- Subject List
- Take a Tour
- For Authors
- Subscriber Services
- Publications
- African American Studies
- African Studies
- American Literature
- Anthropology
- Architecture Planning and Preservation
- Art History
- Atlantic History
- Biblical Studies
- British and Irish Literature
- Childhood Studies
- Chinese Studies
- Cinema and Media Studies
- Communication
- Criminology
- Environmental Science
- Evolutionary Biology
- International Law
- International Relations
- Islamic Studies
- Jewish Studies
- Latin American Studies
- Latino Studies
- Linguistics
- Literary and Critical Theory
- Medieval Studies
- Military History
- Political Science
- Public Health
- Renaissance and Reformation
- Social Work
- Urban Studies
- Victorian Literature
- Browse All Subjects
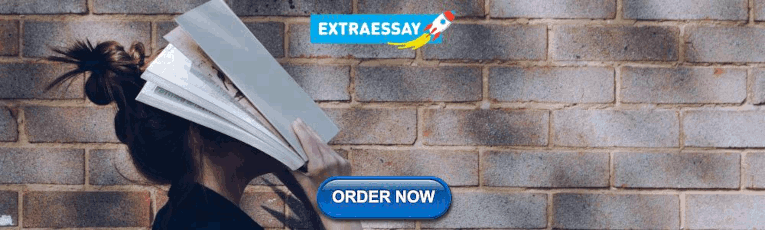
How to Subscribe
- Free Trials
In This Article Expand or collapse the "in this article" section Single-Case Experimental Designs
Introduction, general overviews and primary textbooks.
- Textbooks in Applied Behavior Analysis
- Types of Single-Case Experimental Designs
- Model Building and Randomization in Single-Case Experimental Designs
- Visual Analysis of Single-Case Experimental Designs
- Effect Size Estimates in Single-Case Experimental Designs
- Reporting Single-Case Design Intervention Research
Related Articles Expand or collapse the "related articles" section about
About related articles close popup.
Lorem Ipsum Sit Dolor Amet
Vestibulum ante ipsum primis in faucibus orci luctus et ultrices posuere cubilia Curae; Aliquam ligula odio, euismod ut aliquam et, vestibulum nec risus. Nulla viverra, arcu et iaculis consequat, justo diam ornare tellus, semper ultrices tellus nunc eu tellus.
- Action Research
- Ambulatory Assessment in Behavioral Science
- Effect Size
- Mediation Analysis
- Path Models
- Research Methods for Studying Daily Life
Other Subject Areas
Forthcoming articles expand or collapse the "forthcoming articles" section.
- Data Visualization
- Melanie Klein
- Remote Work
- Find more forthcoming articles...
- Export Citations
- Share This Facebook LinkedIn Twitter
Single-Case Experimental Designs by S. Andrew Garbacz , Thomas R. Kratochwill LAST MODIFIED: 29 July 2020 DOI: 10.1093/obo/9780199828340-0265
Single-case experimental designs are a family of experimental designs that are characterized by researcher manipulation of an independent variable and repeated measurement of a dependent variable before (i.e., baseline) and after (i.e., intervention phase) introducing the independent variable. In single-case experimental designs a case is the unit of intervention and analysis (e.g., a child, a school). Because measurement within each case is conducted before and after manipulation of the independent variable, the case typically serves as its own control. Experimental variants of single-case designs provide a basis for determining a causal relation by replication of the intervention through (a) introducing and withdrawing the independent variable, (b) manipulating the independent variable across different phases, and (c) introducing the independent variable in a staggered fashion across different points in time. Due to their economy of resources, single-case designs may be useful during development activities and allow for rapid replication across studies.
Several sources provide overviews of single-case experimental designs. Barlow, et al. 2009 includes an overview for the development of single-case experimental designs, describes key considerations for designing and conducting single-case experimental design research, and reviews procedural elements, assessment strategies, and replication considerations. Kazdin 2011 provides detailed coverage of single-case experimental design variants as well as approaches for evaluating data in single-case experimental designs. Kratochwill and Levin 2014 describes key methodological features that underlie single-case experimental designs, including philosophical and statistical foundations and data evaluation. Ledford and Gast 2018 covers research conceptualization and writing, design variants within single-case experimental design, definitions of variables and associated measurement, and approaches to organize and evaluate data. Riley-Tillman and Burns 2009 provides a practical orientation to single-case experimental designs to facilitate uptake and use in applied settings.
Barlow, D. H., M. K. Nock, and M. Hersen, eds. 2009. Single case experimental designs: Strategies for studying behavior change . 3d ed. New York: Pearson.
A comprehensive reference about the process of designing and conducting single-case experimental design studies. Chapters are integrative but can stand alone.
Kazdin, A. E. 2011. Single-case research designs: Methods for clinical and applied settings . 2d ed. New York: Oxford Univ. Press.
A complete overview and description of single-case experimental design variants as well as information about data evaluation.
Kratochwill, T. R., and J. R. Levin, eds. 2014. Single-case intervention research: Methodological and statistical advances . New York: Routledge.
The authors describe in depth the methodological and analytic considerations necessary for designing and conducting research that uses a single-case experimental design. In addition, the text includes chapters from leaders in psychology and education who provide critical perspectives about the use of single-case experimental designs.
Ledford, J. R., and D. L. Gast, eds. 2018. Single case research methodology: Applications in special education and behavioral sciences . New York: Routledge.
Covers the research process from writing literature reviews, to designing, conducting, and evaluating single-case experimental design studies.
Riley-Tillman, T. C., and M. K. Burns. 2009. Evaluating education interventions: Single-case design for measuring response to intervention . New York: Guilford Press.
Focuses on accelerating uptake and use of single-case experimental designs in applied settings. This book provides a practical, “nuts and bolts” orientation to conducting single-case experimental design research.
back to top
Users without a subscription are not able to see the full content on this page. Please subscribe or login .
Oxford Bibliographies Online is available by subscription and perpetual access to institutions. For more information or to contact an Oxford Sales Representative click here .
- About Psychology »
- Meet the Editorial Board »
- Abnormal Psychology
- Academic Assessment
- Acculturation and Health
- Action Regulation Theory
- Addictive Behavior
- Adolescence
- Adoption, Social, Psychological, and Evolutionary Perspect...
- Advanced Theory of Mind
- Affective Forecasting
- Affirmative Action
- Ageism at Work
- Allport, Gordon
- Alzheimer’s Disease
- Analysis of Covariance (ANCOVA)
- Animal Behavior
- Animal Learning
- Anxiety Disorders
- Art and Aesthetics, Psychology of
- Artificial Intelligence, Machine Learning, and Psychology
- Assessment and Clinical Applications of Individual Differe...
- Attachment in Social and Emotional Development across the ...
- Attention-Deficit/Hyperactivity Disorder (ADHD) in Adults
- Attention-Deficit/Hyperactivity Disorder (ADHD) in Childre...
- Attitudinal Ambivalence
- Attraction in Close Relationships
- Attribution Theory
- Authoritarian Personality
- Bayesian Statistical Methods in Psychology
- Behavior Therapy, Rational Emotive
- Behavioral Economics
- Behavioral Genetics
- Belief Perseverance
- Bereavement and Grief
- Biological Psychology
- Birth Order
- Body Image in Men and Women
- Bystander Effect
- Categorical Data Analysis in Psychology
- Childhood and Adolescence, Peer Victimization and Bullying...
- Clark, Mamie Phipps
- Clinical Neuropsychology
- Clinical Psychology
- Cognitive Consistency Theories
- Cognitive Dissonance Theory
- Cognitive Neuroscience
- Communication, Nonverbal Cues and
- Comparative Psychology
- Competence to Stand Trial: Restoration Services
- Competency to Stand Trial
- Computational Psychology
- Conflict Management in the Workplace
- Conformity, Compliance, and Obedience
- Consciousness
- Coping Processes
- Correspondence Analysis in Psychology
- Counseling Psychology
- Creativity at Work
- Critical Thinking
- Cross-Cultural Psychology
- Cultural Psychology
- Daily Life, Research Methods for Studying
- Data Science Methods for Psychology
- Data Sharing in Psychology
- Death and Dying
- Deceiving and Detecting Deceit
- Defensive Processes
- Depressive Disorders
- Development, Prenatal
- Developmental Psychology (Cognitive)
- Developmental Psychology (Social)
- Diagnostic and Statistical Manual of Mental Disorders (DSM...
- Discrimination
- Dissociative Disorders
- Drugs and Behavior
- Eating Disorders
- Ecological Psychology
- Educational Settings, Assessment of Thinking in
- Embodiment and Embodied Cognition
- Emerging Adulthood
- Emotional Intelligence
- Empathy and Altruism
- Employee Stress and Well-Being
- Environmental Neuroscience and Environmental Psychology
- Ethics in Psychological Practice
- Event Perception
- Evolutionary Psychology
- Expansive Posture
- Experimental Existential Psychology
- Exploratory Data Analysis
- Eyewitness Testimony
- Eysenck, Hans
- Factor Analysis
- Festinger, Leon
- Five-Factor Model of Personality
- Flynn Effect, The
- Forensic Psychology
- Forgiveness
- Friendships, Children's
- Fundamental Attribution Error/Correspondence Bias
- Gambler's Fallacy
- Game Theory and Psychology
- Geropsychology, Clinical
- Global Mental Health
- Habit Formation and Behavior Change
- Health Psychology
- Health Psychology Research and Practice, Measurement in
- Heider, Fritz
- Heuristics and Biases
- History of Psychology
- Human Factors
- Humanistic Psychology
- Implicit Association Test (IAT)
- Industrial and Organizational Psychology
- Inferential Statistics in Psychology
- Insanity Defense, The
- Intelligence
- Intelligence, Crystallized and Fluid
- Intercultural Psychology
- Intergroup Conflict
- International Classification of Diseases and Related Healt...
- International Psychology
- Interviewing in Forensic Settings
- Intimate Partner Violence, Psychological Perspectives on
- Introversion–Extraversion
- Item Response Theory
- Law, Psychology and
- Lazarus, Richard
- Learned Helplessness
- Learning Theory
- Learning versus Performance
- LGBTQ+ Romantic Relationships
- Lie Detection in a Forensic Context
- Life-Span Development
- Locus of Control
- Loneliness and Health
- Mathematical Psychology
- Meaning in Life
- Mechanisms and Processes of Peer Contagion
- Media Violence, Psychological Perspectives on
- Memories, Autobiographical
- Memories, Flashbulb
- Memories, Repressed and Recovered
- Memory, False
- Memory, Human
- Memory, Implicit versus Explicit
- Memory in Educational Settings
- Memory, Semantic
- Meta-Analysis
- Metacognition
- Metaphor, Psychological Perspectives on
- Microaggressions
- Military Psychology
- Mindfulness
- Mindfulness and Education
- Minnesota Multiphasic Personality Inventory (MMPI)
- Money, Psychology of
- Moral Conviction
- Moral Development
- Moral Psychology
- Moral Reasoning
- Nature versus Nurture Debate in Psychology
- Neuroscience of Associative Learning
- Nonergodicity in Psychology and Neuroscience
- Nonparametric Statistical Analysis in Psychology
- Observational (Non-Randomized) Studies
- Obsessive-Complusive Disorder (OCD)
- Occupational Health Psychology
- Olfaction, Human
- Operant Conditioning
- Optimism and Pessimism
- Organizational Justice
- Parenting Stress
- Parenting Styles
- Parents' Beliefs about Children
- Peace Psychology
- Perception, Person
- Performance Appraisal
- Personality and Health
- Personality Disorders
- Personality Psychology
- Phenomenological Psychology
- Placebo Effects in Psychology
- Play Behavior
- Positive Psychological Capital (PsyCap)
- Positive Psychology
- Posttraumatic Stress Disorder (PTSD)
- Prejudice and Stereotyping
- Pretrial Publicity
- Prisoner's Dilemma
- Problem Solving and Decision Making
- Procrastination
- Prosocial Behavior
- Prosocial Spending and Well-Being
- Protocol Analysis
- Psycholinguistics
- Psychological Literacy
- Psychological Perspectives on Food and Eating
- Psychology, Political
- Psychoneuroimmunology
- Psychophysics, Visual
- Psychotherapy
- Psychotic Disorders
- Publication Bias in Psychology
- Reasoning, Counterfactual
- Rehabilitation Psychology
- Relationships
- Reliability–Contemporary Psychometric Conceptions
- Religion, Psychology and
- Replication Initiatives in Psychology
- Research Methods
- Risk Taking
- Role of the Expert Witness in Forensic Psychology, The
- Sample Size Planning for Statistical Power and Accurate Es...
- Schizophrenic Disorders
- School Psychology
- School Psychology, Counseling Services in
- Self, Gender and
- Self, Psychology of the
- Self-Construal
- Self-Control
- Self-Deception
- Self-Determination Theory
- Self-Efficacy
- Self-Esteem
- Self-Monitoring
- Self-Regulation in Educational Settings
- Self-Report Tests, Measures, and Inventories in Clinical P...
- Sensation Seeking
- Sex and Gender
- Sexual Minority Parenting
- Sexual Orientation
- Signal Detection Theory and its Applications
- Simpson's Paradox in Psychology
- Single People
- Single-Case Experimental Designs
- Skinner, B.F.
- Sleep and Dreaming
- Small Groups
- Social Class and Social Status
- Social Cognition
- Social Neuroscience
- Social Support
- Social Touch and Massage Therapy Research
- Somatoform Disorders
- Spatial Attention
- Sports Psychology
- Stanford Prison Experiment (SPE): Icon and Controversy
- Stereotype Threat
- Stereotypes
- Stress and Coping, Psychology of
- Student Success in College
- Subjective Wellbeing Homeostasis
- Taste, Psychological Perspectives on
- Teaching of Psychology
- Terror Management Theory
- Testing and Assessment
- The Concept of Validity in Psychological Assessment
- The Neuroscience of Emotion Regulation
- The Reasoned Action Approach and the Theories of Reasoned ...
- The Weapon Focus Effect in Eyewitness Memory
- Theory of Mind
- Therapies, Person-Centered
- Therapy, Cognitive-Behavioral
- Thinking Skills in Educational Settings
- Time Perception
- Trait Perspective
- Trauma Psychology
- Twin Studies
- Type A Behavior Pattern (Coronary Prone Personality)
- Unconscious Processes
- Video Games and Violent Content
- Virtues and Character Strengths
- Women and Science, Technology, Engineering, and Math (STEM...
- Women, Psychology of
- Work Well-Being
- Wundt, Wilhelm
- Privacy Policy
- Cookie Policy
- Legal Notice
- Accessibility
Powered by:
- [66.249.64.20|81.177.182.159]
- 81.177.182.159
Single-Case Design, Analysis, and Quality Assessment for Intervention Research
Affiliation.
- 1 Biomechanics & Movement Science Program, Department of Physical Therapy, University of Delaware, Newark, Delaware (M.A.L., A.B.C., I.B.); and Division of Educational Psychology & Methodology, State University of New York at Albany, Albany, New York (M.M.).
- PMID: 28628553
- PMCID: PMC5492992
- DOI: 10.1097/NPT.0000000000000187
Background and purpose: The purpose of this article is to describe single-case studies and contrast them with case studies and randomized clinical trials. We highlight current research designs, analysis techniques, and quality appraisal tools relevant for single-case rehabilitation research.
Summary of key points: Single-case studies can provide a viable alternative to large group studies such as randomized clinical trials. Single-case studies involve repeated measures and manipulation of an independent variable. They can be designed to have strong internal validity for assessing causal relationships between interventions and outcomes, as well as external validity for generalizability of results, particularly when the study designs incorporate replication, randomization, and multiple participants. Single-case studies should not be confused with case studies/series (ie, case reports), which are reports of clinical management of a patient or a small series of patients.
Recommendations for clinical practice: When rigorously designed, single-case studies can be particularly useful experimental designs in a variety of situations, such as when research resources are limited, studied conditions have low incidences, or when examining effects of novel or expensive interventions. Readers will be directed to examples from the published literature in which these techniques have been discussed, evaluated for quality, and implemented.
- Cohort Studies
- Medical Records*
- Quality Assurance, Health Care*
- Randomized Controlled Trials as Topic
- Research Design*
Grants and funding
- R21 HD076092/HD/NICHD NIH HHS/United States

An official website of the United States government
The .gov means it’s official. Federal government websites often end in .gov or .mil. Before sharing sensitive information, make sure you’re on a federal government site.
The site is secure. The https:// ensures that you are connecting to the official website and that any information you provide is encrypted and transmitted securely.
- Publications
- Account settings
Preview improvements coming to the PMC website in October 2024. Learn More or Try it out now .
- Advanced Search
- Journal List
- Healthcare (Basel)

Randomized Single-Case Experimental Designs in Healthcare Research: What, Why, and How?
Health problems are often idiosyncratic in nature and therefore require individualized diagnosis and treatment. In this paper, we show how single-case experimental designs (SCEDs) can meet the requirement to find and evaluate individually tailored treatments. We give a basic introduction to the methodology of SCEDs and provide an overview of the available design options. For each design, we show how an element of randomization can be incorporated to increase the internal and statistical conclusion validity and how the obtained data can be analyzed using visual tools, effect size measures, and randomization inference. We illustrate each design and data analysis technique using applied data sets from the healthcare literature.
1. Introduction
“Averaging data across many subjects can hide a multitude of sins: The experimental treatment may fail to affect the behavior of some subjects, and may even lead to contrary effects in others. As a consequence, statistically significant results based on large sample sizes are not persuasive.” [ 1 ]. Most people trust their healthcare providers with adequate care of a large variety of symptoms, ranging from a simple cold to more complex health problems, such as chronic pain, chronic diseases, or allergies. The opening quote by Perone highlights that rigorous experimental testing and persuasive results are needed to justify the patients’ trust in their healthcare providers. Perone also highlights the individuality of each person when it comes to finding an effective treatment, which by definition limits the applicability of large-scale group studies for situations in which symptoms are highly idiosyncratic in nature.
Following this logic, a shift towards individualized testing and experimental results in healthcare followed the accumulation of evidence that general healthcare diagnoses and interventions often fail to accurately describe and relieve patient symptoms (e.g., [ 2 , 3 , 4 ]). Already 25 years ago, McHorney and Tarlov recognized that the available health status surveys at the time were not adequate for individual patient monitoring in clinical practice [ 5 ]. McHorney and Tarlov reviewed five available health status measures and concluded that “the most problematic feature of the five surveys was their lack of precision for individual-patient applications. There was little evidence of the validity of the five surveys for screening, diagnosing, or monitoring individual patients. At this time, however, it seems that new instruments, or adaptation of existing measures and scaling methods, are needed for individual-patient assessment and monitoring”. Similarly, finding effective treatments for individual patients is difficult to achieve without individually tailored interventions. Turk argued in his influential paper on customizing pain treatments that a substantial proportion of patients does not benefit from generic treatments in spite of an increasingly better understanding of the mechanisms of pain [ 4 ]. According to Turk, limited success of chronic pain treatments at the time was caused by assuming homogeneity between patients, which Turk labeled as the “patient and treatment uniformity myths”. In Turk’s view, the remedy to this myth is a better matching of empirical data to patient characteristics in order to design individual treatment plans.
These limits of group studies, specifically in healthcare, were recognized in clinical practice after decades, in which they were thought to be the gold standard and the “ N -of-1 randomized controlled trial” was included among the highest levels of evidence in the Oxford Centre for Evidence-Based Medicine and in the Evidence-Based Medicine Guidelines of the American Medical Association [ 6 , 7 , 8 ]. Outside the medical field, N -of-1 randomized controlled trials have already long been in use under the name single-case experimental designs (SCEDs). In this paper, we review and empirically demonstrate the use of a specific form of individualized experimentation: randomized SCEDs. Vohra [ 9 ] accurately summarized a shift towards SCEDs in healthcare and evidence-based medicine, when she said that “although evidence-based medicine has embraced large parallel group trials as the gold standard for health research, there are limitations in the ability to apply data from these trials into routine clinical practice. Rigorous research methods that yield high-quality data from individual patients have the opportunity to not only inform the care of that individual, but also the group of individuals who suffer from the same condition(s). Rather than starting with a group, and extrapolating inferences at the level of the individual, single-case experimental designs ( sic ) evaluate treatment response at the level of the individual, and when combined, may inform how we should treat groups of patients with similar conditions”.
As Vohra explained, SCEDs turn the logic of group studies upside down to find effective treatments in healthcare applications, in which the individual is the unit of analysis and intervention. SCEDs come with the additional advantage that they require fewer resources and are often practically more feasible, for example when many variants of a therapy exist and they cannot all be tested in large-group studies [ 10 ].
SCEDs are thus viable and powerful alternatives to group studies in healthcare research. To draw valid conclusions from SCEDs about novel or existing treatments, it is pivotal to choose a strong design and adequate data analysis tools. In this paper, we showcase how an element of randomization can be incorporated into the design of an SCED to strengthen the internal validity of the experiment. We first define SCEDs, distinguish SCEDs from other non-experimental forms of case research, and present a typology of different types of SCEDs. We then move on to define and discuss each type accompanied by an applied publication from the healthcare literature. For each applied data set, we explain stepwise how an element of randomization can be implemented and how the obtained data can be analyzed using visual analysis, effect size calculation, and randomization tests.
2. Single-Case Experimental Designs: Definition and Overview of Design Options
Contemporary textbooks on SCEDs follow a long tradition. An early and fierce proponent of SCEDs was B.F. Skinner [ 11 ], proclaiming in his 1956 seminal paper on the scientific method that “we are within reach of a science of the individual. This will be achieved, not by resorting to some special theory of knowledge in which intuition or understanding takes the place of observation and analysis, but through an increasing grasp of relevant conditions to produce order in the individual case”.
Excellent introductory texts on SCEDs for healthcare professionals are available in Morgan and Morgan [ 12 , 13 ], who credited Skinner as an important figure in the advancement of SCEDs for behavioral and healthcare sciences. Other recommended textbooks on the methodology of SCEDs include Barlow et al. [ 14 ], Kazdin [ 10 ], and Ledford and Gast [ 15 ]. In spite of the fact that many different (sub-)forms of SCEDs exist, they all have some common underlying features. All the forms of SCEDs comprise of repeated measurements (e.g., daily disability ratings) taken from a single entity (e.g., a pain patient) under different levels of at least one independent variable (e.g., treatment for chronic pain) [ 16 , 17 ]. Table 1 provides a typology of SCEDs with four overarching categories: phase designs, alternation designs, multiple baseline designs, and changing criterion designs (cf. [ 18 , 19 ]). Within each of these categories, different design options exist. For further information about each design example, interested readers are referred to the key references in Table 1 .
Overview of single-case experimental design (SCED) options with references for further reading.
A systematic review of published SCEDs with health behavior outcomes is available in a study by McDonald et al. [ 35 ]. As the authors pointed out, it is important to clearly distinguish these types of single-case experimental research from other types of non-experimental case research. In non-experimental case studies, no intervention takes place. Instead, the behavior of interest is observed and measured over time as it occurs naturally. As such, observational case studies can give valuable insights about how behaviors evolve naturally over time. Contrary to that, the ultimate goal of SCEDs as a form of individualized experimentation is to assess whether a causal relationship exists between the independent and dependent variables. This can give information about which treatment works best for a patient by observing changes in the health outcome behavior under different manipulations of the independent variable (e.g., different therapies for decreasing self-harming behavior in a patient with depressive symptoms). McDonald et al. pointed out that, in experimental forms of single-case research with health behavior outcomes, it is advised to incorporate randomization into the design if possible. In the following paragraphs, we define the concept of randomization, define each type of SCED, present published data sets from the healthcare literature for each type, and show how an element of randomization can be included in each category to strengthen the internal validity and analyze the data using the randomization tests.
3. Randomization in Single-Case Experimental Designs
The incorporation of an element of randomization in the design of experiments has a long-standing history and interested readers are referred to Finch [ 36 ], Kempthorne [ 37 ], and Onghena [ 38 ] for an extensive historical discussion of the concept. Randomization is not a prerequisite for conducting an SCED. However, the added value of incorporating an element of randomization in the design of an SCED has been extensively discussed in the literature. It is recommended that an element of randomization should be incorporated whenever the research conditions under consideration allow it to enhance the scientific credibility of SCEDs [ 39 , 40 , 41 , 42 ]. Onghena and Edgington further contended that randomized experiments are in many aspects superior to non-randomized experiments due to stronger control over confounding factors, such as time, the participants, or the setting [ 18 ]. Furthermore, randomization facilitates some difficult decisions in the planning phase of an SCED. For example, the randomization procedure helps make decisions about the order, in which treatments are presented to a patient [ 43 ].
In addition to the advantages that randomization offers in terms of enhanced scientific credibility, control over internal validity threats in the planning of an SCED, randomization has great added value to the statistical conclusion validity. Kempthorne pointed out that randomization in the design of experiments frees the researcher from having to use statistical models, of which assumptions might not actually have been met by the experimental procedure and resulting data [ 44 ]. Similarly, Todman and Dugard asserted that the incorporation of randomization in the design of experiments makes such experiments eligible for statistical tests based on the random assignment procedure actually utilized in the experiment [ 45 ].
These statistical tests are called randomization tests. Randomization tests are valid and powerful significance tests under the assumption that “in experiments in which randomization is performed, the actual arrangement of treatments … is one chosen at random from a predetermined set of possible arrangements” [ 24 ]. Comprehensive textbooks on randomization tests in general and in particular for SCEDs are available in Edgington and Onghena [ 46 ] and Todman et al. [ 21 ], respectively. The steps involved in conducting a randomized SCED and analyzing the obtained data with a randomization test are explained in Heyvaert and Onghena [ 47 ] and Tanious et al. [ 48 ]. Briefly, these steps are as follows: hypothesis formulation and determination of the significance level and the number of measurements; determination of the randomization scheme; conduct of the experiment and calculation of the observed test statistic; and obtaining of the reference distribution and p -value.
To better understand the concept of randomization in SCEDs, it might be helpful to reconsider how randomization is used in group studies. In group studies, participants are assigned randomly to the different experimental conditions. In SCEDs, where one entity is exposed to all the levels of the independent variable(s), this is per definition not possible. Instead, measurement occasions are randomly assigned to the different levels of the independent variable(s) [ 18 , 49 ]. In research practice, however, oftentimes there are restrictions for this random assignment procedure of measurement occasions to treatments in SCEDs due to ethical or financial reasons. It might, for example, be unethical to withhold treatment from a patient with chronic pain just for the sake of adhering to the randomization scheme. Similarly, the duration of a study and the length of an intervention phase depend, in part, on the financial resources available. If financial resources are limited and the study duration is accordingly short, a fast introduction of the intervention is preferred, which places restrictions on the random assignment procedure. Additionally, the chosen SCED might place restrictions on the random assignment procedure. These restrictions will be discussed per type of SCED in subsequent sections.
4. Phase Designs
Phase designs consist of measurements taken in consecutive phases that implement different levels of the independent variable(s). In the terminology of phase designs, “A” stands for baseline measures, i.e., measurements of the dependent variable(s) without any manipulation of the independent variable(s), and “B” stands for experimental measures, i.e., measurements of the dependent variable with the manipulation(s) of the independent variable(s) in place [ 14 , 15 ]. According to the What Works Clearinghouse guidelines [ 50 ], three measurements per phase are required to meet the minimum evidence standards and five measurements per phase are recommended to meet evidence standards without reservation for all the designs under the phase category (see also [ 51 , 52 , 53 ]).
The most basic forms of phase designs are AB and ABA designs. In the former, initial baseline measurements are followed by measurements taken under the manipulation of the independent variable(s). In the latter, the intervention is withdrawn and the B-phase measurements are followed by a second A-phase. The ABA design is often also referred to as the withdrawal design [ 14 ]. While both of these designs are initially appealing due to their simplicity, they come with significant drawbacks. Guidelines on the conduct of SCEDs require at least three potential demonstrations of an effect to demonstrate experimental control over a dependent variable (e.g., [ 50 , 54 ]). An effect can be demonstrated with each phase change, and thus the AB design offers one potential demonstration, while the ABA design offers two potential demonstrations. A related concern is that, with few phase changes, any observed effect might coincide with external circumstances [ 50 ]. Ledford and Gast summarized the problems associated with the AB and ABA designs as follows:
- (a) “The ABA design is more useful than the basic AB design from an experimental perspective. However, you would not select this design at the outset to evaluate intervention effectiveness due to the practical and ethical considerations of terminating a study with a participant in baseline conditions. From a research perspective, if ethically defensible and practical, it would be more appropriate to expand to an ABAB design, thereby replicating the effect of the independent variable on the target behavior.” [ 15 ].
- (b) As Ledford and Gast pointed out, sometimes practical and ethical reasons render it impossible to implement more intricate designs [ 15 ]. Therefore, not to dismiss the AB design altogether, Michiels and Onghena [ 55 ] and Onghena et al. [ 56 ] discussed techniques for increasing the experimental validity of this design. These techniques include incorporating randomization into the design, colleting a sufficiently large number of data points, and replicating across participants.
- (c) As Ledford and Gast further explained, an ABAB design offers one more possibility of demonstrating the effect of an independent variable than an ABA design. With three potential demonstrations of an effect, the ABAB design is therefore the minimum phase design to meet the quality standards. If phase designs implement more than one distinct manipulations of the independent variable, each level is labeled with a distinct letter in alphabetical order. For example, in the ABACA design, two additional levels of the independent variable are present (B and C). It is also possible for two treatments to be administered within the same phase. The use of hyphens between each distinctive phase is then recommended to delineate the phases from one another. For example, in an A-B-A-BC design, intervention B is first administered separately and in the second experimental phase together with intervention C.
Figure 1 presents the results of an ABAB design used to investigate the effectiveness of occupational therapy with adults demonstrating agitation and post-traumatic amnesia following brain injury [ 57 ]. During the A-phases, subjects received daily standard occupational therapy, including systematic instruction, task adaptation, environmental modification, physical guidance, and facilitation. During the B-phases, daily occupational therapy was provided using the Perceive, Recall, Plan and Perform (PRPP) System approach. As Nott et al. explained, the PRPP System is a dynamic intervention process based upon all the stages of information processing [ 57 ]. During both phases, the subject’s information processing capacity was measured daily as a percentage score of task performance. Thus, a higher score indicates better information processing by the subject. Figure 1 shows the results of a 35-year-old female with diffuse axonal injury resulting in restlessness, excessive response to external stimuli, poor attention, and memory impairment.

Example of an ABAB design. Data from Nott et al. (2008) [ 57 ].
In phase designs, all the data are graphed as one continuous time-series, facilitating the observation of changes over time. Vertical dashed lines indicate phase changes. For all analyses in this paper, we look at the data aspect level, which may be operationalized as the mean score in a given phase [ 54 ]. A visual inspection of the means in each phase reveals that there is a noticeable difference in the level of the scores between A1 and B1. This change in level is not reverted when the intervention is withdrawn, even though the level of the scores in A2 is lower than in B1. When the intervention is introduced again (B2), there is a noticeable level change in score when compared to that in the preceding baseline phase (A2).
A randomization test can supplement this visual assessment with a quantification (test statistic) and information about the statistical significance of the effect ( p -value). The general null hypothesis is that the treatment is ineffective. The researchers expected that the intervention would lead to an increase in information processing. Therefore, we chose this as our one-sided alternative hypothesis. We chose the conventional significance level α of 0.05. To quantify the differences in level, we used the sum of B-phase means minus the sum of A-phase means as our test statistic [ 58 ], which can be written as: ( B ¯ 1 + B ¯ 2 ) − ( A ¯ 1 + A ¯ 2 ) . If a researcher expects the scores to be lower in the B-phases, ( A ¯ 1 + A ¯ 2 ) − ( B ¯ 1 + B ¯ 2 ) might be a more suitable test statistic. Two-sided tests can be performed by using the absolute difference between the sum of the B-phase means and the sum of the A-phase means written as: | ( A ¯ 1 + A ¯ 2 ) − ( B ¯ 1 + B ¯ 2 ) | .
In total, 24 measurements were taken. For the validity of the randomization test, it is important to assume that this had been determined a priori. In a phase design, an unrestricted randomization scheme, in which each measurement can either be A or B, is, per definition, not possible. Therefore, a restricted randomization scheme that takes the phase nature of the design into account should be chosen. Following guidelines on the conduct and analysis of SCEDs, we chose a restricted randomization scheme that allows for at least three measurements per phase. The number of possible ways of randomizing 24 measurements was calculated as: ( 24 − 3 ( 3 + 1 ) + 3 3 ) = 455, so that each of the four phases contains at least three measurements (cf. 58). Below is a non-exhaustive list of randomizations for illustrative purposes (the experiment, as it was carried out, is marked in bold):
- AAABBBAAABBBBBBBBBBBBBBB
- AAAABBBAAABBBBBBBBBBBBBB
- AAAAABBBAAABBBBBBBBBBBBB
- AAAAAABBBAAABBBBBBBBBBBB
- …
- AAAAAABBBBBBAAAAAABBBBBB
- AAAAAAAAAAABBBBBAAAABBBB
- AAAAAAAAAAAABBBBAAABBBBB.
The observed test statistic was calculated as: ( 63.67 + 85.67 ) − ( 29.67 + 54.33 ) = 65.33 , meaning that, on average, task performance by the subject increased by 65.33% during the intervention phases. How does this observed test statistic compare to the test statistics that would have been obtained with the other randomizations? To answer this question, we needed to locate the observed test statistic in the reference distribution of all the test statistics possible, given the randomization scheme (see Figure 2 ).

Reference distribution of test statistics for an ABAB design by Nott et al. [ 57 ].
The vertical red line indicates the observed test statistic. All the test statistics on this line or further to the right indicate test statistics at least as large as the observed one. In this case, none of the other randomizations would have led to a test statistic as high as or higher than the observed one. The p -value of a randomization test equals the number of test statistics as large as or larger than the observed one (when the expected effect is an increase in the dependent variable). Thus, for the data in Figure 2 , the p -value was calculated as: 1 455 = 0.002 , and we rejected the null hypothesis that the treatment is ineffective.
5. Alternation Designs
Contrary to phases designs, alternation designs do not consist of distinctive phases. Rather, as the name suggests, alternation designs rely on the fast alternation of treatments that are each associated with a distinct stimulus [ 25 ]. Another contrast to phase designs is that, in alternation designs, there is no requirement for a minimum number of measurements under the same condition to establish a steady pattern of responding before alternating the treatments [ 59 ]. In alternation designs, “A” refers to measurements taken under the first treatment or baseline and “B” refers to measurements taken under the second treatment. If more treatments are tested, each subsequent treatment is labeled with a distinct letter in alphabetical order. The completely randomized design is the simplest design in the alternation category [ 16 , 60 ]. In a completely randomized design, each measurement has an equal chance of being either A or B. As Edgington pointed out, this random assignment procedure is analogous to the random assignment of subjects to treatments in between-group studies [ 22 ]. While this design has a strong internal validity, a researcher might end up with an undesirable order of treatments [ 61 ]. For example, if a researcher conducts an experiment with 10 measurement occasions to test the effect of a novel exercise therapy for patients with arthritis, one of the possible randomizations would be AAAAABBBBB. Such a design has weak control over internal validity threats such as history and maturation as discussed in the section over AB designs. Alternating treatments designs and randomized block designs rule out such undesirable sequences. In an alternating treatments design, a limit is placed on the maximum number of measurements taken consecutively under the same condition. Recommended limits are two consecutive measures [ 27 , 28 ] or three consecutive measures [ 14 ]. In some research situations, however, it is not feasible or undesirable to administer the same treatment twice or three times in a row within a given timeframe. A randomized SCED that takes this constraint into account is the randomized block design. Edgington [ 22 ] gave an example of a randomized block SCED by referring to a study of Smith [ 62 ]. Smith tested the effectiveness of three different drugs to relieve narcoleptic symptoms over a period of 15 days. Smith divided the 15 days into five segments of three days. During each segment of three days, each drug was administered once and the order of administration was determined randomly with one drug per day. The logic of a randomized block SCED becomes clearer in comparison to the block logic in group studies. In group studies using a randomized block design, participants are allocated randomly to treatment blocks consisting of different conditions, whereas in a randomized block SCED treatments closely together in time are grouped into blocks and the order of treatment administration within each block is randomized [ 61 ].
Figure 3 presents the results of an alternating treatments design used to examine the effect of androgen supplementation in six healthy oral contraceptive users, who experience mood disturbances during regular oral contraceptive use [ 63 ]. Each study phase consisted of one menstrual cycle, during which the subjects continued their regular contraceptive use, supplemented either with a placebo (A-measurements) or with supplemental androgen (B-measurements). For both treatments, daily mood measures were taken with a single item question to be answered on a 5-point Likert scale, where 5 indicated a very positive mood. Figure 3 shows the results of a 23-year-old female who had been an oral contraceptive user for nine years prior to the study.

Example of an alternating treatment design. Data from Roumen et al. [ 63 ].
Whereas in phase designs the data for all treatments are plotted as one continuous time-series, in alternation designs each treatment is plotted as its own time-series. Each data point represents the mean mood score for that menstrual cycle. A visual inspection of Figure 3 reveals that two of the A-measurements are higher—indicating a better mood—than all B-measurements. This is contrary to the expected treatment effect.
For the randomization test, the null hypothesis and significance level remain the same as for the phase design. The alternative hypothesis is that the treatment leads to an increase in mood score. The researchers determined to take six measurements in total for each participant with each treatment for three menstrual cycles. The researchers further determined that the same treatment should not be administered for more than two consecutive menstrual cycles. With these constraints, there are 14 possible randomizations. Below is an exhaustive list of all possible randomizations for illustrative purposes (the experiment, as it was carried out, is marked in bold):
To quantify the difference between the A- and B-measurements, Roumen et al. subtracted the mean of the intervention measurements from the mean of the baseline measurements by using ( A ¯ − B ¯ ). The observed test statistic for the data in Figure 3 was calculated as: 3.97 − 3.89 = 0.08, meaning that, on average, the subject’s mood score was 0.08 higher on the 5-point Likert scale during the baseline measures. Figure 4 shows the reference distribution for the alternating treatments design.

Reference distribution of test statistics for an alternating treatment design by Roumen, et al. [ 63 ].
As the researchers expected the treatment to lead to an increase in mood scores, all the test statistics on the red line or to its left indicate randomizations that would have led to a higher treatment effect. There are 11 randomizations, for which the treatment effect is higher than the observed one. Thus, the p -value was calucated as: 11 14 = 0.79 . Accordingly, we did not reject the null hypothesis that the treatment is ineffective for this subject.
6. Multiple Baseline Design
Conceptually, multiple baseline designs are closely related to phase designs. The multiple baseline designs consist of a series of replicated AB designs. The term was coined by Baer et al. [ 29 ], “An alternative to the reversal technique may be called the ‘multiple baseline’ technique. This alternative may be of particular value when a behavior appears to be irreversible or when reversing the behavior is undesirable. In the multiple-baseline technique, a number of responses are identified and measured over time to provide baselines against which changes can be evaluated. With these baselines established, the experimenter then applies an experimental variable to one of the behaviors, produces a change in it, and perhaps notes little or no change in the other baselines.”
As Baer et al. [ 29 ] pointed out, there are situations, in which a change in a dependent variable is irreversible. For example, if a patient suffering from cardiac arrhythmia receives a pacemaker to reduce his/her feelings of dizziness, the pacemaker cannot simply be removed again to assess whether the feelings of dizziness increase again as a result (as is for example done in an ABAB design). In situations like that, the multiple baseline design provides a valid alternative. Baer et al. first defined the multiple baseline across outcomes design. However, replications in the multiple baseline design can also be established across participants or settings, and the intervention is introduced in a staggered way to the different units [ 64 ].
The staggered introduction of the intervention is an important feature for the validity of all variants of the multiple baseline design. Consider a novel therapy designed to reduce feelings of claustrophobia in a patient, who avoids crowds, narrow spaces, and windowless rooms. The staggered introduction of the intervention implies that, while the therapy is applied to the first setting (crowds), the other settings (narrow spaces and windowless rooms) remain in the baseline measures. When the therapy is applied to the second setting (narrow spaces), the third setting (windowless rooms) remains in the baseline measures. Finally, the intervention is introduced to the third setting (windowless rooms). If the feelings of claustrophobia decrease only in the setting to which the therapy is applied, and in the other settings that are in the baseline measures, no changes in feelings of claustrophobia are observed, then the multiple baseline design gives powerful evidence for the effectiveness of the therapy. In general, “the power of such designs comes from demonstrating that change occurs when, and only when, the intervention is directed at the behavior, setting, or subject in question” [ 14 ] (p. 202, emphasis in original).
Figure 5 shows the results of a multiple baseline design across participants used to investigate the effectiveness of video-based cognitive behavior therapy for treating eating disorders in five patients living far from urban centers [ 65 ]. During the baseline phases, subjects registered their daily eating patterns and symptoms of disordered eating. During the intervention phases, subjects received cognitive behavioral therapy sessions via a mobile video application. These sessions focused on establishing a regular meal schedule. Furthermore, subjects were encouraged to regularly record their weight, but these data were not recorded by the researchers. Figure 5 displays the number of daily meals consumed by the subjects, which Abrahamsson et al. [ 65 ] chose as the main outcome variable. Subjects self-recorded their daily eating frequency by means of a treatment-specific food diary. The researchers hypothesized that the treatment would lead to a higher frequency of daily meals, indicative of less binge eating.

Example of a multiple baseline design across cases. Data from Abrahamsson et al. [ 65 ].
In a multiple baseline design, each participant, outcome, or setting is plotted as its own time-series. For the first participant, the intervention was introduced on the 17th day; for the second participant, the intervention was introduced on the 19th day; for the third participant, the intervention was introduced on the 22nd day; for the fourth participant, the intervention was introduced on the 27th day; and for the fifth participant, the intervention was introduced on the 28th day. A visual inspection of the graphed data reveals that the magnitude of change after the introduction of the intervention differs between participants. For participants three, four, and five, the increases in level seem higher than for participants one and two.
The null hypothesis and the significance level remain the same as in the previous examples. The alternative hypothesis is that the video-based cognitive behavior therapy leads to a higher frequency of daily meals. A restricted randomization scheme for multiple baseline designs has to take into account the staggered introduction of the intervention across participants, meaning that the intervention cannot start on the same day for more than one participant. Abrahamsson et al. determined a priori that the total duration of the study is 55 days [ 65 ]. Furthermore, the researchers determined a priori that the moment of phase change from the baseline to the intervention would occur randomly for each participant between the 15th and 36th day. This randomization scheme was chosen so that each participant has a baseline length of at least two weeks and an intervention phase length of at least 20 days. For the chosen randomization scheme, there are 3,160,080 (calculated by 22 ! ( 22 − 5 ) ! ) randomizations that allow for a staggered introduction of the intervention. Below is a non-exhaustive list of baseline phase lengths per participant (the ones actually used in the experiment is marked in bold):
- 14,15,16,18,30
- 16,18,21,26,27
- 18,19,21,22,28
- 22,24,28,30,34
- …
- 26,29,30,32,35
- 28,29,30,31,32
- 29,30,31,32,33
- 31,32,33,34,35.
To quantify the intervention effectiveness, we choose the mean difference between the intervention and the baseline measurements as our test statistic, which can be calculated by ( B ¯ 1 + B ¯ 2 + B ¯ 3 + B ¯ 4 + B ¯ 5 ) − ( A ¯ 1 + A ¯ 2 + A ¯ 3 + A ¯ 4 + A ¯ 5 ) 5 . The observed test statistic for the data displayed in Figure 5 was calculated as: ( 3.59 + 3.46 + 3.68 + 4.97 + 5.5 ) − ( 2.81 + 2.67 + 1.86 + 2.85 + 2.96 ) 5 = 1.61 , meaning that the intervention leads, on average, to an increase of 1.61 in the frequency of daily meals. Given the large number of possible randomizations, it is computationally not feasible to locate the observed test statistic in the reference distribution of all possible randomizations. Therefore, Abrahamsson et al. used a Monte Carlo random sampling procedure [ 65 ]. This procedure takes a random sample of 1000 randomizations based on all permissible randomizations. Figure 6 shows how the observed test statistic compared to the test statistics that would have been obtained by the other 999 randomizations.

Reference distribution of test statistics for a multiple baseline design i [ 65 ].
Two randomizations would have led to a test statistic as large as the observed one or even larger. The p -value thus equals 0.002 , calculate by 2 1000 , and we can reject the null hypothesis that the treatment is ineffective.
7. Changing Criterion Design
The last category in the typology of SCEDs is the changing criterion design. This design was first introduced and demonstrated by Hartmann and Hall [ 31 ]. In the changing criterion design, a criterion that the subject has to meet is set. This criterion changes constantly between adjacent phases to systematically decrease or increase the frequency of the dependent variable. After the initial baseline measures, treatment is never withdrawn [ 43 ]. Barlow et al. argued that the lack of treatment withdrawal throughout the course of the study is a major strength of the changing criterion design and that this feature makes the design especially attractive for clinical studies, for example in the treatment of dangerous behaviors such as self-harming [ 14 ]. Klein et al. elaborated that the changing criterion design is especially valuable in situations, in which an immediate, abrupt increase or decrease in a dependent variable may be difficult to achieve or undesirable [ 32 ]. Furthermore, the stepwise changes in the frequency of the dependent variable may facilitate habitual changes in a subject’s behavior [ 66 ]. In their seminal paper, Hartmann and Hall conducted two experiments using the changing criterion design. In the first study, Hartmann and Hall used a reward strategy to increase the number of math problems correctly solved by a behaviorally disordered boy. In the second study, a financial incentive strategy was used to stepwise reduce the number of cigarettes smoked by a heavy smoker. Hartmann and Hall [ 31 ] emphasized several important factors to ensure a valid implementation of the changing criterion design: “Successful implementation of the changing criterion design requires particular attention to three design factors: length of baseline and treatment phases, magnitude of changes in the criterion, and number of treatment phases or changes in criterion. All phases should be long enough to ensure that successive changes in a therapeutic direction are not naturally occurring due to either historical, maturational, or measurement factors (see Campbell and Stanley, 1963). In addition, treatment phases should differ in length, or if of a constant length, should be preceded by a baseline phase longer than each of the separate treatment phases. This is to ensure that stepwise changes in the rate of the target behavior are not occurring naturally in synchrony with criterion changes” (p. 530).
Klein et al. recommended incorporating “mini-reversals” into the changing criterion design [ 32 ]. Such a reversal entails reverting to a previous criterion. For example, if the daily caloric intake for an obese person has been reduced by 200 and 400 calories per day in the first two phases compared to mean caloric intake during the baseline measures, a mini-reversal would entail going back to the 200 calories phase. In actual research practice, such reversals depend, of course, on ethical and practical considerations. If ethically and practically feasible, such reversals can greatly strengthen the demonstration of experimental control over the dependent variable. Klein et al. further recommended that at least three criterion changes should be implemented for repeated observation of intervention effectiveness [ 32 ]. Regarding the minimum number of data points required per phase, clear guidelines are still lacking for the changing criterion design. Given the phase structure of the changing criterion design, a reasonable recommendation might be at least three and preferably five data points. The effectiveness of an intervention is demonstrated with a changing criterion design, when the dependent variable consistently changes to criterion levels set by the researcher [ 66 ]. In the range-bound version of the changing criterion design [ 33 ], the researcher sets a range of acceptable occurrences of the dependent variable instead of a single criterion. Barker et al. gave an example of an injured athlete in rehabilitation [ 43 ]. To prevent overtraining and the likelihood of reinjury, it may be useful to place an upper limit on the number of training sessions per week. Similarly, to prevent stagnation in the rehabilitation process, it may be useful to set a lower limit on the acceptable number of weekly training sessions. If the athlete trains no more than the upper limit criterion and no less than the lower limit criterion, then the intervention leads to an acceptable amount of exercising. Thus, the only difference between the classical changing criterion design and the range-bound changing criterion design is that in the former a single-point criterion is set that has to be met by the participant while in the latter an acceptable range is specified.
Figure 7 shows the results of a changing criterion design using a mindfulness-based health wellness program to reduce the weight of a morbidly obese man [ 67 ]. After an initial baseline phase of 12 weeks, during which the subject’s weight was recorded, the intervention was introduced consisting of physical exercise, a food awareness program, mindful eating to manage rapid eating, visualizing and labeling hunger, and a mindfulness procedure as a self-control strategy. Adherence to the physical exercise program resulted in a reward in the form of purchasing an item from the subject’s wish list of reinforcers. During the baseline and all the intervention phases, the subject’s weight was recorded weekly. After the baseline phases, the criterion for each consecutive phase was to lose five pounds. The criterion changed after three successful measures (i.e., the subject’s weight equaled or was below the criterion). Due to the nature of the experiment, ethical considerations rendered it impossible to incorporate a reversal. Neither did the researchers vary the magnitude of criterion changes between phases in consideration with the patient to keep him motivated. In total, the researchers applied the intervention for 270 weeks. To be able to present all the data in a single time-series graph, Figure 7 only shows the data for the first 95 weeks.

Example of a changing criterion design. The red horizontal lines indicate the criterion in each phase. Data from Singh et al. [ 67 ].
Similar to the phase designs, all the data in a changing criterion design are plotted as a single continuous time-series. It is customary to label each phase chronologically in alphabetical order. A visual inspection of the graphed data reveals that the participant lost weight continuously during the course of the experiment. However, it can also be seen that there are several data points in each phase that clearly deviate from the criterion.
Specific randomization schemes for changing criterion designs have only recently been proposed by Onghena et al. [ 7 ] and Ferron et al. [ 68 ]. Similar to phase designs and multiple baseline designs, a changing criterion design is not eligible for an unrestricted randomization scheme. The specific structure of the changing criterion design has to be taken into account when determining the randomization scheme. The specific structure of the changing criterion design needs to be preserved with its successive phases and criteria when constructing the reference distribution [ 7 ]. Another factor that has to be taken into account is that Singh et al. determined that the subject had to record the criterion weight for three weeks before changing the criterion and moving to the next phase [ 67 ].
To introduce an element of randomization, we constructed a randomization scheme under the assumption that the researchers determined a priori that the phase change occurs randomly within the next two weeks after the criterion weight had been recorded for at least three consecutive weeks. This leaves us with two possible phase change moments per phase: weeks 19 and 20 in phase B, weeks 26 and 27 in phase C, and so on. There are 256 (calculated by 2 8 ) possibilities to assign the eight phase change moments in this way.
However, this does not take into account the baseline phase as there is no criterion present in the baseline phase. The different possibilities for incorporating the baseline phase in the randomization procedure and calculation of the test statistic are discussed in Onghena et al. [ 7 ]. One possibility would be to drop the baseline measures. As this would result in a loss of possibly valuable information, we do not recommend this option. Another option would be to select a score based on the subject’s characteristics. For example, the subject recorded a weight of 308 pounds when entering the study. One might argue that this would be a sensible criterion under the assumption that the subject will not gain weight. However, this does not take into account how the data pattern in the baseline phase evolves over time and basing a criterion on a single data point seems arbitrary given that this data point might be an outlier. Therefore, we followed the recommendation to take the median value of the baseline phase (311 lbs.) as a criterion [ 7 ].
Still, the question—which possible phase change moments to identify for the change from the A- to the B-phase—remains. Given that there are two possible phase change moments in the other phases, we might follow the same logic for the baseline phase, so that in total there are 512 (calculated by 2 9 ) randomizations. If Singh et al. had incorporated an element of randomization in the planning phase of the experiment, the B-phase might have started randomly after at least five weeks of baseline measures (cf. earlier discussion on the minimum phase length required to meet the evidence standards). Below is a non-exhaustive list of the 512 possible phase change moments for illustrative purposes (the experiment that has actually been carried out is marked in bold):
- 12, 19, 26, 36, 44, 54, 64, 77, 85
- 12, 19, 26, 37, 44, 55, 64, 77, 85
- 12, 20, 26, 36, 44, 54, 64, 77, 85
- …
- 13, 20, 26, 37, 44, 54, 64, 77, 86
- 13, 20, 27, 36, 45, 54, 65, 77, 86
- 13, 20, 27, 37, 45, 55, 65, 78, 86 .
To quantify the intervention effectiveness, we chose the mean absolute deviation as a test statistic [ 7 ]. The mean absolute deviation equals the sum of the absolute differences between each individual data point and the criterion within that phase divided by the total number of data points N, which can be written as: ∑ | C i − m i j | N , where C i stands for the criterion in phase i and m i stands for the jth measurement in phase i. The observed test statistic for the data in Figure 7 equals 2.11, meaning that, on average, the subject’s recorded weight deviated 2.11 lbs. from the criterion. For the mean absolute deviation, lower scores indicate a better match between the scores and the criteria. Thus, a score of zero for the absolute mean deviation would indicate a perfect match between the scores and the criterion for all the measurements. Figure 8 shows the distribution of test statistics for all the possible randomizations.

Reference distribution of test statistics for a changing criterion design by Singh et al. [ 67 ].
Since for the mean absolute deviance smaller values indicate better adherence to the criterion, we had to look at the left tail of the distribution to calculate the p -value. There are 511 randomizations in the distribution that would have led to a lower mean absolute deviance. The p -value thus equals 0.998 (calculated by 511 512 ), and we did not reject the null hypothesis that the treatment is ineffective.
8. Discussion
In the present paper, we showed how randomized SCEDs can be utilized in healthcare research to find individually tailored interventions. For each design type of SCEDs, we presented published studies from the healthcare literature, illustrated how an element of randomization can be incorporated, and how the obtained data can be analyzed by means of visual analysis, effect size measures, and randomization tests. We put the emphasis on the randomization tests because they are a flexible and versatile data analysis technique that can be adapted to many situations encountered in applied research. This emphasis on the randomization tests, however, does not mean that the obtained p -value is an all-or-nothing indicator of intervention effectiveness. Visual analysis and effect size calculation, as well as qualitative data, should be considered when judging the success of an intervention for the patient. For example, the p -value for the changing criterion design example was nearly 1. At the same time, visual analysis indicated that the patient continuously lost weight throughout the course of the experiment. The patient deviated on average only a bit over 2 lbs. from the criteria. Even though the randomization test indicated a non-significant treatment effect, the weight loss of the patient throughout the experiment can increase his quality of life and overall health. Therefore, we always recommend an integrated approach to analyzing data obtained through SCEDs.
It should be noted that some of the example data sets used in this paper did not incorporate an element of randomization in the planning phase of the study. The analysis of the changing criterion design in particular was loaded with heavy assumptions that were not met in the actual design of the study and the randomization test was calculated only on a subset of data from the original study. However, at the same time, this enabled us to illustrate a possible randomization procedure for the changing criterion design if the researchers incorporated an element of randomization in the planning phase of the study. The randomization tests for the changing criterion design and the ABAB design were therefore carried out under the assumptions that an element of randomization was incorporated a priori and that the experiments as they were carried out were actually chosen at random from all possible randomizations. Alternative ways of analyzing SCED data by means of masked graphs have been proposed for situations, in which the randomization procedure has not (entirely) been determined a priori [ 69 , 70 ]. If the results are analyzed by means of a randomization test when the randomization assumption has not been met, the Type I error might deviate from the predetermined α [ 71 ].
An important consideration when conducting any kind of significance testing is the power of the test to detect a treatment effect. The power of randomization tests for phase designs varies as a function of both the number of phases and the number of observations per phase [ 72 ]. For multiple baseline designs, it has been found that the power of randomization tests depends, among other things, on the between-case stagger separation, meaning that if the introduction of the intervention is further apart from one case to the next, the power increases [ 30 ]. Another important factor in the power of randomization tests for multiple baseline designs is the number of cases, behaviors, or settings under investigation. The power increases considerably when comparing at least three different cases, behaviors, or settings with at least 20 measurements each [ 18 ]. For the alternating treatments designs, the power of randomization tests depends largely on the number of observations and the number of permissible successive observations under the same treatment [ 18 ]. Research on the specific factors influencing the power of randomization tests for the changing criterion designs is still needed. For SCED randomization tests in general, the lowest possible p -value is the inverse of the number of possible randomizations. For the alternating treatments design example, the lowest possible p -value was: 1 14 = 0.07 . When less than 20 randomizations are possible, a randomization test has zero power at a conventional level α of 0.05 [ 73 ]. Conversely, as was the case in the multiple baseline design example, the number of possible randomizations can be so high that it becomes computationally unfeasible to calculate the exact p -value. As shown, in such cases, a Monte Carlo random sampling procedure can be employed to approximate the exact p -value.
Another important consideration in the analysis of SCED data is the choice of data aspects that are of interest to the researcher. Widely accepted guidelines regarding the conduct and analysis of SCEDs recommend inspecting six data aspects: level, trend, variability, overlap, immediacy of the effect, and consistency of data patterns [ 50 ]. In the present paper, we focused only on the data aspect level for illustrative purposes. A procedure for assessing all the six data aspects simultaneously through multiple randomization tests has been proposed by Tanious et al. [ 48 ]. We do not recommend isolating one data aspect and base a conclusion regarding the effectiveness of an intervention on that data aspect alone. A user-friendly web-based application, where the analyses for phase designs, multiple baseline designs, and alternation designs can be executed, is available at https://tamalkd.shinyapps.io/scda/ [ 74 ]. Generic R-code for analyzing changing criterion designs is available in [ 7 ]. Further discussion of randomization tests for phase and alternation designs and available software for analyzing these designs are available in a study of Heyvaert and Onghena [ 75 ].
9. Conclusions
Randomized SCEDs are valid alternatives to large-group studies for applied healthcare professionals, especially when patient symptoms are highly idiosyncratic in nature. Randomization tests allow for powerful inferences regarding treatment effectiveness based on the random assignment procedure actually used in the experiment.
Author Contributions
Conceptualization, R.T. and P.O.; formal analysis, R.T.; project administration, P.O.; software, R.T. and P.O.; supervision, P.O.; visualization, R.T.; writing of an original draft, R.T.; writing of review and editing, P.O.
This research received no external funding.
Conflicts of Interest
The authors declare no conflicts of interest.
Leveraging Single-Case Experimental Designs to Promote Personalized Psychological Treatment: Step-by-Step Implementation Protocol with Stakeholder Involvement of an Outpatient Clinic for Personalized Psychotherapy
- Original Article
- Open access
- Published: 11 March 2024
Cite this article
You have full access to this open access article
- Saskia Scholten ORCID: orcid.org/0000-0002-1439-8684 1 ,
- Lea Schemer ORCID: orcid.org/0000-0001-7830-4889 1 ,
- Philipp Herzog ORCID: orcid.org/0000-0002-8267-2083 1 , 2 ,
- Julia W. Haas ORCID: orcid.org/0000-0002-6501-0221 1 ,
- Jens Heider 1 ,
- Dorina Winter ORCID: orcid.org/0000-0002-5096-7995 1 ,
- Dorota Reis ORCID: orcid.org/0000-0002-0027-9713 3 &
- Julia Anna Glombiewski 1
222 Accesses
5 Altmetric
Explore all metrics
Our objective is to implement a single-case experimental design (SCED) infrastructure in combination with experience-sampling methods (ESM) into the standard diagnostic procedure of a German outpatient research and training clinic. Building on the idea of routine outcome monitoring, the SCED infrastructure introduces intensive longitudinal data collection, individual effectiveness measures, and the opportunity for systematic manipulation to push personalization efforts further. It aims to empower psychotherapists and patients to evaluate their own treatment ( idiographic perspective ) and to enable researchers to analyze open questions of personalized psychotherapy ( nomothetic perspective) . Organized around the principles of agile research, we plan to develop, implement, and evaluate the SCED infrastructure in six successive studies with continuous stakeholder involvement: In the project development phase, the business model for the SCED infrastructure is developed that describes its vision in consideration of the context (Study 1). Also, the infrastructure's prototype is specified, encompassing the SCED procedure, ESM protocol, and ESM survey (Study 2 and 3). During the optimization phase, feasibility and acceptability are tested and the infrastructure is adapted accordingly (Study 4). The evaluation phase includes a pilot implementation study to assess implementation outcomes (Study 5), followed by actual implementation using a within-institution A-B design (Study 6). The sustainability phase involves continuous monitoring and improvement. We discuss to what extent the generated data could be used to address current questions of personalized psychotherapy research. Anticipated barriers and limitations during the implementation processes are outlined.
Similar content being viewed by others
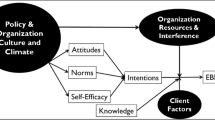
Assessing Causal Pathways and Targets of Implementation Variability for EBP use (Project ACTIVE): a study protocol
Emily M. Becker-Haimes, David S. Mandell, … Torrey A. Creed
The Importance of Stepped-Care Protocols for the Redesign of Behavioral Health Care in Patient-Centered Medical Homes
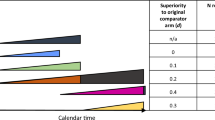
Using the ‘Leapfrog’ Design as a Simple Form of Adaptive Platform Trial to Develop, Test, and Implement Treatment Personalization Methods in Routine Practice
Simon E. Blackwell
Avoid common mistakes on your manuscript.
Introduction
Clinicians face the challenge that they work with individuals, while most research is still conducted on the group level. Nomothetic research was intended to identify general psychological processes (Allport, 1937 ). However, even relatively homogenous groups are composed of heterogeneous individuals. Trying to understand intraindividual variation inferring from results based on interindividual differences may lead to invalid conclusions (Molenaar, 2004 ). In psychotherapy research, heterogeneity of treatment effects (Varadhan et al., 2013 ) indicates that patients respond differently to psychological treatments even if they show similar symptoms and are treated by the same therapist with similar methods (Kiesler, 1966 ). Recently, meta-analyses empirically demonstrated the considerable heterogeneity in treatment effects for several mental disorders such as depression (Kaiser et al., 2022a , 2022b ), posttraumatic stress disorder (Herzog & Kaiser, 2022 ) and borderline personality disorder (Kaiser & Herzog, 2023 ) with small to medium effects on average depending on the mental disorder studied. These findings underly the need for optimization efforts to maximize treatment outcomes of the individual patient. In this regard and analogous to personalized medicine (Simon & Perlis, 2010 ), personalizing psychological treatments to the individual patient might be a promising approach to achieve this goal (Chekroud et al., 2021 ).
Personalized Treatment Approaches
Personalized psychological treatments aim at investigating the dynamics in psychopathology (Fisher, 2015 ) and mechanisms of change within an individual patient over the course of treatment (Altman et al., 2020 ). Generally speaking, mental disorders are considered complex systems of contextualized dynamic processes that are specific to the individual and need to be considered in deriving personalized treatment decisions and recommendations (Wright & Woods, 2020 ).
Personalized treatment approaches can be based on intuitive, theoretical, data-informed, or data-driven models (Cohen et al., 2021 ). Sources of information for clinical decision-making are intuition and anecdotes for intuitive models, and theory and concepts for theoretical models, respectively (Cohen et al., 2021 ). Yet, the resulting clinical judgements may be error-prone due to several clinician biases such as representativeness heuristic, selection, and confirmation biases, as well as overoptimism (Lutz et al., 2022 ). In contrast, data-informed and data-driven models are based on evidence or statistical algorithms (Cohen et al., 2021 ). One data-based line of research led to the development of empirically derived decision-support tools such as systematic routine outcome monitoring (Lutz et al., 2022 ). Objective feedback allows individuals to form accurate pattern-recognition abilities (Kahneman & Klein, 2009 ). Another line of research comprises treatment selection procedures based on clinical prediction models. They aim to achieve a better match between the individual and the treatment received (Cohen & DeRubeis, 2018 ). Both approaches are highly valuable to address clinician biases, as a complementary tool, and could yield a potential to increase treatment outcomes. Limitations to these data-informed approaches include that many studies are underpowered or lack hold-out samples and are therefore likely to overestimate effects of personalized treatment (Lorenzo-Luaces et al., 2021 ). In addition, routine outcome monitoring is currently observational, raising questions about the internal validity, and thus limiting the causal inferences (Kaiser et al., 2023 ). Prediction models are also limited due to their reliance on group level information and large datasets which are not feasible to collect in routine clinical care and private practices.
To push personalization efforts even further, clinician scientists or scientific practitioners need to be empowered to implement successful personalized psychological treatment (Piccirillo & Rodebaugh, 2019 ). An experimental setting within general practice that focuses on the individual patient is needed (Howard et al., 1996 ). New technologies, such as experience sampling methods, wearables, open-source software for analyzing time-series data, and more advanced statistical models could assist the integration of individual level designs into clinical practice (Piccirillo & Rodebaugh, 2019 ).
Promoting Idiographic Research with Single-Case Designs
Single-case experimental designs (SCEDs Footnote 1 ) are time- and cost-efficient, yet methodologically sound, prospective idiographic research designs (Nikles et al., 2021 ) that could be particularly promising to be implemented in routine clinical care (Schemer et al., 2022 ). Per definition, SCEDs have the following key features: (1) One entity (e.g., a person) that serves as its own control, (2) is observed repeatedly during a certain period of time (for example via experience sampling methodology, ESM Footnote 2 ), and (3) under systematic manipulation (e.g., introduction of a psychological treatment) (Vlaeyen et al., 2022 ). SCEDs seek to estimate meaningful treatment effects for individual patients while safeguarding internal validity and causal inference (Kazdin, 2019 ; Tanious & Onghena, 2019 ; Vlaeyen et al., 2020 ). In combination with ESM, the methodological rigor of SCEDs can be further increased (Schemer et al., 2022 ). Graphical examples of SCEDs are presented as Supplemental Material (S1 Design illustrations, https://osf.io/dkytg ).
SCEDs, especially in combination with ESM, are considered powerful and adaptive research designs for routine clinical care that could reduce the research-practice gap (Bentley et al., 2019 ; Berg et al., 2023 ; Kravitz et al., 2014 ). Both methodological approaches bring different advantages. ESM has shown to support clinical care usefully (e.g., problematic cannabis use: Piccirillo et al., 2023 ) . In particular, both researchers and practitioners highlight that ESM has an incremental value for mental health care, with the assessment of context specificity of symptoms as the most useful part (Piot et al., 2022 ). SCEDs on the other hand allow to evaluate the efficacy of existing interventions or intervention packages for a particular patient in clinical practice and to easily pilot novel interventions or modifications of known interventions (Krasny-Pacini & Evans, 2018 ; Selker et al., 2022 ).
Existing evidence indicates that 79% of participating patients found n-of-1 trials useful (Kaplan & Gabler, 2014 ) with high perceived system usability (Kravitz et al., 2020 ). In a medical context, SCEDs are used for example to study the ideal dose of melatonin for sleep disturbance in Parkinson's disease in a way that individual study participants also gained valuable insights (Nikles et al., 2019 ). Likewise, SCEDs are also particularly suitable for treating mental health problems lacking (adequate) evidence for the efficacy of specific psychological treatments (Duan et al., 2013 ). Due to high comorbidities and the fact that individuals are not alike, it is often the case that existing protocols are not suitable for patients in routine clinical practice (Berg et al., 2023 ). By quickly identifying ineffective psychological treatments, SCEDs can help to reduce mental health care costs (Kravitz et al., 2014 ). In addition, patients may be involved more closely in treatment planning through self-monitoring (Kravitz et al., 2014 ) and shared decision-making is encouraged through continuous discussion (Riese et al., 2021 ). The result is a patient-centered, genuine learning system in mental health care (Selker et al., 2022 ).
In practice, this means that the effects of specific interventions carried out by a practitioner on a particular patient could be evaluated throughout the ongoing treatment (mind: Paul, 1967 ). A SCED infrastructure allows to set up research studies more easily. For example, a multiple baseline design could be implemented to evaluate isolated intervention strategies (Schemer et al., 2018 ). Collected data could then be analyzed on an individual level (e.g., single-case randomization tests) and on a group level (e.g., single-case meta-analysis) (Heyvaert & Onghena, 2014 ).
Our ultimate objective is to establish an Outpatient Clinic for Personalized Psychotherapy within a German outpatient research and training center. Its key component is a SCED infrastructure creating an experimental setting centered on individual patients within routine clinical care serving the following aims:
Idiographic perspective: Enriching current diagnostic procedures mainly comprising clinical interviews and questionnaires by ESM methods that allow continuous monitoring of relevant psychological processes.
Causal inference: Facilitating systematic manipulation going beyond current standards of routine outcome monitoring systems to find effective treatments for challenging or non-responsive patients and to optimize new psychological treatments for future studies.
Nomothetic perspective: Over time, as data accumulates, large numbers of individual intensive-longitudinal datasets could be used for data-driven personalization efforts.
To achieve these long-term goals, we plan to develop, implement, and evaluate an SCED infrastructure following agile research principles (Wilson et al., 2018 a). The current paper serves as a study protocol for this step-by-step implementation process. The infrastructure serves two areas of application: routine clinical care and future research projects. While this study protocol outlines the planned procedure in routine clinical care, future research projects may adapt their methodological approaches. Unlike typical implementation research focused on effective interventions (Pinnock et al., 2017 ), we plan to implement a methodological infrastructure. Reporting standards for implementation studies recommend a twofold reporting strategy always reporting on the implementation strategy (e.g., training of therapists) and the intervention (e.g., exposure therapy). Yet, the SCED infrastructure is not seen as an intervention. Therefore, we focus on typical implementation outcomes such as feasibility, acceptability, and sustainability of the infrastructure (Proctor et al., 2011 ) as oppose to its effect on clinical outcomes.
Methods and Analysis
To promote an open research culture (Nosek et al., 2015 ), an Open Science Framework (OSF) project is created to bundle the projects that will be realized using the SCED infrastructure, including respective pre-registrations using the SCED infrastructure, research materials, and preprints ( https://osf.io/yex48/ ).
The SCED infrastructure will be implemented in the outpatient clinic of the RPTU Kaiserslautern-Landau in Germany. The outpatient clinic is part of a project for coordinating research efforts in German university outpatient clinics for psychotherapy that guides current standard diagnostic procedures (Velten et al., 2017 ). Standard diagnostic procedures comprise up to four diagnostic sessions and include an intake interview by a licensed psychotherapist, further clinical interviews and questionnaires. The Symptom Checklist 90 (SCL-90, Derogatis & Unger, 2010 ; Franke, 2014 ) together with symptom-specific questionnaires is administered at intake, during the diagnostic sessions, during treatment (every 10th session), at posttreatment and at 6-month follow-up.
Around 800 adults with mental disorders are treated per year. Eighty therapists in training and 18 licensed psychotherapists deliver about 45 treatments per day. In Germany, public health insurance usually covers short-term treatment of 24 sessions, which can be extended to up to 80 sessions in total, depending on the mental state of patients. In addition, therapies are implemented within the framework of third-party funded and self-funded psychotherapy studies. The treatment delivered is Cognitive Behavioral Therapy (CBT). The outpatient clinic is a facility that teaches bachelor's graduates who are pursuing their master's degree in psychotherapy and master's graduates who are training to become licensed psychotherapists with a special emphasis on CBT. Adherence to standard CBT methods is safeguarded by regular supervision. Bachelor graduates receive intensive one-on-one supervision by a licensed psychotherapist, who could directly intervene in the case of an emergency, while working with patients. Master graduates receive regular group and individual supervision by a licensed psychotherapist with more than five years of work experience.
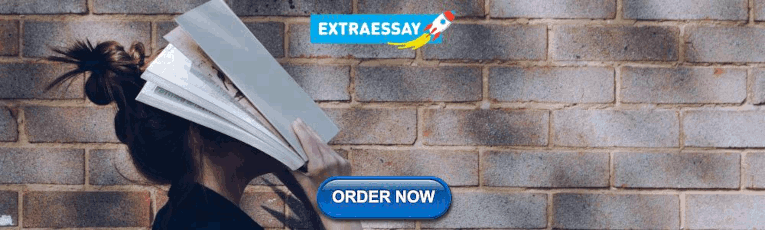
Identification of Stakeholders
The following stakeholders have to be considered during the implementation process (Eslick & Sim, 2014 ; Krasny-Pacini & Evans, 2018 ; Selker et al., 2022 ): Patients (and their family members, caregivers…) have to be able to easily enter and access their own data and to view and interpret their results. Therapists (and their supervisors) should be able to recruit and manage a sample of patients, to set up their own SCDs, monitor the data collection progress, intervene if needed, and to view and interpret results. Clinical researchers should be able to create and implement SCEDs, intervene if needed, capture deviations and adjust the protocol, and assess fidelity measures and interrater-reliability. The administrative team provides institutional oversight and management (e.g., creating user accounts). System administrators and developers support the operation of the IT system, provide user tech support, and maintain and problem-solve the operational code. The statistician reviews the trial design and collected data for validity and/or aggregate analysis, download identified or de-identified data for offline analysis, and runs the analysis. Other potentially relevant stakeholders are healthcare payers, healthcare delivery systems, and regulatory agencies. In addition, experts in the field form the scientific advisory board that supervises the implementation of the Outpatient Clinic for Personalized Psychotherapy and training staff of the psychotherapy training consider SCD and m-Path in the curriculum to enable future therapists to easily use the methodology. Stakeholders and their key functions for the SCED infrastructure are summarized in the Supplemental Material (S2 Stakeholder identification, https://osf.io/z4uf5 ).
Implementation Strategy: Step-by-Step Implementation with Stakeholder Involvement
A successful implementation of the SCED infrastructure needs to emphasize the context where the SCED infrastructure is introduced (Bauer & Kirchner, 2020 ). Implementation strategies are methods or techniques used to enhance the adoption, sustainability, and scaling up (Powell et al., 2015 ; Proctor et al., 2013 ). Discrete implementation strategies involve single approaches or techniques, while the complexity of implementing clinical innovations often necessitates multifaceted strategies that combine two or more of these discrete strategies (Kirchner et al., 2020 a). Implementation strategies should be considered from the outset of the planned research (Pinnock et al., 2017 ), even though they are iterative by nature and need to be adapted when facing unexpected challenges (Kirchner et al., 2020a ). We plan to use a step-by-step implementation with stakeholder involvement as multifaceted implementation strategy (compare “stage implementation scale up” and “using advisory boards and workgroups” as recommended implementation strategies by the Expert Recommendations for Implementing Change (ERIC) project; Powell et al., 2015 ).
Following the recommendations of agile research (Bartels et al., 2022 ; Wilson et al., 2018 ), different steps in the implementation process can inform and potentially modify subsequent steps (Fig. 1 ): In the project identification phase , the SCED infrastructure is envisioned (see Objectives) while acknowledging the context of the SCED infrastructure (see Setting and Stakeholder Identification). It is dedicated to plan the step-by-step implementation and to describe it in this study protocol. In the project development phase, a prototype of the infrastructure is specified. This includes finalizing a business model in consideration of the context (Study 1), the SCED procedure, ESM protocol and ESM survey (Study 2 and 3). In the optimization phase , feasibility and acceptability are tested and the infrastructure is customized accordingly (Study 4). The evaluation phase includes a pilot implementation study to assess implementation outcomes (Study 5) and the actual implementation using a within-institutional A-B design to evaluate the implementation (Study 6). In the sustainability phase, the application is continuously monitored and improved regarding relevance, safety, and effectiveness. Throughout the entire process, we will establish and consult an advisory board consisting of international experts in SCEDs that meets twice a year to guide project decision making.
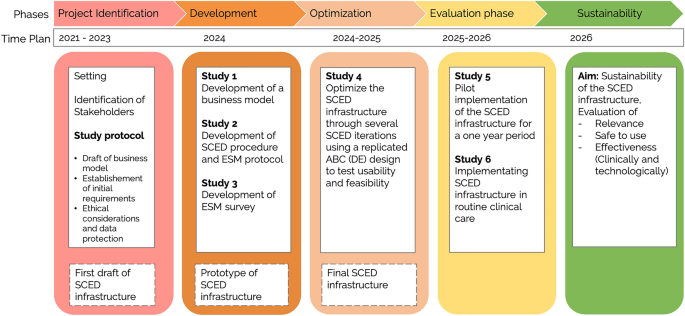
Step-by-step implementation process
Stakeholders will serve as research partners (Anampa-Guzmán et al., 2022 ) who will be consulted at several stages through various methods (e.g., via stakeholder meetings and interviews) to develop, optimize, and implement the SCED infrastructure. This involvement provides stakeholders with the opportunity to express what their group needs during the implementation process (such as special trainings or workshops). As outlined above, we consider different types of stakeholders: patients, therapists, supervisors, and clinical researchers as users of the SCED infrastructure; statisticians, administrative staff, and developers as user support (e.g., administrators, developers), and the scientific advisory board and our training staff as collaborators . Stakeholders are engaged using various methods (see separate pre-registrations for detailed descriptions of the respective methods) such as the World Café Method (Brown & Isaacs, 2005 ; Schiele et al., 2022 ), stakeholder meetings (Doria et al., 2018 ), cognitive interviews (Beatty & Willis, 2007 ), and semi-structural interviews (Domecq et al., 2014 ). Involvement will be evaluated using the Public and Patient Engagement Evaluation Tool (PPEET) (Abelson et al., 2016 ) and reported according to the GRIPP2 checklist (Staniszewska et al., 2017 ).
Study 1: Exploring Barriers and Facilitators to Develop a Business Model
The aim of this study is (1) to explore barriers and facilitators regarding the prospective implementation process and (2) to develop a business model that enables sustainable implementation. For this purpose, a preliminary business model is being formulated, initial technical requirements are being considered, and ethical and privacy issues are being assessed to identify potential questions and challenges. We will conduct a World Café (Brown & Isaacs, 2005 ; Schiele et al., 2022 ) at least three persons of all relevant stakeholder groups (patients, therapists, supervisors, researchers) to identify further questions and challenges. In qualitative research, information saturation in interview studies is reached after 6–15 interviews (Guest et al., 2006 ; Turner-Bowker et al., 2018 ). Because recommendations for group sizes in World Cafés are missing, we aimed at a group size of 12 persons based on this information. The World Café is followed by in-depth stakeholder interviews with key experts for specific questions (e.g., regarding data protection, psychotherapy training) to develop a business model that addresses these issues.
Draft of the Business Model
The business model is intended to describe how the Outpatient Clinic for Personalized Psychotherapy will create, deliver, and capture value (Osterwalder et al., 2010 ). Business models have a long tradition in entrepreneurial practice and have been recommended for clinical trials (McDonald et al., 2011 ) and health care service planning (Sibalija et al., 2021 ). Developing a business model obliges to think through economic, operational, and strategic aspects that will lead to sustainable benefits in defined markets such as health care services (Morris et al., 2005 ). Figure 2 shows the first draft of the business model we developed for the Outpatient Clinic for Personalized Psychotherapy following Osterwalder and colleagues' nine building blocks ( 2010 ). Subsequently, we will outline each block and highlight questions and challenges (Q&C) that need to be clarified in the development phase:
The Outpatient Clinic for Personalized Psychotherapy aims to reach and serve patients with mental health conditions and their family members, therapists in training and licensed therapists, their supervisors, and clinical researchers (called “ users”, see “stakeholders” for more information). Q&C: How interested are these users in the Outpatient Clinic for Personalized Psychotherapy? What are the incentives and the support needed to use the Outpatient Clinic for Personalized Psychotherapy?
The Outpatient Clinic for Personalized Psychotherapy provides the following services that create value for the users: an easy-to-use data assessment tool, intra- and interindividual ESM data, ongoing progress feedback, and data-based clinical decision-making support. Q&C: What is needed to make the service (organizationally and technically) easily accessible? How should it be set up that it is accepted and feasible?
Communication or channels connect the users with the services within the Outpatient Clinic for Personalized Psychotherapy. They include the research co-design process to raise awareness and evaluate the concept of the Outpatient Clinic for Personalized Psychotherapy, the ESM app m-Path to use and deliver the services, and personal communication to provide support and to stay in touch (Mestdagh et al., 2022 ). Q&C: How are different channels connected to each other, e.g., how is feedback provided within m-Path or to a particular therapist channeled to the administrative staff or the research team?
Types of relationships between the Outpatient Clinic for Personalized Psychotherapy and its users (formerly: customer relationships) range from personal assistance to automated services. For a successful implementation, the Outpatient Clinic for Personalized Psychotherapy needs a user support system that includes a statistician, an administrative team, system administrators, developers, the healthcare delivery system, and regulatory agencies (see “stakeholders” for more information). Q&C: How can the Outpatient Clinic for Personalized Psychotherapy be embedded into the existing routine clinical care structure and procedures?
Anticipated benefits are improvements of personalized psychological treatments that can continuously be adapted to the patient’s specific needs and an increase in patient engagement. These benefits are intended to produce reduced mental health care costs in the long run allowing to negotiate with healthcare insurances to pay for the service. Q&C: How can empirical evidence be provided that examine these anticipated benefits? What are the relevant outcomes?
Key resources describe the most important assets (physical, human, financial, and intellectual) that are required to make the business model work. The outpatient clinic is a major asset because it provides the facilities, but also the infrastructure of the healthcare provision including patients, therapists, supervisors, and staff members, likewise generating financial resources. The affiliation to the university is also a key resource because it includes clinical researchers, student assistants, and an intellectual network, e.g., to the scientific advisory board. Q&C: How the Outpatient Clinic for Personalized Psychotherapy integrated into the existing workflow and -packages to ensure long-term implementation?
Key activities that are needed to make the business model work include the implementation of a platform that allows to collect and visualize ESM data, the evaluation and analysis of the collected data, and the publication strategy. Q&C: Which platform should be selected? How are workflows covered that are not implemented in the platform yet?
K ey partnerships or collaborators (see “stakeholders” for more information) make the business model work. Q&C: How are stakeholders selected, engaged, and continuously involved into the process?
The cost structure describes all the costs incurred to operate the business model. In our case, the costs to buy and maintain the license for the platform, the salaries of the employees and student assistants, and, if needed, publication fees. Currently, all costs are covered by the university and the outpatient clinic. Q&C: How can costs be covered in the long run to guarantee sustainable funding for the Outpatient Clinic for Personalized Psychotherapy?
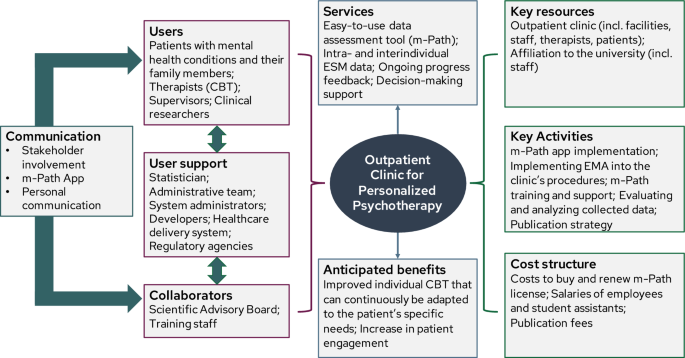
Draft of the Business Model of the Outpatient Clinic for Personalized Psychotherapy
Establishment of Technical Requirements
The Outpatient Clinic for Personalized Psychotherapy's key service is a platform facilitating all SCED phases: goal selection, treatment, measures, trial design (analysis, sampling, randomization, blinding, scheduling, and reviewing), data collection, and analysis (Eslick & Sim, 2014 ). Frequent graphing of data and adaptability to new information are crucial (Hayes, 1981 ). To facilitate shared decision-making, data presentation (e.g., treatment effect size, confidence intervals, graphical trajectories, means) must avoid overinterpretation, incorporate user preferences, and maintain scientific rigor (Duan et al., 2013 ). Using Eslick and Sim's ( 2014 ) checklist for platform selection, we decided to use m-Path (Mestdagh et al., 2022 ). M-path is an easy-to-use, closed-source platform with flexible ESM protocols, varied item formats, data collection and visualization, and just-in-time interventions, ideal for personalized research in clinical settings. m-Path's flexibility and support allowed us to implement a hierarchical account structure embedding therapists', supervisors', and patients' accounts under the research team. Patient data adheres to EU General Data Protection Regulation. Although m-Path visualizes patient data well, it lacks specific features for single case designs, like various trial designs or automated visual/statistical analysis. Q&C: How will the participant flow be organized (especially, if a randomization is used in the design?) How will data analysis and visualization be conducted?
Ethical Considerations and Data Protection
For all studies of the implementation process, the ethical approval is currently being obtained by the institutional ethics committee of the Department of Psychology at the RPTU Kaiserslautern-Landau. However, using the SCED infrastructure in routine clinical care and for research purposes comes with the challenge to meet requirements of treatment-related data and research data. For example, documentation and retention obligation apply to treatment-related data, research data may have to be deleted if the patient requests it. Specific in-depth interviews of Study 1 will be used to clarify questions regarding ethical considerations and data protection. An updated ethical approval will be obtained based on the results. Q&C: Should there be a line between treatment-related data and research data? Can patients opt-out of using the SCED infrastructure and still receive psychological treatment for their condition? How is privacy secured including data gathering, transport, storage, analyses, and presentation?
Study 2: Development of SCED Procedure and ESM Protocol for Routine Clinical Care
The aim of the study is (1) to develop a SCED procedure for routine clinical care, (2) to develop an ESM protocol that describes the different parameters that need to be considered such as assessment duration, frequency, and sampling scheme, and (3) to find a balance between optimal and pragmatic study settings. The ESM protocol will be reviewed in discussion groups with a minimum of six psychotherapists and six patients as the stakeholders who are mostly affected by the ESM protocol (for a justification of the sample size see Study 1). The SCED protocol, along with the outcomes of the discussion groups, will be presented to the international advisory board and to the management board of the outpatient clinic to come to a final decision.
SCED Procedure
We created a working version based on the Risk of Bias in N-of-1 Trials (RoBiNT) scale, a critical evaluation tool to evaluate the methodological quality of intervention studies using single-case methodology (Tate et al., 2013 ). We outlined the procedure for routine clinical care and research projects that could use the infrastructure of the single-case clinic in the future. For routine clinical care, we plan to use a replicated ABCDE design in which EMA data is collected after the initial appointment (phase A: baseline), during up to five diagnostic sessions (phase B: diagnostic), and during the first treatment sessions (phase C: intervention). Whenever psychotherapists alter their treatment focus or change their therapeutic strategies, they can initiate a new intervention phase (phase C’, phase C’’, etc.). This allows them to directly continuously evaluate their respective therapeutic approach. Furthermore, ESM data will be collected after treatment has ended (phase D: posttreatment) and at 6-months follow-up (phase E: follow-up). Further details can be found in Table 1 .
ESM Protocol
We followed systematic guidelines to consider different arguments when making methodological decisions (Janssens et al., 2018 ). A decision matrix was created that organizes different arguments along methodological questions (Supplemental Material, S3 Decision matrix for methodological questions, https://osf.io/54ewu ). Arguments include the nature of the variable of interest, reliability and feasibility issues, as well as statistical requirements. Open methodological questions include study duration, measurement frequency, number of items, sampling scheme, instruction of items, and delay allowed to respond. The matrix was filled with typical practices or recommendations for ESM studies found in the literature (Eisele et al., 2020 ; Janssens et al., 2018 ; Wrzus & Neubauer, 2023 ). Based on the matrix, the research team formulated methodical suggestions for the SCED infrastructure regarding each methodological question. We decided to omit the issue of statistical requirements. Instead, future studies using the SCED infrastructure should discuss statistical requirements for the respective research question and analysis in a separate pre-registration in the Open Science Framework ( https://osf.io/yex48/ ).
We propose to collect ESM data on 14 consecutive days during each SCED phase. We decided to not limit the study duration during the intervention phase with the aim to gather information about the extent that the daily surveys are used during treatment. Alternatively, a second (or more) 14-day assessment periods could be prompted during treatment (phase C’ or C’’ etc.). We suggest to collect one signal-contingent survey per day with the option of additional event-contingent surveys whenever the patient feels something important had happened. Each event-contingent survey will trigger a measurement burst with additional five surveys, randomly presented within the following hour. This measurement burst enables to monitor momentary mood on a microlevel. We decided to apply a semi-random sampling scheme with random beeps during an individualized time in the evening (e.g., 18-22 h). As delay allowed to respond, we suggest 60 min for the signal-contingent survey with a reminder after 30 min. For the event-contingent survey, we decided to close the survey after 30 min.
Study 3: Development of the ESM Survey
This study aims (1) to identify a relevant and suitable clinical behavior and (2) to develop an ESM survey to assess this target behavior in routine clinical care. The survey will be part of our standard diagnostic procedure, allowing patients and psychotherapists to add individualized items if desired. The survey's specific content is less critical for implementing the SCED infrastructure and can be replaced or expanded in future research studies based on other variables of interest. However, it is a significant gap in SCED infrastructure implementation that validated ESM surveys are still limited (for a positive example see https://esmitemrepositoryinfo.com/ ). It is needed if it should be possible to aggregate the generated SCED data across patients (e.g., to investigate overarching research questions). We plan to follow recommendations in questionnaire development and validation, following sequential phases of item generation, scale development and scale validation (Boateng et al., 2018 ). During this process, a minimum of six stakeholders each will be consulted at several stages until information saturation is reached (for a justification of the sample size see Study 1). For example, patients will be involved via cognitive interviews to ensure the comprehensibility of the items (Darnall et al., 2017 ). Psychotherapists will be involved to ensure that the m-path data dashboard is used in a meaningful way for clinicians (Guest et al., 2006 ). Besides content validity, it will be crucial to assess its suitability as a SCED instrument, e.g. in terms of sensitivity to intrapersonal change (Lavefjord et al., 2021 ), and to consider the potential non-stationarity of the data (Ryan et al., 2023 ).
We propose to apply a twofold assessment strategy which yields the potential to understand the individual’s model in reference to what is normative (Wright & Zimmermann, 2019 ) and to capture complex change processes that are of greatest relevance to individual clients being also most consistent with the clinical reality of psychotherapeutic work (Lloyd et al., 2019 ).
Emotion Regulation
As a first draft of an ESM survey, we decided to focus on emotion regulation as an important transdiagnostic mechanism to complement the thus far primarily symptom-oriented diagnostics with a mechanistically informed approach. Emotion regulation refers to the ‘ability to modulate the intensity, frequency, and duration of positive and/or negative emotions’ (Boemo et al., 2022 , p. 1). It is a dynamic, multi-stage process (Gross, 2015 ), making it well-suited for ESM measurement. Emotion regulation is crucial in psychological disorder development and maintenance (Fernandez et al., 2016 ). Understanding its complexity in everyday life can reveal regulation difficulties and inform targeted therapeutic interventions (Aldao et al., 2015 ; Gross & Jazaieri, 2014 ). Moreover, emotion regulation could be studied more rigorously as mechanism of change in psychotherapy (Palmieri et al., 2022 ). We plan to include the following emotion regulation processes in the ESM survey: momentary affect (Cloos et al., 2022 ), information on the appraisal of specific situational contexts (Doré et al., 2016 ), emotion regulation strategies (Boemo et al., 2022 ), emotion regulation motives (Tamir, 2016 ), and emotion regulation success (Gruber et al., 2012 ). This would allow a differentiated assessment of an important transdiagnostic mechanism within and across patients. Data provides important insight for psychotherapy independent from specific diagnosis and could still be aggregated across patients.
Idiographic Items
Above that, two idiographic items are formulated, a problem-focused and goal-focused idiographic measure. Idiographic measures offer personalized insights and empower clients (Elliott et al., 2016 ; Wright & Zimmermann, 2019 ). Especially, goal-setting has been shown to have effects of d = 0.34-0.40 of its own (Epton et al., 2017 ; Harkin et al., 2016 ). However, the item generation is not without challenges (Sales et al., 2023 ). The identification of relevant problems and goals is a difficult task as patients might not always be able to report their problems and goals in clear and precise manners. Also, problems and goals might change throughout the process of psychotherapy. For those reasons, we plan to build upon existing measures. According to two recent systematic reviews (Lloyd et al., 2019 ; Sales & Alves, 2016 ) examples for short problem-focused measures that could serve as a starting point are the Simplified Personal Questionnaire (PQ; Elliott et al., 2016 ; Shapiro, 1961 ) and the Psychological Outcome Profiles (PSYCHLOPS; Ashworth et al., 2005 ) and goal-focused measures are Goal Attainment Scaling (Kiresuk & Sherman, 1968 ), Goals Form (Cooper & Xu, 2023 ), and Youth TOP Problems (Weisz et al., 2011 ).
Study 4: Testing Usability and Feasibility
In the optimization phase , the aim is to test usability and feasibility and to customize the SCED infrastructure accordingly. We plan to carry out several SCED iterations using a replicated ABC(DE) design (see Table 1 ; Tate et al., 2013 ). Each iteration will comprise 6–12 patient-therapist dyads (Bartels et al., 2022 ). To accelerate the optimization process, only phases A, B, and C are initially included to identify critical points of improvement. Phases D and E will be carried out, but they will not be decisive for the optimization process. Based on the draft of the ESM protocol, the respective phases should comprise 14 assessment days. Patient-therapist dyads are informed that participation also includes a subsequent stakeholder meeting. The results of each iteration will be presented at the respective stakeholder meeting. Stakeholders will collaboratively identify areas for improvement in each component of the infrastructure. Based on this, they may choose to initiate further rounds of iterations. The optimization loop continues until stakeholders agree that the infrastructure is ready for the implementation phase. Decisions will be consensus-based, requiring agreement from all stakeholders. Figure 3 presents an overview about the design and process of the optimization phase.
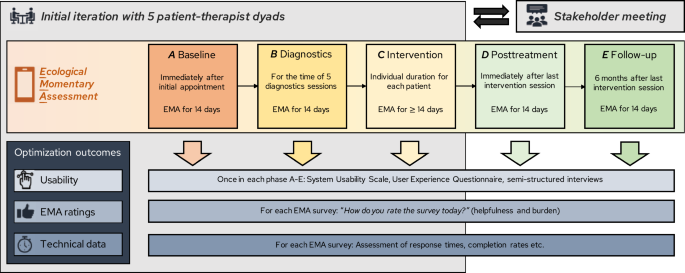
Design and process of the optimization phase
Usability Measures
A mixed methods approach will be used to determine the usability of the SCED infrastructure, including questionnaires, technical data, and semi-structured interviews (see Table 2 ). Questionnaires will be administered at the end of each SCED phase with the concluding assessment comprising questionnaires and a semi-structural interview 6 weeks after the intervention started. To facilitate its evaluation, we decomposed the infrastructure into the following components: initial expectations, the onboarding process, the practical use of the m-path app, the feasibility of the ESM protocol and survey (including possible negative side effects), the clarity of the visual dashboard, the utility for clinical practice, and data management aspects.
Feasibility Measures
Feasibility is evaluated along the outcome domains of implementation research that are commonly used in feasibility studies (Arain et al., 2010 ; Proctor et al., 2011 ): Acceptability and appropriateness are assessed as part of the usability measures. Feasible adoption is reflected in participation (≥ 50% offered using the SCED infrastructure will enroll in the study and ≥ 70% of the participants who enrolled will complete participation; Frumkin et al., 2021 ). Reasons for drop-out will be assessed if participants consent to provide information. In addition, penetration, compliance and retention will be monitored.
Study 5: Piloting the Implementation of the SCED Infrastructure
The study aims (1) to determine how users accept, perceive, adopt, and integrate the SCED infrastructure into routine clinical care and (2) to use results to initiate strategies for further improvement. For that reason, the SCED infrastructure will be offered in routine clinical care to generate first evidence regarding perceptual and behavioral implementation domains. We intend to offer all new admissions to our clinic to use the SCED infrastructure for a 1-year period. Both patients (irrespective of their clinical diagnosis except for acute suicidal tendencies and psychotic symptoms) and psychotherapists will be invited to participate in the implementation study. We expect to have approximately 250 new admissions in a 1-year period. On average, during the last five years we had 295 new admissions per year, but due to the change of the system we expect a slight decrease. In 2021 and 2022, we had 77 active psychotherapists and 72 respectively. The number of patients and psychotherapists who decide to use the infrastructure will be used to estimate the adoption rate as one implementation outcome (see Section “Implementation Outcome Measures” and Table 3 for more information).
To evaluate our implementation process, we assess several perceptual and behavioral outcome domains (Proctor et al., 2011 ). We hope to obtain some outcome measures from people who are willing to use the SCED infrastructure, as well as those who are not interested. This will allow us to conduct some exploratory analyzes about how these groups differ. For patients, we expect to collect a relatively diverse sample which will probably allow such a comparison.
Results of the initial implementation study will be a first indicator for the expected sustainability of the SCED infrastructure in our clinic. In case, results do not meet our predefined criteria for a successful implementation, the research team will develop a proposal for another implementation strategy plan (Kirchner et al., 2020 b; Powell et al., 2015 ) to maintain and further improve the uptake of the SCED infrastructure. Other implementation strategies could comprise the provision of interactive assistance or the training of stakeholders. Again, the implementation plan will be optimized and finalized together with different stakeholders. In either case, we plan to replicate the pilot implementation study for another year, with the goal to either test whether implementation outcomes substantially improve compared to the first implementation round or to evaluate its sustainability.
Implementation Outcome Measures
Perceptual and behavioral outcome measures were selected in accordance with a generally recognized taxonomy for outcome domains in implementation research (see Table 3 ; Proctor et al., 2011 ). For our study purposes, we considered the outcome domains acceptability, appropriateness, adoption, and penetration. In addition, we plan to report on compliance and retention rates as indicators inherently linked to ESM studies. Criteria for a successful implementation were chosen according to previous research (Schleider et al., 2020 ). Criteria for compliance and retention rates were formulated based on systematic reviews (Rintala et al., 2019 ; Vachon et al., 2019 ; Wrzus & Neubauer, 2023 ). We decided to omit the domains of feasibility, fidelity, and sustainability as these issues are addressed in the other phases of the agile research framework. For example, feasibility will be ameliorated and tested in phase 3, while sustainability will be addressed in phase 5. Likewise, it is difficult to include fidelity in an agile research framework as methodological decisions can change based on outcomes from previous phases. We have chosen behavioral measures whenever possible. Given the general lack of unified implementation measures with mostly unknown psychometric quality, questionnaires to assess perceptual outcomes were selected based on a recent systematic review evaluating the psychometric properties of implementation measures (Mettert et al., 2020 ). Perceptual implementation outcome measures will be collected from both patients and psychotherapists when they decide whether they want to use the SCED infrastructure prior to treatment. Perceptional implementation outcomes will also be administered after each SCED phase to record their development over time. In the intervention phase, implementation measures will be administered 6 weeks after the intervention started. If the post-treatment and follow-up phases are not yet available for all patients at the time of data analysis, patients will be invited to take part in another 14 ESM assessment days and to provide concluding information on perceptual implementation outcome measures.
Study 6: Implementation of the SCED Infrastructure
The aim of the study during the implementation phase is to evaluate the SCED infrastructure compared to routine diagnostic procedures prior implementation. A quasi-experimental single case AB-design with the outpatient clinic as entity will be conducted. The design has the advantages that it reduces confounders (e.g., secular trends, seasonality) and allows to measure long-term effects (Bernal et al., 2017 ). We will use a continuous sequence of outcome data taken repeatedly over time from the outpatient clinic prior implementation and compare it to outcome data post implementation. This time series is used to estimate an interrupted time series regression. An underlying trend is established, which is ‘interrupted’ by the implementation of the SCED infrastructure at a known point in time (Bernal et al., 2017 ). Therefore, pre- and post-implementation need to be clearly distinguished (Miller et al., 2020 ). A graphical example of an interrupted time series is presented as Supplemental Material (S1 Design illustrations, https://osf.io/dkytg ).
In our case, data are considered for the pre-implementation phase up to the start of Study 5 and for the post-implementation phase after the completion of Study 5, when the SCED infrastructure is introduced for everyone into routine clinical care. Two items assessing satisfaction and burden of the diagnostic procedure (that includes the ESM assessments after the implementation of the SCED infrastructure) will be used to evaluate the new diagnostic procedure. In addition, we will take an exploratory look at clinical effectiveness using the Symptom Checklist – 90 (Derogatis & Unger, 2010 ; Franke, 2014 ). We expect a slight level and slope change regarding clinical effectiveness because research has shown that self-monitoring already effects outcomes positively leading to level change (Bartels et al., 2019 ; Guo & Albright, 2018 ; McBain et al., 2015 ; Simons et al., 2015 ). In addition, the continuous feedback might reduce treatment failure and improve the positive effects of psychotherapy for example through enhanced tailoring (Lambert & Harmon, 2018 ; Lutz et al., 2019 ), leading to a temporary slope change resulting in a level change.
SCEDs are prospective idiographic research designs aiming to estimate meaningful treatment effects for individual patients (Vlaeyen et al., 2020 ). We argue that the method is highly suitable to foster the development and evaluation of personalized psychological treatments for two main reasons: First, current data-driven personalization efforts are mainly based on nomothetic data that come with limitations due to power (Lorenzo-Luaces et al., 2021 ) and due to invalid inferences to the individual. In contrast, the SCED infrastructure offers a truly idiographic approach to research and practice. Nevertheless, generalization is enabled through replication creating data that can also be accumulated to answer nomothetic research questions. Second, since the SCED infrastructure allows systematic manipulation, it is valid to draw causal inferences and estimate treatment effects for the individual patient which extends the observational character of current routine outcome monitoring systems. For those reasons, we outlined the step-by-step implementation with stakeholder involvement of a SCED infrastructure including ESM into routine clinical care of a German outpatient research and training clinic. While focusing on routine clinical care in this protocol, the infrastructure can also serve future research studies using SCED methodology.
To our knowledge, such a SCED infrastructure has not been implemented in the context of mental health up till now. Yet, such an infrastructure could be associated with several advantages: The SCED infrastructure could link everyday life to the psychotherapy process more closely and brings the psychotherapy process back into daily life. For example, it could empower patients through self-monitoring and feedback and enhance clinical and collaborative decision-making through continuous evaluation (Herzog et al., 2022 ; Schemer et al., 2022 ), also overcoming biases of clinical judgements (Kaiser et al., 2022a , 2022b ). Therapists will probably find this feedback highly credible because it results from multiple assessments in the daily life of patients. The feedback provides new information about the patients' progress with high precision. This accurate information from a credible source facilitates the integration of feedback and update of beliefs about patients’ therapy progress—a necessary prerequisite for the feedback to effect treatment outcome (Herzog et al., 2023 ).
Practitioners in routine clinical care could also use systematic manipulation in an ongoing treatment to evaluate their own clinical practice for their particular patients (Piccirillo & Rodebaugh, 2019 ). Thus, the infrastructure could bring science into practice allowing a truly individualized and at the same time evidence-based treatment to deliver the most effective treatment for the respective patient. Above that, it provides an infrastructure for a range of idiographic and/or nomothetic research questions that need intensive longitudinal data assessment and/or systematic manipulation: What specific emotional regulation difficulties does the individual patient have and how can they best be addressed in therapy? How effective is a particular treatment strategy such as high-intensity exposure for an individual patient or specific patient groups? To what extent does a change in therapeutic strategy lead to an improvement in the process outcome if there was no improvement or even a deterioration in the previous phase? Asked differently, how does changing the therapeutic strategy too quickly affect the therapeutic process? How valid are inferences from group data to the individual vs. from individual data to the group regarding the psychotherapy process (ergodicity problem; see Adolf & Fried, 2019 ; Fisher et al., 2018 )? Methodologically, the SCED infrastructure allows to focus on patterns within a person using intensive longitudinal data that can be used to inform decisions about personalized treatment strategies for this specific person (Delgadillo & Lutz, 2020 ). For example, it might be interesting to take into account the dynamic nature of psychological mechanisms. Once data has accumulated, personalized advantage indexes could be developed to guide personalized treatment selection on a very fine-grained level (DeRubeis et al., 2014 ).
The step-by-step implementation of a SCED infrastructure including EMA with stakeholder involvement comes with the following limitations: First, the step-by-step implementation is initiated expecting that the SCED infrastructure will enable studies that will push personalization forward leading to more effective treatments for individual patients. However, evidence that will support this expectation can only be generated after implementation. Therefore, we did not describe a stopping rule for the implementation process, even though there could be a high patient burden, lacking penetration, or it might not lead to better treatment in the long run.
Second, it must be kept in mind that evidence for the effectiveness of stakeholder engagement from experimental or empirical studies is lacking (Slattery et al., 2020 ). At the same time, it comes with an increase in research resources such as time, costs, and training efforts, changes that might not be feasibly and uncertainty to resolve conflicts (Holzer et al., 2022 ; Tindall et al., 2021 ). Further barriers are the use of technical jargon, power imbalances between the researcher and stakeholders, difficulty for stakeholders to understand how their input is reflected in the final research (Holzer et al., 2022 ). Therefore, it is important to introduce the research purpose, the questions being addressed and the requirements for the studies carefully (Holzer et al., 2022 ). Role descriptions and responsibilities should be clearly defined and the stakeholders’ time and input should be valued to build trust and rapport between researchers and stakeholders (Slattery et al., 2020 ).
Third, there are many obstacles when implementing a new infrastructure into an existing routine. As the consolidated framework for implementation research outlines, many domains need to be taken into consideration including the inner and outer setting, the individuals involved in the implementation, and the implementation process itself (Damschroder et al., 2009 , 2022 ). While this protocol outlines the implementation process and some aspects of the other domains, the adaptation to the inner and outer setting will present numerous challenges including the accounting of the service with insurances, patient data protection and handling policies, or the maintenance of the service with existing staff. Even though we try to address these challenges from early on, most of them will come up throughout the process. The implementation strategies were chosen to respond to those upcoming issues flexibly on the way.
Despite these limitations, we are optimistic that challenges can be faced and overall, the potential of the SCED infrastructure will outweigh the burden. We will propose a business model for a Outpatient Clinic for Personalized Psychotherapy—a learning mental health care system that can reduce the research-practice gap. We will implement this SCED infrastructure in combination with EMA into routine clinical care step-by-step with stakeholder involvement to leverage the potential of person-specific data-based and data-driven methods (Schemer et al., 2022 ).
Different terms are used in the context of SCEDs such as Single-Case Experimental Design (SCED), Single-Subject Research Design, Single-Case Observational Design (SCOD), N-of-1 trial, Single Patient Open Trial (SPOT) and Single-Case Design (SCD) (Krasny-Pacini & Evans, 2018 ; Nikles et al., 2021 ). Although SCEDs is widely used, we use from here on SCD as an umbrella term, allowing also observational and open designs and thus fitting better with the intended use in our routine clinical care setting.
Experience Sampling Methodology (ESM), Ecological Momentary Assessments, and Ambulatory Assessments comprise data collection methods used to study individuals’ daily life experiences and environmental features in naturalistic settings (see Myin-Germeys & Kuppens, 2021). In particular, ESM and Ecological Momentary Assessment prompt individuals to respond to surveys assessing psychological constructs as they unfold in daily life (Schemer et al., 2022 ). For consistency reasons, we use the term “ESM”.
Abelson, J., Li, K., Wilson, G., Shields, K., Schneider, C., & Boesveld, S. (2016). Supporting quality public and patient engagement in health system organizations: Development and usability testing of the Public and Patient Engagement Evaluation Tool. Health Expectations, 19 (4), 817–827. https://doi.org/10.1111/hex.12378
Article PubMed Google Scholar
Adolf, J. K., & Fried, E. I. (2019). Ergodicity is sufficient but not necessary for group-to-individual generalizability. Proceedings of the National Academy of Sciences, 116 (14), 6540–6541. https://doi.org/10.1073/pnas.1818675116
Article ADS CAS Google Scholar
Aldao, A., Sheppes, G., & Gross, J. J. (2015). Emotion regulation flexibility. Cognitive Therapy and Research, 39 (3), 263–278. https://doi.org/10.1007/s10608-014-9662-4
Article Google Scholar
Allport, G. W. (1937). Personality: A psychological interpretation (pp. xiv, 588). Holt.
Altman, A. D., Shapiro, L. A., & Fisher, A. J. (2020). Why does therapy work? An idiographic approach to explore mechanisms of change over the course of psychotherapy using digital assessments. Frontiers in Psychology, 11 , 782. https://doi.org/10.3389/fpsyg.2020.00782
Article PubMed PubMed Central Google Scholar
Anampa-Guzmán, A., Freeman-Daily, J., Fisch, M., Lou, E., Pennell, N. A., Painter, C. A., Sparacio, D., Lewis, M. A., Karmo, M., Anderson, P. F., Graff, S. L., For the Collaboration for Outcomes using Social Media in Oncology. (2022). The rise of the expert patient in cancer: From backseat passenger to co-navigator. JCO Oncology Practice, 18 (8), 578–583. https://doi.org/10.1200/OP.21.00763
Arain, M., Campbell, M. J., Cooper, C. L., & Lancaster, G. A. (2010). What is a pilot or feasibility study? A review of current practice and editorial policy. BMC Medical Research Methodology, 10 (1), 67. https://doi.org/10.1186/1471-2288-10-67
Ashworth, M., Robinson, S. I., Godfrey, E., Shepherd, M., Evans, C., Seed, P., Parmentier, H., & Tylee, A. (2005). Measuring mental health outcomes in primary care: The psychometric properties of a new patient-generated outcome measure’, PSYCHLOPS’('psychological outcome profiles’). Primary Care Mental Health, 3 , 4.
Google Scholar
Bartels, S. L., Johnsson, S. I., Boersma, K., Flink, I., McCracken, L. M., Petersson, S., Christie, H. L., Feldman, I., Simons, L. E., Onghena, P., Vlaeyen, J. W. S., & Wicksell, R. K. (2022). Development, evaluation and implementation of a digital behavioural health treatment for chronic pain: Study protocol of the multiphase DAHLIA project. British Medical Journal Open, 12 (4), e059152. https://doi.org/10.1136/bmjopen-2021-059152
Bartels, S. L., van Knippenberg, R. J. M., Dassen, F. C. M., Asaba, E., Patomella, A.-H., Malinowsky, C., Verhey, F. R. J., & de Vugt, M. E. (2019). A narrative synthesis systematic review of digital self-monitoring interventions for middle-aged and older adults. Internet Interventions, 18 , 100283. https://doi.org/10.1016/j.invent.2019.100283
Bauer, M. S., & Kirchner, J. (2020). Implementation science: What is it and why should I care? Psychiatry Research, 283 , 112376. https://doi.org/10.1016/j.psychres.2019.04.025
Beatty, P. C., & Willis, G. B. (2007). Research synthesis: The practice of Cognitive Interviewing. Public Opinion Quarterly, 71 (2), 287–311. https://doi.org/10.1093/poq/nfm006
Bentley, K. H., Kleiman, E. M., Elliott, G., Huffman, J. C., & Nock, M. K. (2019). Real-time monitoring technology in single-case experimental design research: Opportunities and challenges. Behaviour Research and Therapy, 117 , 87–96. https://doi.org/10.1016/j.brat.2018.11.017
Berg, M., Schemer, L., Kirchner, L., & Scholten, S. (2023). Mind the gap—Ideas for making clinical research more relevant for practitioners and patients. PsyArXiv . https://doi.org/10.31234/osf.io/2qvhy
Bernal, J. L., Cummins, S., & Gasparrini, A. (2017). Interrupted time series regression for the evaluation of public health interventions: A tutorial. International Journal of Epidemiology, 46 (1), 348–355. https://doi.org/10.1093/ije/dyw098
Boateng, G. O., Neilands, T. B., Frongillo, E. A., Melgar-Quiñonez, H. R., & Young, S. L. (2018). Best practices for developing and validating scales for health, social, and behavioral research: A primer. Frontiers in Public Health, 6 , 149. https://doi.org/10.3389/fpubh.2018.00149
Boemo, T., Nieto, I., Vazquez, C., & Sanchez-Lopez, A. (2022). Relations between emotion regulation strategies and affect in daily life: A systematic review and meta-analysis of studies using ecological momentary assessments. Neuroscience & Biobehavioral Reviews, 139 , 104747. https://doi.org/10.1016/j.neubiorev.2022.104747
Brooke, J. (1996). SUS: A quick and dirty usability scale. In Usability evaluation in industry (pp. 189–194).
Brown, J., & Isaacs, D. (2005). The World Café: Shaping our futures through conversations that matter (1st ed.). Berrett-Koehler.
Chekroud, A. M., Bondar, J., Delgadillo, J., Doherty, G., Wasil, A., Fokkema, M., Cohen, Z., Belgrave, D., DeRubeis, R., Iniesta, R., Dwyer, D., & Choi, K. (2021). The promise of machine learning in predicting treatment outcomes in psychiatry. World Psychiatry, 20 (2), 154–170. https://doi.org/10.1002/wps.20882
Cloos, L., Ceulemans, E., & Kuppens, P. (2022). Development, validation, and comparison of self-report measures for positive and negative affect in intensive longitudinal research. Psychological Assessment . https://doi.org/10.1037/pas0001200
Cohen, Z. D., Delgadillo, J., & DeRubeis, R. J. (2021). Personalized treatment approaches. In M. Barkham, W. Lutz, & L. G. Castonguay (Eds.), Bergin and Garfield’s handbook of psychotherapy and behavior change. Wiley.
Cohen, Z. D., & DeRubeis, R. J. (2018). Treatment Selection in Depression. Annual Review of Clinical Psychology, 14 , 1–28. https://doi.org/10.1146/annurev-clinpsy-050817
Cooper, M., & Xu, D. (2023). Goals Form: Reliability, validity, and clinical utility of an idiographic goal-focused measure for routine outcome monitoring in psychotherapy. Journal of Clinical Psychology, 79 (3), 641–666. https://doi.org/10.1002/jclp.23344
Damschroder, L. J., Aron, D. C., Keith, R. E., Kirsh, S. R., Alexander, J. A., & Lowery, J. C. (2009). Fostering implementation of health services research findings into practice: A consolidated framework for advancing implementation science. Implementation Science, 4 (1), 50. https://doi.org/10.1186/1748-5908-4-50
Damschroder, L. J., Reardon, C. M., Widerquist, M. A. O., & Lowery, J. (2022). The updated consolidated framework for implementation research based on user feedback. Implementation Science, 17 (1), 75. https://doi.org/10.1186/s13012-022-01245-0
Darnall, B. D., Sturgeon, J. A., Cook, K. F., Taub, C. J., Roy, A., Burns, J. W., Sullivan, M., & Mackey, S. C. (2017). Development and validation of a daily Pain Catastrophizing Scale. The Journal of Pain, 18 (9), 1139–1149. https://doi.org/10.1016/j.jpain.2017.05.003
Delgadillo, J., & Lutz, W. (2020). A development pathway towards precision mental health care. JAMA Psychiatry . https://doi.org/10.1001/jamapsychiatry.2020.1048
Derogatis, L. R., & Unger, R. (2010). Symptom checklist-90-revised. In The Corsini Encyclopedia of Psychology (pp. 1–2). American Cancer Society. https://doi.org/10.1002/9780470479216.corpsy0970
DeRubeis, R. J., Cohen, Z. D., Forand, N. R., Fournier, J. C., Gelfand, L. A., & Lorenzo-Luaces, L. (2014). The Personalized Advantage Index: Translating research on prediction into individualized treatment recommendations. A demonstration. PLoS ONE, 9 (1), e83875. https://doi.org/10.1371/journal.pone.0083875
Article ADS CAS PubMed PubMed Central Google Scholar
Domecq, J. P., Prutsky, G., Elraiyah, T., Wang, Z., Nabhan, M., Shippee, N., Brito, J. P., Boehmer, K., Hasan, R., Firwana, B., Erwin, P., Eton, D., Sloan, J., Montori, V., Asi, N., Abu Dabrh, A. M., & Murad, M. H. (2014). Patient engagement in research: A systematic review. BMC Health Services Research, 14 (1), 89. https://doi.org/10.1186/1472-6963-14-89
Doré, B. P., Silvers, J. A., & Ochsner, K. N. (2016). Toward a personalized science of emotion regulation. Social and Personality Psychology Compass, 10 (4), 171–187. https://doi.org/10.1111/spc3.12240
Doria, N., Condran, B., Boulos, L., Curtis Maillet, D. G., Dowling, L., & Levy, A. (2018). Sharpening the focus: Differentiating between focus groups for patient engagement vs. qualitative research. Research Involvement and Engagement, 4 (1), 19. https://doi.org/10.1186/s40900-018-0102-6
Duan, N., Kravitz, R. L., & Schmid, C. H. (2013). Single-patient (n-of-1) trials: A pragmatic clinical decision methodology for patient-centered comparative effectiveness research. Journal of Clinical Epidemiology, 66 (8), S21–S28. https://doi.org/10.1016/j.jclinepi.2013.04.006
Eisele, G., Vachon, H., Lafit, G., Kuppens, P., Houben, M., Myin-Germeys, I., & Viechtbauer, W. (2020). The effects of sampling frequency and questionnaire length on perceived burden, compliance, and careless responding in experience sampling data in a student population. Assessment, 29 (2), 136–151. https://doi.org/10.1177/1073191120957102
Elliott, R., Wagner, J., Sales, C. M. D., Rodgers, B., Alves, P., & Café, M. J. (2016). Psychometrics of the Personal Questionnaire: A client-generated outcome measure. Psychological Assessment, 28 (3), 263–278. https://doi.org/10.1037/pas0000174
Epton, T., Currie, S., & Armitage, C. J. (2017). Unique effects of setting goals on behavior change: Systematic review and meta-analysis. Journal of Consulting and Clinical Psychology, 85 (12), 1182–1198. https://doi.org/10.1037/ccp0000260
Eslick, I., & Sim, I. (2014). Information Technology (IT) infrastructure for N-of-1 trials. In R. L. Kravitz, N. Duan, & DEcIDE Methods Center N-of-1 Guidance Panel (Duan N, Eslick I, Gabler NB, Kaplan HC, Kravitz RL, Larson EB, Pace WD, Schmid CH, Sim I, Vohra S), Design and implementation of N-of-1 trials: A user’s guide: Vol. AHRQ Publication No. 13(14)-EHC122-EF (pp. 55–70). Agency for Healthcare Research and Quality.
Fernandez, K. C., Jazaieri, H., & Gross, J. J. (2016). Emotion regulation: A transdiagnostic perspective on a new RDoC domain. Cognitive Therapy and Research, 40 (3), 426–440. https://doi.org/10.1007/s10608-016-9772-2
Fisher, A. J. (2015). Toward a dynamic model of psychological assessment: Implications for personalized care. Journal of Consulting and Clinical Psychology, 83 (4), 825–836. https://doi.org/10.1037/ccp0000026
Fisher, A. J., Medaglia, J. D., & Jeronimus, B. F. (2018). Lack of group-to-individual generalizability is a threat to human subjects research. Proceedings of the National Academy of Sciences, 115 (27), E6106–E6115. https://doi.org/10.1073/pnas.1711978115
Franke, G. H. (2014). Symptom-Checklist-90-Standard (1 . Hogrefe.
Frumkin, M. R., Piccirillo, M. L., Beck, E. D., Grossman, J. T., & Rodebaugh, T. L. (2021). Feasibility and utility of idiographic models in the clinic: A pilot study. Psychotherapy Research: Journal of the Society for Psychotherapy Research, 31 (4), 520–534. https://doi.org/10.1080/10503307.2020.1805133
Gross, J. J. (2015). Emotion regulation: Current status and future prospects. Psychological Inquiry, 26 (1), 1–26. https://doi.org/10.1080/1047840X.2014.940781
Article ADS MathSciNet Google Scholar
Gross, J. J., & Jazaieri, H. (2014). Emotion, emotion regulation, and psychopathology: An affective science perspective. Clinical Psychological Science, 2 (4), 387–401. https://doi.org/10.1177/2167702614536164
Gruber, J., Harvey, A. G., & Gross, J. J. (2012). When trying is not enough: Emotion regulation and the effort–success gap in bipolar disorder. Emotion, 12 (5), 997–1003. https://doi.org/10.1037/a0026822
Guest, G., Bunce, A., & Johnson, L. (2006). How many interviews are enough? An experiment with data saturation and variability. Field Methods, 18 (1), 59–82. https://doi.org/10.1177/1525822X05279903
Guo, Y., & Albright, D. (2018). The effectiveness of telehealth on self-management for older adults with a chronic condition: A comprehensive narrative review of the literature. Journal of Telemedicine and Telecare, 24 (6), 392–403. https://doi.org/10.1177/1357633X17706285
Harkin, B., Webb, T. L., Chang, B. P. I., Prestwich, A., Conner, M., Kellar, I., Benn, Y., & Sheeran, P. (2016). Does monitoring goal progress promote goal attainment? A meta-analysis of the experimental evidence. Psychological Bulletin, 142 (2), 198–229. https://doi.org/10.1037/bul0000025
Hayes, S. C. (1981). Single case experimental design and empirical clinical practice. Journal of Consulting and Clinical Psychology, 49 (2), 193–211. https://doi.org/10.1037/0022-006X.49.2.193
Article CAS PubMed Google Scholar
Herzog, P., & Kaiser, T. (2022). Is it worth it to personalize the treatment of PTSD? - A variance-ratio meta-analysis and estimation of treatment effect heterogeneity in RCTs of PTSD. Journal of Anxiety Disorders . https://doi.org/10.1016/j.janxdis.2022.102611
Herzog, P., Kaiser, T., & Brakemeier, E.-L. (2022). Praxisorientierte Forschung in der Psychotherapie: Entwicklung, Gegenwart und Zukunft. Zeitschrift Für Klinische Psychologie Und Psychotherapie, 51 (2), 127–148. https://doi.org/10.1026/1616-3443/a000665
Herzog, P., Kube, T., & Rubel, J. (2023). Why some psychotherapists benefit from feedback on treatment progress more than others: A belief updating perspective. Clinical Psychology: Science and Practice, 30 (4), 468–479. https://doi.org/10.1037/cps0000174
Heyvaert, M., & Onghena, P. (2014). Randomization tests for single-case experiments: State of the art, state of the science, and state of the application. Journal of Contextual Behavioral Science, 3 (1), 51–64. https://doi.org/10.1016/j.jcbs.2013.10.002
Holzer, K. J., Veasley, C., Kerns, R. D., Edwards, R. R., Gewandter, J. S., Langford, D. J., Yaeger, L. H., McNicol, E., Ferguson, M., Turk, D. C., Dworkin, R. H., & Haroutounian, S. (2022). Partnering with patients in clinical trials of pain treatments: A narrative review. Pain, 163 (10), 1862–1873. https://doi.org/10.1097/j.pain.0000000000002600
Howard, K. I., Moras, K., Brill, P. L., Martinovich, Z., & Lutz, W. (1996). Evaluation of psychotherapy: Efficacy, effectiveness, and patient progress. American Psychologist, 51 (10), 1059–1064. https://doi.org/10.1037/0003-066X.51.10.1059
Janssens, K. A. M., Bos, E. H., Rosmalen, J. G. M., Wichers, M. C., & Riese, H. (2018). A qualitative approach to guide choices for designing a diary study. BMC Medical Research Methodology, 18 (1), 140. https://doi.org/10.1186/s12874-018-0579-6
Kahneman, D., & Klein, G. (2009). Conditions for intuitive expertise: A failure to disagree. American Psychologist, 64 (6), 515–526. https://doi.org/10.1037/a0016755
Kaiser, T., Brakemeier, E.-L., & Herzog, P. (2023). What if we wait? Using synthetic waiting lists to estimate treatment effects in routine outcome data. Psychotherapy Research . https://doi.org/10.1080/10503307.2023.2182241
Kaiser, T., & Herzog, P. (2023). Is personalized treatment selection a promising avenue in bpd research? A meta-regression estimating treatment effect heterogeneity in RCTs of BPD. Journal of Consulting and Clinical Psychology . https://doi.org/10.1037/ccp0000803
Kaiser, T., Herzog, P., Voderholzer, U., & Brakemeier, E.-L. (2022a). Out of sight, out of mind? High discrepancy between observer- and patient-reported outcome after routine inpatient treatment for depression. Journal of Affective Disorders, 300 , 322–325. https://doi.org/10.1016/j.jad.2022.01.019
Kaiser, T., Volkmann, C., Volkmann, A., Karyotaki, E., Cuijpers, P., & Brakemeier, E.-L. (2022b). Heterogeneity of treatment effects in trials on psychotherapy of depression. Clinical Psychology: Science and Practice. https://doi.org/10.1037/cps0000079
Kaplan, H. C., & Gabler, N. B. (2014). User engagement, training, and support for conducting N-of-1 trials. In R. L. Kravitz, N. Duan, & DEcIDE Methods Center N-of-1 Guidance Panel (Duan N, Eslick I, Gabler NB, Kaplan HC, Kravitz RL, Larson EB, Pace WD, Schmid CH, Sim I, Vohra S), Design and implementation of N-of-1 trials: A user’s guide: Vol. AHRQ Publication No. 13(14)-EHC122-EF (pp. 71–81). Agency for Healthcare Research and Quality.
Kazdin, A. E. (2019). Single-case experimental designs Evaluating interventions in research and clinical practice. Behaviour Research and Therapy, 117 , 3–17. https://doi.org/10.1016/j.brat.2018.11.015
Kiesler, D. J. (1966). Some myths of psychotherapy research and the search for a paradigm. Psychological Bulletin, 65 (2), 110–136. https://doi.org/10.1037/h0022911
Kirchner, J. E., Smith, J. L., Powell, B. J., Waltz, T. J., & Proctor, E. K. (2020). Getting a clinical innovation into practice: An introduction to implementation strategies. Psychiatry Research, 283 , 112467. https://doi.org/10.1016/j.psychres.2019.06.042
Kiresuk, T. J., & Sherman, R. E. (1968). Goal attainment scaling: A general method for evaluating comprehensive community mental health programs. Community Mental Health Journal, 4 (6), 443–453. https://doi.org/10.1007/BF01530764
Krasny-Pacini, A., & Evans, J. (2018). Single-case experimental designs to assess intervention effectiveness in rehabilitation: A practical guide. Annals of Physical and Rehabilitation Medicine, 61 (3), 164–179. https://doi.org/10.1016/j.rehab.2017.12.002
Kravitz, R. L., Aguilera, A., Chen, E. J., Choi, Y. K., Hekler, E., Karr, C., Kim, K. K., Phatak, S., Sarkar, S., Schueller, S. M., Sim, I., Yang, J., & Schmid, C. H. (2020). Feasibility, acceptability, and influence of mHealth-supported N-of-1 trials for enhanced cognitive and emotional well-being in US volunteers. Frontiers in Public Health, 8 , 260. https://doi.org/10.3389/fpubh.2020.00260
Kravitz, R. L., Duan, N., Vohra, S., & Li, J. (2014). Introduction to N-of-1 trials: Indications and barriers. In R. L. Kravitz, N. Duan, & DEcIDE Methods Center N-of-1 Guidance Panel (Duan N, Eslick I, Gabler NB, Kaplan HC, Kravitz RL, Larson EB, Pace WD, Schmid CH, Sim I, Vohra S), Design and implementation of N-of-1 Trials: A user’s guide: Vol. AHRQ Publication No. 13(14)-EHC122-EF (pp. 1–11). Agency for Healthcare Research and Quality.
Lambert, M. J., & Harmon, K. L. (2018). The merits of implementing routine outcome monitoring in clinical practice. Clinical Psychology: Science and Practice, 25 (4), e12268. https://doi.org/10.1111/cpsp.12268
Lavefjord, A., Sundström, F. T. A., Buhrman, M., & McCracken, L. M. (2021). Assessment methods in single case design studies of psychological treatments for chronic pain: A scoping review. Journal of Contextual Behavioral Science, 21 , 121–135. https://doi.org/10.1016/j.jcbs.2021.05.005
Lloyd, C. E. M., Duncan, C., & Cooper, M. (2019). Goal measures for psychotherapy: A systematic review of self-report, idiographic instruments. Clinical Psychology: Science and Practice, 26 (3), e12281. https://doi.org/10.1111/cpsp.12281
Lorenzo-Luaces, L., Peipert, A., De Jesús Romero, R., Rutter, L. A., & Rodriguez-Quintana, N. (2021). Personalized medicine and cognitive behavioral therapies for depression: Small effects, big problems, and bigger data. International Journal of Cognitive Therapy, 14 (1), 59–85. https://doi.org/10.1007/s41811-020-00094-3
Lutz, W., Rubel, J. A., Schwartz, B., Schilling, V., & Deisenhofer, A.-K. (2019). Towards integrating personalized feedback research into clinical practice: Development of the Trier Treatment Navigator (TTN). Behaviour Research and Therapy, 120 , 103438. https://doi.org/10.1016/j.brat.2019.103438
Lutz, W., Schwartz, B., & Delgadillo, J. (2022). Measurement-based and data-informed psychological therapy. Annual Review of Clinical Psychology, 18 (1), 071720–114821. https://doi.org/10.1146/annurev-clinpsy-071720-014821
Lyon, A. R., Koerner, K., & Chung, J. (2020). Usability Evaluation for Evidence-Based Psychosocial Interventions (USE-EBPI): A methodology for assessing complex intervention implementability. Implementation Research and Practice, 1 , 263348952093292. https://doi.org/10.1177/2633489520932924
McBain, H., Shipley, M., & Newman, S. (2015). The impact of self-monitoring in chronic illness on healthcare utilisation: A systematic review of reviews. BMC Health Services Research, 15 (1), 565. https://doi.org/10.1186/s12913-015-1221-5
McDonald, A. M., Treweek, S., Shakur, H., Free, C., Knight, R., Speed, C., & Campbell, M. K. (2011). Using a business model approach and marketing techniques for recruitment to clinical trials. Trials, 12 (1), 74. https://doi.org/10.1186/1745-6215-12-74
Mestdagh, M., Verdonck, S., Piot, M., Niemeijer, K., Tuerlinckx, F., Kuppens, P., & Dejonckheere, E. (2022). m-Path: An easy-to-use and flexible platform for ecological momentary assessment and intervention in behavioral research and clinical practice. PsyArXiv . https://doi.org/10.31234/osf.io/uqdfs
Mettert, K., Lewis, C., Dorsey, C., Halko, H., & Weiner, B. (2020). Measuring implementation outcomes: An updated systematic review of measures’ psychometric properties. Implementation Research and Practice, 1 , 263348952093664. https://doi.org/10.1177/2633489520936644
Miller, C. J., Smith, S. N., & Pugatch, M. (2020). Experimental and quasi-experimental designs in implementation research. Psychiatry Research, 283 , 112452. https://doi.org/10.1016/j.psychres.2019.06.027
Molenaar, P. C. M. (2004). A manifesto on psychology as idiographic science: Bringing the person back into scientific psychology, this time forever. Measurement: Interdisciplinary Research & Perspective, 2 (4), 201–218. https://doi.org/10.1207/s15366359mea0204_1
Moore, G. C., & Benbasat, I. (1991). Development of an instrument to measure the perceptions of adopting aniInformation technology innovation. Information Systems Research, 2 (3), 192–222. https://doi.org/10.1287/isre.2.3.192
Morris, M., Schindehutte, M., & Allen, J. (2005). The entrepreneur’s business model: Toward a unified perspective. Journal of Business Research, 58 (6), 726–735. https://doi.org/10.1016/j.jbusres.2003.11.001
Myin-Germeys, I., & Kuppens, P. (Eds.). (2021). The open handbook of experience sampling methodology: A step-by-step guide to designing, conducting, and analyzing ESM studies . Amazon.
Nikles, J., Onghena, P., Vlaeyen, J. W. S., Wicksell, R. K., Simons, L. E., McGree, J. M., & McDonald, S. (2021). Establishment of an international collaborative network for n-of-1 trials and single-case designs. Contemporary Clinical Trials Communications, 23 , 100826. https://doi.org/10.1016/j.conctc.2021.100826
Nikles, J., O’Sullivan, J. D., Mitchell, G. K., Smith, S. S., McGree, J. M., Senior, H., Dissanyaka, N., & Ritchie, A. (2019). Protocol: Using N-of-1 tests to identify responders to melatonin for sleep disturbance in Parkinson’s disease. Contemporary Clinical Trials Communications, 15 , 100397. https://doi.org/10.1016/j.conctc.2019.100397
Article CAS PubMed PubMed Central Google Scholar
Nosek, B. A., Alter, G., Banks, G. C., Borsboom, D., Bowman, S. D., Breckler, S. J., Buck, S., Chambers, C. D., Chin, G., Christensen, G., Contestabile, M., Dafoe, A., Eich, E., Freese, J., Glennerster, R., Goroff, D., Green, D. P., Hesse, B., Humphreys, M., & Yarkoni, T. (2015). Promoting an open research culture. Science, 348 (6242), 1422–1425. https://doi.org/10.1126/science.aab2374
Osterwalder, A., Pigneur, Y., & Clark, T. (2010). Business model generation: A handbook for visionaries, game changers, and challengers . Wiley.
Palmieri, A., Fernandez, K. C., Cariolato, Y., Kleinbub, J. R., Salvatore, S., & Gross, J. J. (2022). Emotion regulation in psychodynamic and cognitive-behavioural therapy: An integrative perspective. Clinical Neuropsychiatry., 19 (2), 103–113.
PubMed PubMed Central Google Scholar
Paul, G. L. (1967). Strategy of outcome research in psychotherapy. Journal of Consulting Psychology, 31 (2), 109–118. https://doi.org/10.1037/h0024436
Piccirillo, M. L., Enkema, M. C., & Foster, K. T. (2023). Using the experience sampling method to support clinical practice: An illustration with problematic cannabis use. Psychotherapy: Research Journal of the Society for Psychotherapy Research . https://doi.org/10.1080/10503307.2023.2184284
Piccirillo, M. L., & Rodebaugh, T. L. (2019). Foundations of idiographic methods in psychology and applications for psychotherapy. Clinical Psychology Review, 71 , 90–100. https://doi.org/10.1016/j.cpr.2019.01.002
Pinnock, H., Barwick, M., Carpenter, C. R., Eldridge, S., Grandes, G., Griffiths, C. J., Rycroft-Malone, J., Meissner, P., Murray, E., Patel, A., Sheikh, A., & Taylor, S. J. C. (2017). Standards for reporting implementation studies (StaRI) statement. BMJ, 356 , i6795. https://doi.org/10.1136/bmj.i6795
Piot, M., Mestdagh, M., Riese, H., Weermeijer, J., Brouwer, J. M. A., Kuppens, P., Dejonckheere, E., & Bos, F. M. (2022). Practitioner and researcher perspectives on the utility of ecological momentary assessment in mental health care: A survey study. Internet Interventions, 30 , 100575. https://doi.org/10.1016/j.invent.2022.100575
Powell, B. J., Waltz, T. J., Chinman, M. J., Damschroder, L. J., Smith, J. L., Matthieu, M. M., Proctor, E. K., & Kirchner, J. E. (2015). A refined compilation of implementation strategies: Results from the Expert Recommendations for Implementing Change (ERIC) project. Implementation Science, 10 (1), 21. https://doi.org/10.1186/s13012-015-0209-1
Proctor, E. K., Powell, B. J., & McMillen, J. C. (2013). Implementation strategies: Recommendations for specifying and reporting. Implementation Science, 8 (1), 139. https://doi.org/10.1186/1748-5908-8-139
Proctor, E. K., Silmere, H., Raghavan, R., Hovmand, P., Aarons, G., Bunger, A., Griffey, R., & Hensley, M. (2011). Outcomes for implementation research: Conceptual distinctions, measurement challenges, and research agenda. Administration and Policy in Mental Health, 38 (2), 65–76. https://doi.org/10.1007/s10488-010-0319-7
Riese, H., von Klipstein, L., Schoevers, R. A., van der Veen, D. C., & Servaas, M. N. (2021). Personalized ESM monitoring and feedback to support psychological treatment for depression: A pragmatic randomized controlled trial (Therap-i). BMC Psychiatry, 21 (1), 143. https://doi.org/10.1186/s12888-021-03123-3
Rintala, A., Wampers, M., Myin-Germeys, I., & Viechtbauer, W. (2019). Response compliance and predictors thereof in studies using the experience sampling method. Psychological Assessment, 31 (2), 2. https://doi.org/10.1037/pas0000662
Ryan, O., Haslbeck, J. M. B., & Waldorp, L. (2023). Non-stationarity in time-series analysis: Modeling stochastic and deterministic trends. PsyArXiv . https://doi.org/10.31234/osf.io/z7ja2
Sales, C. M. D., & Alves, P. C. G. (2016). Patient-centered assessment in psychotherapy: A review of individualized tools. Clinical Psychology: Science and Practice, 23 (3), 265–283. https://doi.org/10.1037/h0101737
Article ADS Google Scholar
Sales, C. M. D., Ashworth, M., Ayis, S., Barkham, M., Edbrooke-Childs, J., Faísca, L., Jacob, J., Xu, D., & Cooper, M. (2023). Idiographic patient reported outcome measures (I-PROMs) for routine outcome monitoring in psychological therapies: Position paper. Journal of Clinical Psychology, 79 (3), 596–621. https://doi.org/10.1002/jclp.23319
Schemer, L., Glombiewski, J. A., & Scholten, S. (2022). All good things come in threes: A systematic review and Delphi study on the advances and challenges of ambulatory assessments, network analyses, and single-case experimental designs. Clinical Psychology: Science and Practice . https://doi.org/10.1037/cps0000083
Schemer, L., Vlaeyen, J. W. S., Doerr, J. M., Skoluda, N., Nater, U. M., Rief, W., & Glombiewski, J. A. (2018). Treatment processes during exposure and cognitive-behavioral therapy for chronic back pain: A single-case experimental design with multiple baselines. Behaviour Research and Therapy, 108 , 58–67. https://doi.org/10.1016/j.brat.2018.07.002
Schiele, H., Krummaker, S., Hoffmann, P., & Kowalski, R. (2022). The “research world café” as method of scientific enquiry: Combining rigor with relevance and speed. Journal of Business Research, 140 , 280–296. https://doi.org/10.1016/j.jbusres.2021.10.075
Schleider, J. L., Sung, J., Bianco, A., Gonzalez, A., Vivian, D., & Mullarkey, M. C. (2020). Open pilot trial of a single-session consultation service for clients on psychotherapy wait-lists. PsyArXiv . https://doi.org/10.31234/osf.io/fdwqk
Schrepp, M., Hinderks, A., & Thomaschewski, J. (2017). Design and evaluation of a short version of the User Experience Questionnaire (UEQ-S). International Journal of Interactive Multimedia and Artificial Intelligence, 4 (6), 103. https://doi.org/10.9781/ijimai.2017.09.001
Selker, H. P., Cohen, T., D’Agostino, R. B., Dere, W. H., Ghaemi, S. N., Honig, P. K., Kaitin, K. I., Kaplan, H. C., Kravitz, R. L., Larholt, K., McElwee, N. E., Oye, K. A., Palm, M. E., Perfetto, E., Ramanathan, C., Schmid, C. H., Seyfert-Margolis, V., Trusheim, M., & Eichler, H. (2022). A useful and sustainable role for N-of-1 trials in the healthcare ecosystem. Clinical Pharmacology & Therapeutics, 112 (2), 224–232. https://doi.org/10.1002/cpt.2425
Shapiro, M. B. (1961). A method of measuring psychological changes specific to the individual psychiatric patient*. British Journal of Medical Psychology, 34 (2), 151–155. https://doi.org/10.1111/j.2044-8341.1961.tb00940.x
Sibalija, J., Barrett, D., Subasri, M., Bitacola, L., & Kim, R. B. (2021). Understanding value in a healthcare setting: An application of the business model canvas. Methodological Innovations, 14 (3), 205979912110504. https://doi.org/10.1177/20597991211050477
Simon, G. E., & Perlis, R. H. (2010). Personalized medicine for depression: Can we match patients with treatments? The American Journal of Psychiatry, 167 (12), 1445–1455. https://doi.org/10.1176/appi.ajp.2010.09111680
Simons, C. J. P., Hartmann, J., Kramer, I., Menne-Lothmann, C., Höhn, P., van Bemmel, A. L., Myin-Germeys, I., Delespaul, P., van Os, J., & Wichers, M. (2015). Effects of momentary self-monitoring on empowerment in a randomized controlled trial in patients with depression. European Psychiatry, 30 (8), 8. https://doi.org/10.1016/j.eurpsy.2015.09.004
Slattery, P., Saeri, A. K., & Bragge, P. (2020). Research co-design in health: A rapid overview of reviews. Health Research Policy and Systems, 18 (1), 17. https://doi.org/10.1186/s12961-020-0528-9
Staniszewska, S., Brett, J., Simera, I., Seers, K., Mockford, C., Goodlad, S., Altman, D. G., Moher, D., Barber, R., Denegri, S., Entwistle, A., Littlejohns, P., Morris, C., Suleman, R., Thomas, V., & Tysall, C. (2017). GRIPP2 reporting checklists: Tools to improve reporting of patient and public involvement in research. Research Involvement and Engagement, 3 (1), 13. https://doi.org/10.1186/s40900-017-0062-2
Tamir, M. (2016). Why do people regulate their emotions? A taxonomy of motives in emotion regulation. Personality and Social Psychology Review, 20 (3), 199–222. https://doi.org/10.1177/1088868315586325
Tanious, R., & Onghena, P. (2019). Randomized single-case experimental designs in healthcare research: What, why, and how? Healthcare, 7 , 143.
Tate, R. L., Perdices, M., Rosenkoetter, U., Wakim, D., Godbee, K., Togher, L., & McDonald, S. (2013). Revision of a method quality rating scale for single-case experimental designs and n-of-1 trials: The 15-item Risk of Bias in N-of-1 Trials (RoBiNT) Scale. Neuropsychological Rehabilitation, 23 (5), 619–638.
Tindall, R. M., Ferris, M., Townsend, M., Boschert, G., & Moylan, S. (2021). A first-hand experience of co-design in mental health service design: Opportunities, challenges, and lessons. International Journal of Mental Health Nursing, 30 (6), 1693–1702. https://doi.org/10.1111/inm.12925
Turner-Bowker, D. M., Lamoureux, R. E., Stokes, J., Litcher-Kelly, L., Galipeau, N., Yaworsky, A., Solomon, J., & Shields, A. L. (2018). Informing a priori sample size estimation in qualitative concept elicitation interview studies for clinical outcome assessment instrument development. Value in Health, 21 (7), 839–842. https://doi.org/10.1016/j.jval.2017.11.014
Vachon, H., Viechtbauer, W., Rintala, A., & Myin-Germeys, I. (2019). Compliance and retention with the experience sampling method over the continuum of severe mental disorders: Meta-analysis and recommendations. Journal of Medical Internet Research, 21 (12), 12. https://doi.org/10.2196/14475
Varadhan, R., Segal, J. B., Boyd, C. M., Wu, A. W., & Weiss, C. O. (2013). A framework for the analysis of heterogeneity of treatment effect in patient-centered outcomes research. Journal of Clinical Epidemiology, 66 (8), 818–825. https://doi.org/10.1016/j.jclinepi.2013.02.009
Velten, J., Margraf, J., Benecke, C., Berking, M., In-Albon, T., Tania, L., Lutz, W., Schlarb, A., Schöttke, H., Willutzki, U., & Jürgen H. (2017). Methodenpapier zur Koordination der Datenerhebung und -auswertung an Hochschul- und Ausbildungsambulanzen für Psychotherapie (KODAP). Zeitschrift Für Klinische Psychologie Und Psychotherapie, 46 (3), 169–175. https://doi.org/10.1026/1616-3443/a000431
Vlaeyen, J. W. S., Onghena, P., Vannest, K. J., & Kratochwill, T. R. (2022). Single-case experimental designs: Clinical research and practice. In Comprehensive clinical psychology (pp. 1–28). Elsevier. https://doi.org/10.1016/B978-0-12-818697-8.00191-6
Vlaeyen, J. W. S., Wicksell, R. K., Simons, L. E., Gentili, C., De, T. K., Tate, R. L., Vohra, S., Punja, S., Linton, S. J., Sniehotta, F. F., & Onghena, P. (2020). From Boulder to Stockholm in 70 years: Single case experimental designs in clinical research. The Psychological Record . https://doi.org/10.1007/s40732-020-00402-5
Weisz, J. R., Chorpita, B. F., Frye, A., Ng, M. Y., Lau, N., Bearman, S. K., Ugueto, A. M., Langer, D. A., Hoagwood, K. E., & The Research Network on Youth Mental Health. (2011). Youth top problems: Using idiographic, consumer-guided assessment to identify treatment needs and to track change during psychotherapy. Journal of Consulting and Clinical Psychology, 79 (3), 369–380. https://doi.org/10.1037/a0023307
Wilson, K., Bell, C., Wilson, L., & Witteman, H. (2018). Agile research to complement agile development: A proposal for an mHealth research lifecycle. Npj Digital Medicine, 1 (1), 46. https://doi.org/10.1038/s41746-018-0053-1
Wright, A. G. C., & Woods, A. C. (2020). Personalized models of psychopathology. In T. Widiger & T. D. Cannon (Eds.), Annual review of clinical psychology, Vol 16, 2020 (Vol. 16, pp. 49–74). Annual Reviews. https://doi.org/10.1146/annurev-clinpsy-102419-125032
Wright, A. G. C., & Zimmermann, J. (2019). Applied ambulatory assessment: Integrating idiographic and nomothetic principles of measurement. Psychological Assessment, 31 (12), 1467–1480. https://doi.org/10.1037/pas0000685
Wrzus, C., & Neubauer, A. B. (2023). Ecological momentary assessment: A meta-analysis on designs, samples, and compliance across research fields. Assessment . https://doi.org/10.1177/10731911211067
Download references
Acknowledgements
We would like to thank Johan W. S. Vlaeyen for his continuous reflections regarding the implementation of a SCED infrastructure.
Open Access funding enabled and organized by Projekt DEAL. No funding was received to assist with the preparation of this manuscript.
Author information
Authors and affiliations.
Department of Psychology, Pain and Psychotherapy Research Lab, RPTU Kaiserslautern-Landau, Ostbahnstr. 10, 76829, Landau, Germany
Saskia Scholten, Lea Schemer, Philipp Herzog, Julia W. Haas, Jens Heider, Dorina Winter & Julia Anna Glombiewski
Department of Psychology, Harvard University, 33 Kirkland Street, Cambridge, MA, 02138, USA
Philipp Herzog
Applied Statistical Modeling, Universität des Saarlandes, Campus, 66123, Saarbrücken, Germany
Dorota Reis
You can also search for this author in PubMed Google Scholar
Contributions
All authors contributed to the manuscript conception and design. The original draft of the manuscript was written by Saskia Scholten and Lea Schemer. Methodological considerations were developed by Saskia Scholten, Lea Schemer, and Philipp Herzog. Visualization was realized by Saskia Scholten and Julia W. Haas. Supervision was provided by Julia Anna Glombiewski. All authors reviewed and edited previous versions of the manuscript. All authors read and approved the final manuscript.
Corresponding author
Correspondence to Saskia Scholten .
Ethics declarations
Conflict of interest.
The authors have no competing interests to declare that are relevant to the content of this article.
Ethical Approval
All procedures performed in studies involving human participants will be in accordance with the ethical standards of the institutional and/or national research committee and with the 1964 Helsinki Declaration and its later amendments or comparable ethical standards. We considered the Single-Case Reporting Guideline in Behavioral Intervenions (SCRIBE) 2016 Statement (Tate et al., 2016) and Standards for Reporting Implementation Studies (StaRI) Statement (Pinnock et al., 2017 ) to draft this manuscript.
Additional information
Publisher's note.
Springer Nature remains neutral with regard to jurisdictional claims in published maps and institutional affiliations.
Supplementary Information
Below is the link to the electronic supplementary material.
Supplementary file1 (PDF 118 kb)
Supplementary file2 (pdf 62 kb), supplementary file3 (pdf 70 kb), rights and permissions.
Open Access This article is licensed under a Creative Commons Attribution 4.0 International License, which permits use, sharing, adaptation, distribution and reproduction in any medium or format, as long as you give appropriate credit to the original author(s) and the source, provide a link to the Creative Commons licence, and indicate if changes were made. The images or other third party material in this article are included in the article's Creative Commons licence, unless indicated otherwise in a credit line to the material. If material is not included in the article's Creative Commons licence and your intended use is not permitted by statutory regulation or exceeds the permitted use, you will need to obtain permission directly from the copyright holder. To view a copy of this licence, visit http://creativecommons.org/licenses/by/4.0/ .
Reprints and permissions
About this article
Scholten, S., Schemer, L., Herzog, P. et al. Leveraging Single-Case Experimental Designs to Promote Personalized Psychological Treatment: Step-by-Step Implementation Protocol with Stakeholder Involvement of an Outpatient Clinic for Personalized Psychotherapy. Adm Policy Ment Health (2024). https://doi.org/10.1007/s10488-024-01363-5
Download citation
Accepted : 27 February 2024
Published : 11 March 2024
DOI : https://doi.org/10.1007/s10488-024-01363-5
Share this article
Anyone you share the following link with will be able to read this content:
Sorry, a shareable link is not currently available for this article.
Provided by the Springer Nature SharedIt content-sharing initiative
- Single-case experimental design
- Experience sampling method
- Ecological momentary assessment
- Personalized psychotherapy
- Stakeholder involvement
- Implementation research
- Find a journal
- Publish with us
- Track your research
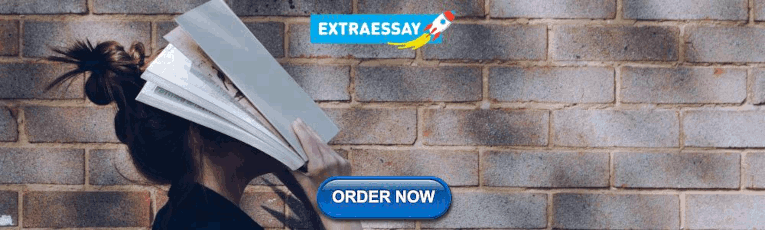
IMAGES
VIDEO
COMMENTS
Single-case studies can provide a viable alternative to large group studies such as randomized clinical trials. Single case studies involve repeated measures, and manipulation of and independent variable. They can be designed to have strong internal validity for assessing causal relationships between interventions and outcomes, and external ...
Abstract. This chapter addresses the peculiarities, characteristics, and major fallacies of single case research designs. A single case study research design is a collective term for an in-depth analysis of a small non-random sample. The focus on this design is on in-depth.
The single-case experiment has a storied history in psychology dating back to the field's founders: Fechner (1889), Watson (1925), and Skinner (1938).It has been used to inform and develop theory, examine interpersonal processes, study the behavior of organisms, establish the effectiveness of psychological interventions, and address a host of other research questions (for a review, see ...
Within a case study research, one may study a single case or multiple cases. Single case studies are most common in case study researches. Yin (2014, p. 59) says that single cases are 'eminently justifiable' under certain conditions: (a) when the case under study is unique or atypical, and hence, its study is revelatory, (b) when the case ...
Studies that use a single-case design (SCD) measure outcomes for cases (such as a child or family) repeatedly during multiple phases of a study to determine the success of an intervention. The number of phases in the study will depend on the research questions, intervention, and outcome(s) of interest (see Types of SCDs on page 4 for examples).
This book is a compendium of tools and information for researchers considering single-case design (SCD) research, a newly viable and often essential methodology in applied psychology, education, and related fields. ... Single-Case Designs and Large-N Studies: The Best of Both Worlds Susan M. Sheridan; Using Single-Case Research Designs in ...
In designing single-case research studies, it is essential that researchers select the appropriate research design for the specific research question and dependent variable. ... Single-case design research can be used to address a number of different types of research questions regarding treatment effectiveness that are likely to be of interest ...
Tactics of Scientific Research (Sidman, 1960) provides a visionary treatise on single-case designs, their scientific underpinnings, and their critical role in understanding behavior. Since the foundational base was provided, single-case designs have proliferated especially in areas of application where they have been used to evaluate interventions with an extraordinary range of clients ...
Either single-case or multiple-case designs may be used in case study research. Single-case designs are usually appropriate where the case represents a critical case (it meets all the necessary conditions for testing a theory), where it is an extreme or unique case, where it is a revelatory case, or where the research is exploratory (Yin 1994 ...
A comprehensive reference about the process of designing and conducting single-case experimental design studies. Chapters are integrative but can stand alone. Kazdin, A. E. 2011. Single-case research designs: Methods for clinical and applied settings. 2d ed. New York: Oxford Univ. Press.
Single-case design has a long history of use for assessing intervention effectiveness for children with disabilities. Although these designs have been widely employed for more than 50 years, recent years have been especially dynamic in terms of growth in the use of single-case design and application of standards designed to improve the validity and applicability of findings.
We highlight current research designs, analysis techniques, and quality appraisal tools relevant for single-case rehabilitation research. Summary of key points: Single-case studies can provide a viable alternative to large group studies such as randomized clinical trials. Single-case studies involve repeated measures and manipulation of an ...
A single-case design is an experimental design for a study in which one entity is observed repeatedly during a certain period under different levels of at least one independent variable. Single ...
Abstract. Single-case experimental designs (SCEDs) represent a family of research designs that use experimental methods to study the effects of treatments on outcomes. The fundamental unit of analysis is the single case—which can be an individual, clinic, or community—ideally with replications of effects within and/or between cases.
A special issue of Perspectives on Behavior Science focused on methodological advances needed for single-case research is a timely contribution to the field. There are growing efforts to both articulate professional standards for single-case methods (Kratochwill et al., 2010; Tate et al., 2016), and advance new procedures for analysis and interpretation of single-case studies (Manolov ...
In this article, we discuss how open-science practices align with other recent developments in single-case design research, describe four prominent open-science practices (i.e., preregistration, registered reports, data and materials sharing, and open access), and discuss potential benefits and limitations of each practice for single-case design.
The clinician in practice is apt to select the AB 1 B 2, changing criterion design. The single case approach provides a means of measuring the increased amount of an intervention. For example, in Kazdin, 2 increased expected levels of quiz performance are used across math objectives 1, 2, 3 and 4 as measured during daily school sessions. 3, 5.
General Readings on Single-Case Research Design and Analysis: Barlow DH, Nock MK, Hersen M. Single Case Experimental Designs: Strategies for Studying Behavior Change. 3rd ed. Needham Heights, MA: Allyn & Bacon; 2008. 3. Kazdin AE. Single-Case Research Designs: Methods for Clinical and Applied Settings. New York, NY: Oxford University Press; 2010. 4
This chapter addresses single-case research designs' peculiarities, characteristics, and significant fallacies. A single case research design is a collective term for an in-depth analysis of a small non-random sample. The focus of this design is in-depth. ... How does the single case study research design differ from the action research design?
Single-case experimental designs (SCEDs) have become a popular research methodology in educational science, psychology, and beyond. The growing popularity has been accompanied by the development of specific guidelines for the conduct and analysis of SCEDs. In this paper, we examine recent practices in the conduct and analysis of SCEDs by systematically reviewing applied SCEDs published over a ...
Direct replication is the strategy of repeating a study with no procedural changes to assess the reliability of a finding. This can be accomplished in the original study or in a separate study by the original or new researchers. In single-case design research, this type of replication is most apparent in the ABAB design, which includes an ...
Method: The single case study design involved a 35-year-old woman with a comorbid diagnosis of C-PTSD and BED, ... which she was stable on at least 2 months before starting the research study. When treatment began, Anne was bingeing multiple times per day in a self-reported attempt to "numb" negative emotions and intrusive thoughts.
We adopted an embedded single-case design with two units of analysis (Yin Citation 2015, p. 50): the project organization and the social media message. ... Research limitations. The present case study design has some limitations. The study was based on a single case where, for instance, social and environmental issues were not particularly ...
2. Single-Case Experimental Designs: Definition and Overview of Design Options. Contemporary textbooks on SCEDs follow a long tradition. An early and fierce proponent of SCEDs was B.F. Skinner [], proclaiming in his 1956 seminal paper on the scientific method that "we are within reach of a science of the individual.This will be achieved, not by resorting to some special theory of knowledge ...
Single-case experimental designs (SCEDs) are increasingly used in fields such as clinical psychology and educational psychology for the evaluation of treatments and interventions in individual participants. The AB phase design, also known as the interrupted time series design, is one of the most basic SCEDs used in practice. Randomization can be included in this design by randomly determining ...
Our objective is to implement a single-case experimental design (SCED) infrastructure in combination with experience-sampling methods (ESM) into the standard diagnostic procedure of a German outpatient research and training clinic. Building on the idea of routine outcome monitoring, the SCED infrastructure introduces intensive longitudinal data collection, individual effectiveness measures ...