Have a language expert improve your writing
Run a free plagiarism check in 10 minutes, generate accurate citations for free.
- Knowledge Base
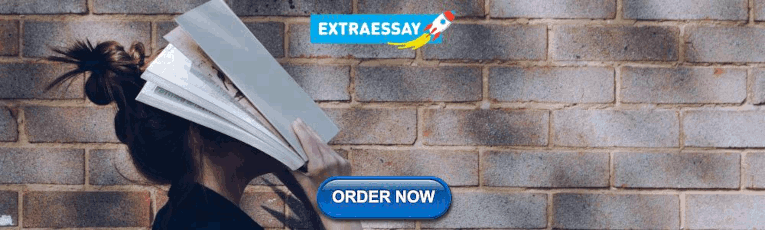
The Beginner's Guide to Statistical Analysis | 5 Steps & Examples
Statistical analysis means investigating trends, patterns, and relationships using quantitative data . It is an important research tool used by scientists, governments, businesses, and other organizations.
To draw valid conclusions, statistical analysis requires careful planning from the very start of the research process . You need to specify your hypotheses and make decisions about your research design, sample size, and sampling procedure.
After collecting data from your sample, you can organize and summarize the data using descriptive statistics . Then, you can use inferential statistics to formally test hypotheses and make estimates about the population. Finally, you can interpret and generalize your findings.
This article is a practical introduction to statistical analysis for students and researchers. We’ll walk you through the steps using two research examples. The first investigates a potential cause-and-effect relationship, while the second investigates a potential correlation between variables.
Table of contents
Step 1: write your hypotheses and plan your research design, step 2: collect data from a sample, step 3: summarize your data with descriptive statistics, step 4: test hypotheses or make estimates with inferential statistics, step 5: interpret your results, other interesting articles.
To collect valid data for statistical analysis, you first need to specify your hypotheses and plan out your research design.
Writing statistical hypotheses
The goal of research is often to investigate a relationship between variables within a population . You start with a prediction, and use statistical analysis to test that prediction.
A statistical hypothesis is a formal way of writing a prediction about a population. Every research prediction is rephrased into null and alternative hypotheses that can be tested using sample data.
While the null hypothesis always predicts no effect or no relationship between variables, the alternative hypothesis states your research prediction of an effect or relationship.
- Null hypothesis: A 5-minute meditation exercise will have no effect on math test scores in teenagers.
- Alternative hypothesis: A 5-minute meditation exercise will improve math test scores in teenagers.
- Null hypothesis: Parental income and GPA have no relationship with each other in college students.
- Alternative hypothesis: Parental income and GPA are positively correlated in college students.
Planning your research design
A research design is your overall strategy for data collection and analysis. It determines the statistical tests you can use to test your hypothesis later on.
First, decide whether your research will use a descriptive, correlational, or experimental design. Experiments directly influence variables, whereas descriptive and correlational studies only measure variables.
- In an experimental design , you can assess a cause-and-effect relationship (e.g., the effect of meditation on test scores) using statistical tests of comparison or regression.
- In a correlational design , you can explore relationships between variables (e.g., parental income and GPA) without any assumption of causality using correlation coefficients and significance tests.
- In a descriptive design , you can study the characteristics of a population or phenomenon (e.g., the prevalence of anxiety in U.S. college students) using statistical tests to draw inferences from sample data.
Your research design also concerns whether you’ll compare participants at the group level or individual level, or both.
- In a between-subjects design , you compare the group-level outcomes of participants who have been exposed to different treatments (e.g., those who performed a meditation exercise vs those who didn’t).
- In a within-subjects design , you compare repeated measures from participants who have participated in all treatments of a study (e.g., scores from before and after performing a meditation exercise).
- In a mixed (factorial) design , one variable is altered between subjects and another is altered within subjects (e.g., pretest and posttest scores from participants who either did or didn’t do a meditation exercise).
- Experimental
- Correlational
First, you’ll take baseline test scores from participants. Then, your participants will undergo a 5-minute meditation exercise. Finally, you’ll record participants’ scores from a second math test.
In this experiment, the independent variable is the 5-minute meditation exercise, and the dependent variable is the math test score from before and after the intervention. Example: Correlational research design In a correlational study, you test whether there is a relationship between parental income and GPA in graduating college students. To collect your data, you will ask participants to fill in a survey and self-report their parents’ incomes and their own GPA.
Measuring variables
When planning a research design, you should operationalize your variables and decide exactly how you will measure them.
For statistical analysis, it’s important to consider the level of measurement of your variables, which tells you what kind of data they contain:
- Categorical data represents groupings. These may be nominal (e.g., gender) or ordinal (e.g. level of language ability).
- Quantitative data represents amounts. These may be on an interval scale (e.g. test score) or a ratio scale (e.g. age).
Many variables can be measured at different levels of precision. For example, age data can be quantitative (8 years old) or categorical (young). If a variable is coded numerically (e.g., level of agreement from 1–5), it doesn’t automatically mean that it’s quantitative instead of categorical.
Identifying the measurement level is important for choosing appropriate statistics and hypothesis tests. For example, you can calculate a mean score with quantitative data, but not with categorical data.
In a research study, along with measures of your variables of interest, you’ll often collect data on relevant participant characteristics.
Here's why students love Scribbr's proofreading services
Discover proofreading & editing
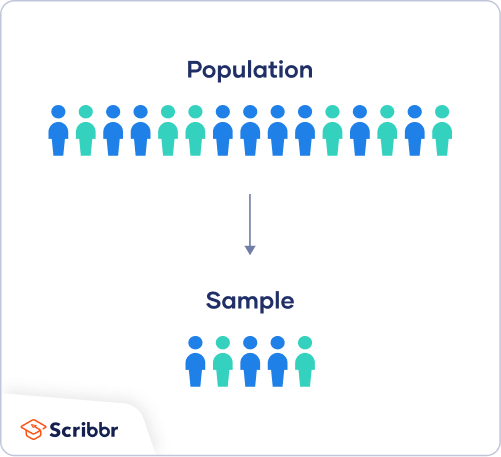
In most cases, it’s too difficult or expensive to collect data from every member of the population you’re interested in studying. Instead, you’ll collect data from a sample.
Statistical analysis allows you to apply your findings beyond your own sample as long as you use appropriate sampling procedures . You should aim for a sample that is representative of the population.
Sampling for statistical analysis
There are two main approaches to selecting a sample.
- Probability sampling: every member of the population has a chance of being selected for the study through random selection.
- Non-probability sampling: some members of the population are more likely than others to be selected for the study because of criteria such as convenience or voluntary self-selection.
In theory, for highly generalizable findings, you should use a probability sampling method. Random selection reduces several types of research bias , like sampling bias , and ensures that data from your sample is actually typical of the population. Parametric tests can be used to make strong statistical inferences when data are collected using probability sampling.
But in practice, it’s rarely possible to gather the ideal sample. While non-probability samples are more likely to at risk for biases like self-selection bias , they are much easier to recruit and collect data from. Non-parametric tests are more appropriate for non-probability samples, but they result in weaker inferences about the population.
If you want to use parametric tests for non-probability samples, you have to make the case that:
- your sample is representative of the population you’re generalizing your findings to.
- your sample lacks systematic bias.
Keep in mind that external validity means that you can only generalize your conclusions to others who share the characteristics of your sample. For instance, results from Western, Educated, Industrialized, Rich and Democratic samples (e.g., college students in the US) aren’t automatically applicable to all non-WEIRD populations.
If you apply parametric tests to data from non-probability samples, be sure to elaborate on the limitations of how far your results can be generalized in your discussion section .
Create an appropriate sampling procedure
Based on the resources available for your research, decide on how you’ll recruit participants.
- Will you have resources to advertise your study widely, including outside of your university setting?
- Will you have the means to recruit a diverse sample that represents a broad population?
- Do you have time to contact and follow up with members of hard-to-reach groups?
Your participants are self-selected by their schools. Although you’re using a non-probability sample, you aim for a diverse and representative sample. Example: Sampling (correlational study) Your main population of interest is male college students in the US. Using social media advertising, you recruit senior-year male college students from a smaller subpopulation: seven universities in the Boston area.
Calculate sufficient sample size
Before recruiting participants, decide on your sample size either by looking at other studies in your field or using statistics. A sample that’s too small may be unrepresentative of the sample, while a sample that’s too large will be more costly than necessary.
There are many sample size calculators online. Different formulas are used depending on whether you have subgroups or how rigorous your study should be (e.g., in clinical research). As a rule of thumb, a minimum of 30 units or more per subgroup is necessary.
To use these calculators, you have to understand and input these key components:
- Significance level (alpha): the risk of rejecting a true null hypothesis that you are willing to take, usually set at 5%.
- Statistical power : the probability of your study detecting an effect of a certain size if there is one, usually 80% or higher.
- Expected effect size : a standardized indication of how large the expected result of your study will be, usually based on other similar studies.
- Population standard deviation: an estimate of the population parameter based on a previous study or a pilot study of your own.
Once you’ve collected all of your data, you can inspect them and calculate descriptive statistics that summarize them.
Inspect your data
There are various ways to inspect your data, including the following:
- Organizing data from each variable in frequency distribution tables .
- Displaying data from a key variable in a bar chart to view the distribution of responses.
- Visualizing the relationship between two variables using a scatter plot .
By visualizing your data in tables and graphs, you can assess whether your data follow a skewed or normal distribution and whether there are any outliers or missing data.
A normal distribution means that your data are symmetrically distributed around a center where most values lie, with the values tapering off at the tail ends.
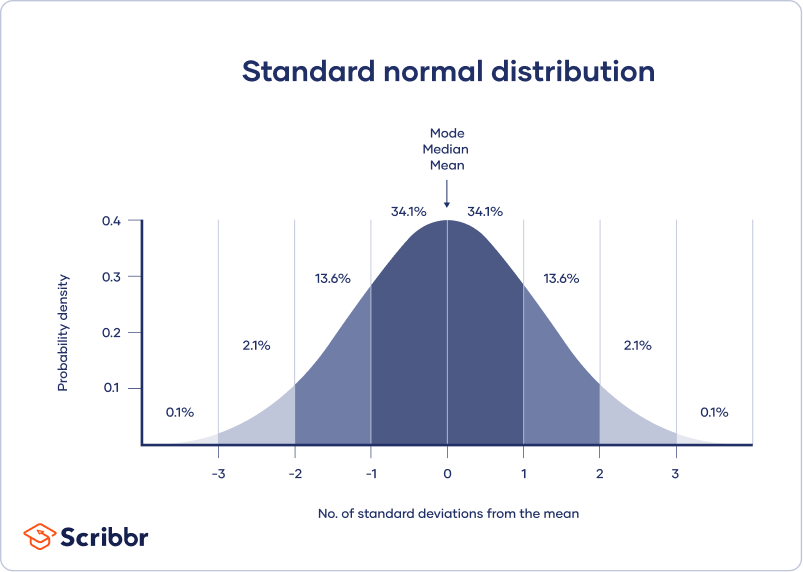
In contrast, a skewed distribution is asymmetric and has more values on one end than the other. The shape of the distribution is important to keep in mind because only some descriptive statistics should be used with skewed distributions.
Extreme outliers can also produce misleading statistics, so you may need a systematic approach to dealing with these values.
Calculate measures of central tendency
Measures of central tendency describe where most of the values in a data set lie. Three main measures of central tendency are often reported:
- Mode : the most popular response or value in the data set.
- Median : the value in the exact middle of the data set when ordered from low to high.
- Mean : the sum of all values divided by the number of values.
However, depending on the shape of the distribution and level of measurement, only one or two of these measures may be appropriate. For example, many demographic characteristics can only be described using the mode or proportions, while a variable like reaction time may not have a mode at all.
Calculate measures of variability
Measures of variability tell you how spread out the values in a data set are. Four main measures of variability are often reported:
- Range : the highest value minus the lowest value of the data set.
- Interquartile range : the range of the middle half of the data set.
- Standard deviation : the average distance between each value in your data set and the mean.
- Variance : the square of the standard deviation.
Once again, the shape of the distribution and level of measurement should guide your choice of variability statistics. The interquartile range is the best measure for skewed distributions, while standard deviation and variance provide the best information for normal distributions.
Using your table, you should check whether the units of the descriptive statistics are comparable for pretest and posttest scores. For example, are the variance levels similar across the groups? Are there any extreme values? If there are, you may need to identify and remove extreme outliers in your data set or transform your data before performing a statistical test.
From this table, we can see that the mean score increased after the meditation exercise, and the variances of the two scores are comparable. Next, we can perform a statistical test to find out if this improvement in test scores is statistically significant in the population. Example: Descriptive statistics (correlational study) After collecting data from 653 students, you tabulate descriptive statistics for annual parental income and GPA.
It’s important to check whether you have a broad range of data points. If you don’t, your data may be skewed towards some groups more than others (e.g., high academic achievers), and only limited inferences can be made about a relationship.
A number that describes a sample is called a statistic , while a number describing a population is called a parameter . Using inferential statistics , you can make conclusions about population parameters based on sample statistics.
Researchers often use two main methods (simultaneously) to make inferences in statistics.
- Estimation: calculating population parameters based on sample statistics.
- Hypothesis testing: a formal process for testing research predictions about the population using samples.
You can make two types of estimates of population parameters from sample statistics:
- A point estimate : a value that represents your best guess of the exact parameter.
- An interval estimate : a range of values that represent your best guess of where the parameter lies.
If your aim is to infer and report population characteristics from sample data, it’s best to use both point and interval estimates in your paper.
You can consider a sample statistic a point estimate for the population parameter when you have a representative sample (e.g., in a wide public opinion poll, the proportion of a sample that supports the current government is taken as the population proportion of government supporters).
There’s always error involved in estimation, so you should also provide a confidence interval as an interval estimate to show the variability around a point estimate.
A confidence interval uses the standard error and the z score from the standard normal distribution to convey where you’d generally expect to find the population parameter most of the time.
Hypothesis testing
Using data from a sample, you can test hypotheses about relationships between variables in the population. Hypothesis testing starts with the assumption that the null hypothesis is true in the population, and you use statistical tests to assess whether the null hypothesis can be rejected or not.
Statistical tests determine where your sample data would lie on an expected distribution of sample data if the null hypothesis were true. These tests give two main outputs:
- A test statistic tells you how much your data differs from the null hypothesis of the test.
- A p value tells you the likelihood of obtaining your results if the null hypothesis is actually true in the population.
Statistical tests come in three main varieties:
- Comparison tests assess group differences in outcomes.
- Regression tests assess cause-and-effect relationships between variables.
- Correlation tests assess relationships between variables without assuming causation.
Your choice of statistical test depends on your research questions, research design, sampling method, and data characteristics.
Parametric tests
Parametric tests make powerful inferences about the population based on sample data. But to use them, some assumptions must be met, and only some types of variables can be used. If your data violate these assumptions, you can perform appropriate data transformations or use alternative non-parametric tests instead.
A regression models the extent to which changes in a predictor variable results in changes in outcome variable(s).
- A simple linear regression includes one predictor variable and one outcome variable.
- A multiple linear regression includes two or more predictor variables and one outcome variable.
Comparison tests usually compare the means of groups. These may be the means of different groups within a sample (e.g., a treatment and control group), the means of one sample group taken at different times (e.g., pretest and posttest scores), or a sample mean and a population mean.
- A t test is for exactly 1 or 2 groups when the sample is small (30 or less).
- A z test is for exactly 1 or 2 groups when the sample is large.
- An ANOVA is for 3 or more groups.
The z and t tests have subtypes based on the number and types of samples and the hypotheses:
- If you have only one sample that you want to compare to a population mean, use a one-sample test .
- If you have paired measurements (within-subjects design), use a dependent (paired) samples test .
- If you have completely separate measurements from two unmatched groups (between-subjects design), use an independent (unpaired) samples test .
- If you expect a difference between groups in a specific direction, use a one-tailed test .
- If you don’t have any expectations for the direction of a difference between groups, use a two-tailed test .
The only parametric correlation test is Pearson’s r . The correlation coefficient ( r ) tells you the strength of a linear relationship between two quantitative variables.
However, to test whether the correlation in the sample is strong enough to be important in the population, you also need to perform a significance test of the correlation coefficient, usually a t test, to obtain a p value. This test uses your sample size to calculate how much the correlation coefficient differs from zero in the population.
You use a dependent-samples, one-tailed t test to assess whether the meditation exercise significantly improved math test scores. The test gives you:
- a t value (test statistic) of 3.00
- a p value of 0.0028
Although Pearson’s r is a test statistic, it doesn’t tell you anything about how significant the correlation is in the population. You also need to test whether this sample correlation coefficient is large enough to demonstrate a correlation in the population.
A t test can also determine how significantly a correlation coefficient differs from zero based on sample size. Since you expect a positive correlation between parental income and GPA, you use a one-sample, one-tailed t test. The t test gives you:
- a t value of 3.08
- a p value of 0.001
Receive feedback on language, structure, and formatting
Professional editors proofread and edit your paper by focusing on:
- Academic style
- Vague sentences
- Style consistency
See an example
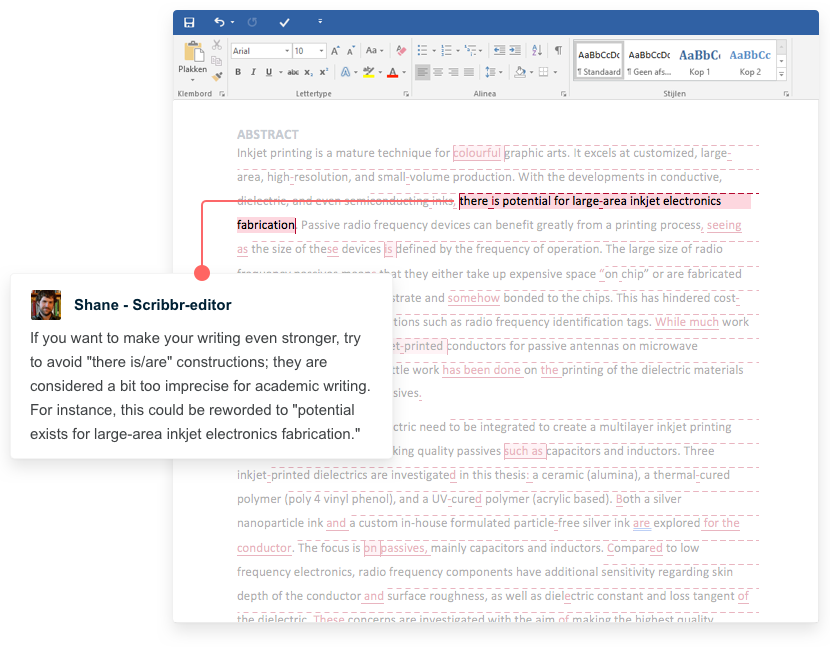
The final step of statistical analysis is interpreting your results.
Statistical significance
In hypothesis testing, statistical significance is the main criterion for forming conclusions. You compare your p value to a set significance level (usually 0.05) to decide whether your results are statistically significant or non-significant.
Statistically significant results are considered unlikely to have arisen solely due to chance. There is only a very low chance of such a result occurring if the null hypothesis is true in the population.
This means that you believe the meditation intervention, rather than random factors, directly caused the increase in test scores. Example: Interpret your results (correlational study) You compare your p value of 0.001 to your significance threshold of 0.05. With a p value under this threshold, you can reject the null hypothesis. This indicates a statistically significant correlation between parental income and GPA in male college students.
Note that correlation doesn’t always mean causation, because there are often many underlying factors contributing to a complex variable like GPA. Even if one variable is related to another, this may be because of a third variable influencing both of them, or indirect links between the two variables.
Effect size
A statistically significant result doesn’t necessarily mean that there are important real life applications or clinical outcomes for a finding.
In contrast, the effect size indicates the practical significance of your results. It’s important to report effect sizes along with your inferential statistics for a complete picture of your results. You should also report interval estimates of effect sizes if you’re writing an APA style paper .
With a Cohen’s d of 0.72, there’s medium to high practical significance to your finding that the meditation exercise improved test scores. Example: Effect size (correlational study) To determine the effect size of the correlation coefficient, you compare your Pearson’s r value to Cohen’s effect size criteria.
Decision errors
Type I and Type II errors are mistakes made in research conclusions. A Type I error means rejecting the null hypothesis when it’s actually true, while a Type II error means failing to reject the null hypothesis when it’s false.
You can aim to minimize the risk of these errors by selecting an optimal significance level and ensuring high power . However, there’s a trade-off between the two errors, so a fine balance is necessary.
Frequentist versus Bayesian statistics
Traditionally, frequentist statistics emphasizes null hypothesis significance testing and always starts with the assumption of a true null hypothesis.
However, Bayesian statistics has grown in popularity as an alternative approach in the last few decades. In this approach, you use previous research to continually update your hypotheses based on your expectations and observations.
Bayes factor compares the relative strength of evidence for the null versus the alternative hypothesis rather than making a conclusion about rejecting the null hypothesis or not.
If you want to know more about statistics , methodology , or research bias , make sure to check out some of our other articles with explanations and examples.
- Student’s t -distribution
- Normal distribution
- Null and Alternative Hypotheses
- Chi square tests
- Confidence interval
Methodology
- Cluster sampling
- Stratified sampling
- Data cleansing
- Reproducibility vs Replicability
- Peer review
- Likert scale
Research bias
- Implicit bias
- Framing effect
- Cognitive bias
- Placebo effect
- Hawthorne effect
- Hostile attribution bias
- Affect heuristic
Is this article helpful?
Other students also liked.
- Descriptive Statistics | Definitions, Types, Examples
- Inferential Statistics | An Easy Introduction & Examples
- Choosing the Right Statistical Test | Types & Examples
More interesting articles
- Akaike Information Criterion | When & How to Use It (Example)
- An Easy Introduction to Statistical Significance (With Examples)
- An Introduction to t Tests | Definitions, Formula and Examples
- ANOVA in R | A Complete Step-by-Step Guide with Examples
- Central Limit Theorem | Formula, Definition & Examples
- Central Tendency | Understanding the Mean, Median & Mode
- Chi-Square (Χ²) Distributions | Definition & Examples
- Chi-Square (Χ²) Table | Examples & Downloadable Table
- Chi-Square (Χ²) Tests | Types, Formula & Examples
- Chi-Square Goodness of Fit Test | Formula, Guide & Examples
- Chi-Square Test of Independence | Formula, Guide & Examples
- Coefficient of Determination (R²) | Calculation & Interpretation
- Correlation Coefficient | Types, Formulas & Examples
- Frequency Distribution | Tables, Types & Examples
- How to Calculate Standard Deviation (Guide) | Calculator & Examples
- How to Calculate Variance | Calculator, Analysis & Examples
- How to Find Degrees of Freedom | Definition & Formula
- How to Find Interquartile Range (IQR) | Calculator & Examples
- How to Find Outliers | 4 Ways with Examples & Explanation
- How to Find the Geometric Mean | Calculator & Formula
- How to Find the Mean | Definition, Examples & Calculator
- How to Find the Median | Definition, Examples & Calculator
- How to Find the Mode | Definition, Examples & Calculator
- How to Find the Range of a Data Set | Calculator & Formula
- Hypothesis Testing | A Step-by-Step Guide with Easy Examples
- Interval Data and How to Analyze It | Definitions & Examples
- Levels of Measurement | Nominal, Ordinal, Interval and Ratio
- Linear Regression in R | A Step-by-Step Guide & Examples
- Missing Data | Types, Explanation, & Imputation
- Multiple Linear Regression | A Quick Guide (Examples)
- Nominal Data | Definition, Examples, Data Collection & Analysis
- Normal Distribution | Examples, Formulas, & Uses
- Null and Alternative Hypotheses | Definitions & Examples
- One-way ANOVA | When and How to Use It (With Examples)
- Ordinal Data | Definition, Examples, Data Collection & Analysis
- Parameter vs Statistic | Definitions, Differences & Examples
- Pearson Correlation Coefficient (r) | Guide & Examples
- Poisson Distributions | Definition, Formula & Examples
- Probability Distribution | Formula, Types, & Examples
- Quartiles & Quantiles | Calculation, Definition & Interpretation
- Ratio Scales | Definition, Examples, & Data Analysis
- Simple Linear Regression | An Easy Introduction & Examples
- Skewness | Definition, Examples & Formula
- Statistical Power and Why It Matters | A Simple Introduction
- Student's t Table (Free Download) | Guide & Examples
- T-distribution: What it is and how to use it
- Test statistics | Definition, Interpretation, and Examples
- The Standard Normal Distribution | Calculator, Examples & Uses
- Two-Way ANOVA | Examples & When To Use It
- Type I & Type II Errors | Differences, Examples, Visualizations
- Understanding Confidence Intervals | Easy Examples & Formulas
- Understanding P values | Definition and Examples
- Variability | Calculating Range, IQR, Variance, Standard Deviation
- What is Effect Size and Why Does It Matter? (Examples)
- What Is Kurtosis? | Definition, Examples & Formula
- What Is Standard Error? | How to Calculate (Guide with Examples)
What is your plagiarism score?
The Data Deep Dive: Statistical Analysis Guide

Table of contents
- 1 What Is Statistical Analysis and Its Role?
- 2 Preparing for Statistical Analysis
- 3 Data Collection and Management
- 4 Performing Descriptive Statistical Analysis
- 5 Performing Inferential Statistics Analysis
- 6 Writing the Statistical Research Paper
- 7 Common Mistakes in Statistical Analysis
- 8 Ethical Considerations
- 9 Concluding the Research Paper
- 10 Examples of Good and Poor Statistical Analysis in Research Paper
- 11 Key Insights: Navigating Statistical Analysis
Statistical analysis is fundamental if you need to reveal patterns or identify trends in datasets, employing numerical data analysis to eliminate bias and extract meaningful vision. Accordingly, it is crucial in research explanation, model evolution, and survey planning.
Statistical analysts make valuable results from the raw data, facilitating informed decision-making and predictive statistical analytics based on historical information.
Do you need to make a statistical analysis for your university studies? In this statistical study article, you will find instructions on how to write statistical analysis, as well as types of statistical analysis, statistical tools, and common mistakes students face.
What Is Statistical Analysis and Its Role?
Statistical analysis is the systematic process of collecting, organizing, and interpreting numbers to reveal patterns and identify trends and relationships. It plays a crucial role in research by providing tools to analyze data objectively, remove bias, and draw conclusions. Moreover, statistical analysis aids in identifying correlations, testing hypotheses, and making predictions, thereby informing decision-making in various fields such as computer science, medicine, economics, and social sciences. Thus, it enables quantitative data and statistical analytics researchers to assess results.
Struggling with how to analyze data in research? Feel free to address our specialists to get skilled and qualified help with research paper data analysis.
Preparing for Statistical Analysis
Preparing for statistical analysis requires some essential steps to ensure the validity and reliability of results.
- Firstly, formulating understandable and measurable questions is critical for valid statistics in a research paper. Questions lead the entire data analysis process and help define the scope of the study. Accordingly, scientists should develop specific, relevant, and capable issues that can be answered through statistical methods.
- Secondly, identifying appropriate data is vital. Picking an accordant data set that aligns with the investigations guarantees the analysis and business intelligence are focused and meaningful. For this purpose, researchers should consider data origin, quality, and responsibility when selecting data for analysis.
By scrupulously formulating problems and selecting appropriate statistical analytics data, researchers can lay a solid foundation for statistical analysis, guiding to strong and prudent results.
Data Collection and Management
Information collection and management are integral components of the statistical analysis process, ensuring the accuracy and reliability of results. Firstly, considering the techniques of data collection is essential. Here, researchers may employ primary approaches, such as examinations, interviews, or experiments, to gather direct information. Secondary methods involve utilizing existing data sources like databases, statistical analysis software, literature reviews, or archival records.
To collect data, specialists need to analyze:
- dependent variable;
- categorical variables;
- outcome variable;
- patterns and trends;
- alternative hypothesis states.
Once data is collected, organizing it is crucial for efficient analysis. As a rule, researchers utilize statistical analysis software tools or spreadsheets to manage data systematically, ensuring clarity and accessibility. Besides, proper organization includes labeling variables, formatting data consistently, and documenting any transformations or cleaning statistical procedures undertaken.
Effective data management also facilitates coherent analysis, empowering scientists to get meaningful insights and draw valid conclusions. By using suitable data collection approaches and organizing data systematically, researchers can unlock the full potential of statistical analysis, advancing knowledge and driving proof-based replies.
Performing Descriptive Statistical Analysis
Performing descriptive statistics is essential in knowing and summarizing data sets for statistics in research. Usually, it involves exploring the crucial characteristics of the data to gain insights into its normal allotting and changeability.
The basics of descriptive statistics encompass measures of central tendency, dispersion, and graphical representations.
- Measures of main bias , such as mean, median, and mode, summarize a dataset’s typical or main value.
- Dispersion repeated measures , including range, variance, and standard deviation, quantify the spread or variability of the data points.
- Graphical representations , such as histograms, box plots, and scatter plots, offer visual insights into the distribution and patterns within the data based on statistical observations.
Explaining descriptive statistical analysis results involves understanding and presenting the findings effectively. Accordingly, researchers should show understandable explanations of the descriptive statistics in the research paper calculated, highlighting key insights and trends within the data. Indeed, visual representations can enhance understanding by illustrating the distribution and relationships in the data. Hence, it’s essential to consider the context of the analysis and the questions when interpreting the results, ensuring that the conclusions drawn are suggestive and relevant.
Overall, performing descriptive statistical data analysis enables researchers to summarize and derive the crucial characteristics after collecting data. It is vital to provide a foundation for further research study and interpretation. By mastering the basics of different types of statistical analysis and correctly explaining the results, experimentals can uncover valuable insights and communicate their findings clearly and precisely.
If you struggle on this step, ask for help from our writers. They can’t write an essay or paper for you but are eager to assist you with each step.
Performing Inferential Statistics Analysis
When students perform statistical analysis, it involves making statistical inference and drawing conclusions about a population based on sample data. For this reason, the inferential statistical tool in research revolves around hypothesis testing, confidence intervals, and significance levels.
On the one side, hypothesis testing allows researchers to assess the validity of assumptions underlying entire population parameters by comparing one sample data to theoretical expectations. On the other side, sample data, null hypothesis, and confidence intervals provide a range of normal and extreme values within which the proper population parameter will likely fall. Lastly, significance levels indicate the probability of obtaining the observed results by chance alone, helping researchers determine the reliability of their findings.
Choosing the proper approach is crucial for conducting meaningful inferential statistics analysis. Accordingly, researchers must select appropriate parametric tests based on the research design, collect data type, null hypothesis, and hypothesis being tested. For example, standard parametric tests and non parametric tests include:
- T-tests: a parametric statistical test used to determine if there is a significant difference between the means of two groups. It is commonly used when the sample size is small, and the population standard deviation is unknown.
- Z test: similar to the t-test, the z-test is a parametric test used to compare means, but it is typically employed when the sample size is large and/or the population standard deviation is known.
- ANOVA: this parametric statistical test compares the means of three or more groups simultaneously. It assesses whether there are any statistically significant differences between the means of the groups.
- Regression: a statistical method used to examine the relationship between one dependent variable (often denoted as Y) and one or more independent variables (often denoted as X) within one case study analysis . Thus, it helps in understanding how the value of the dependent variable changes when one or more independent variables are varied. Here, case study analysis refers to applying regression analysis in specific scenarios or case studies to explore relationships between quantitative variables.
Importantly to note, interpreting results from inferential studies requires a nuanced understanding of statistical concepts and diligent consideration of the context. Here, investigators should assess the strength of evidence supporting their conclusions, considering factors such as effect size, statistical power, and potential biases. Besides, communicating inferential statistics results involves presenting findings and standard deviation to highlight the implications for the research question or troublesome under investigation.
Writing the Statistical Research Paper
Writing a research paper involves integrating and presenting your findings coherently. You need to know the answers to the questions: “What is statistical analysis?” and “How do you do a statistical analysis?”. As a rule, the typical structure includes several essential sections:
- Introduction : This section provides backdrop information on the research theme, states the research questions or null hypothesis, patterns, and trends, and outlines the study’s objectives and statistical attention.
- Methodology : Here, researchers detail the methods and procedures for analyzing and collecting data. This section should be thorough enough for other researchers to replicate the study.
- Results : This section presents the study’s findings, often through descriptive and inferential statistical data analysis. It’s essential to present results objectively and accurately, using appropriate statistical study measures and techniques.
- Discussion : In this segment, investigators interpret statistics and the results, discuss their implications, and compare them to existing literature. It’s an opportunity to critically evaluate the findings and address any limitations or potential biases.
- Conclusion : The conclusion summarizes the study’s key findings, discusses their significance, and suggests avenues for future research.
When you present or write a statistical report in each section, it’s crucial to clearly and concisely explain the methods, results, and research design. Therefore, students usually need to test it in the sample group. In the methodology section, describe the statistical techniques used and justify their appropriateness for the research question. Otherwise, use descriptive statistics to summarize data and inferential statistics to test hypotheses or explore relationships between variables.
Whereas, graphics and tables are potent statistical instruments for presenting data effectively. Choose the most appropriate format for your data, whether it’s a bar graph, scatter plot, or table of descriptive statistics for research.
As a result, writing your research essay must involve such steps:
- Arranging your decisions analytically;
- Integrating statistical analysis throughout;
- Using visuals and tables to enhance clarity and understanding.
Common Mistakes in Statistical Analysis
Common mistakes in statistical analysis can undermine the validity and reliability of research findings. Here are some key pitfalls to avoid:
- Confusing terms like ‘mean’ and ‘median’ or misinterpreting p value and confidence intervals can lead to incorrect conclusions.
- Selecting the wrong test for the research question or ignoring test assumptions can compromise the accuracy of the results.
- Ignoring missing data and outliers or failing to preprocess data properly can introduce bias and skew results.
- Focusing solely on statistical significance without considering practical significance or engaging in p-hacking practices can lead to misleading conclusions.
- Failing to share facts or selectively report results can hinder research reproducibility and transparency.
- Both small and large sample sizes can impact the reliability and generalizability of findings.
- Repeatedly testing hypotheses on the same data set or creating overly complicated models can result in spurious decisions.
- Failing to interpret statistical results within the broader context or generalize findings appropriately can limit the practical relevance of research.
- Misrepresenting graphics or neglecting to label and interval scale graphs correctly can distort the statistical analysis of data.
- Managing redundant analyses or ignoring existing knowledge in the field can hinder the promotion of research.
Avoiding common mistakes in statistical analysis requires diligence and attention to detail. Consequently, researchers should prioritize understanding statistical concepts systematically and using appropriate methods for exploratory data analysis. Thus, it’s essential to double-check calculations, verify assumptions, and seek guidance from statistical analysts if needed.
Furthermore, maintaining transparency and reproducibility in research practices is leading. It includes sharing data, code, and methodology details to facilitate equivalent surveys and replication of findings.
Continuous data learning and staying updated on best practices in statistical analysis are also vital for avoiding pitfalls and improving the quality of research. By addressing these common mistakes and adopting robust practices, researchers can ensure the morality and reliability of their findings, contributing to advancing knowledge in their respective fields.
Ethical Considerations
Ethical considerations in statistical analysis encompass safeguarding data privacy and integrity. That being said, researchers must uphold ethical practices in handling data, ensuring confidentiality, and respecting participants’ rights. Indeed, transparent reporting of results is vital, as is disclosing potential conflicts of passion and holding to moral guidelines data dedicated to relevant institutions and controlling bodies. By prioritizing ethical principles, researchers can maintain trust and integrity in their work, fostering a culture of responsible data analysis in research and decision-making.
Concluding the Research Paper
Concluding a research paper involves summarizing key findings and suggesting future research directions. Here, reiterating the paper’s main points and highlighting the significance of the results is essential. Statistical analysts can also discuss limitations and areas for further investigation, providing context for future studies. By showing insightful outcomes and figuring out avenues for future research, scientists can contribute to the ongoing discourse in their field and inspire further inquiry and exploration.
Examples of Good and Poor Statistical Analysis in Research Paper
Good statistical analysis examples in research:
- A study on the effectiveness of a new drug uses appropriate parametric tests, presents results clearly with confidence intervals, and discusses both statistical and practical significance.
- A survey-based research project employs stratified random sampling, ensuring a representative sample, and utilizes advanced regression analysis to explore complex relationships between variables.
- An experiment investigating the impact of a teaching method on student performance controls for potential confounding variables and conducts power statistical analysis basics to determine sample size, ensuring adequate statistical power.
Examples of poor stat analysis in research:
- A study fails to report key details about information collection and statistical methods, making it impossible to evaluate the validity of the findings.
- A research paper relies solely on p value to conclude without considering effect sizes or practical significance, leading to misleading interpretations.
- An analysis uses an inappropriate statistical test for the research question, resulting in flawed conclusions and misinterpretation of the data.
Here are two good examples.
Example 1: The Effect of Regular Exercise on Anxiety Levels among College Students
Introduction: In recent years, mental health issues among college students have become a growing concern. Anxiety, in particular, is prevalent among this demographic. This study aims to investigate the potential impact of regular exercise on anxiety levels among college students. Understanding this relationship could inform interventions aimed at improving mental well-being in this population.
Methodology: Participants (N = 100) were recruited from a local university and randomly assigned to either an exercise or control group. The exercise group engaged in a supervised 30-minute aerobic exercise session three times a week for eight weeks, while the control group maintained regular activities. Anxiety levels were assessed using the State-Trait Anxiety Inventory (STAI) before and after the intervention period.
Results: The results revealed a significant decrease in anxiety levels among participants in the exercise group compared to the control group (t(98) = -2.45, p < 0.05). Specifically, the mean anxiety score decreased from 45.2 (SD = 7.8) to 38.6 (SD = 6.4) in the exercise group, while it remained relatively stable in the control group (mean = 44.5, SD = 8.2).
Discussion: These findings suggest that regular aerobic exercise may have a beneficial effect on reducing anxiety levels among college students. Engaging in physical activity could serve as a potential non-pharmacological intervention for managing anxiety symptoms in this population. Further research is warranted to explore this relationship’s underlying mechanisms and determine optimal exercise duration and intensity for maximum mental health benefits.
Example 2: The Relationship between Service Quality, Customer Satisfaction, and Loyalty in Retail Settings
Introduction: Maintaining high levels of customer satisfaction and loyalty is essential for the success of retail businesses. This study investigates the relationship between service quality, customer satisfaction, and loyalty in a local retail chain context. Understanding these dynamics can help businesses identify areas for improvement and develop strategies to enhance customer retention.
Methodology: A survey was conducted among the retail chain’s customers (N = 300) to assess their perceptions of service quality, satisfaction with their shopping experience, and intention to repurchase from the store. Service quality was measured using the SERVQUAL scale, while customer satisfaction and loyalty were assessed using Likert-type scales.
Results: The results indicated a strong positive correlation between service quality, customer satisfaction, and loyalty (r = 0.75, p < 0.001). Furthermore, regression analysis revealed that service quality significantly predicted both customer satisfaction (β = 0.60, p < 0.001) and loyalty (β = 0.45, p < 0.001). Additionally, customer satisfaction emerged as a significant predictor of loyalty (β = 0.50, p < 0.001), indicating its mediating role in the relationship between service quality and loyalty.
Discussion: These findings underscore the importance of high-quality service in enhancing customer satisfaction and fostering loyalty in retail settings. Businesses should prioritize investments in service training, infrastructure, and customer relationship management to ensure positive shopping experiences and promote repeat patronage. Future research could explore additional factors influencing customer loyalty and examine the effectiveness of specific loyalty programs and incentives in driving repeat business.
Key Insights: Navigating Statistical Analysis
To sum up, mastering a statistical analysis system is essential for researchers to derive meaningful insights from data. Understanding statistical concepts, choosing appropriate methods, and adhering to ethical guidelines are paramount.
Additionally, transparent reporting, rigorous methodology, and careful interpretation ensure the integrity and reliability of research findings. By avoiding common pitfalls and embracing best practices, researchers can contribute to advancing knowledge and making informed decisions across various fields.
Ultimately, statistical analysis is a powerful tool for unlocking the mysteries hidden within data, guiding us toward more profound understanding and innovation.
Readers also enjoyed

WHY WAIT? PLACE AN ORDER RIGHT NOW!
Just fill out the form, press the button, and have no worries!
We use cookies to give you the best experience possible. By continuing we’ll assume you board with our cookie policy.
When you choose to publish with PLOS, your research makes an impact. Make your work accessible to all, without restrictions, and accelerate scientific discovery with options like preprints and published peer review that make your work more Open.
- PLOS Biology
- PLOS Climate
- PLOS Complex Systems
- PLOS Computational Biology
- PLOS Digital Health
- PLOS Genetics
- PLOS Global Public Health
- PLOS Medicine
- PLOS Mental Health
- PLOS Neglected Tropical Diseases
- PLOS Pathogens
- PLOS Sustainability and Transformation
- PLOS Collections
- How to Report Statistics

Ensure appropriateness and rigor, avoid flexibility and above all never manipulate results
In many fields, a statistical analysis forms the heart of both the methods and results sections of a manuscript. Learn how to report statistical analyses, and what other context is important for publication success and future reproducibility.
A matter of principle
First and foremost, the statistical methods employed in research must always be:
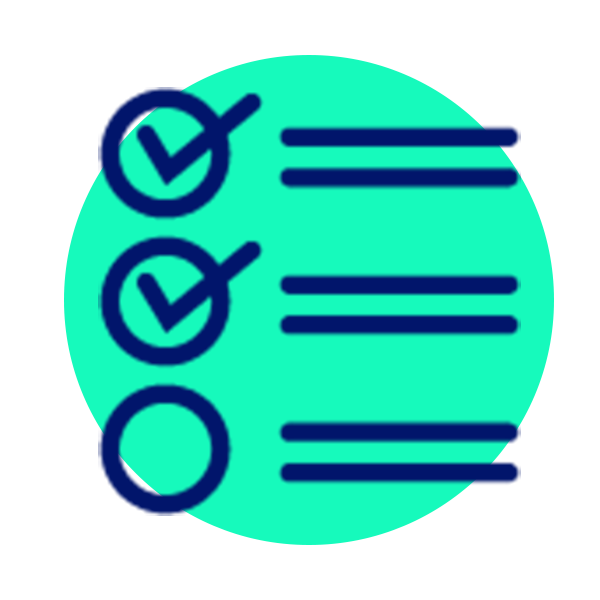
Appropriate for the study design

Rigorously reported in sufficient detail for others to reproduce the analysis

Free of manipulation, selective reporting, or other forms of “spin”
Just as importantly, statistical practices must never be manipulated or misused . Misrepresenting data, selectively reporting results or searching for patterns that can be presented as statistically significant, in an attempt to yield a conclusion that is believed to be more worthy of attention or publication is a serious ethical violation. Although it may seem harmless, using statistics to “spin” results can prevent publication, undermine a published study, or lead to investigation and retraction.
Supporting public trust in science through transparency and consistency
Along with clear methods and transparent study design, the appropriate use of statistical methods and analyses impacts editorial evaluation and readers’ understanding and trust in science.
In 2011 False-Positive Psychology: Undisclosed Flexibility in Data Collection and Analysis Allows Presenting Anything as Significant exposed that “flexibility in data collection, analysis, and reporting dramatically increases actual false-positive rates” and demonstrated “how unacceptably easy it is to accumulate (and report) statistically significant evidence for a false hypothesis”.
Arguably, such problems with flexible analysis lead to the “ reproducibility crisis ” that we read about today.
A constant principle of rigorous science The appropriate, rigorous, and transparent use of statistics is a constant principle of rigorous, transparent, and Open Science. Aim to be thorough, even if a particular journal doesn’t require the same level of detail. Trust in science is all of our responsibility. You cannot create any problems by exceeding a minimum standard of information and reporting.

Sound statistical practices
While it is hard to provide statistical guidelines that are relevant for all disciplines, types of research, and all analytical techniques, adherence to rigorous and appropriate principles remains key. Here are some ways to ensure your statistics are sound.
Define your analytical methodology before you begin Take the time to consider and develop a thorough study design that defines your line of inquiry, what you plan to do, what data you will collect, and how you will analyze it. (If you applied for research grants or ethical approval, you probably already have a plan in hand!) Refer back to your study design at key moments in the research process, and above all, stick to it.
To avoid flexibility and improve the odds of acceptance, preregister your study design with a journal Many journals offer the option to submit a study design for peer review before research begins through a practice known as preregistration. If the editors approve your study design, you’ll receive a provisional acceptance for a future research article reporting the results. Preregistering is a great way to head off any intentional or unintentional flexibility in analysis. By declaring your analytical approach in advance you’ll increase the credibility and reproducibility of your results and help address publication bias, too. Getting peer review feedback on your study design and analysis plan before it has begun (when you can still make changes!) makes your research even stronger AND increases your chances of publication—even if the results are negative or null. Never underestimate how much you can help increase the public’s trust in science by planning your research in this way.
Imagine replicating or extending your own work, years in the future Imagine that you are describing your approach to statistical analysis for your future self, in exactly the same way as we have described for writing your methods section . What would you need to know to replicate or extend your own work? When you consider that you might be at a different institution, working with different colleagues, using different programs, applications, resources — or maybe even adopting new statistical techniques that have emerged — you can help yourself imagine the level of reporting specificity that you yourself would require to redo or extend your work. Consider:
- Which details would you need to be reminded of?
- What did you do to the raw data before analysis?
- Did the purpose of the analysis change before or during the experiments?
- What participants did you decide to exclude?
- What process did you adjust, during your work?
Even if a necessary adjustment you made was not ideal, transparency is the key to ensuring this is not regarded as an issue in the future. It is far better to transparently convey any non-optimal techniques or constraints than to conceal them, which could result in reproducibility or ethical issues downstream.
Existing standards, checklists, guidelines for specific disciplines
You can apply the Open Science practices outlined above no matter what your area of expertise—but in many cases, you may still need more detailed guidance specific to your own field. Many disciplines, fields, and projects have worked hard to develop guidelines and resources to help with statistics, and to identify and avoid bad statistical practices. Below, you’ll find some of the key materials.
TIP: Do you have a specific journal in mind?
Be sure to read the submission guidelines for the specific journal you are submitting to, in order to discover any journal- or field-specific policies, initiatives or tools to utilize.
Articles on statistical methods and reporting
Makin, T.R., Orban de Xivry, J. Science Forum: Ten common statistical mistakes to watch out for when writing or reviewing a manuscript . eLife 2019;8:e48175 (2019). https://doi.org/10.7554/eLife.48175
Munafò, M., Nosek, B., Bishop, D. et al. A manifesto for reproducible science . Nat Hum Behav 1, 0021 (2017). https://doi.org/10.1038/s41562-016-0021
Writing tips
Your use of statistics should be rigorous, appropriate, and uncompromising in avoidance of analytical flexibility. While this is difficult, do not compromise on rigorous standards for credibility!

- Remember that trust in science is everyone’s responsibility.
- Keep in mind future replicability.
- Consider preregistering your analysis plan to have it (i) reviewed before results are collected to check problems before they occur and (ii) to avoid any analytical flexibility.
- Follow principles, but also checklists and field- and journal-specific guidelines.
- Consider a commitment to rigorous and transparent science a personal responsibility, and not simple adhering to journal guidelines.
- Be specific about all decisions made during the experiments that someone reproducing your work would need to know.
- Consider a course in advanced and new statistics, if you feel you have not focused on it enough during your research training.
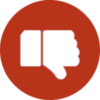
Don’t
- Misuse statistics to influence significance or other interpretations of results
- Conduct your statistical analyses if you are unsure of what you are doing—seek feedback (e.g. via preregistration) from a statistical specialist first.
- How to Write a Great Title
- How to Write an Abstract
- How to Write Your Methods
- How to Write Discussions and Conclusions
- How to Edit Your Work
The contents of the Peer Review Center are also available as a live, interactive training session, complete with slides, talking points, and activities. …
The contents of the Writing Center are also available as a live, interactive training session, complete with slides, talking points, and activities. …
There’s a lot to consider when deciding where to submit your work. Learn how to choose a journal that will help your study reach its audience, while reflecting your values as a researcher…
Reporting statistical methods and outcome of statistical analyses in research articles
- Published: 15 June 2020
- Volume 72 , pages 481–485, ( 2020 )
Cite this article
- Mariusz Cichoń 1
17k Accesses
12 Citations
1 Altmetric
Explore all metrics
Avoid common mistakes on your manuscript.
Introduction
Statistical methods constitute a powerful tool in modern life sciences. This tool is primarily used to disentangle whether the observed differences, relationships or congruencies are meaningful or may just occur by chance. Thus, statistical inference is an unavoidable part of scientific work. The knowledge of statistics is usually quite limited among researchers representing the field of life sciences, particularly when it comes to constraints imposed on the use of statistical tools and possible interpretations. A common mistake is that researchers take for granted the ability to perform a valid statistical analysis. However, at the stage of data analysis, it may turn out that the gathered data cannot be analysed with any known statistical tools or that there are critical flaws in the interpretation of the results due to violations of basic assumptions of statistical methods. A common mistake made by authors is to thoughtlessly copy the choice of the statistical tests from other authors analysing similar data. This strategy, although sometimes correct, may lead to an incorrect choice of statistical tools and incorrect interpretations. Here, I aim to give some advice on how to choose suitable statistical methods and how to present the results of statistical analyses.
Important limits in the use of statistics
Statistical tools face a number of constraints. Constraints should already be considered at the stage of planning the research, as mistakes made at this stage may make statistical analyses impossible. Therefore, careful planning of sampling is critical for future success in data analyses. The most important is ensuring that the general population is sampled randomly and independently, and that the experimental design corresponds to the aims of the research. Planning a control group/groups is of particular importance. Without a suitable control group, any further inference may not be possible. Parametric tests are stronger (it is easier to reject a null hypothesis), so they should always be preferred, but such methods can be used only when the data are drawn from a general population with normal distribution. For methods based on analysis of variance (ANOVA), residuals should come from a general population with normal distribution, and in this case there is an additional important assumption of homogeneity of variance. Inferences made from analyses violating these assumptions may be incorrect.
Statistical inference
Statistical inference is asymmetrical. Scientific discovery is based on rejecting null hypotheses, so interpreting non-significant results should be taken with special care. We never know for sure why we fail to reject the null hypothesis. It may indeed be true, but it is also possible that our sample size was too small or variance too large to capture the differences or relationships. We also may fail just by chance. Assuming a significance level of p = 0.05 means that we run the risk of rejecting a null hypothesis in 5% of such analyses. Thus, interpretation of non-significant results should always be accompanied by the so-called power analysis, which shows the strength of our inference.
Experimental design and data analyses
The experimental design is a critical part of study planning. The design must correspond to the aims of the study presented in the Introduction section. In turn, the statistical methods must be suited to the experimental design so that the data analyses will enable the questions stated in the Introduction to be answered. In general, simple experimental designs allow the use of simple methods like t-tests, simple correlations, etc., while more complicated designs (multifactor designs) require more advanced methods (see, Fig. 1 ). Data coming from more advanced designs usually cannot be analysed with simple methods. Therefore, multifactor designs cannot be followed by a simple t-test or even with one-way ANOVA, as factors may not act independently, and in such a case the interpretation of the results of one-way ANOVA may be incorrect. Here, it is particularly important that one may be interested in a concerted action of factors (interaction) or an action of a given factor while controlling for other factors (independent action of a factor). But even with one factor design with more than two levels, one cannot use just a simple t-test with multiple comparisons between groups. In such a case, one-way ANOVA should be performed followed by a post hoc test. The post hoc test can be done only if ANOVA rejects the null hypothesis. There is no point in using the post hoc test if the factors have only two levels (groups). In this case, the differences are already clear after ANOVA.
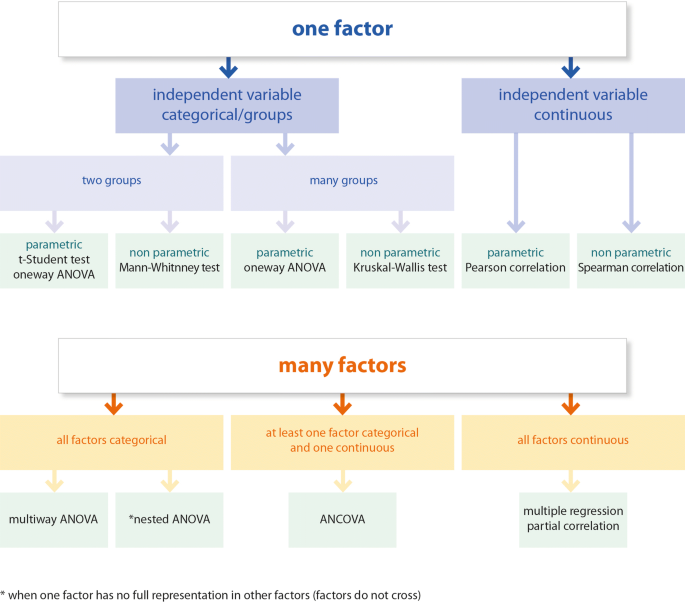
Test selection chart
Description of statistical methods in the Materials and methods section
It is in the author’s interest to provide the reader with all necessary information to judge whether the statistical tools used in the paper are the most suitable to answer the scientific question and are suited to the data structure. In the Materials and methods section, the experimental design must be described in detail, so that the reader may easily understand how the study was performed and later why such specific statistical methods were chosen. It must be clear whether the study is planned to test the relationships or differences between groups. Here, the reader should already understand the data structure, what the dependent variable is, what the factors are, and should be able to determine, even without being directly informed, whether the factors are categorical or continuous, and whether they are fixed or random. The sample size used in the analysis should be clearly stated. Sometimes sample sizes used in analyses are smaller than the original. This can happen for various reasons, for example if one fails to perform some measurements, and in such a case, the authors must clearly explain why the original sample size differs from the one used in the analyses. There must be a very good reason to omit existing data points from the analyses. Removing the so-called outliers should be an exception rather than the rule.
A description of the statistical methods should come at the end of the Materials and methods section. Here, we start by introducing the statistical techniques used to test predictions formulated in the Introduction. We describe in detail the structure of the statistical model (defining the dependent variable, the independent variables—factors, interactions if present, character of the factors—fixed or random). The variables should be defined as categorical or continuous. In the case of more advanced models, information on the methods of effects estimation or degrees of freedom should be provided. Unless there are good reasons, interactions should always be tested, even if the study is not aimed at testing an interaction. If the interaction is not the main aim of the study, non-significant interactions should be dropped from the model and new analyses without interactions should be carried out and such results reported. If the interaction appears to be significant, one cannot remove it from the model even if the interaction is not the main aim of the study. In such a case, only the interaction can be interpreted, while the interpretation of the main effects is not allowed. The author should clearly describe how the interactions will be dealt with. One may also consider using a model selection procedure which should also be clearly described.
The authors should reassure the reader that the assumptions of the selected statistical technique are fully met. It must be described how the normality of data distribution and homogeneity of variance was checked and whether these assumptions have been met. When performing data transformation, one needs to explain how it was done and whether the transformation helped to fulfil the assumptions of the parametric tests. If these assumptions are not fulfilled, one may apply non-parametric tests. It must be clearly stated why non-parametric tests are performed. Post hoc tests can be performed only when the ANOVA/Kruskal–Wallis test shows significant effects. These tests are valid for the main effects only when the interaction is not included in the model. These tests are also applicable for significant interactions. There are a number of different post hoc tests, so the selected test must be introduced in the materials and methods section.
The significance level is often mentioned in the materials and methods section. There is common consensus among researchers in life sciences for a significance level set at p = 0.05, so it is not strictly necessary to report this conventional level unless the authors always give the I type error (p-value) throughout the paper. If the author sets the significance level at a lower value, which could be the case, for example, in medical sciences, the reader must be informed about the use of a more conservative level. If the significance level is not reported, the reader will assume p = 0.05. In general, it does not matter which statistical software was used for the analyses. However, the outcome may differ slightly between different software, even if exactly the same model is set. Thus, it may be a good practice to report the name of the software at the end of the subsection describing the statistical methods. If the original code of the model analysed is provided, it would be sensible to inform the reader of the specific software and version that was used.
Presentation of the outcome in the Results section
Only the data and the analyses needed to test the hypotheses and predictions stated in the Introduction and those important for discussion should be placed in the Results section. All other outcome might be provided as supplementary materials. Some descriptive statistics are often reported in the Results section, such as means, standard errors (SE), standard deviation (SD), confidence interval (CI). It is of critical importance that these estimates can only be provided if the described data are drawn from a general population with normal distribution; otherwise median values with quartiles should be provided. A common mistake is to provide the results of non-parametric tests with parametric estimates. If one cannot assume normal distribution, providing arithmetic mean with standard deviation is misleading, as they are estimates of normal distribution. I recommend using confidence intervals instead of SE or SD, as confidence intervals are more informative (non-overlapping intervals suggest the existence of potential differences).
Descriptive statistics can be calculated from raw data (measured values) or presented as estimates from the calculated models (values corrected for independent effects of other factors in the model). The issue whether estimates from models or statistics calculated from the raw data provided throughout the paper should be clearly stated in the Materials and methods section. It is not necessary to report the descriptive statistics in the text if it is already reported in the tables or can be easily determined from the graphs.
The Results section is a narrative text which tells the reader about all the findings and guides them to refer to tables and figures if present. Each table and figure should be referenced in the text at least once. It is in the author’s interest to provide the reader the outcome of the statistical tests in such a way that the correctness of the reported values can be assessed. The value of the appropriate statistics (e.g. F, t, H, U, z, r) must always be provided, along with the sample size (N; non-parametric tests) or degrees of freedom (df; parametric tests) and I type error (p-value). The p-value is an important information, as it tells the reader about confidence related to rejecting the null hypothesis. Thus one needs to provide an exact value of I type error. A common mistake is to provide information as an inequality (p < 0.05). There is an important difference for interpretation if p = 0.049 or p = 0.001.
The outcome of simple tests (comparing two groups, testing relationship between two variables) can easily be reported in the text, but in case of multivariate models, one may rather report the outcome in the form of a table in which all factors with their possible interactions are listed with their estimates, statistics and p-values. The results of post hoc tests, if performed, may be reported in the main text, but if one reports differences between many groups or an interaction, then presenting such results in the form of a table or graph could be more informative.
The main results are often presented graphically, particularly when the effects appear to be significant. The graphs should be constructed so that they correspond to the analyses. If the main interest of the study is in an interaction, then it should be depicted in the graph. One should not present interaction in the graph if it appeared to be non-significant. When presenting differences, the mean or median value should be visualised as a dot, circle or some other symbol with some measure of variability (quartiles if a non-parametric test was performed, and SD, SE or preferably confidence intervals in the case of parametric tests) as whiskers below and above the midpoint. The midpoints should not be linked with a line unless an interaction is presented or, more generally, if the line has some biological/logical meaning in the experimental design. Some authors present differences as bar graphs. When using bar graphs, the Y -axis must start from a zero value. If a bar graph is used to show differences between groups, some measure of variability (SD, SE, CI) must also be provided, as whiskers, for example. Graphs may present the outcome of post hoc tests in the form of letters placed above the midpoint or whiskers, with the same letter indicating lack of differences and different letters signalling pairwise differences. The significant differences can also be denoted as asterisks or, preferably, p-values placed above the horizontal line linking the groups. All this must be explained in the figure caption. Relationships should be presented in the form of a scatterplot. This could be accompanied by a regression line, but only if the relationship is statistically significant. The regression line is necessary if one is interested in describing a functional relationship between two variables. If one is interested in correlation between variables, the regression line is not necessary, but could be placed in order to visualise the relationship. In this case, it must be explained in the figure caption. If regression is of interest, then providing an equation of this regression is necessary in the figure caption. Remember that graphs serve to represent the analyses performed, so if the analyses were carried out on the transformed data, the graphs should also present transformed data. In general, the tables and figure captions must be self-explanatory, so that the reader is able to understand the table/figure content without reading the main text. The table caption should be written in such a way that it is possible to understand the statistical analysis from which the results are presented.
Guidelines for the Materials and methods section:
Provide detailed description of the experimental design so that the statistical techniques will be understandable for the reader.
Make sure that factors and groups within factors are clearly introduced.
Describe all statistical techniques applied in the study and provide justification for each test (both parametric and non-parametric methods).
If parametric tests are used, describe how the normality of data distribution and homogeneity of variance (in the case of analysis of variance) was checked and state clearly that these important assumptions for parametric tests are met.
Give a rationale for using non-parametric tests.
If data transformation was applied, provide details of how this transformation was performed and state clearly that this helped to achieve normal distribution/homogeneity of variance.
In the case of multivariate analyses, describe the statistical model in detail and explain what you did with interactions.
If post hoc tests are used, clearly state which tests you use.
Specify the type of software and its version if you think it is important.
Guidelines for presentation of the outcome of statistical analyses in the Results section:
Make sure you report appropriate descriptive statistics—means, standard errors (SE), standard deviation (SD), confidence intervals (CI), etc. in case of parametric tests or median values with quartiles in case of non-parametric tests.
Provide appropriate statistics for your test (t value for t-test, F for ANOVA, H for Kruskal–Wallis test, U for Mann–Whitney test, χ 2 for chi square test, or r for correlation) along with the sample size (non-parametric tests) or degrees of freedom (df; parametric tests).
t 23 = 3.45 (the number in the subscript denotes degree of freedom, meaning the sample size of the first group minus 1 plus the sample size of the second group minus 1 for the test with independent groups, or number of pairs in paired t-test minus 1).
F 1,23 = 6.04 (first number in the subscript denotes degrees of freedom for explained variance—number of groups within factor minus 1, second number denotes degree of freedom for unexplained variance—residual variance). F-statistics should be provided separately for all factors and interactions (only if interactions are present in the model).
H = 13.8, N 1 = 15, N 2 = 18, N 3 = 12 (N 1, N 2, N 3 are sample sizes for groups compared).
U = 50, N 1 = 20, N 2 = 19 for Mann–Whitney test (N 1 and N 2 are sample sizes for groups).
χ 2 = 3.14 df = 1 (here meaning e.g. 2 × 2 contingency table).
r = 0.78, N = 32 or df = 30 (df = N − 2).
Provide exact p-values (e.g. p = 0.03), rather than standard inequality (p ≤ 0.05)
If the results of statistical analysis are presented in the form of a table, make sure the statistical model is accurately described so that the reader will understand the context of the table without referring to the text. Please ensure that the table is cited in the text.
The figure caption should include all information necessary to understand what is seen in the figure. Describe what is denoted by a bar, symbols, whiskers (mean/median, SD, SE, CI/quartiles). If you present transformed data, inform the reader about the transformation you applied. If you present the results of a post hoc test on the graph, please note what test was used and how you denote the significant differences. If you present a regression line on the scatter plot, give information as to whether you provide the line to visualise the relationship or you are indeed interested in regression, and in the latter case, give the equation for this regression line.
Further reading in statistics:
Sokal and Rolf. 2011. Biometry. Freeman.
Zar. 2010. Biostatistical analyses. Prentice Hall.
McDonald, J.H. 2014. Handbook of biological statistics. Sparky House Publishing, Baltimore, Maryland.
Quinn and Keough. 2002. Experimental design and data analysis for biologists. Cambridge University Press.
Author information
Authors and affiliations.
Institute of Environmental Sciences, Jagiellonian University, Gronostajowa 7, 30-376, Kraków, Poland
Mariusz Cichoń
You can also search for this author in PubMed Google Scholar
Corresponding author
Correspondence to Mariusz Cichoń .
Rights and permissions
Reprints and permissions
About this article
Cichoń, M. Reporting statistical methods and outcome of statistical analyses in research articles. Pharmacol. Rep 72 , 481–485 (2020). https://doi.org/10.1007/s43440-020-00110-5
Download citation
Published : 15 June 2020
Issue Date : June 2020
DOI : https://doi.org/10.1007/s43440-020-00110-5
Share this article
Anyone you share the following link with will be able to read this content:
Sorry, a shareable link is not currently available for this article.
Provided by the Springer Nature SharedIt content-sharing initiative
- Find a journal
- Publish with us
- Track your research
Have a thesis expert improve your writing
Check your thesis for plagiarism in 10 minutes, generate your apa citations for free.
- Knowledge Base
The Beginner's Guide to Statistical Analysis | 5 Steps & Examples
Statistical analysis means investigating trends, patterns, and relationships using quantitative data . It is an important research tool used by scientists, governments, businesses, and other organisations.
To draw valid conclusions, statistical analysis requires careful planning from the very start of the research process . You need to specify your hypotheses and make decisions about your research design, sample size, and sampling procedure.
After collecting data from your sample, you can organise and summarise the data using descriptive statistics . Then, you can use inferential statistics to formally test hypotheses and make estimates about the population. Finally, you can interpret and generalise your findings.
This article is a practical introduction to statistical analysis for students and researchers. We’ll walk you through the steps using two research examples. The first investigates a potential cause-and-effect relationship, while the second investigates a potential correlation between variables.
Table of contents
Step 1: write your hypotheses and plan your research design, step 2: collect data from a sample, step 3: summarise your data with descriptive statistics, step 4: test hypotheses or make estimates with inferential statistics, step 5: interpret your results, frequently asked questions about statistics.
To collect valid data for statistical analysis, you first need to specify your hypotheses and plan out your research design.
Writing statistical hypotheses
The goal of research is often to investigate a relationship between variables within a population . You start with a prediction, and use statistical analysis to test that prediction.
A statistical hypothesis is a formal way of writing a prediction about a population. Every research prediction is rephrased into null and alternative hypotheses that can be tested using sample data.
While the null hypothesis always predicts no effect or no relationship between variables, the alternative hypothesis states your research prediction of an effect or relationship.
- Null hypothesis: A 5-minute meditation exercise will have no effect on math test scores in teenagers.
- Alternative hypothesis: A 5-minute meditation exercise will improve math test scores in teenagers.
- Null hypothesis: Parental income and GPA have no relationship with each other in college students.
- Alternative hypothesis: Parental income and GPA are positively correlated in college students.
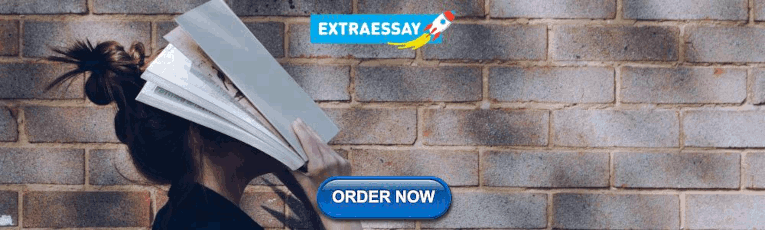
Planning your research design
A research design is your overall strategy for data collection and analysis. It determines the statistical tests you can use to test your hypothesis later on.
First, decide whether your research will use a descriptive, correlational, or experimental design. Experiments directly influence variables, whereas descriptive and correlational studies only measure variables.
- In an experimental design , you can assess a cause-and-effect relationship (e.g., the effect of meditation on test scores) using statistical tests of comparison or regression.
- In a correlational design , you can explore relationships between variables (e.g., parental income and GPA) without any assumption of causality using correlation coefficients and significance tests.
- In a descriptive design , you can study the characteristics of a population or phenomenon (e.g., the prevalence of anxiety in U.S. college students) using statistical tests to draw inferences from sample data.
Your research design also concerns whether you’ll compare participants at the group level or individual level, or both.
- In a between-subjects design , you compare the group-level outcomes of participants who have been exposed to different treatments (e.g., those who performed a meditation exercise vs those who didn’t).
- In a within-subjects design , you compare repeated measures from participants who have participated in all treatments of a study (e.g., scores from before and after performing a meditation exercise).
- In a mixed (factorial) design , one variable is altered between subjects and another is altered within subjects (e.g., pretest and posttest scores from participants who either did or didn’t do a meditation exercise).
- Experimental
- Correlational
First, you’ll take baseline test scores from participants. Then, your participants will undergo a 5-minute meditation exercise. Finally, you’ll record participants’ scores from a second math test.
In this experiment, the independent variable is the 5-minute meditation exercise, and the dependent variable is the math test score from before and after the intervention. Example: Correlational research design In a correlational study, you test whether there is a relationship between parental income and GPA in graduating college students. To collect your data, you will ask participants to fill in a survey and self-report their parents’ incomes and their own GPA.
Measuring variables
When planning a research design, you should operationalise your variables and decide exactly how you will measure them.
For statistical analysis, it’s important to consider the level of measurement of your variables, which tells you what kind of data they contain:
- Categorical data represents groupings. These may be nominal (e.g., gender) or ordinal (e.g. level of language ability).
- Quantitative data represents amounts. These may be on an interval scale (e.g. test score) or a ratio scale (e.g. age).
Many variables can be measured at different levels of precision. For example, age data can be quantitative (8 years old) or categorical (young). If a variable is coded numerically (e.g., level of agreement from 1–5), it doesn’t automatically mean that it’s quantitative instead of categorical.
Identifying the measurement level is important for choosing appropriate statistics and hypothesis tests. For example, you can calculate a mean score with quantitative data, but not with categorical data.
In a research study, along with measures of your variables of interest, you’ll often collect data on relevant participant characteristics.
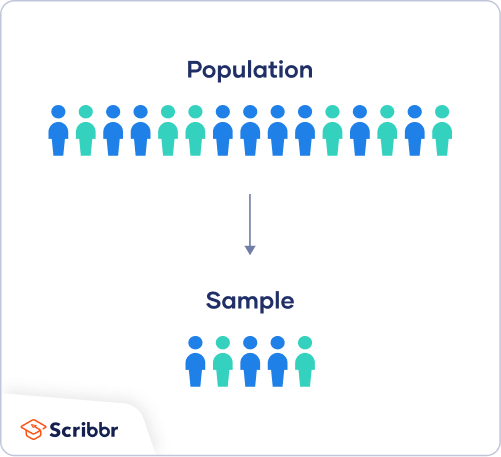
In most cases, it’s too difficult or expensive to collect data from every member of the population you’re interested in studying. Instead, you’ll collect data from a sample.
Statistical analysis allows you to apply your findings beyond your own sample as long as you use appropriate sampling procedures . You should aim for a sample that is representative of the population.
Sampling for statistical analysis
There are two main approaches to selecting a sample.
- Probability sampling: every member of the population has a chance of being selected for the study through random selection.
- Non-probability sampling: some members of the population are more likely than others to be selected for the study because of criteria such as convenience or voluntary self-selection.
In theory, for highly generalisable findings, you should use a probability sampling method. Random selection reduces sampling bias and ensures that data from your sample is actually typical of the population. Parametric tests can be used to make strong statistical inferences when data are collected using probability sampling.
But in practice, it’s rarely possible to gather the ideal sample. While non-probability samples are more likely to be biased, they are much easier to recruit and collect data from. Non-parametric tests are more appropriate for non-probability samples, but they result in weaker inferences about the population.
If you want to use parametric tests for non-probability samples, you have to make the case that:
- your sample is representative of the population you’re generalising your findings to.
- your sample lacks systematic bias.
Keep in mind that external validity means that you can only generalise your conclusions to others who share the characteristics of your sample. For instance, results from Western, Educated, Industrialised, Rich and Democratic samples (e.g., college students in the US) aren’t automatically applicable to all non-WEIRD populations.
If you apply parametric tests to data from non-probability samples, be sure to elaborate on the limitations of how far your results can be generalised in your discussion section .
Create an appropriate sampling procedure
Based on the resources available for your research, decide on how you’ll recruit participants.
- Will you have resources to advertise your study widely, including outside of your university setting?
- Will you have the means to recruit a diverse sample that represents a broad population?
- Do you have time to contact and follow up with members of hard-to-reach groups?
Your participants are self-selected by their schools. Although you’re using a non-probability sample, you aim for a diverse and representative sample. Example: Sampling (correlational study) Your main population of interest is male college students in the US. Using social media advertising, you recruit senior-year male college students from a smaller subpopulation: seven universities in the Boston area.
Calculate sufficient sample size
Before recruiting participants, decide on your sample size either by looking at other studies in your field or using statistics. A sample that’s too small may be unrepresentative of the sample, while a sample that’s too large will be more costly than necessary.
There are many sample size calculators online. Different formulas are used depending on whether you have subgroups or how rigorous your study should be (e.g., in clinical research). As a rule of thumb, a minimum of 30 units or more per subgroup is necessary.
To use these calculators, you have to understand and input these key components:
- Significance level (alpha): the risk of rejecting a true null hypothesis that you are willing to take, usually set at 5%.
- Statistical power : the probability of your study detecting an effect of a certain size if there is one, usually 80% or higher.
- Expected effect size : a standardised indication of how large the expected result of your study will be, usually based on other similar studies.
- Population standard deviation: an estimate of the population parameter based on a previous study or a pilot study of your own.
Once you’ve collected all of your data, you can inspect them and calculate descriptive statistics that summarise them.
Inspect your data
There are various ways to inspect your data, including the following:
- Organising data from each variable in frequency distribution tables .
- Displaying data from a key variable in a bar chart to view the distribution of responses.
- Visualising the relationship between two variables using a scatter plot .
By visualising your data in tables and graphs, you can assess whether your data follow a skewed or normal distribution and whether there are any outliers or missing data.
A normal distribution means that your data are symmetrically distributed around a center where most values lie, with the values tapering off at the tail ends.
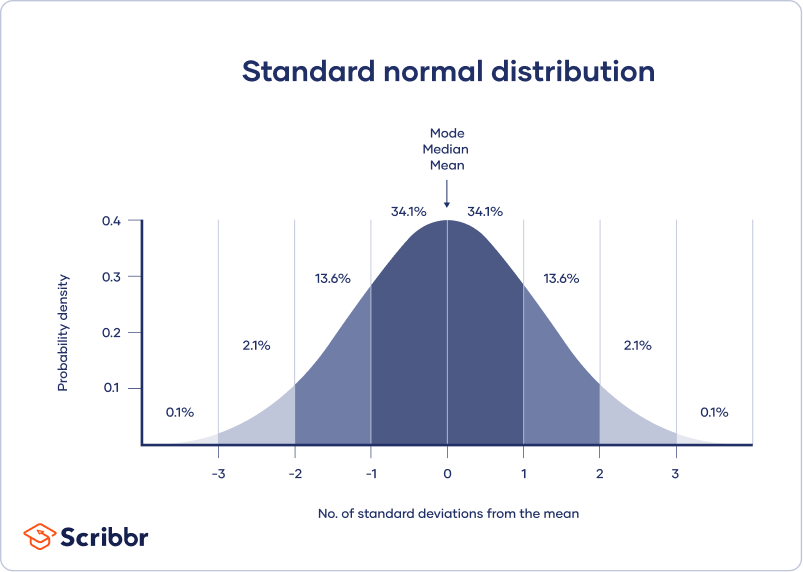
In contrast, a skewed distribution is asymmetric and has more values on one end than the other. The shape of the distribution is important to keep in mind because only some descriptive statistics should be used with skewed distributions.
Extreme outliers can also produce misleading statistics, so you may need a systematic approach to dealing with these values.
Calculate measures of central tendency
Measures of central tendency describe where most of the values in a data set lie. Three main measures of central tendency are often reported:
- Mode : the most popular response or value in the data set.
- Median : the value in the exact middle of the data set when ordered from low to high.
- Mean : the sum of all values divided by the number of values.
However, depending on the shape of the distribution and level of measurement, only one or two of these measures may be appropriate. For example, many demographic characteristics can only be described using the mode or proportions, while a variable like reaction time may not have a mode at all.
Calculate measures of variability
Measures of variability tell you how spread out the values in a data set are. Four main measures of variability are often reported:
- Range : the highest value minus the lowest value of the data set.
- Interquartile range : the range of the middle half of the data set.
- Standard deviation : the average distance between each value in your data set and the mean.
- Variance : the square of the standard deviation.
Once again, the shape of the distribution and level of measurement should guide your choice of variability statistics. The interquartile range is the best measure for skewed distributions, while standard deviation and variance provide the best information for normal distributions.
Using your table, you should check whether the units of the descriptive statistics are comparable for pretest and posttest scores. For example, are the variance levels similar across the groups? Are there any extreme values? If there are, you may need to identify and remove extreme outliers in your data set or transform your data before performing a statistical test.
From this table, we can see that the mean score increased after the meditation exercise, and the variances of the two scores are comparable. Next, we can perform a statistical test to find out if this improvement in test scores is statistically significant in the population. Example: Descriptive statistics (correlational study) After collecting data from 653 students, you tabulate descriptive statistics for annual parental income and GPA.
It’s important to check whether you have a broad range of data points. If you don’t, your data may be skewed towards some groups more than others (e.g., high academic achievers), and only limited inferences can be made about a relationship.
A number that describes a sample is called a statistic , while a number describing a population is called a parameter . Using inferential statistics , you can make conclusions about population parameters based on sample statistics.
Researchers often use two main methods (simultaneously) to make inferences in statistics.
- Estimation: calculating population parameters based on sample statistics.
- Hypothesis testing: a formal process for testing research predictions about the population using samples.
You can make two types of estimates of population parameters from sample statistics:
- A point estimate : a value that represents your best guess of the exact parameter.
- An interval estimate : a range of values that represent your best guess of where the parameter lies.
If your aim is to infer and report population characteristics from sample data, it’s best to use both point and interval estimates in your paper.
You can consider a sample statistic a point estimate for the population parameter when you have a representative sample (e.g., in a wide public opinion poll, the proportion of a sample that supports the current government is taken as the population proportion of government supporters).
There’s always error involved in estimation, so you should also provide a confidence interval as an interval estimate to show the variability around a point estimate.
A confidence interval uses the standard error and the z score from the standard normal distribution to convey where you’d generally expect to find the population parameter most of the time.
Hypothesis testing
Using data from a sample, you can test hypotheses about relationships between variables in the population. Hypothesis testing starts with the assumption that the null hypothesis is true in the population, and you use statistical tests to assess whether the null hypothesis can be rejected or not.
Statistical tests determine where your sample data would lie on an expected distribution of sample data if the null hypothesis were true. These tests give two main outputs:
- A test statistic tells you how much your data differs from the null hypothesis of the test.
- A p value tells you the likelihood of obtaining your results if the null hypothesis is actually true in the population.
Statistical tests come in three main varieties:
- Comparison tests assess group differences in outcomes.
- Regression tests assess cause-and-effect relationships between variables.
- Correlation tests assess relationships between variables without assuming causation.
Your choice of statistical test depends on your research questions, research design, sampling method, and data characteristics.
Parametric tests
Parametric tests make powerful inferences about the population based on sample data. But to use them, some assumptions must be met, and only some types of variables can be used. If your data violate these assumptions, you can perform appropriate data transformations or use alternative non-parametric tests instead.
A regression models the extent to which changes in a predictor variable results in changes in outcome variable(s).
- A simple linear regression includes one predictor variable and one outcome variable.
- A multiple linear regression includes two or more predictor variables and one outcome variable.
Comparison tests usually compare the means of groups. These may be the means of different groups within a sample (e.g., a treatment and control group), the means of one sample group taken at different times (e.g., pretest and posttest scores), or a sample mean and a population mean.
- A t test is for exactly 1 or 2 groups when the sample is small (30 or less).
- A z test is for exactly 1 or 2 groups when the sample is large.
- An ANOVA is for 3 or more groups.
The z and t tests have subtypes based on the number and types of samples and the hypotheses:
- If you have only one sample that you want to compare to a population mean, use a one-sample test .
- If you have paired measurements (within-subjects design), use a dependent (paired) samples test .
- If you have completely separate measurements from two unmatched groups (between-subjects design), use an independent (unpaired) samples test .
- If you expect a difference between groups in a specific direction, use a one-tailed test .
- If you don’t have any expectations for the direction of a difference between groups, use a two-tailed test .
The only parametric correlation test is Pearson’s r . The correlation coefficient ( r ) tells you the strength of a linear relationship between two quantitative variables.
However, to test whether the correlation in the sample is strong enough to be important in the population, you also need to perform a significance test of the correlation coefficient, usually a t test, to obtain a p value. This test uses your sample size to calculate how much the correlation coefficient differs from zero in the population.
You use a dependent-samples, one-tailed t test to assess whether the meditation exercise significantly improved math test scores. The test gives you:
- a t value (test statistic) of 3.00
- a p value of 0.0028
Although Pearson’s r is a test statistic, it doesn’t tell you anything about how significant the correlation is in the population. You also need to test whether this sample correlation coefficient is large enough to demonstrate a correlation in the population.
A t test can also determine how significantly a correlation coefficient differs from zero based on sample size. Since you expect a positive correlation between parental income and GPA, you use a one-sample, one-tailed t test. The t test gives you:
- a t value of 3.08
- a p value of 0.001
The final step of statistical analysis is interpreting your results.
Statistical significance
In hypothesis testing, statistical significance is the main criterion for forming conclusions. You compare your p value to a set significance level (usually 0.05) to decide whether your results are statistically significant or non-significant.
Statistically significant results are considered unlikely to have arisen solely due to chance. There is only a very low chance of such a result occurring if the null hypothesis is true in the population.
This means that you believe the meditation intervention, rather than random factors, directly caused the increase in test scores. Example: Interpret your results (correlational study) You compare your p value of 0.001 to your significance threshold of 0.05. With a p value under this threshold, you can reject the null hypothesis. This indicates a statistically significant correlation between parental income and GPA in male college students.
Note that correlation doesn’t always mean causation, because there are often many underlying factors contributing to a complex variable like GPA. Even if one variable is related to another, this may be because of a third variable influencing both of them, or indirect links between the two variables.
Effect size
A statistically significant result doesn’t necessarily mean that there are important real life applications or clinical outcomes for a finding.
In contrast, the effect size indicates the practical significance of your results. It’s important to report effect sizes along with your inferential statistics for a complete picture of your results. You should also report interval estimates of effect sizes if you’re writing an APA style paper .
With a Cohen’s d of 0.72, there’s medium to high practical significance to your finding that the meditation exercise improved test scores. Example: Effect size (correlational study) To determine the effect size of the correlation coefficient, you compare your Pearson’s r value to Cohen’s effect size criteria.
Decision errors
Type I and Type II errors are mistakes made in research conclusions. A Type I error means rejecting the null hypothesis when it’s actually true, while a Type II error means failing to reject the null hypothesis when it’s false.
You can aim to minimise the risk of these errors by selecting an optimal significance level and ensuring high power . However, there’s a trade-off between the two errors, so a fine balance is necessary.
Frequentist versus Bayesian statistics
Traditionally, frequentist statistics emphasises null hypothesis significance testing and always starts with the assumption of a true null hypothesis.
However, Bayesian statistics has grown in popularity as an alternative approach in the last few decades. In this approach, you use previous research to continually update your hypotheses based on your expectations and observations.
Bayes factor compares the relative strength of evidence for the null versus the alternative hypothesis rather than making a conclusion about rejecting the null hypothesis or not.
Hypothesis testing is a formal procedure for investigating our ideas about the world using statistics. It is used by scientists to test specific predictions, called hypotheses , by calculating how likely it is that a pattern or relationship between variables could have arisen by chance.
The research methods you use depend on the type of data you need to answer your research question .
- If you want to measure something or test a hypothesis , use quantitative methods . If you want to explore ideas, thoughts, and meanings, use qualitative methods .
- If you want to analyse a large amount of readily available data, use secondary data. If you want data specific to your purposes with control over how they are generated, collect primary data.
- If you want to establish cause-and-effect relationships between variables , use experimental methods. If you want to understand the characteristics of a research subject, use descriptive methods.
Statistical analysis is the main method for analyzing quantitative research data . It uses probabilities and models to test predictions about a population from sample data.
Is this article helpful?
Other students also liked, a quick guide to experimental design | 5 steps & examples, controlled experiments | methods & examples of control, between-subjects design | examples, pros & cons, more interesting articles.
- Central Limit Theorem | Formula, Definition & Examples
- Central Tendency | Understanding the Mean, Median & Mode
- Correlation Coefficient | Types, Formulas & Examples
- Descriptive Statistics | Definitions, Types, Examples
- How to Calculate Standard Deviation (Guide) | Calculator & Examples
- How to Calculate Variance | Calculator, Analysis & Examples
- How to Find Degrees of Freedom | Definition & Formula
- How to Find Interquartile Range (IQR) | Calculator & Examples
- How to Find Outliers | Meaning, Formula & Examples
- How to Find the Geometric Mean | Calculator & Formula
- How to Find the Mean | Definition, Examples & Calculator
- How to Find the Median | Definition, Examples & Calculator
- How to Find the Range of a Data Set | Calculator & Formula
- Inferential Statistics | An Easy Introduction & Examples
- Levels of measurement: Nominal, ordinal, interval, ratio
- Missing Data | Types, Explanation, & Imputation
- Normal Distribution | Examples, Formulas, & Uses
- Null and Alternative Hypotheses | Definitions & Examples
- Poisson Distributions | Definition, Formula & Examples
- Skewness | Definition, Examples & Formula
- T-Distribution | What It Is and How To Use It (With Examples)
- The Standard Normal Distribution | Calculator, Examples & Uses
- Type I & Type II Errors | Differences, Examples, Visualizations
- Understanding Confidence Intervals | Easy Examples & Formulas
- Variability | Calculating Range, IQR, Variance, Standard Deviation
- What is Effect Size and Why Does It Matter? (Examples)
- What Is Interval Data? | Examples & Definition
- What Is Nominal Data? | Examples & Definition
- What Is Ordinal Data? | Examples & Definition
- What Is Ratio Data? | Examples & Definition
- What Is the Mode in Statistics? | Definition, Examples & Calculator

Statistical Analysis in Research: Meaning, Methods and Types
Home » Videos » Statistical Analysis in Research: Meaning, Methods and Types
The scientific method is an empirical approach to acquiring new knowledge by making skeptical observations and analyses to develop a meaningful interpretation. It is the basis of research and the primary pillar of modern science. Researchers seek to understand the relationships between factors associated with the phenomena of interest. In some cases, research works with vast chunks of data, making it difficult to observe or manipulate each data point. As a result, statistical analysis in research becomes a means of evaluating relationships and interconnections between variables with tools and analytical techniques for working with large data. Since researchers use statistical power analysis to assess the probability of finding an effect in such an investigation, the method is relatively accurate. Hence, statistical analysis in research eases analytical methods by focusing on the quantifiable aspects of phenomena.
What is Statistical Analysis in Research? A Simplified Definition
Statistical analysis uses quantitative data to investigate patterns, relationships, and patterns to understand real-life and simulated phenomena. The approach is a key analytical tool in various fields, including academia, business, government, and science in general. This statistical analysis in research definition implies that the primary focus of the scientific method is quantitative research. Notably, the investigator targets the constructs developed from general concepts as the researchers can quantify their hypotheses and present their findings in simple statistics.
When a business needs to learn how to improve its product, they collect statistical data about the production line and customer satisfaction. Qualitative data is valuable and often identifies the most common themes in the stakeholders’ responses. On the other hand, the quantitative data creates a level of importance, comparing the themes based on their criticality to the affected persons. For instance, descriptive statistics highlight tendency, frequency, variation, and position information. While the mean shows the average number of respondents who value a certain aspect, the variance indicates the accuracy of the data. In any case, statistical analysis creates simplified concepts used to understand the phenomenon under investigation. It is also a key component in academia as the primary approach to data representation, especially in research projects, term papers and dissertations.
Most Useful Statistical Analysis Methods in Research
Using statistical analysis methods in research is inevitable, especially in academic assignments, projects, and term papers. It’s always advisable to seek assistance from your professor or you can try research paper writing by CustomWritings before you start your academic project or write statistical analysis in research paper. Consulting an expert when developing a topic for your thesis or short mid-term assignment increases your chances of getting a better grade. Most importantly, it improves your understanding of research methods with insights on how to enhance the originality and quality of personalized essays. Professional writers can also help select the most suitable statistical analysis method for your thesis, influencing the choice of data and type of study.
Descriptive Statistics
Descriptive statistics is a statistical method summarizing quantitative figures to understand critical details about the sample and population. A description statistic is a figure that quantifies a specific aspect of the data. For instance, instead of analyzing the behavior of a thousand students, research can identify the most common actions among them. By doing this, the person utilizes statistical analysis in research, particularly descriptive statistics.
- Measures of central tendency . Central tendency measures are the mean, mode, and media or the averages denoting specific data points. They assess the centrality of the probability distribution, hence the name. These measures describe the data in relation to the center.
- Measures of frequency . These statistics document the number of times an event happens. They include frequency, count, ratios, rates, and proportions. Measures of frequency can also show how often a score occurs.
- Measures of dispersion/variation . These descriptive statistics assess the intervals between the data points. The objective is to view the spread or disparity between the specific inputs. Measures of variation include the standard deviation, variance, and range. They indicate how the spread may affect other statistics, such as the mean.
- Measures of position . Sometimes researchers can investigate relationships between scores. Measures of position, such as percentiles, quartiles, and ranks, demonstrate this association. They are often useful when comparing the data to normalized information.
Inferential Statistics
Inferential statistics is critical in statistical analysis in quantitative research. This approach uses statistical tests to draw conclusions about the population. Examples of inferential statistics include t-tests, F-tests, ANOVA, p-value, Mann-Whitney U test, and Wilcoxon W test. This
Common Statistical Analysis in Research Types
Although inferential and descriptive statistics can be classified as types of statistical analysis in research, they are mostly considered analytical methods. Types of research are distinguishable by the differences in the methodology employed in analyzing, assembling, classifying, manipulating, and interpreting data. The categories may also depend on the type of data used.
Predictive Analysis
Predictive research analyzes past and present data to assess trends and predict future events. An excellent example of predictive analysis is a market survey that seeks to understand customers’ spending habits to weigh the possibility of a repeat or future purchase. Such studies assess the likelihood of an action based on trends.
Prescriptive Analysis
On the other hand, a prescriptive analysis targets likely courses of action. It’s decision-making research designed to identify optimal solutions to a problem. Its primary objective is to test or assess alternative measures.
Causal Analysis
Causal research investigates the explanation behind the events. It explores the relationship between factors for causation. Thus, researchers use causal analyses to analyze root causes, possible problems, and unknown outcomes.
Mechanistic Analysis
This type of research investigates the mechanism of action. Instead of focusing only on the causes or possible outcomes, researchers may seek an understanding of the processes involved. In such cases, they use mechanistic analyses to document, observe, or learn the mechanisms involved.
Exploratory Data Analysis
Similarly, an exploratory study is extensive with a wider scope and minimal limitations. This type of research seeks insight into the topic of interest. An exploratory researcher does not try to generalize or predict relationships. Instead, they look for information about the subject before conducting an in-depth analysis.
The Importance of Statistical Analysis in Research
As a matter of fact, statistical analysis provides critical information for decision-making. Decision-makers require past trends and predictive assumptions to inform their actions. In most cases, the data is too complex or lacks meaningful inferences. Statistical tools for analyzing such details help save time and money, deriving only valuable information for assessment. An excellent statistical analysis in research example is a randomized control trial (RCT) for the Covid-19 vaccine. You can download a sample of such a document online to understand the significance such analyses have to the stakeholders. A vaccine RCT assesses the effectiveness, side effects, duration of protection, and other benefits. Hence, statistical analysis in research is a helpful tool for understanding data.
Sources and links For the articles and videos I use different databases, such as Eurostat, OECD World Bank Open Data, Data Gov and others. You are free to use the video I have made on your site using the link or the embed code. If you have any questions, don’t hesitate to write to me!
Support statistics and data, if you have reached the end and like this project, you can donate a coffee to “statistics and data”..
Copyright © 2022 Statistics and Data

An official website of the United States government, Department of Justice.
Here's how you know
Official websites use .gov A .gov website belongs to an official government organization in the United States.
Secure .gov websites use HTTPS A lock ( Lock A locked padlock ) or https:// means you’ve safely connected to the .gov website. Share sensitive information only on official, secure websites.
Evaluating the Robustness and Ruggedness of a Statistical Model for Comparison of Mass Spectral Data for Seized Drug Identification
This paper addresses the increasing challenges of identifying novel psychoactive substances based on visual evaluation of spectra in gas chromatography-mass spectrometry; it lays out a summary of the research project, including major goals and objectives, research design, methods, and data analysis, outcomes, limitations, artifacts, references cited, and five appendices.
The authors report on their efforts to evaluate the robustness and ruggedness of the statistical comparison method for compounds representing different novel psychoactive substance (NPS) classes. They suggest that the statistical method described and demonstrated in this report will provide an objective method to statistically compare mass spectra, and note that the method is an extension of current methods to compare spectra, with a statistical evaluation of the reference spectrum and the sample spectrum rather than a visual assessment. For their evaluation, the authors selected compounds representing different NPS classes including structural and positional isomers that were previously documented as being difficult to distinguish based on electron-ionization (EI) mass spectra. The four specific research were: to access the effect of sample concentration on statistical association and discrimination of positional isomers (robustness); to assess the effect of different instruments on statistical association and discrimination of positional isomers (ruggedness); to develop and implement testing of the statistical comparison method in operational forensic science laboratories (testing); and to develop and host training sessions to provide recommendations for implementing the method in forensic laboratories (training). The report describes the authors’ research design, methods, and data analysis techniques. Outcomes are discussed in detail, and descriptions are provided of the six data sets that were generated by the project. The appendices include: Instrument Parameters; Association and Discrimination of Synthetic Cathinones; Association and Discrimination of Fentanyl Analogs; Association and Discrimination of Synthetic Cannabinoids; and Association and Discrimination of Fluoroisobutyryl Fentanyl (FIBF) Position Isomers.
Additional Details
Related topics, similar publications.
- Use of Laser-Induced Breakdown Spectrometry (LIBS) in Sorting Commingled Human Remains
- Law Enforcement Officers Safety and Wellness: A Multi-Level Study
- Examining Financial Fraud Against Older Adults
AI Index Report
The AI Index Report tracks, collates, distills, and visualizes data related to artificial intelligence. Our mission is to provide unbiased, rigorously vetted, broadly sourced data in order for policymakers, researchers, executives, journalists, and the general public to develop a more thorough and nuanced understanding of the complex field of AI. The report aims to be the world’s most credible and authoritative source for data and insights about AI.
Subscribe to receive the 2024 report in your inbox!
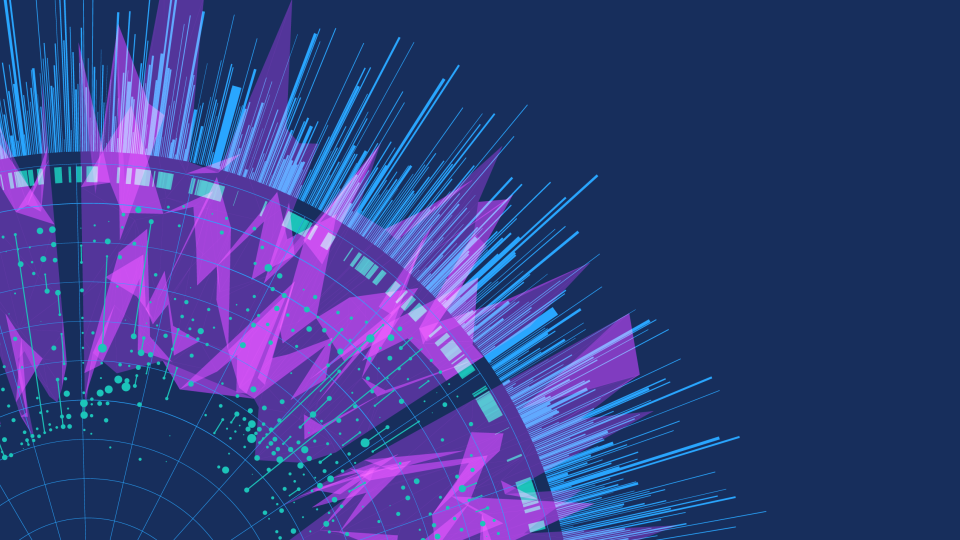
Coming Soon: 2024 AI Index Report!
The 2024 AI Index Report will be out April 15! Sign up for our mailing list to receive it in your inbox.
Steering Committee Co-Directors
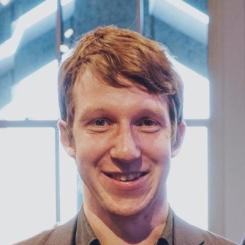
Ray Perrault
Steering committee members.
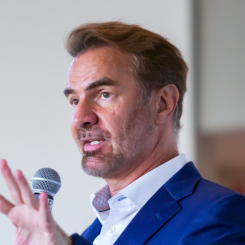
Erik Brynjolfsson
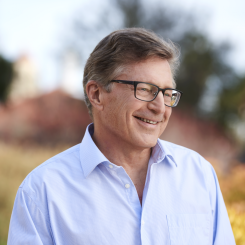
John Etchemendy
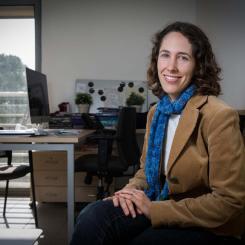
Katrina Ligett
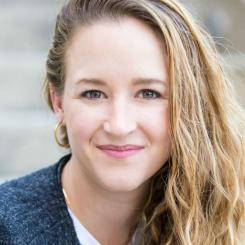
Terah Lyons
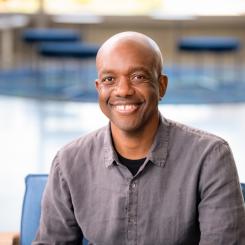
James Manyika
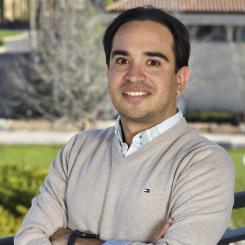
Juan Carlos Niebles
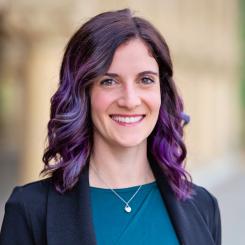
Vanessa Parli
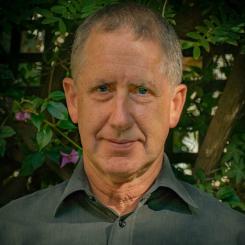
Yoav Shoham
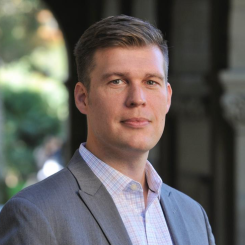
Russell Wald
Staff members.
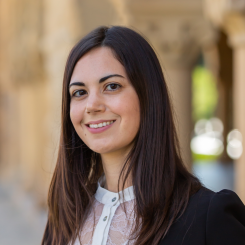
Loredana Fattorini
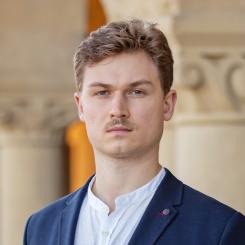
Nestor Maslej
Letter from the co-directors.
AI has moved into its era of deployment; throughout 2022 and the beginning of 2023, new large-scale AI models have been released every month. These models, such as ChatGPT, Stable Diffusion, Whisper, and DALL-E 2, are capable of an increasingly broad range of tasks, from text manipulation and analysis, to image generation, to unprecedentedly good speech recognition. These systems demonstrate capabilities in question answering, and the generation of text, image, and code unimagined a decade ago, and they outperform the state of the art on many benchmarks, old and new. However, they are prone to hallucination, routinely biased, and can be tricked into serving nefarious aims, highlighting the complicated ethical challenges associated with their deployment.
Although 2022 was the first year in a decade where private AI investment decreased, AI is still a topic of great interest to policymakers, industry leaders, researchers, and the public. Policymakers are talking about AI more than ever before. Industry leaders that have integrated AI into their businesses are seeing tangible cost and revenue benefits. The number of AI publications and collaborations continues to increase. And the public is forming sharper opinions about AI and which elements they like or dislike.
AI will continue to improve and, as such, become a greater part of all our lives. Given the increased presence of this technology and its potential for massive disruption, we should all begin thinking more critically about how exactly we want AI to be developed and deployed. We should also ask questions about who is deploying it—as our analysis shows, AI is increasingly defined by the actions of a small set of private sector actors, rather than a broader range of societal actors. This year’s AI Index paints a picture of where we are so far with AI, in order to highlight what might await us in the future.
- Jack Clark and Ray Perrault
Our Supporting Partners
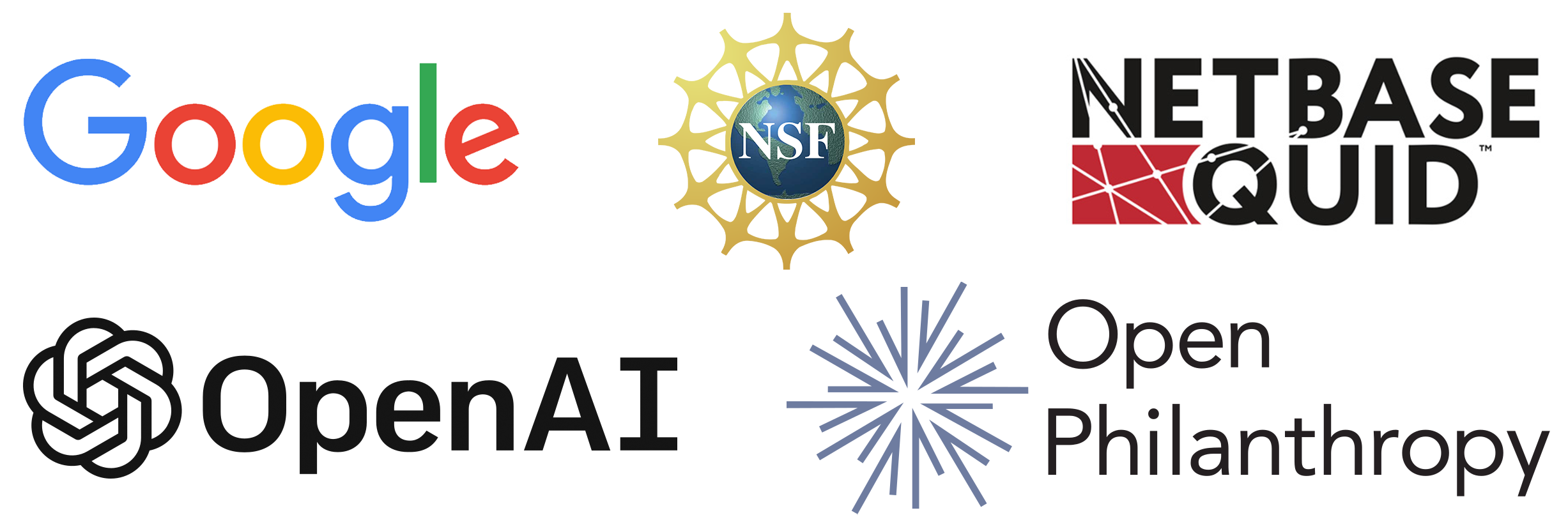
Analytics & Research Partners

Stay up to date on the AI Index by subscribing to the Stanford HAI newsletter.

An official website of the United States government
The .gov means it’s official. Federal government websites often end in .gov or .mil. Before sharing sensitive information, make sure you’re on a federal government site.
The site is secure. The https:// ensures that you are connecting to the official website and that any information you provide is encrypted and transmitted securely.
- Publications
- Account settings
Preview improvements coming to the PMC website in October 2024. Learn More or Try it out now .
- Advanced Search
- Journal List
- Korean J Anesthesiol
- v.71(2); 2018 Apr
Introduction to systematic review and meta-analysis
1 Department of Anesthesiology and Pain Medicine, Inje University Seoul Paik Hospital, Seoul, Korea
2 Department of Anesthesiology and Pain Medicine, Chung-Ang University College of Medicine, Seoul, Korea
Systematic reviews and meta-analyses present results by combining and analyzing data from different studies conducted on similar research topics. In recent years, systematic reviews and meta-analyses have been actively performed in various fields including anesthesiology. These research methods are powerful tools that can overcome the difficulties in performing large-scale randomized controlled trials. However, the inclusion of studies with any biases or improperly assessed quality of evidence in systematic reviews and meta-analyses could yield misleading results. Therefore, various guidelines have been suggested for conducting systematic reviews and meta-analyses to help standardize them and improve their quality. Nonetheless, accepting the conclusions of many studies without understanding the meta-analysis can be dangerous. Therefore, this article provides an easy introduction to clinicians on performing and understanding meta-analyses.
Introduction
A systematic review collects all possible studies related to a given topic and design, and reviews and analyzes their results [ 1 ]. During the systematic review process, the quality of studies is evaluated, and a statistical meta-analysis of the study results is conducted on the basis of their quality. A meta-analysis is a valid, objective, and scientific method of analyzing and combining different results. Usually, in order to obtain more reliable results, a meta-analysis is mainly conducted on randomized controlled trials (RCTs), which have a high level of evidence [ 2 ] ( Fig. 1 ). Since 1999, various papers have presented guidelines for reporting meta-analyses of RCTs. Following the Quality of Reporting of Meta-analyses (QUORUM) statement [ 3 ], and the appearance of registers such as Cochrane Library’s Methodology Register, a large number of systematic literature reviews have been registered. In 2009, the Preferred Reporting Items for Systematic Reviews and Meta-Analyses (PRISMA) statement [ 4 ] was published, and it greatly helped standardize and improve the quality of systematic reviews and meta-analyses [ 5 ].

Levels of evidence.
In anesthesiology, the importance of systematic reviews and meta-analyses has been highlighted, and they provide diagnostic and therapeutic value to various areas, including not only perioperative management but also intensive care and outpatient anesthesia [6–13]. Systematic reviews and meta-analyses include various topics, such as comparing various treatments of postoperative nausea and vomiting [ 14 , 15 ], comparing general anesthesia and regional anesthesia [ 16 – 18 ], comparing airway maintenance devices [ 8 , 19 ], comparing various methods of postoperative pain control (e.g., patient-controlled analgesia pumps, nerve block, or analgesics) [ 20 – 23 ], comparing the precision of various monitoring instruments [ 7 ], and meta-analysis of dose-response in various drugs [ 12 ].
Thus, literature reviews and meta-analyses are being conducted in diverse medical fields, and the aim of highlighting their importance is to help better extract accurate, good quality data from the flood of data being produced. However, a lack of understanding about systematic reviews and meta-analyses can lead to incorrect outcomes being derived from the review and analysis processes. If readers indiscriminately accept the results of the many meta-analyses that are published, incorrect data may be obtained. Therefore, in this review, we aim to describe the contents and methods used in systematic reviews and meta-analyses in a way that is easy to understand for future authors and readers of systematic review and meta-analysis.
Study Planning
It is easy to confuse systematic reviews and meta-analyses. A systematic review is an objective, reproducible method to find answers to a certain research question, by collecting all available studies related to that question and reviewing and analyzing their results. A meta-analysis differs from a systematic review in that it uses statistical methods on estimates from two or more different studies to form a pooled estimate [ 1 ]. Following a systematic review, if it is not possible to form a pooled estimate, it can be published as is without progressing to a meta-analysis; however, if it is possible to form a pooled estimate from the extracted data, a meta-analysis can be attempted. Systematic reviews and meta-analyses usually proceed according to the flowchart presented in Fig. 2 . We explain each of the stages below.

Flowchart illustrating a systematic review.
Formulating research questions
A systematic review attempts to gather all available empirical research by using clearly defined, systematic methods to obtain answers to a specific question. A meta-analysis is the statistical process of analyzing and combining results from several similar studies. Here, the definition of the word “similar” is not made clear, but when selecting a topic for the meta-analysis, it is essential to ensure that the different studies present data that can be combined. If the studies contain data on the same topic that can be combined, a meta-analysis can even be performed using data from only two studies. However, study selection via a systematic review is a precondition for performing a meta-analysis, and it is important to clearly define the Population, Intervention, Comparison, Outcomes (PICO) parameters that are central to evidence-based research. In addition, selection of the research topic is based on logical evidence, and it is important to select a topic that is familiar to readers without clearly confirmed the evidence [ 24 ].
Protocols and registration
In systematic reviews, prior registration of a detailed research plan is very important. In order to make the research process transparent, primary/secondary outcomes and methods are set in advance, and in the event of changes to the method, other researchers and readers are informed when, how, and why. Many studies are registered with an organization like PROSPERO ( http://www.crd.york.ac.uk/PROSPERO/ ), and the registration number is recorded when reporting the study, in order to share the protocol at the time of planning.
Defining inclusion and exclusion criteria
Information is included on the study design, patient characteristics, publication status (published or unpublished), language used, and research period. If there is a discrepancy between the number of patients included in the study and the number of patients included in the analysis, this needs to be clearly explained while describing the patient characteristics, to avoid confusing the reader.
Literature search and study selection
In order to secure proper basis for evidence-based research, it is essential to perform a broad search that includes as many studies as possible that meet the inclusion and exclusion criteria. Typically, the three bibliographic databases Medline, Embase, and Cochrane Central Register of Controlled Trials (CENTRAL) are used. In domestic studies, the Korean databases KoreaMed, KMBASE, and RISS4U may be included. Effort is required to identify not only published studies but also abstracts, ongoing studies, and studies awaiting publication. Among the studies retrieved in the search, the researchers remove duplicate studies, select studies that meet the inclusion/exclusion criteria based on the abstracts, and then make the final selection of studies based on their full text. In order to maintain transparency and objectivity throughout this process, study selection is conducted independently by at least two investigators. When there is a inconsistency in opinions, intervention is required via debate or by a third reviewer. The methods for this process also need to be planned in advance. It is essential to ensure the reproducibility of the literature selection process [ 25 ].
Quality of evidence
However, well planned the systematic review or meta-analysis is, if the quality of evidence in the studies is low, the quality of the meta-analysis decreases and incorrect results can be obtained [ 26 ]. Even when using randomized studies with a high quality of evidence, evaluating the quality of evidence precisely helps determine the strength of recommendations in the meta-analysis. One method of evaluating the quality of evidence in non-randomized studies is the Newcastle-Ottawa Scale, provided by the Ottawa Hospital Research Institute 1) . However, we are mostly focusing on meta-analyses that use randomized studies.
If the Grading of Recommendations, Assessment, Development and Evaluations (GRADE) system ( http://www.gradeworkinggroup.org/ ) is used, the quality of evidence is evaluated on the basis of the study limitations, inaccuracies, incompleteness of outcome data, indirectness of evidence, and risk of publication bias, and this is used to determine the strength of recommendations [ 27 ]. As shown in Table 1 , the study limitations are evaluated using the “risk of bias” method proposed by Cochrane 2) . This method classifies bias in randomized studies as “low,” “high,” or “unclear” on the basis of the presence or absence of six processes (random sequence generation, allocation concealment, blinding participants or investigators, incomplete outcome data, selective reporting, and other biases) [ 28 ].
The Cochrane Collaboration’s Tool for Assessing the Risk of Bias [ 28 ]
Data extraction
Two different investigators extract data based on the objectives and form of the study; thereafter, the extracted data are reviewed. Since the size and format of each variable are different, the size and format of the outcomes are also different, and slight changes may be required when combining the data [ 29 ]. If there are differences in the size and format of the outcome variables that cause difficulties combining the data, such as the use of different evaluation instruments or different evaluation timepoints, the analysis may be limited to a systematic review. The investigators resolve differences of opinion by debate, and if they fail to reach a consensus, a third-reviewer is consulted.
Data Analysis
The aim of a meta-analysis is to derive a conclusion with increased power and accuracy than what could not be able to achieve in individual studies. Therefore, before analysis, it is crucial to evaluate the direction of effect, size of effect, homogeneity of effects among studies, and strength of evidence [ 30 ]. Thereafter, the data are reviewed qualitatively and quantitatively. If it is determined that the different research outcomes cannot be combined, all the results and characteristics of the individual studies are displayed in a table or in a descriptive form; this is referred to as a qualitative review. A meta-analysis is a quantitative review, in which the clinical effectiveness is evaluated by calculating the weighted pooled estimate for the interventions in at least two separate studies.
The pooled estimate is the outcome of the meta-analysis, and is typically explained using a forest plot ( Figs. 3 and and4). 4 ). The black squares in the forest plot are the odds ratios (ORs) and 95% confidence intervals in each study. The area of the squares represents the weight reflected in the meta-analysis. The black diamond represents the OR and 95% confidence interval calculated across all the included studies. The bold vertical line represents a lack of therapeutic effect (OR = 1); if the confidence interval includes OR = 1, it means no significant difference was found between the treatment and control groups.

Forest plot analyzed by two different models using the same data. (A) Fixed-effect model. (B) Random-effect model. The figure depicts individual trials as filled squares with the relative sample size and the solid line as the 95% confidence interval of the difference. The diamond shape indicates the pooled estimate and uncertainty for the combined effect. The vertical line indicates the treatment group shows no effect (OR = 1). Moreover, if the confidence interval includes 1, then the result shows no evidence of difference between the treatment and control groups.

Forest plot representing homogeneous data.
Dichotomous variables and continuous variables
In data analysis, outcome variables can be considered broadly in terms of dichotomous variables and continuous variables. When combining data from continuous variables, the mean difference (MD) and standardized mean difference (SMD) are used ( Table 2 ).
Summary of Meta-analysis Methods Available in RevMan [ 28 ]
The MD is the absolute difference in mean values between the groups, and the SMD is the mean difference between groups divided by the standard deviation. When results are presented in the same units, the MD can be used, but when results are presented in different units, the SMD should be used. When the MD is used, the combined units must be shown. A value of “0” for the MD or SMD indicates that the effects of the new treatment method and the existing treatment method are the same. A value lower than “0” means the new treatment method is less effective than the existing method, and a value greater than “0” means the new treatment is more effective than the existing method.
When combining data for dichotomous variables, the OR, risk ratio (RR), or risk difference (RD) can be used. The RR and RD can be used for RCTs, quasi-experimental studies, or cohort studies, and the OR can be used for other case-control studies or cross-sectional studies. However, because the OR is difficult to interpret, using the RR and RD, if possible, is recommended. If the outcome variable is a dichotomous variable, it can be presented as the number needed to treat (NNT), which is the minimum number of patients who need to be treated in the intervention group, compared to the control group, for a given event to occur in at least one patient. Based on Table 3 , in an RCT, if x is the probability of the event occurring in the control group and y is the probability of the event occurring in the intervention group, then x = c/(c + d), y = a/(a + b), and the absolute risk reduction (ARR) = x − y. NNT can be obtained as the reciprocal, 1/ARR.
Calculation of the Number Needed to Treat in the Dichotomous table
Fixed-effect models and random-effect models
In order to analyze effect size, two types of models can be used: a fixed-effect model or a random-effect model. A fixed-effect model assumes that the effect of treatment is the same, and that variation between results in different studies is due to random error. Thus, a fixed-effect model can be used when the studies are considered to have the same design and methodology, or when the variability in results within a study is small, and the variance is thought to be due to random error. Three common methods are used for weighted estimation in a fixed-effect model: 1) inverse variance-weighted estimation 3) , 2) Mantel-Haenszel estimation 4) , and 3) Peto estimation 5) .
A random-effect model assumes heterogeneity between the studies being combined, and these models are used when the studies are assumed different, even if a heterogeneity test does not show a significant result. Unlike a fixed-effect model, a random-effect model assumes that the size of the effect of treatment differs among studies. Thus, differences in variation among studies are thought to be due to not only random error but also between-study variability in results. Therefore, weight does not decrease greatly for studies with a small number of patients. Among methods for weighted estimation in a random-effect model, the DerSimonian and Laird method 6) is mostly used for dichotomous variables, as the simplest method, while inverse variance-weighted estimation is used for continuous variables, as with fixed-effect models. These four methods are all used in Review Manager software (The Cochrane Collaboration, UK), and are described in a study by Deeks et al. [ 31 ] ( Table 2 ). However, when the number of studies included in the analysis is less than 10, the Hartung-Knapp-Sidik-Jonkman method 7) can better reduce the risk of type 1 error than does the DerSimonian and Laird method [ 32 ].
Fig. 3 shows the results of analyzing outcome data using a fixed-effect model (A) and a random-effect model (B). As shown in Fig. 3 , while the results from large studies are weighted more heavily in the fixed-effect model, studies are given relatively similar weights irrespective of study size in the random-effect model. Although identical data were being analyzed, as shown in Fig. 3 , the significant result in the fixed-effect model was no longer significant in the random-effect model. One representative example of the small study effect in a random-effect model is the meta-analysis by Li et al. [ 33 ]. In a large-scale study, intravenous injection of magnesium was unrelated to acute myocardial infarction, but in the random-effect model, which included numerous small studies, the small study effect resulted in an association being found between intravenous injection of magnesium and myocardial infarction. This small study effect can be controlled for by using a sensitivity analysis, which is performed to examine the contribution of each of the included studies to the final meta-analysis result. In particular, when heterogeneity is suspected in the study methods or results, by changing certain data or analytical methods, this method makes it possible to verify whether the changes affect the robustness of the results, and to examine the causes of such effects [ 34 ].
Heterogeneity
Homogeneity test is a method whether the degree of heterogeneity is greater than would be expected to occur naturally when the effect size calculated from several studies is higher than the sampling error. This makes it possible to test whether the effect size calculated from several studies is the same. Three types of homogeneity tests can be used: 1) forest plot, 2) Cochrane’s Q test (chi-squared), and 3) Higgins I 2 statistics. In the forest plot, as shown in Fig. 4 , greater overlap between the confidence intervals indicates greater homogeneity. For the Q statistic, when the P value of the chi-squared test, calculated from the forest plot in Fig. 4 , is less than 0.1, it is considered to show statistical heterogeneity and a random-effect can be used. Finally, I 2 can be used [ 35 ].
I 2 , calculated as shown above, returns a value between 0 and 100%. A value less than 25% is considered to show strong homogeneity, a value of 50% is average, and a value greater than 75% indicates strong heterogeneity.
Even when the data cannot be shown to be homogeneous, a fixed-effect model can be used, ignoring the heterogeneity, and all the study results can be presented individually, without combining them. However, in many cases, a random-effect model is applied, as described above, and a subgroup analysis or meta-regression analysis is performed to explain the heterogeneity. In a subgroup analysis, the data are divided into subgroups that are expected to be homogeneous, and these subgroups are analyzed. This needs to be planned in the predetermined protocol before starting the meta-analysis. A meta-regression analysis is similar to a normal regression analysis, except that the heterogeneity between studies is modeled. This process involves performing a regression analysis of the pooled estimate for covariance at the study level, and so it is usually not considered when the number of studies is less than 10. Here, univariate and multivariate regression analyses can both be considered.
Publication bias
Publication bias is the most common type of reporting bias in meta-analyses. This refers to the distortion of meta-analysis outcomes due to the higher likelihood of publication of statistically significant studies rather than non-significant studies. In order to test the presence or absence of publication bias, first, a funnel plot can be used ( Fig. 5 ). Studies are plotted on a scatter plot with effect size on the x-axis and precision or total sample size on the y-axis. If the points form an upside-down funnel shape, with a broad base that narrows towards the top of the plot, this indicates the absence of a publication bias ( Fig. 5A ) [ 29 , 36 ]. On the other hand, if the plot shows an asymmetric shape, with no points on one side of the graph, then publication bias can be suspected ( Fig. 5B ). Second, to test publication bias statistically, Begg and Mazumdar’s rank correlation test 8) [ 37 ] or Egger’s test 9) [ 29 ] can be used. If publication bias is detected, the trim-and-fill method 10) can be used to correct the bias [ 38 ]. Fig. 6 displays results that show publication bias in Egger’s test, which has then been corrected using the trim-and-fill method using Comprehensive Meta-Analysis software (Biostat, USA).

Funnel plot showing the effect size on the x-axis and sample size on the y-axis as a scatter plot. (A) Funnel plot without publication bias. The individual plots are broader at the bottom and narrower at the top. (B) Funnel plot with publication bias. The individual plots are located asymmetrically.

Funnel plot adjusted using the trim-and-fill method. White circles: comparisons included. Black circles: inputted comparisons using the trim-and-fill method. White diamond: pooled observed log risk ratio. Black diamond: pooled inputted log risk ratio.
Result Presentation
When reporting the results of a systematic review or meta-analysis, the analytical content and methods should be described in detail. First, a flowchart is displayed with the literature search and selection process according to the inclusion/exclusion criteria. Second, a table is shown with the characteristics of the included studies. A table should also be included with information related to the quality of evidence, such as GRADE ( Table 4 ). Third, the results of data analysis are shown in a forest plot and funnel plot. Fourth, if the results use dichotomous data, the NNT values can be reported, as described above.
The GRADE Evidence Quality for Each Outcome
N: number of studies, ROB: risk of bias, PON: postoperative nausea, POV: postoperative vomiting, PONV: postoperative nausea and vomiting, CI: confidence interval, RR: risk ratio, AR: absolute risk.
When Review Manager software (The Cochrane Collaboration, UK) is used for the analysis, two types of P values are given. The first is the P value from the z-test, which tests the null hypothesis that the intervention has no effect. The second P value is from the chi-squared test, which tests the null hypothesis for a lack of heterogeneity. The statistical result for the intervention effect, which is generally considered the most important result in meta-analyses, is the z-test P value.
A common mistake when reporting results is, given a z-test P value greater than 0.05, to say there was “no statistical significance” or “no difference.” When evaluating statistical significance in a meta-analysis, a P value lower than 0.05 can be explained as “a significant difference in the effects of the two treatment methods.” However, the P value may appear non-significant whether or not there is a difference between the two treatment methods. In such a situation, it is better to announce “there was no strong evidence for an effect,” and to present the P value and confidence intervals. Another common mistake is to think that a smaller P value is indicative of a more significant effect. In meta-analyses of large-scale studies, the P value is more greatly affected by the number of studies and patients included, rather than by the significance of the results; therefore, care should be taken when interpreting the results of a meta-analysis.
When performing a systematic literature review or meta-analysis, if the quality of studies is not properly evaluated or if proper methodology is not strictly applied, the results can be biased and the outcomes can be incorrect. However, when systematic reviews and meta-analyses are properly implemented, they can yield powerful results that could usually only be achieved using large-scale RCTs, which are difficult to perform in individual studies. As our understanding of evidence-based medicine increases and its importance is better appreciated, the number of systematic reviews and meta-analyses will keep increasing. However, indiscriminate acceptance of the results of all these meta-analyses can be dangerous, and hence, we recommend that their results be received critically on the basis of a more accurate understanding.
1) http://www.ohri.ca .
2) http://methods.cochrane.org/bias/assessing-risk-bias-included-studies .
3) The inverse variance-weighted estimation method is useful if the number of studies is small with large sample sizes.
4) The Mantel-Haenszel estimation method is useful if the number of studies is large with small sample sizes.
5) The Peto estimation method is useful if the event rate is low or one of the two groups shows zero incidence.
6) The most popular and simplest statistical method used in Review Manager and Comprehensive Meta-analysis software.
7) Alternative random-effect model meta-analysis that has more adequate error rates than does the common DerSimonian and Laird method, especially when the number of studies is small. However, even with the Hartung-Knapp-Sidik-Jonkman method, when there are less than five studies with very unequal sizes, extra caution is needed.
8) The Begg and Mazumdar rank correlation test uses the correlation between the ranks of effect sizes and the ranks of their variances [ 37 ].
9) The degree of funnel plot asymmetry as measured by the intercept from the regression of standard normal deviates against precision [ 29 ].
10) If there are more small studies on one side, we expect the suppression of studies on the other side. Trimming yields the adjusted effect size and reduces the variance of the effects by adding the original studies back into the analysis as a mirror image of each study.
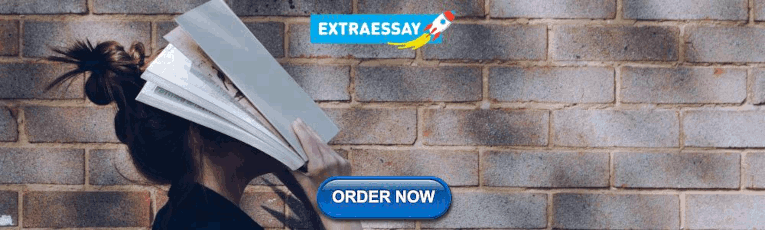
IMAGES
VIDEO
COMMENTS
Table of contents. Step 1: Write your hypotheses and plan your research design. Step 2: Collect data from a sample. Step 3: Summarize your data with descriptive statistics. Step 4: Test hypotheses or make estimates with inferential statistics.
1 Introduction. Statistics is a set of methods used to analyze data. The statistic is present in all areas of science involving the. collection, handling and sorting of data, given the insight of ...
Introduction. Statistical analysis is necessary for any research project seeking to make quantitative conclusions. The following is a primer for research-based statistical analysis. It is intended to be a high-level overview of appropriate statistical testing, while not diving too deep into any specific methodology.
Statistical analysis is fundamental if you need to reveal patterns or identify trends in datasets, employing numerical data analysis to eliminate bias and extract meaningful vision. Accordingly, it is crucial in research explanation, model evolution, and survey planning. Statistical analysts make valuable results from the raw data, facilitating ...
Statistical methods involved in carrying out a study include planning, designing, collecting data, analysing, drawing meaningful interpretation and reporting of the research findings. The statistical analysis gives meaning to the meaningless numbers, thereby breathing life into a lifeless data. The results and inferences are precise only if ...
Overview. Statistical Papers is a forum for presentation and critical assessment of statistical methods encouraging the discussion of methodological foundations and potential applications. The Journal stresses statistical methods that have broad applications, giving special attention to those relevant to the economic and social sciences.
Provides well-organized coverage of statistical analysis and applications in biology, kinesiology, and physical anthropology with comprehensive insights into the techniques and interpretations of R, SPSS®, Excel®, and Numbers® output An Introduction to Statistical Analysis in Research: With Applications in the Biological and Life Sciences develops a conceptual foundation in statistical ...
In many fields, a statistical analysis forms the heart of both the methods and results sections of a manuscript. Learn how to report statistical analyses, and what other context is important for publication success and future reproducibility. A matter of principle. First and foremost, the statistical methods employed in research must always be:
Type and distribution of the data used. For the same objective, selection of the statistical test is varying as per data types. For the nominal, ordinal, discrete data, we use nonparametric methods while for continuous data, parametric methods as well as nonparametric methods are used.[] For example, in the regression analysis, when our outcome variable is categorical, logistic regression ...
This article is a practical guide to conducting data analysis in general literature reviews. The general literature review is a synthesis and analysis of published research on a relevant clinical issue, and is a common format for academic theses at the bachelor's and master's levels in nursing, physiotherapy, occupational therapy, public health and other related fields.
The knowledge of statistics is usually quite limited among researchers representing the field of life sciences, particularly when it comes to constraints imposed on the use of statistical tools and possible interpretations. A common mistake is that researchers take for granted the ability to perform a valid statistical analysis.
Measuring variables. When planning a research design, you should operationalise your variables and decide exactly how you will measure them.. For statistical analysis, it's important to consider the level of measurement of your variables, which tells you what kind of data they contain:. Categorical data represents groupings. These may be nominal (e.g., gender) or ordinal (e.g. level of ...
A Simplified Definition. Statistical analysis uses quantitative data to investigate patterns, relationships, and patterns to understand real-life and simulated phenomena. The approach is a key analytical tool in various fields, including academia, business, government, and science in general. This statistical analysis in research definition ...
Study Design and Statistical Analysis A Practical Guide for Clinicians This book takes the reader through the entire research process: choosing a question, designing a study, collecting the data, using univariate, bivariate and multivariable analysis, and publishing the results. It does so by using plain language rather than complex
12. Summary. As you read papers also notice the construction of the papers (learn from the good and bad examples). Abstract and Introduction { keys for getting readers engaged. Be gentle with your audience. Tell them your story. Writing is work { but ultimately rewarding! 13. Created Date.
The choice of statistical test used for analysis of data from a research study is crucial in interpreting the results of the study. This article gives an overview of the various factors that determine the selection of a statistical test and lists some statistical testsused in common practice. How to cite this article: Ranganathan P. An ...
for statistical data analysis without additional costs. With the help of the R system for statistical computing, research really becomes reproducible when both the data and the results of all data analysis steps reported in a paper are available to the readers through an Rtranscript file. Ris most widely used for
This paper addresses the increasing challenges of identifying novel psychoactive substances based on visual evaluation of spectra in gas chromatography-mass spectrometry; it lays out a summary of the research project, including major goals and objectives, research design, methods, and data analysis, outcomes, limitations, artifacts, references cited, and five appendices.
Results. Although biostatistical inputs are critical for the entire research study (online supplemental table 2), biostatistical consultations were mostly used for statistical analyses only 15.Even though the conduct of statistical analysis mismatched with the study objective and DGP was identified as the major problem in articles submitted to high-impact medical journals. 16 In addition ...
AI Index Report. The AI Index Report tracks, collates, distills, and visualizes data related to artificial intelligence. Our mission is to provide unbiased, rigorously vetted, broadly sourced data in order for policymakers, researchers, executives, journalists, and the general public to develop a more thorough and nuanced understanding of the ...
In our analysis, only 13.4% of the trials exhibited a negative effect size, while 37.1% of the trials indicated a positive effect size. Therefore, our results support the notion that both Gadgil and priming effects can occur in nature, but their influences on decomposition are highly context-dependent (Fernandez & Kennedy, 2016 ; Smith & Wan ...
It is easy to confuse systematic reviews and meta-analyses. A systematic review is an objective, reproducible method to find answers to a certain research question, by collecting all available studies related to that question and reviewing and analyzing their results. A meta-analysis differs from a systematic review in that it uses statistical ...