- Learning Tips
- Exam Guides
- School Life
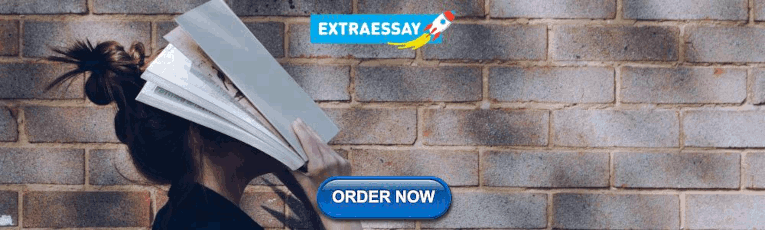
Thesis Statements about Social Media: 21 Examples and Tips
- by Judy Jeni
- January 27, 2024
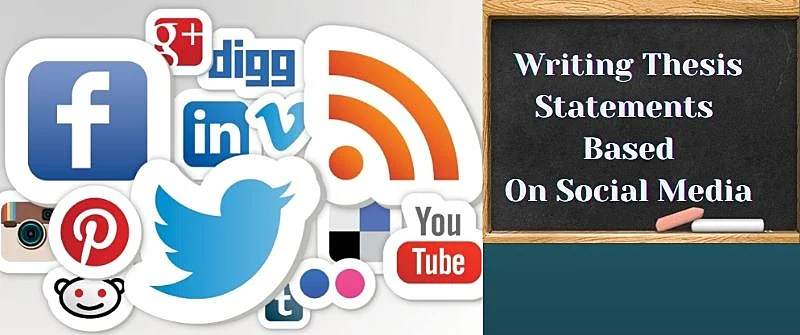
A thesis statement is a sentence in the introduction paragraph of an essay that captures the purpose of the essay. Using thesis statements about social media as an example, I will guide you on how to write them well.
It can appear anywhere in the first paragraph of the essay but it is mostly preferred when it ends the introduction paragraph. learning how to write a thesis statement for your essay will keep you focused.
A thesis statement can be more than one sentence only when the essay is on complex topics and there is a need to break the statement into two. This means, a good thesis statement structures an essay and tells the reader what an essay is all about.
A good social media thesis statement should be about a specific aspect of social media and not just a broad view of the topic.
The statement should be on the last sentence of the first paragraph and should tell the reader about your stand on the social media issue you are presenting or arguing in the essay.
Reading an essay without a thesis statement is like solving a puzzle. Readers will have to read the conclusion to at least grasp what the essay is all about. It is therefore advisable to craft a thesis immediately after researching an essay.
Throughout your entire writing, every point in every paragraph should connect to the thesis. In case it doesn’t then probably you have diverged from the main issue of the essay.
How to Write a Thesis Statement?
Writing a thesis statement is important when writing an essay on any topic, not just about social media. It is the key to holding your ideas and arguments together into just one sentence.
The following are tips on how to write a good thesis statement:
Start With a Question and Develop an Answer
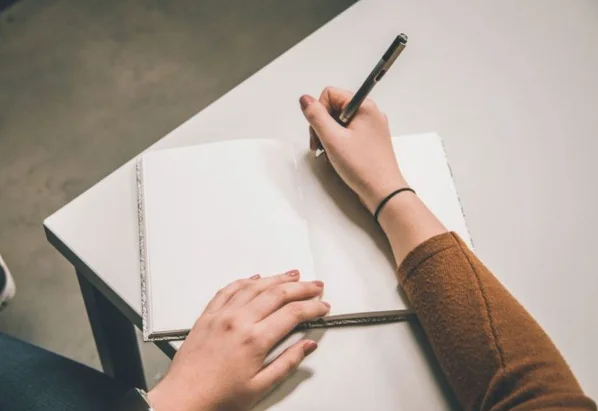
If the question is not provided, come up with your own. Start by deciding the topic and what you would like to find out about it.
Secondly, after doing some initial research on the topic find the answers to the topic that will help and guide the process of researching and writing.
Consequently, if you write a thesis statement that does not provide information about your research topic, you need to construct it again.
Be Specific
The main idea of your essay should be specific. Therefore, the thesis statement of your essay should not be vague. When your thesis statement is too general, the essay will try to incorporate a lot of ideas that can contribute to the loss of focus on the main ideas.
Similarly, specific and narrow thesis statements help concentrate your focus on evidence that supports your essay. In like manner, a specific thesis statement tells the reader directly what to expect in the essay.
Make the Argument Clear
Usually, essays with less than one thousand words require the statement to be clearer. Remember, the length of a thesis statement should be a single sentence, which calls for clarity.
In these short essays, you do not have the freedom to write long paragraphs that provide more information on the topic of the essay.
Likewise, multiple arguments are not accommodated. This is why the thesis statement needs to be clear to inform the reader of what your essay is all about.
If you proofread your essay and notice that the thesis statement is contrary to the points you have focused on, then revise it and make sure that it incorporates the main idea of the essay. Alternatively, when the thesis statement is okay, you will have to rewrite the body of your essay.
Question your Assumptions
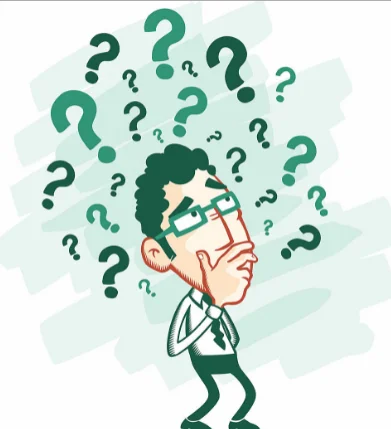
Before formulating a thesis statement, ask yourself the basis of the arguments presented in the thesis statement.
Assumptions are what your reader assumes to be true before accepting an argument. Before you start, it is important to be aware of the target audience of your essay.
Thinking about the ways your argument may not hold up to the people who do not subscribe to your viewpoint is crucial.
Alongside, revise the arguments that may not hold up with the people who do not subscribe to your viewpoint.
Take a Strong Stand
A thesis statement should put forward a unique perspective on what your essay is about. Avoid using observations as thesis statements.
In addition, true common facts should be avoided. Make sure that the stance you take can be supported with credible facts and valid reasons.
Equally, don’t provide a summary, make a valid argument. If the first response of the reader is “how” and “why” the thesis statement is too open-ended and not strong enough.
Make Your Thesis Statement Seen
The thesis statement should be what the reader reads at the end of the first paragraph before proceeding to the body of the essay. understanding how to write a thesis statement, leaves your objective summarized.
Positioning may sometimes vary depending on the length of the introduction that the essay requires. However, do not overthink the thesis statement. In addition, do not write it with a lot of clever twists.
Do not exaggerate the stage setting of your argument. Clever and exaggerated thesis statements are weak. Consequently, they are not clear and concise.
Good thesis statements should concentrate on one main idea. Mixing up ideas in a thesis statement makes it vague. Read on how to write an essay thesis as part of the steps to write good essays.
A reader may easily get confused about what the essay is all about if it focuses on a lot of ideas. When your ideas are related, the relation should come out more clearly.
21 Examples of Thesis Statements about Social Media
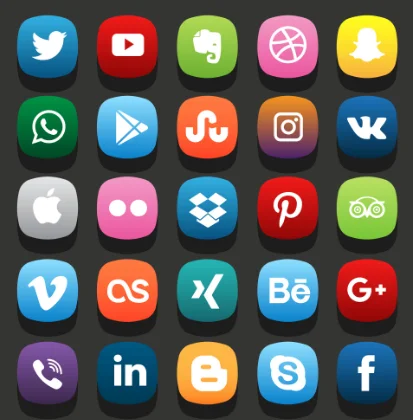
- Recently, social media is growing rapidly. Ironically, its use in remote areas has remained relatively low.
- Social media has revolutionized communication but it is evenly killing it by limiting face-to-face communication.
- Identically, social media has helped make work easier. However,at the same time it is promoting laziness and irresponsibility in society today.
- The widespread use of social media and its influence has increased desperation, anxiety, and pressure among young youths.
- Social media has made learning easier but its addiction can lead to bad grades among university students.
- As a matter of fact, social media is contributing to the downfall of mainstream media. Many advertisements and news are accessed on social media platforms today.
- Social media is a major promoter of immorality in society today with many platforms allowing sharing of inappropriate content.
- Significantly, social media promotes copycat syndrome that positively and negatively impacts the behavior adapted by different users.
- In this affluent era, social media has made life easy but consequently affects productivity and physical strength.
- The growth of social media and its ability to reach more people increases growth in today’s business world.
- The freedom on social media platforms is working against society with the recent increase in hate speech and racism.
- Lack of proper verification when signing up on social media platforms has increased the number of minors using social media exposing them to cyberbullying and inappropriate content.
- The freedom of posting anything on social media has landed many in trouble making the need to be cautious before posting anything important.
- The widespread use of social media has contributed to the rise of insecurity in urban centers
- Magazines and journals have spearheaded the appreciation of all body types but social media has increased the rate of body shaming in America.
- To stop abuse on Facebook and Twitter the owners of these social media platforms must track any abusive post and upload and ban the users from accessing the apps.
- Social media benefits marketing by creating brand recognition, increasing sales, and measuring success with analytics by tracking data.
- Social media connects people around the globe and fosters new relationships and the sharing of ideas that did not exist before its inception.
- The increased use of social media has led to the creation of business opportunities for people through social networking, particularly as social media influencers.
- Learning is convenient through social media as students can connect with education systems and learning groups that make learning convenient.
- With most people spending most of their free time glued to social media, quality time with family reduces leading to distance relationships and reduced love and closeness.
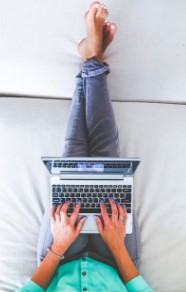
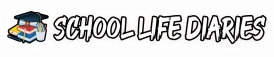
Crafting A Thesis Statement About Social Media: Tips And Tricks
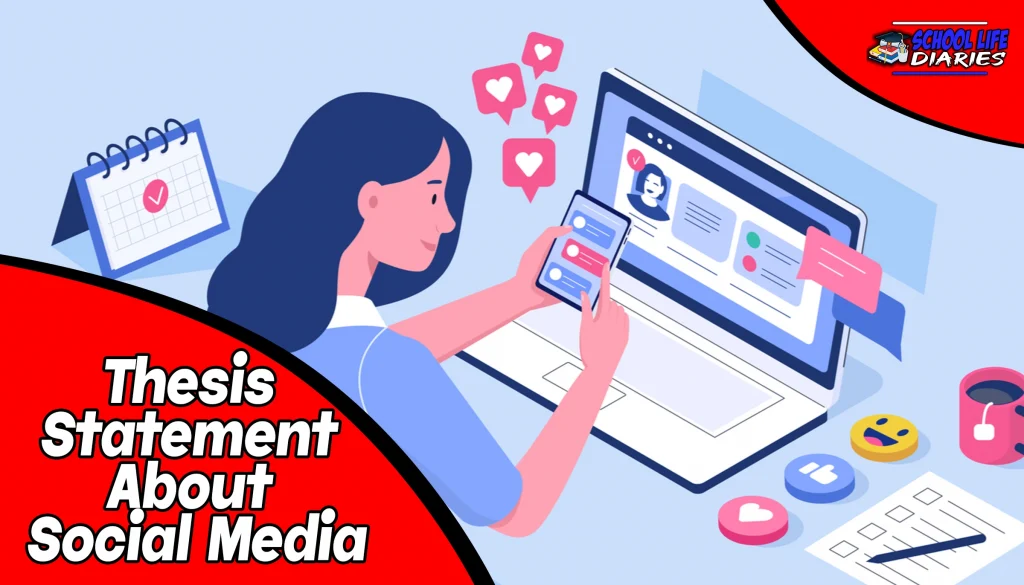
With the rise of online platforms and thesis statement social media in our lives, students and teachers alike are increasingly asking questions about whether or not this technology is beneficial to academic performance. While it can be argued that there are both pros and cons to using social media. Many studies now show how integrating different forms of social media into classroom-based teaching can have tremendous positive benefits such as promoting greater collaboration between peers.
Asynchronous learning opportunities for a wider variety of topics, increased engagement among students to participate in activities related to areas of study, improved access to resources outside the textbook, as well as an overall boost in communication with all stakeholders involved within any given educational setting. In this blog post, we will explore these topics and provide a thesis statement about the impacts that social media has today on modern education.
How To Write a Thesis Statement About Social Media?
1. understand the topic:.
Before attempting to write a thesis statement on social media, it is important to understand the topic. In this case, you need to familiarize yourself with what social media is and what aspects are associated with it. Researching different sources such as news articles, blogs, and academic papers can help provide insight into the topic.
2. Brainstorm Potential Ideas:
After gaining an understanding of the topic, you should brainstorm potential ideas for your thesis statement. Think about what information you found during your research and develop a few statements that capture the essence of it. It is also useful to consider any questions or opinions you have on the subject matter.
3. Refine the Ideas:
Once you have a few potential ideas for your thesis statement, it is time to narrow them down. Evaluate each idea and determine which one best encapsulates the points you want to make about social media. Re-write this statement in its strongest form and consider how it can be further refined.
4. Finalize Your Thesis Statement:
After you have thoroughly examined each idea, you can finalize your thesis statement. Make sure it accurately reflects the points you want to make about social media and that it is written in a clear and concise manner. Once this is done, your thesis statement on social media will be complete.
5. Support Your Thesis Statement:
To make your thesis statement even stronger, it is important to provide evidence and support for your claims. This may involve conducting additional research or gathering statistics that back up what you are saying. Doing this will create a well-rounded argument and help communicate the message of the thesis statement more effectively.
6. Revise and Edit:
The final step in writing your thesis statement is to revise and edit it. Read through the statement several times, paying attention to spelling, grammar, and syntax. You can also ask someone else to read over it as well to ensure that it is clear and accurate. Once everything has been checked, your thesis statement on social media is ready to be presented.
What Is a Social Media Thesis Statement?
A Social Media Thesis Statement is a statement that expresses the key idea of an argument about the influence of social media platforms on modern society. It could be argued that social media has had both positive and negative impacts on people, from creating new opportunities for communication and connection to contributing to issues like cyberbullying, addiction, and data security.
The overall effect of social media on our lives is still unclear and needs further exploration. This thesis statement serves as a starting point for further research into the role of social media in today’s society. Through exploring the effects of social media on individuals, communities, and even global society, we can gain insight into how these platforms are shaping the world we live in.
What Are Social Networks On The Internet?
Social networks on the Internet are online platforms that allow individuals to connect and interact with one another in a virtual space. These networks often involve user profiles, friends lists, and other features that allow users to post content such as photos, text, and videos. Many social networks allow users to comment on others’ posts, join groups, and join conversations.
The use of social networks has become a popular part of everyday life, providing users with a convenient way to stay connected and share information. From personal connections to professional opportunities, these networks offer various benefits that can be utilized by both individuals and businesses.
Do We Know Where Social Networks Originate?
Social networks have become an increasingly popular way of connecting with people all over the world, allowing us to communicate with friends, family, and acquaintances. However, it can be difficult to determine the exact origin of these networks.
The first concept resembling a social network was Six Degrees, which was created by Andrew Weinreich in 1996. This website allowed users to create a profile and connect with other users, as well as find people from around the world. It was successful for a while but eventually failed due to legal issues and a lack of funding.
In 1997, Classmates.com was launched, allowing users to search for former classmates and make connections with them. This was the beginning of social networks becoming an integral part of our lives. Soon after, other popular networks emerged such as Friendster in 2002, LinkedIn in 2003, and MySpace in 2004.
Advantages and Disadvantages of Social Networks On The Internet
Advantages of social networks on the internet.
1. Social networks on the internet provide unprecedented levels of connectivity, allowing users to easily interact with each other regardless of geographical location.
2. Social networks can be used to build communities and create conversations around particular topics or ideas.
3. Many social networks have also opened up their platforms to entrepreneurs, allowing small businesses to have the opportunity to market their products and services more effectively.
4. While social networks do offer many benefits, it is important that users remain cautious when using them.
5. Social networks can provide many opportunities for people to make connections, learn about new topics, and gain insight into different perspectives.
Disadvantages of Social Networks On The Internet
1. Excessive personal information sharing can lead to identity theft and fraud.
2. Unregulated content can be misleading or inappropriate for younger viewers.
3. Connecting with strangers online can put users at risk for cyberbullying, scams, and other malicious acts.
4. Increased distractions from social media use can negatively impact school performance and work productivity.
5. Addiction to social networking sites is common among users, leading to a lack of balance between online/offline relationships and activities.
How To Write A Good Social Media Essay?
1. Choose a topic that interests you and your audience
2. Do extensive research to understand the subject better
3. Brainstorm ideas
4. Outline the essay structure
5. Write an attention-grabbing introduction
6. Create well-structured paragraphs with clear arguments supported by evidence
7. Incorporate visuals (e.g., images, videos, gifs) where appropriate to keep readers engaged
8. Make sure your content is relevant and up-to-date according to social media trends
9. End with a strong conclusion summarizing the main points
10. Proofread for grammar and formatting errors before posting.
Social Media Essay Outline
1. introduction:.
The introduction should provide a brief overview of the topic, explaining what social media is and why it is important in our lives today. It should also introduce the main points to be discussed in the essay and provide an overview of relevant research that has been done. The Introduction should conclude with a clearly defined thesis statement.
2. Thesis Statement:
The purpose of this essay is to explore the effects that social media has had on our lives, both positive and negative, and to provide insight into how we can use it in constructive ways.
The body of the essay should begin by looking at the positives of social media, such as its ability to connect people across the globe and how it can be used to share ideas. It should discuss how social media has created new opportunities for businesses and organizations to reach a wider audience and how it can be used to raise awareness about important issues.
4. Conclusion:
In conclusion, although there are both positives and negatives associated with social media, it is clear that used in the right way it can be an incredibly useful and powerful tool for communication. With a few simple steps, such as using proper caution when consuming information and taking breaks from constant use, anyone can enjoy the benefits of this technology without many of its potential drawbacks.
21 Examples of Thesis Statements About Social Media
1. For individuals, social media can be both a blessing and a curse; it provides a platform for increased connectivity, but can also create or exacerbate feelings of loneliness and depression.
2. Social media has been associated with the rise of “echo chambers” that limit the diversity of perspectives people encounter.
3. Social media can also have a serious impact on mental health, as users are exposed to a barrage of both positive and negative information all at once.
4. It is important for individuals to be conscious of their own use of social media, as well as that of others in order to minimize any potential risks associated with it.
5. Setting limits on time spent online, engaging in meaningful conversations, and taking a break from social media can help to reduce any potential negative effects of its use.
6. It is also important to remember that the content posted on social media does not always reflect the reality of people’s lives.
7. It is crucial to remain mindful of what we see on social media and understand its potential impact on our own mental health.
8. We can ensure a safe and healthy online environment for ourselves and those around us.
9. Social media can be an amazing tool to maintain relationships and foster meaningful dialogue, but it is important to keep in mind the potential pitfalls associated with its use.
10. We can make sure that our experiences on social media are both positive and productive.
11. By understanding the potential risks and actively managing our own use of social media, we can ensure that it remains a force for good in our lives.
12. Through increased self-awareness and mindfulness, we can make sure to get the most out of our interactions on social media while avoiding any potential negative effects.
13. It is important to remember that social media should use in moderation and with care so that it can continue to have a positive impact on the lives of its users.
14. By remaining aware of our own use and encouraging others to do the same, we can ensure that social media remains a safe and healthy space for all.
15. It is important to remember the potential benefits and pitfalls of social media and make sure to use it responsibly.
16. We can ensure a safe and enjoyable experience for everyone who uses it
17. Social media can also be a powerful tool for spreading awareness and promoting positive change in the world.
18. Social media can use to advocate for causes that matter and amplify the voices of those who are often unheard.
19. Through thoughtful engagement and meaningful conversations, we can use social media as a platform to create positive change in the world.
20. When used responsibly, social media can be an invaluable tool to help us build better communities and create a more just and equitable world.
21. By understanding the potential impacts of using social media and taking steps to ensure responsible use, we can make sure that it remains a force for good in our lives.
Related Article: Term Paper: Structure And Tips For Writing A Successful Paper
Conclusion:
As we can see from this study, students who use social media in an educational setting perform better than those who don’t. This is likely because social media provides a more engaging and interactive learning environment. While there are many potential benefits to incorporating social media into instruction, such as promoting collaboration and interaction among students.
There are also some challenges that need to take into account, such as managing student screen time and ensuring online safety. If you’re a teacher, consider incorporating social media into your lesson plans. And if you’re a student, be sure to take advantage of the resources that social media has to offer.
Related Posts
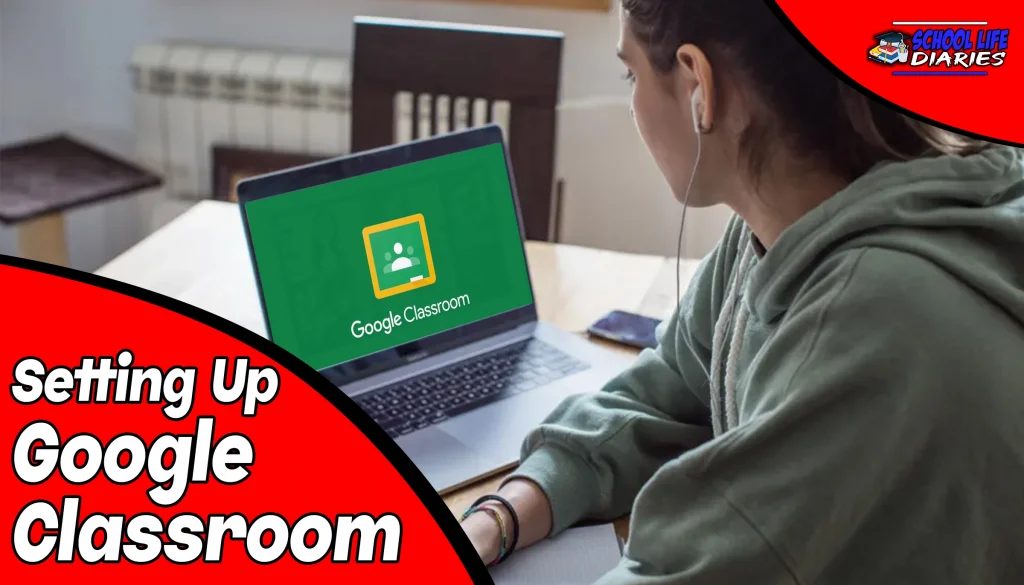
A Complete Parent Guide for Setting Up Google Classroom
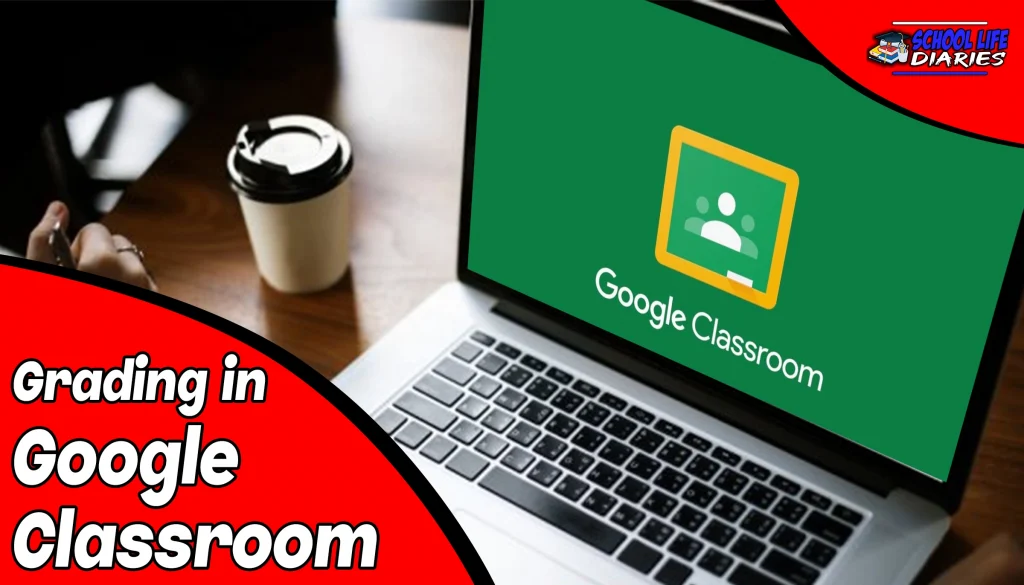
Master the Art of Grading in Google Classroom: Ultimate Guide
Leave a comment cancel reply.
Your email address will not be published. Required fields are marked *
Save my name, email, and website in this browser for the next time I comment.
School Life Diaries
Our website provides interesting and informative content related to school life, teachers, and students. Our articles are written by experienced professionals and provide valuable insights into the world of education.
Whether you’re a student looking for advice or a teacher searching for new ideas, our website is a great resource for anyone interested in learning more about the school experience.
- Teachers Tote
Legal Pages
- Privacy Policy
- Terms of Use
Social Media
A Survey on Social Media Influence Environment and Influencers Identification
- Review Paper
- Published: 03 October 2022
- Volume 12 , article number 145 , ( 2022 )
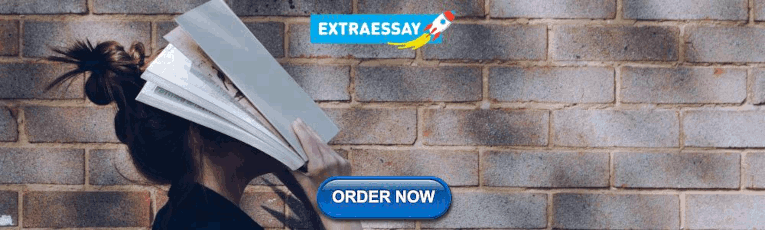
Cite this article
- Feriel Gammoudi ORCID: orcid.org/0000-0002-4715-0028 1 , 2 ,
- Mondher Sendi 2 &
- Mohamed Nazih Omri 2
7276 Accesses
6 Citations
Explore all metrics
Social media users can be influenced directly by their close relationships, such as their friends, family, and colleagues. They can also be influenced by those who follow them through shared information, goals, news, and opinions. Generally, an influencer is someone who entices an influence to do the same action, make the same decision, or change their behavior. He can also communicate information, ideas, and thoughts to multiple users. There are many tools to identify influencers. It can not be found simply through their big follower number or their shared media number. Thus, influencer identification is one of the essential tasks in social media research. Several approaches and metrics have been proposed in the literature to identify influencers. In this article, we explored the issue of identifying social media influencers while providing a generic view of social media influence. First, we presented a literature synthesis on the influence of social media. Then, we categorized the works and illustrated the leading solutions in literature to identify influencers in social media. A discussion and suggestions for potential future directions in this area accompanied this presentation. We believe these briefings are critical to resolving the issue discussed in this article.
Similar content being viewed by others
How to Measure Influence in Social Networks?
What Do We Know About Influencers on Social Media? Toward a New Conceptualization and Classification of Influencers
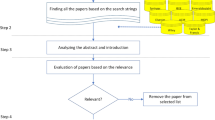
Systematic literature review on identifying influencers in social networks
Seyed Farid Seyfosadat & Reza Ravanmehr
Avoid common mistakes on your manuscript.
1 Introduction
1.1 context.
Digital networks have a huge impact on people’s daily lives, leading dozens of new researchers to study this rapidly growing issue that extends throughout multiple sub-domains. As social networks become more prominent, a researcher’s first logical thought upon analyzing these networks is how to get specific information from the data. That makes marketing applications explore social media to understand user trends and offer new products. Military applications have analyzed them to detect terrorist groups and their further socialization Cialdini and Goldstein ( 2004 ). The health and biomedical fields Sendi and Omri ( 2015 ) have also benefited from social media analysis by exploring discussions on health topics and analyzing patient behaviors. Analysis can also predict relationship quality, event detection and characterization as good or bad Iraklis and Williams ( 2020 ) anticipating the number of followers they might have Imamori and Tajima ( 2016 ), enhancing interaction activity and user similarities Xiang et al. ( 2010 ). And by combining temporal information with a social network structure, user interests Bao et al. ( 2013 ) are predicted.
As the researchers’ results found, the network structure proves to be of utmost importance. A graph with nodes and edges, where each edge symbolizes the connection between two users, whereas each node represents one user, can be used to model a social network. Users have a personal web page and connect with different friends to share content and interact with each other, and build social relationships by following others or adding a theme as a friend.
In associated with social influence Peng et al. ( 2018 ) an influencer entices an influence to do the same action or make the same decision, and there can also be no uncertainty Peng et al. ( 2017 ). Users might well be instantly influenced by their true and intimate relationships (friends, family, classmates, coworkers, etc.) while they spend more time with them, and by famous content creators that they follow, by consulting their shares daily: actuality, goals, news, and opinion. Thus, users are sharing influence while sharing media and posts. The information and knowledge management community has recently attached importance to the topic of identifying significant users from social media as “the source of influence”. Although studies have concentrated on measuring levels of ability to identify knowledgeable users and determining levels of influence to identify influential users. The process starts by analyzing the influence of the nodes and then progressively maximizes the influence of an initial set of nodes to obtain the influential nodes. It is a challenging task that has the potential for considerable usefulness in many applications.
Several important works in the literature deal with influence and many new works have recently been attracted by identifying important and significant influencer nodes. In this paper, we will deal with the social media influence environment, influencer identification, and important nodes, and we will classify important existing works.
The following section discusses the main related surveys cited in the literature.
1.2 Related surveys
Many important works dealing with social media analysis have been proposed in the literature. We are going to classify them according to their state of the art and their metrics. The first social media analysis technique Adedoyin-Olowe et al. ( 2014 ), Tabassum et al. ( 2018 ) was classified as an unsupervised, semi-supervised, or supervised learning method. The SM community detection Azaouzi et al. ( 2019 ) and social media structure discovery have two types of structure networks: static or dynamic according to space and time. Some others worked to drown the social media users’ relationships as a graph with nodes and edges. Authors Bian et al. ( 2019 ) reviewed, analyzed, and classified some main literature works into two main categories: the most influential nodes in the graph and the most significant nodes. Works dealing with the inferring of user interest profiles have been classified in Piao and Breslin ( 2018 ). Another target user personality has been detailed by its authors in Andreassen et al. ( 2016 ). Depending on the sort of machine learning utilized in each approach, the information transmitted could be rumors Alzanin and M.Azmi ( 2018 ). The authors proceeded by dividing the problem of rumor detection into three different categories: hybrid approaches; supervised approaches; and unsupervised approaches. There are also important works dealing with the analysis of social media influence, such as the works detailed in Peng et al. ( 2018 ); Sun and Tang ( 2011 ); Peng et al. ( 2017 ). The authors of the contribution presented in Peng et al. ( 2018 ) classified several notable works using switch algorithms and models. In Sun and Tang ( 2011 ), the authors also described various methods and algorithms for calculating measures related to social influence. An important new work Azaouzi et al. ( 2021 ) deals with the problem of influence maximization under privacy protection. They divide models into two categories: group node-based models and individual models. And, by proposing different algorithms, different methodologies, and also diverse frameworks, they demonstrated that one key solves the problem of maximizing influence at the individual level Kempe et al. ( 2003 ). The perspectives of influencer marketing, their content strategies, and the attractiveness of their sponsored recommendations are reviewed to promote the marketing tactic. The three research factors used by authors to categorize papers were the source, message, and audience Hudders et al. ( 2021 ). Social network applications are becoming highly interested in the topic of community detection Plantie and Crampes ( 2013 ).
There is much work dealing with the influence of others on social media. We aimed to classify some of them subjectively according to their aims and the taxonomy used in classification. Table 1 below summarizes the related surveys.
To the best of our knowledge and after these related surveys studies, no one proposed a classification of influencer node. Moreover, no work has been found to deal with the global problem of social media influencer identification. By comparison with the main related surveys, such as Bian et al. ( 2019 ); Adedoyin-Olowe et al. ( 2014 ); Tabassum et al. ( 2018 ); Piao and Breslin ( 2018 ); Alzanin and M.Azmi ( 2018 ); Hudders et al. ( 2021 ); Andreassen et al. ( 2016 ); Peng et al. ( 2018 ); Plantie and Crampes ( 2013 ) presented previously, our study presents the following major differences:
We are not only dealing with influence maximization or significant node identification. Our aim is the identification of many types of social network influencers.
Unlike some existing surveys, dealing with only one type of social network, that of Twitter, our study exploits different types of social networks, such as micro-blogging, egocentric networks, Google+ dataset, Facebook, Amazon, DBLP, and Wiki.
Our study is characterized by two important points: exhaustiveness and recent bibliographical references. Indeed, our study represents coverage of different fields of application.
1.3 Motivation and contribution
1.3.1 motivation.
Numerous important works in the literature deal with user interests and influence, which have changed over time and have played a vital role in all analysis processes. Thus, we have dealt with social media strategy, the first user influencer, to simulate the real environment of users.
The social media strategy makes users more dependent: content is organized and categorized, and switching algorithms, user activity, and past choices increase the usage rate. One of its strategies is that publication occurrence depends on users’ last choices and their degree of importance, the rate of publication consulted, and users’ litigation. These networks use cookies and algorithms to collect information about them and their behavior. Other websites consult, search, and try to identify the users’ interests. When the latter is looking for something, it starts appearing as a suggestion on social media homepages. This further influences brands to consume their offer. User interest was also guided by photos, videos, and information inspired by his friends. On the homepage are their trending topics, most viewed videos, and their latest picks; similar and international trending topics; international action or famous events; and personal activity.
Social media allows users to follow each other and share details about their activities and postings. They can like each other’s publications, photos, videos, opinions, and disputes. It allows the user to see similar consulted publications, and it offers the possibility to chat and create direct communication and influence. Their friend or an influencer can be inspired by seeing the publication. That is why we thought that social media users were sharing influence.
All of those influence the user’s choice, use, ideas, focus, and personality. Users prefer personalized and guided services that are matched by their choice of searches and hope. So we are dealing with the influence and influencers on social media networks.
1.3.2 Contributions
The main contributions of this article can be summarized in the following points:
First, we dealt with the social media influence environment to study the general concept of our aims.
Secondly, our principal contribution was influencer identification and important nodes, followed by classifying important works dealing with them and reviewing them.
Finally, in a third step, new research directions concerning our survey review are discussed.
1.4 Paper Organization
The rest of this work is organized as follows: In Section 2 , we presented the review methodology adopted in this work by providing the information sources and the research questions. In section 3 , the social media influence background is presented and discussed. Section 4 is devoted to detailing an overview of social media influence. Then, section 5 contained a literature synthesis on the social media influence that we have treated in this work. Section 6 presented the discussions and research challenges that are synthesized at the end of this research work. We concluded the study by proposing several research challenges in Section 7 .
2 Review methodology, sources of information and research questions
2.1 review methodology.
We have assumed a systematic methodology to elaborate on this current work on social media influence. The following is a description of the review approach used, inspired by Kitchenham and Charters ( 2007 ); Helali and Omri ( 2021 ); Souiden et al. ( 2022 ). The review procedure is a series of processes that make up the review protocol. We begin by providing the various information sources and then the search and selection criteria for the main bibliographical references that we used to undertake this study. Next, we present the questions section that we have to provide answers to throughout this literary work.
According to our objective, we do not aim to create a proper list dealing with social media influence but to introduce some important work. We focused on works from reputable publications. The initial research was based on keywords from recent works. After that, we filtered our search results by choosing according to a few norms, such as the publication date and/or writer competence. If the same, we find a similar approach or idea. Then, based on a full-text screening, title, and abstract, we obtain our primary studies list. Finally, to ensure the quality of the assessment form, we choose several articles arbitrarily based on the previous stage’s selected results.
2.2 Sources of information considered
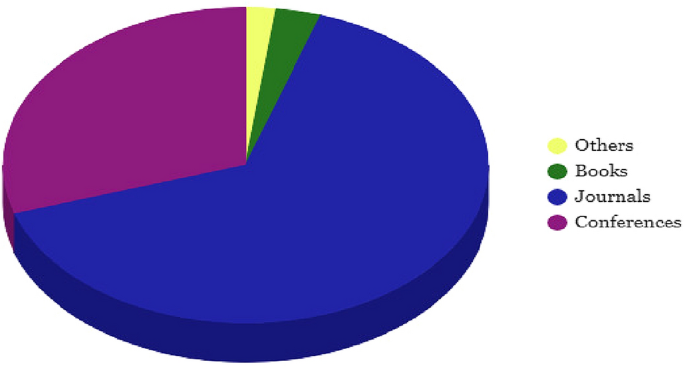
Articles from different types of sources
Concerning the bibliography used, we proceed to search various information sources to lead to the realization of this study. To choose sources related to our focus problem, we selected research articles from journals, conference proceedings, books, and magazines. Thus, we considered the following databases in our research: IEEE Xplore Springer Footnote 1 , ScienceDirect Footnote 2 , Scopus Footnote 3 , ACM Digital Library Footnote 4 ,Taylor Footnote 5 , Francis Footnote 6 , Google Scholar Footnote 7 .
We also screened the majority of related high-profile conferences, such as SIGKDD, ICDT, SIGMOD, ICML, WWW, VLDB, ICDE, and EDBT, to find out about recent work. Figure 1 shows that papers reviewed percentages from different types of resources.
2.3 Research questions
In our research, we are going to answer some proposed questions that are identified in this current section. Therefore, this part focuses on question determination, as below. Then, we will answer those questions through the research review.
RQ1 : Who can influence social media users?
RQ2 : Which is the way to detect influencers?
RQ3 : How do they influence social media users?
3 Social Media Influence : background
3.1 social networks representation.
Social media networks can be represented as a set of profile sites Aggarwal and Subbian ( 2014 ) or virtual communities. Their structure, by default, is considered static, with a static topology and information. After that, it can be transformed into dynamic networks at a specific time t if new nodes and edges are added. Networks have a specific topology Peng et al. ( 2018 ): internet sites where users connect and communicate with others; and mobile social networks. According to the contribution Bian et al. ( 2019 ), the authors show that we can model a social network by a graph G = {V, E} where V = { \(v_1\) , \(v_2\) , . . . , \(v_i\) } represents a set of nodes and E = { \(e_1\) , \(e_2\) ,..., \(e_j\) } represents a set of edges. In the network graph, actors are denoted by V , and social interactions and relations between them are denoted by E . Edge measures relay the influence built on the concepts of a pair of nodes. Each node \(v_i\) can be active or inactive, and the diffusion of influence can propagate from the active node to its neighbor, and the links correspond to social relations.
3.2 Influence on Social Media
Social influence is a concept that includes a wide range of phenomena, such as:
Socialization is the process through which individuals acquire the knowledge, skills, attitudes, values, norms, and appropriate actions of their community Shahr et al. ( 2019 ), which powerfully directs behavior in private settings as well Cialdini and Goldstein ( 2004 ) .
Obedience and authority Individuals are frequently rewarded for behaving under the opinions, advice, and directives of authority figures Cialdini and Goldstein ( 2004 ).
Compliance professionals are forever attempting to establish that they are working for the same goals as they are, in essence, their teammates Cialdini ( 2001 ).
Conformity refers to the act of changing one’s behavior to match the responses of others Cialdini and Goldstein ( 2004 ).
Persuasion, the ability to use its effect, is frequently a crucial element of success Cialdini and NJ. ( 2002 ).
Social networking users tend to act like their “friends” or “neighbors” Singlaand and Richardson ( 2008 ); Sun and Tang ( 2011 ). This phenomenon can be summarized in the following points:
Social Influence: Social media users are more likely to imitate their friends’ actions. Thus, social influence causes people to endorse the behaviors of others.
Selection: People who are characterized as close to them are more likely to bond with each other.
Variables that may be confusing: Other unidentified variables exist, and people may act similarly to each other.
Social influence Cercel and Trausan-Matu ( 2014 ) can be defined as the influence of a person or a group of people on each other. This influence is manifested through the imposition of a set of attitudes and behaviors. Considering two users, \(U_1\) and \(U_2\) , from the social network, \(U_1\) has a direct influence on \(U_2\) or indirectly influences the point of view of \(U_2\) , and transmits influence to \(U_2\) . According to Peng et al. ( 2018 ), social influence is defined as a level of uncertainty or a binary relationship between one (the influencer) and the other (the influenced) to do the same action or choice. The level of social influence can be quantified by a continuous real number or by a degree of uncertainty (e.g., weaker, weak, strong, stronger, etc.) and can have different values Peng et al. ( 2017 ). The fact that \(U_1\) influences \(U_2\) , does not imply that \(U_2\) necessarily affects \(U_1\) .
3.3 Two-step and multi-step communication flow theory
Since relationships are organized around a network of peers, with no centralized entities of control in social media, users are thrown into a global stream of communication Elanor ( 2013 ). Social media networks have reshaped communication at different levels, such as mass communication’s two-step flow of communication theory Oren ( 2019 ) and multi-step communication. The two-step flow communication theory can be used to describe how influence works on social media. According to Ognyanova ( 2017 ) in the two-step model, the theory’s concept of personalized content through opinion leaders Oren ( 2019 ) and direct media effects are hampered by social interactions and audience selectivity in exposure, perception, and retention. The two-step flow theory was expanded upon by the multi-step paradigm Ognyanova ( 2017 ). The multi-step flow paradigm describes the way media and interpersonal influence shape public opinion. Understanding whether someone’s opinion was influenced by their social links or if they simply chose social ties that shared that opinion, to begin with, is important in the context of the multi-step flow of communication Ognyanova ( 2017 ).
3.4 Influencers identification
Nowadays, social media homepages are limited to showing their users priority on the activities of their friends who are used to communicating with or reacting to their statuses, pics, media, or stories. This task can be studied by utilizing the rich text and user interactions on social media Zheng et al. ( 2020 ). Some research deals with the person who influences others, each defines a type and researches its impact on others. Nowadays, social media homepages are limited to showing their users the activities of their friends, who are used to communicating with or reacting to their statuses, pics, media, or stories. Some research deals with the person who influences others, each defines one influencer type and researches its impact on others. In the book, Shah et al. ( 2018 ) and according to its authors, influential people play an important role in information sharing. Being the most popular or the first to affirm a new concept are two examples of being influential. They also provide a strategy for identifying network infrastructure pioneers based on a specific topic of interest. People who adopt and introduce new ideas before they are known, influencing others, are known as trendsetters. They are not always famous or popular. In the same way, not all the latest inventors are trendsetters since only a few of them can spread their ideas in their social circles. They also suggest a reliable way to describe the spread of creativity by presenting each issue as a collection of trends that can be applied to many different scenarios. Results from algorithm simulations on a large Twitter dataset show an ability to quantify indirect and direct influence, and early adoption is also an important trait for influencing others.
All this is to distinguish between the trendsetters and the others, who, although having a high degree, only accept trends when they have become well known. Other specified innovation hubs score a lower degree of acceptance of a new idea than follower hubs. Followers Innovation Hubs are trendsetters, while hubs are influencers. In the dissemination of information, influential people have a crucial effect. Having more Wang et al. ( 2020 ) followers than others does not mean more interaction. There are several ways to be influential, such as being the most famous or being the first to implement a new idea. The most influential users Erlandsson et al. ( 2016 ); Zareie et al. ( 2019 ); Zhao et al. ( 2019 ); Sun and Ng ( 2012 ) played an important role in spreading the information. Others aim to find influencers Rodríguez-Vidal et al. ( 2019 ); Subbian et al. ( 2014 ); Harrigan et al. ( 2021 ); Tsugawa and Kimura ( 2018 ); Kaple et al. ( 2017 ); Chia et al. ( 2021 ); Harrigan et al. ( 2021 ) in different ways. The authors of the works presented in Cervellini et al. ( 2016 ); Saez-Trumper et al. ( 2012 ) deal with a trendsetter who is not automatically popular or famous but whose thoughts have been broadcast on the network. As a result, they serve the same purpose with a different name Zhou et al. ( 2019 ): influence nodes. Identifying influential users has important implications in e-commerce and media Jain and Sinha ( 2020 ); Sun et al. ( 2016 ); Yang et al. ( 2019 ); Sheikhahmadi et al. ( 2017 ). Another example is influential actors Qasem et al. ( 2015 , 2017 ), who have followers Probst ( 2013 ), are famous on Instagram Jin et al. ( 2019 ), and have popular content Ding et al. ( 2015 ). This phenomenon causes a significant increase in the size of the social network. In addition, news influencers Alp and Ögüdücü ( 2018 ) have been identified as specialists on a specific topic. Table 2 summarizes this work and gives further details.
Trendsetters are people who support and propagate innovative thoughts and have an influence on others before they become famous. Cervellini et al. ( 2016 ) propose a methodology for identifying themes in network infrastructure based on a certain topic of interest. And Saez-Trumper et al. ( 2012 ) used different ranking algorithms to identify themes. Popular content is by and about famous people on social media. A random walk model was presented by Ding Ding et al. ( 2015 ) to quantify the influence of users and the popularity of tweets. Another influencer type is called the prophet, who are knowledgeable bloggers and have a strong capacity to predict the future. Zhang et al. ( 2015 ) propose a method for identifying influential blogs that can predict trending hashtags. It is based on keywords or phrases that describe topics or events that are well-known in the community. Influencers are people who have the power to influence someone or something’s personality, evolution, or attitude. Authors like Rodríguez-Vidal et al. ( 2019 ) used a topic modeling approach to manage the textual signal in tweets after experimenting with numerous signals and machine learning algorithms. Harrigan et al. ( 2021 )are using freely available data from social media APIs, and influential mavens on social media may be discovered. Chia et al. ( 2021 ) integrated the social capital and social exchange theories, as well as the social learning theory, to identify ideal Kaple et al. ( 2017 ) proposed a method to maximize public participation and build smart cities through the use of social networks. To detect influencers, Pudjajana et al. ( 2018 ) employ SNA metrics (DC, CC, BC) and weight on the SNA measurement. A method for identifying network influencers based on their social capital value was proposed by Subbian et al. ( 2014 ). Switch Sunil and Lingam ( 2019 ) most of the currently used algorithms created to address these issues have the greedy algorithm as their foundation. And the two-level approach was proposed (SI) for an epidemic model for maximizing the influence spread and a multithreading approach for implementation. Tsugawa and Kimura ( 2018 ) used degree, betweenness, proximity, PageRank, and the k-core index, and used the common sample methodologies SEC, BFS, DFS, and random sampling to find influencers in different social networks. Switch topic Alp and Ögüdücü ( 2018 ) defined the topical influencers as experts on a given topic. And to identify the theme, an approach that combines related features and network feature information was used. It is widely acknowledged that the distribution of influence varies over topics. The work of Zheng et al. ( 2020 ) is based on the language attention network and influence convolution network to detect on-demand topic-specific influencers. Another work dealing with Topic-Sensitive Influencer Fang et al. ( 2014 ). They used a unified hypergraph to model users, images, and various types of relations. Influencers of airline services Izdihardian and Ruldeviyani ( 2021 ) are also detected on Twitter and the results showed their higher influence. Influential nodes in complex networks are often those that increase an interest propagation process’s asymptotic reach. Zhou et al. ( 2019 ) a thorough evaluation of centrality metrics based on their capacity to detect early and late influencer nodes. Lead or follow the influence of mobilizers and propagators in networks and society funded by Probst ( 2013 ) using a systematic literature review to find relevant publications. Influential users are authoritative actors and are a term used to describe influential users. The work of Jain and Sinha ( 2020 ) used WCI (Weighted Correlated Influence), a new concept that integrates the relative influence of 10 different elements into two different feature sets: timeline and trend specific. Yang et al. ( 2019 ) developed the component study of the characteristics of Houston’s top 1% of Twitter users in terms of the following growth. Another important work by Sheikhahmadi et al. ( 2017 ) where weights are allocated to neighbors based on their adoption delays and the spreading influence of nodes is identified. Via a multi-features model, Sun et al. ( 2016 ) and based on a user identification solution with a lot of clouts. The model investigates if a user’s attributes, such as follower count, can be used to infer whether he or she is an influential user, and then ranks influential individuals based on their impact using the Page-Rank concept. Others aim to find the most influential users, or “top k” users, who are considered the most influential. Erlandsson et al. ( 2016 ) found the Degree Centrality and Page Rank Centrality and compared the theme to the outcomes of association rule learning. Zareie et al. ( 2019 ) proposed the idea of a user behavior analysis-based high-influence user discovery algorithm (HIUD). Also, influential actors are individuals whose tweets generate an enormous number of retweets, resulting in a larger social network, proposed in 2015 by Qasem et al. ( 2015 ) find via a method for detecting influential people utilizing a novel influence degree. Then, Qasem et al. ( 2017 ), an extended method for identifying important actors, was formed based on the attractiveness model defined with the T measure.
Other works have defined special influencers that can be an inspiration for many important other works by trying to identify and find those influencer nodes on social media networks. MARWICK ( 2013 ) wrote a book that describes content creators who enjoy fame and influence others by using social media to maintain an active communication network. Other works could use the larger social graph or friends list and broadcast audience recordings to Marwick, who proved its importance. In the work Enke and Borchers ( 2019 ), the authors described three types of influencers: digital opinion leaders, those who are likely to have an impact on others in their immediate area, and content creators, who are people who develop content for social media using their talents to create social media content. They also defined moderators, who initiate interaction procedures and utilize their position to try to lead and continue conversations, and they proved the importance and strategy of influencer communication, in which social media influencers are performed or addressed within tasks that are strategically important to the objectives of business goals. Campbell and Grimm ( 2019 ); Campbell and Farrell ( 2020 ) aimed at users with large communities of followers. Like celebrity influencers and mega-influencers, last but not least, are individuals who have already earned 1 million or more followers on social media, have seen considerable follower growth, and have gained celebrity status through a well-established skill. Recording Campbell and Grimm ( 2019 ) authors’ social media influencers’ posts on their social media channels in exchange for compensation. In their next work, they affirmed that influencers can be simultaneously social media personae, celebrities, experts in particular fields, and peer consumers. They add another definition of influencers and micro-influencers, whose ads are indistinguishable from other consumer-generated content. And also, they defined influencers as individuals who post to their social media accounts in exchange for compensation and as consumers paid to endorse products and promote them to their followers. They affirmed that micro-influencers, Campbell and Grimm ( 2019 ); Campbell and Farrell ( 2020 ); Leonardi and Monti ( 2020 ) or influencers with relatively small online followings, are more effective, often implying enjoyment or simply usage than other influencers and celebrities.
Using social media to identify influential users is a complex process, but one that is extremely important for several Zhang et al. ( 2015 ) applications. Previous research has determined skill levels for detecting knowledgeable users and influence degrees for recognizing influential users. Generally, they mainly depend on the analysis of textual content but also on links to the structure of social media networks. Bloggers use the blogosphere as a platform to post articles, communicate ideas and discuss views. Blogosphere data is dynamic and changes with information updates over time. In the work presented in Zhang et al. ( 2015 ), the authors attempted to find bloggers who anticipate future popular trends, known as prophetic bloggers. In this work, the authors examined the chronological characteristics and content of blog data and proposed a method to analyze the ability of bloggers to predict buzzwords. Bloggers are judged on the types of words used in their blogs. For example, four recent, related, frequent, and/or thorough factors are considered for this goal: content similarity, post-precocity, buzzword coverage, and frequency of entry. In the work of Zhang et al. ( 2015 ), the authors stated that by inferring buzzwords, they detected buzzwords, which allows the assessment of bloggers’ buzzword prediction ability. The contribution proposed by Bian et al. ( 2014a ), was interested in the detection of epidemics. To discover the spread of a virus as soon as possible, they have selected many social network nodes that they consider most relevant. To maximize the benefits of companies’ products and services, consumer profiles are detected and analyzed Hernandez et al. ( 2013 ). By analyzing a dataset from Twitter, the linguistic indicators of partisan conflict in mainstream and social media during political upheaval are explored Karamshuk et al. ( 2016 ). Others deal with pathogenic social media accounts, such as terrorist supporters exploiting large communities Alvari et al. ( 2018 ) and critical nodes, Alorainy et al. ( 2022 ) and a high detection of top-k central nodes Mahyar et al. ( 2018 ). Identifying the users who are most influential in spreading information can aid in the creation of effective tactics Adnan et al. ( 2022 ).
4 Overview of Social Media Influence
4.1 users’ interest.
The degree of influence a social media user has on their friends, as well as their desire to spread additional information, can be detected from interest similarity. Authors have submitted that people users are more affected and influenced by their friends’ opinions than by other influencers Domingos and Richardson ( 2001 ); Staab et al. ( 2005 ). In Xie et al. ( 2014 ), the measure of similarity between two users is based on a random walk distance based on tags and image correlation and was examined in folksonomy data. It outperforms proof in terms of user profile enrichment. Generally, social media users demonstrate Bian et al. ( 2014b ) different interests and hold different expertise levels for numerous kinds of interests, such as sports, music, history, and so on. Authors Sendi et al. ( 2017 ) assumed that users’ interests were discovered from uncertain information. Another way to categorize users’ profiles Mabrouk et al. ( 2020 , 2018 ) as an outcome, the culture of the user’s interest profile will be critical for a full analysis of their influence on their friends, diffusion actions, and future action prediction.
4.2 User’s relationship
The main reason that social networks influence users’ behavior changes is their relationship. As users’ relationship strengths are not equal, social media users do not have the same degree of friendship and intimacy. Relationship strength can be detected by interaction comments, likes, chats, etc. Previously, social network studies centered on binary friendship relationships. Switch authors Xiang et al. ( 2010 ) can lead to a wide variety of relationship strengths (for example, mixing acquaintances and best friends) to estimate relationship strength. They create an unsupervised model based on user similarities and interaction activity, weak and strong relationships. Their model represents the full spectrum and infers a continuous-valued relationship strength for links. For the inference, they used a coordinate ascent optimization approach to create their link-based latent variable model. They have proved and evaluated it on Facebook and LinkedIn. The authors Chader et al. ( 2017 ) did not accept the binary relation. They had related weights that recorded the strength of people’s relationships; they tested the effectiveness of the CoBSP process. The motive comes from the belief that those with the closest relationships with users can give more information about them. In the work of Zarrinkalam et al. ( 2017 , 2019 ), they detect user interests by focusing on social relationships, user-generated content, and temporal factors.
4.3 Interests change
After taking into account the users’ utterances, gender Ouni et al. ( 2022b ), their activeness, and vulnerability to their friends’ influence, the work of Budak et al. ( 2014 ) proved that interests vary during life. They established an inference algorithm that strikes a balance between the latest estimates of interests and the old estimate of interests, and using Twitter data, they funded a precision of 0.9 for the top-5 interests. Users have a variety of interests, such as music, history, sports, and so on Bian et al. ( 2014a ). The degree of influence he has on his friends is determined by his interest in distributing new information and must be varied for different categories. The interest profile learning is decisive for a complete analysis of a user’s impact on his contacts, spread actions, and future action prediction. Another idea Wang et al. ( 2018 ) is to use the UNITE systemic framework with interesting extraction, which considers both text and information. Their approach to experimental results on Sina Weibo. An important work Sendi et al. ( 2019 ) aims to derive and monitor on Twitter the temporal interest topics and also handle the user’s interest, social various dimensions, and dynamical characteristics. Moreover, over time on social media, through user activities’ frequent tags, users, and organizations from their social media posts Shah et al. ( 2018 ), the evolution of one’s attitude through time is observed. The suggested approach also incorporates the use of the semantic web, particularly the DBpedia ontology, which analyzes the content of tweets in the user’s Twitter feed.
4.4 Infer interests
To deduce users’ interests, authors have taken into account their communication, their activity state, and their sensitivity according to the influence of their friends. A probabilistic model of social data has been proposed by Budak et al. ( 2014 ). This model is unsupervised and well-scalable with many users and interests. The authors of Han et al. ( 2015 ), were interested in determining the similarities of interest given to two users, without having any idea about their interests. They showed that similarities between people depend on similar demographic information and having more common friends. Attribute inference can be performed by mixing social graphs and Jia et al. ( 2017 ) behaviors, by first collecting public data and then using machine learning techniques to estimate the private attributes of certain target users. They analyzed user behavior to determine the probability that all users have a given property. Many applications, such as targeted advertising and news suggestions, rely on user locations, which can help solve the problem of profiling users’ Ouni et al. ( 2022c ) home locations Li et al. ( 2012 ). The authors propose a unified discriminatory influence model UDI and overcome the challenge of rare signals in a unified probabilistic framework, combining user-centric and social media data. They produce local and global locations with their prediction methods. Important work has also been proposed in Utz ( 2010 ), their authors found that the target’s acknowledged popularity, community orientation, target’s self-generated data, the affectivity of the target’s contacts, and the volume of friends all have an impact on social attractiveness. So users can create accounts with photos and detailed descriptions to use as a starting point for building an impression. In addition to self-provided data, social media offers two other types of data: the target’s friend data and system data, such as the number of friends. Another interesting work has been proposed in Xie et al. ( 2014 ) where the authors aimed to improve various user tags for a simple image by establishing a collaborative description and making them more meaningful and useful to users.
4.5 Emerging and trending topic
The work proposed in Bao et al. ( 2013 ) was occupied by trending topics. It could be related to important news or subjects being discussed by a large number of microbloggers. The authors supposed that publishing posts on a popular topic proved the user’s interest. The latter are replicated in posts published on the same trending topics. In the work presented in Dang et al. ( 2016 ), the authors aim at emerging topics and are concerned with the substance of important emerging events such as regulatory enforcement, election campaigns, natural disasters, and traffic accidents. Dynamic Bayesian networks are used to uncover developing topics within their methodology. For this, they started the topic diffusion characteristics in the early phase, as well as non-emerging topics with several topology features, and they compared emerging and then, in a given time interval, generated an emerging keyword candidate term list based on term frequency. Next, create a DBN-based model for each candidate to calculate the probability of the candidate emerging as a keyword based on the joint conditional probabilities of the specified features.
5 Social Media influence and literature synthesis
In this section, we proceed to an illustration and a classification of the main relevant works in the literature that are related to the problem treated in this work. The Table 3 presents a summary of the works covered and discussed in this survey. We can see that the majority of previous studies have dealt with the goals and interests of users. Many other studies have addressed the influencers, reasons for interest, and change of all new users.
This Table 3 also presents the approaches used, the experimental results, as well as the years of publication, the authors, and the methodologies adopted. The different approaches to this survey are listed with their concepts. The table also shows the average scores and proof.
For example, in 2013, Bao et al. ( 2013 ) propose a PMF-based method to predict user interests by combining temporal information and social network structure. In this work, the authors confirmed the accuracy of user interest predictions. Then, in 2014, Bian et al. ( 2014a ) focused on the prediction of trending messages and diffusion participants. Three types of influence have been determined: epidemic influence, interest influence, and social influence, which has proven its superiority. In 2015, Han et al. ( 2015 ) were interested in inferring similarities in interests using user demographic information, friendships, and interests. The proposed model was tested on a Facebook dataset. The authors were able to conclude that people with similar demographic information (e.g., age, location) or more mutual friends tend to have more similar tastes. Another interesting work is that of Xiang et al. ( 2010 ). This work consists of modeling the strength or weight of the relationship using a latent variable model on Facebook and LinkedIn. The simulation results of this model showed better autocorrelation and classification performance compared to the results obtained using different raw data elements. The contribution proposed by Zarrinkalam et al. ( 2017 , 2019 )leveraged influencers’ interests in active social media users. By using a graphical description model with three types of information, this model showed its performance. Important work was also proposed by Shah et al. ( 2018 ), where the authors focused on modeling user interests through the social media network graph and the semantic web. This model has also proven its effectiveness. In the same year, i.e., 2018, Wang et al. ( 2018 ) used both content and network structure to extract interest from microblogging and significantly outperform basic methods. Another unsupervised approach that allows inferring user interests from microblogs has been proposed by Budak et al. ( 2014 ). The most direct measure of their inference technique is quality. For the top five interests, this model yielded an accuracy of 0,9. Zhang et al. ( 2015 ) targeted the chronological and content aspects underlying blog data, and a method of analyzing the power of bloggers to predict hashtags has been implemented and tested. This technique has also shown its performance. In 2017, Chader et al. ( 2017 ) show that targeted friendships are not created equal. A methodology for detecting emerging trends has been proposed by Dang Dang et al. ( 2016 ). Their DBN model is based on DBN and uses an early detection strategy based on a dynamic Bayesian network for emerging topics in microblogging networks. They choose features among the topological attributes of the subject distribution and use dynamic changes in the conditional probabilities computed by DBNs to recognize new trends. Jia et al. ( 2017 ) proposed an AttriInfer model to infer user attributes in online social networks using Markov random fields. They incorporated behaviors and social graphics, benefited from both positive people and people with negative training experience, and scaled across large online communities. They proved that the optimized version of AttriInfer is much more flexible than the fundamental version. Li et al. ( 2012 ) proposed their model called “Home Locations Using a Universal and Discriminative Influence Model,” which proved its effectiveness. Saez-Trumper et al. ( 2012 ) tried to find trendsetters in news networks by proposing a robust method. They showed their ability to locate a large portion of trendsetters, regardless of the top 10% of trend adopters, in authentic situations. In their contribution, when nodes with high degree trends are late, the temporal corrosion function diminishes their impact on outcome ranking and helps to underlie variations in the behavior of TS and PR outcomes over time. Rather than using the tags or just the photos, they used both user-generated tags and image correlation. The Multifaceted Folksonomy Graph (MFG) was proposed by Xie et al. ( 2014 ). The Multifaceted Folksonomy Graph (MFG) was proposed. Recently, Wang et al. ( 2020 ), examined the impact of influential leaders on the spread of famous games: Sina Weibo’s Travel Frog and assessed the spread trends, as well as the growth of KOL group networks and keywords UGC. They show that the information propagation event continues via periods of robust and slow forward growth, reaching its peak. In the same year, Mabrouk et al. ( 2020 ) proposed two approaches: the first is a hybridization of ontology and linear SVM, while the second is a hybridization of ontology and FSVM, where they proved that the SVM fuzzy semantic classifier works exceptionally well Mabrouk et al. ( 2018 ). A unique weighted correlated influence metric for Covid-19 is used to identify influential users on Twitter and was also proposed Jain and Sinha ( 2020 ) In this work, the authors verified that the individual with the most followers or the greatest number of tweets is not always the most impactful. Trend-specific influence metrics are insufficient to identify influential users. Harrigan et al. ( 2021 ) attempted to identify influencers on social networks using data widely accessible from the APIs of these networks, and influential mavens have been identified. They found that big social data can be used by decision-makers to identify influential customers. Also, Chia et al. ( 2021 ) proposed searching for the best influencer by combining theories of social capital and socialization with social theories of learning. A complete framework for identifying ideal SMIs has been developed. They proved that the effects of trust and social identification on PMIs’ desire to share unboxing journals in the cognitive dimension of the social capital theory were found to be insignificant. The cognitive factor did not influence the propensity of PMIs to post unboxing notices in the community, unlike the structural and relational dimensions. Finding the most influential entities within the network has proved to be NP-hard. The proposed approach More and Lingam ( 2019 ) provides a balance between influence spread and execution time. Others Yang et al. ( 2014 ) deal with uncovering social network sybils in the wild and terrorist community evolution detection Chaabani and Akaichi ( 2022 ) proposing an artificial bee colony optimization, and then applying the BCTTC to track terrorist evolution. In the work of , Hodas et al. ( 2016 ), an experiment exploring social media usage during disaster scenarios, combining electroencephalograms, personality surveys, and prompts, is proposed. To detect spammer Aswani et al. ( 2018 ) using bio-inspired computing and compromised accounts, the authors Bohacik et al. ( 2017 ); Ouni et al. ( 2022a ) used an anomaly model trained on the previous login data of users. Another important work Arora et al. ( 2019 ) proposes a mechanism for measuring the influencer index across popular social media platforms to identify buzz in social media Aswani et al. ( 2017 ). This is also detected via a hybrid artificial bee colony approach integrated with k-nearest neighbors to identify and segregate buzz and a proposed hybrid bio-inspired approach.
6 Discussion and research challenges
One could wonder if we have adequately addressed the majority of the important issues underlying the identification of influencers, considering the volume of works that have been published in this area, such that the solutions suggested are sufficient for the majority of social network analysis tasks. Yet, in our opinion, a large variety of critical research issues should be solved until we can regard influencer identification as a problem-solving technique. We conclude that other significant fields have not yet been fully explored or studied, such as social network graphs and topology, and the relationship between influencers’ identification and community detection, their networked audience switch MARWICK ( 2013 ) instead of studied fields, of which we have shown some relevant examples. Thus, we ponder unless we have successfully handled almost all of the critical problems related to this subject in a way that the solutions provided seem suitably sophisticated for most social network analysis tasks.
Using social media, users first put their basic information, which can be false, into the social media guide to find their interesting topics. After they add friends and some aims or topics, social media algorithms expand their interests. Social media stars propose helpful suggestions for users to enrich their lives: friends from the same region; old school and faculty; and phone contact. It also suggests hobbies related to his work domain or studies. Then, after setting some preferences, social media stars suggest similar pages and friend types and eliminate ignored suggestions. Its suggestions are based on an intelligent system that analysts change needs, trembling all the time to suggest the most helpful. It certainly encourages users to use it more. The most important thing that users can do here is to search for new aims, new friends, or a new place and all the suggested updates. The challenge was that users could have a period or ephemeral aims. We think time has a big impact on social media users and the importance of a dynamic social media structure for researchers. There are many works in literature dealing with this idea, but no one has put into consideration the social media algorithms and strategies that determine social media influence.
The majority of works in the literature have focused on the identification of the most influential people and significant nodes, called top-k. It represents an interesting research area. Without considering Users can be influenced directly by their friends or groups of friends. They may influence them at the same time. Users share influence. All types of influencers have an important effect and can be the most influential because of their direct contact.
Influence maximization is the trendiest topic that is being exploited and hopes to maximize users’ influence like marketing publicity. But we do not have a search engine that deals with protecting users from negative and also positive users, who may likewise pose a risk by fostering wrath, resentment, hostility, hatred, and indignation, to minimize influence.
User interests that change throughout time and trendy topics have a direct relationship with influence detection by simply comparing users’ aims or interest topics. User interests change according to place or locality and season, influencing them, so we can predict influencers’ node switches. For example, they can also change locales.
Different works deal with the relationship strength and still cannot know the real life and direct relationship. Users may not communicate and discuss clear ideas on social media and may just exchange media or some symbols. Other contributions where the authors have aimed to exploit the users’ locality information without considering that some users have double nationality or can aim with other country topics. The great challenge is, therefore, to find a solution to all these situations. The work of Sinha and Swearingen ( 2001 ) showed that suggestions from subject friends are better than recommender systems. The other challenge now is to study users’ influencers’ friends and predict their new aims.
There are many types of influencers defined as: content creators MARWICK ( 2013 ) digital opinion leaders, moderators Enke and Borchers ( 2019 ) celebrity influencers, mega-influencers, and other works dealing with micro-influencers Campbell and Grimm ( 2019 ); Campbell and Farrell ( 2020 ); Leonardi and Monti ( 2020 ) that can be identified and found. So research is anticipated to rise.
7 Conclusion and prospects
7.1 summary.
Social media has emerged as a popular platform for members to discuss anything, give opinions, and express feelings and important moments about ideas and facts from everyday life Bao et al. ( 2013 ). In this article, we have provided an overview of the current state and future directions of influencer identification on social media. We have reviewed and classified the main existing contributions in the literature into the identification influencers category. At first, we tried to talk more about this domain and alleged it in some works. Then, we cited some other classifications that deal with the same subject, and at least we have applied our classification proposition in some works according to our knowledge. We have proposed some new ideas that can change the orientation of searches on social media users’ interest topics, and why not aim to influence new works?
Our study has some limitations. Most importantly, we did not aim at the structure and topology of social media. We have also noted that we identify significant nodes that influence other nodes in the influenced community and also detect them.
We also know that social media is virtual, but behind this virtual exists a real person whose acts, interactions, and shows are real. They are influenced by internal factors like friends and family, college classmates, and next-door neighbors, and external factors like life coaches, travel bloggers, influence, fashionistas, fitness models, and makeup artists. So we have detailed some important influencers’ identification.
7.2 Prospects
In this state, to conclude our survey, we aimed to synthesize the main research works proposed in the literature, solve the problem of identifying influencers, and present the various algorithms, methods, methodologies, and proposed frameworks. It remains a topical problem, given the importance of the social media community in recent years, and it will continue in the future. Three new avenues may be outlined for this work’s prospects. The first step is to undertake more complete comparison research to provide more information to scholars and particularly on how to find social media influencers. In the second part of the study, we will run more experiments on a multitude of other standard data sets to confirm the principal approaches provided in the literature’s performance and robustness. The third part consists of proposing a new approach to finding new influencers on social networks by using other techniques and criteria based on the new networks’ users’ characteristics.
https://link.springer.com
http://www.sciencedirect.com
https://www.scopus.com
https://www.acm.org/digital-library
https://www.taylorandfrancis.com
http://ieeexplore.ieee.org )
https://scholar.google.co.in
Adedoyin-Olowe M, Gaber MM, Stahl FT (2014) A survey of data mining techniques for social media analysis. Data Mining and Digital Humanities
Adnan TMT, Islam MS, Papon TI, Nath S, Adnan MA (2022) Uacd: a local approach for identifying the most influential spreaders in twitter in a distributed environment. Soc Netw Anal Min 12:37
Google Scholar
Aggarwal C, Subbian K (2014) Evolutionary network analysis: a survey. ACM Comput Survey 47:1–36
MATH Google Scholar
Alorainy W, Burnap P, Liu H, Williams M, Giommoni L (2022) Disrupting networks of hate: characterising hateful networks and removing critical nodes. Soc Netw Anal Min 12:27
Alp ZZ, Ögüdücü SG (2018) Identifying topical influencers on twitter based on user behavior and network topology. Knowl-Based Syst 141:211–221
Alvari H, Shaabani E, Shakarian P (2018) Early identification of pathogenic social media accounts. in IEEE Xplore
Alzanin SM, Azmi AM (2018) Detecting rumors in social media: a survey. Procedia Comput Sci 142:294–300
Andreassen CS, Pallesen S, Griffiths MD (2016) The relationship between addictive use of social media, narcissism, and self-esteem: findings from a large national survey. Addictive Behav. 64:287–293
Arora A, Bansal S, Kandpal C, Aswani R, Dwivedi YK (2019) Measuring social media influencer index- insights from facebook, twitter and instagram. J Retail Consum Services 49:86–101
Aswani R, Ghrera SP, Kar AK, Chandra S (2017) Identifying buzz in social media: a hybrid approach using artificial bee colony and k-nearest neighbors for outlier detection. Soc Netw Anal Min. 7(1)
Aswani R, Kar AK, Ilavarasan PV (2018) Detection of spammers in twitter marketing: a hybrid approach using social media analytics and bio inspired computing. Inform Syst Frontiers 20(3):515–530
Azaouzi M, Mnasri W, Romdhane LB (2021) New trends in influence maximization models. Comput Sci Rev 40:100393
Azaouzi M, Rhoum D, Romdhane LB (2019) Community detection in large-scale social networks: state-of-the-art and future directions. Soc Netw Anal Min 9:23
Bao H, Li Q, Liao SS, Song S, Gao H (2013) A new temporal and social pmf-based method to predict users’ interests in micro-blogging. Decision Support Syst 55:698–709
Bian J, Yang Y, Chua T-S (2014a) Predicting trending messages and diffusion participants in microblogging network. In ‘Proceedings of the 37th international ACM SIGIR conference on Research and development in information retrieval’. p. 537–546
Bian J, Yang Y, Chua T-S (2014b) Predicting trending messages and diffusion participants in microblogging network. In. ‘Proceedings of the 37th international ACM SIGIR conference on Research and development in information retrieval’. p. 537–546
Bian R, Koh YS, Dobbie G, Divoli A (2019) Identifying top-k nodes in social networks: a survey. ACM Comput Surveys 52:1–33
Bohacik J, Fuchs A, Benedikovic M (2017) Detecting compromised accounts on the pokec online social network. in International Conference on Information and Digital Technologies (IDT)
Budak C, Kannan A, Agrawal R, Pedersen J (2014) Inferring user interests from microblogs
Campbell C, Farrell JR (2020) More than meets the eye: The functional components underlying influencer marketing. Business Horizons. 63(4):469–479
Campbell C, Grimm PE (2019) The challenges native advertising poses: Exploring potential federal trade commission responses and identifying research needs. J Public Policy Marketing 38(1):110–123
Cercel D, Trausan-Matu S (2014) Opinion propagation in online social networks: a survey. in: Proceedings of the 4th acm international conference on web intelligence. in ‘Opinion propagation in online social networks: a survey. In: Proceedings of the 4th ACM International Conference on Web Intelligence’. pp. 5–8
Cervellini P, Menezes AG, Mago VK (2016) Finding trendsetters on yelp dataset. in ‘IEEE Symposium Series on Computational Intelligence (SSCI)’. pp. 1–7
Chaabani Y, Akaichi J (2022) Bees colonies for terrorist communities evolution detection. Soc Netw Anal Min. 12
Chader A, Haddadou H, Hidouci W-K (2017) All friends are not equal: weight-aware egocentric network-based user profiling. In ‘IEEE/ACS international conference on computer systems and applications’. pp. 482–488
Chia K-C, Hsu C-C, Lin L-T, Tseng H H (2021) The identification of ideal social media influencers: Integrating the social capital, social exchange, and social learning theories. J Electron Commerce Res. 22
Cialdini RB (2001) Influence: Science and practice (4th ed.). allyn and bacon
Cialdini RB, Goldstein NJ (2004) Social influence: compliance and conformity. Annu Rev Psychol 55(1):591–621
Cialdini RB, NJ G (2002) The science and practice of persuasion. in ‘Cornell Hotel Restaur Adm’ p. 43 40–50
Dang Q, Gao F, Zhou Y (2016) Early detection method for emerging topics based on dynamic bayesian networks in micro-blogging networks. Expert Syst Appl 57:285–295
Ding Z, Wang H, Guo L, Qiao F, Cao J, Shen D (2015) Finding influential users and popular contents on twitter. In ‘International conference on web information systems engineering’. pp. 267–275
Domingos P, Richardson M (2001) Mining the network value of customers. In ‘Proceedings of the seventh ACM SIGKDD international conference on Knowledge discovery and data mining’. p. 57–66
Elanor C (2013) Csr communication strategies for organizational legitimacy in social media. Corporate Communicat 18(2):228–248
Enke N, Borchers NS (2019) Social media influencers in strategic communication: A conceptual framework for strategic social media influencer communication. Inter J Strategic Communicat 13(4):261–277
Erlandsson F, Bródka P, Borg A, Johnson H (2016) Finding influential users in social media using association rule learning. Entropy 18:164
Fang Q, Sang J, Xu C, Rui Y (2014) Topic-sensitive influencer mining in interest-based social media networks via hypergraph learning. IEEE Transct Multi 16:796–812
Han X, Wang L, Crespi N, Park S, Cuevas A (2015) Alike people, alike interests? inferring interest similarity in online social networks. Decis Support Syst 69:92–106
Harrigan P, Daly TM, Coussement K, Lee JA, Soutar GN, Evers U (2021) Identifying influencers on social media. Inter J Inform Manage 56:102246
Helali L, Omri MN (2021) A survey of data center consolidation in cloud computing systems. Comput Sci Rev 39:100366
Hernandez M, Hildrum K, Jain P, Wagle R (2013) Constructing consumer profiles from social media data. In ‘IEEE international conference on big data’. pp. 710–716
Hodas NO, Butner R, Corley C (2016) How a user’s personality influences content engagement in social media. Social Informatics. p. 481–493
Imamori D, Tajima K (2016) Predicting popularity of twitter accounts through the discovery of link-propagating early adopters. in ‘CIKM’
Iraklis M, Williams HT (2020) Good and bad events: combining network-based event detection with sentiment analysis. Soc Netw Anal Min 10(1):1–12
Izdihardian WA, Ruldeviyani Y (2021) Detecting social media influencers of airline services through social network analysis on twitter: A case study of the indonesian airline industry. In ‘3rd East Indonesia Conference on Computer and Information Technology (EIConCIT). IEEE’
Jain S, Sinha A (2020) Identification of influential users on twitter: A novel weighted correlated influence measure for covid-19. Chaos Solitons and Fractals. 139:110037
MathSciNet Google Scholar
Jia J, Wang B, Zhang L, Gong NZ (2017) Attriinfer: Inferring user attributes in online social networks using markov random fields. In ‘Proceedings of the 26th International Conference on World Wide Web’. p. 1561–1569
Jin SV, Muqaddam A, Ryu E (2019) Instafamous and social media influencer marketing. Market Intell Plan Emerald Publishing LimiT 37:567–579
Jin SV, Ryu E (2020) “i’ll buy what she’s wearing’’: The roles of envy toward and parasocial interaction with influencers in instagram celebrity-based brand endorsement and social commerce. J Retail Consumer Service 55:102121
Kaple M, Kulkarni K, Potika K (2017) Viral marketing for smart cities: Influencers in social network communities. In ‘IEEE Third International Conference on Big Data Computing Service and Applications (BigDataService)’. pp. 106 – 111
Karamshuk D, Lokot T, Pryymak O, Sastry N (2016) Identifying partisan slant in news articles and twitter during political crises. in ‘International Conference on Social Informatics (SocInfo)’
Kempe D, Kleinberg J, Tardos E (2003) Maximizing the spread of influence through a social network. In ‘Proceedings of the ninth ACM SIGKDD international conference on Knowledge discovery and data mining’. p. 137–146
Kitchenham B, Charters S (2007) Guidelines for performing systematic literature reviews in software engineering (version 2.3). EBSE Technical Report
Leonardi S, Monti D (2020) Mining micro-influencers from social media posts. In ‘Proceedings of the 35th Annual ACM Symposium on Applied Computing’
Li R, Wang S, Deng H, Wang R, Chang KC-C (2012) Towards social user profiling: Unified and discriminative influence model for inferring home locations. In ‘Proceedings of the 18th ACM SIGKDD international conference on Knowledge discovery and data mining’. p. 1023–1031
Liselot Hudders, De Jans Steffi, DV M (2021) The commercialization of social media stars: A literature review and conceptual framework on the strategic use of social media influencers. International Journal of Advertising. 40(3):327–375
Mabrouk O, Hlaoua L, Omri MN (2018) Fuzzy twin svm based-profile categorization approach. In ‘14th International Conference on Natural Computation, Fuzzy Systems and Knowledge Discovery (ICNC-FSKD)’. pp. 547 – 553
Mabrouk O, Hlaoua L, Omri MN (2020) Exploiting ontology information in fuzzy svm social media profile classification’’, applied intelligence. Appl Intell 51:3757–3774
Mahajan P, Kaur PD (2021) Harnessing user’s social influence and iot data for personalized event recommendation in event-based social networks. Soc NetW Anal Min. 11(1)
Mahyar H, Hasheminezhad R, Ghalebi E, Ali Nazemian RG, Movaghar A, Rabiee HR (2018) Identifying central nodes for information flow in social networks using compressive sensing. Social Network Analysis and Mining
MARWICK AE (2013) Status update: Celebrity, publicity, and branding in the social media age. yale university press
More JS, Lingam C (2019) A gradient-based methodology for optimizing time for influence diffusion in social networks. Soc Netw Anal Min. 9(1)
Ognyanova K (2017) Multistep flow of communication: Network effects. in ‘New York NY: Wiley-Blackwell’. pp. 1–10
Oren S (2019) Algorithmic personalization and the two-step flow of communication. Communication Theory
Ouni S, Fkih F, Omri MN (2022a) Bert and cnn based tobeat approach for unwelcome tweets detection. Social Network Analysis and Mining. 12
Ouni S, Fkih F, Omri MN (2022b) Bots and gender detection on twitter using stylistic features. In ‘14th International Conference on Computational Collective Intelligence’
Ouni S, Fkih F, Omri MN (2022c) Novel semantic and statistic features-based author profiling approach. J Ambient Intell Human Comput
Peng S, Wang G, Xie D (2017) Social influence analysis in social networking big data: opportunities and challenges. IEEE Netw 31:11–17
Peng S, Wang G, Zhou C, Wang C, Yu S, Niu J (2017) An immunization framework for social networks through big data based influence modeling. IEEE Transact Dependable Secure Comput 16:984–995
Peng S, Zhou Y, Caoc L, Yud S, Niue J, Jiaf W (2018) Influence analysis in social networks: a survey. Netw Comput Appl 106:17–32
Piao G, Breslin JG (2018) Inferring user interests in microblogging social networks: a survey. User Modeling User-Adapted Interact 28:277–329
Plantie M, Crampes M (2013) Survey on social community detection. Computer Communications and Networks, Springer-Verlag, London, Social Media Retrieval
Probst F (2013) Who will lead and who will follow: identifying influential users in online social networks. Business Inform Syst Eng 5:179–193
Pudjajana AM, Manongga D, Iriani A, Purnomo HD (2018) Identification of influencers in social media using social network analysis (sna). In ‘International Seminar on Research of Information Technology and Intelligent Systems (ISRITI)’. pp. 400 – 404
Qasem Z, Jansen M, Hecking T, Hoppe H (2015) On the detection of influential actors in social media. In ‘th International Conference on Signal-Image Technology and Internet-Based Systems (SITIS)’. pp. 421–427
Qasem Z, Jansen M, Hecking T, Hoppe H (2017) Influential actors detection using attractiveness model in social media networks. Inter Workshop Complex Netw Appl 693:123–134
Rodríguez-Vidal J, Gonzalo J, Plaza L, Sánchez HA (2019) Automatic detection of influencers in social networks: authority versus domain signals. J Associat Inform sci technol 70:675–684
Saez-Trumper D, Comarela G, Almeida V, Baeza-Yates R, Benevenuto F (2012) Finding trendsetters in information networks. In ‘Proceedings of the 18th ACM SIGKDD international conference on Knowledge discovery and data mining’ p. 1014–1022
Sendi M, Omri MN (2015) Biomedical concept extraction based information retrieval model: application on the mesh. In ‘International Conference on Intelligent Systems Design and Applications (ISDA)’, 14–16 December 2015. pp. 40–45
Sendi M, Omri MN, Abed M (2017) Possibilistic interest discovery from uncertain information in social networks. Intell Data Anal 21:1425–1442
Sendi M, Omri MN, Abed M (2019) Discovery and tracking of temporal topics of interest based on belief-function and aging theories. J Ambient Intell Human Comput 10:3409–3425
Shah B, Verma AP, Tiwari S (2018) User interest modeling from social media network graph, enriched with semantic web. In ‘Proceedings of International Conference on Computational Intelligence and Data Engineering’. pp. 55–64
Shahr HSA, Yazdani S, Afshar L (2019) Professional socialization: an analytical definition. J Medical Ethics History of Med. pp. 12–17
Sheikhahmadi A, Nematbakhsh MA, Zareie A (2017) Identification of influential users by neighbors in online social networks. Physica A: Statist Mech Appl 486:517–534
Singlaand P, Richardson M (2008) Yes, there is a correlation: - from social networks to personal behavior on the web. in ‘In Proceeding of the 17th international conference on World Wide Web (WWW’08)’. p. 655–664
Sinha R, Swearingen K (2001) Comparing recommendations made by online systems and friends. DELOS Personalisation and Recommender Systems in Digital Libraries
Souiden I, Omri MN, Brahmi Z (2022) A survey of outlier detection in high dimensional data streams. Comput Sci Rev 44:100463
Staab S, Domingos P, Mika P, Golbeck J, Ding L, Finin T, Joshi A, Nowak A, Vallacher R (2005) Social networks applied. IEEE Intell Syst 20:80–93
Subbian K, Sharma D, Wen Z, Srivastava J (2014) Finding influencers in networks using social capital. Soc Netw Anal Min 11:219
Sun B, Ng VT (2012) Identifying influential users by their postings in social networks. Inter Workshop Model Soc Med 8329:128–151
Sun J, Tang J (2011) A survey of models and algorithms for social influence analysis. in ‘Social Network Data Analytics’. pp. 177–214
Sun Q, Wang N, Zhou Y, Luo Z (2016) Identification of influential online social network users based on multi-features. Inter J Pattern Recognition Artifi Intell 30:1659015
Sunil MJ, Lingam C (2019) A si model for social media influencer maximization. Appl Comput Inform 15(2):102–108
Tabassum S, Pereira FSF, Fernandes S, Gama J (2018) Social network analysis: an overview. Wiley Interdisciplinary Rev: Data Mining and Knowledge Discovery. 8(5):e1256
Tsugawa S, Kimura K (2018) Identifying influencers from sampled social networks. Physica A. 507:294–303
Utz S (2010) Show me your friends and i will tell you what type of person you are: How one’s profile, number of friends, and type of friends influence impression formation on social network sites. Comput-Mediated Communicat 15:314–335
Wang H, Huang X, Li L (2018) Microblog oriented interest extraction with both content and network structure. Intellt Data Anal 22:515–532
Wang Z, Liu H, Liu W, Wang S (2020) Understanding the power of opinion leaders’ influence on the diffusion process of popular mobile games: Travel frog on sina weibo. Comput Human Behavior. 109:106354
Xiang R, Neville J, Rogati M (2010) Modeling relationship strength in online social networks. In ‘Proceedings of the 19th international conference on World wide web’. p. 981–990
Xie H, Li Q, Mao X, Li X, Cai Y, Rao Y (2014) Community-aware user profile enrichment in folksonomy. Neural Netw 58:111–121
Yang Y, Zhang C, Fan C, Yao W, Huang R, Mostafavi A (2019) Exploring the emergence of influential users on social media during natural disasters. Inter J Disaster Risk Reduct 38:101204
Yang Z, Wilison C, Wang X, Gao T, Zhao BY, Dai Y (2014) Uncovering social network sybils in the wild. ACM Transact on Knowl Discovery from Data 8(1):1–29
Zareie A, Sheikhahmadi A, Jalili M (2019) Identification of influential users in social networks based on users’ interest. Inform Sci 493:217–231
MathSciNet MATH Google Scholar
Zarrinkalam F, Kahani M, Bagheri E (2017) Mining user interests over active topics on social networks. Inform Process Manage 54:339–357
Zarrinkalam F, Kahani M, Bagheri E (2019) User interest prediction over future unobserved topics on social networks. Inform Retrieval J 22:93–128
Zhang J, Tomonaga S, Nakajima S, Inagaki Y, Nakamoto R (2015) Finding prophets in the blogosphere: Bloggers who predicted buzzwords before they become popular. In ‘Proceedings of the 17th International Conference on Information Integration and Web-based Applications and Services’. p. 1–10
Zhao Z, Zhou H, Zhang B, Ji F, Li C (2019) Identifying high influential users in social media by analyzing users’ behaviors. J Intelligent Fuzzy Syst 36:6207–6218
Zheng C, Zhang Q, Young SD, Wang W (2020) On-demand influencer discovery on social media. In ‘Proceedings of the 29th ACM international conference on information and knowledge management’
Zhou F, Lü L, Mariani MS (2019) Fast influencers in complex networks. Communicat Nonlinear Sci Num Simulat 74:69–83
Download references
Author information
Authors and affiliations.
Monastir Faculty of Science, University of Monastir, Monastir, Tunisia
Feriel Gammoudi
MARS Research Laboratory LR17ES05, University of Sousse, Sousse, Tunisia
Feriel Gammoudi, Mondher Sendi & Mohamed Nazih Omri
You can also search for this author in PubMed Google Scholar
Corresponding author
Correspondence to Feriel Gammoudi .
Ethics declarations
Conflict of interest.
The authors have no affiliation with any organization with a direct or indirect financial interest in the subject matter discussed in the manuscript.
Ethical approval
This article does not contain any studies with human participants or animals performed by any of the authors.
Additional information
Publisher's note.
Springer Nature remains neutral with regard to jurisdictional claims in published maps and institutional affiliations.
Rights and permissions
Springer Nature or its licensor holds exclusive rights to this article under a publishing agreement with the author(s) or other rightsholder(s); author self-archiving of the accepted manuscript version of this article is solely governed by the terms of such publishing agreement and applicable law.
Reprints and permissions
About this article
Gammoudi, F., Sendi, M. & Omri, M.N. A Survey on Social Media Influence Environment and Influencers Identification. Soc. Netw. Anal. Min. 12 , 145 (2022). https://doi.org/10.1007/s13278-022-00972-y
Download citation
Received : 10 May 2022
Revised : 10 September 2022
Accepted : 17 September 2022
Published : 03 October 2022
DOI : https://doi.org/10.1007/s13278-022-00972-y
Share this article
Anyone you share the following link with will be able to read this content:
Sorry, a shareable link is not currently available for this article.
Provided by the Springer Nature SharedIt content-sharing initiative
- Social media
- Influencer identification
- Finding influencer
- Users’ interest
- Find a journal
- Publish with us
- Track your research
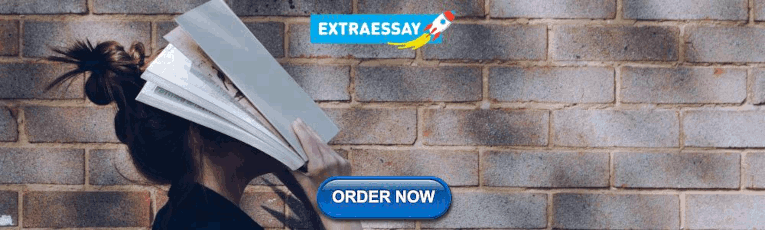
IMAGES
VIDEO
COMMENTS
21 Examples of Thesis Statements about Social Media. Recently, social media is growing rapidly. Ironically, its use in remote areas has remained relatively low. Social media has revolutionized communication but it is evenly killing it by limiting face-to-face communication. Identically, social media has helped make work easier.
Parasocial interaction is a theory derived from communication literature that attempts to explain consumers' relationships with mass media personalities (Horton and Wohl 1956). Feelings of PSI occur when a consumer feels a sense of friendship with someone who they see in the media (Lee and Watkins 2016).
Social media has become an integral part of modern society, revolutionizing the way people communicate, interact, and share information.With the rise of platforms such as Facebook, Twitter, Instagram, and Snapchat, social media has transformed the way we connect with others and consume content. This essay will explore the impact of social media on society, focusing on the thesis statement that ...
SOCIAL MEDIA INFLUENCER MOTIVATIONS 5 . Abstract . Social media influencers are online celebrities who have the power to shape the opinions of audience members due to their relatable qualities. Influencer sponsorships have changed the nature of social media platforms and the strategy by which the content is posted. But besides the
Title of Thesis The Impact of Social Media Influencers on Purchase Decisions Date 28.4.2023 Pages/Number of appendices 32+5 Client Organisation /Partners - Abstract The main objective of this thesis was to investigate how social media influencers impact Savonia University of Applied Sciences students' purchasing decisions.
1 INTRODUCTION. Social media influencers are individuals who have built up a large following on social media and are able to influence their audience's attitudes and behaviors (Hudders et al., 2021).They have become the subject of much scholarly research due to the powerful impact they have on consumer behavior, from influencing purchase decisions to changing societal norms (IZEA Insights, 2022).
The increase in the use of social media in recent years has enabled users to obtain vast amounts of information from different sources. Unprecedented technological developments are currently enabling social media influencers to build powerful interactivity with their followers. These interactions have, in one way or another, influenced young people's behaviors, attitudes, and choices. Thus ...
For social media influencers, to be more popular, it would be a good idea for them to know how they behave will affect the college students' purchase intention. 1.2 Research topic 1.2.1 Social media influencers and college students The reason why social media influencers can be the new marketing strategy is
The Social Media Influencer Reigns Supreme An exploratory study on the influencer concept within social media and the interplay of consumers, influencers, and brands on Instagram Written by: Simisola Ololade Ojuri MSc Strategic Market Creation Master Thesis Supervisor: Karin Tollin Student Number: 113808 Number of Pages: 57
A Social Media Thesis Statement is a statement that expresses the key idea of an argument about the influence of social media platforms on modern society. It could be argued that social media has had both positive and negative impacts on people, from creating new opportunities for communication and connection to contributing to issues like ...
The thesis concludes that macro social media influencers affect consumers' behavior through their content and formed communities, to consume more and repeatedly, desire to reach a certain experience through consumption or behave in a certain ... collaborate with social media influencers (SMIs) (Forbes, 2016). As the internet continues to
Within the past decade, communication online has grown and become a phenomenon of influencer culture on social media. Brands have turned to SMI to disseminate information to influence consumer's product preceptions using the using the social media platforms. One of the most important trends is the use of the influencer marketing concept.
Thesis Statement: Social media allows users to curate their own unique brand of self-presentation. Focusing on social media influencers who "type themselves into being" (Sundén, 2003) online through the self-brand they create and establish, promoted through user-generated data uploads, and gauged on audiences' responses.
Influencers are taking over social media by storm by not only working for and promoting. brands but creating a brand for themselves. According to Influencer MarketingHub, the. influencer market grew from $1.7 billion in 2016 to $9.7 billion in 2020, and in 2021 it soared to. $13.8 billion (Santora, 2022).
The Influence of Social Media 7 . Literature Review . Social media (SM), also known as Social Networking Sites (SNS) have increasingly become a source of discussion among researchers. The growing presence of SM in the lives of young adults and the effects . of . SM on mental health among this
Social media users can be influenced directly by their close relationships, such as their friends, family, and colleagues. They can also be influenced by those who follow them through shared information, goals, news, and opinions. Generally, an influencer is someone who entices an influence to do the same action, make the same decision, or change their behavior. He can also communicate ...
Social media influencers (SMI) are people who advertise products and services through content they publish on their social media channels. Influencers can affect people's opinions with their recommendations and content. These social media influencers are considered to have similar effects to people's opinion as traditional opinion leaders
This research paper aims to examine social media influencers and their impact on. consumers' intention and behaviour. Multiple attributes of social media influencers. and followers were considered, including the influencer's popularity, leverage, fashionability and affinity, and the follower's homophily with the.
Report/thesis title The impact of social media on consumer purchasing behaviour Number of pages and appendix pages 29+4 ... this research the use of social media influencers is a beneficial part of marketing plan since they reach social media users of all ages. Also, brands should keep on concentrating on ...
By. Alyssa C. Carman. May 2023. Objective: Social media has been proposed to play an important role in the. presence of body image issues among young individuals, with many comparing. themselves to unrealistic beauty standards portrayed online. The results of this research.
This Thesis is brought to you for free and open access by BYU ScholarsArchive. It has been accepted for inclusion in All Theses and Dissertations by an ... social media is that the five main components of U&G proposed by Katz et al. (1974) can be applied to social media use. U&G is widely considered a pro-social theory that highlights the
denounce those who oppress them (Miller et al. 2020). Together this suggests that social media can be a positive way to cope with racial discrimination. Project Description In this study, I answer the question, does social media usage influence the effects of racial discrimination on mental health? Specifically, this study examines African ...
As a result, the major findings revealed that the influence of social media distractions on the behavior of the students were students' loss of eagerness, behavioral interruptions and self ...