- Open access
- Published: 05 September 2017
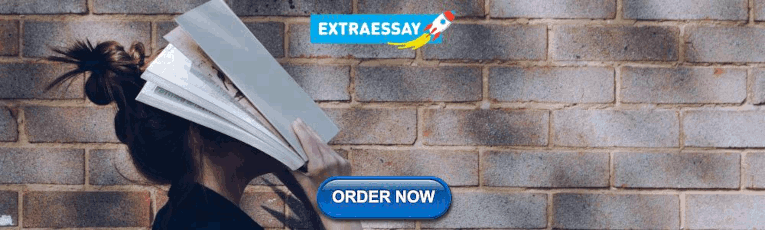
The volatility of returns from commodity futures: evidence from India
- Isita Mukherjee 1 &
- Bhaskar Goswami 1
Financial Innovation volume 3 , Article number: 15 ( 2017 ) Cite this article
5756 Accesses
10 Citations
Metrics details
This paper examines the pattern of the volatility of the daily return of select commodity futures in India and explores the extent to which the select commodity futures satisfy the Samuelson hypothesis.
One commodity future from each group of futures is chosen for the analysis. The select commodities are potato, gold, crude oil, and mentha oil. The data are collected from MCX India over the period 2004–2012. This study uses several econometric techniques for the analysis. The GARCH model is introduced for examining the volatility of commodity futures. One of the key contributions of the paper is the use of the β term of the GARCH model to address the Samuelson hypothesis.
The Samuelson hypothesis, when tested by daily returns and using standard deviation as a crude measure of volatility, is supported for gold futures only, as per the value of β (the GARCH effect). The values of the rolling standard deviation, used as a measure of the trend in the volatility of daily returns, exhibits a decreasing volatility trend for potato futures and an increasing volatility trend for gold futures in all contract cycles. The result of the GARCH (1,1) model suggests the presence of persistent volatility and the prevalence of long memory for the select commodity futures, except potato futures.
Conclusions
The study sheds light on significant characteristics of the daily return volatility of the commodity futures under analysis. The results suggest the existence of a developed market for the gold and crude oil futures (with volatility clustering) and show that the maturity effect is only valid for the gold futures.
Volatility plays a vital role in derivative pricing, hedging, risk management, and optimal portfolio selection. The concept of volatility relates to the uncertainty or risk about an asset’s value. A higher volatility means that an asset can assume a large range of values, while a lower volatility implies that an asset’s value does not fluctuate dramatically, even though it changes over time. Accurate modeling and forecasting of volatility in asset returns are major issues in financial economics. Derivative markets, particularly commodity futures markets, have become more sophisticated now a day. The futures price depends on the availability of information. A small change in price may have large effects on the trading results across futures markets. Researchers around the world showed increasing interest in the volatility of commodity futures. In the present analysis, an attempt is made to examine the trend and pattern of the volatility of daily returns of few select commodity futures in the Indian context.
As a first step, we examine the characteristics of the commodity futures. In particular, we analyze whether the price variability of a future increases or decreases when the contract approaches maturity. The Samuelson hypothesis for the selected commodity futures is tested. Samuelson ( 1965 ) argued that the volatility of the change in futures price increases as the contract approaches maturity. This phenomenon is also called the “Maturity Effect.” The purpose of testing the Samuelson hypothesis is to assess the degree of maturity of Indian commodity futures. From the view point of the Samuelson hypothesis, the prediction of price volatility is very useful for all participants in the futures market, such as hedgers, speculators, and traders. We also address the trend in daily return’s volatility across the contract cycles to decipher the volatility characteristics of the select commodity futures. To this end, we introduce the concept of rolling standard deviation.
We, then, proceed to examine the volatility aspects of the commodity futures. The steps involved in this exercise are the graphical plotting of the daily returns series, followed by its descriptive statistics. The daily returns are tested for stationarity. Then, we explored the GARCH (1, 1) model for the return volatility of the select futures. Footnote 1
The present paper derives its motivation from the following considerations. First, commodity futures as a financial asset is gaining prominence in the Indian capital market. The uninterrupted transactions in futures contracts from 2004, with a volume of trade surging from Rs 1.29 lakh crore in 2003–2004 to a peak of Rs 181 lakh crore in 2011–2012, Footnote 2 confirms the phenomenal importance of commodity futures. Second, empirically testing the Samuelson hypothesis as an indicator of developed and mature futures market seems necessary for the Indian commodity futures market. One of the key contributions of this paper is to use the GARCH (1,1) process for testing the Samuelson hypothesis on select commodity futures. Testing the Samuelson hypothesis through the β term of the GARCH (1,1) yields meaningful results, as the GARCH (1,1) assumes that the returns are uncorrelated, with zero mean. Moreover, in the GARCH (1,1) process, the present volatility does not depend on past returns, and thereby makes it a suitable methodology to test the Samuelson hypothesis. In this respect, the present analysis aims at filling a gap in the existing literature. Finally, in India, while the volatility issues related to dominant financial assets, such as company shares, have been well researched and documented, only a few studies on commodity futures have been carried out. More specifically, the trend and pattern of the volatility in the daily returns from commodities have been largely ignored in the existing literature. The remainder of this paper is organized as follows. The second section presents the literature. The third section deals with the methodology used in this paper and describes the relevant data sources. The result and discussion of the analysis are carried out in the fourth section. Finally, the fifth section provides our concluding remarks.
Literature review
Many researchers, such as W. R. Anderson ( 1985 ), examined the Samuelson hypothesis using selected agricultural futures contracts and found support for wheat, oat, soybeans, and soybeans meal futures. Bessembinder et al. ( 1996 ) provided a new framework for the maturity effect, the ‘BCSS hypothesis’ (based on Bessembinder, Coughenour, Seguin and Smoller). This hypothesis is an extension of the Samuelson hypothesis. The authors found that the Samuelson hypothesis is more likely to hold for those commodities whose price changes can be reversed in future. Black and Tonks ( 2000 ) investigated the pattern of the volatility of commodity futures prices over time and revealed the conditions which support the Samuelson hypothesis. Allen and Cruickshank ( 2000 ) analyzed the Samuelson hypothesis for selected commodity futures of three different futures markets in three different countries. They performed a regression analysis complemented by ARCH models, and the result suggests that the Samuelson hypothesis holds in the case of maximum selected contracts. Floros and Vougas ( 2006 ) investigated the Samuelson hypothesis in the context of the Greek stock index futures market and examined the maturity effect through linear regressions and GARCH models. The result of the study suggests that volatility depends on time to maturity and gives a stronger support to the Samuelson hypothesis compared to linear regressions. Duong and Kalev ( 2008 ) examined the Samuelson hypothesis for 336 selected commodities from five futures exchanges observed between 1996 and 2003. Using the Jonckheere-Terpstra Test, OLS regressions with realized volatility, and various GARCH models, the authors find mixed evidence concerning the support for the Samuelson hypothesis. Even though many studies investigated the Samuelson hypothesis, very few contributions analyzed it in the context of the Indian commodity futures market.
Notable exceptions are Verma and Kumar ( 2010 ), who examined the application of the Samuelson hypothesis and BCSS hypothesis in the Indian commodity futures market. Gupta and Rajib ( 2012 ) also examined this issue for eight commodities, and they concluded that the Samuelson hypothesis does not hold for the majority of the considered commodity contracts.
Numerous studies investigate the volatility of futures prices worldwide.
Locke and Sarkar ( 1996 ) examined the changes in market liquidity following changes in price volatility. The results of the study suggest that market makers are most hurt by volatility in the case of inactive contracts. Richter and Sorensen ( 2002 ) analyzed a volatility model for soybean futures and options using panel data. The study suggests the existence of a seasonal pattern in convenience yields and volatility, in line with the storage theory. Chang et al. ( 2012 ) examined a long memory volatility model for 16 agricultural commodity futures. The empirical results are obtained using unit root tests, GARCH, EGARCH, APARCH, FIGARCH, FIEGARCH, and FIAPARCH model. Manera et al. ( 2013 ) examined the effect of different types of speculation on the volatility of commodity futures prices. The authors selected four energy and seven non-energy commodity futures observed over the period 1986–2010. Using GARCH models, the study suggests that speculation affects the volatility of returns, and long-term speculation has a negative impact, whereas short term speculation has a positive effect. Christoffersen et al. ( 2014 ) analyzed the stylized facts of volatility in the post-financialization period using data of 750 million futures observed between 2004 and 2013.
Two strands in the existing literature focused on volatility in the Indian commodity futures market. First, the literature is largely dominated by spot price volatility and its spillover effect on future price volatility, that is, the price discovery mechanism of the futures market. Brajesh and Kumar ( 2009 ) examined the relationship between future trading activity and spot price volatility for different commodity groups, such as agricultural, metal, precious metal, and energy commodities in the perspective of the Indian commodity derivatives market. P. Srinivasan ( 2012 ) examined the price discovery process and volatility spillovers in Indian spot-futures commodity markets and the result points to dominant volatility spillovers from spot to futures market. Sehgal et al. ( 2012 ) examined the futures trading activity on spot price volatility of seven agricultural commodities and found that unexpected futures trading has strong correlation on spot volatility. Chauhan et al. ( 2013 ) analyzed the market efficiency of the Indian commodity market. They found that for guar seed, the volatility in futures prices influences the volatility in spot prices and the opposite result holds for chana. The work by Chakrabarti and Rajvanshi ( 2013 ) also explored the determinants of return volatility of select commodity futures in the Indian context. Sendhil et al. ( 2013 ) examined the efficiency of commodity futures through price discovery, transmission, and the extent of volatility in four agricultural commodities and found persistence volatility in spot market. Kumar et al. ( 2014 ) examined the price discovery and volatility spillovers in the Indian spot-futures commodity market. Gupta and Varma ( 2015 ) reviewed the impact of futures trading on spot markets of rubber in India and observed bidirectional flow of volatility between spot and futures market. Vivek Rajvanshi ( 2015 ) presented a comparative study on the performance of range and return-based volatility estimators for crude oil commodity futures. Malhotra and Sharma ( 2016 ) investigated the information transmission process between the spot and futures market and found that bidirectional volatility spillovers exists between the spot and futures market.
Second, a few studies specifically focus on the volatility of commodity futures. Kumar and Singh ( 2008 ) examined the volatility clustering and asymmetric nature of Indian commodity and stock market using S&P CNX Nifty for the stock market, and gold and soybean for the commodity futures market. Kumar and Pandey ( 2010 ) examined the relationship between volatility and trading activity for different categories of Indian commodity derivatives. They find a positive and significant correlation between volatility and trading volume for all commodities, no significant relationship between volatility and open interest, and an asymmetric relationship between trading volume and open interest. Kumar and Pandey ( 2011 ) examined the cross market linkages of Indian commodity futures with futures markets outside India. However, all these studies focus on the price volatility of commodity futures. In contrast with the above-mentioned studies on the Indian commodity futures market, the present study attempts to examine the return volatility of select commodity futures as financial assets. Footnote 3
The data on commodity futures are obtained from the official website of Multi Commodity Exchange (MCX), Mumbai, and cover the period from 2004 to 2012. We selected four commodities (potato, crude oil, gold, and mentha oil) from four different categories of commodity futures: Agricultural Commodity Futures, Energy, Bullions and Oil, and Oil Related Products, respectively. This choice satisfies two basic criteria: (i) the high frequency of future contracts; (ii) the large volume/value of such futures within the study period. Table 1 justifies the choice of the commodity futures.
In the commodity futures exchanges, trading takes place for 1-month, 2-month, and 3-month contract expiry cycles. However, in India, the 4-month, 5-month, and up to 1-year contract expiry cycles exist, in some cases, and we treat them as unusual exceptions. We only focus on the 1-month (near), 2-month (next-near), and 3-month (far) expiry cycles for futures. All futures contracts expire on the last Thursday of the month.
Hereafter, we provide a hypothetical example to demonstrate the steps involved in calculating the return in the logarithm form. We introduce a case based on crude oil.
The contract starts on July 30, 2010, and expires on October 20, 2010.
Nominal return for 1-month contract = ln(closing price on October 20)-ln(opening price on October 1); (October 1 is the Friday following the last Thursday of September, with 1 month to expiry, approximately.).
Nominal return for 2-month contract = ln(closing price on October 20)-ln(opening price on August 27); (August 27 is the Friday following the last Thursday of August, with 2 months to expiry, approximately).
Nominal return for 3-month contract = ln(closing price on October 20) -ln(opening price on July 30).
Here, the daily return on futures is calculated as the value of the continuously compounded rate of the return multiplied by 100. As such, the Log return of the price series = ln(Ft /Ft-1) *100, where Ft and Ft-1 are the closing prices on day t and ( t-1 ) of a futures contract. The standard deviation of the daily return is also calculated for all the three categories of contract cycles.
We use the conventional standard deviation approach as the measure of the volatility of daily returns. A hypothetical example is as follows (Table 2 ).
We also introduce the concept of 25-day moving standard deviation (also known as the rolling standard deviation) as a measure of the trend in the volatility of the daily returns.
The method for calculating the rolling standard deviation is explained with the help of a hypothetical example based on crude oil futures.
We consider the first 25 days starting from July 30, 2010 to calculate the standard deviation.
For the next period, the initial day (July 30, 2010) is left out and 1 day is added to the end of the period (August 24, 2010) so that the 25 days begin from July 31, 2010, and end on August 24, 2010. The standard deviation is calculated for these 25 days.
The above process is repeated for the entire length of the contract cycles to obtain the rolling standard deviation for the concerned futures.
In this example, 25-days are considered as the average number of trading days per month (leaving aside Sundays and other holidays). Therefore, the total annual trading days for commodity futures is 305 days.
We then proceed to plot graphically the daily returns series over time so that volatility clustering can be verified.
Descriptive statistics
To analyze the characteristics of the daily return series of the commodity futures market during the study period, the descriptive statistics show the mean ( X ), standard deviation (σ), Skewness ( S ), Kurtosis ( K ), and Jarque-Bera statistics results.
We calculated the coefficients of Skewness and Kurtosis to verify whether the return series is skewed or leptokurtic. To test the null hypothesis of normality, the Jarque-Bera statistic (JB) has been applied, as follows:
where N is the number of observations, S is the coefficient of Skewness, K is the coefficient of Kurtosis, k is the number of estimated coefficients used to create the series, and JB follows a Chi-square distribution with 2 degrees of freedom (d. f.). We perform a joint test of normality where the joint hypothesis of s = 0 and k = 3 is tested. If the JB statistic is greater than the table value of chi-square with 2 d. f., the null hypothesis of a normal distribution of residuals is rejected.
Test for stationarity
For testing whether the data are stationary or not, we performed the Augmented Dickey-Fuller (Dickey and Fuller 1979 ) and Philips-Perron Test (PP) (Phillips and Perron 1988 ). The stationarity of the return series has been checked by ADF test by fitting a regression equation based on a random walk with an intercept, or drift term (φ), as follows:
where μ t is a disturbance term with white noise. Here the null hypothesis is H 0 : ∂ = 0 (with alternative hypothesis H 1 : ∂ < 0). If this hypothesis is accepted, there is a unit root in the yt sequence, and the time series is non-stationary. If the magnitude of the ADF test statistic exceeds the magnitude of Mackinnon critical value, the null hypothesis is rejected, and there is no unit root in the daily return series.
Phillips and Perron ( 1988 ) suggested an alternative (non-parametric) method to control for serial correlation when testing for the presence of a unit root. The PP method estimates the non- augmented DF test equation, and it can be seen as a generalization of the ADF test procedure, which allows for fairly mild assumptions concerning the distribution of errors. The PP regression equation is as follows:
where the ADF test corrects for higher order serial correlation by adding lagged differenced terms on the right-hand side, while the PP test corrects the t statistic of the coefficient ∂ obtained from the AR(1) regression to account for the serial correlation μt . The null hypothesis is H 0 : ∂ = 0 (with alternative hypothesis H 1 : ∂ < 0).
Test for heteroskedasticity
The presence of heteroskedasticity in asset returns has been well documented in the existing literature. If the error variance is not constant (heteroskedastic), then, the OLS estimation is inefficient. The tendency of volatility clustering in financial data can be well captured by a Generalized Autoregressive Conditional Heteroskedastic (GARCH) model. Therefore, we modeled the time-varying conditional variance in our study as a GARCH process.
To identify the type of GARCH model that is more appropriate for our data, we performed the ARCH LM test (Engle 1982 ). This is a Lagrange Multiplier test for the presence of an ARCH effect in the residuals. We first regressed the return series on their one-period lagged return series and obtained the residuals \( \left({\widehat{\varepsilon}}^2\right) \) . Then, the residuals have been squared and regressed on their own lags of order one to four to test for the ARCH effect. The estimated equation is:
where K t is the error term. We, then, obtained the coefficient of determination ( R 2). The null hypothesis is the absence of ARCH error, H 0 : ϑ i = 0, against the alternative hypothesis H 1 : ϑ i ≠ 0. Under the null hypothesis, the ARCH LM statistic is defined as TR 2 , where T represents the number of observations. The LM statistic converges to a χ 2 distribution. Hence, we use the Lagrange Multiplier (LM) test for Autoregressive Conditional Heteroskedasticity (ARCH) to verify the presence of heteroskedasticity in the residuals of the daily return series for all commodity futures. If the ARCH LM statistic is significant, we confirm the presence of an ARCH effect.
The ARCH model as developed by Engle ( 1982 ) is an extensively used time-series models in the finance-related research. The ARCH model suggests that the variance of residuals depends on the squared error terms from the past periods. The residual terms are conditionally normally distributed and serially uncorrelated. A generalization of this model is the GARCH specification. Bollerslev ( 1986 ) extended the ARCH model based on the assumption that forecasts of the time-varying variance depend on the lagged variance of the variable under consideration. The GARCH specification is consistent with the return distribution of most financial assets, which is leptokurtic and it allows long memory in the variance of the conditional return distribution.
The Generalized Arch Model (GARCH)
The GARCH model (Bollerslev 1986 ) assumes that the volatility at time t is not only affected by q past squared returns but also by p lags of past estimated volatility. The specification of a GARCH (1, 1) is given as:
mean equation:
variance equation:
where ω > 0, α ≥ 0, β ≥ 0, and r t. is the return of the asset at time t , μ is the average return, and ε t is the residual return. The parameters α and β capture the ARCH effect and GARCH effect, respectively, and they determine the short-run dynamics of the resulting time series. If the value of the GARCH term β is sufficiently large, the volatility is persistent. On the other hand, a large value of α indicates an insensitive reaction of the volatility to market movements. If the sum of the coefficients is close to one, then, any shock will lead to a permanent change in all future values. Hence, the shock is persistent in the conditional variance, implying a long memory.
The Wald test estimates the test statistic by computing the unrestricted regression equation, without imposing any coefficient restrictions, as specified by the null hypothesis. The Wald statistic (under the null hypothesis) measures how the unrestricted estimates satisfy the restrictions. If the restrictions are valid, then, the unrestricted estimates should fulfill the restrictions.
We consider a general nonlinear regression model:
where β is a k vector of parameters to estimate. Any restrictions on the parameters can be written as:
where g is a smooth q dimensional vector imposing q restrictions on β .
Under the null hypothesis H˳, the Wald statistic has an asymptotic χ 2 ( q ) distribution, where q is the number of restrictions.
The result of the above tests is derived using Eviews 7.
Result and discussion
The Samuelson hypothesis is tested by the daily returns for the select commodity futures, and the results are reported in Table 3 . There is no clear trend and pattern in the percentage of the standard deviation among the selected commodities, except the gold futures, for which the Samuelson hypothesis holds. For other commodity futures (potato, crude oil, and mentha oil) this assumption is not confirmed. Footnote 4 For crude oil and mentha oil, the volatility of daily returns is greater for the 3-month (far) contract, followed by the 1-month (near) contract and the 2-months (next near) contract. The only exception is observed for potato futures, for which the volatility of daily returns for the 2-month (next near) contract is greater than that for the 1-month (near) contract. This phenomenon may be attributed to two possible reasons: (1) the underdeveloped and/or developing futures market in India, which acts as a barrier to the fulfillment of the Samuelson hypothesis; (2) since the volatility of daily returns for the 3-month (far) contract is greater for the selected three commodity futures (potato, crude oil, and mentha oil), the trend may be attributed to the initial euphoric behavior in the futures market, resulting from the initiation of a future contract.
Table 3 also presents the rolling standard deviation of the four commodity futures for all the three types of contract cycles.
To explore the trend in the volatility of daily returns for the selected commodity futures, we used the methodology known as 25-days rolling standard deviation. Figures 1 , 2 and 3 depict the trends of the volatility for each commodity futures, where the x-axis measures the number of contracts traded and the y-axis measures the standard deviation in percentage (%) terms.
Trends based on rolling standard deviation for 1 (Near) month contract
Trends based on rolling standard deviation for 2 (next near) month contract
Trends based on rolling standard deviation for 3 (far) month contract
For potato futures, there is a decreasing trend in volatility for near, next near, and far month contracts, with near contract exhibiting the least declining trend in volatility, and far month contract showing the maximum declining trend in volatility.
For crude oil and mentha oil futures, the near month volatility trend of daily returns is almost constant, and the magnitude of rolling standard deviation (volatility trend) is the highest for the far month contract.
For gold futures, the trend in volatility is increasing for all types of contract (1-month, 2-month, and 3-month). Moreover, this rise in the trend in volatility is greater for the 1-month contract, suggesting that the gold futures trend is more volatile as the contract approaches the maturity date.
The descriptive statistics for daily return series of the select commodity futures are summarized in Table 4 .
The average daily returns for all commodity futures are either close to zero or negative throughout the study period. The descriptive statistics show that the returns are negatively skewed. Since the estimated coefficients for the Skewness of the return series are different from zero, the underlying return distributions are not symmetric. The estimated coefficients for the Kurtosis of the daily return series are relatively high, suggesting that the underlying distributions are leptokurtic or heavily tailed and sharply peaked toward the mean compared to a normal distribution. The observed Skewness and Kurtosis indicate that the distribution of daily return series is non-normal. The Jarque-Bera normality test also shows the non-normality of the return distributions, as the estimated values of the Jarque-Bera statistic of all the return series are statistically significant at the 1% level (Figs. 4 , 5 , 6 and 7 ).
Daily return series graph of Potato futures
Daily return series graph of mentha oil futures
Daily return series graph of crude oil futures
Daily return series graph of gold futures
The correlogram test is conducted to address the presence of serial correlation in the residuals. We observe no serial correlation in the residuals up to 24 lags for the gold and crude oil futures in all types of contract cycles. This result holds for the 3-month (far) mentha oil contracts and potato near and next near contracts, as reported in Table 5 .
The ADF and PP tests are performed to verify the stationarity of the daily return series, and the statistics are presented in Table 6 . The p values of the ADF and PP tests are <0.05, which leads to conclude that the data used for the entire study period are stationary.
Both the test statistics reported in Table 6 reject the null hypothesis at the 1% significance level, with the critical value of −3.43 for both the ADF and PP tests. These results confirm that the series are stationary.
The graphs of daily returns confirm the absence of a clustering effect for potato futures and menthe oil futures. Only the 3 month contracts for menthe oil futures exhibits a small clustering effect for some periods. The graphs of crude oil and gold futures for all types of contracts show that the daily return series exhibits a clustering effect or volatility.
Table 7 presents the result of the ARCH-LM test (Engle 1982 ) of heteroskedasticity. This test detects the presence of the ARCH effect in the residuals of the daily return series. The ARCH-LM test statistic is significant for all types of contract cycles of gold commodity futures and the near and next near month contract of crude oil commodity futures, as well as for the mentha oil next near Footnote 5 contracts. The result confirms the presence of ARCH effects in the residuals as the test statistics are significant at 1% level. Hence, the results confirm the need for the analysis of the GARCH effect. The ARCH-LM statistic is not statistically significant for all types of potato contracts and mentha oil near contracts. Moreover, in the case of far month contracts of crude oil and mentha oil futures, we find no evidence of ARCH effect in the residuals. These findings are in line with the negligible amount of volatility clustering exhibited by the daily returns’ volatility graph. Hence, the results seem to confirm the need for the analysis of the GARCH effect. Footnote 6
The GARCH model is used for modeling the volatility of daily return series for the three types of contracts (near, next near, and far contracts) for crude oil and gold commodity futures and only for next near and far month contracts for mentha oil futures. The result of the GARCH (1,1) model is shown in Table 8 . All the parameters of the GARCH analysis are statistically significant.
The constant (ω), ARCH term (α), and GARCH term (β) are statistically significant at the 1% level. In the variance equation, the estimated β coefficient is considerably greater than the α coefficient, which implies that the volatility is more sensitive to its lagged values. The result suggests that the volatility is persistent. Moreover, the β term is greater for the near month contract cycles for gold futures, which confirms the validity of the Samuelson hypothesis. The sum of these coefficients ( α and β ) is close to unity, which indicates that a shock will persist for many future periods, suggesting the prevalence of long memory. However, the Wald test indicates the acceptance of the null hypothesis that α + β = 1 for far month contract cycles of gold futures only.
To check the robustness of the GARCH (1,1) model, we employed the ARCH-LM test (Engle 1982 ) to verify the presence of any further ARCH effect. As shown in the Table 7 , the ARCH- LM test statistic for the GARCH (1,1) model does not show any additional ARCH effect in the residuals of the model, which implies that the variance equation is well specified for the select commodity futures.
As a result, we can conclude that, among the select commodity futures, the clustering effect is present in the volatility of daily returns for crude oil and gold commodity futures in all contract cycles. Mentha oil futures also present a clustering effect in far month contracts.
This paper addresses the volatility of four select commodity futures: potato, mentha oil, crude oil, and gold. All the three types of contract cycles (near month, next near month, and far month) are considered for volatility analysis. The conventional approach based on standard deviation as a measure of volatility is considered to test the Samuelson hypothesis. To further corroborate the findings, the β -term of the GARCH (1,1) is also used to verify the Samuelson hypothesis. The results suggest that the Samuelson hypothesis does not hold for the select commodity futures in the Indian context, except for the gold futures. These results are in line with the findings of Gupta and Rajib ( 2012 ) and suggest that the Indian gold futures market is as developed as in the advanced countries.
The trend in the volatility of daily returns is captured by the concept of rolling standard deviation. The volatility trends in crude oil and mentha oil futures highlight the significance of the available information as the far month volatility is higher than the near month volatility. The fluctuations in the world markets for oil commodities have a lagged impact on the domestic market. Finally, the objective of futures market in terms of price discovery and hedging against future risks seems to be satisfied for potato futures. To test the presence of a unit root in the daily return series, we performed the ADF and PP tests. The results confirmed the stationarity of the daily return series for all the commodity futures.
For volatility modeling, we first considered the graphical representation of volatility clustering along with the descriptive statistics for all contract cycles of each commodity future. We, then, introduced a correlogram to check for serial correlation in the residuals, and, finally, the ARCH-LM test was conducted to check for the presence of an ARCH effect. All contract cycles of potato futures did not show any volatility clustering, and the result of the ARCH-LM test ruled out any ARCH effects in the daily return series. However, for all types of contract cycles of gold futures, we found unambiguous volatility clustering, and the ARCH-LM test results also suggested the presence of an ARCH effect. These results are in line with the findings of Kumar and Singh ( 2008 ) for gold futures.
For mentha oil and crude oil futures, the result obtained from the volatility clustering and ARCH- LM test was ambiguous for different contract cycles. Although the result of the ARCH-LM test implied no ARCH effect for the far month of mentha oil and crude oil futures, a trace of volatility clustering was observed in the daily return graph. Hence, we considered the far month contracts of mentha oil and crude oil futures for the GARCH analysis.
Furthermore, the result of the GARCH (1,1) model shows that three parameters, the constant(ω), ARCH (α) term, and GARCH (β) term, are significant at the 1% level. In the variance equation, the estimated β coefficient is greater than the α coefficient, which implies that the volatility is more sensitive to its lagged values. Hence, the volatility is persistent. The sum of these coefficients ( α and β ) are close to the unit, which suggests that a shock will persist for many future periods. This is particularly true for gold futures of far month contract, in line with the findings of Kumar and Singh ( 2008 ).
The volatility clustering effect shows that the crude oil and gold futures markets are rather similar. The crude oil futures market is largely dependent on the global market conditions, which are highly volatile. The spillover effect of global volatility has an impact on the Indian crude oil futures market. Other significant macroeconomic variables (such as the interest rate, exchange rate, and so on, which are fluctuating in nature) have a significant impact on gold futures market in India. Thus, after examining the Samuelson hypothesis and volatility features, we concluded that, out of the selected commodity futures, gold futures are well developed and organized in the Indian market.
The aim of this paper is to portrait the simplest form of return volatility of the select commodity futures. Therefore, advanced volatility models (like EGARCH, TGARCH, PGARCH) are not considered, although the inclusion of such models would definitely enrich the present study.
Data source: www.fmc.gov.in
The factors affecting the return volatility of commodity futures (like trading volume and open interest) are not under the purview of the present study as that would unnecessarily complicate and shift the focus out of the presented issue.
Identical results hold for gold futures, for which we test the Samuelson hypothesis using the β term of GARCH (1, 1) model as a measure of volatility, as reported in Table 8 .
The graph for the next near month contract of menthe oil shows volatility clustering although the Jarque-Bera value suggests that the residuals are not normally distributed. In addition, the correlogram shows that the residuals are serially correlated. Therefore we perform the ARCH-LM test and we observe the presence of ARCH effect.
Although the result of the ARCH-LM test implies no ARCH effect for the far month contract of mentha oil and crude oil futures, a trace of volatility clustering is observed in the daily return graph. Hence, we also consider the far month contracts of mentha oil and crude oil futures for the GARCH analysis.
Allen DE, Cruickshank SN (2000) Empirical testing of the Samuelson hypothesis: an application to futures markets in Australia, Singapore and the UK. Working paper, School of Finance and Business Economics, Edith Cowan University
Google Scholar
Anderson RW (1985) Some determinants of the volatility of futures prices. J Futur Mark 5(3):331–348
Article Google Scholar
Bessembinder H, Coughenour JF, Seguin PJ, Smoller MM (1996) Is there a term structure of futures volatilities? Reevaluating the Samuelson hypothesis. J Deriv 4:45–58
Black J, Tonks I (2000) Time series volatility of commodity futures prices. J Futur Mark 20(2):127–144
Bollerslev T (1986) Generalized autoregressive conditional Heteroskedasticity. J Econ 31(3):307–327
Chakrabarti BB, Rajvanshi V (2013) Determinants of return volatility: evidence from Indian commodity futures market. J Int Financ Econ 13(1):91–108
Chang C, McAleer M, Tansuchat R (2012) Modelling long memory volatility in agricultural commodity futures returns. Unpublished Working Paper 15093:10, Complutense University of Madrid
Chauhan KA, Singh S, Arora A (2013) Market efficiency and volatility spillovers in futures and spot commodity market. The agricultural sector perspective. SBIM VI(2):61–84
Christoffersen P, Lunde A, Olesen K (2014) Factor structure in commodity futures return and volatility. Working Paper, CREATES
David A. Dickey and Wayne A. Fuller (1979) Distribution of the Estimators for Autoregressive Time Series With a Unit Root. J Amer Stat Asso 74(366):427–431.
Duong NH, Kalev SP (2008) The Samuelson hypothesis in futures markets: an analysis using intraday data. J Bank Financ 32(4):489–500
Engle RF (1982) Autoregressive conditional Heteroskedasticity with estimates of the variance of UK inflation. Econometrica 50(4):987–1007
Floros C, Vougas VD (2006) Samuelson’s Hypothesis in Greek stock index futures market. Invest Manag Financ Innov 3(2):154–170
Gupta A, Varma P (2015) Impact of futures trading on spot markets: an empirical analysis of rubber in India. East Econ J 42(3):1–14
Gupta KS, Rajib P (2012) Samuelson hypothesis & Indian commodity derivatives market. Asia-Pacific Finan Markets 19:331–352
Kumar B (2009) Effect of futures trading on spot market volatility: evidence from Indian commodity derivatives markets. Social Science Research Network
Kumar B, Pandey A (2010) Price volatility, trading volume and open interest: evidence from Indian commodity futures markets. Social science research network
Kumar B, Pandey A (2011) International linkages of the Indian commodity futures market. Mod Econ 2:213–227
Kumar B, Singh P (2008) Volatility modeling, seasonality and risk-return relationship in GARCH-in-mean framework: the case of Indian stock and commodity markets. W.P. No. 2008-04-04, IIMA, 1-35
Kumar MM, Acharya D, Suresh BM (2014) Price discovery and volatility spillovers in futures and spot commodity markets : some Indian evidence. J Adv Manag Res 11(2):211–226
Locke P, A Sarkar (1996) Volatility and liquidity in futures markets. Research paper #9612, Federal Reserve Bank of New York
Malhotra M, Sharma KD (2016) Volatility dynamics in oil and oilseeds spot and futures market in India. Vikalpa 41(2):132–148
Manera M, Nicolini M, Vignati I (2013) Futures price volatility in commodities markets: the role of short term vs long term speculation. Università di Pavia, Department of Economics and Management, DEM Working Paper Series 42
Phillips PCB, Perron P (1988) Testing for unit roots in time series regression. Biometrika 75:335–346
Rajvanshi V (2015) Performance of range and return based volatility estimators:evidence from Indian crude oil futures market. Glob Econ Financ J 8(1):46–66
Richter M, Sorensen C (2002) Stochastic volatility and seasonality in commodity futures and options: the case of soybeans. Working Paper, Copenhagen Business School
Samuelson PA (1965) Proof that properly anticipated prices fluctuates randomly. Ind Manag Rev 6:41–49
Sehgal S, Rajput N, Dua KR (2012) Futures trading and spot market volatility: evidence from Indian commodity markets. Asian J Finan Acc 4(2):199–217
Sendhil R, Kar A, Mathur CV, Jha KG (2013) Price discovery, transmission and volatility: agricultural commodity futures. Agric Econ Res Rev 26(1):41–54
Srinivasan P (2012) Price discovery and volatility spillovers in Indian spot – futures commodity market. IUP J Behav Finance 9:70–85
Verma A, Kumar VSRVC (2010) An examination of the maturity effect in the Indian commodities futures market. Agric Econ Res Rev 23:335–342
Download references
Acknowledgements
The authors are indebted to three anonymous referee of this journal for their constructive comments of on the earlier draft of the manuscript. However, the usual disclaimer applies.
There is no financial assistance received in carrying out this particular research activity.
Availability of data and materials
The dataset is obtained from the publicly available repository, MCX, India website.
Author information
Authors and affiliations.
Department of Economics, The University of Burdwan, Burdwan, India
Isita Mukherjee & Bhaskar Goswami
You can also search for this author in PubMed Google Scholar
Contributions
BG initiated the thematic concept of the current research while IM carried out the exercise using statistical tools and techniques with the help of EViews 7. Both authors read and approved the final manuscript.
Corresponding author
Correspondence to Isita Mukherjee .
Ethics declarations
Ethics approval and consent to participate.
Not Applicable.
Consent for publication
Competing interests.
The authors declare that they have no competing interests.
Publisher’s Note
Springer Nature remains neutral with regard to jurisdictional claims in published maps and institutional affiliations.
Rights and permissions
Open Access This article is distributed under the terms of the Creative Commons Attribution 4.0 International License ( http://creativecommons.org/licenses/by/4.0/ ), which permits unrestricted use, distribution, and reproduction in any medium, provided you give appropriate credit to the original author(s) and the source, provide a link to the Creative Commons license, and indicate if changes were made.
Reprints and permissions
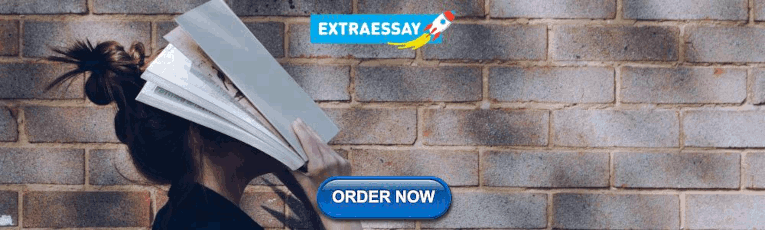
About this article
Cite this article.
Mukherjee, I., Goswami, B. The volatility of returns from commodity futures: evidence from India. Financ Innov 3 , 15 (2017). https://doi.org/10.1186/s40854-017-0066-9
Download citation
Received : 23 September 2016
Accepted : 02 September 2017
Published : 05 September 2017
DOI : https://doi.org/10.1186/s40854-017-0066-9
Share this article
Anyone you share the following link with will be able to read this content:
Sorry, a shareable link is not currently available for this article.
Provided by the Springer Nature SharedIt content-sharing initiative
- Commodity futures
- Daily return
- Samuelson hypothesis
Academia.edu no longer supports Internet Explorer.
To browse Academia.edu and the wider internet faster and more securely, please take a few seconds to upgrade your browser .
Enter the email address you signed up with and we'll email you a reset link.
- We're Hiring!
- Help Center
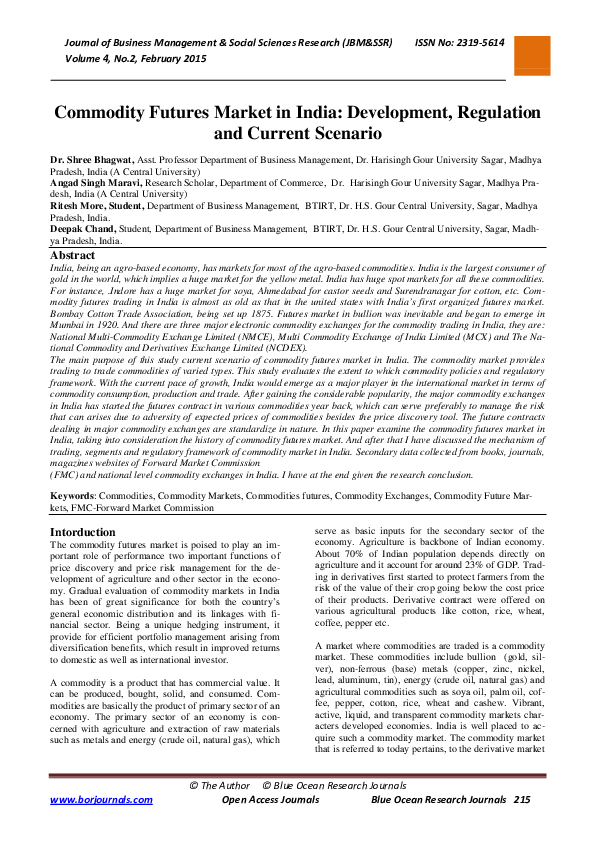
Commodity Futures Market in India: Development, Regulation and Current Scenario

2015, Journal of Business Management Social Sciences Research
Related Papers
IAEME PUBLICATION
IAEME Publication
Commodity futures market, in India, which started working way back in 1875, provides a platform for various stakeholders of commodity to hedge their price risk. However, it was assessed that the market would promote speculation which in turn would prove detrimental for the pricing of the agricultural commodities. However, since 2002, the market gained momentum in number of exchanges; commodities and value of contracts. The futures market or contracts, dealing in recognised commodity exchanges, are standardised in nature. The proposed study attempts to examine the current situation of agricultural commodity futures market in India in terms of its regulatory policy. Besides, the paper also evaluates the mechanism of current trading status and regulatory framework of commodity futures contract in India
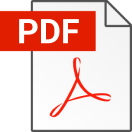
dharen pandey kumar
After a long time India has experienced the commodity trading at a time when its people has forgot the trading process with a very low interest gaining a good pace after ten years of its introduction. It is necessary to thus introduce the new generation with the modern commodity trading mechanism. This paper in short aims at introducing three points: what commodity trading means; where it takes place i.e. Commodity exchanges, and, how is it regulated in the Indian economy.
ANGAD MARAVI
The concept of commodity market is not new in India. The history of commodity market in India dates back to the ancient times, but the first organized market was established in 1875. However commodity market in India remained in a state of hibernation for four decades, which was market by suspicion on the benefits of futures trading. A Commodity Market has gained momentum since, its introduction in India and has played a major role in Indian financial markets. More importantly, Commodity Market is one of the important tools of hedging risk. A Commodity market broadly is an Agriculture market whose payoff structure is determined by the value of underlying commodities, exchange rate, oil price, and the like. So a Commodity market comprises of trade instruments which derive their value from some underlying variable assets like food grains such as wheat, rice pulses etc. All commodity markets are based on some " cash " products. Though the agricultural sector contributes significantly to the Indian economy, it faces several bottlenecks, one of those being the antiquated laws governing agricultural marketing and price discovery, leading to low price realization by Indian farmers. In India, six national level exchanges offer commodity derivatives contracts on commodities, with some having electronic spot commodity exchanges to facilitate spot trading of commodities. The present study is analysis into agricultural and non-agricultural commodities in Indian commodity markets.
Indian markets have recently thrown open a new avenue for retail investors and traders to participate commodity derivatives. For those who want to diversify their portfolios beyond shares, bonds and real estate, commodities are the best option. Till some months ago, this wouldn't have made sense. For retail investors could have done very little to actually invest in commodities such as gold and silver or oilseeds in the futures market. This was nearly impossible in commodities except for gold and silver as there was practically no retail avenue for pumping in commodities.The history of commodity derivative market in India dates back to the ancient times, but the first organized market was established in 1875. However, by mid 1960s government took a drastic step by banning derivatives trade altogether. The commodity derivative market remained virtually absent in next four decades and it made the restart only in early 2000s. Since its reintroduction it is thriving and the current trend shows strong growth potential of the market, although, the actual growth trajectory will depend upon the attitude of the policy makers and the efficiency of the regulatory mechanism. The present study makes an overview of the Indian commodity derivatives market. The study discusses the evolution and performance of the market, its present status and the future prospect.
IJAR Indexing
India is among the top producers of a number of commodities and has a long history of trading in commodity derivatives. Futures, as a derivative tool of risk management have existed in India. Major industries of its economy like sugar, textile, metal, energy etc. are based on various commodities. Today, India enjoys world ranking with respect to trading volume in certain commodities like Silver, Gold, Copper, Guar Seed etc. So, far the financial returns are concerned commodity futures markets allow commercial producers and commercial consumers to offset the risk of adverse future price movements in the commodities that they are selling or buying besides the price discovery tool. So considering these points, an attempt has been made to study the performance of commodity futures trading in India.
International Journal of Pure & Applied Bioscience
Mohammad Irshad VK
Against the well-established fact that the usefulness and suitability of futures trading in developing the underlying agricultural commodity market, especially in agriculture based country like India are been questioned by various bodies. Through this work it has been analysing the role of futures trading on agricultural commodities. The effect of futures trading may be of two kinds, that is by way of hedging the price risk and the other way is through price discovery. The data collected for the analysis are the daily price history of spot and futures of five major agricultural commodities (Castor Seed, Chana, Chilli, Jeera, and Wheat) for a period of 7 years started from 2007 to 2014. Here it is revalidated the relationship between price movements of agricultural commodity futures and underlying spot prices by applying econometric analysis tools like Unit Root Test, and Engel-Granger test of Cointegration. The empirical findings proved that an existence of interrelationship between futures price movement and respective underlying spot prices in Indian agricultural commodity market.
roshini philip
Bhaskar Goswami , Isita Mukherjee
The paper is a humble attempt to address the issues related to agricultural commodity futures market in India. An empirical analysis is carried out in terms of 10(ten) agricultural commodity futures (Chana, Jute, Kapas, Pepper, Wheat, Rice, Potato, Yellow peas, Sugar and Urad) for the period 2004-2012. The study reveals that in an environment of persistent inflation in the economy coupled with global recession, agricultural commodity futures market is not a favourite destination for the investors to put in their savings.
On agricultural commodity futures, there is always been a doubt, expressed by different bodies and experts on the usefulness and suitability of futures contract in developing in underlying agricultural commodity market, especially in agricultural based economy like India. Here an attempt is made to revalidate the impact of futures trading on agricultural commodity market. The daily price information in spot and futures market for a period of seven years ranging from 2007 to 14 for five major agricultural commodities are VAR (Vector Auto Regression) and GARCH (1,1) to test the dynamic interrelationship among the variables. The empirical findings significantly shows that comparative advantage of futures market in disseminating information, leading to a significant price discovery and risk management, that can help to successfully develop the underlying commodity market in India.
Statistical analysis of impact of COVID 19 on India commodity markets
How did a fitness crisis translate to a financial crisis? Why did coronavirus bring the worlds financial system to its knees? The solution is to be found in two approaches. Initially, this contagious virus resulted in a lockdown of the business world and economy. A ratio at which the virus has been proliferating, the increased uncertainty is exactly the reason for this bad situation which resulted as unsecured initialization investment between traders. We rely on the present environment in evaluating the constricting procedures, fiscal policies and public health actions that were implemented in that time. This study examines volatility transmission over the financial crisis. Recently established causality in impulse response functions and variance test to everyday data from January 2020 has been implied. To recognize the effect of the food cost crisis, statistics are separated into two intervals i.e. post-COVID period and the pre-COVID period. The variance causality test indicates that the risk transmission among agricultural commodity is zero, but oil market volatility spills on the markets for agricultural products excluding sugar in the post-crisis period. Thus, this paper signifies that the statistical volatility transmission differs post food price crisis. Following, risk transmission materializes as an additional element of the statistical interrelations among agricultural and energy markets.
1. Introduction
By and large Implications of COVID-19 for creating nation economies Worldwide, the quantity of instances of coronavirus has outperformed the emblematic limit of 100,000. In the wake of watching the encounters of China, Italy, Iran, South Korea, France and other early-influenced nations, an ever increasing number of nations are receiving measures to contain the spread of the infection that include: travel limitations, daily curfews, a prohibition on open occasions, the conclusion of schools, cinemas, exhibition halls, bars and shops. These uncommon measures are pushing down on the world economy, which is debilitating quick. The hit to Gross Domestic Product (GDP) is relied upon to be high, particularly if these limitations most recent a while.
In any case, nobody can dependably anticipate the full monetary effect of the outbreak and theories are evolving quickly. A lot of relies upon what is mysterious—to what extent the outbreak endures, what number of nations it harrows, and the degree to which an organized, coordinated, quick track strategy reaction is prepared and continued. In any case, what we cannot deny is that the flare-up showed up at a powerless point for the world economy, exactly when worldwide development was starting to get from its most reduced rate since the 2009 money related emergency.
That has upsetting ramifications for creating economies: Tighter credit conditions, more fragile development, and the preoccupation of government assets to support human services frameworks and battle the flare-up would decrease finances accessible for key advancement needs. A financial droop would likewise hamper the battle against extraordinary neediness. It is basic, in this way, that policymakers wherever perceive how financial damage can be transmitted starting with one nation then onto the next—and to act rapidly to forestall its spread.
That transmission of monetary damage is probably going to happen through a few channels .
- • The first is through worldwide exchange and costs: worldwide worth chains, which represent almost 50% of worldwide exchange, are being upset by processing plant shutdowns and deferred resumption of activities. Ware costs therefore will likewise be disturbed
- • The second is through remote money related streams, which could decrease (on account of settlements) or be moved away from coronavirus-influenced nations.
- • The third is through the travel industry, a significant income stream for some creating nations that is contracting with declining request and growing travel limitations; and
- • The fourth is through local capital—human just as monetary—which is turning out to be underutilized as processing plants are sat and individuals remain at home.
2. Literature review
The connection among oil and farming costs as far as oil as a creation expense in horticulture was inspected by Harri and Hudson [13] , Baffes [3] , [4] , Alghalith [4] , Chang and Su [8] , Alom et al. [2] , Du et al. [10] . Baffes [3] broke down how unrefined petroleum costs spill on the costs of 35 globally exchanged essential items and found that the go through of raw petroleum value variations to the general non-vitality product list, the compost list, farming and metals are 0.11, 0.17, 0.33 and 0.16, individually. At a greater number ofdisaggregatinglevels, Baffes [4] rethought this relationship and reasoned that the most elevated go through of oil value changes is to the compost file followed by farming. The connection among oil and agrarian costs regarding biofuels was inspected by Zhang et al. [25] , Busse et al. [5] , Hassouneh et al. [15] , Kristoufek et al. [19] , and Serra [23] . Serra [23] examined the instability overflow among unrefined petroleum, sugar and ethanol costs in Brazil. She noticed that there are solid instability connections among the costs and that raw petroleum and sugar advertise stuns trigger an expansion in the unpredictability of the ethanol cost. This demonstrates the presence of a powerful connection among fuel, biofuel, and agrarian markets. In an alternate report, Serra et al. [24] dissected the value relationships and transmission designs in the U.S. ethanol industry and found that there exist since quite a while ago run connections among the costs of ethanol, pulses, oil and gas just as solid connections among vitality and food costs. Like Serra [23] , they reveal a since a long time ago run synchronization connection among oil, biofuel and horticultural marketplaces. Hassouneh et al. [15] inspect the value relationships and value transmission designs among food and vitality costs in Spain. They discovered that there is a since quite a while ago run, synchronisation connection among sunflower, biodiesel, and raw petroleum costs; that biodiesel is the main variable that acclimates to deviations from the since quite a while ago run relationship and that sunflower oil costs are affected by vitality costs through short-run value elements. Kristoufek et al. [19] dissect the presence of the connection among ethanol, biodiesel, and related fills and ware costs in the Germany and US. Their outcomes demonstrate that even though biofuel is influenced by fuel and food costs, biofuel costs has a restricted limit in the assurance of food costs. They additionally found that the connection between costs changes relying upon the information recurrence utilized. Busse et al. [5] explored the vertical value transmission in the biodiesel flexibly chain in Germany by concentrating on the associations between costs of vegetable oil, biodiesel, soy oil, and raw petroleum. They discovered proof for a solid effect of unrefined petroleum cost on biodiesel costs, and of biodiesel costs on vegetable oil costs. Zhang et al. [25] break down both short-and since quite a while ago run connection between costs of fuel and horticultural wares. Their outcomes show that there is no immediate since quite a while ago run value connection among fuel and agrarian ware costs and there is possibly constrained, assuming any, immediate short-run connections. Concerning the writing as far as co-development of ware costs with macroeconomic variables and budgetary pointers, Krichene [18] explores oil value developments between January 2000 and October 2007. He contends that the fast increment as of late saw in oil and other item costs can be ascribed to the expansionary financial strategies during the mid-2000s. Because of the effect of expansionary arrangements, world interest for wares rose while gracefully falled behind, squeezing all product costs. He calls attention to the new elements in ware costs because of facilitating of money related position in the late 2007 and mid-2008. Du et al. [10] examined the job of hypothesis in driving unrefined petroleum value variety subsequent to controlling for other impacting factors. They additionally endeavor to measure the degree to which instability in the raw petroleum showcase go through into rural ware markets (wheat and pulses) in the U.S. They discovered proof of unpredictability overflow among unrefined petroleum, pulses, and wheat showcases after the fall in 2006, suggesting hazard move among these product markets. Cevik and Sedik [7] inspect the outrageous changes in product costs (wine and oil) somewhere in the range of 1990 and 2010. As indicated by their outcomes, macroeconomic variables rise as the primary determinants of product costs. They additionally call attention to that, albeit significant economies represent around half of world utilization, developing markets make up a critical steady option to request. Whatever the purpose for the since quite a while ago run pattern or short run vacillations is, raw petroleum as well as other ware spot costs followed a comparable example. They all accomplished consistently expanding patterns first and afterward an exceptional droop after the worldwide emergency. Evaluating a few elective hypotheses of the raw petroleum value climb in 2008, going from interest and flexibly elements to product hypothesis, Hamilton [12] presumes that as opposed to being elective clarifications, they may together be liable at the cost stun. As respects to hazard move among vitality and rural markets, in one of the early endeavors to find unpredictability overflow. Study Data Method Commodity Key discoveries Baffes [3] 1960–2005 (yearly) OLS Crude oil 35 products Pass-through of raw petroleum cost changes to the general non-vitality ware file, the compost list, agribusiness and metals Harri and Hudson [13] 2003–2009 (day by day) Causality Crude oil, pulses, mazies Volatility overflow from oil costs to pulses costs after the food cost emergency Krichene [18] 2000–2007 (every day) LPGHT Crude oil Expansionary fiscal approaches during the mid 2000s influence oil costs Alghalith [1] 1974–2007 (yearly) NOLS Crude oil, food bushel An increment in oil cost and its instability yields a higher food value Baffes [4] 1960–200 (yearly) OLS Energy record Non-vitality list The manure list shows the biggest go through followed by horticulture and metals. Busse et al. [5] 2002–2009 (week by week) MS-VECM Biodiesel, rapeseed oil, soya oil, raw petroleum Strong effect of raw petroleum cost on biodiesel costs, and of biodiesel costs on rapeseed oil costs Chang and Su [8] 2000–2008 (day by day) EGARCH Crude oil, pulses, soybean Volatility overflow from raw petroleum to pulses and soybean costs Zhang et al. [25] 1989–2008 (month to month) VECM Ethanol, pulses, rice, mazies, sugar, wheat, gas, raw petroleum No direct since a long time ago run value relations among fuel and agrarian product costs Alom et al. [2] 1995–2010 (every day) VAR Crude oil, food value list Positive connection between's records of world oil and food makers value instability. The outcomes fluctuate across nations and sub-periods. Cevik and Sedik [7] . 1990–2010 (month to month) OLS Crude oil, fine wine Macroeconomic components are the principle determinants of product costs Du et al. [10] 1998–2009 (week after week) SVM Crude oil, pulses, wheat Volatility overflow among unrefined petroleum, pulses, and wheat markets Serra [23] 2000–2009 (month to month) SP-GARCH Ethanol, raw petroleum, sugar Shocks on raw petroleum and sugar showcase cause an expansion in the unpredictability of ethanol cost. Serra et al. [24] 1990–2008 (month to month) ST-VECM Ethanol, pulses, unrefined petroleum, gas Long-run connections among the costs just as solid connections among vitality and food costs. Harri and Hudson [13] noticed that there is unpredictability overflow from oil prospects costs to pulses fates costs after the food value emergency. In accordance with this discovering, Chang and Su [8] gave proof of unpredictability overflow from raw petroleum to pulses and arraigned that soybean costs are emphatically critical throughout the sophisticated raw petroleum value period, inferring a monetary replacement impact throughout the higher raw petroleum value period. Notwithstanding unpredictability transmission among world farming and world oil wares, a few examinations focused on the instability transmission from globe oil costs to residential horticultural item costs. For example, Alghalith [1] examined the effect of oil cost vulnerabilities on food costs in Tobago and Trinidad and found that an expansion in oil cost and its instability produces a greater food cost. The finding that a higher hazard in the oil showcase prompts a greater food cost proposes that there exists a hazard move instrument between the two item advertises. Alom et al. [2] explored instability overflow from world oil costs to food costs for the chose Pacific and Asia nations and noticed that food value unpredictability is decidedly related with world oil value unpredictability however the outcomes shift around nations and sub-periods. Besides, their outcomes infer that unpredictability overflow from globe oil to domestic’s food costs are more grounded for the later sub-time frame, demonstrating expanding interdependency among Asia Pacific horticultural and world oil markets throughout the ongoing years. Evidently there is a quickly developing writing on the elements.
3. Objectives
This articleexplores the volatility spilloverimpactsamong the agriculture products and crude oil market and estimates statistical conditional correlations. The major contributions of the article are as follows.
- (1) The variousqualities of volatility spilloverimpactsamong each commodity market and crude oil after and before the COVID-19 are evaluatedsystematically, and the impact of crude oil fluctuations in the priceson different commodity markets is being compared.
- (2) Ultimately, we assess the statistical conditional correlation among each commodity market andthe crude oil market andevaluate the time-differingqualities of correlations after and before the COVID-19.
4. Econometric methodology
To evaluate the volatility spill over between world oil and horticultural item costs, this examination receives causality in change test as of late created by Hafner and Herwartz [11] . In analyzing volatility spill over among two arrangement, Cheung and Ng [9] and Hong [16] built up a causality-in-difference test which depends on CCF (cross-relationship capacities) of normalized residuals acquired from univariate GARCH (general autoregressive restrictive heteroscedasticity) assessments. The CCF in accordance with the Portmanteau test is probably going to be experiencing huge oversized in little and moderate examples while the unpredictability forms are leptokurtic [11] . Furthermore, findings from CCF on the basis of testing methodology are delicate to the sets of leads and slacks which thus addresses the heartiness of discoveries. The instability overflow trial of Hafner and Herwartz [11] consideringLM (Lagrange multiplier) rule defeats the setbacks of Cheung and Ng's technique and is handy for exact outlines. Moreover, the Monte Carlo test completed in Hafner and Herwartz [11] demonstrates that the LM methodology is increasingly powerful against leptokurtic developments in little examples and the addition from conveying the LM test increments with test size. The outcomes further demonstrate that an unseemly lead and fellow request decision in the CCF test contorts its exhibition and along these lines prompts the danger of choosing an off-base request of the CCF measurement. In what happens next, we quickly clarify the subtleties of Hafner and Herwartz [11] causality in change test. In the Hafner and Herwartz [11] methodology, experimentation for causality in change depends on assessing univariate GARCH models. The invalid theory of non-causality in fluctuation among two return arrangement is depicted as in the following example
where ε it and Ft (j) = Ft\σ(εjτ, τ ≤ t) are the residuals from GARCH model. The next modelis deemed to be test for the null assumption.
where conditional variance σit 2 = ωi + αiε i,t−1 2 + βiσ i,t−1 2 and ξitsignifies the consistent residuals of GARCH model. In Eq. (2) , the adequate condition for Eq. (1) is π = 0 which certifies that the null assumption of non-causality in difference H0:π = 0 is tested against the alternate assumption H1:π ≠ 0. The total of the Gaussian loglikelihood function of εit is provided by xit(ξit 2–1)/2 where the derivatives xit = σit − 2 (∂σit 2 /∂θi) and θi=(ωi, αi, βi)′. Hafner and Herwartz [11] recommend the subsequent LM test to test the volatility transmission among the two sequences:
Asymptoticalallocation of the test value in Eq. (3) will rely upon the quantity ofspecification pointers in z jt . While there are two specification pointers in λ LM , the test has an approximate chi-square circulation with twoDoF.
Notwithstanding testing for causality in difference between world oil and horticultural ware costs, this examination utilizes impulseresponse investigation so as to decide how the instability of farming item costs react to a stun in world oil value unpredictability. In such manner, we use the summed up drive reaction strategy supported by Pesaran and Shin [22] and Koop et al. [17] which is better than the conventional methodology since it isn't dependent upon the symmetry study emerging from Cholesky requesting and the outcomes from the summed up drive reaction capacities are not delicate to the requesting of factors in the vector autoregression (VAR) framework.
We utilize day by day information covering the period before COVID-19 at the spot costs of world oil, pulses, maize’s, sugar and wheat. The information on all arrangement is accumulated from DataStream.
The arrival arrangement — ln(p t / pt−1 ) where p t is the cost at day t — are utilized in the exact investigation and the characteristic logarithms of the factors are organized in 5-day during the week. With respect to oil cost rural ware costs nexus, it has currently been contended that horticultural item costs are not receptive to the oil costs till 2006 [6] . Before COVID-19, the food value emergency brings about higher relationship between the financial crisis. To represent the effect of the food value emergency, it is helpful to isolate the full example into subcategories (for instance, [6] , [13] , [14] , [20] , [21] ). We thusly work with twogroups: (i) the pre-emergency period crossing before COVID-19, and (ii) the post-emergency period which will cover the time structure after COVID-19.
Table 1 addresses to the elucidating measurements for the sub-time frames. It appears that information qualities of the arrival arrangement are marginally unique in the pre-and post-emergency periods. As a matter of first importance, true to form, the mean and the unpredictability of the profits in the post-emergency period are higher in comparison to the pre-emergency period. Furthermore, the kurtosis, skewness, standard deviation, and meanof the world oil returns are more prominent than those of the horticultural product returns in the pre-emergency period. Then again, the rural product returns have greater skewness and mean in comparison to the oil returns. In the post-emergency period, standard deviation for maize’s and pulses are significantly greaterin comparison to different factors. This in truth is relied upon because of the way that the oil cost flood during the ongoing years expands the determined interest for the agrarian items, for example, pulses and maize’s which are utilized in biofuels creation which thusly prompts a considerable ascent in the costs of those products. The various information attributes in the post-and pre-emergency periods bring up the issue of whether the relationship among the world oil and horticultural ware returns change over the sub-time frames.
NarrativeStatistics.
6. Analysis approach
6.1. statistical conditional correlation.
To further examine the time-differingqualities of correlation among the crude oil market and each commodity market and to evaluateadjustments before and after the COVID-19, we projected the statistical conditional correlation coefficients. Fig. 2 a represents the statistical conditional correlation among the agricultural products and crude oil market. The correlation demonstrates markedly dissimilarqualities after and before the COVID-19. Before the COVID-19, the conditional correlation coefficient initiallyreduced and then improved, demonstrating a different V-type trend, with the turning point in the vicinity. Though, following the financial crisis, correlation among the agricultural products and crude oil marketimproved, surpassing the pre-crisis high point and changingto a lesser degreeat a greater level. By contemplating trends for the agricultural products and crude oil price index in Fig. 1 , the adjustments in correlation could be explained. Though, price differences for crude oil and other goods are not completely synchronized. Global investors were enthusiastic about the outlook for the crude oil reason for this is that it is a key strategic resource, so a huge amount of unpredictable capitalwas flowing to the crude oil market, which additionally drove up oil prices, so an stockreplacement impact of crude oil on other raw materials marketsis evident. Later, to prevent risks, the market livestockimpacttriggered a flow of speculative moneyfrom the economic markets to the commodities markets. In this phase, the cost of most basic goods rapidly improved, so the relationship among the agricultural products and crude oil market continuously improved.

Developments for the crude oil price and the agricultural products.

Conditional correlation between crude oil and commodity markets.
7. Impacted nations
A decline in Chinese flexibly of rational information sources can affect the profitable limit and along these lines the fares of some arbitrary nation relying upon how dependent its enterprises are on Chinese providers. For instance, some’s European vehicle producers may confront the deficiency of basic segments for their activities, organizations in Japan may discover hard to acquire parts important for the gathering of computerized cameras, etc. For some organizations, the restricted utilization of inventories brought by a lean and without a moment to spare assembling procedure would bring about deficiencies that will affect their creation abilities and by and large fares. Table 1 states by division the potential impact of COVID-19 on trades in the most presented nations to Chinese flexibly interruptions. Generally, the most affected economies will be the European Union (hardware, automobiles, and synthetic compounds), the United States (apparatus, automobiles, and exactness instruments), Japan (hardware and automobiles), the Republic of Korea (transmission equipment and hardware), Taiwan Province of China (office equipment and transmission equipment) and Viet Nam (transmission equipment).
8. Conclusion
This article researches volatility spillover among oil and chose farming item advertises (wheat, maizes, pulses and sugar) that are important agrarian items for biofuel production and for food on the planet. The information crossing is separated into two periods as when COVID-19 (the post-emergency period) to represent the item emergency. In the elucidating examination, we saw that item advertise returns follow comparable properties as budgetary returns. It is settled that oil markets have consistently pulled in worldwide financial specialists. The way that noteworthy hazard transmissions are seen from oil to agrarian product showcases after the emergency recommends that speculators looking for places of refuge increment the financialization of the rural item advertises. This proposes nearby measures to smother value vulnerability in farming markets might not be compelling in the short term. The worldwide factors, for example, the hazard in vitality markets appear to drive the short run unpredictability in farming markets. The significance of this impact is better for nations that are generally helpless against food value variances. Even more light can be dropped on this problem by contemplating the reactions of neighborhood horticultural costs to worldwide vitality stuns. Moreover, financial specialists keen on item markets ought to understand that hazard in one ware market may not be autonomous of hazard in other ware markets. Further examination is fundamental so as to discover how chance transmission system functions among various money related and item advertises. The elements of product costs are muddled, and various components might be influencing these business sectors. The ongoing conversation recommends that notwithstanding the vitality farming linkage, the budgetary factors, for example, trade rates, prospects markets, theory, and financing costs may assume job on the ongoing elements of product costs. In this manner, examining agrarian value assurance proposes the requirement for increasingly experimental examination to mutually recognize the effects of different factors on rural costs. In that regard, future investigations can expand the writing in any event in two different ways. In the first place, inquiries about can profit by the adaptability of auxiliary vector autoregression models to force hypothetical limitations to recognize effects of vitality and money showcases on agrarian markets. Besides, multivariate unpredictability overflow examination which gives space to apply causality in fluctuation tests and drive reaction investigation dependent on multivariate models may give new experiences.
Declaration of Competing Interest
The authors declare that they have no known competing financial interests or personal relationships that could have appeared to influence the work reported in this paper.
Further Reading
Baltimore bridge collapse: What happened and what is the death toll?
What is the death toll in the bridge collapse, when did the baltimore bridge collapse, what factors played a role in the bridge collapse, how much will the baltimore bridge cost to replace, how long will it take to rebuild the baltimore bridge.
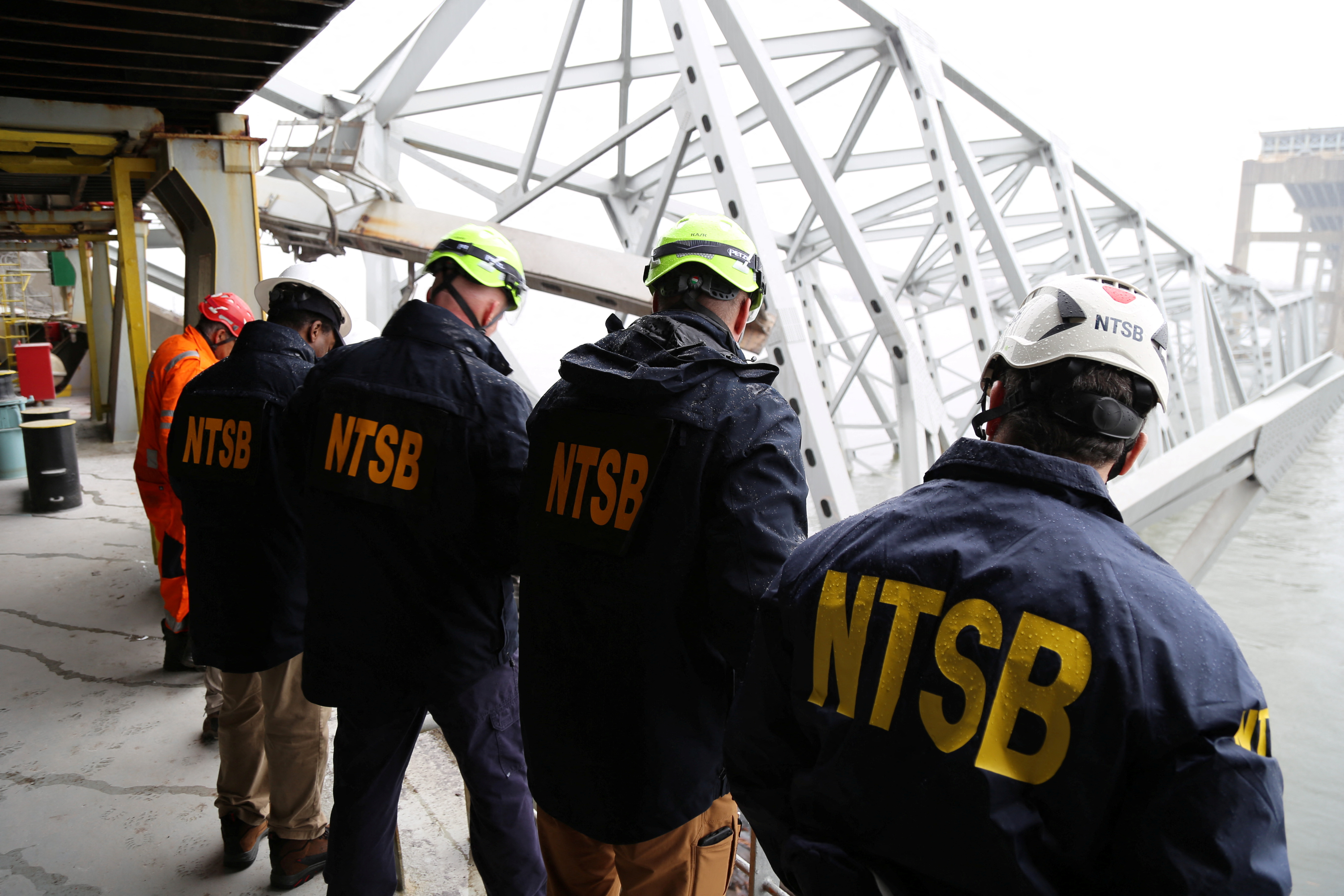
WHAT TO KNOW ABOUT THE CARGO SHIP DALI AND ITS STRANDED CREW
What do we know about the bridge that collapsed.

HOW WILL THE BRIDGE COLLAPSE IMPACT THE BALTIMORE PORT?

Get weekly news and analysis on the U.S. elections and how it matters to the world with the newsletter On the Campaign Trail. Sign up here.
Writing by Lisa Shumaker; Editing by Steve Gorman, Daniel Wallis and Bill Berkrot
Our Standards: The Thomson Reuters Trust Principles. , opens new tab

Thomson Reuters
Lisa's journalism career spans two decades, and she currently serves as the Americas Day Editor for the Global News Desk. She played a pivotal role in tracking the COVID pandemic and leading initiatives in speed, headline writing and multimedia. She has worked closely with the finance and company news teams on major stories, such as the departures of Twitter CEO Jack Dorsey and Amazon’s Jeff Bezos and significant developments at Apple, Alphabet, Facebook and Tesla. Her dedication and hard work have been recognized with the 2010 Desk Editor of the Year award and a Journalist of the Year nomination in 2020. Lisa is passionate about visual and long-form storytelling. She holds a degree in both psychology and journalism from Penn State University.
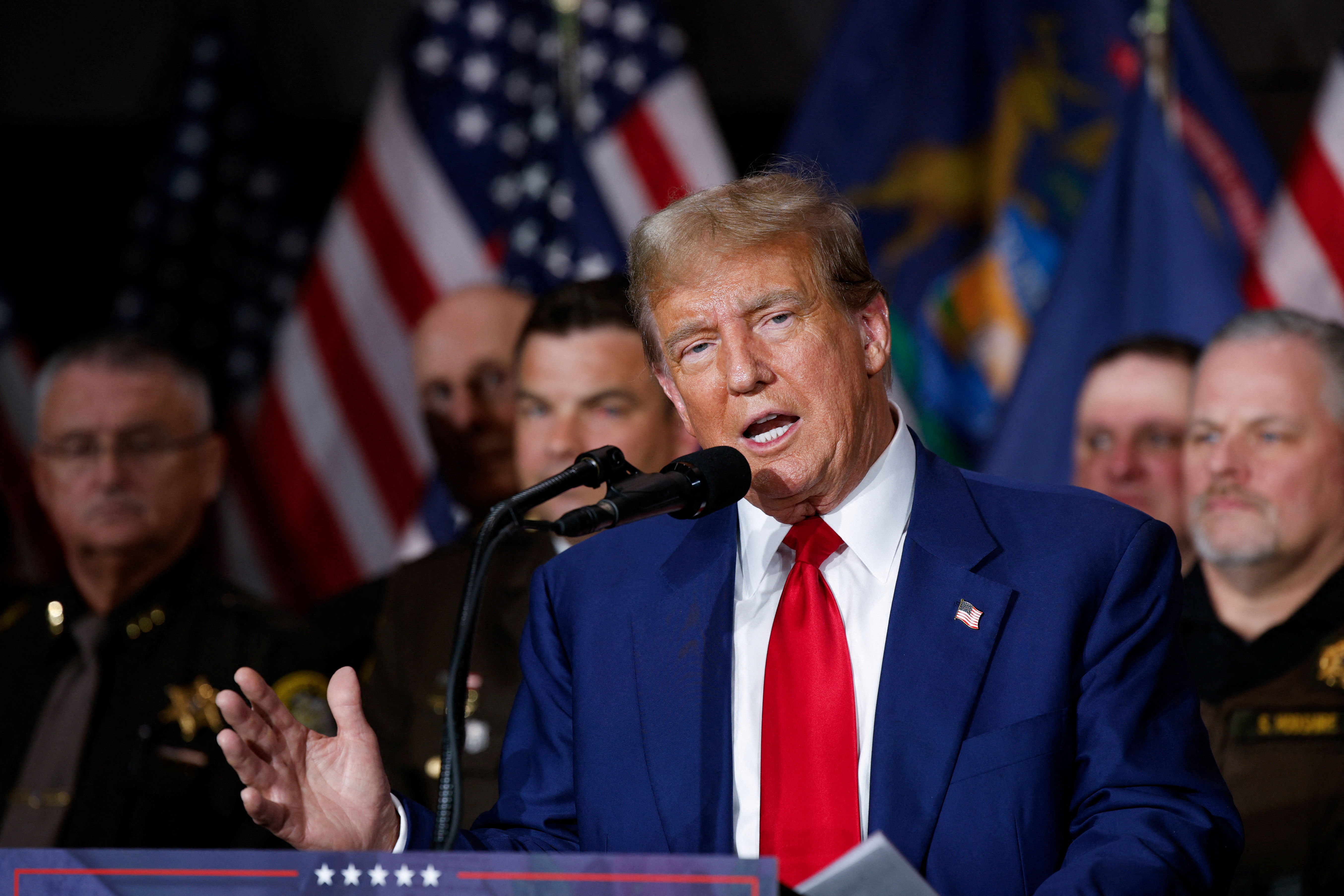
Trump campaign, RNC triple fundraising in March to $65.6 million
The election campaign of former U.S. President Donald Trump and the Republican National Committee said on Wednesday they raised over $65.6 million in March, more than three times the amount raised in February.
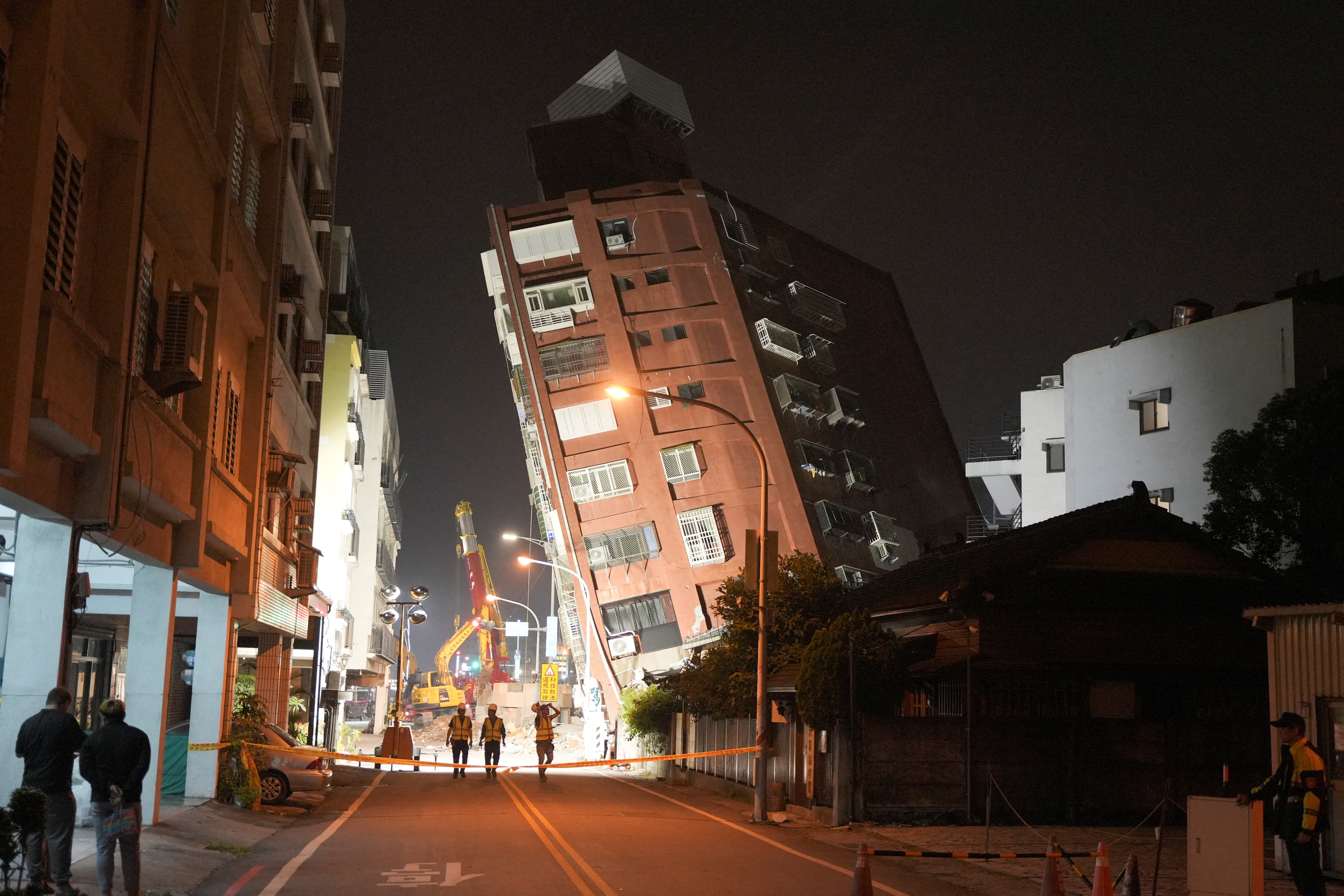
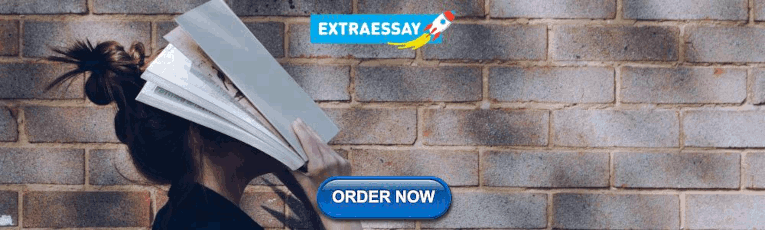
COMMENTS
Kaul, S. (2007), "Commodity Futures Trading in India-Myths and Misconceptions", NCDEX Institute of Commodity Markets & Research (NICR) Working Paper. Emerging Commodity Exchanges in a Globalized ...
for pumping in commodities.The history of commodity derivative market in India dates back t o the ancient times, but the first organized market was established in 1875. However, by mid 1960s
The prime objective of the research is to observe the macroeconomic variables causes of the volatility of the commodity market in India. This research study has another two minor objectives; the first one is to investigate the extrapolative power of changes in macroeconomic factors for the Indian commodity future market volatility.
This article aims to find out interlinkages between equity and commodity markets through the channel of investors' outlook in the equity market. ... Commodity futures market efficiency in India and effect on inflation. Indian ... The relationships between sentiment, returns and volatility. EFMA 2004 Basel Meetings Paper. Cass Business School ...
This paper attempts to throw light on commodity market in India and to find out the impact of the SEBI-FMC merger and also to analyze future growth prospects and challenges of commodity market ...
The total value of agri-commodities futures had a steady increasing trend till 2011-2012, as depicted in Table 4 and Figure 1. Thereafter, the growth momentum began to show a declining trend till 2014-2015. There was a marginal increase in the value of trade comprising ₹56.36 trillion in 2015-2016.
Abstract: The research investigated the impact of macroeconomic variables on the volatility of the commodity futures market in India (together with oil futures, agricultural commodity futures and metal futures). The monetary policies, financial market infor-mation and economic environments are determined by the macroeconomic variables.
Our current paper is related to a field of research highlighting the importance of strategic commodities such as crude oil and gold to the risk of ... Commodity market volatility indexes have a positive impact on the volatility of green companies ... It is noteworthy that India is one of the leading commodity importers in the world. In 2018 ...
The study used the daily closing futures price of gold, silver, aluminum, copper, lead, nickel, zinc, crude oil, and natural gas traded at MCX. According to Table 3, MCX has the highest share of the total value of trade (from 63.53% to 86.64%) among commodity exchanges in India over the period from 2006 to 2018.
Background This paper examines the pattern of the volatility of the daily return of select commodity futures in India and explores the extent to which the select commodity futures satisfy the Samuelson hypothesis. Methods One commodity future from each group of futures is chosen for the analysis. The select commodities are potato, gold, crude oil, and mentha oil. The data are collected from ...
Commodity Markets - Jayant Nalawade 08 Special Focus: Precious Metals Theme Note: Precious Metals - Prospects, Issues and Perspectives 14 2. Gold as an Asset class for Investment - Latha Chari, Pradiptarathi Panda and V. R. Narasimhan 16 3. What would it take India to be a price-setter in global gold market? - G. Chandrashekhar 22 4.
commodity markets in India. Data is collected for a 5 year period which ranges from 2015 to 2019. ... The research has been conducted based on past one year data using Exponential Moving Average (EMA) and line chart. ... commodity markets functioning in India. The paper indicates, Overview of the commodity market, commodity market development ...
Commodity futures market, in India, which started working way back in 1875, provides a platform for various stakeholders of commodity to hedge their price risk. ... Kamlesh Gakhar and Ms. Meetu (2013) in Sagar Suresh Dhole (2014) in his research paper "Derivatives Market in India: Evaluation, Trading "Commodity Futures Market in India: The ...
Narender L Ahuja (2006) in his research on "Commodity Derivatives market in India: Development, Regulation and Future Prospective", concluded that Indian commodity market has made enormous progress since 2003 with increased number of modern commodity exchanges, transparency and trading activity. The volume and value of
With respect to commodity derivatives, the research "Commodity Futures Market in India: A Study of Trends in the "Notional Multi-Commodity Indices" by Bose (2009) aimed to comprehend the ...
The variance causality test indicates that the risk transmission among agricultural commodity is zero, but oil market volatility spills on the markets for agricultural products excluding sugar in the post-crisis period. Thus, this paper signifies that the statistical volatility transmission differs post food price crisis.
3) To study the different segments of Indian Commodity Markets. 4) To analyze the regulatory framework of commodity market in India. 5) To study the challenges as faced by commodity market in India. III. Review Of Literature Pravakar shoo and Rajiv Kumar (2009) has evaluated that trading in commodity derivatives on
1Bangalore University, Bengaluru, India _____ Abstract: This study is descriptive in nature and the paper reviews the literature on commodity market and risk associated with commodity investment, the different sources of return in commodity market and the effect of different macro-economic variables like interest rate, inflation on the return.
commodity market. But, the spread of the commodity market network and value of trade shows the high performance in this field. 4) (Bansal, Dadhich, & Ahmad, 2014) examines that over the years the commodity market in India has grown and especially certain commodities like metals that have been traded excessively. There was a
The present study aims to understand the status of Agri-Commodity Markets in India with special focus on Market Efficiency, Price Discovery and Risk Management. IV. RESEARCH METHODOLOGY ... (2014)in their research paper, "Market Efficiency of commodity futures in India" analyses the market efficiency and long run equilibrium relationship of ...
Kaul, S. (2007), "Commodity Futures Trading in India-Myths and Misconceptions", NCDEX Institute of Commodity Markets & Research (NICR) Working Paper. Recommended publications Discover more about ...
JETIR1705068 Journal of Emerging Technologies and Innovative Research (JETIR) www.jetir.org 355 Commodity Derivatives Market in India- A Study Manjunath Narasagondar Asst Professor of Commerce ... This paper seeks 1. To study the Commodity Derivatives Market in India. 2. To identify the road blocks in providing interface to execute the ...
CPI. The analysis presented in our paper exploits a large monthly dataset on agricultural price movements for 21 different commodities and 60 different markets (mandis) in India. The measure of market integration we consider is the cross-market price differential across each market pair in our sample.
After the bridge collapse in 2007 in Minnesota, Congress allocated $250 million. Initial estimates put the cost of rebuilding the bridge at $600 million, according to economic analysis company ...