- Search Menu
- Browse content in Arts and Humanities
- Browse content in Archaeology
- Anglo-Saxon and Medieval Archaeology
- Archaeological Methodology and Techniques
- Archaeology by Region
- Archaeology of Religion
- Archaeology of Trade and Exchange
- Biblical Archaeology
- Contemporary and Public Archaeology
- Environmental Archaeology
- Historical Archaeology
- History and Theory of Archaeology
- Industrial Archaeology
- Landscape Archaeology
- Mortuary Archaeology
- Prehistoric Archaeology
- Underwater Archaeology
- Urban Archaeology
- Zooarchaeology
- Browse content in Architecture
- Architectural Structure and Design
- History of Architecture
- Residential and Domestic Buildings
- Theory of Architecture
- Browse content in Art
- Art Subjects and Themes
- History of Art
- Industrial and Commercial Art
- Theory of Art
- Biographical Studies
- Byzantine Studies
- Browse content in Classical Studies
- Classical Literature
- Classical Reception
- Classical History
- Classical Philosophy
- Classical Mythology
- Classical Art and Architecture
- Classical Oratory and Rhetoric
- Greek and Roman Archaeology
- Greek and Roman Papyrology
- Greek and Roman Epigraphy
- Greek and Roman Law
- Late Antiquity
- Religion in the Ancient World
- Digital Humanities
- Browse content in History
- Colonialism and Imperialism
- Diplomatic History
- Environmental History
- Genealogy, Heraldry, Names, and Honours
- Genocide and Ethnic Cleansing
- Historical Geography
- History by Period
- History of Agriculture
- History of Education
- History of Emotions
- History of Gender and Sexuality
- Industrial History
- Intellectual History
- International History
- Labour History
- Legal and Constitutional History
- Local and Family History
- Maritime History
- Military History
- National Liberation and Post-Colonialism
- Oral History
- Political History
- Public History
- Regional and National History
- Revolutions and Rebellions
- Slavery and Abolition of Slavery
- Social and Cultural History
- Theory, Methods, and Historiography
- Urban History
- World History
- Browse content in Language Teaching and Learning
- Language Learning (Specific Skills)
- Language Teaching Theory and Methods
- Browse content in Linguistics
- Applied Linguistics
- Cognitive Linguistics
- Computational Linguistics
- Forensic Linguistics
- Grammar, Syntax and Morphology
- Historical and Diachronic Linguistics
- History of English
- Language Variation
- Language Families
- Language Evolution
- Language Reference
- Language Acquisition
- Lexicography
- Linguistic Theories
- Linguistic Typology
- Linguistic Anthropology
- Phonetics and Phonology
- Psycholinguistics
- Sociolinguistics
- Translation and Interpretation
- Writing Systems
- Browse content in Literature
- Bibliography
- Children's Literature Studies
- Literary Studies (Modernism)
- Literary Studies (Romanticism)
- Literary Studies (American)
- Literary Studies (Asian)
- Literary Studies (European)
- Literary Studies (Eco-criticism)
- Literary Studies - World
- Literary Studies (1500 to 1800)
- Literary Studies (19th Century)
- Literary Studies (20th Century onwards)
- Literary Studies (African American Literature)
- Literary Studies (British and Irish)
- Literary Studies (Early and Medieval)
- Literary Studies (Fiction, Novelists, and Prose Writers)
- Literary Studies (Gender Studies)
- Literary Studies (Graphic Novels)
- Literary Studies (History of the Book)
- Literary Studies (Plays and Playwrights)
- Literary Studies (Poetry and Poets)
- Literary Studies (Postcolonial Literature)
- Literary Studies (Queer Studies)
- Literary Studies (Science Fiction)
- Literary Studies (Travel Literature)
- Literary Studies (War Literature)
- Literary Studies (Women's Writing)
- Literary Theory and Cultural Studies
- Mythology and Folklore
- Shakespeare Studies and Criticism
- Browse content in Media Studies
- Browse content in Music
- Applied Music
- Dance and Music
- Ethics in Music
- Ethnomusicology
- Gender and Sexuality in Music
- Medicine and Music
- Music Cultures
- Music and Culture
- Music and Media
- Music and Religion
- Music Education and Pedagogy
- Music Theory and Analysis
- Musical Scores, Lyrics, and Libretti
- Musical Structures, Styles, and Techniques
- Musicology and Music History
- Performance Practice and Studies
- Race and Ethnicity in Music
- Sound Studies
- Browse content in Performing Arts
- Browse content in Philosophy
- Aesthetics and Philosophy of Art
- Epistemology
- Feminist Philosophy
- History of Western Philosophy
- Metaphysics
- Moral Philosophy
- Non-Western Philosophy
- Philosophy of Action
- Philosophy of Law
- Philosophy of Religion
- Philosophy of Language
- Philosophy of Mind
- Philosophy of Perception
- Philosophy of Science
- Philosophy of Mathematics and Logic
- Practical Ethics
- Social and Political Philosophy
- Browse content in Religion
- Biblical Studies
- Christianity
- East Asian Religions
- History of Religion
- Judaism and Jewish Studies
- Qumran Studies
- Religion and Education
- Religion and Health
- Religion and Politics
- Religion and Science
- Religion and Law
- Religion and Art, Literature, and Music
- Religious Studies
- Browse content in Society and Culture
- Cookery, Food, and Drink
- Cultural Studies
- Customs and Traditions
- Ethical Issues and Debates
- Hobbies, Games, Arts and Crafts
- Lifestyle, Home, and Garden
- Natural world, Country Life, and Pets
- Popular Beliefs and Controversial Knowledge
- Sports and Outdoor Recreation
- Technology and Society
- Travel and Holiday
- Visual Culture
- Browse content in Law
- Arbitration
- Browse content in Company and Commercial Law
- Commercial Law
- Company Law
- Browse content in Comparative Law
- Systems of Law
- Competition Law
- Browse content in Constitutional and Administrative Law
- Government Powers
- Judicial Review
- Local Government Law
- Military and Defence Law
- Parliamentary and Legislative Practice
- Construction Law
- Contract Law
- Browse content in Criminal Law
- Criminal Procedure
- Criminal Evidence Law
- Sentencing and Punishment
- Employment and Labour Law
- Environment and Energy Law
- Browse content in Financial Law
- Banking Law
- Insolvency Law
- History of Law
- Human Rights and Immigration
- Intellectual Property Law
- Browse content in International Law
- Private International Law and Conflict of Laws
- Public International Law
- IT and Communications Law
- Jurisprudence and Philosophy of Law
- Law and Society
- Law and Politics
- Browse content in Legal System and Practice
- Courts and Procedure
- Legal Skills and Practice
- Primary Sources of Law
- Regulation of Legal Profession
- Medical and Healthcare Law
- Browse content in Policing
- Criminal Investigation and Detection
- Police and Security Services
- Police Procedure and Law
- Police Regional Planning
- Browse content in Property Law
- Personal Property Law
- Study and Revision
- Terrorism and National Security Law
- Browse content in Trusts Law
- Wills and Probate or Succession
- Browse content in Medicine and Health
- Browse content in Allied Health Professions
- Arts Therapies
- Clinical Science
- Dietetics and Nutrition
- Occupational Therapy
- Operating Department Practice
- Physiotherapy
- Radiography
- Speech and Language Therapy
- Browse content in Anaesthetics
- General Anaesthesia
- Neuroanaesthesia
- Clinical Neuroscience
- Browse content in Clinical Medicine
- Acute Medicine
- Cardiovascular Medicine
- Clinical Genetics
- Clinical Pharmacology and Therapeutics
- Dermatology
- Endocrinology and Diabetes
- Gastroenterology
- Genito-urinary Medicine
- Geriatric Medicine
- Infectious Diseases
- Medical Oncology
- Medical Toxicology
- Pain Medicine
- Palliative Medicine
- Rehabilitation Medicine
- Respiratory Medicine and Pulmonology
- Rheumatology
- Sleep Medicine
- Sports and Exercise Medicine
- Community Medical Services
- Critical Care
- Emergency Medicine
- Forensic Medicine
- Haematology
- History of Medicine
- Medical Ethics
- Browse content in Medical Skills
- Clinical Skills
- Communication Skills
- Nursing Skills
- Surgical Skills
- Browse content in Medical Dentistry
- Oral and Maxillofacial Surgery
- Paediatric Dentistry
- Restorative Dentistry and Orthodontics
- Surgical Dentistry
- Medical Statistics and Methodology
- Browse content in Neurology
- Clinical Neurophysiology
- Neuropathology
- Nursing Studies
- Browse content in Obstetrics and Gynaecology
- Gynaecology
- Occupational Medicine
- Ophthalmology
- Otolaryngology (ENT)
- Browse content in Paediatrics
- Neonatology
- Browse content in Pathology
- Chemical Pathology
- Clinical Cytogenetics and Molecular Genetics
- Histopathology
- Medical Microbiology and Virology
- Patient Education and Information
- Browse content in Pharmacology
- Psychopharmacology
- Browse content in Popular Health
- Caring for Others
- Complementary and Alternative Medicine
- Self-help and Personal Development
- Browse content in Preclinical Medicine
- Cell Biology
- Molecular Biology and Genetics
- Reproduction, Growth and Development
- Primary Care
- Professional Development in Medicine
- Browse content in Psychiatry
- Addiction Medicine
- Child and Adolescent Psychiatry
- Forensic Psychiatry
- Learning Disabilities
- Old Age Psychiatry
- Psychotherapy
- Browse content in Public Health and Epidemiology
- Epidemiology
- Public Health
- Browse content in Radiology
- Clinical Radiology
- Interventional Radiology
- Nuclear Medicine
- Radiation Oncology
- Reproductive Medicine
- Browse content in Surgery
- Cardiothoracic Surgery
- Gastro-intestinal and Colorectal Surgery
- General Surgery
- Neurosurgery
- Paediatric Surgery
- Peri-operative Care
- Plastic and Reconstructive Surgery
- Surgical Oncology
- Transplant Surgery
- Trauma and Orthopaedic Surgery
- Vascular Surgery
- Browse content in Science and Mathematics
- Browse content in Biological Sciences
- Aquatic Biology
- Biochemistry
- Bioinformatics and Computational Biology
- Developmental Biology
- Ecology and Conservation
- Evolutionary Biology
- Genetics and Genomics
- Microbiology
- Molecular and Cell Biology
- Natural History
- Plant Sciences and Forestry
- Research Methods in Life Sciences
- Structural Biology
- Systems Biology
- Zoology and Animal Sciences
- Browse content in Chemistry
- Analytical Chemistry
- Computational Chemistry
- Crystallography
- Environmental Chemistry
- Industrial Chemistry
- Inorganic Chemistry
- Materials Chemistry
- Medicinal Chemistry
- Mineralogy and Gems
- Organic Chemistry
- Physical Chemistry
- Polymer Chemistry
- Study and Communication Skills in Chemistry
- Theoretical Chemistry
- Browse content in Computer Science
- Artificial Intelligence
- Computer Architecture and Logic Design
- Game Studies
- Human-Computer Interaction
- Mathematical Theory of Computation
- Programming Languages
- Software Engineering
- Systems Analysis and Design
- Virtual Reality
- Browse content in Computing
- Business Applications
- Computer Games
- Computer Security
- Computer Networking and Communications
- Digital Lifestyle
- Graphical and Digital Media Applications
- Operating Systems
- Browse content in Earth Sciences and Geography
- Atmospheric Sciences
- Environmental Geography
- Geology and the Lithosphere
- Maps and Map-making
- Meteorology and Climatology
- Oceanography and Hydrology
- Palaeontology
- Physical Geography and Topography
- Regional Geography
- Soil Science
- Urban Geography
- Browse content in Engineering and Technology
- Agriculture and Farming
- Biological Engineering
- Civil Engineering, Surveying, and Building
- Electronics and Communications Engineering
- Energy Technology
- Engineering (General)
- Environmental Science, Engineering, and Technology
- History of Engineering and Technology
- Mechanical Engineering and Materials
- Technology of Industrial Chemistry
- Transport Technology and Trades
- Browse content in Environmental Science
- Applied Ecology (Environmental Science)
- Conservation of the Environment (Environmental Science)
- Environmental Sustainability
- Environmentalist Thought and Ideology (Environmental Science)
- Management of Land and Natural Resources (Environmental Science)
- Natural Disasters (Environmental Science)
- Nuclear Issues (Environmental Science)
- Pollution and Threats to the Environment (Environmental Science)
- Social Impact of Environmental Issues (Environmental Science)
- History of Science and Technology
- Browse content in Materials Science
- Ceramics and Glasses
- Composite Materials
- Metals, Alloying, and Corrosion
- Nanotechnology
- Browse content in Mathematics
- Applied Mathematics
- Biomathematics and Statistics
- History of Mathematics
- Mathematical Education
- Mathematical Finance
- Mathematical Analysis
- Numerical and Computational Mathematics
- Probability and Statistics
- Pure Mathematics
- Browse content in Neuroscience
- Cognition and Behavioural Neuroscience
- Development of the Nervous System
- Disorders of the Nervous System
- History of Neuroscience
- Invertebrate Neurobiology
- Molecular and Cellular Systems
- Neuroendocrinology and Autonomic Nervous System
- Neuroscientific Techniques
- Sensory and Motor Systems
- Browse content in Physics
- Astronomy and Astrophysics
- Atomic, Molecular, and Optical Physics
- Biological and Medical Physics
- Classical Mechanics
- Computational Physics
- Condensed Matter Physics
- Electromagnetism, Optics, and Acoustics
- History of Physics
- Mathematical and Statistical Physics
- Measurement Science
- Nuclear Physics
- Particles and Fields
- Plasma Physics
- Quantum Physics
- Relativity and Gravitation
- Semiconductor and Mesoscopic Physics
- Browse content in Psychology
- Affective Sciences
- Clinical Psychology
- Cognitive Neuroscience
- Cognitive Psychology
- Criminal and Forensic Psychology
- Developmental Psychology
- Educational Psychology
- Evolutionary Psychology
- Health Psychology
- History and Systems in Psychology
- Music Psychology
- Neuropsychology
- Organizational Psychology
- Psychological Assessment and Testing
- Psychology of Human-Technology Interaction
- Psychology Professional Development and Training
- Research Methods in Psychology
- Social Psychology
- Browse content in Social Sciences
- Browse content in Anthropology
- Anthropology of Religion
- Human Evolution
- Medical Anthropology
- Physical Anthropology
- Regional Anthropology
- Social and Cultural Anthropology
- Theory and Practice of Anthropology
- Browse content in Business and Management
- Business History
- Business Ethics
- Business Strategy
- Business and Technology
- Business and Government
- Business and the Environment
- Comparative Management
- Corporate Governance
- Corporate Social Responsibility
- Entrepreneurship
- Health Management
- Human Resource Management
- Industrial and Employment Relations
- Industry Studies
- Information and Communication Technologies
- International Business
- Knowledge Management
- Management and Management Techniques
- Operations Management
- Organizational Theory and Behaviour
- Pensions and Pension Management
- Public and Nonprofit Management
- Strategic Management
- Supply Chain Management
- Browse content in Criminology and Criminal Justice
- Criminal Justice
- Criminology
- Forms of Crime
- International and Comparative Criminology
- Youth Violence and Juvenile Justice
- Development Studies
- Browse content in Economics
- Agricultural, Environmental, and Natural Resource Economics
- Asian Economics
- Behavioural Finance
- Behavioural Economics and Neuroeconomics
- Econometrics and Mathematical Economics
- Economic Methodology
- Economic History
- Economic Systems
- Economic Development and Growth
- Financial Markets
- Financial Institutions and Services
- General Economics and Teaching
- Health, Education, and Welfare
- History of Economic Thought
- International Economics
- Labour and Demographic Economics
- Law and Economics
- Macroeconomics and Monetary Economics
- Microeconomics
- Public Economics
- Urban, Rural, and Regional Economics
- Welfare Economics
- Browse content in Education
- Adult Education and Continuous Learning
- Care and Counselling of Students
- Early Childhood and Elementary Education
- Educational Equipment and Technology
- Educational Strategies and Policy
- Higher and Further Education
- Organization and Management of Education
- Philosophy and Theory of Education
- Schools Studies
- Secondary Education
- Teaching of a Specific Subject
- Teaching of Specific Groups and Special Educational Needs
- Teaching Skills and Techniques
- Browse content in Environment
- Applied Ecology (Social Science)
- Climate Change
- Conservation of the Environment (Social Science)
- Environmentalist Thought and Ideology (Social Science)
- Natural Disasters (Environment)
- Social Impact of Environmental Issues (Social Science)
- Browse content in Human Geography
- Cultural Geography
- Economic Geography
- Political Geography
- Browse content in Interdisciplinary Studies
- Communication Studies
- Museums, Libraries, and Information Sciences
- Browse content in Politics
- African Politics
- Asian Politics
- Chinese Politics
- Comparative Politics
- Conflict Politics
- Elections and Electoral Studies
- Environmental Politics
- European Union
- Foreign Policy
- Gender and Politics
- Human Rights and Politics
- Indian Politics
- International Relations
- International Organization (Politics)
- International Political Economy
- Irish Politics
- Latin American Politics
- Middle Eastern Politics
- Political Theory
- Political Behaviour
- Political Economy
- Political Institutions
- Political Methodology
- Political Communication
- Political Philosophy
- Political Sociology
- Politics and Law
- Public Policy
- Public Administration
- Quantitative Political Methodology
- Regional Political Studies
- Russian Politics
- Security Studies
- State and Local Government
- UK Politics
- US Politics
- Browse content in Regional and Area Studies
- African Studies
- Asian Studies
- East Asian Studies
- Japanese Studies
- Latin American Studies
- Middle Eastern Studies
- Native American Studies
- Scottish Studies
- Browse content in Research and Information
- Research Methods
- Browse content in Social Work
- Addictions and Substance Misuse
- Adoption and Fostering
- Care of the Elderly
- Child and Adolescent Social Work
- Couple and Family Social Work
- Developmental and Physical Disabilities Social Work
- Direct Practice and Clinical Social Work
- Emergency Services
- Human Behaviour and the Social Environment
- International and Global Issues in Social Work
- Mental and Behavioural Health
- Social Justice and Human Rights
- Social Policy and Advocacy
- Social Work and Crime and Justice
- Social Work Macro Practice
- Social Work Practice Settings
- Social Work Research and Evidence-based Practice
- Welfare and Benefit Systems
- Browse content in Sociology
- Childhood Studies
- Community Development
- Comparative and Historical Sociology
- Economic Sociology
- Gender and Sexuality
- Gerontology and Ageing
- Health, Illness, and Medicine
- Marriage and the Family
- Migration Studies
- Occupations, Professions, and Work
- Organizations
- Population and Demography
- Race and Ethnicity
- Social Theory
- Social Movements and Social Change
- Social Research and Statistics
- Social Stratification, Inequality, and Mobility
- Sociology of Religion
- Sociology of Education
- Sport and Leisure
- Urban and Rural Studies
- Browse content in Warfare and Defence
- Defence Strategy, Planning, and Research
- Land Forces and Warfare
- Military Administration
- Military Life and Institutions
- Naval Forces and Warfare
- Other Warfare and Defence Issues
- Peace Studies and Conflict Resolution
- Weapons and Equipment

- < Previous chapter
- Next chapter >
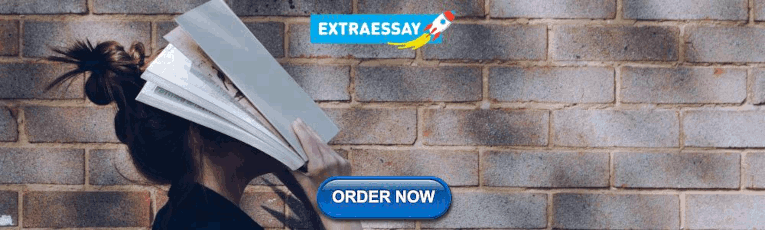
28 Case Selection for Case‐Study Analysis: Qualitative and Quantitative Techniques
John Gerring is Professor of Political Science, Boston University.
- Published: 02 September 2009
- Cite Icon Cite
- Permissions Icon Permissions
This article presents some guidance by cataloging nine different techniques for case selection: typical, diverse, extreme, deviant, influential, crucial, pathway, most similar, and most different. It also indicates that if the researcher is starting from a quantitative database, then methods for finding influential outliers can be used. In particular, the article clarifies the general principles that might guide the process of case selection in case-study research. Cases are more or less representative of some broader phenomenon and, on that score, may be considered better or worse subjects for intensive analysis. The article then draws attention to two ambiguities in case-selection strategies in case-study research. The first concerns the admixture of several case-selection strategies. The second concerns the changing status of a case as a study proceeds. Some case studies follow only one strategy of case selection.
Case ‐study analysis focuses on one or several cases that are expected to provide insight into a larger population. This presents the researcher with a formidable problem of case selection: Which cases should she or he choose?
In large‐sample research, the task of case selection is usually handled by some version of randomization. However, in case‐study research the sample is small (by definition) and this makes random sampling problematic, for any given sample may be wildly unrepresentative. Moreover, there is no guarantee that a few cases, chosen randomly, will provide leverage into the research question of interest.
In order to isolate a sample of cases that both reproduces the relevant causal features of a larger universe (representativeness) and provides variation along the dimensions of theoretical interest (causal leverage), case selection for very small samples must employ purposive (nonrandom) selection procedures. Nine such methods are discussed in this chapter, each of which may be identified with a distinct case‐study “type:” typical, diverse, extreme, deviant, influential, crucial, pathway, most‐similar , and most‐different . Table 28.1 summarizes each type, including its general definition, a technique for locating it within a population of potential cases, its uses, and its probable representativeness.
While each of these techniques is normally practiced on one or several cases (the diverse, most‐similar, and most‐different methods require at least two), all may employ additional cases—with the proviso that, at some point, they will no longer offer an opportunity for in‐depth analysis and will thus no longer be “case studies” in the usual sense ( Gerring 2007 , ch. 2 ). It will also be seen that small‐ N case‐selection procedures rest, at least implicitly, upon an analysis of a larger population of potential cases (as does randomization). The case(s) identified for intensive study is chosen from a population and the reasons for this choice hinge upon the way in which it is situated within that population. This is the origin of the terminology—typical, diverse, extreme, et al. It follows that case‐selection procedures in case‐study research may build upon prior cross‐case analysis and that they depend, at the very least, upon certain assumptions about the broader population.
In certain circumstances, the case‐selection procedure may be structured by a quantitative analysis of the larger population. Here, several caveats must be satisfied. First, the inference must pertain to more than a few dozen cases; otherwise, statistical analysis is problematic. Second, relevant data must be available for that population, or a significant sample of that population, on key variables, and the researcher must feel reasonably confident in the accuracy and conceptual validity of these variables. Third, all the standard assumptions of statistical research (e.g. identification, specification, robustness) must be carefully considered, and wherever possible, tested. I shall not dilate further on these familiar issues except to warn the researcher against the unreflective use of statistical techniques. 1 When these requirements are not met, the researcher must employ a qualitative approach to case selection.
The point of this chapter is to elucidate general principles that might guide the process of case selection in case‐study research, building upon earlier work by Harry Eckstein, Arend Lijphart, and others. Sometimes, these principles can be applied in a quantitative framework and sometimes they are limited to a qualitative framework. In either case, the logic of case selection remains quite similar, whether practiced in small‐ N or large‐ N contexts.
Before we begin, a bit of notation is necessary. In this chapter “ N ” refers to cases, not observations. Here, I am concerned primarily with causal inference, rather than inferences that are descriptive or predictive in nature. Thus, all hypotheses involve at least one independent variable ( X ) and one dependent variable ( Y ). For convenience, I shall label the causal factor of special theoretical interest X 1 , and the control variable, or vector of controls (if there are any), X 2 . If the writer is concerned to explain a puzzling outcome, but has no preconceptions about its causes, then the research will be described as Y‐centered . If a researcher is concerned to investigate the effects of a particular cause, with no preconceptions about what these effects might be, the research will be described as X‐centered . If a researcher is concerned to investigate a particular causal relationship, the research will be described as X 1 / Y‐centered , for it connects a particular cause with a particular outcome. 2 X ‐ or Y ‐centered research is exploratory; its purpose is to generate new hypotheses. X 1 / Y‐centered research, by contrast, is confirmatory/disconfirmatory; its purpose is to test an existing hypothesis.
1 Typical Case
In order for a focused case study to provide insight into a broader phenomenon it must be representative of a broader set of cases. It is in this context that one may speak of a typical‐case approach to case selection. The typical case exemplifies what is considered to be a typical set of values, given some general understanding of a phenomenon. By construction, the typical case is also a representative case.
Some typical cases serve an exploratory role. Here, the author chooses a case based upon a set of descriptive characteristics and then probes for causal relationships. Robert and Helen Lynd (1929/1956) selected a single city “to be as representative as possible of contemporary American life.” Specifically, they were looking for a city with
1) a temperate climate; 2) a sufficiently rapid rate of growth to ensure the presence of a plentiful assortment of the growing pains accompanying contemporary social change; 3) an industrial culture with modern, high‐speed machine production; 4) the absence of dominance of the city's industry by a single plant (i.e., not a one‐industry town); 5) a substantial local artistic life to balance its industrial activity …; and 6) the absence of any outstanding peculiarities or acute local problems which would mark the city off from the midchannel sort of American community. ( Lynd and Lynd 1929/1956 , quoted in Yin 2004 , 29–30)
After examining a number of options the Lynds decided that Muncie, Indiana, was more representative than, or at least as representative as, other midsized cities in America, thus qualifying as a typical case.
This is an inductive approach to case selection. Note that typicality may be understood according to the mean, median, or mode on a particular dimension; there may be multiple dimensions (as in the foregoing example); and each may be differently weighted (some dimensions may be more important than others). Where the selection criteria are multidimensional and a large sample of potential cases is in play, some form of factor analysis may be useful in identifying the most‐typical case(s).
However, the more common employment of the typical‐case method involves a causal model of some phenomenon of theoretical interest. Here, the researcher has identified a particular outcome ( Y ), and perhaps a specific X 1 / Y hypothesis, which she wishes to investigate. In order to do so, she looks for a typical example of that causal relationship. Intuitively, one imagines that a case selected according to the mean values of all parameters must be a typical case relative to some causal relationship. However, this is by no means assured.
Suppose that the Lynds were primarily interested in explaining feelings of trust/distrust among members of different social classes (one of the implicit research goals of the Middletown study). This outcome is likely to be affected by many factors, only some of which are included in their six selection criteria. So choosing cases with respect to a causal hypothesis involves, first of all, identifying the relevant parameters. It involves, secondly, the selection of a case that has a “typical” value relative to the overall causal model; it is well explained. Cases with untypical scores on a particular dimension (e.g. very high or very low) may still be typical examples of a causal relationship. Indeed, they may be more typical than cases whose values lie close to the mean. Thus, a descriptive understanding of typicality is quite different from a causal understanding of typicality. Since it is the latter version that is more common, I shall adopt this understanding of typicality in the remainder of the discussion.
From a qualitative perspective, causal typicality involves the selection of a case that conforms to expectations about some general causal relationship. It performs as expected. In a quantitative setting, this notion is measured by the size of a case's residual in a large‐ N cross‐case model. Typical cases lie on or near the regression line; their residuals are small. Insofar as the model is correctly specified, the size of a case's residual (i.e. the number of standard deviations that separate the actual value from the fitted value) provides a helpful clue to how representative that case is likely to be. “Outliers” are unlikely to be representative of the target population.
Of course, just because a case has a low residual does not necessarily mean that it is a representative case (with respect to the causal relationship of interest). Indeed, the issue of case representativeness is an issue that can never be definitively settled. When one refers to a “typical case” one is saying, in effect, that the probability of a case's representativeness is high, relative to other cases. This test of typicality is misleading if the statistical model is mis‐specified. And it provides little insurance against errors that are purely stochastic. A case may lie directly on the regression line but still be, in some important respect, atypical. For example, it might have an odd combination of values; the interaction of variables might be different from other cases; or additional causal mechanisms might be at work. For this reason, it is important to supplement a statistical analysis of cases with evidence drawn from the case in question (the case study itself) and with our deductive knowledge of the world. One should never judge a case solely by its residual. Yet, all other things being equal, a case with a low residual is less likely to be unusual than a case with a high residual, and to this extent the method of case selection outlined here may be a helpful guide to case‐study researchers faced with a large number of potential cases.
By way of conclusion, it should be noted that because the typical case embodies a typical value on some set of causally relevant dimensions, the variance of interest to the researcher must lie within that case. Specifically, the typical case of some phenomenon may be helpful in exploring causal mechanisms and in solving identification problems (e.g. endogeneity between X 1 and Y , an omitted variable that may account for X 1 and Y , or some other spurious causal association). Depending upon the results of the case study, the author may confirm an existing hypothesis, disconfirm that hypothesis, or reframe it in a way that is consistent with the findings of the case study. These are the uses of the typical‐case study.
2 Diverse Cases
A second case‐selection strategy has as its primary objective the achievement of maximum variance along relevant dimensions. I refer to this as a diverse‐case method. For obvious reasons, this method requires the selection of a set of cases—at minimum, two—which are intended to represent the full range of values characterizing X 1 , Y , or some particular X 1 / Y relationship. 3
Where the individual variable of interest is categorical (on/off, red/black/blue, Jewish/Protestant/Catholic), the identification of diversity is readily apparent. The investigator simply chooses one case from each category. For a continuous variable, the choices are not so obvious. However, the researcher usually chooses both extreme values (high and low), and perhaps the mean or median as well. The researcher may also look for break‐points in the distribution that seem to correspond to categorical differences among cases. Or she may follow a theoretical hunch about which threshold values count, i.e. which are likely to produce different values on Y .
Another sort of diverse case takes account of the values of multiple variables (i.e. a vector), rather than a single variable. If these variables are categorical, the identification of causal types rests upon the intersection of each category. Two dichotomous variables produce a matrix with four cells. Three trichotomous variables produce a matrix of eight cells. And so forth. If all variables are deemed relevant to the analysis, the selection of diverse cases mandates the selection of one case drawn from within each cell. Let us say that an outcome is thought to be affected by sex, race (black/white), and marital status. Here, a diverse‐case strategy of case selection would identify one case within each of these intersecting cells—a total of eight cases. Things become slightly more complicated when one or more of the factors is continuous, rather than categorical. Here, the diversity of case values do not fall neatly into cells. Rather, these cells must be created by fiat—e.g. high, medium, low.
It will be seen that where multiple variables are under consideration, the logic of diverse‐case analysis rests upon the logic of typological theorizing—where different combinations of variables are assumed to have effects on an outcome that vary across types ( Elman 2005 ; George and Bennett 2005 , 235; Lazarsfeld and Barton 1951 ). George and Smoke, for example, wish to explore different types of deterrence failure—by “fait accompli,” by “limited probe,” and by “controlled pressure.” Consequently, they wish to find cases that exemplify each type of causal mechanism. 4
Diversity may thus refer to a range of variation on X or Y , or to a particular combination of causal factors (with or without a consideration of the outcome). In each instance, the goal of case selection is to capture the full range of variation along the dimension(s) of interest.
Since diversity can mean many things, its employment in a large‐ N setting is necessarily dependent upon how this key term is defined. If it is understood to pertain only to a single variable ( X 1 or Y ), then the task is fairly simple. A categorical variable mandates the choice of at least one case from each category—two if dichotomous, three if trichotomous, and so forth. A continuous variable suggests the choice of at least one “high” and “low” value, and perhaps one drawn from the mean or median. But other choices might also be justified, according to one's hunch about the underlying causal relationship or according to natural thresholds found in the data, which may be grouped into discrete categories. Single‐variable traits are usually easy to discover in a large‐ N setting through descriptive statistics or through visual inspection of the data.
Where diversity refers to particular combinations of variables, the relevant cross‐ case technique is some version of stratified random sampling (in a probabilistic setting) or Qualitative Comparative Analysis (in a deterministic setting) ( Ragin 2000 ). If the researcher suspects that a causal relationship is affected not only by combinations of factors but also by their sequencing , then the technique of analysis must incorporate temporal elements ( Abbott 2001 ; Abbott and Forrest 1986 ; Abbott and Tsay 2000 ). Thus, the method of identifying causal types rests upon whatever method of identifying causal relationships is employed in the large‐ N sample.
Note that the identification of distinct case types is intended to identify groups of cases that are internally homogeneous (in all respects that might affect the causal relationship of interest). Thus, the choice of cases within each group should not be problematic, and may be accomplished through random sampling or purposive case selection. However, if there is suspected diversity within each category, then measures should be taken to assure that the chosen cases are typical of each category. A case study should not focus on an atypical member of a subgroup.
Indeed, considerations of diversity and typicality often go together. Thus, in a study of globalization and social welfare systems, Duane Swank (2002) first identifies three distinctive groups of welfare states: “universalistic” (social democratic), “corporatist conservative,” and “liberal.” Next, he looks within each group to find the most‐typical cases. He decides that the Nordic countries are more typical of the universalistic model than the Netherlands since the latter has “some characteristics of the occupationally based program structure and a political context of Christian Democratic‐led governments typical of the corporatist conservative nations” ( Swank 2002 , 11; see also Esping‐Andersen 1990 ). Thus, the Nordic countries are chosen as representative cases within the universalistic case type, and are accompanied in the case‐study portion of his analysis by other cases chosen to represent the other welfare state types (corporatist conservative and liberal).
Evidently, when a sample encompasses a full range of variation on relevant parameters one is likely to enhance the representativeness of that sample (relative to some population). This is a distinct advantage. Of course, the inclusion of a full range of variation may distort the actual distribution of cases across this spectrum. If there are more “high” cases than “low” cases in a population and the researcher chooses only one high case and one low case, the resulting sample of two is not perfectly representative. Even so, the diverse‐case method probably has stronger claims to representativeness than any other small‐ N sample (including the standalone typical case). The selection of diverse cases has the additional advantage of introducing variation on the key variables of interest. A set of diverse cases is, by definition, a set of cases that encompasses a range of high and low values on relevant dimensions. There is, therefore, much to recommend this method of case selection. I suspect that these advantages are commonly understood and are applied on an intuitive level by case‐study researchers. However, the lack of a recognizable name—and an explicit methodological defense—has made it difficult for case‐study researchers to utilize this method of case selection, and to do so in an explicit and self‐conscious fashion. Neologism has its uses.
3 Extreme Case
The extreme‐case method selects a case because of its extreme value on an independent ( X 1 ) or dependent ( Y ) variable of interest. Thus, studies of domestic violence may choose to focus on extreme instances of abuse ( Browne 1987 ). Studies of altruism may focus on those rare individuals who risked their lives to help others (e.g. Holocaust resisters) ( Monroe 1996 ). Studies of ethnic politics may focus on the most heterogeneous societies (e.g. Papua New Guinea) in order to better understand the role of ethnicity in a democratic setting ( Reilly 2000–1 ). Studies of industrial policy often focus on the most successful countries (i.e. the NICS) ( Deyo 1987 ). And so forth. 5
Often an extreme case corresponds to a case that is considered to be prototypical or paradigmatic of some phenomena of interest. This is because concepts are often defined by their extremes, i.e. their ideal types. Italian Fascism defines the concept of Fascism, in part, because it offered the most extreme example of that phenomenon. However, the methodological value of this case, and others like it, derives from its extremity (along some dimension of interest), not its theoretical status or its status in the literature on a subject.
The notion of “extreme” may now be defined more precisely. An extreme value is an observation that lies far away from the mean of a given distribution. This may be measured (if there are sufficient observations) by a case's “Z score”—the number of standard deviations between a case and the mean value for that sample. Extreme cases have high Z scores, and for this reason may serve as useful subjects for intensive analysis.
For a continuous variable, the distance from the mean may be in either direction (positive or negative). For a dichotomous variable (present/absent), extremeness may be interpreted as unusual . If most cases are positive along a given dimension, then a negative case constitutes an extreme case. If most cases are negative, then a positive case constitutes an extreme case. It should be clear that researchers are not simply concerned with cases where something “happened,” but also with cases where something did not. It is the rareness of the value that makes a case valuable, in this context, not its positive or negative value. 6 Thus, if one is studying state capacity, a case of state failure is probably more informative than a case of state endurance simply because the former is more unusual. Similarly, if one is interested in incest taboos a culture where the incest taboo is absent or weak is probably more useful than a culture where it is present or strong. Fascism is more important than nonfascism. And so forth. There is a good reason, therefore, why case studies of revolution tend to focus on “revolutionary” cases. Theda Skocpol (1979) had much more to learn from France than from Austro‐Hungary since France was more unusual than Austro‐Hungary within the population of nation states that Skocpol was concerned to explain. The reason is quite simple: There are fewer revolutionary cases than nonrevolutionary cases; thus, the variation that we explore as a clue to causal relationships is encapsulated in these cases, against a background of nonrevolutionary cases.
Note that the extreme‐case method of case selection appears to violate the social science folk wisdom warning us not to “select on the dependent variable.” 7 Selecting cases on the dependent variable is indeed problematic if a number of cases are chosen, all of which lie on one end of a variable's spectrum (they are all positive or negative), and if the researcher then subjects this sample to cross‐case analysis as if it were representative of a population. 8 Results for this sort of analysis would almost assuredly be biased. Moreover, there will be little variation to explain since the values of each case are explicitly constrained.
However, this is not the proper employment of the extreme‐case method. (It is more appropriately labeled an extreme‐ sample method.) The extreme‐case method actually refers back to a larger sample of cases that lie in the background of the analysis and provide a full range of variation as well as a more representative picture of the population. It is a self‐conscious attempt to maximize variance on the dimension of interest, not to minimize it. If this population of cases is well understood— either through the author's own cross‐case analysis, through the work of others, or through common sense—then a researcher may justify the selection of a single case exemplifying an extreme value for within‐case analysis. If not, the researcher may be well advised to follow a diverse‐case method, as discussed above.
By way of conclusion, let us return to the problem of representativeness. It will be seen that an extreme case may be typical or deviant. There is simply no way to tell because the researcher has not yet specified an X 1 / Y causal proposition. Once such a causal proposition has been specified one may then ask whether the case in question is similar to some population of cases in all respects that might affect the X 1 / Y relationship of interest (i.e. unit homogeneous). It is at this point that it becomes possible to say, within the context of a cross‐case statistical model, whether a case lies near to, or far from, the regression line. However, this sort of analysis means that the researcher is no longer pursuing an extreme‐case method. The extreme‐case method is purely exploratory—a way of probing possible causes of Y , or possible effects of X , in an open‐ended fashion. If the researcher has some notion of what additional factors might affect the outcome of interest, or of what relationship the causal factor of interest might have with Y , then she ought to pursue one of the other methods explored in this chapter. This also implies that an extreme‐case method may transform into a different kind of approach as a study evolves; that is, as a more specific hypothesis comes to light. Useful extreme cases at the outset of a study may prove less useful at a later stage of analysis.
4 Deviant Case
The deviant‐case method selects that case(s) which, by reference to some general understanding of a topic (either a specific theory or common sense), demonstrates a surprising value. It is thus the contrary of the typical case. Barbara Geddes (2003) notes the importance of deviant cases in medical science, where researchers are habitually focused on that which is “pathological” (according to standard theory and practice). The New England Journal of Medicine , one of the premier journals of the field, carries a regular feature entitled Case Records of the Massachusetts General Hospital. These articles bear titles like the following: “An 80‐Year‐Old Woman with Sudden Unilateral Blindness” or “A 76‐Year‐Old Man with Fever, Dyspnea, Pulmonary Infiltrates, Pleural Effusions, and Confusion.” 9 Another interesting example drawn from the field of medicine concerns the extensive study now devoted to a small number of persons who seem resistant to the AIDS virus ( Buchbinder and Vittinghoff 1999 ; Haynes, Pantaleo, and Fauci 1996 ). Why are they resistant? What is different about these people? What can we learn about AIDS in other patients by observing people who have built‐in resistance to this disease?
Likewise, in psychology and sociology case studies may be comprised of deviant (in the social sense) persons or groups. In economics, case studies may consist of countries or businesses that overperform (e.g. Botswana; Microsoft) or underperform (e.g. Britain through most of the twentieth century; Sears in recent decades) relative to some set of expectations. In political science, case studies may focus on countries where the welfare state is more developed (e.g. Sweden) or less developed (e.g. the United States) than one would expect, given a set of general expectations about welfare state development. The deviant case is closely linked to the investigation of theoretical anomalies. Indeed, to say deviant is to imply “anomalous.” 10
Note that while extreme cases are judged relative to the mean of a single distribution (the distribution of values along a single variable), deviant cases are judged relative to some general model of causal relations. The deviant‐case method selects cases which, by reference to some (presumably) general relationship, demonstrate a surprising value. They are “deviant” in that they are poorly explained by the multivariate model. The important point is that deviant‐ness can only be assessed relative to the general (quantitative or qualitative) model. This means that the relative deviant‐ness of a case is likely to change whenever the general model is altered. For example, the United States is a deviant welfare state when this outcome is gauged relative to societal wealth. But it is less deviant—and perhaps not deviant at all—when certain additional (political and societal) factors are included in the model, as discussed in the epilogue. Deviance is model dependent. Thus, when discussing the concept of the deviant case it is helpful to ask the following question: Relative to what general model (or set of background factors) is Case A deviant?
Conceptually, we have said that the deviant case is the logical contrary of the typical case. This translates into a directly contrasting statistical measurement. While the typical case is one with a low residual (in some general model of causal relations), a deviant case is one with a high residual. This means, following our previous discussion, that the deviant case is likely to be an un representative case, and in this respect appears to violate the supposition that case‐study samples should seek to reproduce features of a larger population.
However, it must be borne in mind that the primary purpose of a deviant‐case analysis is to probe for new—but as yet unspecified—explanations. (If the purpose is to disprove an extant theory I shall refer to the study as crucial‐case, as discussed below.) The researcher hopes that causal processes identified within the deviant case will illustrate some causal factor that is applicable to other (more or less deviant) cases. This means that a deviant‐case study usually culminates in a general proposition, one that may be applied to other cases in the population. Once this general proposition has been introduced into the overall model, the expectation is that the chosen case will no longer be an outlier. Indeed, the hope is that it will now be typical , as judged by its small residual in the adjusted model. (The exception would be a circumstance in which a case's outcome is deemed to be “accidental,” and therefore inexplicable by any general model.)
This feature of the deviant‐case study should help to resolve questions about its representativeness. Even if it is not possible to measure the new causal factor (and thus to introduce it into a large‐ N cross‐case model), it may still be plausible to assert (based on general knowledge of the phenomenon) that the chosen case is representative of a broader population.
5 Influential Case
Sometimes, the choice of a case is motivated solely by the need to verify the assumptions behind a general model of causal relations. Here, the analyst attempts to provide a rationale for disregarding a problematic case or a set of problematic cases. That is to say, she attempts to show why apparent deviations from the norm are not really deviant, or do not challenge the core of the theory, once the circumstances of the special case or cases are fully understood. A cross‐case analysis may, after all, be marred by several classes of problems including measurement error, specification error, errors in establishing proper boundaries for the inference (the scope of the argument), and stochastic error (fluctuations in the phenomenon under study that are treated as random, given available theoretical resources). If poorly fitting cases can be explained away by reference to these kinds of problems, then the theory of interest is that much stronger. This sort of deviant‐case analysis answers the question, “What about Case A (or cases of type A)? How does that, seemingly disconfirming, case fit the model?”
Because its underlying purpose is different from the usual deviant‐case study, I offer a new term for this method. The influential case is a case that casts doubt upon a theory, and for that reason warrants close inspection. This investigation may reveal, after all, that the theory is validated—perhaps in some slightly altered form. In this guise, the influential case is the “case that proves the rule.” In other instances, the influential‐case analysis may contribute to disconfirming, or reconceptualizing, a theory. The key point is that the value of the case is judged relative to some extant cross‐case model.
A simple version of influential‐case analysis involves the confirmation of a key case's score on some critical dimension. This is essentially a question of measurement. Sometimes cases are poorly explained simply because they are poorly understood. A close examination of a particular context may reveal that an apparently falsifying case has been miscoded. If so, the initial challenge presented by that case to some general theory has been obviated.
However, the more usual employment of the influential‐case method culminates in a substantive reinterpretation of the case—perhaps even of the general model. It is not just a question of measurement. Consider Thomas Ertman's (1997) study of state building in Western Europe, as summarized by Gerardo Munck. This study argues
that the interaction of a) the type of local government during the first period of statebuilding, with b) the timing of increases in geopolitical competition, strongly influences the kind of regime and state that emerge. [Ertman] tests this hypothesis against the historical experience of Europe and finds that most countries fit his predictions. Denmark, however, is a major exception. In Denmark, sustained geopolitical competition began relatively late and local government at the beginning of the statebuilding period was generally participatory, which should have led the country to develop “patrimonial constitutionalism.” But in fact, it developed “bureaucratic absolutism.” Ertman carefully explores the process through which Denmark came to have a bureaucratic absolutist state and finds that Denmark had the early marks of a patrimonial constitutionalist state. However, the country was pushed off this developmental path by the influence of German knights, who entered Denmark and brought with them German institutions of local government. Ertman then traces the causal process through which these imported institutions pushed Denmark to develop bureaucratic absolutism, concluding that this development was caused by a factor well outside his explanatory framework. ( Munck 2004 , 118)
Ertman's overall framework is confirmed insofar as he has been able to show, by an in‐depth discussion of Denmark, that the causal processes stipulated by the general theory hold even in this apparently disconfirming case. Denmark is still deviant, but it is so because of “contingent historical circumstances” that are exogenous to the theory ( Ertman 1997 , 316).
Evidently, the influential‐case analysis is similar to the deviant‐case analysis. Both focus on outliers. However, as we shall see, they focus on different kinds of outliers. Moreover, the animating goals of these two research designs are quite different. The influential‐case study begins with the aim of confirming a general model, while the deviant‐case study has the aim of generating a new hypothesis that modifies an existing general model. The confusion stems from the fact that the same case study may fulfill both objectives—qualifying a general model and, at the same time, confirming its core hypothesis.
Thus, in their study of Roberto Michels's “iron law of oligarchy,” Lipset, Trow, and Coleman (1956) choose to focus on an organization—the International Typographical Union—that appears to violate the central presupposition. The ITU, as noted by one of the authors, has “a long‐term two‐party system with free elections and frequent turnover in office” and is thus anything but oligarchic ( Lipset 1959 , 70). As such, it calls into question Michels's grand generalization about organizational behavior. The authors explain this curious result by the extraordinarily high level of education among the members of this union. Michels's law is shown to be true for most organizations, but not all. It is true, with qualifications. Note that the respecification of the original model (in effect, Lipset, Trow, and Coleman introduce a new control variable or boundary condition) involves the exploration of a new hypothesis. In this instance, therefore, the use of an influential case to confirm an existing theory is quite similar to the use of a deviant case to explore a new theory.
In a quantitative idiom, influential cases are those that, if counterfactually assigned a different value on the dependent variable, would most substantially change the resulting estimates. They may or may not be outliers (high‐residual cases). Two quantitative measures of influence are commonly applied in regression diagnostics ( Belsey, Kuh, and Welsch 2004 ). The first, often referred to as the leverage of a case, derives from what is called the hat matrix . Based solely on each case's scores on the independent variables, the hat matrix tells us how much a change in (or a measurement error on) the dependent variable for that case would affect the overall regression line. The second is Cook's distance , a measure of the extent to which the estimates of all the parameters would change if a given case were omitted from the analysis. Cases with a large leverage or Cook's distance contribute quite a lot to the inferences drawn from a cross‐case analysis. In this sense, such cases are vital for maintaining analytic conclusions. Discovering a significant measurement error on the dependent variable or an important omitted variable for such a case may dramatically revise estimates of the overall relationships. Hence, it may be quite sensible to select influential cases for in‐depth study.
Note that the use of an influential‐case strategy of case selection is limited to instances in which a researcher has reason to be concerned that her results are being driven by one or a few cases. This is most likely to be true in small to moderate‐sized samples. Where N is very large—greater than 1,000, let us say—it is extremely unlikely that a small set of cases (much less an individual case) will play an “influential” role. Of course, there may be influential sets of cases, e.g. countries within a particular continent or cultural region, or persons of Irish extraction. Sets of influential observations are often problematic in a time‐series cross‐section data‐set where each unit (e.g. country) contains multiple observations (through time), and hence may have a strong influence on aggregate results. Still, the general rule is: the larger the sample, the less important individual cases are likely to be and, hence, the less likely a researcher is to use an influential‐case approach to case selection.
6 Crucial Case
Of all the extant methods of case selection perhaps the most storied—and certainly the most controversial—is the crucial‐case method, introduced to the social science world several decades ago by Harry Eckstein. In his seminal essay, Eckstein (1975 , 118) describes the crucial case as one “that must closely fit a theory if one is to have confidence in the theory's validity, or, conversely, must not fit equally well any rule contrary to that proposed.” A case is crucial in a somewhat weaker—but much more common—sense when it is most, or least, likely to fulfill a theoretical prediction. A “most‐likely” case is one that, on all dimensions except the dimension of theoretical interest, is predicted to achieve a certain outcome, and yet does not. It is therefore used to disconfirm a theory. A “least‐likely” case is one that, on all dimensions except the dimension of theoretical interest, is predicted not to achieve a certain outcome, and yet does so. It is therefore used to confirm a theory. In all formulations, the crucial‐case offers a most‐difficult test for an argument, and hence provides what is perhaps the strongest sort of evidence possible in a nonexperimental, single‐case setting.
Since the publication of Eckstein's influential essay, the crucial‐case approach has been claimed in a multitude of studies across several social science disciplines and has come to be recognized as a staple of the case‐study method. 11 Yet the idea of any single case playing a crucial (or “critical”) role is not widely accepted among most methodologists (e.g. Sekhon 2004 ). (Even its progenitor seems to have had doubts.)
Let us begin with the confirmatory (a.k.a. least‐likely) crucial case. The implicit logic of this research design may be summarized as follows. Given a set of facts, we are asked to contemplate the probability that a given theory is true. While the facts matter, to be sure, the effectiveness of this sort of research also rests upon the formal properties of the theory in question. Specifically, the degree to which a theory is amenable to confirmation is contingent upon how many predictions can be derived from the theory and on how “risky” each individual prediction is. In Popper's (1963 , 36) words, “Confirmations should count only if they are the result of risky predictions ; that is to say, if, unenlightened by the theory in question, we should have expected an event which was incompatible with the theory—and event which would have refuted the theory. Every ‘good’ scientific theory is a prohibition; it forbids certain things to happen. The more a theory forbids, the better it is” (see also Popper 1934/1968 ). A risky prediction is therefore one that is highly precise and determinate, and therefore unlikely to be achieved by the product of other causal factors (external to the theory of interest) or through stochastic processes. A theory produces many such predictions if it is fully elaborated, issuing predictions not only on the central outcome of interest but also on specific causal mechanisms, and if it is broad in purview. (The notion of riskiness may also be conceptualized within the Popperian lexicon as degrees of falsifiability .)
These points can also be articulated in Bayesian terms. Colin Howson and Peter Urbach explain: “The degree to which h [a hypothesis] is confirmed by e [a set of evidence] depends … on the extent to which P(eČh) exceeds P (e) , that is, on how much more probable e is relative to the hypothesis and background assumptions than it is relative just to background assumptions.” Again, “confirmation is correlated with how much more probable the evidence is if the hypothesis is true than if it is false” ( Howson and Urlbach 1989 , 86). Thus, the stranger the prediction offered by a theory—relative to what we would normally expect—the greater the degree of confirmation that will be afforded by the evidence. As an intuitive example, Howson and Urbach (1989 , 86) offer the following:
If a soothsayer predicts that you will meet a dark stranger sometime and you do in fact, your faith in his powers of precognition would not be much enhanced: you would probably continue to think his predictions were just the result of guesswork. However, if the prediction also gave the correct number of hairs on the head of that stranger, your previous scepticism would no doubt be severely shaken.
While these Popperian/Bayesian notions 12 are relevant to all empirical research designs, they are especially relevant to case‐study research designs, for in these settings a single case (or, at most, a small number of cases) is required to bear a heavy burden of proof. It should be no surprise, therefore, that Popper's idea of “riskiness” was to be appropriated by case‐study researchers like Harry Eckstein to validate the enterprise of single‐case analysis. (Although Eckstein does not cite Popper the intellectual lineage is clear.) Riskiness, here, is analogous to what is usually referred to as a “most‐ difficult” research design, which in a case‐study research design would be understood as a “least‐likely” case. Note also that the distinction between a “must‐fit” case and a least‐likely case—that, in the event, actually does fit the terms of a theory—is a matter of degree. Cases are more or less crucial for confirming theories. The point is that, in some circumstances, a paucity of empirical evidence may be compensated by the riskiness of the theory.
The crucial‐case research design is, perforce, a highly deductive enterprise; much depends on the quality of the theory under investigation. It follows that the theories most amenable to crucial‐case analysis are those which are lawlike in their precision, degree of elaboration, consistency, and scope. The more a theory attains the status of a causal law, the easier it will be to confirm, or to disconfirm, with a single case. Indeed, risky predictions are common in natural science fields such as physics, which in turn served as the template for the deductive‐nomological (“covering‐law”) model of science that influenced Eckstein and others in the postwar decades (e.g. Hempel 1942 ).
A frequently cited example is the first important empirical demonstration of the theory of relativity, which took the form of a single‐event prediction on the occasion of the May 29, 1919, solar eclipse ( Eckstein 1975 ; Popper 1963 ). Stephen Van Evera (1997 , 66–7) describes the impact of this prediction on the validation of Einstein's theory.
Einstein's theory predicted that gravity would bend the path of light toward a gravity source by a specific amount. Hence it predicted that during a solar eclipse stars near the sun would appear displaced—stars actually behind the sun would appear next to it, and stars lying next to the sun would appear farther from it—and it predicted the amount of apparent displacement. No other theory made these predictions. The passage of this one single‐case‐study test brought the theory wide acceptance because the tested predictions were unique—there was no plausible competing explanation for the predicted result—hence the passed test was very strong.
The strength of this test is the extraordinary fit between the theory and a set of facts found in a single case, and the corresponding lack of fit between all other theories and this set of facts. Einstein offered an explanation of a particular set of anomalous findings that no other existing theory could make sense of. Of course, one must assume that there was no—or limited—measurement error. And one must assume that the phenomenon of interest is largely invariant; light does not bend differently at different times and places (except in ways that can be understood through the theory of relativity). And one must assume, finally, that the theory itself makes sense on other grounds (other than the case of special interest); it is a plausible general theory. If one is willing to accept these a priori assumptions, then the 1919 “case study” provides a very strong confirmation of the theory. It is difficult to imagine a stronger proof of the theory from within an observational (nonexperimental) setting.
In social science settings, by contrast, one does not commonly find single‐case studies offering knockout evidence for a theory. This is, in my view, largely a product of the looseness (the underspecification) of most social science theories. George and Bennett point out that while the thesis of the democratic peace is as close to a “law” as social science has yet seen, it cannot be confirmed (or refuted) by looking at specific causal mechanisms because the causal pathways mandated by the theory are multiple and diverse. Under the circumstances, no single‐case test can offer strong confirmation of the theory ( George and Bennett 2005 , 209).
However, if one adopts a softer version of the crucial‐case method—the least‐likely (most difficult) case—then possibilities abound. Indeed, I suspect that, implicitly , most case‐study work that makes a positive argument focusing on a single case (without a corresponding cross‐case analysis) relies largely on the logic of the least‐ likely case. Rarely is this logic made explicit, except perhaps in a passing phrase or two. Yet the deductive logic of the “risky” prediction is central to the case‐study enterprise. Whether a case study is convincing or not often rests on the reader's evaluation of how strong the evidence for an argument might be, and this in turn—wherever cross‐ case evidence is limited and no manipulated treatment can be devised—rests upon an estimation of the degree of “fit” between a theory and the evidence at hand, as discussed.
Lily Tsai's (2007) investigation of governance at the village level in China employs several in‐depth case studies of villages which are chosen (in part) because of their least‐likely status relative to the theory of interest. Tsai's hypothesis is that villages with greater social solidarity (based on preexisting religious or familial networks) will develop a higher level of social trust and mutual obligation and, as a result, will experience better governance. Crucial cases, therefore, are villages that evidence a high level of social solidarity but which, along other dimensions, would be judged least likely to develop good governance, e.g. they are poor, isolated, and lack democratic institutions or accountability mechanisms from above. “Li Settlement,” in Fujian province, is such a case. The fact that this impoverished village nonetheless boasts an impressive set of infrastructural accomplishments such as paved roads with drainage ditches (a rarity in rural China) suggests that something rather unusual is going on here. Because her case is carefully chosen to eliminate rival explanations, Tsai's conclusions about the special role of social solidarity are difficult to gainsay. How else is one to explain this otherwise anomalous result? This is the strength of the least‐likely case, where all other plausible causal factors for an outcome have been minimized. 13
Jack Levy (2002 , 144) refers to this, evocatively, as a “Sinatra inference:” if it can make it here, it can make it anywhere (see also Khong 1992 , 49; Sagan 1995 , 49; Shafer 1988 , 14–6). Thus, if social solidarity has the hypothesized effect in Li Settlement it should have the same effect in more propitious settings (e.g. where there is greater economic surplus). The same implicit logic informs many case‐study analyses where the intent of the study is to confirm a hypothesis on the basis of a single case.
Another sort of crucial case is employed for the purpose of dis confirming a causal hypothesis. A central Popperian insight is that it is easier to disconfirm an inference than to confirm that same inference. (Indeed, Popper doubted that any inference could be fully confirmed, and for this reason preferred the term “corroborate.”) This is particularly true of case‐study research designs, where evidence is limited to one or several cases. The key proviso is that the theory under investigation must take a consistent (a.k.a. invariant, deterministic) form, even if its predictions are not terrifically precise, well elaborated, or broad.
As it happens, there are a fair number of invariant propositions floating around the social science disciplines (Goertz and Levy forthcoming; Goertz and Starr 2003 ). It used to be argued, for example, that political stability would occur only in countries that are relatively homogeneous, or where existing heterogeneities are mitigated by cross‐cutting cleavages ( Almond 1956 ; Bentley 1908/1967 ; Lipset 1960/1963 ; Truman 1951 ). Arend Lijphart's (1968) study of the Netherlands, a peaceful country with reinforcing social cleavages, is commonly viewed as refuting this theory on the basis of a single in‐depth case analysis. 14
Granted, it may be questioned whether presumed invariant theories are really invariant; perhaps they are better understood as probabilistic. Perhaps, that is, the theory of cross‐cutting cleavages is still true, probabilistically, despite the apparent Dutch exception. Or perhaps the theory is still true, deterministically, within a subset of cases that does not include the Netherlands. (This sort of claim seems unlikely in this particular instance, but it is quite plausible in many others.) Or perhaps the theory is in need of reframing; it is true, deterministically, but applies only to cross‐ cutting ethnic/racial cleavages, not to cleavages that are primarily religious. One can quibble over what it means to “disconfirm” a theory. The point is that the crucial case has, in all these circumstances, provided important updating of a theoretical prior.
Heretofore, I have treated causal factors as dichotomous. Countries have either reinforcing or cross‐cutting cleavages and they have regimes that are either peaceful or conflictual. Evidently, these sorts of parameters are often matters of degree. In this reading of the theory, cases are more or less crucial. Accordingly, the most useful—i.e. most crucial—case for Lijphart's purpose is one that has the most segregated social groups and the most peaceful and democratic track record. In these respects, the Netherlands was a very good choice. Indeed, the degree of disconfirmation offered by this case study is probably greater than the degree of disconfirmation that might have been provided by other cases such as India or Papua New Guinea—countries where social peace has not always been secure. The point is that where variables are continuous rather than dichotomous it is possible to evaluate potential cases in terms of their degree of crucialness .
Note that the crucial‐case method of case‐selection, whether employed in a confirmatory or disconfirmatory mode, cannot be employed in a large‐ N context. This is because an explicit cross‐case model would render the crucial‐case study redundant. Once one identifies the relevant parameters and the scores of all cases on those parameters, one has in effect constructed a cross‐case model that confirms or disconfirms the theory in question. The case study is thenceforth irrelevant, at least as a means of decisive confirmation or disconfirmation. 15 It remains highly relevant as a means of exploring causal mechanisms, of course. Yet, because this objective is quite different from that which is usually associated with the term, I enlist a new term for this technique.
7 Pathway Case
One of the most important functions of case‐study research is the elucidation of causal mechanisms. But which sort of case is most useful for this purpose? Although all case studies presumably shed light on causal mechanisms, not all cases are equally transparent. In situations where a causal hypothesis is clear and has already been confirmed by cross‐case analysis, researchers are well advised to focus on a case where the causal effect of X 1 on Y can be isolated from other potentially confounding factors ( X 2 ). I shall call this a pathway case to indicate its uniquely penetrating insight into causal mechanisms. In contrast to the crucial case, this sort of method is practicable only in circumstances where cross‐case covariational patterns are well studied and where the mechanism linking X 1 and Y remains dim. Because the pathway case builds on prior cross‐case analysis, the problem of case selection must be situated within that sample. There is no standalone pathway case.
The logic of the pathway case is clearest in situations of causal sufficiency—where a causal factor of interest, X 1 , is sufficient by itself (though perhaps not necessary) to account for Y 's value (0 or 1). The other causes of Y , about which we need make no assumptions, are designated as a vector, X 2 .
Note that wherever various causal factors are substitutable for one another, each factor is conceptualized (individually) as sufficient ( Braumoeller 2003 ). Thus, situations of causal equifinality presume causal sufficiency on the part of each factor or set of conjoint factors. An example is provided by the literature on democratization, which stipulates three main avenues of regime change: leadership‐initiated reform, a controlled opening to opposition, or the collapse of an authoritarian regime ( Colomer 1991 ). The case‐study format constrains us to analyze one at a time, so let us limit our scope to the first one—leadership‐initiated reform. So considered, a causal‐pathway case would be one with the following features: (a) democratization, (b) leadership‐initiated reform, (c) no controlled opening to the opposition, (d) no collapse of the previous authoritarian regime, and (e) no other extraneous factors that might affect the process of democratization. In a case of this type, the causal mechanisms by which leadership‐initiated reform may lead to democratization will be easiest to study. Note that it is not necessary to assume that leadership‐initiated reform always leads to democratization; it may or may not be a deterministic cause. But it is necessary to assume that leadership‐initiated reform can sometimes lead to democratization on its own (given certain background features).
Now let us move from these examples to a general‐purpose model. For heuristic purposes, let us presume that all variables in that model are dichotomous (coded as 0 or 1) and that the model is complete (all causes of Y are included). All causal relationships will be coded so as to be positive: X 1 and Y covary as do X 2 and Y . This allows us to visualize a range of possible combinations at a glance.
Recall that the pathway case is always focused, by definition, on a single causal factor, denoted X 1 . (The researcher's focus may shift to other causal factors, but may only focus on one causal factor at a time.) In this scenario, and regardless of how many additional causes of Y there might be (denoted X 2 , a vector of controls), there are only eight relevant case types, as illustrated in Table 28.2 . Identifying these case types is a relatively simple matter, and can be accomplished in a small‐ N sample by the construction of a truth‐table (modeled after Table 28.2 ) or in a large‐ N sample by the use of cross‐tabs.
Notes : X 1 = the variable of theoretical interest. X 2 = a vector of controls (a score of 0 indicates that all control variables have a score of 0, while a score of 1 indicates that all control variables have a score of 1). Y = the outcome of interest. A–H = case types (the N for each case type is indeterminate). G, H = possible pathway cases. Sample size = indeterminate.
Assumptions : (a) all variables can be coded dichotomously (a binary coding of the concept is valid); (b) all independent variables are positively correlated with Y in the general case; ( c ) X 1 is (at least sometimes) a sufficient cause of Y .
Note that the total number of combinations of values depends on the number of control variables, which we have represented with a single vector, X 2 . If this vector consists of a single variable then there are only eight case types. If this vector consists of two variables ( X 2a , X 2b ) then the total number of possible combinations increases from eight (2 3 ) to sixteen (2 4 ). And so forth. However, none of these combinations is relevant for present purposes except those where X 2a and X 2b have the same value (0 or 1). “Mixed” cases are not causal pathway cases, for reasons that should become clear.
The pathway case, following the logic of the crucial case, is one where the causal factor of interest, X 1 , correctly predicts Y while all other possible causes of Y (represented by the vector, X 2 ) make “wrong” predictions. If X 1 is—at least in some circumstances—a sufficient cause of Y , then it is these sorts of cases that should be most useful for tracing causal mechanisms. There are only two such cases in Ta b l e 28.2—G and H. In all other cases, the mechanism running from X 1 to Y would be difficult to discern either because X 1 and Y are not correlated in the usual way (constituting an unusual case, in the terms of our hypothesis) or because other confounding factors ( X 2 ) intrude. In case A, for example, the positive value on Y could be a product of X 1 or X 2 . An in‐depth examination of this case is not likely to be very revealing.
Keep in mind that because the researcher already knows from her cross‐case examination what the general causal relationships are, she knows (prior to the case‐ study investigation) what constitutes a correct or incorrect prediction. In the crucial‐ case method, by contrast, these expectations are deductive rather than empirical. This is what differentiates the two methods. And this is why the causal pathway case is useful principally for elucidating causal mechanisms rather than verifying or falsifying general propositions (which are already more or less apparent from the cross‐case evidence). Of course, we must leave open the possibility that the investigation of causal mechanisms would invalidate a general claim, if that claim is utterly contingent upon a specific set of causal mechanisms and the case study shows that no such mechanisms are present. However, this is rather unlikely in most social science settings. Usually, the result of such a finding will be a reformulation of the causal processes by which X 1 causes Y —or, alternatively, a realization that the case under investigation is aberrant (atypical of the general population of cases).
Sometimes, the research question is framed as a unidirectional cause: one is interested in why 0 becomes 1 (or vice versa) but not in why 1 becomes 0. In our previous example, we asked why democracies fail, not why countries become democratic or authoritarian. So framed, there can be only one type of causal‐pathway case. (Whether regime failure is coded as 0 or 1 is a matter of taste.) Where researchers are interested in bidirectional causality—a movement from 0 to 1 as well as from 1 to 0—there are two possible causal‐pathway cases, G and H. In practice, however, one of these case types is almost always more useful than the other. Thus, it seems reasonable to employ the term “pathway case” in the singular. In order to determine which of these two case types will be more useful for intensive analysis the researcher should look to see whether each case type exhibits desirable features such as: (a) a rare (unusual) value on X 1 or Y (designated “extreme” in our previous discussion), (b) observable temporal variation in X 1 , ( c ) an X 1 / Y relationship that is easier to study (it has more visible features; it is more transparent), or (d) a lower residual (thus indicating a more typical case, within the terms of the general model). Usually, the choice between G and H is intuitively obvious.
Now, let us consider a scenario in which all (or most) variables of concern to the model are continuous, rather than dichotomous. Here, the job of case selection is considerably more complex, for causal “sufficiency” (in the usual sense) cannot be invoked. It is no longer plausible to assume that a given cause can be entirely partitioned, i.e. rival factors eliminated. However, the search for a pathway case may still be viable. What we are looking for in this scenario is a case that satisfies two criteria: (1) it is not an outlier (or at least not an extreme outlier) in the general model and (2) its score on the outcome ( Y ) is strongly influenced by the theoretical variable of interest ( X 1 ), taking all other factors into account ( X 2 ). In this sort of case it should be easiest to “see” the causal mechanisms that lie between X 1 and Y .
Achieving the second desiderata requires a bit of manipulation. In order to determine which (nonoutlier) cases are most strongly affected by X 1 , given all the other parameters in the model, one must compare the size of the residuals for each case in a reduced form model, Y = Constant + X 2 + Res reduced , with the size of the residuals for each case in a full model, Y = Constant + X 2 + X 1 + Res full . The pathway case is that case, or set of cases, which shows the greatest difference between the residual for the reduced‐form model and the full model (ΔResidual). Thus,
Note that the residual for a case must be smaller in the full model than in the reduced‐ form model; otherwise, the addition of the variable of interest ( X 1 ) pulls the case away from the regression line. We want to find a case where the addition of X 1 pushes the case towards the regression line, i.e. it helps to “explain” that case.
As an example, let us suppose that we are interested in exploring the effect of mineral wealth on the prospects for democracy in a society. According to a good deal of work on this subject, countries with a bounty of natural resources—particularly oil—are less likely to democratize (or once having undergone a democratic transition, are more likely to revert to authoritarian rule) ( Barro 1999 ; Humphreys 2005 ; Ross 2001 ). The cross‐country evidence is robust. Yet as is often the case, the causal mechanisms remain rather obscure. In order to better understand this phenomenon it may be worthwhile to exploit the findings of cross‐country regression models in order to identify a country whose regime type (i.e. its democracy “score” on some general index) is strongly affected by its natural‐research wealth, all other things held constant. An analysis of this sort identifies two countries— the United Arab Emirates and Kuwait—with high Δ Residual values and modest residuals in the full model (signifying that these cases are not outliers). Researchers seeking to explore the effect of oil wealth on regime type might do well to focus on these two cases since their patterns of democracy cannot be well explained by other factors—e.g. economic development, religion, European influence, or ethnic fractionalization. The presence of oil wealth in these countries would appear to have a strong independent effect on the prospects for democratization in these cases, an effect that is well modeled by general theory and by the available cross‐case evidence.
To reiterate, the logic of causal “elimination” is much more compelling where variables are dichotomous and where causal sufficiency can be assumed ( X 1 is sufficient by itself, at least in some circumstances, to cause Y ). Where variables are continuous, the strategy of the pathway case is more dubious, for potentially confounding causal factors ( X 2 ) cannot be neatly partitioned. Even so, we have indicated why the selection of a pathway case may be a logical approach to case‐study analysis in many circumstances.
The exceptions may be briefly noted. Sometimes, where all variables in a model are dichotomous, there are no pathway cases, i.e. no cases of type G or H (in Table 28.2 ). This is known as the “empty cell” problem, or a problem of severe causal multicollinearity. The universe of observational data does not always oblige us with cases that allow us to independently test a given hypothesis. Where variables are continuous, the analogous problem is that of a causal variable of interest ( X 1 ) that has only minimal effects on the outcome of interest. That is, its role in the general model is quite minor. In these situations, the only cases that are strongly affected by X 1 —if there are any at all—may be extreme outliers, and these sorts of cases are not properly regarded as providing confirmatory evidence for a proposition, for reasons that are abundantly clear by now.
Finally, it should be clarified that the identification of a causal pathway case does not obviate the utility of exploring other cases. One might, for example, want to compare both sorts of potential pathway cases—G and H—with each other. Many other combinations suggest themselves. However, this sort of multi‐case investigation moves beyond the logic of the causal‐pathway case.
8 Most‐similar Cases
The most‐similar method employs a minimum of two cases. 16 In its purest form, the chosen pair of cases is similar in all respects except the variable(s) of interest. If the study is exploratory (i.e. hypothesis generating), the researcher looks for cases that differ on the outcome of theoretical interest but are similar on various factors that might have contributed to that outcome, as illustrated in Table 28.3 (A) . This is a common form of case selection at the initial stage of research. Often, fruitful analysis begins with an apparent anomaly: two cases are apparently quite similar, and yet demonstrate surprisingly different outcomes. The hope is that intensive study of these cases will reveal one—or at most several—factors that differ across these cases. These differing factors ( X 1 ) are looked upon as putative causes. At this stage, the research may be described by the second diagram in Table 28.3 (B) . Sometimes, a researcher begins with a strong hypothesis, in which case her research design is confirmatory (hypothesis testing) from the get‐go. That is, she strives to identify cases that exhibit different outcomes, different scores on the factor of interest, and similar scores on all other possible causal factors, as illustrated in the second (hypothesis‐testing) diagram in Table 28.3 (B) .
The point is that the purpose of a most‐similar research design, and hence its basic setup, often changes as a researcher moves from an exploratory to a confirmatory mode of analysis. However, regardless of where one begins, the results, when published, look like a hypothesis‐testing research design. Question marks have been removed: (A) becomes (B) in Table 28.3 .
As an example, let us consider Leon Epstein's classic study of party cohesion, which focuses on two “most‐similar” countries, the United States and Canada. Canada has highly disciplined parties whose members vote together on the floor of the House of Commons while the United States has weak, undisciplined parties, whose members often defect on floor votes in Congress. In explaining these divergent outcomes, persistent over many years, Epstein first discusses possible causal factors that are held more or less constant across the two cases. Both the United States and Canada inherited English political cultures, both have large territories and heterogeneous populations, both are federal, and both have fairly loose party structures with strong regional bases and a weak center. These are the “control” variables. Where they differ is in one constitutional feature: Canada is parliamentary while the United States is presidential. And it is this institutional difference that Epstein identifies as the crucial (differentiating) cause. (For further examples of the most‐similar method see Brenner 1976 ; Hamilton 1977 ; Lipset 1968 ; Miguel 2004 ; Moulder 1977 ; Posner 2004 .)
X 1 = the variable of theoretical interest. X 2 = a vector of controls. Y = the outcome of interest.
Several caveats apply to any most‐similar analysis (in addition to the usual set of assumptions applying to all case‐study analysis). First, each causal factor is understood as having an independent and additive effect on the outcome; there are no “interaction” effects. Second, one must code cases dichotomously (high/low, present/absent). This is straightforward if the underlying variables are also dichotomous (e.g. federal/unitary). However, it is often the case that variables of concern in the model are continuous (e.g. party cohesion). In this setting, the researcher must “dichotomize” the scoring of cases so as to simplify the two‐case analysis. (Some flexibility is admissible on the vector of controls ( X 2 ) that are “held constant” across the cases. Nonidentity is tolerable if the deviation runs counter to the predicted hypothesis. For example, Epstein describes both the United States and Canada as having strong regional bases of power, a factor that is probably more significant in recent Canadian history than in recent American history. However, because regional bases of power should lead to weaker parties, rather than stronger parties, this element of nonidentity does not challenge Epstein's conclusions. Indeed, it sets up a most‐difficult research scenario, as discussed above.)
In one respect the requirements for case control are not so stringent. Specifically, it is not usually necessary to measure control variables (at least not with a high degree of precision) in order to control for them. If two countries can be assumed to have similar cultural heritages one needn't worry about constructing variables to measure that heritage. One can simply assert that, whatever they are, they are more or less constant across the two cases. This is similar to the technique employed in a randomized experiment, where the researcher typically does not attempt to measure all the factors that might affect the causal relationship of interest. She assumes, rather, that these unknown factors have been neutralized across the treatment and control groups by randomization or by the choice of a sample that is internally homogeneous.
The most useful statistical tool for identifying cases for in‐depth analysis in a most‐ similar setting is probably some variety of matching strategy—e.g. exact matching, approximate matching, or propensity‐score matching. 17 The product of this procedure is a set of matched cases that can be compared in whatever way the researcher deems appropriate. These are the “most‐similar” cases. Rosenbaum and Silber (2001 , 223) summarize:
Unlike model‐based adjustments, where [individuals] vanish and are replaced by the coefficients of a model, in matching, ostensibly comparable patterns are compared directly, one by one. Modern matching methods involve statistical modeling and combinatorial algorithms, but the end result is a collection of pairs or sets of people who look comparable, at least on average. In matching, people retain their integrity as people, so they can be examined and their stories can be told individually.
Matching, conclude the authors, “facilitates, rather than inhibits, thick description” ( Rosenbaum and Silber 2001 , 223).
In principle, the same matching techniques that have been used successfully in observational studies of medical treatments might also be adapted to the study of nation states, political parties, cities, or indeed any traditional paired cases in the social sciences. Indeed, the current popularity of matching among statisticians—relative, that is, to garden‐variety regression models—rests upon what qualitative researchers would recognize as a “case‐based” approach to causal analysis. If Rosenbaum and Silber are correct, it may be perfectly reasonable to appropriate this large‐ N method of analysis for case‐study purposes.
As with other methods of case selection, the most‐similar method is prone to problems of nonrepresentativeness. If employed in a qualitative fashion (without a systematic cross‐case selection strategy), potential biases in the chosen case must be addressed in a speculative way. If the researcher employs a matching technique of case selection within a large‐ N sample, the problem of potential bias can be addressed by assuring the choice of cases that are not extreme outliers, as judged by their residuals in the full model. Most‐similar cases should also be “typical” cases, though some scope for deviance around the regression line may be acceptable for purposes of finding a good fit among cases.
X 1 = the variable of theoretical interest. X 2a–d = a vector of controls. Y = the outcome of interest.
9 Most‐different Cases
A final case‐selection method is the reverse image of the previous method. Here, variation on independent variables is prized, while variation on the outcome is eschewed. Rather than looking for cases that are most‐similar, one looks for cases that are most‐ different . Specifically, the researcher tries to identify cases where just one independent variable ( X 1 ), as well as the dependent variable ( Y ), covary, while all other plausible factors ( X 2a–d ) show different values. 18
The simplest form of this two‐case comparison is illustrated in Table 28.4 . Cases A and B are deemed “most different,” though they are similar in two essential respects— the causal variable of interest and the outcome.
As an example, I follow Marc Howard's (2003) recent work, which explores the enduring impact of Communism on civil society. 19 Cross‐national surveys show a strong correlation between former Communist regimes and low social capital, controlling for a variety of possible confounders. It is a strong result. Howard wonders why this relationship is so strong and why it persists, and perhaps even strengthens, in countries that are no longer socialist or authoritarian. In order to answer this question, he focuses on two most‐different cases, Russia and East Germany. These two countries were quite different—in all ways other than their Communist experience— prior to the Soviet era, during the Soviet era (since East Germany received substantial subsidies from West Germany), and in the post‐Soviet era, as East Germany was absorbed into West Germany. Yet, they both score near the bottom of various cross‐ national indices intended to measure the prevalence of civic engagement in the current era. Thus, Howard's (2003 , 6–9) case selection procedure meets the requirements of the most‐different research design: Variance is found on all (or most) dimensions aside from the key factor of interest (Communism) and the outcome (civic engagement).
What leverage is brought to the analysis from this approach? Howard's case studies combine evidence drawn from mass surveys and from in‐depth interviews of small, stratified samples of Russians and East Germans. (This is a good illustration, incidentally, of how quantitative and qualitative evidence can be fruitfully combined in the intensive study of several cases.) The product of this analysis is the identification of three causal pathways that, Howard (2003 , 122) claims, help to explain the laggard status of civil society in post‐Communist polities: “the mistrust of communist organizations, the persistence of friendship networks, and the disappointment with post‐communism.” Simply put, Howard (2003 , 145) concludes, “a great number of citizens in Russia and Eastern Germany feel a strong and lingering sense of distrust of any kind of public organization, a general satisfaction with their own personal networks (accompanied by a sense of deteriorating relations within society overall), and disappointment in the developments of post‐communism.”
The strength of this most‐different case analysis is that the results obtained in East Germany and Russia should also apply in other post‐Communist polities (e.g. Lithuania, Poland, Bulgaria, Albania). By choosing a heterogeneous sample, Howard solves the problem of representativeness in his restricted sample. However, this sample is demonstrably not representative across the population of the inference, which is intended to cover all countries of the world.
More problematic is the lack of variation on key causal factors of interest— Communism and its putative causal pathways. For this reason, it is difficult to reach conclusions about the causal status of these factors on the basis of the most‐different analysis alone. It is possible, that is, that the three causal pathways identified by Howard also operate within polities that never experienced Communist rule.
Nor does it seem possible to conclusively eliminate rival hypotheses on the basis of this most‐different analysis. Indeed, this is not Howard's intention. He wishes merely to show that whatever influence on civil society might be attributed to economic, cultural, and other factors does not exhaust this subject.
My considered judgment is that the most‐different research design provides minimal leverage into the problem of why Communist systems appear to suppress civic engagement, years after their disappearance. Fortunately, this is not the only research design employed by Howard in his admirable study. Indeed, the author employs two other small‐ N cross‐case methods, as well as a large‐ N cross‐country statistical analysis. These methods do most of the analytic work. East Germany may be regarded as a causal pathway case (see above). It has all the attributes normally assumed to foster civic engagement (e.g. a growing economy, multiparty competition, civil liberties, a free press, close association with Western European culture and politics), but nonetheless shows little or no improvement on this dimension during the post‐ transition era ( Howard 2003 , 8). It is plausible to attribute this lack of change to its Communist past, as Howard does, in which case East Germany should be a fruitful case for the investigation of causal mechanisms. The contrast between East and West Germany provides a most‐similar analysis since the two polities share virtually everything except a Communist past. This variation is also deftly exploited by Howard.
I do not wish to dismiss the most‐different research method entirely. Surely, Howard's findings are stronger with the intensive analysis of Russia than they would be without. Yet his book would not stand securely on the empirical foundation provided by most‐different analysis alone. If one strips away the pathway‐case (East Germany) and the most‐similar analysis (East/West Germany) there is little left upon which to base an analysis of causal relations (aside from the large‐ N cross‐national analysis). Indeed, most scholars who employ the most‐different method do so in conjunction with other methods. 20 It is rarely, if ever, a standalone method. 21
Generalizing from this discussion of Marc Howard's work, I offer the following summary remarks on the most‐different method of case analysis. (I leave aside issues faced by all case‐study analyses, issues that are explored in Gerring 2007 .)
Let us begin with a methodological obstacle that is faced by both Millean styles of analysis—the necessity of dichotomizing every variable in the analysis. Recall that, as with most‐similar analysis, differences across cases must generally be sizeable enough to be interpretable in an essentially dichotomous fashion (e.g. high/low, present/absent) and similarities must be close enough to be understood as essentially identical (e.g. high/high, present/present). Otherwise the results of a Millean style analysis are not interpretable. The problem of “degrees” is deadly if the variables under consideration are, by nature, continuous (e.g. GDP). This is a particular concern in Howard's analysis, where East Germany scores somewhat higher than Russia in civic engagement; they are both low, but Russia is quite a bit lower. Howard assumes that this divergence is minimal enough to be understood as a difference of degrees rather than of kinds, a judgment that might be questioned. In these respects, most‐different analysis is no more secure—but also no less—than most‐similar analysis.
In one respect, most‐different analysis is superior to most‐similar analysis. If the coding assumptions are sound, the most‐different research design may be quite useful for eliminating necessary causes . Causal factors that do not appear across the chosen cases—e.g. X 2a–d in Table 28.4 —are evidently unnecessary for the production of Y . However, it does not follow that the most‐different method is the best method for eliminating necessary causes. Note that the defining feature of this method is the shared element across cases— X 1 in Table 28.4 . This feature does not help one to eliminate necessary causes. Indeed, if one were focused solely on eliminating necessary causes one would presumably seek out cases that register the same outcomes and have maximum diversity on other attributes. In Table 28.4 , this would be a set of cases that satisfy conditions X 2a–d , but not X 1 . Thus, even the presumed strength of the most‐different analysis is not so strong.
Usually, case‐study analysis is focused on the identification (or clarification) of causal relations, not the elimination of possible causes. In this setting, the most‐ different technique is useful, but only if assumptions of causal uniqueness hold. By “causal uniqueness,” I mean a situation in which a given outcome is the product of only one cause: Y cannot occur except in the presence of X . X is necessary, and in some situations (given certain background conditions) sufficient, to cause Y . 22
Consider the following hypothetical example. Suppose that a new disease, about which little is known, has appeared in Country A. There are hundreds of infected persons across dozens of affected communities in that country. In Country B, located at the other end of the world, several new cases of the disease surface in a single community. In this setting, we can imagine two sorts of Millean analyses. The first examines two similar communities within Country A, one of which has developed the disease and the other of which has not. This is the most‐similar style of case comparison, and focuses accordingly on the identification of a difference between the two cases that might account for variation across the sample. A second approach focuses on communities where the disease has appeared across the two countries and searches for any similarities that might account for these similar outcomes. This is the most‐different research design.
Both are plausible approaches to this particular problem, and we can imagine epidemiologists employing them simultaneously. However, the most‐different design demands stronger assumptions about the underlying factors at work. It supposes that the disease arises from the same cause in any setting. This is often a reasonable operating assumption when one is dealing with natural phenomena, though there are certainly many exceptions. Death, for example, has many causes. For this reason, it would not occur to us to look for most‐different cases of high mortality around the world. In order for the most‐different research design to effectively identify a causal factor at work in a given outcome, the researcher must assume that X 1 —the factor held constant across the diverse cases—is the only possible cause of Y (see Table 28.4 ). This assumption rarely holds in social‐scientific settings. Most outcomes of interest to anthropologists, economists, political scientists, and sociologists have multiple causes. There are many ways to win an election, to build a welfare state, to get into a war, to overthrow a government, or—returning to Marc Howard's work—to build a strong civil society. And it is for this reason that most‐different analysis is rarely applied in social science work and, where applied, is rarely convincing.
If this seems a tad severe, there is a more charitable way of approaching the most‐different method. Arguably, this is not a pure “method” at all but merely a supplement, a way of incorporating diversity in the sub‐sample of cases that provide the unusual outcome of interest. If the unusual outcome is revolutions, one might wish to encompass a wide variety of revolutions in one's analysis. If the unusual outcome is post‐Communist civil society, it seems appropriate to include a diverse set of post‐Communist polities in one's sample of case studies, as Marc Howard does. From this perspective, the most‐different method (so‐called) might be better labeled a diverse‐case method, as explored above.
10 Conclusions
In order to be a case of something broader than itself, the chosen case must be representative (in some respects) of a larger population. Otherwise—if it is purely idiosyncratic (“unique”)—it is uninformative about anything lying outside the borders of the case itself. A study based on a nonrepresentative sample has no (or very little) external validity. To be sure, no phenomenon is purely idiosyncratic; the notion of a unique case is a matter that would be difficult to define. One is concerned, as always, with matters of degree. Cases are more or less representative of some broader phenomenon and, on that score, may be considered better or worse subjects for intensive analysis. (The one exception, as noted, is the influential case.)
Of all the problems besetting case‐study analysis, perhaps the most persistent— and the most persistently bemoaned—is the problem of sample bias ( Achen and Snidal 1989 ; Collier and Mahoney 1996 ; Geddes 1990 ; King, Keohane, and Verba 1994 ; Rohlfing 2004 ; Sekhon 2004 ). Lisa Martin (1992 , 5) finds that the overemphasis of international relations scholars on a few well‐known cases of economic sanctions— most of which failed to elicit any change in the sanctioned country—“has distorted analysts view of the dynamics and characteristics of economic sanctions.” Barbara Geddes (1990) charges that many analyses of industrial policy have focused exclusively on the most successful cases—primarily the East Asian NICs—leading to biased inferences. Anna Breman and Carolyn Shelton (2001) show that case‐study work on the question of structural adjustment is systematically biased insofar as researchers tend to focus on disaster cases—those where structural adjustment is associated with very poor health and human development outcomes. These cases, often located in sub‐Saharan Africa, are by no means representative of the entire population. Consequently, scholarship on the question of structural adjustment is highly skewed in a particular ideological direction (against neoliberalism) (see also Gerring, Thacker, and Moreno 2005) .
These examples might be multiplied many times. Indeed, for many topics the most‐studied cases are acknowledged to be less than representative. It is worth reflecting upon the fact that our knowledge of the world is heavily colored by a few “big” (populous, rich, powerful) countries, and that a good portion of the disciplines of economics, political science, and sociology are built upon scholars' familiarity with the economics, political science, and sociology of one country, the United States. 23 Case‐study work is particularly prone to problems of investigator bias since so much rides on the researcher's selection of one (or a few) cases. Even if the investigator is unbiased, her sample may still be biased simply by virtue of “random” error (which may be understood as measurement error, error in the data‐generation process, or as an underlying causal feature of the universe).
There are only two situations in which a case‐study researcher need not be concerned with the representativeness of her chosen case. The first is the influential case research design, where a case is chosen because of its possible influence on a cross‐case model, and hence is not expected to be representative of a larger sample. The second is the deviant‐case method, where the chosen case is employed to confirm a broader cross‐case argument to which the case stands as an apparent exception. Yet even here the chosen case is expected to be representative of a broader set of cases—those, in particular, that are poorly explained by the extant model.
In all other circumstances, cases must be representative of the population of interest in whatever ways might be relevant to the proposition in question. Note that where a researcher is attempting to disconfirm a deterministic proposition the question of representativeness is perhaps more appropriately understood as a question of classification: Is the chosen case appropriately classified as a member of the designated population? If so, then it is fodder for a disconfirming case study.
If the researcher is attempting to confirm a deterministic proposition, or to make probabilistic arguments about a causal relationship, then the problem of representativeness is of the more usual sort: Is case A unit‐homogeneous relative to other cases in the population? This is not an easy matter to test. However, in a large‐ N context the residual for that case (in whatever model the researcher has greatest confidence in) is a reasonable place to start. Of course, this test is only as good as the model at hand. Any incorrect specifications or incorrect modeling procedures will likely bias the results and give an incorrect assessment of each case's “typicality.” In addition, there is the possibility of stochastic error, errors that cannot be modeled in a general framework. Given the explanatory weight that individual cases are asked to bear in a case‐study analysis, it is wise to consider more than just the residual test of representativeness. Deductive logic and an in‐depth knowledge of the case in question are often more reliable tools than the results of a cross‐case model.
In any case, there is no dispensing with the question. Case studies (with the two exceptions already noted) rest upon an assumed synecdoche: The case should stand for a population. If this is not true, or if there is reason to doubt this assumption, then the utility of the case study is brought severely into question.
Fortunately, there is some safety in numbers. Insofar as case‐study evidence is combined with cross‐case evidence the issue of sample bias is mitigated. Indeed, the suspicion of case‐study work that one finds in the social sciences today is, in my view, a product of a too‐literal interpretation of the case‐study method. A case study tout court is thought to mean a case study tout seul . Insofar as case studies and cross‐case studies can be enlisted within the same investigation (either in the same study or by reference to other studies in the same subfield), problems of representativeness are less worrisome. This is the virtue of cross‐level work, a.k.a. “triangulation.”
11 Ambiguities
Before concluding, I wish to draw attention to two ambiguities in case‐selection strategies in case‐study research. The first concerns the admixture of several case‐ selection strategies. The second concerns the changing status of a case as a study proceeds.
Some case studies follow only one strategy of case selection. They are typical , diverse , extreme , deviant , influential , crucial , pathway , most‐similar , or most‐different research designs, as discussed. However, many case studies mix and match among these case‐selection strategies. Indeed, insofar as all case studies seek representative samples, they are always in search of “typical” cases. Thus, it is common for writers to declare that their case is, for example, both extreme and typical; it has an extreme value on X 1 or Y but is not, in other respects, idiosyncratic. There is not much that one can say about these combinations of strategies except that, where the cases allow for a variety of empirical strategies, there is no reason not to pursue them. And where the same cases can serve several functions at once (without further effort on the researcher's part), there is little cost to a multi‐pronged approach to case analysis.
The second issue that deserves emphasis is the changing status of a case during the course of a researcher's investigation—which may last for years, if not decades. The problem is acute wherever a researcher begins in an exploratory mode and proceeds to hypothesis‐testing (that is, she develops a specific X 1 / Y proposition) or where the operative hypothesis or key control variable changes (a new causal factor is discovered or another outcome becomes the focus of analysis). Things change. And it is the mark of a good researcher to keep her mind open to new evidence and new insights. Too often, methodological discussions give the misleading impression that hypotheses are clear and remain fixed over the course of a study's development. Nothing could be further from the truth. The unofficial transcripts of academia— accessible in informal settings, where researchers let their guards down (particularly if inebriated)—are filled with stories about dead‐ends, unexpected findings, and drastically revised theory chapters. It would be interesting, in this vein, to compare published work with dissertation prospectuses and fellowship applications. I doubt if the correlation between these two stages of research is particularly strong.
Research, after all, is about discovery, not simply the verification or falsification of static hypotheses. That said, it is also true that research on a particular topic should move from hypothesis generating to hypothesis‐testing. This marks the progress of a field, and of a scholar's own work. As a rule, research that begins with an open‐ended ( X ‐ or Y ‐centered) analysis should conclude with a determinate X 1 / Y hypothesis.
The problem is that research strategies that are ideal for exploration are not always ideal for confirmation. The extreme‐case method is inherently exploratory since there is no clear causal hypothesis; the researcher is concerned merely to explore variation on a single dimension ( X or Y ). Other methods can be employed in either an open‐ ended (exploratory) or a hypothesis‐testing (confirmatory/disconfirmatory) mode. The difficulty is that once the researcher has arrived at a determinate hypothesis the originally chosen research design may no longer appear to be so well designed.
This is unfortunate, but inevitable. One cannot construct the perfect research design until (a) one has a specific hypothesis and (b) one is reasonably certain about what one is going to find “out there” in the empirical world. This is particularly true of observational research designs, but it also applies to many experimental research designs: Usually, there is a “good” (informative) finding, and a finding that is less insightful. In short, the perfect case‐study research design is usually apparent only ex post facto .
There are three ways to handle this. One can explain, straightforwardly, that the initial research was undertaken in an exploratory fashion, and therefore not constructed to test the specific hypothesis that is—now—the primary argument. Alternatively, one can try to redesign the study after the new (or revised) hypothesis has been formulated. This may require additional field research or perhaps the integration of additional cases or variables that can be obtained through secondary sources or through consultation of experts. A final approach is to simply jettison, or de‐emphasize, the portion of research that no longer addresses the (revised) key hypothesis. A three‐case study may become a two‐case study, and so forth. Lost time and effort are the costs of this downsizing.
In the event, practical considerations will probably determine which of these three strategies, or combinations of strategies, is to be followed. (They are not mutually exclusive.) The point to remember is that revision of one's cross‐case research design is normal and perhaps to be expected. Not all twists and turns on the meandering trail of truth can be anticipated.
12 Are There Other Methods of Case Selection?
At the outset of this chapter I summarized the task of case selection as a matter of achieving two objectives: representativeness (typicality) and variation (causal leverage). Evidently, there are other objectives as well. For example, one wishes to identify cases that are independent of each other. If chosen cases are affected by each other (sometimes known as Galton's problem or a problem of diffusion), this problem must be corrected before analysis can take place. I have neglected this issue because it is usually apparent to the researcher and, in any case, there are no simple techniques that might be utilized to correct for such biases. (For further discussion of this and other factors impinging upon case selection see Gerring 2001 , 178–81.)
I have also disregarded pragmatic/logistical issues that might affect case selection. Evidently, case selection is often influenced by a researcher's familiarity with the language of a country, a personal entrée into that locale, special access to important data, or funding that covers one archive rather than another. Pragmatic considerations are often—and quite rightly—decisive in the case‐selection process.
A final consideration concerns the theoretical prominence of a particular case within the literature on a subject. Researchers are sometimes obliged to study cases that have received extensive attention in previous studies. These are sometimes referred to as “paradigmatic” cases or “exemplars” ( Flyvbjerg 2004 , 427).
However, neither pragmatic/logistical utility nor theoretical prominence qualifies as a methodological factor in case selection. That is, these features of a case have no bearing on the validity of the findings stemming from a study. As such, it is appropriate to grant these issues a peripheral status in this chapter.
One final caveat must be issued. While it is traditional to distinguish among the tasks of case selection and case analysis, a close look at these processes shows them to be indistinct and overlapping. One cannot choose a case without considering the sort of analysis that it might be subjected to, and vice versa. Thus, the reader should consider choosing cases by employing the nine techniques laid out in this chapter along with any considerations that might be introduced by virtue of a case's quasi‐experimental qualities, a topic taken up elsewhere ( Gerring 2007 , ch. 6 ).
Abadie, A. , Drukker, D. , Herr, J. L. , and Imbens, G. W. 2001 . Implementing matching estimators for average treatment effects in Stata. Stata Journal , 1: 1–18.
Google Scholar
Abbott, A. 2001 . Time Matters: On Theory and Method . Chicago: University of Chicago Press.
Google Preview
—— and Tsay, A. 2000 . Sequence analysis and optimal matching methods in sociology. Sociological Methods and Research , 29: 3–33. 10.1177/0049124100029001001
—— and Forrest, J. 1986 . Optimal matching methods for historical sequences. Journal of Interdisciplinary History , 16: 471–94. 10.2307/204500
Achen, C. H. , and Snidal, D. 1989 . Rational deterrence theory and comparative case studies. World Politics , 41: 143–69. 10.2307/2010405
Allen, W. S. 1965 . The Nazi Seizure of Power: The Experience of a Single German Town, 1930–1935 . New York: Watts.
Almond, G. A. 1956 . Comparative political systems. Journal of Politics , 18: 391–409.
Amenta, E. 1991 . Making the most of a case study: theories of the welfare state and the American experience. Pp. 172–94 in Issues and Alternatives in Comparative Social Research ed. C. C. Ragin . Leiden: E. J. Brill.
Barro, R. J. 1999 . Determinants of democracy. Journal of Political Economy , 107: 158–83. 10.1086/250107
Belsey, D. A. , Kuh, E. , and Welsch, R. E. 2004 . Regression Diagnostics: Identifying Influential Data and Sources of Collinearity . New York: Wiley.
Bennett, A. , Lepgold, J. , and Unger, D. 1994 . Burden‐sharing in the Persian Gulf War. International Organization , 48: 39–75. 10.1017/S0020818300000813
Bentley, A. 1908/ 1967 . The Process of Government . Cambridge, Mass.: Harvard University Press.
Brady, H. E. , and Collier, D. (eds.) 2004 . Rethinking Social Inquiry: Diverse Tools, Shared Standards . Lanham, Md.: Rowman and Littlefield.
Braumoeller, B. F. 2003 . Causal complexity and the study of politics. Political Analysis , 11: 209–33. 10.1093/pan/mpg012
Breman, A. , and Shelton, C. 2001. Structural adjustment and health: a literature review of the debate, its role‐players and presented empirical evidence. CMH Working Paper Series, Paper No. WG6: 6. WHO, Commission on Macroeconomics and Health.
Brenner, R. 1976 . Agrarian class structure and economic development in pre‐industrial Europe. Past and Present , 70: 30–75. 10.1093/past/70.1.30
Browne, A. 1987 . When Battered Women Kill . New York: Free Press.
Buchbinder, S. , and Vittinghoff, E. 1999 . HIV‐infected long‐term nonprogressors: epidemiology, mechanisms of delayed progression, and clinical and research implications. Microbes Infect , 1: 1113–20. 10.1016/S1286-4579(99)00204-X
Cohen, M. R. , and Nagel, E. 1934 . An Introduction to Logic and Scientific Method . New York: Harcourt, Brace and Company.
Collier, D. , and Mahoney, J. 1996 . Insights and pitfalls: selection bias in qualitative research. World Politics , 49: 56–91. 10.1353/wp.1996.0023
Collier, R. B. , and Collier, D. 1991/ 2002 . Shaping the Political Arena: Critical Junctures, the Labor Movement, and Regime Dynamics in Latin America . Notre Dame, Ind.: University of Notre Dame Press.
Colomer, J. M. 1991 . Transitions by agreement: modeling the Spanish way. American Political Science Review , 85: 1283–302. 10.2307/1963946
Converse, P. E. , and Dupeux, G. 1962 . Politicization of the electorate in France and the United States. Public Opinion Quarterly , 16: 1–23. 10.1086/267067
Coppedge, M. J. 2004. The conditional impact of the economy on democracy in Latin America. Presented at the conference “Democratic Advancements and Setbacks: What Have We Learnt?”, Uppsala University, June 11–13.
De Felice, E. G. 1986 . Causal inference and comparative methods. Comparative Political Studies , 19: 415–37. 10.1177/0010414086019003005
Desch, M. C. 2002 . Democracy and victory: why regime type hardly matters. International Security , 27: 5–47. 10.1162/016228802760987815
Deyo, F. (ed.) 1987 . The Political Economy of the New Asian Industrialism . Ithaca, NY: Cornell University Press.
Dion, D. 1998 . Evidence and inference in the comparative case study. Comparative Politics , 30: 127–45. 10.2307/422284
Eckstein, H. 1975 . Case studies and theory in political science. In Handbook of Political Science , vii: Political Science: Scope and Theory , ed. F. I. Greenstein and N. W. Polsby . Reading, Mass.: Addison‐Wesley.
Eggan, F. 1954 . Social anthropology and the method of controlled comparison. American Anthropologist , 56: 743–63. 10.1525/aa.1954.56.5.02a00020
Elman, C. 2003 . Lessons from Lakatos. In Progress in International Relations Theory: Appraising the Field , ed. C. Elman and M. F. Elman . Cambridge, Mass.: MIT Press.
—— 2005 . Explanatory typologies in qualitative studies of international politics. International Organization , 59: 293–326.
Emigh, R. 1997 . The power of negative thinking: the use of negative case methodology in the development of sociological theory. Theory and Society , 26: 649–84. 10.1023/A:1006896217647
Epstein, L. D. 1964 . A comparative study of Canadian parties. American Political Science Review , 58: 46–59. 10.2307/1952754
Ertman, T. 1997 . Birth of the Leviathan: Building States and Regimes in Medieval and Early Modern Europe . Cambridge: Cambridge University Press.
Esping‐Andersen, G. 1990 . The Three Worlds of Welfare Capitalism . Princeton, NJ: Princeton University Press.
Flyvbjerg, B. 2004 . Five misunderstandings about case‐study research. Pp. 420–34 in Qualitative Research Practice , ed. C. Seale , G. Gobo , J. F. Gubrium , and D. Silverman . London: Sage.
Geddes, B. 1990 . How the cases you choose affect the answers you get: selection bias in comparative politics. In Political Analysis , vol. ii, ed. J. A. Stimson . Ann Arbor: University of Michigan Press.
—— 2003 . Paradigms and Sand Castles: Theory Building and Research Design in Comparative Politics . Ann Arbor: University of Michigan Press.
George, A. L. , and Bennett, A. 2005 . Case Studies and Theory Development . Cambridge, Mass.: MIT Press.
—— and Smoke, R. 1974 . Deterrence in American Foreign Policy: Theory and Practice . New York: Columbia University Press.
Gerring, J. 2001 . Social Science Methodology: A Criterial Framework . Cambridge: Cambridge University Press.
—— 2007 . Case Study Research: Principles and Practices . Cambridge: Cambridge University Press.
—— Thacker, S. and Moreno, C. 2005. Do neoliberal policies save lives? Unpublished manuscript.
Goertz, G. and Starr, H. (eds.) 2003 . Necessary Conditions: Theory, Methodology and Applications . New York: Rowman and Littlefield.
—— and Levy, J. (eds.) forthcoming. Causal explanations, necessary conditions, and case studies: World War I and the end of the Cold War. Manuscript.
Goodin, R. E. and Smitsman, A. 2000 . Placing welfare states: the Netherlands as a crucial test case. Journal of Comparative Policy Analysis , 2: 39–64. 10.1080/13876980008412635
Gujarati, D. N. 2003 . Basic Econometrics , 4th edn. New York: McGraw‐Hill.
Hamilton, G. G. 1977 . Chinese consumption of foreign commodities: a comparative perspective. American Sociological Review , 42: 877–91. 10.2307/2094574
Haynes, B. F. Pantaleo, G. and Fauci, A. S. 1996 . Toward an understanding of the correlates of protective immunity to HIV infection. Science , 271: 324–8. 10.1126/science.271.5247.324
Hempel, C. G. 1942 . The function of general laws in history. Journal of Philosophy , 39: 35–48. 10.2307/2017635
Ho, D. E. Imai, K. King, G. and Stuart, E. A. 2004. Matching as nonparametric preprocessing for reducing model dependence in parametric causal inference. Manuscript.
Howard, M. M. 2003 . The Weakness of Civil Society in Post‐Communist Europe . Cambridge: Cambridge University Press.
Howson, C. and Urbach, P. 1989 . Scientific Reasoning: The Bayesian Approach . La Salle, Ill.: Open Court.
Humphreys, M. 2005 . Natural resources, conflict, and conflict resolution: uncovering the mechanisms. Journal of Conflict Resolution , 49: 508–37. 10.1177/0022002705277545
Jenicek, M. 2001 . Clinical Case Reporting in Evidence‐Based Medicine , 2nd edn. Oxford: Oxford University Press.
Karl, T. L. 1997 . The Paradox of Plenty: Oil Booms and Petro‐states . Berkeley: University of California Press.
Kazancigil, A. 1994 . The deviant case in comparative analysis: high stateness in comparative analysis. Pp. 213–38 in Comparing Nations: Concepts, Strategies, Substance , ed. M. Dogan and A. Kazancigil . Cambridge: Blackwell.
Kemp, K. A. 1986 . Race, ethnicity, class and urban spatial conflict: Chicago as a crucial case Urban Studies , 23: 197–208. 10.1080/00420988620080231
Kendall, P. L. and Wolf, K. M. 1949/ 1955 . The analysis of deviant cases in communications research. In Communications Research, 1948–1949 , ed. P. F. Lazarsfeld and F. N. Stanton. New York: Harper and Brothers. Reprinted as pp. 167–70 in The Language of Social Research , ed. P. F. Lazarsfeld and M. Rosenberg . New York: Free Press.
Kennedy, C. H. 2005 . Single‐case Designs for Educational Research . Boston: Allyn and Bacon.
Kennedy, P. 2003 . A Guide to Econometrics , 5th edn. Cambridge, Mass.: MIT Press.
Khong, Y. F. 1992 . Analogies at War: Korea, Munich, Dien Bien Phu, and the Vietnam Decisions of 1965 . Princeton, NJ: Princeton University Press.
King, G. Keohane, R. O. and Verba, S. 1994 . Designing Social Inquiry: Scientific Inference in Qualitative Research . Princeton, NJ: Princeton University Press.
Lakatos, I. 1978 . The Methodology of Scientific Research Programmes . Cambridge: Cambridge University Press.
Lazarsfeld, P. F. and Barton, A. H. 1951 . Qualitative measurement in the social sciences: classification, typologies, and indices. In The Policy Sciences , ed. D. Lerner and H. D. Lass‐ well. Stanford, Calif.: Stanford University Press.
Levy, J. S. 2002 . Qualitative methods in international relations. In Evaluating Methodology in International Studies , ed. F. P. Harvey and M. Brecher. Ann Arbor: University of Michigan Press.
Lijphart, A. 1968 . The Politics of Accommodation: Pluralism and Democracy in the Netherlands . Berkeley: University of California Press.
—— 1969 . Consociational democracy. World Politics , 21: 207–25. 10.2307/2009820
—— 1971 . Comparative politics and the comparative method. American Political Science Review , 65: 682–93.
—— 1975 . The comparable cases strategy in comparative research. Comparative Political Studies , 8: 158–77.
Lipset, S. M. 1959 . Some social requisites of democracy: economic development and political development. American Political Science Review , 53: 69–105. 10.2307/1951731
—— 1960/ 1963 . Political Man: The Social Bases of Politics . Garden City, NY: Anchor.
—— 1968 . Agrarian Socialism: The Cooperative Commonwealth Federation in Saskatchewan. A Study in Political Sociology . Garden City, NY: Doubleday.
—— Trow, M. A. and Coleman, J. S. 1956 . Union Democracy: The Internal Politics of the International Typographical Union . New York: Free Press.
Lynd, R. S. and Lynd, H. M. 1929/ 1956 . Middletown: A Study in American Culture . New York: Harcourt, Brace.
Mahoney, J. and Goertz, G. 2004 . The possibility principle: choosing negative cases in comparative research. American Political Science Review , 98: 653–69.
Martin, L. L. 1992 . Coercive Cooperation: Explaining Multilateral Economic Sanctions .Princeton, NJ: Princeton University Press.
Mayo, D. G. 1996 . Error and the Growth of Experimental Knowledge . Chicago: University of Chicago Press.
Meckstroth, T. 1975 . “Most different systems” and “most similar systems:” a study in the logic of comparative inquiry. Comparative Political Studies , 8: 133–77.
Miguel, E. 2004 . Tribe or nation: nation‐building and public goods in Kenya versus Tanzania. World Politics , 56: 327–62. 10.1353/wp.2004.0018
Mill, J. S. 1843/ 1872 . The System of Logic , 8th edn. London: Longmans, Green.
Monroe, K. R. 1996 . The Heart of Altruism: Perceptions of a Common Humanity . Princeton, NJ: Princeton University Press.
Moore, B., Jr. 1966 . Social Origins of Dictatorship and Democracy: Lord and Peasant in the Making of the Modern World . Boston: Beacon Press.
Morgan, S. L. and Harding, D. J. 2005. Matching estimators of causal effects: from stratification and weighting to practical data analysis routines. Manuscript.
Moulder, F. V. 1977 . Japan, China and the Modern World Economy: Toward a Reinterpretation of East Asian Development ca. 1600 to ca. 1918 . Cambridge: Cambridge University Press.
Munck, G. L. 2004 . Tools for qualitative research. Pp. 105–21 in Rethinking Social Inquiry: Diverse Tools, Shared Standards , ed. H. E. Brady and D. Collier . Lanham, Md. : Rowman and Littlefield.
Njolstad, O. 1990 . Learning from history? Case studies and the limits to theory‐building. Pp. 220–46 in Arms Races: Technological and Political Dynamics , ed. O. Njolstad . Thousand Oaks, Calif.: Sage.
Patton, M. Q. 2002 . Qualitative Evaluation and Research Methods . Newbury Park, Calif.: Sage.
Popper, K. 1934/ 1968 . The Logic of Scientific Discovery . New York: Harper and Row.
—— 1963 . Conjectures and Refutations . London: Routledge and Kegan Paul.
Posner, D. 2004 . The political salience of cultural difference: why Chewas and Tumbukas are allies in Zambia and adversaries in Malawi. American Political Science Review , 98: 529–46.
Przeworski, A. and Teune, H. 1970 . The Logic of Comparative Social Inquiry . New York: John Wiley.
Queen, S. 1928 . Round table on the case study in sociological research. Publications of the American Sociological Society, Papers and Proceedings , 22: 225–7.
Ragin, C. C. 2000 . Fuzzy‐set Social Science . Chicago: University of Chicago Press.
—— 2004 . Turning the tables. Pp. 123–38 in Rethinking Social Inquiry: Diverse Tools, Shared Standards , ed. H. E. Brady and D. Collier. Lanham, Md. : Rowman and Littlefield.
Reilly, B. 2000 –1. Democracy, ethnic fragmentation, and internal conflict: confused theories, faulty data, and the “crucial case” of Papua New Guinea. International Security , 25: 162–85. 10.1162/016228800560552
—— and Phillpot, R. 2003 . “Making democracy work” in Papua New Guinea: social capital and provincial development in an ethnically fragmented society. Asian Survey , 42: 906–27. 10.1525/as.2002.42.6.906
Rogowski, R. 1995 . The role of theory and anomaly in social‐scientific inference. American Political Science Review , 89: 467–70. 10.2307/2082443
Rohlfing, I. 2004. Have you chosen the right case? Uncertainty in case selection for single case studies. Working Paper, International University, Bremen.
Rosenbaum, P. R. 2004 . Matching in observational studies. In Applied Bayesian Modeling and Causal Inference from an Incomplete‐data Perspective , ed. A. Gelman and X.‐L. Meng . New York: John Wiley.
—— and Silber, J. H. 2001 . Matching and thick description in an observational study of mortality after surgery. Biostatistics , 2: 217–32. 10.1093/biostatistics/2.2.217
Ross, M. 2001 . Does oil hinder democracy? World Politics , 53: 325–61. 10.1353/wp.2001.0011
Sagan, S. D. 1995 . Limits of Safety: Organizations, Accidents, and Nuclear Weapons . Princeton, NJ: Princeton University Press.
Sekhon, J. S. 2004 . Quality meets quantity: case studies, conditional probability and counter‐ factuals. Perspectives in Politics , 2: 281–93.
Shafer, M. D. 1988 . Deadly Paradigms: The Failure of U.S. Counterinsurgency Policy . Princeton, NJ: Princeton University Press.
Skocpol, T. 1979 . States and Social Revolutions: A Comparative Analysis of France, Russia, and China . Cambridge: Cambridge University Press.
—— and Somers, M. 1980 . The uses of comparative history in macrosocial inquiry. Comparative Studies in Society and History , 22: 147–97.
Stinchcombe, A. L. 1968 . Constructing Social Theories . New York: Harcourt, Brace.
Swank, D. H. 2002 . Global Capital, Political Institutions, and Policy Change in Developed Welfare States . Cambridge: Cambridge University Press.
Tendler, J. 1997 . Good Government in the Tropics . Baltimore: Johns Hopkins University Press.
Truman, D. B. 1951 . The Governmental Process . New York: Alfred A. Knopf.
Tsai, L. 2007 . Accountability without Democracy: How Solidary Groups Provide Public Goods in Rural China . Cambridge: Cambridge University Press.
Van Evera, S. 1997 . Guide to Methods for Students of Political Science . Ithaca, NY: Cornell University Press.
Wahlke, J. C. 1979 . Pre‐behavioralism in political science. American Political Science Review , 73: 9–31. 10.2307/1954728
Yashar, D. J. 2005 . Contesting Citizenship in Latin America: The Rise of Indigenous Movements and the Postliberal Challenge . Cambridge: Cambridge University Press.
Yin, R. K. 2004 . Case Study Anthology . Thousand Oaks, Calif.: Sage.
Gujarati (2003) ; Kennedy (2003) . Interestingly, the potential of cross‐case statistics in helping to choose cases for in‐depth analysis is recognized in some of the earliest discussions of the case‐study method (e.g. Queen 1928 , 226).
This expands on Mill (1843/1872 , 253), who wrote of scientific enquiry as twofold: “either inquiries into the cause of a given effect or into the effects or properties of a given cause.”
This method has not received much attention on the part of qualitative methodologists; hence, the absence of a generally recognized name. It bears some resemblance to J. S. Mill's Joint Method of Agreement and Difference ( Mill 1843/1872 ), which is to say a mixture of most‐similar and most‐different analysis, as discussed below. Patton (2002 , 234) employs the concept of “maximum variation (heterogeneity) sampling.”
More precisely, George and Smoke (1974 , 534, 522–36, ch. 18 ; see also discussion in Collier and Mahoney 1996 , 78) set out to investigate causal pathways and discovered, through the course of their investigation of many cases, these three causal types. Yet, for our purposes what is important is that the final sample includes at least one representative of each “type.”
For further examples see Collier and Mahoney (1996) ; Geddes (1990) ; Tendler (1997) .
Traditionally, methodologists have conceptualized cases as having “positive” or “negative” values (e.g. Emigh 1997 ; Mahoney and Goertz 2004 ; Ragin 2000 , 60; 2004 , 126).
Geddes (1990) ; King, Keohane, and Verba (1994) . See also discussion in Brady and Collier (2004) ; Collier and Mahoney (1996) ; Rogowski (1995) .
The exception would be a circumstance in which the researcher intends to disprove a deterministic argument ( Dion 1998 ).
Geddes (2003 , 131). For other examples of casework from the annals of medicine see “Clinical reports” in the Lancet , “Case studies” in Canadian Medical Association Journal , and various issues of the Journal of Obstetrics and Gynecology , often devoted to clinical cases (discussed in Jenicek 2001 , 7). For examples from the subfield of comparative politics see Kazancigil (1994) .
For a discussion of the important role of anomalies in the development of scientific theorizing see Elman (2003) ; Lakatos (1978) . For examples of deviant‐case research designs in the social sciences see Amenta (1991) ; Coppedge (2004) ; Eckstein (1975) ; Emigh (1997) ; Kendall and Wolf (1949/1955) .
For examples of the crucial‐case method see Bennett, Lepgold, and Unger (1994) ; Desch (2002) ; Goodin and Smitsman (2000) ; Kemp (1986) ; Reilly and Phillpot (2003) . For general discussion see George and Bennett (2005) ; Levy (2002) ; Stinchcombe (1968 , 24–8).
A third position, which purports to be neither Popperian or Bayesian, has been articulated by Mayo (1996 , ch. 6 ). From this perspective, the same idea is articulated as a matter of “severe tests.”
It should be noted that Tsai's conclusions do not rest solely on this crucial case. Indeed, she employs a broad range of methodological tools, encompassing case‐study and cross‐case methods.
See also the discussion in Eckstein (1975) and Lijphart (1969) . For additional examples of case studies disconfirming general propositions of a deterministic nature see Allen (1965); Lipset, Trow, and Coleman (1956) ; Njolstad (1990) ; Reilly (2000–1) ; and discussion in Dion (1998) ; Rogowski (1995) .
Granted, insofar as case‐study analysis provides a window into causal mechanisms, and causal mechanisms are integral to a given theory, a single case may be enlisted to confirm or disconfirm a proposition. However, if the case study upholds a posited pattern of X/Y covariation, and finds fault only with the stipulated causal mechanism, it would be more accurate to say that the study forces the reformulation of a given theory, rather than its confirmation or disconfirmation. See further discussion in the following section.
Sometimes, the most‐similar method is known as the “method of difference,” after its inventor ( Mill 1843/1872 ). For later treatments see Cohen and Nagel (1934) ; Eggan (1954) ; Gerring (2001 , ch. 9 ); Lijphart (1971 ; 1975) ; Meckstroth (1975) ; Przeworski and Teune (1970) ; Skocpol and Somers (1980) .
For good introductions see Ho et al. (2004) ; Morgan and Harding (2005) ; Rosenbaum (2004) ; Rosenbaum and Silber (2001) . For a discussion of matching procedures in Stata see Abadie et al. (2001) .
The most‐different method is also sometimes referred to as the “method of agreement,” following its inventor, J. S. Mill (1843/1872) . See also De Felice (1986) ; Gerring (2001 , 212–14); Lijphart (1971 ; 1975) ; Meckstroth (1975) ; Przeworski and Teune (1970) ; Skocpol and Somers (1980) . For examples of this method see Collier and Collier (1991/2002) ; Converse and Dupeux (1962) ; Karl (1997) ; Moore (1966) ; Skocpol (1979) ; Yashar (2005 , 23). However, most of these studies are described as combining most‐similar and most‐different methods.
In the following discussion I treat the terms social capital, civil society, and civic engagement interchangeably.
E.g. Collier and Collier (1991/2002) ; Karl (1997) ; Moore (1966) ; Skocpol (1979) ; Yashar (2005 , 23). Karl (1997) , which affects to be a most‐different system analysis (20), is a particularly clear example of this. Her study, focused ostensibly on petro‐states (states with large oil reserves), makes two sorts of inferences. The first concerns the (usually) obstructive role of oil in political and economic development. The second sort of inference concerns variation within the population of petro‐states, showing that some countries (e.g. Norway, Indonesia) manage to avoid the pathologies brought on elsewhere by oil resources. When attempting to explain the constraining role of oil on petro‐states, Karl usually relies on contrasts between petro‐states and nonpetro‐states (e.g. ch. 10 ). Only when attempting to explain differences among petro‐states does she restrict her sample to petro‐states. In my opinion, very little use is made of the most‐different research design.
This was recognized, at least implicitly, by Mill (1843/1872 , 258–9). Skepticism has been echoed by methodologists in the intervening years (e.g. Cohen and Nagel 1934 , 251–6; Gerring 2001 ; Skocpol and Somers 1980 ). Indeed, explicit defenses of the most‐different method are rare (but see De Felice 1986 ).
Another way of stating this is to say that X is a “nontrivial necessary condition” of Y .
Wahlke (1979 , 13) writes of the failings of the “behavioralist” mode of political science analysis: “It rarely aims at generalization; research efforts have been confined essentially to case studies of single political systems, most of them dealing …with the American system.”
- About Oxford Academic
- Publish journals with us
- University press partners
- What we publish
- New features
- Open access
- Institutional account management
- Rights and permissions
- Get help with access
- Accessibility
- Advertising
- Media enquiries
- Oxford University Press
- Oxford Languages
- University of Oxford
Oxford University Press is a department of the University of Oxford. It furthers the University's objective of excellence in research, scholarship, and education by publishing worldwide
- Copyright © 2024 Oxford University Press
- Cookie settings
- Cookie policy
- Privacy policy
- Legal notice
This Feature Is Available To Subscribers Only
Sign In or Create an Account
This PDF is available to Subscribers Only
For full access to this pdf, sign in to an existing account, or purchase an annual subscription.

- SUGGESTED TOPICS
- The Magazine
- Newsletters
- Managing Yourself
- Managing Teams
- Work-life Balance
- The Big Idea
- Data & Visuals
- Reading Lists
- Case Selections
- HBR Learning
- Topic Feeds
- Account Settings
- Email Preferences
HBS Case Selections

Innovation at Moog Inc.
- Brian J. Hall
- Ashley V. Whillans
- Davis Heniford
- Dominika Randle
- Caroline Witten
Innovation at Google Ads: The Sales Acceleration and Innovation Labs (SAIL) (A)
- Linda A. Hill
- Emily Tedards
Juan Valdez: Innovation in Caffeination
- Michael I. Norton
- Jeremy Dann
UGG Steps into the Metaverse
- Shunyuan Zhang
- Sharon Joseph
- Sunil Gupta
- Julia Kelley
Metaverse Wars
- David B. Yoffie
- Matt Higgins
Roblox: Virtual Commerce in the Metaverse
- Ayelet Israeli
- Nicole Tempest Keller
Timnit Gebru: "SILENCED No More" on AI Bias and The Harms of Large Language Models
- Tsedal Neeley
- Stefani Ruper
Hugging Face: Serving AI on a Platform
- Shane Greenstein
- Kerry Herman
- Sarah Gulick
SmartOne: Building an AI Data Business
- Karim R. Lakhani
- Pippa Tubman Armerding
- Gamze Yucaoglu
- Fares Khrais
Honeywell and the Great Recession (A)
- Sandra J. Sucher
- Susan Winterberg
Target: Responding to the Recession
- Ranjay Gulati
- Catherine Ross
- Richard S. Ruback
- Royce Yudkoff
Hometown Foods: Changing Price Amid Inflation
- Julian De Freitas
- Jeremy Yang
- Das Narayandas
Elon Musk's Big Bets
- Eric Baldwin
Elon Musk: Balancing Purpose and Risk
- Shikhar Ghosh
- Sarah Mehta
Tesla's CEO Compensation Plan
- Krishna G. Palepu
- John R. Wells
- Gabriel Ellsworth
China Rapid Finance: The Collapse of China's P2P Lending Industry
- William C. Kirby
- Bonnie Yining Cao
- John P. McHugh
Forbidden City: Launching a Craft Beer in China
- Christopher A. Bartlett
- Carole Carlson
Booking.com
- Stefan Thomke
- Daniela Beyersdorfer
Innovation at Uber: The Launch of Express POOL
- Chiara Farronato
- Alan MacCormack
Racial Discrimination on Airbnb (A)
- Michael Luca
- Scott Stern
- Hyunjin Kim
GitLab and the Future of All-Remote Work (A)
- Prithwiraj Choudhury
- Emma Salomon
TCS: From Physical Offices to Borderless Work
Creating a virtual internship at goldman sachs.
- Iavor Bojinov
Unilever's Response to the Future of Work
- William R. Kerr
- Emilie Billaud
- Mette Fuglsang Hjortshoej
AT&T, Retraining, and the Workforce of Tomorrow
- Joseph B. Fuller
- Carl Kreitzberg
Leading Change in Talent at L'Oreal
- Lakshmi Ramarajan
- Vincent Dessain
- Emer Moloney
- William W. George
- Andrew N. McLean
Eve Hall: The African American Investment Fund in Milwaukee
- Steven S. Rogers
- Alterrell Mills
United Housing - Otis Gates
- Mercer Cook
The Home Depot: Leadership in Crisis Management
- Herman B. Leonard
- Marc J. Epstein
- Melissa Tritter
The Great East Japan Earthquake (B): Fast Retailing Group's Response
- Hirotaka Takeuchi
- Kenichi Nonomura
- Dena Neuenschwander
- Meghan Ricci
- Kate Schoch
- Sergey Vartanov
Insurer of Last Resort?: The Federal Financial Response to September 11
- David A. Moss
- Sarah Brennan
Under Armour
- Rory McDonald
- Clayton M. Christensen
- Daniel West
- Jonathan E. Palmer
- Tonia Junker
Hunley, Inc.: Casting for Growth
- John A. Quelch
- James T. Kindley
Bitfury: Blockchain for Government
- Mitchell B. Weiss
- Elena Corsi
Deutsche Bank: Pursuing Blockchain Opportunities (A)
- Lynda M. Applegate
- Christoph Muller-Bloch
Maersk: Betting on Blockchain
- Scott Johnson
Yum! Brands
- Jordan Siegel
- Christopher Poliquin
Bharti Airtel in Africa
- Tanya Bijlani
Li & Fung 2012
- F. Warren McFarlan
- Michael Shih-ta Chen
- Keith Chi-ho Wong
Sony and the JK Wedding Dance
- John Deighton
- Leora Kornfeld
United Breaks Guitars
David dao on united airlines.
- Benjamin Edelman
- Jenny Sanford
Marketing Reading: Digital Marketing
- Joseph Davin
Social Strategy at Nike
- Mikolaj Jan Piskorski
- Ryan Johnson
The Tate's Digital Transformation
Social strategy at american express, mellon financial and the bank of new york.
- Carliss Y. Baldwin
- Ryan D. Taliaferro
The Walt Disney Company and Pixar, Inc.: To Acquire or Not to Acquire?
- Juan Alcacer
- David J. Collis
Dow's Bid for Rohm and Haas
- Benjamin C. Esty
Finance Reading: The Mergers and Acquisitions Process
- John Coates
Apple: Privacy vs. Safety? (A)
- Henry W. McGee
- Nien-he Hsieh
- Sarah McAra
Sidewalk Labs: Privacy in a City Built from the Internet Up
- Leslie K. John
Data Breach at Equifax
- Suraj Srinivasan
- Quinn Pitcher
- Jonah S. Goldberg
Apple's Core
- Noam Wasserman
Design Thinking and Innovation at Apple
- Barbara Feinberg
Apple Inc. in 2012
- Penelope Rossano
Iz-Lynn Chan at Far East Organization (Abridged)
- Anthony J. Mayo
- Dana M. Teppert
Barbara Norris: Leading Change in the General Surgery Unit
- Boris Groysberg
- Nitin Nohria
- Deborah Bell
Adobe Systems: Working Towards a "Suite" Release (A)
- David A. Thomas
- Lauren Barley
- Jan W. Rivkin
Starbucks Coffee Company: Transformation and Renewal
- Nancy F. Koehn
- Kelly McNamara
- Nora N. Khan
- Elizabeth Legris
JCPenney: Back in Business
- K. Shelette Stewart
- Christine Snively
Home Nursing of North Carolina
Castronics, llc, gemini investors, angie's list: ratings pioneer turns 20.
- Robert J. Dolan
Basecamp: Pricing
- Frank V. Cespedes
- Robb Fitzsimmons
J.C. Penney's "Fair and Square" Pricing Strategy
J.c. penney's 'fair and square' strategy (c): back to the future.
- Jose B. Alvarez
Osaro: Picking the best path
- James Palano
- Bastiane Huang
HubSpot and Motion AI: Chatbot-Enabled CRM
- Thomas Steenburgh
GROW: Using Artificial Intelligence to Screen Human Intelligence
- Ethan S. Bernstein
- Paul D. McKinnon
- Paul Yarabe

Arup: Building the Water Cube
- Robert G. Eccles
- Amy C. Edmondson
- Dilyana Karadzhova
(Re)Building a Global Team: Tariq Khan at Tek
Managing a global team: greg james at sun microsystems, inc. (a).
- Thomas J. DeLong
Organizational Behavior Reading: Leading Global Teams
Ron ventura at mitchell memorial hospital.
- Heide Abelli
Anthony Starks at InSiL Therapeutics (A)
- Gary P. Pisano
- Vicki L. Sato
Wolfgang Keller at Konigsbrau-TAK (A)
- John J. Gabarro
The 2010 Chilean Mining Rescue (A)
- Faaiza Rashid
IDEO: Human-Centered Service Design
- Ryan W. Buell
- Andrew Otazo
- Benjamin Jones
- Alexis Brownell

David Neeleman: Flight Path of a Servant Leader (A)
- Matthew D. Breitfelder
Coach Hurley at St. Anthony High School
- Scott A. Snook
- Bradley C. Lawrence
Shapiro Global
- Michael Brookshire
- Monica Haugen
- Michelle Kravetz
- Sarah Sommer
Kathryn McNeil (A)
- Joseph L. Badaracco Jr.
- Jerry Useem
Carol Fishman Cohen: Professional Career Reentry (A)
- Myra M. Hart
- Robin J. Ely
- Susan Wojewoda
Alex Montana at ESH Manufacturing Co.
- Michael Kernish
Michelle Levene (A)
- Tiziana Casciaro
- Victoria W. Winston
John and Andrea Rice: Entrepreneurship and Life
- Howard H. Stevenson
- Janet Kraus
- Shirley M. Spence
Partner Center

Phase 3 Consulting
Read more about Kate Wadia
- August 11, 2017
System selection: creating the right process
- By Kate Wadia
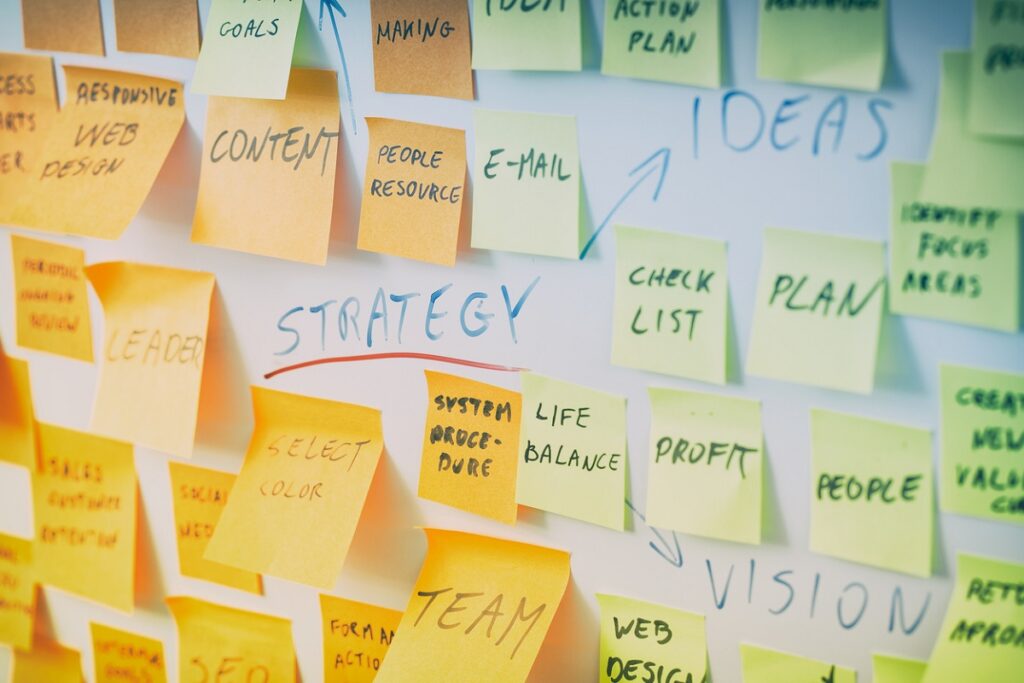
In our 12-part series, Kate Wadia – Managing Director at Phase 3 Consulting – guides the HR professional through how to navigate, succeed and lead with HR tech project-work . From the inception of the business case to the handover into BAU, we’ll follow an indicative project timeframe to explain the way and the why of a project step-by- step, to give you a full toolkit of practical points, a deciphering of definitions and the top tips to get results important to HR and the wider business.
So far in this series we’ve considered how to put together the business case for HR systems implementation and in part 2 I helped you to understand how complex the choice of solution now is and how hard it is to position yourself in the market.
This month’s guide aims to make sure the process of selecting HR technology does not derail you from making the right choice. I’ve seen many implementations de-railed on process or, more accurately, on too much worry about process. Unfortunately for most of us, system selection comes up once in blue moon and so we don’t get an opportunity to practice the process.
Here is a practical guide to the steps you’ll need to take to creating a process and getting the most out of it.
What steps are involved in the process of system selection?
Most clients I work with find it helpful to have a clear map of the typical process between identification of the business case (link to part 1) and arriving at a project start (“project initiation”). If you are an experienced project manager, you might find it handy to share an outline with your less-experienced colleagues:
The role you expect to perform in managing the project will afford you more or less choice about precisely how these steps are organised in terms of style, method and depth. The well-known project methodology Prince 2 offers the principle of tailoring to suit your environment. Formality needs to fit. Beware bureaucracy but do justice to the detail where deserved.
It is unlikely that elsewhere you have written out the activities of selection, except against a calendar or project planning tool:
- Pre-Selection and Requirements Analysis – the business case is taken forward into stakeholder discussions and requirements analysis, which is the fullest part of the pre-selection activity. Examine in detail each area of the system scope, aiming to capture in a clear specification. I recommend ( see below ) (link to ‘Take 1 step on part 3’ below) including a ‘lessons learned’ workshop. Outcomes may be a refined version of the business case with closer scrutiny of costs, benefits and ROI (link to tip on ROI in part 1), as well as a shortlist of product vendors to approach.
TIP: Systems are more commonly over-specified rather than under-specified. Whilst tempting, this can lead to unnecessarily ruling out great solutions or embarking on project plans that flounder in over-ambition. Over-specification encourages salespeople to concentrate on the whizziest features (or future features!). You should think about your aspirations in terms of essentials and desirables, rather like a person specification, to help you structure your thinking.
The Tender Process – tendering is the approach to the market of suppliers. Invitations, replies and countering detail can be formal and I’ll explain in this article how to decide which and what to use.
Larger organisations typically involve a procurement department and with associated guidelines and frameworks to work within to support you through this part of the process.
Product Demonstrations – you will wish to see a showcase demonstration of a handful of different products selected upon examination of responses to your tendering process. Most organisations choose to see between three and five systems. Second rounds are advisable. ( Read on for some tips on how to enjoy the excitement of new stuff without falling into the booby traps!)
Decision-making and Due Diligence – there is a short step in between the decision you’d like to reach and informing the sales personnel, but it’s a key one. Due diligence checks include following up references, often with site visits, asking more questions of the supplier company, exploring company background, financials and client-base. There may be more work to be done to confirm options for technical hosting or licensing (for a broad understanding, look back to part 2 )
TIP: Whilst it’s ideal to get expert and objective help with the full system selection process, if your budget is limited then spend wisely. Pre-selection requirements analysis and contract negotiation are the stretches of this selection road-map where the greatest specialist knowledge add most leverage in the least consultancy time. Consider using the same person to help you out later on with scoping too at project start – this needs to join up.
Contract Negotiations – work with a legal adviser or someone expert in handling the substance of technology contracts to agree on precise terms. Familiarity of your own context should allow you to judge how extensive this process is likely to be as a time-factor but do – right now – take a moment to consider quite how influential a step this is! ( I will look at some of the grey areas in the next article).
Project Initiation – off the back of happy agreements, project teams are put in place and there is a start to the project. Expect this to begin with a “kick-off” meeting.
KEY QUESTION: How long will the selection process take? How much time should I invest? Put this on the agenda for your exercise in ‘lessons learned’, because timescales are much determined by your own organisational habit, structure and the associated complexity of project. In my experience, selection itself takes between two and four months. Subsequent implementation, by comparison, may well span 12-18 months but if all parties share a greater agility, then you could be live with an integrated HR and payroll system within as little as 2-3 months from the point of contract. Don’t hold me to it – much about timescales is within your gift to control! Consultants tend to plan on spending between 10 and 20 days to steer a client organisation through selection work. But note my tip above if funds are hard to find.
Handling the Tender Process
For HR, tendering can be tough due to technical complexity and unfamiliar processes.
What is the role of procurement? If available, then procurement professionals will help you prepare documentation for tenders, keep to purchasing strategies, budgets and legal requirements and (later) to draw up contracts. A procurement framework agreement may make it easier to ensure compliance by suppliers.
Procurement structures can feel restrictive, but they are designed to help. Ask for a brief from that department so that you can work out together from the outset how to benefit from the safety, the groundwork and the expertise without restricting choice.
If you need to manage tendering directly, then the choices are yours about how to tender. Be pleased with this choice! There is no need to be bamboozled by the business-speak of tender terminology….
The key point is to convey your requirements to potential suppliers as accurately as possible and to help those organisations to present accurately by reply. Do not confuse accuracy with length and detail. A traditional tendering process can include extensive documentation – but question the value of lengthy tick-lists. Instead you could aim to draw out the points of differentiation between your specification and others’.
For example, whether a payroll system is complaint with current HMRC rules can be checked on the HMRC website . Instead explain the need for accommodation of a particularly complex pay element or a business process for authorising pay change that is atypical.
Key question: How do you know which type of tender process format and documentation to use?
Phrases used can be confusing. Here is some deciphering:
- Request for Information (RFI) – is an initial ask for information to find out more about your requirements before you make a more formal request.
- Expression of Interest (EOI) – gives potential suppliers notice that you’d like to hear from them. You could also achieve the same as part of an RFI.
- Invitation to Tender (ITT) – in HR technology is perhaps the acronym most commonly used. An ITT is a documentation of requirements that is detailed and specific.
For your central market enquiry, you could otherwise use:
Request for Proposal/Tender/Quotation (RFP/RFT/RFQ) – the formal stage of market enquiry. Nearly interchangeable, choose “quotation” for simple price enquiries, “tender” for specified but complex projects and “proposal” where there are different kinds of solutions to address a business need, rather than a clear solution.
You do not need to work with any of these terms if you do not wish to do so!
Familiarise yourself with these phrases, particularly as others will use them. But have confidence that terminology doesn’t greatly matter. The essence of tender is an ask and a reply, a to-and-fro of information sharing.
Why not send a ‘Supplier Invitation’ and ask for a ‘Reply to Invitation’ and then ‘Project Proposal and Quotation’ ? These are my words and they aim to avoid the ambiguities of the above. It is helpful to potential suppliers to provide a written guidance note about the process you expect of them, giving contact details, next steps, dates and deadlines. If you want to work with a certain format, then say so.
Product Demonstrations: to be seen, to be believed?
You will be asking those shortlisted to visit you (or sometimes attend remotely) to provide a full demonstration of their product. After tedious documentation, introductions and admin this tends to be something of a high-five high-point in system selection processes. It’s a lot of fun to see the aspirations you have for your HR vision come to life and screen in front of you!
But you should be wary of product demonstrations. Not without reason is the concept referred to as a ‘beauty parade’. Bear in mind:
- Sales personnel are more likely to show you what is great about the product and less likely to fill in the gaps
- Those representatives are not the same technicians who will be responsible for your project initiation or post-sales support
- You are seeing a test system which is especially built and carefully set up to look good. I have even heard of a case where demonstrators have used a slide show of what a product should look like, rather than logging in to any system at all. Ask how much was pre-configured to show you what you are seeing
- Be extra wary when future developments on the product roadmap are relied upon
In the representations made to you, understand why things are sold as recommendations or benefits, and whether therefore stuff matters to you.
How can you get the best out of product demonstrations?
- Prepare. Send in advance some specific, differentiating functions or processes that you wish to see modelled as you’ll require them.
- Help the sales people to help you! There is no point in making this too tough a test. Offer information in your supplier invitations about the context for your project. In the session, steer them back on course if they are not showing you what you’d ideally like to see.
- Invite a manageable number of people to attend. Too many and you won’t achieve the full agenda; too few and you are unlikely to have representation broad enough to cover the different concerns (don’t miss out the senior stakeholder, an IT representative and an end-user)
- Manage your own excitement and that of your team. Stick to an agenda, take notes and have in mind a second round
- Enjoy this part of the process. And see the sizzle for the steak!
Key question: Is HR system selection an art or a science? Experience teaches me that project teams in practice reach verdicts based on something beyond a purely analytical assessment against the requirements. It is proven that decision-making is a complex interplay between the rational and the emotional. This means paying attention not only to the ‘art’ of managing the process (plenty tips through this series!) but to the emotional resonance of the project issues themselves that are in scope. Encourage those insistent or resistant to understand earlier rather than later that things will make a difference to them. It is easy with HR technology to care too late in the day.
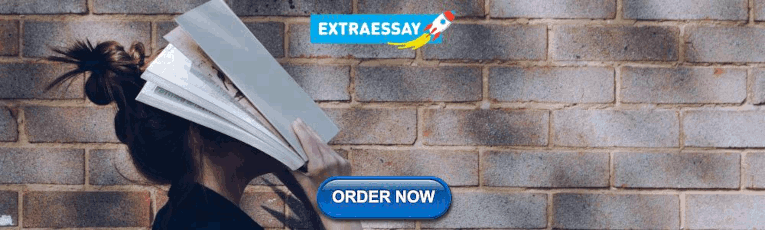
Step 3 in short!
We concluded last time that making the ‘right’ choice is something that is contextual and therefore can’t be done for you; by contrast managing the selection process is a question that can clutter the key thinking you need to do about those choices. Process should be a facilitator of the right choices. Here it is no more than a structure of asking, hearing and deciding.
In setting out your requirements you do not need all the answers, but you do need to know which questions to put. Selection is about mutual information-sharing.
Make sure you are confident on both the art and the science of a simple set of steps that will take you from business case to project initiation. A key point is that you and your organisation are in charge.
Next time I will help you with your homework to get set for a successful ‘kick-off’, forewarned, forearmed and future-proofed in early project stages.
Do ONE thing on Step 3…
Arrange a “lessons learned” workshop. It is rarely done, but easy to do and both rewarding and valuable. Getting internal stakeholders together to review previous HR system projects (and ideally include projects carried out in other parts of the organisation too) is a great way to engage team members from early days, as well as deriving useful insights and evidence from history. You may well be able to start making very practical plans for the weeks ahead – for example in looking at your project management method or the resourcing allocation you can give.
How? Decide who you want to involve and get everyone in a room together, having shared the aim of the session in advance. Make sure the group has a chair person to keep discussion on track. Keep notes on points of consensus and those contested so that there are clear outcomes. You can go grander, but it’s as simple as that to be effective.
- Tags: Communication , Innovation , Technology
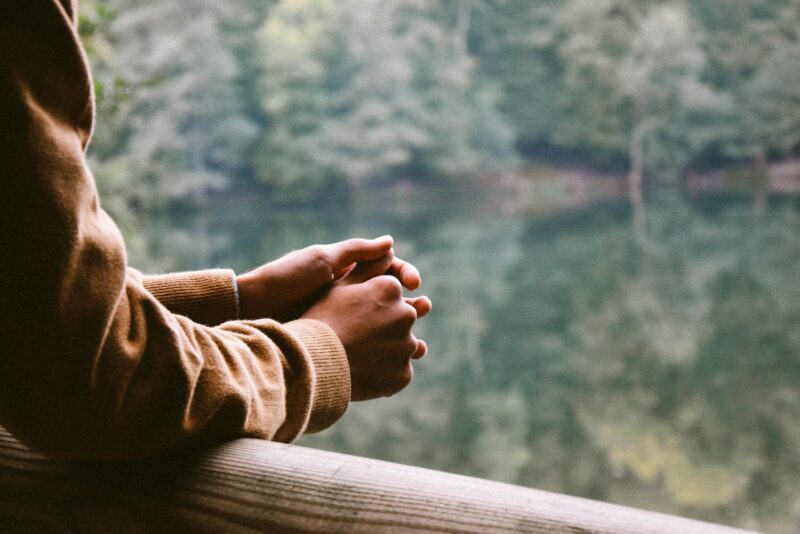
Identifying the risk factors for suicide within our organisations
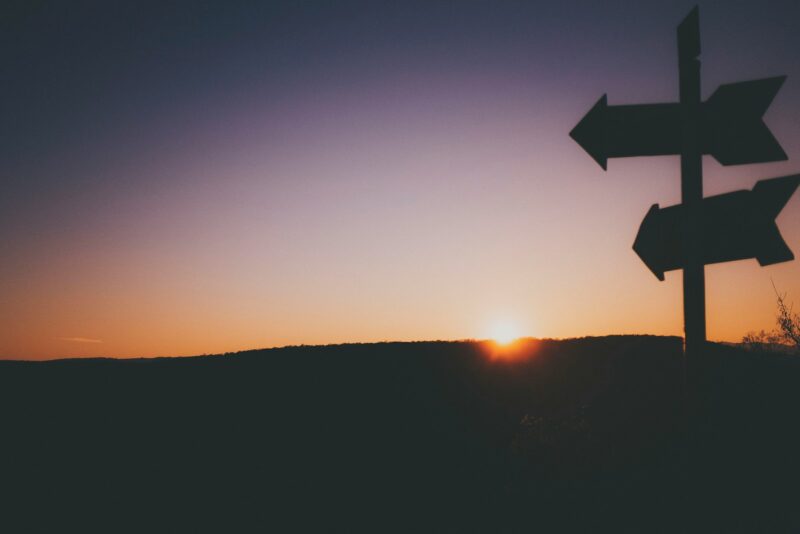
Home, office or hybrid? You’re asking the wrong question
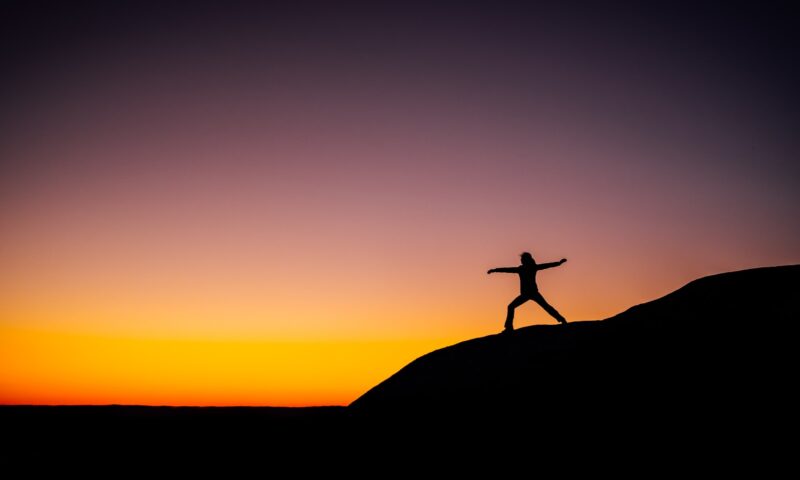
Menstrual leave at work: Is better flexibility enough?
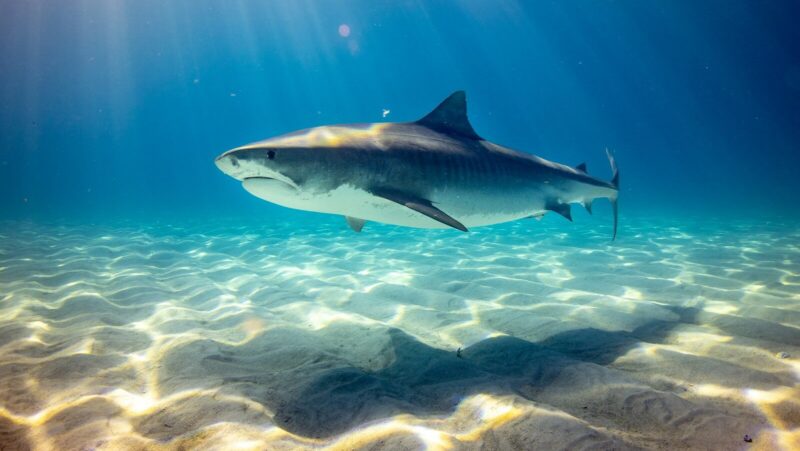
Three signs of stress at work you should not ignore
Former Managing Director
Most read this week
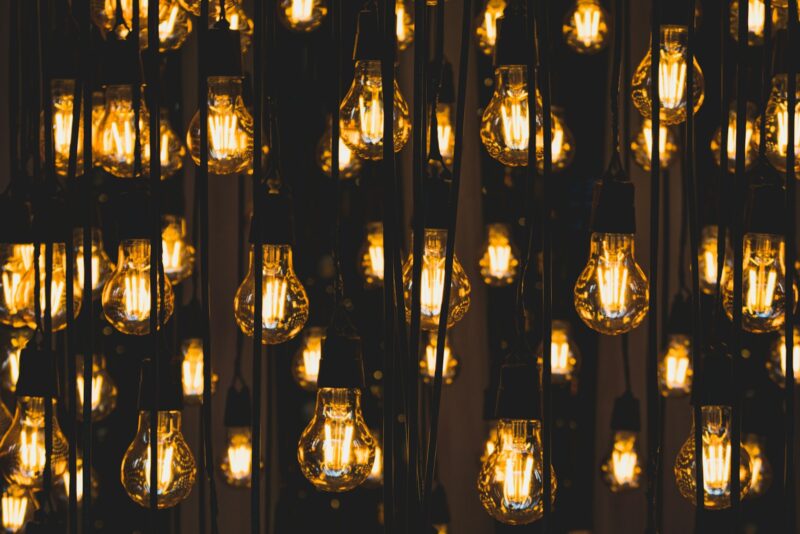
- Culture , Lead
- 19th April 2024
Ten ways to inspire and encourage intrapreneurship
- Thom Dennis
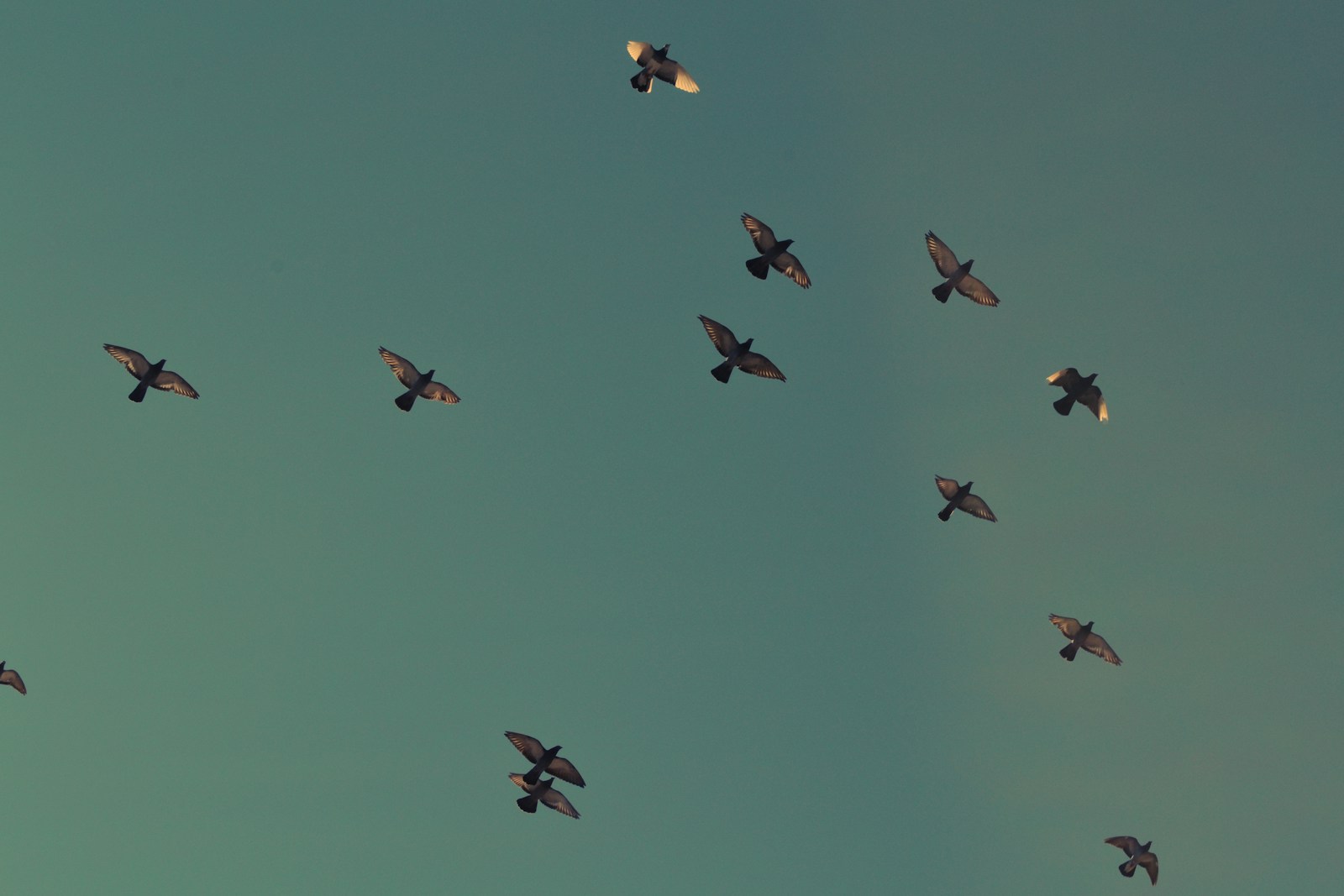
- People , Perform
- 12th March 2024
Achieving connection: The gold dust of high performance
- Andrew Loveless
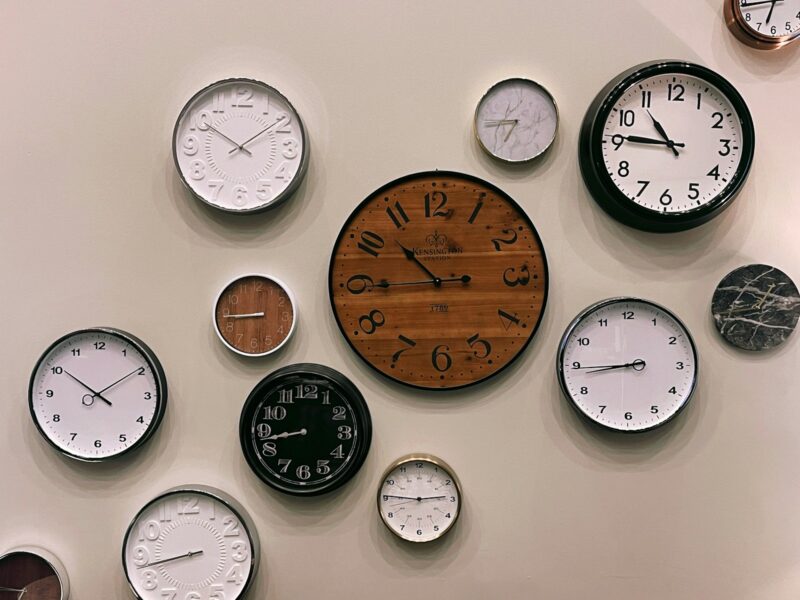
- Employees , Engage
- 15th April 2024
Human resources: Whose return on whose investment?
- Quentin Millington
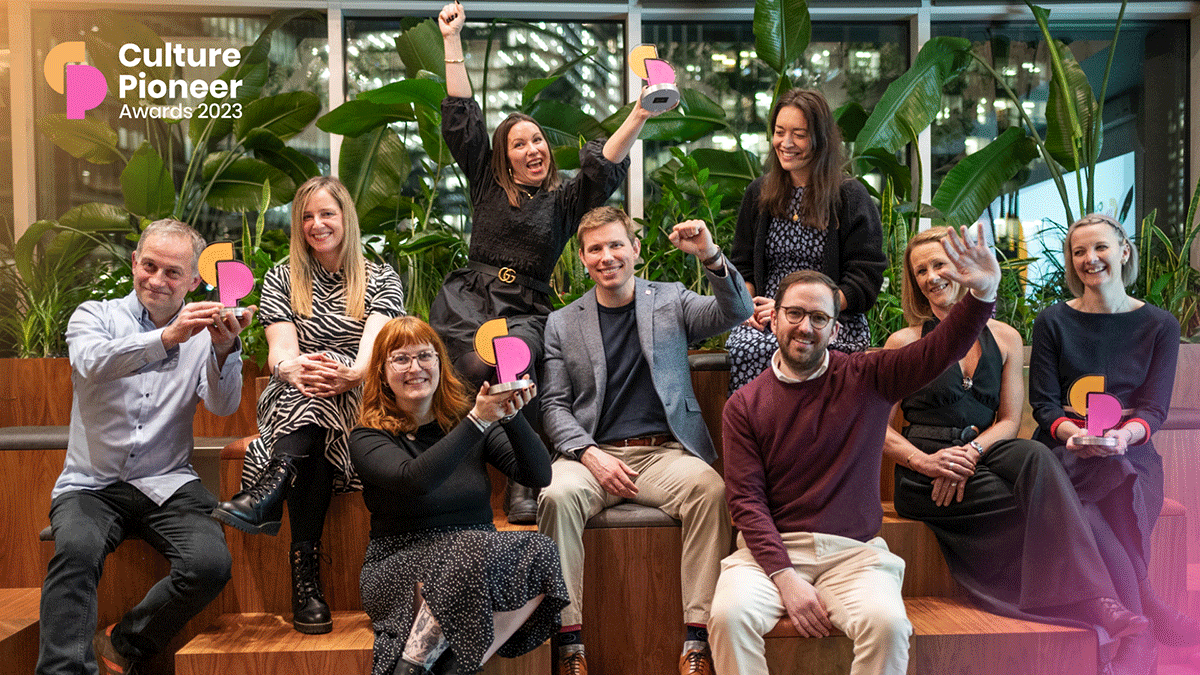
- 22nd March 2024
Winners announced for Culture Pioneer Awards 2023
- Becky Norman
Matt Somers - Coaching Culture Series
How to build a coaching culture
The fundamentals to crafting a coaching culture
Part one: Coaching for success
Building a coaching culture
Part two: What’s the story?
Why stories are a powerful tool for creating a coaching culture
Part three: Coaching as a ritual
Why coaching needs to be embedded as a cultural ritual
- About HRZone
- Privacy policy
- Sub-Processors List
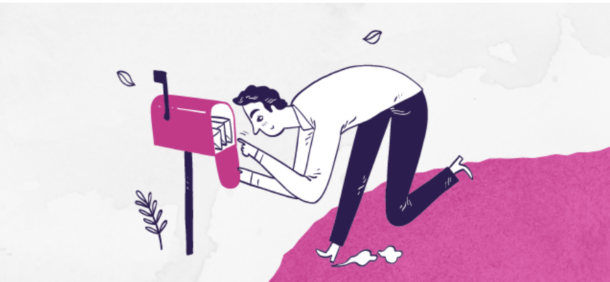
Get the latest from HRZone
Subscribe to expert insights on how to create a better workplace for both your business and its people.
Company size 1-9 10-49 50 to 99 100 - 499 500 - 999 1,000 - 4,999 5,000 - 9,999 10,000 - 49,999 50,000 - 99,999 100,000 +
Role responsibility CIO CEO Chairman HR Director Head of HR HR Manager HR Officer/Assistant Manager But Not Dept Head Board Level Dir But Not HR HR Consultant Supplier to HR Training Dir/Head Training Manager Training Consultant Training Officer/Exec/Assistant Trainer/Coach/Mentor/Tutor Sales and Marketing/Bus Dev Academic/Lecturer Student
DEMYSTIFY THE SYSTEM SELECTION PROCESS
System selection shouldn't be overwhelming.
Recently DelCor engaged with a large professional association to manage their system selection process for contracting a new certification management vendor. Discover DelCor's holistic approach to system selection that resulted in the identification of a new certificate management system by defining the association's selection criteria as well as streamlining the association's certification process.
Download Your Case Study Today!
©2024 DelCor Technology Solutions | DelCor.com | Washington, DC | Chicago
- Mini Projects
- Web Development
- Career Guidance
- Developer Essentials
- Data Structures and Algorithms
- Programming Languages
- Crio Community
- In the News
A Comprehensive Guide to System Design
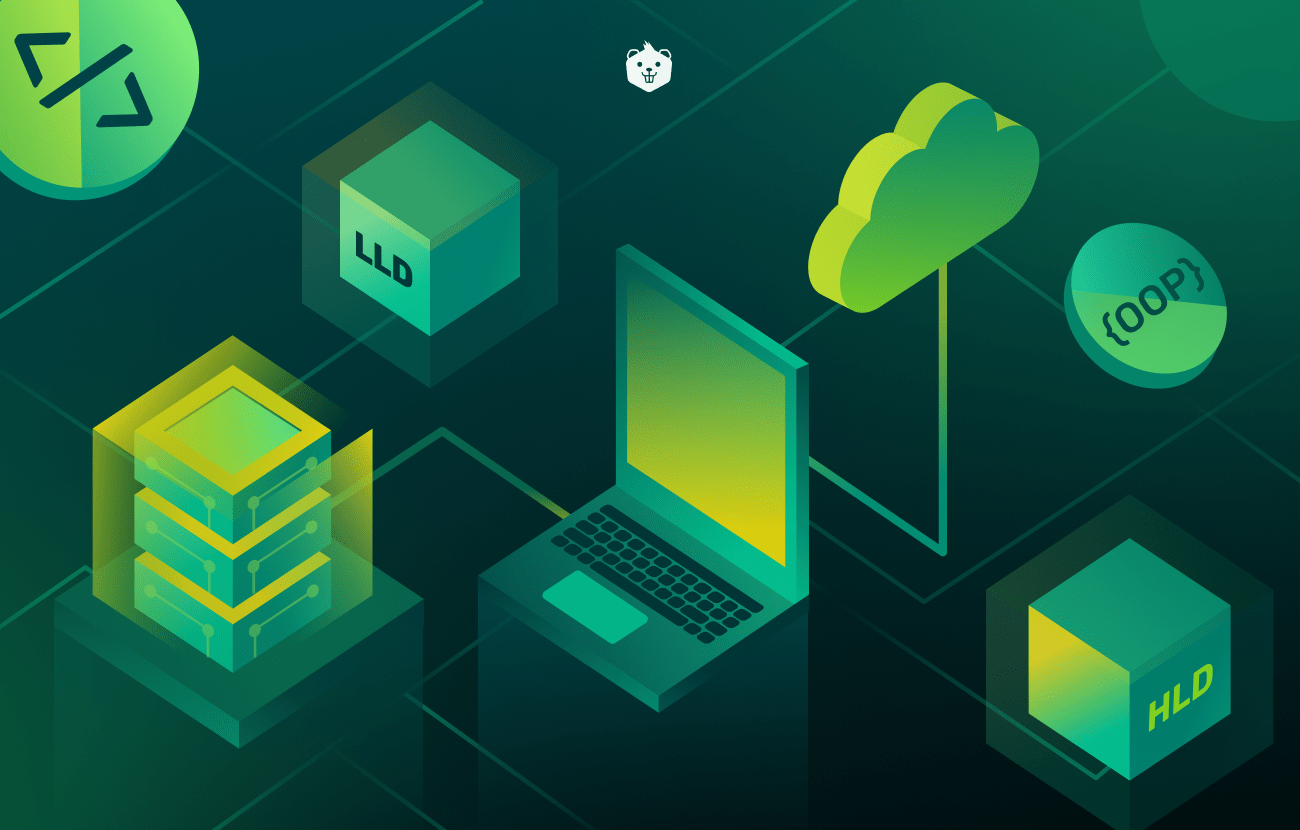
In the dynamic landscape of software engineering, system design stands as a pivotal phase that lays the foundation for robust and scalable applications. Mastering the art of system design involves understanding intricate design concepts that fuel innovation and ensure seamless functionality. In this comprehensive guide, we'll delve into the world of system design, explore its core concepts, and equip you with the knowledge to excel in this crucial aspect of software engineering.
Table of Contents
What is system design, key concepts in system design, understanding the components of system design, the process of system design, best practices for effective system design, case studies of successful system designs.
At its essence, system design is the blueprint that transforms concepts and requirements into tangible software structures. It is the strategic process of defining the architecture, components, and interactions of a software system to fulfill specific functional and non-functional requirements. It involves translating high-level concepts into concrete designs that can be implemented and executed efficiently.
Modularity and Abstraction: Breaking down a complex system into modular components promotes reusability, ease of maintenance, and efficient collaboration among developers.
Coupling and Cohesion: Striking the right balance between component interdependence (coupling) and the functional relatedness within a component (cohesion) is crucial for a well-structured system.
Architectural Patterns: Different architectural patterns, such as client-server, microservices , and monolithic, offer guidelines for organizing components and handling interactions.
Data Flow and Communication: Designing effective data flow and communication mechanisms ensures seamless information exchange between system components.
Trade-offs and Constraints: System designers often face trade-offs between factors like performance, cost, and development time. Understanding these trade-offs is vital to making informed decisions.
A well-crafted software system is a composition of carefully crafted components, each serving a specific purpose and collaborating seamlessly to bring a digital vision to life. Every component is designed and precisely placed to ensure smooth operation and optimal performance. These components encompass a spectrum of functionalities, from managing data and user interactions to securing sensitive information and facilitating communication between different parts of the system.
Let's take a closer look at these fundamental components that form the backbone of a well-structured system design:
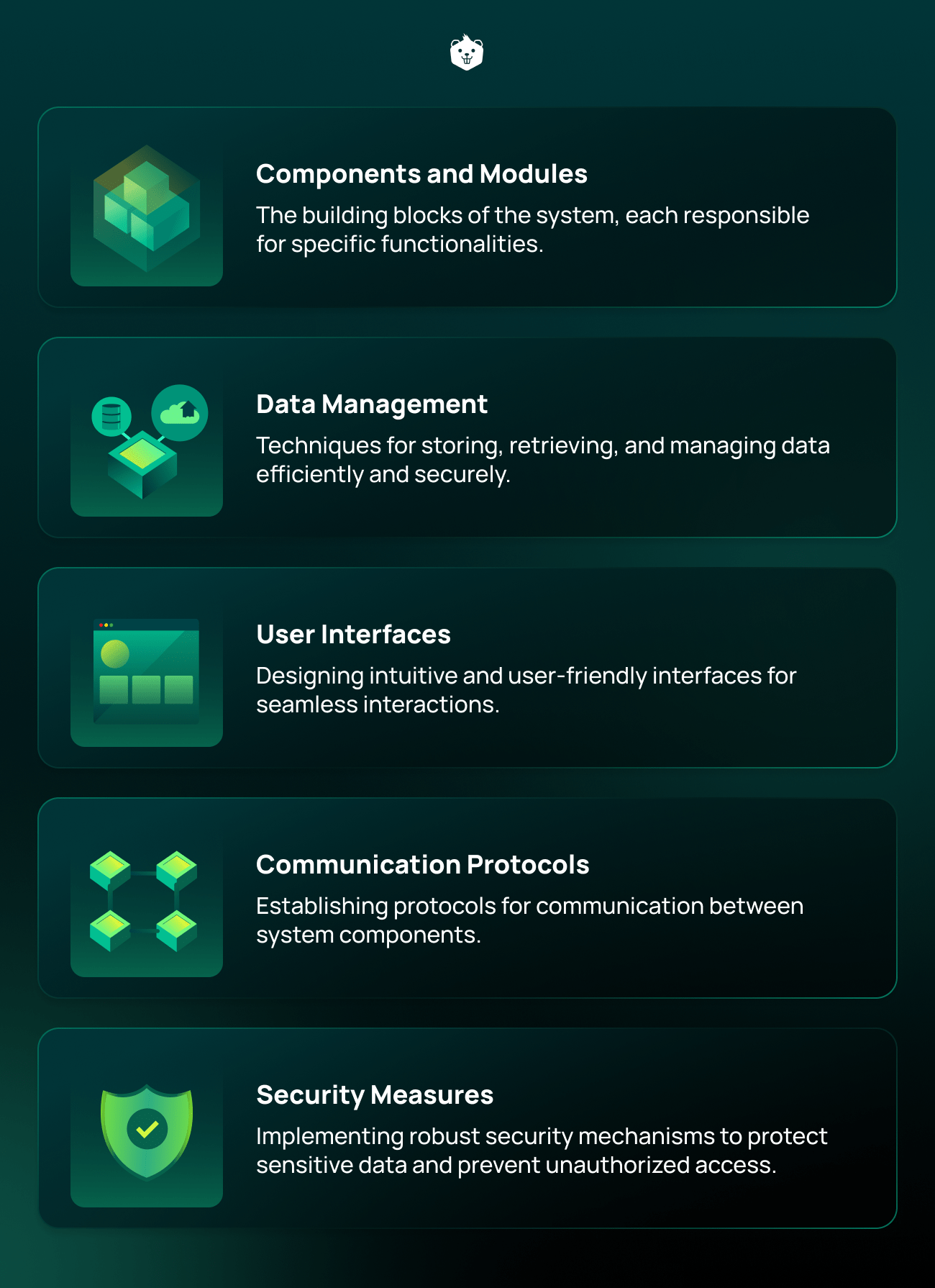
Components and Modules: Each component encapsulates a specific functionality, allowing for modularity and reusability. This means that developers can focus on refining and enhancing individual components without disrupting the entire system.
Data Management: Data management involves designing databases , defining schemas, and implementing strategies for data storage, retrieval, and maintenance. A well-designed data management component ensures that information flows seamlessly through the system, enabling accurate and timely decision-making.
User Interfaces: A user interface component involves creating intuitive, aesthetically pleasing, and user-friendly interfaces that facilitate smooth interactions. From buttons and menus to forms and visual elements, a well-designed user interface component enhances user experience, making it easy for users to navigate and engage with the application.
Communication Protocols: In a world where systems often need to talk to each other, communication protocols act as the language that enables effective dialogue. These protocols define the rules and conventions for data exchange between different components or even between separate systems. Whether it's a web service requesting information from a server or two modules sharing critical data, a robust communication protocol ensures that information is transmitted accurately and efficiently.
Security Measures: With the increasing importance of data privacy and cybersecurity, a dedicated security component is essential. This component encompasses encryption, authentication, access control, and other measures to safeguard sensitive information and prevent unauthorized access. A well-designed security component shields the software system from potential threats and vulnerabilities.
System design is a structured process that involves several stages. It involves a methodical process of conceptualization, refinement, and construction. This process serves as the blueprint for transforming abstract ideas into tangible and functional software systems.
Now, let's delve into the phases that constitute the process of system design:
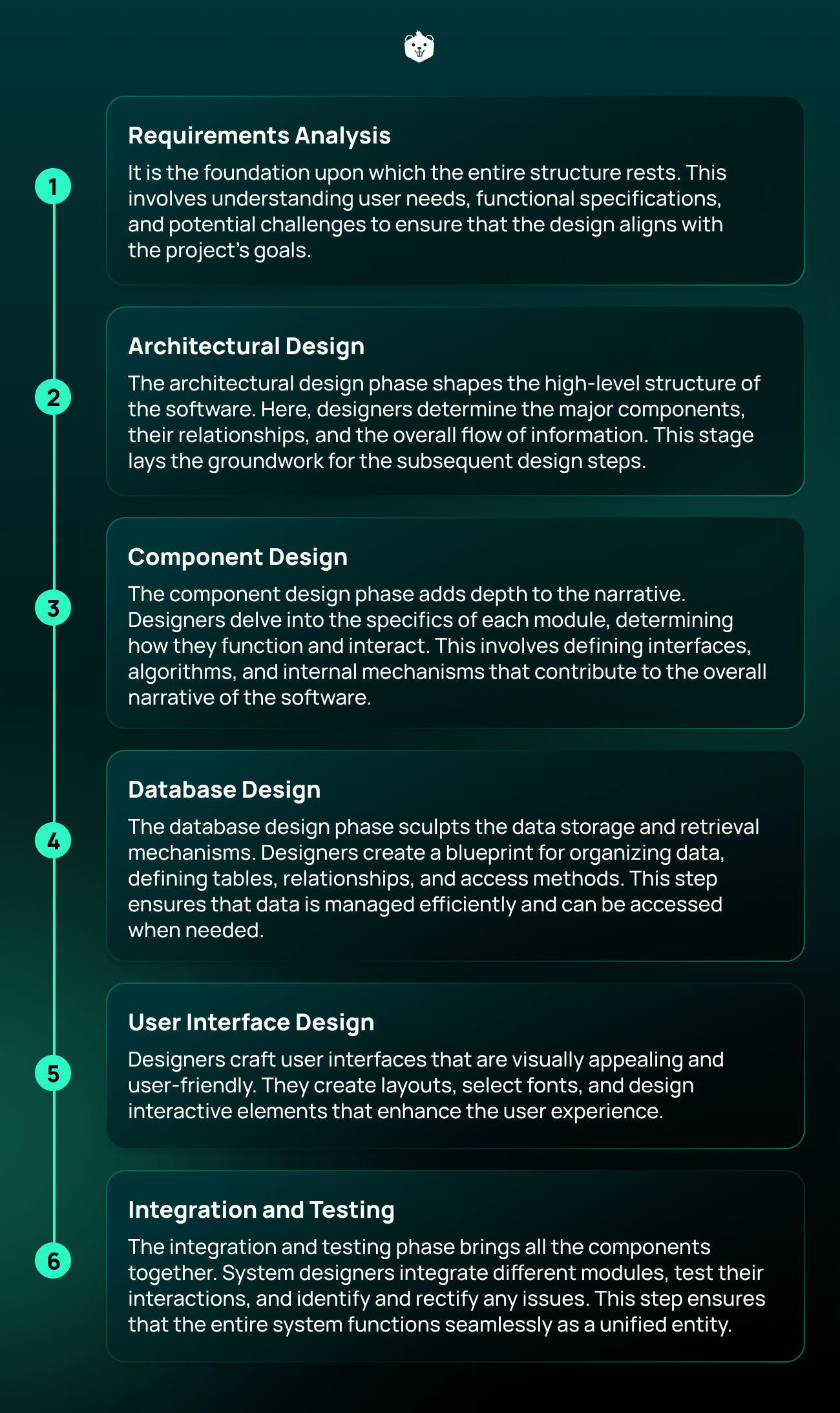
Requirements Analysis : It is the foundation upon which the entire structure rests. This involves understanding user needs, functional specifications, and potential challenges to ensure that the design aligns with the project's goals.
Architectural Design : The architectural design phase shapes the high-level structure of the software. Here, designers determine the major components, their relationships, and the overall flow of information. This stage lays the groundwork for the subsequent design steps.
Component Design : The component design phase adds depth to the narrative. Designers delve into the specifics of each module, determining how they function and interact. This involves defining interfaces, algorithms, and internal mechanisms that contribute to the overall narrative of the software.
Database Design : The database design phase sculpts the data storage and retrieval mechanisms. Designers create a blueprint for organizing data, defining tables, relationships, and access methods. This step ensures that data is managed efficiently and can be accessed when needed.
User Interface Design : Designers craft user interfaces that are visually appealing and user-friendly. They create layouts, select fonts, and design interactive elements that enhance the user experience.
Integration and Testing : The integration and testing phase brings all the components together. System designers integrate different modules, test their interactions, and identify and rectify any issues. This step ensures that the entire system functions seamlessly as a unified entity.
- Thoroughly understand user needs and requirements before diving into design.
- Create modular components to enhance reusability and maintainability.
- Maintain comprehensive documentation to aid understanding and future development.
- Develop prototypes to validate design concepts and gather user feedback.
- Keep It Simple. Strive for simplicity in design to reduce complexity and improve system clarity.
Let's dive into some fascinating real-world examples that showcase how the principles of system design have been applied to create impressive and impactful digital experiences. These case studies provide a glimpse into how thoughtful design choices can lead to revolutionary solutions and redefine entire industries.
Netflix Streaming Service
Netflix, the world's leading streaming platform, is a prime example of successful system design. Behind the scenes, Netflix uses a microservices-based architecture . Think of this as a city with different neighborhoods, each serving a unique purpose. These microservices allow Netflix to deliver content seamlessly to millions of users worldwide. Just like each neighborhood has its own shops and services, each microservice handles a specific task, like user authentication or video streaming. This modular approach ensures scalability, easy maintenance, and quick updates, contributing to the smooth streaming experience that millions enjoy daily.
Uber's Real-time Matching
Uber's success is built on a robust system design that enables real-time matching of drivers and riders. Imagine a magic matchmaking spell that instantly connects people needing a ride with available drivers. Uber's system uses advanced algorithms and location data to make this happen. It's like having a digital GPS guiding drivers to their passengers in real-time. This efficient system design ensures that users get reliable rides quickly, making urban transportation more convenient and accessible.
Amazon Web Services (AWS)
Amazon Web Services (AWS) is a game-changing example of system design in the cloud computing world. Imagine having a supercharged toolbox of digital tools and resources that you can access from anywhere. AWS provides this by offering a wide range of services, like storage, computing power, and databases , through the cloud. It's like having a virtual workshop where developers can build and deploy applications without worrying about the hardware. AWS's scalable and flexible system design has transformed how businesses manage their IT infrastructure, enabling startups and large enterprises alike to innovate and grow rapidly.
System design is the backbone of software engineering, shaping the way applications function, scale, and deliver value to users. By understanding the core concepts, following a systematic design process, and considering key factors, developers can create software systems that meet user expectations and adapt to changing technological landscapes. Learning system design empowers software engineers to craft innovative, reliable, and efficient solutions that drive the digital world forward.

Written by Amani Undru
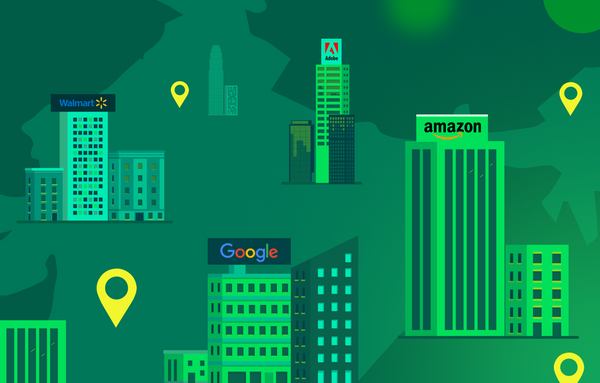
Top Skills to Land Tech Roles at Global MNCs in India
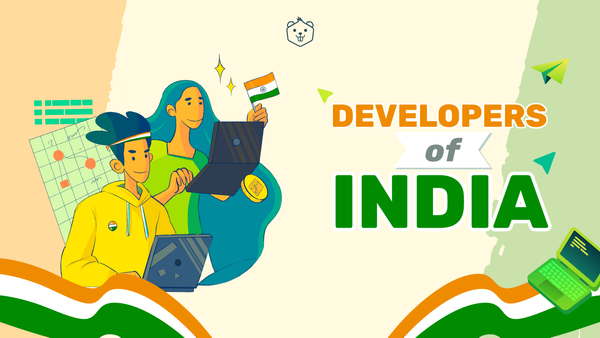
Empowering the Future: Celebrating Developers of India
Academia.edu no longer supports Internet Explorer.
To browse Academia.edu and the wider internet faster and more securely, please take a few seconds to upgrade your browser .
Enter the email address you signed up with and we'll email you a reset link.
- We're Hiring!
- Help Center
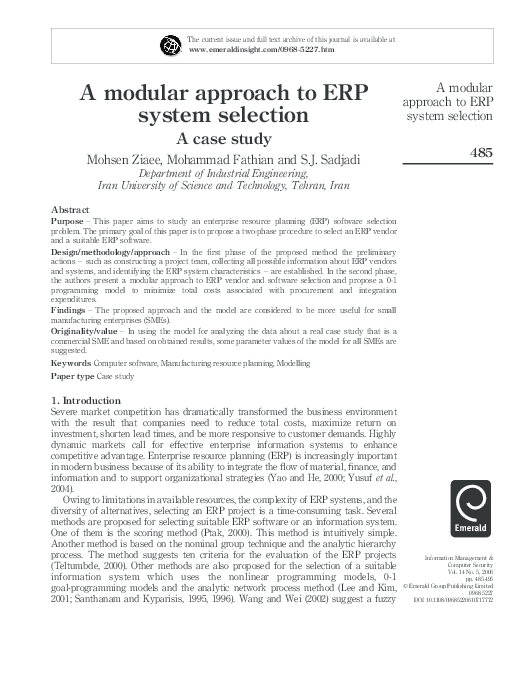
A modular approach to ERP system selection: A case study

2006, Information Management & Computer Security
Related Papers
Bálint Molnár , Benczúr András , Szabo Gyula
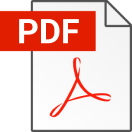
Journal Européen des Systèmes Automatisés
Irene Fahim
Harsh Bajpai
Bálint Molnár , Benczúr András
International Journal of Business Information Systems
Dr. Majed Albraithen
International Journal of Industrial and Systems Engineering
igor rivera
Constantinos Stefanou
Stefan Koch
Moutaz Haddara
Enterprise resource planning (ERP) systems implementation projects are challenging and resource consuming. There are several phases in the ERP implementation process. One of the earliest and most critical phases is the ERP selection phase. If an organization selects an inadequate ERP to fit their needs, the project will most likely destine to fail. Research and practice have provided several cases of ERP project failures because of a faulty selection process. No matter what amendments the adopting company undertakes in the later phases, if there is no fit, there is no success. This research, presents an explanatory case study, which employed a simple multi-attribute rating technique (SMART)-based ERP selection method. The selection method was mainly based on developing process maps for all the critical business processes inside the organization, and then checking the degree of compliance of the potential ERP packages with those developed process maps. In addition, other factors were also included in the ERP evaluation and selection process. As the ERP was assured to be matching the business requirements, the method and the selection factors have been proven adequate for the selection process. In addition, this minimizes the risk of a non-fitting ERP system and consequent project failures.
Philseok Kim
RELATED PAPERS
Acta Anaesthesiologica Taiwanica
Mei-Yung Tsou
Bekir Elmas
Acta Crystallographica Section A Foundations of Crystallography
Richard Matyi
Journal of Advanced Academic Research
kanchan gautam
Fatemeh Ravari
Expert Systems with Applications
Electric Power Systems Research
Patrick Panciatici
Russian Journal of Forensic Medicine
Vasiliy Vlassov
Livestock research for rural development
Anderson Medina
Sustainability
Elsayed Tag eldin
Tibor Denes
Journal of Molecular Structure
Colin Reeves
marco zavatarelli
Jurnal Kelautan dan Perikanan Terapan (JKPT)
Muhammad Agus
rosete pescador
Journal of Design History
Barbara Brownie
LWT - Food Science and Technology
Radovan Borojevic
Journal of Pharmacovigilance and Drug Research
Kenneth Gossett
Technovation
amit poonia
Perspectives of New Music
Robert P Sholl
Journal of Clinical Medicine
Stella Mazurova
Samuel Parra León
UPenn毕业证书 宾夕法尼亚大学学位证
RELATED TOPICS
- We're Hiring!
- Help Center
- Find new research papers in:
- Health Sciences
- Earth Sciences
- Cognitive Science
- Mathematics
- Computer Science
- Academia ©2024
Regional-scale landslide modeling using machine learning and GIS: a case study for Idukki district, Kerala, India
- Original Paper
- Published: 23 April 2024
Cite this article
- Dhanya Madhu ORCID: orcid.org/0000-0002-6958-2825 1 , 2 ,
- G. K. Nithya 1 ,
- S. Sreekala 1 &
- Maneesha Vinodini Ramesh 1
Globally, landslides impact in a site-specific and regional scale, and have affected 4.8 million human beings during1998–2017. Landslides, being a highly complex phenomenon involving real-time and near real-time interactions between hydrological, geomorphological, climatological as well as anthropological factors impacting large spatial areas, demand the development of regional-scale warning systems. Even though an extensive body of research already exists in the field of landslide early warning, the prediction of the actual location and the time of landslide initiation is still a major challenge. In the current study, we compare the performance of ten machine learning (ML) algorithms useful for landslide early warning. The current study is performed in the Idukki district of Kerala state in India. A database with landslide incidents is created using research literature, reports from the Geological Survey of India (GSI) as well as news articles. Landslide causative factors, as indicated by previous literature, have been mapped using Geographic Information System (GIS). The different ML algorithms considered for this study are Decision Tree, Logistic Regression, K Nearest Neighbor, Gaussian Naive Bayes, Support Vector Machine, and its different kernel functions such as linear, Polynomial, Gaussian and ensemble algorithms namely Random Forest and AdaBoost. The performance of the different algorithms is quantified and compared utilizing established statistical metrics such as G-Mean, F1 score, and ROC-AUC score. Our results follow the similar ones in literature where the machine learning techniques provide an efficient tool for landslide susceptibility mapping. All the algorithms considered produce reasonable results.
This is a preview of subscription content, log in via an institution to check access.
Access this article
Price includes VAT (Russian Federation)
Instant access to the full article PDF.
Rent this article via DeepDyve
Institutional subscriptions
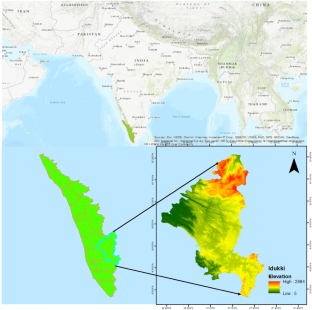
Abraham MT, Pothuraju D, Satyam N (2019) Rainfall thresholds for prediction of landslides in Idukki, India: an empirical approach. Water 11(10):2113
Article Google Scholar
Abraham MT, Satyam N, Rosi A, Pradhan B, Segoni S (2020) The selection of rain gauges and rainfall parameters in estimating intensity-duration thresholds for landslide occurrence: case study from Wayanad (India). Water 12(4):1000
Chan HC, Chen PA, Lee JT (2018) Rainfall-induced landslide susceptibility using a rainfall–runoff model and logistic regression. Water 10(10):1354
Ferri C, Hernández-Orallo J, Modroiu R (2009) An experimental comparison of performance measures for classification. Pattern Recogn Lett 30(1):27–38
Goetz JN, Brenning A, Petschko H, Leopold P (2015) Evaluating machine learning and statistical prediction techniques for landslide susceptibility modeling. Comput Geosci 81:1–11
He S, Wang J, Liu S (2020) Rainfall event–duration thresholds for landslide occurrences in China. Water 12(2):494
Hemalatha T, Ramesh MV, Rangan VP (2019) Effective and accelerated forewarning of landslides using wireless sensor networks and machine learning. IEEE Sens J 19(21):9964–9975
Hong H, Biswajeet P, Bui DT, Xu C (2015) Landslide susceptibility assessment at the Suichuan area (China) using support vector machine model. In: emerging economies, risk and development, and intelligent technology. In: Proceedings of the 5th international conference on risk analysis and crisis response. Tangier Morocco pp. 1–3
Hong H, Pradhan B, Jebur MN, Bui DT, Xu C, Akgun A (2016) Spatial prediction of landslide hazard at the Luxi area (China) using support vector machines. Environmental Earth Sciences 75:1–14
Article CAS Google Scholar
Hossin M, Sulaiman MN (2015) A review on evaluation metrics for data classification evaluations. Int J Data Min Knowl Manag Process 5(2):1
Hu Q, Zhou Y, Wang S, Wang F, Wang H (2019) Improving the accuracy of landslide detection in “off-site” area by machine learning model portability comparison: a case study of Jiuzhaigou earthquake. China Remote Sensing 11(21):2530
Jeni LA, Cohn JF, De La Torre F (2013) Facing imbalanced data–recommendations for the use of performance metrics. In: 2013 Humaine association conference on affective computing and intelligent interaction. pp. 245–251 IEEE
Kothari UC, Momayez M (2018) Machine learning: a novel approach to predicting slope instabilities. Int J Geophy 2018:1–9
Kuradusenge M, Kumaran S, Zennaro M (2020) Rainfall-induced landslide prediction using machine learning models: the case of Ngororero district, Rwanda. Int J Environ Res Public Health 17(11):4147
Leonarduzzi E, Molnar P, McArdell BW (2017) Predictive performance of rainfall thresholds for shallow landslides in Switzerland from gridded daily data. Water Resour Res 53(8):6612–6625
Liu Z, Gilbert G, Cepeda JM, Lysdahl AOK, Piciullo L, Hefre H, Lacasse S (2021) Modelling of shallow landslides with machine learning algorithms. Geosci Front 12(1):385–393
Liu S, Wang L, Zhang W, He Y, Pijush S (2023) A comprehensive review of machine learning-based methods in landslide susceptibility mapping. Geol J 58(6):2283–2301. https://doi.org/10.1002/gj.4666
Lukose Kuriakose S, Sankar G, Muraleedharan C (2010) Landslide fatalities in the Western Ghats of Kerala, India. In: EGU general assembly conference abstracts. p. 8645
Ma Z, Mei G, Piccialli F (2021) Machine learning for landslides prevention: a survey. Neural Comput Appl 33:10881–10907
Maalouf M (2011) Logistic regression in data analysis: an overview. Int J Data Anal Tech Strateg 3(3):281–299
Merghadi A, Yunus AP, Dou J, Whiteley J, ThaiPham B, Bui DT, Abderrahmane B (2020) Machine learning methods for landslide susceptibility studies: a comparative overview of algorithm performance. Earth-Sci Rev 207:103225
Mersha T, Meten M (2020) GIS-based landslide susceptibility mapping and assessment using bivariate statistical methods in Simada area, northwestern Ethiopia. Geoenviron Disaster 7(1):1–22
Nam K, Wang F (2020) An extreme rainfall-induced landslide susceptibility assessment using autoencoder combined with random forest in Shimane prefecture. Japan Geoenviron Disaster 7(1):1–16
Google Scholar
Nsengiyumva JB, Valentino R (2020) Predicting landslide susceptibility and risks using GIS-based machine learning simulations, case of upper Nyabarongo catchment. Geomat Nat Haz Risk 11(1):1250–1277
Omadlao ZRD, Tuguinay NMA, Saturay Jr RM (2019) Machine learning-based prediction system for rainfall-induced landslides in Benguet first Engineering district
Pai DS, Rajeevan M, Sreejith OP, Mukhopadhyay B, Satbha NS (2014) Development of a new high spatial resolution (0.25× 0.25) long period (1901–2010) daily gridded rainfall data set over India and its comparison with existing data sets over the region. Mausam 65(1):1–18
Ramesh MV (2014) Design, development, and deployment of a wireless sensor network for detection of landslides. Ad Hoc Netw 13:2–18
Sajinkumar KS, Anbazhagan S, Pradeepkumar AP, Rani VR (2011) Weathering and landslide occurrences in parts of Western Ghats, Kerala. J Geol Soc India 78:249–257
Sajinkumar KS, Rinu S, Oommen T, Vishnu CL, Praveen KR, Rani VR, Muraleedharan C (2020) Improved rainfall threshold for landslides in data sparse and diverse geomorphic milieu: a cluster analysis based approach. Nat Hazards 103:639–657
Sasidhar TT, Sreelakshmi K, Vyshnav MT, Sowmya V, Soman KP (2019) Land cover satellite image classification using ndvi and simplecnn. In: 2019 10th International conference on computing, communication and networking technologies (ICCCNT) pp. 1–5 IEEE
Tavakkoli Piralilou S, Shahabi H, Jarihani B, Ghorbanzadeh O, Blaschke T, Gholamnia K, Aryal J (2019) Landslide detection using multi-scale image segmentation and different machine learning models in the higher Himalayas. Remote Sens 11(21):2575
Tien Bui D, Pradhan B, Lofman O, Revhaug I (2012) Landslide susceptibility assessment in Vietnam using support vector machines, decision tree, and Naive Bayes Models. Mathematical problems in Engineering 2012
Tehrani FS, Santinelli G, Herrera M (2019) A framework for predicting rainfall induced landslides using machine learning methods. In: 17th European conference on soil mechanics and geotechnical engineering. ECSMGE 2019-Proceedings
Tehrani FS, Calvello M, Liu Z, Zhang L, Lacasse S (2022) Machine learning and landslide studies: recent advances and applications. Nat Hazards 114(2):1197–1245
Tien Bui D, Pradhan B, Lofman O, Revhaug I, Dick ØB (2013) Regional prediction of landslide hazard using probability analysis of intense rainfall in the Hoa Binh province. Vietnam Nat Hazard 66:707–730
Tien Bui D, Ho TC, Pradhan B, Pham BT, Nhu VH, Revhaug I (2016) GIS-based modeling of rainfall-induced landslides using data mining-based functional trees classifier with AdaBoost, Bagging, and MultiBoost ensemble frameworks. Environ Earth Sci 75:1–22
Tien Bui D, Tuan TA, Hoang ND, Thanh NQ, Nguyen DB, Van Liem N, Pradhan B (2017) Spatial prediction of rainfall-induced landslides for the Lao Cai area (Vietnam) using a hybrid intelligent approach of least squares support vector machines inference model and artificial bee colony optimization. Landslides 14:447–458
Vakhshoori V, Pourghasemi HR, Zare M, Blaschke T (2019) Landslide susceptibility mapping using GIS-based data mining algorithms. Water 11(11):2292
Vijayakumar A, Mahesh AS (2019) Quality assessment of ground water in pre and post-monsoon using various classification technique. Int J Recent Technol Eng (IJRTE) 8(2):5996–6003
Wang H, Zhang L, Yin K, Luo H, Li J (2021) Landslide identification using machine learning. Geosci Front 12(1):351–364
Xiao T, Yin K, Yao T, Liu S (2019) Spatial prediction of landslide susceptibility using GIS-based statistical and machine learning models in Wanzhou country, three gorges reservoir, China. Acta Geochimica 38:654–669
Download references
Acknowledgements
The authors express their gratitude for the immeasurable motivation and guidance provided by Sri. (Dr) Mata Amritanandamayi Devi, the Chancellor of Amrita Vishwa Vidyapeetham. The authors acknowledge the Indian Meteorological Department for the daily rainfall data. We gratefully acknowledge the landslide research team members at Amrita Vishwa Vidyapeetham for the landslide photos from field. The authors are thankful for the effort of those who have directly or indirectly helped in doing this work.
No grants, or funds were received during the preparation of this manuscript.
Author information
Authors and affiliations.
Center for Wireless Networks & Applications (WNA), Amrita Vishwa Vidyapeetham, Amritapuri, India
Dhanya Madhu, G. K. Nithya, S. Sreekala & Maneesha Vinodini Ramesh
Department of Physics, Amrita Vishwa Vidyapeetham, Amritapuri, India
Dhanya Madhu
You can also search for this author in PubMed Google Scholar
Corresponding author
Correspondence to Dhanya Madhu .
Ethics declarations
Conflicts of interest.
The authors declare they have no competing interests.
Additional information
Publisher's note.
Springer Nature remains neutral with regard to jurisdictional claims in published maps and institutional affiliations.
Rights and permissions
Springer Nature or its licensor (e.g. a society or other partner) holds exclusive rights to this article under a publishing agreement with the author(s) or other rightsholder(s); author self-archiving of the accepted manuscript version of this article is solely governed by the terms of such publishing agreement and applicable law.
Reprints and permissions
About this article
Madhu, D., Nithya, G.K., Sreekala, S. et al. Regional-scale landslide modeling using machine learning and GIS: a case study for Idukki district, Kerala, India. Nat Hazards (2024). https://doi.org/10.1007/s11069-024-06592-3
Download citation
Received : 18 December 2022
Accepted : 29 March 2024
Published : 23 April 2024
DOI : https://doi.org/10.1007/s11069-024-06592-3
Share this article
Anyone you share the following link with will be able to read this content:
Sorry, a shareable link is not currently available for this article.
Provided by the Springer Nature SharedIt content-sharing initiative
- Machine learning
- Geoscience data
- Find a journal
- Publish with us
- Track your research
- Skip to main content
- Keyboard shortcuts for audio player

- LISTEN & FOLLOW
- Apple Podcasts
- Google Podcasts
- Amazon Music
Your support helps make our show possible and unlocks access to our sponsor-free feed.
What Donald Trump's Indictments Mean For The Election And Our Legal System

Former U.S. President Donald Trump arrives ahead of the start of jury selection at Manhattan Criminal Court in New York City. Pool/Getty Images hide caption
Former U.S. President Donald Trump arrives ahead of the start of jury selection at Manhattan Criminal Court in New York City.
We've never been here before. A former president is being tried in criminal court while he's running for reelection.
Donald Trump faces four separate indictments. And only one of them will go to trial before November. That's a case that got underway yesterday in a Manhattan courtroom with jury selection. Trump is charged with falsifying business documents ahead of the 2016 election to cover up payments he made to adult film star Stormy Daniels.
With a former president facing an unprecedented four indictments, what sets this case in New York apart? And what could the treatment of a former president reveal about our legal system more broadly
Find more of our programs online . Listen to 1A sponsor-free by signing up for 1A+ at plus.npr.org/the1a .
Donald Trump trial updates: Man sets himself on fire at Manhattan courthouse
Editor's note: This file recaps the news from Donald Trump's criminal trial on Friday, April 19. For the latest news from Trump's hush money trial , please read our live updates for Monday, April 22 .
NEW YORK — A man threw conspiracy pamphlets into the air and set himself on fire Friday outside the courthouse where former President Donald Trump's hush money trial is taking place, critically injuring himself and horrifying onlookers.
Police identified him as Max Azzarello, 37, of Florida. The victim was seen dousing himself with a liquid around 1:35 p.m. in a park across the street from the Manhattan courthouse, witnesses told USA TODAY.
The shocking incident took place in full view of news cameras shortly after a full panel of 12 jurors and six alternates was seated for his hush money trial . Witnesses said the victim tossed the flyers into the air before dousing himself and setting himself ablaze with what appeared to be a lighter. Six first responders suffered minor injuries trying to put out the fire and provide medical attention, the New York City Fire Department said.
Keep up with USA TODAY's live updates from inside and outside the Manhattan courthouse:
Prep for the polls: See who is running for president and compare where they stand on key issues in our Voter Guide
Manifesto shows Max Azzarello was mired in conspiracy theories before setting himself on fire outside Trump trial
In a post on a Substack newsletter called "The Ponzi Papers," Max Azzarello said he had burned himself alive to draw attention to the U.S. political and economic system.
"My name is Max Azzarello, and I am an investigative researcher who has set himself on fire outside of the Trump trial in Manhattan," the post says.
Azzarello's manifesto took aim at Trump, President Joe Biden, cryptocurrency, and the federal government.
"To my friends and family, witnesses and first responders, I deeply apologize for inflicting this pain upon you," he wrote.
Witness saw it coming: 'I'm going to see something bad here'
A bystander who asked to be identified by only his first name, Dave, told USA TODAY he watched the victim's actions with a growing sense of panic before the man went up in flames.
"I heard this clap, and this fellow was throwing these papers into the air," the 73-year-old Manhattan resident said. "He had a can and he poured fluid all over himself, at which point I thought, 'Oof, I'm going to see something bad here.'"
"And sure enough, he pulled out a lighter. And he set himself on fire."
One person blasted the burning man with a fire extinguisher as a New York emergency medical technician joined the fray.
By the time the flames were doused, "his face was completely black," another witness, Julie Berman, told reporters.
−Aysha Bagchi
'Don't interrupt me,' judge scolds Trump lawyer. 'Have a seat'
With court back in session, Judge Merchan arrived and the prosecution raised an issue about restricting access to information tied to Michael Cohen, Trump's former lawyer and a potentially key witness in the case. Merchan engaged in a back-and-forth with Trump lawyer Emil Bove when the judge scolded the lawyer.
"Don't interrupt me," Merchan said. "I'm not interrupting you," Bove shot back. "You are. Have a seat," Merchan instructed.
New York Supreme Court Justice Marsha Michael denied a request Friday from Trump’s attorneys to halt the criminal trial. Another motion to move the case out of Manhattan is still pending.
Opening statements in Trump’s trial are slated to begin Monday morning.
– Aysha Bagchi
Trump: Judicial system in New York 'an outrage'
If Donald Trump testifies at his New York hush money trial, prosecutors want to introduce evidence about other his other legal disputes. Trump harshly criticized judges in other cases as he left the criminal trial.
New York Judge Arthur Engoron ordered Trump to pay $454 million in a civil fraud trial for exaggerating the value of his real estate. Trump is appealing.
“Engoron is a whack job,” Trump said. “What he did was a disgrace.”
U.S. District Judge Lewis Kaplan presided over a trial where columnist E. Jean Carroll won $83 million in a defamation lawsuit for denying that he assaulted her in a department story changing room in the 1990s. Trump appealed that verdict, too.
“I had no idea who this person was,” Trump said of Carroll.
“What’s happening with the judicial system is an outrage,” Trump added.
Judge Juan Merchan, who is presiding over the criminal trial, said he would rule Monday on what additional evidence prosecutors can introduce if Trump testifies.
– Bart Jansen
Trump's historic trial now has a full slate of 12 jurors and 6 alternates
The chosen alternate jurors have been were sworn in after two more were chosen.
They swore to try the case "in a fair and impartial manner." Judge Merchan told the jurors he expects to begin the trial proceedings on Monday. He advised them proceedings will end at 2 p.m. ET on Monday and Tuesday and will run through lunch on those days.
Trump legal team opposed information from E. Jean Carroll trials
The Trump "Sandoval hearing" this afternoon is going into whether the prosecution can introduce multiple negative outcomes for Trump from past legal cases to undermine his credibility if he chooses to testify in his New York criminal case.
Those negative outcomes include Trump's losses in two civil cases against New York writer E. Jean Carroll, where Trump was found liable for sexually abusing and defaming her. The former president was ordered to pay her $88.3 million in damages.
Trump lawyer Emil Bove argued that bringing in information from the Carroll trials is inappropriate because it "pushes the salaciousness" onto another level.
Merchan asked why not just keep out the allegations and just allow the findings in the cases to be introduced in the trial. Bove said that would still allow for inappropriate evidence.
'Egomaniacal' and 'the devil': Another potential alternate struck by judg e
Another potential alternate juror was struck for impartiality concerns after the defense brought social media posts to the judge's attention that they believed were from an account belonging to the man.
On questioning, the potential juror didn't recall making certain posts he was shown (which were not shown to reporters). Some posts included descriptions of Trump as "egomaniacal" and "the devil."
Asked by Judge Merchan if the man believed Trump is the devil, the man replied: "At that time, yeah, I may have felt that."
Judge advises Trump defense to stop flooding court with filings
Judge Juan Merchan is addressing additional issues with prosecutors and Trump's defense team after wrapping up the Sandoval hearing." Merchan advised Trump's defense that they need to accept his rulings at a certain point. He complained that the defense was targeting individual court decisions "one by one by one" and submitting filings challenging them.
Social media comes back to haunt yet another potential juror
Defense attorney Susan Necheles asked Judge Merchan to strike another potential alternate based social media posts she showed to the judge (the posts weren't shown to reporters).
After bringing the man in for questioning, Merchan said he found the prospective juror credible − adding that he didn't know that the sentiments in the posts were anti-Trump. But Merchan said he was concerned that one of the photographs that was featured included text describing "a massive anti-Trump rally."
Too many people have worked too hard to get the case this far, Merchan said. "I'm going to grant the challenge for cause."
More: How Donald Trump's hush money trial team is using social media to weed out New York jurors
Third and fourth alternate jurors chosen
Two more alternate jurors have been chosen, making four alternates at this point. One is a man who is an audio professional. He earlier said he believed Trump is being treated fairly. The other is a woman who is active in a school parents' association.
Dismissed juror number 4 speaks out: 'I feel sorry for the other jurors'
USA TODAY Network had an exclusive interview Thursday with Herson Cabreras , who was chosen for the jury on Tuesday but kicked off two days later, after the prosecution raised questions about whether he accurately described his background.
The kerfuffle over his jury service arose after prosecutors raised the issue of a 1991 incident in which Cabreras and an associate were accused of tearing down political campaign signs in Harrison, New York.
"I feel sorry for the other jurors," he said, expressing concern about other jurors potentially being dragged into the center of a fiercely contested legal battle.
- Asher Stockler
Judge strikes Women's March attendee from alternate juror contention
Judge Merchan struck a potential alternate juror based on impartiality concerns.
"I don't believe she's being disingenuous. I do believe that she's credible," Merchan said. However, leaning on the side of caution and "looking at her answers as a whole," he said he thought the safer course is to grant the defense's challenge for cause.
Trump team trying to get woman who attended Women's March struck by judge
Trump lawyer Susan Necheles is arguing that a woman who attended the Women's March protest after Trump's presidential election should be struck by the judge based on impartiality concerns. Necheles said the potential juror made statements such as that Trump enabled racist and homophobic comments.
The potential alternate said earlier she didn't know what Trump's rhetoric is, but she is familiar with people who made homophobic and racist comments and cited Trump.
The potential alternate has been brought in for additional questioning. She echoed her previous comments, but also told the judge she can be fair and impartial.
Second alternate juror chosen
A second alternate juror, a woman who said she doesn't watch news, was chosen after neither side used a challenge to strike her. She will be the second alternate juror. The first alternate was chosen on Thursday.
Trump lawyer's questioning of potential jurors ends. We could have more alternates soon
Trump lawyer Susan Necheles ' questioning of potential alternate jurors has ended. The judge has given the lawyers some time to confer and review their notes before they will go through any challenges they have to the alternate juror candidates.
Potential juror says his opinion on Trump is neutral, leaning hostile
Pressed by Trump lawyer Susan Necheles about his opinion on Trump, a potential juror said he likes Trump's tax policy, but doesn't like where the Republican Party is when it comes to women's rights over their bodies. The man said his opinion on Trump is neutral, leaning hostile.
Alternate juror candidate says Trump's base 'can feel enabled by his rhetoric'
A potential juror who attended the Women's March protest after Trump's presidential election said Trump's base can sometimes "feel enabled by his rhetoric." But she also said she didn't know what his rhetoric is. She said she was familiar with people who made homophobic and racist comments and cited President Trump.
Necheles asked if the potential juror would hold Trump responsible for that, and the woman said no. She said she thought of it as an issue at the ballot box, not in the court room.
Potential juror questioned about attending Women's March
Trump defense attorney Susan Necheles asked a potential juror about having attended the Women's March protest after Trump was elected president in 2016. Asked if there was strong anger toward Trump, the potential juror said she remembered the event as being about women's solidarity.
Another potential juror is excused after saying she was feeling anxiety
A woman being considered for the remaining alternate juror spots spoke up as questioning continued between potential jurors and Trump lawyer Susan Necheles. She said she has started to feel anxiety and asked to approach the judge's bench. After a brief private conversation, Judge Merchan announced she was excused.
Trump lawyer Susan Necheles begins questioning potential jurors
Trump defense lawyer Susan Necheles has begun questioning the potential alternate jurors.
"Biases color the way that we look at the world," she tells them. She asks them to continue to do the best they can "to really be honest."
Hoffinger asking potential jurors about issue that may tie to Michael Cohen
Prosecutor Susan Hoffinger is asking potential jurors how they would feel about the testimony of someone who has pleaded guilty to a crime in the past. Former Trump lawyer Michael Cohen is a potential witness in the case and has pleaded guilty to multiple crimes, including lying to Congress.
Everyone said they would keep an open mind.
Woman who said father is a lifelong Chris Christie friend gets emotional, is excused from jury duty
A prospective juror who earlier in the day said her father is a lifelong friend of former New Jersey Gov. Chris Christie – a one-time Trump ally who broke publicly with Trump after the former president refused to accept the 2020 election results and ran against him in the 2024 Republican primaries – has now been excused.
During individualized questioning by prosecutor Susan Hoffinger, the potential juror suddenly said she thought she "could do this," but it is more stressful than she expected. The woman was emotional, her voice cracking as she spoke.
The judge held a private conversation at his bench, and then stated the woman is excused.
Potential juror disqualified. 'Good luck,' she said as she left
A potential juror who earlier described having a criminal history and doubted she was allowed to serve as a juror has now been formally disqualified. The woman was the 21st potential juror to speak today, and she spoke emotionally about having been incarcerated, at one point mentioning some charges related to drugs. The judge still had her respond to the full jury questionnaire.
The woman said she is a firm believer that when people do something they should be accountable for their actions. In response to one question about following the judge's instructions on the law, she said, "I promise to follow your instructions, sir."
After the break, the judge said the woman was disqualified because she needs a particular certificate to be able to serve. He added that, depending on the nature of her charges, she may be able to serve in the future
"Okay, thank you," the woman said as the judge dismissed her. "Good luck," she added. It wasn't clear to whom she directed that last comment.
Court proceedings resume
Court proceedings resumed around 11:37 a.m. ET.
Court takes 10-minute break
The judge announced a 10-minute break. The potential jurors who spoke today and weren't excused still need to be directly questioned by attorneys for the prosecution and defense. Trump was especially attentive yesterday as potential jurors responded to his lawyer's questioning about their opinions of him.
Potential juror participated in Women's March
We are nearing the end of this batch of potential alternate jurors responding to the jury questionnaire. The current potential juror speaking was born in India and grew up in Minnesota. She said she participated in the Women's March, a protest after Trump was elected president in 2016.
Potential juror's father is lifelong friend of Chris Christie
The 17th potential juror to speak today said her father is a lifelong friend of former Republican New Jersey Governor Chris Christie. Christie was a strong supporter of Trump during the 2016 general election and most of Trump's presidency, but he broke with Trump after Trump refused to accept the results of the 2020 presidential election.
14th potential juror volunteered for Clinton campaign
The 14th potential juror to speak today may not be someone the Trump legal team wants as an alternate. He said he volunteered for the Clinton campaign. That appears to be the Hillary Clinton presidential campaign. The juror questionnaire asks if the jury candidates have ever attended a campaign event for any anti-Trump group or organization.
The man said he gets his news from The New York Times. He doesn't watch much TV, but his television news would be from MSNBC, he said.
Third potential alternate juror excused over impartiality concerns
A third potential juror was excused at the get-go of her time to speak today. She said after reading the 42 questions on the jury questionnaire yesterday, she doesn't think she can be impartial. Judge Merchan released her.
Trump awake, alert again for past several potential jurors
After appearing to be sleepy and struggling to stay awake as potential jurors responded to the jury questionnaire earlier this morning, Trump has been fully awake through the past several potential jurors speaking.
We are on the eleventh potential juror to speak this morning.
Trump Media stock price
At open on Thursday April 19, Trump Media & Technology Group Corp's share price rose to $36.95, up 11.33% from Wednesday April 18's close.
– Kinsey Crowley
Another potential juror says she doesn't think she can be impartial
A woman was excused after she said, upon learning of the questions posed to potential jurors, she doesn't think she can be impartial.
Trump continues to appear sleepy
Trump's eyes have often been closed for extended periods – dozens of seconds at a time – as the third potential juror to respond to the jury questionnaire this morning is speaking.
At one point, his mouth went agape for seconds as his eyes were closed. He just opened his eyes as the jury candidate said she might have read his book, "Trump: The Art of the Deal," when she was younger.
Trump appears sleepy
Trump's eyes have been closed almost entirely for more than two minutes as the second potential juror to be questioned this morning is speaking.
His head dropped down at least three times before he lifted it back up. After the third time, he opened his eyes and said something to his lawyer, Emil Bove. He is now looking around.
First potential alternate juror excused
The first potential juror to be questioned this morning was quickly excused. She raised at the get-go her anxiety about participating in the trial. She said she worried people in her life would figure out she was on the jury over time, even without her telling them, and that could prevent her from being fair and impartial.
Judge Merchan excused her.
Potential jurors arrive and receive questionnaire
The potential alternate jurors who will be questioned to start off the morning have arrived and been passed a copy of the 42-question jury questionnaire.
– Aysha Bagch i
Judge Juan Merchan arrives
Judge Juan Merchan just arrived. One lawyer from each side introduced those at their table, including Trump. The judge said generally, "Good morning," and continued his common practice of greeting Trump directly: "Good morning, Mr. Trump."
Trump enters the courtroom
Former President Donald Trump just entered the courtroom and took a seat at the defense table in between defense lawyers Todd Blanche and Emil Bove. Prosecutors are also seated at their table in the courtroom.
What is Trump on trial for?
Trump has pleaded not guilty to charges that he falsified business records to cover up a hush money payment to porn star Stormy Daniels that was designed to unlawfully interfere in the 2016 presidential election.
The payment was made by former Trump lawyer Michael Cohen and violated federal campaign finance laws, according to prosecutors. They say Trump authorized the payment to help his presidential campaign, and falsified records to cover up the checks he sent to reimburse Cohen for the hush money.
Who are Donald Trump's lawyers?
Trump's defense team is led by Todd Blanche and Susan Necheles .
Blanche was a federal prosecutor for nine years in the Southern District of New York, which includes Manhattan. As a prominent white-collar defense lawyer he has defended Trump advisor Boris Epshteyn and Trump’s former campaign chairman, Paul Manafort.
Necheles is ranked among the top criminal defense lawyers in New York by the legal rating and head-hunting firm Chambers and Partners. She was also a former counsel to Venero Mangano , the former Genovese crime family underboss known as Benny Eggs..
– Josh Meyer
Who is Juan Merchan?
Juan Merchan is the New York judge presiding over Donald Trump's hush money trial, the first criminal trial of a former U.S. president in history.
He was appointed to a family court by Former New York City Mayor Michael Bloomberg in 2006 and appointed to the felony court a few years later.
Trump has repeatedly attacked Merchan, calling him biased because Merchan's daughter works for a marketing firm with Democratic politicians as clients, but an ethics committee has not found grounds to question Merchan's objectivity.
Merchan has ruled against Trump in the past when he presided over a 2022 tax-fraud trial of two parts of the Trump Organization.
So far in this trial, Merchan has imposed a gag order against Trump commenting on witnesses, court staff or prosecutors, expanded it to prohibit comments on family members of himself and Manhattan District Attorney Alvin Bragg, and refused Trump's request to delay the trial
– Kinsey Crowley & Bart Jansen
Will the Trump trial be televised or live streamed?
New York court rules state that audio-visual coverage of trials is not permitted unless a representative of the news media submits an application and the judge allows it, which has not happened for this trial.
– Kinsey Crowley & Aysha Bagchi
What is a Sandoval hearing?
The judge said he could hold a "Sandoval hearing" today. That's a hearing where the judge would consider what kind of evidence the prosecution may introduce on Trump's prior "bad acts" if he chooses to testify.
Trump said last week he plans to testify, although he also said he would testify when the defense had a chance to put on witnesses in his civil fraud trial, only to back out the day before he was scheduled to take the stand.
According to March court filings , the prosecution wants to question Trump about several legal determinations, including a civil jury's finding that Trump sexually abused New York writer E. Jean Carroll.
Advertisement
Supported by
What to Know About the Trial Donald Trump Faces in Manhattan
Prosecutors accused Mr. Trump of falsifying business records to cover up a sex scandal. It is the first criminal trial of a former president.
- Share full article

By Ben Protess , Kate Christobek and Jonah E. Bromwich
Follow our live coverage of Trump’s hush money trial in Manhattan.
Donald J. Trump is now on trial in Manhattan — the first former U.S. president to be criminally prosecuted.
Opening arguments in the trial are set for Monday, following a week of jury selection that whittled hundreds of prospective jurors to a panel of seven men and five women. The trial, which is expected to last about six weeks, will oscillate between salacious testimony on sex scandals and granular detail about corporate documents.
Mr. Trump faces 34 felony counts of falsifying business records in the first degree, all tied to the former president’s role in a hush-money payment to a porn star, Stormy Daniels.
But that payoff is not the only such deal that prosecutors plan to highlight. The prosecutors, from the Manhattan district attorney’s office, have accused Mr. Trump of orchestrating a broader scheme to influence the 2016 presidential election by directing his allies to purchase damaging stories about him to keep them under wraps.
It is the first of Mr. Trump’s four criminal cases to go to trial — and it could be the only one to do so before Election Day.
Mr. Trump, who is again the presumptive Republican nominee for president, has denied all wrongdoing. He also assailed the district attorney, Alvin L. Bragg, for bringing the charges, accusing him of carrying out a politically motivated witch hunt. And he has attacked the judge, Juan M. Merchan.
Here are answers to some key questions about the trial.
Will the trial be televised?
No. There will be no audio or video broadcast of the trial available, though cameras will be stationed in the hallway outside the courtroom to capture Mr. Trump’s remarks going in and out of the trial.
Court will generally be in session every weekday except Wednesdays, from 9:30 a.m. to 4:30 p.m.
What is Mr. Trump accused of?
The charges trace back to a $130,000 hush-money payment that Mr. Trump’s fixer, Michael D. Cohen, made to Ms. Daniels in the final days of the 2016 campaign. The payment, which Mr. Cohen said he had made at Mr. Trump’s direction, suppressed her story of a sexual liaison that she said she had with Mr. Trump.
Paying hush money is not always illegal.

The Donald Trump Indictment, Annotated
The indictment unveiled in April 2023 centers on a hush-money deal with a porn star, but a related document alleges a broader scheme to protect Donald J. Trump’s 2016 campaign.
But while serving as the commander in chief, Mr. Trump reimbursed Mr. Cohen, and the way he did so constituted fraud, prosecutors say.
In internal records, Mr. Trump’s company classified the repayment to Mr. Cohen as legal expenses, citing a retainer agreement. Yet there were no such expenses, the prosecutors say, and the retainer agreement was fictional too.
Those records underpin the 34 counts of falsifying business records: 11 counts involve the checks, 11 center on monthly invoices Mr. Cohen submitted to the company, and 12 involve entries in the general ledger for Mr. Trump’s trust.

Why did prosecutors cite other hush-money payments?
Mr. Bragg’s office linked Mr. Trump to three hush-money deals. While Mr. Trump is indicted only in connection with the business records related to Ms. Daniels, the prosecutors most likely mentioned the other deals to begin the work of proving that Mr. Trump intended to conceal a second crime.
In addition to the indictment, the prosecutors filed a so-called statement of facts that referenced the other payoffs.
That document, common in complex white-collar cases, provides something of a road map for what the prosecutors could reveal at trial. And based on evidence presented to the grand jury, the document details the two hush-money deals involving The National Enquirer, which has longstanding ties to Mr. Trump.
The first involved the tabloid’s payment of $30,000 to a former Trump Tower doorman who claimed to know that Mr. Trump had fathered a child out of wedlock. The publication later determined that the claim was untrue.
The National Enquirer also made a payment to Karen McDougal, Playboy’s Playmate of the Year in 1998, who wanted to sell her story of an affair with Mr. Trump during the 2016 campaign. She reached a $150,000 agreement with the tabloid, which bought the rights to her story to suppress it — a practice known as “catch and kill.”
The deals suggest that the payment to Ms. Daniels was not an isolated incident but rather part of a broader strategy to influence the 2016 election.
Why is it a felony to falsify records?
Falsifying business records in New York State can be a misdemeanor. But it can be elevated to a felony if prosecutors prove that the records were falsified to conceal another crime.
In this case, there are three potential additional crimes that Mr. Bragg has accused Mr. Trump of concealing: a federal campaign finance violation, a state election-law crime and tax fraud.
The campaign crimes, prosecutors say, involve the hush-money payoffs to Ms. Daniels and Ms. McDougal. The payments, they argue, were illegal donations to Mr. Trump’s campaign.
The potential tax fraud stems from the way in which Mr. Cohen was reimbursed for his payment to Ms. Daniels.
Do prosecutors need to convict Mr. Trump of the other crimes?
No. Prosecutors do not have to charge Mr. Trump with any secondary crime or prove that he committed it.
They still must show, however, that there was intent to “commit or conceal” a second crime.
What do we know about the jury?
Because of concerns about the privacy and safety of the jury, the judge restricted the release of identifying information. The panel includes seven men and five women from neighborhoods around the city. Some are middle-aged, and some are young.
Six alternates were also selected and will listen to the testimony in case one of the seated jurors drops out.
The jury was drawn from a pool of residents of Manhattan, where Mr. Trump is deeply unpopular, and during jury selection, dozens of prospective jurors were excused because they said they could not be impartial in deciding a case involving the polarizing former president. Several others were dismissed because of critical social media posts about Mr. Trump.
But the 18 New Yorkers who were selected each pledged to be impartial and decide the case based on the facts. And two of them, during the jury selection process, expressed some affection for the former president.
Who will the witnesses be?
Mr. Cohen is expected to be a crucial witness for the prosecution. His testimony could take days.
Mr. Bragg’s prosecutors are also expected to call David Pecker, the former publisher of the National Enquirer, as well as Hope Hicks, a former campaign and White House aide to Mr. Trump, to shed light on the tumultuous period surrounding the hush-money payments.
Ms. Daniels and Ms. McDougal could be witnesses as well.

Who Are Key Players in the Trump Manhattan Criminal Trial?
The first criminal trial of former President Donald J. Trump is underway. Take a closer look at central figures related to the case.
What will the defense do?
The defense will most likely try to paint Mr. Cohen as a Trump-hating liar, noting that he and the former president had a falling-out years ago. Mr. Trump’s lawyers are expected to emphasize that Mr. Cohen pleaded guilty to a variety of federal crimes in 2018 — including for his role in the hush-money payment.
Much of Mr. Cohen’s testimony is expected to be corroborated by other witnesses, but he might be the only one who can directly tie Mr. Trump to the false business records, a potential weakness of the case that Mr. Trump’s lawyers could seek to exploit.
Whether Mr. Trump’s lawyers will call any witnesses is unclear, but Mr. Trump has said he plans to take the stand in his own defense.
Will Trump attend the trial?
Nothing is ever certain with Mr. Trump, but he is currently expected to attend much of the trial, and he attended each day during jury selection. To be absent, he would need to seek a waiver from the judge. Mr. Trump has already indicated a potential conflict — his youngest son’s high school graduation in May — though it is unclear whether the judge will pause the trial that day or excuse him from attending.
When Mr. Trump is there, it will create a host of security and logistical issues around the Lower Manhattan courthouse. In addition to the U.S. Secret Service protecting Mr. Trump, there will be a heavy police presence outside the building, as protesters and counterprotesters could fill the streets.
Who is the judge?
Justice Merchan is a veteran judge known as a no-nonsense, drama-averse jurist. This case is already testing his patience.
Since the Manhattan district attorney charged Mr. Trump last year, the former president has used campaign emails, social media and repetitive legal filings to attack the judge’s integrity and family. Recently, the former president demanded for a second time that Justice Merchan step aside , citing his daughter’s position at a Democratic consulting firm that worked for the 2020 Biden campaign.
The judge, who denied that request on Monday, has also issued a gag order to protect prosecutors, witnesses and his own family from Mr. Trump’s vitriol. And yet the former president has continued to post articles with pictures of the justice’s daughter.
During the trial, Justice Merchan will be in charge of keeping order in the courtroom and ruling on objections made by prosecutors and Mr. Trump’s lawyers. The jury will ultimately decide whether Mr. Trump is guilty.
What is the maximum sentence if Mr. Trump is convicted?
The charges against Mr. Trump are all Class E felonies, the lowest category of felonies in New York. Each count carries a maximum prison sentence of four years. Justice Merchan has made it clear that he takes white-collar crime seriously and could throw Mr. Trump behind bars. It’s likely, however, that Justice Merchan would impose a concurrent sentence — under which Mr. Trump would serve all prison time simultaneously — if the former president were convicted of more than one count.
And nothing in the law requires Justice Merchan to imprison Mr. Trump if he’s convicted by a jury. The judge could instead sentence him to probation.
Can Trump appeal?
Yes, if he is convicted, Mr. Trump will appeal, a process that could take months or longer.
Mr. Trump could first take the case to the Appellate Division in Manhattan, and, ultimately seek review from the state’s highest court, the Court of Appeals in Albany. In other words, any appeal is unlikely to be resolved before Election Day. And he would most likely remain free at least until it is resolved.
Ben Protess is an investigative reporter at The Times, writing about public corruption. He has been covering the various criminal investigations into former President Trump and his allies. More about Ben Protess
Kate Christobek is a reporter covering the civil and criminal cases against former president Donald J. Trump for The Times. More about Kate Christobek
Jonah E. Bromwich covers criminal justice in New York, with a focus on the Manhattan district attorney’s office and state criminal courts in Manhattan. More about Jonah E. Bromwich
Our Coverage of the Trump Hush-Money Trial
News and Analysis
Manhattan prosecutors delivered a raw recounting of Donald Trump’s seamy past as they debuted their case against him, reducing the former president to a co-conspirator in a plot to cover up three sex scandals that threatened his 2016 election win. Here are five takeaways .
Trump has assembled a team of defense lawyers with extensive experience representing people charged with white-collar crimes to defend him. Here’s a look at his defense team .
With support from demonstrators in Lower Manhattan spotty so far, Donald Trump issued a call to “rally behind MAGA,” and suggested the poor turnout was a result of a plot against his supporters.
More on Trump’s Legal Troubles
Key Inquiries: Trump faces several investigations at both the state and the federal levels, into matters related to his business and political careers.
Case Tracker: Keep track of the developments in the criminal cases involving the former president.
What if Trump Is Convicted?: Could he go to prison ? And will any of the proceedings hinder Trump’s presidential campaign? Here is what we know , and what we don’t know .
Trump on Trial Newsletter: Sign up here to get the latest news and analysis on the cases in New York, Florida, Georgia and Washington, D.C.
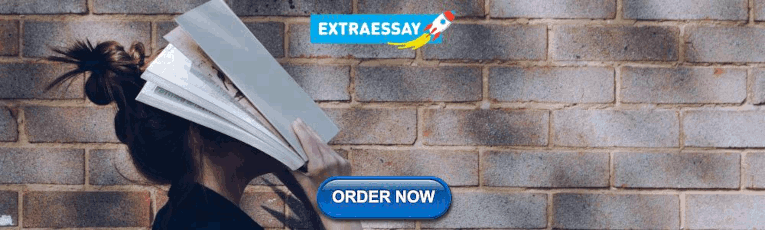
IMAGES
VIDEO
COMMENTS
For causal case studies that employ causal mechanisms, Gerring divides them into exploratory case-selection, estimating case-selection, and diagnostic case-selection. The differences revolve around how the central hypothesis is utilized in the study. Exploratory case studies are used to identify a potential causal hypothesis.
The article then draws attention to two ambiguities in case-selection strategies in case-study research. The first concerns the admixture of several case-selection strategies. The second concerns the changing status of a case as a study proceeds. Some case studies follow only one strategy of case selection.
Yet, while the existing qualitative literature on case selection offers a wide range of suggestions for case selection, most techniques discussed require in-depth familiarity of each case. Seven case selection procedures are considered, each of which facilitates a different strategy for within-case analysis. The case selection procedures ...
Although case studies have been discussed extensively in the literature, little has been written about the specific steps one may use to conduct case study research effectively (Gagnon, 2010; Hancock & Algozzine, 2016).Baskarada (2014) also emphasized the need to have a succinct guideline that can be practically followed as it is actually tough to execute a case study well in practice.
A case study is one of the most commonly used methodologies of social research. This article attempts to look into the various dimensions of a case study research strategy, the different epistemological strands which determine the particular case study type and approach adopted in the field, discusses the factors which can enhance the effectiveness of a case study research, and the debate ...
For case-study analysis, it is often the rareness of the value that makes a case valuable, not its positive or negative value (contrast Emigh 1997; Mahoney and Goertz 2004; Ragin 2000: 60; Ragin 2004: 126). Large-N Analysis. As we have said, extreme cases lie far from the mean of a variable. _.
The second part of the case study discusses the improvements that were made following a system review by the HR manager. Recruitment and Selection Case Study:
Comparative Research Designs and Case Selection 33 3.1 Overview In general, research designs can be located in the following two-dimensional matrix, listing numbers of variables and numbers of cases (see Figure 3.1): Cases 1 2 Small "l" Large "m" n k World system Variables Large "j" Description C 1 V j Paired comparison C 2V j ...
Three case studies have been conducted in the context of two SPOs to evaluate and signify the usefulness and efficiency of the DSS to address MCDM problems, specifically DBMS selection problem in this study. The case study companies considered a number of feasible DBMS technologies for their organisations through multiple internal expert ...
The literature about ERP systems can be analyzed under four main categories: ERP selection, ERP implementation, ERP risk management and general ERP projects. The published research on ERP system selection (as is the case in this study) and implementations constitute roughly about 75% of all published studies [2]. In order to provide a good ...
The goal of this paper is to develop a case study (i.e., problem statement) and a Unified Modeling Language (UML) solution for an automated system that will 1) create and track projects, 2) assign teams to projects, 3) assign tasks to teams, 4) assign the most qualified team members to the teams, and 5) address project costing.
The published research on ERP system selection (as is the case in this study) and implementations constitute roughly about 75% of all published studies [2]. In order to provide a good coverage of the related literature in a concise manner, the scope of the review will be focused on the studies related specifically to ERP system selection ...
Case studies featuring Black protagonists. Curated: August 03, 2020 . Oprah! William W. George ... one lower and one upper case letter, and one special character. Sync Your Subscription Create ...
System selection: creating the right process. By Kate Wadia. In our 12-part series, Kate Wadia - Managing Director at Phase 3 Consulting - guides the HR professional through how to navigate, succeed and lead with HR tech project-work. From the inception of the business case to the handover into BAU, we'll follow an indicative project ...
Discover DelCor's holistic approach to system selection that resulted in the identification of a new certificate management system by defining the association's selection criteria as well as streamlining the association's certification process. Don't let system selection overwhelm your association or nonprofit. DelCor System Selection Case ...
In the second phase, the authors present a modular approach to ERP vendor and software selection and propose a 0‐1 programming model to minimize total costs associated with procurement and integration expenditures., - The proposed approach and the model are considered to be more useful for small manufacturing enterprises (SMEs)., - In ...
ERP case study examples would include an ERP selection project for a manufacturer or distributor, or an ERP implementation for an e-commerce company or retail chain. Our free library of ERP software case studies gives you access to ERP success stories from different perspectives: vendors, resellers, and service providers.
System design is a structured process that involves several stages. It involves a methodical process of conceptualization, refinement, and construction. This process serves as the blueprint for transforming abstract ideas into tangible and functional software systems. Now, let's delve into the phases that constitute the process of system design:
5. A framework for selecting an appropriate ERP system The proposed framework aimed to enhance the overall ERP implementation outcomes, and identify an overall roadmap for SMEs to assist decision-making to select the appropriate ERP system. The developed framework consist of three main phases: Identifying current company processes and ...
To illustrate the proposed simulation approach for the multi-echelon inventory system selection problem, we provide an application through the comparison of the 4 alternatives defined in Table 3 for the case of the Moroccan pharmaceutical products supply chain in the public sector. The model parameters are based on data provided by the ...
The approach taken in this w ork consist of two phases: the first phase is a literature review of studies in ERP selection, to develop a theoretical framew ork, second phase, present s a ...
This research, presents an explanatory case study, which employed a simple multi-attribute rating technique (SMART)-based ERP selection method. The selection method was mainly based on developing process maps for all the critical business processes inside the organization, and then checking the degree of compliance of the potential ERP packages ...
Keywords: building construction project; formwork system selection; decision making; rough numbers; rough AHP; rough EDAS; case study 1. Introduction The formwork system (FWS) gives the geometry and strength required by the rein-forced concrete (RC) structure to attain the desired form and structural design properties of the cured concrete [1,2].
A detailed comparative study of the landslide prediction system in a site-specific application using different ML classification algorithms such as Gaussian ... The selection of reference rain gauge stations for predicting rainfall-induced landslides in a regional-specific application a challenging factor, especially when considering sparsely ...
Donald Trump faces four separate indictments. And only one of them will go to trial before November. That's a case that got underway yesterday in a Manhattan courtroom with jury selection. Trump ...
1:29. NEW YORK — A man threw conspiracy pamphlets into the air and set himself on fire Friday outside the courthouse where former President Donald Trump's hush money trial is taking place ...
According to research findings, this system has a cost of energy of 0.186 $/kWh, net present cost of 2.68 × 10 8 $, and pollutants emission of 53,902.7 tons, which are 80.11%, 76.89%, and 79.14% less than the DIG-only system, respectively. Furthermore, dump energy is found to be the lowest when both BB and FC are installed together, which ...
April 18, 2024. Follow our live coverage of Trump's hush money criminal trial in Manhattan. At 4:34 p.m. on Thursday, a jury of 12 citizens was selected to determine the fate of an indicted ...
W. weigh-in-motion (WIM). - A process of estimating a moving vehicle's gross weight and the portion of that weight that is carried by each wheel, axle, or axle group, or combination thereof, by measurement and analysis of dynamic vehicle tire forces. [2.25] weigh-in-motion screening scale.
Mr. Trump faces 34 felony counts of falsifying business records in the first degree, all tied to the former president's role in a hush-money payment to a porn star, Stormy Daniels. But that ...