11 real and famous cases of malware attacks
- Updated at June 4, 2021
- Blog , Threat Research
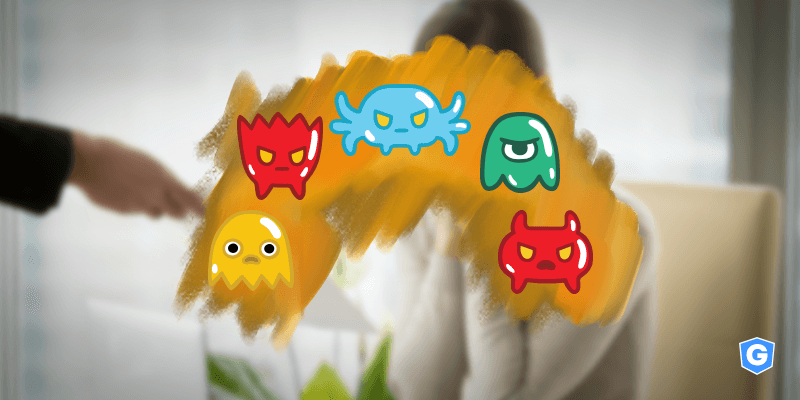
Many cases of famous hacker attacks use malware at some point. For example, first, the cybercriminal can send you a phishing email . No attachment. No links. Text only. After he gains your trust , in a second moment, he can send you a malicious attachment , that is, malware disguised as a legitimate file.
Malware is a malicious software designed to infect computers and other devices. The intent behind the infection varies. Why? Because the cybercriminal can use malware to make money, to steal secret information that can give strategic advantages, to prevent a business from running or even just to have fun.
Yes, there are hackers who act for pleasure.
In fact, malware is a broad term. It’s like a category. Within this category are different types of threats, such as virus , worm , trojan , and ransomware .
To fight malware delivered via email, here at Gatefy we offer a secure email gateway solution and an anti-fraud solution based on DMARC . You can request a demo or more information .
To get an idea, according to the FBI , damages caused by ransomware amounted to more than USD 29.1 million just in 2020. And one of the most widely used form of malware spreading continues to be via email . As a Verizon report confirmed : 30% of the malware was directly installed by the actor, 23% was sent there by email and 20% was dropped from a web application.
The cases listed below show how malware attacks can work and give you a glimpse of the harm they cause to businesses and individuals.
In this post, we’ll cover the following malware cases:
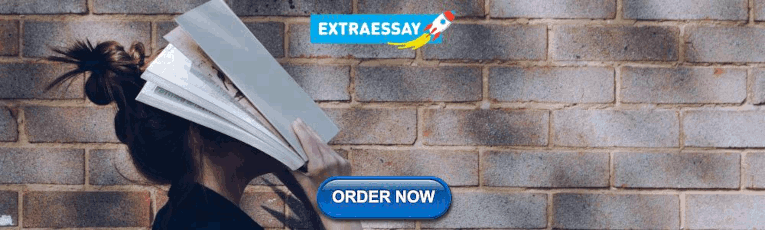
Table of Contents
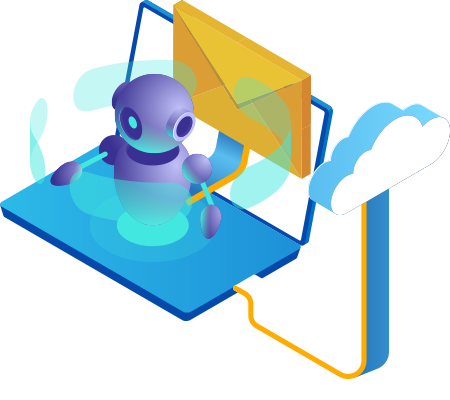
Check out 11 real cases of malware attacks
1. covidlock, ransomware, 2020.
Fear in relation to the Coronavirus (COVID-19) has been widely exploited by cybercriminals. CovidLock ransomware is an example. This type of ransomware infects victims via malicious files promising to offer more information about the disease.
The problem is that, once installed, CovidLock encrypts data from Android devices and denies data access to victims. To be granted access, you must pay a ransom of USD 100 per device.
2. LockerGoga, ransomware, 2019
LockerGoga is a ransomware that hit the news in 2019 for infecting large corporations in the world, such as Altran Technologies and Hydro. It’s estimated that it caused millions of dollars in damage in advanced and targeted attacks.
LockerGoga infections involve malicious emails , phishing scams and also credentials theft. LockerGoga is considered a very dangerous threat because it completely blocks victims’ access to the system.
3. Emotet, trojan, 2018
Emotet is a trojan that became famous in 2018 after the U.S. Department of Homeland Security defined it as one of the most dangerous and destructive malware. The reason for so much attention is that Emotet is widely used in cases of financial information theft, such as bank logins and cryptocurrencies.
The main vectors for Emotet’s spread are malicious emails in the form of spam and phishing campaigns . 2 striking examples are the case of the Chilean bank Consorcio, with damages of USD 2 million, and the case of the city of Allentown, Pennsylvania, with losses of USD 1 million.
4. WannaCry, ransomware, 2017
One of the worst ransomware attacks in history goes by the name of WannaCry , introduced via phishing emails in 2017. The threat exploits a vulnerability in Windows.
It’s estimated that more than 200,000 people have been reached worldwide by WannaCry, including hospitals, universities and large companies, such as FedEx, Telefonica, Nissan and Renault. The losses caused by WannaCry exceed USD 4 billion.
By the way, have you seen our article about the 7 real and famous cases of ransomware attacks ?
5. Petya, ransomware, 2016
Unlike most ransomware , Petya acts by blocking the machine’s entire operating system. We mean, Windows system. To release it, the victim has to pay a ransom.
It’s estimated that the losses involving Petya and its more new and destructive variations amount to USD 10 billion since it was released in 2016. Among the victims are banks, airports and oil and shipping companies from different parts of the world.
6. CryptoLocker, ransomware, 2013
The CryptoLocker is one of the most famous ransomware in history because, when it was released in 2013, it used a very large encryption key, which made the experts’ work difficult. It’s believed that it has caused more than USD 3 million in damage, infecting more than 200,000 Windows systems.
This type of ransomware was mainly distributed via emails, through malicious files that looked like PDF files , but, obviously, weren’t.
7. Stuxnet, worm, 2010
The Stuxnet deserves special mention on this list for being used in a political attack, in 2010, on Iran’s nuclear program and for exploiting numerous Windows zero-day vulnerabilities . This super-sophisticated worm has the ability to infect devices via USB drives, so there is no need for an internet connection.
Once installed, the malware is responsible for taking control of the system. It’s believed that it has been developed at the behest of some government. Read: USA and Israel.
8. Zeus, trojan, 2007
Zeus is a trojan distributed through malicious files hidden in emails and fake websites, in cases involving phishing . It’s well known for propagating quickly and for copying keystrokes, which led it to be widely used in cases of credential and passwords theft, such as email accounts and bank accounts.
The Zeus attacks hit major companies such as Amazon, Bank of America and Cisco. The damage caused by Zeus and its variations is estimated at more than USD 100 million since it was created in 2007.
9. MyDoom, worm, 2004
In 2004, the MyDoom worm became known and famous for trying to hit major technology companies, such as Google and Microsoft. It used to be spread by email using attention-grabbing subjects, such as “Error”, “Test” and “Mail Delivery System”.
MyDoom was used for DDoS attacks and as a backdoor to allow remote control. The losses are estimated, according to reports, in millions of dollars.
10. ILOVEYOU, worm, 2000
The ILOVEYOU worm was used to disguise itself as a love letter, received via email. Reports say that it infected more than 45 million people in the 2000s, causing more than USD 15 billion in damages.
ILOVEYOU is also considered as one of the first cases of social engineering used in malware attacks. Once executed, it had the ability to self-replicate using the victim’s email.
Also see 10 real and famous cases of social engineering .
11. Melissa, virus, 1999
The Melissa virus infected thousands of computers worldwide by the end of 1999. The threat was spread by email, using a malicious Word attachment and a catchy subject: “Important Message from (someone’s name)”.
Melissa is considered one of the earliest cases of social engineering in history. The virus had the ability to spread automatically via email. Reports from that time say that it infected many companies and people, causing losses estimated at USD 80 million.
How to fight malware attacks
There are 2 important points or fronts to fight and prevent infections caused by malware.
1. Cybersecurity awareness
The first point is the issue regarding cybersecurity awareness. You need to be aware on the internet. That means: watch out for suspicious websites and emails . And that old tip continues: if you’re not sure what you’re doing, don’t click on the links and don’t open attachments.
2. Technology to fight malware
The second point involves the use of technology . It’s important that you have an anti-malware solution on your computer or device. For end-users, there are several free and good options on the market.
For companies, in addition to this type of solution, we always recommend strengthening the protection of your email network. As already explained, email is the main malware vector. So, an email security solution can rid your business of major headaches.
Here at Gatefy we offer an email gateway solution and a DMARC solution . By the way, you can request a demo by clicking here or ask for more information . Our team of cybersecurity experts will contact you shortly to help.
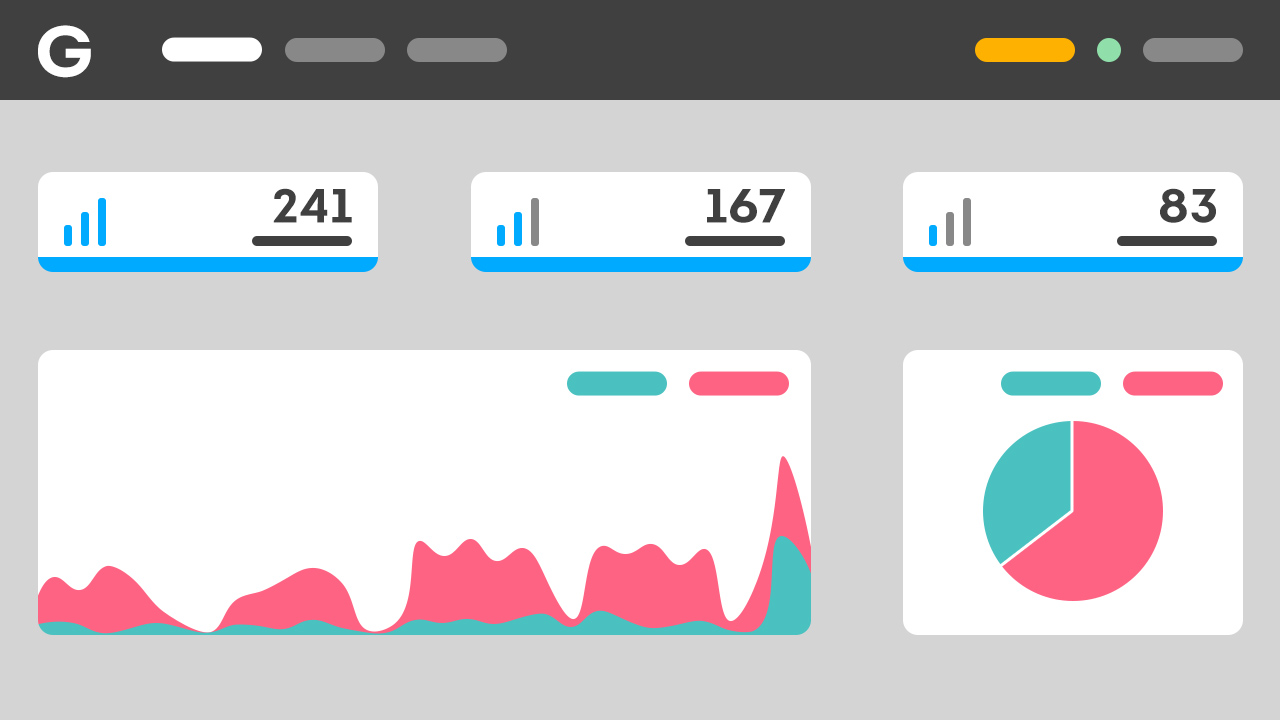
Latest news
10 real and famous cases of bec (business email compromise), 8 reasons to use dmarc in your business, what is mail server.
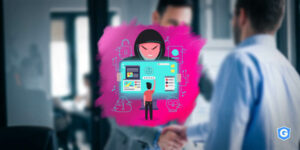
- Artificial Intelligence
- Generative AI
- Business Operations
- IT Leadership
- Application Security
- Business Continuity
- Cloud Security
- Critical Infrastructure
- Identity and Access Management
- Network Security
- Physical Security
- Risk Management
- Security Infrastructure
- Vulnerabilities
- Software Development
- Enterprise Buyer’s Guides
- United States
- United Kingdom
- Newsletters
- Foundry Careers
- Terms of Service
- Privacy Policy
- Cookie Policy
- Member Preferences
- About AdChoices
- E-commerce Links
- Your California Privacy Rights
Our Network
- Computerworld
- Network World

17 types of Trojans and how to defend against them
Trojan malware comes in many different types, but all require a user action to initiate.

Computer Trojans received their name from the infamous mythological horse. The Trojan’s basic mission is to mislead people of its real goal. A Trojan is malicious software that usually needs to be launched by the user or another malicious program.
Malicious code typically penetrates the system under the guise of a useful utility or tool. However, the main task of such software is to perform one or more of these malicious actions:
- Delete user or system data
- Change user or system data
- Encrypt user or system data
- Copy user or system data
- Send and receive files
- Slow computers
- Slow networks
Trojans have evolved into different complex forms like backdoors (that can manage distant computers) and downloaders (that can download and install other malicious programs. These are the types of Trojans that you need to be aware of:
1. ArcBombs
These Trojans represent special archives that are designed to behave abnormally when users try to unpack them. ArcBomb archives either freeze or seriously slow the system. Malicious archives use different techniques to achieve their goal. They may use malcrafted headers or corrupt data that lead to a malfunction of an archiver or an unpacking algorithm. They may also contain a heavyweight object that consists of identical, repeating data that can be packed into a small archive. For example, 10 GB of data is packed into a 400 KB archive.
2. Backdoors
Backdoors allow criminals to control computers remotely. Attackers can do a wide range of actions on an infected computer including receiving, sending, deleting or launching files; displaying screen alerts; or rebooting PCs. These Trojans can also help attackers install and launch third-party code on the victim’s device, record keystrokes (acting like keyloggers ), or turn on the camera and microphone. Sometimes backdoors are used to manage a group of infected computers (or recently IoT devices ) united into a botnet .
A separate type of backdoor can spread over the network (just like a worm ). Unlike woks, they do not spread by themselves, but only after receiving a command from their creator.
3. Banking Trojans
Banking Trojans are created to steal confidential user data such as login credentials, passwords, SMS authentication, or bank card info.
Emotet Discovered in 2014, Emotet was initially created to steal banking data. Spam functions and malware download options were added to later versions.
TrickBot Created in 2016, TrickBot is still one of the most prevalent banking Trojans. Besides targeting banks, TrickBot also steals cryptocurrency from Bitcoin wallets. TrickBot consists of several modules coupled with a config file. Modules have specific tasks such as stealing credentials, gaining persistence, or encryption. Hacked wireless routers are used as command-and-control servers.
4. Clickers
These Trojans are created to access internet sites and servers. Users are not aware of such activity as clickers send commands to browsers. Clickers may also replace Windows host files where standard addresses are indicated. Clickers are used to:
- Increase website traffic volumes to get more money from ads
- Run DDoS attacks
- Redirect potential victims to web pages containing hoaxes or malware
DDoS Trojans are intended to launch denial of service attacks targeting the victim’s IP address. During such attacks, a flood of requests get sent from multiple infected devices to the DDoS victim thus overloading it and causing it to stop functioning. To run a successful DDoS attack , cyber crooks need to infect plenty of computers with DDoS Trojans. Mass spam and phishing are often used for this. Once a botnet is ready, all infected computers start to simultaneously attack the victim.
6. Downloaders
Downloaders can download and launch malicious software, including other Trojans. Data about the location and name of the programs that need to be downloaded is stored inside the Trojan code or can be obtained by the Trojan from the server controlled by the Trojan author. Downloaders are frequently used to get the initial foothold on the system. Innocent users visit infected webpages that contain exploits. These exploits deliver downloaders that then download the rest of the malicious payload.
7. Droppers
These software pieces are designed to install malware covertly. They contain other malware that is obfuscated and deeply hidden inside the dropper’s code. This is done to prevent detection by antivirus software. Many antivirus tools cannot analyze all components of droppers. They usually are saved to a Windows temporary directory. Then they are executed without any user notifications.
Malicious software like fakeAV impersonates the operation of antivirus tools. Fake AV shows numerous security warnings to users trying to extort money from them. Inexperienced users get frightened and buy full versions of fakeAVs to get rid of the non-existent security threats.
9. Game thieves
Similar to bankers, game thieves are intended to steal confidential information. Instead of stealing financial records, game thieves steal information related to online gaming accounts. These Trojans then use email, FTP and other data transfer methods to pass stolen info to hackers .
10. Instant messaging Trojans
IM Trojans steal logins and passwords used to access instant messaging services like Skype or WhatsApp. Trojans then pass this data to attackers via email, web requests, FTP and other methods.
11. Loaders
A Loader is a small piece of code needed to install the full version of the virus. A tiny loader enters the computer system (for example, when the user is viewing a malicious image file). During this process, the loader connects to a server and downloads and installs the rest of its components.
12. Mailfinders
Mailfinders steal email addresses from user computers and transmit them to hackers. Criminals then use the collected addresses for spamming and phishing attacks.
13. Notifiers
This type of Trojans sends its developer information about the infected computer and its status. It may include info on open ports, launched software, and running services. Notifiers are used during complex attacks involving multi-component malware. Attackers need to be sure all parts of their malware are successfully installed.
14. Proxies
These Trojans allow attackers to get anonymous access to internet websites using the victim’s computer as an intermediary. Crooks use this type of viruses to send spam while hiding behind victims’ IP addresses.
15. Password stealing ware
Password Stealing Ware (PSW) steals passwords from infected computers. Trojans of this type can search for passwords kept by users inside files or by web browsers. Some variants may steal software licenses and system/network passwords.
16. Ransom Trojans
Ransom Trojans may encrypt files andor block access to a PC, so users are unable to use it. Attackers offer victims to pay the ransom in exchange for their data or restoring the regular operation of the device. These Trojans are extremely popular now. Some operators earn millions of dollars in short periods of time.
17. SMS Trojans
These rogue programs send SMS messages from infected devices (smartphones) to premium-rate numbers. Sometimes, they can be used as SMS interceptors during multi-stage attacks involving two-factor authentication .
How do Trojans work?
All Trojans consist of two parts: server and client. The client connects to the server with the help of the TCP/IP protocol. The client may have a user interface and a set of buttons and input fields for remote administration.
The server part is installed on the victim’s device. The server-side processes (executes) commands from the client and transfers various data. Once entering the computer, the server-side listens on a specific port waiting for commands. An attacker pings a port on an infected host. In case the server part is successfully installed, it responds with the computer IP address and network name. When the connection is established the client starts sending commands to the server part.
Trojan infection symptoms
- New applications in the Start-up menu
- Fake alerts informing about viruses, downloaded porn videos, etc.
- Sudden screenshots
- Playing sounds or displaying photos
- Sudden computer rebooting
How to defend against Trojans
Most Trojans require user permission to run. Users usually launch the malware when they click on an email attachment or allow macros in office docs. So, the best protection against Trojans is to train users to watch what they click or open. Users should be 100% sure of the sources of the files sent to them or downloaded from the internet. In today’s busy world, this is not always possible, so a few additional measures should be taken.
Keep all software updated, especially the OS, antivirus, and web browsers. Malefactors exploit security holes in these programs to place Trojans on your computers. Set up and use firewalls to keep the internet connections secure. Firewalls filter out malicious traffic and prevent Trojans from getting delivered onto your device.
As there are many types of Trojan, no single method will get rid of them. The first step is to clean the temporary folder, locate malicious entries in the registry, and manually delete them while in Safe Mode. The best antivirus tools can detect and remove Trojans automatically. Regular antivirus updates are essential to better detection accuracy.
Related content
Exploit available for critical flaw in forticlient server, fbi and cisa warn government systems against increased ddos attacks, top cybersecurity product news of the week, new phishing campaign targets us organizations with netsupport rat, from our editors straight to your inbox.

David Balaban is a computer security researcher with over 17 years of experience in malware analysis and antivirus software evaluation. David runs MacSecurity.net and Privacy-PC.com projects that present expert opinions on contemporary information security matters, including social engineering, malware, penetration testing, threat intelligence, online privacy, and white hat hacking. David has a strong malware troubleshooting background, with a recent focus on ransomware countermeasures.
More from this author
How to select a dlp solution: 9 unusual considerations, 4 ways attackers exploit hosted services: what admins need to know, why you should consider outsourcing dlp, most popular authors.

Show me more
Udp-based network communications face critical denial-of-service attacks.

Why more women aren't CISOs and how to change that

Study: Cybersecurity burnout impacts 88% of cybersecurity and IT roles in Singapore. What can you do as an MSP to help?

CSO Executive Sessions: 2024 International Women's Day special

CSO Executive Sessions: Former convicted hacker Hieu Minh Ngo on blindspots in data protection

CSO Executive Sessions Australia with Sunil Sale, CISO at MinterEllison

LockBit feud with law enforcement feels like a TV drama

Sponsored Links
- Tomorrow’s cybersecurity success starts with next-level innovation today. Join the discussion now to sharpen your focus on risk and resilience.
- IDC report: Life-cycle services can help align technology, operational, and business outcomes.
- Digital infrastructure plays a big role in business outcomes. Read this IDC report to learn more.
Trojan malware is back and it's the biggest hacking threat to your business
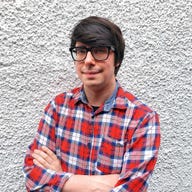
Trojan malware attacks against business targets have rocketed in the last year, as cyber criminals alter their tactics away from short-term gain and in-your-face ransomware attacks towards more subtle, long-term campaigns with the aim of stealing information including banking information, personal data and even intellectual property.
- 8 habits of highly secure remote workers
- How to find and remove spyware from your phone
- The best VPN services: How do the top 5 compare?
- How to find out if you are involved in a data breach -- and what to do next
Figures from security company Malwarebytes Labs in a new report suggest that trojan and backdoor attacks have risen to become the most detected against businesses – and the number of trojan attacks has more than doubled in the last year, increasing by 132 percent between 2017 and 2018, with backdoors up by 173 percent.
Malwarebytes classifies trojans and backdoors separately, describing a trojan as a program "that claim to perform one function but actually do another", Meanwhile, a backdoor is defined as "a type of trojan that allows a threat actor access to a system by bypassing its security" and gaining access to systems undetected.
Attacks using spyware — malware that gathers information on a device and sends it to a third-party actor — have also jumped hugely, up by 142 percent in the same period.
"When you say spyware, people think of how it's been around for a decade or more and it's old and boring — but it's really effective and it's really come back into fashion with the rise in attacks on businesses and a thirst for data exfiltration," Chris Boyd, lead malware intelligence analyst at Malwarebytes told ZDNet. "Despite its potentially mundane trappings, spyware is quite a big deal again"
In contrast, file-encrypting ransomware attacks have only risen by 9 percent over the course of the same period.
SEE: What is malware? Everything you need to know about viruses, trojans and malicious software
A particularly prolific information-stealing campaign throughout 2018, and into 2019, came in the form of the Emotet trojan , which among other things, steals data, monitors network traffic, can move through networks, and is capable of dropping other trojans onto infected systems.
Emotet is dangerous in of itself but the ability to install other malware onto compromised systems makes it a real menace — and those behind the campaign are intentionally attempting to spread it to business targets, warns the report.
The paper also points to TrickBot as a particularly prolific trojan — in part, being helped along because it's a secondary payload dropped by Emotet.
Like Emotet, TrickBot is constantly being updated with with new capabilities, with the malware recently adding the ability to steal passwords and browser histories from victims in a move that improves TrickBot's ability to gather the information needed for those behind it to secretly traverse across networks and work towards the ultimate goal of stealing sensitive data.
That targeted data is more than just simply personal information and banking details – with Malwarebytes warning that consultancy firms are the primary target for trojan campaigns.
For cyber criminals, breaching one of these could provide a treasure trove of data, not just about the companies and their clients, but it could potentially provide them access to intellectual property or other secrets.
Trojan malware attacks aren't a new phenomenon, but organisations underestimate cyber criminals deploying them at their peril.
"It's almost going a little bit old-school," said Boyd. "The stuff we thought was boring or a bit old hat still works really well"
READ MORE ON CYBER CRIME
- How one hacked laptop led to an entire network being compromised
- Global hacking campaign targets critical infrastructure CNET
- Data breaches, cyberattacks are top global risks alongside natural disasters and climate change
- Cyberattacks are becoming more clever than ever, here's what to look for TechRepublic
- Cyber security: Hackers step out of the shadows with bigger, bolder attacks
The best security keys you can buy: Expert tested
Ai is changing cybersecurity and businesses must wake up to the threat, google chrome now better protects you against risky websites and weak passwords.
Red Team vs. Blue Team: A Real-World Hardware Trojan Detection Case Study Across Four Modern CMOS Technology Generations
Ieee account.
- Change Username/Password
- Update Address
Purchase Details
- Payment Options
- Order History
- View Purchased Documents
Profile Information
- Communications Preferences
- Profession and Education
- Technical Interests
- US & Canada: +1 800 678 4333
- Worldwide: +1 732 981 0060
- Contact & Support
- About IEEE Xplore
- Accessibility
- Terms of Use
- Nondiscrimination Policy
- Privacy & Opting Out of Cookies
A not-for-profit organization, IEEE is the world's largest technical professional organization dedicated to advancing technology for the benefit of humanity. © Copyright 2024 IEEE - All rights reserved. Use of this web site signifies your agreement to the terms and conditions.
To revisit this article, visit My Profile, then View saved stories .
- Backchannel
- Newsletters
- WIRED Insider
- WIRED Consulting
Geoff White
The 20-Year Hunt for the Man Behind the Love Bug Virus
This story is adapted from Crime Dot Com: From Viruses to Vote Rigging, How Hacking Went Global , by Geoff White.
It’s 30 degrees in the shade and I’m standing, sweating, at the entrance to a sprawling street market in the Quiapo district of Manila, capital of the Philippines. On a piece of paper I’ve written the name of the person I’m searching for: a Filipino man named Onel de Guzman. I’ve heard he might have worked among the mass of stalls spread out before me ... maybe ... several years ago.
I start showing the piece of paper to people at random. It seems an impossible task. The wildest of goose chases. I don’t know what de Guzman looks like now, because the only photo I have of him is almost 20 years old. Even worse: In the grainy shot, taken at a chaotic press conference, de Guzman is wearing sunglasses and covering his face with a handkerchief.
The young student had good reason to hide. He’d been accused of unleashing the Love Bug , a high-profile and extremely successful virus that had infected an estimated 45 million computers worldwide and caused billions of dollars’ worth of damage.
The virus was groundbreaking. Not because of its technical complexity or the disruption it caused, but because it showed how to utilize something far more powerful than code. It perfectly exploited a weakness not in computers, but in the humans who use them—a tactic that has been used in countless cybercrimes since. But de Guzman had never admitted to anything. He’d mumbled his way through the press conference, given a couple of noncommittal interviews to the media, and escaped without prosecution. Then he’d gone to ground and hadn’t surfaced in two decades. No social media, no online profile. A ghost in the digital world he’d once been accused of terrorizing.
It had taken me a year to get any kind of lead as to his whereabouts. There were rumors he was in Germany, that he worked for the United Nations in Austria, that he’d moved to the United States, or even that he’d been hired by Microsoft. And now I was stumbling through a market in Manila, showing his name in the hope someone would recognize it.
If I could find him, maybe I could ask him about the virus and whether he understood its impact. And perhaps I could get him to tell me, after 20 years, whether he was really the one behind it. But as I brandished his name, all I got were blank looks and suspicious questions. Then one of the market stallholders grinned at me.
“The virus guy? Yeah, I know him.”
The Love Bug virus was unleashed on May 4, 2000. It was simple, but devastatingly effective and highly contagious. Once infected, many of the user’s files would be overwritten with copies of the virus, so that whenever the victim tried to open the files, they’d reinfect their system. The virus also tried to steal people’s passwords. But the true genius lay in how it spread. Once infected, the victim’s computer would send an email to everyone in their Microsoft Outlook contacts book. The emails read: “kindly check the attached love letter coming from me,” and attached was a copy of the virus, disguised as a text file with the title “love-letter-for-you.”
Andy Greenberg
Steven Levy
Matt Reynolds
Jeremy White
Faced with such a tempting message, many people took the bait, opened the attachment, and got infected. It didn’t take long for the virus to spread around the world. When you think about the math, its success becomes easy to understand, and quite frightening: If the initial victim had sent it to 50 people, and then each of them infected another 50 people, and so on, it would only take six jumps for the virus to infect everyone in the world (presuming they all had computers).
Panic ensued: Systems in banks and factories were infected. In the UK, Parliament shut down its email network for several hours to prevent infection. Even the Pentagon was reportedly affected.
Just a few months previously, the world had been fretting about the risk of a so-called Y2K bug—the fear that computers would fail to cope with the switch from dates in the 1900s to the 2000s. The damage predictions had been massively exaggerated, and the vast majority of systems were unaffected. But just as the tech industry breathed a sigh of relief, the Love Bug virus showed the true scale of devastation that could be caused in an increasingly connected world. Estimates of the damage ran into the tens of billions of dollars—much of it spent on fixing infected computers and preventing reinfection. Once it was released, the virus code could be downloaded and tweaked by anyone: within days, researchers were seeing dozens of copycat versions being unleashed.
As the news coverage became ever more shrill, investigators got to work trying to trace the source of the bug. The passwords stolen by the virus were being sent to an email address registered in the Philippines. Local police traced the email account to an apartment in Manila. The net was closing in.
After some initial questioning, they identified one Onel de Guzman, a 23-year-old computer science student at AMA Computer College, studying at the Makati campus, a grim, gray concrete building in the center of the city. The virus had mentioned the phrase grammersoft , which investigators quickly established was an underground hacking cell made up of AMA students, some of whom had started experimenting with viruses. De Guzman was a leading member.
As journalists poured into town, de Guzman’s lawyer hastily arranged a press conference so the world’s media could put their questions to the man increasingly assumed to be at the heart of global virus outbreak. De Guzman appeared, seemingly terrified, hiding behind dark glasses and holding a handkerchief over his face, covering his prominent acne scars. He hung onto his sister, Irene, who lived in the flat that the police had originally raided. Flashguns popped and news cameras zoomed in as de Guzman took his seat. But anyone expecting clarification was soon disappointed. De Guzman’s lawyer fielded many of the questions with vague non-answers.
De Guzman himself seemingly didn’t speak much English. Finally, one of the assembled media managed to ask a key question: Did de Guzman, perhaps, release the virus accidentally?
“It is possible,” mumbled de Guzman.
And that was it. There were no more questions. The press conference ended, and de Guzman’s solitary non-answer was the closest anyone got to an explanation of a virus that infected 45 million machines worldwide.
De Guzman was never prosecuted because, at that time, the Philippines had no law against computer hacking. Soon, the cameras packed up, the news crews left, and the story slipped off the agenda.
With the true author unconfirmed, suspicion fell on de Guzman’s schoolfriend Michael Buen, whose name had appeared on a previous virus, called Mykl-B. Buen denied having anything to do with the Love Bug outbreak, but his pleas were largely ignored. Most online sources still list de Guzman and Buen as the creators of the virus, either jointly or separately, and that’s how it’s been for 20 years. Until now.
The Minor Basilica of the Black Nazarene is one of Manila’s most revered Catholic shrines, and in its shadow lies the labyrinthine expanse of Quiapo market, home to everything from Hello Kitty backpacks to LED-lit Virgin Mary statuettes. It was here, acting on a tip-off, that I came to look for Onel de Guzman.
Eventually, the friendly stall-holder who remembered him directed me across town to a different shopping district. I went down another rabbit hole of market stalls, flashing the piece of paper with de Guzman’s name written on it, looking like a tourist dad who’d lost his kids. After many blank looks and suspicious questions, a bored-looking trader pointed me in the direction of a nearby commercial unit. It was empty, but after 10 hours of waiting for him to turn up to work, I finally came face to face with Onel de Guzman.
Now 43, his juvenile acne scars have all but disappeared, and his diamond-shaped face has filled out into comfortable middle age. Still as shy as he was at the press conference all those years ago, he hides his gaze under a mop of jet-black hair, his face occasionally breaking into a smile displaying a distinctive set of uniform teeth. He’d changed so much, I began to doubt I was actually speaking to the real de Guzman, so I started making a furtive sketch in my notepad of the position of the moles on his face, to compare later on with the photo of him from 20 years ago. Back then, in the chaotic press conference, he’d swerved the question of whether he had written the virus, giving the half-answer that’s remained hanging in the air ever since. According to de Guzman, it wasn’t his idea to be so evasive.
“That’s what my lawyer told me to do,” he says, in halting English.
I’d expected to have to extract the truth from de Guzman by forensic interview, and I’d lined up my evidence like an amateur barrister. Remarkably, he wasted no time in confessing to a wrongdoing he’d ducked ever since the turn of the millennium. “It wasn’t a virus, it was a Trojan,” he says, correcting my terminology to point out that his malicious software worked by sneaking onto a victim’s computer disguised as something benign. “I didn’t expect it would get to the US and Europe. I was surprised.”
The story he went on to tell is strikingly straightforward. De Guzman was poor, and internet access was expensive. He felt that getting online was almost akin to a human right (a view that was ahead of its time). Getting access required a password, so his solution was to steal the passwords from those who’d paid for them. Not that de Guzman regarded this as stealing: He argued that the password holder would get no less access as a result of having their password unknowingly “shared.” (Of course, his logic conveniently ignored the fact that the internet access provider would have to serve two people for the price of one.)
De Guzman came up with a solution: a password-stealing program. In hindsight, perhaps his guilt should have been obvious, because this was almost exactly the scheme he’d mapped out in a thesis proposal that had been rejected by his college the previous year.
At the time, he says, designing such software wasn’t difficult. “There was a bug in Windows 95,” he says. “If someone clicks the attachment, [the program] will run through their machine.”
But there’s the rub: how to get people to click on the attachment? De Guzman says he would hang out in internet chat rooms where Manila internet users gathered, and strike up conversations. He would then send his victims an infected file, pretending it was his picture. It worked. “I chatted only to people that had no knowledge of computers, to experiment on them,” he says.
De Guzman had good reason to confine his hacking to Manila residents. At this time, internet access relied on dialup. Since Manila’s dialup passwords would only work on Filipino phones, and de Guzman was stealing passwords to use on his home phone line, he had no need to target victims outside the city. If he’d kept it that way, his life might have been very different. But, like many hackers, de Guzman was curious, and wanted to push his virus forward.
In May 2000, he tweaked his original code so that it would not simply be restricted to Manila residents. He also made two other changes that would ensure his place in hacker history. First, he programmed the virus so that once it had infected a computer, it would send a copy of itself to each person in the victim’s email address book. By doing so, he created a so-called worm virus, a self-spreading monster with no off switch. Once released, de Guzman would have no control.
His second change was the work of true, if perhaps unconscious, genius. Once the virus spread beyond de Guzman’s hands, he needed a way of tempting recipients into opening the attachment that contained the code. His old trick of pretending it was a photo wouldn’t work, so he came up with a new tactic: He gave the virus a title that had universal and near-irresistible appeal. “I figured out that many people want a boyfriend, they want each other, they want love, so I called it that,” he says.
The Love Bug was born.
Like many hackers, de Guzman is a night owl. He finds the dark hours quieter, making it easier to concentrate. It was 1 am when de Guzman found his patient zero, the person whose initial infection would go on to spread the virus. He was chatting online to a fellow Filipino who was living in Singapore. De Guzman can’t remember who the man was, but he remembers sending him a copy of his new, improved virus.
Unaware of the worldwide chaos he’d just unleashed, de Guzman says he then went out and got drunk with a friend. Within a day, though, his virus had spread like wildfire and investigators were closing in on their suspect.
His mother contacted him. She’d received word the police were hunting a hacker in Manila, and she knew of her son’s illicit hobby. She hid his computer but crucially left the disks, one of which had the Mykl-B virus on it, pulling Michael Buen and several dozen other AMA students onto the police’s radar.
For 20 years, de Guzman’s silence left a cloud hanging over his classmate Buen, who is commonly listed as the joint author of the virus. Yet according to de Guzman, he had nothing to do with it. The pair had written viruses before, he says, but the Love Bug was written by de Guzman alone.
De Guzman says he had to take a year off after the incident to let the heat die down, during which he didn’t touch a computer. He never went back to AMA and never graduated. He later became a mobile-phone technician. He says he regrets writing the virus, but he now faces the fate of all wrongdoers in the internet age: infamy that will never decay. “Sometimes I get my picture on the internet,” he says. “My friends said, ‘It’s you, it’s you!’ They find my name. I’m a shy person, I don’t want this.” His children are ages 7 and 14. He knows one day soon they will find out about his role in one of the world’s most infamous viruses. He’s not sure how he’ll deal with that.
At the end of our interview, de Guzman goes back to his job, disappearing into the mall’s mass of tiny tech repair stalls, where he sits surrounded by soldering irons, multimeters, and disassembled mobile phones. He says he loves his work and that he’s content, but as I make my way out of the Blade Runner -esque fluorescent-lit maze of cramped computer shops, I get the feeling this isn’t where he’d imagined his life would end up.
The Love Bug wasn’t the smartest computer virus, nor the most disruptive, and it certainly wasn’t the most profitable. But it’s the perfect illustration of a basic truth about much of the computer crime currently plaguing society today: It’s not about the tech, it’s about the people. Twenty years later, many of the biggest hacks and manipulations carried out on the internet—the digital burglary of Sony Pictures Entertainment, the hi-tech heist of $81 million from Bangladesh Bank, the interference in the 2016 US presidential election—aren’t, at their heart, about code, software, or hardware. They’re about exploiting human frailty. The hacker’s first step is to fool people into doing things they shouldn’t. The real trick is how to convince their victims to perform such actions, and that relies on psychological acumen every bit as much as technical skill. A good hacker needs an instinctive grasp of human behavior, and a deep understanding of our desires and fears.
De Guzman was absolutely not the first person to realize this, but in naming his virus he had, almost inadvertently, come up with the greatest lure of all time. His attack succeeded and became a global menace because he hit upon the one thing sought by everyone on the planet: love.
- 📩 Want the latest on tech, science, and more? Sign up for our newsletters !
- The furious hunt for the MAGA bomber
- How to ditch those phone apps you never use—or wanted
- She helped wreck the news business. Here’s her plan to fix it
- This cobalt-free battery is good for the planet— and it actually works
- Is your chart a detective story? Or a police report ?
- ✨ Optimize your home life with our Gear team’s best picks, from robot vacuums to affordable mattresses to smart speakers
Edited extract from Crime Dot Com: From Viruses to Vote Rigging, How Hacking Went Global by Geoff White, published 12 September 2020. Reproduced by permission of Reaktion Books.
- Gravity, gizmos, and a grand theory of interstellar travel
- Meet this year’s WIRED25: People who are making things better
- How financial apps get you to spend more and question less
- Parenting in the age of the pandemic pod
- TikTok and the evolution of digital blackface
- 🏃🏽♀️ Want the best tools to get healthy? Check out our Gear team’s picks for the best fitness trackers , running gear (including shoes and socks ), and best headphones
Hemal Jhaveri
Lauren Goode
Robert Peck
Home > Learning Center > Trojans
Article's content
What is a trojan virus.
Trojans are deceptive programs that appear to perform one function, but in fact perform another, malicious function. They might be disguised as free software, videos or music, or seemingly legitimate advertisements.
The term “trojan virus” is not technically accurate; according to most definitions, trojans are not viruses. A virus is a program that spreads by attaching itself to other software, while a trojan spreads by pretending to be useful software or content. Many experts consider spyware programs, which track user activity and send logs or data back to the attacker, as a type of trojan.
Trojans can act as standalone tools for attackers, or can be a platform for other malicious activity. For example, trojan downloaders are used by attackers to deliver future payloads to a victim’s device. Trojan rootkits can be used to establish a persistent presence on a user’s device or a corporate network.
Trojan Infection Methods
Here are common ways trojans can infect computers in your corporate network:
- A user is targeted by phishing or other types of social engineering , opens an infected email attachment or clicks a link to a malicious website
- A user visits a malicious website and experiences a drive-by download pretending to be useful software, or is prompted to download a codec to play a video or audio stream
- A user visits a legitimate website infected with malicious code (for example, malvertising or cross-site scripting )
- A user downloads a program whose publisher is unknown or unauthorized by organizational security policies
- Attackers install a trojan by exploiting a software vulnerability , or through unauthorized access
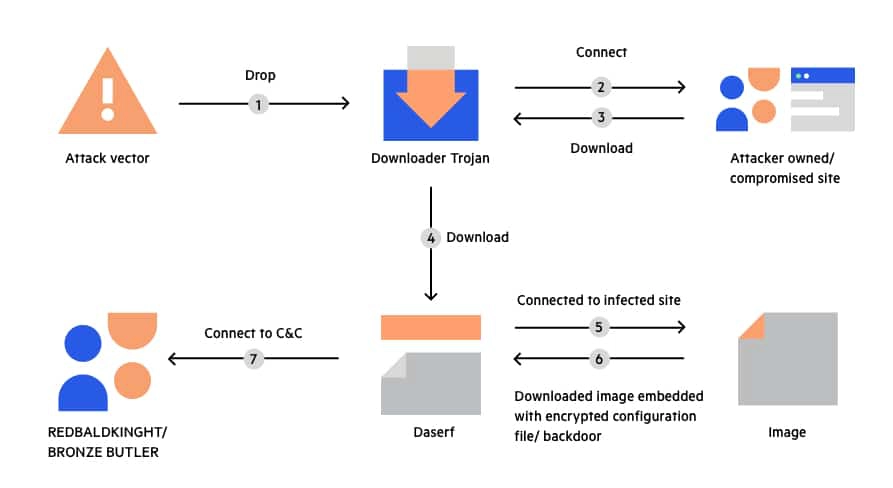
“Daserf” Trojan created by the cyber-espionage group REDBALDKNIGHT is often installed through the use of decoy documents attached in emails.
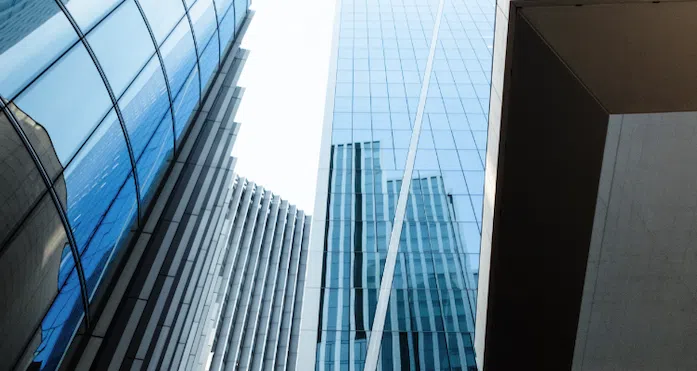
Cracking the Code: Defending APIs from Business Logic Attacks
Register Now
Types of Trojans
The first trojan was seen in the wild was ANIMAL, released in 1975. Since then, many millions of trojan variants have emerged, which may be classified into many types. Here are some of the most common types.
Downloader Trojan
A downloader trojan downloads and deploy other malicious code, such as rootkits, ransomware or keyloggers. Many types of ransomware distribute themselves via a “dropper”, a downloader trojan that installs on a user’s computer and deploys other malware components.
A dropper is often the first stage in a multi-phase trojan attack, followed by the installation of another type of trojan that provides attackers with a persistent foothold in an internal system. For example, a dropper can be used to inject a backdoor trojan into a sensitive server.
Backdoor Trojan
A backdoor trojan opens up a secret communication tunnel, allowing the local malware deployment to communicate with an attacker’s Command & Control center. It may allow hackers to control the device, monitor or steal data, and deploy other software.
Spyware is software that observes user activities, collecting sensitive data like account credentials or banking details. They send this data back to the attacker. Spyware is typically disguised as useful software, so it is generally considered as a type of trojan.
Rootkit Trojans
Rootkit trojans acquire root-level or administrative access to a machine, and boots together with the operating system, or even before the operating system. This makes them very difficult to detect and remove.
DDoS Attack Trojan (Botnet)
A DDoS trojan turns the victim’s device into a zombie participating in a larger botnet. The attacker’s objective is to harvest as many machines as possible and use them for malicious purposes without the knowledge of the device owners—typically to flood servers with fake traffic as part of a Distributed Denial of Service (DoS) attack.
Trojan Horse Malware Examples
Following are some of the fastest-spreading and most dangerous trojan families.
Zeus/Zbot is a malware package operating in a client/server model, with deployed instances calling back home to the Zeus Command & Control (C&C) center. It is estimated to have infected over 3.6 million computers in the USA, including machines owned by NASA, Bank of America and the US Department of Transportation.
Zeus infects Windows computers, and sends confidential data from the victim’s computer to the Zeus server. It is particularly effective at stealing credentials, banking details and other financial information and transmit them to the attackers.
The weak point of the Zeus system is the single C&C server, which was a primary target for law enforcement agencies. Later versions of Zeus added a domain generation algorithm (GDA), which lets Zbots connect to a list of alternative domain names if the Zeus server is not available.
Zeus has many variants, including:
- Zeus Gameover —a peer-to-peer version of the Zeus botnet without a centralized C&C.
- SpyEye —designed to steal money from online bank accounts.
- Ice IX —financial malware that can control content in a browser during a financial transaction, and extract credentials and private data from forms.
- Citadel —an open-source variant of Zeus that has been worked on and improved by a community of cybercriminals, and was succeeded by Atmos.
- Carberp —one of the most widely spread financial malware in Russia. Can exploit operating system vulnerabilities to gain root access to target systems.
- Shylock —uses a domain generation algorithm (DGA), used to receive commands from a large number of malicious servers.
ILOVEYOU (commonly referred to as the “ILOVEYOU virus”) was a trojan released in 2000, which was used in the world’s most damaging cyberattack, which caused $8.7 billion in global losses.
The trojan was distributed as a phishing email, with the text “Kindly check the attached love letter coming from me”, with an attachment named “ILOVEYOU” that appeared to be a text file. Recipients who were curious enough to open the attachment became infected, the trojan would overwrite files on the machine and then send itself to their entire contact list. This simple but effective propagation method caused the virus to spread to millions of computers.
Cryptolocker
Cryptolocker is a common form of ransomware. It distributes itself using infected email attachments; a common message contains an infected password-protected ZIP file, with the password contained in the message. When the user opens the ZIP using the password and clicks the attached PDF, the trojan is activated. It searches for files to encrypt on local drives and mapped network drives, and encrypts the files using asymmetric encryption with 1024 or 2048-bit keys. The attackers then demand a ransom to release the files.
Stuxnet was a specialized Windows Trojan designed to attack Industrial Control Systems (ICS). It was allegedly used to attack Iran’s nuclear facilities. The virus caused operator monitors to show business as usual, while it changed the speed of Iranian centrifuges, causing them to spin too long and too quickly, and destroying the equipment.
How to Detect Trojans in Your Organization
Trojans are a major threat to organizational systems and a tool commonly used as part of Advanced Persistent Threats (APT). Security teams can use the following technologies and methods to detect and prevent trojans:
Endpoint protection platforms
Modern endpoint protection systems include device traditional antivirus, next-generation antivirus (NGAV) that can prevent zero-day and unknown trojans, and behavioral analytics that identifies anomalous activity on user devices. This combination of protective measures is effective against most trojans.
Web application firewall (WAF)
A WAF is deployed at the network edge, and is able to prevent trojan infections, by preventing downloads of trojan payloads from suspicious sources. In addition, it can detect and block any unusual or suspicious network communication. WAFs can block trojans when they “phone home” to their C&C center, rendering them ineffective, and can help identify the affected systems.
Threat hunting
Threat hunting is the practice of actively searching for threats on corporate networks by skilled security analysts. Analysts use Security Information and Event Management (SIEM) systems to collect data from hundreds of IT systems and security tools, and use advanced searches and data analytics techniques to uncover traces of trojans and other threats present in the local environment.
Triaging user complaints
Often, a simple user complaint about a slow machine or strange user interface behavior could signal a trojan. Triaging IT support requests with behavioral analytics and data from other security tools can help identify hidden trojans.
The following are common symptoms of trojans which may be reported by users:
- Popups appear, launched by the user’s browser or operating system
- Disk space disappears, unexplained persistent disk errors
- Poor system performance, machine suddenly slows down with no apparent cause
- Mouse or keyboard operate on their own
- Computer shuts down or restarts with no user action
- Change to desktop image or configuration
- Change to browser homepage or start page
- Searches redirect to an unknown domain
- System firewall or antivirus turned off without user intervention
- Unusual network activity when the user is not active
- New programs, favorites or bookmarks not added by the user
Imperva Data Protection Solutions
Imperva helps detect and prevent trojans via user rights management—it monitors data access and activities of privileged users to identify excessive, inappropriate, and unused privileges. It also offers the industry’s leading web application firewall (WAF), which can detect and b lock trojans when they attempt to contact their Command & Control center.
In addition to ransomware detection and prevention, Imperva’s data security solution protects your data wherever it lives — on premises, in the cloud and hybrid environments. It also provides security and IT teams with full visibility into how the data is being accessed, used, and moved around the organization.
Our comprehensive approach relies on multiple layers of protection, including:
- Database firewall — blocks SQL injection and other threats, while evaluating for known vulnerabilities.
- Data masking and encryption — obfuscates sensitive data so it would be useless to the bad actor, even if somehow extracted.
- Data loss prevention (DLP) — inspects data in motion, at rest on servers, in cloud storage, or on endpoint devices.
- User behavior analytics — establishes baselines of data access behavior, uses machine learning to detect and alert on abnormal and potentially risky activity.
- Data discovery and classification — reveals the location, volume, and context of data on premises and in the cloud.
- Database activity monitoring — monitors relational databases, data warehouses, big data and mainframes to generate real-time alerts on policy violations.
- Alert prioritization — Imperva uses AI and machine learning technology to look across the stream of security events and prioritize the ones that matter most.
Latest Blogs
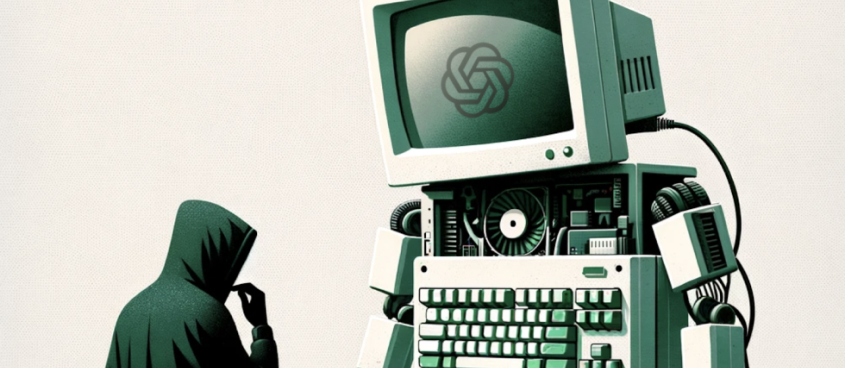
Feb 19, 2024 6 min read
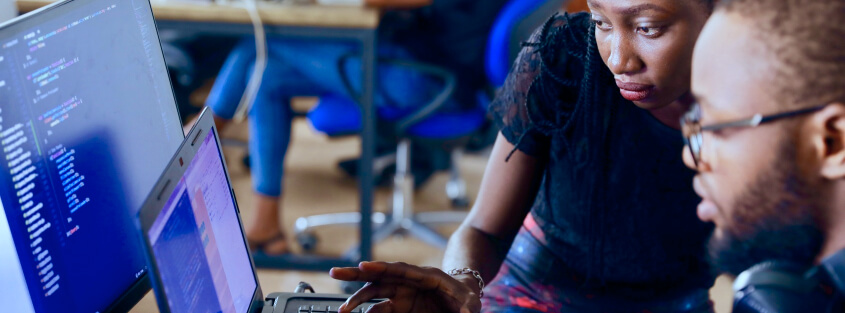
, Sofia Naer
Dec 19, 2023 2 min read
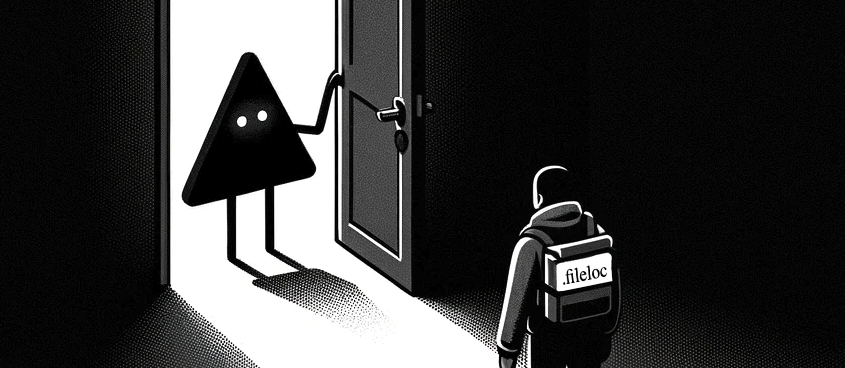
Dec 14, 2023 5 min read
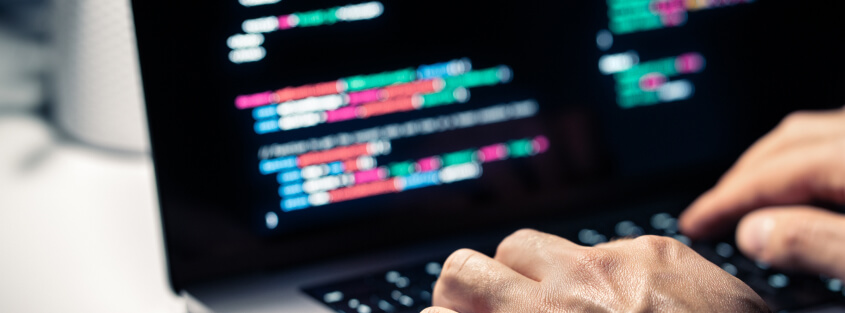
Daniel Johnston
Dec 14, 2023 3 min read
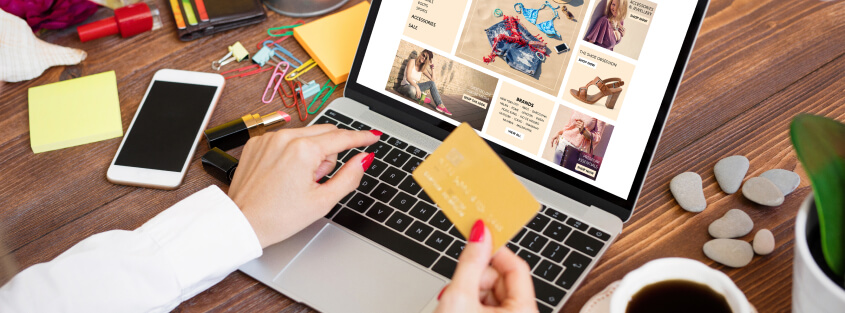
- Imperva Threat Research

Erez Hasson
, Gabi Stapel
Nov 8, 2023 13 min read
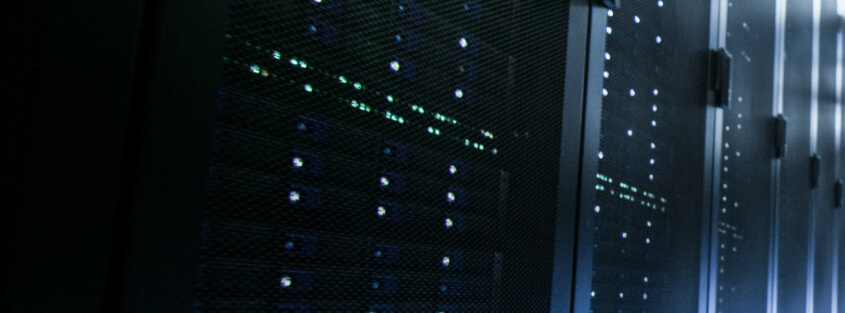
Oct 24, 2023 3 min read
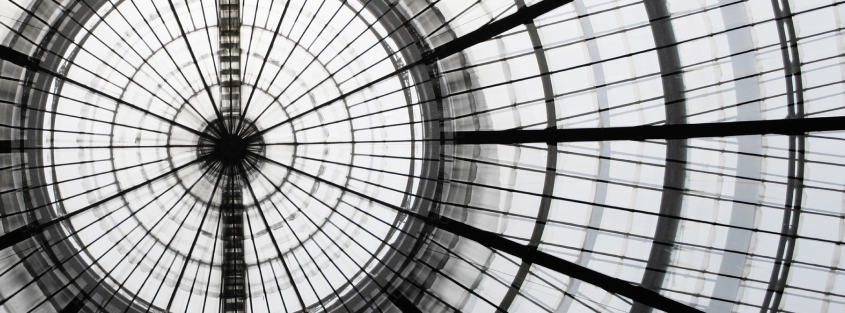
Oct 19, 2023 7 min read
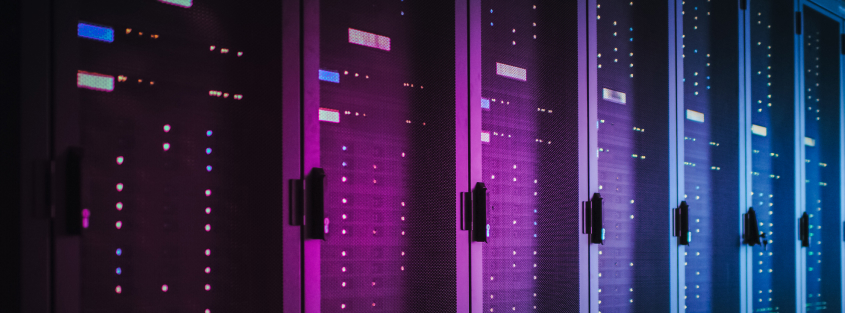
Kunal Anand
, Nadav Avital
Oct 10, 2023 1 min read
Latest Articles
- Attack Types
709.3k Views
627.5k Views
460.1k Views
327.1k Views
308.9k Views
281.2k Views
277.1k Views
254.5k Views
Protect Against Business Logic Abuse
Identify key capabilities to prevent attacks targeting your business logic
The 10th Annual Bad Bot Report
The evolution of malicious automation over the last decade
The State of Security Within eCommerce in 2022
Learn how automated threats and API attacks on retailers are increasing
Prevoty is now part of the Imperva Runtime Protection
Protection against zero-day attacks
No tuning, highly-accurate out-of-the-box
Effective against OWASP top 10 vulnerabilities
An Imperva security specialist will contact you shortly.
Top 3 US Retailer
- GTA 5 Cheats
- Print on a Chromebook
- Nothing Phone 2 Review
- Best YouTube TV Alternatives
- Asus ROG Ally vs. Steam Deck
- Gameshare on Nintendo Switch
‘Most Dangerous Trojan Virus Ever’ Steals Over $1 Million

The thefts were discovered after M86 gained access to the command-and-control server in the Eastern Europe country of Moldova. As for the money, M86 could not give an exact location for where it was going, other than to suggest that it was heading into the former Soviet states- which likely meant that the money was going to the Russian mafia, or another Eastern European gang.
“We’ve never seen such a sophisticated and dangerous threat. Always check your balance and have a good idea of what it is.” M86 said in a security report released on Tuesday.
- Laptop with some of world’s most dangerous malware sells for $1.35 million
The Zeus Trojan, also known as Zbot has infected more than 37,000 computers in the UK through a drive-by download. Users visiting a compromised site would unknowingly receive the virus as a cookie, hidden as part of a legitimate ad on potentially any website.
Once the virus is on the computer, it waits until the user goes to their bank’s website — it is only one specific bank that has yet to be named as the investigation is ongoing — and then it intercepts password information before it can be encrypted. Once the trojan has access to the account, it checks to make sure that there is at least £800 available, then it begins to transfer anywhere up to £5,000 to various bank accounts. Once the transfer is complete, the virus then creates a false electronic statement to conceal the missing funds.
Most, if not all, of the victims will have their money reimbursed by the bank.
“In the vast majority of cases, if people had kept their computer’s operating systems and software such as Internet Explorer up to date they would not have been attacked,” Ed Rowley, product manager at M86 said, according to the Daily Mail .
“More often than not Trojans exploit known vulnerabilities that can be simply patched and fixed by downloading updates.”
Earlier this month, the UK based security group, Trusteer warned that more than 100,000 computers may have been infected with the Zeus Trojan, meaning that this is far from over.
Editors' Recommendations
- Hacker steals 1 billion people’s records in unprecedented data breach
HP is one of the best laptop brands, so if you need a new device, you're going to want to take advantage of the offers in HP's laptop sale. Prices start at $220, and you can enjoy savings of as much as $500, but only if you hurry because we're not sure how much time is remaining before these bargains get taken down. Whether you spot something interesting among our recommendations below, or you want to check out everything that's part of the sale, you need to complete your purchase as soon as possible to make sure that you get an HP laptop for cheaper than usual.
What to buy in HP's laptop sale
When it comes to computer brands, few names are as well-known and respected as Dell and HP. Both companies have been churning out popular laptops for decades, with their catalogs now filled with iconic lineups like the Dell XPS 15 and HP Pavilion. Both HP and Dell have a lot to offer -- and because they sell so many products vying for the same niche, it can be difficult to determine which one is best for your lifestyle.
Whether you're looking for a premium gaming laptop or something budget-friendly for a job or small business, you'll find that both Dell and HP have a product that fit your needs. The two catalogs feature products with a variety of processors, graphics cards, and price tags, though there are some key differences you should know about the brands before making a purchase.
You’ve just bought a Meta Quest 3 and had your mind blown by how awesome games are in this VR headset that has finally demonstrated what we’ve all been waiting for. While you could stop there, it’s a smart move to buy some of the best Quest 3 accessories too. That way, you’ll get the most out of your coveted VR headset. We’ve picked out some of the very best Meta Quest 3 accessories to help you out now you’ve picked up one of the Meta Quest 3 deals going on at the moment. Here’s what we recommend. Meta Quest 3 Elite Strap Best strap
An ergonomic strap that fits most head sizes with a simple turn of a dial, the Meta Quest 3 Elite Strap just made everything comfier. The lightweight strap helps reduce facial pressure while evenly distributing weight so it feels infinitely better to wear. There’s a soft and flexible silicone support that cradles the back of your head while a flexible top strap helps you make sure the headset fits over your eyes appropriately.
Malware found on laptops given out by government
- Published 22 January 2021
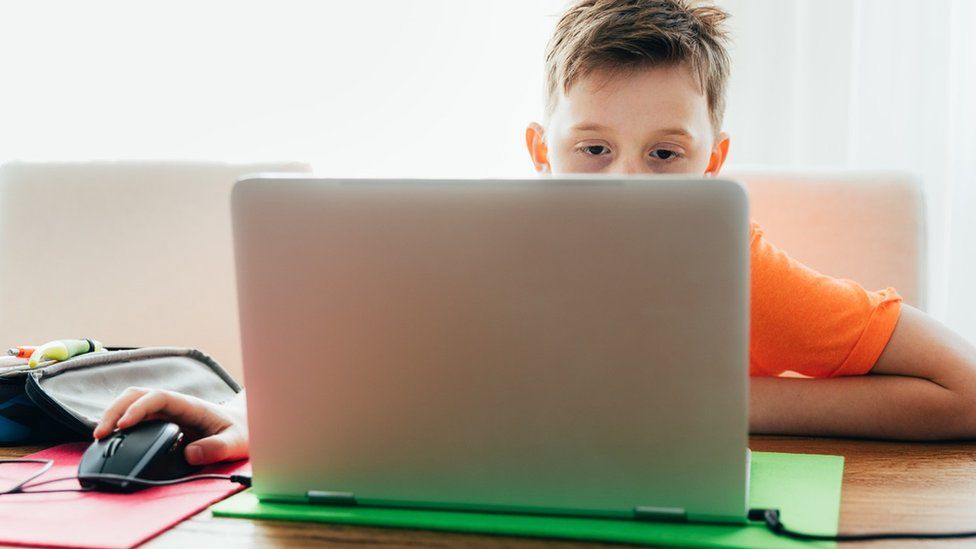
Some of the laptops given out in England to support vulnerable children home-schooling during lockdown contain malware, BBC News has learned.
Teachers shared details on an online forum about suspicious files found on devices sent to a Bradford school.
The malware, which they said appeared to be contacting Russian servers, is believed to have been found on laptops given to a handful of schools.
The Department for Education said it was aware and urgently investigating.
- Can you get a free laptop or cheap broadband?
- Covid 19: The huge challenges of home-schooling
A DfE official told BBC News: "We are aware of an issue with a small number of devices. And we are investigating as an urgent priority to resolve the matter as soon as possible.
"DfE IT teams are in touch with those who have reported this issue."
"We believe this is not widespread."
Geo, the firm which made the laptops, told the BBC: "We have been working closely with the Department for Education regarding a reported issue on a very small number of devices. We are providing our full support during their investigation.
"We take all matters of security extremely seriously. Any schools that have concerns should contact the Department for Education."
According to the forum, the Windows laptops contained Gamarue.I, a worm identified by Microsoft in 2012.
The government has so far sent schools more than 800,000 laptops, as it tries to distribute more than a million devices to disadvantaged pupils who may not have access at home.
"Upon unboxing and preparing them, it was discovered that a number of the laptops were infected with a self-propagating network worm," wrote Marium Haque, deputy director of Education and Learning at Bradford Council.
She recommended that schools also check their networks "as an added precaution".
Information security consultant Paul Moore told the BBC that the Gamarue worm "presents a very severe threat to any PC or network".
"Ideally users should reboot into safe mode and run a full scan with an anti-virus product," he said.
"However with this type of malware, it is advisable to seek professional assistance in order to ensure it has been correctly removed."
The malware in question installs spyware which can gather information about browsing habits, as well as harvest personal information such as banking details.
"The fact that these devices were not checked and scrubbed before being sent to vulnerable children is a concern," said George Glass, head of threat intelligence at security firm Redscan.
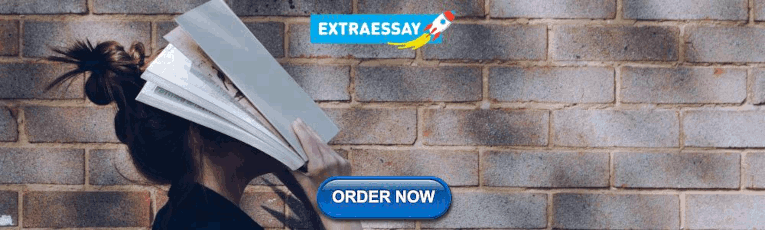
The huge challenges of home-schooling
- Published 11 January 2021
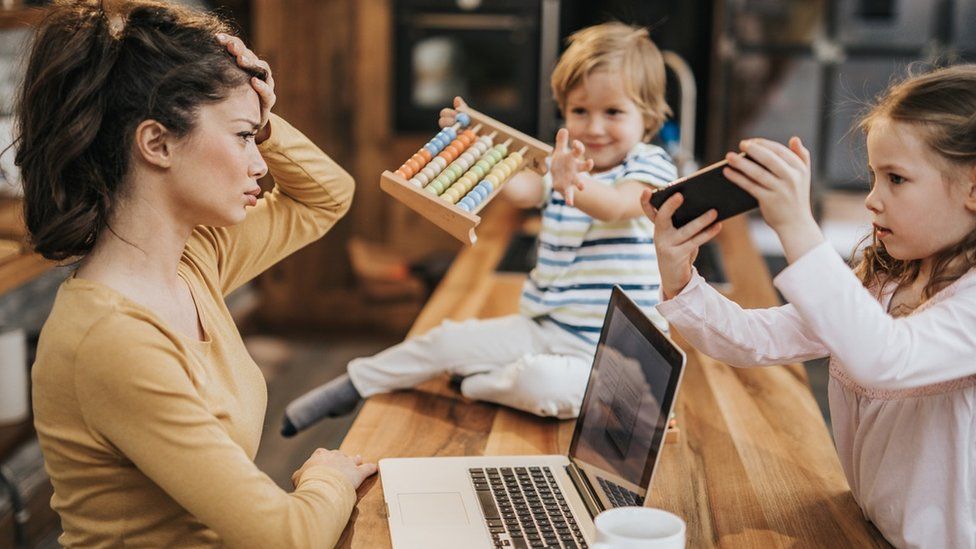
Home-school: Can you get a free laptop or cheaper broadband?
- Published 10 February 2021
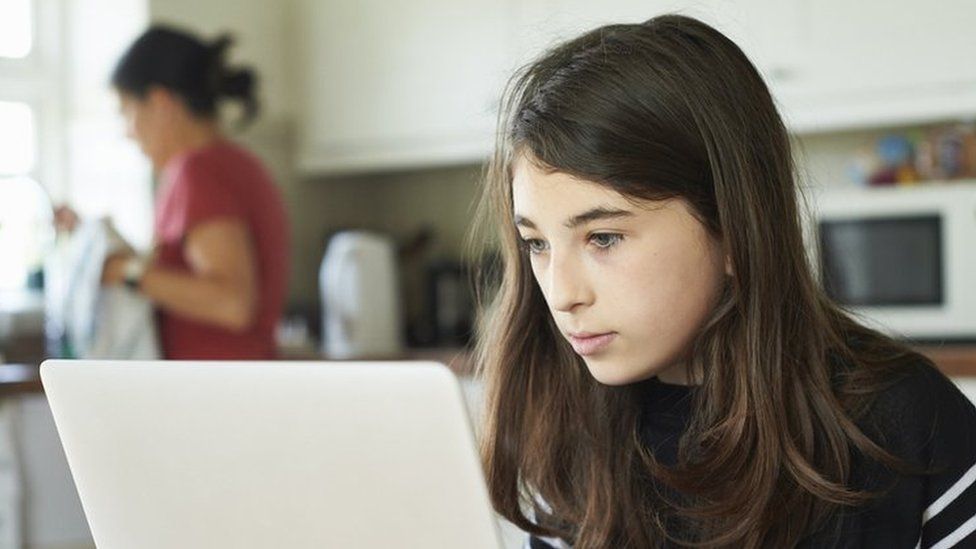
Trojan Horse Virus
- Download Ebook - Use AI to Detect Malware

What Is a Trojan Horse Virus?
A Trojan Horse Virus is a type of malware that downloads onto a computer disguised as a legitimate program. The delivery method typically sees an attacker use social engineering to hide malicious code within legitimate software to try and gain users' system access with their software.
A simple way to answer the question " what is Trojan " is it is a type of malware that typically gets hidden as an attachment in an email or a free-to-download file, then transfers onto the user’s device. Once downloaded, the malicious code will execute the task the attacker designed it for, such as gain backdoor access to corporate systems, spy on users’ online activity, or steal sensitive data.
Indications of a Trojan being active on a device include unusual activity such as computer settings being changed unexpectedly.
History of the Trojan Horse
The original story of the Trojan horse can be found in the Aeneid by Virgil and the Odyssey by Homer. In the story, the enemies of the city of Troy were able to get inside the city gates using a horse they pretended was a gift. The soldiers hid inside the huge wooden horse and once inside, they climbed out and let the other soldiers in.
There are a few elements of the story that make the term “Trojan horse” an appropriate name for these types of cyber attacks :
- The Trojan horse was a unique solution to the target’s defenses. In the original story, the attackers had laid siege to the city for 10 years and hadn’t succeeded in defeating it. The Trojan horse gave them the access they had been wanting for a decade. A Trojan virus, similarly, can be a good way to get behind an otherwise tight set of defenses.
- The Trojan horse appeared to be a legitimate gift. In a similar vein, a Trojan virus looks like legitimate software.
- The soldiers in the Trojan horse controlled the city’s defense system. With a Trojan virus, the malware takes control of your computer, potentially leaving it vulnerable to other “invaders.”

How Do Trojans Work?
Unlike computer viruses , a Trojan horse cannot manifest by itself, so it needs a user to download the server side of the application for it to work. This means the executable (.exe) file should be implemented and the program installed for the Trojan to attack a device’s system.
A Trojan virus spreads through legitimate-looking emails and files attached to emails, which are spammed to reach the inboxes of as many people as possible. When the email is opened and the malicious attachment is downloaded, the Trojan server will install and automatically run every time the infected device is turned on.
Devices can also be infected by a Trojan through social engineering tactics, which cyber criminals use to coerce users into downloading a malicious application. The malicious file could be hidden in banner advertisements, pop-up advertisements, or links on websites.
A computer infected by Trojan malware can also spread it to other computers. A cyber criminal turns the device into a zombie computer, which means they have remote control of it without the user knowing. Hackers can then use the zombie computer to continue sharing malware across a network of devices, known as a botnet.
For example, a user might receive an email from someone they know, which includes an attachment that also looks legitimate. However, the attachment contains malicious code that executes and installs the Trojan on their device. The user often will not know anything untoward has occurred, as their computer may continue to work normally with no signs of it having been infected.
The malware will reside undetected until the user takes a certain action, such as visiting a certain website or banking app. This will activate the malicious code, and the Trojan will carry out the hacker’s desired action. Depending on the type of Trojan and how it was created, the malware may delete itself, return to being dormant, or remain active on the device.
Trojans can also attack and infect smartphones and tablets using a strand of mobile malware. This could occur through the attacker redirecting traffic to a device connected to a Wi-Fi network and then using it to launch cyberattacks.
Most Common Types of Trojan Malware
There are many types of Trojan horse viruses that cyber criminals use to carry out different actions and different attack methods. The most common types of Trojan used include:
- Backdoor Trojan : A backdoor Trojan enables an attacker to gain remote access to a computer and take control of it using a backdoor. This enables the malicious actor to do whatever they want on the device, such as deleting files, rebooting the computer, stealing data, or uploading malware. A backdoor Trojan is frequently used to create a botnet through a network of zombie computers.
- Banker Trojan : A banker Trojan is designed to target users’ banking accounts and financial information. It attempts to steal account data for credit and debit cards, e-payment systems, and online banking systems.
- Distributed denial-of-service (DDoS) Trojan : These Trojan programs carry out attacks that overload a network with traffic. It will send multiple requests from a computer or a group of computers to overwhelm a target web address and cause a denial of service.
- Downloader Trojan : A downloader Trojan targets a computer that has already been infected by malware, then downloads and installs more malicious programs to it. This could be additional Trojans or other types of malware like adware .
- Exploit Trojan : An exploit malware program contains code or data that takes advantage of specific vulnerabilities within an application or computer system. The cyber criminal will target users through a method like a phishing attack, then use the code in the program to exploit a known vulnerability.
- Fake antivirus Trojan : A fake antivirus Trojan simulates the actions of legitimate antivirus software. The Trojan is designed to detect and remove threats like a regular antivirus program, then extort money from users for removing threats that may be nonexistent.
- Game-thief Trojan : A game-thief Trojan is specifically designed to steal user account information from people playing online games.
- Instant messaging (IM) Trojan : This type of Trojan targets IM services to steal users’ logins and passwords. It targets popular messaging platforms such as AOL Instant Messenger, ICQ, MSN Messenger, Skype, and Yahoo Pager.
- Infostealer Trojan : This malware can either be used to install Trojans or prevent the user from detecting the existence of a malicious program. The components of infostealer Trojans can make it difficult for antivirus systems to discover them in scans.
- Mailfinder Trojan : A mailfinder Trojan aims to harvest and steal email addresses that have been stored on a computer.
- Ransom Trojan : Ransom Trojans seek to impair a computer’s performance or block data on the device so that the user can no longer access or use it. The attacker will then hold the user or organization ransom until they pay a ransom fee to undo the device damage or unlock the affected data.
- Remote access Trojan : Similar to a backdoor Trojan, this strand of malware gives the attacker full control of a user’s computer. The cyber criminal maintains access to the device through a remote network connection, which they use to steal information or spy on a user.
- Rootkit Trojan : A rootkit is a type of malware that conceals itself on a user’s computer. Its purpose is to stop malicious programs from being detected, which enables malware to remain active on an infected computer for a longer period.
- Short message service (SMS) Trojan : An SMS Trojan infects mobile devices and is capable of sending and intercepting text messages. This includes sending messages to premium-rate phone numbers, which increases the costs on a user’s phone bill.
- Spy Trojan : Spy Trojans are designed to sit on a user’s computer and spy on their activity. This includes logging their keyboard actions, taking screenshots, accessing the applications they use, and tracking login data.
- SUNBURST : The SUNBURST trojan virus was released on numerous SolarWinds Orion Platform. Victims were compromised by trojanized versions of a legitimate SolarWinds digitally signed file named: SolarWinds.Orion.Core.BusinessLayer.dll. The trojanized file is a backdoor. Once on a target machine, it remains dormant for a two-week period and will then retrieve commands that allow it to transfer, execute, perform reconnaissance, reboot and halt system services. Communication occurs over http to predetermined URI's.
How To Recognize a Trojan Virus
A Trojan horse virus can often remain on a device for months without the user knowing their computer has been infected. However, telltale signs of the presence of a Trojan include computer settings suddenly changing, a loss in computer performance, or unusual activity taking place. The best way to recognize a Trojan is to search a device using a Trojan scanner or malware-removal software.
How To Protect Yourself from Trojan Viruses
Examples of trojan horse virus attacks.
Trojan attacks have been responsible for causing major damage by infecting computers and stealing user data. Well-known examples of Trojans include:
- Rakhni Trojan: The Rakhni Trojan delivers ransomware or a cryptojacker tool—which enables an attacker to use a device to mine cryptocurrency—to infect devices.
- Tiny Banker: Tiny Banker enables hackers to steal users’ financial details. It was discovered when it infected at least 20 U.S. banks.
- Zeus or Zbot: Zeus is a toolkit that targets financial services and enables hackers to build their own Trojan malware. The source code uses techniques like form grabbing and keystroke logging to steal user credentials and financial details.

Ransomware Hub
Ransomware is a leading cyberthreat to corporate, government, and personal cybersecurity. Learn how Fortinet protects your organization against ransomware and related cyber threats.
Fortinet's ransomware hub introduces you to the world of protection that Fortinet products and services brings to your organization.
Quick Links

Free Product Demo
Explore key features and capabilities, and experience user interfaces.

Resource Center
Download from a wide range of educational material and documents.

Free Trials
Test our products and solutions.

Contact Sales
Have a question? We're here to help.

An official website of the United States government
The .gov means it’s official. Federal government websites often end in .gov or .mil. Before sharing sensitive information, make sure you’re on a federal government site.
The site is secure. The https:// ensures that you are connecting to the official website and that any information you provide is encrypted and transmitted securely.
- Publications
- Account settings
Preview improvements coming to the PMC website in October 2024. Learn More or Try it out now .
- Advanced Search
- Journal List
- Elsevier - PMC COVID-19 Collection

Ransomware: Recent advances, analysis, challenges and future research directions
Craig beaman.
a Canadian Institute for Cybersecurity, Faculty of Computer Science, University of New Brunswick, Canada
Ashley Barkworth
Toluwalope david akande, saqib hakak, muhammad khurram khan.
b Center of Excellence in Information Assurance, College of Computer and Information Sciences, King Saud University, Riyadh 11653, Saudi Arabia
The COVID-19 pandemic has witnessed a huge surge in the number of ransomware attacks. Different institutions such as healthcare, financial, and government have been targeted. There can be numerous reasons for such a sudden rise in attacks, but it appears working remotely in home-based environments (which is less secure compared to traditional institutional networks) could be one of the reasons. Cybercriminals are constantly exploring different approaches like social engineering attacks, such as phishing attacks, to spread ransomware. Hence, in this paper, we explored recent advances in ransomware prevention and detection and highlighted future research challenges and directions. We also carried out an analysis of a few popular ransomware samples and developed our own experimental ransomware, AESthetic, that was able to evade detection against eight popular antivirus programs.
1. Introduction
The COVID-19 pandemic has led to an increase in the rate of cyberattacks. As the workplace paradigm shifted to home-based scenarios—resulting in weaker security controls—attackers lured people through COVID-19 themed ransomware phishing emails. For example, many phishing campaigns prompted users to click on specific links to get sensitive information related to a COVID-19 vaccine, shortage of surgical masks, etc. Attackers made good use of fake COVID-19 related information as a hook to launch more successful phishing campaigns. Higher levels of unemployment can be another factor that motivates people towards cybercrime, such as launching ransomware attacks and disrupting critical IT services, in order to support themselves ( Lallie et al., 2020 ).
Cyber extortion methods have existed since the 1980s. The first ransomware sample dates back to 1989 with the PC Cyborg Trojan ( Tailor and Patel, 2017 ). After the target computer was restarted 90 times, PC Cyborg hid directories and encrypted the names of all files on the C drive, rendering the system unusable. In the 1990s and early 2000s, ransomware attacks were mostly carried out by hobbyist hackers who aimed to gain notoriety through cyber pranks and vandalism ( Srinivasan, 2017 ). Modern ransomware emerged around 2005 and quickly became a viable business strategy for attackers ( Richardson, North, 2017 , Wilner, Jeffery, Lalor, Matthews, Robinson, Rosolska, Yorgoro, 2019 ). Targets shifted from individuals to companies and organizations in order to fetch larger ransoms ( Muslim et al., 2019 ). The following industries were particularly targeted: transportation, healthcare, financial services, and government ( Alshaikh et al., 2020 ). The number of ransomware attacks has grown exponentially thanks to easily obtainable ransomware toolkits and ransomware-as-a-service (RaaS) that allows novices to launch ransomware attacks ( Sharmeen et al., 2020 ).
Ransomware is a type of malware designed to facilitate different nefarious activities, such as preventing access to personal data unless a ransom is paid ( Khammas, 2020 , Komatwar, Kokare, 2020 , Meland, Bayoumy, Sindre, 2020 ). This ransom typically uses cryptocurrency like Bitcoin, which makes it difficult to track the recipient of the transaction and is ideal for attackers to evade law enforcement agencies ( Kara, Aydos, 2020 , Karapapas, Pittaras, Fotiou, Polyzos, 2020 ). There has been a surge in ransomware attacks in the past few years. For example, during the ongoing COVID-19 pandemic, an Android app called CovidLock was developed to monitor heat map visuals and statistics on COVID-19 ( Saeed, 2020 ). The application tricked users by locking user contacts, pictures, videos, and access to social media accounts as soon as they installed it. To regain access, users were asked to pay some ransom in Bitcoin; otherwise, their data was made public ( Hakak et al., 2020c ). Another notorious example of ransomware is the WannaCry worm, which spread rapidly across many computer networks in May 2017 ( Akbanov, Vassilakis, Logothetis, 2019 , Mackenzie, 2019 ). Within days, it had infected over 200,000 computers spanning across 150 countries ( Mattei, 2017 ). Hospitals across the U.K. were knocked offline ( Chen and Bridges, 2017 ); government systems, railway networks, and private companies were affected as well ( Cosic et al., 2019 ).
Ransomware can be categorized into three main forms - locker, crypto, and scareware ( Gomez-Hernandez, Alvarez-Gonzalez, Garcia-Teodoro, 2018 , Kok, Abdullah, Jhanjhi, Supramaniam, 2019 ) - as shown in Fig. 1 . Scareware may use pop-up ads to manipulate users into assuming that they are required to download certain software, thereby using coercion techniques for downloading malware. In scareware, the cyber crooks exploit the fear rather than lock the device or encrypt any data ( Andronio et al., 2015 ). This form of ransomware does not do any harm to the victim’s computer. The aim of locker ransomware is to block primary computer functions. Locker ransomware may encrypt certain files which can lock the computer screen and/or keyboard, but it is generally easy to overcome and can often be resolved by rebooting the computer in safe mode or running an on-demand virus scanner ( Adamu and Awan, 2019 ). Locker ransomware may allow limited user access. Crypto ransomware encrypts the user’s sensitive files but does not interfere with basic computer functions. Unlike locker ransomware, crypto ransomware is often irreversible as current encryption techniques (e.g., AES and RSA) are nearly impossible to revert if implemented properly ( Gomez-Hernandez, Alvarez-Gonzalez, Garcia-Teodoro, 2018 , Nadir, Bakhshi, 2018 ). Table 1 presents a few popular ransomware families. Crypto ransomware can use one of three encryption schemes: symmetric, asymmetric, or hybrid ( Cicala and Bertino, 2020 ). A purely symmetric approach is problematic as the encryption key must be embedded in the ransomware ( Dargahi et al., 2019 ). This makes this approach vulnerable to reverse engineering. The second approach is to use asymmetric encryption. The issue with this approach is that asymmetric encryption is slow compared to symmetric encryption and hence struggles to encrypt larger files ( Bajpai et al., 2018 ).

Categories of ransomware ( Andronio et al., 2015 ).
List of popular ransomware strains.
The most effective approach (i.e., the hardest to decrypt) is hybrid encryption, which uses both symmetric and asymmetric encryption. An overview of the hybrid approach is given in Fig. 2 . For hybrid encryption, the first step is to create a random symmetric key. The ransomware usually creates this key by calling a cryptographic API on the user’s operating system ( Zimba et al., 2019 ). The symmetric key encrypts the victim’s files as the ransomware traverses through the file system. Once all files are encrypted, a public-private key pair is generated by a command and control (C&C) server which the ransomware connects to. The public key is sent to the ransomware and is used to encrypt the symmetric key, while the private key is held by the C&C server. The plaintext version of the symmetric key is then deleted to ensure that the victim cannot use it to recover their files. Instructions for how to pay the ransom are left for the victim. If the ransom is paid, then the decryption process will begin. Decryption starts by requesting the private key from the C&C server. Once obtained, the private key is used to decrypt the symmetric key. Finally, the symmetric key is used to recover the victim’s files. Generally, a unique public-private key pair is generated for each new ransomware infection; this prevents victims from sharing private keys with other victims to enable them to recover the symmetric key.

The typical steps used by ransomware to encrypt and decrypt a user’s data. This illustrates a hybrid approach where both symmetric and asymmetric cryptography are used.
Ransomware attacks can cause significant financial damage, reduce productivity, disrupt normal business operations, and harm the reputations of individuals or companies ( Jain, Rani, 2020 , Zhang-Kennedy, Assal, Rocheleau, Mohamed, Baig, Chiasson, 2018 ). The global survey ‘The State of Ransomware 2021’ commissioned by Sophos announced in its findings that, among roughly 2000 respondents whose organizations had been hit by a ransomware attack, the average total cost to an organization to rectify the impacts of a ransomware attack (considering downtime, people time, device cost, network cost, lost opportunity, ransom paid etc.) was US$1.85 million, which is more than double the US$761,106 cost reported in 2020 ( ran, 2021 ). These attacks may also result in a permanent loss of information or files. Paying the ransom does not guarantee that the locked system or files will be released ( for Cyber Security, 2018 ). For companies who pay the ransom, the cost of recovering from the attack doubles on average ( Ltd., 2020 ). By the end of the year 2021, ransomware attacks are expected to cost the world $20 billion, up from $325 million in 2015 ( Alshaikh et al., 2020 ). These attacks have been particularly devastating since the COVID-19 pandemic and started by targeting hospitals, vaccine research labs, and contact tracing apps ( Pranggono and Arabo, 2020 ). From all these statistics, it is clear that we need to understand the behaviour of ransomware and its variants to effectively detect and mitigate future attacks. Due to its profitability, new variants of ransomware continue to emerge that circumvent traditional antivirus applications and other detection methods. Hence, it is critical to come up with a new generation of efficient countermeasures.
There is an emerging need to highlight the recent advancements in the area of ransomware. The contribution of this paper is as follows:
- • Recent state-of-the-art ransomware detection and prevention approaches are presented.
- • Different ransomware samples are tested in a virtual environment.
- • A new experimental ransomware known as AESthetic is proposed and tested on eight popular antivirus programs.
- • The effectiveness of a few popular ransomware countermeasures on implemented ransomware samples is analyzed.
- • Future research challenges and directions are identified and elaborated on.
The rest of the article is organized as follows. Section 2 surveys the recent literature on ransomware detection and prevention approaches. Section 3 presents our new ransomware sample, AESthetic, and the experimental test-bed setup along with in-depth analysis. A discussion of our literature survey and test results is in Section 4 . Section 5 highlights future research challenges and directions. Finally, Section 6 concludes the article.
2. Literature review
Before our own survey, we searched for and identified relevant surveys on ransomware and summarized their contributions in Table 2 . Most existing surveys were outdated and focused on papers from 2014 to 2017. Hence, for our own literature review, we sourced papers on ransomware solutions from 2017 onwards. The papers came from the following article databases: IEEE Xplore, ACM, Science Direct, and Springer. Our searches were made using combinations of the following keywords: ‘ransomware detection’, ‘ransomware prevention’, ‘crypto-ransomware’, ‘malware detection’, ‘key backup’, ‘data backup’, ‘access control’, ‘honeypots’, ‘machine learning’, and ‘intrusion/anomaly detection’. We categorized the surveyed papers into ransomware prevention and detection approaches. Most of the existing works within these two categories involved the preliminary step of malware analysis, which is explained below:
Existing review studies.
2.1. Malware analysis
Malware analysis is a standard approach to understand the components and behaviour of malware, ransomware included. This analysis is useful to detect malware attacks and prevent similar attacks in the future. Malware analysis is broadly categorized into static and dynamic analysis. Static analysis analyzes binary file contents, whereas dynamic analysis studies the behaviour and actions of a process during execution ( Or-Meir, Nissim, Elovici, Rokach, 2019 , Sharafaldin, Lashkari, Hakak, Ghorbani, 2019 , Shijo, Salim, 2015 ).
Signature-based malware detection is a static analysis approach that uses the unique patterns within the malicious file in order to detect it. For ransomware, this includes the unique sequences of bytes within the binary file, the order of function calls, or the analysis of ransomware notes ( Alshaikh, Nagy, Hefny, 2020 , Aslan, Samet, 2020 , Nahmias, Cohen, Nissim, Elovici, 2020 ). The signature can then be checked against the signatures of known malware samples. The main advantages of signature-based detection are that it is fast and has a low false-positive rate; for these reasons, signature-based detection is very popular. However, if malware is concealed through code obfuscation techniques like binary packing, then it may evade detection ( Khan et al., 2020 ). Dynamic analysis is less susceptible to these evasion techniques because, unlike static analysis, it does not rely on analyzing the binary code itself and instead looks for meaningful patterns or signatures that imply the maliciousness of the analyzed file ( Or-Meir et al., 2019 ). Additionally, signature-based approaches will fail against newly created malware ( Aghakhani, Gritti, Mecca, Lindorfer, Ortolani, Balzarotti, Vigna, Kruegel, 2020 , Kok, Abdullah, Jhanjhi, Supramaniam, 2019 ).
Analysis can reveal some of the steps ransomware takes to infect a user’s computer. For example, Bajpai and Enbody ( Bajpai and Enbody, 2020a ) performed static and dynamic analysis on decompiled .NET ransomware samples and found that .NET ransomware first attempts to gain execution privileges and then contacts a C&C server to obtain the encryption key. Zimba and Mulenga ( Zimba and Mulenga, 2018 ) examined the static and behavioural properties of WannaCry ransomware; they discovered that WannaCry retrieves the network adapter properties to determine whether it’s residing in a private or public subnet in order to effectuate substantial network propagation and subsequent damage. Malware analysis can discover the unique characteristics of ransomware which can then be used to help design prevention or detection mechanisms.
2.2. Recent advances in ransomware research
As mentioned previously, most existing studies have analyzed the nature of malware. Based on their analysis, they have proposed different approaches to prevent or detect ransomware. We have classified the existing studies based on their goal, which is to either prevent ransomware infection or to detect ransomware once it has infected the system. A classification diagram of the utilized tools from the reviewed studies can be found in Fig. 3 .

An overview of the utilized tools observed in literature for both ransomware prevention/mitigation and detection.
2.2.1. Ransomware prevention approaches
Preventative solutions aim to block, mitigate, or reverse the damage done by ransomware. Common preventative approaches include: enforcing strict access control, storing data and/or key backups, and increasing user awareness and training. Raising user awareness of ransomware attacks and training users on how to avoid them can prevent attacks before they occur. A summary of the utilized tools found to be used in the surveyed literature on ransomware prevention can be found in Table 3 .
Overview of surveyed literature on ransomware prevention.
Access Control
Access control prevents ransomware encryption by restricting access to the file system.
Parkinson Parkinson (2017) examined how to use built-in security controls to prevent ransomware from executing in the host computer via elevated privileges. One way that ransomware gains access to files is through a user’s credentials if the user has a high level of permissions. He proposed implementing least privilege and separation of duties through role-based access control; restricting data access as far up the directory hierarchy as possible; and routinely auditing permissions and roles.
Kim and Lee Kim and Lee (2020) proposed an access control list that whitelists specific programs for each file type. Only whitelisted programs are allowed to access files. This implicitly blocks malicious processes from accessing and encrypting files. Whereas a blacklist cannot stop ransomware that it does not contain a code signature for, a whitelist can effectively block new and unknown ransomware.
Ami et al. Ami et al. (2018) developed a solution known as AntiBotics containing three key components: a policy enforcement driver, a policy specification interface, and a challenge-response. This program makes use of both biometric authentication (e.g., a fingerprint) and human response (e.g., CAPTCHA) to prevent the deletion or modification of data. AntiBotics enforces access control by presenting periodic identification challenges. This program assigns access permissions to executable objects based on a rule specified by an administrator as well as the feedback of the challenges presented upon attempts to modify or delete files. One of this program’s limitations is that it is only tested on Windows OS. Also, although modern ransomware failed to evade AntiBotics , it’s possible that future ransomware could adapt to AntiBotics . For example, ransomware could avoid AntiBotics by injecting itself into a permitted process while waiting until the process is granted permission. A case where ransomware may attempt to rename a protected folder and conceal itself may arise, but AntiBotics can block such a process by presenting a challenge when a rename operation is carried out.
McIntosh et al. McIntosh et al. (2021) proposed a framework that enables access control decision making to a filesystem to be deferred when required, in order to observe the consequence of such an access request to the file system and to roll back changes if required. The authors suggested that their framework could be applied to implement a malware-resilient file system and potentionally deter ransomware attacks. They demonstrated the practicality of their framework through a prototype testing, capturing relevant ransomware situations. The experimental results against a large ransomware dataset showed that their framework can be effectively applied in practice.
Genç et al. Genç et al. (2018) developed an access control mechanism with the insight that without access to true randomness, ransomware relies on the pseudo random number generators that modern operating systems make available to applications in order to generate keys. They proposed a strategy to mitigate ransomware attacks that considers pseudo random number generator functions as critical resources, controls accesses on their APIs, and stops unauthorized applications that call them. Their strategy was tested against 524 active real-world ransomware samples and stopped 94% of them, including WannaCry, Locky, CryptoLocker, CryptoWall, and NotPetya samples.
Data Backup
Keeping regular backups of the data stored on a computer or network can greatly minimize the impact of ransomware. Instead, the damage is simply limited to any data that has been created since the last backup. There is overhead in backing up large amounts of data, and so choosing how often backups should be taken and how long they will be kept are important decisions to be made.
Huang et al. Huang et al. (2017) proposed a solution called FlashGuard that does not rely on software at all. Instead, it uses the fact that Solid State Drives (SSD) don’t overwrite data right away - a garbage collector does this after a while. The authors modified SSD firmware so the garbage collector doesn’t remove data as quickly, and hence lost data can be restored. When tested against ransomware samples, FlashGuard successfully recovered encrypted data with little impact on SSD performance and life span.
Thomas and Galligher Thomas and Galligher (2018) conducted a literature review of the ransomware process, functional backup architecture paradigms, and the ability of backups to address ransomware attacks. They also provided suggestions to improve the information security risk assessments to better address ransomware threats, and presented a new tool for conducting backup system evaluations during information security risk assessments that enables auditors to effectively analyze backup systems and improve and organization’s ability to combat and recover from a ransomware attack.
Min et al. Min et al. (2018) proposed Amoeba, an autonomous backup and recovery SSD system to defend against ransomware attacks. Amoeba contains a hardware accelerator to detect the infection of pages by ransomware attacks at high speed, as well as a fine-grained backup control mechanism to minimize space overhead for original data backup. To evaluate their system, the authors extended the Microsoft SSD simulator to implement Amoeba and evaluated it using realistic block-level traces collected while running the actual ransomware. Their experiments found that Amoeba had negligible overhead and outperformed in performance and space efficiency over the state-of-the-art SSD, FlashGuard.
Kharraz and Kirda Kharraz and Kirda (2017) proposed Redemption, a system that requires minimal modification of the operating system to maintain a transparent buffer for all storage I/O. Redemption monitors the I/O request patterns of applications on a per-process basis for signs of ransomware-like behavior. If I/O request patterns are observed that indicate possible ransomware activity, the offending processes can be terminated and the data restored. The evaluation of their system showed that Redemption can ensure zero data loss against current ransomware families without detracting from the user experience or inducing alarm fatigue. Additionally, they proved that Redemption incurs modest overhead, averaging 2.6% for realistic workloads.
Key Management
Key management refers to recovering the encryption key that was used to encrypt files and using that to decrypt them without paying the ransom. For some ransomware samples, such as samples that hard code the key directly into their executable binary, this may be rather straightforward. For hybrid models, this can be more challenging, as the key is only available in plaintext while the files are actively being encrypted.
Bajpai and Enbody Bajpai and Enbody (2020a) decompiled eight different .NET ransomware variants and determined that some ransomware samples use poor key generation techniques that call common libraries. This insight can be utilized by ransomware countermeasures by keeping a backup of an attacker’s symmetric encryption key. This key can be used to recover any encrypted files later on. For example, Lee et al. Lee et al. (2018) observed that many ransomware programs use the CNG library, a cryptographic library for Windows machines, to generate the encryption key. They developed a prevention system that hooks these functions such that when ransomware calls them, the system stores the encryption key. For the evaluation of their system, Lee et al. Lee et al. (2018) implemented a sample ransomware program. They also implemented their prevention solution which attempts hooking into the process from the ransomware program that performs encryption so that it can extract the encryption key. After hooking, the prevention program displays the extracted encryption key when the sample ransomware generates the key for the encryption. In experiments where the ransomware program attempted encryption 10, 100, 1,000, 10,000, and 100,000 times, their ransomware prevention program was able to extract the encryption key 100% of the time. One limitation of this solution is the assumption that ransomware calls a specific library to obtain the encryption key; if the assumption is invalid, the solution fails.
Some ransomware programs use a symmetric session key for encryption. This key is stored in the victim’s computer which then encrypts the user’s files. Kolodenker et al. Kolodenker et al. (2017) developed a key backup solution called Paybreak which relies on signatures. PayBreak implements a key escrow approach that stores session keys in a vault, including the symmetric key that the attacker uses. When tested, PayBreak successfully recovered all files encrypted with known encryption signatures.
The security of the symmetric encryption key is vital for ransomware developers. Furthermore, a large subset of current ransomware exclusively deploy AES for data encryption. With this in mind, Bajpai and Enbody Bajpai and Enbody (2020) developed a side-channel attack on ransomware’s key management to extract exposed ransomware keys from system memory during the encryption process. Their attack leverages the knowledge that the encryption process is a white box on the host system; this approach is successful regardless of which cryptographic API is being used by the malware and regardless of whether a cryptographic API is being used by the malware at all. Their attack was able to identify exposed AES keys in ransomware process memory with a 100% success rate in preliminary experiments, including against NotPetya, WannaCry, LockCrypt, CryptoRoger, and AutoIT samples.
User Awareness
Chung Chung (2019) looked at preventing ransomware attacks within companies and organizations, arguing that they should help individual employees take precautions against ransomware scams. This is especially important since, as mentioned previously, ransomware attacks are increasingly targeting institutions such as financial or healthcare organizations. The author listed five prevention tips for employees to follow: install antivirus or anti-malware software on every computer and mobile device in use; choose strong and unique passwords for personal and work accounts; regularly back up files to an external hard drive; never open suspicious email attachments; and use mirror shielding technology such as NeuShield as a failsafe data protection measure.
Thomas Thomas (2018) also examined how users and employees within organizations can avoid ransomware attacks, but this paper focused on how individuals can avoid falling for phishing attacks, which are a common first step for ransomware. The author surveyed several security professionals and, based on the findings from the survey, proposed several recommendations. The first recommendation was to segment company employees based on factors such as their familiarity with phishing and the impact level of their jobs. After segmentation, the next recommendation was to develop targeted training for each group; this training should include real-life examples highlighting the seriousness and damage caused by phishing, use real case studies, and include actual incidents within the company. Sharing these actual and personal examples will result in a strong realization of the dangerous impact of spear phishing and will evoke a more personal protection response.
2.2.2. Ransomware detection approaches
Researchers have proposed various detection solutions to spot ongoing ransomware attacks. Once ransomware programs have been spotted, they can be stopped and removed. Below is a classification of different detection approaches. A summary of the tools used in the surveyed literature on ransomware detection can be found in Table 4 . An overview of the experimental results, which includes sensitivity and specificity rates, of the surveyed literature on ransomware detection can be found in Table 5 .
Overview of surveyed literature on ransomware detection.
Experimental results from the surveyed ransomware detection literature.
* Entries that contain a dash were not found in the reviewed source.
Analyzing System Information
A few of the surveyed papers used system information, such as log files or changes to the Windows Registry, as a method of detecting ransomware. A brief summary of all those works is presented below.
Monika et al. Monika et al. (2016) noted that ransomware samples tend to add and modify many Windows registry values. They suggested that the continuous monitoring of Windows registry values, along with file system activity, can be used to detect ransomware attacks. Chen et al. Chen and Bridges (2017) analyzed system log files to detect ransomware activity. This was done by extracting various features from the log files that are relevant to malware activity. Ultimately they found that malware (ransomware included) can be effectively detected using their approach, even when the logs contain mostly benign events, and that their solution is resilient to polymorphism.
Ransom Note Analysis
After the execution of a ransomware attack, a ransom note is usually left behind. This note could be saved to the user’s computer in the form of a text file or displayed on the user’s screen. This note informs the user that their personal files have been encrypted - or, in the case of locker ransomware, are inaccessible - and gives steps on how to pay and retrieve them. Static and dynamic analysis can reveal the traits of ransomware notes. For example, Groenewegen et al. Groenewegen et al. (2020) performed static and dynamic behaviour analysis to identify the traits of the NEFILIM ransomware strain that targets Windows machines. They found that if a NEFILIM sample is executed with administrative privileges, the accompanying ransom note is written to the root directory of the machine (C:); otherwise, it is written to the user’s ”AppData” directory. Furthermore, the ransomware calls the ”CreateFileW” and ”WriteFile” Windows functions to create the ransomware note and write to it, respectively. Lastly, they determined that the ransomware note file is always named ”NEFILIM-DECRYPT.txt”. In the case where the ransom note is displayed on the screen, some researchers took screen captures and used image and text analysis methods to detect the presence of a ransom note ( Alzahrani, Alshehri, Alshahrani, Alharthi, Fu, Liu, Zhu, 2018 , Kharaz, Arshad, Mulliner, Robertson, Kirda, 2016 ).
As mentioned in Section 2.1 , ransomware typically displays a ransom note on the user’s computer to receive payment. Some researchers used static and/or dynamic analysis to detect the presence of such a note to ascertain whether a ransomware attack is underway.
Alzahrani et al. Alzahrani et al. (2018) proposed RanDroid, a framework to detect ransomware embedded in malicious Android applications by looking for ransom notes displayed during the app’s execution. RanDroid measures the structural similarity between a set of images collected from the inspected application and a set of threatening images collected from known ransomware variants. The framework first decompiles the Android Application Package (APK) which contains a set of files and folders. It then extracts images from the resources folder and XML layout files using static analysis. Dynamic analysis is performed with a UI-guided test input generator to interact with the application without instrumentation, in order to trigger the app’s events, capture the activities that appear while the app is running, and collect additional images. Several pre-processing steps are applied to the images, including extracting the text from the images. Image and text similarity measurements are calculated against a database of images and texts collected from known ransomware variants; both measurements are used for a final classification. RanDroid was tested by running 300 applications (100 ransomware and 200 goodware applications) and achieved a 91% accuracy rate.
Kharraz et al. Kharaz et al. (2016) designed a system called UNVEIL to detect ransomware; a core component of UNVEIL is aimed at detecting screen locker ransomware, with the key insight that ransom notes generally cover a significant part, if not all, of the display. UNVEIL monitors the desktop of the victim machine and takes screenshots of the desktop before and after a sample is executed. The series of screenshots are then analyzed and compared with image analysis methods to determine if a large part of the screen has changed substantially between captures. When evaluated against 148,223 samples, UNVEIL achieved a 96.3% detection rate with zero false positives.
File Analysis
Crypto ransomware modifies a file when encrypting it. Large changes made to many files in a computer’s file system could indicate that a ransomware attack is underway. There are several metrics that can be used to detect significant changes in files. The three metrics identified from the surveyed literature are entropy, file type, and file differences (i.e. similarity). In addition, several researchers analyzed file I/O operations to detect suspicious activity. These four methods of file analysis are defined below.
- • File entropy: This measures the ”randomness” of a file. Encrypted and compressed files have high entropy compared to plaintext files. Hence, calculating the entropy of the file and comparing the value to previous calculations for the same file can be used to determine whether a file has been infected by ransomware. Scaife et al. Scaife et al. (2016) calculated file entropy with Shannon’s formula and used it as one feature to detect ransomware. Mehnaz et al. Mehnaz et al. (2018) also used Shannon entropy as a metric for detecting ransomware. Lee et al. Lee et al. (2019) applied machine learning to classify infected files based on file entropy analysis.
- • File type: A file’s type refers to its extension. Ransomware typically changes the extension of any file that it encrypts. In addition to entropy, both Scaife et al. Scaife et al. (2016) and Mehnaz et al. Mehnaz et al. (2018) used file type changes as a feature to determine the presence of ransomware. The detection system designed by Ramesh and Menen Ramesh and Menen (2020) monitors for changes such as large numbers of files being created with the same extension or any files with more than one extension.
- • Similarity: In comparison with benign file changes, such as modifying parts of a file or adding new text, the contents of a file encrypted by ransomware should be completely dissimilar from the original plaintext content. Hence, measuring the similarity of two versions of the same file can be used to detect whether ransomware is present. Scaife et al. Scaife et al. (2016) measured the similarity between two files with a hash function sdhash, which outputs a similarity score from 0 to 100 that describes the confidence of similarity between two files. Comparisons between previous versions of a file and the encrypted version of the file should yield a score close to 0, as the ciphertext should be indistinguishable from random data. Mehnaz et al. Mehnaz et al. (2018) also used sdhash to perform similarity checks between file versions to determine if a file has been encrypted by ransomware.
- • File I/O: These operations are used to access the host computer’s file system. Examples of I/O operations include open, close, read, and write fil (2021) . Ransomware typically performs read operations to read user files without the user’s permission. It executes write operations either to create encrypted copies of the target files or to overwrite the original files. In the case of the former option, ransomware performs additional operations to delete the original files. Baek et al. Baek et al. (2018) developed a system to detect ransomware in SSDs which learns the behavioural characteristics of ransomware by observing the request headers of the I/O operations that it performs on data blocks. These request headers include the logical block address, the type of operation (read/write), and the size of the data. Natanzon et al. Natanzon et al. (2018) developed a system that generates a ransomware probability by comparing recent I/O activity to historical I/O activity; if the ransomware probability exceeds a specified threshold value, the system takes actions to mitigate the effects of ransomware within the host. The detection system proposed by Kharraz et al. Kharaz et al. (2016) extracts features from I/O requests during a sample’s execution such as the type of request (e.g., open, read, write). These events are then matched against a set of I/O access pattern signatures as evidence that the sample is in fact ransomware.
Finite State Machines
An abstract mathematical model that can be used to represent the state of a system and track changes. It has been noted that many ransomware samples tend to carry out similar sets of actions once they reach a target system. Also, the changes made by ransomware differ significantly from benign programs. Hence, ransomware can be quickly identified in most cases. FSM’s can be used to track those actions by associating system events with transitions between the states in the FSM. The state of the FSM can be monitored and if certain states are reached, the FSM can signal that a ransomware attack is underway. Monitoring the state changes that occur in the computer system in terms of utilization, persistence, and the lateral movement of resources can detect ransomware ( Ramesh and Menen, 2020 ).
Ramesh and Menen Ramesh and Menen (2020) proposed a finite state machine (FSM) with eight total states. The changes represented in the FSM include: changes in file entropy, as encrypted files have higher levels of entropy; changes in retention state, which occurs if a process has been added to the Run registry or startup directory; lateral movement, which checks for suspicious file names such as doubled file extensions (e.g..pdf.exe); and system resources, which looks for processes that modify the system-restore settings or stop a large number of other processes in a short amount of time. If the FSM ever moves into one of its four final states, then the system is considered to be under a ransomware attack. Their method was tested against 475 different ransomware samples and 1500 benign programs. It detected 98.1% of the tested samples and had a 0% false positive rate. The main drawbacks of this approach are its inability to detect locker-type ransomware and its inability to detect ransomware samples that use sophisticated code-obfuscation and incremental unpacking techniques, such as NotPetya.
Honeypots (or honeyfiles) are decoy files set up for the ransomware to attack. Once these files are attacked, the attack is detected and stopped. Honeyfiles are easy to set up and require little maintenance. However, there is no guarantee the attacker will target these decoys, so an attacker may encrypt other files while leaving the honeyfiles untouched Moore (2016) . Gómez-Hernández and Álvarez-González Gomez-Hernandez et al. (2018) proposed R-Locker , a tool for Unix platforms containing a ”trap layer” with a series of honeyfiles. Any process or application that accesses the trap layer is detected and stopped. Unfortunately, R-Locker only protects part of the complete file system, and the tool can be defeated by deleting the central trap file.
Similarly, Kharraz et al. Kharaz et al. (2016) designed UNVEIL to limit the damage that can be done by attackers before they are detected with honeyfiles. UNVEIL generates a virtual environment that aims to attract attackers. It then monitors its file system I/O and detects any presence of a screen locker. Their solution detected 96.3% of ransomware samples and had zero false positives.
Shaukat and Rebeiro Shaukat and Ribeiro (2018) proposed RansomWall , a multi-layered defense system that incorporates honeyfiles to protect against crypto-ransomware. When the trap layer suspects a process is malicious, any modified files are backed up until it is classified as either ransomware or benign by other layers. When tested, RansomWall had a 98.25% accuracy rate and generated zero false positives. One challenge is that some ransomware samples have limited file system activity.
Network Traffic Analysis
Network traffic analysis intercepts network packets and analyzes communication traffic patterns to detect ongoing malware attacks. For certain ransomware families, the communication between the victim host and the C&C server behaves much differently compared to normal conditions. This anomalous behavior can be revealed by studying certain traffic features. The four main features of network traffic used by researchers to detect ransomware are discussed below.
- • Packet size: The size of messages exchanged may be unusually large if they contain an encryption key or encryption instructions. Cabaj et al. Cabaj et al. (2018) analyzed CryptoLocker and Locky ransomware samples under execution and extracted the message size from HTTP packet headers to determine the average size of messages exchanged between the infected host and the C&C server, then used these statistics to build an anomaly detection system based on message size. Bekerman et al. Bekerman et al. (2015) used TCP packet size as a feature in a supervised-based system for detecting ransomware.
- • Message frequency: Determining an uptick in certain kinds of traffic can be used to detect the presence of a ransomware attack. Almasshadani et al. Almashhadani et al. (2019) observed that Locky ransomware significantly increases the number of HTTP POST request packets within the traffic stream compared to the normal traffic. Additionally, they found that there are numerous TCP RST and TCP ACK packets in Locky’s traffic used to terminate the malicious TCP connections abnormally. The authors used these features and others as part of a multi-classifier intrusion detection system. Bekerman et al. Bekerman et al. (2015) used the number of TCP RST packets, TCP ACK packets, and duplicate ACK packets as well as the number of sessions in communication as features for their supervised ransomware classification model.
- • Malicious domains: Communication between the ransomware and the C&C server can be blocked if the server’s domain is identified as malicious. Cabaj and Mazurczyk Cabaj and Mazurczyk (2016) proposed a software-defined networking solution that relies on dynamic blacklisting of proxy servers to block communication between the infected computer and the C&C server. Their proposal forwards all DNS traffic to a controller that checks the domains with a blacklist database. If a malicious domain is detected, the DNS message is discarded and traffic from the host is blocked.
- • DGA detection: Rather than using hardcoded domain addresses, which are susceptible to domain blacklisting, some types of ransomware employ a Domain Generation Algorithm (DGA) to generate a large number of domain names that can be used as rendezvous points for their C&C servers. Some detection systems such as the one proposed by Chadha and Kumar Chadha and Kumar (2017) and Salehi et al. Salehi et al. (2018) work by determining the DGA and subsequently blocking all generated domains.
- • Other features: Hundreds of other extracted network features from various OSI layers can also be used for ransomware detection. Many of these are outlined in Bekerman et al. (2015) , where they did not focus on ransomware detection specifically, but instead on general malware detection.
Machine Learning
Many studies proposed machine learning models that detect ransomware by classifying computer programs as either benign or ransomware based on their behaviour. With sufficient training data, these models can spot attacks with a high degree of accuracy. Additionally, they are frequently able to detect ransomware before it has a chance to encrypt any files. However, finding a suitable model requires trial and error, and biasness or overfitting may occur if proper measures are not taken ( Kok et al., 2019b ). What distinguishes the models proposed by different researchers are the classifier algorithms that are applied and the features that are used for training. The features used in the surveyed literature include the following:
- • APIs / System calls : API calls are functions that facilitate the exchange of data among applications, while system calls are service requests made by the ransomware to the OS or kernel api (2018) . Often, ransomware makes API calls to the C&C server to obtain an encryption or decryption key. Other API calls can be made to maintain execution privileges on the host computer, enumerate the list of files to encrypt, and access or modify files. Ransomware and benign programs have specific call patterns or a unique order of calls that can be used to differentiate them. Examples of system calls include create, delete, execute, and terminate Bajpai and Enbody (2020b) ; Qin et al. (2020) ; api (2018) .
- • Log files: Log files can come from a variety of sources and record information that can indicate whether a ransomware attack is underway. For instance, Herrera Silva and Hernández-Alvarez ( Silva and Hernandez-Alvarez, 2017 ) found that both WannaCry and Petya ransomware exploit DNS and NetBIOS and can be spotted by analyzing DNS and NetBIOS logs. I/O request packets are generated for each file operation and contain parameters such as the type of operation and the address and size of the data being read or written to. These parameters can be extracted from I/O request packet logs and used as features.
- • File I/O: Ransomware typically executes many more read operations than benign programs, since it must read every file it encrypts. Additionally, it executes more write operations on average. File operation metrics such as the number of files written to or read from; the average entropy of file-write operations; the number of file operations performed for each file extension; and the total number of files accessed can be used to gauge if the file operations being performed are benign or part of a ransomware attack( Continella, Guagnelli, Zingaro, Pasquale, Barenghi, Zanero, Maggi, 2016 , Sgandurra, Muñoz-González, Mohsen, Lupu, 2016 ).
- • HPC values: Hardware Performance Counters (HPCs) are a set of special-purpose registers that were first introduced to verify the static and dynamic integrity of programs in order to detect any malicious modifications to them ( Alam et al., 2020 ). The time-series data collected from these counters can be fed into a model to learn the behaviour of a system and detect malicious programs through any statistical deviations in the data.
- • Network traffic: Network traffic features include average packet size, the number of packets exchanged between the host and other machines, and the source and/or destination IP addresses contained within packet headers. Ransomware frequently displays anomalous communications patterns. For example, the work by Cabaj et al. Cabaj and Mazurczyk (2016) found that CryptoWall and Locky ransomware samples involve a defined sequence of HTTP packets exchanged between the host and a C&C server to distribute the encryption key; in addition, these packets tend to be larger than average. Machine learning models can learn normal and anomalous traffic features to distinguish normal communication from malicious communication. Chadha and Kumar Chadha and Kumar (2017) analyzed network traffic to obtain the names of benign and malicious domains to use as features for their model, which detects ransomware by predicting if incoming or outgoing packets transmitted to or from the host contains a malicious domain.
- • Opcode/Bytecode sequences: Opcodes (”operation codes”) specify the basic processor instructions to be performed by a machine, whereas bytecode is a form of instruction designed to be executed by a program interpreter (e.g., Java Virtual Machine). These sequences have rich context and semantic information that provide a snapshot of the program’s behaviour. This information can be extracted through dynamic analysis and fed into a model to predict if a given program is benign or malicious.
- • Process actions: This refers to the sequence of events that occur while a program or application is running. Ransomware will typically cause different events to occur compared to a benign program; these events can be transformed into feature vectors and learned by a model by extracting information such as text and encoding it as numerical values ( Homayoun et al., 2019 ).
- • Others: Many other features were used by researchers and extracted from assorted sources. Some of these features are derived from the raw bytes extracted from executable files using static analysis ( Khammas, 2020 ). Other features related to web domains (e.g., the length of the domain name, the number of days a domain is registered for Quinkert et al. (2018b) ) or DNS (e.g., the number of DNS name errors, the number of meaningless domain names ( Almashhadani et al., 2019 )). Portable Executable (PE) file headers, which show the structure of a file and contain important information about the nature of the executable file, have components that be used as features. Other sources for features include the CPU (e.g., power usage), k-mer substrings (e.g., frequencies), volatile memory, and the Windows Registry ( Azmoodeh, Dehghantanha, Conti, Choo, 2018 , Cohen, Nissim, 2018 , Sgandurra, Muñoz-González, Mohsen, Lupu, 2016 ).
A complete list of the works that focused on detecting ransomware using machine learning is highlighted in Table 6 .
Overview of surveyed machine learning detection approaches.
SVM: Support Vector Machines, ANN: Artificial Neural Networks, KNN: k -nearest neighbors, LDA: Linear discriminant analysis, CART: Classification and regression trees, SGD: Stochastic Gradient Descent, CNN: Convolutional Neural Networks, LSTM: Long short-term memory
3. Ransomware implementation and evaluation
In this section, we have highlighted the motivation of implementing existing ransomware samples and testing the effectiveness of existing countermeasures against those ransomware samples. A brief description of our new ransomware is also presented.
3.1. Motivation
From the literature review, few studies were found to test the effectiveness of existing ransomware countermeasures, such as antivirus products. There seems to be a research gap between research-based proposed solutions and existing practical solutions. To validate our claim, we decided to test different AV products against random known ransomware samples and a simple ransomware created by us. This was done to evaluate the effectiveness of existing practical countermeasures against both known and unknown ransomware samples. Also, our aim is not to claim that existing AV products are not able to detect ransomware samples, as it is possible that the tested AV products are able to detect other samples from other known ransomware families. Through these experiments, our motive is just to highlight the need of effective countermeasures against known/unknown ransomware samples.
3.2. Experimental setup
Testing was done using a VirtualBox virtual machine running the latest version of Windows 10. VirtualBox Guest Additions were not installed as some malware samples are known to detect these additions ( gue, 2017 ). Ransomware samples were taken from the work of sam (2021) . The samples were in a binary format and had to be extracted from an encrypted ZIP file before use. In most cases, the file extensions were manually added before the execution of the ransomware. To conduct the tests safely on these ransomware samples, a few precautions were taken. This included setting the network adaptor to host only, ensuring all software was up-to-date, and removing any shared folders between the guest and the host operating systems. On the host side, data was backed up to an external hard drive and the internet connection was disconnected. The reason for disconnecting the internet was to make sure ransomware did not escape the environment of the virtual machine. The ransomware samples were all taken from https://github.com/ytisf/theZoo in January of 2021.
Several test folders were placed in different areas of the file system including Desktop, Documents, and Picture folders. Test folders were also placed in protected areas of the file system such as Program Files, Program Files (x86), and Windows. One of the folders was placed in the Recycle Bin to analyze if the ransomware scans Recycle Bin or not. The test folders contained four different file formats that included rich-text, text, PDF, and image files. All these respective files had a non-zero size.
3.3. Testing
Testing consisted of three parts, where in each part various ransomware samples are pitted against various antivirus products. The first test was on well-known ransomware samples. The second test used a RaaS generator. The third and final test used a novel custom-made ransomware sample. All of the antivirus products were the most up-to-date versions as of January, 2021.
3.3.1. Well-Known ransomware tests
The first round of testing was simply a control test to see the impact of the ransomware samples when no security controls were in place; all antivirus applications were turned off. The User Access Control Settings of Windows were set to default. The ransomware samples tested were WannaCry ( Akbanov et al., 2019 ), Cerber ( Hassan, 2019 ), Thanos, and Jigsaw ( Hull et al., 2019 ). The results are shown in Table 7 , where it can be seen that most of the files within the Desktop, Documents, etc., got encrypted except for the protected operating system folders. Cerber ransomware failed to encrypt folders that the other samples encrypted. The explanation for this behaviour is unknown, but it could have just been programmed in that way.
Control test results where ransomware samples were tested without any form of protection.
Other ransomware samples were also tested, but unfortunately, we were not able to analyze them. As mentioned earlier, some forms of ransomware need to connect via the internet to a C&C server before they can be executed. In our scenario, due to the testing being done offline, it was not possible to analyze that category of ransomware.
The same ransomware samples were then tested against eight popular antivirus programs. In all cases, the ransomware samples were rapidly detected and removed before any test files became encrypted. The samples were often removed before they were even clicked on.
3.3.2. RAASNet Testing
The second round of testing was done using a RaaS generator called RAASNet, which can be downloaded from https://github.com/leonv024/RAASNet . RAASNet is a free, cross-platform, and open-source software project designed to educate the public about how easy it is to create and use ransomware. It allows for custom ransomware to be created and tested. Although RAASNet generates real ransomware, the decryption key can be freely obtained from the author’s website.
A control test was performed for two different RAASNet generated ransomware samples with no antivirus software running. These two samples were identical except for the fact that one ran with administrator privileges while the other did not. The payloads of both samples were generated using the default settings of RAASNet. The results of this control test can be seen in Table 8 . Both of the samples were set to target all of the listed folder locations. The sample with administrator privileges was tested to see if it would be able to infect the protected operating system folders, but this was unsuccessful. The only difference between the two tests was that the one with administrator privileges generated a user account control (UAC) prompt message, but allowing access still did not let the ransomware modify the files.
A control test of two different RAASNet payloads, one with administrator privileges and one without.
The advantage of testing RAASNet ransomware over well-known ransomware samples (e.g. Jigsaw) is that RAASNet generated samples are not included in all antivirus signature databases. One of the generated payloads was uploaded to VirusTotal.com, and only 20 out of 72 antivirus engines detected the payload as malicious. Comparatively, Jigsaw’s sample was also uploaded and this was detected by 67 out of 72 engines. This means that the antivirus programs can be tested for their dynamic detection abilities rather than strictly through static-based detection. This is important since it is a better indication of how they might do against novel ransomware samples in the future where static analysis is more likely to fail.
A RAASNet generated payload (created with default settings and without administrator privileges) was then tested against several popular antivirus programs. The results of these tests can be found in Table 9 . Folders were placed in different locations across the file system and marked as either encrypted or safe depending on whether the ransomware encrypted them or not. The worst performing antivirus programs were Microsoft Defender, MalwareBytes (Free), and Avira (Free). All of the antivirus programs had real-time protection turned on. Overall, the antivirus programs did quite well and quickly caught the ransomware before it could do any real damage. However, the antivirus programs with the best results appeared to detect the ransomware samples through static analysis. This is evidenced by the fact that many of these antivirus programs gave messages indicating that they detected the ransomware by preemptively scanning the file, seemingly before they could run.
RAASNet test results for different antivirus software. Both Microsoft Defender and Avira failed to stop the sample.
It is worth noting that many antivirus programs, such as Microsoft Defender, do have an effective form of ransomware protection built-in. This protection comes in the form of folder protection which checks if a process is trusted. If it is not, the antivirus software denies the process from modifying the folder contents. A protected folder was set up on the Desktop using Microsoft Defender, and the contents in this folder were successfully protected. It would appear that a similar form of protection also safeguards important operating system folders, as evidenced by the fact that no ransomware sample was able to encrypt files in these areas of the file system.
3.3.3. AESthetic Ransomware testing
The final tests were done using the AESthetic ransomware sample. This sample was custom-made for this research and was created in Java. We created AESthetic using Java’s standard cryptographic package, javax.crypto. AESthetic uses a hybrid encryption approach with the help of a C&C server that runs on localhost. It starts by generating a symmetric key using secure cryptographic modules. It then recursively crawls through the file system from a specified target directory and will encrypt all specified file types using AES-256 in CBC mode. A unique and randomly generated initialization vector is used for each file, which gets appended to the beginning of the encrypted file for later use. A ransom note is placed in every directory that AESthetic traverses through. Once all of the files are encrypted, AESthetic connects to the C&C server to obtain an RSA public key that it uses to encrypt the symmetric key. Once the symmetric key is encrypted, the plaintext version of the symmetric key is deleted. New files are created to store the encrypted data and the original plaintext files are deleted. After ten seconds, it will automatically start to decrypt the encrypted files. To do this, it once again connects to the C&C server to obtain the corresponding RSA private key to decrypt the encrypted AES symmetric key. This sample was tested against eight popular antivirus programs (which are the same as those listed in Table 9 ). All of the test files got encrypted by AESthetic. None of the antivirus programs reported any suspicious activity. Both the source code and an executable JAR file were uploaded to VirusTotal.com, and in both cases, this resulted in zero detections. There were zero detections since the malware was made just for this research and its signature has not yet been added to any signature database.
4. Discussion
From the results of our literature review and experiments, we can make several observations on the current trends and limitations of ransomware countermeasure solutions. Most papers preferred to study ransomware using dynamic analysis over static analysis, or used a combination of the two. This is perhaps unsurprising, as static analysis can frequently be evaded through code obfuscation or polymorphic/metamorphic attacks ( Shaukat and Ribeiro, 2018 ). However, some papers found that certain dynamic analysis approaches can be evaded as well. For instance, the virtual environment in UNVEIL ( Kharaz et al., 2016 ) could potentially be detected and avoided by attackers. One limitation of both types of analysis is that the results cannot usually be generalized to all ransomware variants. For example, the key backup technique proposed by Lee et al. Lee et al. (2018) relies on their analysis that ransomware calls specific functions in the CNG library. The HTTP traffic characteristics that Cabaj et al. Cabaj et al. (2018) used to detect ransomware comes from studying ransomware families: CryptoWall and Locky. Almashhadani et al. Almashhadani et al. (2019) based their detection system on the behavioural analysis of one family – Locky.
Preventative techniques such as access control and key or data backups can reduce the damage that ransomware can inflict on systems and possibly deter future attacks. However, these prevention-based approaches suffer from several shortcomings as well. Firstly, they can have significant overhead. Access control or key backup schemes can incur significant computational costs ( Wang et al., 2015 ). Creating data backups can cause the system to take a significant performance hit, especially under high workloads ( Alshaikh et al., 2020 ).
Machine learning models were the most common technique for detecting ransomware. These models can be trained to recognize the general behaviour patterns of ransomware through suspicious behaviour or specific basic processor instruction patterns. The ability for machine learning to detect the general behaviour of ransomware is important, as ransomware is constantly evolving and can easily change its code signature, but has difficulty changing its attack pattern ( Kok et al., 2019b ). However, many of these models require an attack to already be underway in order to detect suspicious activity, such as file access or communication to a malicious domain. Khan et al.’s Khan et al. (2020) use of digital DNA sequencing is a promising approach since it is designed to detect ransomware before infection.
Based on the results of our experiments, which were conducted on a number of different ransomware samples, we have learned a few interesting things about ransomware. Our tests using RAASNet have shown how easy it is to acquire and use ransomware through RaaS software. RaaS lets ransomware developers sell or lease their ransomware variants to affiliates, who use these variants to perform attacks; both developers and affiliates get a cut of any profits. As previously mentioned, RaaS enables users without technical expertise to launch ransomware attacks, meaning that ransomware is no longer limited to the developers who create it. For developers, RaaS reduces their risk since they do not launch the attacks themselves. The RaaS model has gained popularity amongst cybercriminals and has caused a dramatic increase in the rate of ransomware attacks in recent years ( Al-rimy et al., 2018 ).
Although antivirus programs were successful against previously known samples, they did not fare quite so well against the lesser-known RAASNet sample and the completely novel AESthetic sample. The novel sample of course is not present in antivirus signature databases and it was completely undetected. This highlights that current antivirus software likely rely too heavily on simple signature-based static analysis detection and hence should invest more into the approaches seen in literature, especially in regards to dynamic analysis or honeypot approaches. For example, our ransomware AESthetic was designed with many tell-tale ransomware behaviors in mind, such as leaving ransom notes, reading and writing to many files throughout the file system, and using cryptographic libraries. These behaviors could have potentially been used to detect AESthetic as malicious using dynamic analysis. The only tested antivirus countermeasure that successfully repelled all of the tested ransomware samples was ransomware folder protection, such as ”Controlled folder access” which is offered by Windows Defender. Such an approach requires the user to manually decide which folders to protect however and it is not very user-friendly, as one needs to manually allow benign programs through the protection wall.
5. Research challenges and future research directions
In this section, we have highlighted key research challenges based on the literature review and explored future research directions. The identified research challenges include unawareness among users, lack of open-access ransomware libraries, and inadequate detection and false-positive rates for ransomware. Future research directions include edge and fog-assisted ransomware, DeepFake ransomware, remote working vulnerabilities, blockchain-based countermeasures, increases in RaaS attacks, and expansion to AESthetic.
5.1. Research challenges
1. Unawareness among users: Awareness among users is one of the fundamental challenges that needs to be addressed to reduce the impact of ransomware. For example, there is no full-proof automatic system that is able to consistently counter ransomware attacks that propagate through phishing campaigns. Although existing spam filters are efficient, there is always a possibility that some malicious emails will make their way into your inbox. In that scenario, basic knowledge of recognizing spam can save a victim from being infected. There are currently many workshops, programs, and online websites available to educate users of such threats, but based on the statistics of ransomware attacks, it seems more efforts are needed.
2. Lack of Open-Access Ransomware Libraries: In order to propose and develop new solutions that can tackle ransomware, there is an emerging need for open ransomware libraries. The availability of such libraries will help researchers to better understand the varying features behind existing ransomware samples, including their working mechanism, etc. Based on that understanding, researchers can propose better solutions in a faster time span. As it stands, it is a tedious task to implement a particular ransomware sample and then test out the countermeasure. However, collecting many of the existing ransomware samples is itself a big research challenge that needs international research collaboration, as well as a huge amount of funding to obtain the necessary resources, etc.
3. Inadequate Detection and False Positive Rates: Existing ransomware detection systems face a difficult challenge achieving both a high detection rate and few false alarms. A large number of false alarms is frustrating for administrators, whereas a low detection rate makes the system ineffective ( Maimó et al., 2019 ). Signature-based detection systems may miss attacks if the signature is too specific; conversely, the system may flag too many benign programs as ransomware if the signature is too generic. Anomaly-based detection systems flag behaviour that is sufficiently far from normal ( Kathareios et al., 2017 ). However, not all abnormal behaviour is malicious. Consequently, these systems can generate a high number of false alarms and require a human to manually review each alarm. This manual validation adds to the system workload and reduces the system’s practicality. Al-Rimy et al. Al-rimy et al. (2018) were able to achieve both high detection and low false-positive rates by combining two behavioural detection methods into a single model. However, their system relies on a time-based threshold. Hence, more research is needed to improve ransomware detection models and to increase their applicability.
5.2. Future research directions
1. Edge and Fog-assisted Ransomware Detection and Prevention using Federated Learning: There have been huge advancements in the area of Edge and Fog-based related technologies. Mukherjee et al. (2018) , Hakak et al. (2020c) , Hakak et al. (2020) , Pham et al. (2020) . Besides, with the arrival of federated learning ( Yang et al., 2019 ), numerous opportunities in terms of improving state-of-the-art machine-learning-based approaches have emerged. There is a huge possibility of utilizing these concepts to detect and prevent ransomware, based on machine learning approaches ( Liu et al., 2020 ). One of the possibilities arises by training and deploying machine learning-based algorithms into Edge/Fog-based nodes to detect and prevent ransomware. Through Federated learning, we can personalize the learning process of each respective node.
2. DeepFake Ransomware : Deepfakes are the manipulated digital representations such as images, videos where an attacker tries to mimic the real person ( Güera and Delp, 2018 ). In the future, it could be possible for attackers to create ransomware that will automatically generate DeepFake content of a victim performing some incriminatory or intimate action which he/she never did. The victim will be asked to pay the ransom in order to avoid that content being published online. To mitigate such ransomware attacks will be challenging due to the velocity of data and the availability of numerous social media channels to spread the content.
3. Remote Working Vulnerabilities : The recent COVID-19 pandemic made it mandatory for several institutions to initiate the work-from-home scenarios or implement bring your own devices (BYOD) policies ( Palanisamy et al., 2020 ). As a result of which, several vulnerabilities ( Curran, 2020 ) were exploited by the attackers that resulted in several ransomware attacks. In one of the reports by SkyBox Security, the ransomware attacks witnessed 72 percent growth compared to the previous years. Hence, it is one of the future research directions to look at mitigating such attacks during remote working scenarios.
4. Blockchain-based Countermeasures : Blockchain is an immutable decentralized ledger that makes tampering difficult ( Hakak et al., 2020a ) due to its decentralized nature along with linked hash function, timestamp function and consensus mechanism ( Hakak, Khan, Gilkar, Imran, Guizani, 2020 , Hakak, W.Z. Khan, Gilkar, Haider, Imran, Alkatheiri, 2020 ). It seems to have potential and it is an interesting research direction where blockchain-based solutions can be used to mitigate ransomware-based attacks. The first step in this direction is the work of Delgado-Mohatar et al. (2020) where the authors have highlighted the use of smart contracts for the limited payment of ransoms to get the decryption keys.
5. Increase in Ransomware-as-a-service (RaaS) Attacks : Ransomware as a service or RaaS is gaining popularity from the past few years ( Keijzer, 2020 ). In RaaS model, an experienced attacker creates ransomware and offers that code to script kiddies or gray-hat hackers for some price ( Meland, Bayoumy, Sindre, 2020 , Puat, Rahman, 2020 ). The script kiddies or gray-hat hackers then use that code to carry out their own attacks. The Cerber ransomware attack is one example of the RaaS model in action. With emerging technologies and an increasing number of internet users, there is a strong possibility for a surge in these types of attacks. Hence, mitigating such attacks in the future seems to be a potential research direction.
6. AESthetic Ransomware Artifact Development : The source code of AESthetic ransomware has been posted to GitHub at https://github.com/kregg34/AESthetic and has been made private. As we are still in initial phases of developing decryption tool for AESthetic, we aim to create artifacts for AESthetic ransomware so that researchers can evaluate the efficacy of their solutions against ransomware. On the other hand, once the decryption tool is finalised, we will release the code of AESthetic.
7. AESthetic Performance : The antivirus products were likely able to detect the other, well-known samples due to their known signatures. However, our ransomware AESthetic has no known signatures and went undetected. This may indicate that these products are relying on static analysis too much, and not effectively utilizing dynamic analysis. Dynamic analysis may be able to detect AESthetic as this was designed to have many of the tell-tale-signs of ransomware behaviour. However, to validate this claim, more research is needed owing to the blackbox nature of antivirus products.
6. Conclusion
In this work, recent advances in ransomware analysis, detection, and prevention were explored. It was found that the focus of the state-of-the-art ransomware detection techniques mostly revolve around honeypots, network traffic analysis, and machine learning based approaches. Prevention techniques mostly focused on access control, data and key backups, and hardware-based solutions. However, it seems that there is a trend in using machine learning based approaches to detect ransomware. We have conducted a number of experiments on ransomware samples, through which it was observed that there is a need for more intelligent approaches to detect and prevent ransomware. Through the experiments, it was also observed that ransomware can be easily created and used. In the end, we highlighted the existing research challenges and enumerated some future research directions in the field of ransomware.
Credit Author Statment
Craig Beaman conducted the literature review, worked on implementation details, and was involved in drafting the manuscript.
Ashley Barkworth conducted the literature review and was involved in drafting the manuscript, with particular focus on Ransomware Prevention Approaches and subsections 2.2.2.3 and 2.2.2.5-2.2.2.7 under Section 2.2.2 (“Ransomware Detection Approaches”).
Toluwalope David Akande conducted the literature review and was involved in drafting the manuscript.
Saqib Hakak designed the study, assisted in classification, worked on future research challenges & directions section, and coordinated the whole work.
M.Khurram Khan provided potential useful recommendations and directions to improve the work, assisted in addressing reviewer comments and proof-reading.
Declaration of Competing Interest
The authors declare that they have no known competing financial interests or personal relationships that could have appeared to influence the work reported in this paper.
Acknowledgements
All persons who have made substantial contributions to the work reported in the manuscript (e.g., technical help, writing and editing assistance, general support), but who do not meet the criteria for authorship, are named in the Acknowledgements and have given us their written permission to be named. If we have not included an Acknowledgements, then that indicates that we have not received substantial contributions from non-authors. The work of Muhammad Khurram Khan is supported by King Saud University, Riyadh, Saudi Arabia under the project number (RSP-2021/12).
Biographies
Craig Beaman is a graduate student at the University of New Brunswick, where he is completing a Master of Applied Cybersecurity. Craig received a B.Sc. (Honours) from the University of New Brunswick with a major in physics and minors in mathematics and computer science. His research interests include cryptography, network security, and malware detection and prevention.
Ashley Barkworth is a graduate student at the University of New Brunswick, where she is completing a masters in applied cybersecurity. Ashley received a B.Sc. (Honours) from the University of British Columbia with a major in computer science and a minor in mathematics in 2020. Her research interests include information security, cryptography, and data management in centralized systems.
Toluwalope David Akande is a graduate student at the University of New Brunswick, where he is completing a Master of Applied Cybersecurity. He received a B.Sc. (Honours) from Obafemi Awolowo University with a major in Computer Engineering. His research interests include network security, intrusion detection using machine learning and cloud computing security.
Saqib Hakak is an assistant professor at the Canadian Institute for Cybersecurity (CIC), Faculty of Computer Science, University of New Brunswick (UNB). Having more than 5+ years of industrial and academic experience, he has received several Gold/Silver awards in international innovation competitions and is serving as the technical committee member/reviewer of several reputed conference/journal venues. His current research interests include Risk management, Fake news detection using AI, Security and Privacy concerns in IoE, Applications of Federated Learning in IoT, and blockchain technology.
Muhammad Khurram Khan is currently working as a Professor of Cybersecurity at the Center of Excellence in Information Assurance, King Saud University, Kingdom of Saudi Arabia. He is founder and CEO of the ‘Global Foundation for Cyber Studies and Research’, an independent and non-partisan cybersecurity think-tank in Washington D.C, USA. He is the Editor-in-Chief of ‘Telecommunication Systems’ published by Springer-Nature with its recent impact factor of 2.314 (JCR 2021). He is also the Editor-in-Chief of Cyber Insights Magazine. He is on the editorial board of several journals including, IEEE Communications Surveys & Tutorials, IEEE Communications Magazine, IEEE Internet of Things Journal, IEEE Transactions on Consumer Electronics, Journal of Network & Computer Applications (Elsevier), IEEE Access, IEEE Consumer Electronics Magazine, PLOS ONE, and Electronic Commerce Research, etc. He has published more than 400 papers in the journals and conferences of international repute. In addition, he is an inventor of 10 US/PCT patents. He has edited 10 books/proceedings published by Springer-Verlag, Taylor & Francis and IEEE. His research areas of interest are Cybersecurity, digital authentication, IoT security, biometrics, multimedia security, cloud computing security, cyber policy, and technological innovation management. He is a fellow of the IET (UK), a fellow of the BCS (UK), and a fellow of the FTRA (Korea). His detailed profile can be visited at http://www.professorkhurram.com .
- Adamu U., Awan I. 2019 7th International Conference on Future Internet of Things and Cloud (FiCloud) 2019. Ransomware prediction using supervised learning algorithms; pp. 57–63. [ CrossRef ] [ Google Scholar ]
- Aghakhani H., Gritti F., Mecca F., Lindorfer M., Ortolani S., Balzarotti D., Vigna G., Kruegel C. Network and Distributed Systems Security (NDSS) Symposium 2020. 2020. When malware is packin’heat; limits of machine learning classifiers based on static analysis features. [ Google Scholar ]
- Akbanov M., Vassilakis V., Logothetis M. Wannacry ransomware: analysis of infection, persistence, recovery prevention and propagation mechanisms. Journal of Telecommunications and Information Technology. 2019 [ Google Scholar ]
- Al-Rimy B., Maarof M., Alazab M., Alsolami F., Shaid S., Ghaleb F., Al-Hadhrami T., Ali A. A pseudo feedback-based annotated tf-idf technique for dynamic crypto-ransomware pre-encryption boundary delineation and features extraction. IEEE Access. 2020; 8 :140586–140598. [ Google Scholar ]
- Al-rimy B., Maarof M., Prasetyo Y., Shaid S., Ariffin A. Zero-day aware decision fusion-based model for crypto-ransomware early detection. International Journal of Integrated Engineering. 2018; 10 (6) [ Google Scholar ]
- Al-rimy B., Maarof M., Shaid S. Ransomware threat success factors, taxonomy, and countermeasures: a survey and research directions. Computers & Security. 2018; 74 :144–166. [ Google Scholar ]
- Al-rimy B., Maarof M., Shaid S. Crypto-ransomware early detection model using novel incremental bagging with enhanced semi-random subspace selection. Future Generation Computer Systems. 2019; 101 :476–491. [ Google Scholar ]
- Alam M., Bhattacharya S., Dutta S., Sinha S., Mukhopadhyay D., Chattopadhyay A. 2019 IEEE International Symposium on Hardware Oriented Security and Trust (HOST) 2019. Ratafia: ransomware analysis using time and frequency informed autoencoders; pp. 218–227. [ Google Scholar ]
- Alam M., Sinha S., Bhattacharya S., Dutta S., Mukhopadhyay D., Chattopadhyay A. Rapper: ransomware prevention via performance counters. arXiv preprint arXiv:2004.01712. 2020 [ Google Scholar ]
- Alhawi O., Baldwin J., Dehghantanha A. Cyber Threat Intelligence. Springer; 2018. Leveraging Machine Learning Techniques for Windows Ransomware Network Traffic Detection; pp. 93–106. [ Google Scholar ]
- Almashhadani A., Kaiiali M., Sezer S., O’Kane P. A multi-classifier network-based crypto ransomware detection system: a case study of locky ransomware. IEEE Access. 2019; 7 :47053–47067. [ Google Scholar ]
- Alshaikh H., Nagy N.R., Hefny H. Ransomware prevention and mitigation techniques. Int J Comput Appl. 2020; 177 (40):31–39. [ Google Scholar ]
- Alzahrani A., Alshehri A., Alshahrani H., Alharthi R., Fu H., Liu A., Zhu Y. 2018 IEEE International Conference on Electro/Information Technology (EIT) IEEE; 2018. Randroid: Structural similarity approach for detecting ransomware applications in android platform; pp. 0892–0897. [ Google Scholar ]
- Ami O., Elovici Y., Hendler D. Proceedings of the 33rd Annual ACM Symposium on Applied Computing. 2018. Ransomware prevention using application authentication-based file access control; pp. 1610–1619. [ Google Scholar ]
- Andronio N., Zanero S., Maggi F. Springer-Verlag; Berlin, Heidelberg: 2015. Heldroid: Dissecting and detecting mobile ransomware; pp. 382–404. [ CrossRef ] [ Google Scholar ]
- Aslan O., Samet R. A comprehensive review on malware detection approaches. IEEE Access. 2020; 8 :6249–6271. [ Google Scholar ]
- Aurangzeb S., Aleem M., Iqbal M., Islam M., et al. Ransomware: a survey and trends. J. Inf. Assur. Secur. 2017; 6 (2):48–58. [ Google Scholar ]
- Ayub M.A., Continella A., Siraj A. 2020. An i/o request packet (irp) driven effective ransomware detection scheme using artificial neural network; pp. 319–324. [ CrossRef ] [ Google Scholar ]
- Azmoodeh A., Dehghantanha A., Conti M., Choo K.-K.R. Detecting crypto-ransomware in iot networks based on energy consumption footprint. J Ambient Intell Humaniz Comput. 2018; 9 (4):1141–1152. [ Google Scholar ]
- Bae S., Lee G., Im E. Ransomware detection using machine learning algorithms. Concurrency and Computation: Practice and Experience. 2020; 32 (18):e5422. [ Google Scholar ]
- Baek S., Jung Y., Mohaisen A., Lee S., Nyang D. 2018 IEEE 38th International Conference on Distributed Computing Systems (ICDCS) IEEE; 2018. Ssd-insider: Internal defense of solid-state drive against ransomware with perfect data recovery; pp. 875–884. [ Google Scholar ]
- Bajpai P., Enbody R. Attacking key management in ransomware. IT Prof. 2020; 22 (2):21–27. [ Google Scholar ]
- Bajpai P., Enbody R. Dissecting.net ransomware: key generation, encryption and operation. Network Security. 2020; 2020 (2):8–14. [ Google Scholar ]
- Bajpai P., Enbody R. 2020 IEEE International Conference on Electro Information Technology (EIT) 2020. An empirical study of api calls in ransomware; pp. 443–448. [ CrossRef ] [ Google Scholar ]
- Bajpai P., Sood A.K., Enbody R. 2018 APWG Symposium on Electronic Crime Research (eCrime) 2018. A key-management-based taxonomy for ransomware; pp. 1–12. [ CrossRef ] [ Google Scholar ]
- Baldwin J., Dehghantanha A. Cyber Threat Intelligence. Springer; 2018. Leveraging Support Vector Machine for Opcode Density Based Detection of Crypto-ransomware; pp. 107–136. [ Google Scholar ]
- Bekerman D., Shapira B., Rokach L., Bar A. 2015 IEEE Conference on Communications and Network Security (CNS) IEEE; 2015. Unknown malware detection using network traffic classification; pp. 134–142. [ Google Scholar ]
- Berrueta Irigoyen E., Morató Osés D., Magaña Lizarrondo E., Izal Azcárate M. A survey on detection techniques for cryptographic ransomware. IEEE Access, 2019, 7, 144925–144944. 2019 [ Google Scholar ]
- Brewer R. Ransomware attacks: detection, prevention and cure. Network Security. 2016; 2016 (9):5–9. [ Google Scholar ]
- Cabaj K., Gregorczyk M., Mazurczyk W. Software-defined networking-based crypto ransomware detection using http traffic characteristics. Computers & Electrical Engineering. 2018; 66 :353–368. [ Google Scholar ]
- Cabaj K., Mazurczyk W. Using software-defined networking for ransomware mitigation: the case of cryptowall. IEEE Netw. 2016; 30 (6):14–20. [ Google Scholar ]
- Chadha S., Kumar U. 2017 International Conference on Computing, Communication and Automation (ICCCA) IEEE; 2017. Ransomware: Let’s fight back! pp. 925–930. [ Google Scholar ]
- Chen Q., Bridges R.A. 2017 16th IEEE International Conference on Machine Learning and Applications (ICMLA) 2017. Automated behavioral analysis of malware: A case study of wannacry ransomware; pp. 454–460. [ CrossRef ] [ Google Scholar ]
- Chung M. Why employees matter in the fight against ransomware. Computer Fraud & Security. 2019; 2019 (8):8–11. [ Google Scholar ]
- Cicala F., Bertino E. Analysis of encryption key generation in modern crypto ransomware. IEEE Trans Dependable Secure Comput. 2020 doi: 10.1109/TDSC.2020.3005976. [ CrossRef ] [ Google Scholar ] 1–1
- Cohen A., Nissim N. Trusted detection of ransomware in a private cloud using machine learning methods leveraging meta-features from volatile memory. Expert Syst Appl. 2018; 102 :158–178. [ Google Scholar ]
- Continella A., Guagnelli A., Zingaro G., Pasquale G.D., Barenghi A., Zanero S., Maggi F. Proceedings of the 32nd Annual Conference on Computer Security Applications. 2016. Shieldfs: a self-healing, ransomware-aware filesystem; pp. 336–347. [ Google Scholar ]
- Cosic J., Schlehuber C., Morog D. 2019 IEEE 15th International Scientific Conference on Informatics. 2019. New challenges in forensic analysis in railway domain; pp. 000061–000064. [ CrossRef ] [ Google Scholar ]
- Creating a simple free malware analysis environment, 2017 https://www.malwaretech.com/2017/11/creating-a-simple-free-malware-analysis-environment.html .
- Curran K. Cyber security and the remote workforce. Computer Fraud & Security. 2020; 2020 (6):11–12. [ Google Scholar ]
- Cusack G., Michel O., Keller E. Proceedings of the 2018 ACM International Workshop on Security in Software Defined Networks & Network Function Virtualization. 2018. Machine learning-based detection of ransomware using sdn; pp. 1–6. [ Google Scholar ]
- file i/o, 2021 https://www.pcmag.com/encyclopedia/term/file-io .
- for Cyber Security, C. C., 2018. Ransomware: How to prevent and recover (itsap.00.099). https://www.cyber.gc.ca/en/guidance/ransomware-how-prevent-and-recover-itsap00099 .
- Dargahi T., Dehghantanha A., Bahrami P.N., Conti M., Bianchi G., Benedetto L. A cyber-kill-chain based taxonomy of crypto-ransomware features. Journal of Computer Virology and Hacking Techniques. 2019; 15 :277–305. [ Google Scholar ]
- Delgado-Mohatar O., Sierra-Cámara J., Anguiano E. Blockchain-based semi-autonomous ransomware. Future Generation Computer Systems. 2020 [ Google Scholar ]
- Genç Z., Lenzini G., Ryan P. International Conference on Detection of Intrusions and Malware, and Vulnerability Assessment. Springer; 2018. No random, no ransom: a key to stop cryptographic ransomware; pp. 234–255. [ Google Scholar ]
- Gomez-Hernandez J., Alvarez-Gonzalez L., Garcia-Teodoro P. R-Locker: thwarting ransomware action through a honeyfile-based approach. Computers & Security. 2018; 73 :389–398. [ Google Scholar ]
- Groenewegen A., Alqabandi M., Elamin M., Paardekooper P. 2020. A behavioral analysis of the ransomware strain nefilim. [ CrossRef ] [ Google Scholar ]
- Güera D., Delp E. 2018 15th IEEE International Conference on Advanced Video and Signal Based Surveillance (AVSS) IEEE; 2018. Deepfake video detection using recurrent neural networks; pp. 1–6. [ Google Scholar ]
- Hakak S., Khan W., Gilkar G., Assiri B., Alazab M., Bhattacharya S., Reddy G. Recent advances in blockchain technology: a survey on applications and challenges. arXiv preprint arXiv:2009.05718. 2020 [ Google Scholar ]
- Hakak S., Khan W., Gilkar G., Imran M., Guizani N. Securing smart cities through blockchain technology: architecture, requirements, and challenges. IEEE Netw. 2020; 34 (1):8–14. [ Google Scholar ]
- Hakak S., Khan W., Imran M., Choo K., Shoaib M. Have you been a victim of covid-19-related cyber incidents? survey, taxonomy, and mitigation strategies. IEEE Access. 2020; 8 :124134–124144. [ PMC free article ] [ PubMed ] [ Google Scholar ]
- Hakak, S., Ray, S., Khan, W., Scheme, E., 2020. A framework for edge-assisted healthcare data analytics using federated learning.
- Hakak S., W.Z. Khan W.Z., Gilkar G.A., Haider N., Imran M., Alkatheiri M.S. Industrial wastewater management using blockchain technology: architecture, requirements, and future directions. IEEE Internet of Things Magazine. 2020; 3 (2):38–43. [ Google Scholar ]
- Hassan N. Ransomware Revealed. Springer; 2019. Ransomware Families; pp. 47–68. [ Google Scholar ]
- Homayoun S., Dehghantanha A., Ahmadzadeh M., Hashemi S., Khayami R., Choo K., Newton D. Drthis: deep ransomware threat hunting and intelligence system at the fog layer. Future Generation Computer Systems. 2019; 90 :94–104. [ Google Scholar ]
- Huang J., Xu J., Xing X., Liu P., Qureshi M.K. Proceedings of the 2017 ACM SIGSAC Conference on Computer and Communications Security. 2017. Flashguard: Leveraging intrinsic flash properties to defend against encryption ransomware; pp. 2231–2244. [ Google Scholar ]
- Hull G., John H., Arief B. Ransomware deployment methods and analysis: views from a predictive model and human responses. Crime Sci. 2019; 8 (1):2. [ Google Scholar ]
- Jain G., Rani N. Springer Singapore; 2020. Awareness learning analysis of malware and ransomware in bitcoin; pp. 765–776. [ Google Scholar ]
- Javaheri D., Hosseinzadeh M., Rahmani A. Detection and elimination of spyware and ransomware by intercepting kernel-level system routines. IEEE Access. 2018; 6 :78321–78332. [ Google Scholar ]
- Jung S., Won Y. Ransomware detection method based on context-aware entropy analysis. Soft comput. 2018; 22 (20):6731–6740. [ Google Scholar ]
- Kara I., Aydos M. 2020 11th IEEE Annual Ubiquitous Computing, Electronics Mobile Communication Conference (UEMCON) 2020. Cyber fraud: Detection and analysis of the crypto-ransomware; pp. 0764–0769. [ CrossRef ] [ Google Scholar ]
- Karapapas C., Pittaras I., Fotiou N., Polyzos G.C. 2020 IEEE International Conference on Blockchain and Cryptocurrency (ICBC) 2020. Ransomware as a service using smart contracts and ipfs; pp. 1–5. [ CrossRef ] [ Google Scholar ]
- Kathareios G., Anghel A., Mate A., Clauberg R., Gusat M. Catch it if you can: real-time network anomaly detection with low false alarm rates. 2017 16th IEEE International Conference on Machine Learning and Applications (ICMLA) 2017 doi: 10.1109/icmla.2017.00-36. [ CrossRef ] [ Google Scholar ]
- Keijzer N. The new generation of ransomware: an in depth study of Ransomware-as-a-Service. University of Twente; 2020. [ Google Scholar ]
- Khammas B. Ransomware detection using random forest technique. ICT Express. 2020; 6 (4):325–331. [ Google Scholar ]
- Khan F., Ncube C., Ramasamy L.K., Kadry S., Nam Y. A digital dna sequencing engine for ransomware detection using machine learning. IEEE Access. 2020; 8 :119710–119719. doi: 10.1109/ACCESS.2020.3003785. [ CrossRef ] [ Google Scholar ]
- Kharaz A., Arshad S., Mulliner C., Robertson W., Kirda E. 25th { USENIX } Security Symposium ( { USENIX } Security 16) 2016. { UNVEIL } : A large-scale, automated approach to detecting ransomware; pp. 757–772. [ Google Scholar ]
- Kharraz A., Kirda E. International Symposium on Research in Attacks, Intrusions, and Defenses. Springer; 2017. Redemption: Real-time protection against ransomware at end-hosts; pp. 98–119. [ Google Scholar ]
- Kim D., Lee J. Blacklist vs. whitelist-based ransomware solutions. IEEE Consum. Electron. Mag. 2020; 9 (3):22–28. doi: 10.1109/MCE.2019.2956192. [ CrossRef ] [ Google Scholar ]
- Kok S., Abdullah A., Jhanjhi N. Early detection of crypto-ransomware using pre-encryption detection algorithm. Journal of King Saud University-Computer and Information Sciences. 2020 [ Google Scholar ]
- Kok S., Abdullah A., Jhanjhi N., Supramaniam M. Prevention of crypto-ransomware using a pre-encryption detection algorithm. Computers. 2019; 8 (4):79. [ Google Scholar ]
- Kok S., Abdullah A., Jhanjhi N., Supramaniam M. Ransomware, threat and detection techniques: areview. Int. J. Comput. Sci. Netw. Secur. 2019; 19 (2):136. [ Google Scholar ]
- Kolodenker E., Koch W., Stringhini G., Egele M. Proceedings of the 2017 ACM on Asia Conference on Computer and Communications Security. 2017. Paybreak: Defense against cryptographic ransomware; pp. 599–611. [ Google Scholar ]
- Komatwar R., Kokare M. A survey on malware detection and classification. Journal of Applied Security Research. 2020:1–31. [ Google Scholar ]
- Lallie H., Shepherd L., Nurse J., Erola A., Epiphaniou G., Maple C., Bellekens X. Cyber security in the age of covid-19: a timeline and analysis of cyber-crime and cyber-attacks during the pandemic. arXiv preprint arXiv:2006.11929. 2020 [ Google Scholar ]
- Lee K., Lee S., Yim K. Machine learning based file entropy analysis for ransomware detection in backup systems. IEEE Access. 2019; 7 :110205–110215. [ Google Scholar ]
- Lee K., Yim K., Seo J. Ransomware prevention technique using key backup. Concurrency and Computation: Practice and Experience. 2018; 30 (3):e4337. [ Google Scholar ]
- Liu X., Li H., Xu G., Lu R., He M. Adaptive privacy-preserving federated learning. PEER-TO-PEER NETWORKING AND APPLICATIONS. 2020 [ Google Scholar ]
- Ltd., S., 2020. Paying the ransom doubles cost of recovering from a ransomware attack, according to sophos. https://www.globenewswire.com/news-release/2020/05/12/2031961/0/en/Paying-the-Ransom-Doubles-Cost-of-Recovering-from-a-Ransomware-Attack-According-to-Sophos.html .
- Mackenzie P. Wannacry aftershock. Sophos, disponible en ligne: https://www. sophos. com/en-us/medialibrary/PDFs/technical-papers/WannaCry-Aftershock. pdf. 2019 [ Google Scholar ]
- Maimó L., Celdran A., Gomez A., Clemente F., Weimer J., Lee I. Intelligent and dynamic ransomware spread detection and mitigation in integrated clinical environments. Sensors. 2019; 19 (5):1114. doi: 10.3390/s19051114. [ PMC free article ] [ PubMed ] [ CrossRef ] [ Google Scholar ]
- Manavi F., Hamzeh A. A new method for ransomware detection based on pe header using convolutional neural networks. 2020 17th International ISC Conference on Information Security and Cryptology (ISCISC) 2020 doi: 10.1109/ISCISC51277.2020.9261903. [ CrossRef ] [ Google Scholar ]
- Mattei T. Privacy, confidentiality, and security of health care information: lessons from the recent wannacry cyberattack. World Neurosurg. 2017; 104 :972–974. [ PubMed ] [ Google Scholar ]
- McIntosh T., Watters P., Kayes A., Ng A., Chen Y. Enforcing situation-aware access control to build malware-resilient file systems. Future Generation Computer Systems. 2021; 115 :568–582. doi: 10.1016/j.future.2020.09.035. [ CrossRef ] [ Google Scholar ]
- Mehnaz S., Mudgerikar A., Bertino E. International Symposium on Research in Attacks, Intrusions, and Defenses. Springer; 2018. Rwguard: A real-time detection system against cryptographic ransomware; pp. 114–136. [ Google Scholar ]
- Meland P., Bayoumy Y., Sindre G. The ransomware-as-a-service economy within the darknet. Computers & Security. 2020:101762. [ Google Scholar ]
- Min D., Park D., Ahn J., Walker R., Lee J., Park S., Kim Y. Amoeba: an autonomous backup and recovery ssd for ransomware attack defense. IEEE Comput. Archit. Lett. 2018; 17 (2):245–248. [ Google Scholar ]
- Monika, Zavarsky P., Lindskog D. Experimental analysis of ransomware on windows and android platforms: evolution and characterization. Procedia Comput Sci. 2016; 94 :465–472. [ Google Scholar ]
- Moore C. 2016 Cybersecurity and Cyberforensics Conference (CCC) IEEE; 2016. Detecting ransomware with honeypot techniques; pp. 77–81. [ Google Scholar ]
- Morato D., Berrueta E., Magaña E., Izal M. Ransomware early detection by the analysis of file sharing traffic. Journal of Network and Computer Applications. 2018; 124 :14–32. [ Google Scholar ]
- Mukherjee M., Shu L., Wang D. Survey of fog computing: fundamental, network applications, and research challenges. IEEE Communications Surveys & Tutorials. 2018; 20 (3):1826–1857. [ Google Scholar ]
- Muslim A., Dzulkifli D., Nadhim M.H., Abdellah R. 2019. A study of ransomware attacks: Evolution and prevention. [ Google Scholar ]
- Nadir I., Bakhshi T. 2018 International Conference on Computing, Mathematics and Engineering Technologies (iCoMET) 2018. Contemporary cybercrime: A taxonomy of ransomware threats mitigation techniques; pp. 1–7. [ CrossRef ] [ Google Scholar ]
- Nahmias D., Cohen A., Nissim N., Elovici Y. Deep feature transfer learning for trusted and automated malware signature generation in private cloud environments. Neural Networks. 2020; 124 :243–257. [ PubMed ] [ Google Scholar ]
- Naseer A., Mir R., Mir A., Aleem M. Windows-based ransomware: a survey. Journal of Information Assurance & Security. 2020; 15 (3) [ Google Scholar ]
- Natanzon, A., Derbeko, P., Stern, U., Bakshi, M., Manusov, Y., 2018. Ransomware detection using i/o patterns. US Patent 10,078,459.
- Or-Meir O., Nissim N., Elovici Y., Rokach L. Dynamic malware analysis in the modern era’a state of the art survey. ACM Computing Surveys (CSUR) 2019; 52 (5):1–48. [ Google Scholar ]
- Or-Meir O., Nissim N., Elovici Y., Rokach L. Dynamic malware analysis in the modern era’a state of the art survey. ACM Comput. Surv. 2019; 52 (5) doi: 10.1145/3329786. [ CrossRef ] [ Google Scholar ]
- Palanisamy R., Norman A., Kiah M. Byod policy compliance: risks and strategies in organizations. Journal of Computer Information Systems. 2020:1–12. [ Google Scholar ]
- Parkinson S. Use of access control to minimise ransomware impact. Network Security. 2017; 2017 (7):5–8. [ Google Scholar ]
- Pham Q., Fang F., Ha V., Piran M., Le M., Le L., Hwang W., Ding Z. A survey of multi-access edge computing in 5g and beyond: fundamentals, technology integration, and state-of-the-art. IEEE Access. 2020; 8 :116974–117017. [ Google Scholar ]
- Poudyal S., Dasgupta D., Akhtar Z., Gupta K. 14th International Conference on Malicious and Unwanted Software” MALCON. 2019. A multi-level ransomware detection framework using natural language processing and machine learning. [ Google Scholar ]
- Poudyal S., Subedi K.P., Dasgupta D. 2018 IEEE Symposium Series on Computational Intelligence (SSCI) IEEE; 2018. A framework for analyzing ransomware using machine learning; pp. 1692–1699. [ Google Scholar ]
- Pranggono B., Arabo A. Covid-19 pandemic cybersecurity issues. Internet Technology Letters. 2020; n/a (n/a) doi: 10.1002/itl2.247. [ CrossRef ] [ Google Scholar ]
- Puat H., Rahman N. Ransomware as a service and public awareness. PalArch’s Journal of Archaeology of Egypt/Egyptology. 2020; 17 (7):5277–5292. [ Google Scholar ]
- Qin B., Wang Y., Ma C. 2020 International Conference on Big Data, Artificial Intelligence and Internet of Things Engineering (ICBAIE) 2020. Api call based ransomware dynamic detection approach using textcnn; pp. 162–166. [ CrossRef ] [ Google Scholar ]
- Quinkert, F., Holz, T., Hossain, K., Ferrara, E., Lerman, K., 2018a. Raptor: Ransomware attack predictor. 1803.01598.
- Quinkert F., Holz T., Hossain K., Ferrara E., Lerman K. Raptor: ransomware attack predictor. arXiv preprint arXiv:1803.01598. 2018 [ Google Scholar ]
- Ramesh G., Menen A. Automated dynamic approach for detecting ransomware using finite-state machine. Decis Support Syst. 2020; 138 :113400. [ Google Scholar ]
- Richardson R., North M. Ransomware: evolution, mitigation and prevention. International Management Review. 2017; 13 (1):10–21. [ Google Scholar ]
- Saeed M. Malware in computer systems: problems and solutions. IJID (International Journal on Informatics for Development) 2020; 9 (1):1–8. [ Google Scholar ]
- Salehi S., Shahriari H., Ahmadian M.M., Tazik L. 2018 15th International ISC (Iranian Society of Cryptology) Conference on Information Security and Cryptology (ISCISC) 2018. A novel approach for detecting dga-based ransomwares; pp. 1–7. [ CrossRef ] [ Google Scholar ]
- Scaife N., Carter H., Traynor P., Butler K.R.B. 2016 IEEE 36th International Conference on Distributed Computing Systems (ICDCS) 2016. Cryptolock (and drop it): Stopping ransomware attacks on user data; pp. 303–312. [ CrossRef ] [ Google Scholar ]
- Sgandurra D., Muñoz-González L., Mohsen R., Lupu E.C. Automated dynamic analysis of ransomware: benefits, limitations and use for detection. arXiv preprint arXiv:1609.03020. 2016 [ Google Scholar ]
- Sharafaldin I., Lashkari A., Hakak S., Ghorbani A. 2019 International Carnahan Conference on Security Technology (ICCST) IEEE; 2019. Developing realistic distributed denial of service (ddos) attack dataset and taxonomy; pp. 1–8. [ Google Scholar ]
- Sharmeen S., Ahmed Y.A., Huda S., Koçer B.A., Hassan M.M. Avoiding future digital extortion through robust protection against ransomware threats using deep learning based adaptive approaches. IEEE Access. 2020; 8 :24522–24534. doi: 10.1109/ACCESS.2020.2970466. [ CrossRef ] [ Google Scholar ]
- Shaukat S., Ribeiro V. 2018 10th International Conference on Communication Systems & Networks (COMSNETS) IEEE; 2018. Ransomwall: A layered defense system against cryptographic ransomware attacks using machine learning; pp. 356–363. [ Google Scholar ]
- Shijo P., Salim A. Integrated static and dynamic analysis for malware detection. Procedia Comput Sci. 2015; 46 :804–811. [ Google Scholar ]
- Silva J., Hernandez-Alvarez M. 2017 IEEE Second Ecuador Technical Chapters Meeting (ETCM) IEEE; 2017. Large scale ransomware detection by cognitive security; pp. 1–4. [ Google Scholar ]
- Srinivasan C. Hobby hackers to billion-dollar industry: the evolution of ransomware. Computer Fraud & Security. 2017; 2017 (11):7–9. doi: 10.1016/S1361-3723(17)30081-7. [ CrossRef ] [ Google Scholar ]
- Tailor J., Patel A. A comprehensive survey: ransomware attacks prevention, monitoring and damage control. International Journal of Research and Scientific Innovation (IJRSI) 2017; 4 :2321–2705. [ Google Scholar ]
- Takeuchi Y., Sakai K., Fukumoto S. Proceedings of the 47th International Conference on Parallel Processing Companion. 2018. Detecting ransomware using support vector machines; pp. 1–6. [ Google Scholar ]
- Thezoo, 2021 https://github.com/ytisf/theZoo/tree/master/malwares/Binaries .
- Thomas J. Individual cyber security: empowering employees to resist spear phishing to prevent identity theft and ransomware attacks. Thomas, JE (2018). Individual cyber security: Empowering employees to resist spear phishing to prevent identity theft and ransomware attacks. International Journal of Business Management. 2018; 12 (3):1–23. [ Google Scholar ]
- Thomas J., Galligher G. Improving backup system evaluations in information security risk assessments to combat ransomware. Computer and Information Science. 2018; 11 (1) [ Google Scholar ]
- url, 2021 https://www.sophos.com/en-us/press-office/press-releases/2021/04/ransomware-recovery-cost-reaches-nearly-dollar-2-million-more-than-doubling-in-a-year.aspx .
- Walker A., Sengupta S. MILCOM 2019-2019 IEEE Military Communications Conference (MILCOM) IEEE; 2019. Insights into malware detection via behavioral frequency analysis using machine learning; pp. 1–6. [ Google Scholar ]
- Wang Z., Huang D., Zhu Y., Li B., Chung C. Efficient attribute-based comparable data access control. IEEE Trans. Comput. 2015; 64 (12):3430–3443. [ Google Scholar ]
- What is the difference between api and system call. 2018 https://pediaa.com/what-is-the-difference-between-api-and-system-call .
- Wilner A., Jeffery A., Lalor J., Matthews K., Robinson K., Rosolska A., Yorgoro C. On the social science of ransomware: technology, security, and society. Comparative Strategy. 2019; 38 (4):347–370. [ Google Scholar ]
- Yang Q., Liu Y., Chen T., Tong Y. Federated machine learning: concept and applications. ACM Transactions on Intelligent Systems and Technology (TIST) 2019; 10 (2):1–19. [ Google Scholar ]
- Yaqoob I., Ahmed E., ur Rehman M., Ahmed A., Al-garadi M., Imran M., Guizani M. The rise of ransomware and emerging security challenges in the internet of things. Comput. Networks. 2017; 129 :444–458. [ Google Scholar ]
- Zhang B., Xiao W., Xiao X., Sangaiah A., Zhang W., Zhang J. Ransomware classification using patch-based cnn and self-attention network on embedded n-grams of opcodes. Future Generation Computer Systems. 2020; 110 :708–720. [ Google Scholar ]
- Zhang-Kennedy L., Assal H., Rocheleau J., Mohamed R., Baig K., Chiasson S. 27th { USENIX } Security Symposium ( { USENIX } Security 18) 2018. The aftermath of a crypto-ransomware attack at a large academic institution; pp. 1061–1078. [ Google Scholar ]
- Zimba A., Mulenga M. A dive into the deep: demystifying wannacry crypto ransomware network attacks via digital forensics. International Journal on Information Technologies and Security. 2018; 10 :57–68. [ Google Scholar ]
- Zimba A., Wang Z., Chen H., Mulenga M. Recent advances in cryptovirology: state-of-the-art crypto mining and crypto ransomware attacks. KSII Trans. Internet Inf. Syst. 2019; 13 :3258–3279. doi: 10.3837/tiis.2019.06.027. [ CrossRef ] [ Google Scholar ]
We use cookies for security purposes, to improve your experience on our site and tailor content for you. Our Privacy Statement explains how we use cookies.
The following form allows you to search all of BT.
- Practice management
- Managing your business
Case studies – malware attacks
As our lives increasingly move online, cybersecurity is an important consideration for all businesses, including financial advice businesses. For many financial advisers understanding how to protect sensitive client information from cyber attacks is becoming an important part of sound practice management.
A cyber attack is essentially an attempt by hackers to damage or destroy a computer network or system. One of the ways they can do this, is by installing malware (also known as malicious software)on your computer that allows unauthorised access to your files and can allow your activity to be watched without you knowing. Cyber criminals can then steal personal information and login details for secure websites to commit fraudulent activities.
In this article we discuss steps financial advisers can take to protect themselves from cyber attacks and explore different scenarios that demonstrate what a cyber attack can look like and how it can be prevented.
How can financial advisers improve their cyber security?
- Turn on auto-updates for your business operating system – such as windows or Apple’s ios, and be sure to keep computer security up to date with anti-virus and anti-spyware, as well as a good firewall.
- Back up important data – to an external hard drive, to a USB or a cloud to protect your business from lost data.
- Enable multi-factor authentication – start using two or more proofs of identity such as a PIN, passphrase, card or token, or finger print before access is enabled.
- Implement premissions on a ‘need to know’ basis – your employees don’t need to access everything. Be selective about what permissions are allowed to which staff.
- Conduct regular employee cyber training. Show staff how to ‘recognise, avoid, report, remove and recover’. Your employees can be your defence against cyber crime. Reward staff for their efforts; and
- Always be cautious of the below when receiving emails: - requests for money, especially urgent or overdue - Bank account changes - Attachments, especially from unknown or suspicious email addresses - Requests to check or confirm login details
Case studies - malware attacks
Protect yourself and your business
Cyber security assessment tool
The Department of Industry, Science, Energy and Resources has developed a tool to help you identify your business' cyber security strengths and areas where your business can improve. This tool will ask you a series of questions about how you manage your cyber security risks and based on your answers, you will receive a list of recommendations to action. You can download the recommendations as a PDF and access the tool here.
Scenario 1 – Advisory practices attacked by a trojan virus
Scenario 2 - Adviser subject to a malware attack causing account lock
Scenario 3 - opening email attachment causes all pcs in the office to shutdown, scenario 1 - advisory practices attacked by a trojan virus.
In this scenario, a number of advisory practices were subject to a targeted malware attack via a Trojan virus. This virus helped the cyber criminals access several advisers’ PCs and obtain the login details for systems that had been used.
This attempted fraud took place while the practice was closed over the Christmas holidays.
"We locked up the office that afternoon just before Christmas and went home. We were all looking forward to a nice long break, it’d been a busy year. We wouldn’t be back in the office until the New Year."
Transactions were submitted to the platform over the Christmas period using several advisers’ user IDs.
Direct credit (EFT) bank account details were edited to credit the cyber criminals' ‘mule’ Australian bank account. From this account the cyber criminals would be free to transfer the funds overseas.
Luckily for the practice, the fraud was uncovered before any funds were paid out.
"Even though we were on holiday, we all continued to check our transaction updates via the platform each day. We called the platform right away and they were able to stop the fraudulent payments in time."
Preventing this type of fraud
- Be diligent about checking platform transaction updates sent by email or displayed online. Specifically look out for withdrawal requests, new accounts opened, asset sell downs and changes to contact details.
- When taking annual leave, nominate a colleague to check platform transaction updates on your behalf in your absence.
- Call us immediately if you suspect fraud or malware on your system. We’ll suspend your login ID to attempt to prevent further fraudulent transactions.
- Bring in a tech specialist immediately to run and update security software and restore your systems back to normal.
A Melbourne advisory practice was the target of a malware attack, having found malware on their system which locked their access to the platform. The malware allowed the cyber criminal to gain access to an adviser’s login details for all systems he had used recently.
The cyber criminals now had access to every website or account that required a login. This included personal banking, platform desktop software, Xplan software and Facebook.
The next time the adviser tried to log in to his platform desktop software, he was locked out.
He rang our account executive team to report his access was locked. He couldn’t login, even though he was using his correct user name and password.
The platform reset his password. The next day when the adviser tried again to login, he was locked out of the system again.
It became obvious that the adviser’s user ID had been compromised. At this point, the user ID was deleted.
Where you have had your platform access locked or you suspect fraud or malware on your system call us immediately as part of your reporting response so we can suspend your login ID to attempt to prevent further fraudulent transactions. Bring in a tech specialist immediately to run and update security software and restore your systems back to normal.
- Be on the lookout for requests to check and confirm login details.
- Increase the strength of your identifiers and ensure two or more proofs of identity are required before access to company systems is enabled.
- Use virus protection software to prevent hackers from accessing your information and to help protect you if you click on a suspicious link or visit a fake website.
- Schedule regular training for employees so that they can better detect malicious links or avoid downloading content from untrustworthy sources.
A staff member in an advisory practice opened a file attached to an email received one morning.
It turned out the attachment contained a ‘worm’ that infected not only the staff member’s PC, it also spread to all other PCs in the practice network.
This malware caused all PCs in the office to shut down.
The adviser needed to use the platform software that day to ensure his clients participated in a Corporate Action that was closing the following day.
With help from their Business Development Manager, the office worked through the issue so they were able to log into the platform software to complete this critical work from a home laptop that hadn’t been infected with the virus.
- Never open attachments in emails if you don’t know or trust the source.
- Ensure your office network is protected with up-to-date anti-virus software.
- Call us immediately if you suspect fraud or malware on your system. We’ll suspend your login ID to attempt to prevent any further criminal activity.
- Bring in a tech specialist immediately to run and update security software and restore your systems back to normal.
MIT Technology Review
- Newsletters
How scientists traced a mysterious covid case back to six toilets
When wastewater surveillance turns into a hunt for a single infected individual, the ethics get tricky.
- Cassandra Willyard archive page
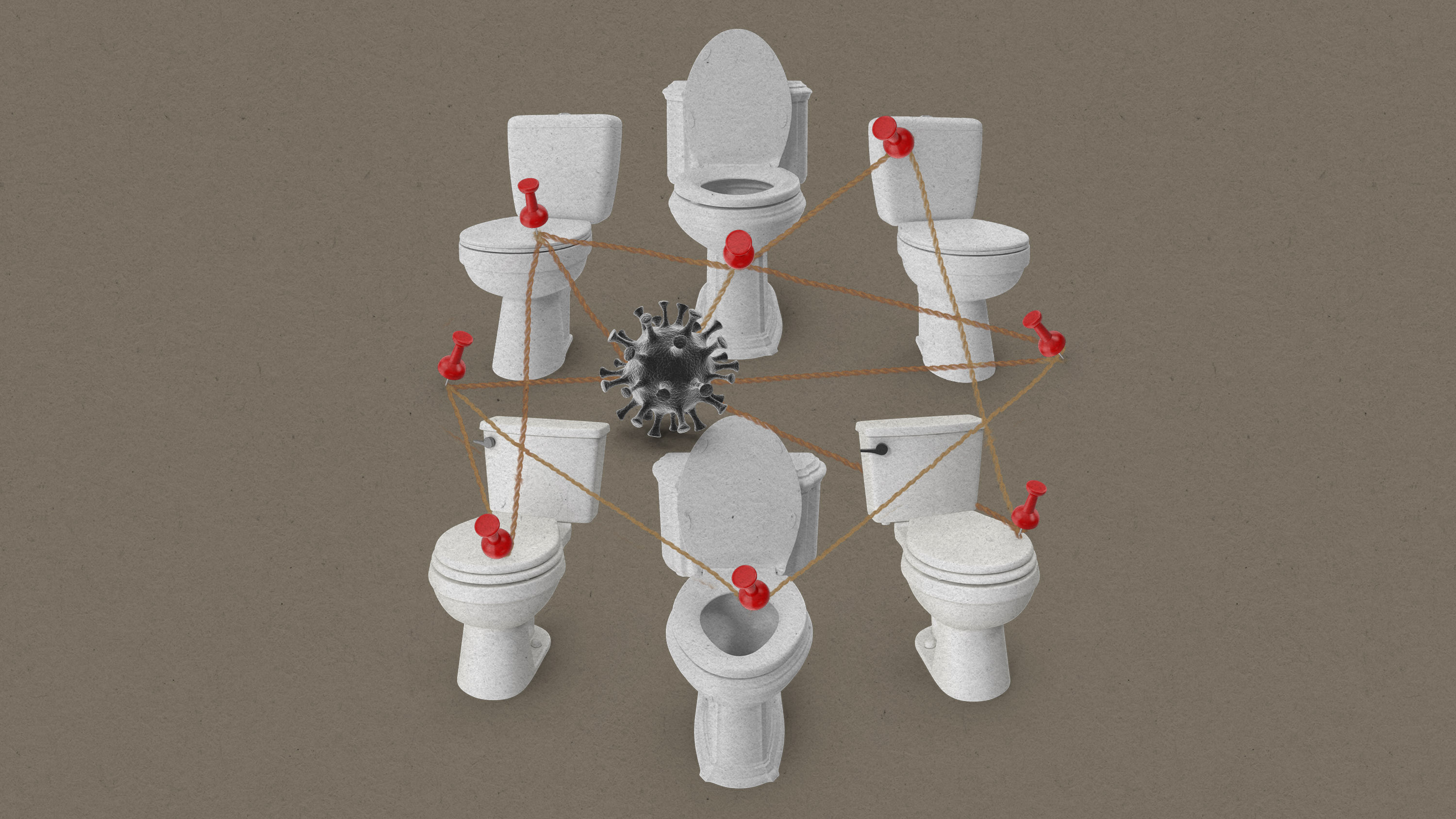
This article first appeared in The Checkup, MIT Technology Review’s weekly biotech newsletter. To receive it in your inbox every Thursday, and read articles like this first, sign up here .
This week I have a mystery for you. It’s the story of how a team of researchers traced a covid variant in Wisconsin from a wastewater plant to six toilets at a single company. But it’s also a story about privacy concerns that arise when you use sewers to track rare viruses back to their source.
That virus likely came from a single employee who happened to be shedding an enormous quantity of a very weird variant. The researchers would desperately like to find that person. But what if that person doesn’t want to be found?
A few years ago, Marc Johnson, a virologist at the University of Missouri, became obsessed with weird covid variants he was seeing in wastewater samples. The ones that caught his eye were odd in a couple of different ways: they didn’t match any of the common variants, and they didn’t circulate. They would pop up in a single location, persist for some length of time, and then often disappear—a blip. Johnson found his first blip in Missouri. “It drove me nuts,” he says. “I was like, ‘What the hell was going on here?’”
Then he teamed up with colleagues in New York, and they found a few more.
Hoping to pin down even more lineages, Johnson put a call out on Twitter (now X) for wastewater. In January 2022, he got another hit in a wastewater sample shipped from a Wisconsin treatment plant. He and David O’Connor, a virologist at the University of Wisconsin, started working with state health officials to track the signal—from the treatment plant to a pumping station and then to the outskirts of the city, “one manhole at a time,” Johnson says. “Every time there was a branch in the road, we would check which branch [the signal] was coming from.”
They chased some questionable leads. The researchers were suspicious the virus might be coming from an animal. At one point O’Connor took people from his lab to a dog park to ask dog owners for poop samples. “There were so many red herrings,” Johnson says.
Finally, after sampling about 50 manholes, the researchers found the manhole, the last one on the branch that had the variant. They got lucky. “The only source was this company,” Johnson says. Their results came out in March in Lancet Microbe .
Wastewater surveillance might seem like a relatively new phenomenon, born of the pandemic, but it goes back decades. A team of Canadian researchers outlines several historical examples in this story . In one example , a public health official traced a 1946 typhoid outbreak to the wife of a man who sold ice cream at the beach. Even then, the researcher expressed some hesitation. The study didn’t name the wife or the town, and he cautioned that infections probably shouldn’t be traced back to an individual “except in the presence of an outbreak.”
In a similar study published in 1959 , scientists traced another typhoid epidemic to one woman, who was then banned from food service and eventually talked into having her gallbladder removed to eliminate the infection. Such publicity can have a “devastating effect on the carrier,” they remarked in their write-up of the case. “From being a quiet and respected citizen, she becomes a social pariah.”
When Johnson and O’Connor traced the virus to that last manhole, things got sticky. Until that point, the researchers had suspected these cryptic lineages were coming from animals. Johnson had even developed a theory involving organic fertilizer from a source further upstream. Now they were down to a single building housing a company with about 30 employees. They didn’t want to stigmatize anyone or invade their privacy. But someone at the company was shedding an awful lot of virus. “Is it ethical to not tell them at that point?” Johnson wondered.
O’Connor and Johnson had been working with state health officials from the very beginning. They decided the best path forward would be to approach the company, explain the situation, and ask if they could offer voluntary testing. The decision wasn’t easy. “We didn’t want to cause panic and say there’s a dangerous new variant lurking in our community,” Ryan Westergaard, the state epidemiologist for communicable diseases at the Wisconsin Department of Health Services, told Nature . But they also wanted to try to help the person who was infected.
The company agreed to testing, and 19 of its 30 employees turned up for nasal swabs. They were all negative.
That may mean one of the people who didn’t test was carrying the infection. Or could it mean that the massive covid infection in the gut didn’t show up on a nasal swab? “This is where I would use the shrug emoji if we were doing this over email,” O’Connor says.
At the time, the researchers had the ability to test stool samples for the virus, but they didn’t have approval. Now they do, and they’re hoping stool will lead them to an individual infected with one of these strange viruses who can help answer some of their questions. Johnson has identified about 50 of these cryptic covid variants in wastewater. “The more I study these lineages, the more I am convinced that they are replicating in the GI tract,” Johnson says. “It wouldn’t surprise me at all if that’s the only place they were replicating.”
But how far should they go to find these people? That’s still an open question. O’Connor can imagine a dizzying array of problems that might arise if they did identify an individual shedding one of these rare variants. The most plausible hypothesis is that the lineages arise in individuals who have immune disorders that make it difficult for them to eliminate the infection. That raises a whole host of other thorny questions: what if that person had a compromised immune system due to HIV in addition to the strange covid variant? What if that person didn’t know they were HIV positive, or didn’t want to divulge their HIV status? What if the researchers told them about the infection, but the person couldn’t access treatment? “If you imagine what the worst-case scenarios are, they’re pretty bad,” O’Connor says.
On the other hand, O’Connor says, they think there are a lot of these people around the country and the world. “Isn't there also an ethical obligation to try to learn what we can so that we can try to help people who are harboring these viruses?” he asks.
Now read the rest of The Checkup
More from mit technology review.
Longevity specialists aim to help people live longer and healthier lives. But they have yet to establish themselves as a credible medical field. Expensive longevity clinics that cater to the wealthy worried well aren’t helping. Jessica Hamzelou takes us inside the quest to legitimize longevity medicine .
Drug developers bet big on AI to help speed drug development. But when will we see our first generative drug? Antonio Regalado has the story .
Read more from MIT Technology Review’s archive
The covid pandemic brought the tension between privacy and public health into sharp relief, wrote Karen Hao in 2020 .
That same year Genevieve Bell argued that we can reimagine contact tracing in a way that protects privacy.
In 2021, Antonio Regalado covered some of the first efforts to track the spread of covid variants using wastewater.
Earlier this year I wrote about using wastewater to track measles .
From around the web
Surgeons have transplanted a kidney from a genetically engineered pig into a 62-year-old man in Boston. ( New York Times ) → Surgeons transplanted a similar kidney into a brain-dead patient in 2021. ( MIT Technology Review ) → Researchers are also looking into how to transplant other organs. Just a few months ago, surgeons connected a genetically engineered pig liver to another brain-dead patient. ( MIT Technology Review )
The FDA has approved a new gene therapy for a rare but fatal genetic disorder in children. Its $4.25 million price tag will make it the world’s most expensive medicine, but it promises to give children with the disease a shot at a normal life. ( CNN ) → Read Antonio Regalado’s take on the curse of the costliest drug. ( MIT Technology Review )
People who practice intermittent fasting have an increased risk of dying of heart disease, according to new research presented at the American Heart Association meeting in Chicago. There are, of course, caveats. ( Washington Post and Stat )
Biotechnology and health
This baby with a head camera helped teach an ai how kids learn language.
A neural network trained on the experiences of a single young child managed to learn one of the core components of language: how to match words to the objects they represent.
The next generation of mRNA vaccines is on its way
Adding a photocopier gene to mRNA vaccines could make them last longer and curb side effects.
An AI-driven “factory of drugs” claims to have hit a big milestone
Insilico is part of a wave of companies betting on AI as the "next amazing revolution" in biology
- Antonio Regalado archive page
Ready, set, grow: These are the biotech plants you can buy now
For $73, I bought genetically modified tomato seeds and a glowing petunia.
Stay connected
Get the latest updates from mit technology review.
Discover special offers, top stories, upcoming events, and more.
Thank you for submitting your email!
It looks like something went wrong.
We’re having trouble saving your preferences. Try refreshing this page and updating them one more time. If you continue to get this message, reach out to us at [email protected] with a list of newsletters you’d like to receive.
Study finds bivalent COVID vaccine not tied to stroke risk
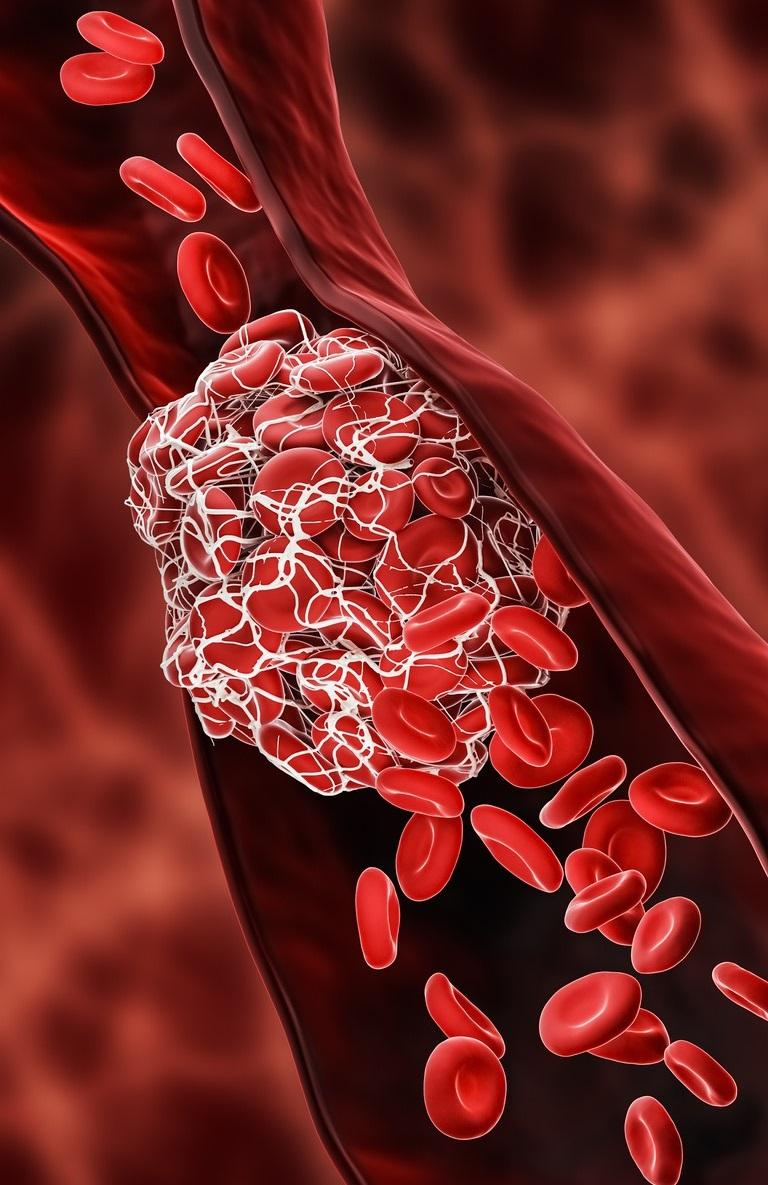
Earlier this week in JAMA , researchers published data on the risk of stroke among Medicare beneficiaries aged 65 years and older in the immediate weeks following a bivalent (two-strain) COVID-19 vaccine dose, finding no significantly elevated risk during the first 6 weeks following injection.
The study comes a year after reports appeared in the US Vaccine Safety Datalink showing that during the immediate period (1 to 21 days) after vaccination, older adults were suffering more strokes if they received both the Pfizer bivalent COVID vaccine and a high-dose or adjuvanted influenza vaccine on the same day.
The authors used Medicare recipient data to estimate the risk among those who received either the Pfizer or Moderna COVID-19 bivalent vaccine, concomitant (same-day) administration of either brand of COVID vaccine plus a high-dose or adjuvanted flu vaccine, and a high-dose or adjuvanted flu vaccine within the first 1 to 21-day or 22-to-42-day risk window compared with the 43-to-90-day control window. Adjuvanted vaccines include a substance that boosts immune response.
Possible stroke risk with high-dose flu vaccine
The study included 5,397,278 Medicare beneficiaries who received either brand of the COVID-19 bivalent vaccine (median age 74 years; 56% were women), 11,001 of whom had a stroke during the study period.
The authors found no significant association for stroke with either COVID vaccine, but same-day administration of either brand and a high-dose or adjuvanted influenza vaccine was associated with an elevated risk of stroke.
The observed association between vaccination and stroke in the concomitant subgroup was likely driven by a high-dose or adjuvanted influenza vaccination.
"The current study additionally found an association that was small in magnitude between stroke and administration of a high-dose or adjuvanted influenza vaccine when the vaccine was administered without concomitant administration of either brand of the COVID-19 bivalent vaccine," the authors concluded. "This finding suggests that the observed association between vaccination and stroke in the concomitant subgroup was likely driven by a high-dose or adjuvanted influenza vaccination.”
US flu activity declines, along with other respiratory viruses
Flu activity finally declined last week following a lengthy post-holiday bump, though levels are still elevated, the Centers for Disease Control and Prevention (CDC) said today in its latest weekly FluView report .
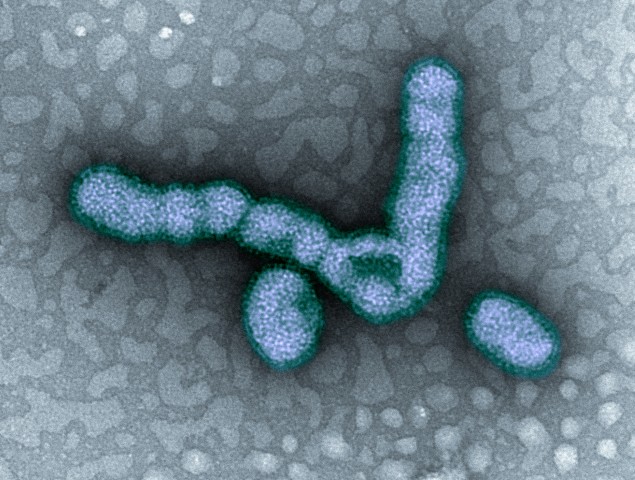
In its weekly respiratory virus snapshot , the CDC said indicators of COVID and respiratory syncytial virus (RSV) also declined. For all three viruses, test positivity and emergency department visits declined last week. Nine jurisdictions reported high or very high activity, down from 17 the previous week.
Flu up slightly in Pacific Northwest
For flu, activity decreased or was stable in nine regions but increased slightly in the Pacific Northwest. Of respiratory samples that were positive for flu at public health labs, 64.6% were influenza A, and of subtyped influenza A viruses, 56.1% were H3N2, and 43.9% were 2009 H1N1.
Hospitalizations have been declining since January, and overall deaths also trended downward. However, the CDC reported five more pediatric flu deaths, raising the total to 121 for the season. Two were due to H1N1, and three involved influenza B.
COVID wastewater detections decline to low level
In its latest COVID data updates , most indicators show a continuing steady decline, though deaths were level.
Wastewater detections of SARS-CoV-2 are now classified as low. Levels are still highest in the south, but they have declined steeply in recent weeks.
Vietnam probes human H5 avian flu case
Health officials in Vietnam's Khanh Hoa province have confirmed an H5 avian flu infection in a 21-year-old university student, though there are no details yet on the strain or how the patient contracted the virus.
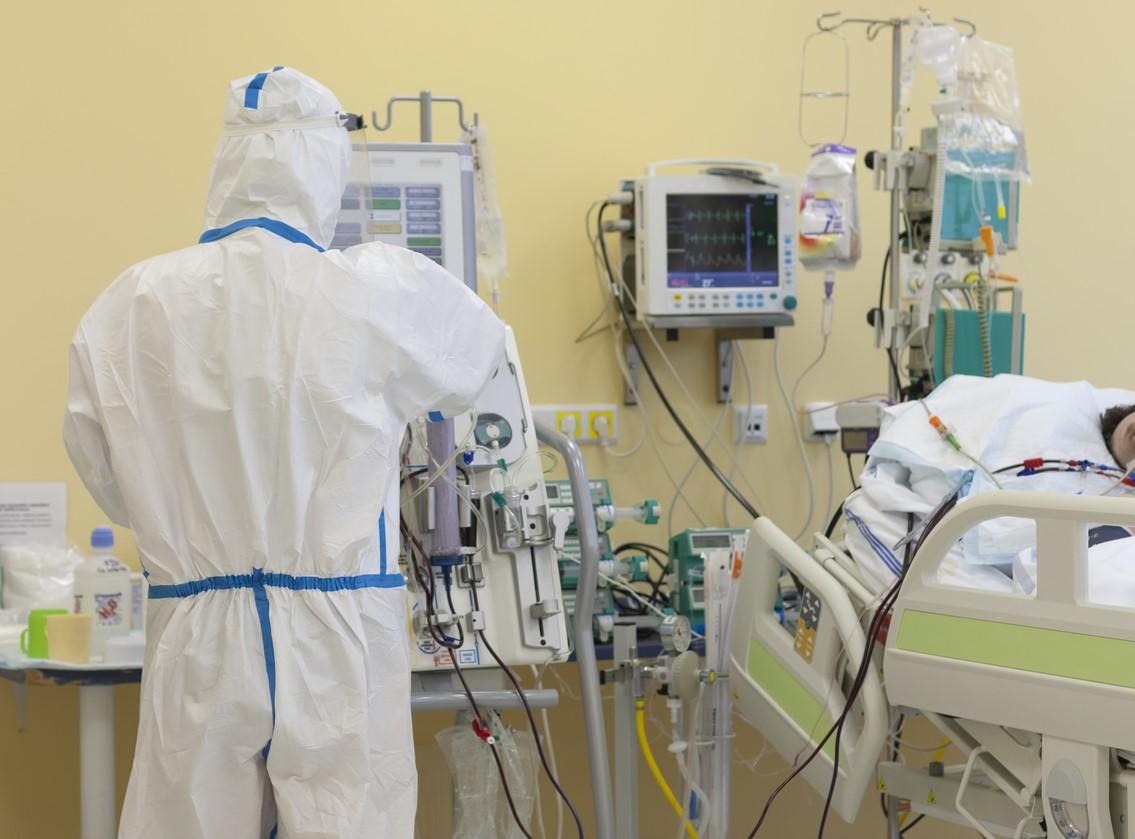
Details about the case were reported in Vietnamese media reports translated and posted by Avian Flu Diary , an infectious disease news blog. An official statement on the case from provincial health officials was translated and posted by FluTrackers , an inflection disease news message board. Khanh Hoa is on Vietnam's southeastern coast.
Man hospitalized in serious condition
The patient's symptoms began on March 11, and he returned home a few days later, where he stayed with his mother and sister while seeking medical care. When his symptoms worsened, he was hospitalized at a regional hospital. Soon after, he was transferred to Khanh Hoa provincial hospital where healthcare workers collected samples and sent them to the Pasteur Institute in Nha Trang, which revealed the H5 influenza virus.
The man is hospitalized in serious condition and in isolation at the Provincial Hospital of Tropical Diseases. Health officials are monitoring contacts, including family members, health workers, and university students.
So far, the subtype isn't known, but a few human cases involving older and recent H5N1 virus clades have been reported over the past few years, and China and Laos have reported H5N6 infections.
The reports didn't say how the patient likely contracted the virus.
Scientists caution about CDC guidance on over-the-counter drugs for COVID-19
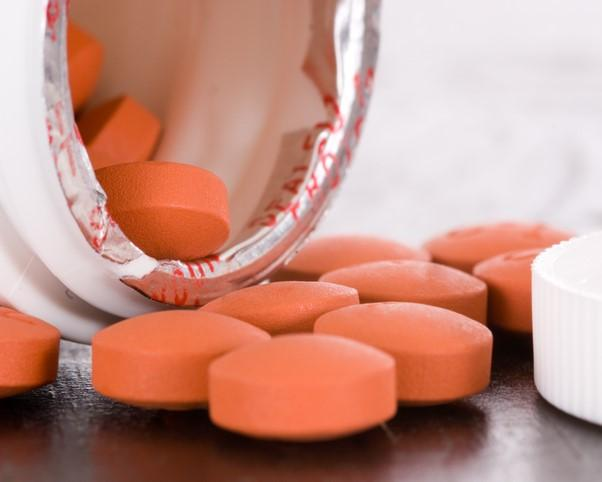
In its most recent guidelines on the use of over-the-counter (OTC) drugs for COVID-19, the US Centers for Disease Control and Prevention (CDC) says that mildly ill patients can relieve symptoms such as fever and sore throat with acetaminophen or ibuprofen, but a team led by Florida Atlantic University (FAU) researchers says it's not that simple. .
In a review in the American Journal of Medicine , the investigators conclude that the decision to take acetaminophen (eg, Tylenol), aspirin, or ibuprofen (eg, Motrin, Advil) should be done on an individual basis under the guidance of a clinician.
This is because the drugs aren't risk-free, with overuse of acetaminophen tied to irreversible liver damage, liver failure, the need for liver transplant, and kidney damage, the authors noted.
While aspirin's anti-inflammatory properties may be useful in treating moderate to severe COVID-19, it carries the risk of bleeding, especially in the gastrointestinal (GI) tract. This is of particular concern in COVID-19, which may itself lead to bleeding and clotting abnormalities.
Patient profile should guide decision, authors say
Compared with aspirin, ibuprofen, naproxen (eg, Aleve), and other non-steroidal anti-inflammatory drugs (NSAIDs) have greater adverse-event profiles, namely for gastroenteritis (inflammation of the GI tract) and peptic ulcers. As with acetaminophen, long-term use of these drugs can lead to liver and kidney toxicity.
We believe that health care providers should make individual clinical judgments for each of his or her patients in the selection of OTC drugs to treat symptoms of COVID-19.
Charles Hennekens, MD, DrPH
"Further, NSAIDs do not confer the cardiovascular benefits of aspirin," the authors wrote. "Since NSAIDs may induce a procoagulant state, the risks of thrombotic and thromboembolic events that can occur with a COVID-19 infection suggests that aspirin may have more beneficial effects in patients with acute COVID-19 infection."
They added that national guidelines should be based on evidence from large randomized trials and directed only to clinicians.
"We believe that health care providers should make individual clinical judgments for each of his or her patients in the selection of OTC drugs to treat symptoms of COVID-19," senior author Charles Hennekens, MD, DrPH, of FAU, said in a university news release . "This judgement should be based on the entire benefit to risk profile of the patient."
Quick takes: Polio in Pakistan, yellow fever in the Americas, intranasal RSV vaccine trial
- Pakistan reported two wild poliovirus type 1 (WPV1) cases this week, marking its first of 2024. Both patients experienced paralysis onset in February and are from Balochistan province, which has been one of the country's hot spots, the Global Polio Eradication Initiative (GPEI) said in its latest weekly update . Also, the country reported 21 more environmental detections of the virus, including 5 in Balochistan, 11 in Sindh, 3 in Khyber Pakhtunkhwa, and 1 each in Islamabad and Punjab. Elsewhere, four countries reported more cases involving circulating vaccine-derived poliovirus type 2: Guinea, Mali, Somalia, and Yemen.
- Three countries in the Americas have reported a total of seven yellow fever cases this year, according to an update yesterday from the Pan American Health Organization (PAHO). Of the seven cases, four were fatal. Countries reporting cases include Colombia, which confirmed three cases, all fatal, in people from different cities in Putumayo department in the south. Guyana reported two cases in people who worked at the same logging camp near the border with Brazil. Peru noted two confirmed case-patients in different departments. Both had been exposed to wooded or wild areas in their agricultural work. In a related development, Brazil reported detections in nonhuman primates. For comparison, four countries reported 41 cases in 2023. PAHO said the risk in the Americas remains high, because impacts from the COVID-19 pandemic have led to gaps in vaccine coverage.
- Blue Lake Biotechnology, a US-based vaccine company, yesterday announced promising preliminary findings from a phase 1/2a clinical trial of its intranasal vaccine against respiratory syncytial virus (RSV) in young children. In a press release, the company said the vaccine, called BLB201, is immunogenic and well tolerated, with no significant safety signals after a single intranasal dose. The trial is under way in seropositive children ages 18 to 59 months old. The company is currently enrolling seronegative and seropositive kids as young as 8 months old in the ongoing trial. The US Food and Drug Administration (FDA) has granted the company fast-track designation for the vaccine. The vaccine encodes the RSV F protein and uses a proprietary parainfluenza virus 5 vector, not known to cause human disease.
In case you missed it
This week's top reads, cdc continues to receive reports of mis-c in kids following covid infections.
The CDC said the cases are a reminder that vaccination is an important tool for preventing MIS-C, which can be severe or even fatal.
Vaccines cut risk of post-COVID heart failure, blood clots for at least 6 months, data suggest
COVID vaccine effectiveness against heart failure and blood clots in the veins and arteries was 22%, 53%, and 45%, respectively, in the month after SARS-CoV-2 infection.
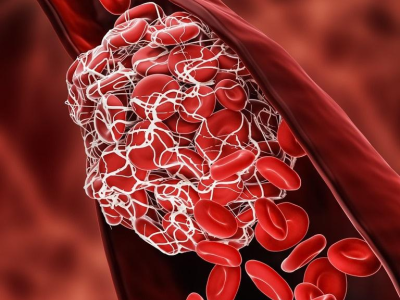
Avian flu detected for first time in US livestock
Minnesota veterinary officials said animals with weakened or immature immune systems, such as goat kids, are at increased risk.
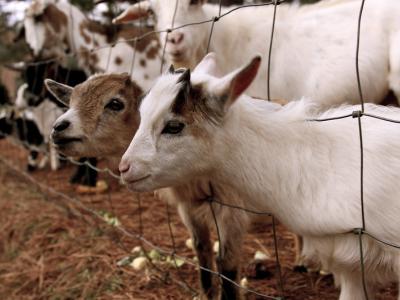
More than 600 dead in spreading DR Congo mpox outbreak as Republic of Congo reports its first cases
The test the US Centers for Disease Control and Prevention uses to identify clade I mpox cases is 'most likely not reliable' for detection of the substrain identified in the study, the authors say.
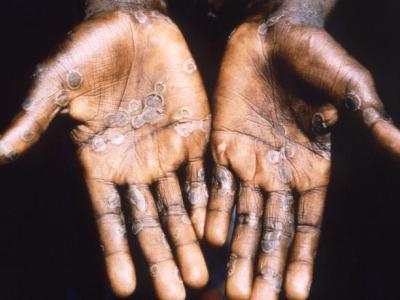
Analysis finds positive risk-benefit for Novavax COVID vaccine
A drop in overall infections, hospitalizations, and deaths outweighed the risk from rare reports of myocarditis or pericarditis.
Probe links COVID spread to school bus riders from sick driver
After being told to quarantine following exposure to the virus, the driver continued to ferry passengers on a public bus, school bus, and charter bus.
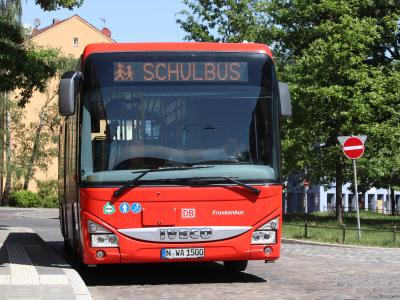
Brisk US flu activity continues as COVID indicators drop further
Some states in the Central and Midwest regions are reporting high or very high flu activity.
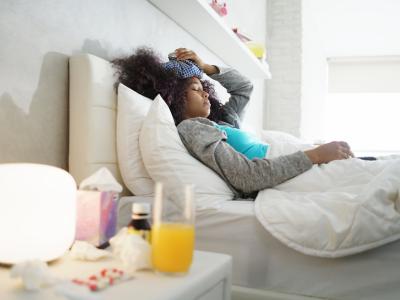
Study details why phase 3 trial of GSK's RSV vaccine for pregnant women was halted early
A total of 7% of infants in the vaccine group were born early, compared with 5% in the placebo group, a 37% difference.
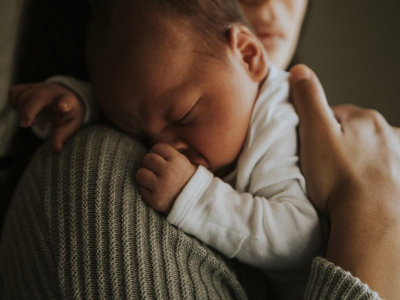
Climate change unleashing torrent of infectious disease threats, physicians caution
Healthcare providers must stay current on climate-related changes in the geographic range, seasonality, and frequency of transmission of infectious diseases, the authors say.
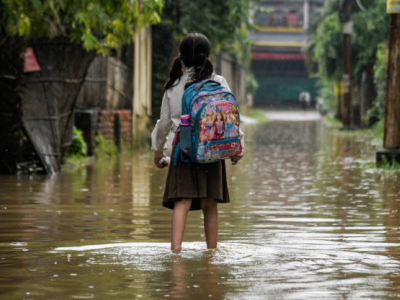
Could cleaner air be driving rise in Legionnaire's disease?
Researchers said a drop in sulfur dioxide levels might allow the bacteria to survive longer in aerosolized water droplets.
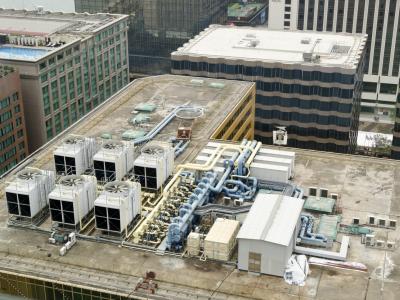
Our underwriters
Unrestricted financial support provided by.
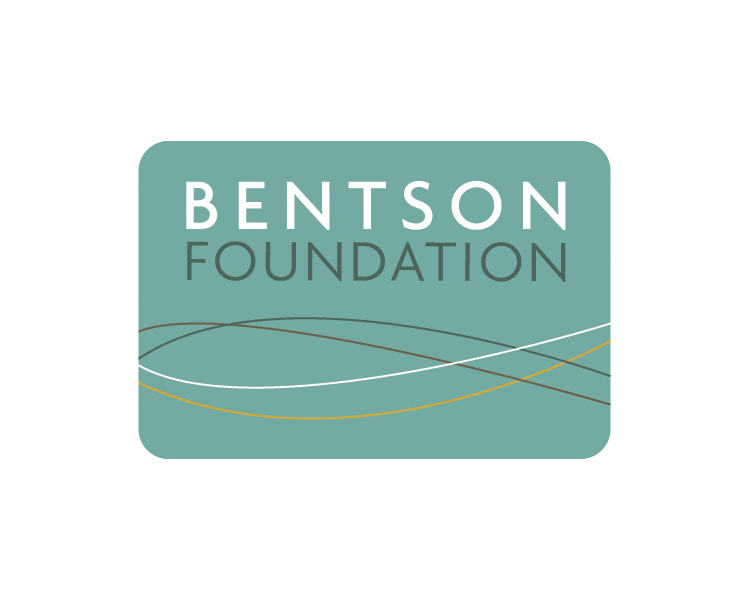
- Antimicrobial Resistance
- Chronic Wasting Disease
- All Topics A-Z
- Resilient Drug Supply
- Influenza Vaccines Roadmap
- CIDRAP Leadership Forum
- WHO Roadmap Development
- Coronavirus Vaccines Roadmap
- Antimicrobial Stewardship
- Osterholm Update
- Newsletters
- About CIDRAP
- CIDRAP in the News
- Our Director
- Osterholm in the Press
- Shop Merchandise
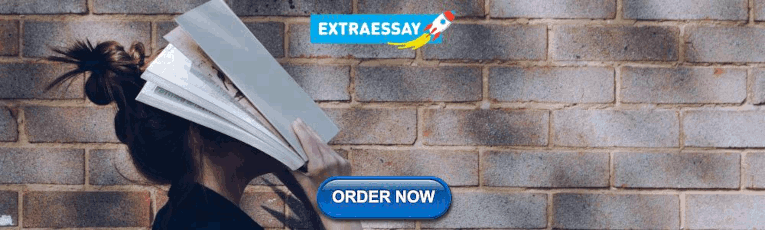
IMAGES
VIDEO
COMMENTS
Authors: Senesh Wijayarathne. Sri Lanka Institute of Information Technology. This study mainly focuses on Trojan Horse Viruses. This study thoroughly describes about what is a Trojan Horse Viruses ...
Check out 11 real cases of malware attacks. 1. CovidLock, ransomware, 2020. Fear in relation to the Coronavirus (COVID-19) has been widely exploited by cybercriminals. CovidLock ransomware is an example. This type of ransomware infects victims via malicious files promising to offer more information about the disease.
The Trojan AIDS/PC Cyborg virus was the first known ransomware attack. It gained access to users' computers through a mailed floppy disc disguised as a survey program. The malware encrypted C ...
2. Brain virus (1986) Creeper was designed to leap across computer networks, but for most of the 1970s and '80s that infection vector was in limited simply because most computers operated in ...
Malware, short for "malicious software," includes any software (such as a virus, Trojan, or spyware) that is installed on your computer or mobile device. The software is then used, usually covertly, to compromise the integrity of your device. Most commonly, malware is designed to give attackers access to your infected computer.
In some cases, that additional malware was a banking trojan that recorded online banking credentials and then stole from victims' accounts. In other cases, Emotet allowed the installation of ...
Hacked wireless routers are used as command-and-control servers. 4. Clickers. These Trojans are created to access internet sites and servers. Users are not aware of such activity as clickers send ...
Figures from security company Malwarebytes Labs in a new report suggest that trojan and backdoor attacks have risen to become the most detected against businesses - and the number of trojan ...
In this work, we aim to improve upon this state of the art by presenting a public and open hardware Trojan detection case study based on four different digital ICs using a Red Team vs. Blue Team approach. Hereby, the Red Team creates small changes acting as surrogates for inserted Trojans in the layouts of 90 nm, 65 nm, 40 nm, and 28 nm ICs.
"The virus guy? Yeah, I know him." The Love Bug virus was unleashed on May 4, 2000. It was simple, but devastatingly effective and highly contagious. Once infected, many of the user's files ...
The Trojan Horse. The COVID-19 pandemic has massively accelerated a global shift toward new digital technologies in health, a trend underway before the crisis. In response to the pandemic, many countries are rapidly scaling up the use of new digital tools and artificial intelligence (AI) for tasks ranging from digital contact tracing, to ...
A virus is a program that spreads by attaching itself to other software, while a trojan spreads by pretending to be useful software or content. Many experts consider spyware programs, which track user activity and send logs or data back to the attacker, as a type of trojan.
Earlier this week, the internet security group M86 uncovered a Trojan virus targeting an unnamed financial institution in the UK. The "Zeus Trojan" has already siphoned off over $1 million ...
"Ideally users should reboot into safe mode and run a full scan with an anti-virus product," he said. "However with this type of malware, it is advisable to seek professional assistance in order ...
In computing, a Trojan horse (or simply Trojan) is any malware that misleads users of its true intent by disguising itself as a standard program. The term is derived from the ancient Greek story of the deceptive Trojan Horse that led to the fall of the city of Troy.. Trojans are generally spread by some form of social engineering.For example, where a user is duped into executing an email ...
A Trojan Horse Virus is a type of malware that downloads onto a computer disguised as a legitimate program. The delivery method typically sees an attacker use social engineering to hide malicious code within legitimate software to try and gain users' system access with their software.. A simple way to answer the question "what is Trojan" is it is a type of malware that typically gets hidden as ...
The first ransomware sample dates back to 1989 with the PC Cyborg Trojan ... is generally easy to overcome and can often be resolved by rebooting the computer in safe mode or running an on-demand virus ... A multi-classifier network-based crypto ransomware detection system: a case study of locky ransomware. IEEE Access. 2019; 7:47053 ...
Scenario 1 - Advisory practices attacked by a Trojan virus. In this scenario, a number of advisory practices were subject to a targeted malware attack via a Trojan virus. This virus helped the cyber criminals access several advisers' PCs and obtain the login details for systems that had been used. This attempted fraud took place while the ...
Published: July 23, 2020 4 min read. Trojan horse, or Trojan, is a type of malicious code or software that can take control of your computer. It is designed to damage, disrupt, steal, or in general inflict some other harmful action on your data or network. Once installed, a Trojan can perform the action it was designed for.
The study didn't name the wife or the town, and he cautioned that infections probably shouldn't be traced back to an individual "except in the presence of an outbreak."
Pakistan reported two wild poliovirus type 1 (WPV1) cases this week, marking its first of 2024. Both patients experienced paralysis onset in February and are from Balochistan province, which has been one of the country's hot spots, the Global Polio Eradication Initiative (GPEI) said in its latest weekly update.Also, the country reported 21 more environmental detections of the virus, including ...
The study included 5,397,278 Medicare beneficiaries who received either brand of the COVID-19 bivalent vaccine (median age 74 years; 56% were women), 11,001 of whom had a stroke during the study period. ... In its weekly respiratory virus snapshot, the CDC said indicators of COVID and respiratory syncytial virus (RSV) also declined. For all ...