Computational Social Science

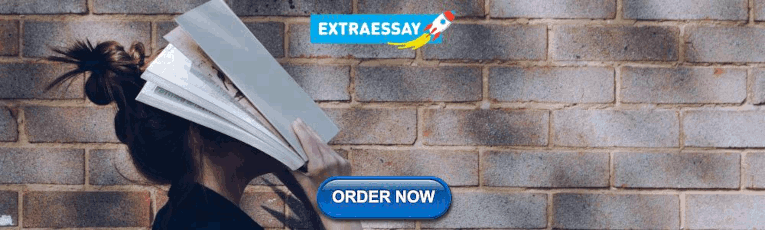
Using data science to understand changes in human interactions
The Center supports research in the overlapping areas of the social sciences and computer science.
The field of computational social science exists because advances in technology have generated an unprecedented volume of digital data that can be used to research traditional social science topics like democracy, security, economic growth, and inequality. Computational methods include:
- Data mining
- Natural language processing
- Text analysis
- Web scraping
- Data visualization
- Machine learning
Sign up to get funding and event announcements
For Stanford affiliates only.
Recent News
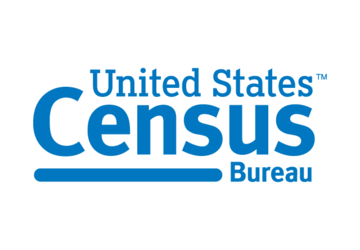
Stanford IRiSS opens expanded Federal Statistical Research Data Center
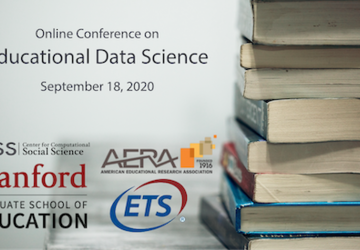
Conference on Educational Data Science provides researchers and practitioners lasting resources
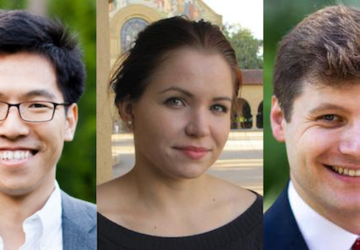
CSS Fellowships yield cross-disciplinary insights
See More CSS News
CSS Fellowships

The IRiSS Center for Computational Social Science runs a competitive grant proposal program that awards five Computational Social Science Fellowships per year. The funding assists graduate students conducting computational research to acquire data, pay RAs to process and validate data, and other research activities.
The 2019-2020 CSS Fellows conducted innovate computational research that pushed disciplinary boundaries and yielded a trove of rich insights. The research reports provided by the fellows describe their groundbreaking findings and the impact of the fellowships on their research. Read the 2019-2020 research reports .
2023-2024 General Catalog

Computational Social Science, Designated Emphasis College of Letters & Science
Office & Contact Information
Martin Hilbert, Ph.D., Chairperson of the Program
Program Office
Communication; 370 Kerr Hall; 530-752-3464; Computational Social Science ; Faculty
Graduate Study
Computational social science provides scholars with the tools to deepen the understanding of long–standing questions in the social sciences, as well as explore new ones. The DE in Computational Social Science is both interdisciplinary in terms of requiring foundations in computational methods, data analysis, and social science theory, and it is multidisciplinary in terms of blurring the traditional boundaries between disciplines in the social sciences. This DE allows students with computational and mathematical skills to deepen their understanding of social science theory and to study unanswered social science research questions; and it allows students from the social sciences to improve their analytical skills in areas like big data analysis, computer simulations, network analysis and machine learning.
Graduate Advisor
Consult Communication Program office.
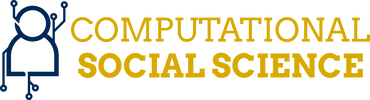
Designated Emphasis CSS
Since Fall 2019, the DE in Computational Social Science has been offered to PhD students from the following associated programs: Animal Biology; Anthropology; Communication; Computer Science; Ecology; Economics; Education; Human Development; Geography; Linguistics; Nursing Science and Health-Care Leadership; Political Science; Public Health Sciences; Psychology; Sociology. Completion of the requirements for the DE and an affiliated Ph.D. are reflected in the notation on the Doctoral Diploma: “ Ph.D. in X with Emphasis in Computational Social Science ”.
Computational Social Science is about developing and applying computational tools to deepen our understanding of long–standing questions in the social sciences, as well as exploring new ones. As suggested by the term itself, the approach is per definition interdisciplinary in terms of requiring foundations in computational methods and social science theory, and it is multidisciplinary in terms of blurring the traditional boundaries between disciplines in the social sciences. Some 30 faculty members from 10 Departments of UC Davis are part of the DE.
More on CSS People Requirements
The DE CSS allows students with computational and mathematical skills to deepen their understanding of social science theory and it allows students from the social sciences to improve their analytical skills in areas like big data analysis, computer simulations, network analysis, and machine learning. The DE requires at least 4 courses, consisting of one required course and 3 electives, with one elective required from each of the following 3 different categories:
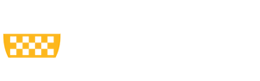
- 2022 Update
- 2021 Update
- 2020 Update
- Reputation and History
- Departments and Programs
- Faculty Recruiting
- Zoom Backgrounds
- Board of Visitors
- SCI Learning Academy
- Administration
- Faculty Directory
- Staff Directory
- PhD Students
- Diversity, Equity and Inclusion at SCI
- Diversity, Equity and Inclusion Committee
- School Initiatives and Resources
- University Initiatives and Resources
- Carving the path to safer and smarter buildings
- A holistic approach to intelligent social learning
- How to anticipate hiccups in health care
- Preserving a shared digital memory
- Holding information technologies accountable and addressing misinformation on the web
- More than an afterthought: Dr. Ibrahim shows students the necessity of cybersecurity
- Current Grants
- Faculty Accepting Undergraduate Students for Research
- Submit Research for Undergraduate Students
- Labs, Centers, and Institutes
- Visiting Scholars
- Undergraduate Research Scholars
- Degrees and Programs
- Find the Right Major for You
- Computational Biology
Computational Social Science
- Computer Science
- Data Science
- Digital Narrative and Interactive Design
- Information Science
- BS + MS in Computer Science
- Physics and Quantum Computing
- Library and Information Science
- Intelligent Systems
- Telecommunications
- Computational Modeling and Simulation
- Information Science with a focus in Telecommunications
- Applied Data Driven Methods
- Big Data Analytics
- Cybersecurity, Policy, and Law
- Information and Network Security
- Professional Institute
- Types of Opportunities
- Experiential Learning Courses
- Meet Alexa Spaventa
- Meet J. Stephanie Rose
- Meet Lydon Pelletier
- Meet Pedro Bustamante
- Meet Nico Campuzano
- Meet Andrea Michael
- Meet Kinori Rosnow
- Take the Next Step
- Undergraduate Admissions FAQ
- Master's Admissions
- Doctoral Admissions
- Certificate Admissions
- GRE Requirements
- Financial Aid
- Scholarships
- Campus Life
- Information Sessions
- A-Z Student Resources
- Responsibilities
- Placement Assessments
- General Education Requirements
- Major and Minor Declaration
- Faculty Mentors
- Contact the SCI Advising Center
- Building Hours
- Career Resources
- Post-Graduate Outcomes
- Enrollment Resources
- Graduation Process and Expectations
- Apply for Graduation
- School Recognition Ceremony
- Information Technology
- Graduate Student Orientation
- New Graduate Student FAQ
- Undergraduate Student Orientation
- Ombudsperson
- Academic Integrity Policy
- Experiential Learning Policies
- School Forms
- Student Appeals
- Student Organizations
- Academic Support and Tutoring
- Student Success Workshops
- Who to Contact
- Submit a News Item
- Event Assistance & Promotion
- Undergraduate Majors
The Computational Social Science (CSS) major educates students to build, compute, and improve theoretically informed models of social processes, bridging domain and technical expertise. This major will prepare students to understand, engage with, and innovatively solve evolving, complex multi-scale challenges such as climate change, transnational political violence, cybersecurity and privacy, social polarization, and inequality. This major will draw on and enhance Pitt’s strength in both social science theory, broadly construed, as well as computing, informatics, and networked systems. Students will gain an understanding of modern computational tools and resources, and social, political, and economic concepts from core social science classes.
Computational Social Science Major Requirements
The major will comprise of the following requirements for a total of 52-53 credits.
Pre-Requisite Mathematics Class
- MATH 0220 - ANALYTIC GEOMETRY AND CALCULUS 1
Foundations of Computational Social Science
The foundational courses introduce students to enduring puzzles in social science research, emerging themes of computational social science, and the approaches that social scientists and information & network scientists use to solve problems. This section consists of eight required courses that will provide foundations of subject matter knowledge and the basic computational tools that are relevant to social scientific theories and empirics.
One Introduction to CSS Class
- PS 0702 - INTRODUCTION TO COMPUTATIONAL SOCIAL SCIENCE OR
- INFSCI 0702 - INTRODUCTION TO COMPUTATIONAL SOCIAL SCIENCE
One Introduction to Social Science Class
Choose one class from this list:
- PS 0200 - AMERICAN POLITICS
- PS 0500 - INTERNATIONAL RELATIONS
- PS 0300 - COMPARATIVE POLITICS
Four Classes Introducing Computational Approaches and Basic Tools
Two required courses:
- CMPINF 0401 - INTERMEDIATE PROGRAMMING *
- INFSCI 0510 - DATA ANALYSIS
And then, select two of the following courses:
- INFSCI 0410 - HUMAN-CENTERED SYSTEMS
- INFSCI 0610 - NETWORKS AND INFORMATION
- INFSCI 1500 - DATABASE MANAGEMENT CONCEPTS AND APPLICATIONS
*Students should have some programming experience (usually acquired in high school) before taking CMPINF 0401 . Any high school course that includes the writing of several Python, C++, or Java programs would be sufficient. It is also possible to take one of CS 0010, CS 0011, or CS 0012 as preparation. Preparatory classes of this nature do not count toward the student's major requirements.
One Social Science Research Design Class
- PS 0700 - RESEARCH METHODS IN POLITICAL SCIENCE
One Modeling Social Interactions and Motivations Class
Select one of the following courses.
- PS 1250 - GAMES, POLITICS, AND STRATEGY
- PS 1514 - POLITICAL STRATEGY INTERNATIONAL RELATIONS
Ethics and Computational Social Science
This section of the major will expose students to critical debates on the ethics of governance, computing, and technological change. Issues covered will include tradeoffs between privacy/security and censorship/freedom, as well as topics related to surveillance, propaganda, cyber-security, and regulation. The goal is to expose students to both the moral and social consequences of technology at a conceptual level, and the specific technical implementations that cause potential social problems (e.g., packet-sniffing) and could potentially expand the space for solutions (e.g., differential privacy).
Select two courses from the following:
- CMPINF 1205 - COMPARATIVE DIGITAL PRIVACIES
- CS 0590 - SOCIAL IMPLICATIONS OF COMPUTING TECHNOLOGY
- INFSCI 1600 - SECURITY AND PRIVACY
- PS 1693 - POLITICAL THEORY & THE FUTURE-ANALYSIS
- INFSCI 1049 - INTRODUCTION TO INFORMATION SECURITY
- PS 1675 - POLITICS OF HUMAN RIGHTS
Intermediate Techniques Applied to Social Science Content
The third set of requirements will empower students to use computational tools to explore enduring social science puzzles and theories at scale. These paths are not meant to be formal areas of concentration, but options to gain competence in more focused areas. For example, some students might be interested in applying data mining techniques to problems in campaigns in American politics. Other students might want to focus on cyber-security and international relations. The computational techniques, domains, and analytics course sets are organized to broaden interest in the major. Together, these courses allow our students to have an evolved understanding of how computing and digital tools can be used in government, businesses, and NGOs.
Two Computational Skills Classes
These classes specifically focus on relevant computational skills, including data mining, the web, visualizations, social computing, and advanced security and privacy.
Select two of the following courses to satisfy this subset:
- INFSCI 1440 - SOCIAL COMPUTING
- INFSCI 1520 - INFORMATION VISUALIZATION
- INFSCI 1530 - DATA MINING
- INFSCI 1550 - SPATIAL INFORMATION
- INFSCI 1570 - NETWORK AND WEB DATA TECHNOLOGIES
- INFSCI 1620 - ADVANCED SECURITY AND PRIVACY
Two Domain Specialization Classes
Students will delve deeper into their domain specialization with two classes. One class should pair with the theme chosen for the student's Introductory Social Science Class (under Foundations of CSS). Students then choose another substantive class that can be outside the previous theme. Select two domain specializations classes from the themes below. Although only a few classes are listed for each theme, more are options available to students. Students should refer to the major's academic advisement (degree progress) report for a complete list of course options.
American Politics: A subset of courses within the 1200-range are approved domain specialization courses to pair with PS 0200 .
- PS 1201 - CONSTITUTION AND CIVIL LIBERTIES
- PS 1202 - AMERICAN CONSTITUTIONAL LAW
- PS 1203 - JUDICIAL POLITICS
- PS 1204 - WOMEN IN POLITICS
- PS 1211 - LEGISLATIVE PROCESS
- PS 1213 - LAW AND POLITICS
- PS 1232 - POLITICAL ATTITUDE & PUBLIC OPIN ION
Comparative Politics: A subset of courses offered within the 1300-range are approved domain specialization courses to pair with PS 0300 .
- PS 1302 - POLITICAL DEVELOPMENT
- PS 1303 - PEACE MOVEMENTS AND PEACE EDUCATION
- PS 1311 - WESTERN EUROPEAN GOVERNMENT AND POLITICS
- PS 1316 - ASIAN POLITICS
- PS 1317 - POLITICS OF THE EUROPEAN UNION
- PS 1322 - LATIN AMERICAN POLITICAL DEVELOPMENT
- PS 1327 - POLITICS OF REVOLUTION
International Relations: A subset of courses offered within the 1500-range are approved domain specialization courses to pair with PS 0500 .
- PS 1501 - THEORY OF INTERNATIONAL RELATIONS
- PS 1502 - INTERNATIONAL LAW AND PROBLEMS OF WORLD ORDER
- PS 1503 - INTERNATIONAL ORGANIZATION
- PS 1504 - NATIONALISM
- PS 1511 - AMERICAN FOREIGN POLICY
- PS 1512 - THE POLITICS OF MIGRATION
- PS 1513 - FOREIGN POLICIES--CHANGING WORLD
One Integrated Analytics Content Class
The final section in this requirement will marry a deep analytical component, such as predictive analytics, causal inference, game theory, data visualization, and other topics with complicated social problems such as inequality, trade, climate change, political violence, or polarization. The class will focus on integrating computational tools into the measurement of core social science concepts, including democracy, human rights, happiness, and peace. There will be a focus on using text and images as data.
Select one of the following courses:
- PS 1291 - WOMEN IN POLITICS - ANALYSIS
- PS 1292 - RACE, GENDER, AND POLITICS - ANALYSIS
- PS 1293 - LEGISLATIVE PROCESS - ANALYSIS
- PS 1294 - POLITICAL ATTITUDE - ANALYSIS
- PS 1295 - POLITICAL PSYCHOLOGY - ANALYSIS
- PS 1299 - ANALYSIS OF AMERICAN POLITICS
- PS 1391 - AUTHORITARIAN STATECRAFT AND THE ARTS OF RESISTANCE - ANALYSIS
- PS 1392 - EUROPEAN POLITICS-ANALYSIS IN COMPARATIVE POLITICS
- PS 1395 - LATIN AMERICAN POLITICS-ANALYSIS
- PS 1399 - ANALYSIS OF COMPARATIVE POLITICS
- PS 1591 - ANALYSIS OF CIVIL WARS
- PS 1592 - POLITICS OF GLOBAL ECONOMIC RELATIONS - ANALYSIS
- PS 1599 - ANALYSIS OF INTERNATIONAL RELATIONS
- PS 1702 - VISUALIZING AND UNDERSTANDING SOCIAL DATA
Application Development Capstone
The major culminates in the production of a research project that uses computational tools to create either a) an online, interactive data visualization, b) a replicable research report that uses unstructured data or c) a module/library. In all cases, the project will engage with or help to resolve an important social problem. This project can be created through independent or directed research, or in one of the classes listed below. All students will present their projects as digital posters to faculty, alumni, and potential employers from around the Pittsburgh area at an end-of-the-year event.
- INFSCI 1700 - DATA-DRIVEN COMMUNICATION
- INFSCI 1710 - DIRECTED RESEARCH
- INFSCI 1720 - INTERNSHIP
- INFSCI 1730 - INDEPENDENT STUDY
- INFSCI 1740 - TEAM-BASED CAPSTONE PROJECT
- PS 1782 - APPLICATION IN COMPUTATIONAL SOCIAL SCIENCE
- PS 1900 - INTERNSHIP
- PS 1901 - INDEPENDENT STUDY
- PS 1903 - DIRECTED RESEARCH
For full major requirement details, visit the Computational Social Science course catalog .
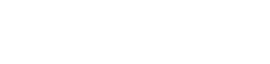
- Message from the Chair
- Reflections on Disinformation, Democracy, and Free Expression
- Tech / Law Colloquium
- Graduation Info
- Artificial Moral Behavior
- Stop Predicting? Machine Learning and Measurement
- Towards a Theory of Proxies (for Race)
- List of Info Sci Courses
- Enrollment/Waitlist
Computational Social Science
- Critical Data Studies
- Data Science
- Economics and Information
- Education Technology
- Ethics, Law and Policy
- Human-Computer Interaction
- Human-Robot Interaction
- Incentives and Computation
- Infrastructure Studies
- Interface Design and Ubiquitous Computing
- Natural Language Processing
- Network Science
- Social Computing and Computer-supported Cooperative Work
- Technology and Equity
- Research Staff
- Student Services Assistant
- Lead Chair’s Assistant
- Tenure-track and Tenured Faculty – Ithaca
- Tenure-track and Tenured Faculty - Cornell Tech
- Lecturer or Senior Lecturer Positions – Ithaca
- Ugrad Course Staff
- Resources for Students
- For Employers
- Admission Requirements
- Core Courses
- Programming and Math Requirements
- Behavioral Science
- Digital Culture and Production
- Information Ethics, Law, and Policy
- Interactive Technologies
- Networks, Crowds, and Markets
- UX (User Experience) Design
- Criteria for Good Standing
- Honors Requirements
- Admission to the Major
- Core Requirements
- Math Requirements
- Major Approved Electives
- MPS Early Credit Option
- Independent Research
- CPT Procedures
- Cornell Design & Tech Initiative
- Cornell Data Science
- Undergraduate Minor in Info Sci
- Our Students and Alumni
- How to Apply
- Admissions FAQs
- Scholarship
- Teaching Assistants
- Early MPS Credit Option
- Degree Requirements
- Focus Areas
- Recent MPS Projects
- Digital Tech Immersion
- Conference Travel Grant
- Third Semester Extension
- Student Spotlights
- Career Success
- Program Contacts and Student Advising
- Connective Media - Cornell Tech
- Health Tech - Cornell Tech
- Prospective PhD Students
- Ph.D. Admissions FAQs
- Ph.D. Visit Day
- Graduate Housing
- Degree Requirements and Curriculum
- Statistics Graduate Society
- Computer Science Graduate Organization
- Graduate School Requirements
- Graduate School Resources
- Ph.D. Field Requirements
- Field Specific Items, Helpful Tips, and Resources
- Conference and Travel Grants
- Outside Fellowship Opportunities
- Inter-Campus Travel Fund
- Graduate Minor
- Human Participant Compensation
- Travel Planning and Reimbursement Process
- Purchasing Goods for Research for PhDs
- Diversity and Inclusion
Search form

Related News
- Info Sci Ph.D. Student Named 2023 Quad Fellow
- Koenecke and Garg selected for Forbes 30 Under 30: Science
- New recommender system provides fairer search results
- Inaugural Grants Announced from Strategic Partnership with LinkedIn
- Gender Bias Not a Factor in Physics Recommendations Letters
Related Activities
Cornell Social Media Lab Social Technologies Lab
Growing use of the Internet and social media in the past decade has led to an explosion in the amount of social and behavioral data available to researchers. This in turn has created huge opportunities for social scientists to study human behavior and social interaction in unprecedented detail.
Leveraging these opportunities requires collaborative, interdisciplinary efforts involving computer and information scientists, physicists, and mathematicians who know how to build the "telescope" and economists, political scientists, and sociologists who know where to aim it.
Computational social science exists at the intersection of these varied disciplines.
Contributing Faculty and Researchers
- Larry Blume
- Cristobal Cheyre
- Morten Christiansen
- Cristian Danescu-Niculescu-Mizil
- David Easley
- Nikhil Garg
- Joseph Halpern
- René Kizilcec
- Jon Kleinberg
- Allison Koenecke
- Lillian Lee
- Ian Lundberg
- Michael Macy
- J. Nathan Matias
- David Mimno
- Benjamin Soltoff
- Matthew Wilkens

Academic Programs
- CSE PhD Overview
- Dept-CSE PhD Overview
- CSE Doctoral Theses
- Program Overview and Curriculum
- For New CCSE Students
- Terms of Reference
MIT Doctoral Programs in Computational Science and Engineering
The Center for Computational Science and Engineering (CCSE) offers two doctoral programs in computational science and engineering (CSE) – one leading to a standalone PhD degree in CSE offered entirely by CCSE (CSE PhD) and the other leading to an interdisciplinary PhD degree offered jointly with participating departments in the School of Engineering and the School of Science (Dept-CSE PhD).
While both programs enable students to specialize at the doctoral level in a computation-related field via focused coursework and a thesis, they differ in essential ways. The standalone CSE PhD program is intended for students who intend to pursue research in cross-cutting methodological aspects of computational science. The resulting doctoral degree in Computational Science and Engineering is awarded by CCSE via the the Schwarzman College of Computing. In contrast, the interdisciplinary CSE PhD program is intended for students who are interested in computation in the context of a specific engineering or science discipline. For this reason, this degree is offered jointly with participating departments across the Institute; the interdisciplinary degree is awarded in a specially crafted thesis field that recognizes the student’s specialization in computation within the chosen engineering or science discipline.
For more information about CCSE’s doctoral programs, please explore the links on the left. Information about our application and admission process is available via the ‘ Admissions ‘ tab in our menu. MIT Registrar’s Office provides graduate tuition and fee rates as set by the MIT Corporation and the Graduate Admissions section of MIT’s Office of Graduate Education (OGE) website contains additional information about costs of attendance and funding .
- Search This Site All UCSD Sites Faculty/Staff Search Term
- Instructional Assistantships
- Postdoctoral Scholars
- M.S. Students
- Administration
- Prospective Students
- Graduate Courses
- Masters FAQ
- Program Cost
- Capstone Project
Computational Social Science
Welcome to computational social science.
Dynamic pricing of rideshare services, automated tutoring systems, and mitigation strategies for disinformation campaigns have one thing in common: they all require combining formal theories from the social sciences with efficient analytics of internet-scale data. This novel computational social science skillset is increasingly in demand to face modern challenges and develop future solutions in science, government, and business.
UC San Diego offers Computational Social Science programs to train students in this exciting, interdisciplinary field at the undergraduate and graduate levels. Our CSS training combines coursework in formal models from across social science disciplines with modern computational data analysis techniques. Most important of all, the hands-on curriculum provides substantive and varied practice applying these skillsets to real-world problems. This integrative training prepares the next generation of scientists and practitioners to understand the past, explore the present, and build the future.
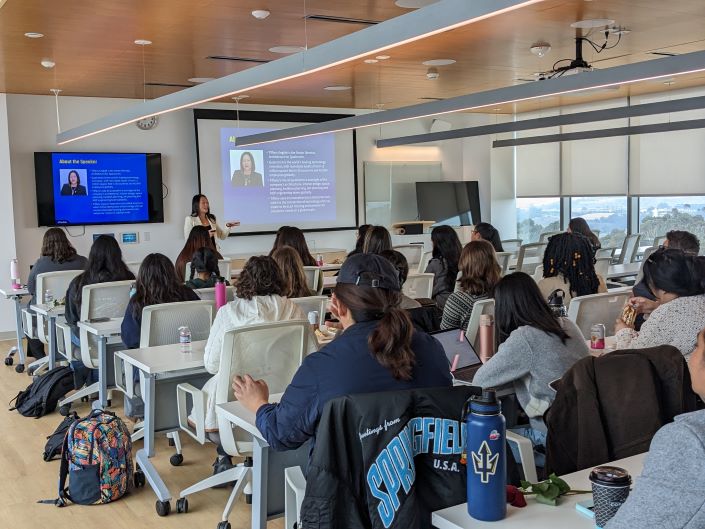
CSS in UC San Diego Today
Code and Community: Computational Social Science Program Addresses Social Questions with Data
Read the Article
Connect With Us
- Departments and Units
- Majors and Minors
- LSA Course Guide
- LSA Gateway
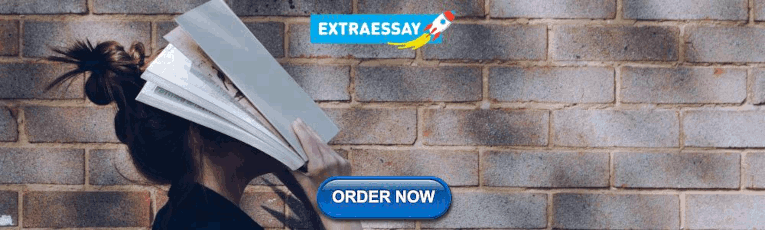
Search: {{$root.lsaSearchQuery.q}}, Page {{$root.page}}
- CSS Initiative
- News and Events
- Undergraduate Students
- Graduate Students
- Alumni and Friends

- Complex Systems Minor
- Featured CSCS Minor Alumni
- Complex Systems Undergrad Courses
- Undergraduate Awards
- Transfer Credit Policies and Procedures
- Complex Systems Graduate Certificate
- Graduate Courses
- Gifts to CSCS
- Featured CSCS Certificate Alumni
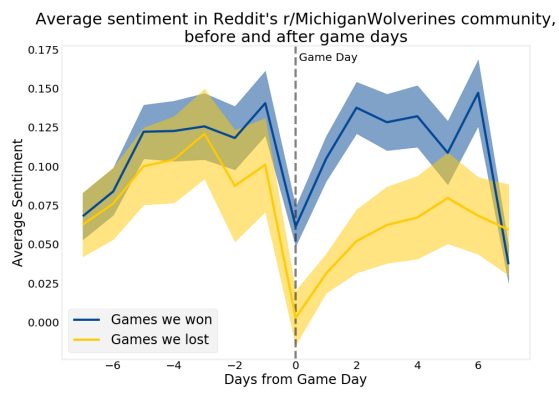
Computational Social Science (CSS) Initiative
The Center for the Study of Complex Systems announced the Computational Social Science (CSS) initiative in the fall of 2017. Under the leadership of core faculty member Elizabeth Bruch, the CSS Initiative aim is to develop new courses in computational social science and to build an interdisciplinary community of Michigan students and faculty who work and collaborate in this area. Vast streams of activity data from electronic sources make it possible to study human behavior with an unparalleled richness of detail. Social scientists can, for the first time, avail themselves of granular, disintermediated data to assemble individual narratives, motivations, and behavioral arcs as people go about living their lives.
The plan is for this community to be able to discuss topics of mutual interest, learn new skills, and create interdisciplinary collaborations. As part of this effort, we are organizing a series of methods workshops designed to provide introductions to important CSS-related models and techniques (see below).
Core faculty member Daniel Romero is co-organizing several initiative activities.
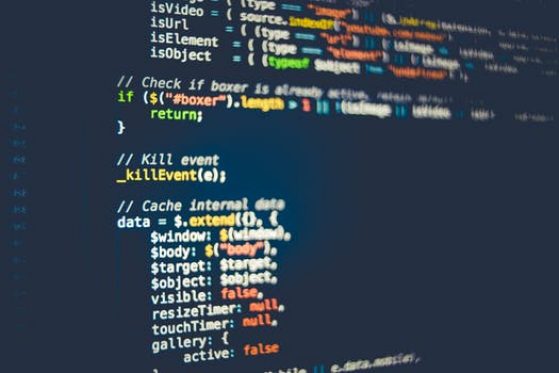
CSS Course Development
The CSS Initiative will develop new courses at both the undergraduate and graduate levels including hands-on labs for social science undergraduates without a strong programming background. These labs will provide an opportunity for undergraduates to collect and analyze online data from places like Reddit and Twitter to study topics such as trolling behavior and its consequences or networks of influence. At the graduate level, the CSS Initiative is developing hands-on course materials on a range of topics including discrete choice modeling, network analysis, Bayesian statistics, and reproducible research.
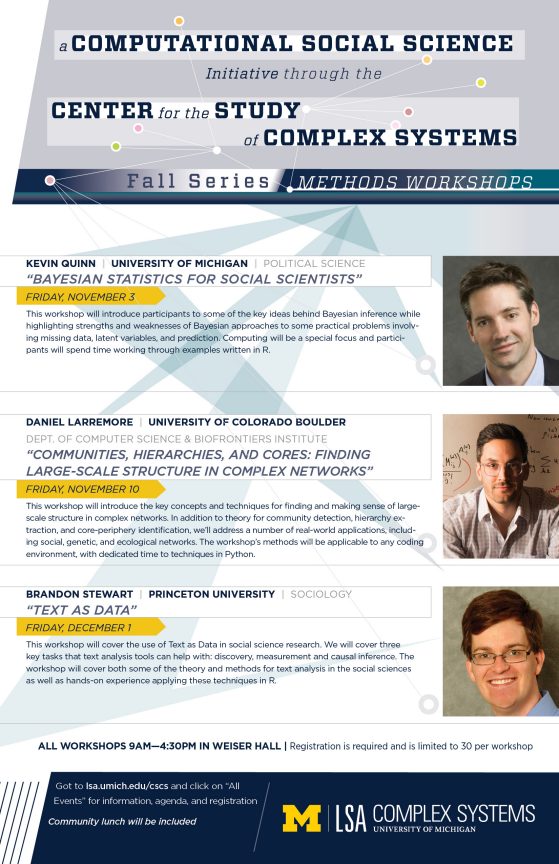
CSS Workshops
To complement course development, a series of one-day technical workshops were offered during the 2017/2018 academic year that introduced students and faculty to quantitative methods for modeling human behavior and social dynamics. The CSS Initiative will also cultivate cross-disciplinary interaction between faculty and students within the College of LSA and throughout the University of Michigan. Planned activities include a computational social science speaker series, a CSS faculty Working Group, a book group to discuss recent publications of interest, and coordinated activities with related groups such as the Michigan Data Science (MIDAS) initiative. For more information, please contact [email protected].
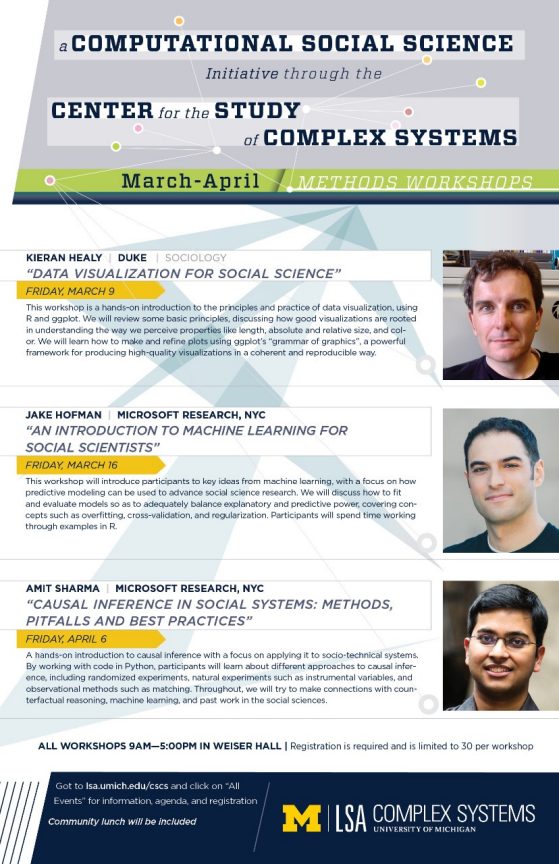
For other CSS sponsored Events and Seminars click "CSS Events" in the left list.

- Information For
- Prospective Students
- Current Students
- Faculty and Staff
- More about LSA
- How Do I Apply?
- LSA Magazine
- Student Resources
- Academic Advising
- Global Studies
- LSA Opportunity Hub
- Social Media
- Update Contact Info
- Privacy Statement
- Report Feedback
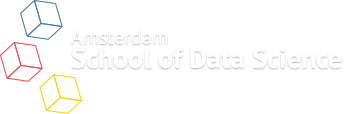
- Courses & Programmes /
Computational Social Science
Use our tool to find your future, courses & programmes.
- z)">name (a > z)
- a)">name (z > a)
- high)">level (low > high)
- low)">level (high > low)
Digitisation poses new questions and challenges for our societies, but it also brings new opportunities to intervene in societal issues such as inequality and climate change. Would you like to learn how to make the world a better place? Scientifically grounded in social sciences and humanities expertise, Computational Social Science students develop skills to design, create, apply and appropriate digital technology and data science to benefit society.

- Chancellor’s Message
Print Options
2023-24 edition, computational science, ph.d..
Lee Swindlehurst, UCI Director 949-824-2818 computationalscience.uci.edu
Joint Doctoral Program with UC Irvine and San Diego State University
A joint offering with San Diego State University (SDSU), the Ph.D. program in Computational Science trains professionals capable of developing novel computational approaches to solve complex problems in both fundamental sciences and applied sciences and engineering. A program of study combining applied mathematics, computing, and a solid training in basic science culminates in doctoral research focused on an unsolved scientific problem.
The Ph.D. in Computational Science produces broadly educated, research-capable scientists that are well prepared for diverse careers in academia, industry, business, and government research laboratories.
Students are admitted into the joint program via a Joint Admissions Committee. Applicants apply to UCI directly using the UCI graduate application.
Applicants are expected to hold a Bachelor’s degree in one of the science, technology, engineering, and mathematics (STEM) fields.
Applicants are evaluated on the basis of their prior academic record and their potential for creative research and teaching, as demonstrated in submitted materials. These materials include official university transcripts, three letters of recommendation, a Statement of Purpose, and a Personal History statement.
Program Requirements
The normative time to completion is five years. The maximum time to completion is seven years. A total minimum of 66 units of course work, independent study, and research must be completed. These units must be distributed as follows:
- Minimum of 18 units of graduate-level coursework as SDSU.
- Minimum of 24 units of graduate-level coursework at UCI.
- Minimum of 24 units of combined research, practicum, and dissertation research units at either institution.
Summer Research Survey
Students are required to attend the annual summer seminar series featuring participating faculty members describing their current research and possible projects.
Course Requirements
Core courses at sdsu.
MATH 636 - Mathematical Modeling OR MATH 638 - Continuous Dynamical Systems and Chaos MATH 693B - Advanced Computational PDEs COMP 605 - Scientific Computing
Elective Courses at SDSU
Students select 9 units from the following list, or appropriate substitutions, with the approval of the program director and their research mentor
AE 601 - Computational Fluid Mechanics AE 641 - Structural Optimization AE 670 - Optimal Control BIOL 606 - Biological Data BIOL 668 - Advanced Biological Data Analysis BIOL 740 - Phylogenetic Systematics BIOMI 608 - Programming Problems in Bioinformatics CHEM 711 - Chemical Thermodynamics CHEM 712 - Chemical Kinetics CHEM 713 - Quantum Chemistry CIVE 620 - Traffic Flow and Control CIVE 697 - Traffic Signals Systems Operations and Control COMP 526 - Computational Methods for Scientists COMP 607 - Computational Database Fundamentals COMP 670 - Seminar: Problems in Computational Science CS 600 - Bioinformatics CS 610 - Computational Genomics CS 653 - Data Mining and Knowledge CS 666 - Advanced Distributed Systems CS 696 - Programming Problems in Bioinformatics EE 645 - Antennas and Wave Propagation EE 657 - Digital Signal Processing EE 658 - Advanced Digital Signal Processing EE 665 - Multimedia Wireless Networks EE 740 - Advanced Topics in Physical Electronics Antenna Design MATH 693A - Advanced Computational Optimization MATH 693B - Advanced Computational PDEs MB 610A-B - Advanced Topics in Molecular Biology ME 610 - Finite Element Methods PHYS 604 - Electricity and Magnetism PHYS 606 - Statistical Mechanics PHYS 608 - Classical Mechanics PHYS 610 - Quantum Mechanics STAT 657 - Statistical and Machine Learning Methods STAT 658 - Advanced Data Analytics STAT 676 - Bayesian Statistics STAT 678 - Survival Analysis STAT 700 - Data Analysis STAT 701 - Monte Carlo Methods STAT 702 - Data Mining
Core Courses at UCI
Elective courses at uci.
Students select 8 units from the following list, or appropriate substitutions, with the approval of the program director and their research mentor
Research Units at SDSU
COMP 897 - Doctoral Research COMP 898 - Practicum COMP 899 - Dissertation
Research Units at UCI
Practicum and doctoral research.
Dissertation research is carried out at either UCI or SDSU, or at an industry or national laboratory under the supervision of the Doctoral Advisor. While conducting dissertation research, students must enroll in the appropriate research units at the campus of the Doctoral Advisor. If research is done outside of UCI or SDSU, students should register in-absentia if appropriate.
Research Report Exam
The student is expected to pass the Research Report Exam within three years of admittance. This examination consists of a term research project supervised by a faculty mentor. The student is required to prepare a written account of research work performed and its results, and offer an oral presentation before the members of the Doctoral Committee. The student must submit a paper based on their research report before giving the oral presentation to the Doctoral Committee. Should a student fail the Research Report Exam, one retake is allowed.
Dissertation Proposal
Students must submit a dissertation proposal to the doctoral committee by the end of their third year in the program. This proposal should take the form of a scientific grant proposal to a major funding agency. It should describe the research project that the student intends to carry out and upon which their doctoral dissertation will be based. The student must also offer an oral presentation of the proposal before the Computational Science faculty. Upon successful completion of this presentation, the student will be recommended for advancement to candidacy for the doctoral degree.
Advancement to Doctoral Candidacy
After successful completion of the dissertation proposal and certification that all other requirements are fulfilled, the student is advanced to candidacy at both campuses. Students not registered at UCI will need to formally advance to candidacy in the summer term. Advancement to candidacy for the Ph.D. must occur at least one term prior to dissertation defense.
Dissertation and Final Oral Examination
On completion of the research, the student prepares the dissertation in accordance with UCI regulations. A final draft of the dissertation is presented to each member of the doctoral committee at least three weeks prior to the final oral examination. The oral defense is held on the campus of the primary faculty advisor. Students must follow UCI filing deadlines. Students are required to be registered for Dissertation Research (3 units) at SDSU and Dissertation Research (4 units) simultaneously at UCI during the semester in which they present their doctoral defense. Alternatively, students can request filing fee status at UCI in the quarter in which they present their doctoral defense.
University of California, Irvine Faculty
Mohammad A. Al Faruque, Ph.D. University of Kaiserslautern, Chair of Emulex Career Development and Associate Professor of Electrical Engineering and Computer Science; Mechanical and Aerospace Engineering (system-level design, embedded systems, cyber-physical-systems, multi-core systems)
Jun F. Allard, Ph.D. University of British Columbia, Assistant Professor of Mathematics; Physics and Astronomy (Mathematical and computational biology, biopolymers, biomembranes, force-sensitive biomolecular bonds)
Ioan Andricioaei, Ph.D. Boston University, Professor of Chemistry (Theoretical Chemistry and Biophysics: Developing novel theoretical techniques and applying computer and modeling methods to describe, in terms of dynamics and thermodynamics, biologically important molecular processes, with the aim to complement, enhance or predict experimental findings.)
Pierre F. Baldi, Ph.D. California Institute of Technology, UCI Chancellor's Professor of Computer Science; Biological Chemistry; Biomedical Engineering; Developmental and Cell Biology (Bioinformatics, computational biology, AI and machine learning with particular emphasis on: Deep Learning, Neural Networks, Reinforcement Learning, and their Theoretical Foundations and Applications)
Kieron Burke, Ph.D. University of California, Santa Barbara, Professor of Chemistry; Physics and Astronomy (Physical chemistry and chemical physics, polymer, materials, nanoscience, theoretical and computational)
Filippo Capolino, Ph.D. University of Florence, Italy, Professor of Electrical and Computer Science (Optics/electromagnetics in nanostructures and sensors, antennas/microwaves, RF and wireless systems)
Ann Marie Carlton, Ph.D. Rutgers University, Associate Professor of Chemistry (Atmospheric chemistry, aerosol liquid water, cloud processing, secondary organic aerosol)
Peter Chang, M.D. Northwestern University, Assistant Professor in Residence of Radiological Sciences; Computer Science; Pathology and Laboratory Medicine
Olivier Cinquin, Ph.D. University College London, Assistant Professor of Developmental and Cell Biology (Mathematical modeling of networks, systems biology)
Donald A. Dabdub, Ph.D. California Institute of Technology, Professor of Mechanical and Aerospace Engineering; Civil and Environmental Engineering (Mathematical modeling of urban and global air pollution, dynamics of atmospheric aerosols, secondary organic aerosols, impact of energy generation on air quality, chemical reactions at gas-liquid interfaces)
Kristen Davis, Ph.D. Stanford University, Assistant Professor of Civil and Environmental Engineering; Earth System Science (Coastal Dynamics)
Franco De Flaviis, Ph.D. University of California, Los Angeles, Professor of Electrical Engineering and Computer Science (microwave systems, wireless communications, electromagnetic circuit simulations)
Russell L. Detwiler, Ph.D. University of Colorado Boulder, Associate Professor of Civil and Environmental Engineering (groundwater hydrology, contaminant fate and transport, subsurface process modeling, groundwater/surface-water interaction)
Efi Foufoula-Georgiou, Ph.D. University of Florida, Distinguished Professor of Civil and Environmental Engineering (hydrology and geomorphology with emphasis on modeling the interactions between the atmosphere, land, and the terrestrial environment at plot to large-watershed scale)
Filipp Furche, Ph.D. University of Karlsruhe, Professor of Chemistry (Physical chemistry and chemical physics, theoretical and computational)
Robert Benny Gerber, Ph.D. University of Oxford, Professor of Chemistry (Vibrational spectroscopy, chemical reaction dynamics, biological molecules, molecular dynamics)
Wayne B. Hayes, Ph.D. University of Toronto, Associate Professor of Computer Science (Biomedical Informatics and Computational Biology, Computer Vision Scientific and Numerical Computing)
Alexander Ihler, Ph.D. Massachusetts Institute of Technology, Associate Professor of Information and Computer Science (Artificial intelligence and machine learning, focusing on statistical methods for learning from data and on approximate inference techniques for graphical models)
Perry Johnson, Ph.D. John Hopkins University, Assistant Professor of Mechanical and Aerospace Engineering (turbulent flows, particle-laden and multiphase flows, turbulent boundary layers, large-eddy simulations, scientific computing)
Frithjof Kruggel, M.D., Ph.D. Ludwig Maximilian University of Munich, Professor of Biomedical Engineering; Electrical Engineering and Computer Science (Biomedical signal and image processing, anatomical and functional neuroimaging in humans, structure-function relationship in the human brain)
Arthur D. Lander, Ph.D. University of California, San Francisco, Donald Bren Professor and Professor of Developmental and Cell Biology; Biomedical Engineering; Logic and Philosophy of Science; Pharmacology (Systems biology of development, pattern formation, growth control)
Marco Levorato, Ph.D. University of Padua, Associate Professor of Computer Science; Electrical Engineering and Computer Science (artificial intelligence and machine learning, networks and distributed systems, statistics and statistical theory, stochastic modeling, signal processing)
Mo Li, Ph.D. University of Michigan, Assistant Professor of Civil and Environmental Engineering (ultra-damage-tolerant and multifunctional composite materials for protective and resilient structures, built environments, and energy infrastructure)
Feng Liu, Ph.D. Princeton University, Professor of Mechanical and Aerospace Engineering (Computational fluid dynamics and combustion, aerodynamics, aeroelasticity, propulsion, turbomachinery aerodynamics and aeromechanics)
John S. Lowengrub, Ph.D. Courant Institute of Mathematical Sciences, UCI Chancellor's Professor of Mathematics; Biomedical Engineering; Chemical Engineering and Materials Science (Applied and computational mathematics, mathematical and computational biology)
Ray Luo, Ph.D. University of Maryland, College Park, Professor of Molecular Biology and Biochemistry; Biomedical Engineering; Chemical Engineering and Materials Science (Protein structure, non-covalent associations involving proteins)
Vladimir A. Mandelshtam, Ph.D. Institute of Spectroscopy, Academy of Sciences of USSR, Professor of Chemistry (Theoretical and Computational Chemistry)
Craig C. Martens, Ph.D. Cornell University, Professor of Chemistry (Theoretical Chemistry, Chemical Physics)
Eric D. Mjolsness, Ph.D. California Institute of Technology, Professor of Computer Science; Mathematics (Applied mathematics, mathematical biology, modeling languages)
David L. Mobley, Ph.D. University of California, Davis, Associate Professor of Pharmaceutical Sciences; Chemistry (Chemical biology, physical chemistry and chemical physics, theoretical and computational)
Mathieu Morlighem, Ph.D. Ecole Centrale Paris, Vice Chair and Associate Professor of Earth System Science
Seyed Ali Mortazavi, Ph.D. California Institute of Technology, Assistant Professor of Developmental and Cell Biology (Functional genomics to study transcriptional regulation in development)
Shaul Mukamel, Ph.D. Tel Aviv University, UCI Distinguished Professor of Chemistry; Physics and Astronomy (Physical chemistry and chemical physics, polymer, materials, nanoscience, theoretical and computational)
Alexandru Nicolau, Ph.D. Yale University, Department Chair and Professor of Computer Science; Electrical Engineering and Computer Science (Architecture, parallel computation, programming languages and compilers)
Qing Nie, Ph.D. Ohio State University, C hancellor's Professor, Developmental & Cell Biology (Computational Biology; Systems Biology; Stem Cells; Regulatory Networks; Stochastic Dynamics; Scientific Computing and Numerical Analysis)
Francois W. Primeau, Ph.D. Massachusetts Institute of Technology, Professor of Earth System Science
Michael S. Pritchard, Ph.D. University of California, San Diego, Associate Professor of Earth System Science
Roger H. Rangel, Ph.D. University of California, Berkeley, Professor of Mechanical and Aerospace Engineering (Fluid dynamics and heat transfer of multiphase systems including spray combustion, atomization and metal spray solidification, applied mathematics and computational methods)
Elizabeth L. Read, Ph.D. University of California, Berkeley, Assistant Professor of Chemical Engineering and Materials Science; Molecular Biology and Biochemistry (Dynamics of complex biochemical systems, regulation of immune responses)
Eric Rignot, Ph.D. University of Southern California, Donald Bren Professor of Earth System Science (Glaciology, climate change, radar remote sensing, ice sheet modeling, interferometry, radio echo sounding, ice-ocean interactions)
Timothy Rupert, Ph.D. Massachusetts Institute of Technology, Assistant Professor of Mechanical and Aerospace Engineering; Chemical Engineering and Materials Science (Mechanical behavior, nanomaterials, structure property relationships, microstructural stability, grain boundaries and interfaces, materials characterization)
Manabu Shiraiwa, Ph.D. Max Planck Institute for Chemistry, Associate Professor of Chemistry (Atmospheric Chemistry, Heterogeneous and Multiphase Chemistry, Aerosol Particles, Reactive Oxygen Species, Kinetic Modeling)
Hal S. Stern, Ph.D. Stanford University, Professor of Statistics; Cognitive Sciences (Bayesian methods, model diagnostics, forensic statistics, and statistical applications in biology/health, social sciences, and sports)
Lizhi Sun, Ph.D. University of California, Los Angeles, Professor of Civil and Environmental Engineering; Chemical Engineering and Materials Science (Micro- and nano-mechanics, composites and nanocomposites, smart materials and structures, multiscale modeling, elastography)
A. Lee Swindlehurst, Ph.D. Stanford University, Professor of Electrical Engineering and Computer Science (Signal processing, estimation and detection theory, applications in wireless communications, geo-positioning, radar, sonar, biomedicine)
Kevin Thornton, Ph.D. University of Chicago, Associate Professor of Ecology and Evolutionary Biology School of Biological Sciences (Genome evolution, gene duplication, population genetics, adaptation)
Douglas J. Tobias, Ph.D. Carnegie Mellon University, Professor of Chemistry (Atmospheric and environmental, chemical biology, physical chemistry and chemical physics, theoretical and computational)
Isabella Velicogna, Ph.D. Università degli Studi di Trieste, UCI Chancellor's Fellow and Professor of Earth System Science
Nalini Venkatasubramanian, Ph.D., University of Illinois at Urbana-Champaign, Professor of Computer Science (Distributed Systems Middleware, Multimedia Systems and Applications, Mobile and Pervasive Computing, Formal Methods, Data Management, and Grid Computing)
Jasper A. Vrugt, Ph.D. University of Amsterdam, Associate Professor of Civil and Environmental Engineering; Earth System Science (Complex systems, modeling, statistics, hydrology, geophysics, ecology, data, optimization, hydropower, data assimilation)
Yun Wang, Ph.D. Pennsylvania State University, Associate Professor of Mechanical and Aerospace Engineering (Fuel cells, computational modeling, thermo-fluidics, two-phase flows, electrochemistry, Computational Fluid Dynamics (CFD), turbulent combustion)
Zhiying Wang, Ph.D. California Institute of Technology, Assistant Professor of Electrical Engineering and Computer Science (information theory, coding theory for data storage, modeling, compression, and computation for genomic data)
Daniel Whiteson, Ph.D. University of California, Berkeley, Professor of Physics and Astronomy; Logic and Philosophy of Science (Particle Physics: Experimental High Energy Physics, structure of matter and the nature of its interactions at the very smallest scales)
Dominik Franz X. Wodarz, Ph.D. University of Oxford, Professor of Ecology and Evolutionary Biology; Mathematics; Program in Public Health (Dynamics of virus infections and the immune system, dynamics of cancer and its treatment, and general evolutionary dynamics and population dynamics)
Xiaohui Xie, Ph.D. Massachusetts Institute of Technology, Professor of Computer Science; Developmental and Cell Biology (computational biology, bioinformatics, genomics, neural computation, machine learning)
Charles S. Zender, Ph.D. University of Colorado Boulder, Professor of Earth System Science; Computer Science
San Diego State University Faculty
Reza Akhavian, Ph.D. University of Central Florida, Assistant Professor of Department of Civil, Construction, and Environmental Engineering (Construction Engineering and Management, Internet of Things (IoT), Data Analytics, Machine Learning, Robotics, Cyber-Physical Systems, Building Information Modeling (BIM)
Ashkan Ashrafi, Ph.D. University of Alabama, Huntsville, Associate Professor of Electrical and Computer Engineering (Digital and Statistical Signal Processing, Real-Time DSP, Biomedical Signal Processing, Fourier Analysis, Direct Digital Frequency Synthesizers, Multivariate Spectral Analysis, Hilbert Spaces, Matrix Theory and Applications)
Barbara Ann Bailey, Ph.D. North Carolina State University, Associate Professor of Statistics (Nonlinear Time Series, Dynamical Systems, and Clouds. Visualization of Nonlinear Models. Environmental Monitoring. Population Dynamics and Embryonic Mortality. Model Validation)
Arlette Baljon, Ph.D. University of Chicago, Associate Professor of Physics (Biophysics, Complex Networks, Polymer Science and computational soft matter physics)
Amneet Bhalla, Ph.D. Northwestern University, Assistant Professor of Mechnical Engineering (Fluid-Structure Interaction, Multiphase Flows, Aquatic Locomotion, Renewable Energy Device Modeling, Numerical Methods, High Performance Computing, Scientific Software Design)
Peter Blomgren, Ph.D. University of California, Los Angeles, Professor of Mathematics (Image Processing, Wave Propagation in Complex Media, Numerical Solutions of PDEs, Scientific Computing, Nonlinear Dynamical Systems)
Joaquin Camacho, Ph.D. University of California, Los Angeles, Assistant Professor of Mechanical Engineering (Multiphase Flows, Sustainable Energy, Nanomaterial Theory and Fabrication, Combustion, Aerosol Dynamics, Carbon Materials)
Margherita Capriotti, Ph.D. University of California, San Diego, Assistant Professor of Aerospace Engineering (Develop novel and efficient tools to characterize aerospace composite structures using wave propagation of different physical nature)
Ricardo Carretero, Ph.D. University College London, Professor of Mathematics (Nonlinear Dynamics, Nonlinear Waves, Bose-Einstein Condensation (BEC))
Jose Castillo, Ph.D. University of New Mexico, Professor of Mathematics (Numerical Solution of Partial Differential Equations, Scientific Computing, and Modeling)
Jianwei Chen, Ph.D. Chinese University of Hong Kong, Associate Professor of Statistics (Statistical Inferences for Nonlinear Dynamic Models, Bayesian Methods, MCMC, and Computational Statistics)
Andy Cooksy, Ph.D. University of California, Berkeley, Professor of Chemistry and Biochemistry (Laser Spectroscopy, Reaction Dynamics, and Ab Initio Calculation of Free Radicals and Other Transient Molecule) Chris Curtis, Ph.D., University of Washington, Assistant Professor of Mathematics (Fluid Mechanics, Modeling and Simulation, Computational Fluid Dynamics and Numerical Simulation)
Bryan Donyanavard, Ph.D., University of California, Irvine, Assistant Professor of Computer Science (Runtime Resource Management for Energy-Efficient Execution of Cyber-Physical Systems) Robert Edwards, Ph.D. University of Sussex, Brighton, England, Professor of Computer Science (Microbiology, Bioinformatics, and High Performance Computing) Juanjuan Fan, Ph.D. University of Washington, Professor of Statistics (Multivariate Failure Time Data, Tree Based Methods, Genetic Epidemiology)
Uduak George, Ph.D. University of Sussex, Brighton, UK, Assistant Professor of Mathematics and Statistics (Mathematical biology, fluid dynamics, continuum mechanics of tissues, morphogenesis, solute transport) Jerome Gilles, Ph.D. Ecole Normale Supeieure, France, Assistant Professor of Mathematics (Applied Harmonic/Functional Analysis, Signal/Image Processing, data driven methods, Functional analysis)
Kyle Hasenstab, Ph.D. University of California, Los Angeles, Assistant Professor of Statistics (Deep neural networks, medical image analysis, interpretability of AI algorithms, functional data analysis)
Hajar Homayouni, Ph.D. Colorado State University, Assistant Professor of Computer Science (Data Quality Testing, Big Data, and Machine Learning)
Ke Huang, Ph.D. University of Grenoble, France, Assistant Professor of Electrical and Computer Engineering (VLSI Testing, Fault Modeling and Diagnosis. Machine Learning, Data Mining. Trustworthy ICs. Computer-Aided Design) Gustav Jacobs, Ph.D., University of Illinois at Chicago, Professor of Aerospace Engineering (Computational Physics, High-Order Methods, Fluid and Plasma Dynamics) Calvin Johnson, Ph.D. University of Washington, Professor of Physics (Theoretical and Computational Nuclear Structure and Nuclear Astrophysics) Parag Katira, Ph.D. University of Florida, Assistant Professor of Mechanical Engineering (Biomolecular Motors, Cell Mechanics, Mechanosensing, Tissue Dynamics, Soft Matter Interactions, Design of Active Materials)
Alicia Kinoshita, Ph.D. University of California, Los Angeles, Associate Professor of Civil Engineering (Hydrologic change in coupled human-natural systems) Sunil Kumar, Ph.D. Birla Institute of Technology and Science, India, Professor of Electrical and Computer Engineering and Thomas G. Pine Faculty Fellow (Wireless Networks, Multimedia Traffic, and Video Processing Techniques) Lyuba Kuztnesova, Ph.D. Cornell University, Assistant Professor of Physics (Nanophotonics, ultrafast lasers, and cavity quantum electrodynamics and high energy short-pulse generation in fiber laser systems, mode-locking in quantum cascade lasers, blue LEDs, microcavities, and metamaterials) Richard Levine, Ph.D. Cornell University, Professor of Statistics (Markov Chain Monte Carlo Methods, Environmental Statistics, Biostatistics, Bayesian Decision Theory) Xiaobai Liu, Ph.D. Huazhong University of Science and Technology, China, Associate Professor of Computer Science (Computer Vision, Machine Learning, Computational Statistics and their applications to clinic diagnosis, sports, transportation, surveillance, video games and others) Antonio Luque, Ph.D. University of Barcelona, Assistant Professor of Mathematics (Applied Mathematics, Biophysics, Physical Virology+ theoretical and computational biophysics as well as mathematical modeling, molecular and physicochemical properties of viruses in viral ecology)
Sahar Ghanipoor Machiani, Ph.D. Virginia Tech University, Assistant Professor of Civil, Construction, and Environmental Engineering (Traffic Safety and Signal Operation, Human Behavior Modeling, Connected/Automated Vehicles, Evacuation Modeling Infrastructure-Based Safety Systems)
Marta Miletic, Ph.D. Kansas State University, Assistant Professor of Civil, Construction, and Environmental Engineering (Geotech Engineering)
Duy Nguyen, Ph.D. McGill University, Canada, Assistant Professor of Electrical and Computer Engineering (Signal Processing, Communications, and Information Theories for Wireless Systems and Networks) Kenneth Nollett, Ph.D. University of Chicago, Assistant Professor of Physics (Theoretical and computational physics, spanning the interface between nuclear physics and astrophysics) Christopher Paolini, Ph.D. San Diego State University, Assistant Professor of Electrical and Computer Engineering (Cyberinfrastructure, Computational Geochemistry and Combustion Science)
Pavel Popov, Ph.D. Cornell University, Professor of Aerospace Engineering (Computational combustion with applications to aerospace propulsion. His research interests include combustion instability in aerospace engines, stochastic modelling of turbulent combustion, plasma-combustion interactions simulation of multiphase flow, turbulence modelling and high-performance computing.)
Shangping Ren, Ph.D. University of Illinois at Urbana-Champaign, Professor of Computer Science (Cyber-Physical Systems, Real-Time Scheduling, and Cloud Computing) Forest Rohwer, Ph.D. San Diego State University, Professor of Biology (Genomic Analysis of Phage, Diversity of Coral-associated Bacteria, Opportunistic Infections and Coral Disease)
Eric Sandquist, Ph.D. University of California, Santa Cruz, Professor of Astronomy (Physics of Stars and the Way They Age) Anca Segal, Ph.D. University of Utah, Professor of Biology (The Mechanism of Site-Specific Recombination; Structure/Function Analysis of Recombination Proteins)
Ignacio Sepulveda, Ph.D. Cornell University, Assistant Professor of Civil Engineering (Coastal Hazards, Coastal Engineering, Tsunami Science, Seismology, Stochastic Calculus for Uncertainty Quantification, Remote sensing, Wave Mechanics, Inversions)
Arun Sethuraman, Ph.D. Iowa State University, Assistant Professor of Bioinformatics (Population Genomics, Evolution, Bioinformatics)
Satish Sharma, Ph.D. Banaras Hindu University, India, Professor of Electrical and Computer Engineering (Electromagnetics antennas and waves, microwave devices and systems) Samuel Shen, Ph.D. University of Wisconsin, Madison, Albert W. Johnson Distinguished Professor of Mathematics (Statistical Climatology & Agroclimatology, Fluid Dynamcis & Forced Nonlinear Waves) Nicholas Shikuma, Ph.D. University of California, Santa Cruz, Assistant Professor of Biology (Molecular Mechanisms of Bacteria/Bacteriophage/Animal Interactions) Usha Sinha, Ph.D. Indian Institute of Science, Bangalore, India, Professor of Physics (Medical and Imaging Physics, Magnetic Resonance Imaging (MRI), and Informatics)
Jeet Sukumaran, Ph.D. University of Kansas, Assistant Professor of Biology (Process-based modeling of macroevolutionary dynamics, diversification, and biogeography/phylogeography; species delimitation; host-parasite coevolution, phylogenetics) Mauro Tambasco, Ph.D. University of Western Ontario, Associate Professor of Physics (Medical Physics: Biophysics effects of ionizing radiation in the presence of strong magnetic fields) Naveen Vaidya, Ph.D. York University, Canada, Assistant Professor of Mathematics (Applied Mathematics, Mathematical Biology, Disease Modeling, Differential Equations) Satchi Venkataraman, Ph.D. University of Florida, Professor of Aerospace Engineering (Structural Mechanics, Design Optimization, Composite Materials, Biomechanics) Wei Wang, Ph.D. University of Nebraska, Lincoln, Associate Professor of Computer Science (Cyber-Physical Systems, Wireless Multimedia Networking, Breast Cancer Image Processing)
Qi Wang, Ph.D. Johns Hopkins University, Assistant Professor of Aerospace Engineering (Data Assimilation in Turbulent Environments, Adjoint-Based Optimization, Measurement-Enhanced Simulations, Drag Reduction and Optimal Sensor Placement, Pollution Source Localization in Stratified or Non-Stratified Turbulence) Fridolin Weber, Ph.D. University of Munich, Germany, Albert W. Johnson Distinguished Professor of Physics (Superdense Matter, Astrophysics, General Relativity) Tao Xie, Ph.D. New Mexico Institute of Mining and Technology, Professor of Computer Science (High-Performance Computing, Energy-Efficient Storage Systems, Parallel/Distributed Systems, and Security-Aware Scheduling)
Yang Xu, Ph.D. Penn State University, Assistant Professor of Computer Science (Cognitive science, computer science, linguistics and psychology)
Ahmad Bani Younes, Ph.D. Texas A&M University, Assistant Professor of Aerospace Engineering (Space research topics: including the development of fast and high fidelity gravity model for the earth anomalies; fast and efficient trajectories propagation for satellite motions; optimal control theory, and, algorithms development for optimization theory, perturbation theory, orbital motion, and very broadly algorithmic differentiation for automatically generating mixed sets of high-order partial derivatives.)
Send Page to Printer
Print this page.
Download Page (PDF)
The PDF will include all information unique to this page.
2023-2024 Catalogue
A PDF of the entire 2023-2024 catalogue.
Suggestions or feedback?
MIT News | Massachusetts Institute of Technology
- Machine learning
- Social justice
- Black holes
- Classes and programs
Departments
- Aeronautics and Astronautics
- Brain and Cognitive Sciences
- Architecture
- Political Science
- Mechanical Engineering
Centers, Labs, & Programs
- Abdul Latif Jameel Poverty Action Lab (J-PAL)
- Picower Institute for Learning and Memory
- Lincoln Laboratory
- School of Architecture + Planning
- School of Engineering
- School of Humanities, Arts, and Social Sciences
- Sloan School of Management
- School of Science
- MIT Schwarzman College of Computing
To build a better AI helper, start by modeling the irrational behavior of humans
Press contact :.
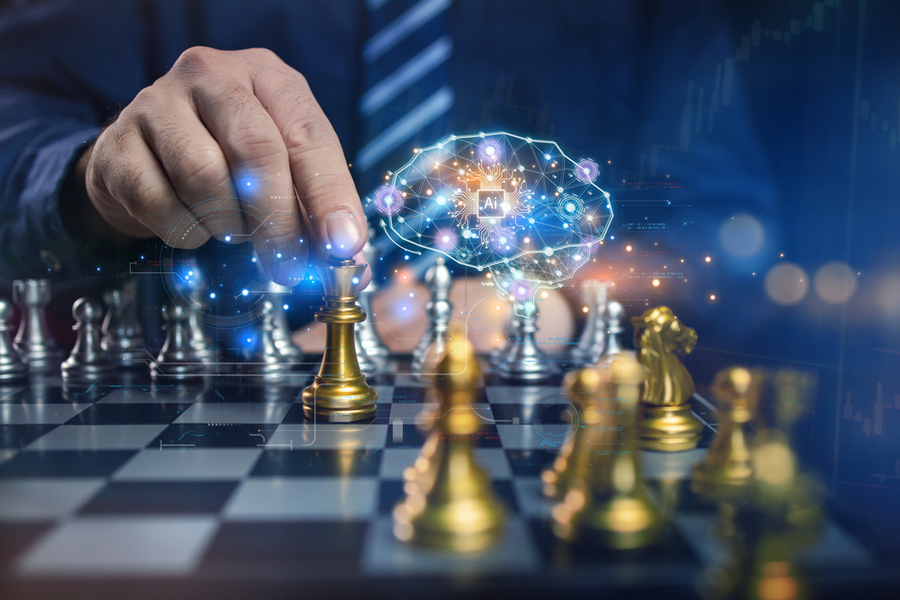
Previous image Next image
To build AI systems that can collaborate effectively with humans, it helps to have a good model of human behavior to start with. But humans tend to behave suboptimally when making decisions.
This irrationality, which is especially difficult to model, often boils down to computational constraints. A human can’t spend decades thinking about the ideal solution to a single problem.
Researchers at MIT and the University of Washington developed a way to model the behavior of an agent, whether human or machine, that accounts for the unknown computational constraints that may hamper the agent’s problem-solving abilities.
Their model can automatically infer an agent’s computational constraints by seeing just a few traces of their previous actions. The result, an agent’s so-called “inference budget,” can be used to predict that agent’s future behavior.
In a new paper, the researchers demonstrate how their method can be used to infer someone’s navigation goals from prior routes and to predict players’ subsequent moves in chess matches. Their technique matches or outperforms another popular method for modeling this type of decision-making.
Ultimately, this work could help scientists teach AI systems how humans behave, which could enable these systems to respond better to their human collaborators. Being able to understand a human’s behavior, and then to infer their goals from that behavior, could make an AI assistant much more useful, says Athul Paul Jacob, an electrical engineering and computer science (EECS) graduate student and lead author of a paper on this technique .
“If we know that a human is about to make a mistake, having seen how they have behaved before, the AI agent could step in and offer a better way to do it. Or the agent could adapt to the weaknesses that its human collaborators have. Being able to model human behavior is an important step toward building an AI agent that can actually help that human,” he says.
Jacob wrote the paper with Abhishek Gupta, assistant professor at the University of Washington, and senior author Jacob Andreas, associate professor in EECS and a member of the Computer Science and Artificial Intelligence Laboratory (CSAIL). The research will be presented at the International Conference on Learning Representations.
Modeling behavior
Researchers have been building computational models of human behavior for decades. Many prior approaches try to account for suboptimal decision-making by adding noise to the model. Instead of the agent always choosing the correct option, the model might have that agent make the correct choice 95 percent of the time.
However, these methods can fail to capture the fact that humans do not always behave suboptimally in the same way.
Others at MIT have also studied more effective ways to plan and infer goals in the face of suboptimal decision-making.
To build their model, Jacob and his collaborators drew inspiration from prior studies of chess players. They noticed that players took less time to think before acting when making simple moves and that stronger players tended to spend more time planning than weaker ones in challenging matches.
“At the end of the day, we saw that the depth of the planning, or how long someone thinks about the problem, is a really good proxy of how humans behave,” Jacob says.
They built a framework that could infer an agent’s depth of planning from prior actions and use that information to model the agent’s decision-making process.
The first step in their method involves running an algorithm for a set amount of time to solve the problem being studied. For instance, if they are studying a chess match, they might let the chess-playing algorithm run for a certain number of steps. At the end, the researchers can see the decisions the algorithm made at each step.
Their model compares these decisions to the behaviors of an agent solving the same problem. It will align the agent’s decisions with the algorithm’s decisions and identify the step where the agent stopped planning.
From this, the model can determine the agent’s inference budget, or how long that agent will plan for this problem. It can use the inference budget to predict how that agent would react when solving a similar problem.
An interpretable solution
This method can be very efficient because the researchers can access the full set of decisions made by the problem-solving algorithm without doing any extra work. This framework could also be applied to any problem that can be solved with a particular class of algorithms.
“For me, the most striking thing was the fact that this inference budget is very interpretable. It is saying tougher problems require more planning or being a strong player means planning for longer. When we first set out to do this, we didn’t think that our algorithm would be able to pick up on those behaviors naturally,” Jacob says.
The researchers tested their approach in three different modeling tasks: inferring navigation goals from previous routes, guessing someone’s communicative intent from their verbal cues, and predicting subsequent moves in human-human chess matches.
Their method either matched or outperformed a popular alternative in each experiment. Moreover, the researchers saw that their model of human behavior matched up well with measures of player skill (in chess matches) and task difficulty.
Moving forward, the researchers want to use this approach to model the planning process in other domains, such as reinforcement learning (a trial-and-error method commonly used in robotics). In the long run, they intend to keep building on this work toward the larger goal of developing more effective AI collaborators.
This work was supported, in part, by the MIT Schwarzman College of Computing Artificial Intelligence for Augmentation and Productivity program and the National Science Foundation.
Share this news article on:
Related links.
- Athul Paul Jacob
- Jacob Andreas
- Language and Intelligence Group
- Computer Science and Artificial Intelligence Laboratory
- Department of Electrical Engineering and Computer Science
Related Topics
- Computer science and technology
- Artificial intelligence
- Human-computer interaction
- Computer modeling
- Computer Science and Artificial Intelligence Laboratory (CSAIL)
- Electrical Engineering & Computer Science (eecs)
- National Science Foundation (NSF)
Related Articles
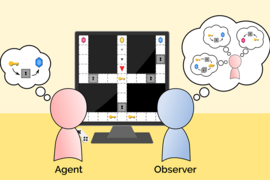
Building machines that better understand human goals
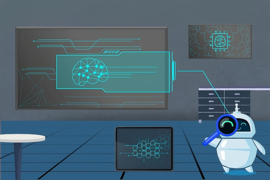
AI agents help explain other AI systems
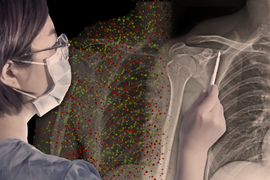
Automated system teaches users when to collaborate with an AI assistant
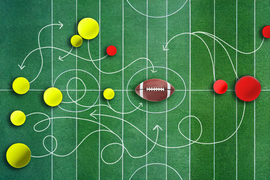
A far-sighted approach to machine learning
Previous item Next item
More MIT News
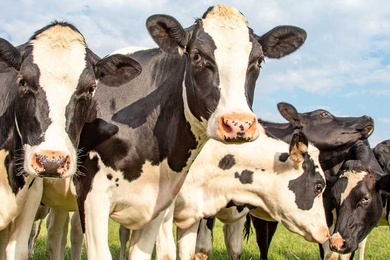
Featured video: Moooving the needle on methane
Read full story →
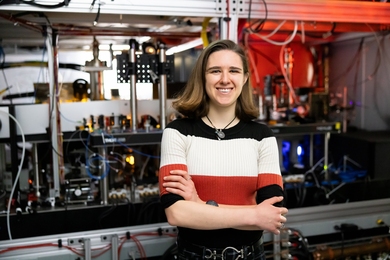
The many-body dynamics of cold atoms and cross-country running
Heather Paxson named associate dean for faculty of the School of Humanities, Arts, and Social Sciences
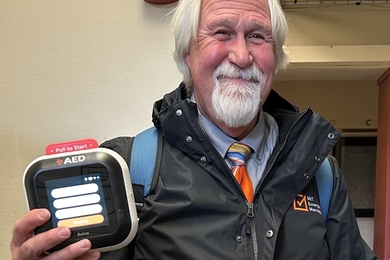
Preparing MIT’s campus for cardiac emergencies
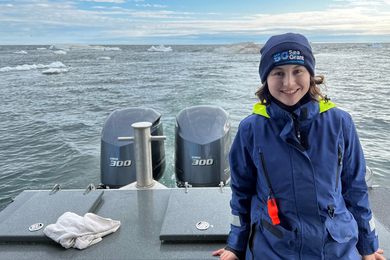
Researching extreme environments
Advancing technology for aquaculture
- More news on MIT News homepage →
Massachusetts Institute of Technology 77 Massachusetts Avenue, Cambridge, MA, USA
- Map (opens in new window)
- Events (opens in new window)
- People (opens in new window)
- Careers (opens in new window)
- Accessibility
- Social Media Hub
- MIT on Facebook
- MIT on YouTube
- MIT on Instagram
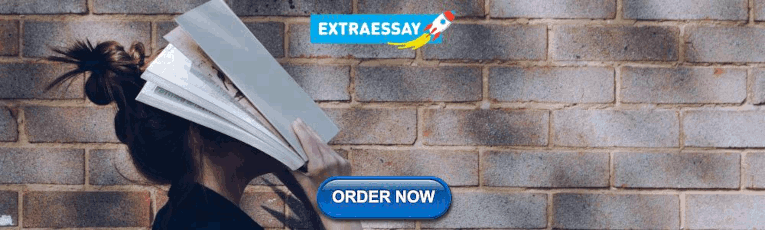
IMAGES
VIDEO
COMMENTS
Hello Reddit, I am currently in a conundrum on how to best proceed with getting PhD in Computational Social Science which is a relatively new interdisciplinary field that is not offered at many Universities. George Mason is pretty much the only well known that offers it. ... Computational Social Science which is a relatively new ...
NetSci 2023 -- Int'l School and Conference on Network Science (10-14 July, Vienna, AU) Important Dates: Satellite proposals deadline: January 15, 2023. Abstract submission deadline: February 1, 2023. Decisions on acceptance of abstracts: February 28, 2023. Early bird registration: before May 1, 2023.
Most "computational social science" happens within social science departments. See e.g. Chris Bail, Matt Salganik, and Duncan Watts in sociology. Noshir Contractor in communication. in fact getting a PhD in comp social science could be a bit of a disadvantage if you're pursuing an academic career, as there are very few "comp soc sci" depts and ...
I am in the process of choosing between two PhD schools in Computational Social Science. I want to know what considerations I need to prioritize. School A. School B. In Ireland, with pay of ~€18000. In Denmark, with pay of ~DKK384000. I don't know what work environment is there, although I don't expect it to be bad.
I am in the process of choosing between two PhD schools in Computational Social Science. I want to know what considerations I need to prioritize. School A School B In Ireland, with pay of ~€18000 ... The official Python community for Reddit! Stay up to date with the latest news, packages, and meta information relating to the Python ...
As an incoming international graduate student, would really appreciate inputs on how this course is w.r.t. Placements and Career opportunities after completion w.r.t. packages and firms Quality of the Faculty and Alumni Relevance in the Data Science/ Data Analytics domain w.r.t. similar courses at other top Unis
Dear scientists I have decided to pursue a PhD. My background is in sociology and applied statistics. Am currently looking for US based social science departments / PhD programs which have a strong focus, or at least a strong presence of computational social science.
The way things are going, big data is going to be of huge importance to social science research and gaining funding. Particularly with growing number of big data sets and advent of social media. In terms of securing your first grants it'll be strongly in your favour to be in that side of the social sciences.
The core objective of the computational social science (CSS) PhD program is to train graduate students to be professional computational social scientists in academia, government, or business. The program offers a unique and innovative interdisciplinary academic environment for systematically exploring, discovering, and developing skills to ...
However, because computational social science (CSS) is still relatively new to the social sciences, it can feel like a hidden curriculum for many PhD students. To support social science PhD students, this article is an accessible guide to CSS training based on previous literature and our collective working experiences in academic, public-, and ...
The Ph.D. Specialization in Computational Social Science brings together classes and community across the School of Social Sciences. The CSS Specialization supplements social science Ph.D. curricula with technical coursework and practice leveraging modern computational modeling methods and large naturalistic datasets. These skills, in turn ...
The field of computational social science exists because advances in technology have generated an unprecedented volume of digital data that can be used to research traditional social science topics like democracy, security, economic growth, and inequality. Computational methods include: Data mining. Natural language processing.
Computational social science provides scholars with the tools to deepen the understanding of long-standing questions in the social sciences, as well as explore new ones. The DE in Computational Social Science is both interdisciplinary in terms of requiring foundations in computational methods, data analysis, and social science theory, and it ...
The Computational Social Science Lab is an interdisciplinary research group that works at the ... A Social Map of Music on Reddit. What connects the Foo Fighers, James Blunt, and Eminem? ... Generative AI for social good. 3rd-year PhD. Jessica (Yi Fei) Bo. Human-centered design of intelligent systems. 1st-year PhD. Karim Hamadeh. Building ...
The DE CSS allows students with computational and mathematical skills to deepen their understanding of social science theory and it allows students from the social sciences to improve their analytical skills in areas like big data analysis, computer simulations, network analysis, and machine learning. The DE requires at least 4 courses ...
The Computational Social Science (CSS) major educates students to build, compute, and improve theoretically informed models of social processes, bridging domain and technical expertise. This major will prepare students to understand, engage with, and innovatively solve evolving, complex multi-scale challenges such as climate change, transnational political violence, cybersecurity and privacy ...
Computational Social Science. Growing use of the Internet and social media in the past decade has led to an explosion in the amount of social and behavioral data available to researchers. This in turn has created huge opportunities for social scientists to study human behavior and social interaction in unprecedented detail.
The standalone CSE PhD program is intended for students who intend to pursue research in cross-cutting methodological aspects of computational science. The resulting doctoral degree in Computational Science and Engineering is awarded by CCSE via the the Schwarzman College of Computing. In contrast, the interdisciplinary CSE PhD program is ...
This novel computational social science skillset is increasingly in demand to face modern challenges and develop future solutions in science, government, and business. UC San Diego offers Computational Social Science programs to train students in this exciting, interdisciplinary field at the undergraduate and graduate levels. Our CSS training ...
Computational Social Science (CSS) Initiative ... These labs will provide an opportunity for undergraduates to collect and analyze online data from places like Reddit and Twitter to study topics such as trolling behavior and its consequences or networks of influence. At the graduate level, the CSS Initiative is developing hands-on course ...
Admissions. University-wide admissions policies can be found in the Graduate Admissions Policies section of this catalog. To apply for this program, please complete the George Mason University Admissions Application. Eligibility. Students interested in applying for admission should have a bachelor's degree in computational science, any natural science, mathematics, engineering, or computer ...
Scientifically grounded in social sciences and humanities expertise, Computational Social Science students develop skills to design, create, apply and appropriate digital technology and data science to benefit society. organisation UvA. language English. coordinator (s) Education Desk Social Sciences. duration 3 years. more info.
The Ph.D. in Computational Science produces broadly educated, research-capable scientists that are well prepared for diverse careers in academia, industry, business, and government research laboratories. Students are admitted into the joint program via a Joint Admissions Committee. Applicants apply to UCI directly using the UCI graduate ...
Researchers have been building computational models of human behavior for decades. Many prior approaches try to account for suboptimal decision-making by adding noise to the model. Instead of the agent always choosing the correct option, the model might have that agent make the correct choice 95 percent of the time.