- Skip to main content
- Skip to primary sidebar
- Skip to footer
- QuestionPro

- Solutions Industries Gaming Automotive Sports and events Education Government Travel & Hospitality Financial Services Healthcare Cannabis Technology Use Case NPS+ Communities Audience Contactless surveys Mobile LivePolls Member Experience GDPR Positive People Science 360 Feedback Surveys
- Resources Blog eBooks Survey Templates Case Studies Training Help center

Home Market Research Research Tools and Apps
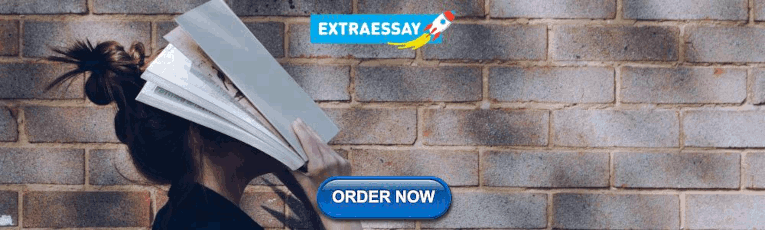
Analytical Research: What is it, Importance + Examples
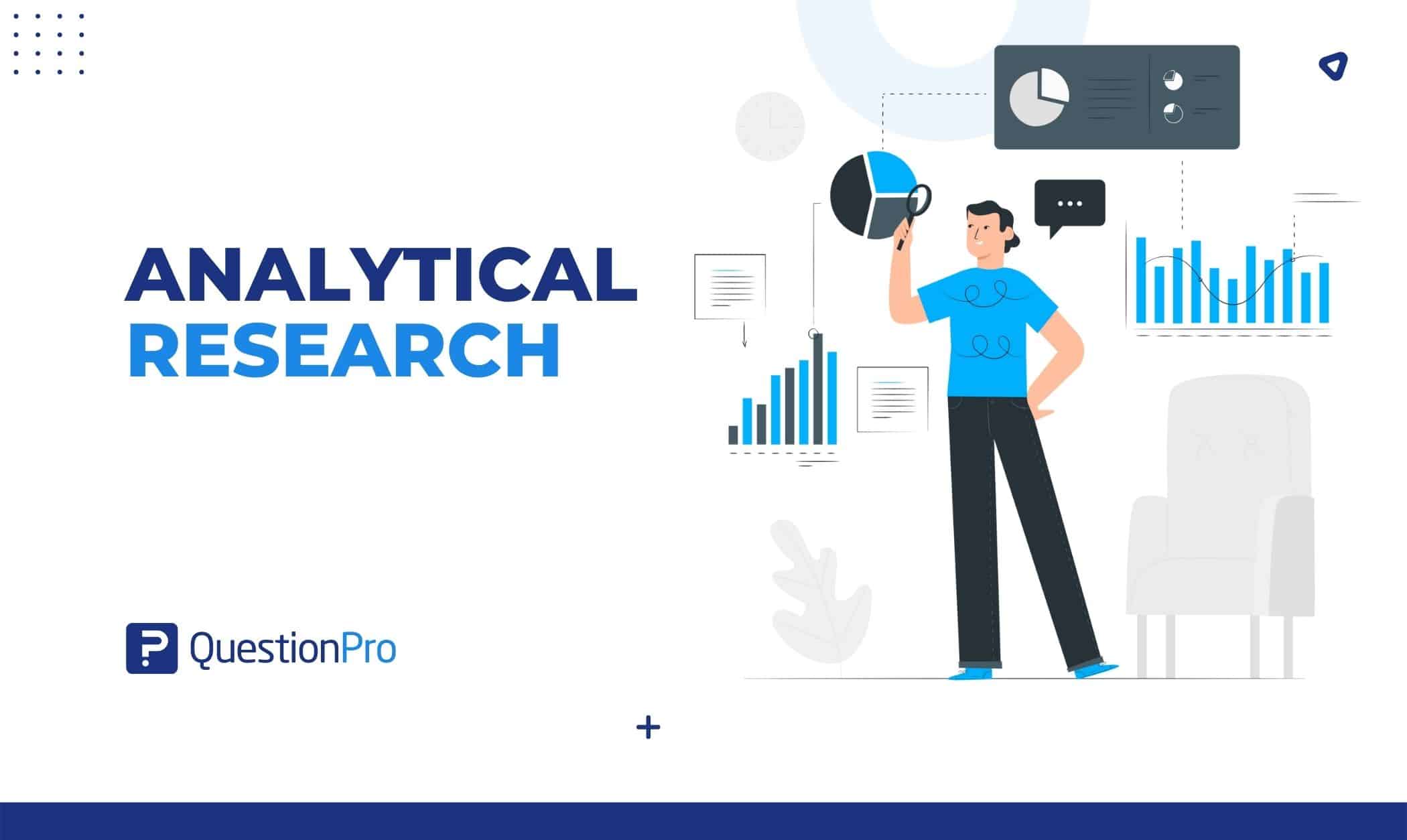
Finding knowledge is a loose translation of the word “research.” It’s a systematic and scientific way of researching a particular subject. As a result, research is a form of scientific investigation that seeks to learn more. Analytical research is one of them.
Any kind of research is a way to learn new things. In this research, data and other pertinent information about a project are assembled; after the information is gathered and assessed, the sources are used to support a notion or prove a hypothesis.
An individual can successfully draw out minor facts to make more significant conclusions about the subject matter by using critical thinking abilities (a technique of thinking that entails identifying a claim or assumption and determining whether it is accurate or untrue).
What is analytical research?
This particular kind of research calls for using critical thinking abilities and assessing data and information pertinent to the project at hand.
Determines the causal connections between two or more variables. The analytical study aims to identify the causes and mechanisms underlying the trade deficit’s movement throughout a given period.
It is used by various professionals, including psychologists, doctors, and students, to identify the most pertinent material during investigations. One learns crucial information from analytical research that helps them contribute fresh concepts to the work they are producing.
Some researchers perform it to uncover information that supports ongoing research to strengthen the validity of their findings. Other scholars engage in analytical research to generate fresh perspectives on the subject.
Various approaches to performing research include literary analysis, Gap analysis , general public surveys, clinical trials, and meta-analysis.
Importance of analytical research
The goal of analytical research is to develop new ideas that are more believable by combining numerous minute details.
The analytical investigation is what explains why a claim should be trusted. Finding out why something occurs is complex. You need to be able to evaluate information critically and think critically.
This kind of information aids in proving the validity of a theory or supporting a hypothesis. It assists in recognizing a claim and determining whether it is true.
Analytical kind of research is valuable to many people, including students, psychologists, marketers, and others. It aids in determining which advertising initiatives within a firm perform best. In the meantime, medical research and research design determine how well a particular treatment does.
Thus, analytical research can help people achieve their goals while saving lives and money.
Methods of Conducting Analytical Research
Analytical research is the process of gathering, analyzing, and interpreting information to make inferences and reach conclusions. Depending on the purpose of the research and the data you have access to, you can conduct analytical research using a variety of methods. Here are a few typical approaches:
Quantitative research
Numerical data are gathered and analyzed using this method. Statistical methods are then used to analyze the information, which is often collected using surveys, experiments, or pre-existing datasets. Results from quantitative research can be measured, compared, and generalized numerically.
Qualitative research
In contrast to quantitative research, qualitative research focuses on collecting non-numerical information. It gathers detailed information using techniques like interviews, focus groups, observations, or content research. Understanding social phenomena, exploring experiences, and revealing underlying meanings and motivations are all goals of qualitative research.
Mixed methods research
This strategy combines quantitative and qualitative methodologies to grasp a research problem thoroughly. Mixed methods research often entails gathering and evaluating both numerical and non-numerical data, integrating the results, and offering a more comprehensive viewpoint on the research issue.
Experimental research
Experimental research is frequently employed in scientific trials and investigations to establish causal links between variables. This approach entails modifying variables in a controlled environment to identify cause-and-effect connections. Researchers randomly divide volunteers into several groups, provide various interventions or treatments, and track the results.
Observational research
With this approach, behaviors or occurrences are observed and methodically recorded without any outside interference or variable data manipulation . Both controlled surroundings and naturalistic settings can be used for observational research . It offers useful insights into behaviors that occur in the actual world and enables researchers to explore events as they naturally occur.
Case study research
This approach entails thorough research of a single case or a small group of related cases. Case-control studies frequently include a variety of information sources, including observations, records, and interviews. They offer rich, in-depth insights and are particularly helpful for researching complex phenomena in practical settings.
Secondary data analysis
Examining secondary information is time and money-efficient, enabling researchers to explore new research issues or confirm prior findings. With this approach, researchers examine previously gathered information for a different reason. Information from earlier cohort studies, accessible databases, or corporate documents may be included in this.
Content analysis
Content research is frequently employed in social sciences, media observational studies, and cross-sectional studies. This approach systematically examines the content of texts, including media, speeches, and written documents. Themes, patterns, or keywords are found and categorized by researchers to make inferences about the content.
Depending on your research objectives, the resources at your disposal, and the type of data you wish to analyze, selecting the most appropriate approach or combination of methodologies is crucial to conducting analytical research.
Examples of analytical research
Analytical research takes a unique measurement. Instead, you would consider the causes and changes to the trade imbalance. Detailed statistics and statistical checks help guarantee that the results are significant.
For example, it can look into why the value of the Japanese Yen has decreased. This is so that an analytical study can consider “how” and “why” questions.
Another example is that someone might conduct analytical research to identify a study’s gap. It presents a fresh perspective on your data. Therefore, it aids in supporting or refuting notions.
Descriptive vs analytical research
Here are the key differences between descriptive research and analytical research:
Aspect | Descriptive Research | Analytical Research |
Objective | Describe and document characteristics or phenomena. | Analyze and interpret data to understand relationships or causality. |
Focus | “What” questions | “Why” and “How” questions |
Data Analysis | Summarizing information | Statistical research, hypothesis testing, qualitative research |
Goal | Provide an accurate and comprehensive description | Gain insights, make inferences, provide explanations or predictions |
Causal Relationships | Not the primary focus | Examining underlying factors, causes, or effects |
Examples | Surveys, observations, case-control study, content analysis | Experiments, statistical research, qualitative analysis |
The study of cause and effect makes extensive use of analytical research. It benefits from numerous academic disciplines, including marketing, health, and psychology, because it offers more conclusive information for addressing research issues.
QuestionPro offers solutions for every issue and industry, making it more than just survey software. For handling data, we also have systems like our InsightsHub research library.
You may make crucial decisions quickly while using QuestionPro to understand your clients and other study subjects better. Make use of the possibilities of the enterprise-grade research suite right away!
LEARN MORE FREE TRIAL
MORE LIKE THIS
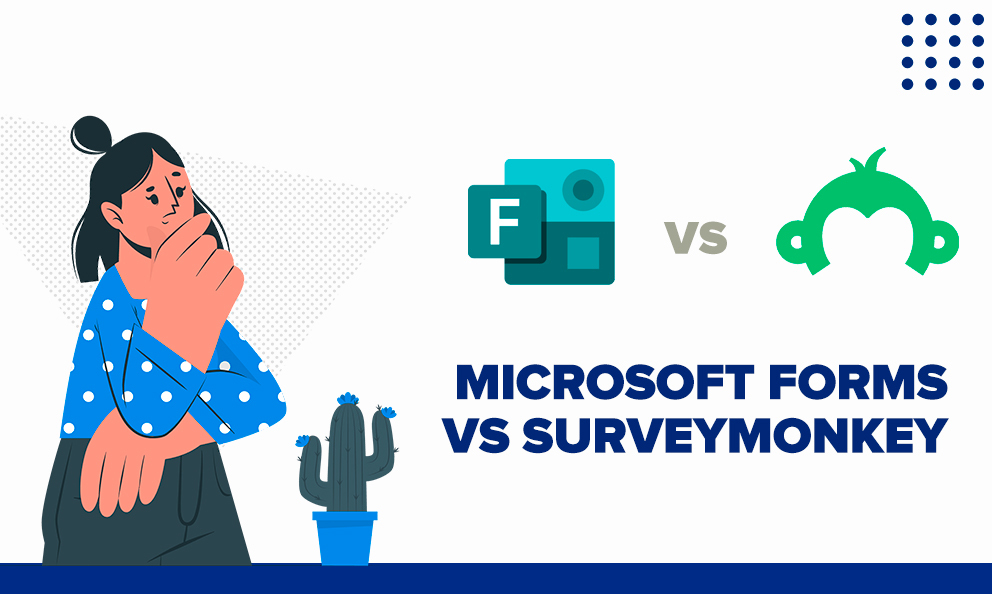
Microsoft Forms vs SurveyMonkey: Complete Analysis
Jul 29, 2024
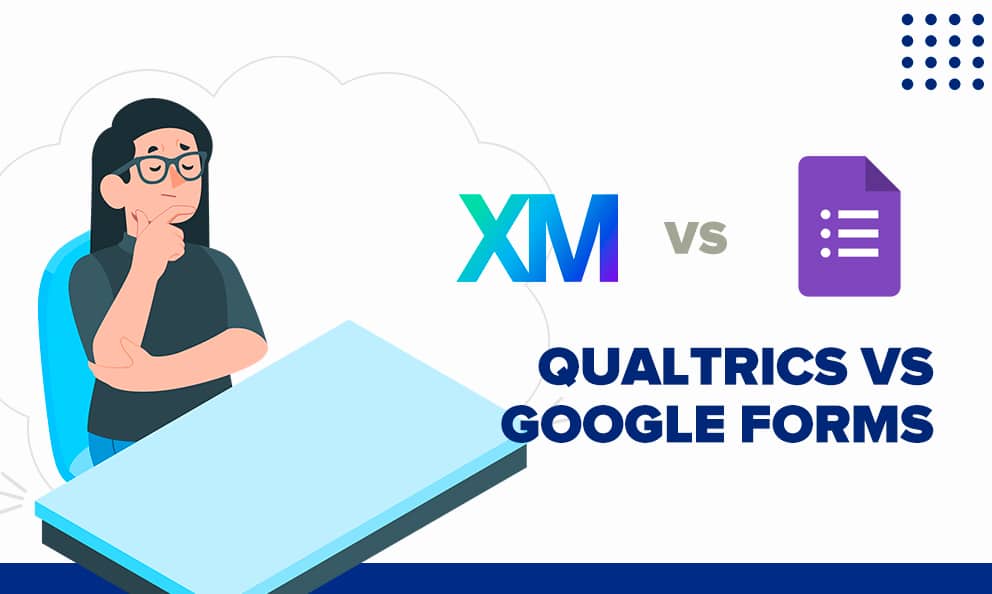
Qualtrics vs Google Forms: Which is the Best Platform?
Jul 24, 2024
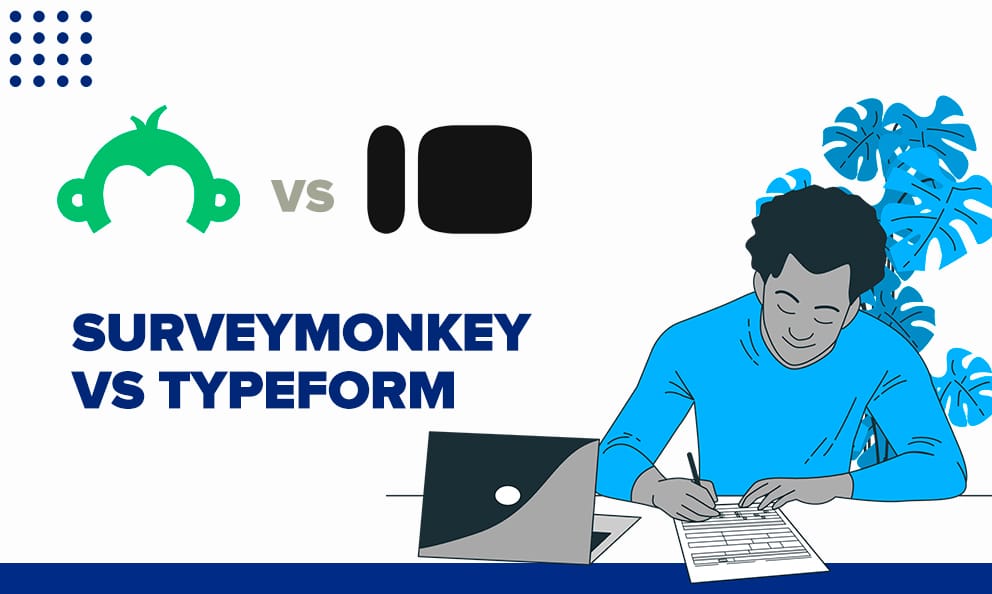
TypeForm vs. SurveyMonkey: Which is Better in 2024?
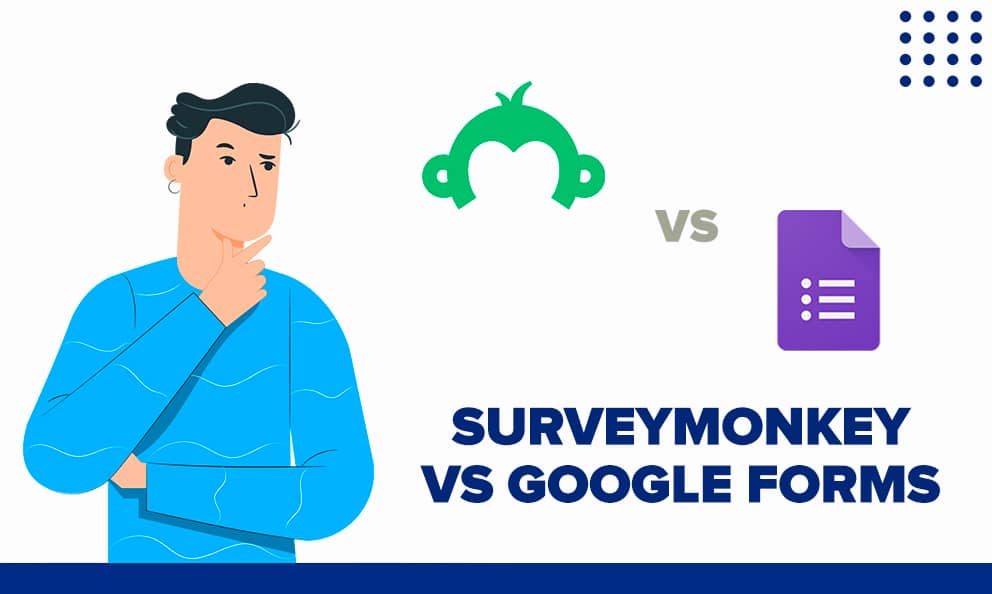
SurveyMonkey vs Google Forms: A Detailed Comparison
Jul 23, 2024
Other categories
- Academic Research
- Artificial Intelligence
- Assessments
- Brand Awareness
- Case Studies
- Communities
- Consumer Insights
- Customer effort score
- Customer Engagement
- Customer Experience
- Customer Loyalty
- Customer Research
- Customer Satisfaction
- Employee Benefits
- Employee Engagement
- Employee Retention
- Friday Five
- General Data Protection Regulation
- Insights Hub
- Life@QuestionPro
- Market Research
- Mobile diaries
- Mobile Surveys
- New Features
- Online Communities
- Question Types
- Questionnaire
- QuestionPro Products
- Release Notes
- Research Tools and Apps
- Revenue at Risk
- Survey Templates
- Training Tips
- Tuesday CX Thoughts (TCXT)
- Uncategorized
- What’s Coming Up
- Workforce Intelligence
A Step-by-Step Guide to Analytical Method Development and Validation
Method development and validation are essential components of drug development and chemistry manufacturing and controls (CMC). The goal of method development and validation is to ensure that the methods used to measure the identity, purity, potency, and stability of drugs are accurate, precise, and reliable. Analytical methods are critical tools for ensuring the quality, safety, and efficacy of pharmaceutical products in the drug development process. Analytical development services performed at Emery Pharma are outlined below.
Analytical Method Development Overview:
Analytical method development is the process of selecting and optimizing analytical methods to measure a specific attribute of a drug substance or drug product. This process involves a systematic approach to evaluating and selecting suitable methods that are sensitive, specific, and robust, and can be used to measure the target attribute within acceptable limits of accuracy and precision.
Method Validation Overview:
Method validation is the process of demonstrating that an analytical method is suitable for its intended use, and that it is capable of producing reliable and consistent results over time. The validation process involves a set of procedures and tests designed to evaluate the performance characteristics of the method.
Components of method validation include:
- Specificity
- Limit of detection (LOD)
- Limit of quantification (LOQ)
Depending on the attribute being assayed, we use state-of-the-art instrumentation such as HPLC (with UV-Vis/DAD, IR, CAD, etc. detectors), LC-MS, HRMS, MS/MS, GC-FID/MS, NMR, plate readers, etc.
At Emery Pharma, we follow a prescribed set of key steps per regulatory (FDA, EMA, etc.) guidance, as well as instructions from the International Council for Harmonisation of Technical Requirements for Pharmaceuticals for Human Use (ICH) for any analytical method development and validation.
Step 1: Define the Analytical Method Objectives
The first step in analytical method development and validation is to define the analytical method objectives, including the attribute to be measured, the acceptance criteria, and the intended use of the method. This step involves understanding the critical quality attributes (CQAs) of the drug product or drug substance and selecting appropriate analytical methods to measure them.
For example, impurity profile of a drug substance assessment require suitable HPLC-based methods for small molecules, whereas host cell proteins (impurity-equivalent) for a Biologic drug substance require ligand binding assays (LBAs) such as ELISA for overview, and LC-HRMS-based analysis for thorough understanding.
Step 2: Conduct a Literature Review
Next, a literature review is conducted to identify existing methods and establish a baseline for the method development process. This step involves reviewing scientific literature, regulatory guidance, and industry standards to determine the current state of the art and identify potential methods that may be suitable for the intended purpose.
At Emery Pharma, we have worked on and have existing programs on virtually all type of drug modalities, thus we have access to many validated internal methods to tap into as well.
Step 3: Develop a Method Plan
The next step is to develop a method plan that outlines the methodology, instrumentation, and experimental design for method development and validation. The plan includes the selection of suitable reference standards, the establishment of performance characteristics, and the development of protocols for analytical method validation.
Step 4: Optimize the Method
Next, the analytical method is optimized to ensure that it is sensitive, specific, and robust. This step involves evaluating various parameters, such as sample preparation, column selection, detector selection, mobile phase composition, and gradient conditions, to optimize the method performance.
Step 5: Validate the Method
The critical next step is to validate the analytical method to ensure that it meets the performance characteristics established in the method plan. This step involves evaluating the method's accuracy, precision, specificity, linearity, range, LOD, LOQ, ruggedness, and robustness.
Depending on the stage of development, validation may be performed under Research and Development (R&D), however, most Regulatory submissions require method validation be conducted per 21 CFR Part 58 on Good Laboratory Practices (GLP). To that end, Emery Pharma has an in-house Quality Assurance department that ensures compliance and can play host to regulators/auditors.
Step 6: (Optional) Transfer the Method
In some instances, e.g., clinical trials with multiple international sites, the validated method may need to be transferred to another qualified laboratory. We routinely help our Clients get several parallel sites up to speed on new validated methods, and support with training analysts on the method, documenting the method transfer process, and conducting ongoing monitoring and maintenance of the method.
Step 7: Sample Analysis
The final step of an analytical method development Validation process is developing a protocol and initiate sample analysis.
At Emery Pharma, depending on the stage of development, sample analysis is conducted under R&D or in compliance with 21 CFR Part 210 and 211 for current Good Manufacturing Procedures (cGMP). We boast an impressive array of qualified instrumentation that can be deployed for cGMP sample analysis, which is overseen by our Quality Assurance Director for compliance and proper reporting.
Let us be a part of your success story
Emery Pharma has decades of experience in analytical method development and validation. We strive to implement procedures that help to ensure new drugs are manufactured to the highest quality standards and are safe and effective for patient use.
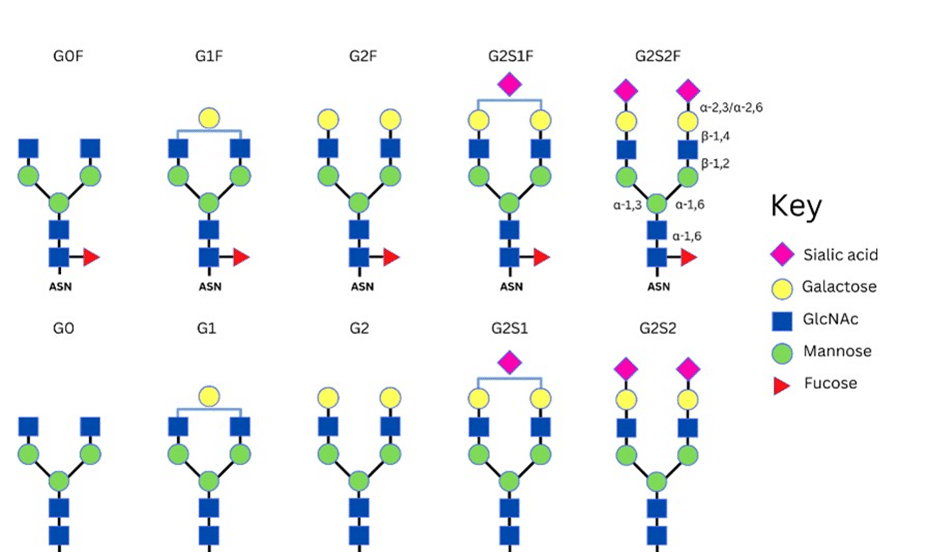
Emery Pharma
Request for proposal, let us be a part of your success story..
Do you have questions regarding a potential project? Or would you like to learn more about our services? Please reach out to a member of the Emery Pharma team via the contact form, and one of our experts will be in touch soon as possible. We look forward to working with you!
By continuing, you will be directed to a site intended only for residents of the United States and Canada. We are called MSD everywhere, except in the United States and Canada where we are known as Merck & Co., Inc., Rahway, NJ, USA.
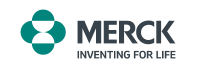
The link you have selected will take you to a third-party website, owned and operated by AgileOne. Our company does not control, and is not responsible for, the accuracy, content, practices, or standards of the pharmatempjobs.com website and/or AgileOne.
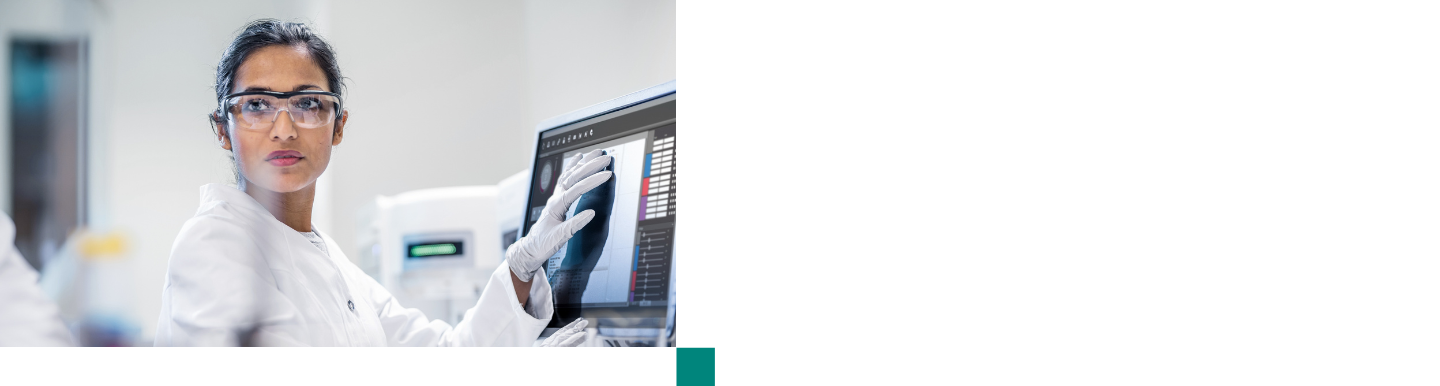
Analytical Research and Development (AR&D)
“leveraging diverse expertise in measurement science to be partners in problem solving”, worldwide operations, chemical characterization methods such as separation science, mass spectrometry, spectroscopy, and bioassays, structure-function analyses, biophysical and physical characterization and structure elucidation, cheminformatics and predictive sciences, automation, high throughput analytics, and predictive and digital tools, our scientists operate across eight sites globally, including key scientific innovation hub cities, and leverage collaborations with academic institutions, contract research organizations, and other pharmaceutical companies. five of these sites are located in the us., our organization, what we do, how we work, our commitment to talent development, lorem ipsum, join our talent community today..
Receive updates on career opportunities tailored to you.
Connect with us on social
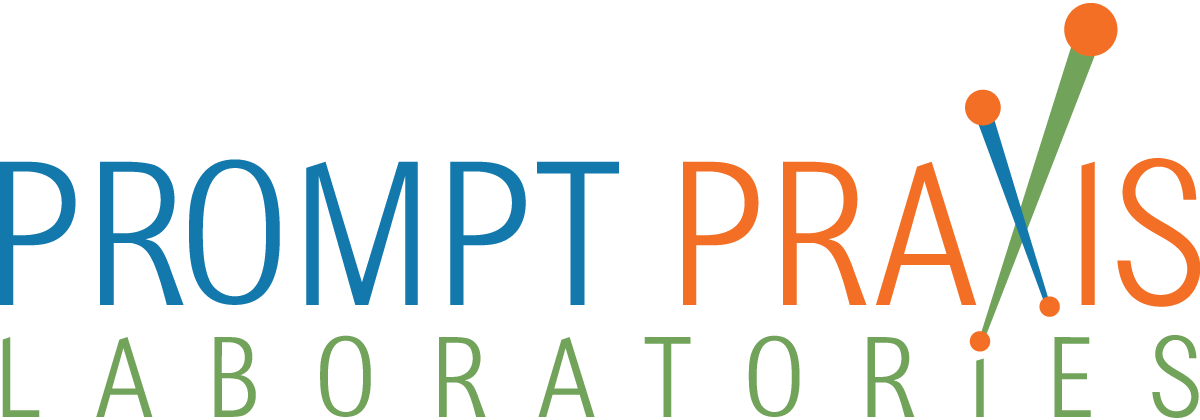
Raise Your Laboratory Testing Benchmark!
- October 29, 2022
- / Analytical Testing Lab Resources , Analytical Testing Lab Services
What Is Analytical Method Development And Validation?
Table of contents.
Analytical Method Development and Validation (AMV) involves the process of creating and testing new analytical techniques to accurately measure components of products.
This method development can include both fundamental research and applying existing theories to predict unknowns. Once a method has been created, it must then be validated to ensure that it produces consistent results when compared against recognized standards.
This article will focus on the validation of newly developed methods, not revalidation of those already in use.
The process of revalidation is when another test is conducted after changing component parts to make sure that the same conclusions are still drawn from the data collected. Different types of validation exist depending on what part of the analyte being measured is being tested, but all validations must demonstrate accurate results in order for them to be accepted and used.
Steps of analytical method development
The first step in developing an analytic technique is to determine if the current methods are adequate or sufficient. If they are, then your task is done!
You have already started the process by defining what you want to measure and determining how to go about measuring it. The next steps should be familiar to you as a scientist — experimentation, theory, and application.
Experimentation means putting your new methodology to use by testing it on samples that you have already analyzed using other methods. This way you can compare your results with those of the past techniques without investing more money in equipment and reagents.
Theory refers to mathematical equations used to predict the result of experiments. While not always needed, there are times when theoretical calculations help make sense of experimental data.
Application comes last because no matter how well designed and rigorous your new analysis technique is, it will not prove its quality unless you apply it to something real.
Analytical method development does not necessarily mean creating and adapting a brand-new technique, but rather improving upon existing ones . By doing this, you shift the focus away from reinventing the wheel and onto making things better for future researchers.
Laboratory equipment needed
The first step in developing an analytical method is to evaluate whether the current methods are adequate for your research or not. If they are, then you can simply keep using them!
Many times however, researchers will need to make some changes to these already established protocols to make them more effective or improve the accuracy of the analysis. This is where method development comes into play!
By defining what steps should be done during method development, we as scientists get rid of poor quality laboratory practices that may have worked well back when the method was created, but no longer.
There are several different types of method development, such as changing reagents, altering reaction conditions, or even changing how the sample is treated once it’s got there. All of these affect the way the chemical being analyzed reacts with other chemicals, and this impacts the outcome of the test.
So, how do you know if something is working? You could try running a few samples by the new protocol to see if it works better than before, but that would require taking extra precautions to ensure that the results remain consistent.
Alternatively, you could run a lot of samples at one time under controlled settings to determine if the altered protocol produces similar , if not better, results. Both of those strategies are examples of method validation.
Sample preparation
Sample preparation is one of the most important steps in any analysis you perform. If done improperly, there can be disastrous consequences for your test results.
Most analytical tests require samples of blood or other fluids, tissue pieces, or substances such as urine or feces to determine what chemicals are present in the experiment. These samples must be properly collected, stored, and processed to ensure accurate results.
Proper sample collection includes using correct needles or lancets to get the right amount of fluid, storing the sample correctly, and processing the sample correctly. All these steps depend on well-established protocols that work for your specific testing method!
Analysts who perform chemical analyses often have specialised equipment and reagents available to them. They may also develop their own internal procedures or use “standard” methods published by national or international laboratories with certified experts overseeing everything .
When performing an analysis on a new specimen, it is very helpful to run some preliminary experiments to see if the protocol works and whether the required controls are included.
Analytical instrument
An analytical instrument is any device used to analyze samples for chemical compounds or elements. This could be something as simple as a test tube with reagents, or more advanced devices such as gas chromatography (GC) machines or mass spectrometers (MS).
Many of these instruments require an initial step of method development before you can use them. This means figuring out what part of the sample you want to run in the machine, what reagent(s) needed to be added, and how much of each reagent should be given to the sample.
Once this has been done, the analyst runs the experiment! The experimentalist takes their prepared sample and places it into the analyzer. They then start the equipment and wait for results.
The experimentalist also needs to make sure that they have enough of each reagent until the analysis is complete so that the right amount of chemicals are in the solution when the sample enters the machine.
Method validation
Beyond determining if a method works, or is working for your lab tests, you will want to evaluate how well it performs its function. This is referred to as method verification or validation.
Methods that have been verified are good tools for your business, but there are many ways to do this! There are different types of methods validation, with some being more important than others.
Generalized accuracy, precision, and/or specificity testing are usually the first steps in most method validations. These tests determine whether the method is performing its job correctly by comparing results to a known “truth” or benchmark.
By doing this before using the test result too seriously, you can avoid wasting time and resources proving something that has already proven itself to work.
Testing the same sample twice within an hour or day is not enough- you must also test the same sample at multiple concentrations and compare those to see what the normal range is. Generalizing from these samples is then used to calculate what other samples should be.
When calculating cutoffs or normal ranges, you must use the same statistical techniques that you would use in research studies- such as percentiles or z scores.
Factors to consider during method validation
During analytical method development, you will need to determine what factors must be considered for successful analysis. These include instrumentation, reagents, sample preparation, temperature, time, etc.
Instrumentation is the part of the test that produces a signal used to identify the presence or absence of an analyte in your samples. There are many different types of instruments including spectrophotometers, fluorimeters, mass spectrometers, and others. Each one has its benefits and drawbacks so it is important to know which ones work best with your particular analytes.
A common source of error when using an instrument is called carryover. This happens when leftover chemicals from a previous run remain in the system and affect the accuracy of the next measurement. Instruments can have special precautions against this, such as having separate waste containers for each chemical being used.
Using appropriate equipment is an integral part of ensuring accurate results throughout every stage of a testing process. Different manufacturers’ versions may differ slightly, but they all follow similar protocols.
Sample storage
The next major factor in analytical method development is sample preparation or pre-analytics. This includes extracting chemicals from the tissue, breaking down large molecules into smaller ones, and/or purifying the analytes of interest.
Sample preparation can be very specific depending on what type of chemical you want to analyze. For example, if you wanted to determine how much calcium is in a given amount of milk, then you would need to extract it first!
There are many different ways to do this, so no matter which way you choose, just make sure that you do not break down the samples too much as some substances take longer to process than others.
Some examples of sample preparation include hydrolysis (the splitting up of larger molecules) for sugar analysis, drying the specimen before weighing, adding reagents to the specimens, etc. Depending on the analyte, adequate specificity and sensitivity cannot be guaranteed without appropriate pretreatment of the sample.
Quality control
This is perhaps one of the most important steps in any analytical method development or validation. It can be referred to as quality control, precision testing, accuracy testing, verification, etc. The key here is defining what level of analysis this fits under. If you want to test how well your new technique works on a few samples that already have an established method, then this is defined as accuracy testing. Testing whether your modified procedure produces the same results as the existing methods are definitions for precision testing.
If you want to make sure your new way of analyzing something is correct, then quality control is the best term to use. Your final determination will depend on which phase of analytical method development you are working on at the time.
Quality control is typically done after completion of each step in the process, but it can also be done during some stages. For example, if you were performing ICP-MS analysis, you would check to see if there was enough material left over from the previous stage to continue with the next one.
Share This!
Recently Added
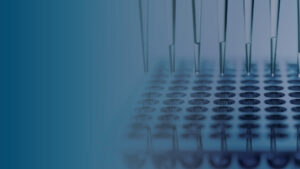
Compounding Pharmacy Compliance 2024, June 18-19, in Boston, MA
Prompt Praxis Labs is sponsoring and attending the Compounding Pharmacy Compliance 2024, June 18-19, in Boston, MA.
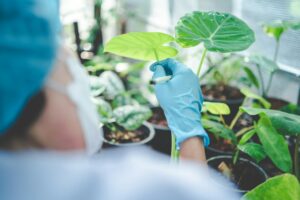
Drug Diversion Testing: What It Is and How It Works
Drug diversion testing is a crucial aspect of healthcare that ensures patient safety and the proper use of controlled substances. Drug diversion refers to the illegal transfer of prescription drugs from the legal supply chain to the illegal market.
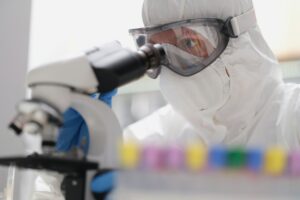
Exploring the Process of Sterility Testing in Pharmaceuticals
When it comes to pharmaceuticals, ensuring quality and safety is of paramount importance. One crucial aspect of this process is sterility testing. In this article, we will dive into the intricate details of sterility testing and explore its significance in the pharmaceutical industry.
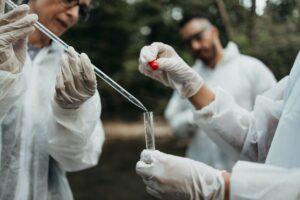
The Importance of Regular Quality Control Product Testing: How Often is Ideal?
Regular quality control product testing is a critical aspect of ensuring the overall quality and reliability of products. From electronics to pharmaceuticals, conducting frequent testing helps identify any potential issues or defects early on, preventing costly consequences down the line. But how often should companies perform these tests to strike the right balance between efficiency and effectiveness?
We are digitized & streamlined.
Creating unrivaled capacity for timely results.
Prompt Praxis Laboratories, analytical testing labs, provide a full range of rapid testing and support services focusing on pharmaceutical products and compounded sterile or nonsterile preparations.
Schedule a Consultation
Complete the form below, and we will be in touch shortly.
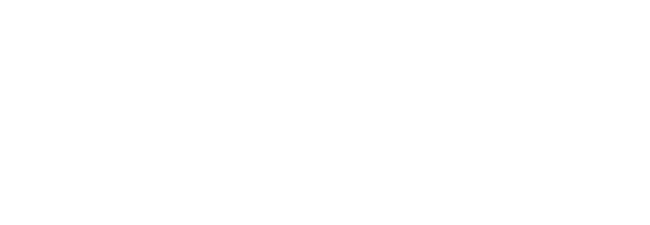
Prompt Praxis Laboratories, LLC, founded in 2011, is a women-owned fully-integrated analytical and microbiological testing lab powered with the current information technology to facilitate rapid drug development.
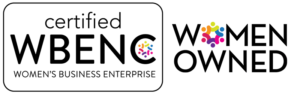
- About Prompt Praxis Labs
- Analytical Testing Capabilities
- Recent Events
- Laboratory Services
- Ready-to-Analyze®Test Methods
- Management Team
- Contact Prompt Praxis Labs
- Ready-to-Analyze®Test Methods, A – L
- Department Teams
- Microbiological Testing Capabilities
FDA & DEA Registrations
FDA Establishment Identifier: 3009381492
GDUFA Self-Identified
Illinois DEA: (Analytical Laboratory, Schedules II-V) 097.001930
Federal DEA: (Analytical Laboratory, Schedules II-V) RM0458097
847-859-3850
50 Lakeview Parkway, Suite 123 Vernon Hills, IL 60061-1590
150 N Fairway Drive, Suites 148-154 Vernon Hills, IL 60061-1860
Employee Portal
What are Analytical Study Designs?
- Research Process
- Peer Review
Analytical study designs can be experimental or observational and each type has its own features. In this article, you'll learn the main types of designs and how to figure out which one you'll need for your study.
Updated on September 19, 2022

A study design is critical to your research study because it determines exactly how you will collect and analyze your data. If your study aims to study the relationship between two variables, then an analytical study design is the right choice.
But how do you know which type of analytical study design is best for your specific research question? It's necessary to have a clear plan before you begin data collection. Lots of researchers, sadly, speed through this or don't do it at all.
When are analytical study designs used?
A study design is a systematic plan, developed so you can carry out your research study effectively and efficiently. Having a design is important because it will determine the right methodologies for your study. Using the right study design makes your results more credible, valid, and coherent.
Descriptive vs. analytical studies
Study designs can be broadly divided into either descriptive or analytical.
Descriptive studies describe characteristics such as patterns or trends. They answer the questions of what, who, where, and when, and they generate hypotheses. They include case reports and qualitative studies.
Analytical study designs quantify a relationship between different variables. They answer the questions of why and how. They're used to test hypotheses and make predictions.
Experimental and observational
Analytical study designs can be either experimental or observational. In experimental studies, researchers manipulate something in a population of interest and examine its effects. These designs are used to establish a causal link between two variables.
In observational studies, in contrast, researchers observe the effects of a treatment or intervention without manipulating anything. Observational studies are most often used to study larger patterns over longer periods.
Experimental study designs
Experimental study designs are when a researcher introduces a change in one group and not in another. Typically, these are used when researchers are interested in the effects of this change on some outcome. It's important to try to ensure that both groups are equivalent at baseline to make sure that any differences that arise are from any introduced change.
In one study, Reiner and colleagues studied the effects of a mindfulness intervention on pain perception . The researchers randomly assigned participants into an experimental group that received a mindfulness training program for two weeks. The rest of the participants were placed in a control group that did not receive the intervention.
Experimental studies help us establish causality. This is critical in science because we want to know whether one variable leads to a change, or causes another. Establishing causality leads to higher internal validity and makes results reproducible.
Experimental designs include randomized control trials (RCTs), nonrandomized control trials (non-RCTs), and crossover designs. Read on to learn the differences.
Randomized control trials
In an RCT, one group of individuals receives an intervention or a treatment, while another does not. It's then possible to investigate what happens to the participants in each group.
Another important feature of RCTs is that participants are randomly assigned to study groups. This helps to limit certain biases and retain better control. Randomization also lets researchers pinpoint any differences in outcomes to the intervention received during the trial. RTCs are considered the gold standard in biomedical research and are considered to provide the best kind of evidence.
For example, one RCT looked at whether an exercise intervention impacts depression . Researchers randomly placed patients with depressive symptoms into intervention groups containing different types of exercise (i.e., light, moderate, or strong). Another group received usual medications or no exercise interventions.
Results showed that after the 12-week trial, patients in all exercise groups had decreased depression levels compared to the control group. This means that by using an RCT design, researchers can now safely assume that the exercise variable has a positive impact on depression.
However, RCTs are not without drawbacks. In the example above, we don't know if exercise still has a positive impact on depression in the long term. This is because it's not feasible to keep people under these controlled settings for a long time.
Advantages of RCTs
- It is possible to infer causality
- Everything is properly controlled, so very little is left to chance or bias
- Can be certain that any difference is coming from the intervention
Disadvantages of RCTs
- Expensive and can be time-consuming
- Can take years for results to be available
- Cannot be done for certain types of questions due to ethical reasons, such as asking participants to undergo harmful treatment
- Limited in how many participants researchers can adequately manage in one study or trial
- Not feasible for people to live under controlled conditions for a long time
Nonrandomized controlled trials
Nonrandomized controlled trials are a type of nonrandomized controlled studies (NRS) where the allocation of participants to intervention groups is not done randomly . Here, researchers purposely assign some participants to one group and others to another group based on certain features. Alternatively, participants can sometimes also decide which group they want to be in.
For example, in one study, clinicians were interested in the impact of stroke recovery after being in an enriched versus non-enriched hospital environment . Patients were selected for the trial if they fulfilled certain requirements common to stroke recovery. Then, the intervention group was given access to an enriched environment (i.e. internet access, reading, going outside), and another group was not. Results showed that the enriched group performed better on cognitive tasks.
NRS are useful in medical research because they help study phenomena that would be difficult to measure with an RCT. However, one of their major drawbacks is that we cannot be sure if the intervention leads to the outcome. In the above example, we can't say for certain whether those patients improved after stroke because they were in the enriched environment or whether there were other variables at play.
Advantages of NRS's
- Good option when randomized control trials are not feasible
- More flexible than RCTs
Disadvantages of NRS's
- Can't be sure if the groups have underlying differences
- Introduces risk of bias and confounds
Crossover study
In a crossover design, each participant receives a sequence of different treatments. Crossover designs can be applied to RCTs, in which each participant is randomly assigned to different study groups.
For example, one study looked at the effects of replacing butter with margarine on lipoproteins levels in individuals with cholesterol . Patients were randomly assigned to a 6-week butter diet, followed by a 6-week margarine diet. In between both diets, participants ate a normal diet for 5 weeks.
These designs are helpful because they reduce bias. In the example above, each participant completed both interventions, making them serve as their own control. However, we don't know if eating butter or margarine first leads to certain results in some subjects.
Advantages of crossover studies
- Each participant serves as their own control, reducing confounding variables
- Require fewer participants, so they have better statistical power
Disadvantages of crossover studies
- Susceptible to order effects, meaning the order in which a treatment was given may have an effect
- Carry-over effects between treatments
Observational studies
In observational studies, researchers watch (observe) the effects of a treatment or intervention without trying to change anything in the population. Observational studies help us establish broad trends and patterns in large-scale datasets or populations. They are also a great alternative when an experimental study is not an option.
Unlike experimental research, observational studies do not help us establish causality. This is because researchers do not actively control any variables. Rather, they investigate statistical relationships between them. Often this is done using a correlational approach.
For example, researchers would like to examine the effects of daily fiber intake on bone density . They conduct a large-scale survey of thousands of individuals to examine correlations of fiber intake with different health measures.
The main observational studies are case-control, cohort, and cross-sectional. Let's take a closer look at each one below.
Case-control study
A case-control is a type of observational design in which researchers identify individuals with an existing health situation (cases) and a similar group without the health issue (controls). The cases and the controls are then compared based on some measurements.
Frequently, data collection in a case-control study is retroactive (i.e., backwards in time). This is because participants have already been exposed to the event in question. Additionally, researchers must go through records and patient files to obtain the records for this study design.
For example, a group of researchers examined whether using sleeping pills puts people at risk of Alzheimer's disease . They selected 1976 individuals that received a dementia diagnosis (“cases”) with 7184 other individuals (“controls”). Cases and controls were matched on specific measures such as sex and age. Patient data was consulted to find out how much sleeping pills were consumed over the course of a certain time.
Case-control is ideal for situations where cases are easy to pick out and compare. For instance, in studying rare diseases or outbreaks.
Advantages of case-control studies
- Feasible for rare diseases
- Cheaper and easier to do than an RCT
Disadvantages of case-control studies
- Relies on patient records, which could be lost or damaged
- Potential recall and selection bias
Cohort study (longitudinal)
A cohort is a group of people who are linked in some way. For instance, a birth year cohort is all people born in a specific year. In cohort studies, researchers compare what happens to individuals in the cohort that have been exposed to some variable compared with those that haven't on different variables. They're also called longitudinal studies.
The cohort is then repeatedly assessed on variables of interest over a period of time. There is no set amount of time required for cohort studies. They can range from a few weeks to many years.
Cohort studies can be prospective. In this case, individuals are followed for some time into the future. They can also be retrospective, where data is collected on a cohort from records.
One of the longest cohort studies today is The Harvard Study of Adult Development . This cohort study has been tracking various health outcomes of 268 Harvard graduates and 456 poor individuals in Boston from 1939 to 2014. Physical screenings, blood samples, brain scans and surveys were collected on this cohort for over 70 years. This study has produced a wealth of knowledge on outcomes throughout life.
A cohort study design is a good option when you have a specific group of people you want to study over time. However, a major drawback is that they take a long time and lack control.
Advantages of cohort studies
- Ethically safe
- Allows you to study multiple outcome variables
- Establish trends and patterns
Disadvantages of cohort studies
- Time consuming and expensive
- Can take many years for results to be revealed
- Too many variables to manage
- Depending on length of study, can have many changes in research personnel
Cross-sectional study
Cross-sectional studies are also known as prevalence studies. They look at the relationship of specific variables in a population in one given time. In cross-sectional studies, the researcher does not try to manipulate any of the variables, just study them using statistical analyses. Cross-sectional studies are also called snapshots of a certain variable or time.
For example, researchers wanted to determine the prevalence of inappropriate antibiotic use to study the growing concern about antibiotic resistance. Participants completed a self-administered questionnaire assessing their knowledge and attitude toward antibiotic use. Then, researchers performed statistical analyses on their responses to determine the relationship between the variables.
Cross-sectional study designs are ideal when gathering initial data on a research question. This data can then be analyzed again later. By knowing the public's general attitudes towards antibiotics, this information can then be relayed to physicians or public health authorities. However, it's often difficult to determine how long these results stay true for.
Advantages of cross-sectional studies
- Fast and inexpensive
- Provides a great deal of information for a given time point
- Leaves room for secondary analysis
Disadvantages of cross-sectional studies
- Requires a large sample to be accurate
- Not clear how long results remain true for
- Do not provide information on causality
- Cannot be used to establish long-term trends because data is only for a given time
So, how about your next study?
Whether it's an RCT, a case-control, or even a qualitative study, AJE has services to help you at every step of the publication process. Get expert guidance and publish your work for the world to see.

The AJE Team
See our "Privacy Policy"
- Expert Interviews
- Press Releases
- The Road To Series
- Market Research
- Thought Leaders
- Small Molecules
- Advanced Therapies
- Manufacturing
- Supply Chain
- Clinical Trials
- Technologies

Analytical Method Development: Key to Process Development
Increasing cost pressures and the move toward value- or evidence-based medicine is placing pressure on pharmaceutical companies to be first to market with novel drugs manufactured via highly efficient and low-cost production methods. accelerating process development efforts is a notable consequence. collaborative interactions with an advanced analytical method development team, with the freedom to explore novel solutions, can facilitate both process optimization activities and technology transfer, from the development lab to the gmp lab and manufacturing floor. , importance of analytical development activities.
Throughout the entire drug development lifecycle, efficient and cost-effective routes to candidate drug substances with high potential for regulatory approval are established. This work involves investigation of the impact of numerous parameters on process performance (yield, selectivity, etc.) and product quality. Timely access to accurate analytical information is fundamental to completing process development projects effectively and efficiently. As a result, methods are continually evaluated and optimized as needed through all stages of the drug development lifecycle for the purposes of product characterization, or to be qualified and validated as release assays.
Analytical data on product quality and impurity identities and properties are needed to gain the detailed process knowledge necessary for determining additional parameters needed to investigate and ultimately optimize the conditions for production of the drug substance at commercial scale according to quality specifications. The data can also be used to develop predictive models of processes to facilitate scale-up and technology transfer. Consequently, both the timeliness and appropriateness of the data can have a direct impact on the efficiency of the drug development process.
Collaboration Is Crucial
To be most effective, analytical method development must not occur in isolation. Extensive interactions between analytical scientists and process development scientists and engineers are necessary to ensure that analytical methods address the specific needs of the process development group. In addition, constant, two-way exchange of data and ongoing communication on the information needs of the process development group is essential for the effective development of fit-for-purpose methods that can accelerate process development efforts.
As a CDMO that operates as an independent group within a large pharmaceutical company, GlaxoSmithKline Biopharmaceuticals offers many advantages to manu-facturers looking for a strong, stable partner with extensive experience in biologics development and commercialization and access to expansive resources, including a wide range of analytical capabilities and in-depth regulatory expertise. The Biopharmaceutical Technology — Analytical (BPT-A) group within GSK Biopharmaceuticals is a separate, additional analytical unit focused on method development and supporting process development efforts. The BPT-A group, which works in conjunction with the process development team, was formed to allow collaboration between analytical scientists and process development scientists at all stages as a means for accelerating process development efforts.
Members of the BPT-A group and process development teams work directly with one another and constantly exchange data regarding the process and analytical results. Having both teams at the same location allows for significantly reduced turnaround times and facilitates process optimization. The BPT-A group also serves as the primary analytical interface for all GSK customers.
Because the BPT-A group also works with the Quality Control (QC) department, the team has an extensive understanding of the testing required for both process development activities and commercial manufacturing, which provides a significant advantage when transitioning processes.
Need For Flexibility
Supporting process development activities requires a higher level of flexibility and freedom to explore new and unconventional analytical methods than typically observed in a traditional quality control laboratory.
While the BPT-A lab at GSK Biopharmaceuticals conducts assay development, qualification, and validation work, and supports routine development testing, the team also develops a wide variety of assays / methods designed specifically to support process development activities, including optimization of processes and characterization of both products and impurities / byproducts. Method development can involve optimization of existing, but insufficient, assays or the establishment of an entirely new method when new processes are brought into the process development lab. Methods may also be modified or alternatives developed to allow the identification and characterization of new species that are generated in a process that is undergoing evaluation.
In the latter case, method development can be quite challenging, because oftentimes there are only very subtle differences in the impurities produced as a result of minor changes in process conditions. Typically, separations of biologic compounds are achieved based on size or charge heterogeneity, and often the species that need to be identified are aggregates or proteins in a denatured state. Detection and identification of such species are crucial because they can have an impact on the efficacy and safety of formulated biopharmaceuticals.
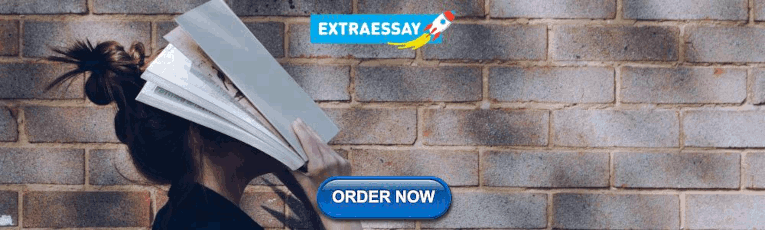
Many Different Process Development Solutions
For instance, in one process development project, a new species was formed during attempts to optimize the process conditions. The species could not be separated using the existing method because it was very similar to another previously identified impurity. The lab determined that the new species was an aggregate with the same molecular weight as the known impurity but with a slightly different size radius. A new method was developed that separated the two dimers so that additional optimization studies could be conducted and process conditions identified that would avoid / minimize the generation of the new dimer.
In another example, the BPT-A team replaced an existing validated ion-exchange release method. Although inconsistency in column performance was the original reason for method redevelopment, the method was further optimized for improved detection of lysine variants on the terminus of the antibody. The improved method allowed better collaboration with the process development group to perform a full characterization of the species in order to conduct a comprehensive investigation of the process. The process development team ultimately used this method to investigate the impact of raw material choices on the levels of lysine variants generated in the process.
Beyond Process Development Support
The flexibility of the BPT-A team at GSK Biopharmaceuticals, and its interaction with the production plant and traditional quality control group, has helped position these analytical scientists to address method development issues for more than just process development activities.
In one case, silicone was detected in a product after its container was changed. The original release size exclusion method was inadequate because the silicone peak interfered with the detection of protein-related impurities. To overcome the problem, a method was developed that bound the silicone to the chromatography column while the protein was allowed to pass through and be analyzed. Additionally, a new method for determination of the protein concentration was developed because the presence of the silicone interfered with the existing spectrometric assay. The alternative method used a reversed-phase method in combination with a calibration curve of known protein concentrations.
To be most effective, analytical method development must not occur in isolation.
When analytical methods for manufacturing processes need to be improved, the BPT-A team often works in conjunction with the manufacturing team or the QC department. There was a need to replace the cuvette-based traditional A 280 measurement with the SoloVPE method. The SoloVPE system utilizes a variable path length and sample absorbance at 280 nm to determine protein concentrations based on the Slope Spectroscopy ® technique, which eliminates the need for a dilution step thereby avoiding the potential for human error and variation. The BPT-A team helped perform the method validation to introduce the method to the plant.
Finally, the BPT-A group is involved in method development work that must be completed in response to new regulatory requirements. When regulatory agencies identify minor chemical modifications that have the potential to appear in a specific type of biologic drug, the agency often requires the implementation of analytical methods that can detect these species. As in the case with process optimization of processes brought into GSK Biologics, in some cases, the existing method can be modified, and in others an entirely new method must be developed.
Accelerating process development activities is crucial for remaining competitive in the pharmaceutical marketplace today and requires strong collaboration with analytical method development efforts. As a contract development and manufacturing organization (CDMO) dedicated to providing advanced process development capabilities, GSK Biopharmaceuticals has established a highly experienced analytical team focused on supporting process development with the specific goal of enabling the rapid scale-up and tech transfer of biopharma partner processes.
Manager, Biopharmaceutical Technology-Analytical
GSK Contract Manufacturing
Investigator, Biopharmaceutical Technology - Analytical

An official website of the United States government
The .gov means it’s official. Federal government websites often end in .gov or .mil. Before sharing sensitive information, make sure you’re on a federal government site.
The site is secure. The https:// ensures that you are connecting to the official website and that any information you provide is encrypted and transmitted securely.
- Publications
- Account settings
Preview improvements coming to the PMC website in October 2024. Learn More or Try it out now .
- Advanced Search
- Journal List
- BMJ Open Access

Analytical studies: a framework for quality improvement design and analysis
Conducting studies for learning is fundamental to improvement. Deming emphasised that the reason for conducting a study is to provide a basis for action on the system of interest. He classified studies into two types depending on the intended target for action. An enumerative study is one in which action will be taken on the universe that was studied. An analytical study is one in which action will be taken on a cause system to improve the future performance of the system of interest. The aim of an enumerative study is estimation, while an analytical study focuses on prediction. Because of the temporal nature of improvement, the theory and methods for analytical studies are a critical component of the science of improvement.
Introduction: enumerative and analytical studies
Designing studies that make it possible to learn from experience and take action to improve future performance is an essential element of quality improvement. These studies use the now traditional theory established through the work of Fisher, 1 Cox, 2 Campbell and Stanley, 3 and others that is widely used in biomedicine research. These designs are used to discover new phenomena that lead to hypothesis generation, and to explore causal mechanisms, 4 as well as to evaluate efficacy and effectiveness. They include observational, retrospective, prospective, pre-experimental, quasiexperimental, blocking, factorial and time-series designs.
In addition to these classifications of studies, Deming 5 defined a distinction between analytical and enumerative studies which has proven to be fundamental to the science of improvement. Deming based his insight on the distinction between these two approaches that Walter Shewhart had made in 1939 as he helped develop measurement strategies for the then-emerging science of ‘quality control.’ 6 The difference between the two concepts lies in the extrapolation of the results that is intended, and in the target for action based on the inferences that are drawn.
A useful way to appreciate that difference is to contrast the inferences that can be made about the water sampled from two different natural sources ( figure 1 ). The enumerative approach is like the study of water from a pond. Because conditions in the bounded universe of the pond are essentially static over time, analyses of random samples taken from the pond at a given time can be used to estimate the makeup of the entire pond. Statistical methods, such as hypothesis testing and CIs, can be used to make decisions and define the precision of the estimates.

Environment in enumerative and analytical study. Internal validity diagram from Fletcher et al . 7
The analytical approach, in contrast, is like the study of water from a river. The river is constantly moving, and its physical properties are changing (eg, due to snow melt, changes in rainfall, dumping of pollutants). The properties of water in a sample from the river at any given time may not describe the river after the samples are taken and analysed. In fact, without repeated sampling over time, it is difficult to make predictions about water quality, since the river will not be the same river in the future as it was at the time of the sampling.
Deming first discussed these concepts in a 1942 paper, 8 as well as in his 1950 textbook, 9 and in a 1975 paper used the enumerative/analytical terminology to characterise specific study designs. 5 While most books on experimental design describe methods for the design and analysis of enumerative studies, Moen et al 10 describe methods for designing and learning from analytical studies. These methods are graphical and focus on prediction of future performance. The concept of analytical studies became a key element in Deming's ‘system of profound knowledge’ that serves as the intellectual foundation for improvement science. 11 The knowledge framework for the science of improvement, which combines elements of psychology, the Shewhart view of variation, the concept of systems, and the theory of knowledge, informs a number of key principles for the design and analysis of improvement studies:
- Knowledge about improvement begins and ends in experimental data but does not end in the data in which it begins.
- Observations, by themselves, do not constitute knowledge.
- Prediction requires theory regarding mechanisms of change and understanding of context.
- Random sampling from a population or universe (assumed by most statistical methods) is not possible when the population of interest is in the future.
- The conditions during studies for improvement will be different from the conditions under which the results will be used. The major source of uncertainty concerning their use is the difficulty of extrapolating study results to different contexts and under different conditions in the future.
- The wider the range of conditions included in an improvement study, the greater the degree of belief in the validity and generalisation of the conclusions.
The classification of studies into enumerative and analytical categories depends on the intended target for action as the result of the study:
- Enumerative studies assume that when actions are taken as the result of a study, they will be taken on the material in the study population or ‘frame’ that was sampled.
More specifically, the study universe in an enumerative study is the bounded group of items (eg, patients, clinics, providers, etc) possessing certain properties of interest. The universe is defined by a frame, a list of identifiable, tangible units that may be sampled and studied. Random selection methods are assumed in the statistical methods used for estimation, decision-making and drawing inferences in enumerative studies. Their aim is estimation about some aspect of the frame (such as a description, comparison or the existence of a cause–effect relationship) and the resulting actions taken on this particular frame. One feature of an enumerative study is that a 100% sample of the frame provides the complete answer to the questions posed by the study (given the methods of investigation and measurement). Statistical methods such as hypothesis tests, CIs and probability statements are appropriate to analyse and report data from enumerative studies. Estimating the infection rate in an intensive care unit for the last month is an example of a simple enumerative study.
- Analytical studies assume that the actions taken as a result of the study will be on the process or causal system that produced the frame studied, rather than the initial frame itself. The aim is to improve future performance.
In contrast to enumerative studies, an analytical study accepts as a given that when actions are taken on a system based on the results of a study, the conditions in that system will inevitably have changed. The aim of an analytical study is to enable prediction about how a change in a system will affect that system's future performance, or prediction as to which plans or strategies for future action on the system will be superior. For example, the task may be to choose among several different treatments for future patients, methods of collecting information or procedures for cleaning an operating room. Because the population of interest is open and continually shifts over time, random samples from that population cannot be obtained in analytical studies, and traditional statistical methods are therefore not useful. Rather, graphical methods of analysis and summary of the repeated samples reveal the trajectory of system behaviour over time, making it possible to predict future behaviour. Use of a Shewhart control chart to monitor and create learning to reduce infection rates in an intensive care unit is an example of a simple analytical study.
The following scenarios give examples to clarify the nature of these two types of studies.
Scenario 1: enumerative study—observation
To estimate how many days it takes new patients to see all primary care physicians contracted with a health plan, a researcher selected a random sample of 150 such physicians from the current active list and called each of their offices to schedule an appointment. The time to the next available appointment ranged from 0 to 180 days, with a mean of 38 days (95% CI 35.6 to 39.6).
This is an enumerative study, since results are intended to be used to estimate the waiting time for appointments with the plan's current population of primary care physicians.
Scenario 2: enumerative study—hypothesis generation
The researcher in scenario 1 noted that on occasion, she was offered an earlier visit with a nurse practitioner (NP) who worked with the physician being called. Additional information revealed that 20 of the 150 physicians in the study worked with one or more NPs. The next available appointment for the 130 physicians without an NP averaged 41 days (95% CI 39 to 43 days) and was 18 days (95% CI 18 to 26 days) for the 20 practices with NPs, a difference of 23 days (a 56% shorter mean waiting time).
This subgroup analysis suggested that the involvement of NPs helps to shorten waiting times, although it does not establish a cause–effect relationship, that is, it was a ‘hypothesis-generating’ study. In any event, this was clearly an enumerative study, since its results were to understand the impact of NPs on waiting times in the particular population of practices. Its results suggested that NPs might influence waiting times, but only for practices in this health plan during the time of the study. The study treated the conditions in the health plan as static, like those in a pond.
Scenario 3: enumerative study—comparison
To find out if administrative changes in a health plan had increased member satisfaction in access to care, the customer service manager replicated a phone survey he had conducted a year previously, using a random sample of 300 members. The percentage of patients who were satisfied with access had increased from 48.7% to 60.7% (Fisher exact test, p<0.004).
This enumerative comparison study was used to estimate the impact of the improvement work during the last year on the members in the plan. Attributing the increase in satisfaction to the improvement work assumes that other conditions in the study frame were static.
Scenario 4: analytical study—learning with a Shewhart chart
Each primary care clinic in a health plan reported its ‘time until the third available appointment’ twice a month, which allowed the quality manager to plot the mean waiting time for all of the clinics on Shewhart charts. Waiting times had been stable for a 12-month period through August, but the manager then noted a special cause (increase in waiting time) in September. On stratifying the data by region, she found that the special cause resulted from increases in waiting time in the Northeast region. Discussion with the regional manager revealed a shortage of primary care physicians in this region, which was predicted to become worse in the next quarter. Making some temporary assignments and increasing physician recruiting efforts resulted in stabilisation of this measure.
Documenting common and special cause variation in measures of interest through the use of Shewhart charts and run charts based on judgement samples is probably the simplest and commonest type of analytical study in healthcare. Such charts, when stable, provide a rational basis for predicting future performance.
Scenario 5: analytical study—establishing a cause–effect relationship
The researcher mentioned in scenarios 1 and 2 planned a study to test the existence of a cause–effect relationship between the inclusion of NPs in primary care offices and waiting time for new patient appointments. The variation in patient characteristics in this health plan appeared to be great enough to make the study results useful to other organisations. For the study, she recruited about 100 of the plan's practices that currently did not use NPs, and obtained funding to facilitate hiring NPs in up to 50 of those practices.
The researcher first explored the theories on mechanisms by which the incorporation of NPs into primary care clinics could reduce waiting times. Using important contextual variables relevant to these mechanisms (practice size, complexity, use of information technology and urban vs rural location), she then developed a randomised block, time-series study design. The study had the power to detect an effect of a mean waiting time of 5 days or more overall, and 10 days for the major subgroups defined by levels of the contextual variables. Since the baseline waiting time for appointments varied substantially across practices, she used the baseline as a covariate.
After completing the study, she analysed data from baseline and postintervention periods using stratified run charts and Shewhart charts, including the raw measures and measures adjusted for important covariates and effects of contextual variables. Overall waiting times decreased 12 days more in practices that included NPs than they did in control practices. Importantly, the subgroup analyses according to contextual variables revealed conditions under which the use of NPs would not be predicted to lead to reductions in waiting times. For example, practices with short baseline waiting times showed little or no improvement by employing NPs. She published the results in a leading health research journal.
This was an analytical study because the intent was to apply the learning from the study to future staffing plans in the health plan. She also published the study, so its results would be useful to primary care practices outside the health plan.
Scenario 6: analytical study—implementing improvement
The quality-improvement manager in another health plan wanted to expand the use of NPs in the plan's primary care practices, because published research had shown a reduction in waiting times for practices with NPs. Two practices in his plan already employed NPs. In one of these practices, Shewhart charts of waiting time by month showed a stable process averaging 10 days during the last 2 years. Waiting time averaged less than 7 days in the second practice, but a period when one of the physicians left the practice was associated with special causes.
The quality manager created a collaborative among the plan's primary care practices to learn how to optimise the use of NPs. Physicians in the two sites that employed NPs served as subject matter experts for the collaborative. In addition to making NPs part of their care teams, participating practices monitored appointment supply and demand, and tested other changes designed to optimise response to patient needs. Thirty sites in the plan voluntarily joined the collaborative and hired NPs. After 6 months, Shewhart charts indicated that waiting times in 25 of the 30 sites had been reduced to less than 7 days. Because waiting times in these practices had been stable over a considerable period of time, the manager predicted that future patients would continue to experience reduced times for appointments. The quality manger began to focus on a follow-up collaborative among the backlog of 70 practices that wanted to join.
This project was clearly an analytical study, since its aim was specifically to improve future waiting-time performance for participating sites and other primary care offices in the plan. Moreover, it focused on learning about the mechanisms through which contextual factors affect the impact of NPs on primary care office functions, under practice conditions that (like those in a river) will inevitably change over time.
Statistical theory in enumerative studies is used to describe the precision of estimates and the validity of hypotheses for the population studied. But since these statistical methods provide no support for extrapolation of the results outside the population that was studied, the subject experts must rely on their understanding of the mechanisms in place to extend results outside the population.
In analytical studies, the standard error of a statistic does not address the most important source of uncertainty, namely, the change in study conditions in the future. Although analytical studies need to take into account the uncertainty due to sampling, as in enumerative studies, the attributes of the study design and analysis of the data primarily deal with the uncertainty resulting from extrapolation to the future (generalisation to the conditions in future time periods). The methods used in analytical studies encourage the exploration of mechanisms through multifactor designs, contextual variables introduced through blocking and replication over time.
Prior stability of a system (as observed in graphic displays of repeated sampling over time, according to Shewhart's methods) increases belief in the results of an analytical study, but stable processes in the past do not guarantee constant system behaviour in the future. The next data point from the future is the most important on a graph of performance. Extrapolation of system behaviour to future times therefore still depends on input from subject experts who are familiar with mechanisms of the system of interest, as well as the important contextual issues. Generalisation is inherently difficult in all studies because ‘whereas the problems of internal validity are solvable within the limits of the logic of probability statistics, the problems of external validity are not logically solvable in any neat, conclusive way’ 3 (p. 17).
The diverse activities commonly referred to as healthcare improvement 12 are all designed to change the behaviour of systems over time, as reflected in the principle that ‘not all change is improvement, but all improvement is change.’ The conditions in the unbounded systems into which improvement interventions are introduced will therefore be different in the future from those in effect at the time the intervention is studied. Since the results of improvement studies are used to predict future system behaviour, such studies clearly belong to the Deming category of analytical studies. Quality improvement studies therefore need to incorporate repeated measurements over time, as well as testing under a wide range of conditions (2, 3 and 10). The ‘gold standard’ of analytical studies is satisfactory prediction over time.
Conclusions and recommendations
In light of these considerations, some important principles for drawing inferences from improvement studies include 10 :
- The analysis of data, interpretation of that analysis and actions taken as a result of the study should be closely tied to the current knowledge of experts about mechanisms of change in the relevant area. They can often use the study to discover, understand and evaluate the underlying mechanisms.
- The conditions of the study will be different from the future conditions under which the results will be used. Assessment by experts of the magnitude of this difference and its potential impact on future events should be an integral part of the interpretation of the results of the intervention.
- Show all the data before aggregation or summary.
- Plot the outcome data in the order in which the tests of change were conducted and annotate with information on the interventions.
- Use graphical displays to assess how much of the variation in the data can be explained by factors that were deliberately changed.
- Rearrange and subgroup the data to study other sources of variation (background and contextual variables).
- Summarise the results of the study with appropriate graphical displays.
Because these principles reflect the fundamental nature of improvement—taking action to change performance, over time, and under changing conditions—their application helps to bring clarity and rigour to improvement science.
Acknowledgments
The author is grateful to F Davidoff and P Batalden for their input to earlier versions of this paper.
Competing interests: None.
Provenance and peer review: Not commissioned; externally peer reviewed.
Have a language expert improve your writing
Run a free plagiarism check in 10 minutes, generate accurate citations for free.
- Knowledge Base
Methodology
- What Is a Research Design | Types, Guide & Examples
What Is a Research Design | Types, Guide & Examples
Published on June 7, 2021 by Shona McCombes . Revised on November 20, 2023 by Pritha Bhandari.
A research design is a strategy for answering your research question using empirical data. Creating a research design means making decisions about:
- Your overall research objectives and approach
- Whether you’ll rely on primary research or secondary research
- Your sampling methods or criteria for selecting subjects
- Your data collection methods
- The procedures you’ll follow to collect data
- Your data analysis methods
A well-planned research design helps ensure that your methods match your research objectives and that you use the right kind of analysis for your data.
Table of contents
Step 1: consider your aims and approach, step 2: choose a type of research design, step 3: identify your population and sampling method, step 4: choose your data collection methods, step 5: plan your data collection procedures, step 6: decide on your data analysis strategies, other interesting articles, frequently asked questions about research design.
- Introduction
Before you can start designing your research, you should already have a clear idea of the research question you want to investigate.
There are many different ways you could go about answering this question. Your research design choices should be driven by your aims and priorities—start by thinking carefully about what you want to achieve.
The first choice you need to make is whether you’ll take a qualitative or quantitative approach.
Qualitative approach | Quantitative approach |
---|---|
and describe frequencies, averages, and correlations about relationships between variables |
Qualitative research designs tend to be more flexible and inductive , allowing you to adjust your approach based on what you find throughout the research process.
Quantitative research designs tend to be more fixed and deductive , with variables and hypotheses clearly defined in advance of data collection.
It’s also possible to use a mixed-methods design that integrates aspects of both approaches. By combining qualitative and quantitative insights, you can gain a more complete picture of the problem you’re studying and strengthen the credibility of your conclusions.
Practical and ethical considerations when designing research
As well as scientific considerations, you need to think practically when designing your research. If your research involves people or animals, you also need to consider research ethics .
- How much time do you have to collect data and write up the research?
- Will you be able to gain access to the data you need (e.g., by travelling to a specific location or contacting specific people)?
- Do you have the necessary research skills (e.g., statistical analysis or interview techniques)?
- Will you need ethical approval ?
At each stage of the research design process, make sure that your choices are practically feasible.
Here's why students love Scribbr's proofreading services
Discover proofreading & editing
Within both qualitative and quantitative approaches, there are several types of research design to choose from. Each type provides a framework for the overall shape of your research.
Types of quantitative research designs
Quantitative designs can be split into four main types.
- Experimental and quasi-experimental designs allow you to test cause-and-effect relationships
- Descriptive and correlational designs allow you to measure variables and describe relationships between them.
Type of design | Purpose and characteristics |
---|---|
Experimental | relationships effect on a |
Quasi-experimental | ) |
Correlational | |
Descriptive |
With descriptive and correlational designs, you can get a clear picture of characteristics, trends and relationships as they exist in the real world. However, you can’t draw conclusions about cause and effect (because correlation doesn’t imply causation ).
Experiments are the strongest way to test cause-and-effect relationships without the risk of other variables influencing the results. However, their controlled conditions may not always reflect how things work in the real world. They’re often also more difficult and expensive to implement.
Types of qualitative research designs
Qualitative designs are less strictly defined. This approach is about gaining a rich, detailed understanding of a specific context or phenomenon, and you can often be more creative and flexible in designing your research.
The table below shows some common types of qualitative design. They often have similar approaches in terms of data collection, but focus on different aspects when analyzing the data.
Type of design | Purpose and characteristics |
---|---|
Grounded theory | |
Phenomenology |
Your research design should clearly define who or what your research will focus on, and how you’ll go about choosing your participants or subjects.
In research, a population is the entire group that you want to draw conclusions about, while a sample is the smaller group of individuals you’ll actually collect data from.
Defining the population
A population can be made up of anything you want to study—plants, animals, organizations, texts, countries, etc. In the social sciences, it most often refers to a group of people.
For example, will you focus on people from a specific demographic, region or background? Are you interested in people with a certain job or medical condition, or users of a particular product?
The more precisely you define your population, the easier it will be to gather a representative sample.
- Sampling methods
Even with a narrowly defined population, it’s rarely possible to collect data from every individual. Instead, you’ll collect data from a sample.
To select a sample, there are two main approaches: probability sampling and non-probability sampling . The sampling method you use affects how confidently you can generalize your results to the population as a whole.
Probability sampling | Non-probability sampling |
---|---|
Probability sampling is the most statistically valid option, but it’s often difficult to achieve unless you’re dealing with a very small and accessible population.
For practical reasons, many studies use non-probability sampling, but it’s important to be aware of the limitations and carefully consider potential biases. You should always make an effort to gather a sample that’s as representative as possible of the population.
Case selection in qualitative research
In some types of qualitative designs, sampling may not be relevant.
For example, in an ethnography or a case study , your aim is to deeply understand a specific context, not to generalize to a population. Instead of sampling, you may simply aim to collect as much data as possible about the context you are studying.
In these types of design, you still have to carefully consider your choice of case or community. You should have a clear rationale for why this particular case is suitable for answering your research question .
For example, you might choose a case study that reveals an unusual or neglected aspect of your research problem, or you might choose several very similar or very different cases in order to compare them.
Data collection methods are ways of directly measuring variables and gathering information. They allow you to gain first-hand knowledge and original insights into your research problem.
You can choose just one data collection method, or use several methods in the same study.
Survey methods
Surveys allow you to collect data about opinions, behaviors, experiences, and characteristics by asking people directly. There are two main survey methods to choose from: questionnaires and interviews .
Questionnaires | Interviews |
---|---|
) |
Observation methods
Observational studies allow you to collect data unobtrusively, observing characteristics, behaviors or social interactions without relying on self-reporting.
Observations may be conducted in real time, taking notes as you observe, or you might make audiovisual recordings for later analysis. They can be qualitative or quantitative.
Quantitative observation | |
---|---|
Other methods of data collection
There are many other ways you might collect data depending on your field and topic.
Field | Examples of data collection methods |
---|---|
Media & communication | Collecting a sample of texts (e.g., speeches, articles, or social media posts) for data on cultural norms and narratives |
Psychology | Using technologies like neuroimaging, eye-tracking, or computer-based tasks to collect data on things like attention, emotional response, or reaction time |
Education | Using tests or assignments to collect data on knowledge and skills |
Physical sciences | Using scientific instruments to collect data on things like weight, blood pressure, or chemical composition |
If you’re not sure which methods will work best for your research design, try reading some papers in your field to see what kinds of data collection methods they used.
Secondary data
If you don’t have the time or resources to collect data from the population you’re interested in, you can also choose to use secondary data that other researchers already collected—for example, datasets from government surveys or previous studies on your topic.
With this raw data, you can do your own analysis to answer new research questions that weren’t addressed by the original study.
Using secondary data can expand the scope of your research, as you may be able to access much larger and more varied samples than you could collect yourself.
However, it also means you don’t have any control over which variables to measure or how to measure them, so the conclusions you can draw may be limited.
Receive feedback on language, structure, and formatting
Professional editors proofread and edit your paper by focusing on:
- Academic style
- Vague sentences
- Style consistency
See an example
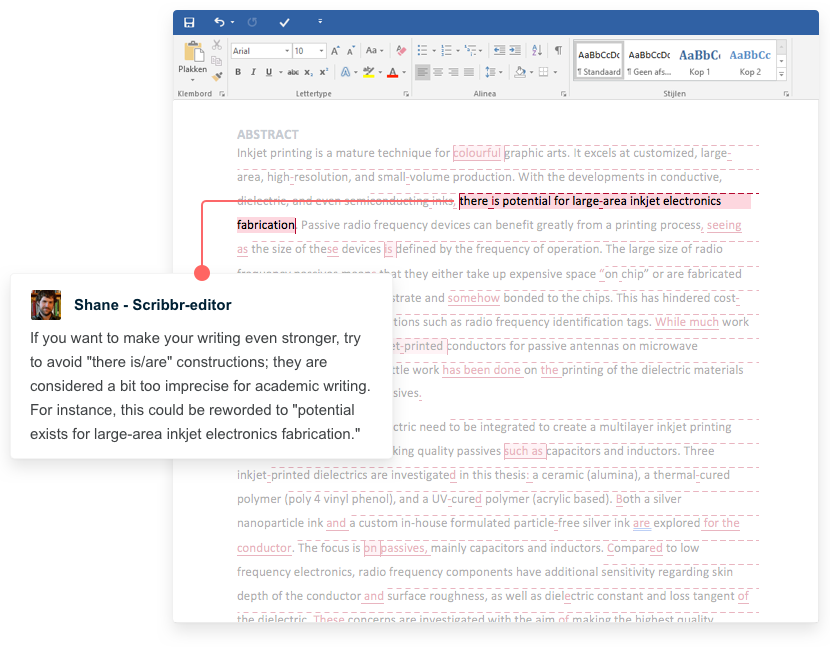
As well as deciding on your methods, you need to plan exactly how you’ll use these methods to collect data that’s consistent, accurate, and unbiased.
Planning systematic procedures is especially important in quantitative research, where you need to precisely define your variables and ensure your measurements are high in reliability and validity.
Operationalization
Some variables, like height or age, are easily measured. But often you’ll be dealing with more abstract concepts, like satisfaction, anxiety, or competence. Operationalization means turning these fuzzy ideas into measurable indicators.
If you’re using observations , which events or actions will you count?
If you’re using surveys , which questions will you ask and what range of responses will be offered?
You may also choose to use or adapt existing materials designed to measure the concept you’re interested in—for example, questionnaires or inventories whose reliability and validity has already been established.
Reliability and validity
Reliability means your results can be consistently reproduced, while validity means that you’re actually measuring the concept you’re interested in.
Reliability | Validity |
---|---|
) ) |
For valid and reliable results, your measurement materials should be thoroughly researched and carefully designed. Plan your procedures to make sure you carry out the same steps in the same way for each participant.
If you’re developing a new questionnaire or other instrument to measure a specific concept, running a pilot study allows you to check its validity and reliability in advance.
Sampling procedures
As well as choosing an appropriate sampling method , you need a concrete plan for how you’ll actually contact and recruit your selected sample.
That means making decisions about things like:
- How many participants do you need for an adequate sample size?
- What inclusion and exclusion criteria will you use to identify eligible participants?
- How will you contact your sample—by mail, online, by phone, or in person?
If you’re using a probability sampling method , it’s important that everyone who is randomly selected actually participates in the study. How will you ensure a high response rate?
If you’re using a non-probability method , how will you avoid research bias and ensure a representative sample?
Data management
It’s also important to create a data management plan for organizing and storing your data.
Will you need to transcribe interviews or perform data entry for observations? You should anonymize and safeguard any sensitive data, and make sure it’s backed up regularly.
Keeping your data well-organized will save time when it comes to analyzing it. It can also help other researchers validate and add to your findings (high replicability ).
On its own, raw data can’t answer your research question. The last step of designing your research is planning how you’ll analyze the data.
Quantitative data analysis
In quantitative research, you’ll most likely use some form of statistical analysis . With statistics, you can summarize your sample data, make estimates, and test hypotheses.
Using descriptive statistics , you can summarize your sample data in terms of:
- The distribution of the data (e.g., the frequency of each score on a test)
- The central tendency of the data (e.g., the mean to describe the average score)
- The variability of the data (e.g., the standard deviation to describe how spread out the scores are)
The specific calculations you can do depend on the level of measurement of your variables.
Using inferential statistics , you can:
- Make estimates about the population based on your sample data.
- Test hypotheses about a relationship between variables.
Regression and correlation tests look for associations between two or more variables, while comparison tests (such as t tests and ANOVAs ) look for differences in the outcomes of different groups.
Your choice of statistical test depends on various aspects of your research design, including the types of variables you’re dealing with and the distribution of your data.
Qualitative data analysis
In qualitative research, your data will usually be very dense with information and ideas. Instead of summing it up in numbers, you’ll need to comb through the data in detail, interpret its meanings, identify patterns, and extract the parts that are most relevant to your research question.
Two of the most common approaches to doing this are thematic analysis and discourse analysis .
Approach | Characteristics |
---|---|
Thematic analysis | |
Discourse analysis |
There are many other ways of analyzing qualitative data depending on the aims of your research. To get a sense of potential approaches, try reading some qualitative research papers in your field.
If you want to know more about the research process , methodology , research bias , or statistics , make sure to check out some of our other articles with explanations and examples.
- Simple random sampling
- Stratified sampling
- Cluster sampling
- Likert scales
- Reproducibility
Statistics
- Null hypothesis
- Statistical power
- Probability distribution
- Effect size
- Poisson distribution
Research bias
- Optimism bias
- Cognitive bias
- Implicit bias
- Hawthorne effect
- Anchoring bias
- Explicit bias
A research design is a strategy for answering your research question . It defines your overall approach and determines how you will collect and analyze data.
A well-planned research design helps ensure that your methods match your research aims, that you collect high-quality data, and that you use the right kind of analysis to answer your questions, utilizing credible sources . This allows you to draw valid , trustworthy conclusions.
Quantitative research designs can be divided into two main categories:
- Correlational and descriptive designs are used to investigate characteristics, averages, trends, and associations between variables.
- Experimental and quasi-experimental designs are used to test causal relationships .
Qualitative research designs tend to be more flexible. Common types of qualitative design include case study , ethnography , and grounded theory designs.
The priorities of a research design can vary depending on the field, but you usually have to specify:
- Your research questions and/or hypotheses
- Your overall approach (e.g., qualitative or quantitative )
- The type of design you’re using (e.g., a survey , experiment , or case study )
- Your data collection methods (e.g., questionnaires , observations)
- Your data collection procedures (e.g., operationalization , timing and data management)
- Your data analysis methods (e.g., statistical tests or thematic analysis )
A sample is a subset of individuals from a larger population . Sampling means selecting the group that you will actually collect data from in your research. For example, if you are researching the opinions of students in your university, you could survey a sample of 100 students.
In statistics, sampling allows you to test a hypothesis about the characteristics of a population.
Operationalization means turning abstract conceptual ideas into measurable observations.
For example, the concept of social anxiety isn’t directly observable, but it can be operationally defined in terms of self-rating scores, behavioral avoidance of crowded places, or physical anxiety symptoms in social situations.
Before collecting data , it’s important to consider how you will operationalize the variables that you want to measure.
A research project is an academic, scientific, or professional undertaking to answer a research question . Research projects can take many forms, such as qualitative or quantitative , descriptive , longitudinal , experimental , or correlational . What kind of research approach you choose will depend on your topic.
Cite this Scribbr article
If you want to cite this source, you can copy and paste the citation or click the “Cite this Scribbr article” button to automatically add the citation to our free Citation Generator.
McCombes, S. (2023, November 20). What Is a Research Design | Types, Guide & Examples. Scribbr. Retrieved July 27, 2024, from https://www.scribbr.com/methodology/research-design/
Is this article helpful?
Shona McCombes
Other students also liked, guide to experimental design | overview, steps, & examples, how to write a research proposal | examples & templates, ethical considerations in research | types & examples, what is your plagiarism score.
- Search Search Please fill out this field.
What Is Research and Development?
- Understanding R&D
- Types of R&D
- Pros and Cons
- Considerations
- R&D vs. Applied Research
- R&D Tax Credits
The Bottom Line
- Business Essentials
What Is Research and Development (R&D)?
:max_bytes(150000):strip_icc():format(webp)/wk_headshot_aug_2018_02__william_kenton-5bfc261446e0fb005118afc9.jpg)
Investopedia / Ellen Lindner
Research and development (R&D) is the series of activities that companies undertake to innovate. R&D is often the first stage in the development process that results in market research product development, and product testing.
Key Takeaways
- Research and development represents the activities companies undertake to innovate and introduce new products and services or to improve their existing offerings.
- R&D allows a company to stay ahead of its competition by catering to new wants or needs in the market.
- Companies in different sectors and industries conduct R&D—pharmaceuticals, semiconductors, and technology companies generally spend the most.
- R&D is often a broad approach to exploratory advancement, while applied research is more geared towards researching a more narrow scope.
- The accounting for treatment for R&D costs can materially impact a company's income statement and balance sheet.
Understanding Research and Development (R&D)
The concept of research and development is widely linked to innovation both in the corporate and government sectors. R&D allows a company to stay ahead of its competition. Without an R&D program, a company may not survive on its own and may have to rely on other ways to innovate such as engaging in mergers and acquisitions (M&A) or partnerships. Through R&D, companies can design new products and improve their existing offerings.
R&D is distinct from most operational activities performed by a corporation. The research and/or development is typically not performed with the expectation of immediate profit. Instead, it is expected to contribute to the long-term profitability of a company. R&D may often allow companies to secure intellectual property, including patents , copyrights, and trademarks as discoveries are made and products created.
Companies that set up and employ departments dedicated entirely to R&D commit substantial capital to the effort. They must estimate the risk-adjusted return on their R&D expenditures, which inevitably involves risk of capital. That's because there is no immediate payoff, and the return on investment (ROI) is uncertain. As more money is invested in R&D, the level of capital risk increases. Other companies may choose to outsource their R&D for a variety of reasons including size and cost.
Companies across all sectors and industries undergo R&D activities. Corporations experience growth through these improvements and the development of new goods and services. Pharmaceuticals, semiconductors , and software/technology companies tend to spend the most on R&D. In Europe, R&D is known as research and technical or technological development.
Many small and mid-sized businesses may choose to outsource their R&D efforts because they don't have the right staff in-house to meet their needs.
Types of Research and Development (R&D)
There are several different types of R&D that exist in the corporate world and within government. The type used depends entirely on the entity undertaking it and the results can differ.
Basic Research
There are business incubators and accelerators, where corporations invest in startups and provide funding assistance and guidance to entrepreneurs in the hope that innovations will result that they can use to their benefit.
M&As and partnerships are also forms of R&D as companies join forces to take advantage of other companies' institutional knowledge and talent.
Applied Research
One R&D model is a department staffed primarily by engineers who develop new products —a task that typically involves extensive research. There is no specific goal or application in mind with this model. Instead, the research is done for the sake of research.
Development Research
This model involves a department composed of industrial scientists or researchers, all of who are tasked with applied research in technical, scientific, or industrial fields. This model facilitates the development of future products or the improvement of current products and/or operating procedures.
The largest companies may also be the ones that drive the most R&D spend. For example, Amazon has reported $1.147 billion of research and development value on its 2023 annual report.
Advantages and Disadvantages of R&D
There are several key benefits to research and development. It facilitates innovation, allowing companies to improve existing products and services or by letting them develop new ones to bring to the market.
Because R&D also is a key component of innovation, it requires a greater degree of skill from employees who take part. This allows companies to expand their talent pool, which often comes with special skill sets.
The advantages go beyond corporations. Consumers stand to benefit from R&D because it gives them better, high-quality products and services as well as a wider range of options. Corporations can, therefore, rely on consumers to remain loyal to their brands. It also helps drive productivity and economic growth.
Disadvantages
One of the major drawbacks to R&D is the cost. First, there is the financial expense as it requires a significant investment of cash upfront. This can include setting up a separate R&D department, hiring talent, and product and service testing, among others.
Innovation doesn't happen overnight so there is also a time factor to consider. This means that it takes a lot of time to bring products and services to market from conception to production to delivery.
Because it does take time to go from concept to product, companies stand the risk of being at the mercy of changing market trends . So what they thought may be a great seller at one time may reach the market too late and not fly off the shelves once it's ready.
Facilitates innovation
Improved or new products and services
Expands knowledge and talent pool
Increased consumer choice and brand loyalty
Economic driver
Financial investment
Shifting market trends
R&D Accounting
R&D may be beneficial to a company's bottom line, but it is considered an expense . After all, companies spend substantial amounts on research and trying to develop new products and services. As such, these expenses are often reported for accounting purposes on the income statement and do not carry long-term value.
There are certain situations where R&D costs are capitalized and reported on the balance sheet. Some examples include but are not limited to:
- Materials, fixed assets, or other assets have alternative future uses with an estimable value and useful life.
- Software that can be converted or applied elsewhere in the company to have a useful life beyond a specific single R&D project.
- Indirect costs or overhead expenses allocated between projects.
- R&D purchased from a third party that is accompanied by intangible value. That intangible asset may be recorded as a separate balance sheet asset.
R&D Considerations
Before taking on the task of research and development, it's important for companies and governments to consider some of the key factors associated with it. Some of the most notable considerations are:
- Objectives and Outcome: One of the most important factors to consider is the intended goals of the R&D project. Is it to innovate and fill a need for certain products that aren't being sold? Or is it to make improvements on existing ones? Whatever the reason, it's always important to note that there should be some flexibility as things can change over time.
- Timing: R&D requires a lot of time. This involves reviewing the market to see where there may be a lack of certain products and services or finding ways to improve on those that are already on the shelves.
- Cost: R&D costs a great deal of money, especially when it comes to the upfront costs. And there may be higher costs associated with the conception and production of new products rather than updating existing ones.
- Risks: As with any venture, R&D does come with risks. R&D doesn't come with any guarantees, no matter the time and money that goes into it. This means that companies and governments may sacrifice their ROI if the end product isn't successful.
Research and Development vs. Applied Research
Basic research is aimed at a fuller, more complete understanding of the fundamental aspects of a concept or phenomenon. This understanding is generally the first step in R&D. These activities provide a basis of information without directed applications toward products, policies, or operational processes .
Applied research entails the activities used to gain knowledge with a specific goal in mind. The activities may be to determine and develop new products, policies, or operational processes. While basic research is time-consuming, applied research is painstaking and more costly because of its detailed and complex nature.
R&D Tax Credits
The IRS offers a R&D tax credit to encourage innovation and significantly reduction their tax liability. The credit calls for specific types of spend such as product development, process improvement, and software creation.
Enacted under Section 41 of the Internal Revenue Code, this credit encourages innovation by providing a dollar-for-dollar reduction in tax obligations. The eligibility criteria, expanded by the Protecting Americans from Tax Hikes (PATH) Act of 2015, now encompass a broader spectrum of businesses. The credit tens to benefit small-to-midsize enterprises.
To claim R&D tax credits, businesses must document their qualifying expenses and complete IRS Form 6765 (Credit for Increasing Research Activities). The credit, typically ranging from 6% to 8% of annual qualifying expenses, offers businesses a direct offset against federal income tax liabilities. Additionally, businesses can claim up to $250,000 per year against their payroll taxes.
Example of Research and Development (R&D)
One of the more innovative companies of this millennium is Apple Inc. As part of its annual reporting, it has the following to say about its research and development spend:
In 2023, Apple reported having spent $29.915 billion. This is 8% of their annual total net sales. Note that Apple's R&D spend was reported to be higher than the company's selling, general and administrative costs (of $24.932 billion).
Note that the company doesn't go into length about what exactly the R&D spend is for. According to the notes, the company's year-over-year growth was "driven primarily by increases in headcount-related expenses". However, this does not explain the underlying basis carried from prior years (i.e. materials, patents, etc.).
Research and development refers to the systematic process of investigating, experimenting, and innovating to create new products, processes, or technologies. It encompasses activities such as scientific research, technological development, and experimentation conducted to achieve specific objectives to bring new items to market.
What Types of Activities Can Be Found in Research and Development?
Research and development activities focus on the innovation of new products or services in a company. Among the primary purposes of R&D activities is for a company to remain competitive as it produces products that advance and elevate its current product line. Since R&D typically operates on a longer-term horizon, its activities are not anticipated to generate immediate returns. However, in time, R&D projects may lead to patents, trademarks, or breakthrough discoveries with lasting benefits to the company.
Why Is Research and Development Important?
Given the rapid rate of technological advancement, R&D is important for companies to stay competitive. Specifically, R&D allows companies to create products that are difficult for their competitors to replicate. Meanwhile, R&D efforts can lead to improved productivity that helps increase margins, further creating an edge in outpacing competitors. From a broader perspective, R&D can allow a company to stay ahead of the curve, anticipating customer demands or trends.
There are many things companies can do in order to advance in their industries and the overall market. Research and development is just one way they can set themselves apart from their competition. It opens up the potential for innovation and increasing sales. However, it does come with some drawbacks—the most obvious being the financial cost and the time it takes to innovate.
Amazon. " 2023 Annual Report ."
Internal Revenue Service. " Research Credit ."
Internal Revenue Service. " About Form 6765, Credit for Increasing Research Activities ."
Apple. " 2023 Annual Report ."
:max_bytes(150000):strip_icc():format(webp)/research_pharma-5bfc322b46e0fb0051bf11a0.jpg)
- Terms of Service
- Editorial Policy
- Privacy Policy
IMAGES
VIDEO
COMMENTS
Analytical research is the process of gathering, analyzing, and interpreting information to make inferences and reach conclusions. Depending on the purpose of the research and the data you have access to, you can conduct analytical research using a variety of methods. Here are a few typical approaches:
Analytical method development is the process of selecting and optimizing analytical methods to measure a specific attribute of a drug substance or drug product. This process involves a systematic approach to evaluating and selecting suitable methods that are sensitive, specific, and robust, and can be used to measure the target attribute within ...
Research study design is a framework, or the set of methods and procedures used to collect and analyze data on variables specified in a particular research problem. Research study designs are of many types, each with its advantages and limitations. The type of study design used to answer a particular research question is determined by the ...
Who we are. Analytical Research and Development (AR&D) aims to advance the Merck pipeline from discovery through commercial filing and launch by delivering integrated physical, analytical, and bioanalytical solutions and applying innovative technologies while collaborating across internal networks and the external scientific and regulatory ...
Analytical Method Development and Validation (AMV) involves the process of creating and testing new analytical techniques to accurately measure components of products. This method development can include both fundamental research and applying existing theories to predict unknowns. Once a method has been created, it must then be validated to ...
Research methods are specific procedures for collecting and analyzing data. Developing your research methods is an integral part of your research design. When planning your methods, there are two key decisions you will make. First, decide how you will collect data. Your methods depend on what type of data you need to answer your research question:
When are analytical study designs used? A study design is a systematic plan, developed so you can carry out your research study effectively and efficiently. Having a design is important because it will determine the right methodologies for your study. Using the right study design makes your results more credible, valid, and coherent.
Chemistry Research Journal, 2020, 5(3):173-186 Chemistry Research Journal 173 Available online www.chemrj.org Re ISSN: 2455 view Article-8990 CODEN(USA): CRJHA5 Analytical Method Development and Validation: A Review Shashi Daksh*, Anju Goyal *B.N. Institute of Pharmaceutical Sciences, Faculty of Pharmacy, B N University, Udaipur, Rajasthan ...
To be most effective, analytical method development must not occur in isolation. When analytical methods for manufacturing processes need to be improved, the BPT-A team often works in conjunction with the manufacturing team or the QC department. There was a need to replace the cuvette-based traditional A 280 measurement with the SoloVPE method.
The definition of surrogate performance criteria (also known as analytical procedure attributes (APAs) linked to the ATP acceptance criteria) underpins the method development process. The APAs defined for a given method are technology specific properties (for example, attributes for chromatography measurements may include peak symmetry factor ...
An analytical study is one in which action will be taken on a cause system to improve the future performance of the system of interest. The aim of an enumerative study is estimation, while an analytical study focuses on prediction. Because of the temporal nature of improvement, the theory and methods for analytical studies are a critical ...
Examples of analytical skills. There are many types of analytical skills that you can apply in professional settings. Here are examples of the main skills that lead to effective analytical thinking: Critical thinking. Research. Communication. Data analysis. Problem-solving. Creative thinking.
Other interesting articles. If you want to know more about statistics, methodology, or research bias, make sure to check out some of our other articles with explanations and examples. Statistics. Normal distribution. Skewness. Kurtosis. Degrees of freedom. Variance. Null hypothesis.
The main purpose of development of. analytical methods are for identification, purification. and finally to quatification any required drug etc., T he. main activities involved in the analytical ...
Validated analytical strategies play a significant role in achieving this goal. Outcomes from methodology validation may be used to choose the standard, reliability, and consistency of analytical results, that is associated as an integral part of any sensible analytical practice. Validation of analytical strategies is also
A research design is a strategy for answering your research question using empirical data. Creating a research design means making decisions about: Your overall research objectives and approach. Whether you'll rely on primary research or secondary research. Your sampling methods or criteria for selecting subjects. Your data collection methods.
Research And Development - R&D: Research and development (R&D) refers to the investigative activities a business conducts to improve existing products and procedures or to lead to the development ...
Analytical method development is a process whose main purpose is to prove if any analytical method in the pharmaceutical industry is suitable to be used in the measurement of API. ... Definition of Requirements Requirements for the method development of the analysis are done and recorded. All the materials, reagents and instruments are procured ...
Written by MasterClass. Last updated: Nov 2, 2021 • 4 min read. Hiring managers and job recruiters value strong analytical skills. Develop and showcase your analytical thinking skills to help your application stand out. Explore.
Research: An Analytical Review . ... This study uses the Research and Development method with stages using Lee & Owens stages. The research instrument used is a questionnaire. ... and reporting a ...
Analytical research is a specific type of research that involves critical thinking skills and the evaluation of facts and information relative to the research being conducted. A variety of people including students, doctors and psychologists use analytical research during studies to find the most relevant information. From analytical research, a person finds out critical details to add new ...
Analytical research is a methodical investigation approach that delves deep into complex subjects through data analysis. It aids in understanding, problem-solving, and informed decision-making in diverse fields. A retail company is using analytical research to enhance its marketing strategies. They gather extensive data on consumer behaviour ...
Western blot (WB) assays are a widely used analytical tool that is designed to detect specific protein and/or fragments in a sample. Since the first successful description of WB in 1979 [], the technique has evolved with a number of modifications and improvements.Typically, WB methods involve three main steps, (1) separation of the proteins (including target (analyte of interest)) in the ...