies . Editors have highlighted the following attributes while ensuring the content's credibility:
fact-checked
trusted source
New AI method captures uncertainty in medical images
by Adam Zewe, Massachusetts Institute of Technology
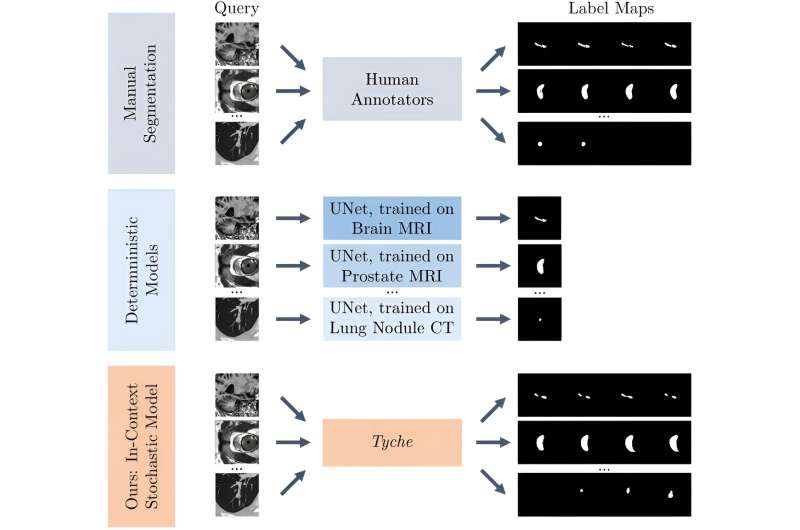
In biomedicine, segmentation involves annotating pixels from an important structure in a medical image, like an organ or cell. Artificial intelligence models can help clinicians by highlighting pixels that may show signs of a certain disease or anomaly.
However, these models typically only provide one answer, while the problem of medical image segmentation is often far from black and white. Five expert human annotators might provide five different segmentations, perhaps disagreeing on the existence or extent of the borders of a nodule in a lung CT image.
"Having options can help in decision-making. Even just seeing that there is uncertainty in a medical image can influence someone's decisions, so it is important to take this uncertainty into account," says Marianne Rakic, an MIT computer science Ph.D. candidate.
Rakic is the lead author of a paper with others at MIT, the Broad Institute of MIT and Harvard, and Massachusetts General Hospital that introduces a new AI tool that can capture the uncertainty in a medical image.
Known as Tyche (named for the Greek divinity of chance), the system provides multiple plausible segmentations that each highlight slightly different areas of a medical image. A user can specify how many options Tyche outputs and select the most appropriate one for their purpose.
Importantly, Tyche can tackle new segmentation tasks without needing to be retrained. Training is a data-intensive process that involves showing a model many examples and requires extensive machine-learning experience.
Because it doesn't need retraining, Tyche could be easier for clinicians and biomedical researchers to use than some other methods. It could be applied "out of the box" for a variety of tasks, from identifying lesions in a lung X-ray to pinpointing anomalies in a brain MRI.
Ultimately, this system could improve diagnoses or aid in biomedical research by calling attention to potentially crucial information that other AI tools might miss.
"Ambiguity has been understudied. If your model completely misses a nodule that three experts say is there and two experts say is not, that is probably something you should pay attention to," adds senior author Adrian Dalca, an assistant professor at Harvard Medical School and MGH and a research scientist in the MIT Computer Science and Artificial Intelligence Laboratory (CSAIL).
Their co-authors include Hallee Wong, a graduate student in electrical engineering and computer science; Jose Javier Gonzalez Ortiz, Ph.D. '23; Beth Cimini, associate director for bioimage analysis at the Broad Institute; and John Guttag, the Dugald C. Jackson Professor of Computer Science and Electrical Engineering. Rakic will present Tyche at the IEEE Conference on Computer Vision and Pattern Recognition, where Tyche has been selected as a highlight.
Addressing ambiguity
AI systems for medical image segmentation typically use neural networks. Loosely based on the human brain, neural networks are machine-learning models comprising many interconnected layers of nodes, or neurons, that process data.
After speaking with collaborators at the Broad Institute and MGH who use these systems, the researchers realized two major issues limit their effectiveness. The models cannot capture uncertainty, and they must be retrained for even a slightly different segmentation task.
Some methods try to overcome one pitfall, but tackling both problems with a single solution has proven especially tricky, Rakic says.
"If you want to take ambiguity into account, you often have to use an extremely complicated model. With the method we propose, our goal is to make it easy to use with a relatively small model so that it can make predictions quickly," she says.
The researchers built Tyche by modifying a straightforward neural network architecture.
A user first feeds Tyche a few examples that show the segmentation task. For instance, examples could include several images of lesions in a heart MRI that different human experts have segmented so the model can learn the task and see that there is ambiguity.
The researchers found that just 16 example images, called a "context set," is enough for the model to make good predictions, but there is no limit to the number of examples one can use. The context set enables Tyche to solve new tasks without retraining.
For Tyche to capture uncertainty, the researchers modified the neural network so it outputs multiple predictions based on one medical image input and the context set. They adjusted the network's layers so that, as data moves from layer to layer, the candidate segmentations produced at each step can "talk" to each other and the examples in the context set.
In this way, the model can ensure that candidate segmentations are all a bit different but still solve the task.
"It is like rolling dice. If your model can roll a two, three, or four but doesn't know you have a two and a four already, then either one might appear again," she says.
They also modified the training process so it is rewarded by maximizing the quality of its best prediction.
If the user asked for five predictions, at the end they can see all five medical image segmentations Tyche produced, even though one might be better than the others.
The researchers also developed a version of Tyche that can be used with an existing, pretrained model for medical image segmentation. In this case, Tyche enables the model to output multiple candidates by making slight transformations to images.
Better, faster predictions
When the researchers tested Tyche with datasets of annotated medical images, they found that its predictions captured the diversity of human annotators and that its best predictions were better than any from the baseline models. Tyche also performed faster than most models.
"Outputting multiple candidates and ensuring they are different from one another really gives you an edge," Rakic says.
The researchers also saw that Tyche could outperform more complex models that have been trained using a large, specialized dataset.
For future work, they plan to try using a more flexible context set, perhaps including text or multiple types of images. In addition, they want to explore methods that could improve Tyche's worst predictions and enhance the system so it can recommend the best segmentation candidates.
The research is published on the arXiv preprint server.
This story is republished courtesy of MIT News ( web.mit.edu/newsoffice/ ), a popular site that covers news about MIT research, innovation and teaching.
Explore further
Feedback to editors

Two key brain systems are central to psychosis, study finds
5 hours ago

COVID-19 vaccine effectiveness: Results from Norway demonstrate the reproducibility of federated analytics
8 hours ago

Elucidating the link between Guillain–Barré syndrome and Takotsubo cardiomyopathy
9 hours ago

Artificial intelligence can help people feel heard, study finds

A new diagnostic model offers hope for Alzheimer's
10 hours ago

New study validates prediction rules for pediatric intra-abdominal and traumatic brain injuries

Chemicals stored in home garages linked to amyotrophic lateral sclerosis risk

In the drive to deprescribe, heartburn drug study teaches key lessons

Researchers identify new genetic risk factors for persistent HPV infections

Survey of mental health and exposure to blasts reveals differences among displaced people who remained in Ukraine
11 hours ago
Related Stories

New method for addressing the reliability challenges of neural networks in inverse imaging problems
Jan 16, 2024

A new method for cardiac image segmentation
Feb 20, 2024

Images of simulated cities help artificial intelligence to understand real streetscapes
Sep 14, 2023

Efficient technique improves machine-learning models' reliability
Feb 13, 2023

Microscopy image segmentation via point and shape regularized data synthesis
Oct 3, 2023

A weakly supervised machine learning model to extract features from microscopy images
May 16, 2022
Recommended for you

Scientists use wearable technology to detect stress levels during sleep

Decoding spontaneous thoughts from the brain via machine learning
12 hours ago

'Virtual biopsy' lets clinicians analyze skin noninvasively
Apr 10, 2024

New AI tool creates virtual model of the infant microbiome and predicts neurodevelopmental deficits
Let us know if there is a problem with our content.
Use this form if you have come across a typo, inaccuracy or would like to send an edit request for the content on this page. For general inquiries, please use our contact form . For general feedback, use the public comments section below (please adhere to guidelines ).
Please select the most appropriate category to facilitate processing of your request
Thank you for taking time to provide your feedback to the editors.
Your feedback is important to us. However, we do not guarantee individual replies due to the high volume of messages.
E-mail the story
Your email address is used only to let the recipient know who sent the email. Neither your address nor the recipient's address will be used for any other purpose. The information you enter will appear in your e-mail message and is not retained by Medical Xpress in any form.
Newsletter sign up
Get weekly and/or daily updates delivered to your inbox. You can unsubscribe at any time and we'll never share your details to third parties.
More information Privacy policy
Donate and enjoy an ad-free experience
We keep our content available to everyone. Consider supporting Science X's mission by getting a premium account.
E-mail newsletter
Suggestions or feedback?
MIT News | Massachusetts Institute of Technology
- Machine learning
- Social justice
- Black holes
- Classes and programs
Departments
- Aeronautics and Astronautics
- Brain and Cognitive Sciences
- Architecture
- Political Science
- Mechanical Engineering
Centers, Labs, & Programs
- Abdul Latif Jameel Poverty Action Lab (J-PAL)
- Picower Institute for Learning and Memory
- Lincoln Laboratory
- School of Architecture + Planning
- School of Engineering
- School of Humanities, Arts, and Social Sciences
- Sloan School of Management
- School of Science
- MIT Schwarzman College of Computing
New AI method captures uncertainty in medical images
Press contact :, media download.
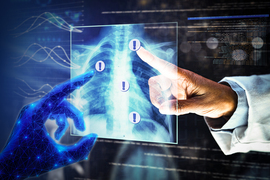
*Terms of Use:
Images for download on the MIT News office website are made available to non-commercial entities, press and the general public under a Creative Commons Attribution Non-Commercial No Derivatives license . You may not alter the images provided, other than to crop them to size. A credit line must be used when reproducing images; if one is not provided below, credit the images to "MIT."
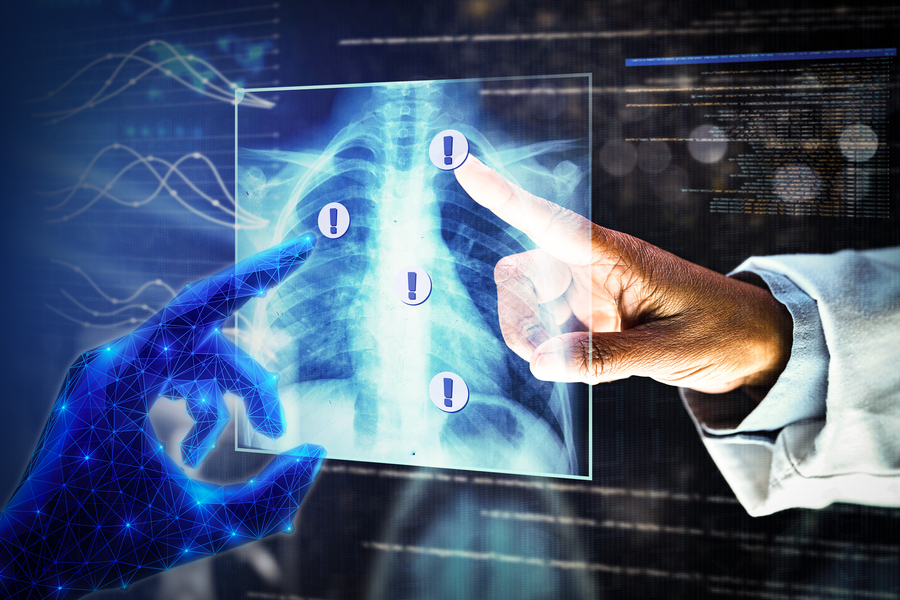
Previous image Next image
In biomedicine, segmentation involves annotating pixels from an important structure in a medical image, like an organ or cell. Artificial intelligence models can help clinicians by highlighting pixels that may show signs of a certain disease or anomaly.
However, these models typically only provide one answer, while the problem of medical image segmentation is often far from black and white. Five expert human annotators might provide five different segmentations, perhaps disagreeing on the existence or extent of the borders of a nodule in a lung CT image.
“Having options can help in decision-making. Even just seeing that there is uncertainty in a medical image can influence someone’s decisions, so it is important to take this uncertainty into account,” says Marianne Rakic, an MIT computer science PhD candidate.
Rakic is lead author of a paper with others at MIT, the Broad Institute of MIT and Harvard, and Massachusetts General Hospital that introduces a new AI tool that can capture the uncertainty in a medical image.
Known as Tyche (named for the Greek divinity of chance), the system provides multiple plausible segmentations that each highlight slightly different areas of a medical image. A user can specify how many options Tyche outputs and select the most appropriate one for their purpose.
Importantly, Tyche can tackle new segmentation tasks without needing to be retrained. Training is a data-intensive process that involves showing a model many examples and requires extensive machine-learning experience.
Because it doesn’t need retraining, Tyche could be easier for clinicians and biomedical researchers to use than some other methods. It could be applied “out of the box” for a variety of tasks, from identifying lesions in a lung X-ray to pinpointing anomalies in a brain MRI.
Ultimately, this system could improve diagnoses or aid in biomedical research by calling attention to potentially crucial information that other AI tools might miss.
“Ambiguity has been understudied. If your model completely misses a nodule that three experts say is there and two experts say is not, that is probably something you should pay attention to,” adds senior author Adrian Dalca, an assistant professor at Harvard Medical School and MGH, and a research scientist in the MIT Computer Science and Artificial Intelligence Laboratory (CSAIL).
Their co-authors include Hallee Wong, a graduate student in electrical engineering and computer science; Jose Javier Gonzalez Ortiz PhD ’23; Beth Cimini, associate director for bioimage analysis at the Broad Institute; and John Guttag, the Dugald C. Jackson Professor of Computer Science and Electrical Engineering. Rakic will present Tyche at the IEEE Conference on Computer Vision and Pattern Recognition, where Tyche has been selected as a highlight.
Addressing ambiguity
AI systems for medical image segmentation typically use neural networks . Loosely based on the human brain, neural networks are machine-learning models comprising many interconnected layers of nodes, or neurons, that process data.
After speaking with collaborators at the Broad Institute and MGH who use these systems, the researchers realized two major issues limit their effectiveness. The models cannot capture uncertainty and they must be retrained for even a slightly different segmentation task.
Some methods try to overcome one pitfall, but tackling both problems with a single solution has proven especially tricky, Rakic says.
“If you want to take ambiguity into account, you often have to use an extremely complicated model. With the method we propose, our goal is to make it easy to use with a relatively small model so that it can make predictions quickly,” she says.
The researchers built Tyche by modifying a straightforward neural network architecture.
A user first feeds Tyche a few examples that show the segmentation task. For instance, examples could include several images of lesions in a heart MRI that have been segmented by different human experts so the model can learn the task and see that there is ambiguity.
The researchers found that just 16 example images, called a “context set,” is enough for the model to make good predictions, but there is no limit to the number of examples one can use. The context set enables Tyche to solve new tasks without retraining.
For Tyche to capture uncertainty, the researchers modified the neural network so it outputs multiple predictions based on one medical image input and the context set. They adjusted the network’s layers so that, as data move from layer to layer, the candidate segmentations produced at each step can “talk” to each other and the examples in the context set.
In this way, the model can ensure that candidate segmentations are all a bit different, but still solve the task.
“It is like rolling dice. If your model can roll a two, three, or four, but doesn’t know you have a two and a four already, then either one might appear again,” she says.
They also modified the training process so it is rewarded by maximizing the quality of its best prediction.
If the user asked for five predictions, at the end they can see all five medical image segmentations Tyche produced, even though one might be better than the others.
The researchers also developed a version of Tyche that can be used with an existing, pretrained model for medical image segmentation. In this case, Tyche enables the model to output multiple candidates by making slight transformations to images.
Better, faster predictions
When the researchers tested Tyche with datasets of annotated medical images, they found that its predictions captured the diversity of human annotators, and that its best predictions were better than any from the baseline models. Tyche also performed faster than most models.
“Outputting multiple candidates and ensuring they are different from one another really gives you an edge,” Rakic says.
The researchers also saw that Tyche could outperform more complex models that have been trained using a large, specialized dataset.
For future work, they plan to try using a more flexible context set, perhaps including text or multiple types of images. In addition, they want to explore methods that could improve Tyche’s worst predictions and enhance the system so it can recommend the best segmentation candidates.
This research is funded, in part, by the National Institutes of Health, the Eric and Wendy Schmidt Center at the Broad Institute of MIT and Harvard, and Quanta Computer.
Share this news article on:
Related links.
- Marianne Rakic
- Adrian Dalca
- John Guttag
- Computer Science and Artificial Intelligence Laboratory
- Department of Electrical Engineering and Computer Science
- Broad Institute of MIT and Harvard
Related Topics
- Computer science and technology
- Artificial intelligence
- Health care
- Computer Science and Artificial Intelligence Laboratory (CSAIL)
- Electrical Engineering & Computer Science (eecs)
- Broad Institute
- National Institutes of Health (NIH)
Related Articles
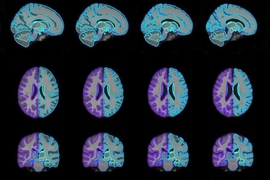
Producing better guides for medical-image analysis
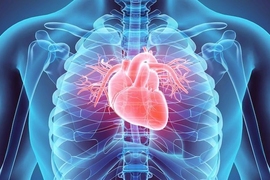
Using machine learning to estimate risk of cardiovascular death
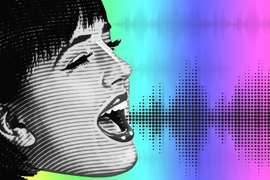
Automating artificial intelligence for medical decision-making
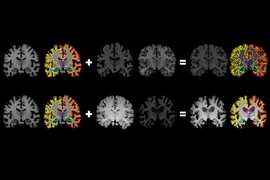
From one brain scan, more information for medical artificial intelligence
Previous item Next item
More MIT News
A biomedical engineer pivots from human movement to women’s health
Read full story →
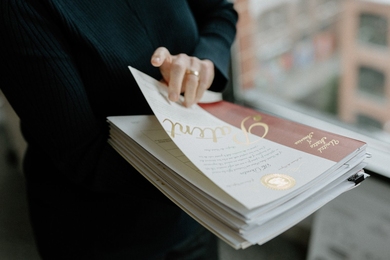
MIT tops among single-campus universities in US patents granted
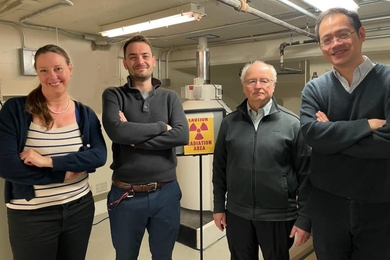
A new way to detect radiation involving cheap ceramics
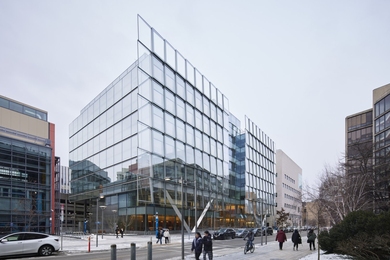
A crossroads for computing at MIT
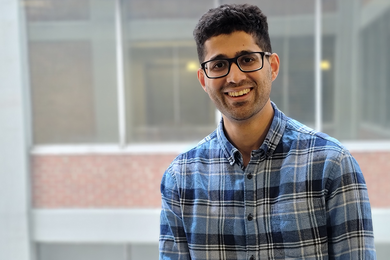
Growing our donated organ supply
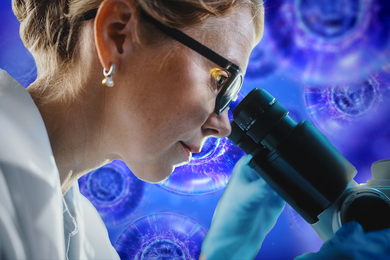
Improving drug development with a vast map of the immune system
- More news on MIT News homepage →
Massachusetts Institute of Technology 77 Massachusetts Avenue, Cambridge, MA, USA
- Map (opens in new window)
- Events (opens in new window)
- People (opens in new window)
- Careers (opens in new window)
- Accessibility
- Social Media Hub
- MIT on Facebook
- MIT on YouTube
- MIT on Instagram

An official website of the United States government
The .gov means it’s official. Federal government websites often end in .gov or .mil. Before sharing sensitive information, make sure you’re on a federal government site.
The site is secure. The https:// ensures that you are connecting to the official website and that any information you provide is encrypted and transmitted securely.
- Publications
- Account settings
Preview improvements coming to the PMC website in October 2024. Learn More or Try it out now .
- Advanced Search
- Journal List
- Elsevier - PMC COVID-19 Collection

Medical image processing and COVID-19: A literature review and bibliometric analysis
Rabab ali abumalloh.
g Computer Department, Applied College, Imam Abdulrahman Bin Faisal University, P.O. Box. 1982, Dammam, Saudi Arabia
Mehrbakhsh Nilashi
h Centre for Global Sustainability Studies (CGSS), Universiti Sains Malaysia, 11800, USM Penang, Malaysia
Muhammed Yousoof Ismail
a Department of MIS, Dhofar University, Oman
Ashwaq Alhargan
b Computer Science Department, College of Computing and Informatics, Saudi Electronic University, Saudi Arabia
Abdullah Alghamdi
c Information Systems Dept., College of Computer Science and Information Systems, Najran University, Najran, Saudi Arabia
Ahmed Omar Alzahrani
d College of Computer Science and Engineering, University of Jeddah, 21959 Jeddah, Saudi Arabia
Linah Saraireh
e Management Information System Department, College of Business, Imam Abdulrahman Bin Faisal University, P.O. Box 1982, Dammam, Saudi Arabia
Shahla Asadi
f Centre of Software Technology and Management, Faculty of Information Science and Technology, Universiti Kebangsaan Malaysia, 43600 Bangi, Selangor, Malaysia
COVID-19 crisis has placed medical systems over the world under unprecedented and growing pressure. Medical imaging processing can help in the diagnosis, treatment, and early detection of diseases. It has been considered as one of the modern technologies applied to fight against the COVID-19 crisis. Although several artificial intelligence, machine learning, and deep learning techniques have been deployed in medical image processing in the context of COVID-19 disease, there is a lack of research considering systematic literature review and categorization of published studies in this field. A systematic review locates, assesses, and interprets research outcomes to address a predetermined research goal to present evidence-based practical and theoretical insights. The main goal of this study is to present a literature review of the deployed methods of medical image processing in the context of the COVID-19 crisis. With this in mind, the studies available in reliable databases were retrieved, studied, evaluated, and synthesized. Based on the in-depth review of literature, this study structured a conceptual map that outlined three multi-layered folds: data gathering and description, main steps of image processing, and evaluation metrics. The main research themes were elaborated in each fold, allowing the authors to recommend upcoming research paths for scholars. The outcomes of this review highlighted that several methods have been adopted to classify the images related to the diagnosis and detection of COVID-19. The adopted methods have presented promising outcomes in terms of accuracy, cost, and detection speed.
Introduction
As an international health crisis, COVID-19 gained the global attention of researchers, health organizations, and governments. As indicated by WHO [ 1 ], the mortality rate of COVID-19 is around 2.06, with around 224,511,226 confirmed cases, entailing 4,627,540 deaths by September 2021. A small ratio of patients can suffer from breathing difficulties, septic shock, or organ malfunctioning [ 2 ]. Several factors have been linked to the mortality rate of COVID-19; such as the availability of healthcare resources [ 3 ], environmental factors [ 4 ], and socioeconomic factors [ 5 ]. In general, elderly people and patients who suffer from severe signs constitute the majority of COVID-19 deaths. Mortality rate increases by 3.5% with every ratio point increase in the proportion of the overweight among people in the high-income regions [ 6 ]. Accordingly, COVID-19 has been regarded as a serious national and international health crisis in the twenty-one decade [ [7] , [8] , [9] , [10] , [11] , [12] ].
Refereeing to specialists’ points of view, the coronavirus primarily impacts the respiratory system causing severe pneumonia with various signs of high temperature, breathlessness, dry cough, and exhaustion [ [13] , [14] , [15] ]. The available regular confirmed clinical examination, reverse transcription-polymerase chain reaction (RT-PCR), for identifying the virus, is manually actuated, complicated, and consumes a considerable time [ 16 ]. The restricted supply of test kits, the lack of field specialists in the health care organizations, and the fast increase in the number of diseased people indicate the need for an automated screening approach, which can be utilized as an additional aid for specialists to rapidly detect the disease, particularly among people who might need fast medical treatment.
Given the quick ratio of scientific development and the utilization of medical data gathered by a huge number of individuals infected by COVID-19, researchers can analyze the available data to present potential solutions that can help in treating and detecting the disease [ 17 ]. Still, the demand for high-efficiency computation has commonly been linked with pricy software systems. On the other hand, many research fields have grown considerably without the necessity for costly investment in computational systems such as modeling and simulation [ 18 ], social sciences [ 19 ], statistical analysis [ 19 ], data-intensive programs [ 19 ], online design automation [ 19 ], and image processing [ [20] , [21] , [22] , [23] , [24] , [25] ]. These research fields have presented promising directions for researchers in several contexts and domains.
Nowadays, researchers in data analysis and data science are considerably inspecting health-related data to aid in medical research development. Regarding this, deep learning, data mining, pattern recognition, and machine learning approaches have been adopted to extract related features from image data sets and categorize them for appropriate disease detection and prediction [ [26] , [27] , [28] , [29] , [30] ]. Medical imaging is an ongoing and developing field in which advanced computer-aided algorithms can be utilized in the recognition, diagnosis, and surgical planning of a particular treatment [ 31 ]. Severe inflammation of the cavities and bronchioles can be detected in COVID-19 infected people; this is caused by the harm caused to their lungs. The indications obtained using X-ray, Lung Ultrasound (US), and Computed Tomography (CT) images are essential in reaching an appropriate medical diagnosis. With the utilization of a suitable approach, researchers can present considerable aid in the inspection of the COVID-19 available image datasets and demonstrate logical results that can help in fighting the disease.
Although image processing has been utilized in medical researches and gets the attention of researchers in numerous fields [ 32 , 33 ], in the context of COVID-19 diagnosis and detection research, image processing is still in its earlier phases. Besides, although the preliminary studies have indicated hopeful outcomes by utilizing imaging modalities for the diagnosis of COVID-19, there has been no previous work to systematically revise and synthesize this area of research, to present a plain perception of image processing for scholars and medical experts.
In order to fill this gap, this study conducted a systematic literature review (SLR) approach to define several articles in previous literature related to the detection and diagnosis of COVID-19 using modalities of medical imaging. SLR presents a means for the assessment and synthesis of the current research which is related to a particular field, addressing a particular question, or exploring a phenomenon that attracts researchers’ interest [ 34 ]. Additionally, this paper investigates the adopted methods used for the detection of COVID-19 based on image processing techniques. This study also presents a conceptual map to describe the research themes related to data gathering, processing, and evaluation in the surveyed studies. The rest of this research is systematized as follows. Section “Methodology of research” describes the research methodology applied in this SLR. Section “Data synthesis” presents the synthesis of the studies and reveals a conceptual map to elaborate on research themes in the surveyed studies. Section “Discussion” discusses the outcomes. Section “Limitation and future research direction”, presents the limitations and future work. Finally, the conclusion of this study is presented in Section 6. To simplify, a list of the abbreviations used in this study is presented in Table 1 .
List of abbreviations.
Methodology of research
Research protocol.
Systematic reviews start by defining a review protocol that considers the research objective being addressed and the methods to perform the study [ 34 ]. In this research, we conducted a SLR to investigate the published studies in the context of COVID-19 and image processing, as a potential approach to disease diagnosis. The research aims particularly to explore the adopted techniques and the utilized imaging modalities in the surveyed studies. To achieve the research objective, we employed a multi-disciplinary analysis of the surveyed studies by assessing and including the articles relevant to the study context. The review protocol of this study is presented in Fig. 1 .

The review protocol.
Search keywords and search process
We conducted a review of eight electronic databases: Elsevier, IEEE, PubMed, Wiley Online Library, Springer, Summon, Google Scholar, and Taylor and Francis to locate the researches that meet the research objective using predetermined keywords. In previous studies of systematic literature review, several and various electronic databases were used to retrieve the studies. The chosen electronic resources have been used in conducting systematic literature reviews in several disciplines and contexts [ [35] , [36] , [37] , [38] , [39] ]. The following search keywords were used to download the relevant articles: “COVID-19” OR “Coronavirus” OR “SARS-CoV-2” AND “Pandemic” OR “Crisis” OR “Disease” AND “Medical Image Analysis” OR “Medical image processing”. These studies are related to the application of image processing for the SARS-CoV-2 diagnosis. Considering the novelty and freshness of the research topic, no previous SLR was conducted in this area. To meet a certain quality level in the surveyed studies, the authors decided not to include case reports, case series, and preprints. The search was enlarged by utilizing a snowballing approach by inspecting the available references in the surveyed studies.
Identification of the inclusion and exclusion criteria
Research abstracts were read carefully to specify the studies that will be included in the SLR. Each study was checked in terms of the inclusion and exclusion conditions to decide to keep it for the next stages or not. The inclusion criteria are (1) area of the study: the study should fall within the image processing area, particularly, related to the COVID-19 crisis; (2) method: the method applied in image processing related to the diagnosis of the COVID-19; (3) complete studies and (4) English-language studies. The exclusion criteria are: (1) another domain rather than the research topic, (2) duplicated studies: we found many studies in which the study can be found in more than one electronic database; (3) preprint studies, commentary, or letter to the editor were excluded from the SLR; (4) uncompleted studies, and (5) non-English studies.
Quality assessment (QA)
This study referred to Kitchenham’s guidelines for conducting a systematic literature review. Hence, each included study should be evaluated, referring to some predefined quality criteria. Thus, we created a checklist to enable the evaluation of the research studies after fully reading the included studies. The quality assessment criteria were proposed to help the researcher to meet the research objective [ 34 ]. The following conditions were explored in the quality evaluation procedure:
QA1. Is the subject considered in the research related directly to image processing and COVID-19 diagnosis?
QA2. Is the research approach elaborated in the research?
QA3. Are the data gathering methods and the adopted dataset clarified in the research?
QA4. Are the analysis methods of the data elaborated in the research?
The four QA criteria presented above were applied to the 73 studies to strengthen our trust in the surveyed studies’ reliability. The authors utilized three degrees of quality to assess the articles as follows: high, medium, and low [ 40 , 41 ], in which the overall quality of each study depends on the sum of the calculated outcomes for all QA criteria. Studies that fulfill the criteria will be granted 2; studies that moderately fulfill the criteria will be granted 1; studies that do not outline the criteria will be given 0. Studies that have a score of 5 or more will be considered high, if they have a score of 4 they will be considered average, and if they get less than 4, they will be regarded as low quality and removed from the SLR. Following the adoption of the QA, 63 studies were kept because they fulfill the QA criteria.
Data extraction
The authors performed manual data extraction focusing on specific items to understand the research approach, research goal, research outcomes, and synthesize the outcomes in the surveyed studies. The gathering and classification of the research allowed us to get various, critical, and accurate findings. Table 2 presents extracted items in the final studies.
Data extraction Items in the final studies.
Distribution of studies by electronic database
This study included eight electronic databases to retrieve the studies. The distribution of the surveyed studies by electronic resources is presented in Fig. 2 . The majority of studies were published in Springer and Elsevier, with a percentage of 25.40%, each. Followed by IEEE and Summon with 19.05% of the surveyed databases, each. PubMed was ranked next with around 4.8% of the surveyed databases. Whereas 2 studies (3.17%) were published in Willey Online Library. Finally, each of Summon, Tylor & Francis has only one published study that is included in the surveyed studies.

Distribution of studies by electronic database.
Data synthesis
Keyword co-occurrence network.
In bibliometric studies, two map classes can be utilized [ 42 ]; distance-based and graph-based diagrams. A distance-based keyword diagram sets up the distance between two keywords. The distance identifies the intensity of the link between the keywords. In this study, we utilized the VOSviewer program to measure distance-based keyword segmentation. A visualization of the keyword co-occurrence network is presented in Fig. 3 . The distance among the chosen items is developed by calculating how many studies that entail both items (which in our study indicate the keywords presented in the study). A huge number of co-occurrences is reflected by a short link among the chosen items. That distance is indicated in the co-occurrence graph and utilized to present the segments. Besides, bigger circles indicate more occurrences of the studies, hence, as the figure presents, “COVID-19” and “deep learning” are the most frequent keywords in the selected studies.

Keyword co-occurrence network visualization.
Term co-occurrence network
Additionally, based on the text data of the abstracts and titles of the studies, a term-co occurrence map was presented. The program was set up to ignore both structured abstract labels and copyright statements. Binary counting was used and the minimum number of occurrences among the selected studies was 3, hence, 236 terms met the pre-determined condition. For each of the 236 terms, a relevance score was measured. Finally, based on the relevance score, the most relevant items were chosen, hence, 142 items were kept. A visualization of the term co-occurrence network based on text data of the surveyed studies is presented in Fig. 4 . In the figure, three clusters of items were generated, each color represents a particular cluster of items. The red cluster focused on the applied technique and the evaluation measures of these techniques. This cluster includes terms like “X-ray image”, “sensitivity”, and “specificity”. The green cluster concentrated on the approaches used in image processing. As it can be seen in the figure, terms like: “screening”, “extraction”, and “ground-glass opacity” are included in this cluster. The blue cluster focused on the virus and the pandemic in general. Terms like: “world health organization”, “contribution”, “review”, “application”, and “survey” are included in this cluster. The relevance scores of the items are presented in Appendix C .

A visualization of term co-occurrence network based on text data.
Co-authorship network
Additionally, co-authorship-countries associations between authors are presented in Fig. 5 . The paths exhibit the number of co-authorship associations between a particular author with other authors. The path strength indicates the strength of the co-authorship association of a particular author with other authors. We set the program to the full counting method. In this method, if the strength of any path in the diagram is represented by A, this means that the two have co-authored A of researches. The total link strength ranges from 0 to 13, in which 14 countries have a total link strength more than three. This reflects the firm cooperation among several researchers in several countries in the study topic.

A visualization of co-authorship-countries network.
A conceptual framework of the surveyed studies
This study is based on an in-depth literature review, giving an overview of the research area to explore the steps involved in the image processing starting from data gathering until COVID-19 diagnosis. Hence, we examine these steps and highlight the basic themes and notions involved in each step. Besides, this study presents a conceptual map that can guide scholars for the future and possible research directions. The three basic folds that arise are presented in Fig. 6 : (1) data gathering and description, (2) the main steps of image processing, and (3) the evaluation metrics. We will outline each fold and its basic themes in which we see possible future research directions and address potential research gaps. In Fig. 7 we present a mind map that includes: (1) a description of research themes related to resources in the surveyed studies, (2) a description of research themes and notions related to the steps involved in COVID-19 diagnosis, and (3) a brief description of each of the main evaluation indicators related to the surveyed studies. We will elaborate more on these figures in the “Discussion” Section.

Conceptual map for research themes and notions.

Mind map for research themes and notions.
COVID-19 tests reverse transcriptase-polymerase chain reaction (RT-PCR) are utilized broadly to detect the disease in regions where the crisis is spreading [ 43 ]. RT-PCR can detect viral nucleotides from specimens gathered by nasopharyngeal swab, oropharyngeal swab, tracheal aspirate, or bronchoalveolar lavage. Still, these tests are restricted in terms of accuracy, speed, cost, and supply [ 44 , 45 ]. Several COVID-19 cases could not be specified because of the low accuracy of RT-PCR tests. Hence, the RT-PCR test might require to be replicated many times in some cases to confirm the results [ 45 ]. Another obstacle faced by health organizations is the speed of obtaining the outcomes of the test, which usually ranges from hours to days [ 46 , 47 ]. The lack of accurate and fast disease detection approach can cause patients to spread the disease to the community without knowing that they are infected [ 48 ]. Furthermore, patients who require urgent medical care might not get suitable therapy at the right time. Additionally, RT-PCR exposes healthcare employees to a greater risk of being infected through the test. Another restriction is the cost of the supply of substances utilized in the testing kits. Although the cost differs from region to region over the world, the test kit price might reach around 60$ [ 49 ]. The cost depends also on the availability of the tests, which relies on the resources and population of the country. All these aspects indicate that other detection approaches need to be adopted to substitute RT- PCR test. Hence, there is a demand to explore other symptomatic approaches for detecting and diagnosis COVID-19. Other methods, which utilize imaging modalities for detecting the disease, can be applied as a replacement to the RT-PCR test for the detection of COVID-19 [ [50] , [51] , [52] , [53] , [54] , [55] , [56] ].
There are three main folds to present regarding the topic of the study: image processing modalities, COVID-19 diagnosis approaches, and steps of COVID-19 diagnosis.
Image processing modalities
Previous literature indicated that COVID-19 induces irregularity in human lungs which appears in the chest X-rays and CT images. This malformation can be in the form of ground-glass opacities. Besides, the general indication in all medical symptoms related to lung ultrasonography is the existence of various line artifacts. This entails the presence of A-lines and B-lines, in which the diagnosis of these lines is highly important. B-lines usually exist in the lung with interstitial edema. The existence or lack of these lines, the kind, and the number of B-lines in chest US can be utilized as an indication of COVID-19 disease. A dense and abnormality-shaped pleural line, along with the presence of focal, varifocal, and confluent vertical B-lines are vital signs of the COVID-19. On the other hand, the presence of A-lines is important evidence in the recovery stage [ 57 ].
People’s lives could be protected, the increase of the illness might be restrained, and large data could be obtained from artificial intelligence patterns with the fast and accurate detection of COVID-19 [ 44 ]. To achieve this goal, health care specialists can utilize X-rays, CT scans, and US imaging modalities in their clinical studies. COVID-19 traditional examinations have possible disadvantages represented by the lack of supply and high expenses of tests [ 46 , 47 ]. On the other hand, all emergency health centers have X-Rays and CT devices. Digital medical images have several kinds that were utilized in the surveyed studies. These types differ from each other in terms of how they are made and the task they perform. Datasets and imaging modalities in the surveyed studies are presented in Appendix B . The main types of imaging modalities explored in the surveyed studies are:
- i. X-ray: is a kind of radiation called electromagnetic waves. X-ray radiology contains radiant X-ray photons that move through body organs [ 58 ]. Based on the kind of body tissue, it allows or reduces the amount of the passed photons, leading to the generation of an image with a range of black and white shadows. This occurs because various tissues consume various quantities of radiation. X-rays penetrate body organs to generate a 2-D photo of the internal human body. Bones consume X-rays the highest, so they appear in the image in white, while other tissues appear in gray, and finally, the lung appears in black because the air consumes the least radiation. In the surveyed studies, the X-ray modality was explored in 39 studies.

Distribution of studies by imaging modality.
- iii. The ultrasound image is a kind of sonography imaging that can be generated using ultrasonic devices. They can be used in health applications because of their reasonable cost and their ability to generate real-time photos with high quality. US images have been utilized in medical applications because they capture body organs’ images using high-frequency sound waves. In the surveyed studies, US images were explored in three studies.
COVID-19 diagnosis approaches using image processing
Artificial intelligence (AI) has been utilized in many aspects such as image classification, big data analysis, disease diagnosis [ 59 ]. AI approaches have been broadly adopted for speeding up the advancement of medical and biological researches [ 60 ]. ML, DL, and PR approaches mainly concentrate on finding solutions and reaching suitable decisions regarding current and emergent problems. In general, in such systems, datasets are pre-processed, segmented, the system is trained, tested, and then new data can be classified. Particularly, in a training step, the input data is pre-processed. Following that, significant features are extracted. The preprocessing stage is required to keep the original space of the data and entails procedures to reduce the noise and to rectify the image. As individuals infected by COVID-19 may experience lung inflammation as the virus attacks the lungs, the diagnosis of the COVID-19 through imaging modalities analysis can be effectively applied using AI techniques [ 61 ].
DL can be adopted to obtain medical image data to present indicators on the molecular condition, disease diagnosis, disease progress, or medicine sensitivity [ 62 ]. The development in image processing research has been utilized by the advancement of DL-underlying methods, allowing the involvement of many images and dealing with image differences. DL is well recognized these days, referring to its performance, especially in image segmentation and classification models [ 63 ]. Several kinds of DL approaches were utilized to serve several aims, e.g., object segmenting, classification, disease diagnosis, and speech recognition. In the surveyed studies, the most adopted DL technique is the convolution neural networks (CNN), as it was applied in 40 studies, as presented in Appendix A .
CNN is a significant approach in image processing, which allows accurate classification of pneumonia affected and normal samples when medical images are provided [ 64 ]. CNN has been broadly utilized as a promising imaging approach since its original launch [ 65 ]. As a kind of deep neural network, CNN has achieved high performance in categorizing and classifying images through the extraction of images’ topological features [ 66 , 67 ]. CNN also adopts a layered perceptron-driven structure that consists of fully connected networks, in which each neuron in one layer is tied to all neurons in other layers. CNN has three kinds of layers, with each type performing a particular function; (1) convolutional, (2) pooling, and (3) fully connected. In this structure, the convolutional layer works to extract the features. Following that, the fully connected layer utilizes the extracted features to determine which class belongs to the existing input. A pooling layer is responsible for minimizing the dimensions of feature maps and network parameters. Although CNN’s presents the best outcomes on huge input data, they need large data and computational resources to train. In the case of limited input data, which might not be adequate to train a CNN from scrape, the performance of CNNs can be leveraged and the computational costs can be reduced by using transfer learning approaches [ 68 ].
In the surveyed studies, several architectures of CNN were deployed. Karthik et al. [ 69 ] used two architectures of CNN; CSDB and CSDB-DFL. These two architectures provide double residual connectivity among blocks, connected network links, the relation of variably sized receptive fields, and channel-shuffling. Heidari et al. [ 70 ] utilized the VGG16 model in a transfer learning methodology regarding its effectiveness in image classification tasks. Turkoglu [ 71 ] utilized CNN-based AlexNet architecture in a transfer learning methodology. Compared to VGG16, the proposed AlexNet architecture presented a better performance in terms of classification accuracy. Goel et al. [ 72 ] proposed a new architecture named OptCoNet, which was used for feature extraction and classification tasks. The proposed system presented a high performance in terms of accuracy (97.78%). Sahlol et al. [ 73 ] proposed a new approach (FO-MPA), in which a CNN was used to perform the feature extraction task, while the Marine Predators Algorithm (MPA) was used for feature selection. Panwar et al. [ 49 ] proposed a new model for COVID-19 detection (nCOVnet), in which VGG16 was used for extracting the features. The proposed model was based on transfer learning in the training stage. Pathak et al. [ 74 ] deployed the ResNet-50 network for the feature extraction task. The transfer learning approach was used in the COVID-19 detection and presented effective outcomes compared to other related models. Duran-Lopez et al. [ 57 ] proposed a new model, entitled COVID-XNet, based on a deep learning approach. The proposed model presented an overall accuracy of 94.43%. Xu et al. [ 75 ] proposed a new 3D deep learning approach. In the proposed approach, two classification schemes were used based on the ResNet-18 model and presented an overall accuracy of 86.7%. Bahadur Chandra et al. [ 76 ] proposed a new system for COVID-19 diagnosis using radiomic texture descriptors to classify CXR images. Toğaçar et al. [ 60 ] deployed two deep learning models for the training process; MobileNetV2 and SqueezeNet. The classification process was performed using SVM tecnique. The overall accuracy of the proposed system was 99.27%, which outperform other state of art approaches. Ismael and Şengür [ 77 ] used the ResNet50 model for feature extraction and fine-tuning tasks while SVM was used for the classification process, in which an overall accuracy of 99.29% was acheived. A new model was proposed by Ucar and Korkmaz [ 78 ], entitled as COVIDiagnosis-Net. The new system was based on Bayes-SqueezeNet. Loey et al. [ 79 ] deployed DTL tecnique through using two models; Googlenet and Alexnet. The deployed tecnique was evaluated in different contexts and presnted promising outcomes. Several deep learning models; VGG19, VGG16, Inception-ResNet-V2, InceptionV3, DenseNet121, Xception, and Resnet50, were used in a comparative study by Shazia et al. [ 80 ]. Among the deployed models, DenseNet121 presented the best performance in terms of classification accuracy.
The deep transfer learning (DTL) approach could be used to overcome overfitting and under-fitting issues in small training input data, by taking benefits of a primary trained CNN using large-scale input data [ 70 ]. DTL methods can train the weights of networks on big input data and hyper-tuning the weights of pre-trained networks on small input data [ 81 ]. Furthermore, by utilizing DTL, the training time can be minimized, mathematical measurements can be reduced, and the intake of the obtainable hardware resources can be minimized [ 79 ]. The transfer learning approach was used in several models in the surveyed literature [ 49 , 59 , [69] , [70] , [71] , 73 , 74 , 79 , [81] , [82] , [83] ].
Usually, the TL method is adopted when the available number of samples for the training step, in models entailing CNN architecture, is inadequate. Still, the transfer of trained features with general images can influence the performance of the deployed model. To overcome this limitation, a robust FE method is required. It is not often suitable to deploy traditional supervised approaches to inspect newly emerged input data entailing an inadequate number of observations. Furthermore, unbalanced input data might be unsuitable for the training process. When it is not feasible to locate additional labeled data, these input data can be efficiently delineated by features. Unbalanced input data issues can be addressed by conducting double-step data replication instead of one-sided data replication to present acceptable outcomes [ 84 ].
Steps of COVID-19 diagnosis
In the following subsections, we will present the main procedures that were utilized in the surveyed studies starting from data pre-processing, feature extraction, feature selection, to classification.
Image pre-processing and augmentation
Image pre-processing basically aims to enhance the quality of images included in the dataset; which accordingly enhances the display of particular pathologists and enhances the outcomes of FE and segmentation approaches [ 85 ]. The use of image processing approaches entails the management of digital photos by adopting several stages. Pre-processing step improves the quality and size of the obtained dataset. Several procedures can be conducted to achieve the following goals: reducing the noise in the initial images, enhancing the quality through raising the contrast, and removing the high/low frequencies [ 32 ]. This entails rifting the insufficient dataset into a substantial one. Several transformations can be applied to the dataset, including scaling, cropping, flipping, filtering, and rotation. Hence, each image can be transformed into a new one, by including required information to promote the following steps. Grayscaling can also be applied to initial datasets because they are usually generated by different types of machines. Hence, a histogram matching procedure can be performed on all samples by considering one sample as a reference to unify the histogram distribution of all samples [ 57 ].
The lack of data or unreliable data can influence the effectiveness of ML and DL due to a shortage of sufficient features [ 86 ]. The overfitting problem happens when a network learns a task with very high variance such as to perfectly model the training data [ 70 , 87 ]. These models of variation usually entail noise, changes in contrast, rotations, and translations. In biased input, data augmentation can be utilized to enhance the counts of infrequent samples. In data augmentation, general causes of variation are specifically added to training data. Particularly medical image processing researchers face limited accessibility to big data, hence, the success of the system can be linked to the deployed augmentation approach. Data Augmentation comprises a set of approaches that improve the quality and size of training datasets such that better DL systems can be utilized. An example, of that, is the application of non-rigid deformations of input samples and the required segmentation. Data augmentation is usually addressed using generative adversarial networks (GAN) [ 88 ]. Several studies considered GAN as a genuine tool to be applied in implementations that need data augmentation [ 88 ]. Still, several kinds of GANs can suffer from instability during the training step and are subject to gradient saturation. In the surveyed literature, several studies utilized image augmentation approaches to increase the number of images in the dataset [ 49 , 69 , 75 , 84 , 88 ]. Studies that used augmented datasets indicated the significant improvement of the system’s performance against systems that used initial datasets [ 76 ].
Feature extraction approaches
COVID-19 patients present several radiological patterns including patchy ground-glass opacities, pneumonic consolidations, reticulonodular opaqueness [ 76 ]. These elusive optical features can be effectively extracted with the aid of a suitable approach. Choosing the best algorithm that enables distinctive and full feature extraction is of great importance. This function is complex to infer, hence, it is essential to plan carefully for every new system. Feature extraction approaches were used to minimize the required time for computation and the complexity of the system, which accordingly enhances the performance of decision-making systems [ 89 ]. Effective FE approaches are needed to get better ML models. On the other hand, DL models are broadly applied in medical imaging applications as they can extract features automatically or by utilizing some pre-trained systems such as ResNet [ 74 ]. It is a primitive stage to extract features when utilizing medical images before the classification and diagnosis functions. Several methods were used to extract the features in the surveyed studies. We will explain the two main deployed approaches in the surveyed studies:
- • The texture feature approach was used to inspect features and to indicate similarities and patterns of images [ 90 ]. In literature this procedure is often called “hand-crafting” and it is utilized to anticipate the right category, which is usually predicted features. It is broadly employed in many medical domains [ 91 ]. In this approach, features can be manually designed, or “by hand” aiming to handle particular problems such as variations and occlusions in scale and illumination. The deployment of handcrafted approaches entails deciding the best trade-off between accuracy and computational performance. GLCM, LBGLCM, GLRLM, and SFTA can be mined to categorize pandemic diseases [ 84 ]. GLCM is the most utilized approach to extract features regarding its good performance in many disciplines particularly in oncology imaging when the texture features of the images are easily detachable [ 91 , 92 ].
- • DL approaches for FE are getting more attention regarding their high capability of extracting features [ 93 ]. Many researchers have adopted DL approaches and achieved outstanding performance in data extraction from images, regarding its capability to discover features on a new dataset on its own, contrasting to previous machine learning algorithms [ 61 , 94 ]. Several techniques were used in the surveyed studies for FE: AlexNet [ 71 ], Resnet50 [ 77 , 95 , 96 ], VGG19 [ 82 , 95 ], VGG16 [ 49 , 95 ], ResExLBP [ 97 ], DenseNet20, DenseNet201, Inception_ResNet_V2, Inception_V3, Resnet50, and MobileNet_V2 [ 95 ].
Feature selection approaches
Feature Selection (FS) entails choosing a group of related features of an input image and deleting the less fitting ones. This step presents many benefits in terms of minimizing the complexity of the proposed approach, minimizing overfitting, and enhancing accuracy. FS approaches can be categorized into three classes: embedded, filter, and wrapper [ 98 ]. The main goal of the FS stage is to establish the feature vector and extract the features with the least knowledge before the final categorization. Hence, a large number of discriminative features can be chosen using the deployed approach.
In the surveyed studies, only a few studies applied FS techniques. A study by Elaziz et al. [ 68 ] presented a new FS technique based on enhancing the behavior of MRFO using differential evolution. Another study by Bahadur Chandra et al. [ 76 ] used the BGWO approach, which mimics the leadership, hunting, and encircling procedure of wolves. This approach can overcome the problem of getting trapped in local minima. The relief algorithm, which is basically based on the KNN algorithm, was used as a feature selection approach that can enhance the diagnosis performance [ 71 ]. The main rule of the relief algorithm is to choose features by measuring the proxy statistical value. The relief algorithm was used in a study by Turkoglu [ 71 ] and Novitasari et al. [ 99 ] to extract the features effectively. Tuncer et al. [ 97 ] adopted an improved version of the Relief algorithm, which used Manhattan distance to produce weights instead of Euclidean distance.
Classification
Classification, which is also called Computer-Aided Diagnosis (CAD), has a vital part in medical image processing. The classification process takes sample images as an input and provides the diagnosis variable as an output [ 100 ]. DL in Computer-Aided Diagnosis was applied for the first time by Lo et al. [ 101 ] to categorize lung X-ray images and has been adopted in several studies since then. As the sample images are provided to the CNN, the classification process is performed by disclosing the content of the image. Following that, image localization is performed, in which bounding boxes are placed around the output position. This process aims to locate the disease in the image. The localization process is essential to locate some basic disease features [ 86 ].
Limitation and future research directions
This section presents potential routes for future studies that can address COVID-19 diagnosis and detection using medical image modalities. Despite the encouraging outcomes of the revised studies, there are some restrictions and obstacles that should be addressed about the utilized approaches for COVID-19 diagnosis. The most important obstacle we noticed in the surveyed studies was the need for an extensive training dataset. This is a general problem in training deep learning models for images in a medical-related context [ 63 ]. DL needs sufficient training data as the DL model’s achievement depends on the volume and quality of the input. It is unavoidable that the preliminary open accessible medical images for new medical cases such as COVID-19 will be limited in quantity and insufficient in quality aspects. Still, the shortage of data is a fundamental restriction to DL’s achievement in medical image processing generally. Additionally, building sufficient medical imaging data is complex because data annotation needs time, work, and cooperation from several professionals to avoid mistakes. Many studies augmented the original training to lower the selection bias and increase the diversity of the dataset [ 102 ]. Augmentation of data can be utilized to enhance the success of the adopted models [ 103 ]. Future studies are thus required to improve the augmentation approaches for developing advanced models and tolerating the lack of data. The second obstacle we faced while conducting this SLR was that each study has utilized a different resource to construct the dataset. Hence, the evaluation of the performance of the adopted models, among several studies, is pretty complex to check and compare. One approach to handle this issue is by presenting comparative studies, in which several models can be evaluated using the same data set and evaluation metrics.
Although the inspected studies have utilized several techniques for COVID-19 diagnosis, these approaches did not consider the incremental updates of the data for the classification process. Incremental learning has become significant with the provision of huge volumes of data that are generated from emerging technologies [ [104] , [105] , [106] ]. As the embedded patterns in big data could be dynamic, the proposed classification method should have the ability to learn incrementally in order to update the current patterns with the accumulation of new data [ 107 ]. Particularly, incremental learning differs from conventional methodologies in data accumulation and ensemble learning [ 108 ]. In the conventional methodologies, ML does not enable the model to update the produced classifier [ 109 ]. Hence, the model will be restricted and can not recognize new divergent images. In general, traditional supervised approaches cannot be utilized for incremental learning and need to recompute all the training data to build prediction models. As computation time and accuracy are two important criteria for the assessment of the medical diagnosis systems, incremental learning can enhance the predictive accuracy and reduce the computation time of COVID-19 diagnosis. Hence, future research direction can be followed by researchers to utilize incremental approaches to improve the performance of COVID-19 diagnosis.
Several other approaches can be used in the classification process, such as ensemble learning. Ensemble learning can aid to enhance the machine learning outcomes. As it can present significant generalization performance, it has gained researchers’ attention [ 110 ]. To improve the efficiency of the generalization of previous single classifiers, ensemble learning integrates two or more ML methods. Ensemble learning has been used in previous literature in image processing studies in various contexts [ [111] , [112] , [113] ].
Considering the collection of studies to be included in this literature review, the databases we choose have been used in several systematic literature review studies and presented robust outcomes. However, referring to the limitation of the study, it is important to mention that although we tried to cover several resources of data, utilizing other databases can provide broader coverage of the research area. Other databases could be used in future work.
COVID-19 is a worldwide issue that should be faced by researchers’ efforts in all scientific means. The COVID-19 crisis has joined researchers from several disciplines together to fight against the spread and the impact of the disease. AI applications depend on the quality of the input data to achieve high performance. The generalizability of the outcomes is also a significant aspect when deploying AI applications, which can be achieved if more data are available for researchers.
Considering the current advent of the COVID-19 crisis, which has put healthcare services under critical conditions over the world, there is a huge need for adequate large image datasets. Hence, to address the data scarcity issue, researchers have adopted data augmentation techniques, which have shown huge possibilities in several disciplines, entailing medical imaging [ 114 ]. Particularly, DL models need a huge volume of training data with clear labels. Still, most images are manually annotated, which indicates a time-consuming procedure that requires expert knowledge [ 115 ]. One way to address this issue is by enabling medical image sharing between different research and health centers over the world, which holds great promises for COVID-19 diagnosis. With this in mind, the post-COVID-19 stage may face more data-sharing among various national and international organizations. This will enable AI researchers to present applications that can provide accurate outcomes.
Medical image processing is a well-recognized method that could be useful in the identification of COVID-19. The results of this SLR indicated that researchers have adopted several approaches to classify the images related to COVID-19 diagnosis and detection, and these methods have presented promising outcomes in terms of the accuracy, cost, and speed of the detection. This review focused on imaging modalities, approaches, and procedures, that were utilized in the surveyed studies aiming to present upcoming directions for future research.
No funding sources.
Competing interests
None declared.
Ethical approval
Not required.
Appendix A. The related studies
Appendix b. datasets and imaging modalities in the surveyed studies, appendix c. the relevance score of the items.
Help | Advanced Search
Quantum Physics
Title: edge detection quantumized: a novel quantum algorithm for image processing.
Abstract: Quantum image processing is a research field that explores the use of quantum computing and algorithms for image processing tasks such as image encoding and edge detection. Although classical edge detection algorithms perform reasonably well and are quite efficient, they become outright slower when it comes to large datasets with high-resolution images. Quantum computing promises to deliver a significant performance boost and breakthroughs in various sectors. Quantum Hadamard Edge Detection (QHED) algorithm, for example, works at constant time complexity, and thus detects edges much faster than any classical algorithm. However, the original QHED algorithm is designed for Quantum Probability Image Encoding (QPIE) and mainly works for binary images. This paper presents a novel protocol by combining the Flexible Representation of Quantum Images (FRQI) encoding and a modified QHED algorithm. An improved edge outline method has been proposed in this work resulting in a better object outline output and more accurate edge detection than the traditional QHED algorithm.
Submission history
Access paper:.
- HTML (experimental)
- Other Formats

References & Citations
- INSPIRE HEP
- Google Scholar
- Semantic Scholar
BibTeX formatted citation

Bibliographic and Citation Tools
Code, data and media associated with this article, recommenders and search tools.
- Institution
arXivLabs: experimental projects with community collaborators
arXivLabs is a framework that allows collaborators to develop and share new arXiv features directly on our website.
Both individuals and organizations that work with arXivLabs have embraced and accepted our values of openness, community, excellence, and user data privacy. arXiv is committed to these values and only works with partners that adhere to them.
Have an idea for a project that will add value for arXiv's community? Learn more about arXivLabs .
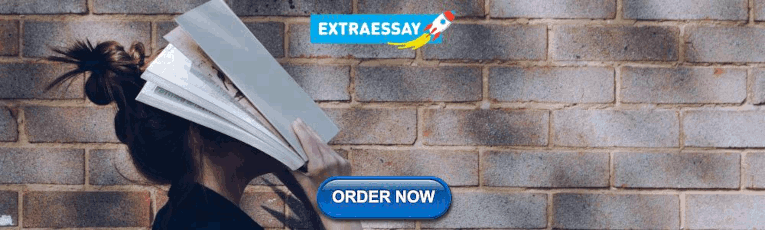
COMMENTS
Medical imaging plays a significant role in different clinical applications such as medical procedures used for early detection, monitoring, diagnosis, and treatment evaluation of various medical conditions. Basicsof the principles and implementations of artificial neural networks and deep learning are essential for understanding medical image analysis in computer vision. Deep Learning ...
In this paper, it is argued that the evolution of medical image processing has been a gradual process, and the diverse factors that contributed to unprecedented progress in the field with the use of AI are explained. ... During the last decades CAD-driven precision diagnosis has been the holy grail of medical image processing research efforts ...
Key Message: In this paper, we will review recent advances in artificial intelligence, machine learning, and deep convolution neural network, focusing on their applications in medical image processing. To illustrate with a concrete example, we discuss in detail the architecture of a convolution neural network through visualization to help ...
Medical image processing is a set of procedures for extracting clinically useful data from various imaging modalities for diagnosis ... As a result, disease detection has become an important topic in medical image processing and medical imaging research. This review studied the articles on disease diagnosis published between 2017 and 2021 ...
Abstract. Medical image processing provides core innovation for medical imaging. This paper is focused on recent developments from science to applications analyzing the past fifteen years of history of the proceedings of the German annual meeting on medical image processing (BVM). Furthermore, some members of the program committee present their ...
1. Introduction. The origin of radiology can be seen as the beginning of medical image processing. The discovery of X-rays by Röntgen and its successful application in clinical practice ended the era of disease diagnosis relying solely on the clinical experience of doctors (Glasser, 1995).The production of medical images provides doctors with more data, enabling them to diagnose and treat ...
During data pre-processing, we obtained 1,570,263 medical image-mask pairs for model development and validation. For internal validation, we randomly split the dataset into 80%, 10%, and 10% as ...
In the field of medical image processing methods and analysis, fundamental information and state-of-the-art approaches with deep learning are presented in this paper. The primary goals of this paper are to present research on medical image processing as well as to define and implement the key guidelines that are identified and addressed.
Medical image processing and analysis techniques play a significant role in diagnosing diseases. Thus, during the last decade, several noteworthy improvements in medical diagnostics have been made based on medical image processing techniques. In this article, we reviewed articles published in the most important journals and conferences that used or proposed medical image analysis techniques to ...
Introduction. For the last decade, the locution Artificial Intelligence (AI) has progressively flooded many scientific journals, including those of image processing and medical physics. Paradoxically, though, AI is an old concept, starting to be formalized in the 1940s, while the term of artificial intelligence itself was coined in 1956 by John McCarthy.
Keywords: Data Mining, Classification, Image Segmentation. 1. Introduction. Medical image processing deals with the development of. problem-specific approaches to the enhancement of raw. medical ...
This paper discusses the different evaluation metrics used in medical imaging classification. Provides a conclusion and future directions in the field of medical image processing using deep learning. This is the outline of the survey paper. In Section 2, medical image analysis is discussed in terms of its applications.
Medical Image Processing regards a set of methodologies that have been developed over recent years with the purpose of improving medical image quality, improving medical data visualization, understanding, and assisting medical diagnosis, and so on. Following past years tendency, it is foreseen that these methodologies will increase in complexity and will also have an increasing range of ...
Read the latest Research articles in Image processing from Scientific Reports. ... Research on improved black widow algorithm for medical image denoising. Hepeng Qu, Kun Liu ... Calls for Papers
Image processing increases the percentage and amount of detected tissues. This chapter presents the application of both simple and sophisticated image analysis techniques in the medical imaging field.
Deep Learning Approach (DLA) in medical image analysis emerges as a fast-growing research field. DLA has been widely used in medical imaging to detect the presence or absence of the disease. This paper presents the development of artificial neural networks, comprehensive analysis of DLA, which delivers promising medical imaging applications.
Medical imaging (MI) [ 1] utilizes various technologies to produce images of the human body's internal structures and functions [ 2 ]. Healthcare professionals (HPs) [ 3] use these medical images for four purposes: diagnosis [ 4 ], treatment planning [ 5 ], monitoring [ 6 ], and research. Firstly, the HPs utilize medical images to identify ...
Image Processing: Research O pportunities and Challenges. Ravindra S. Hegadi. Department of Computer Science. Karnatak University, Dharwad-580003. ravindrahegadi@rediffmail. Abstract. Interest in ...
IET Image Processing journal publishes the latest research in image and video processing, covering the generation, ... Search for more papers by this author. Tan Xin, Tan Xin. ... The one of the main problems with the studies of medical image segmentation is the insufficient contrast between disease region and other skin parts in an image. As a ...
Modern medical image translation methods use generative models for tasks such as the conversion of CT images to MRI. Evaluating these methods typically relies on some chosen downstream task in the target domain, such as segmentation. On the other hand, task-agnostic metrics are attractive, such as the network feature-based perceptual metrics (e.g., FID) that are common to image translation in ...
The major applications of the CNN are in image and signal processing, natural language processing and data analytics. ... The survey includes research papers on various applications of CNNs in medical image understanding. The papers for the survey are queried from various journal websites. ... The papers reviewed for medical image understanding ...
Affine image registration is a cornerstone of medical image processing backed by decades of development. While classical algorithms can achieve excellent accuracy, they solve a time-consuming optimization for every new image pair. In contrast, deep-learning (DL) methods learn a function that maps an image pair to an output transform.
Download. by Minh-Tam (Reynard) Le. Medical Image Processing. Analysis of regional deformation of the heart's left ventricle using invariant SPHARM descriptors. ABSTRACT This paper presents a method to analyze the regional deformation of the heart left ventricle (LV). It consists of two steps.
As a result of the rise of Transformer architectures in medical image analysis, specifically in the domain of medical image segmentation, a multitude of hybrid models have been created that merge the advantages of Convolutional Neural Networks (CNNs) and Transformers. These hybrid models have achieved notable success by significantly improving segmentation accuracy. Yet, this progress often ...
Motivated by this fact, a large number of research works have been proposed and developed for the initial months of 2020. In this paper, we first focus on summarizing the state-of-the-art research works related to deep learning applications for COVID-19 medical image processing.
AI systems for medical image segmentation typically use neural networks. Loosely based on the human brain, neural networks are machine-learning models comprising many interconnected layers of ...
Caption: Researchers from MIT and elsewhere developed a machine-learning framework that can generate multiple plausible answers when asked to identify potential disease in medical images. By capturing the inherent ambiguity in these images, this technique could prevent clinicians from missing crucial information that could inform diagnoses.
Traditionally for improving the segmentation performance of models, most approaches prefer to use adding more complex modules. And this is not suitable for the medical field, especially for mobile medical devices, where computationally loaded models are not suitable for real clinical environments due to computational resource constraints. Recently, state-space models (SSMs), represented by ...
Research protocol. Systematic reviews start by defining a review protocol that considers the research objective being addressed and the methods to perform the study [].In this research, we conducted a SLR to investigate the published studies in the context of COVID-19 and image processing, as a potential approach to disease diagnosis.
Quantum image processing is a research field that explores the use of quantum computing and algorithms for image processing tasks such as image encoding and edge detection. Although classical edge detection algorithms perform reasonably well and are quite efficient, they become outright slower when it comes to large datasets with high-resolution images. Quantum computing promises to deliver a ...