- Introduction to Machine Learning
- Machine Learning with R
- Machine Learning with Python
- Statistics in R
- Math for Machine Learning
- Machine Learning Interview Questions
- Projects in R
- Deep Learning with R
- AI Algorithm
Weather Data Visualization
Visualizing weather data is essential for understanding trends, patterns, and anomalies in meteorological information. R, with its robust packages for data manipulation and visualization, offers powerful tools to create insightful weather data visualizations. This article will guide you through the process of visualizing weather data in R, covering data acquisition, preprocessing, and creating various types of visualizations.
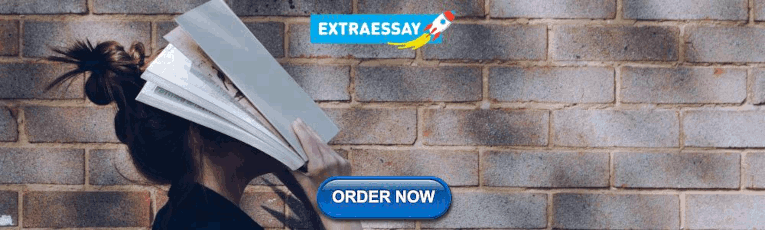
Objectives and Goals
The primary objective of this article is to explore weather data visualization techniques and develop a forecasting model using R Programming Language . By exploring a dataset containing historical weather information, we try to demonstrate the power of data visualization in understanding trends and patterns, as well as the effectiveness of forecasting models in predicting future weather conditions.
Dataset Link: Weather History
The weather dataset has various columns such as:
- Formatted Date
- Precip Type
- Temperature (C)
- Apparent Temperature (C)
- Wind Speed (km/h)
- Wind Bearing (degrees)
- Visibility (km)
- Pressure (millibars)
- Daily Summary
We’re using the weather dataset for our analysis and predictions. By this, we can make sure that our results are reliable and stay the same without adding any extra data from outside sources.
Step 1: Load Required Libraries and Dataset
Loads the necessary libraries ggplot2 and forecast into the R environment. These libraries contain functions and tools that we’ll use for data visualization and forecasting, respectively. Here, we read the weather dataset from the specified file path using the read.csv() function.
Step 2: Display the Structure of the Dataset
It displays the structure of the weather_data dataset, showing information about its variables, data types, and other properties. It helps us to understand the structure of the dataset we’re working with.
Step 3: Visualization of Weather Data
Now we will plot the visualization of our Weather Data to get some valid pieces of information.
Histogram of Precipitation Intensity
A histogram of precipitation intensity reveals the distribution of precipitation events, highlighting the most common precipitation intensities and indicating the range of values.
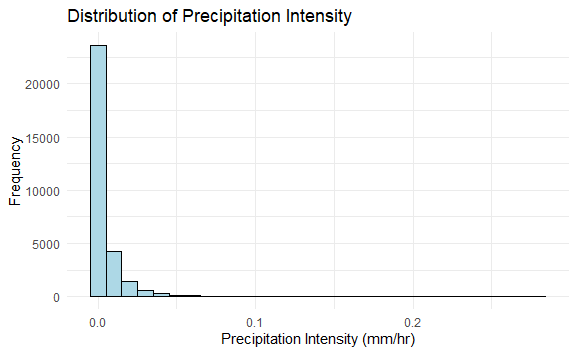
Scatter Plot of Wind Speed vs. Temperature
A scatter plot shows the relationship between wind speed and high temperature. This can help identify any correlations between wind conditions and temperature changes.
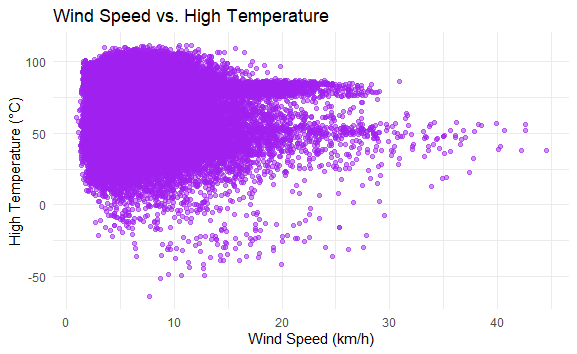
Bar Plot of Precipitation Types
A bar plot displays the frequency of different types of precipitation (e.g., rain, snow), providing an overview of the distribution of precipitation types within the dataset.
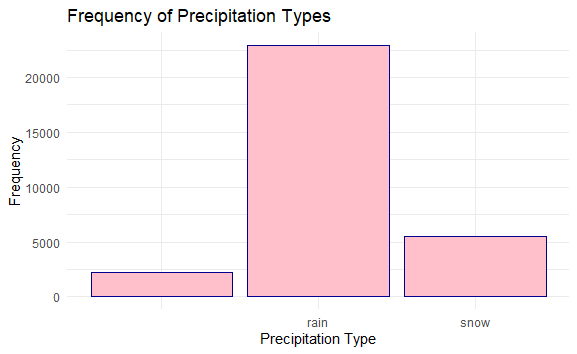
Histogram with Density Plot
We can plot the distribution of temperatures across the dataset, with the histogram showing the frequency of different temperature ranges.
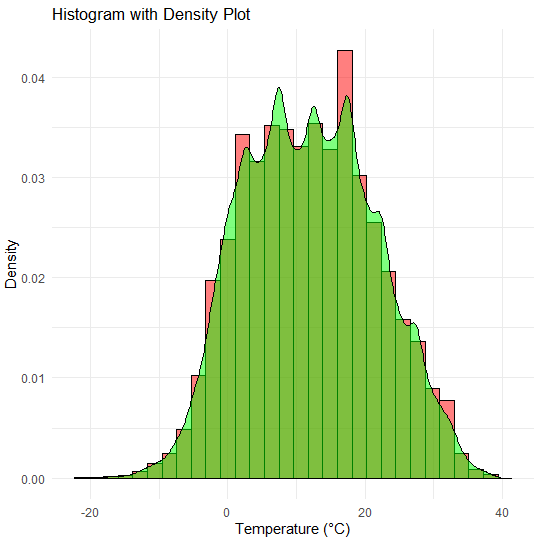
The spread of the histogram and density plot illustrates the variability or range of temperatures present in the dataset. A wider spread indicates a greater variability in temperature readings, while a narrower spread suggests more consistency in temperature values.
Visualizing weather data in R allows you to uncover trends, patterns, and anomalies in meteorological data. By leveraging packages like ggplot2, Plotly, and dygraphs, you can create both static and interactive visualizations that provide valuable insights. Whether you’re analyzing temperature trends, humidity levels, or wind speeds, R offers the tools you need to effectively visualize and interpret weather data.
Please Login to comment...
Similar reads.
- AI-ML-DS With R
- Data Science Blogathon 2024
- R Machine Learning
Improve your Coding Skills with Practice
What kind of Experience do you want to share?

- Rivers and Lakes
- Severe Weather
- Fire Weather
- Long Range Forecasts
- Climate Prediction
- Space Weather
- Past Weather
- Heating/Cooling Days
- Monthly Temperatures
- Astronomical Data
- Beach Hazards
- Air Quality
- Safe Boating
- Rip Currents
- Thunderstorms
- Sun (Ultraviolet Radiation)
- Safety Campaigns
- Winter Weather
- Wireless Emergency Alerts
- Weather-Ready Nation
- Cooperative Observers
- Daily Briefing
- Damage/Fatality/Injury Statistics
- Forecast Models
- GIS Data Portal
- NOAA Weather Radio
- Publications
- SKYWARN Storm Spotters
- TsunamiReady
- Service Change Notices
- Be A Force of Nature
- NWS Education Home
- Pubs/Brochures/Booklets
- NWS Media Contacts
NWS All NOAA
- Organization
- Strategic Plan
- Commitment to Diversity
- For NWS Employees
- International
- National Centers
- Social Media
NWS Education
National Program
Meteorology 101 presentation
- Citizen Science
- Educator Resources
- Data Resources
- Connect with Us
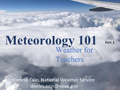
Meteorology 101
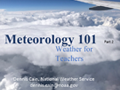
Meteorology 101 Part 2

US Dept of Commerce National Oceanic and Atmospheric Administration National Weather Service NWS Education 1325 East West Highway Silver Spring, MD 20910 Comments? Questions? Please Contact Us.
Announcement: SOA congratulates the new ASAs and CERAs for June 2024.
- CANDIDATE & STUDENT
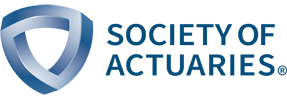
- Actuarial Directory Actuarial Directory Search by: Last Name First Name Company Name City Advanced Search Update Profile
What is an Actuary?
Actuarial education, career development, affiliate membership, designations & credentials, exams & requirements, university resources, major meetings, professional development opportunities, about the soa research institute, research by topic, research opportunities, tables, calculators & modeling tools, naaj practical application essays, actuarial research clearing house (arch), experience studies pro, about soa sections, professional development, actuarial practice, publications, mission and vision, topics in the news, volunteer program, contact soa, job opportunities, legal center.
- search Close
- Actuarial Directory
A Practical Guide for Working with Weather Datasets
Patrick Wiese, ASA
Description
This series of papers is intended to serve as a practical guide for actuaries and researchers who wish to analyze weather datasets. The first paper provides an overview of the main types of weather datasets. The second paper describes computer programming strategies for processing large weather datasets using a standard personal computer. Each subsequent paper describes a particular weather dataset, accompanied by a free, open-source computer program for analyzing the dataset. The computer programs – developed by SOA staff and by volunteers -- reduce the upfront time and effort required to begin working with weather datasets. Because each program is open source, researchers can modify and expand the code to suit their own purposes.
A wide range of datasets will be covered in this series of papers, including (1) data collected by weather stations; (2) data estimated using Doppler radar and/or sensors on satellites; (3) “reanalysis” datasets generated by weather models that assimilate historical data from many sources (land-based stations, ships, planes, weather balloons, buoys, satellites, and radar) and produce, as an output, spatially and temporally complete historical records; (4) short and medium term forecasts, (5) sub-seasonal and seasonal forecasts and (6) long-range climate projections.
Released Papers
Topic #1: The Main Types of Weather Datasets
This report categorizes weather datasets into 8 main types, of which 5 are historical data and 3 are forecast data. Each of the 8 dataset types is described, and an illustrative example of each is provided.
Topic #2: Strategies for Processing Large Weather Datasets
Report Demo Computer Programs
Many weather datasets exceed 100 gigabytes and some are much larger. While most climate scientists have access to servers that can store and process massive weather datasets, other types of researchers may wish to perform weather analyses on a standard personal computer. A personal computer rarely offers more than 1000 gigabytes of storage space and 16 to 32 gigabytes of RAM (active memory for running applications and programs). Given these constraints, a researcher will need a clever approach for working with large weather datasets. This paper describes techniques for running analyses of a large weather dataset despite the storage and memory limitations imposed by a personal computer. This paper is accompanied by 6 small computer programs written in VBA, Python, R, and C++, zipped together into a single file to simplify the download process. These programs illustrate techniques for processing large weather datasets using the limited memory (RAM) available on a personal computer.
Upcoming Papers
Topic #3: Introduction to the GHCN Daily Dataset
The Global Historical Climatology Network Daily (GHCNd) dataset consists of daily temperature, precipitation and wind speed observations collected from over 100,000 land-based weather stations. GHCNd provides geographic coverage of much of the world, but the availability of data varies significantly from one country to another, as well as within each country. In general, the density of weather stations is greatest in urban areas and lowest in rural areas.
Topic #4: Introduction to the CPC Global Temperature and Precipitation Datasets
To address the irregular spacing of ground-based weather stations, researchers have developed algorithms to translate station data into data on a regularly spaced geographic grid. The Climate Prediction Center – a federal agency that is part of the National Oceanic and Atmospheric Administration – uses station data to produce the Global Unified Temperature Dataset and the Global Unified Precipitation Dataset. These datasets cover the period from 1979 to the present and are gridded at 0.5 degrees (i.e. data is available every 0.5 degrees of latitude and longitude). For each day and for each grid point, the data provides minimum temperature, maximum temperature, and total precipitation. Note that the data does not include areas over oceans; rather, the data covers land areas.
Topic #5: Introduction to the ERA5 Dataset
“ERA5” is short for “Fifth generation European Center for Medium-Range Weather Forecasts Atmospheric Reanalysis of the Global Climate”. ERA5 is a “reanalysis” dataset. This type of data is produced by weather models that reconstruct the past in a manner consistent with available historical data, and consistent with the physical laws governing the earth’s atmosphere and oceans. The historical data fed into the model(s) can come from many sources, including weather stations, ships, planes, weather balloons, satellites, and radar. In contrast to station data which has an uneven distribution across space and time, reanalysis data is spatially and temporally complete. Reanalysis data is particularly helpful when analyzing historical trends in a region that has a low density of weather stations. ERA5 data extends from 1950 to the present in hourly time steps and provides coverage of the entire earth using a 0.25-degree geographic grid (i.e. data points are available every 0.25 degrees of latitude and longitude). The ERA5 dataset is updated daily, with a latency of only five days, and provides a comprehensive set of weather metrics including air temperature, air pressure, sea surface temperature, wind speed, total precipitation, and various measures of soil moisture.
Topic #6: Introduction to the IBTrACS Dataset
IBTrACS stands for “International Best Track Archive for Climate Stewardship”. This dataset contains worldwide data on the paths and intensities of tropical cyclones that occurred between 1842 and the present.
Topic #7: Introduction to NOAA’s Storm Events Database
The Storm Events Database, compiled by the National Oceanic and Atmospheric Administration (NOAA), contains data for severe weather events in the United States having sufficient intensity to cause loss of life, injuries, significant property damage, and/or disruption to commerce. This database covers the period from 1950 to the present, and provides loss estimates in addition to data describing each severe weather event.
Topic #8: CMIP6 Climate Projections
A climate projection provides a sense of how the distribution of weather events may gradually change in the decades ahead. Perhaps the most well-known climate projections are those that feed into the climate assessment reports issued by the Intergovernmental Panel on Climate Change (IPCC). The IPCC issued its first climate assessment report in 1990. Since that time, an updated report has been released roughly every 5 years. The most recent report – the sixth in the series – was published in 2021. Because the modeling of climate change is complex, and because different models produce different results, the IPCC uses an “ensemble” of models to produce projections. For the latest IPCC report, the ensemble incudes over 100 models from more than 50 modeling centers. This ensemble is referred to as “CMIP6” which stands for “Coupled Model Intercomparison Project, Phase 6” (corresponding to the sixth report in the series). By running standardized greenhouse gas scenarios through each model in the ensemble, a range of forecasts is produced. The average results across all models serve as a best estimate, while the distribution of results provides some sense of the level of uncertainty.
Topics #9 and beyond: to be determined Topics #9 and beyond: to be determined -->
Many weather datasets exceed 100 gigabytes and some are much larger. While most climate scientists have access to servers that can store and process massive weather datasets, other types of researchers may wish to perform weather analyses on a standard personal computer. A personal computer rarely offers more than 1000 gigabytes of storage space and 16 to 32 gigabytes of RAM (active memory for running applications and programs). Given these constraints, a researcher will need a clever approach for working with large weather datasets. This paper describes techniques for running analyses of a large weather dataset despite the storage and memory limitations imposed by a personal computer.
Computer Programs for Tabulating Weather Datasets
We have not yet released any computer programs. Soon, we expect to release programs for tabulating both the GHCN dataset and the ERA5 dataset.
Questions or Comments?
Give us your feedback! Take a short survey on this report. Take Survey
If you have comments or questions, please send an email to [email protected] .
- Ways to Give
- Contact an Expert
- Explore WRI Perspectives
Filter Your Site Experience by Topic
Applying the filters below will filter all articles, data, insights and projects by the topic area you select.
- All Topics Remove filter
- Climate filter site by Climate
- Cities filter site by Cities
- Energy filter site by Energy
- Food filter site by Food
- Forests filter site by Forests
- Freshwater filter site by Freshwater
- Ocean filter site by Ocean
- Business filter site by Business
- Economics filter site by Economics
- Finance filter site by Finance
- Equity & Governance filter site by Equity & Governance
Search WRI.org
Not sure where to find something? Search all of the site's content.
Climate Watch Slide Deck
This Tool is part of Climate Watch within Climate Program . Reach out to Leandro Vigna for more information.
- Leandro Vigna
Need to know the latest trend in emissions, countries climate targets and how the top emitters compare?
The Climate Watch Slide Deck is a PPT deck of 27 curated slides providing answers to the most common climate change questions with data, visualizations and explanations.

We update the slides every two weeks and even sooner if major countries change their commitments. In the Notes section of each slide, we included source links and additional information to help you better interpret the data for messaging.
You can bookmark this resource and easily copy/paste the slides and visualizations into your presentations.
Access the Climate Watch Resource Bank here
Projects that include this Resource

Climate Watch
Climate Watch offers powerful insights and data on national climate plans, long-term strategies and greenhouse gas emissions to help countries achieve their climate and sustainable development goals.
Primary Contact

Outreach & Data Partnership Manager, Climate Watch
How You Can Help
WRI relies on the generosity of donors like you to turn research into action. You can support our work by making a gift today or exploring other ways to give.
Stay Informed
World Resources Institute 10 G Street NE Suite 800 Washington DC 20002 +1 (202) 729-7600
© 2024 World Resources Institute
Envision a world where everyone can enjoy clean air, walkable cities, vibrant landscapes, nutritious food and affordable energy.
Home Blog Design Understanding Data Presentations (Guide + Examples)
Understanding Data Presentations (Guide + Examples)
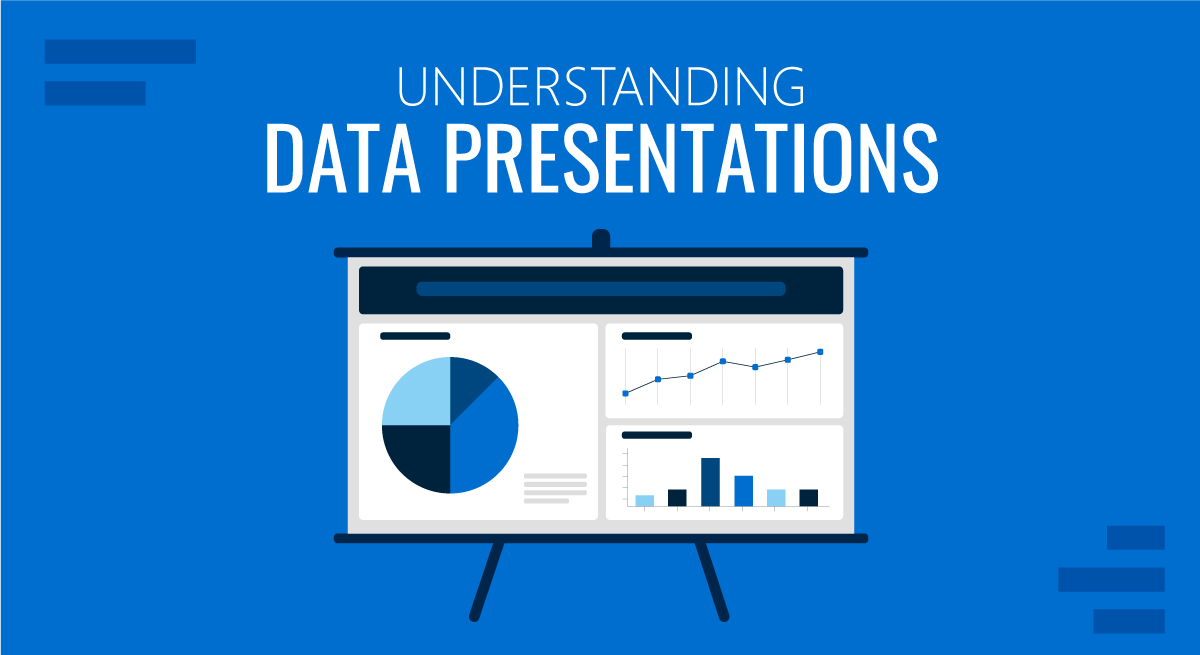
In this age of overwhelming information, the skill to effectively convey data has become extremely valuable. Initiating a discussion on data presentation types involves thoughtful consideration of the nature of your data and the message you aim to convey. Different types of visualizations serve distinct purposes. Whether you’re dealing with how to develop a report or simply trying to communicate complex information, how you present data influences how well your audience understands and engages with it. This extensive guide leads you through the different ways of data presentation.
Table of Contents
What is a Data Presentation?
What should a data presentation include, line graphs, treemap chart, scatter plot, how to choose a data presentation type, recommended data presentation templates, common mistakes done in data presentation.
A data presentation is a slide deck that aims to disclose quantitative information to an audience through the use of visual formats and narrative techniques derived from data analysis, making complex data understandable and actionable. This process requires a series of tools, such as charts, graphs, tables, infographics, dashboards, and so on, supported by concise textual explanations to improve understanding and boost retention rate.
Data presentations require us to cull data in a format that allows the presenter to highlight trends, patterns, and insights so that the audience can act upon the shared information. In a few words, the goal of data presentations is to enable viewers to grasp complicated concepts or trends quickly, facilitating informed decision-making or deeper analysis.
Data presentations go beyond the mere usage of graphical elements. Seasoned presenters encompass visuals with the art of data storytelling , so the speech skillfully connects the points through a narrative that resonates with the audience. Depending on the purpose – inspire, persuade, inform, support decision-making processes, etc. – is the data presentation format that is better suited to help us in this journey.
To nail your upcoming data presentation, ensure to count with the following elements:
- Clear Objectives: Understand the intent of your presentation before selecting the graphical layout and metaphors to make content easier to grasp.
- Engaging introduction: Use a powerful hook from the get-go. For instance, you can ask a big question or present a problem that your data will answer. Take a look at our guide on how to start a presentation for tips & insights.
- Structured Narrative: Your data presentation must tell a coherent story. This means a beginning where you present the context, a middle section in which you present the data, and an ending that uses a call-to-action. Check our guide on presentation structure for further information.
- Visual Elements: These are the charts, graphs, and other elements of visual communication we ought to use to present data. This article will cover one by one the different types of data representation methods we can use, and provide further guidance on choosing between them.
- Insights and Analysis: This is not just showcasing a graph and letting people get an idea about it. A proper data presentation includes the interpretation of that data, the reason why it’s included, and why it matters to your research.
- Conclusion & CTA: Ending your presentation with a call to action is necessary. Whether you intend to wow your audience into acquiring your services, inspire them to change the world, or whatever the purpose of your presentation, there must be a stage in which you convey all that you shared and show the path to staying in touch. Plan ahead whether you want to use a thank-you slide, a video presentation, or which method is apt and tailored to the kind of presentation you deliver.
- Q&A Session: After your speech is concluded, allocate 3-5 minutes for the audience to raise any questions about the information you disclosed. This is an extra chance to establish your authority on the topic. Check our guide on questions and answer sessions in presentations here.
Bar charts are a graphical representation of data using rectangular bars to show quantities or frequencies in an established category. They make it easy for readers to spot patterns or trends. Bar charts can be horizontal or vertical, although the vertical format is commonly known as a column chart. They display categorical, discrete, or continuous variables grouped in class intervals [1] . They include an axis and a set of labeled bars horizontally or vertically. These bars represent the frequencies of variable values or the values themselves. Numbers on the y-axis of a vertical bar chart or the x-axis of a horizontal bar chart are called the scale.
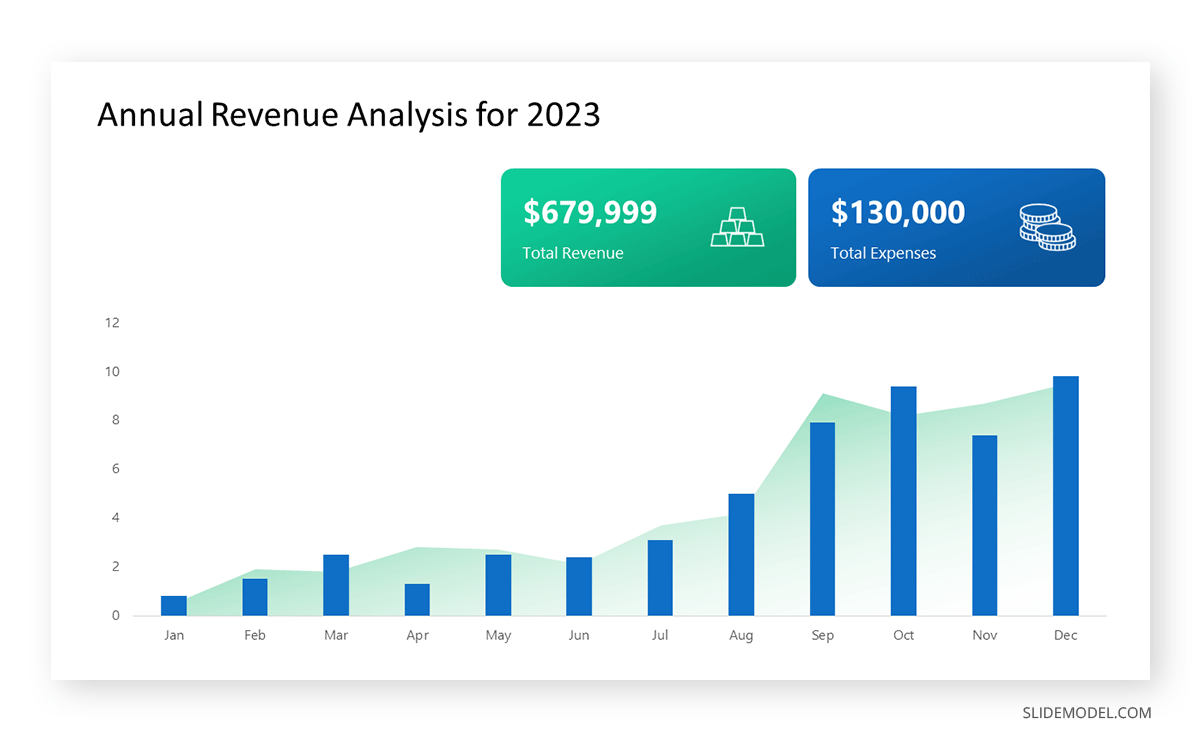
Real-Life Application of Bar Charts
Let’s say a sales manager is presenting sales to their audience. Using a bar chart, he follows these steps.
Step 1: Selecting Data
The first step is to identify the specific data you will present to your audience.
The sales manager has highlighted these products for the presentation.
- Product A: Men’s Shoes
- Product B: Women’s Apparel
- Product C: Electronics
- Product D: Home Decor
Step 2: Choosing Orientation
Opt for a vertical layout for simplicity. Vertical bar charts help compare different categories in case there are not too many categories [1] . They can also help show different trends. A vertical bar chart is used where each bar represents one of the four chosen products. After plotting the data, it is seen that the height of each bar directly represents the sales performance of the respective product.
It is visible that the tallest bar (Electronics – Product C) is showing the highest sales. However, the shorter bars (Women’s Apparel – Product B and Home Decor – Product D) need attention. It indicates areas that require further analysis or strategies for improvement.
Step 3: Colorful Insights
Different colors are used to differentiate each product. It is essential to show a color-coded chart where the audience can distinguish between products.
- Men’s Shoes (Product A): Yellow
- Women’s Apparel (Product B): Orange
- Electronics (Product C): Violet
- Home Decor (Product D): Blue
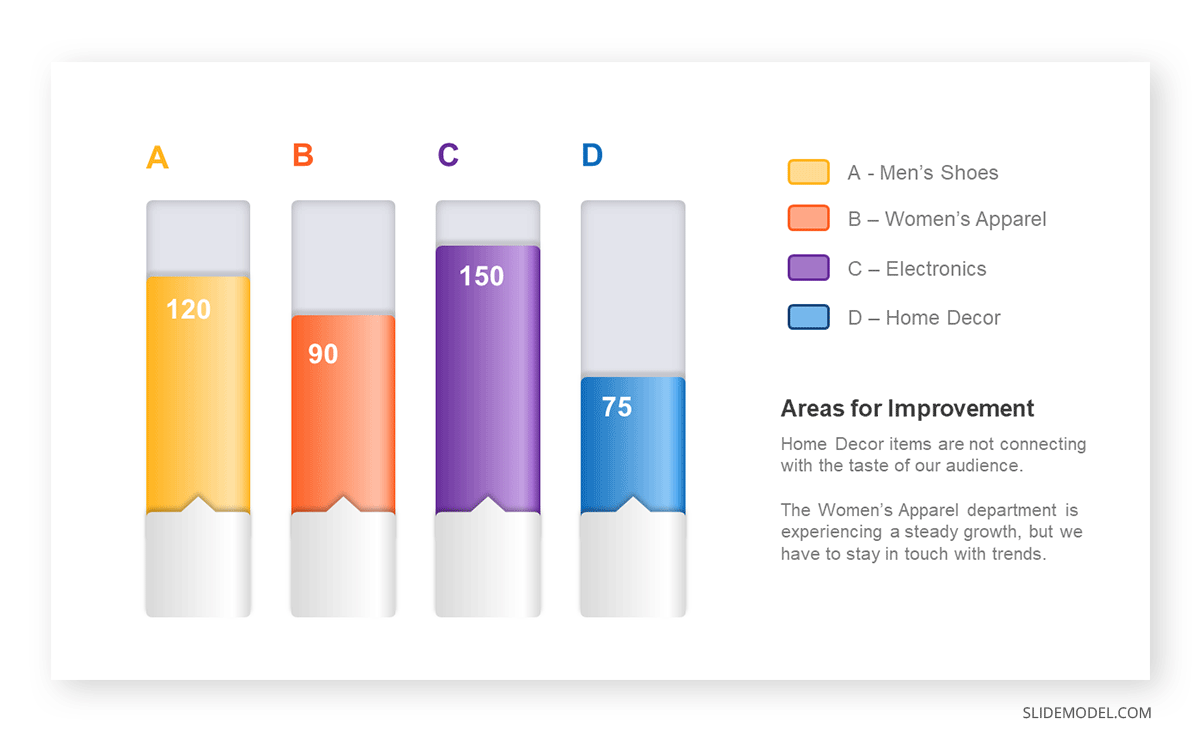
Bar charts are straightforward and easily understandable for presenting data. They are versatile when comparing products or any categorical data [2] . Bar charts adapt seamlessly to retail scenarios. Despite that, bar charts have a few shortcomings. They cannot illustrate data trends over time. Besides, overloading the chart with numerous products can lead to visual clutter, diminishing its effectiveness.
For more information, check our collection of bar chart templates for PowerPoint .
Line graphs help illustrate data trends, progressions, or fluctuations by connecting a series of data points called ‘markers’ with straight line segments. This provides a straightforward representation of how values change [5] . Their versatility makes them invaluable for scenarios requiring a visual understanding of continuous data. In addition, line graphs are also useful for comparing multiple datasets over the same timeline. Using multiple line graphs allows us to compare more than one data set. They simplify complex information so the audience can quickly grasp the ups and downs of values. From tracking stock prices to analyzing experimental results, you can use line graphs to show how data changes over a continuous timeline. They show trends with simplicity and clarity.
Real-life Application of Line Graphs
To understand line graphs thoroughly, we will use a real case. Imagine you’re a financial analyst presenting a tech company’s monthly sales for a licensed product over the past year. Investors want insights into sales behavior by month, how market trends may have influenced sales performance and reception to the new pricing strategy. To present data via a line graph, you will complete these steps.
First, you need to gather the data. In this case, your data will be the sales numbers. For example:
- January: $45,000
- February: $55,000
- March: $45,000
- April: $60,000
- May: $ 70,000
- June: $65,000
- July: $62,000
- August: $68,000
- September: $81,000
- October: $76,000
- November: $87,000
- December: $91,000
After choosing the data, the next step is to select the orientation. Like bar charts, you can use vertical or horizontal line graphs. However, we want to keep this simple, so we will keep the timeline (x-axis) horizontal while the sales numbers (y-axis) vertical.
Step 3: Connecting Trends
After adding the data to your preferred software, you will plot a line graph. In the graph, each month’s sales are represented by data points connected by a line.
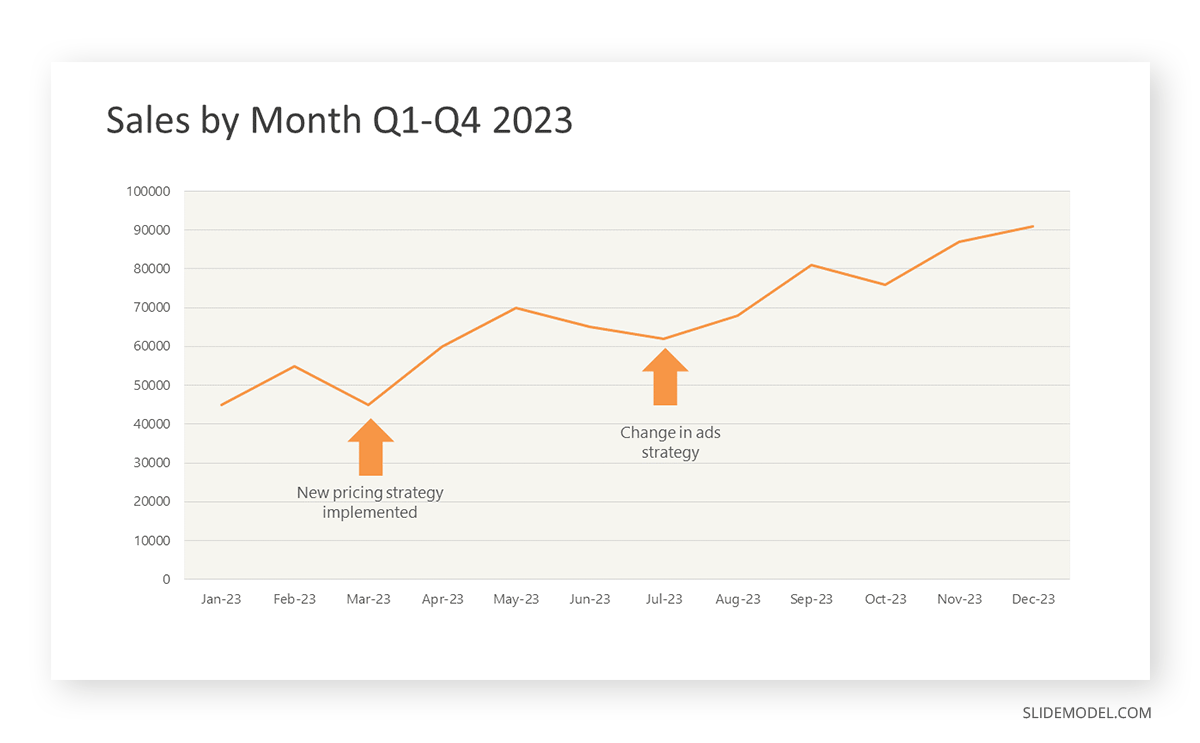
Step 4: Adding Clarity with Color
If there are multiple lines, you can also add colors to highlight each one, making it easier to follow.
Line graphs excel at visually presenting trends over time. These presentation aids identify patterns, like upward or downward trends. However, too many data points can clutter the graph, making it harder to interpret. Line graphs work best with continuous data but are not suitable for categories.
For more information, check our collection of line chart templates for PowerPoint and our article about how to make a presentation graph .
A data dashboard is a visual tool for analyzing information. Different graphs, charts, and tables are consolidated in a layout to showcase the information required to achieve one or more objectives. Dashboards help quickly see Key Performance Indicators (KPIs). You don’t make new visuals in the dashboard; instead, you use it to display visuals you’ve already made in worksheets [3] .
Keeping the number of visuals on a dashboard to three or four is recommended. Adding too many can make it hard to see the main points [4]. Dashboards can be used for business analytics to analyze sales, revenue, and marketing metrics at a time. They are also used in the manufacturing industry, as they allow users to grasp the entire production scenario at the moment while tracking the core KPIs for each line.
Real-Life Application of a Dashboard
Consider a project manager presenting a software development project’s progress to a tech company’s leadership team. He follows the following steps.
Step 1: Defining Key Metrics
To effectively communicate the project’s status, identify key metrics such as completion status, budget, and bug resolution rates. Then, choose measurable metrics aligned with project objectives.
Step 2: Choosing Visualization Widgets
After finalizing the data, presentation aids that align with each metric are selected. For this project, the project manager chooses a progress bar for the completion status and uses bar charts for budget allocation. Likewise, he implements line charts for bug resolution rates.
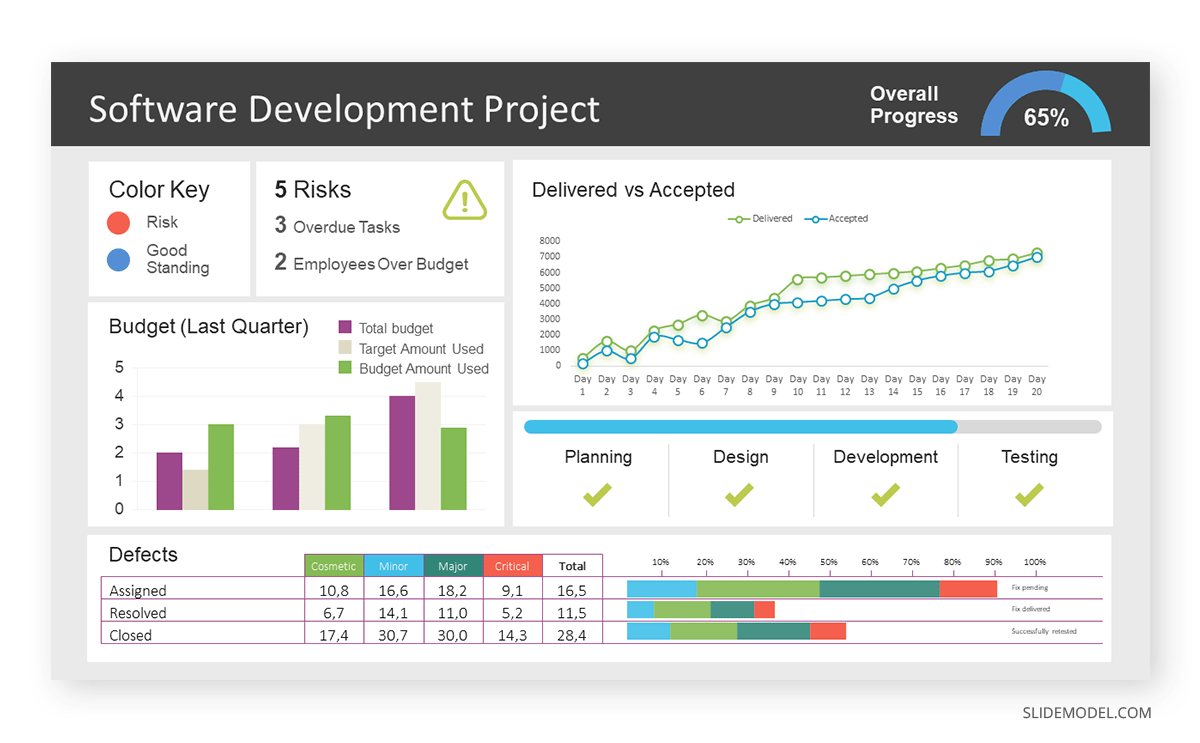
Step 3: Dashboard Layout
Key metrics are prominently placed in the dashboard for easy visibility, and the manager ensures that it appears clean and organized.
Dashboards provide a comprehensive view of key project metrics. Users can interact with data, customize views, and drill down for detailed analysis. However, creating an effective dashboard requires careful planning to avoid clutter. Besides, dashboards rely on the availability and accuracy of underlying data sources.
For more information, check our article on how to design a dashboard presentation , and discover our collection of dashboard PowerPoint templates .
Treemap charts represent hierarchical data structured in a series of nested rectangles [6] . As each branch of the ‘tree’ is given a rectangle, smaller tiles can be seen representing sub-branches, meaning elements on a lower hierarchical level than the parent rectangle. Each one of those rectangular nodes is built by representing an area proportional to the specified data dimension.
Treemaps are useful for visualizing large datasets in compact space. It is easy to identify patterns, such as which categories are dominant. Common applications of the treemap chart are seen in the IT industry, such as resource allocation, disk space management, website analytics, etc. Also, they can be used in multiple industries like healthcare data analysis, market share across different product categories, or even in finance to visualize portfolios.
Real-Life Application of a Treemap Chart
Let’s consider a financial scenario where a financial team wants to represent the budget allocation of a company. There is a hierarchy in the process, so it is helpful to use a treemap chart. In the chart, the top-level rectangle could represent the total budget, and it would be subdivided into smaller rectangles, each denoting a specific department. Further subdivisions within these smaller rectangles might represent individual projects or cost categories.
Step 1: Define Your Data Hierarchy
While presenting data on the budget allocation, start by outlining the hierarchical structure. The sequence will be like the overall budget at the top, followed by departments, projects within each department, and finally, individual cost categories for each project.
- Top-level rectangle: Total Budget
- Second-level rectangles: Departments (Engineering, Marketing, Sales)
- Third-level rectangles: Projects within each department
- Fourth-level rectangles: Cost categories for each project (Personnel, Marketing Expenses, Equipment)
Step 2: Choose a Suitable Tool
It’s time to select a data visualization tool supporting Treemaps. Popular choices include Tableau, Microsoft Power BI, PowerPoint, or even coding with libraries like D3.js. It is vital to ensure that the chosen tool provides customization options for colors, labels, and hierarchical structures.
Here, the team uses PowerPoint for this guide because of its user-friendly interface and robust Treemap capabilities.
Step 3: Make a Treemap Chart with PowerPoint
After opening the PowerPoint presentation, they chose “SmartArt” to form the chart. The SmartArt Graphic window has a “Hierarchy” category on the left. Here, you will see multiple options. You can choose any layout that resembles a Treemap. The “Table Hierarchy” or “Organization Chart” options can be adapted. The team selects the Table Hierarchy as it looks close to a Treemap.
Step 5: Input Your Data
After that, a new window will open with a basic structure. They add the data one by one by clicking on the text boxes. They start with the top-level rectangle, representing the total budget.
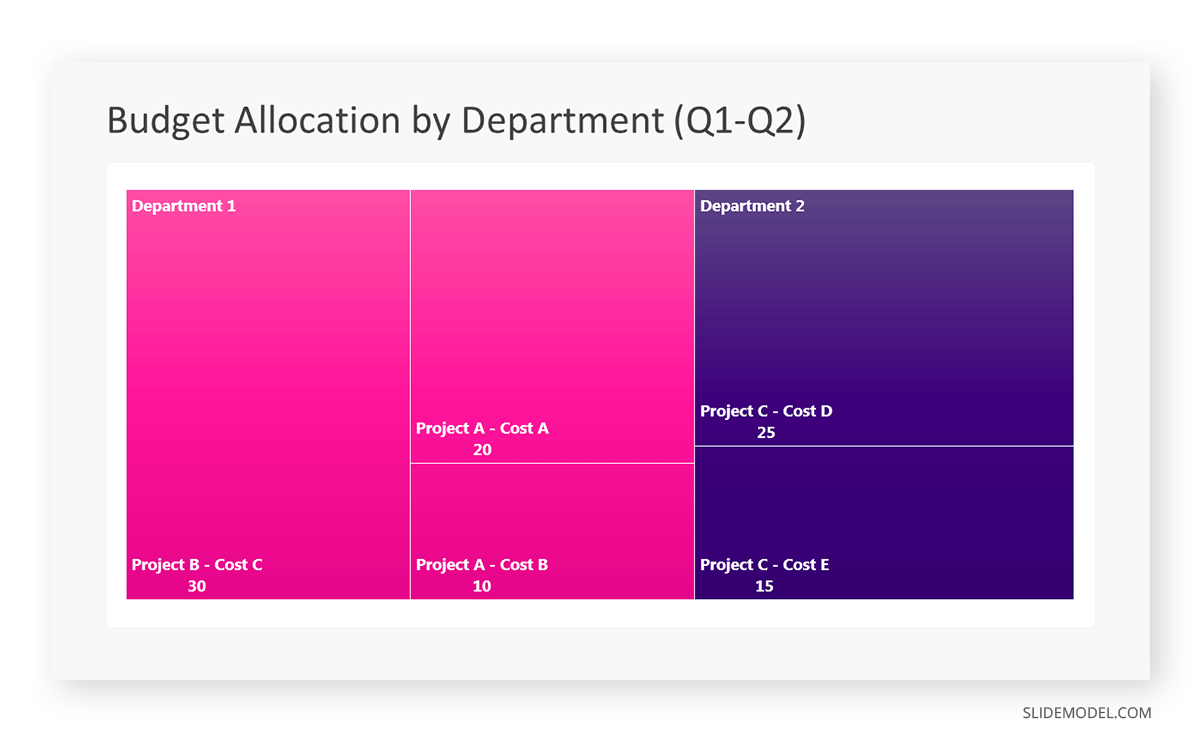
Step 6: Customize the Treemap
By clicking on each shape, they customize its color, size, and label. At the same time, they can adjust the font size, style, and color of labels by using the options in the “Format” tab in PowerPoint. Using different colors for each level enhances the visual difference.
Treemaps excel at illustrating hierarchical structures. These charts make it easy to understand relationships and dependencies. They efficiently use space, compactly displaying a large amount of data, reducing the need for excessive scrolling or navigation. Additionally, using colors enhances the understanding of data by representing different variables or categories.
In some cases, treemaps might become complex, especially with deep hierarchies. It becomes challenging for some users to interpret the chart. At the same time, displaying detailed information within each rectangle might be constrained by space. It potentially limits the amount of data that can be shown clearly. Without proper labeling and color coding, there’s a risk of misinterpretation.
A heatmap is a data visualization tool that uses color coding to represent values across a two-dimensional surface. In these, colors replace numbers to indicate the magnitude of each cell. This color-shaded matrix display is valuable for summarizing and understanding data sets with a glance [7] . The intensity of the color corresponds to the value it represents, making it easy to identify patterns, trends, and variations in the data.
As a tool, heatmaps help businesses analyze website interactions, revealing user behavior patterns and preferences to enhance overall user experience. In addition, companies use heatmaps to assess content engagement, identifying popular sections and areas of improvement for more effective communication. They excel at highlighting patterns and trends in large datasets, making it easy to identify areas of interest.
We can implement heatmaps to express multiple data types, such as numerical values, percentages, or even categorical data. Heatmaps help us easily spot areas with lots of activity, making them helpful in figuring out clusters [8] . When making these maps, it is important to pick colors carefully. The colors need to show the differences between groups or levels of something. And it is good to use colors that people with colorblindness can easily see.
Check our detailed guide on how to create a heatmap here. Also discover our collection of heatmap PowerPoint templates .
Pie charts are circular statistical graphics divided into slices to illustrate numerical proportions. Each slice represents a proportionate part of the whole, making it easy to visualize the contribution of each component to the total.
The size of the pie charts is influenced by the value of data points within each pie. The total of all data points in a pie determines its size. The pie with the highest data points appears as the largest, whereas the others are proportionally smaller. However, you can present all pies of the same size if proportional representation is not required [9] . Sometimes, pie charts are difficult to read, or additional information is required. A variation of this tool can be used instead, known as the donut chart , which has the same structure but a blank center, creating a ring shape. Presenters can add extra information, and the ring shape helps to declutter the graph.
Pie charts are used in business to show percentage distribution, compare relative sizes of categories, or present straightforward data sets where visualizing ratios is essential.
Real-Life Application of Pie Charts
Consider a scenario where you want to represent the distribution of the data. Each slice of the pie chart would represent a different category, and the size of each slice would indicate the percentage of the total portion allocated to that category.
Step 1: Define Your Data Structure
Imagine you are presenting the distribution of a project budget among different expense categories.
- Column A: Expense Categories (Personnel, Equipment, Marketing, Miscellaneous)
- Column B: Budget Amounts ($40,000, $30,000, $20,000, $10,000) Column B represents the values of your categories in Column A.
Step 2: Insert a Pie Chart
Using any of the accessible tools, you can create a pie chart. The most convenient tools for forming a pie chart in a presentation are presentation tools such as PowerPoint or Google Slides. You will notice that the pie chart assigns each expense category a percentage of the total budget by dividing it by the total budget.
For instance:
- Personnel: $40,000 / ($40,000 + $30,000 + $20,000 + $10,000) = 40%
- Equipment: $30,000 / ($40,000 + $30,000 + $20,000 + $10,000) = 30%
- Marketing: $20,000 / ($40,000 + $30,000 + $20,000 + $10,000) = 20%
- Miscellaneous: $10,000 / ($40,000 + $30,000 + $20,000 + $10,000) = 10%
You can make a chart out of this or just pull out the pie chart from the data.
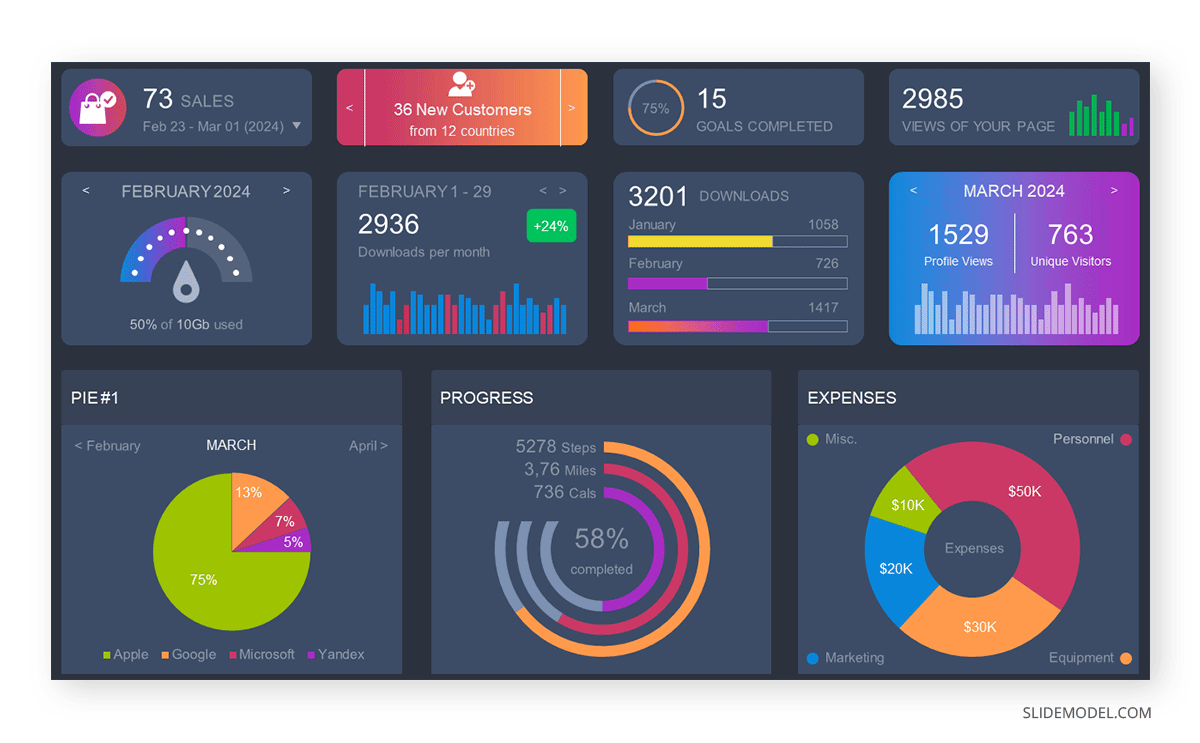
3D pie charts and 3D donut charts are quite popular among the audience. They stand out as visual elements in any presentation slide, so let’s take a look at how our pie chart example would look in 3D pie chart format.
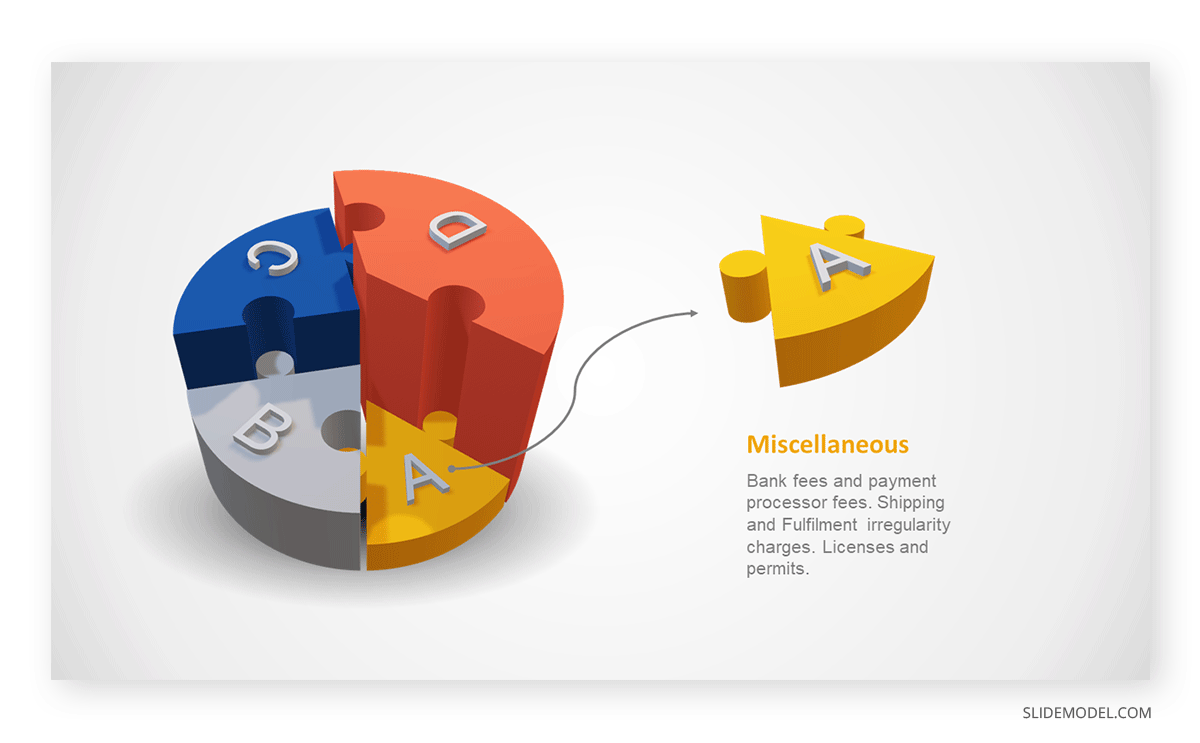
Step 03: Results Interpretation
The pie chart visually illustrates the distribution of the project budget among different expense categories. Personnel constitutes the largest portion at 40%, followed by equipment at 30%, marketing at 20%, and miscellaneous at 10%. This breakdown provides a clear overview of where the project funds are allocated, which helps in informed decision-making and resource management. It is evident that personnel are a significant investment, emphasizing their importance in the overall project budget.
Pie charts provide a straightforward way to represent proportions and percentages. They are easy to understand, even for individuals with limited data analysis experience. These charts work well for small datasets with a limited number of categories.
However, a pie chart can become cluttered and less effective in situations with many categories. Accurate interpretation may be challenging, especially when dealing with slight differences in slice sizes. In addition, these charts are static and do not effectively convey trends over time.
For more information, check our collection of pie chart templates for PowerPoint .
Histograms present the distribution of numerical variables. Unlike a bar chart that records each unique response separately, histograms organize numeric responses into bins and show the frequency of reactions within each bin [10] . The x-axis of a histogram shows the range of values for a numeric variable. At the same time, the y-axis indicates the relative frequencies (percentage of the total counts) for that range of values.
Whenever you want to understand the distribution of your data, check which values are more common, or identify outliers, histograms are your go-to. Think of them as a spotlight on the story your data is telling. A histogram can provide a quick and insightful overview if you’re curious about exam scores, sales figures, or any numerical data distribution.
Real-Life Application of a Histogram
In the histogram data analysis presentation example, imagine an instructor analyzing a class’s grades to identify the most common score range. A histogram could effectively display the distribution. It will show whether most students scored in the average range or if there are significant outliers.
Step 1: Gather Data
He begins by gathering the data. The scores of each student in class are gathered to analyze exam scores.
Names | Score |
---|---|
Alice | 78 |
Bob | 85 |
Clara | 92 |
David | 65 |
Emma | 72 |
Frank | 88 |
Grace | 76 |
Henry | 95 |
Isabel | 81 |
Jack | 70 |
Kate | 60 |
Liam | 89 |
Mia | 75 |
Noah | 84 |
Olivia | 92 |
After arranging the scores in ascending order, bin ranges are set.
Step 2: Define Bins
Bins are like categories that group similar values. Think of them as buckets that organize your data. The presenter decides how wide each bin should be based on the range of the values. For instance, the instructor sets the bin ranges based on score intervals: 60-69, 70-79, 80-89, and 90-100.
Step 3: Count Frequency
Now, he counts how many data points fall into each bin. This step is crucial because it tells you how often specific ranges of values occur. The result is the frequency distribution, showing the occurrences of each group.
Here, the instructor counts the number of students in each category.
- 60-69: 1 student (Kate)
- 70-79: 4 students (David, Emma, Grace, Jack)
- 80-89: 7 students (Alice, Bob, Frank, Isabel, Liam, Mia, Noah)
- 90-100: 3 students (Clara, Henry, Olivia)
Step 4: Create the Histogram
It’s time to turn the data into a visual representation. Draw a bar for each bin on a graph. The width of the bar should correspond to the range of the bin, and the height should correspond to the frequency. To make your histogram understandable, label the X and Y axes.
In this case, the X-axis should represent the bins (e.g., test score ranges), and the Y-axis represents the frequency.
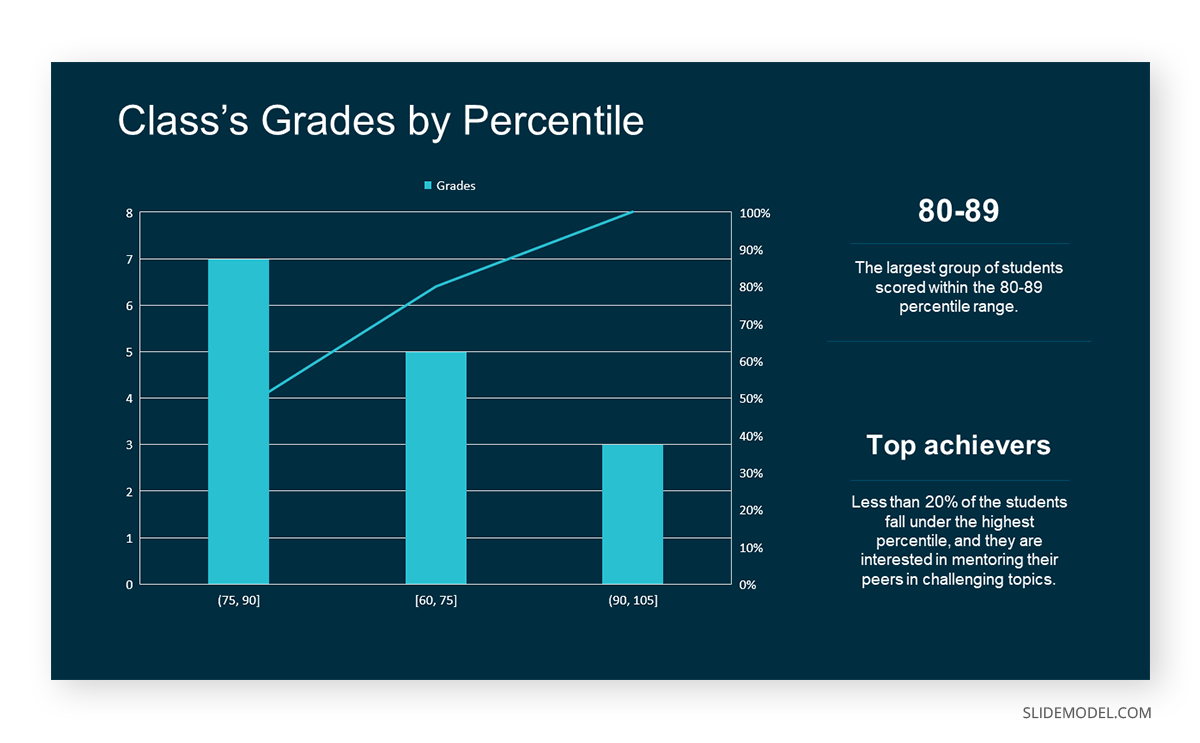
The histogram of the class grades reveals insightful patterns in the distribution. Most students, with seven students, fall within the 80-89 score range. The histogram provides a clear visualization of the class’s performance. It showcases a concentration of grades in the upper-middle range with few outliers at both ends. This analysis helps in understanding the overall academic standing of the class. It also identifies the areas for potential improvement or recognition.
Thus, histograms provide a clear visual representation of data distribution. They are easy to interpret, even for those without a statistical background. They apply to various types of data, including continuous and discrete variables. One weak point is that histograms do not capture detailed patterns in students’ data, with seven compared to other visualization methods.
A scatter plot is a graphical representation of the relationship between two variables. It consists of individual data points on a two-dimensional plane. This plane plots one variable on the x-axis and the other on the y-axis. Each point represents a unique observation. It visualizes patterns, trends, or correlations between the two variables.
Scatter plots are also effective in revealing the strength and direction of relationships. They identify outliers and assess the overall distribution of data points. The points’ dispersion and clustering reflect the relationship’s nature, whether it is positive, negative, or lacks a discernible pattern. In business, scatter plots assess relationships between variables such as marketing cost and sales revenue. They help present data correlations and decision-making.
Real-Life Application of Scatter Plot
A group of scientists is conducting a study on the relationship between daily hours of screen time and sleep quality. After reviewing the data, they managed to create this table to help them build a scatter plot graph:
Participant ID | Daily Hours of Screen Time | Sleep Quality Rating |
---|---|---|
1 | 9 | 3 |
2 | 2 | 8 |
3 | 1 | 9 |
4 | 0 | 10 |
5 | 1 | 9 |
6 | 3 | 7 |
7 | 4 | 7 |
8 | 5 | 6 |
9 | 5 | 6 |
10 | 7 | 3 |
11 | 10 | 1 |
12 | 6 | 5 |
13 | 7 | 3 |
14 | 8 | 2 |
15 | 9 | 2 |
16 | 4 | 7 |
17 | 5 | 6 |
18 | 4 | 7 |
19 | 9 | 2 |
20 | 6 | 4 |
21 | 3 | 7 |
22 | 10 | 1 |
23 | 2 | 8 |
24 | 5 | 6 |
25 | 3 | 7 |
26 | 1 | 9 |
27 | 8 | 2 |
28 | 4 | 6 |
29 | 7 | 3 |
30 | 2 | 8 |
31 | 7 | 4 |
32 | 9 | 2 |
33 | 10 | 1 |
34 | 10 | 1 |
35 | 10 | 1 |
In the provided example, the x-axis represents Daily Hours of Screen Time, and the y-axis represents the Sleep Quality Rating.
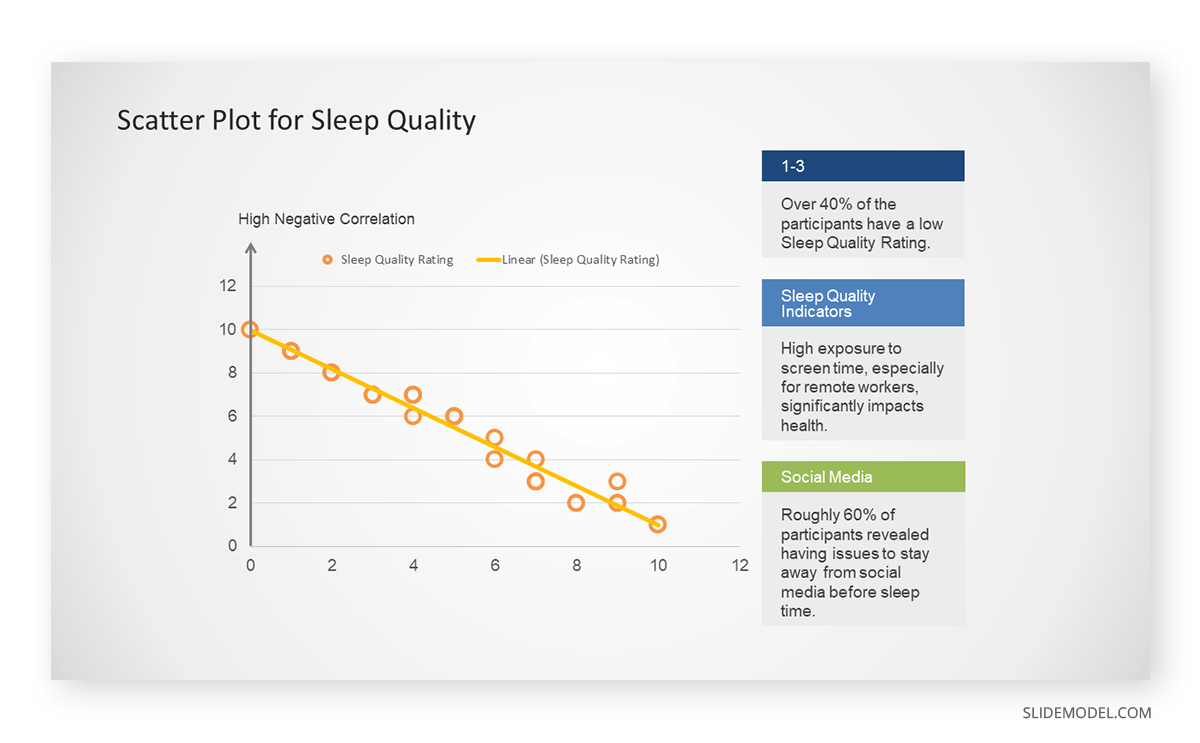
The scientists observe a negative correlation between the amount of screen time and the quality of sleep. This is consistent with their hypothesis that blue light, especially before bedtime, has a significant impact on sleep quality and metabolic processes.
There are a few things to remember when using a scatter plot. Even when a scatter diagram indicates a relationship, it doesn’t mean one variable affects the other. A third factor can influence both variables. The more the plot resembles a straight line, the stronger the relationship is perceived [11] . If it suggests no ties, the observed pattern might be due to random fluctuations in data. When the scatter diagram depicts no correlation, whether the data might be stratified is worth considering.
Choosing the appropriate data presentation type is crucial when making a presentation . Understanding the nature of your data and the message you intend to convey will guide this selection process. For instance, when showcasing quantitative relationships, scatter plots become instrumental in revealing correlations between variables. If the focus is on emphasizing parts of a whole, pie charts offer a concise display of proportions. Histograms, on the other hand, prove valuable for illustrating distributions and frequency patterns.
Bar charts provide a clear visual comparison of different categories. Likewise, line charts excel in showcasing trends over time, while tables are ideal for detailed data examination. Starting a presentation on data presentation types involves evaluating the specific information you want to communicate and selecting the format that aligns with your message. This ensures clarity and resonance with your audience from the beginning of your presentation.
1. Fact Sheet Dashboard for Data Presentation
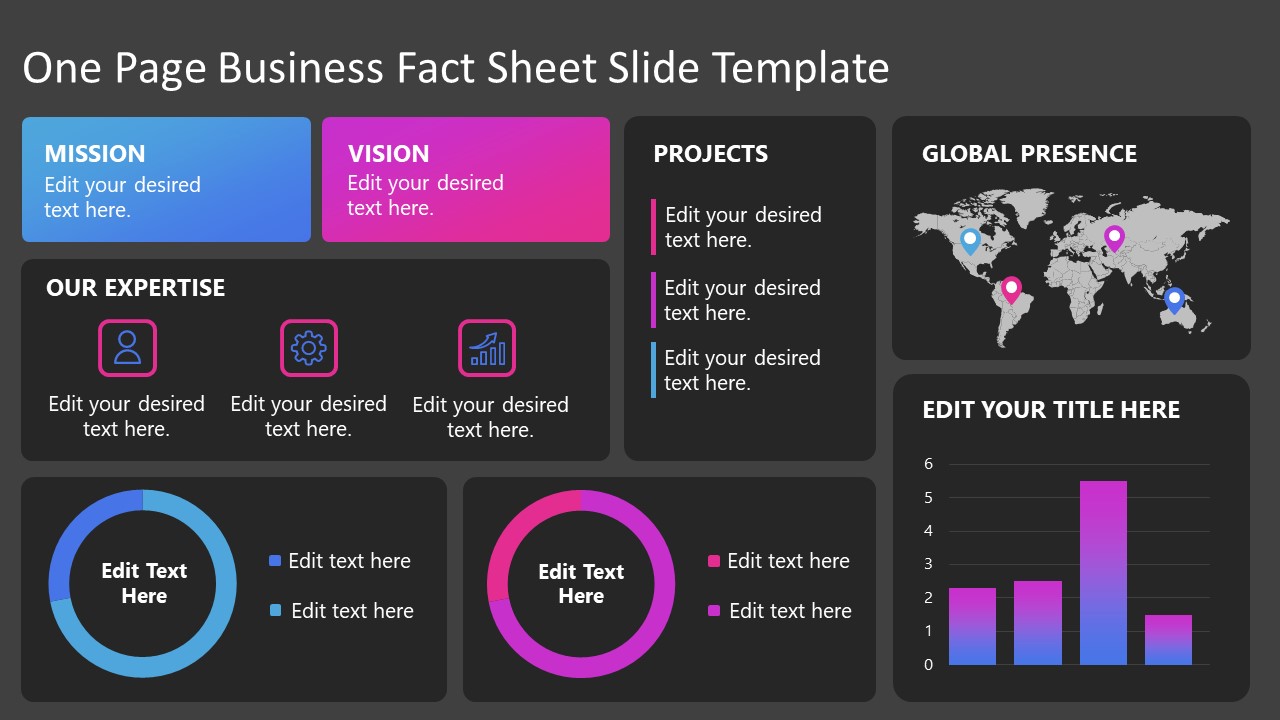
Convey all the data you need to present in this one-pager format, an ideal solution tailored for users looking for presentation aids. Global maps, donut chats, column graphs, and text neatly arranged in a clean layout presented in light and dark themes.
Use This Template
2. 3D Column Chart Infographic PPT Template
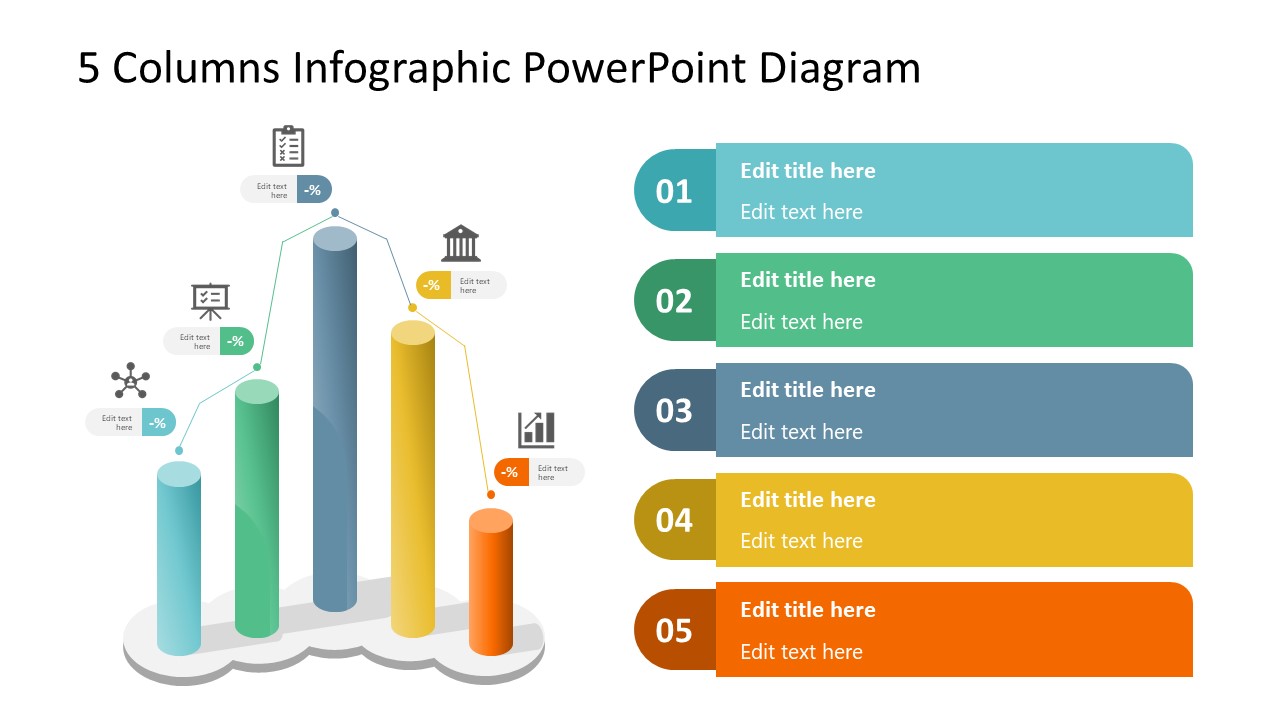
Represent column charts in a highly visual 3D format with this PPT template. A creative way to present data, this template is entirely editable, and we can craft either a one-page infographic or a series of slides explaining what we intend to disclose point by point.
3. Data Circles Infographic PowerPoint Template
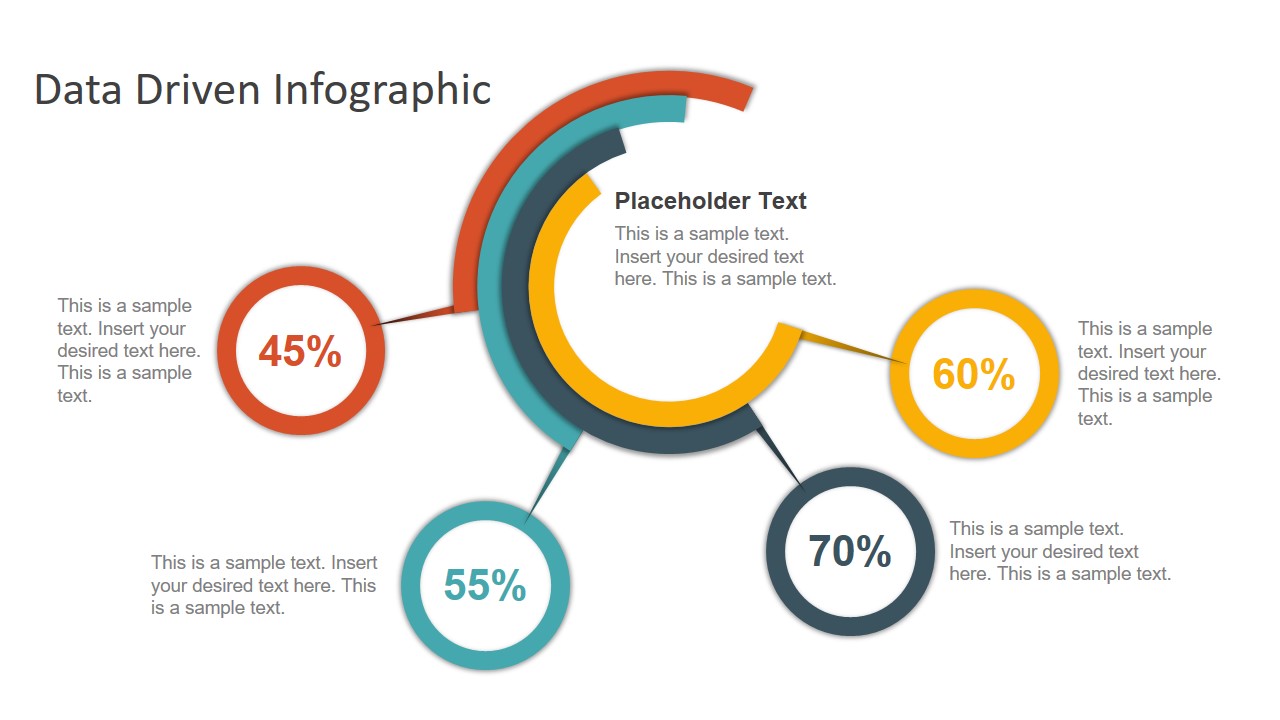
An alternative to the pie chart and donut chart diagrams, this template features a series of curved shapes with bubble callouts as ways of presenting data. Expand the information for each arch in the text placeholder areas.
4. Colorful Metrics Dashboard for Data Presentation
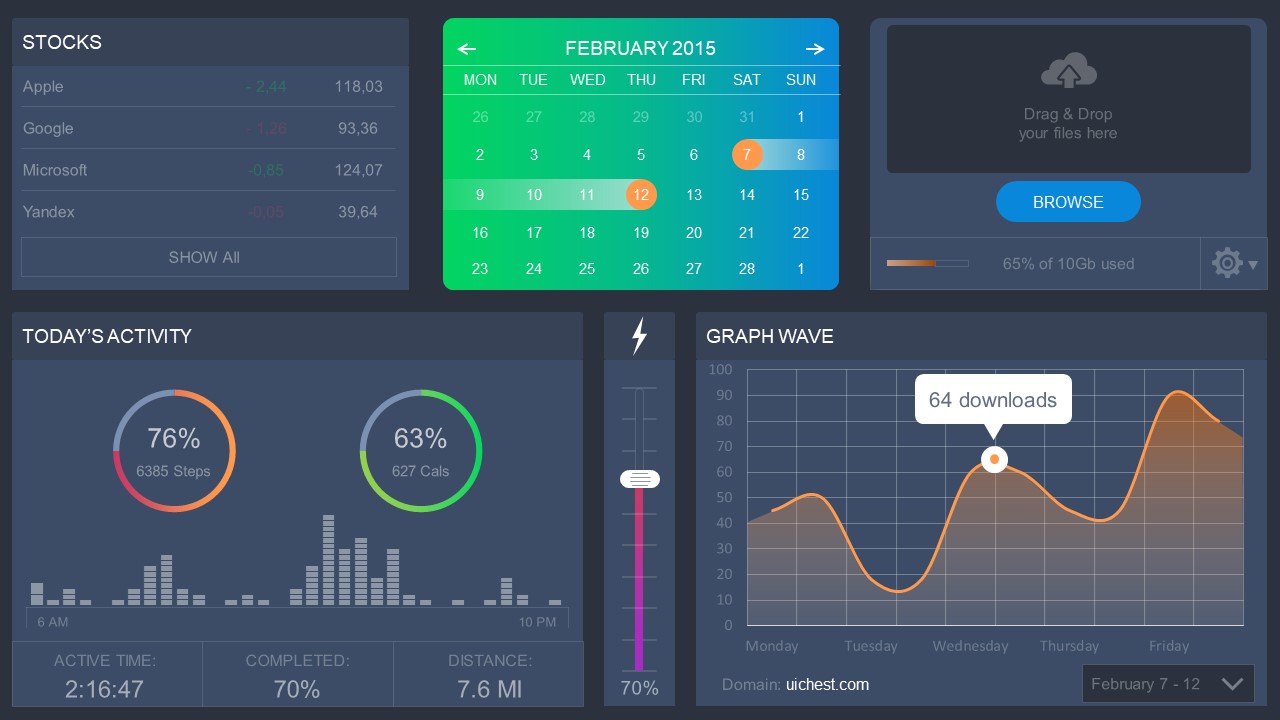
This versatile dashboard template helps us in the presentation of the data by offering several graphs and methods to convert numbers into graphics. Implement it for e-commerce projects, financial projections, project development, and more.
5. Animated Data Presentation Tools for PowerPoint & Google Slides
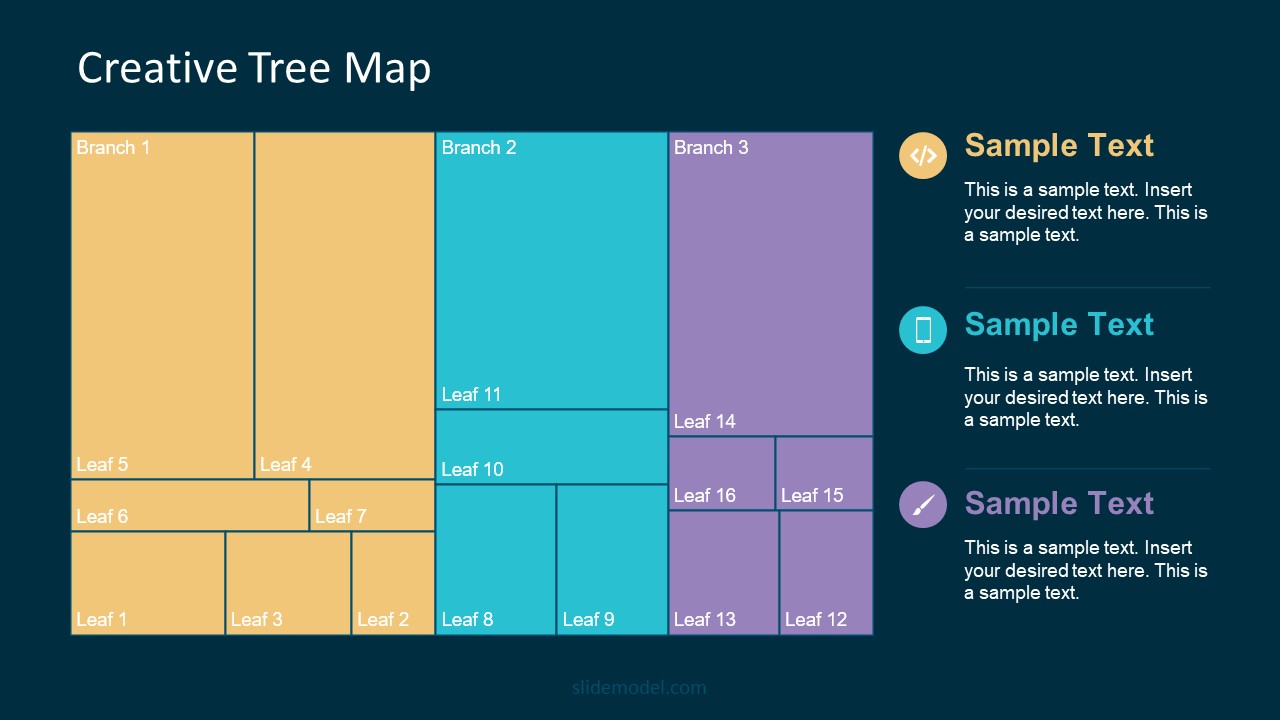
A slide deck filled with most of the tools mentioned in this article, from bar charts, column charts, treemap graphs, pie charts, histogram, etc. Animated effects make each slide look dynamic when sharing data with stakeholders.
6. Statistics Waffle Charts PPT Template for Data Presentations
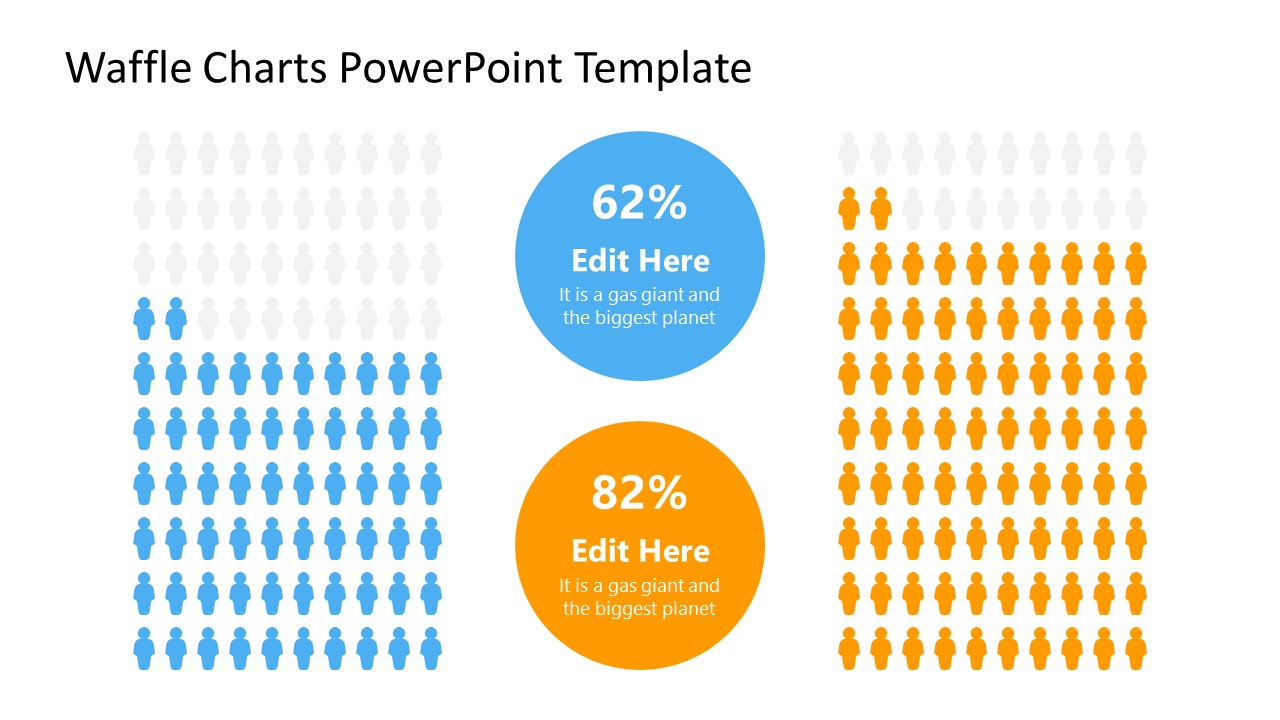
This PPT template helps us how to present data beyond the typical pie chart representation. It is widely used for demographics, so it’s a great fit for marketing teams, data science professionals, HR personnel, and more.
7. Data Presentation Dashboard Template for Google Slides
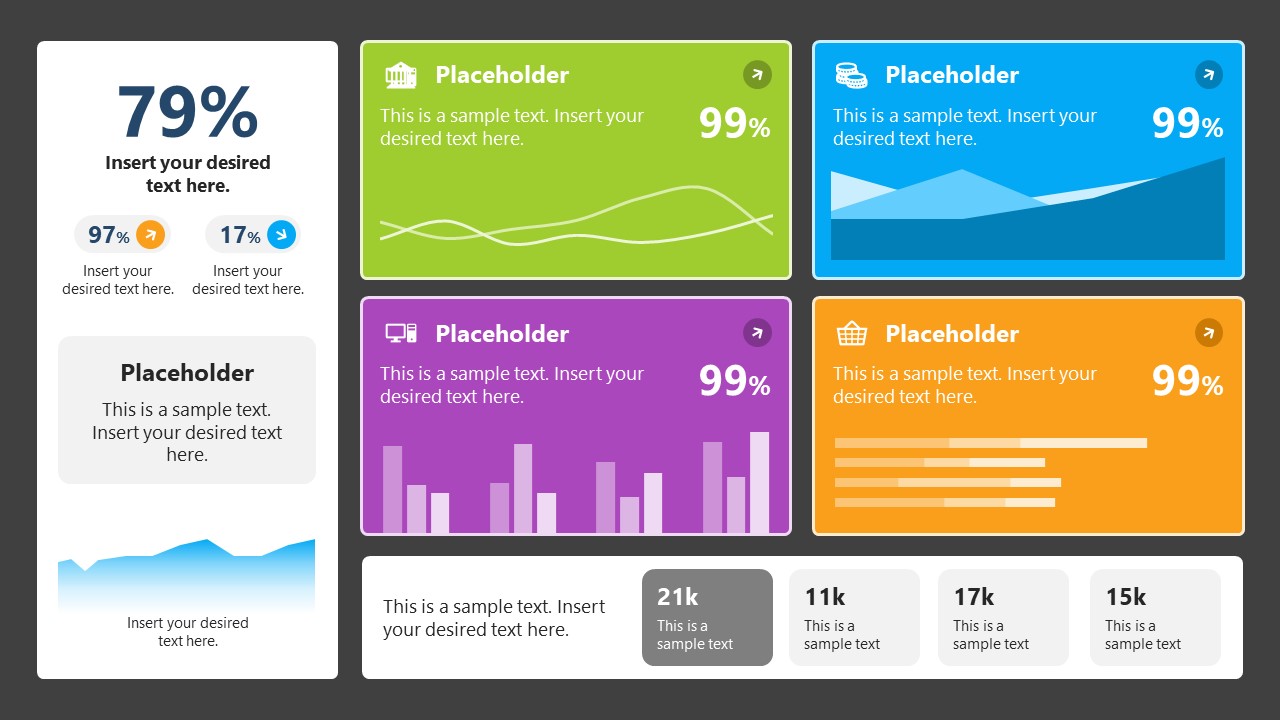
A compendium of tools in dashboard format featuring line graphs, bar charts, column charts, and neatly arranged placeholder text areas.
8. Weather Dashboard for Data Presentation
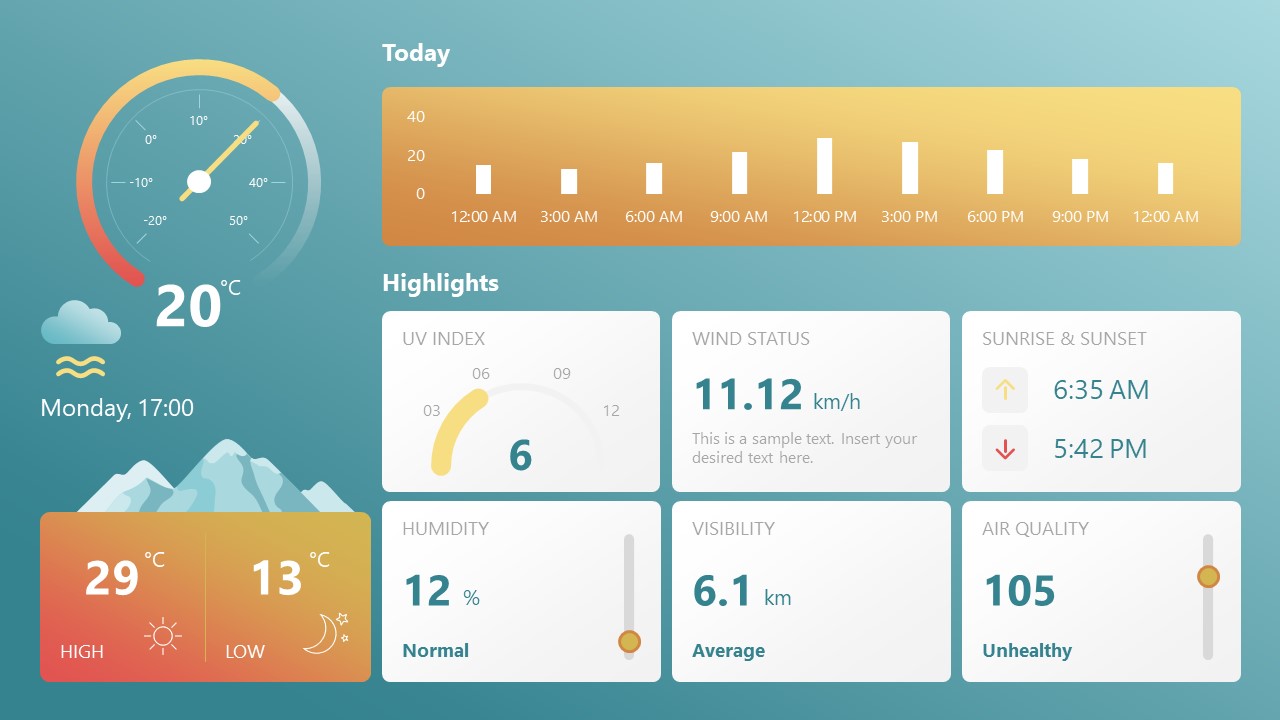
Share weather data for agricultural presentation topics, environmental studies, or any kind of presentation that requires a highly visual layout for weather forecasting on a single day. Two color themes are available.
9. Social Media Marketing Dashboard Data Presentation Template
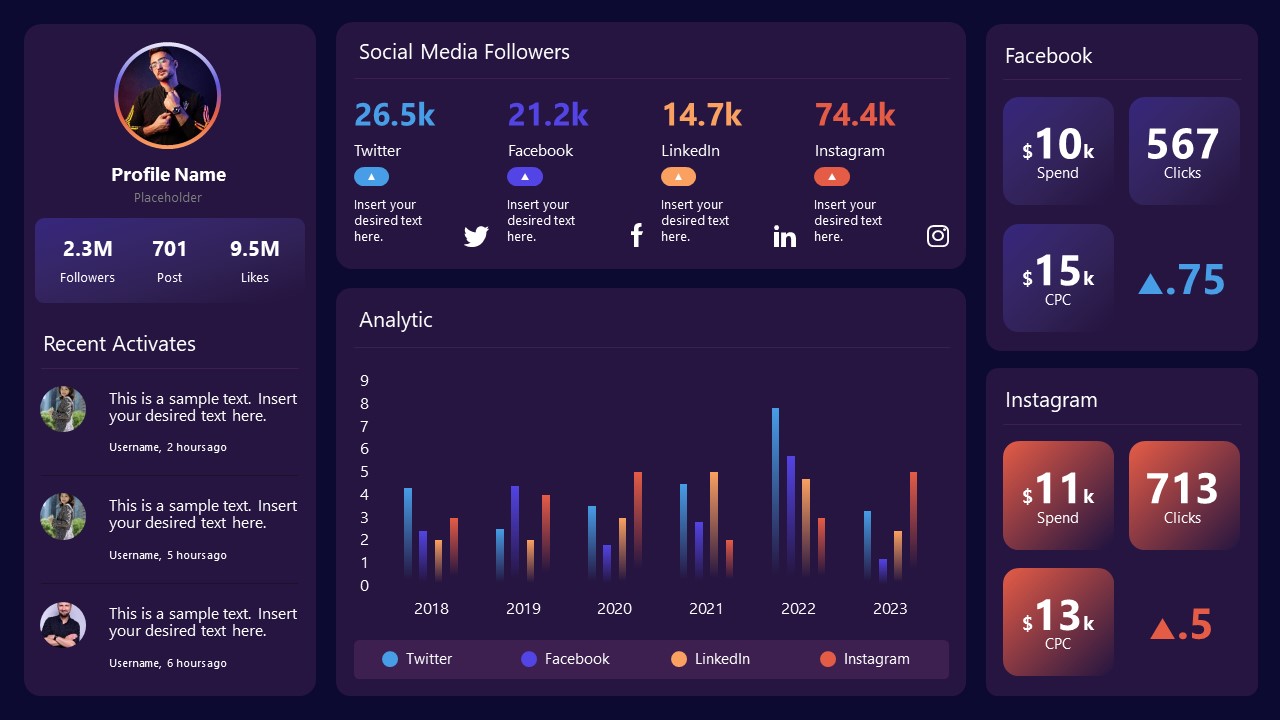
Intended for marketing professionals, this dashboard template for data presentation is a tool for presenting data analytics from social media channels. Two slide layouts featuring line graphs and column charts.
10. Project Management Summary Dashboard Template
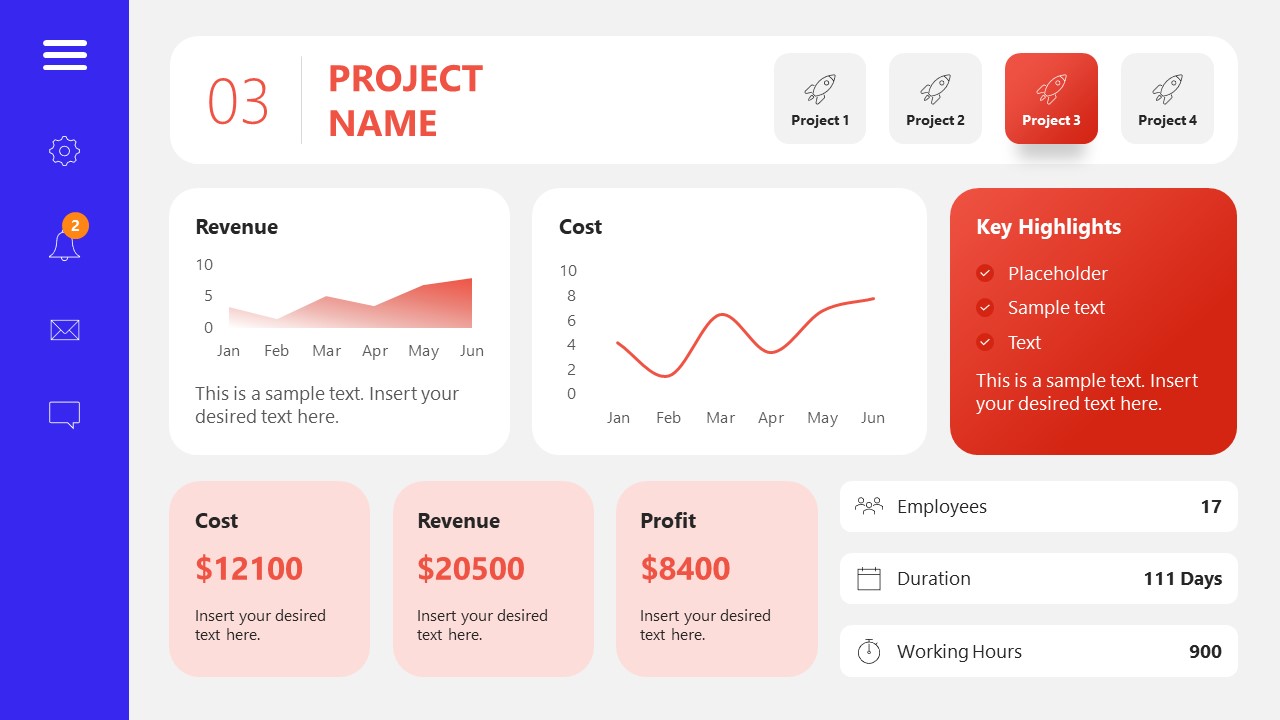
A tool crafted for project managers to deliver highly visual reports on a project’s completion, the profits it delivered for the company, and expenses/time required to execute it. 4 different color layouts are available.
11. Profit & Loss Dashboard for PowerPoint and Google Slides
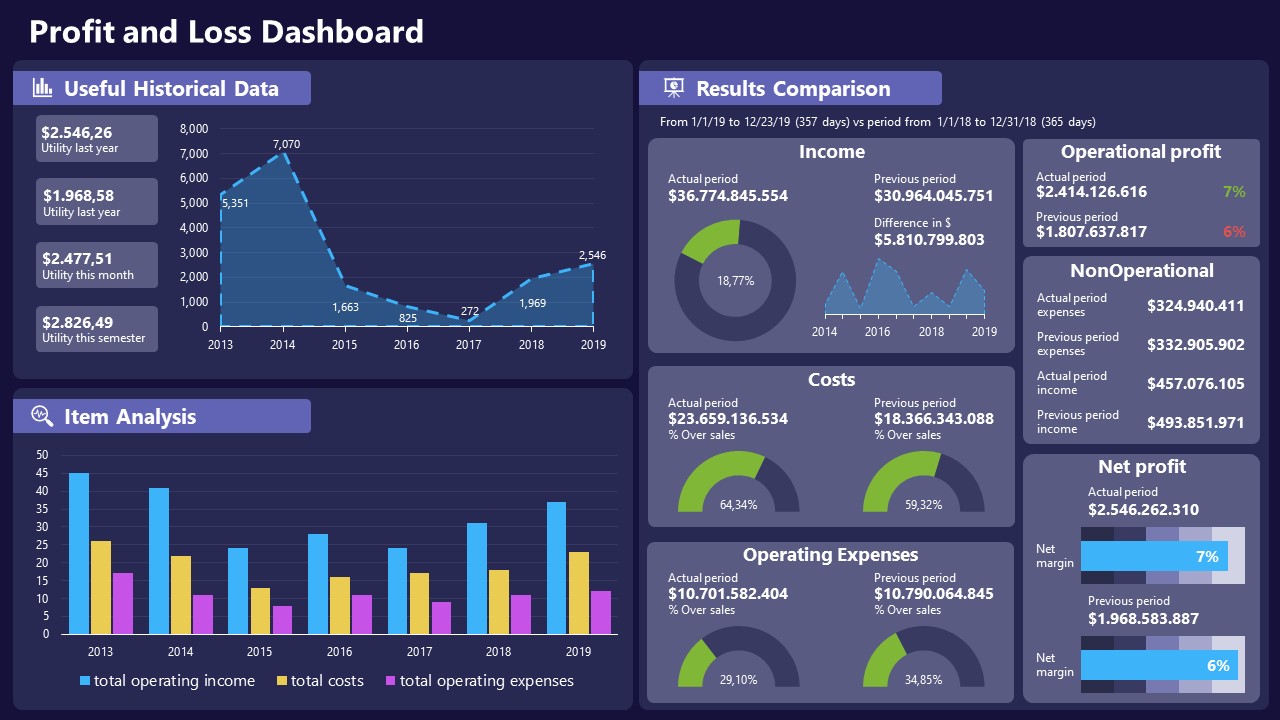
A must-have for finance professionals. This typical profit & loss dashboard includes progress bars, donut charts, column charts, line graphs, and everything that’s required to deliver a comprehensive report about a company’s financial situation.
Overwhelming visuals
One of the mistakes related to using data-presenting methods is including too much data or using overly complex visualizations. They can confuse the audience and dilute the key message.
Inappropriate chart types
Choosing the wrong type of chart for the data at hand can lead to misinterpretation. For example, using a pie chart for data that doesn’t represent parts of a whole is not right.
Lack of context
Failing to provide context or sufficient labeling can make it challenging for the audience to understand the significance of the presented data.
Inconsistency in design
Using inconsistent design elements and color schemes across different visualizations can create confusion and visual disarray.
Failure to provide details
Simply presenting raw data without offering clear insights or takeaways can leave the audience without a meaningful conclusion.
Lack of focus
Not having a clear focus on the key message or main takeaway can result in a presentation that lacks a central theme.
Visual accessibility issues
Overlooking the visual accessibility of charts and graphs can exclude certain audience members who may have difficulty interpreting visual information.
In order to avoid these mistakes in data presentation, presenters can benefit from using presentation templates . These templates provide a structured framework. They ensure consistency, clarity, and an aesthetically pleasing design, enhancing data communication’s overall impact.
Understanding and choosing data presentation types are pivotal in effective communication. Each method serves a unique purpose, so selecting the appropriate one depends on the nature of the data and the message to be conveyed. The diverse array of presentation types offers versatility in visually representing information, from bar charts showing values to pie charts illustrating proportions.
Using the proper method enhances clarity, engages the audience, and ensures that data sets are not just presented but comprehensively understood. By appreciating the strengths and limitations of different presentation types, communicators can tailor their approach to convey information accurately, developing a deeper connection between data and audience understanding.
[1] Government of Canada, S.C. (2021) 5 Data Visualization 5.2 Bar Chart , 5.2 Bar chart . https://www150.statcan.gc.ca/n1/edu/power-pouvoir/ch9/bargraph-diagrammeabarres/5214818-eng.htm
[2] Kosslyn, S.M., 1989. Understanding charts and graphs. Applied cognitive psychology, 3(3), pp.185-225. https://apps.dtic.mil/sti/pdfs/ADA183409.pdf
[3] Creating a Dashboard . https://it.tufts.edu/book/export/html/1870
[4] https://www.goldenwestcollege.edu/research/data-and-more/data-dashboards/index.html
[5] https://www.mit.edu/course/21/21.guide/grf-line.htm
[6] Jadeja, M. and Shah, K., 2015, January. Tree-Map: A Visualization Tool for Large Data. In GSB@ SIGIR (pp. 9-13). https://ceur-ws.org/Vol-1393/gsb15proceedings.pdf#page=15
[7] Heat Maps and Quilt Plots. https://www.publichealth.columbia.edu/research/population-health-methods/heat-maps-and-quilt-plots
[8] EIU QGIS WORKSHOP. https://www.eiu.edu/qgisworkshop/heatmaps.php
[9] About Pie Charts. https://www.mit.edu/~mbarker/formula1/f1help/11-ch-c8.htm
[10] Histograms. https://sites.utexas.edu/sos/guided/descriptive/numericaldd/descriptiven2/histogram/ [11] https://asq.org/quality-resources/scatter-diagram
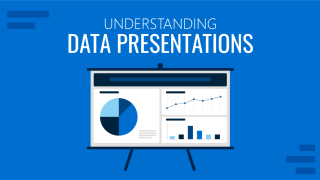
Like this article? Please share
Data Analysis, Data Science, Data Visualization Filed under Design
Related Articles
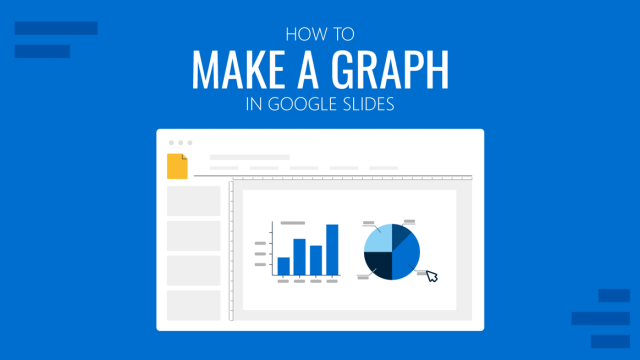
Filed under Google Slides Tutorials • June 3rd, 2024
How To Make a Graph on Google Slides
Creating quality graphics is an essential aspect of designing data presentations. Learn how to make a graph in Google Slides with this guide.
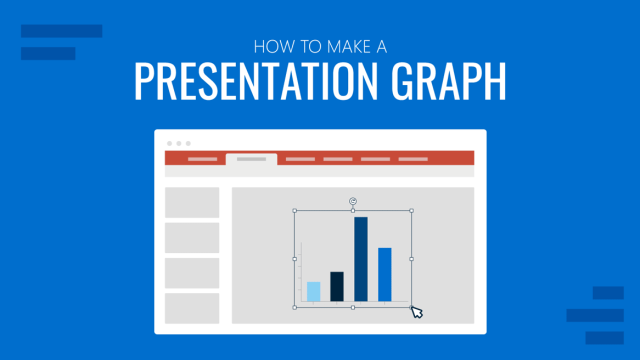
Filed under Design • March 27th, 2024
How to Make a Presentation Graph
Detailed step-by-step instructions to master the art of how to make a presentation graph in PowerPoint and Google Slides. Check it out!
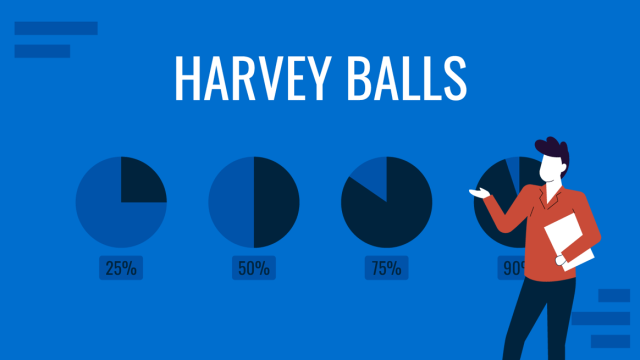
Filed under Presentation Ideas • January 6th, 2024
All About Using Harvey Balls
Among the many tools in the arsenal of the modern presenter, Harvey Balls have a special place. In this article we will tell you all about using Harvey Balls.
Leave a Reply
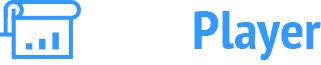
- My presentations
Auth with social network:
Download presentation
We think you have liked this presentation. If you wish to download it, please recommend it to your friends in any social system. Share buttons are a little bit lower. Thank you!
Presentation is loading. Please wait.
To view this video please enable JavaScript, and consider upgrading to a web browser that supports HTML5 video
Gathering Weather Data
Published by Lynne Goodwin Modified over 8 years ago
Similar presentations
Presentation on theme: "Gathering Weather Data"— Presentation transcript:

WEATHER INSTRUMENTS.
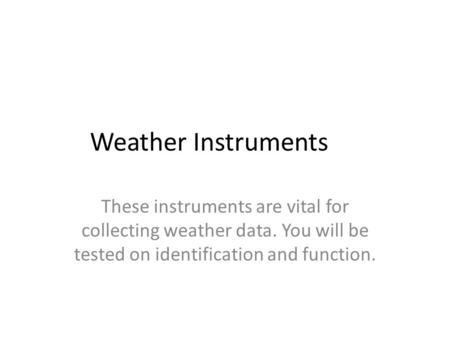
Weather Instruments These instruments are vital for collecting weather data. You will be tested on identification and function.
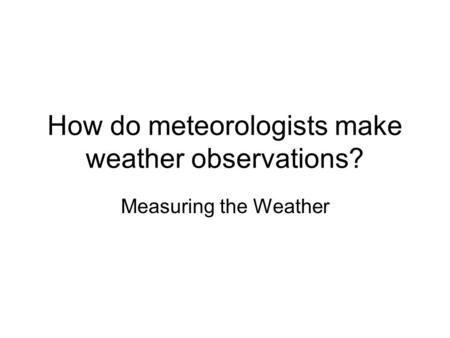
How do meteorologists make weather observations? Measuring the Weather.
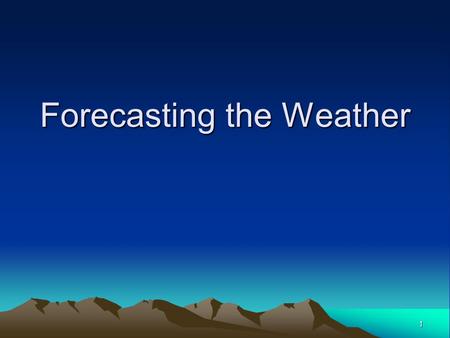
1 Forecasting the Weather. Weather Weather: The daily conditions of a particular area: temp, rain, pressure, wind, etc.Weather: The daily conditions of.

Weather Data Tools. Thermometer ► Measures air temperature.
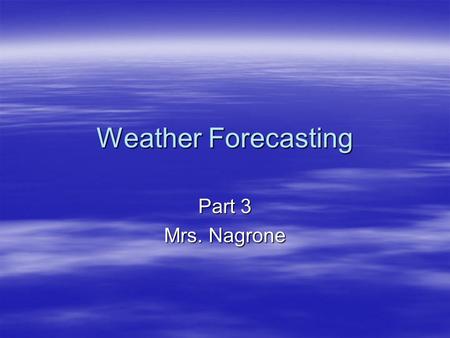
Weather Forecasting Part 3 Mrs. Nagrone. Objectives You will have the understanding of how meteorologists make station models and surface maps. You.
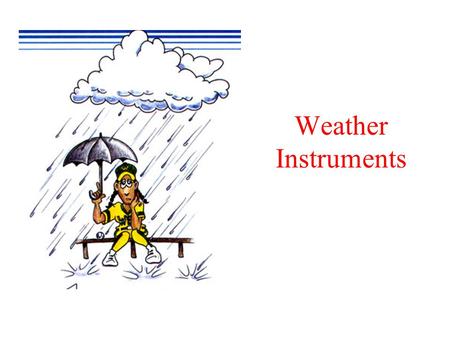
Weather Instruments.
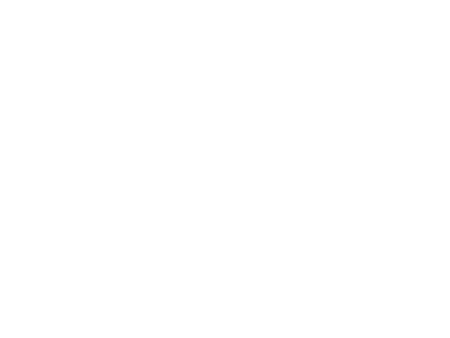
Thermometers Thermometers measure air temperature. Thermometers work because matter expands when heated. Most thermometers are closed glass tubes containing.
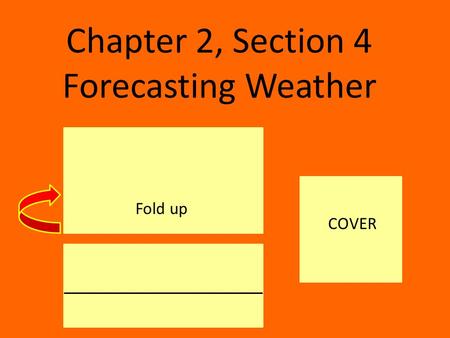
Chapter 2, Section 4 Forecasting Weather COVER Fold up.
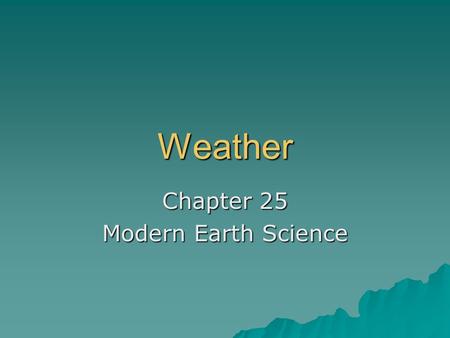
Chapter 25 Modern Earth Science
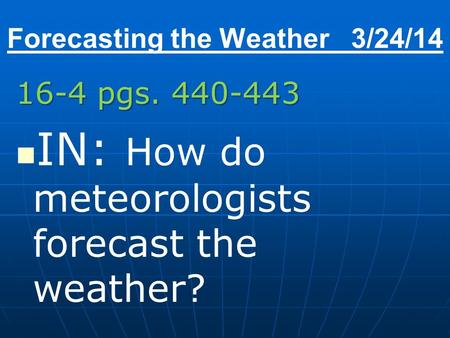
Forecasting the Weather 3/24/14
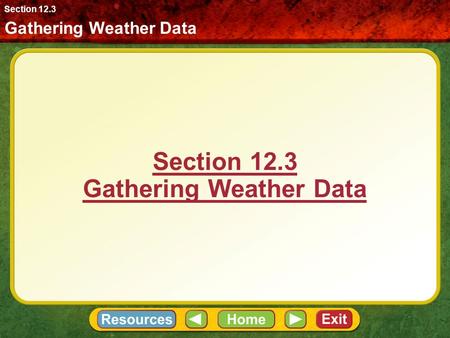
Section 12.3 Gathering Weather Data
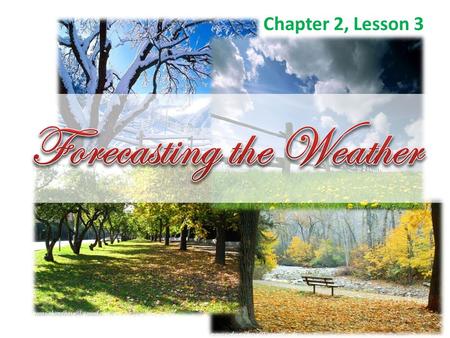
Chapter 2, Lesson 3. A weather forecast is a prediction of weather conditions over the next 3 to 5 days. A meteorologist is a person who observes.
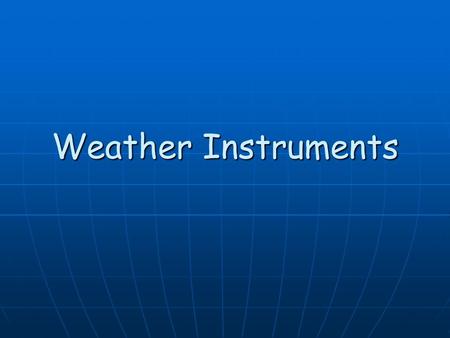
Weather Instruments. Thermometer What does it do? What does it do? It measures the air temperature It measures the air temperature.
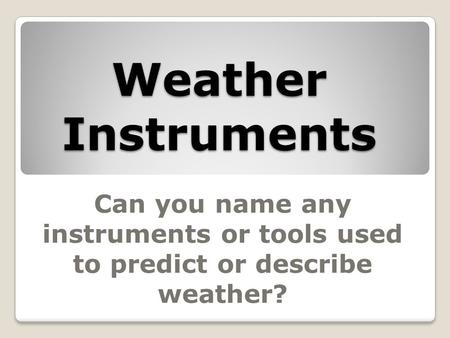
Weather Instruments Can you name any instruments or tools used to predict or describe weather?
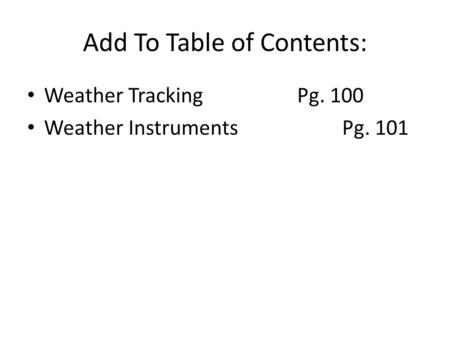
Add To Table of Contents:
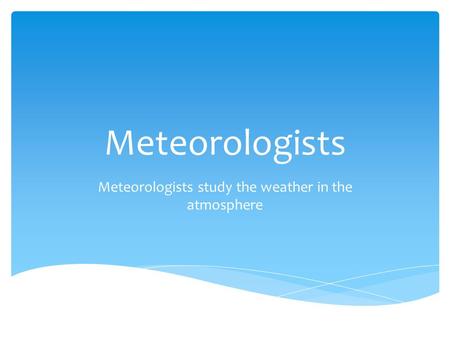
Meteorologists Meteorologists study the weather in the atmosphere.
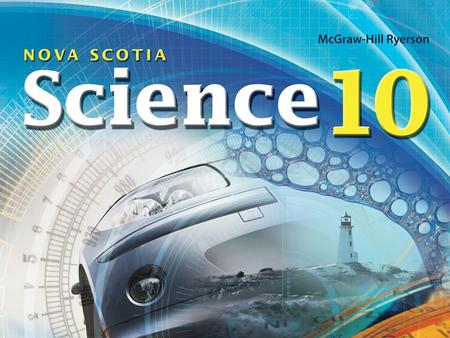
UNIT 1: Weather Dynamics Chapter 1: Inquiring about Weather Chapter 2: Weather Forecasting 2.1 – Measuring Weather Data.
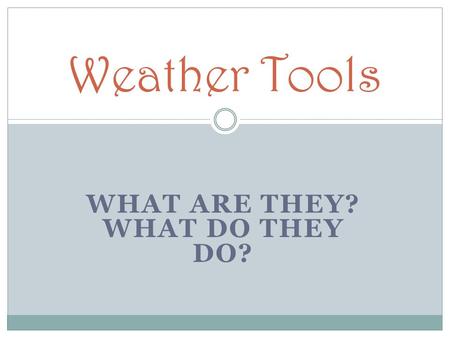
What are they? What do they do?
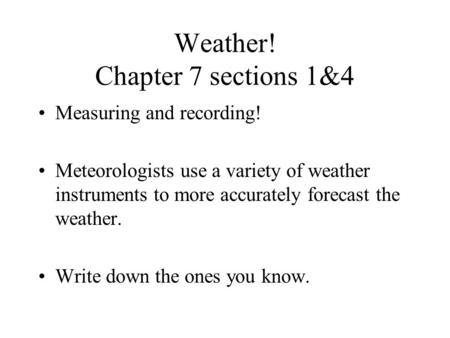
Weather! Chapter 7 sections 1&4 Measuring and recording! Meteorologists use a variety of weather instruments to more accurately forecast the weather.
About project
© 2024 SlidePlayer.com Inc. All rights reserved.
Climate Matters • November 25, 2020
New Presentation: Our Changing Climate
Key concepts:.
Climate Central unveils Our Changing Climate —an informative and customizable climate change presentation that meteorologists, journalists, and others can use for educational outreach and/or a personal Climate 101 tool.
The presentation follows a ”Simple, Serious, Solvable” framework, inspired by climate scientist Scott Denning. This allows the presenter to comfortably explain, and the viewers to easily understand, the causes (Simple), impacts (Serious), and solutions (Solvable) of climate change.
Our Changing Climate is a revamped version of our 2016 climate presentation, and includes the following updates and features:
Up-to-date graphics and topics
Local data and graphics
Fully editable slides (add, remove, customize)
Presenter notes, background information, and references for each slide
Supplementary and bonus slides
Download Outline (PDF, 110KB)
Download Full Presentation (PPT, 148MB)
Updated: April 2021
Climate Central is presenting a new outreach and education resource for meteorologists, journalists, and others—a climate change presentation, Our Changing Climate . This 55-slide presentation is a guide through the basics of climate change, outlining its causes, impacts, and solutions. This climate change overview is unique because it includes an array of local graphics from our ever-expanding media library. By providing these local angles, the presenter can demonstrate that climate change is not only happening at a global-scale, but in our backyards.
This presentation was designed to support your climate change storytelling, but can also double as a great Climate 101 tool for journalists or educators who want to understand climate change better. Every slide contains main points along with background information, so people that are interested can learn at their own pace or utilize graphics for their own content.
In addition to those features, it follows the “Simple, Serious, Solvable” framework inspired by Scott Denning, a climate scientist and professor of atmospheric science at Colorado State University (and a good friend of the program). These three S’s help create the presentation storyline and outline the causes (Simple), impacts (Serious), and solutions (Solvable) of climate change.
Simple. It is simple—burning fossil fuels is heating up the Earth. This section outlines the well-understood science that goes back to the 1800s, presenting local and global evidence that our climate is warming due to human activities.
Serious. More extreme weather, rising sea levels, and increased health and economic risks—the consequences of climate change. In this section, well, we get serious. Climate change impacts are already being felt around the world, and they will continue to intensify until we cut greenhouse gas emissions.
Solvable. With such a daunting crisis like climate change, it is easy to get wrapped up in the negative impacts. This section explains how we can curb climate change and lists the main pathways and solutions to achieving this goal.
With the rollout of our new climate change presentation, we at Climate Central would value any feedback on this presentation. Feel free to reach out to us about how the presentation worked for you, how your audience reacted, or any ideas or topics you would like to see included.
ACKNOWLEDGMENTS & SPECIAL THANKS
Climate Central would like to acknowledge Paul Gross at WDIV-TV in Detroit and the AMS Station Science Committee for the original version of the climate presentation, Climate Change Outreach Presentation , that was created in 2016. We would also like to give special thanks to Scott Denning, professor of atmospheric science at Colorado State University and a member of our NSF advisory board, for allowing us to use this “Simple, Serious, Solvable” framework in this presentation resource.
SUPPORTING MULTIMEDIA
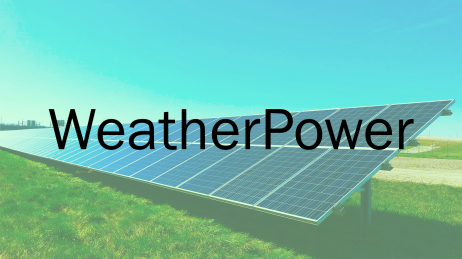
Got any suggestions?
We want to hear from you! Send us a message and help improve Slidesgo
Top searches
Trending searches

education technology
252 templates

meet the teacher
30 templates
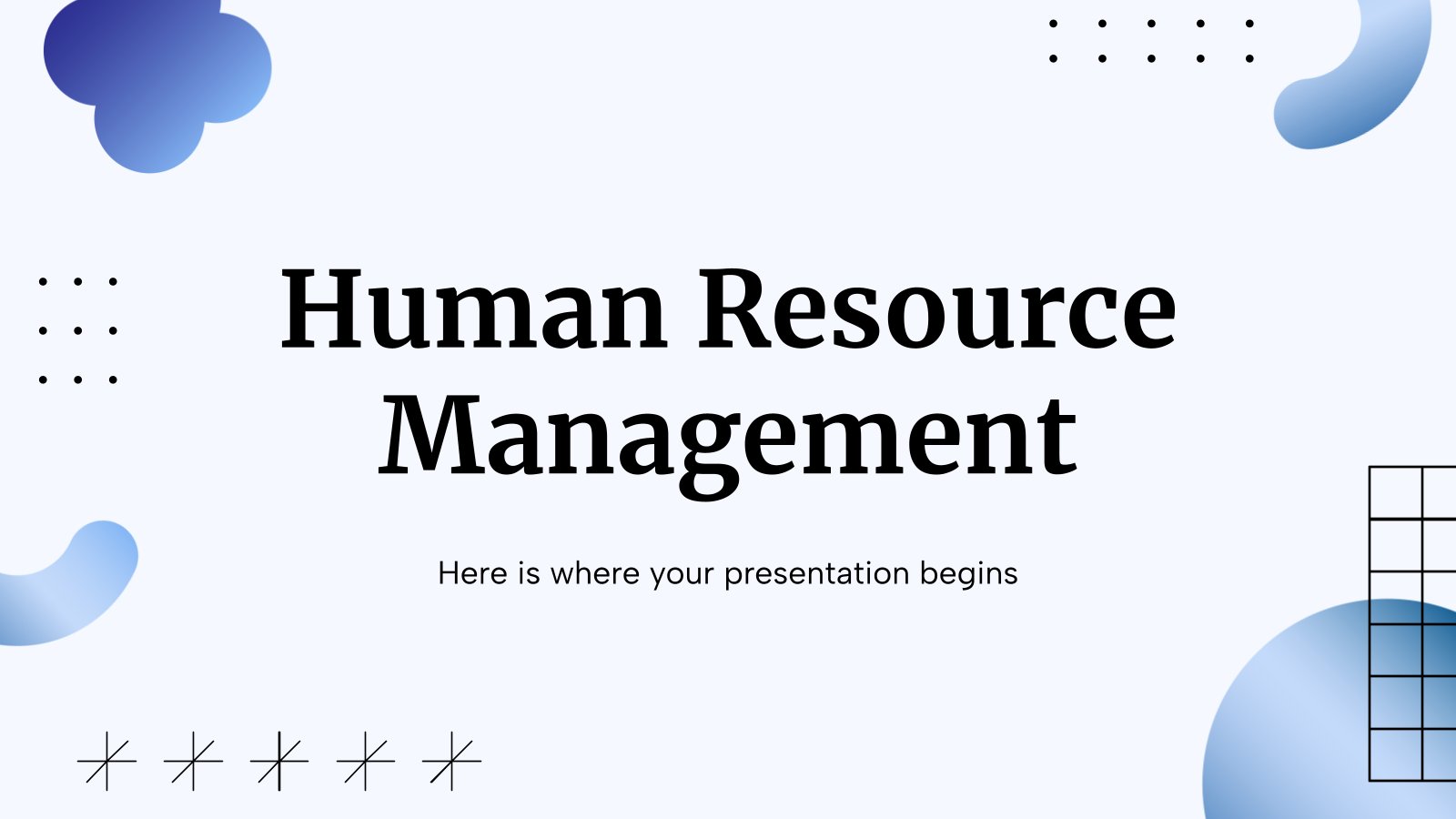
human resource
5 templates
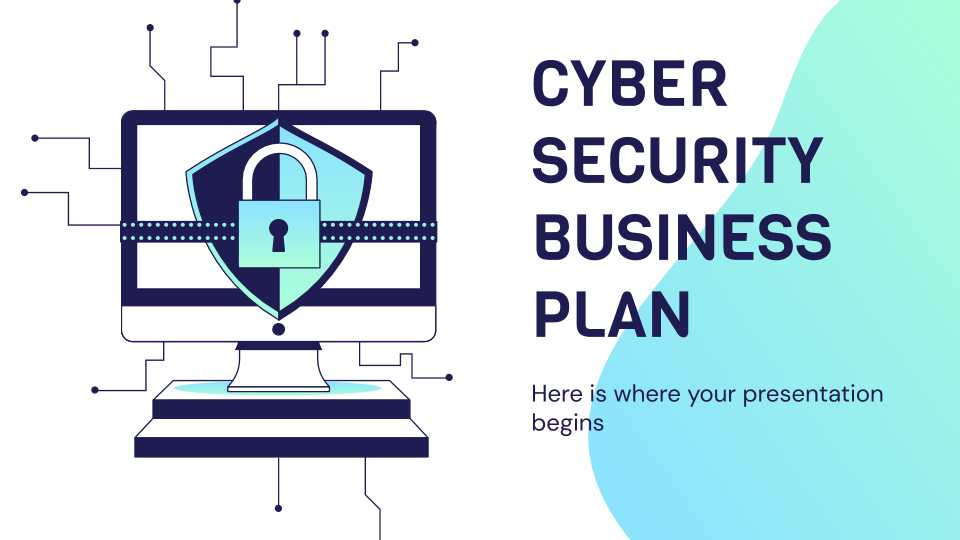
cyber security
11 templates
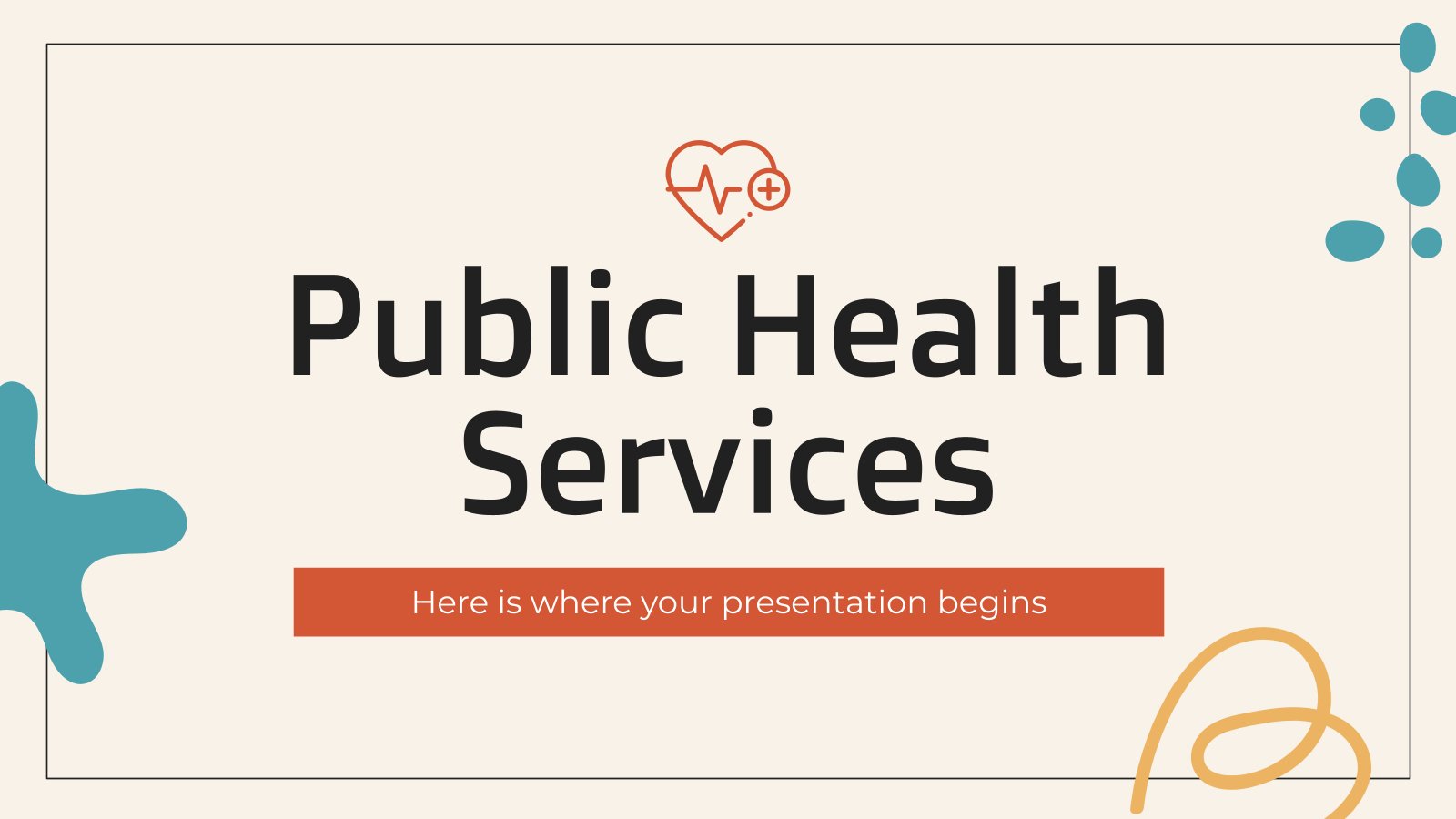
public health
39 templates
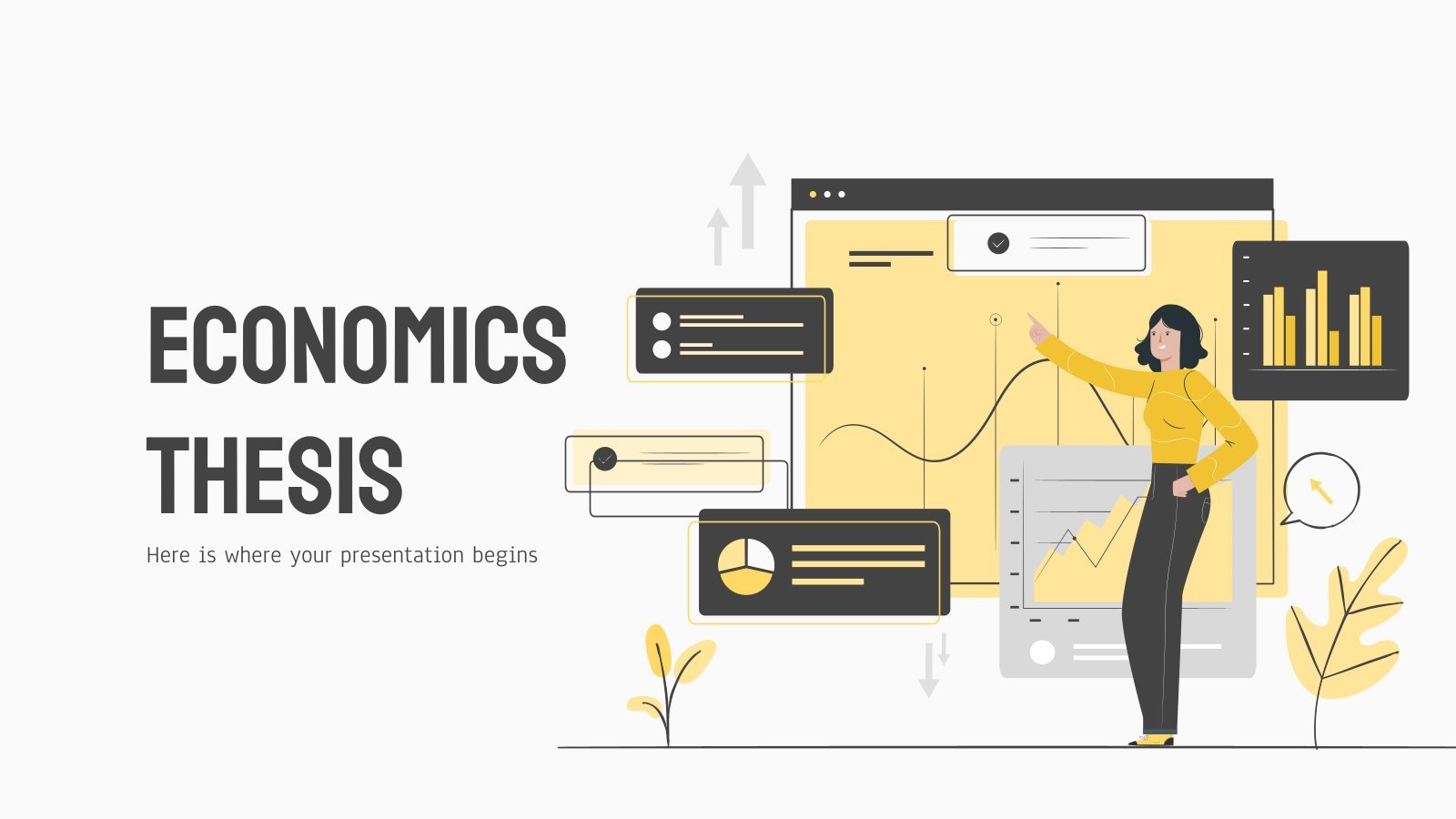
financial analysis
83 templates
What Is Climate?
It seems that you like this template, what is climate presentation, free google slides theme, powerpoint template, and canva presentation template.
Climate refers to the long-term patterns of temperature, rainfall, humidity, wind, and other atmospheric conditions in a region. It's essentially the overall "weather" of a place, averaged over a long period of time. If you want to know more about it, or you want to teach students about climate, you can use this ready-made presentation! Thanks to actual educators, we've been able to create these beautiful slides, illustrated and full of actual content available in different languages.
Features of this template
- Designed for Elementary
- 100% editable and easy to modify
- 19 different slides to impress your audience
- Contains easy-to-edit graphics such as graphs, maps, tables, timelines and mockups
- Includes 500+ icons and Flaticon’s extension for customizing your slides
- Designed to be used in Google Slides, Canva, and Microsoft PowerPoint
- A4 format optimized for printing
- Includes information about fonts, colors, and credits of the resources used
- Available in different languages
How can I use the template?
Am I free to use the templates?
How to attribute?
Attribution required If you are a free user, you must attribute Slidesgo by keeping the slide where the credits appear. How to attribute?
Available in, related posts on our blog.
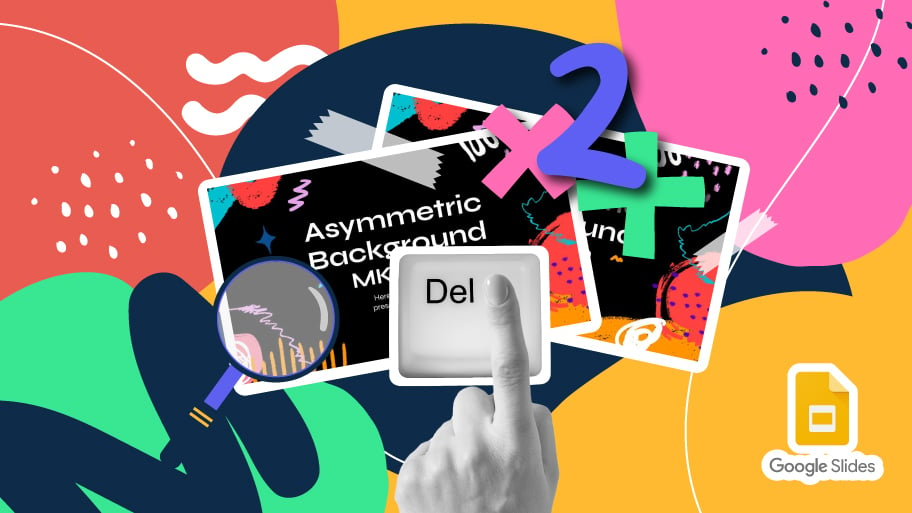
How to Add, Duplicate, Move, Delete or Hide Slides in Google Slides
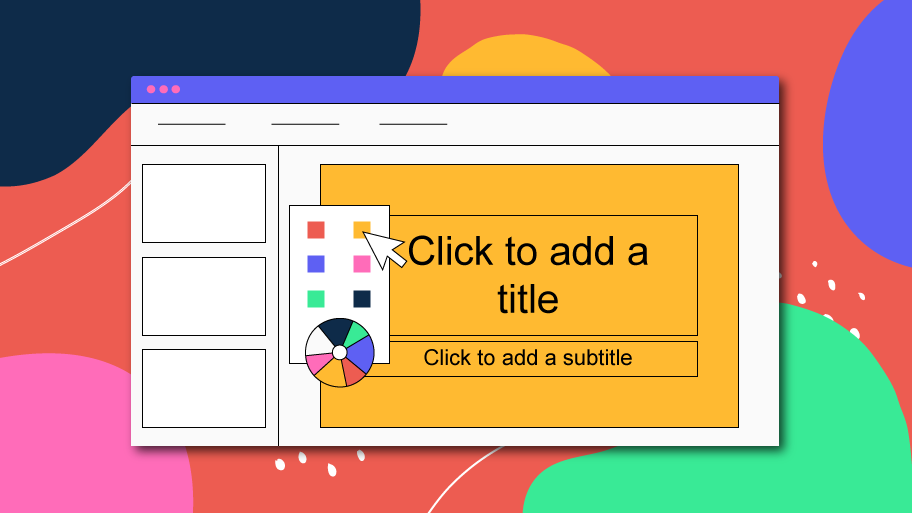
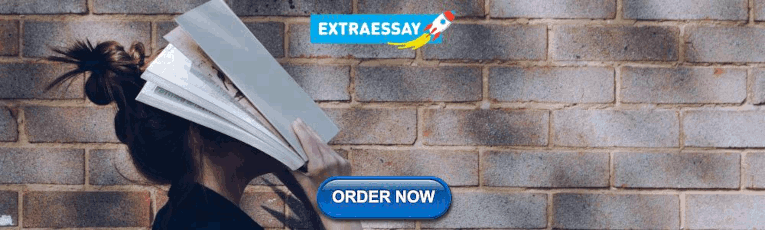
How to Change Layouts in PowerPoint
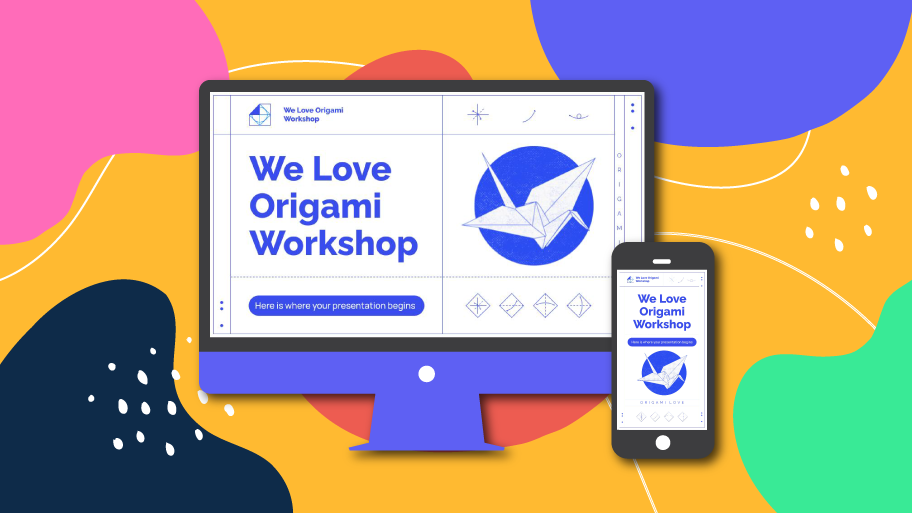
How to Change the Slide Size in Google Slides
Related presentations.
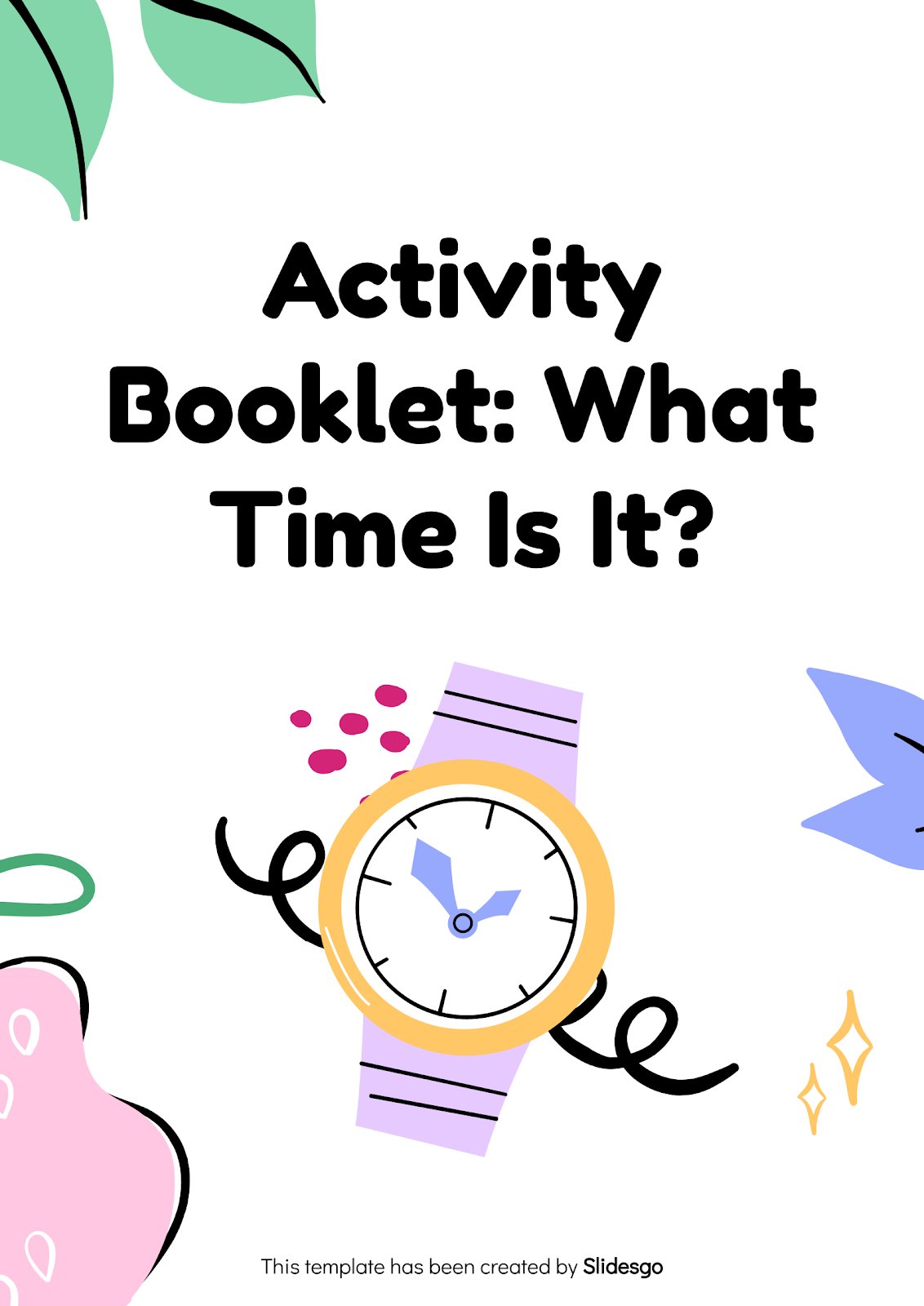
Premium template
Unlock this template and gain unlimited access
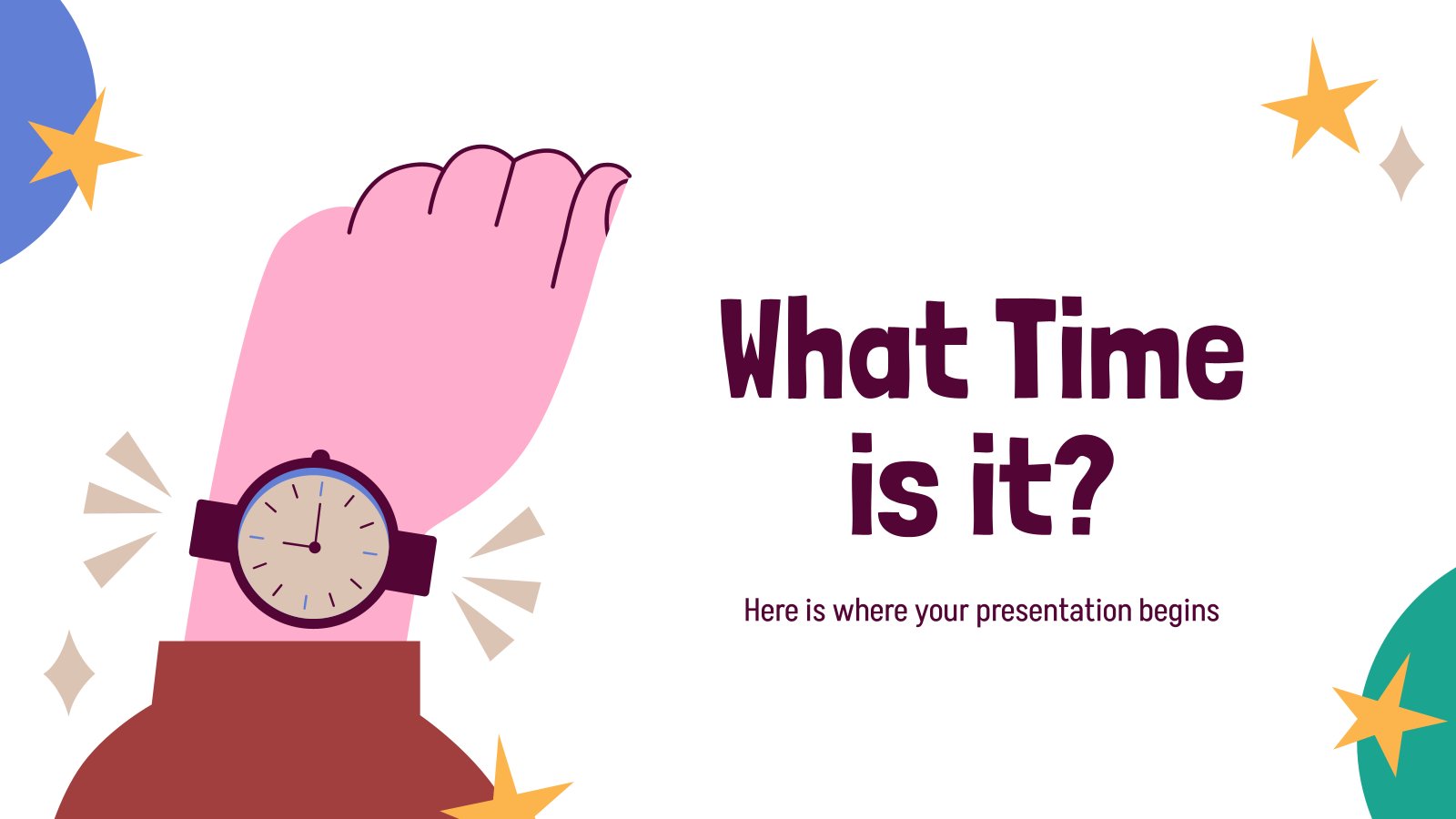
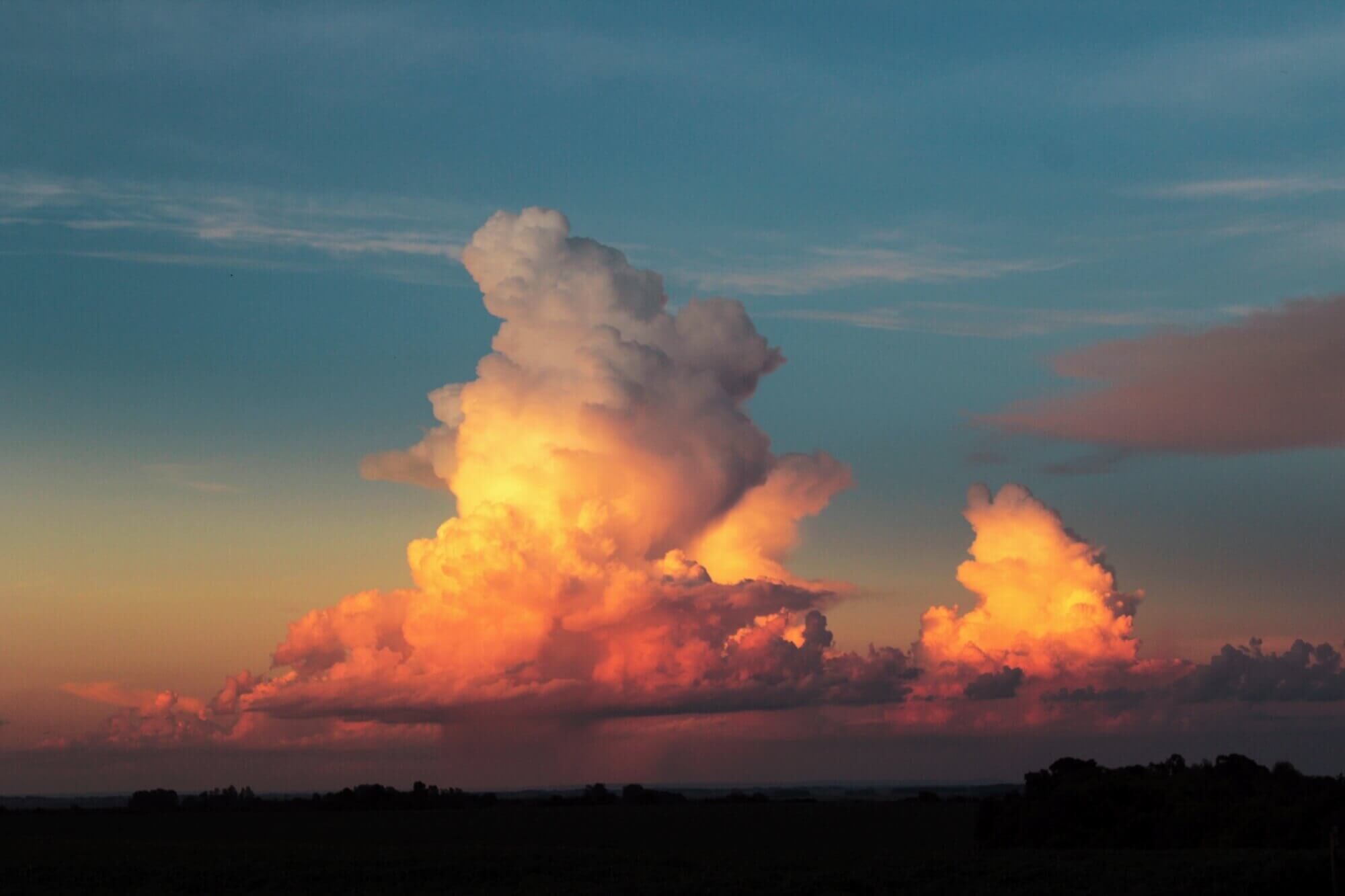
Visual Crossing Weather
Articles, videos, and documentation to help you get the most from Visual Crossing Weather
How do I add weather data into Microsoft PowerPoint?
We are going to walk you through building a PowerPoint that has a graph of your current forecast. We will utilize the ability to pull Visual Crossing Weather Data directly into your PowerPoints and have them update the forecast everytime you open it.
Weather data provider
We are going to use a number of techniques to include the weather data into our Microsoft PowerPoint. The technique we are focusing on is inserting a live web query link into a PowerPoint Slideshow so the weather forecast can update automatically. Before we can start including the weather data into our slides, we need a provider that will provide the weather data in a format suitable for Microsoft PowerPoint. For that we will be using Visual Crossing Weather Data as the weather data services product allows for easily viewing, downloading and web query access to both historical weather data and live weather forecast data.
If you don’t have an account, you can simply sign up for a free trial for Weather Data Services . For help on getting started with the Weather Data Services page, see Getting Started With Weather Data Service .
Now we have an easy way to find weather data, we will open a blank PowerPoint Presentation.
Creating Our Basic Graph
After you open your blank presentation, you can delete the default slide contents or create a new slide such that is doesn’t have any content on the slide. Next, from the ‘Insert’ menu choose the ‘Chart’ icon which will open a window from which you will select the ‘Line’ chart type, then choose the ‘Line with Markers’ icon. Finally we will click ‘OK’ at the bottom of our page.
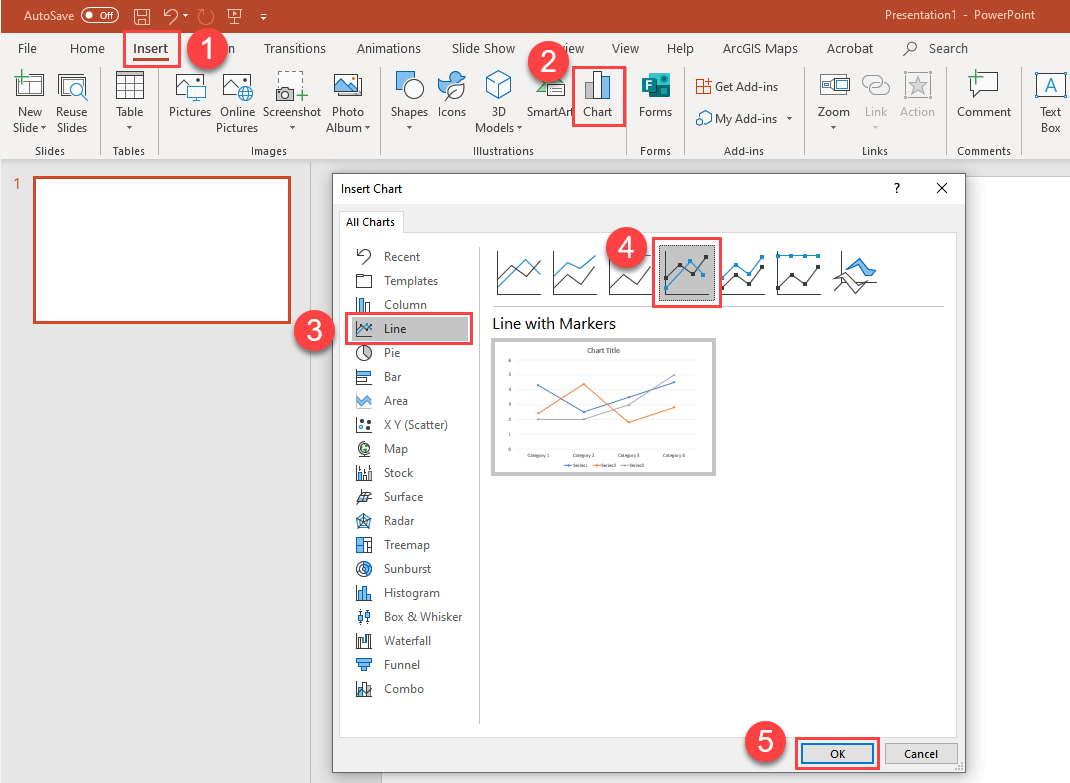
We now have our first chart:

The first thing you may notice is that an embedded Excel workbook named ‘Chart in Microsoft Powerpoint’ is associated with our chart and will contain all of the data you need to create your graph. However the initial version of editing in Excel is too basic for our needs. To open the full Excel editor simply click on the ‘OpenInExcel’ button at the top of the Excel workbook.
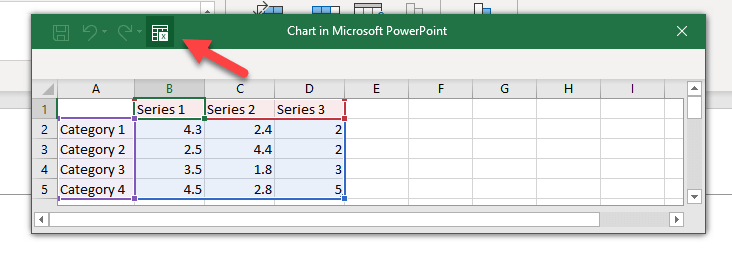
You will notice that a full instance of Excel will open with the chart data in ‘Sheet 1’. You can Delete this sheet later after we load our weather data.
Next we will work on loading in weather data. To do this click on the ‘Data’ tab in Excel, Choose the ‘From Web’ icon and Excel will open an entry box for you to enter a Web Query URL.
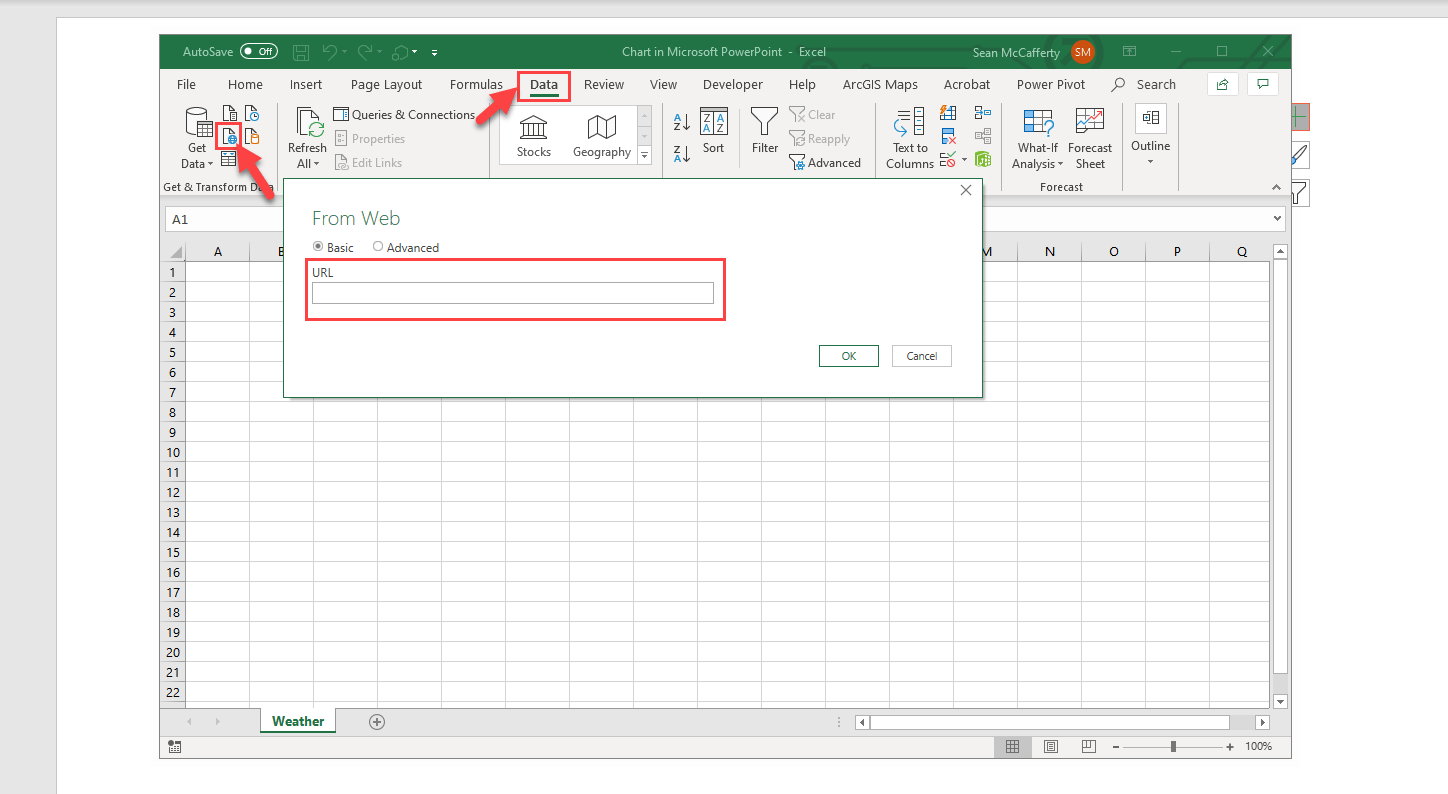
At this point we will take a slight detour from PowerPoint and Excel so that we can get a URL to fetch our weather data. To do this we will use the Visual Crossing Weather Data Service by visiting the weather data service page:
On this page you can login with your account or sign up for a trial. Once you are logged in, the page will request that you add a location for the weather query. We choose ‘Add Manual’ and enter in Herndon, VA as our weather location. Next we will choose the ‘Forecast’ option and we will choose the ‘Day’ interval to get daily forecast data and then we will submit our query by clicking on the ‘Request Weather Data’ button.

You should now see a 15-day forecast view for your location. Next we need to click on the ‘Query API’ button at the top of your forecast page where we can copy our URL that represents the query we just built. Click on the ‘Copy full query’ button to put the URL into your Copy/Paste buffer.
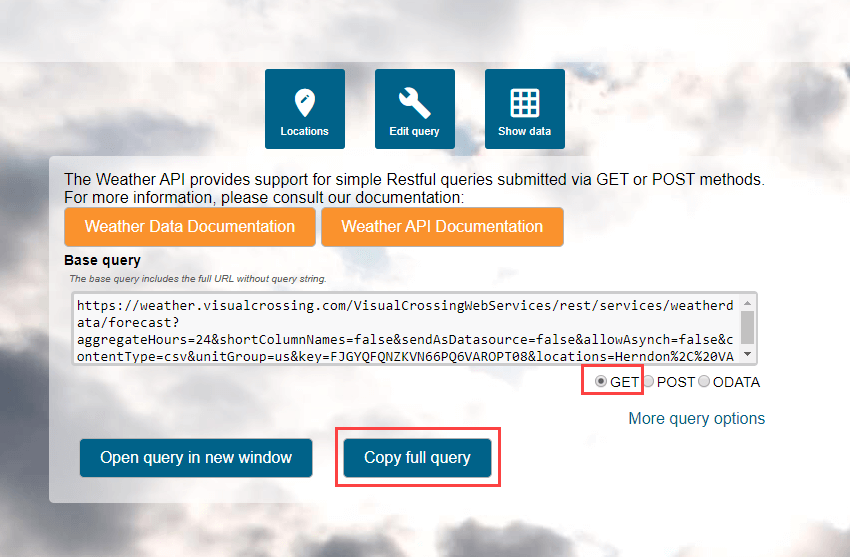
Now that we have our URL String copied we can paste in the string into our Excel window that requested a URL string to fetch our weather data as shown above. Click ‘OK’ and then click ‘Load’ to finish the query loading process. NOTE: You may be asked about security warnings as well as the fact that formulas and references have changed. This is normal and you can click through these to see the result of your query. We can now rename our new sheet ‘Weather’ or something appropriate and delete our previous default sheet that PowerPoint provided.

The final step in connecting the chart to our weather data is to change the chart series definitions. We do this by clicking on the ‘Select Data’ button on the Ribbon Bar while our chart is selected. This will open an editor that allows us to define both Axis series. First choose ‘Edit’ for the ‘Legend Entries Series’ and then select the table data from Address through temperature as seen by the red box below. Then choose ‘Edit’ for the ‘Horizontal Axis Label’ and choose the dates in column B called ‘DateTime’.(don’t choose the date column header as we only need the dates. This selection is highlighted by the purple box below. You should see the following:

After clicking ‘OK’ you should now see the following chart results with Temperature on the Y-axis with 3 series of Temperature data (Min, Max, Temperature) with our forecast dates on the X-axis.
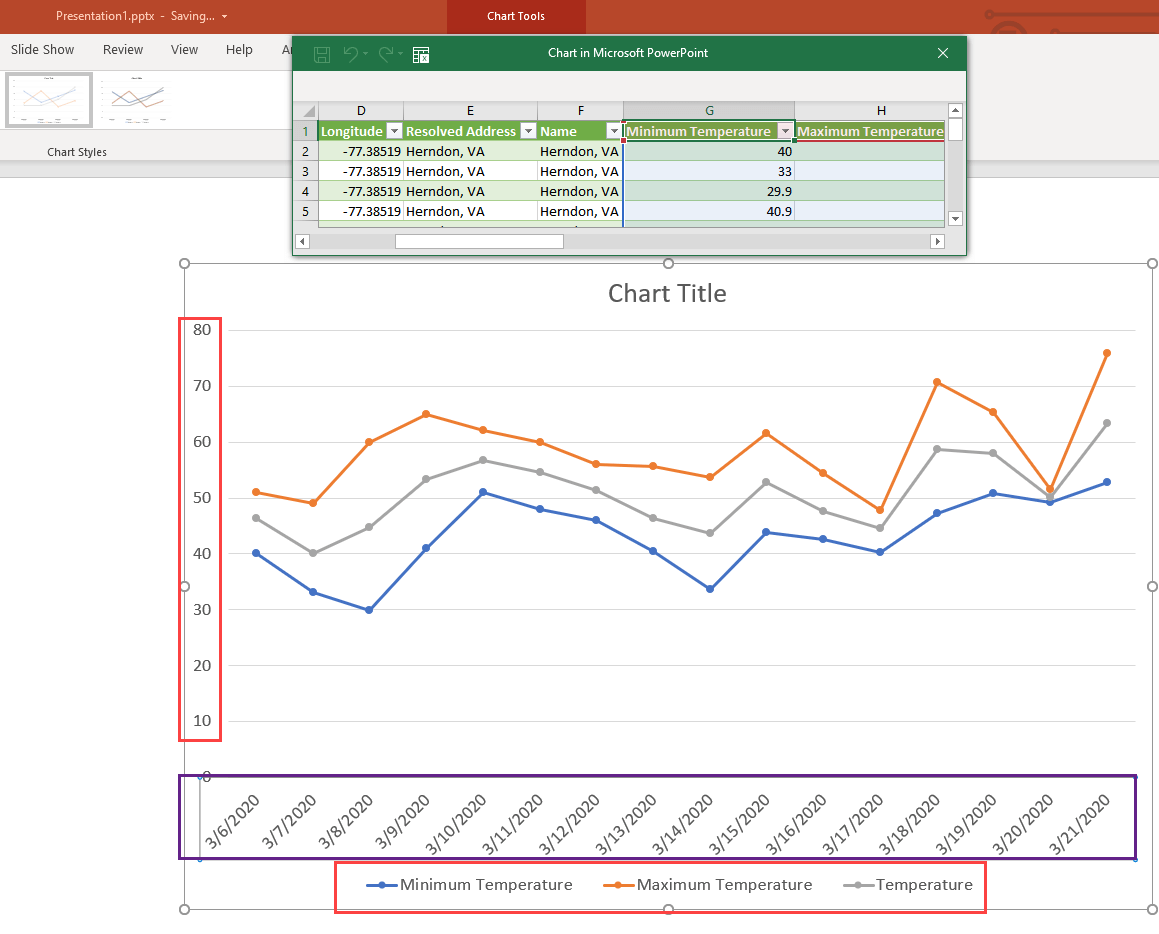
Now we just need to format and save our PowerPoint
Basic Chart Formatting
First we will change our Chart Title to ‘Herndon Weather Forecast’. Next, to keep this exercise simple we will use a predefined style for our chart by using the ‘Chart Styles’ button to the right of the chart. Here we will choose a pre-formatted style that appeals to us, maximize the chart to match the slide and then adjust any fonts as necessary. Here is our result:
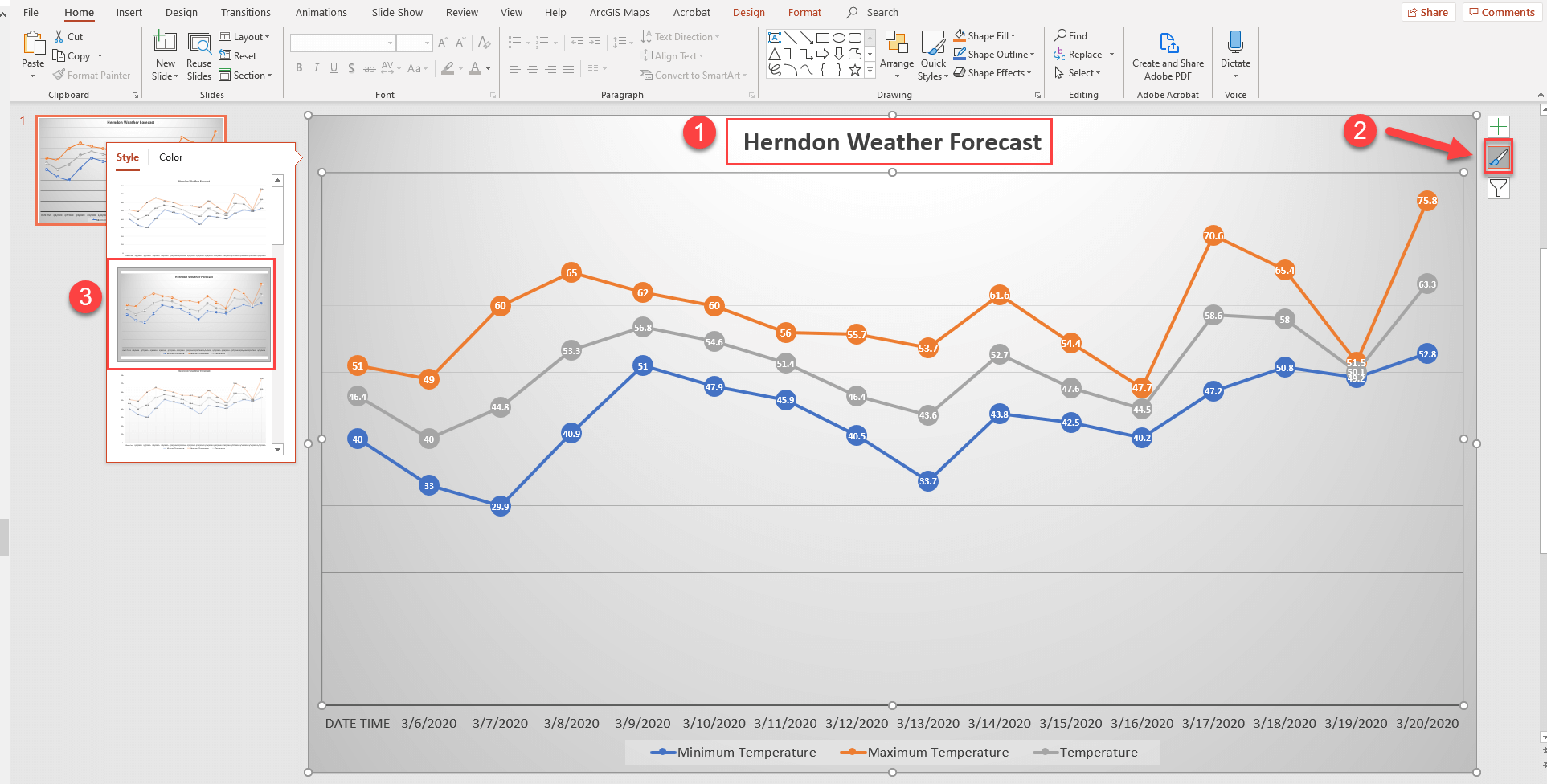
We now have an attractive chart of forecast weather data being loaded live into our PowerPoint Presentation! Very powerful and it only took a few minutes to create. Finally we will make sure the query updates dynamically.
Refreshing your Query Data
There are many ways to trigger updates to our query data. Users can manually refresh on the data, your can code in buttons to refresh the data or you can set up an auto refresh policy. For this example we will choose the latter. To do this, we right-click on the chart and choose ‘Edit Data’. As we did before we will open the full Excel editor. Once opened in Excel, you should see your weather data as before. Now choose the ‘Data’ menu and ‘Connection Properties…’. In this menu, you can select the refresh policy that is your preferred style. We have chosen to refresh the data on opening of the docuement. Everytime this Presentation is opened, the latest forecast data will be fetched.
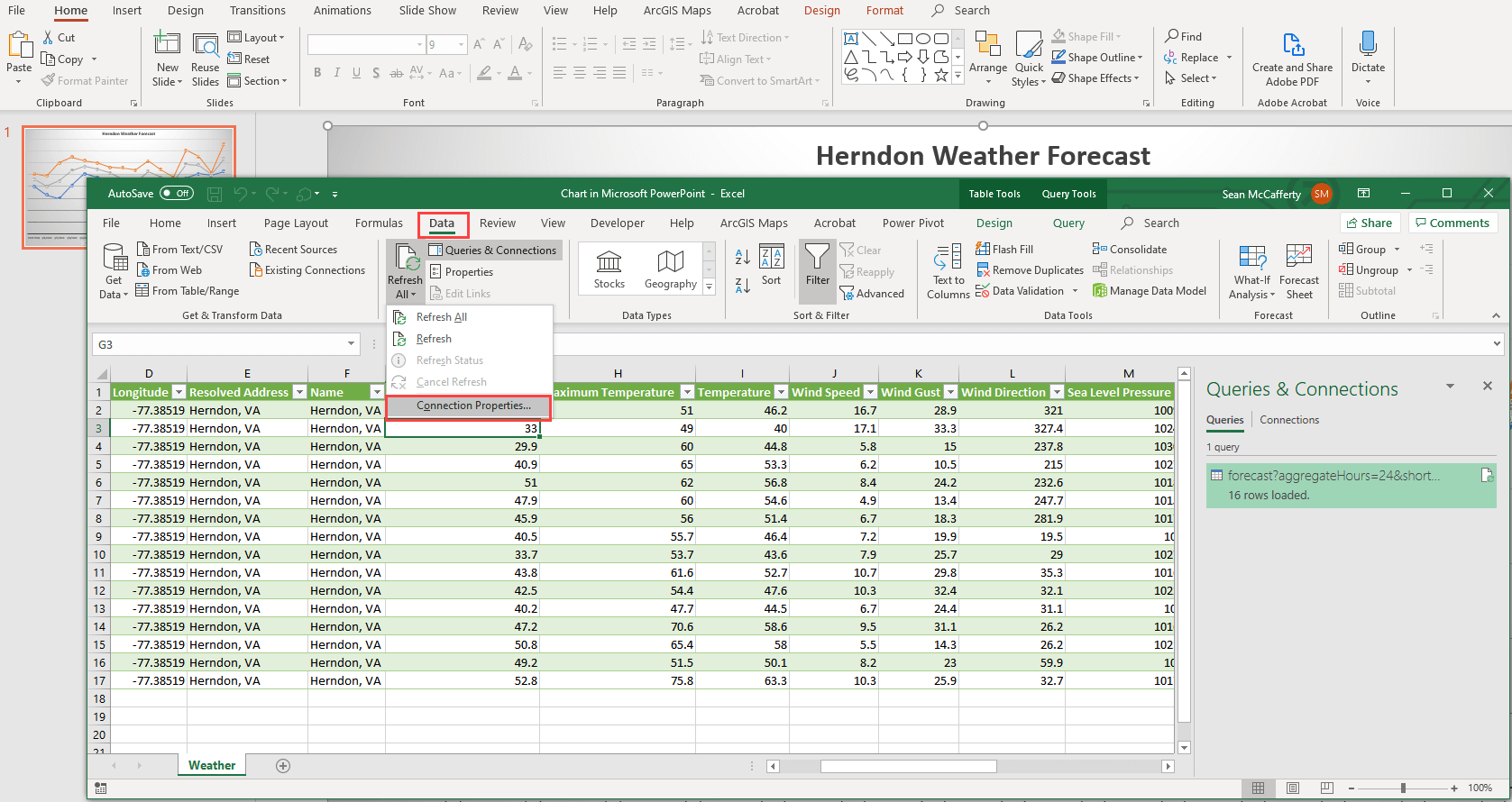
- DynamicPowerPoint.com
- SignageTube.com
- SplitFlapTV.com

The Weather is Always Changing. So Does Our Weather Source.
Feb 3, 2021 | DataPoint , DataPoint Real-time Screens , Dynamic elements
You are probably using our DataPoint or Dynamic Weather plugin to display real-time weather information on your television, and information screens. Our plugins are using an internet-based weather API to get the weather information from, behind the scenes. Recently, we had to stop the Dark Sky Weather API because it was taken over by Apple, and we selected another weather API. But it turned out that the data provided by this weather API was (maybe for some locations only) not accurate enough. Some of our users reported a significant difference between actual outside temperatures, compared to the actual temperatures reported by the weather API. Since we try to deliver good software, we also find it important that we deliver weather data that is correct. We are paying for this weather data, so, it has to be good.
We want to inform you that as of now, our software DataPoint and Dynamic Weather products, are using the new weather API with better weather data.
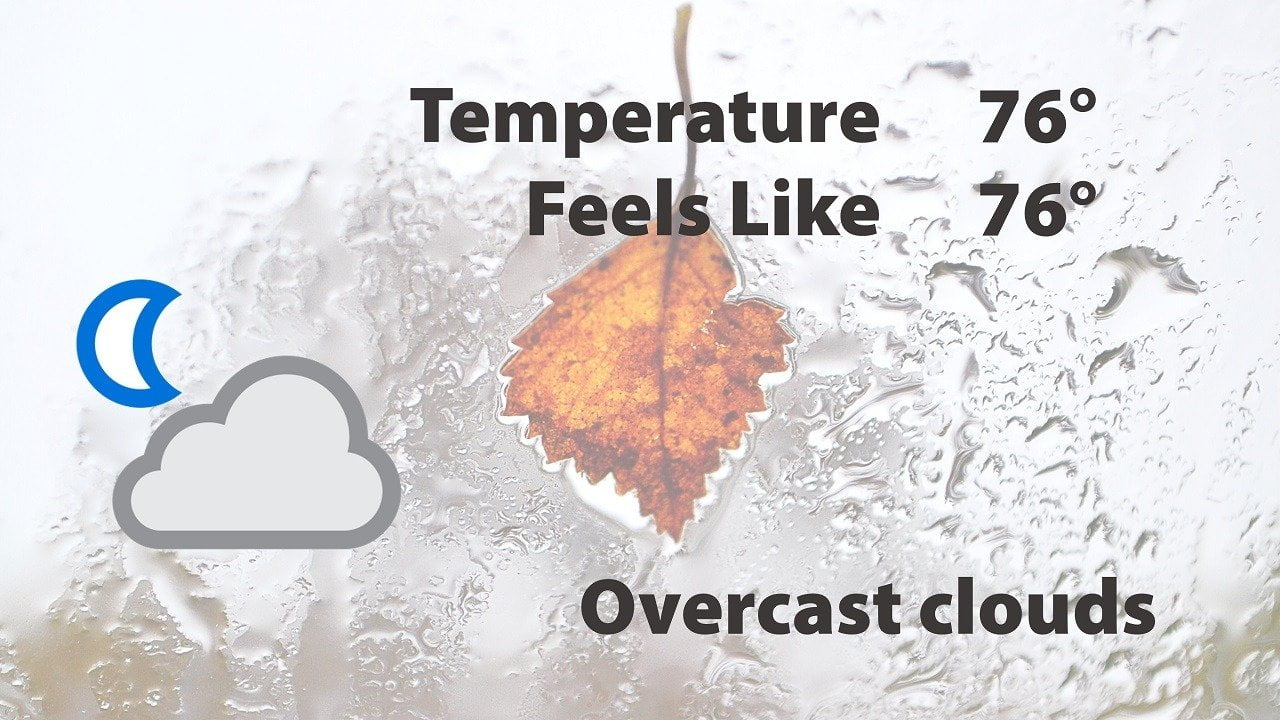
Submit a Comment
Your email address will not be published. Required fields are marked *
Pin It on Pinterest
- StumbleUpon
- Print Friendly
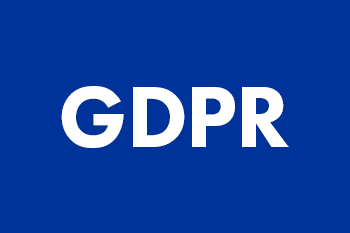
- Privacy Overview
- Strictly Necessary Cookies
This website uses cookies so that we can provide you with the best user experience possible. Cookie information is stored in your browser and performs functions such as recognising you when you return to our website and helping our team to understand which sections of the website you find most interesting and useful.
Strictly Necessary Cookie should be enabled at all times so that we can save your preferences for cookie settings.
If you disable this cookie, we will not be able to save your preferences. This means that every time you visit this website you will need to enable or disable cookies again.
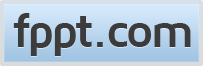
Add Weather Forecast to PowerPoint Presentation
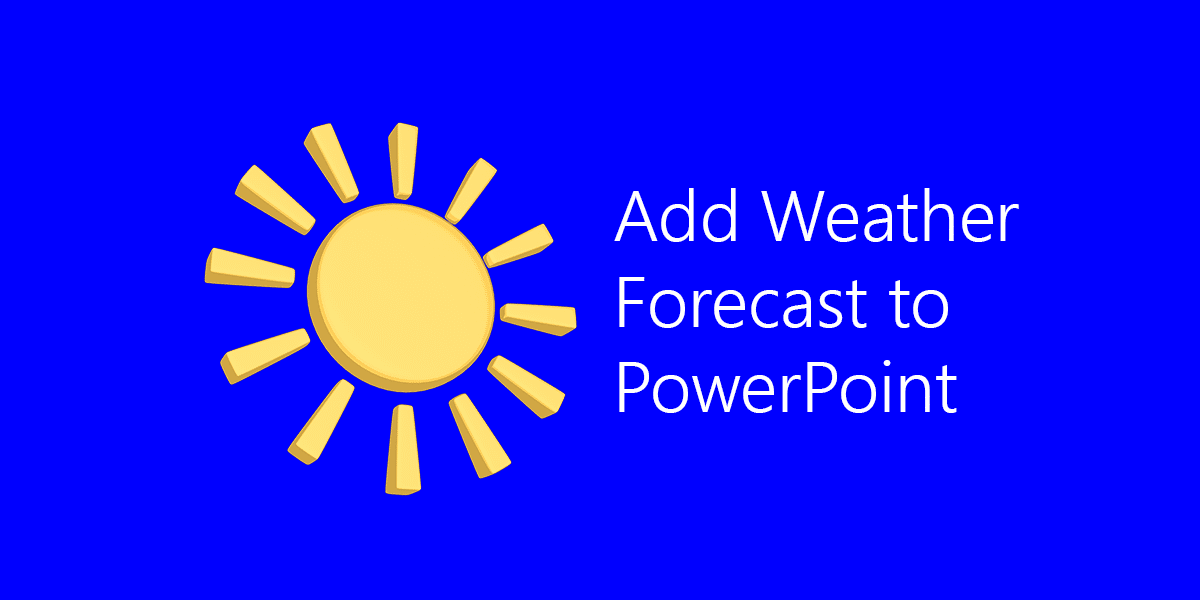
Weather forecast can be embedded in PowerPoint presentations and this is something present in one of the lessons available in Microsoft in Education and Lesson plans. You can take a look at Meteorologist for a Day . Here you will learn how to gather information from Weather.com and embed the weather forecast in a PowerPoint presentation template that is provided for free and can be downloaded from Weather PowerPoint template .
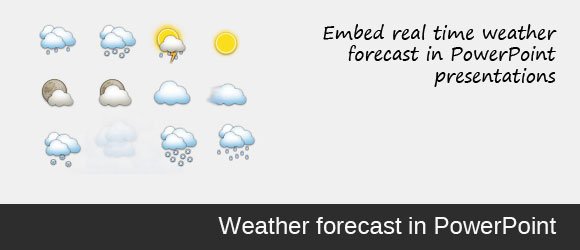
From this lesson we can learn how to embed the following information in a weather report.
Gather data for your weather report. Be sure to include:
- Current conditions
- Temperature
- Precipitation
- Current Doppler image
- 5-day forecast
However, sometimes we need to embed real time weather and forecast information in PowerPoint so we can show real time forecast information to the audience.
How to embed real time weather in PowerPoint?
For live updates you can download and install a free PowerPoint addin named LiveWeb which let you embed webpages in your PowerPoint presentations. If you have security restrictions in your organization then this method may not be ok for you, in this case you can try a different alternative or even use a static weather information. This method requires Internet connection in order to display the web page. We will use LiveWeb to gather information from a publicly available weather forecast and prediction website like weather.com and then display the webpage in the PowerPoint slide.
Once you have installed the plugin, you can insert a new web page. Look for this dialog and add the URL for the weather information page that you want to embed.
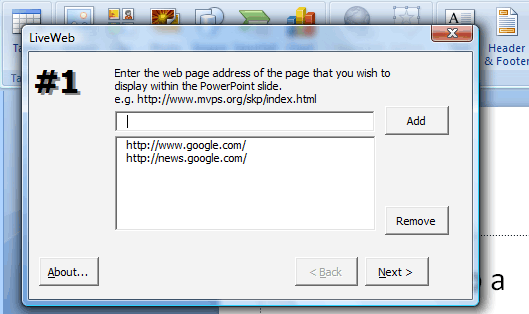
From weather.com you have many options. For example you can use the Weather API that has a monthly fee or you can embed a webpage.
If you want to embed the full web page, look for a city weather like New York weather and then copy the URL and paste it in the dialog above.
https://www.weather.com/weather/today/New+York+NY+USNY0996
Then you can choose where to display the weather page in your slide.
Using Google Weather API
Another interesting approach to embed real time weather report in your PowerPoint presentation templates and backgrounds is to use the Google Weather API that is free. You can use this API however in this case we’d get an XML as an output so we need to process this result from Google Weather API and then embed it into the slides. A possible approach is this one suggested by vba2vsto which explain how to use or parse XML in PowerPoint using VBA.
When you query this URL: http://www.google.com/ig/api?weather=new+york,ny
This will return a XML output like the following:
We can use different approaches to handle XML results in PowerPoint. We will see some of them very soon so stay tuned.
Programmableweb has a list of other 83 weather API’s available for you.
Free Editable Weather PowerPoint template
If you need to embed weather forecast information into a PowerPoint presentation, the free template provided by SlideHunter can be helpful. It is a free Weather PowerPoint template with a modern design and editable elements that will let you customize the slide and prepare awesome weather information or weather reports to an audience. It is great for example if you are showing an agenda to audience and want to show them how’s the weather like today or this week.
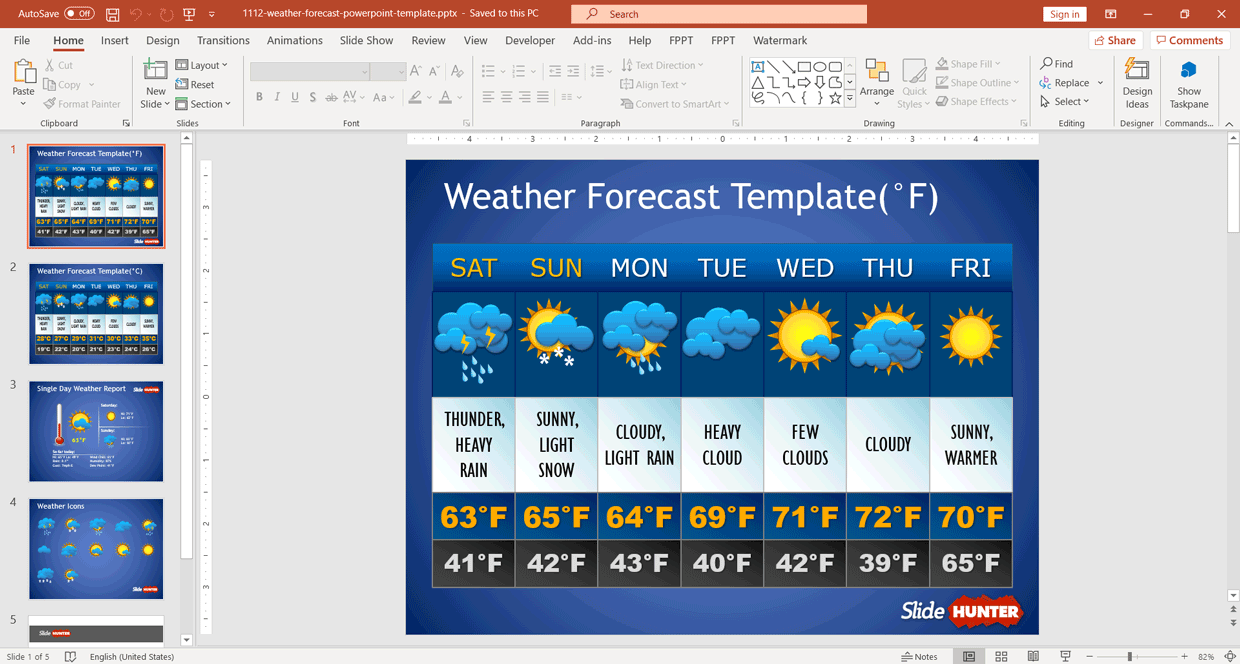
This template is provided for free and once you download it you can customize the slide elements, for example to change the weather from cloudy to sunny. Alternatively, you can download other free weather PowerPoint templates from our catalog, to prepare presentations on forecast, weather situations and more.
We will send you our curated collections to your email weekly. No spam, promise!
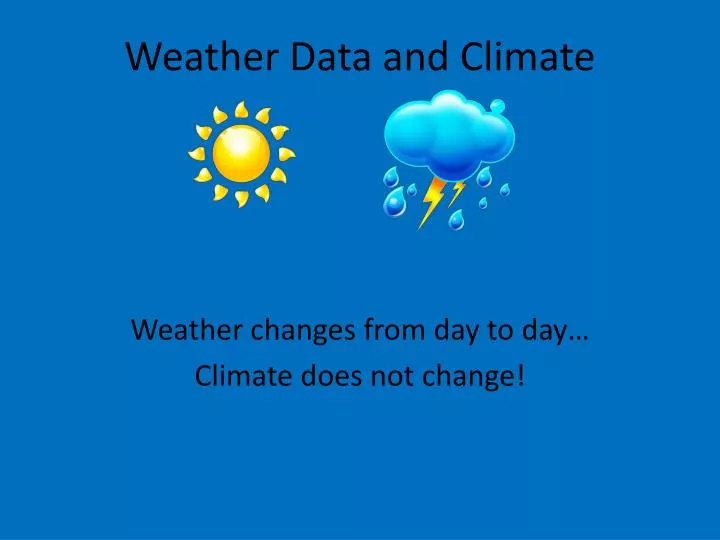
Weather Data and Climate
Nov 20, 2014
90 likes | 353 Views
Weather Data and Climate. Weather changes from day to day… Climate does not change!. Reading Weather Maps. 3 Major Climate Zones. Polar Zone Near Earth’s North & South Poles Tropical Zone Near equator Temperate Zone - between polar & tropical zones. EOG Review.
Share Presentation
- climate zone
- tropical zone
- equator zone
- polar tropical zones
- 3 major climate zones
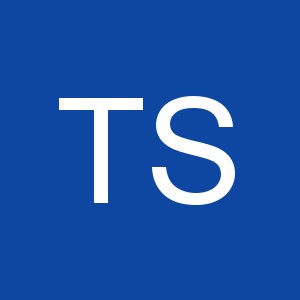
Presentation Transcript
Weather Data and Climate Weather changes from day to day… Climate does not change!
Reading Weather Maps
3 Major Climate Zones • Polar Zone • Near Earth’s North & South Poles • Tropical Zone • Near equator • Temperate Zone - between polar & tropical zones
EOG Review 2. What type of weather will Las Vegas receive in the next few days? a. snow b. sunny and dry c. thunderstorms d. clouds and light rain
EOG Review 3. What does the H near Mexico represent? a. a warm air mass b. an area of high air pressure c. a hot air mass d. high winds
EOG Review • What type of weather might the city of Raleigh receive in the next few days? • Snow • Sunny and dry • Thunderstorms • Clouds and light rain
EOG Review 4. What two factors mostly determine climate? a. temperature and humidity b. thunderstorms and temperature c. temperature and precipitation d. precipitation and humidity
EOG Review 5. Which climate zone does Lumberton, NC fall in? a. polar zone b. equator zone c. tropical zone d. temperate zone
- More by User
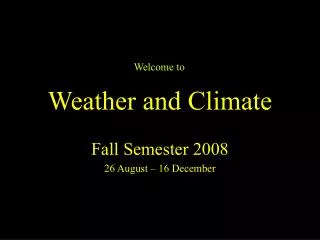
Weather and Climate
Weather and Climate. Welcome to. Fall Semester 2008 26 August – 16 December. Here’s a Course syllabus. Midterm exam – on Tuesday October 14th Final exam – on Tuesday December 16th Quizzes weekly on Blackboard BEFORE class Homework more than monthly In-class participation and quiz “weekly”
1.37k views • 62 slides
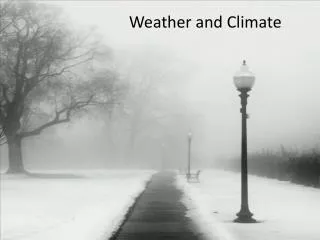
Weather and Climate. You will find the following topics in this Slideshow:. The difference between weather and climate. Microclimates (including the role of aspect, shelter, buildings, surface and natural features)
508 views • 13 slides
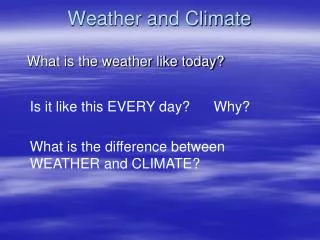
Weather and Climate. What is the weather like today?. Is it like this EVERY day? Why?. What is the difference between WEATHER and CLIMATE?. Weather and Climate. Weather – The temporary environmental conditions in a localized area over a short period of time.
829 views • 27 slides
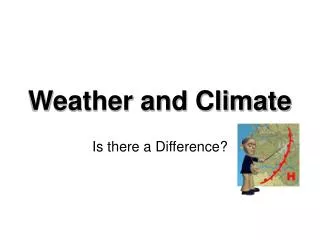
Weather and Climate. Is there a Difference?. Another I Love Science Presentation @ 2006- All Rights Reserved Iteachbio @ mac.com. Weather. Weather is the condition of the atmosphere at a particular time and place. Weather is affected by 4 atmospheric factors- Heat
318 views • 8 slides
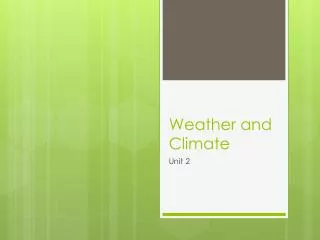
Weather and Climate. Unit 2. Question of the day?. What causes the seasons?. Objective:. - The student will understand that our location on the globe and the globe’s relation to the sun are the biggest determiners of climate. Review:. What is climate?.
406 views • 13 slides
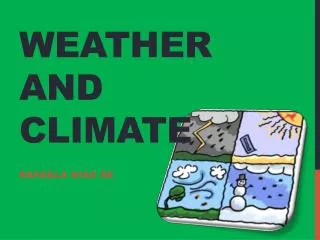
Weather and climate
Weather and climate. Rafaela Diaz 8E. Difference between weather and climate. The difference between weather and climate is a measure of time. Weather is what conditions of the atmosphere are over a short period of time. Climate is how the atmosphere "behaves" over long periods of time.
514 views • 11 slides
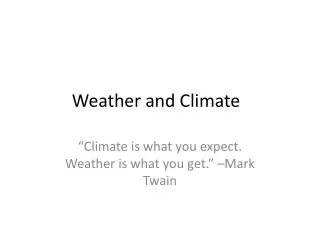
Weather and Climate . “Climate is what you expect. Weather is what you get.” –Mark Twain. Water is also present in varying amounts up to 4.0%. Structure of the atmosphere . Air Pressure and Density. Why do you need an oxygen mask to climb to the Summit of Mt. Everest?.
1.06k views • 67 slides
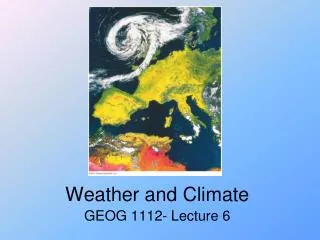
Weather and Climate. GEOG 1112- Lecture 6. Chapter 3: Introduction to the Atmosphere. McKnight’s Physical Geography : A Landscape Appreciation, Tenth Edition, Hess. Image courtesy of cimss.ssec.wisc.edu. Introduction to the Atmosphere. Size of Earth’s Atmosphere
828 views • 50 slides
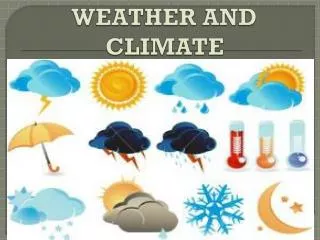
WEATHER AND CLIMATE
WEATHER AND CLIMATE. WEATHER VS CLIMATE. WEATHER. CLIMATE. Weather is the everyday state of the atmosphere, and its short-term (minutes to weeks) variation. In popular, weather is known as the mixture of the temperature, humidity, precipitation, cloudiness, visibility, and wind .
2.42k views • 14 slides
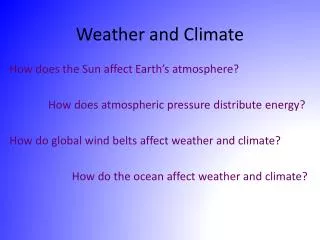
Weather and Climate. How does the Sun affect Earth’s atmosphere? How does atmospheric pressure distribute energy? How do global wind belts affect weather and climate? How do the ocean affect weather and climate?. The Sun and Latitude.
531 views • 18 slides
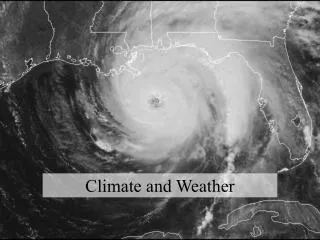
Climate and Weather
Climate and Weather. Today’s class. Map projection leftovers Air pressure and winds Climate comparisons Weather: hurricanes. Map projections. Project a round globe onto a flat surface Options? Stretch out some areas Cut out some areas Shrink some areas. Map projections.
341 views • 25 slides
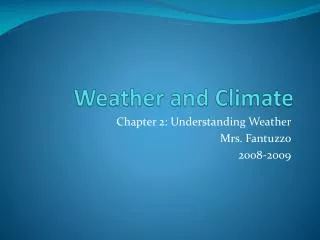
Weather and Climate . Chapter 2: Understanding Weather Mrs. Fantuzzo 2008-2009. DO NOW:. List the four parts to the water cycle. . DO NOW:. List the four parts to the water cycle. . Section 1: Objectives. Explain how water moves through the water cycle.
346 views • 17 slides
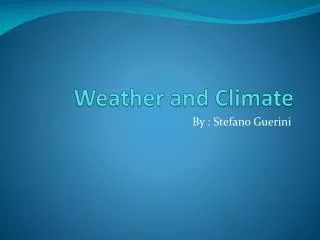
Weather and Climate. By : Stefano Guerini. Climate. Climate : So, the climate of Antarctica is quite different than the climate of a tropical island. Hot summer days are quite typical of climates in many regions of the world, even without the affects of global warming. Weather.
653 views • 9 slides
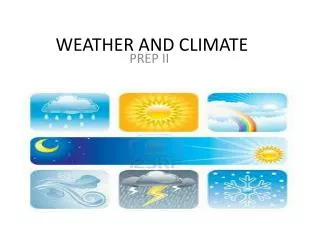
WEATHER AND CLIMATE. PREP II. What is climate?. The weather conditions prevailing in an area in general or over a long period. A region with particular prevailing weather conditions. Difference between weather and climate
266 views • 12 slides
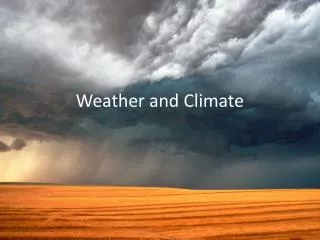
Weather and Climate. Climate vs. Weather. Weather: Short term state of the atmosphere. Temperature, humidity, cloud cover, precipitation, winds, visibility, air pressure, air pollution, etc… Climate: The average weather conditions in an area over a long period of time
742 views • 37 slides
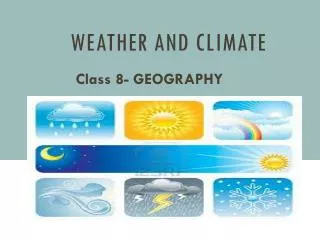
WEATHER AND CLIMATE. Class 8- GEOGRAPHY. Weather And Climate. Difference between weather and climate Weather is what conditions of the atmosphere are over a short period of time C limate is how the atmosphere "behaves" over relatively long periods of time.
400 views • 12 slides
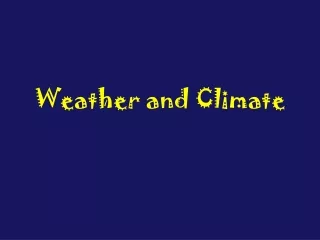
Weather and Climate. Weather. Weather is the state of the atmosphere over a short period of time ie a day Look outside and describe today’s weather. Is it sunny or cloudy? Hot or cold?. Lightning Thunder Rain Sunshine Clouds. Mist Fog Cool breeze Warm breeze Snow.
462 views • 21 slides
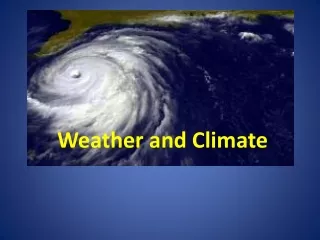
Weather and Climate. Why is weather important in Saskatchewan? Spend 3 minutes sharing your thoughts with 1 partner. Is climate important in Saskatchewan?. Climate & Weather. Climate is what we expect, weather is what we get. – Mark Twain. Climate & Weather.
340 views • 33 slides
PIREP Submit
Pirep entry results.
Daily Temperature and Precipitation Reports - Data Tables
Daily summaries answer questions such as
- What were the high and low temperatures at a station on a specific day?
- How much did it rain last Wednesday?
- How much snow was on the ground on a recent date?
Where do these data come from?
Daily weather records come from automated and human-facilitated observation stations in the Global Historical Climatology Network-Daily database. Data from each station are reviewed regularly for quality and consistency: the data have been checked for obvious inaccuracies, but they have not been adjusted to account for the influences of historical changes in instrumentation or observing practices.
What can I do with these data?
- Find daily records of high and low temperature and precipitation for most localities in the United States.
- Display different variables to look for patterns and compare them among different dates.
- Where did it rain or snow on a specific date?
- How does temperature change with latitude?
- How much snow was on the ground at a station on a specific date?
How do I use the site?
1) Start at https://www.ncei.noaa.gov/maps/daily-summaries/
2) In the first window, click Daily Summary Observations
3) Set the DATE and TYPE of data you want.
- In the Layers tab of the sidebar, use the pull-down menus to select your options:
- Set the calendar to the desired Date (data from today become available in two business days)
- Select the desired Observation (temperature, precipitation, snowfall, snow depth)
- Select the Units (Fahrenheit or Celsius)
- Select Options , if desired and available (not available for all data)
- Click Update Map
4) Choose WHERE you want it for.
Enter a placename or zip code in the SEARCH FOR A LOCATION field. This will zoom the map to your region of interest.
- DO NOT try to click the blinking red dot. It is NOT a station with data; it is only the center of the location your searched for.
- use the identity tool to select a single station at a time
- Stations you selected show up in the Results tab.
5) Preview and/or Download the data
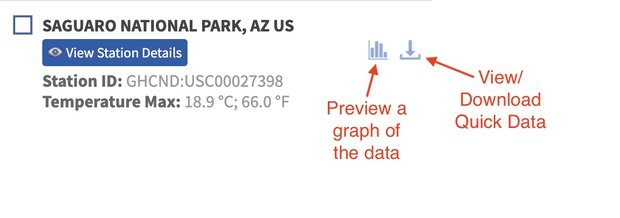
- Click the Graph icon to preview a graph of the data. (Note: Preview graphs show data in tenths of degrees Celsius.
- Click the View/Download icon to download a PDF document of the data.
6) If you want to download a station's data in another format:
Check the box to the left of its name, and then click the Add to Cart button
- Complete the fields in the Cart window (non-certified data are free), then Continue
- Enter your email address.
- You'll receive a email confirming your order right away. Then, you'll receive another email with a link to download your data, usually within the hour.
Access Type | Link & Description |
---|---|
Map viewer and site selection | |
Documentation Type | Link & Description |
---|---|
Download a PDF that describes the data. |
- Point Resolution
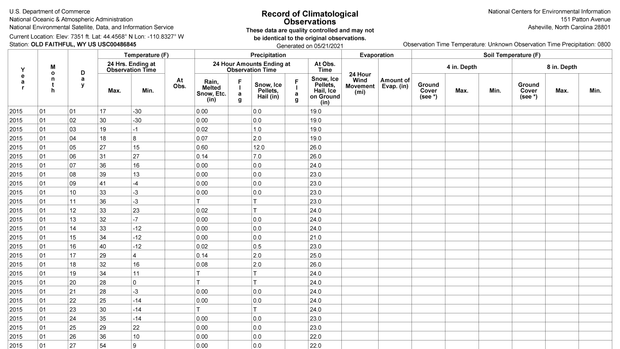
We value your feedback
Help us improve our content
Related Content
News & features, what's the coldest day of the year (1981-2010 normals), what does 2017 look like in your neck of the woods, first dates: when does the climate record say you can expect the season's first snow, maps & data, past weather by zip code - data table, record-setting weather - charts and maps, temperature - us monthly, difference from average, teaching climate, cocorahs — community collaborative rain, hail and snow network: citizen scientists track precipitation, climate youth engagement, toolbox for teaching climate & energy, climate resilience toolkit, snowfall climatology toolbox, extremes database: srcc's climate extremes portal, sc-acis—applied climate information system.
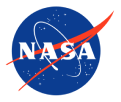
The Ocean and Climate Change
Our ocean is changing. With 70 percent of the planet covered in water, the seas are important drivers of the global climate. Yet increasing greenhouse gases from human activities are altering the ocean before our eyes. NASA and its partners are on a mission to find out more.

The ocean is warming
Rising greenhouse gas concentrations not only warm the air, but the ocean, too. Research shows that around 90 percent of the excess heat from global warming is being absorbed by the ocean. Ocean heat has steadily risen since measurements began in 1955, breaking records in 2023 . All this added heat has led to more frequent and intense marine heat waves. The image visualizes sea surface temperature anomalies in August 2023. Warm colors (red, orange) show where the ocean was warmer than normal. Cool colors (blues) show where temperatures were cooler. The red swath in the Eastern Pacific was due to an El Niño event. El Niño is a climate phenomenon in the tropical Pacific that results in warmer than normal sea surface temperatures leading to weather impacts across the planet. Credit: NASA
Sea levels are rising
Global sea levels have risen more than 4 inches (101 millimeters) since measurements began in 1992, increasing coastal flooding in some places. As ocean water warms, it expands and takes up more space. The added heat in the air and ocean is also melting ice sheets and glaciers, which adds freshwater to the ocean and further raises sea levels. The Surface Water and Ocean Topography (SWOT) mission , launched in 2022, and Sentinel 6 Michael Freilich , launched in 2020, are providing unparalleled views of sea level rise on top of decades of data from other missions. The video shows a 21-day average of sea surface height anomalies highlighting ocean eddies and currents as imaged by the Surface Water and Ocean Topography (SWOT) satellite. The red and orange colors indicate where the sea surface was higher than normal and the blues are where it was lower than normal. Credit: NAS
Explore Earth's Vital Signs
The ocean is getting a little greener
Recent research found that over the past 20 years, the tropical ocean turned greener. Ocean color reflects the life that is found in it. Green colors often correspond to phytoplankton, microscopic plant-like organisms that form the center of the ocean's food web. Observations of changes in phytoplankton populations due to climate change are a key part of the Plankton, Aerosol, Cloud, and ocean Ecosystem (PACE) mission , which launched in 2024.

Ocean warming is altering hurricanes
Hurricanes need warm water to form and strengthen. Recent research points to warmer ocean temperatures as a key factor causing more storms to rapidly intensify. One way to detect rapid intensification before it happens may be through a change in lightning around the eye of the storm. Plus, higher sea levels worsen storm surge flooding when a storm travels over a coastline. NOAA’s GOES-East satellite captures the rapid intensification of Hurricane Lee on Sept. 7, 2023. Credit: NASA/NOAA

Ocean acidification and heating are altering marine ecosystems
Carbon dioxide and heat are both absorbed by the ocean as greenhouse gas levels increase. When carbon dioxide is dissolved in the ocean, the water becomes more acidic. This makes it harder for corals and some other marine life to grow shells and protect themselves. Marine heat waves are complicating the matter by making it too warm for many corals to survive. Satellites are providing important data to scientists measuring such changes in ocean environments. When corals are stressed from changes in their environment, they turn white, or "bleach." Sometimes the coral is able to recover, but other times the bleaching event leads to its death. This image shows the decay of a healthy coral reef to a reef between 2014 and 2015 in the National Marine Sanctuary of American Samoa. Credit: NOAA/ XL Catlin Seaview Survey

Sea ice is thinning and shrinking
Melting sea ice does not affect sea levels, but it does impact global temperatures. Sea ice is light-colored and reflects sunlight back into space; open water is darker and absorbs more sunlight. Warming ocean waters melt sea ice from below, and warmer air helps melt it from above. As ice cover thins and shrinks, more ocean is exposed and less sunlight is reflected, further warming the water and air. Satellites help monitor changes in sea ice which is an area of research for upcoming missions in the Earth Systems Observatory . A photograph of Arctic sea ice breaking up as seen during an overhead flight during NASA’s Operation IceBridge in March 2011. Credit: NASA
Explore the Earth Systems Observatory

El Niño can add to the heat
El Niño occurs when the central and eastern tropical Pacific Ocean become warmer than normal. This periodic ocean warming can add to the long-term global warming that has already accumulated, making a hot year even hotter. That’s because ocean temperatures are major drivers of global temperatures, as seen in 2023 . A visualization showing sea surface height anomalies in the Pacific Ocean in June 2023 based on satellite data. The red and orange colors show a higher-than-normal sea surface height. The blue areas were lower than normal. Credit: NASA
Read More About 2023's Record Heat

Ocean circulation may be changing
Ocean currents are vital transporters of heat around the planet. As the Greenland and Antarctic ice sheets melt, the excess fresh water running into the ocean could disrupt the balance of temperature and salinity that drive deep ocean currents. NASA satellite mission s are monitoring the ocean for changes in heat transport as glaciers continue to melt and the ocean warms. Clouds trace out islands in the Caribbean Sea in this photo taken by an astronaut aboard the International Space Station. Credit: NASA
Read More About Ocean Circulation
Key Satellites and Missions

Aqua is collecting data about Earth's water cycle.

Gravity Recovery and Climate Experiment Follow-On (GRACE-FO)
GRACE-FO is tracking Earth’s water movement across the planet.

Sentinel-6 Michael Freilich
Sentinel-6 Michael Freilich is measuring the height of the ocean.

Surface Water and Ocean Topography (SWOT)
SWOT is providing the first global survey of Earth’s surface water and measuring how it is changing over time.

Plankton, Aerosol, Cloud, and ocean Ecosystem (PACE)
PACE is measuring key variables related to cloud formation, particles and pollutants in the air, and microscopic, floating marine life.

Earth System Observatory
NASA’s Earth System Observatory is a series of satellites working in tandem to create a 3D, holistic view of Earth, from bedrock to atmosphere.
Latest News and Research
Nasa mission flies over arctic to study sea ice melt causes.

How NASA Spotted El Niño Changing the Saltiness of Coastal Waters
Vanishing corals: nasa data helps track coral reefs.
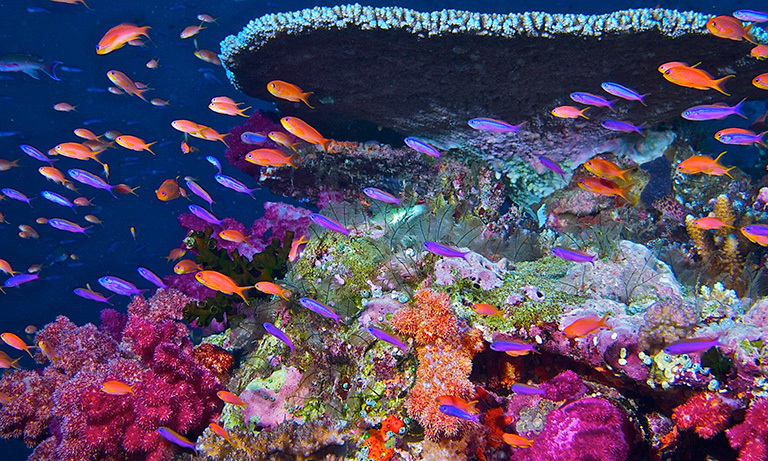
Is the Wilkins Ice Shelf Weakening?
Key ocean and climate resources.

NASA Sea Level Change
NASA’s Sea Level Change portal provides key data, stories, and tools related to NASA’s sea level research.

NASA Earthdata - Ocean
NASA’s Earthdata provides open access to ocean and other datasets produced by NASA satellites and its partners.

Estimating Circulation and Climate of the Ocean (ECCO) Consortium
ECCO combines state-of-the-art ocean circulation models with global ocean datasets to estimate ocean circulation and its role in the climate.

State of the Ocean on NASA Worldview
NASA Worldview State of the Ocean visualizes real data from satellites to show sea surface temperatures and anomalies, and chlorophyll on a daily basis.
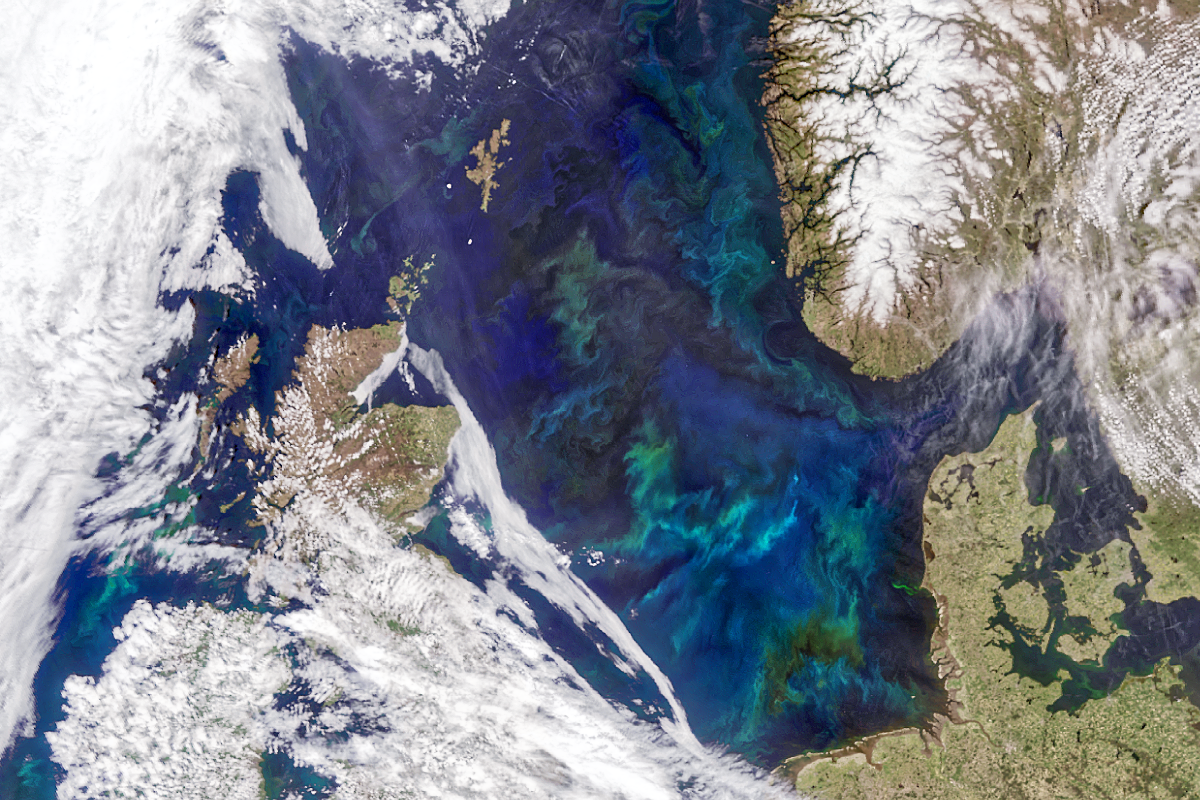
Ocean Color
Ocean Color provides key data, stories, and tools related to NASA’s Ocean Biology Processing Group’s research.
Climate Kids
The ocean covers about 70% of Earth’s surface. So, it’s not surprising that it plays a large part in Earth’s environment. As Earth warms, water in the ocean soaks up energy (heat) and distributes it more evenly across the planet. The ocean also absorbs carbon dioxide from Earth’s atmosphere. The additional heat and carbon dioxide in the ocean can change the environment for the many plants and animals that live there.

Can you blame heat wave on climate change? Eye-popping numbers suggest so.
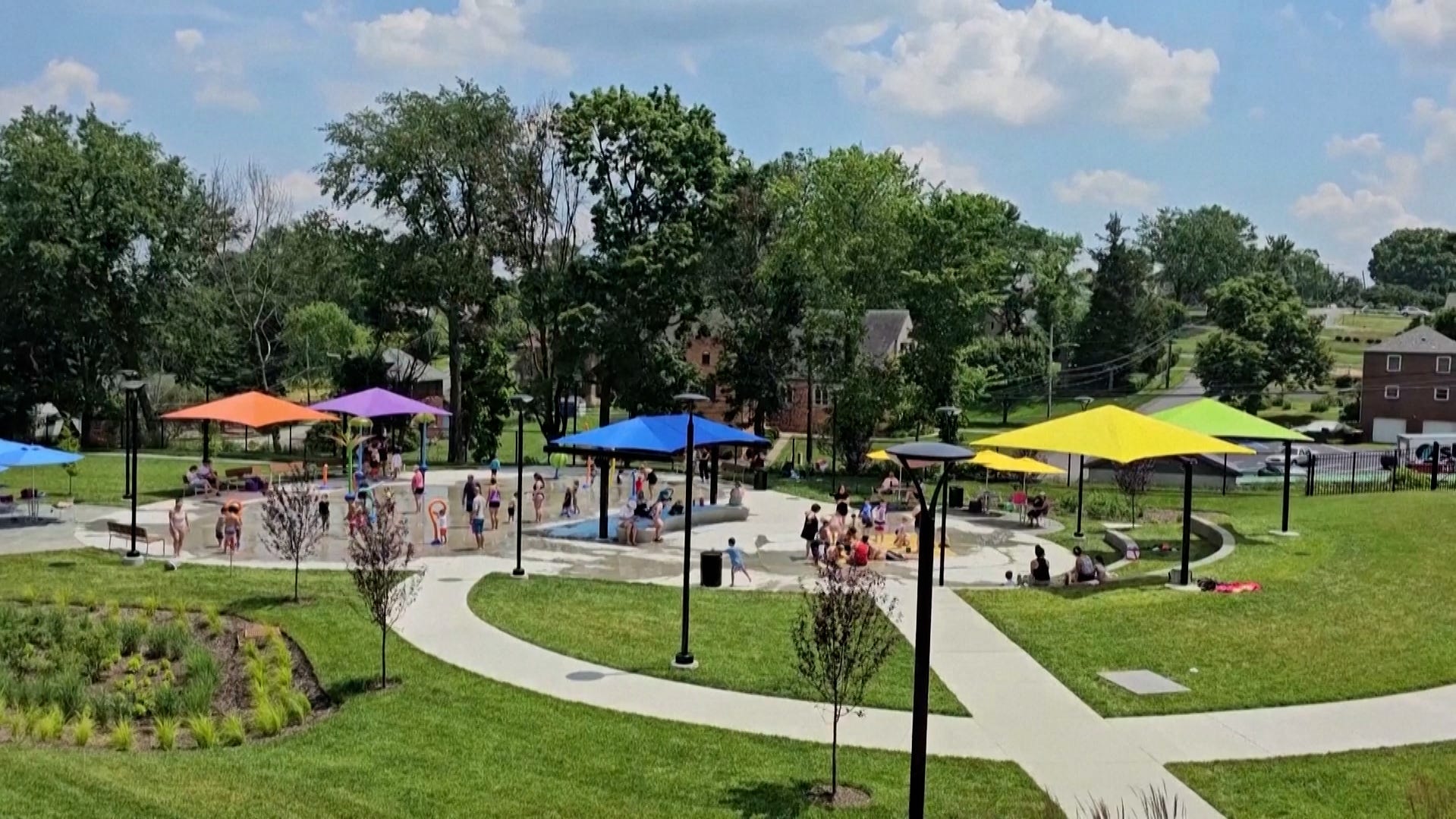
Here's a line you can use at the pool or beach this weekend: "Yep, it's climate change. "
The deadly heat wave that scorched much of North America in early May and early June – and is still baking the central and eastern U.S. – was made 35 times more likely because of human-caused climate change, a scientific study released Thursday says.
The heat wave has killed at least 125 people and led to thousands of heatstroke cases in Mexico, where the heat was particularly intense. Scientists say heat waves will continue to intensify if the world continues to unleash climate-warming emissions from the burning of fossil fuels.
The study was done by World Weather Attribution , an international collaboration of scientists that studies the influence of climate change on extreme weather events.
Deadly and record-breaking heat
"Potentially deadly and record-breaking temperatures are occurring more and more frequently in the U.S., Mexico and Central America due to climate change," said study co-author Izidine Pinto, a researcher at the Royal Netherlands Meteorological Institute.
“The results of our study should be taken as another warning that our climate is heating to dangerous levels," he said.
The study focused on the Southwest U.S. and Mexico, as well as Guatemala, Belize, El Salvador and Honduras, where temperatures were also extreme.
The heat has not been confined to the Americas: May this year was the hottest May on record globally and the 12th month in a row a hottest-month record was broken.
How a heat dome has played a part
According to the World Weather Attribution group , the area has been underneath a large and lingering region of high pressure known as a heat dome, which occurs when hot air is trapped close to the ground and further heated under blue skies and sunshine.
"Whilst heat domes have a well-known mechanism for intensifying heat waves, these past weeks have seen records broken in both daytime and nighttime temperatures in several countries, including Mexico, Guatemala, Honduras and in the southwestern US," the group said in a statement.
They also noted that a heat wave such as this one is four times more likely to occur today than it was in the year 2000.
“Unsurprisingly, heat waves are getting deadlier," study co-author Friederike Otto of Imperial College London said.
Otto added that since 2000, in just 24 years, June heat waves in North and Central America have become 1.4 degrees hotter, exposing millions more people to dangerous heat.
What do others say?
Brett Anderson, AccuWeather climate expert and senior meteorologist, said "climate change is clearly playing a role in enhancing this warming."
"As we continue to put more and more greenhouse gases into the atmosphere, these types of extreme heat and drought conditions across the Southwest and Mexico will almost certainly become more common and perhaps even the norm by the end of this century or even much earlier," Anderson said in an e-mail to USA TODAY.
University of Southern California marine studies chair Carly Kenkel, who wasn’t part of the attribution team’s study, told the Associated Press the analysis is “the logical conclusion based on the data.”
“We’re looking at a shifting baseline – what was once extreme but rare is becoming increasingly common.”
COLLEGE FOOTBALL 25 Rankings Week Showcase
Who are the best teams in ea sports™ college football 25.
Hey College Football Fans,
Welcome back to the Campus Huddle! This week, we have a special “living” edition of the Campus Huddle, centered around Rankings Week.
So what is Rankings Week?
It’s a time to celebrate various EA SPORTS™ College Football 25 rankings, from the Toughest Places to Play, to the Top Offenses and Defenses, to our final Team Power Rankings before the worldwide launch on July 19. Plus, we’ll have our Sights and Sounds Deep Dive coming Wednesday, showcasing the incredible and unique presentation features coming to EA SPORTS™ College Football 25.
The full Rankings Week schedule can be seen here:
TOUGHEST PLACES TO PLAY
We laid out the significant impact that Homefield Advantage can have on the outcome of games in EA SPORTS™ College Football 25 during our Gameplay Deep Dive Campus Huddle . Audio and in-game modifiers such as blurred routes, incorrect play art, confidence and composure affects, and screen shaking are some of the immersive impacts away teams and players will be forced to contend with.
But not all Homefield Advantages are created equal. The Development Team worked to compile a list of the Top 25 Toughest Places to Play, factoring in historical stats such as home winning %, home game attendance, active home winning streaks, team prestige, and more.
Rankings are subject to change in future updates.
- Kyle Field - Texas A&M
- Bryant-Denny Stadium - Alabama
- Tiger Stadium - LSU
- Ohio Stadium - Ohio State
- Sanford Stadium - Georgia
- Beaver Stadium - Penn State
- Camp Randall Stadium - Wisconsin
- Gaylord Family Oklahoma Memorial Stadium - Oklahoma
- Doak S. Campbell Stadium - Florida State
- Ben Hill Griffin Stadium - Florida
- Autzen Stadium - Oregon
- Memorial Stadium - Clemson
- Neyland Stadium - Tennessee
- Jordan-Hare Stadium - Auburn
- Williams-Brice Stadium - South Carolina
- Michigan Stadium - Michigan
- Lane Stadium - Virginia Tech
- Rice-Eccles Stadium - Utah
- Darrell K. Royal - Texas Memorial Stadium - Texas
- Kinnick Stadium - Iowa
- Notre Dame Stadium - Notre Dame
- Spartan Stadium - Michigan State
- Donald W. Reynolds Razorback Stadium - Arkansas
- Albertsons Stadium - Boise State
- Davis Wade Stadium - Mississippi State
SIGHTS AND SOUNDS DEEP DIVE
In case you missed it, Kirk Herbstreit is back with our next Deep Dive, taking a look at the sights and sounds featured in EA SPORTS™ College Football 25. The Development Team spent years capturing countless traditions, mascots, fight songs, and more to the game, ensuring all 134 schools and fan bases were represented with pride. These elements make College Football special and unique, bringing the unmatched feeling of game day to your fingertips.
For even more on the presentation elements and how they come to life, check out the latest Campus Huddle hosted by Senior Game Designer Christian Brandt.
OFFENSIVE/DEFENSIVE POWER RANKINGS
The Development Team meticulously examined hundreds of thousands of data points to arrive at our team power rankings. With help from our friends at Pro Football Focus (PFF), the team analyzed all 134 rosters, thousands of players, years worth of game film, and mountains of stats, ultimately arriving at our Team Power Rankings.
Here are the Top 25 offenses in EA SPORTS™ College Football 25:
- Georgia - 94 OVR
- Oregon - 94 OVR
- Alabama - 91 OVR
- Texas - 91 OVR
- Ohio State - 89 OVR
- LSU - 89 OVR
- Miami - 89 OVR
- Colorado - 89 OVR
- Missouri - 89 OVR
- Clemson - 87 OVR
- Utah - 87 OVR
- Penn State - 87 OVR
- Ole Miss - 87 OVR
- Kansas - 87 OVR
- Arizona - 87 OVR
- NC State - 87 OVR
- Notre Dame - 85 OVR
- Texas A&M - 85 OVR
- Memphis - 85 OVR
- SMU - 85 OVR
- UCF - 85 OVR
- Florida State - 83 OVR
- Oklahoma - 83 OVR
- Virginia Tech - 83 OVR
- USC - 83 OVR
As the old saying goes, “Defense wins championships.” Here are the Top 25 defenses in EA SPORTS™ College Football 25:
- Ohio State - 96 OVR
- Oregon - 90 OVR
- Alabama - 90 OVR
- Clemson - 90 OVR
- Notre Dame - 90 OVR
- Michigan - 90 OVR
- Texas - 88 OVR
- Penn State - 88 OVR
- Utah - 88 OVR
- Florida State - 88 OVR
- Oklahoma - 88 OVR
- Iowa - 88 OVR
- Virginia Tech - 86 OVR
- Wisconsin - 86 OVR
- USC - 86 OVR
- Auburn - 86 OVR
- LSU - 84 OVR
- Texas A&M - 84 OVR
- Colorado - 84 OVR
- Oklahoma State - 84 OVR
- Louisville - 84 OVR
- North Carolina - 84 OVR
- Kansas State - 84 OVR
- Florida - 84 OVR
TEAM POWER RANKINGS
And the moment you’ve all been waiting for! Here are the Top Teams in EA SPORTS™ College Football 25.
- Georgia - 95 OVR
- Ohio State - 93 OVR
- Oregon - 93 OVR
- Alabama - 92 OVR
- Texas - 92 OVR
- LSU - 90 OVR
- Michigan - 88 OVR
- Miami - 88 OVR
- Texas A&M - 88 OVR
- Ole Miss - 88 OVR
- Colorado - 87 OVR
- Oklahoma - 87 OVR
- Wisconsin - 87 OVR
- USC - 87 OVR
- Virginia Tech - 87 OVR
- Oklahoma State - 87 OVR
- Iowa - 87 OVR
Let us know what you think! Join the conversation today by following EA SPORTS™ College Football 25 on social media and rep your school. Next week, we’ll have even more information to share including our Dynasty Deep Dive where we explore the ins and outs of the mode, recruiting, and more!
College Football 25 launches worldwide on July 19th, 2024. Pre-order the Deluxe Edition* or the EA SPORTS™ MVP Bundle** and play 3 days early. Conditions and restrictions apply. See disclaimers for details. Stay in the conversation by following us on Facebook , Twitter , Instagram , YouTube , and Answers HQ .
Pre-order the MVP Bundle*** to make game day every day, and get both Madden NFL 25 and College Football 25 with exclusive content.
FOLLOW US @EASPORTSCollege
Sign-up for our newsletter to be the first to know about new updates.
RELATED NEWS
College football 25 sights and sounds deep dive, college football 25 gameplay deep dive, welcome to college football 25.

R news and tutorials contributed by hundreds of R bloggers
Introduction to standardization in business reporting.
Posted on June 27, 2024 by Numbers around us in R bloggers | 0 Comments
Why Standardization Matters

Hey there! Thanks for joining me on this exciting journey into the world of International Business Communication Standards (IBCS). Before we dive into the nitty-gritty of the SUCCESS acronym, let’s take a step back and chat about why standardization in business reporting is such a game-changer. If you’ve ever felt overwhelmed by messy reports with inconsistent formatting, you’re not alone. I’ve been there too, staring at a sea of numbers that don’t quite add up.
Standardization in business reporting ensures that data is presented in a consistent manner, enhancing comprehensibility and comparability across different reports. Imagine flipping through different reports where each one tells its story in its own unique language — confusing, right? Standardization is like translating all those languages into one that everyone can understand easily.
Consistency is Key
Think of standardized reports as a well-organized bookshelf. You know exactly where to find what you’re looking for, and every book (or in this case, piece of data) is presented in a way that makes sense. This consistency is crucial for making informed business decisions quickly and accurately. No more wasting time trying to figure out what’s what!
I remember a time when I was working on a project that involved analyzing sales data across multiple brands. Each region had its own way of reporting — different formats, different terminologies, and different visualization styles. It was a nightmare to compile all this information into a coherent report. That’s when I discovered the power of standardization. By applying consistent formats and visual styles, the report not only became easier to read but also revealed insights that were previously hidden in the chaos.
Time-Saving and Efficiency
Let’s be honest, who wouldn’t want to save time? Standardization not only reduces the risk of misinterpretation but also enhances the efficiency of report generation and review processes. Once you have a standardized template, creating new reports becomes a breeze. You can focus more on analyzing the data rather than formatting the report.
Understanding IBCS Standards
Now that we’ve established why standardization is so important, let’s get to know IBCS. The International Business Communication Standards provide a comprehensive framework for the design of business communication, particularly in the context of reports, presentations, and dashboards. The goal of IBCS is to improve the clarity, efficiency, and effectiveness of business communications.
The SUCCESS Formula
The heart of IBCS is the SUCCESS formula:
- SAY : Convey a clear message.
- UNIFY : Apply consistent semantic notation.
- CONDENSE : Increase information density.
- CHECK : Ensure visual integrity.
- EXPRESS : Choose proper visualization.
- SIMPLIFY : Avoid clutter.
- STRUCTURE : Organize content logically.
Let’s break down each component briefly:
- SAY : It’s all about making your key message unmistakably clear. Your audience should be able to grasp the main point at a glance. This involves using clear titles, highlighting key figures, and ensuring that the message is front and center.
- UNIFY : Consistency is key. This principle ensures that all visual elements (like colors, shapes, and fonts) are used consistently throughout your reports. This helps in creating a familiar look and feel, making it easier for readers to navigate and understand.
- CONDENSE : More information doesn’t necessarily mean more clutter. This principle focuses on presenting data in a compact and dense format, without overwhelming the reader. Think of using small multiples, sparklines, and condensed tables that pack a lot of information in a small space.
- CHECK : Accuracy and integrity are paramount. This involves verifying the data, ensuring that scales and labels are accurate, and avoiding any visual misrepresentations. It’s about being honest and precise with your visuals.
- EXPRESS : Choosing the right type of visualization for your data is crucial. This principle guides you on selecting the most effective chart types to convey your message clearly, whether it’s bar charts, line charts, scatter plots, or more advanced visualizations.
- SIMPLIFY : Less is more. Avoiding unnecessary elements and focusing on what’s important helps in reducing cognitive load on the reader. This means removing gridlines, reducing colors, and using white space effectively.
- STRUCTURE : Organize your content logically. This involves structuring your reports in a way that guides the reader through the data naturally. Sections, subsections, and a logical flow of information are essential here.
Clarity and Comprehension
I’ve been standardizing reports in my previous roles for quite some time. But I only came across IBCS recently, and let me tell you, I’m absolutely loving it as a framework. It has transformed the way I think about presenting data. Suddenly, my reports are not just a collection of numbers but a coherent story that my audience can easily understand and act upon. Each element of the SUCCESS formula plays a critical role in achieving this clarity.
Practical Steps to Implement Standardization
Alright, let’s get practical. How can you start standardizing your reports? Here’s a step-by-step guide that I’ve found incredibly useful:
- Evaluate Current Practices : Start by evaluating your current reporting practices. Identify inconsistencies and areas for improvement. Trust me, you’ll find plenty of “aha!” moments here.
- Educate and Train : Educate your team about the importance of standardization and the principles of IBCS. Knowledge is power, after all. Conduct workshops or training sessions to get everyone on the same page.
- Develop Templates and Tools : Develop standardized templates and tools that align with IBCS guidelines. This step is crucial for ensuring consistency across all reports. Tools like Quarto can be incredibly helpful here.
- Monitor and Collect Feedback : Regularly review your reports for compliance with the standards and gather feedback from users. Continuous improvement is the name of the game. Set up a feedback loop where users can suggest improvements and share their experiences.
Personal Experience in Implementation
In my previous role, we initiated a project to standardize our sales reports. Initially, there was some resistance — change is always hard. But after a few training sessions and some hands-on practice, the team started to see the benefits. The reports were not only easier to produce but also much more impactful. We even started receiving positive feedback from our clients who appreciated the clarity of our presentations.
Here’s a personal tip: Start small. Implement standardization in one type of report first. This approach allows you to refine the process and make adjustments before rolling it out across all reports.
Challenges and Solutions
Of course, it wasn’t all smooth sailing. We faced challenges like getting everyone to adopt the new standards and ensuring consistency across all reports. But with persistent effort and open communication, we overcame these hurdles. The key was to make everyone understand the long-term benefits of standardization.
One challenge we faced was with custom reports requested by different departments. These reports often deviated from the standard format. Our solution was to create a flexible template that allowed for some customization while still adhering to the core IBCS principles. This compromise ensured that the reports remained standardized but could still meet the specific needs of each department.
Types of Data Analysis
Before we dive deeper into reporting, let’s quickly touch on the different types of data analysis. Understanding these will help you tailor your reports to your specific needs.
Descriptive Analysis: The What
Descriptive analysis is all about summarizing past data to understand what happened. Think of it as the “what” of your data. It’s like looking at your car’s speedometer to see how fast you went. This type of analysis uses statistics like mean, median, and mode to describe the data.
For instance, if we look at the nycflights13 R dataset, a descriptive analysis might involve calculating the average delay time for flights, the total number of flights, or the distribution of flight delays across different months. This helps to paint a clear picture of historical performance.
Diagnostic Analysis: The Why
Diagnostic analysis moves us to the “why.” This type of analysis examines data to understand why something happened. It’s like figuring out why your car’s speed dropped suddenly — maybe there was a traffic jam? Diagnostic analysis involves looking at correlations and potential causal relationships to uncover the reasons behind certain trends or anomalies.
In the context of nycflights13, we might investigate why certain flights are delayed more frequently. This could involve examining variables like weather conditions, carrier performance, or airport congestion. Understanding these factors can help pinpoint the causes of delays.
Predictive Analysis: The What Might Happen
Predictive analysis uses statistical models and forecasting techniques to predict future outcomes based on historical data. It’s like forecasting whether you’ll hit traffic on your next trip based on past experiences. This type of analysis helps in anticipating future trends and making proactive decisions.
Using nycflights13, a predictive analysis might involve forecasting future flight delays based on historical delay patterns and upcoming weather forecasts. This can help airlines and passengers plan better and mitigate potential issues.
Prescriptive Analysis: The What Should We Do
Finally, prescriptive analysis provides recommendations for actions based on predictive analysis. It’s like your GPS suggesting an alternate route to avoid that predicted traffic jam. This type of analysis uses algorithms to suggest various courses of action and their potential outcomes.
For nycflights13, prescriptive analysis could recommend optimal flight schedules or routes to minimize delays. It might also suggest operational changes, like adjusting staffing levels during peak hours or implementing new maintenance protocols.
Reporting Delivery Platforms
Not all reports are created equal, and neither are the platforms we use to deliver them. Let’s break down the different platforms and how they impact standardization:
Interactive Dashboards
Interactive dashboards are dynamic and allow users to explore data in real-time. Standardization here ensures consistency across various views and interactions. Think of platforms like Power BI or Tableau. These dashboards are great for providing an overview and enabling detailed drill-downs.
Using the nycflights13 dataset, an interactive dashboard might include various widgets and filters that allow users to view flight performance by date, carrier, or destination. Ensuring that these elements are standardized makes the dashboard intuitive and user-friendly.
Presentations
Presentations are typically used for communicating key findings to stakeholders. Standardized slides enhance clarity and ensure that key messages are consistently communicated. PowerPoint or Google Slides are your friends here.
Imagine preparing a quarterly review using nycflights13 data. A standardized presentation template would include consistent slide layouts, color schemes, and fonts, making it easier for the audience to follow along and understand the insights.
Static Reports
Static reports provide a fixed snapshot of data. Standardization in static reports ensures that all necessary information is included and presented clearly. PDF reports or printed documents often fall into this category.
For example, a static report using nycflights13 data could be a detailed monthly performance report. Standardized headers, footers, and table formats ensure that the report is easy to read and understand.
How Different Types and Delivery Points Affect Standardization
Alright, let’s tie it all together. Different types of analysis and delivery platforms influence how you apply standardization:
- Descriptive Analysis on Dashboards : Ensure that interactive elements are standardized so users can easily compare past performance across different metrics.
- Diagnostic Analysis in Presentations : Use consistent visuals to explain why certain trends occurred. This helps stakeholders grasp the insights quickly.
- Predictive Analysis in Static Reports : Present forecasts in a standardized format to make it easier for readers to understand and trust the predictions.
- Prescriptive Analysis Across Platforms : Whether it’s a dashboard, presentation, or report, standardized recommendations ensure that the suggested actions are clear and actionable.
Tools for Standardizing Reports in R
In this chapter, we’ll discuss the tools I’ll be using in R to ensure our reports adhere to IBCS standards. Standardizing reports involves a combination of data manipulation, visualization, and documentation tools. Here are the main tools and packages we’ll be using throughout this series:
Data Manipulation with dplyr and tidyr
To start, we need robust tools for data manipulation. The dplyr and tidyr packages from the tidyverse suite are indispensable for cleaning, transforming, and organizing our data.
- dplyr : This package is perfect for data wrangling. With functions like select(), filter(), mutate(), summarize(), and arrange(), we can easily manipulate our data frames to get them into the right shape for analysis.
- tidyr : This package helps in tidying data, ensuring that it follows the tidy data principles. Functions like pivot_longer(), pivot_wider(), unite(), and separate() make it straightforward to reshape data as needed.
Data Visualization with ggplot2
Visualization is a cornerstone of effective reporting, and ggplot2 is the go-to package for creating high-quality graphics in R. It follows the grammar of graphics, which makes it highly flexible and powerful.
- Consistent Themes : We’ll use ggplot2's theming capabilities to apply consistent colors, fonts, and layouts across all our visualizations. This aligns with the UNIFY principle of IBCS.
- Custom Visuals : We’ll create custom visuals that not only look good but also convey the right message clearly, adhering to the EXPRESS principle.
Enhancing ggplot2 with Extensions
There are several extensions to ggplot2 that can help enhance its capabilities and ensure our visualizations are both informative and aesthetically pleasing:
- ggthemes : Provides additional themes and scales that help in standardizing the look and feel of plots.
- gghighlight : Allows us to highlight specific data points in a plot, making it easier to draw attention to key information.
- ggrepel : Helps in adding labels to plots without overlapping, ensuring that our visualizations remain clear and readable.
- patchwork : Facilitates the combination of multiple ggplot2 plots into a single cohesive layout, supporting the CONDENSE principle by increasing information density.
Reporting with Quarto
For generating and maintaining our reports, we’ll use Quarto, a new, powerful tool for creating dynamic documents in R.
- Dynamic Reports : Quarto allows for the integration of R code and markdown, enabling us to create reports that are both reproducible and interactive.
- Standardized Templates : We can create standardized templates that ensure consistency across all reports.
Table Formatting with kableExtra
Tables are a crucial part of any report, and kableExtra is an excellent package for creating well-formatted tables in R.
- Enhanced Tables : kableExtra provides functionality to produce beautiful tables within Quarto documents. It supports various table styling options, including striped rows, column alignment, and more.
- Interactive Tables : This package also supports the creation of interactive tables, making it easier for readers to explore data.
Supplementary Tools
- scales : This package works with ggplot2 to ensure that our scales are appropriately formatted, enhancing readability and accuracy.
- lubridate : Useful for handling date-time data, ensuring our time series data is properly formatted and easy to manipulate.
- stringr : Helps with string manipulation, making it easier to clean and prepare text data for reporting.
So, there you have it — a comprehensive introduction to the importance of standardization in business reporting and an overview of how IBCS can help you achieve it. In the next episodes, we’ll dive deep into each component of the SUCCESS formula, starting with SAY: Convey a Message . We’ll explore how to clearly and effectively communicate the main message in your reports, using practical examples and the nycflights13 dataset to illustrate these principles in action.
Remember, the goal here is to make your reports not just informative but also engaging and easy to understand. Let’s embark on this journey together and transform your business reporting skills!
Stay tuned, and happy reporting!
Introduction to Standardization in Business Reporting was originally published in Numbers around us on Medium, where people are continuing the conversation by highlighting and responding to this story.
Copyright © 2024 | MH Corporate basic by MH Themes
Never miss an update! Subscribe to R-bloggers to receive e-mails with the latest R posts. (You will not see this message again.)
Fueled by climate change, extreme wildfires have doubled in 20 years
A new study analyzing satellite data focused on extreme wildfires, which have severe consequences for humans and the planet.

The frequency and magnitude of extreme wildfires around the globe has doubled in the past two decades due to climate change, according to a study released Monday.
The analysis, published in the journal “Nature Ecology & Evolution ,” focused on massive blazes that release vast amounts of energy from the volume of organic matter burned. Researchers pointed to the historic Australia fires of 2019 and 2020 as an example of blazes that were “unprecedented in their scale and intensity.” The six most extreme fire years have occurred since 2017, the study found.
“It’s absolutely in keeping with what climate change is doing to fire weather around the world,” said lead author Calum Cunningham, a postdoctoral research fellow at the University of Tasmania in Australia. “Climate change is making fire weather more extreme and more frequent in a lot of the world.”
Though previous research found a decrease in the area burned globally by wildfires this century, the new study found that extreme wildfire events have increased 2.2-fold since 2003. Extreme wildfires have severe ecological and societal impacts, leading to deaths and biomass loss while emitting high levels of carbon. According to the study, burn severity, which is a measure of these impacts, has increased in more regions than it has decreased.
Cunningham said the research began as a response to climate skeptics who challenged whether there is a growing fire crisis if area burned globally is in decline. Despite the uptick in media coverage surrounding wildfires, he said there was not yet scientific literature to demonstrate that extreme events are changing.
“We’ve had this paradox where the amount of burning on Earth is declining … and yet we are having fires that are more extreme, more damaging,” said Stephen Pyne, fire historian and emeritus professor at Arizona State University. “How do we reconcile these two?”
Cunningham and his team analyzed data from orbiting NASA satellites, which collected four fire measurements per day over 21 years. The results astounded him.
“I was expecting to see increases, but the rate of the increases surprised and alarmed me because we’re only looking at quite a short period of time,” he said.
John Abatzoglou, a fire researcher at the University of California at Merced, said the focus on extreme fires is critical because they tend to supersede local fire mitigation efforts.
“When we have these hot, highly energetic fires, those are the sort of fires that are very difficult for fire suppression to have the ability to control and stop,” he said.
According to the study, as nighttime temperatures increase, wildfire intensity now continues to stay strong through overnight hours. This poses a problem for firefighters who rely on that window for a break in the blaze, retired firefighter and author Bobbie Scopa said.
“This kind of confirms what we’ve been observing, that the fire intensity is not dying down at night like we used to depend on,” said Scopa, who began firefighting in 1974.
“Rarely did we have 100,000-acre fires 20 years ago,” she added. “But now, it’s not uncommon.”
University of Colorado at Boulder scientist Jennifer Balch said firefighters today bear an undue burden, with a warming climate and tens of millions of U.S. homes facing wildfire risk.
Though the team will continue their research to investigate the scientific phenomena behind this trajectory, Cunningham said climate change has made fire weather hotter and drier, lending the right conditions for wildfires to erupt — even in environments where fires are infrequent.
Extreme wildfires have disproportionately increased in certain regions: North America, Australia, Oceania and the Mediterranean. Researchers especially saw wildfires increase in conifer and boreal forests, which are primarily in North America and Russia. In temperate conifer forests, extreme wildfires have increased 11-fold, accompanied by a sevenfold increase in boreal forests.
“Climate change is not something off in the future,” Cunningham said. “It’s happening before our very eyes. This is the manifestation of the reshaping of the climate we are doing.”

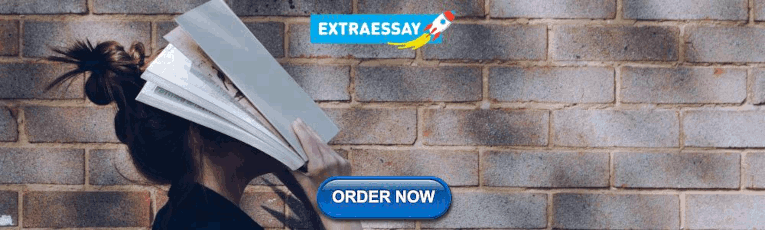
IMAGES
VIDEO
COMMENTS
Visualizing weather data in R allows you to uncover trends, patterns, and anomalies in meteorological data. By leveraging packages like ggplot2, Plotly, and dygraphs, you can create both static and interactive visualizations that provide valuable insights. ... Data Visualization with Pandas is the presentation of data in a graphical format. It ...
Weather and Climate Toolkit. NOAA's Weather and Climate Toolkit (WCT) is free, platform independent software. The WCT allows the visualization and data export of weather and climate data, including Radar, Satellite and Model data. The WCT also provides access to weather/climate web services provided by NCDC and other organizations.
NOAA's Geoplatform provides geospatial data, maps, and analytics in support of NOAA's mission through a GIS application using Esri's ArcGIS Online. Maps, Layers, Scenes, Apps and StoryMaps are available to the public for browsing. The Maps & Data section featuring interactive tools, maps, and additional tools for accessing climate data.
GIS Data Portal; NOAA Weather Radio; Publications; SKYWARN Storm Spotters; ... Weather.gov > NWS Education > Meteorology 101 presentation JetStream. Students. Citizen Science ... US Dept of Commerce National Oceanic and Atmospheric Administration National Weather Service NWS Education 1325 East West Highway Silver Spring, MD 20910
From Raw Data to Informative Products. The most common processing steps used to turn daily weather data into climate data products involve straightforward mathematics. Operations such as adding and averaging turn weather data into climate information. For instance, a station's daily average (mean) temperature is calculated by averaging the ...
Lesson 1 (1 day): The Water Cycle. Lesson 2 (1 day): The Greenhouse Effect. Lessons 3 and 4: Measuring and Analyzing Weather and Climate Data (Precipitation and Temperature) Lesson 5: Mitigating and Adapting to Weather and Climate Extremes in Agriculture and Natural Resources. Rain gauge, Rio Mora National Wildlife Refuge, New Mexico.
Professional Development. Research Institute. Professional Sections. Tools & Resources. About SOA. This report categorizes weather datasets into 8 main types, of which 5 are historical data and 3 are forecast data. Each of the 8 dataset types is described, and an illustrative example of each is provided.
Draw the new text box and click DataPoint, Text box again. Set the data connection to Yahoo Weather | Condition and select the Text column from the columns list. This will display the current weather condition like cloudy and sunny in the text box. Click OK to close. Now add a third text and click DataPoint, Text box.
The Climate Watch Slide Deck is a PPT deck of 27 curated slides providing answers to the most common climate change questions with data, visualizations and explanations. Preview of Climate Watch Slide Deck. Click the image or button below to access the current slides. We update the slides every two weeks and even sooner if major countries ...
Would you like to give PowerPoint Presentations that always show the latest weather data without having to manually reload datasets? This video demonstrates ...
Share weather data for agricultural presentation topics, environmental studies, or any kind of presentation that requires a highly visual layout for weather forecasting on a single day. Two color themes are available. Use This Template. 9. Social Media Marketing Dashboard Data Presentation Template.
Forecasting Tomorrow's Weather. Now the regression model has been trained on past weather data, the exciting part begins! — making predictions on new, unseen data. Let's walk through the process of transforming our model into a weather oracle using a real-world example. Illustrating the Prediction Process: Preparing New Data:
Presentation on theme: "Gathering Weather Data"— Presentation transcript: 1 Gathering Weather Data Section 12.3. 2 Data From Earth's Surface Meteorologists measure ... Began in 1990's 24/7 weather data Provides info for aviation, weather forecasting, and weather research.
Download Full Presentation (PPT, 148MB) Updated: April 2021. Climate Central is presenting a new outreach and education resource for meteorologists, journalists, and others—a climate change ...
DataPoint is a PowerPoint add-in that allows you to display information in real-time from databases and other data stores. Weather APIs and weather sources are included via XML, JSON, and dedicated providers. DataPoint can connect to free weather APIs and is even equipped with a professional weather provider for free.
Ads. What Is Climate? Presentation. Free Google Slides theme, PowerPoint template, and Canva presentation template. Climate refers to the long-term patterns of temperature, rainfall, humidity, wind, and other atmospheric conditions in a region. It's essentially the overall "weather" of a place, averaged over a long period of time.
Find historical weather data for any location and date. Compare past and present conditions with radar, satellite and Wundermap.
To do this, we right-click on the chart and choose 'Edit Data'. As we did before we will open the full Excel editor. Once opened in Excel, you should see your weather data as before. Now choose the 'Data' menu and 'Connection Properties…'. In this menu, you can select the refresh policy that is your preferred style.
In this webinar recording, PresentationPoint partner Kurt Dupont shows how to set up a Live Weather display using PowerPoint and our PowerPoint add-ons, DataPoint, or Dynamic Weather. Have a look at this exclusive and in-depth webinar about showing real-time and live weather conditions and forecasts on a dedicated television screen at your ...
Of course, the real-time weather information that we provide, starts with the observation of the current weather data. DataPoint refreshes the weather data every 10 minutes, while Dynamic Weather is checking for new information every 30 minutes. You can collect weather data of as many locations as you want. The data you get, are: Current ...
Look for this dialog and add the URL for the weather information page that you want to embed. From weather.com you have many options. For example you can use the Weather API that has a monthly fee or you can embed a webpage. If you want to embed the full web page, look for a city weather like New York weather and then copy the URL and paste it ...
Weather Data and Climate. Weather changes from day to day… Climate does not change!. Reading Weather Maps. 3 Major Climate Zones. Polar Zone Near Earth's North & South Poles Tropical Zone Near equator Temperate Zone - between polar & tropical zones. EOG Review.
You understand and consent to the following: you may access this information system for authorized use only; unauthorized use of the system is prohibited and subject to criminal and civil penalties; you have no reasonable expectation of privacy regarding any communication or data transiting or stored on this information system at any time and ...
Click the Graph icon to preview a graph of the data. (Note: Preview graphs show data in tenths of degrees Celsius. Click the View/Download icon to download a PDF document of the data. 6) If you want to download a station's data in another format: Check the box to the left of its name, and then click the Add to Cart button
The ocean is warming. Rising greenhouse gas concentrations not only warm the air, but the ocean, too. Research shows that around 90 percent of the excess heat from global warming is being absorbed by the ocean. Ocean heat has steadily risen since measurements began in 1955, breaking records in 2023.All this added heat has led to more frequent and intense marine heat waves.
Here's a line you can use at the pool or beach this weekend: "Yep, it's climate change.. The deadly heat wave that scorched much of North America in early May and early June - and is still ...
For even more on the presentation elements and how they come to life, ... The Development Team meticulously examined hundreds of thousands of data points to arrive at our team power rankings. With help from our friends at Pro Football Focus (PFF), the team analyzed all 134 rosters, thousands of players, years worth of game film, and mountains ...
You can track the latest information and forecasts on Beryl here. - Articles from The Weather Channel | weather.com
This could involve examining variables like weather conditions, carrier performance, or airport congestion. ... PowerPoint or Google Slides are your friends here.Imagine preparing a quarterly review using nycflights13 data. A standardized presentation template would include consistent slide layouts, color schemes, and fonts, making it easier ...
A new study analyzing satellite data focused on extreme wildfires, which have severe consequences for humans and the planet. By Sarah Raza June 24, 2024 at 11:00 a.m. EDT