hypothesis 6.100.1
pip install hypothesis Copy PIP instructions
Released: Apr 8, 2024
A library for property-based testing
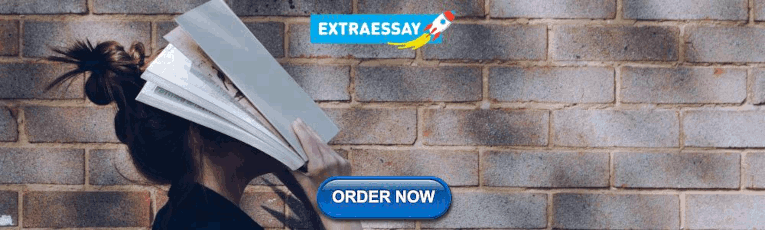
Project links
- Documentation
- Open issues:
View statistics for this project via Libraries.io , or by using our public dataset on Google BigQuery
License: Mozilla Public License 2.0 (MPL 2.0) (MPL-2.0)
Author: David R. MacIver and Zac Hatfield-Dodds
Tags python, testing, fuzzing, property-based-testing
Requires: Python >=3.8
Maintainers
Classifiers
- 5 - Production/Stable
- OSI Approved :: Mozilla Public License 2.0 (MPL 2.0)
- Microsoft :: Windows
- Python :: 3
- Python :: 3 :: Only
- Python :: 3.8
- Python :: 3.9
- Python :: 3.10
- Python :: 3.11
- Python :: 3.12
- Python :: Implementation :: CPython
- Python :: Implementation :: PyPy
- Education :: Testing
- Software Development :: Testing
Project description
Hypothesis is an advanced testing library for Python. It lets you write tests which are parametrized by a source of examples, and then generates simple and comprehensible examples that make your tests fail. This lets you find more bugs in your code with less work.
Hypothesis is extremely practical and advances the state of the art of unit testing by some way. It’s easy to use, stable, and powerful. If you’re not using Hypothesis to test your project then you’re missing out.
Quick Start/Installation
If you just want to get started:
Links of interest
The main Hypothesis site is at hypothesis.works , and contains a lot of good introductory and explanatory material.
Extensive documentation and examples of usage are available at readthedocs .
If you want to talk to people about using Hypothesis, we have both an IRC channel and a mailing list .
If you want to receive occasional updates about Hypothesis, including useful tips and tricks, there’s a TinyLetter mailing list to sign up for them .
If you want to contribute to Hypothesis, instructions are here .
If you want to hear from people who are already using Hypothesis, some of them have written about it .
If you want to create a downstream package of Hypothesis, please read these guidelines for packagers .
Project details
Release history release notifications | rss feed.
Apr 8, 2024
Mar 31, 2024
Mar 24, 2024
Mar 23, 2024
Mar 20, 2024
Mar 19, 2024
Mar 18, 2024
Mar 14, 2024
Mar 12, 2024
Mar 11, 2024
Mar 10, 2024
Mar 9, 2024
Mar 4, 2024
Feb 29, 2024
Feb 27, 2024
Feb 25, 2024
Feb 24, 2024
Feb 22, 2024
Feb 20, 2024
Feb 18, 2024
Feb 15, 2024
Feb 14, 2024
Feb 12, 2024
Feb 8, 2024
Feb 5, 2024
Feb 4, 2024
Feb 3, 2024
Jan 31, 2024
Jan 30, 2024
Jan 27, 2024
Jan 25, 2024
Jan 23, 2024
Jan 22, 2024
Jan 21, 2024
Jan 18, 2024
Jan 17, 2024
Jan 16, 2024
Jan 15, 2024
Jan 13, 2024
Jan 12, 2024
Jan 11, 2024
Jan 10, 2024
Jan 8, 2024
Dec 27, 2023
Dec 16, 2023
Dec 10, 2023
Dec 8, 2023
Nov 27, 2023
Nov 20, 2023
Nov 19, 2023
Nov 16, 2023
Nov 13, 2023
Nov 5, 2023
Oct 16, 2023
Oct 15, 2023
Oct 12, 2023
Oct 6, 2023
Oct 1, 2023
Sep 25, 2023
Sep 18, 2023
Sep 17, 2023
Sep 16, 2023
Sep 10, 2023
Sep 6, 2023
Sep 5, 2023
Sep 4, 2023
Sep 3, 2023
Sep 1, 2023
Aug 28, 2023
Aug 20, 2023
Aug 18, 2023
Aug 12, 2023
Aug 8, 2023
Aug 6, 2023
Aug 5, 2023
Jul 20, 2023
Jul 15, 2023
Jul 11, 2023
Jul 10, 2023
Jul 6, 2023
Jun 27, 2023
Jun 26, 2023
Jun 22, 2023
Jun 19, 2023
Jun 17, 2023
Jun 15, 2023
Jun 13, 2023
Jun 12, 2023
Jun 11, 2023
Jun 9, 2023
Jun 4, 2023
May 31, 2023
May 30, 2023
May 27, 2023
May 26, 2023
May 14, 2023
May 4, 2023
Apr 30, 2023
Apr 28, 2023
Apr 26, 2023
Apr 27, 2023
Apr 25, 2023
Apr 24, 2023
Apr 19, 2023
Apr 16, 2023
Apr 7, 2023
Apr 3, 2023
Mar 27, 2023
Mar 16, 2023
Mar 15, 2023
Feb 17, 2023
Feb 12, 2023
Feb 9, 2023
Feb 5, 2023
Feb 4, 2023
Feb 3, 2023
Feb 2, 2023
Jan 27, 2023
Jan 26, 2023
Jan 24, 2023
Jan 23, 2023
Jan 20, 2023
Jan 14, 2023
Jan 8, 2023
Jan 7, 2023
Jan 6, 2023
Dec 11, 2022
Dec 4, 2022
Dec 2, 2022
Nov 30, 2022
Nov 26, 2022
Nov 19, 2022
Nov 14, 2022
Oct 28, 2022
Oct 17, 2022
Oct 10, 2022
Oct 5, 2022
Oct 2, 2022
Sep 29, 2022
Sep 18, 2022
Sep 5, 2022
Aug 20, 2022
Aug 12, 2022
Aug 10, 2022
Aug 2, 2022
Jul 25, 2022
Jul 22, 2022
Jul 19, 2022
Jul 18, 2022
Jul 17, 2022
Jul 9, 2022
Jul 5, 2022
Jul 4, 2022
Jul 3, 2022
Jun 29, 2022
Jun 27, 2022
Jun 25, 2022
Jun 23, 2022
Jun 15, 2022
Jun 12, 2022
Jun 10, 2022
Jun 7, 2022
Jun 2, 2022
Jun 1, 2022
May 25, 2022
May 19, 2022
May 18, 2022
May 15, 2022
May 11, 2022
May 3, 2022
May 1, 2022
Apr 30, 2022
Apr 29, 2022
Apr 27, 2022
Apr 22, 2022
Apr 21, 2022
Apr 18, 2022
Apr 16, 2022
Apr 13, 2022
Apr 12, 2022
Apr 10, 2022
Apr 9, 2022
Apr 1, 2022
Mar 29, 2022
Mar 27, 2022
Mar 26, 2022
Mar 17, 2022
Mar 7, 2022
Mar 3, 2022
Mar 1, 2022
Feb 26, 2022
Feb 21, 2022
Feb 18, 2022
Feb 13, 2022
Jan 31, 2022
Jan 19, 2022
Jan 17, 2022
Jan 8, 2022
Jan 5, 2022
Dec 31, 2021
Dec 30, 2021
Dec 23, 2021
Dec 15, 2021
Dec 14, 2021
Dec 11, 2021
Dec 10, 2021
Dec 9, 2021
Dec 5, 2021
Dec 3, 2021
Dec 2, 2021
Nov 29, 2021
Nov 28, 2021
Nov 26, 2021
Nov 22, 2021
Nov 21, 2021
Nov 19, 2021
Nov 18, 2021
Nov 16, 2021
Nov 15, 2021
Nov 13, 2021
Nov 5, 2021
Nov 1, 2021
Oct 23, 2021
Oct 20, 2021
Oct 18, 2021
Oct 8, 2021
Sep 29, 2021
Sep 26, 2021
Sep 24, 2021
Sep 19, 2021
Sep 16, 2021
Sep 15, 2021
Sep 13, 2021
Sep 11, 2021
Sep 10, 2021
Sep 9, 2021
Sep 8, 2021
Sep 6, 2021
Aug 31, 2021
Aug 30, 2021
Aug 29, 2021
Aug 27, 2021
Aug 22, 2021
Aug 20, 2021
Aug 16, 2021
Aug 14, 2021
Aug 7, 2021
Jul 27, 2021
Jul 26, 2021
Jul 18, 2021
Jul 12, 2021
Jul 2, 2021
Jun 9, 2021
Jun 4, 2021
Jun 3, 2021
Jun 2, 2021
May 30, 2021
May 28, 2021
May 27, 2021
May 26, 2021
May 24, 2021
May 23, 2021
May 20, 2021
May 18, 2021
May 17, 2021
May 6, 2021
Apr 26, 2021
Apr 17, 2021
Apr 15, 2021
Apr 12, 2021
Apr 11, 2021
Apr 7, 2021
Apr 6, 2021
Apr 5, 2021
Apr 1, 2021
Mar 28, 2021
Mar 27, 2021
Mar 14, 2021
Mar 11, 2021
Mar 10, 2021
Mar 9, 2021
Mar 7, 2021
Mar 4, 2021
Mar 2, 2021
Feb 28, 2021
Feb 26, 2021
Feb 25, 2021
Feb 24, 2021
Feb 20, 2021
Feb 12, 2021
Jan 31, 2021
Jan 29, 2021
Jan 27, 2021
Jan 23, 2021
Jan 14, 2021
Jan 13, 2021
Jan 8, 2021
Jan 7, 2021
Jan 6, 2021
Jan 5, 2021
Jan 4, 2021
Jan 3, 2021
Jan 2, 2021
Jan 1, 2021
Dec 24, 2020
Dec 11, 2020
Dec 10, 2020
Dec 9, 2020
Dec 5, 2020
Nov 28, 2020
Nov 18, 2020
Nov 8, 2020
Nov 3, 2020
Oct 30, 2020
Oct 26, 2020
Oct 24, 2020
Oct 20, 2020
Oct 15, 2020
Oct 14, 2020
Oct 7, 2020
Oct 3, 2020
Oct 2, 2020
Sep 25, 2020
Sep 24, 2020
Sep 21, 2020
Sep 15, 2020
Sep 14, 2020
Sep 11, 2020
Sep 9, 2020
Sep 7, 2020
Sep 6, 2020
Sep 4, 2020
Aug 30, 2020
Aug 28, 2020
Aug 27, 2020
Aug 24, 2020
Aug 20, 2020
Aug 19, 2020
Aug 17, 2020
Aug 16, 2020
Aug 14, 2020
Aug 13, 2020
Aug 12, 2020
Aug 10, 2020
Aug 4, 2020
Aug 3, 2020
Jul 31, 2020
Jul 29, 2020
Jul 27, 2020
Jul 26, 2020
Jul 25, 2020
Jul 23, 2020
Jul 21, 2020
Jul 18, 2020
Jul 17, 2020
Jul 15, 2020
Jul 13, 2020
Jul 12, 2020
Jun 30, 2020
Jun 27, 2020
Jun 26, 2020
Jun 25, 2020
Jun 22, 2020
Jun 21, 2020
Jun 19, 2020
Jun 10, 2020
May 27, 2020
May 21, 2020
May 19, 2020
May 13, 2020
May 12, 2020
May 10, 2020
May 7, 2020
May 4, 2020
Apr 24, 2020
Apr 22, 2020
Apr 19, 2020
Apr 18, 2020
Apr 16, 2020
Apr 15, 2020
Apr 14, 2020
Apr 12, 2020
Mar 24, 2020
Mar 23, 2020
Mar 19, 2020
Mar 18, 2020
Feb 29, 2020
Feb 16, 2020
Feb 14, 2020
Feb 13, 2020
Feb 7, 2020
Feb 6, 2020
Feb 1, 2020
Jan 30, 2020
Jan 26, 2020
Jan 21, 2020
Jan 19, 2020
Jan 12, 2020
Jan 11, 2020
Jan 9, 2020
Jan 6, 2020
Jan 3, 2020
Jan 1, 2020
Dec 29, 2019
Dec 28, 2019
Dec 22, 2019
Dec 21, 2019
Dec 19, 2019
Dec 18, 2019
Dec 17, 2019
Dec 16, 2019
Dec 15, 2019
Dec 11, 2019
Dec 9, 2019
Dec 7, 2019
Dec 5, 2019
Dec 2, 2019
Dec 1, 2019
Nov 29, 2019
Nov 28, 2019
Nov 27, 2019
Nov 26, 2019
Nov 25, 2019
Nov 24, 2019
Nov 23, 2019
Nov 22, 2019
Nov 20, 2019
Nov 12, 2019
Nov 11, 2019
Nov 8, 2019
Nov 7, 2019
Nov 6, 2019
Nov 5, 2019
Nov 4, 2019
Nov 3, 2019
Nov 2, 2019
Nov 1, 2019
Oct 30, 2019
Oct 27, 2019
Oct 21, 2019
Oct 17, 2019
Oct 16, 2019
Oct 14, 2019
Oct 9, 2019
Oct 7, 2019
Oct 4, 2019
Oct 2, 2019
Oct 1, 2019
Sep 28, 2019
Sep 20, 2019
Sep 17, 2019
Sep 9, 2019
Sep 4, 2019
Aug 23, 2019
Aug 21, 2019
Aug 20, 2019
Aug 5, 2019
Jul 30, 2019
Jul 29, 2019
Jul 28, 2019
Jul 24, 2019
Jul 14, 2019
Jul 12, 2019
Jul 11, 2019
Jul 8, 2019
Jul 7, 2019
Jul 5, 2019
Jul 4, 2019
Jul 3, 2019
Jun 26, 2019
Jun 23, 2019
Jun 21, 2019
Jun 7, 2019
Jun 6, 2019
Jun 4, 2019
May 29, 2019
May 28, 2019
May 26, 2019
May 19, 2019
May 16, 2019
May 9, 2019
May 8, 2019
May 7, 2019
May 6, 2019
May 5, 2019
Apr 30, 2019
Apr 29, 2019
Apr 24, 2019
Apr 19, 2019
Apr 16, 2019
Apr 12, 2019
Apr 9, 2019
Apr 7, 2019
Apr 5, 2019
Apr 3, 2019
Mar 31, 2019
Mar 30, 2019
Mar 19, 2019
Mar 18, 2019
Mar 15, 2019
Mar 13, 2019
Mar 12, 2019
Mar 11, 2019
Mar 9, 2019
Mar 6, 2019
Mar 4, 2019
Mar 3, 2019
Mar 1, 2019
Feb 28, 2019
Feb 27, 2019
Feb 25, 2019
Feb 24, 2019
Feb 23, 2019
Feb 22, 2019
Feb 21, 2019
Feb 19, 2019
Feb 18, 2019
Feb 15, 2019
Feb 14, 2019
Feb 12, 2019
Feb 11, 2019
Feb 10, 2019
Feb 8, 2019
Feb 6, 2019
Feb 5, 2019
Feb 3, 2019
Feb 2, 2019
Jan 25, 2019
Jan 24, 2019
Jan 23, 2019
Jan 22, 2019
Jan 16, 2019
Jan 14, 2019
Jan 11, 2019
Jan 10, 2019
Jan 9, 2019
Jan 8, 2019
Jan 7, 2019
Jan 6, 2019
Jan 4, 2019
Jan 3, 2019
Jan 2, 2019
Dec 31, 2018
Dec 30, 2018
Dec 29, 2018
Dec 28, 2018
Dec 21, 2018
Dec 20, 2018
Dec 19, 2018
Dec 18, 2018
Dec 17, 2018
Dec 13, 2018
Dec 12, 2018
Dec 11, 2018
Dec 8, 2018
Oct 29, 2018
Oct 27, 2018
Oct 25, 2018
Oct 23, 2018
Oct 22, 2018
Oct 18, 2018
Oct 16, 2018
Oct 11, 2018
Oct 10, 2018
Oct 9, 2018
Oct 8, 2018
Oct 3, 2018
Oct 1, 2018
Sep 30, 2018
Sep 27, 2018
Sep 26, 2018
Sep 25, 2018
Sep 24, 2018
Sep 18, 2018
Sep 17, 2018
Sep 16, 2018
Sep 15, 2018
Sep 14, 2018
Sep 9, 2018
Sep 8, 2018
Sep 3, 2018
Sep 1, 2018
Aug 30, 2018
Aug 29, 2018
Aug 28, 2018
Aug 27, 2018
Aug 23, 2018
Aug 21, 2018
Aug 20, 2018
Aug 19, 2018
Aug 18, 2018
Aug 15, 2018
Aug 14, 2018
Aug 10, 2018
Aug 9, 2018
Aug 8, 2018
Aug 6, 2018
Aug 5, 2018
Aug 3, 2018
Aug 2, 2018
Aug 1, 2018
Jul 31, 2018
Jul 30, 2018
Jul 28, 2018
Jul 26, 2018
Jul 24, 2018
Jul 23, 2018
Jul 22, 2018
Jul 20, 2018
Jul 19, 2018
Jul 8, 2018
Jul 5, 2018
Jul 4, 2018
Jul 3, 2018
Jun 30, 2018
Jun 27, 2018
Jun 26, 2018
Jun 24, 2018
Jun 20, 2018
Jun 19, 2018
Jun 18, 2018
Jun 16, 2018
Jun 14, 2018
Jun 13, 2018
May 20, 2018
May 16, 2018
May 11, 2018
May 10, 2018
May 9, 2018
Apr 22, 2018
Apr 21, 2018
Apr 20, 2018
Apr 17, 2018
Apr 14, 2018
Apr 13, 2018
Apr 12, 2018
Apr 11, 2018
Apr 6, 2018
Apr 5, 2018
Apr 4, 2018
Apr 1, 2018
Mar 30, 2018
Mar 29, 2018
Mar 24, 2018
Mar 20, 2018
Mar 19, 2018
Mar 15, 2018
Mar 12, 2018
Mar 5, 2018
Mar 2, 2018
Mar 1, 2018
Feb 26, 2018
Feb 25, 2018
Feb 23, 2018
Feb 18, 2018
Feb 17, 2018
Feb 13, 2018
Feb 5, 2018
Jan 27, 2018
Jan 24, 2018
Jan 23, 2018
Jan 22, 2018
Jan 21, 2018
Jan 20, 2018
Jan 13, 2018
Jan 8, 2018
Jan 7, 2018
Jan 6, 2018
Jan 4, 2018
Jan 2, 2018
Dec 23, 2017
Dec 21, 2017
Dec 20, 2017
Dec 17, 2017
Dec 12, 2017
Dec 10, 2017
Dec 9, 2017
Dec 6, 2017
Dec 4, 2017
Dec 2, 2017
Dec 1, 2017
Nov 29, 2017
Nov 28, 2017
Nov 23, 2017
Nov 22, 2017
Nov 21, 2017
Nov 18, 2017
Nov 12, 2017
Nov 10, 2017
Nov 6, 2017
Nov 2, 2017
Nov 1, 2017
Oct 16, 2017
Oct 15, 2017
Oct 13, 2017
Oct 9, 2017
Oct 8, 2017
Oct 6, 2017
Sep 30, 2017
Sep 29, 2017
Sep 27, 2017
Sep 25, 2017
Sep 24, 2017
Sep 22, 2017
Sep 19, 2017
Sep 18, 2017
Sep 16, 2017
Sep 15, 2017
Sep 14, 2017
Sep 13, 2017
Sep 12, 2017
Sep 11, 2017
Sep 6, 2017
Sep 5, 2017
Sep 1, 2017
Aug 31, 2017
Aug 29, 2017
Aug 28, 2017
Aug 26, 2017
Aug 25, 2017
Aug 24, 2017
Aug 23, 2017
Aug 22, 2017
Aug 21, 2017
Aug 20, 2017
Aug 18, 2017
Aug 17, 2017
Aug 16, 2017
Aug 15, 2017
Aug 13, 2017
Aug 7, 2017
Aug 4, 2017
Aug 3, 2017
Aug 2, 2017
Jul 23, 2017
Jul 20, 2017
Jul 16, 2017
Jul 7, 2017
Jun 19, 2017
Jun 17, 2017
Jun 11, 2017
Jun 10, 2017
May 28, 2017
May 23, 2017
May 22, 2017
May 19, 2017
May 17, 2017
May 9, 2017
Apr 26, 2017
Apr 23, 2017
Apr 22, 2017
Apr 21, 2017
Mar 20, 2017
Dec 20, 2016
Oct 31, 2016
Oct 5, 2016
Sep 26, 2016
Sep 23, 2016
Sep 22, 2016
Jul 13, 2016
Jul 7, 2016
May 27, 2016
May 24, 2016
May 1, 2016
Apr 30, 2016
Apr 29, 2016
Mar 6, 2016
Feb 25, 2016
Feb 24, 2016
Feb 23, 2016
Feb 18, 2016
Feb 17, 2016
Jan 10, 2016
Jan 9, 2016
Dec 22, 2015
Dec 21, 2015
Dec 16, 2015
Dec 15, 2015
Dec 8, 2015
Nov 24, 2015
Nov 1, 2015
Oct 29, 2015
Oct 18, 2015
Sep 27, 2015
Sep 23, 2015
Sep 16, 2015
Aug 31, 2015
Aug 26, 2015
Aug 22, 2015
Aug 19, 2015
Aug 4, 2015
Aug 3, 2015
Jul 27, 2015
Jul 24, 2015
Jul 21, 2015
Jul 20, 2015
Jul 18, 2015
Jul 17, 2015
Jul 16, 2015
Jul 10, 2015
Jun 29, 2015
Jun 8, 2015
May 21, 2015
May 14, 2015
May 5, 2015
May 4, 2015
Apr 22, 2015
Apr 15, 2015
Apr 14, 2015
Apr 7, 2015
Apr 6, 2015
Mar 27, 2015
Mar 26, 2015
Mar 25, 2015
Mar 23, 2015
Mar 22, 2015
Mar 21, 2015
Mar 20, 2015
Mar 14, 2015
Feb 10, 2015
Feb 5, 2015
Feb 4, 2015
Feb 3, 2015
Jan 21, 2015
Jan 16, 2015
Jan 13, 2015
Jan 12, 2015
Jan 8, 2015
Jan 7, 2015
Dec 14, 2013
May 3, 2013
Mar 26, 2013
Mar 24, 2013
Mar 23, 2013
Mar 13, 2013
Mar 12, 2013
Mar 10, 2013
Download files
Download the file for your platform. If you're not sure which to choose, learn more about installing packages .
Source Distribution
Uploaded Apr 8, 2024 Source
Built Distribution
Uploaded Apr 8, 2024 Python 3
Hashes for hypothesis-6.100.1.tar.gz
Hashes for hypothesis-6.100.1-py3-none-any.whl.
- português (Brasil)
Supported by

Statistics Made Easy
How to Perform Hypothesis Testing in Python (With Examples)
A hypothesis test is a formal statistical test we use to reject or fail to reject some statistical hypothesis.
This tutorial explains how to perform the following hypothesis tests in Python:
- One sample t-test
- Two sample t-test
- Paired samples t-test
Let’s jump in!
Example 1: One Sample t-test in Python
A one sample t-test is used to test whether or not the mean of a population is equal to some value.
For example, suppose we want to know whether or not the mean weight of a certain species of some turtle is equal to 310 pounds.
To test this, we go out and collect a simple random sample of turtles with the following weights:
Weights : 300, 315, 320, 311, 314, 309, 300, 308, 305, 303, 305, 301, 303
The following code shows how to use the ttest_1samp() function from the scipy.stats library to perform a one sample t-test:
The t test statistic is -1.5848 and the corresponding two-sided p-value is 0.1389 .
The two hypotheses for this particular one sample t-test are as follows:
- H 0 : µ = 310 (the mean weight for this species of turtle is 310 pounds)
- H A : µ ≠310 (the mean weight is not 310 pounds)
Because the p-value of our test (0.1389) is greater than alpha = 0.05, we fail to reject the null hypothesis of the test.
We do not have sufficient evidence to say that the mean weight for this particular species of turtle is different from 310 pounds.
Example 2: Two Sample t-test in Python
A two sample t-test is used to test whether or not the means of two populations are equal.
For example, suppose we want to know whether or not the mean weight between two different species of turtles is equal.
To test this, we collect a simple random sample of turtles from each species with the following weights:
Sample 1 : 300, 315, 320, 311, 314, 309, 300, 308, 305, 303, 305, 301, 303
Sample 2 : 335, 329, 322, 321, 324, 319, 304, 308, 305, 311, 307, 300, 305
The following code shows how to use the ttest_ind() function from the scipy.stats library to perform this two sample t-test:
The t test statistic is – 2.1009 and the corresponding two-sided p-value is 0.0463 .
The two hypotheses for this particular two sample t-test are as follows:
- H 0 : µ 1 = µ 2 (the mean weight between the two species is equal)
- H A : µ 1 ≠ µ 2 (the mean weight between the two species is not equal)
Since the p-value of the test (0.0463) is less than .05, we reject the null hypothesis.
This means we have sufficient evidence to say that the mean weight between the two species is not equal.
Example 3: Paired Samples t-test in Python
A paired samples t-test is used to compare the means of two samples when each observation in one sample can be paired with an observation in the other sample.
For example, suppose we want to know whether or not a certain training program is able to increase the max vertical jump (in inches) of basketball players.
To test this, we may recruit a simple random sample of 12 college basketball players and measure each of their max vertical jumps. Then, we may have each player use the training program for one month and then measure their max vertical jump again at the end of the month.
The following data shows the max jump height (in inches) before and after using the training program for each player:
Before : 22, 24, 20, 19, 19, 20, 22, 25, 24, 23, 22, 21
After : 23, 25, 20, 24, 18, 22, 23, 28, 24, 25, 24, 20
The following code shows how to use the ttest_rel() function from the scipy.stats library to perform this paired samples t-test:
The t test statistic is – 2.5289 and the corresponding two-sided p-value is 0.0280 .
The two hypotheses for this particular paired samples t-test are as follows:
- H 0 : µ 1 = µ 2 (the mean jump height before and after using the program is equal)
- H A : µ 1 ≠ µ 2 (the mean jump height before and after using the program is not equal)
Since the p-value of the test (0.0280) is less than .05, we reject the null hypothesis.
This means we have sufficient evidence to say that the mean jump height before and after using the training program is not equal.
Additional Resources
You can use the following online calculators to automatically perform various t-tests:
One Sample t-test Calculator Two Sample t-test Calculator Paired Samples t-test Calculator

Published by Zach
Leave a reply cancel reply.
Your email address will not be published. Required fields are marked *

Your Data Guide
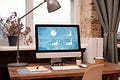
How to Perform Hypothesis Testing Using Python

Step into the intriguing world of hypothesis testing, where your natural curiosity meets the power of data to reveal truths!
This article is your key to unlocking how those everyday hunches—like guessing a group’s average income or figuring out who owns their home—can be thoroughly checked and proven with data.
Thanks for reading Your Data Guide! Subscribe for free to receive new posts and support my work.
I am going to take you by the hand and show you, in simple steps, how to use Python to explore a hypothesis about the average yearly income.
By the time we’re done, you’ll not only get the hang of creating and testing hypotheses but also how to use statistical tests on actual data.
Perfect for up-and-coming data scientists, anyone with a knack for analysis, or just if you’re keen on data, get ready to gain the skills to make informed decisions and turn insights into real-world actions.
Join me as we dive deep into the data, one hypothesis at a time!
Before we get started, elevate your data skills with my expert eBooks—the culmination of my experiences and insights.
Support my work and enhance your journey. Check them out:
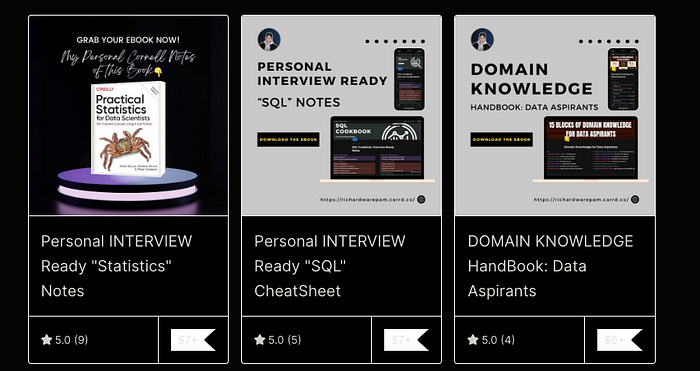
eBook 1: Personal INTERVIEW Ready “SQL” CheatSheet
eBook 2: Personal INTERVIEW Ready “Statistics” Cornell Notes
Best Selling eBook: Top 50+ ChatGPT Personas for Custom Instructions
Data Science Bundle ( Cheapest ): The Ultimate Data Science Bundle: Complete
ChatGPT Bundle ( Cheapest ): The Ultimate ChatGPT Bundle: Complete
💡 Checkout for more such resources: https://codewarepam.gumroad.com/
What is a hypothesis, and how do you test it?
A hypothesis is like a guess or prediction about something specific, such as the average income or the percentage of homeowners in a group of people.
It’s based on theories, past observations, or questions that spark our curiosity.
For instance, you might predict that the average yearly income of potential customers is over $50,000 or that 60% of them own their homes.
To see if your guess is right, you gather data from a smaller group within the larger population and check if the numbers ( like the average income, percentage of homeowners, etc. ) from this smaller group match your initial prediction.
You also set a rule for how sure you need to be to trust your findings, often using a 5% chance of error as a standard measure . This means you’re 95% confident in your results. — Level of Significance (0.05)
There are two main types of hypotheses : the null hypothesi s, which is your baseline saying there’s no change or difference, and the alternative hypothesis , which suggests there is a change or difference.
For example,
If you start with the idea that the average yearly income of potential customers is $50,000,
The alternative could be that it’s not $50,000—it could be less or more, depending on what you’re trying to find out.
To test your hypothesis, you calculate a test statistic —a number that shows how much your sample data deviates from what you predicted.
How you calculate this depends on what you’re studying and the kind of data you have. For example, to check an average, you might use a formula that considers your sample’s average, the predicted average, the variation in your sample data, and how big your sample is.
This test statistic follows a known distribution ( like the t-distribution or z-distribution ), which helps you figure out the p-value.
The p-value tells you the odds of seeing a test statistic as extreme as yours if your initial guess was correct.
A small p-value means your data strongly disagrees with your initial guess.
Finally, you decide on your hypothesis by comparing the p-value to your error threshold.
If the p-value is smaller or equal, you reject the null hypothesis, meaning your data shows a significant difference that’s unlikely due to chance.
If the p-value is larger, you stick with the null hypothesis , suggesting your data doesn’t show a meaningful difference and any change might just be by chance.
We’ll go through an example that tests if the average annual income of prospective customers exceeds $50,000.
This process involves stating hypotheses , specifying a significance level , collecting and analyzing data , and drawing conclusions based on statistical tests.
Example: Testing a Hypothesis About Average Annual Income
Step 1: state the hypotheses.
Null Hypothesis (H0): The average annual income of prospective customers is $50,000.
Alternative Hypothesis (H1): The average annual income of prospective customers is more than $50,000.
Step 2: Specify the Significance Level
Significance Level: 0.05, meaning we’re 95% confident in our findings and allow a 5% chance of error.
Step 3: Collect Sample Data
We’ll use the ProspectiveBuyer table, assuming it's a random sample from the population.
This table has 2,059 entries, representing prospective customers' annual incomes.
Step 4: Calculate the Sample Statistic
In Python, we can use libraries like Pandas and Numpy to calculate the sample mean and standard deviation.
SampleMean: 56,992.43
SampleSD: 32,079.16
SampleSize: 2,059
Step 5: Calculate the Test Statistic
We use the t-test formula to calculate how significantly our sample mean deviates from the hypothesized mean.
Python’s Scipy library can handle this calculation:
T-Statistic: 4.62
Step 6: Calculate the P-Value
The p-value is already calculated in the previous step using Scipy's ttest_1samp function, which returns both the test statistic and the p-value.
P-Value = 0.0000021
Step 7: State the Statistical Conclusion
We compare the p-value with our significance level to decide on our hypothesis:
Since the p-value is less than 0.05, we reject the null hypothesis in favor of the alternative.
Conclusion:
There’s strong evidence to suggest that the average annual income of prospective customers is indeed more than $50,000.
This example illustrates how Python can be a powerful tool for hypothesis testing, enabling us to derive insights from data through statistical analysis.
How to Choose the Right Test Statistics
Choosing the right test statistic is crucial and depends on what you’re trying to find out, the kind of data you have, and how that data is spread out.
Here are some common types of test statistics and when to use them:
T-test statistic:
This one’s great for checking out the average of a group when your data follows a normal distribution or when you’re comparing the averages of two such groups.
The t-test follows a special curve called the t-distribution . This curve looks a lot like the normal bell curve but with thicker ends, which means more chances for extreme values.
The t-distribution’s shape changes based on something called degrees of freedom , which is a fancy way of talking about your sample size and how many groups you’re comparing.
Z-test statistic:
Use this when you’re looking at the average of a normally distributed group or the difference between two group averages, and you already know the standard deviation for all in the population.
The z-test follows the standard normal distribution , which is your classic bell curve centered at zero and spreading out evenly on both sides.
Chi-square test statistic:
This is your go-to for checking if there’s a difference in variability within a normally distributed group or if two categories are related.
The chi-square statistic follows its own distribution, which leans to the right and gets its shape from the degrees of freedom —basically, how many categories or groups you’re comparing.
F-test statistic:
This one helps you compare the variability between two groups or see if the averages of more than two groups are all the same, assuming all groups are normally distributed.
The F-test follows the F-distribution , which is also right-skewed and has two types of degrees of freedom that depend on how many groups you have and the size of each group.
In simple terms, the test you pick hinges on what you’re curious about, whether your data fits the normal curve, and if you know certain specifics, like the population’s standard deviation.
Each test has its own special curve and rules based on your sample’s details and what you’re comparing.
Join my community of learners! Subscribe to my newsletter for more tips, tricks, and exclusive content on mastering Data Science & AI. — Your Data Guide Join my community of learners! Subscribe to my newsletter for more tips, tricks, and exclusive content on mastering data science and AI. By Richard Warepam ⭐️ Visit My Gumroad Shop: https://codewarepam.gumroad.com/

Ready for more?
Search code, repositories, users, issues, pull requests...
Provide feedback.
We read every piece of feedback, and take your input very seriously.
Saved searches
Use saved searches to filter your results more quickly.
To see all available qualifiers, see our documentation .
- Notifications
Hypothesis is a powerful, flexible, and easy to use library for property-based testing.
HypothesisWorks/hypothesis
Folders and files, repository files navigation.
Hypothesis is a family of testing libraries which let you write tests parametrized by a source of examples. A Hypothesis implementation then generates simple and comprehensible examples that make your tests fail. This simplifies writing your tests and makes them more powerful at the same time, by letting software automate the boring bits and do them to a higher standard than a human would, freeing you to focus on the higher level test logic.
This sort of testing is often called "property-based testing", and the most widely known implementation of the concept is the Haskell library QuickCheck , but Hypothesis differs significantly from QuickCheck and is designed to fit idiomatically and easily into existing styles of testing that you are used to, with absolutely no familiarity with Haskell or functional programming needed.
Hypothesis for Python is the original implementation, and the only one that is currently fully production ready and actively maintained.
Hypothesis for Other Languages
The core ideas of Hypothesis are language agnostic and in principle it is suitable for any language. We are interested in developing and supporting implementations for a wide variety of languages, but currently lack the resources to do so, so our porting efforts are mostly prototypes.
The two prototype implementations of Hypothesis for other languages are:
- Hypothesis for Ruby is a reasonable start on a port of Hypothesis to Ruby.
- Hypothesis for Java is a prototype written some time ago. It's far from feature complete and is not under active development, but was intended to prove the viability of the concept.
Additionally there is a port of the core engine of Hypothesis, Conjecture, to Rust. It is not feature complete but in the long run we are hoping to move much of the existing functionality to Rust and rebuild Hypothesis for Python on top of it, greatly lowering the porting effort to other languages.
Any or all of these could be turned into full fledged implementations with relatively little effort (no more than a few months of full time work), but as well as the initial work this would require someone prepared to provide or fund ongoing maintenance efforts for them in order to be viable.
Releases 671
Used by 24.7k.
Contributors 297
- Python 90.0%
- Jupyter Notebook 5.2%
The Hypothesis Testing Library for Python: An Introduction
Hypothesis is a Python library for creating tests which are simple to write and powerful when run, finding cases in your code you wouldn't have thought to look for. It is stable, powerful and easy to add to an existing test suite.
It works by letting you write tests that assert that something should be true for every case, not just the ones you happen to think of.
Think of a normal unit test as being something like the following:
- Set up some data.
- Perform some operations on the data.
- Assert something about the result.
Hypothesis lets you write tests which instead look like this:
- For all data matching some specification.
This is often called property-based testing, and was popularized by the Haskell library Quickcheck . [1]
I found out about the Hypothesis testing library about a year ago, started using it a few hours later, and have been using it ever since. A few months ago, I realized that I felt so strongly about the value and importance of the library that I should give a talk about it, and a few weeks ago that is just what I did. Here is my talk:
http://www.youtube.com/watch?v=CTi2DRvkNLk
[1] https://hypothesis.readthedocs.io/en/latest/
- Red Hat Enterprise Linux
- Red Hat OpenShift
- Red Hat Ansible Automation Platform
- See all products
- See all technologies
- Developer Sandbox
- Developer Tools
- Interactive Tutorials
- API Catalog
- Operators Marketplace
- Learning Resources
- Cheat Sheets
Communicate
- Contact sales
- Find a partner
Report a website issue
- Site Status Dashboard
- Report a security problem
RED HAT DEVELOPER
Build here. Go anywhere.
We serve the builders. The problem solvers who create careers with code.
Join us if you’re a developer, software engineer, web designer, front-end designer, UX designer, computer scientist, architect, tester, product manager, project manager or team lead.
Red Hat legal and privacy links
- About Red Hat
- Contact Red Hat
- Red Hat Blog
- Diversity, equity, and inclusion
- Cool Stuff Store
- Red Hat Summit
- Privacy statement
- Terms of use
- All policies and guidelines
- Digital accessibility
What Is Hypothesis Testing? Types and Python Code Example
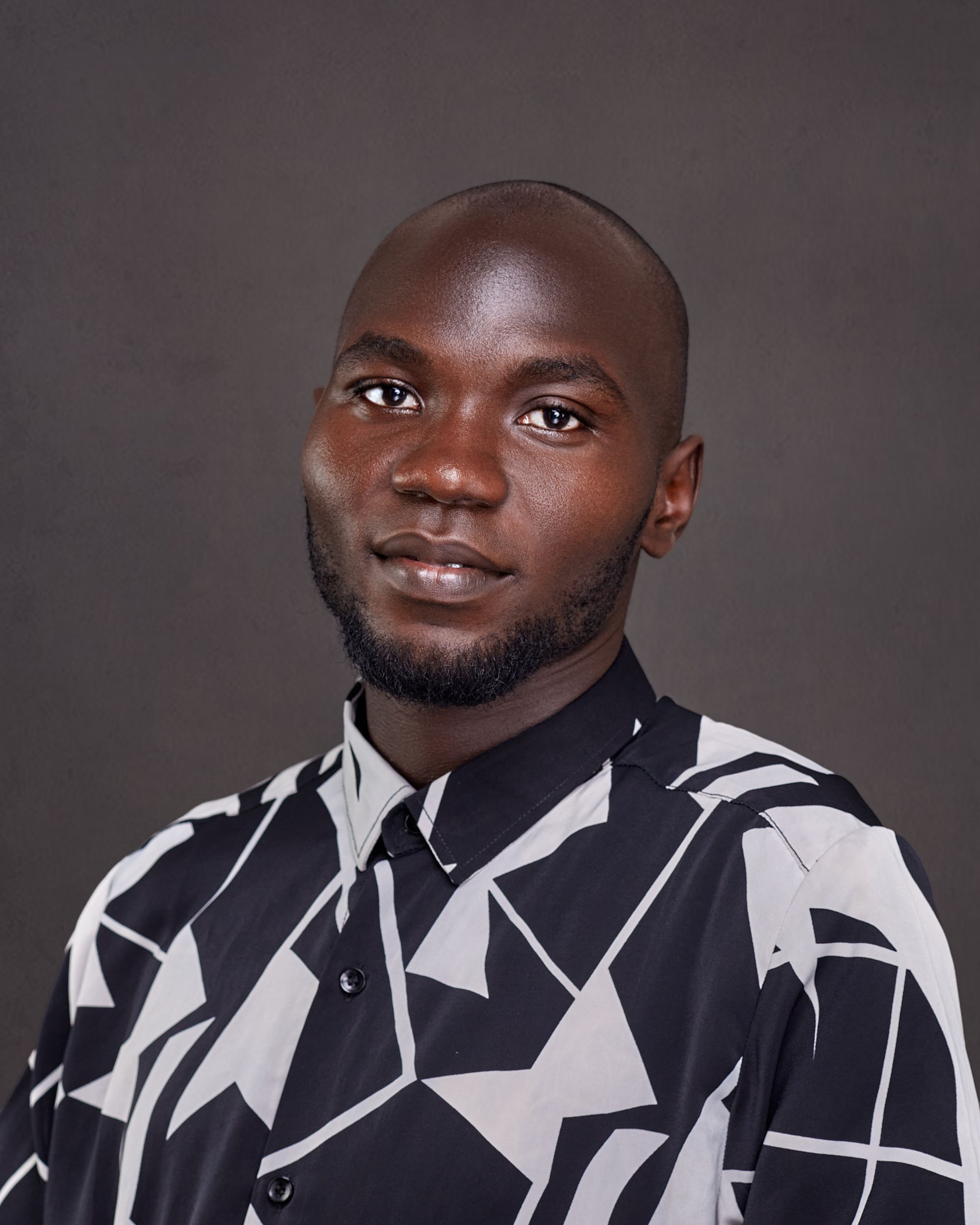
Curiosity has always been a part of human nature. Since the beginning of time, this has been one of the most important tools for birthing civilizations. Still, our curiosity grows — it tests and expands our limits. Humanity has explored the plains of land, water, and air. We've built underwater habitats where we could live for weeks. Our civilization has explored various planets. We've explored land to an unlimited degree.
These things were possible because humans asked questions and searched until they found answers. However, for us to get these answers, a proven method must be used and followed through to validate our results. Historically, philosophers assumed the earth was flat and you would fall off when you reached the edge. While philosophers like Aristotle argued that the earth was spherical based on the formation of the stars, they could not prove it at the time.
This is because they didn't have adequate resources to explore space or mathematically prove Earth's shape. It was a Greek mathematician named Eratosthenes who calculated the earth's circumference with incredible precision. He used scientific methods to show that the Earth was not flat. Since then, other methods have been used to prove the Earth's spherical shape.
When there are questions or statements that are yet to be tested and confirmed based on some scientific method, they are called hypotheses. Basically, we have two types of hypotheses: null and alternate.
A null hypothesis is one's default belief or argument about a subject matter. In the case of the earth's shape, the null hypothesis was that the earth was flat.
An alternate hypothesis is a belief or argument a person might try to establish. Aristotle and Eratosthenes argued that the earth was spherical.
Other examples of a random alternate hypothesis include:
- The weather may have an impact on a person's mood.
- More people wear suits on Mondays compared to other days of the week.
- Children are more likely to be brilliant if both parents are in academia, and so on.
What is Hypothesis Testing?
Hypothesis testing is the act of testing whether a hypothesis or inference is true. When an alternate hypothesis is introduced, we test it against the null hypothesis to know which is correct. Let's use a plant experiment by a 12-year-old student to see how this works.
The hypothesis is that a plant will grow taller when given a certain type of fertilizer. The student takes two samples of the same plant, fertilizes one, and leaves the other unfertilized. He measures the plants' height every few days and records the results in a table.
After a week or two, he compares the final height of both plants to see which grew taller. If the plant given fertilizer grew taller, the hypothesis is established as fact. If not, the hypothesis is not supported. This simple experiment shows how to form a hypothesis, test it experimentally, and analyze the results.
In hypothesis testing, there are two types of error: Type I and Type II.
When we reject the null hypothesis in a case where it is correct, we've committed a Type I error. Type II errors occur when we fail to reject the null hypothesis when it is incorrect.
In our plant experiment above, if the student finds out that both plants' heights are the same at the end of the test period yet opines that fertilizer helps with plant growth, he has committed a Type I error.
However, if the fertilized plant comes out taller and the student records that both plants are the same or that the one without fertilizer grew taller, he has committed a Type II error because he has failed to reject the null hypothesis.
What are the Steps in Hypothesis Testing?
The following steps explain how we can test a hypothesis:
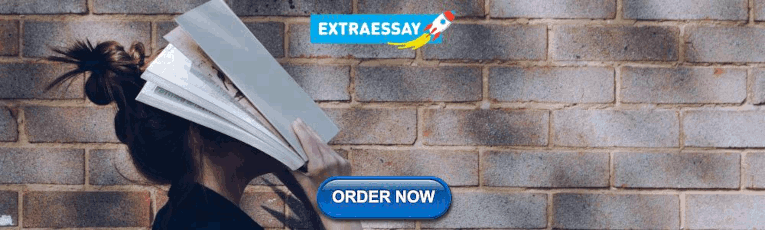
Step #1 - Define the Null and Alternative Hypotheses
Before making any test, we must first define what we are testing and what the default assumption is about the subject. In this article, we'll be testing if the average weight of 10-year-old children is more than 32kg.
Our null hypothesis is that 10 year old children weigh 32 kg on average. Our alternate hypothesis is that the average weight is more than 32kg. Ho denotes a null hypothesis, while H1 denotes an alternate hypothesis.
Step #2 - Choose a Significance Level
The significance level is a threshold for determining if the test is valid. It gives credibility to our hypothesis test to ensure we are not just luck-dependent but have enough evidence to support our claims. We usually set our significance level before conducting our tests. The criterion for determining our significance value is known as p-value.
A lower p-value means that there is stronger evidence against the null hypothesis, and therefore, a greater degree of significance. A p-value of 0.05 is widely accepted to be significant in most fields of science. P-values do not denote the probability of the outcome of the result, they just serve as a benchmark for determining whether our test result is due to chance. For our test, our p-value will be 0.05.
Step #3 - Collect Data and Calculate a Test Statistic
You can obtain your data from online data stores or conduct your research directly. Data can be scraped or researched online. The methodology might depend on the research you are trying to conduct.
We can calculate our test using any of the appropriate hypothesis tests. This can be a T-test, Z-test, Chi-squared, and so on. There are several hypothesis tests, each suiting different purposes and research questions. In this article, we'll use the T-test to run our hypothesis, but I'll explain the Z-test, and chi-squared too.
T-test is used for comparison of two sets of data when we don't know the population standard deviation. It's a parametric test, meaning it makes assumptions about the distribution of the data. These assumptions include that the data is normally distributed and that the variances of the two groups are equal. In a more simple and practical sense, imagine that we have test scores in a class for males and females, but we don't know how different or similar these scores are. We can use a t-test to see if there's a real difference.
The Z-test is used for comparison between two sets of data when the population standard deviation is known. It is also a parametric test, but it makes fewer assumptions about the distribution of data. The z-test assumes that the data is normally distributed, but it does not assume that the variances of the two groups are equal. In our class test example, with the t-test, we can say that if we already know how spread out the scores are in both groups, we can now use the z-test to see if there's a difference in the average scores.
The Chi-squared test is used to compare two or more categorical variables. The chi-squared test is a non-parametric test, meaning it does not make any assumptions about the distribution of data. It can be used to test a variety of hypotheses, including whether two or more groups have equal proportions.
Step #4 - Decide on the Null Hypothesis Based on the Test Statistic and Significance Level
After conducting our test and calculating the test statistic, we can compare its value to the predetermined significance level. If the test statistic falls beyond the significance level, we can decide to reject the null hypothesis, indicating that there is sufficient evidence to support our alternative hypothesis.
On the other contrary, if the test statistic does not exceed the significance level, we fail to reject the null hypothesis, signifying that we do not have enough statistical evidence to conclude in favor of the alternative hypothesis.
Step #5 - Interpret the Results
Depending on the decision made in the previous step, we can interpret the result in the context of our study and the practical implications. For our case study, we can interpret whether we have significant evidence to support our claim that the average weight of 10 year old children is more than 32kg or not.
For our test, we are generating random dummy data for the weight of the children. We'll use a t-test to evaluate whether our hypothesis is correct or not.
For a better understanding, let's look at what each block of code does.
The first block is the import statement, where we import numpy and scipy.stats . Numpy is a Python library used for scientific computing. It has a large library of functions for working with arrays. Scipy is a library for mathematical functions. It has a stat module for performing statistical functions, and that's what we'll be using for our t-test.
The weights of the children were generated at random since we aren't working with an actual dataset. The random module within the Numpy library provides a function for generating random numbers, which is randint .
The randint function takes three arguments. The first (20) is the lower bound of the random numbers to be generated. The second (40) is the upper bound, and the third (100) specifies the number of random integers to generate. That is, we are generating random weight values for 100 children. In real circumstances, these weight samples would have been obtained by taking the weight of the required number of children needed for the test.
Using the code above, we declared our null and alternate hypotheses stating the average weight of a 10-year-old in both cases.
t_stat and p_value are the variables in which we'll store the results of our functions. stats.ttest_1samp is the function that calculates our test. It takes in two variables, the first is the data variable that stores the array of weights for children, and the second (32) is the value against which we'll test the mean of our array of weights or dataset in cases where we are using a real-world dataset.
The code above prints both values for t_stats and p_value .
Lastly, we evaluated our p_value against our significance value, which is 0.05. If our p_value is less than 0.05, we reject the null hypothesis. Otherwise, we fail to reject the null hypothesis. Below is the output of this program. Our null hypothesis was rejected.
In this article, we discussed the importance of hypothesis testing. We highlighted how science has advanced human knowledge and civilization through formulating and testing hypotheses.
We discussed Type I and Type II errors in hypothesis testing and how they underscore the importance of careful consideration and analysis in scientific inquiry. It reinforces the idea that conclusions should be drawn based on thorough statistical analysis rather than assumptions or biases.
We also generated a sample dataset using the relevant Python libraries and used the needed functions to calculate and test our alternate hypothesis.
Thank you for reading! Please follow me on LinkedIn where I also post more data related content.
Technical support engineer with 4 years of experience & 6 months in data analytics. Passionate about data science, programming, & statistics.
If you read this far, thank the author to show them you care. Say Thanks
Learn to code for free. freeCodeCamp's open source curriculum has helped more than 40,000 people get jobs as developers. Get started
Learning Statistics with Python
Hypothesis Testing
12. hypothesis testing #.
The process of induction is the process of assuming the simplest law that can be made to harmonize with our experience. This process, however, has no logical foundation but only a psychological one. It is clear that there are no grounds for believing that the simplest course of events will really happen. It is an hypothesis that the sun will rise tomorrow: and this means that we do not know whether it will rise. – Ludwig Wittgenstein [ 1 ]
In the last chapter, I discussed the ideas behind estimation, which is one of the two “big ideas” in inferential statistics. It’s now time to turn out attention to the other big idea, which is hypothesis testing . In its most abstract form, hypothesis testing really a very simple idea: the researcher has some theory about the world, and wants to determine whether or not the data actually support that theory. However, the details are messy, and most people find the theory of hypothesis testing to be the most frustrating part of statistics. The structure of the chapter is as follows. Firstly, I’ll describe how hypothesis testing works, in a fair amount of detail, using a simple running example to show you how a hypothesis test is “built”. I’ll try to avoid being too dogmatic while doing so, and focus instead on the underlying logic of the testing procedure. [ 2 ] Afterwards, I’ll spend a bit of time talking about the various dogmas, rules and heresies that surround the theory of hypothesis testing.
12.1. A menagerie of hypotheses #
Eventually we all succumb to madness. For me, that day will arrive once I’m finally promoted to full professor. Safely ensconced in my ivory tower, happily protected by tenure, I will finally be able to take leave of my senses (so to speak), and indulge in that most thoroughly unproductive line of psychological research: the search for extrasensory perception (ESP). [ 3 ]
Let’s suppose that this glorious day has come. My first study is a simple one, in which I seek to test whether clairvoyance exists. Each participant sits down at a table, and is shown a card by an experimenter. The card is black on one side and white on the other. The experimenter takes the card away, and places it on a table in an adjacent room. The card is placed black side up or white side up completely at random, with the randomisation occurring only after the experimenter has left the room with the participant. A second experimenter comes in and asks the participant which side of the card is now facing upwards. It’s purely a one-shot experiment. Each person sees only one card, and gives only one answer; and at no stage is the participant actually in contact with someone who knows the right answer. My data set, therefore, is very simple. I have asked the question of \(N\) people, and some number \(X\) of these people have given the correct response. To make things concrete, let’s suppose that I have tested \(N = 100\) people, and \(X = 62\) of these got the answer right… a surprisingly large number, sure, but is it large enough for me to feel safe in claiming I’ve found evidence for ESP? This is the situation where hypothesis testing comes in useful. However, before we talk about how to test hypotheses, we need to be clear about what we mean by hypotheses.
12.1.1. Research hypotheses versus statistical hypotheses #
The first distinction that you need to keep clear in your mind is between research hypotheses and statistical hypotheses. In my ESP study, my overall scientific goal is to demonstrate that clairvoyance exists. In this situation, I have a clear research goal: I am hoping to discover evidence for ESP. In other situations I might actually be a lot more neutral than that, so I might say that my research goal is to determine whether or not clairvoyance exists. Regardless of how I want to portray myself, the basic point that I’m trying to convey here is that a research hypothesis involves making a substantive, testable scientific claim… if you are a psychologist, then your research hypotheses are fundamentally about psychological constructs. Any of the following would count as research hypotheses :
Listening to music reduces your ability to pay attention to other things. This is a claim about the causal relationship between two psychologically meaningful concepts (listening to music and paying attention to things), so it’s a perfectly reasonable research hypothesis.
Intelligence is related to personality . Like the last one, this is a relational claim about two psychological constructs (intelligence and personality), but the claim is weaker: correlational not causal.
Intelligence is speed of information processing . This hypothesis has a quite different character: it’s not actually a relational claim at all. It’s an ontological claim about the fundamental character of intelligence (and I’m pretty sure it’s wrong). It’s worth expanding on this one actually: It’s usually easier to think about how to construct experiments to test research hypotheses of the form “does X affect Y?” than it is to address claims like “what is X?” And in practice, what usually happens is that you find ways of testing relational claims that follow from your ontological ones. For instance, if I believe that intelligence is speed of information processing in the brain, my experiments will often involve looking for relationships between measures of intelligence and measures of speed. As a consequence, most everyday research questions do tend to be relational in nature, but they’re almost always motivated by deeper ontological questions about the state of nature.
Notice that in practice, my research hypotheses could overlap a lot. My ultimate goal in the ESP experiment might be to test an ontological claim like “ESP exists”, but I might operationally restrict myself to a narrower hypothesis like “Some people can `see’ objects in a clairvoyant fashion”. That said, there are some things that really don’t count as proper research hypotheses in any meaningful sense:
Love is a battlefield . This is too vague to be testable. While it’s okay for a research hypothesis to have a degree of vagueness to it, it has to be possible to operationalise your theoretical ideas. Maybe I’m just not creative enough to see it, but I can’t see how this can be converted into any concrete research design. If that’s true, then this isn’t a scientific research hypothesis, it’s a pop song. That doesn’t mean it’s not interesting – a lot of deep questions that humans have fall into this category. Maybe one day science will be able to construct testable theories of love, or to test to see if God exists, and so on; but right now we can’t, and I wouldn’t bet on ever seeing a satisfying scientific approach to either.
The first rule of tautology club is the first rule of tautology club . This is not a substantive claim of any kind. It’s true by definition. No conceivable state of nature could possibly be inconsistent with this claim. As such, we say that this is an unfalsifiable hypothesis, and as such it is outside the domain of science. Whatever else you do in science, your claims must have the possibility of being wrong.
More people in my experiment will say “yes” than “no” . This one fails as a research hypothesis because it’s a claim about the data set, not about the psychology (unless of course your actual research question is whether people have some kind of “yes” bias!). As we’ll see shortly, this hypothesis is starting to sound more like a statistical hypothesis than a research hypothesis.
As you can see, research hypotheses can be somewhat messy at times; and ultimately they are scientific claims. Statistical hypotheses are neither of these two things. Statistical hypotheses must be mathematically precise, and they must correspond to specific claims about the characteristics of the data-generating mechanism (i.e., the “population”). Even so, the intent is that statistical hypotheses bear a clear relationship to the substantive research hypotheses that you care about! For instance, in my ESP study my research hypothesis is that some people are able to see through walls or whatever. What I want to do is to “map” this onto a statement about how the data were generated. So let’s think about what that statement would be. The quantity that I’m interested in within the experiment is \(P(\mbox{"correct"})\) , the true-but-unknown probability with which the participants in my experiment answer the question correctly. Let’s use the Greek letter \(\theta\) (theta) to refer to this probability. Here are four different statistical hypotheses:
If ESP doesn’t exist and if my experiment is well designed, then my participants are just guessing. So I should expect them to get it right half of the time and so my statistical hypothesis is that the true probability of choosing correctly is \(\theta = 0.5\) .
Alternatively, suppose ESP does exist and participants can see the card. If that’s true, people will perform better than chance. The statistical hypotheis would be that \(\theta > 0.5\) .
A third possibility is that ESP does exist, but the colours are all reversed and people don’t realise it (okay, that’s wacky, but you never know…). If that’s how it works then you’d expect people’s performance to be below chance. This would correspond to a statistical hypothesis that \(\theta < 0.5\) .
Finally, suppose ESP exists, but I have no idea whether people are seeing the right colour or the wrong one. In that case, the only claim I could make about the data would be that the probability of making the correct answer is not equal to 50. This corresponds to the statistical hypothesis that \(\theta \neq 0.5\) .
All of these are legitimate examples of a statistical hypothesis because they are statements about a population parameter and are meaningfully related to my experiment.
What this discussion makes clear, I hope, is that when attempting to construct a statistical hypothesis test, the researcher actually has two quite distinct hypotheses to consider. First, he or she has a research hypothesis (a claim about psychology), and this corresponds to a statistical hypothesis (a claim about the data generating population). In my ESP example, these might be
My research hypothesis: “ESP exists”
My statistical hypothesis: \(\theta \neq 0.5\)
And the key thing to recognise is this: a statistical hypothesis test is a test of the statistical hypothesis, not the research hypothesis . If your study is badly designed, then the link between your research hypothesis and your statistical hypothesis is broken. To give a silly example, suppose that my ESP study was conducted in a situation where the participant can actually see the card reflected in a window; if that happens, I would be able to find very strong evidence that \(\theta \neq 0.5\) , but this would tell us nothing about whether “ESP exists”.
12.1.2. Null hypotheses and alternative hypotheses #
So far, so good. I have a research hypothesis that corresponds to what I want to believe about the world, and I can map it onto a statistical hypothesis that corresponds to what I want to believe about how the data were generated. It’s at this point that things get somewhat counterintuitive for a lot of people. Because what I’m about to do is invent a new statistical hypothesis (the “null” hypothesis, \(H_0\) ) that corresponds to the exact opposite of what I want to believe, and then focus exclusively on that, almost to the neglect of the thing I’m actually interested in (which is now called the “alternative” hypothesis, \(H_1\) ). In our ESP example, the null hypothesis is that \(\theta = 0.5\) , since that’s what we’d expect if ESP didn’t exist. My hope, of course, is that ESP is totally real, and so the alternative to this null hypothesis is \(\theta \neq 0.5\) . In essence, what we’re doing here is dividing up the possible values of \(\theta\) into two groups: those values that I really hope aren’t true (the null), and those values that I’d be happy with if they turn out to be right (the alternative). Having done so, the important thing to recognise is that the goal of a hypothesis test is not to show that the alternative hypothesis is (probably) true; the goal is to show that the null hypothesis is (probably) false. Most people find this pretty weird.
The best way to think about it, in my experience, is to imagine that a hypothesis test is a criminal trial [ 4 ] … the trial of the null hypothesis . The null hypothesis is the defendant, the researcher is the prosecutor, and the statistical test itself is the judge. Just like a criminal trial, there is a presumption of innocence: the null hypothesis is deemed to be true unless you, the researcher, can prove beyond a reasonable doubt that it is false. You are free to design your experiment however you like (within reason, obviously!), and your goal when doing so is to maximise the chance that the data will yield a conviction… for the crime of being false. The catch is that the statistical test sets the rules of the trial, and those rules are designed to protect the null hypothesis – specifically to ensure that if the null hypothesis is actually true, the chances of a false conviction are guaranteed to be low. This is pretty important: after all, the null hypothesis doesn’t get a lawyer. And given that the researcher is trying desperately to prove it to be false, someone has to protect it.
12.2. Two types of errors #
Before going into details about how a statistical test is constructed, it’s useful to understand the philosophy behind it. I hinted at it when pointing out the similarity between a null hypothesis test and a criminal trial, but I should now be explicit. Ideally, we would like to construct our test so that we never make any errors. Unfortunately, since the world is messy, this is never possible. Sometimes you’re just really unlucky: for instance, suppose you flip a coin 10 times in a row and it comes up heads all 10 times. That feels like very strong evidence that the coin is biased (and it is!), but of course there’s a 1 in 1024 chance that this would happen even if the coin was totally fair. In other words, in real life we always have to accept that there’s a chance that we did the wrong thing. As a consequence, the goal behind statistical hypothesis testing is not to eliminate errors, but to minimise them.
At this point, we need to be a bit more precise about what we mean by “errors”. Firstly, let’s state the obvious: it is either the case that the null hypothesis is true, or it is false; and our test will either reject the null hypothesis or retain it. [ 5 ] So, as the table below illustrates, after we run the test and make our choice, one of four things might have happened:
As a consequence there are actually two different types of error here. If we reject a null hypothesis that is actually true, then we have made a type I error . On the other hand, if we retain the null hypothesis when it is in fact false, then we have made a type II error .
Remember how I said that statistical testing was kind of like a criminal trial? Well, I meant it. A criminal trial requires that you establish “beyond a reasonable doubt” that the defendant did it. All of the evidentiary rules are (in theory, at least) designed to ensure that there’s (almost) no chance of wrongfully convicting an innocent defendant. The trial is designed to protect the rights of a defendant: as the English jurist William Blackstone famously said, it is “better that ten guilty persons escape than that one innocent suffer.” In other words, a criminal trial doesn’t treat the two types of error in the same way… punishing the innocent is deemed to be much worse than letting the guilty go free. A statistical test is pretty much the same: the single most important design principle of the test is to control the probability of a type I error, to keep it below some fixed probability. This probability, which is denoted \(\alpha\) , is called the significance level of the test (or sometimes, the size of the test). And I’ll say it again, because it is so central to the whole set-up… a hypothesis test is said to have significance level \(\alpha\) if the type I error rate is no larger than \(\alpha\) .
So, what about the type II error rate? Well, we’d also like to keep those under control too, and we denote this probability by \(\beta\) . However, it’s much more common to refer to the power of the test, which is the probability with which we reject a null hypothesis when it really is false, which is \(1-\beta\) . To help keep this straight, here’s the same table again, but with the relevant numbers added:
A “powerful” hypothesis test is one that has a small value of \(\beta\) , while still keeping \(\alpha\) fixed at some (small) desired level. By convention, scientists make use of three different \(\alpha\) levels: \(.05\) , \(.01\) and \(.001\) . Notice the asymmetry here~… the tests are designed to ensure that the \(\alpha\) level is kept small, but there’s no corresponding guarantee regarding \(\beta\) . We’d certainly like the type II error rate to be small, and we try to design tests that keep it small, but this is very much secondary to the overwhelming need to control the type I error rate. As Blackstone might have said if he were a statistician, it is “better to retain 10 false null hypotheses than to reject a single true one”. To be honest, I don’t know that I agree with this philosophy – there are situations where I think it makes sense, and situations where I think it doesn’t – but that’s neither here nor there. It’s how the tests are built.
12.3. Test statistics and sampling distributions #
At this point we need to start talking specifics about how a hypothesis test is constructed. To that end, let’s return to the ESP example. Let’s ignore the actual data that we obtained, for the moment, and think about the structure of the experiment. Regardless of what the actual numbers are, the form of the data is that \(X\) out of \(N\) people correctly identified the colour of the hidden card. Moreover, let’s suppose for the moment that the null hypothesis really is true: ESP doesn’t exist, and the true probability that anyone picks the correct colour is exactly \(\theta = 0.5\) . What would we expect the data to look like? Well, obviously, we’d expect the proportion of people who make the correct response to be pretty close to 50%. Or, to phrase this in more mathematical terms, we’d say that \(X/N\) is approximately \(0.5\) . Of course, we wouldn’t expect this fraction to be exactly 0.5: if, for example we tested \(N=100\) people, and \(X = 53\) of them got the question right, we’d probably be forced to concede that the data are quite consistent with the null hypothesis. On the other hand, if \(X = 99\) of our participants got the question right, then we’d feel pretty confident that the null hypothesis is wrong. Similarly, if only \(X=3\) people got the answer right, we’d be similarly confident that the null was wrong. Let’s be a little more technical about this: we have a quantity \(X\) that we can calculate by looking at our data; after looking at the value of \(X\) , we make a decision about whether to believe that the null hypothesis is correct, or to reject the null hypothesis in favour of the alternative. The name for this thing that we calculate to guide our choices is a test statistic .
Having chosen a test statistic, the next step is to state precisely which values of the test statistic would cause us to reject the null hypothesis, and which values would cause us to keep it. In order to do so, we need to determine what the sampling distribution of the test statistic would be if the null hypothesis were actually true (we talked about sampling distributions earlier). Why do we need this? Because this distribution tells us exactly what values of \(X\) our null hypothesis would lead us to expect. And therefore, we can use this distribution as a tool for assessing how closely the null hypothesis agrees with our data. Using random.binomial from numpy , we can estimate a binomial distribution with a \(\theta = 0.5\) , e.g. estimating from 10,000 trials:

How do we actually determine the sampling distribution of the test statistic? For a lot of hypothesis tests this step is actually quite complicated, and later on in the book you’ll see me being slightly evasive about it for some of the tests (some of them I don’t even understand myself). However, sometimes it’s very easy. And, fortunately for us, our ESP example provides us with one of the easiest cases. Our population parameter \(\theta\) is just the overall probability that people respond correctly when asked the question, and our test statistic \(X\) is the count of the number of people who did so, out of a sample size of \(N\) . We’ve seen a distribution like this before, in the section on the binomial distribution : that’s exactly what the binomial distribution describes! So, to use the notation and terminology that I introduced in that section, we would say that the null hypothesis predicts that \(X\) is binomially distributed, which is written
Since the null hypothesis states that \(\theta = 0.5\) and our experiment has \(N=100\) people, we have the sampling distribution we need. This sampling distribution is plotted in Figure fig-esp-estimation . No surprises really: the null hypothesis says that \(X=50\) is the most likely outcome, and it says that we’re almost certain to see somewhere between 40 and 60 correct responses.
12.4. Making decisions #
Okay, we’re very close to being finished. We’ve constructed a test statistic ( \(X\) ), and we chose this test statistic in such a way that we’re pretty confident that if \(X\) is close to \(N/2\) then we should retain the null, and if not we should reject it. The question that remains is this: exactly which values of the test statistic should we associate with the null hypothesis, and which exactly values go with the alternative hypothesis? In my ESP study, for example, I’ve observed a value of \(X=62\) . What decision should I make? Should I choose to believe the null hypothesis, or the alternative hypothesis?
12.4.1. Critical regions and critical values #
To answer this question, we need to introduce the concept of a critical region for the test statistic \(X\) . The critical region of the test corresponds to those values of \(X\) that would lead us to reject the null hypothesis (which is why the critical region is also sometimes called the rejection region). How do we find this critical region? Well, let’s consider what we know:
\(X\) should be very big or very small in order to reject the null hypothesis.
If the null hypothesis is true, the sampling distribution of \(X\) is Binomial \((0.5, N)\) .
If \(\alpha =.05\) , the critical region must cover 5% of this sampling distribution.
It’s important to make sure you understand this last point: the critical region corresponds to those values of \(X\) for which we would reject the null hypothesis, and the sampling distribution in question describes the probability that we would obtain a particular value of \(X\) if the null hypothesis were actually true. Now, let’s suppose that we chose a critical region that covers 20% of the sampling distribution, and suppose that the null hypothesis is actually true. What would be the probability of incorrectly rejecting the null? The answer is of course 20%. And therefore, we would have built a test that had an \(\alpha\) level of \(0.2\) . If we want \(\alpha = .05\) , the critical region is only allowed to cover 5% of the sampling distribution of our test statistic.

As it turns out, those three things uniquely solve the problem: our critical region consists of the most extreme values , known as the tails of the distribution. This is illustrated in fig-esp-critical . As it turns out, if we want \(\alpha = .05\) , then our critical regions correspond to \(X \leq 40\) and \(X \geq 60\) . [ 6 ] That is, if the number of people saying “true” is between 41 and 59, then we should retain the null hypothesis. If the number is between 0 to 40 or between 60 to 100, then we should reject the null hypothesis. The numbers 40 and 60 are often referred to as the critical values , since they define the edges of the critical region.
At this point, our hypothesis test is essentially complete: (1) we choose an \(\alpha\) level (e.g., \(\alpha = .05\) , (2) come up with some test statistic (e.g., \(X\) ) that does a good job (in some meaningful sense) of comparing \(H_0\) to \(H_1\) , (3) figure out the sampling distribution of the test statistic on the assumption that the null hypothesis is true (in this case, binomial) and then (4) calculate the critical region that produces an appropriate \(\alpha\) level (0-40 and 60-100). All that we have to do now is calculate the value of the test statistic for the real data (e.g., \(X = 62\) ) and then compare it to the critical values to make our decision. Since 62 is greater than the critical value of 60, we would reject the null hypothesis. Or, to phrase it slightly differently, we say that the test has produced a significant result.
12.4.2. A note on statistical “significance” #
Like other occult techniques of divination, the statistical method has a private jargon deliberately contrived to obscure its methods from non-practitioners. – Attributed to G. O. Ashley [ 7 ]
A very brief digression is in order at this point, regarding the word “significant”. The concept of statistical significance is actually a very simple one, but has a very unfortunate name. If the data allow us to reject the null hypothesis, we say that “the result is statistically significant ”, which is often shortened to “the result is significant”. This terminology is rather old, and dates back to a time when “significant” just meant something like “indicated”, rather than its modern meaning, which is much closer to “important”. As a result, a lot of modern readers get very confused when they start learning statistics, because they think that a “significant result” must be an important one. It doesn’t mean that at all. All that “statistically significant” means is that the data allowed us to reject a null hypothesis. Whether or not the result is actually important in the real world is a very different question, and depends on all sorts of other things.
12.4.3. The difference between one sided and two sided tests #
There’s one more thing I want to point out about the hypothesis test that I’ve just constructed. If we take a moment to think about the statistical hypotheses I’ve been using,
we notice that the alternative hypothesis covers both the possibility that \(\theta < .5\) and the possibility that \(\theta > .5\) . This makes sense if I really think that ESP could produce better-than-chance performance or worse-than-chance performance (and there are some people who think that). In statistical language, this is an example of a two-sided test . It’s called this because the alternative hypothesis covers the area on both “sides” of the null hypothesis, and as a consequence the critical region of the test covers both tails of the sampling distribution (2.5% on either side if \(\alpha =.05\) ), as illustrated earlier in fig-esp-critical .
However, that’s not the only possibility. It might be the case, for example, that I’m only willing to believe in ESP if it produces better than chance performance. If so, then my alternative hypothesis would only cover the possibility that \(\theta > .5\) , and as a consequence the null hypothesis now becomes \(\theta \leq .5\) :
When this happens, we have what’s called a one-sided test , and when this happens the critical region only covers one tail of the sampling distribution. This is illustrated in fig-esp-critical-onesided .

12.5. The \(p\) value of a test #
In one sense, our hypothesis test is complete; we’ve constructed a test statistic, figured out its sampling distribution if the null hypothesis is true, and then constructed the critical region for the test. Nevertheless, I’ve actually omitted the most important number of all: the \(p\) value . It is to this topic that we now turn. There are two somewhat different ways of interpreting a \(p\) value, one proposed by Sir Ronald Fisher and the other by Jerzy Neyman. Both versions are legitimate, though they reflect very different ways of thinking about hypothesis tests. Most introductory textbooks tend to give Fisher’s version only, but I think that’s a bit of a shame. To my mind, Neyman’s version is cleaner, and actually better reflects the logic of the null hypothesis test. You might disagree though, so I’ve included both. I’ll start with Neyman’s version…
12.5.1. A softer view of decision making #
One problem with the hypothesis testing procedure that I’ve described is that it makes no distinction at all between a result this “barely significant” and those that are “highly significant”. For instance, in my ESP study the data I obtained only just fell inside the critical region - so I did get a significant effect, but was a pretty near thing. In contrast, suppose that I’d run a study in which \(X=97\) out of my \(N=100\) participants got the answer right. This would obviously be significant too, but by a much larger margin; there’s really no ambiguity about this at all. The procedure that I described makes no distinction between the two. If I adopt the standard convention of allowing \(\alpha = .05\) as my acceptable Type I error rate, then both of these are significant results.
This is where the \(p\) value comes in handy. To understand how it works, let’s suppose that we ran lots of hypothesis tests on the same data set: but with a different value of \(\alpha\) in each case. When we do that for my original ESP data, what we’d get is something like this
When we test ESP data ( \(X=62\) successes out of \(N=100\) observations) using \(\alpha\) levels of .03 and above, we’d always find ourselves rejecting the null hypothesis. For \(\alpha\) levels of .02 and below, we always end up retaining the null hypothesis. Therefore, somewhere between .02 and .03 there must be a smallest value of \(\alpha\) that would allow us to reject the null hypothesis for this data. This is the \(p\) value; as it turns out the ESP data has \(p = .021\) . In short:
\(p\) is defined to be the smallest Type I error rate ( \(\alpha\) ) that you have to be willing to tolerate if you want to reject the null hypothesis.
If it turns out that \(p\) describes an error rate that you find intolerable, then you must retain the null. If you’re comfortable with an error rate equal to \(p\) , then it’s okay to reject the null hypothesis in favour of your preferred alternative.
In effect, \(p\) is a summary of all the possible hypothesis tests that you could have run, taken across all possible \(\alpha\) values. And as a consequence it has the effect of “softening” our decision process. For those tests in which \(p \leq \alpha\) you would have rejected the null hypothesis, whereas for those tests in which \(p > \alpha\) you would have retained the null. In my ESP study I obtained \(X=62\) , and as a consequence I’ve ended up with \(p = .021\) . So the error rate I have to tolerate is 2.1%. In contrast, suppose my experiment had yielded \(X=97\) . What happens to my \(p\) value now? This time it’s shrunk to \(p = 1.36 \times 10^{-25}\) , which is a tiny, tiny [ 8 ] Type I error rate. For this second case I would be able to reject the null hypothesis with a lot more confidence, because I only have to be “willing” to tolerate a type I error rate of about 1 in 10 trillion trillion in order to justify my decision to reject.
12.5.2. The probability of extreme data #
The second definition of the \(p\) -value comes from Sir Ronald Fisher, and it’s actually this one that you tend to see in most introductory statistics textbooks. Notice how, when I constructed the critical region, it corresponded to the tails (i.e., extreme values) of the sampling distribution? That’s not a coincidence: almost all “good” tests have this characteristic (good in the sense of minimising our type II error rate, \(\beta\) ). The reason for that is that a good critical region almost always corresponds to those values of the test statistic that are least likely to be observed if the null hypothesis is true. If this rule is true, then we can define the \(p\) -value as the probability that we would have observed a test statistic that is at least as extreme as the one we actually did get. In other words, if the data are extremely implausible according to the null hypothesis, then the null hypothesis is probably wrong.
12.5.3. A common mistake #
Okay, so you can see that there are two rather different but legitimate ways to interpret the \(p\) value, one based on Neyman’s approach to hypothesis testing and the other based on Fisher’s. Unfortunately, there is a third explanation that people sometimes give, especially when they’re first learning statistics, and it is absolutely and completely wrong . This mistaken approach is to refer to the \(p\) value as “the probability that the null hypothesis is true”. It’s an intuitively appealing way to think, but it’s wrong in two key respects: (1) null hypothesis testing is a frequentist tool, and the frequentist approach to probability does not allow you to assign probabilities to the null hypothesis… according to this view of probability, the null hypothesis is either true or it is not; it cannot have a “5% chance” of being true. (2) even within the Bayesian approach, which does let you assign probabilities to hypotheses, the \(p\) value would not correspond to the probability that the null is true; this interpretation is entirely inconsistent with the mathematics of how the \(p\) value is calculated. Put bluntly, despite the intuitive appeal of thinking this way, there is no justification for interpreting a \(p\) value this way. Never do it.
12.6. Reporting the results of a hypothesis test #
When writing up the results of a hypothesis test, there’s usually several pieces of information that you need to report, but it varies a fair bit from test to test. Throughout the rest of the book I’ll spend a little time talking about how to report the results of different tests (see Section @ref(chisqreport) for a particularly detailed example), so that you can get a feel for how it’s usually done. However, regardless of what test you’re doing, the one thing that you always have to do is say something about the \(p\) value, and whether or not the outcome was significant.
The fact that you have to do this is unsurprising; it’s the whole point of doing the test. What might be surprising is the fact that there is some contention over exactly how you’re supposed to do it. Leaving aside those people who completely disagree with the entire framework underpinning null hypothesis testing, there’s a certain amount of tension that exists regarding whether or not to report the exact \(p\) value that you obtained, or if you should state only that \(p < \alpha\) for a significance level that you chose in advance (e.g., \(p<.05\) ).
12.6.1. The issue #
To see why this is an issue, the key thing to recognise is that \(p\) values are terribly convenient. In practice, the fact that we can compute a \(p\) value means that we don’t actually have to specify any \(\alpha\) level at all in order to run the test. Instead, what you can do is calculate your \(p\) value and interpret it directly: if you get \(p = .062\) , then it means that you’d have to be willing to tolerate a Type I error rate of 6.2% to justify rejecting the null. If you personally find 6.2% intolerable, then you retain the null. Therefore, the argument goes, why don’t we just report the actual \(p\) value and let the reader make up their own minds about what an acceptable Type I error rate is? This approach has the big advantage of “softening” the decision making process – in fact, if you accept the Neyman definition of the \(p\) value, that’s the whole point of the \(p\) value. We no longer have a fixed significance level of \(\alpha = .05\) as a bright line separating “accept” from “reject” decisions; and this removes the rather pathological problem of being forced to treat \(p = .051\) in a fundamentally different way to \(p = .049\) .
This flexibility is both the advantage and the disadvantage to the \(p\) value. The reason why a lot of people don’t like the idea of reporting an exact \(p\) value is that it gives the researcher a bit too much freedom. In particular, it lets you change your mind about what error tolerance you’re willing to put up with after you look at the data. For instance, consider my ESP experiment. Suppose I ran my test, and ended up with a \(p\) value of .09. Should I accept or reject? Now, to be honest, I haven’t yet bothered to think about what level of Type I error I’m “really” willing to accept. I don’t have an opinion on that topic. But I do have an opinion about whether or not ESP exists, and I definitely have an opinion about whether my research should be published in a reputable scientific journal. And amazingly, now that I’ve looked at the data I’m starting to think that a 9% error rate isn’t so bad, especially when compared to how annoying it would be to have to admit to the world that my experiment has failed. So, to avoid looking like I just made it up after the fact, I now say that my \(\alpha\) is .1: a 10% type I error rate isn’t too bad, and at that level my test is significant! I win.
In other words, the worry here is that I might have the best of intentions, and be the most honest of people, but the temptation to just “shade” things a little bit here and there is really, really strong. As anyone who has ever run an experiment can attest, it’s a long and difficult process, and you often get very attached to your hypotheses. It’s hard to let go and admit the experiment didn’t find what you wanted it to find. And that’s the danger here. If we use the “raw” \(p\) -value, people will start interpreting the data in terms of what they want to believe, not what the data are actually saying… and if we allow that, well, why are we bothering to do science at all? Why not let everyone believe whatever they like about anything, regardless of what the facts are? Okay, that’s a bit extreme, but that’s where the worry comes from. According to this view, you really must specify your \(\alpha\) value in advance, and then only report whether the test was significant or not. It’s the only way to keep ourselves honest.
12.6.2. Two proposed solutions #
In practice, it’s pretty rare for a researcher to specify a single \(\alpha\) level ahead of time. Instead, the convention is that scientists rely on three standard significance levels: .05, .01 and .001. When reporting your results, you indicate which (if any) of these significance levels allow you to reject the null hypothesis. This is summarised in the table below. This allows us to soften the decision rule a little bit, since \(p<.01\) implies that the data meet a stronger evidentiary standard than \(p<.05\) would. Nevertheless, since these levels are fixed in advance by convention, it does prevent people choosing their \(\alpha\) level after looking at the data.
Nevertheless, quite a lot of people still prefer to report exact \(p\) values. To many people, the advantage of allowing the reader to make up their own mind about how to interpret \(p = .06\) outweighs any disadvantages. In practice, however, even among those researchers who prefer exact \(p\) values it is quite common to just write \(p<.001\) instead of reporting an exact value for small \(p\) . This is in part because a lot of software doesn’t actually print out the \(p\) value when it’s that small (e.g., SPSS just writes \(p = .000\) whenever \(p<.001\) ), and in part because a very small \(p\) value can be kind of misleading. The human mind sees a number like .0000000001 and it’s hard to suppress the gut feeling that the evidence in favour of the alternative hypothesis is a near certainty. In practice however, this is usually wrong. Life is a big, messy, complicated thing: and every statistical test ever invented relies on simplifications, approximations and assumptions. As a consequence, it’s probably not reasonable to walk away from any statistical analysis with a feeling of confidence stronger than \(p<.001\) implies. In other words, \(p<.001\) is really code for “as far as this test is concerned, the evidence is overwhelming.”
In light of all this, you might be wondering exactly what you should do. There’s a fair bit of contradictory advice on the topic, with some people arguing that you should report the exact \(p\) value, and other people arguing that you should use the tiered approach illustrated in the table above. As a result, the best advice I can give is to suggest that you look at papers/reports written in your field and see what the convention seems to be. If there doesn’t seem to be any consistent pattern, then use whichever method you prefer.
12.7. Running the hypothesis test in practice #
At this point some of you might be wondering if this is a “real” hypothesis test, or just a toy example that I made up. It’s real. In the previous discussion I built the test from first principles, thinking that it was the simplest possible problem that you might ever encounter in real life. However, this test already exists: it’s called the binomial test , and it’s implemented in a function called binom_test() from the scipy.stats package. To test the null hypothesis that the response probability is one-half p = .5 , [ 9 ] using data in which x = 62 of n = 100 people made the correct response, here’s how to do it in Python:
Well. There’s a number, but what does it mean? Sometimes the output of these Python functions can be fairly terse. But here binom_test() is giving us the \(p\) -value for the test we specified. In this case, the \(p\) -value of 0.02 is less than the usual choice of \(\alpha = .05\) , so we can reject the null. Usually we will want to know more than just the \(p\) -value for a test, and Python has ways of giving us this information, but for now, however, I just wanted to make the point that Python packages contain a whole lot of functions corresponding to different kinds of hypothesis test. And while I’ll usually spend quite a lot of time explaining the logic behind how the tests are built, every time I discuss a hypothesis test the discussion will end with me showing you a fairly simple Python command that you can use to run the test in practice.
12.8. Effect size, sample size and power #
In previous sections I’ve emphasised the fact that the major design principle behind statistical hypothesis testing is that we try to control our Type I error rate. When we fix \(\alpha = .05\) we are attempting to ensure that only 5% of true null hypotheses are incorrectly rejected. However, this doesn’t mean that we don’t care about Type II errors. In fact, from the researcher’s perspective, the error of failing to reject the null when it is actually false is an extremely annoying one. With that in mind, a secondary goal of hypothesis testing is to try to minimise \(\beta\) , the Type II error rate, although we don’t usually talk in terms of minimising Type II errors. Instead, we talk about maximising the power of the test. Since power is defined as \(1-\beta\) , this is the same thing.

12.8.1. The power function #
Let’s take a moment to think about what a Type II error actually is. A Type II error occurs when the alternative hypothesis is true, but we are nevertheless unable to reject the null hypothesis. Ideally, we’d be able to calculate a single number \(\beta\) that tells us the Type II error rate, in the same way that we can set \(\alpha = .05\) for the Type I error rate. Unfortunately, this is a lot trickier to do. To see this, notice that in my ESP study the alternative hypothesis actually corresponds to lots of possible values of \(\theta\) . In fact, the alternative hypothesis corresponds to every value of \(\theta\) except 0.5. Let’s suppose that the true probability of someone choosing the correct response is 55% (i.e., \(\theta = .55\) ). If so, then the true sampling distribution for \(X\) is not the same one that the null hypothesis predicts: the most likely value for \(X\) is now 55 out of 100. Not only that, the whole sampling distribution has now shifted, as shown in fig-esp-alternative . The critical regions, of course, do not change: by definition, the critical regions are based on what the null hypothesis predicts. What we’re seeing in this figure is the fact that when the null hypothesis is wrong, a much larger proportion of the sampling distribution distribution falls in the critical region. And of course that’s what should happen: the probability of rejecting the null hypothesis is larger when the null hypothesis is actually false! However \(\theta = .55\) is not the only possibility consistent with the alternative hypothesis. Let’s instead suppose that the true value of \(\theta\) is actually 0.7. What happens to the sampling distribution when this occurs? The answer, shown in fig-esp-alternative2 , is that almost the entirety of the sampling distribution has now moved into the critical region. Therefore, if \(\theta = 0.7\) the probability of us correctly rejecting the null hypothesis (i.e., the power of the test) is much larger than if \(\theta = 0.55\) . In short, while \(\theta = .55\) and \(\theta = .70\) are both part of the alternative hypothesis, the Type II error rate is different.

What all this means is that the power of a test (i.e., \(1-\beta\) ) depends on the true value of \(\theta\) . To illustrate this, I’ve calculated the expected probability of rejecting the null hypothesis for all values of \(\theta\) , and plotted it in fig-powerfunction . This plot describes what is usually called the power function of the test. It’s a nice summary of how good the test is, because it actually tells you the power ( \(1-\beta\) ) for all possible values of \(\theta\) . As you can see, when the true value of \(\theta\) is very close to 0.5, the power of the test drops very sharply, but when it is further away, the power is large.

12.8.2. Effect size #
Since all models are wrong the scientist must be alert to what is importantly wrong. It is inappropriate to be concerned with mice when there are tigers abroad – George Box 1976
The plot shown in fig-powerfunction captures a fairly basic point about hypothesis testing. If the true state of the world is very different from what the null hypothesis predicts, then your power will be very high; but if the true state of the world is similar to the null (but not identical) then the power of the test is going to be very low. Therefore, it’s useful to be able to have some way of quantifying how “similar” the true state of the world is to the null hypothesis. A statistic that does this is called a measure of effect size (e.g. [ Cohen, 1988 ] or [ Ellis, 2010 ] ). Effect size is defined slightly differently in different contexts (and so this section just talks in general terms) but the qualitative idea that it tries to capture is always the same: how big is the difference between the true population parameters, and the parameter values that are assumed by the null hypothesis? In our ESP example, if we let \(\theta_0 = 0.5\) denote the value assumed by the null hypothesis, and let \(\theta\) denote the true value, then a simple measure of effect size could be something like the difference between the true value and null (i.e., \(\theta - \theta_0\) ), or possibly just the magnitude of this difference, \(\mbox{abs}(\theta - \theta_0)\) .
Why calculate effect size? Let’s assume that you’ve run your experiment, collected the data, and gotten a significant effect when you ran your hypothesis test. Isn’t it enough just to say that you’ve gotten a significant effect? Surely that’s the point of hypothesis testing? Well, sort of. Yes, the point of doing a hypothesis test is to try to demonstrate that the null hypothesis is wrong, but that’s hardly the only thing we’re interested in. If the null hypothesis claimed that \(\theta = .5\) , and we show that it’s wrong, we’ve only really told half of the story. Rejecting the null hypothesis implies that we believe that \(\theta \neq .5\) , but there’s a big difference between \(\theta = .51\) and \(\theta = .8\) . If we find that \(\theta = .8\) , then not only have we found that the null hypothesis is wrong, it appears to be very wrong. On the other hand, suppose we’ve successfully rejected the null hypothesis, but it looks like the true value of \(\theta\) is only .51 (this would only be possible with a large study). Sure, the null hypothesis is wrong, but it’s not at all clear that we actually care , because the effect size is so small. In the context of my ESP study we might still care, since any demonstration of real psychic powers would actually be pretty cool [ 10 ] , but in other contexts a 1% difference isn’t very interesting, even if it is a real difference. For instance, suppose we’re looking at differences in high school exam scores between males and females, and it turns out that the female scores are 1% higher on average than the males. If I’ve got data from thousands of students, then this difference will almost certainly be statistically significant , but regardless of how small the \(p\) value is it’s just not very interesting. You’d hardly want to go around proclaiming a crisis in boys education on the basis of such a tiny difference would you? It’s for this reason that it is becoming more standard (slowly, but surely) to report some kind of standard measure of effect size along with the the results of the hypothesis test. The hypothesis test itself tells you whether you should believe that the effect you have observed is real (i.e., not just due to chance); the effect size tells you whether or not you should care.
12.8.3. Increasing the power of your study #
Not surprisingly, scientists are fairly obsessed with maximising the power of their experiments. We want our experiments to work, and so we want to maximise the chance of rejecting the null hypothesis if it is false (and of course we usually want to believe that it is false!) As we’ve seen, one factor that influences power is the effect size. So the first thing you can do to increase your power is to increase the effect size. In practice, what this means is that you want to design your study in such a way that the effect size gets magnified. For instance, in my ESP study I might believe that psychic powers work best in a quiet, darkened room; with fewer distractions to cloud the mind. Therefore I would try to conduct my experiments in just such an environment: if I can strengthen people’s ESP abilities somehow, then the true value of \(\theta\) will go up [ 11 ] and therefore my effect size will be larger. In short, clever experimental design is one way to boost power; because it can alter the effect size.
Unfortunately, it’s often the case that even with the best of experimental designs you may have only a small effect. Perhaps, for example, ESP really does exist, but even under the best of conditions it’s very very weak. Under those circumstances, your best bet for increasing power is to increase the sample size. In general, the more observations that you have available, the more likely it is that you can discriminate between two hypotheses. If I ran my ESP experiment with 10 participants, and 7 of them correctly guessed the colour of the hidden card, you wouldn’t be terribly impressed. But if I ran it with 10,000 participants and 7,000 of them got the answer right, you would be much more likely to think I had discovered something. In other words, power increases with the sample size. This is illustrated in fig-powerfunctionsample , which shows the power of the test for a true parameter of \(\theta = 0.7\) , for all sample sizes \(N\) from 1 to 100, where I’m assuming that the null hypothesis predicts that \(\theta_0 = 0.5\) .

Because power is important, whenever you’re contemplating running an experiment it would be pretty useful to know how much power you’re likely to have. It’s never possible to know for sure, since you can’t possibly know what your effect size is. However, it’s often (well, sometimes) possible to guess how big it should be. If so, you can guess what sample size you need! This idea is called power analysis , and if it’s feasible to do it, then it’s very helpful, since it can tell you something about whether you have enough time or money to be able to run the experiment successfully. It’s increasingly common to see people arguing that power analysis should be a required part of experimental design, so it’s worth knowing about. I don’t discuss power analysis in this book, however. This is partly for a boring reason and partly for a substantive one. The boring reason is that I haven’t had time to write about power analysis yet. The substantive one is that I’m still a little suspicious of power analysis. Speaking as a researcher, I have very rarely found myself in a position to be able to do one – it’s either the case that (a) my experiment is a bit non-standard and I don’t know how to define effect size properly, or (b) I literally have so little idea about what the effect size will be that I wouldn’t know how to interpret the answers. Not only that, after extensive conversations with someone who does stats consulting for a living (my wife, as it happens), I can’t help but notice that in practice the only time anyone ever asks her for a power analysis is when she’s helping someone write a grant application. In other words, the only time any scientist ever seems to want a power analysis in real life is when they’re being forced to do it by bureaucratic process. It’s not part of anyone’s day to day work. In short, I’ve always been of the view that while power is an important concept, power analysis is not as useful as people make it sound, except in the rare cases where (a) someone has figured out how to calculate power for your actual experimental design and (b) you have a pretty good idea what the effect size is likely to be. Maybe other people have had better experiences than me, but I’ve personally never been in a situation where both (a) and (b) were true. Maybe I’ll be convinced otherwise in the future, and probably a future version of this book would include a more detailed discussion of power analysis, but for now this is about as much as I’m comfortable saying about the topic.
12.9. Some issues to consider #
What I’ve described to you in this chapter is the orthodox framework for null hypothesis significance testing (NHST). Understanding how NHST works is an absolute necessity, since it has been the dominant approach to inferential statistics ever since it came to prominence in the early 20th century. It’s what the vast majority of working scientists rely on for their data analysis, so even if you hate it you need to know it. However, the approach is not without problems. There are a number of quirks in the framework, historical oddities in how it came to be, theoretical disputes over whether or not the framework is right, and a lot of practical traps for the unwary. I’m not going to go into a lot of detail on this topic, but I think it’s worth briefly discussing a few of these issues.
12.9.1. Neyman versus Fisher #
The first thing you should be aware of is that orthodox NHST is actually a mash-up of two rather different approaches to hypothesis testing, one proposed by Sir Ronald Fisher and the other proposed by Jerzy Neyman (for a historical summary see [ Lehmann, 2011 ] . The history is messy because Fisher and Neyman were real people whose opinions changed over time, and at no point did either of them offer “the definitive statement” of how we should interpret their work many decades later. That said, here’s a quick summary of what I take these two approaches to be.
First, let’s talk about Fisher’s approach. As far as I can tell, Fisher assumed that you only had the one hypothesis (the null), and what you want to do is find out if the null hypothesis is inconsistent with the data. From his perspective, what you should do is check to see if the data are “sufficiently unlikely” according to the null. In fact, if you remember back to our earlier discussion, that’s how Fisher defines the \(p\) -value. According to Fisher, if the null hypothesis provided a very poor account of the data, you could safely reject it. But, since you don’t have any other hypotheses to compare it to, there’s no way of “accepting the alternative” because you don’t necessarily have an explicitly stated alternative. That’s more or less all that there was to it.
In contrast, Neyman thought that the point of hypothesis testing was as a guide to action, and his approach was somewhat more formal than Fisher’s. His view was that there are multiple things that you could do (accept the null or accept the alternative) and the point of the test was to tell you which one the data support. From this perspective, it is critical to specify your alternative hypothesis properly. If you don’t know what the alternative hypothesis is, then you don’t know how powerful the test is, or even which action makes sense. His framework genuinely requires a competition between different hypotheses. For Neyman, the \(p\) value didn’t directly measure the probability of the data (or data more extreme) under the null, it was more of an abstract description about which “possible tests” were telling you to accept the null, and which “possible tests” were telling you to accept the alternative.
As you can see, what we have today is an odd mishmash of the two. We talk about having both a null hypothesis and an alternative (Neyman), but usually [ 12 ] define the \(p\) value in terms of exreme data (Fisher), but we still have \(\alpha\) values (Neyman). Some of the statistical tests have explicitly specified alternatives (Neyman) but others are quite vague about it (Fisher). And, according to some people at least, we’re not allowed to talk about accepting the alternative (Fisher). It’s a mess: but I hope this at least explains why it’s a mess.
12.9.2. Bayesians versus frequentists #
Earlier on in this chapter I was quite emphatic about the fact that you cannot interpret the \(p\) value as the probability that the null hypothesis is true. NHST is fundamentally a frequentist tool (see the chapter on probability ) and as such it does not allow you to assign probabilities to hypotheses: the null hypothesis is either true or it is not. The Bayesian approach to statistics interprets probability as a degree of belief, so it’s totally okay to say that there is a 10% chance that the null hypothesis is true: that’s just a reflection of the degree of confidence that you have in this hypothesis. You aren’t allowed to do this within the frequentist approach. Remember, if you’re a frequentist, a probability can only be defined in terms of what happens after a large number of independent replications (i.e., a long run frequency). If this is your interpretation of probability, talking about the “probability” that the null hypothesis is true is complete gibberish: a null hypothesis is either true or it is false. There’s no way you can talk about a long run frequency for this statement. To talk about “the probability of the null hypothesis” is as meaningless as “the colour of freedom”. It doesn’t have one!
Most importantly, this isn’t a purely ideological matter. If you decide that you are a Bayesian and that you’re okay with making probability statements about hypotheses, you have to follow the Bayesian rules for calculating those probabilities. I’ll talk more about this in the chapter on Bayesian statistics , but for now what I want to point out to you is the \(p\) value is a terrible approximation to the probability that \(H_0\) is true. If what you want to know is the probability of the null, then the \(p\) value is not what you’re looking for!
12.9.3. Traps #
As you can see, the theory behind hypothesis testing is a mess, and even now there are arguments in statistics about how it “should” work. However, disagreements among statisticians are not our real concern here. Our real concern is practical data analysis. And while the “orthodox” approach to null hypothesis significance testing has many drawbacks, even an unrepentant Bayesian like myself would agree that they can be useful if used responsibly. Most of the time they give sensible answers, and you can use them to learn interesting things. Setting aside the various ideologies and historical confusions that we’ve discussed, the fact remains that the biggest danger in all of statistics is thoughtlessness . I don’t mean stupidity, here: I literally mean thoughtlessness. The rush to interpret a result without spending time thinking through what each test actually says about the data, and checking whether that’s consistent with how you’ve interpreted it. That’s where the biggest trap lies.
To give an example of this, consider the following example see [ Gelman and Stern, 2006 ] . Suppose I’m running my ESP study, and I’ve decided to analyse the data separately for the male participants and the female participants. Of the male participants, 33 out of 50 guessed the colour of the card correctly. This is a significant effect ( \(p = .03\) ). Of the female participants, 29 out of 50 guessed correctly. This is not a significant effect ( \(p = .32\) ). Upon observing this, it is extremely tempting for people to start wondering why there is a difference between males and females in terms of their psychic abilities. However, this is wrong. If you think about it, we haven’t actually run a test that explicitly compares males to females. All we have done is compare males to chance (binomial test was significant) and compared females to chance (binomial test was non significant). If we want to argue that there is a real difference between the males and the females, we should probably run a test of the null hypothesis that there is no difference! We can do that using a different hypothesis test, [ 13 ] but when we do that it turns out that we have no evidence that males and females are significantly different ( \(p = .54\) ). Now do you think that there’s anything fundamentally different between the two groups? Of course not. What’s happened here is that the data from both groups (male and female) are pretty borderline: by pure chance, one of them happened to end up on the magic side of the \(p = .05\) line, and the other one didn’t. That doesn’t actually imply that males and females are different. This mistake is so common that you should always be wary of it: the difference between significant and not-significant is not evidence of a real difference – if you want to say that there’s a difference between two groups, then you have to test for that difference!
The example above is just that: an example. I’ve singled it out because it’s such a common one, but the bigger picture is that data analysis can be tricky to get right. Think about what it is you want to test, why you want to test it, and whether or not the answers that your test gives could possibly make any sense in the real world.
12.10. Summary #
Null hypothesis testing is one of the most ubiquitous elements to statistical theory. The vast majority of scientific papers report the results of some hypothesis test or another. As a consequence it is almost impossible to get by in science without having at least a cursory understanding of what a \(p\) -value means, making this one of the most important chapters in the book. As usual, I’ll end the chapter with a quick recap of the key ideas that we’ve talked about:
Research hypotheses and statistical hypotheses . Null and alternative hypotheses .
Type 1 and Type 2 errors
Test statistics and sampling distributions
Hypothesis testing as a decision making process
\(p\) -values as “soft” decisions
Writing up the results of a hypothesis test
Effect size and power
A few issues to consider regarding hypothesis testing
Later in the book, in the section on Bayesian statistics , I’ll revisit the theory of null hypothesis tests from a Bayesian perspective, and introduce a number of new tools that you can use if you aren’t particularly fond of the orthodox approach. But for now, though, we’re done with the abstract statistical theory, and we can start discussing specific data analysis tools.
- Pydantic Settings
- Pydantic People
Hypothesis is the Python library for property-based testing . Hypothesis can infer how to construct type-annotated classes, and supports builtin types, many standard library types, and generic types from the typing and typing_extensions modules by default.
Pydantic v2.0 drops built-in support for Hypothesis and no more ships with the integrated Hypothesis plugin.
We are temporarily removing the Hypothesis plugin in favor of studying a different mechanism. For more information, see the issue annotated-types/annotated-types#37 .
The Hypothesis plugin may be back in a future release. Subscribe to pydantic/pydantic#4682 for updates.
- History of cooperation
- Areas of cooperation
- Procurement policy
- Useful links
- Becoming a supplier
- Procurement
- Rosatom newsletter
© 2008–2024Valtiollinen Rosatom-ydinvoimakonserni

- Rosatom Global presence
- Rosatom in region
- For suppliers
- Preventing corruption
- Press centre
Rosatom Starts Life Tests of Third-Generation VVER-440 Nuclear Fuel
- 16 June, 2020 / 13:00
This site uses cookies. By continuing your navigation, you accept the use of cookies. For more information, or to manage or to change the cookies parameters on your computer, read our Cookies Policy. Learn more
Toroidally focused ultrasonic flaw detectors
- Acoustic Methods
- Published: 28 July 2011
- Volume 47 , pages 308–310, ( 2011 )
Cite this article
- A. V. Shevelev 1 &
- Zh. V. Zatsepilova 2
33 Accesses
Explore all metrics
New-type toroidally focused ultrasonic flaw detectors, whose application provides an appreciable increase in the flaw detection rate with retention of high sensitivity to flaws, are considered. The construction of a flaw detector is presented, the sizes of a gauge for the formation of the toroidal surface of a lens are given, and the technology of the manufacturing of a toroidal lens is described.
This is a preview of subscription content, log in via an institution to check access.
Access this article
Price includes VAT (Russian Federation)
Instant access to the full article PDF.
Rent this article via DeepDyve
Institutional subscriptions
Similar content being viewed by others
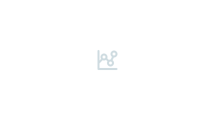
Remote diagnostics of soft solids using nonlinear acoustic methods
A. I. Korobov, M. Yu. Izosimova, … N. I. Odina
Ultrasonic Flaw Detection: Adjustment and Calibration of Equipment Using Samples with Cylindrical Drilling
L. Yu. Mogilner & Ya. G. Smorodinskii
Analyzing the Phase of DFA Image for Determining the Type of Detected Reflector
E. G. Bazulin, A. Kh. Vopilkin, … D. S. Tikhonov
Ermolov, I.N., Aleshin, N.P., and Potapov, A.I., Nerazrushayushchii control’ (Nondestructive Testing), book 2: Akusticheskie metody kontrolya (Acoustic Testing), Moscow: Vysshaya shkola, 1991.
Google Scholar
Nerazrushayushchii kontrol’ (Spravochnik) (Nondestructive Testing: Handbook), Klyuev, V.V., Ed., vol. 3: Ul’trazvukovoi kontrol’ (Ultrasonic Testing), Moscow: Mashinostroenie, 2006.
Download references
Author information
Authors and affiliations.
Elektrostal Polytechnic Institute, Branch of the National University of Science and Technology “MISIS”, ul. Pervomaiskaya 7, Elektrostal, Moscow oblast, 144000, Russia
A. V. Shevelev
Elektrostal Heavy Engineering Plant JSC, ul. Krasnaya 19, Elektrostal, Moscow oblast, 144005, Russia
Zh. V. Zatsepilova
You can also search for this author in PubMed Google Scholar
Corresponding author
Correspondence to Zh. V. Zatsepilova .
Additional information
Original Russian Text © A.V. Shevelev, Zh.V. Zatsepilova, 2011, published in Defektoskopiya, 2011, Vol. 47, No. 5, pp. 19–22.
Rights and permissions
Reprints and permissions
About this article
Shevelev, A.V., Zatsepilova, Z.V. Toroidally focused ultrasonic flaw detectors. Russ J Nondestruct Test 47 , 308–310 (2011). https://doi.org/10.1134/S1061830911050093
Download citation
Received : 14 January 2011
Published : 28 July 2011
Issue Date : May 2011
DOI : https://doi.org/10.1134/S1061830911050093
Share this article
Anyone you share the following link with will be able to read this content:
Sorry, a shareable link is not currently available for this article.
Provided by the Springer Nature SharedIt content-sharing initiative
- ultrasonic flaw detectors
- focusing lens
- flaw detection
- nondestructive testing
- inspection of pipes
- Find a journal
- Publish with us
- Track your research
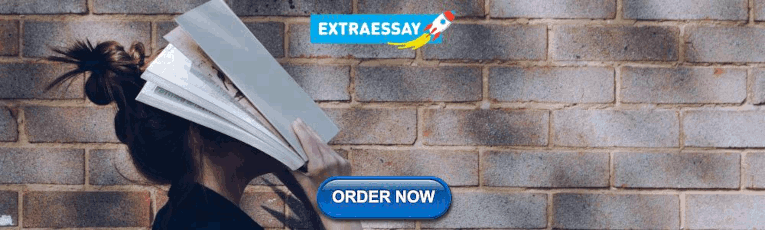
IMAGES
VIDEO
COMMENTS
Welcome to Hypothesis! Hypothesis is a Python library for creating unit tests which are simpler to write and more powerful when run, finding edge cases in your code you wouldn't have thought to look for. It is stable, powerful and easy to add to any existing test suite. It works by letting you write tests that assert that something should be ...
Hypothesis is an advanced testing library for Python. It lets you write tests which are parametrized by a source of examples, and then generates simple and comprehensible examples that make your tests fail. This lets you find more bugs in your code with less work. e.g. xs=[1.7976321109618856e+308, 6.102390043022755e+303] Hypothesis is extremely ...
Example 1: One Sample t-test in Python. A one sample t-test is used to test whether or not the mean of a population is equal to some value. For example, suppose we want to know whether or not the mean weight of a certain species of some turtle is equal to 310 pounds. To test this, we go out and collect a simple random sample of turtles with the ...
It tests the null hypothesis that the population variances are equal (called homogeneity of variance or homoscedasticity). Suppose the resulting p-value of Levene's test is less than the significance level (typically 0.05).In that case, the obtained differences in sample variances are unlikely to have occurred based on random sampling from a population with equal variances.
In this article, we interactively explore and visualize the difference between three common statistical tests: T-test, ANOVA test and Chi-Squared test. We also use examples to walkthrough essential steps in hypothesis testing: 1. define the null and alternative hypothesis. 2. choose the appropriate test.
Dive into the fascinating process of hypothesis testing with Python in this comprehensive guide. Perfect for aspiring data scientists and analytical minds, learn how to validate your predictions using statistical tests and Python's robust libraries. From understanding the basics of hypothesis formulation to executing detailed statistical analysis, this article illuminates the path to data ...
The process of hypothesis testing involves four steps: Now that we have a basic understanding of the concept, let's move on to the implementation in Python. We will use the scipy library to ...
This sort of testing is often called "property-based testing", and the most widely known implementation of the concept is the Haskell library QuickCheck, but Hypothesis differs significantly from QuickCheck and is designed to fit idiomatically and easily into existing styles of testing that you are used to, with absolutely no familiarity with ...
Apart from academic research, hypothesis testing is particularly useful to data scientists, as it lets them conduct A/B tests and other experiments. In this article, we are going to examine a case study of hypothesis testing on the seeds dataset, by using the Pingouin Python library. The Basic Steps of Hypothesis Testing
In this post, you will discover a cheat sheet for the most popular statistical hypothesis tests for a machine learning project with examples using the Python API. Each statistical test is presented in a consistent way, including: The name of the test. What the test is checking. The key assumptions of the test. How the test result is interpreted.
June 24, 2016. Python. Anne Mulhern. Hypothesis is a Python library for creating tests which are simple to write and powerful when run, finding. cases in your code you wouldn't have thought to look for. It is stable, powerful and easy to add to an existing test suite. It works by letting you write tests that assert that something should be true ...
Hypothesis testing is the act of testing whether a hypothesis or inference is true. When an alternate hypothesis is introduced, we test it against the null hypothesis to know which is correct. ... Numpy is a Python library used for scientific computing. It has a large library of functions for working with arrays. Scipy is a library for ...
12. Hypothesis Testing #. The process of induction is the process of assuming the simplest law that can be made to harmonize with our experience. This process, however, has no logical foundation but only a psychological one. It is clear that there are no grounds for believing that the simplest course of events will really happen.
1. In that case, try: logger.debug('silly_example(%s) called', some_number). - unutbu. Oct 31, 2018 at 18:41. 2. The problem with logging or printing from a Hypothesis test is that the output does not distinguish between test cases, so it can be hard to tell which lines came from a particular failing case.
Yes, they're essentially the same thing - rejection sampling. However, there's an important difference in practice: s.filter() allows Hypothesis to reject part of an example and try again (within limits!), whereas assume() has to throw away the whole test case and start over. If we ignore all the heuristics, runtime feedback, splicing, etc. for the sake of illustration... if we generate length ...
Hypothesis. Hypothesis is the Python library for property-based testing.Hypothesis can infer how to construct type-annotated classes, and supports builtin types, many standard library types, and generic types from the typing and typing_extensions modules by default. Pydantic v2.0 drops built-in support for Hypothesis and no more ships with the integrated Hypothesis plugin.
Elektrostal is a city in Moscow Oblast, Russia, located 58 kilometers east of Moscow. Elektrostal has about 158,000 residents. Mapcarta, the open map.
In 1954, Elemash began to produce fuel assemblies, including for the first nuclear power plant in the world, located in Obninsk. In 1959, the facility produced the fuel for the Soviet Union's first icebreaker. Its fuel assembly production became serial in 1965 and automated in 1982. 1. Today, Elemash is one of the largest TVEL nuclear fuel ...
The life tests started after successful completion of hydraulic tests (hydraulic filling) of the mock-up with the aim to determine RK3+ hydraulic resistance. Life tests are carried out on a full-scale research hot run-in test bench V-440 and will last for full 1500 hours. The aim of tests is to study mechanical stability of RK3+ components ...
New-type toroidally focused ultrasonic flaw detectors, whose application provides an appreciable increase in the flaw detection rate with retention of high sensitivity to flaws, are considered. The construction of a flaw detector is presented, the sizes of a gauge for the formation of the toroidal surface of a lens are given, and the technology of the manufacturing of a toroidal lens is described.