Causal Arguments
Sample causal argument.
Now that you have had the chance to learn about writing a causal argument, it’s time to see what one might look like. Linked, you’ll see a sample causal argument essay written following MLA formatting guidelines.
- Sample Causal Argument. Authored by : OWL Excelsior Writing Lab. Provided by : Excelsior College. Located at : http://owl.excelsior.edu/argument-and-critical-thinking/argumentative-purposes/argumentative-purposes-sample-causal-argument/ . Project : ENG 101. License : CC BY-SA: Attribution-ShareAlike
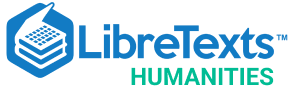
- school Campus Bookshelves
- menu_book Bookshelves
- perm_media Learning Objects
- login Login
- how_to_reg Request Instructor Account
- hub Instructor Commons
- Download Page (PDF)
- Download Full Book (PDF)
- Periodic Table
- Physics Constants
- Scientific Calculator
- Reference & Cite
- Tools expand_more
- Readability
selected template will load here
This action is not available.
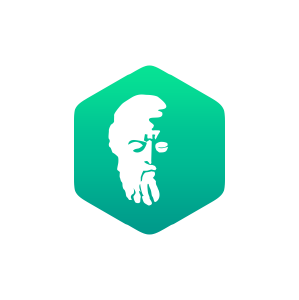
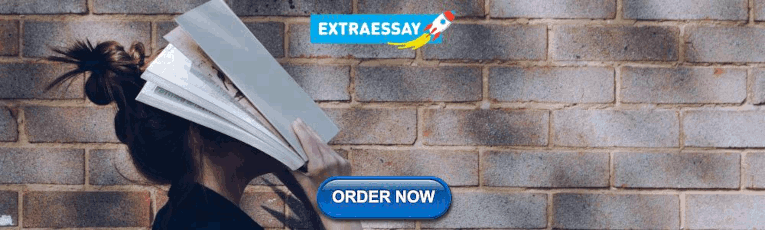
14.2: Organizing the Causal Analysis Essay
- Last updated
- Save as PDF
- Page ID 4998
The causal analysis essay can be split into four basic sections: introduction, body, conclusion, and Works Cited page. There are three basic formats for writing a cause/effect:
- Single effect with multiple causes–air pollution is the effect, and students would identify several causes;
- Single cause with multiple effects–bullying is the cause, and students would establish several effects it has on children;
- Causal Chain–This is a more complex format. Causal chains show a series of causes and effects. For example, dust storms between Tucson and Phoenix can be deadly causing a chain reaction of accidents. The dust is the initial catalyst. It causes car A to stop. Car B crashes into Car A. Car C crashes into Car B., etc. Climate change is a good example of a causal chain topic. Population increase is causing an increase in traffic and greenhouse gases. It is also causing an increase in deforestation for housing, roads and farming. Deforestation means less plants to take up the CO2 and release O2 into the environment. Each item causes an effect. That effect causes another effect. All of this contributes to climate change.
Introduction
The introduction introduces the reader to the topic. We’ve all heard that first impressions are important. This is very true in writing as well. The goal is to engage the readers, hook them so they want to read on. One way is to write a narrative. Topics like bullying or divorce hit home. Beginning with a real case study highlights the issue for readers. This becomes an example that you can refer to throughout the paper. The final sentence in the introduction is usually the thesis statement.
Another way to introduce the topic is to ask a question or set of questions then provide background and context for the topic or issue. For example, if you are writing an essay about schizophrenia, opening questions might be “What are the main causes of schizophrenia? Who is susceptible?” The student would then begin a brief discussion defining schizophrenia and explaining its significance. Once again, the final sentence of the introduction would be a thesis statement introducing the main points that will be covered in the paper.
Body Paragraphs
The body of the essay is separated into paragraphs. Each paragraph covers a single cause or effect. For example, according to the National Institute of Mental Health, the two main causes of schizophrenia are genetic and environmental. Thus, if you were writing about the causes of schizophrenia, then you would have a body paragraph on genetic causes of schizophrenia and a body paragraph on the environmental causes. A second example is climate change where separate paragraphs explain each cause/effect relationship: population increases, increases in air pollution due to traffic exhaust and manufacturing, increases in food production and agriculture, deforestation. All are causes for climate change, and all are intricately linked.
A body paragraph should include the following:
- Topic sentence that identifies the topic for the paragraph,
- Several sentences that describes the causal relationship,
- Evidence from outside sources that corroborates your claim that the causal relationship exists,
- MLA formatted in-text citations indicating which source listed on the Works Cited page has provided the evidence,
- Quotation marks placed around any information taken verbatim (word for word) from the source,
- Summary sentence(s) that draws conclusions from the evidence,
- Remember: information from outside sources should be placed in the middle of the paragraph and not at the beginning or the end of the paragraph;
- Be sure and use transitions or bridge sentences between paragraphs.
- Draw final conclusions from the key points and evidence provided in the paper;
- Tie in the introduction. If you began with a story, draw final conclusions from that story;
- If you began with a question(s), refer back to the question(s) and be sure to provide the answer(s).
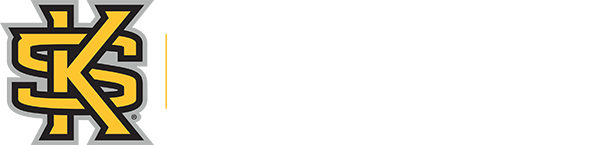
- Writing Center
- Current Students
- Online Only Students
- Faculty & Staff
- Parents & Family
- Alumni & Friends
- Community & Business
- Student Life
- Video Introduction
- Become a Writing Assistant
- All Writers
- Graduate Students
- ELL Students
- Campus and Community
- Testimonials
- Encouraging Writing Center Use
- Incentives and Requirements
- Open Educational Resources
- How We Help
- Get to Know Us
- Conversation Partners Program
- Workshop Series
- Professors Talk Writing
- Computer Lab
- Starting a Writing Center
- A Note to Instructors
- Annotated Bibliography
- Literature Review
- Research Proposal
- Argument Essay
- Rhetorical Analysis
Argument Essay Guidelines
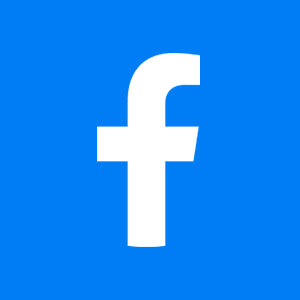
Note to instructors: These guidelines provide possible choices for instructors assigning argument essays. They include options for proposal arguments, definition arguments, and causal arguments. You are encouraged to adopt, adapt, or remix these guidelines to suit your goals for your class.
Due Dates
- Rough Draft:
- Peer Review:
- Final Draft:
This assignment will help you learn to create an effective argument (i.e., convey your meaning persuasively) using evidence, sound reasoning, and awareness of rhetorical principles.
Skills: This assignment will help you practice skills essential to success in and beyond this course:
- Support claims effectively using varied forms of evidence.
- Analyze how style, audience, social context, and purpose shape your writing in electronic and print spaces.
Knowledge: This assignment will help you become familiar with the following important knowledge:
- Demonstrate an understanding of the rhetorical situation.
- Apply elements of rhetoric and argumentation in writing.
You may choose to write a proposal argument , a definition argument , or a causal argument . Directions for each type of argument are provided below. No matter what type of argument you choose to write, the following apply:
- Topic : Seek approval from your instructor on your topic before beginning this assignment. Audience: Select a primary audience who you think should care about your argument. Use appropriate language, organization, and examples to suit your audience. Note that your secondary audience is your classmates and instructor.
- Purpose and significance : The culprits or consequences shouldn't be so obvious that no argument is necessary. Consider the following questions as you establish the purpose and significance of your argument: Why is the argument important? Why is it controversial? What is at stake?
- Counterarguments : Take alternative viewpoints seriously. Your goal should be to choose the strongest support for your argument, but remember that real-world problems are rarely simple, so avoid taking an all-or-nothing position. Take opposing viewpoints into consideration and make concessions and offer counterarguments as appropriate.
- Research : If directed to incorporate research by your instructor, include information from at least three credible sources. Integrate source information effectively by using lead-in phrases; summarizing, paraphrasing, and quoting as appropriate; and citing correctly, both within the body of your essay and on the Works Cited page.
- Length and format : Your essay should be 4–5 pages long, double-spaced.
Formatting requirements: Follow MLA format. Use black Calibri or Times New Roman font in size 12. Double-space the entire document. Use 1-inch margins on all sides.
Option 1: Proposal Argument
Write a practical proposal that in which you recommend a solution to a problem that you perceive in the world. Choose an important topic that matters to you and that you believe will matter to others; craft a proposal in which you argue for positive change.
In general, a proposal argument essay should have three main sections:
- A description of the problem
- Your proposed solution
- Justification for the solution you are proposing
(You may [and should] include additional sections or subsections as needed.)
Example of a proposal argument thesis statement : Our school should require all incoming freshmen to take a philosophy class so they can learn to understand and analyze multiple perspectives.
Examples of questions you might consider:
- Should students be required to take philosophy classes?
- Should new and expecting parents be given the option of enrolling in parenting classes at no cost to them?
- Should the county install cameras on all traffic lights?
- Should the school cut down a forested acre of land to build a new parking lot?
- Should public schools teach both English and Spanish beginning in kindergarten?
Option 2: Definition Argument
Construct a definition argument based on your definition of a controversial term: argue that an object or concept qualifies as a certain term, which you define based on specific criteria. Choose an important term that matters to you and that you believe will matter to others; base your argument on your own definition of that term.
Example of a definition argument thesis statement: Caffeine is a drug because it has predictable, measurable effects on the user’s central nervous system. Potential words for definition: animal, animal cruelty, art, beautiful, cheating, disease, drug, evil, food, hate crime, healthy, natural, poison, role model, sport You may choose a word not on the list. However, be sure to 1) check with your instructor, and 2) choose something that can be reasonably debated.
- Is caffeine a drug?
- Is alcoholism a disease?
- Are human beings animals?
- Is refined sugar a poison?
- Is kayaking a sport?
Examples of definition argument thesis statements:
- Caffeine is a drug because it causes physiological changes and is addictive.
- Kayaking is a sport because it requires physical exertion and can be done competitively.
Option 3: Causal Argument
Construct a causal argument in which you argue that a specific cause or causes led to a specific outcome, or that a specific action will result in a specific consequence. (Do NOT argue that a person or group should or should not take an action; this would be a proposal rather than a causal argument.)
Example of a causal argument thesis statement: Regular journaling leads to improved academic outcomes for incoming freshmen by helping them cope with stress, improve their writing skills, and develop their ability to concentrate for sustained periods of time.
- Does journaling lead to benefits for students?
- Do stimulants cause psychosis?
- Does advertising cause men and women to have unrealistic ideals of physical attractiveness?
- What causes, has caused, or will cause unemployment rates to rise or lower? (For an issue like this, it would be a good idea to consider a specific place and time—for example, in the state of Georgia from 2018-2019.)
- Why or how do people become addicted to a specific substance (sugar, illicit drugs, alcohol, etc.)?
Criteria for success
The argument essay should adhere to the following guidelines: .
- The essay meets requirements for the type of argument it is intended to convey (definition argument, proposal argument, or causal argument).
- The essay is based on a clearly stated, arguable thesis statement.
- The thesis is appropriately developed and supported: the writer has provided evidence, examples, and analysis as appropriate.
- The essay is cohesive/stays on topic.
- The writing is clear and coherent/makes sense.
- The tone and language are appropriate for the audience and purpose.
- The essay has an interesting, relevant title.
- The writer has gone through the entire writing process, revising substantially and thoughtfully.
- The writing adheres to grammar and punctuation rules.
The argument essay should adhere to all formatting criteria:
- MLA format, in the essay and on the Works Cited page
- Essay length: 800-1200 words
- Font: Size 12, Times New Roman font throughout
- Double-spaced lines
- 1-inch margins on all sides

Contact Info
Kennesaw Campus 1000 Chastain Road Kennesaw, GA 30144
Marietta Campus 1100 South Marietta Pkwy Marietta, GA 30060
Campus Maps
Phone 470-KSU-INFO (470-578-4636)
kennesaw.edu/info
Media Resources
Resources For
Related Links
- Financial Aid
- Degrees, Majors & Programs
- Job Opportunities
- Campus Security
- Global Education
- Sustainability
- Accessibility
470-KSU-INFO (470-578-4636)
© 2024 Kennesaw State University. All Rights Reserved.
- Privacy Statement
- Accreditation
- Emergency Information
- Report a Concern
- Open Records
- Human Trafficking Notice
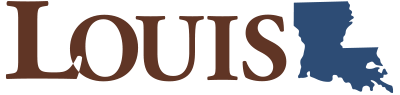
63 5.8 Causal and Proposal Arguments
Causal arguments attempt to make a case that one thing led to another. They answer the question “What caused it?” Causes are often complex and multiple. Before we choose a strategy for a causal argument it can help to identify our purpose. Why do we need to know the cause? How will it help us?
Purposes of causal arguments
To get a complete picture of how and why something happened: In this case, we will want to look for multiple causes, each of which may play a different role. Some might be background conditions, others might spark the event, and others may be influences that sped up the event once it got started. In this case, we often speak of near causes that are close in time or space to the event itself, and remote causes , which are further away or further in the past. We can also describe a chain of causes , with one thing leading to the next, which leads to the next. It may even be the case that we have a feedback loop where a first event causes a second event and the second event triggers more of the first, creating an endless circle of causation. For example, as sea ice melts in the arctic, the dark water absorbs more heat, which warms it further, which melts more ice, which makes the water absorb more heat, etc. If the results are bad, this is called a vicious circle.
To decide who is responsible: Sometimes if an event has multiple causes, we may be most concerned with deciding who bears responsibility and how much. In a car accident, the driver might bear responsibility and the car manufacturer might bear some as well. We will have to argue that the responsible party caused the event but we will also have to show that there was a moral obligation not to do what the party did. That implies some degree of choice and knowledge of possible consequences. If the driver was following all good driving regulations and triggered an explosion by activating the turn signal, clearly the driver cannot be held responsible.
To figure out how to make something happen: In this case we need to zero in on a factor or factors that will push the event forward. Such a factor is sometimes called a precipitating cause . The success of this push will depend on circumstances being right for it, so we will likely also need to describe the conditions that have to be in place for the precipitating cause to actually precipitate the event. If there are likely factors that could block the event, we need to show that those can be eliminated. For example, if we propose a particular surgery to fix a heart problem, we will also need to show that the patient can get to a hospital that performs the surgery and get an appointment. We will certainly need to show that the patient is likely to tolerate the surgery.
To stop something from happening: In this case, we do not need to describe all possible causes. We want to find a factor that is so necessary to the bad result that if we get rid of that factor, the result cannot occur. Then if we eliminate that factor, we can block the bad result. If we cannot find a single such factor, we may at least be able to find one that will make the bad result less likely. For example, to reduce wildfire risk in California, we cannot get rid of all fire whatsoever, but we can repair power lines and aging gas and electric infrastructure to reduce the risk that defects in this system will spark a fire. Or we could try to reduce the damage fires cause by focusing on clearing underbrush.
To predict what might happen in future: As Jeanne Fahnestock and Marie Secor put it in A Rhetoric of Argument , “When you argue for a prediction, you try to convince your reader that all the causes needed to bring about an event are in place or will fall into place.” You also may need to show that nothing will intervene to block the event from happening. One common way to support a prediction is by comparing it to a past event that has already played out. For example, we might argue that humans have survived natural disasters in the past, so we will survive the effects of climate change as well. As Fahnestock and Secor point out, however, “the argument is only as good as the analogy, which sometimes must itself be supported.” How comparable are the disasters of the past to the likely effects of climate change? The argument would need to describe both past and possible future events and convince us that they are similar in severity.
Techniques and cautions for causal argument
So how does a writer make a case that one thing causes another? The briefest answer is that the writer needs to convince us that the factor and the event are correlated and also that there is some way in which the factor could plausibly lead to the event. Then the writer will need to convince us that they have done due diligence in considering and eliminating alternate possibilities for the cause and alternate explanations for any correlation between the factor and the event.
Identify possible causes
If other writers have already identified possible causes, an argument simply needs to refer back to those and add in any that have been missed. If not, the writer can put themselves in the role of detective and imagine what might have caused the event.
Determine which factor is most correlated with the event
If we think that a factor may commonly cause an event, the first question to ask is whether they go together. If we are looking for a sole cause, we can ask if the factor is always there when the event happens and always absent when the event doesn’t happen. Do the factor and the event follow the same trends? The following methods of arguing for causality were developed by philosopher John Stuart Mill, and are often referred to as “Mill’s methods.”
If the event is repeated and every time it happens, a common factor is present, that common factor may be the cause.
If there is a single difference between cases where the event takes place and cases where it doesn’t.
If an event and a possible cause are repeated over and over and they happen to varying degrees, we can check whether they always increase and decrease together. This is often best done with a graph so we can visually check whether the lines follow the same pattern.
Finally, ruling out other possible causes can support a case that the one remaining possible cause did in fact operate.
Explain how that factor could have caused the event
In order to believe that one thing caused another, we usually need to have some idea of how the first thing could cause the second. If we cannot imagine how one would cause another, why should we find it plausible? If we are talking about human behavior, then we are looking for motivation: love, hate, envy, greed, desire for power, etc. If we are talking about a physical event, then we need to look at physical forces. Scientists have dedicated much research to establishing how carbon dioxide in the atmosphere could effectively trap heat and warm the planet.
If there is enough other evidence to show that one thing caused another but the way it happened is still unknown, the argument can note that and perhaps point toward further studies that would establish the mechanism. The writer may want to qualify their argument with “may” or “might” or “seems to indicate,” if they cannot explain how the supposed cause led to the effect.
Eliminate alternative explanations
The catchphrase “correlation is not causation” can help us to remember the dangers of the methods above. It’s usually easy to show that two things happen at the same time or in the same pattern, but hard to show that one actually causes another. Correlation can be a good reason to investigate whether something is the cause, and it can provide some evidence of causality, but it is not proof. Sometimes two unrelated things may be correlated, like the number of women in Congress and the price of milk. We can imagine that both might follow an upward trend, one because of the increasing equality of women in society and the other because of inflation. Describing a plausible agency, or way in which one thing led to another, can help show that the correlation is not random. If we find a strong correlation, we can imagine various causal arguments that would explain it and argue that the one we support has the most plausible agency.
Sometimes things vary together because there is a common cause that affects both of them. An argument can explore possible third factors that may have led to both events. For example, students who go to elite colleges tend to make more money than students who go to less elite colleges. Did the elite colleges make the difference? Or are both the college choice and the later earnings due to a third cause, such as family connections? In his book Food Rules: An Eater’s Manual , journalist Michael Pollan assesses studies on the effects of supplements like multivitamins and concludes that people who take supplements are also those who have better diet and exercise habits, and that the supplements themselves have no effect on health. He advises, “Be the kind of person who takes supplements — then skip the supplements.”
If we have two phenomena that are correlated and happen at the same time, it’s worth considering whether the second phenomenon could actually have caused the first rather than the other way around. For example, if we find that gun violence and violence within video games are both on the rise, we shouldn’t leap to blame video games for the increase in shootings. It may be that people who play video games are being influenced by violence in the games and becoming more likely to go out and shoot people in real life. But could it also be that as gun violence increases in society for other reasons, such violence is a bigger part of people’s consciousness, leading video game makers and gamers to incorporate more violence in their games? It might be that causality operates in both directions, creating a feedback loop as we discussed above.
Proving causality is tricky, and often even rigorous academic studies can do little more than suggest that causality is probable or possible. There are a host of laboratory and statistical methods for testing causality. The gold standard for an experiment to determine a cause is a double-blind, randomized control trial in which there are two groups of people randomly assigned. One group gets the drug being studied and one group gets the placebo, but neither the participants nor the researchers know which is which. This kind of study eliminates the effect of unconscious suggestion, but it is often not possible for ethical and logistical reasons.
The ins and outs of causal arguments are worth studying in a statistics course or a philosophy course, but even without such a course we can do a better job of assessing causes if we develop the habit of looking for alternate explanations.
Practice Exercise
Reflect on the following to construct a causal argument. What would be the best intervention to introduce in society to reduce the rate of violent crime? Below are some possible causes of violent crime. Choose one and describe how it could lead to violent crime. Then think of a way to intervene in that process to stop it. What method from among those described in this section would you use to convince someone that your intervention would work to lower rates of violent crime? Make up an argument using your chosen method and the kind of evidence, either anecdotal or statistical, you would find convincing.
Possible causes of violent crime:
Homophobia and transphobia
Testosterone
Child abuse
Violence in the media
Role models who exhibit toxic masculinity
Violent video games
Systemic racism
Lack of education on expressing emotions
Unemployment
Not enough law enforcement
Economic inequality
The availability of guns
Proposal arguments
Proposal arguments attempt to push for action of some kind. They answer the question “What should be done about it?”
In order to build up to a proposal, an argument needs to incorporate elements of definition argument, evaluation argument, and causal argument. First, we will need to define a problem or a situation that calls for action. Then we need to make an evaluation argument to convince readers that the problem is bad enough to be worth addressing. This will create a sense of urgency within the argument and inspire the audience to seek and adopt proposed action. In most cases, it will need to make causal arguments about the roots of the problem and the good effects of the proposed solution.
Common elements of proposal arguments
Background on the problem, opportunity, or situation.
Often just after the introduction, the background section discusses what has brought about the need for the proposal—what problem, what opportunity exists for improving things, what the basic situation is. For example, management of a chain of daycare centers may need to ensure that all employees know CPR because of new state mandates requiring it, or an owner of pine timberland in eastern Oregon may want to make sure the land can produce saleable timber without destroying the environment.
While the named audience of the proposal may know the problem very well, writing the background section is useful in demonstrating our particular view of the problem. If we cannot assume readers know the problem, we will need to spend more time convincing them that the problem or opportunity exists and that it should be addressed. For a larger audience not familiar with the problem, this section can give detailed context.
Description of the proposed solution
Here we define the nature of what we are proposing so readers can see what is involved in the proposed action. For example, if we write an essay proposing to donate food scraps from restaurants to pig farms, we will need to define what will be considered food scraps. In another example, if we argue that organic produce is inherently healthier for consumers than non-organic produce, and we propose governmental subsidies to reduce the cost of organic produce, we will need to define “organic” and describe how much the government subsidies will be and which products or consumers will be eligible. These examples illustrate the frequency with which different types of argument overlap within a single work.
If we have not already covered the proposal’s methods in the description, we may want to add this. How will we go about completing the proposed work? For example, in the above example about food scraps, we would want to describe how the leftover food will be stored and delivered to the pig farms. Describing the methods shows the audience we have a sound, thoughtful approach to the project. It serves to demonstrate that we have the knowledge of the field to complete the project.
Feasibility of the project
A proposal argument needs to convince readers that the project can actually be accomplished. How can enough time, money, and will be found to make it happen? Have similar proposals been carried out successfully in the past? For example, we might observe that according to the U.S. Environmental Protection Agency, Rutgers University runs a program that sends a ton of food scraps a day from its dining halls to a local farm. If we describe how other efforts overcame obstacles, we will persuade readers that if they can succeed, this proposal can as well.
Benefits of the proposal
Most proposals discuss the advantages or benefits that will come from the solution proposed. Describing the benefits helps you win the audience to your side, so readers become more invested in adopting your proposed solution. In the food scraps example, we might emphasize that the Rutgers program, rather than costing more, led to $100,000 a year in savings because the dining halls no longer needed to pay to have the food scraps hauled away. We could calculate the predicted savings for our new proposed program as well.
In order to predict the positive effects of the proposal and show how implementing it will lead to good results, we will want to use causal arguments.
Sample annotated proposal argument
The sample essay “Why We Should Open Our Borders” by student Laurent Wenjun Jiang can serve as an example. Annotations point out how Jiang uses several proposal argument strategies.
Sample proposal essay “Why We Should Open Our Borders” in PDF with margin notes
Browse news and opinion websites to find a proposal argument that you strongly support. Once you have chosen a proposal, read it closely and look for the elements discussed in this section. Do you find enough discussion of the background, methods, feasibility, and benefits of the proposal? Discuss at least one way in which you think the proposal could be revised to be even more convincing.
Attributions
Parts of this section on proposal arguments are original content by Anna Mills and Darya Myers. Parts were adapted from Technical Writing, which was derived in turn by Annemarie Hamlin, Chris Rubio, and Michele DeSilva, Central Oregon Community College, from Online Technical Writing by David McMurrey – CC BY 4.0 .
5.9 Argumentative Reasoning
By Adam Falik
Once you have clearly articulated a thesis, you need to support that claim with reasons. Reasons answer the question: Why should the claim be? Reasons justify the claim, and in an argument, support the claim’s validity.
The reasons for an argument should follow a “because.” That “because” can either be present or implied. Consider this example:
College athletes should be paid [Claim] because they generate income for their school [Reason] while being unable to obtain employment of their own due to the demands of academic and athletic schedules. [Reason]
Reasons are the backbone of your argument. Your argumentative paper will be mostly comprised of the articulation of your claim, an explanation of reasons, and evidence that back up your reasons.
Not All Reasons Are The Same
Not all reasons are of equal validity. The truth is that some of your reasons may be more urgent or stronger than others. Let’s say you make the rather simple claim that a cigarette smoker should quit smoking. Your claim: A cigarette smoker should break the habit and quit smoking , can be supported by (at a minimum) three reasons:
1) Smoking is damaging for one’s health
2) Second-hand smoking is damaging to other people’s health
3) Cigarette butts have a negative environmental impact on the planet
It can be argued that compared to the risk of heart disease, emphysema, and lung cancer threatening habitual cigarette smokers, as well as the health dangers to those who are impacted by second-hand smoke, that the environmental impact of cigarette butts is of lesser value. And that might be true. Though the majority of this paper might be focused on health risks, the environmental impact is still significant and warrants inclusion in the paper. The point is that not all reasons are equal in value, and not all reasons will be supported with equal amounts of evidence.
There is no exact number of reasons that should be included in support of a claim, just as there is no precise number of cited evidence that should support a reason. Generally, quality will reign over quantity. A few strong reasons that are supported by credible evidence are better than lots of reasons that are either unsupported by evidence, or supported by weaker evidence.
CLAIMS/REASONS/EVIDENCE GRAPHIC (coming)
5.10 SUPPORTING EVIDENCE
Amanda Lloyd, Adam Falik and Doreen Piano
Adding Supporting Evidence to Body Paragraphs
Supporting your ideas effectively is essential to establishing your credibility as a writer, so you should choose your supporting evidence wisely and clearly explain it to your audience.
Present your supporting evidence in the form of paraphrases and direct quotations. Quotations should be used sparingly; that said, direct quotations are often handy when you would like to illustrate a particularly well- written passage or draw attention to an author’s use of tone, diction, or syntax that would likely become lost in a paraphrase.
Types of support might include the following:
• Statistics and data
• Research studies and scholarship
• Hypothetical and real-life examples
• Historical facts
• Analogies
• Precedents
• Case histories
• Expert testimonies or opinions
• Eye-witness accounts
• Applicable personal experiences or anecdotes
Varying your means of support will lend further credibility to your essay and help to maintain your reader’s interest. Keep in mind, though, that some types of support are more appropriate for certain academic disciplines than for others.
Remember that in an argumentative paper, your evidence supports your reason. In the paragraph referred to above with the topic sentence “Colleges athletes often bring in a great deal of income to their college and university through sponsorships,” your evidence might be data that and statistics of athletes who have brought in sponsorship deals from which their colleges and universities have profited.
Direct quotations and paraphrases must be integrated effortlessly and documented appropriately.
Providing Context for Supporting Evidence
Before introducing your supporting evidence, it may occasionally be necessary to provide some context for that information. You should assume that your audience has not read your source texts in their entirety, if at all, so including some background or connecting material between your topic sentence and supporting evidence is frequently essential.
The information contained in your evidence selection might need to be introduced, explained, or defined so that your supporting evidence is perfectly clear to an audience unfamiliar with the source material. For example, your supporting evidence might contain a reference to a concept or term that is not explained or defined in the excerpt or elsewhere in your essay. In this instance, you would need to provide some clarification for your audience. Anticipating your audience is particularly important when incorporating supporting evidence into your essay.
Now that we have a good idea what it means to develop support for the main ideas of your paragraphs, let’s talk about how to make sure that those supporting details are solid and convincing.
Good vs. Weak Support
When you’re developing paragraphs, you should already have a plan for your essay, at least at the most basic level. You know what your topic is, you have a working claim, and you have at least a couple of supporting ideas/reasons in mind that will further develop and support your claim. You need to make sure that the support that you develop for these ideas is solid. Understanding and appealing to your audience can also be helpful in determining what your readers will consider good support and what they’ll consider to be weak. Here are some tips on what to strive for and what to avoid when it comes to supporting evidence.
Good Support
• is relevant and focused (sticks to the point)
• is well developed
• provides sufficient detail
• is vivid and descriptive
• is well organized
• is coherent and consistent
• highlights key terms and ideas
Weak Support
• lacks a clear connection to the point that it’s meant to support
• lacks development
• lacks detail or gives too much detail
• is vague and imprecise
• lacks organization
• seems disjointed (ideas don’t clearly relate to each other)
• lacks emphasis of key terms and ideas
How Much Evidence Do I Need?[DP1]
(NOTE: This should be one paragraph but the formatting is off)
Students often ask: How much evidence do I need? The answer is:
You need exactly the amount of evidence that makes your reason supportable.
In other words: There is no exact quantity of direct and indirect quotations you should be providing.
What matters is that you’ve supported your idea/reason with good enough support to convince your
reader of the integrity of your reason.
This chapter contains material from “The Word on College Reading and Writing” by Monique Babin, Carol Burnell, Susan Pesznecker, Nicole Rosevear, Jaime Wood, OpenOregon Educational Resources , Higher Education Coordination Commission: Office of Community Colleges and Workforce Development is licensed under CC BY-NC 4.0
5.11 EXPLAINING EVIDENCE
Remember not to conclude your body paragraph with supporting evidence. Rather than assuming that the evidence you have provided speaks for itself, it is important to explain why that evidence proves or supports the key idea you present in your topic sentence and (ultimately) the claim you make in your thesis statement.
This explanation can appear in one or more of the following forms:
• Evaluation
• Relevance or significance
• Comparison or contrast
• Cause and effect
• Refutation or concession
• Suggested action or conclusion
• Proposal of further study
• Personal reaction
Try to avoid simply repeating the source material in a different way or using phrases like “This quote means” to begin your explanation. Keep in mind that your voice should control your essay and guide your audience to a greater understanding of the source material’s relevance to your claim. Also, be mindful of the rhythm of your body paragraphs and the placement of your evidence. Try not to structure the same paragraphs over and over with a topic sentence, a quote, then the explanation of that quote. Seek variety. Paragraphs that repeat themselves in structures run the risk of boring readers.
Sandbox: Interactive OER for Dual Enrollment Copyright © by LOUIS: The Louisiana Library Network is licensed under a Creative Commons Attribution 4.0 International License , except where otherwise noted.
Share This Book
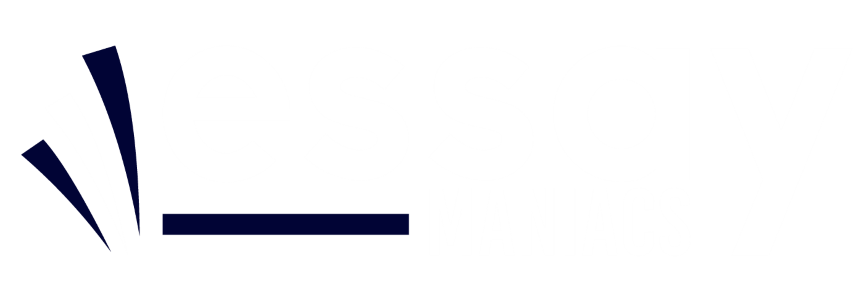
- Place order
How to write a causal Analysis Essay that scores an A
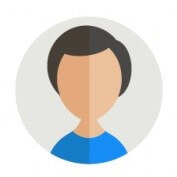
It is human nature to want to understand things and what causes them. When we see, hear, or experience something we do not understand, we often try to find out the cause or the explanation either from family and friends or from the internet.
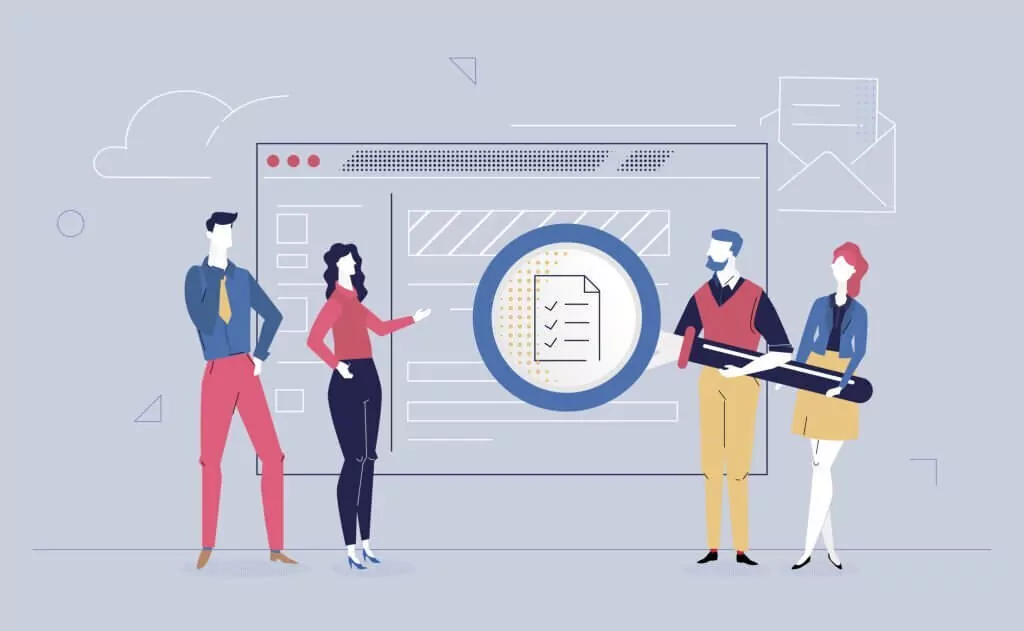
In college, you will be required occasionally to find out the causes, effects, or reasons for various phenomena via causal analysis essay assignments.
This article details everything important about causal analysis essay assignments, including the structure and the steps for writing one.
What Is a Causal Analysis Essay?
Strictly put, a causal analysis essay assignment or an academic writing task requires you to explain the cause of a specific phenomenon analytically.
Causal analysis essays are sometimes referred to as cause-and-effect essays. Because they also reveal how one thing (cause) leads to another (effect). In this respect, when writing a causal analysis essay, you first begin by stating your claim and then backing it up using arguments and supporting facts. You need to show how a specific issue correlates to an underlying problem.
For example, you can be tasked with writing about how the global COVID-19 pandemic led to the rise of e-learning. You need to explore e-learning adoption before and post the pandemic to unravel the real issues that amount as cause and the effects of these issues on e-learning adoption.
Structure of a Causal Analysis Essay
The structure of a causal analysis essay is the typical short essay structure. It is a five-paragraph structure essay with an introduction paragraph, 3 body paragraphs, and a conclusion paragraph. If you follow this structure to write your essay, as your professor expects, you will end up with an academic paper with a strong logical flow.
Find out what to include in each paragraph of your causal analysis essay in the subsection below.
1. Introduction Paragraph
In the introduction paragraph of an essay , you introduce the topic you want to discuss in your essay. You should aim to make your introduction paragraph as interesting as possible. Failure to do so may make your paper uninteresting or boring for the reader. And you do not want this if you are aiming for an excellent grade.
In addition, make sure your introduction paragraph provides background information to make the reader understand what will be discussed. You should restrict the background information to 50 words to avoid overloading the reader with unnecessary information in your intro.
After providing background information, you should include your thesis statement or central argument. Your thesis statement is your most important statement. This is because it sets the tone or the theme for the essay. So, you should be very keen when writing it to ensure it is on point. Remember, a good thesis statement is detailed enough to leave room for argumentation.
2. Body Paragraphs
In your first body paragraph, your first sentence should be your strongest argument supporting your thesis statement. Since this is a causal analysis essay, the strongest argument will naturally be the most significant cause or effect of the phenomena described in your introduction paragraph. The first sentence should be followed by evidence or explanation, plus examples where possible. The evidence should be followed by a closing sentence that wraps everything up nicely. Ensure that you follow the basic rules of paragraphing in essay writing .
The second body paragraph should focus on the second strongest argument favoring your thesis statement. As with the first paragraph, the argument should be followed by the evidence/explanation and a closing sentence. The first sentence in the third body paragraph should state the third strongest argument in favor of your thesis statement. The rest of the paragraph should follow the structure of the other body paragraphs.
3. Conclusion
After writing a good introduction with your thesis statement and three body paragraphs, each focusing on a single cause or critical point, you must wrap up the essay with an excellent conclusion . Your conclusion should restate the thesis and the key causes in your causal analysis essay. It should also include a nice closing sentence that wraps the entire essay up.
Causal Analysis Essay Outline
Now that you know the causal analysis essay structure, it is time to discover the outline and how to create one. Knowing how to create one will help you create one and ensure your paper is well-structured and organized.
How to create a causal analysis essay outline
I. Introduction
- Hook statement (Write an interesting fact or statement about the topic)
- Background information (Highlight the background information about the topic that you will include)
- Thesis statement (State the central argument you will be discussing in your essay)
II. Body paragraph 1
- Topic sentence (State the strongest argument (the first cause) in support of your thesis)
- Evidence (Highlight the critical evidence you will use to support the argument above)
- Concluding sentence (Write the sentence you will use to close out this paragraph)
III. Body paragraph 2
- Topic sentence (State the second strongest argument (the second cause) in support of your thesis)
IV. Body paragraph 3
- Topic sentence (State the third strongest argument (the third cause) in support of your thesis)
V. Conclusion
- Restate your thesis
- Summarize your key arguments
- Closing statement (Write the sentence you will use to conclude your essay)
As you can see above, a causal analysis essay outline is similar to the standard outline for short academic papers. To create your own causal analysis essay, follow the instructions above. Just make sure your outline is as comprehensive as possible to make writing the actual easy a walk in the park.
Steps For Writing a Causal Analysis Essay
In this section, you will discover how to write an actual causal analysis essay. Let’s begin.
1. Read the essay prompt carefully
The fact that you are reading this article means you already know you need to write a causal analysis essay. You probably got this information from the essay prompt. If you did, it means you are already on the right track. You now need to read the prompt carefully again.
Read it carefully to understand the essay question or prompt entirely. Also, read it carefully to understand the essay requirements. Failure to follow the requirements in your essay assignment could be costly for you; it could result in an inferior grade.
So, the first step to writing a causal analysis essay is to read the prompt carefully to understand the question and the requirements.
2. Research the essay topic and create a thesis
After reading and understanding the essay topic, the next thing you need to do is to research the essay topic. Research is important as it will help you understand the topic better and decide how you will answer it.
When conducting research, you should note the key points related to the essay topic. The typical causal analysis essay assignment will require you to discuss the causes of a specific phenomenon. Therefore, focus on noting the key causes of the phenomenon you have been asked to write an essay on in the prompt.
Once you have researched to the extent that you fully understand the topic, you should create a thesis statement. The statement should explain what your entire essay will be focusing on. A typical thesis statement for a causal analysis essay sounds like this, “The main causes of global poverty are conflict, climate change, and inequality.”
3. Create an outline
You should create an outline after researching your paper and creating a thesis statement. Simply follow the instructions we have provided in the section above this one to create your causal analysis essay outline. You should find it easy to create an outline for your essay since you have already created a thesis statement and you know the leading causes you will be discussing in your essay.
Make sure your outline is as comprehensive as it can be. When you create a comprehensive outline, you make your work easy. In other words, you make writing the actual essay very easy. When creating an outline, the most important things you should not forget to outline include the opening sentence, the thesis statement, the main supporting arguments, and the closing sentence.
4. Write the essay
When you are done creating an outline for your essay, you should take a short break and then embark on writing the essay following the outline as a guide. With a comprehensive outline, you shouldn’t find writing your causal analysis essay challenging. You should refer to the outline for guidance when you get stuck in any part of your essay.
The best way to write the essay is sequential. Begin with the introduction, then the body paragraphs, and lastly, the conclusion. Write your essay in a simple and easy-to-understand language. And keep in mind that your goal is to make it as smooth flowing as possible.
5. Add in-text citations
Once you are done writing your causal analysis essay, you need to add the in-text citations. Don’t just add them randomly. Add citations to the ideas or points that are not yours. Add in-text citations throughout your essay. This will make your work look credible. You will also get points for proper referencing if you follow the required format or style.
Of course, the only way you can have in-text citations to add to your essay in this step is if you note the source of each note you made during your research. So, indicate the source next to each note you make during research.
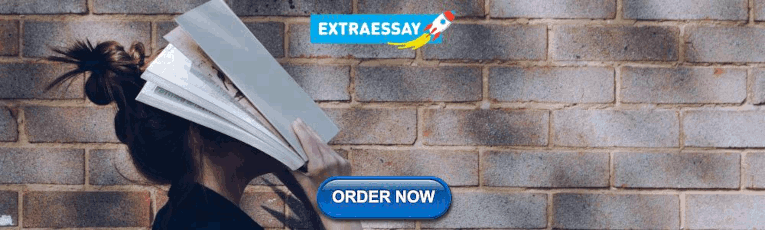
6. Take a break
You should take a break after writing your essay, adding in-text citations and a references page. This is very important at this juncture. Because it allows your brain to rest and forget about the essay, at least momentarily, ensure the break lasts for at least six hours. If you take such a break, you will have a fresh pair of eyes when you look at your essay in the next step.
7. Edit your essay
After taking a break, you should edit your essay. Since you took a break in the previous step, you will have a fresh pair of eyes that should make it easy to catch mistakes. Read your essay aloud to make sure you catch all the issues, errors, and mistakes. Read it slowly to make sure you do not miss anything.
After editing your essay , give it to someone to read it and identify any mistakes you might have missed. Then check the identified mistakes if they are actual mistakes and edit them out of your essay. When you complete this step, your causal analysis essay will be ready for submission.
Tips For Writing an Excellent Causal Analysis Essay
If you want to write a good causal analysis essay, follow the above steps. If you want to write an excellent causal analysis essay, incorporate the tips below when following the steps above.
- Ensure your essay is straightforward to read and understand to give your professor an easy time grading it. This will increase your chances of getting an excellent grade.
- Ensure you include a strong thesis statement at the end of your introduction paragraph. Without a strong thesis statement, your essay will be challenging to follow.
- Ensure there is no vague phrase or statement in your essay. This will make your essay stronger and more credible. It will also ensure you don’t lose marks for clarity.
- Use examples generously in your essay. This will make it easier to understand. It will also make it more authentic and useful.
- Do not belabor points in your essay. Simply explain your key points clearly and concisely. Do not go round and round in circles saying the same thing in different words.
- Support any specific idea or point you include with evidence. You are just a high school or undergraduate student; nobody will take your word for all the key claims and arguments you make. So back everything important you say with evidence from credible sources.
- Do not forget to proofread your essay thoroughly. Doing this is the only way you will convert your ordinary causal analysis essay into something extraordinary.
Example Of a Causal Analysis Essay
A typical causal analysis essay will describe the causes of a problem or a phenomenon. It is a cause-and-effect essay. This section will provide an example of a causal analysis essay. We hope this short causal analysis essay example will make it easy for you to write your own causal analysis essay.
Why do teenagers use drugs, and the negative effects of using drugs? Drug use is prevalent nowadays among teenagers, especially in urban areas across the country. Most teenagers who use drugs use it because of peer pressure or as a reaction to bullying and other sorts of trauma. Drugs use among young people often results in various negative effects, including poor well-being, negative self-image, and addiction. Teenager drug use often leads to poor well-being. Various studies have shown that teenagers who use drugs often suffer from poor health and well-being. This is because the drugs they use without a prescription are dangerous and often produce unpleasant symptoms. The only way drug-using teenagers can reverse this trend is if they say no to drugs. Teenagers who use drugs often end up having a negative self-image. The negative self-image is often brought out by the secrecy surrounding drug use and the negativity associated with drug use. The negative self-image can sometimes lead to depression or even attempted suicide. The best thing about this effect of drug use is that it can be reversed through therapy or an intervention. Teenagers who use drugs usually end up getting addicted. Drug addiction is a terrible condition that forces those with it to repeatedly seek the “high” the drugs offer. This can lead to dependence and a terrible addiction. It can also lead to the addict stealing to get money for the drugs. Despite the negative effects of addiction, it can also be eliminated. In conclusion, Drugs use among youth can lead to negative effects such as addiction, poor self-image, and poor well-being. These negative effects show that drug use can be hazardous for young people and that efforts should be made to put an end to it. Without robust efforts to put an end to drug use among youth, likely, a section of the youth will forever be lost to drugs.
Causal Analysis Topics
Choosing a good causal analysis essay topic will help ensure your essay is exciting and fun to read. Check out our causal analysis topics below to get inspiration to create your fun causal analysis essay topic.
- What are the effects of too much internet on the personalities of children?
- Why is cyberbullying such a big issue in the current world?
- What has been the positive impact of technology in the healthcare industry?
- What is the impact of technology on teaching methods?
- What are the negative effects of misinformation on the internet?
- What causes the increasing number of mass shooting incidents in the country?
- What caused the emergence of the feminist movement?
- Why is there gender bias in American politics?
- What has led to the calls for stricter gun laws in the United States?
- What led to the most recent US government shutdown?
- Why did the coronavirus pandemic have a huge negative impact on the world economy?
- What are the causes of the age-old Palestinian conflict?
- What led to the separation of the KOREA peninsula?
- Why are cryptocurrencies such as Bitcoin rapidly gaining popularity?
- What has led to the recent increase in cases of cyberbullying?
- What is the cause of global warming and its impact on the world?
- What are the negative effects of water pollution?
- The biggest causes of environmental pollution
- What caused the Iranian Revolution?
- What caused the French Revolution?
- What were the biggest causes of the First World War?
- Why was Mussolini very successful in spreading fascism in Italy?
- Explain why homeschooling is gaining popularity across the world.
- What made China halt its one-child policy?
- Why do so many people still oppose the Obamacare Act?
Parting Shot!
The information presented in this article is sufficient for any college student to write an excellent causal analysis essay. So, if you have time, all you need to do is to follow the structure, the steps, and the tips presented in this article to develop the perfect causal analysis essay.
Related Reading:
- How to write a good case study
- Tips and steps for writing an excellent analytical essay
- How to know that an article is peer-reviewed.
If you do not have the time to write the essay or are not confident enough in your writing skills, you should order one from us. Hire someone to write your essay from EssayManiacs, and rest assured, you will nail the paper. We are an assignment-help business with dozens of experienced tutors and writers who can handle almost any academic assignment.
If you order your causal analysis essay from us, you will get a well-researched, well-developed, and 100% original essay with zero grammar errors. Start working with us today for excellent essays, research papers, and other academic assignments.
Need a Discount to Order?
15% off first order, what you get from us.
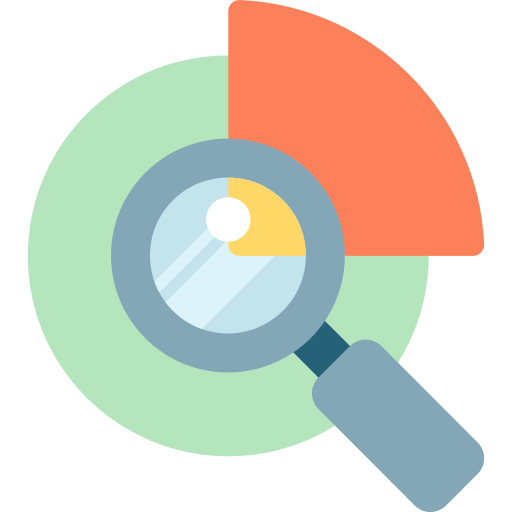
Plagiarism-free papers
Our papers are 100% original and unique to pass online plagiarism checkers.
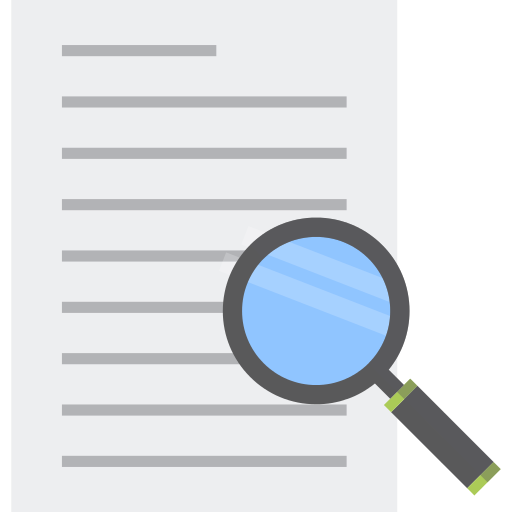
Well-researched academic papers
Even when we say essays for sale, they meet academic writing conventions.
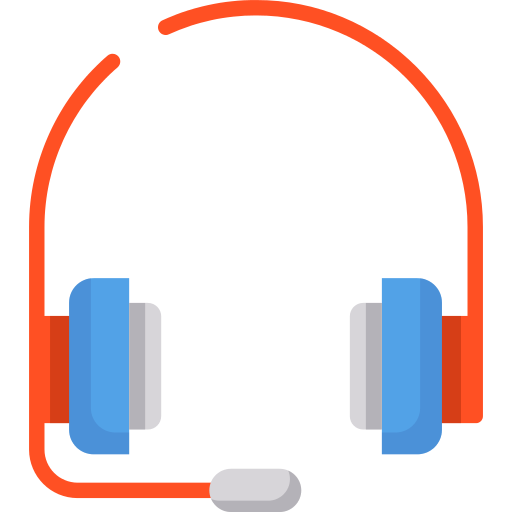
24/7 online support
Hit us up on live chat or Messenger for continuous help with your essays.
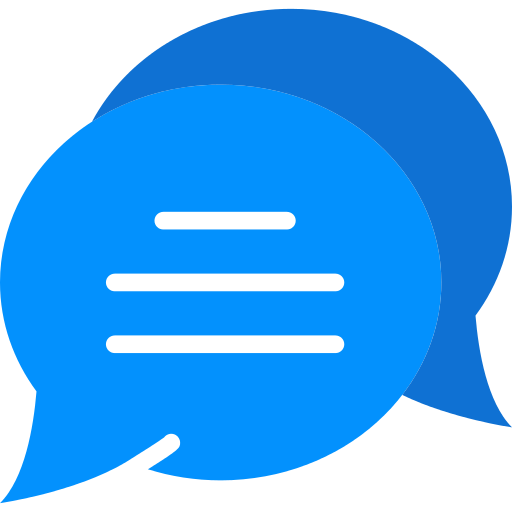
Easy communication with writers
Order essays and begin communicating with your writer directly and anonymously.
- Search Menu
- Browse content in Arts and Humanities
- Browse content in Archaeology
- Anglo-Saxon and Medieval Archaeology
- Archaeological Methodology and Techniques
- Archaeology by Region
- Archaeology of Religion
- Archaeology of Trade and Exchange
- Biblical Archaeology
- Contemporary and Public Archaeology
- Environmental Archaeology
- Historical Archaeology
- History and Theory of Archaeology
- Industrial Archaeology
- Landscape Archaeology
- Mortuary Archaeology
- Prehistoric Archaeology
- Underwater Archaeology
- Urban Archaeology
- Zooarchaeology
- Browse content in Architecture
- Architectural Structure and Design
- History of Architecture
- Residential and Domestic Buildings
- Theory of Architecture
- Browse content in Art
- Art Subjects and Themes
- History of Art
- Industrial and Commercial Art
- Theory of Art
- Biographical Studies
- Byzantine Studies
- Browse content in Classical Studies
- Classical History
- Classical Philosophy
- Classical Mythology
- Classical Literature
- Classical Reception
- Classical Art and Architecture
- Classical Oratory and Rhetoric
- Greek and Roman Epigraphy
- Greek and Roman Law
- Greek and Roman Papyrology
- Greek and Roman Archaeology
- Late Antiquity
- Religion in the Ancient World
- Digital Humanities
- Browse content in History
- Colonialism and Imperialism
- Diplomatic History
- Environmental History
- Genealogy, Heraldry, Names, and Honours
- Genocide and Ethnic Cleansing
- Historical Geography
- History by Period
- History of Emotions
- History of Agriculture
- History of Education
- History of Gender and Sexuality
- Industrial History
- Intellectual History
- International History
- Labour History
- Legal and Constitutional History
- Local and Family History
- Maritime History
- Military History
- National Liberation and Post-Colonialism
- Oral History
- Political History
- Public History
- Regional and National History
- Revolutions and Rebellions
- Slavery and Abolition of Slavery
- Social and Cultural History
- Theory, Methods, and Historiography
- Urban History
- World History
- Browse content in Language Teaching and Learning
- Language Learning (Specific Skills)
- Language Teaching Theory and Methods
- Browse content in Linguistics
- Applied Linguistics
- Cognitive Linguistics
- Computational Linguistics
- Forensic Linguistics
- Grammar, Syntax and Morphology
- Historical and Diachronic Linguistics
- History of English
- Language Acquisition
- Language Evolution
- Language Reference
- Language Variation
- Language Families
- Lexicography
- Linguistic Anthropology
- Linguistic Theories
- Linguistic Typology
- Phonetics and Phonology
- Psycholinguistics
- Sociolinguistics
- Translation and Interpretation
- Writing Systems
- Browse content in Literature
- Bibliography
- Children's Literature Studies
- Literary Studies (Asian)
- Literary Studies (European)
- Literary Studies (Eco-criticism)
- Literary Studies (Romanticism)
- Literary Studies (American)
- Literary Studies (Modernism)
- Literary Studies - World
- Literary Studies (1500 to 1800)
- Literary Studies (19th Century)
- Literary Studies (20th Century onwards)
- Literary Studies (African American Literature)
- Literary Studies (British and Irish)
- Literary Studies (Early and Medieval)
- Literary Studies (Fiction, Novelists, and Prose Writers)
- Literary Studies (Gender Studies)
- Literary Studies (Graphic Novels)
- Literary Studies (History of the Book)
- Literary Studies (Plays and Playwrights)
- Literary Studies (Poetry and Poets)
- Literary Studies (Postcolonial Literature)
- Literary Studies (Queer Studies)
- Literary Studies (Science Fiction)
- Literary Studies (Travel Literature)
- Literary Studies (War Literature)
- Literary Studies (Women's Writing)
- Literary Theory and Cultural Studies
- Mythology and Folklore
- Shakespeare Studies and Criticism
- Browse content in Media Studies
- Browse content in Music
- Applied Music
- Dance and Music
- Ethics in Music
- Ethnomusicology
- Gender and Sexuality in Music
- Medicine and Music
- Music Cultures
- Music and Religion
- Music and Media
- Music and Culture
- Music Education and Pedagogy
- Music Theory and Analysis
- Musical Scores, Lyrics, and Libretti
- Musical Structures, Styles, and Techniques
- Musicology and Music History
- Performance Practice and Studies
- Race and Ethnicity in Music
- Sound Studies
- Browse content in Performing Arts
- Browse content in Philosophy
- Aesthetics and Philosophy of Art
- Epistemology
- Feminist Philosophy
- History of Western Philosophy
- Metaphysics
- Moral Philosophy
- Non-Western Philosophy
- Philosophy of Science
- Philosophy of Language
- Philosophy of Mind
- Philosophy of Perception
- Philosophy of Action
- Philosophy of Law
- Philosophy of Religion
- Philosophy of Mathematics and Logic
- Practical Ethics
- Social and Political Philosophy
- Browse content in Religion
- Biblical Studies
- Christianity
- East Asian Religions
- History of Religion
- Judaism and Jewish Studies
- Qumran Studies
- Religion and Education
- Religion and Health
- Religion and Politics
- Religion and Science
- Religion and Law
- Religion and Art, Literature, and Music
- Religious Studies
- Browse content in Society and Culture
- Cookery, Food, and Drink
- Cultural Studies
- Customs and Traditions
- Ethical Issues and Debates
- Hobbies, Games, Arts and Crafts
- Lifestyle, Home, and Garden
- Natural world, Country Life, and Pets
- Popular Beliefs and Controversial Knowledge
- Sports and Outdoor Recreation
- Technology and Society
- Travel and Holiday
- Visual Culture
- Browse content in Law
- Arbitration
- Browse content in Company and Commercial Law
- Commercial Law
- Company Law
- Browse content in Comparative Law
- Systems of Law
- Competition Law
- Browse content in Constitutional and Administrative Law
- Government Powers
- Judicial Review
- Local Government Law
- Military and Defence Law
- Parliamentary and Legislative Practice
- Construction Law
- Contract Law
- Browse content in Criminal Law
- Criminal Procedure
- Criminal Evidence Law
- Sentencing and Punishment
- Employment and Labour Law
- Environment and Energy Law
- Browse content in Financial Law
- Banking Law
- Insolvency Law
- History of Law
- Human Rights and Immigration
- Intellectual Property Law
- Browse content in International Law
- Private International Law and Conflict of Laws
- Public International Law
- IT and Communications Law
- Jurisprudence and Philosophy of Law
- Law and Politics
- Law and Society
- Browse content in Legal System and Practice
- Courts and Procedure
- Legal Skills and Practice
- Primary Sources of Law
- Regulation of Legal Profession
- Medical and Healthcare Law
- Browse content in Policing
- Criminal Investigation and Detection
- Police and Security Services
- Police Procedure and Law
- Police Regional Planning
- Browse content in Property Law
- Personal Property Law
- Study and Revision
- Terrorism and National Security Law
- Browse content in Trusts Law
- Wills and Probate or Succession
- Browse content in Medicine and Health
- Browse content in Allied Health Professions
- Arts Therapies
- Clinical Science
- Dietetics and Nutrition
- Occupational Therapy
- Operating Department Practice
- Physiotherapy
- Radiography
- Speech and Language Therapy
- Browse content in Anaesthetics
- General Anaesthesia
- Neuroanaesthesia
- Browse content in Clinical Medicine
- Acute Medicine
- Cardiovascular Medicine
- Clinical Genetics
- Clinical Pharmacology and Therapeutics
- Dermatology
- Endocrinology and Diabetes
- Gastroenterology
- Genito-urinary Medicine
- Geriatric Medicine
- Infectious Diseases
- Medical Toxicology
- Medical Oncology
- Pain Medicine
- Palliative Medicine
- Rehabilitation Medicine
- Respiratory Medicine and Pulmonology
- Rheumatology
- Sleep Medicine
- Sports and Exercise Medicine
- Clinical Neuroscience
- Community Medical Services
- Critical Care
- Emergency Medicine
- Forensic Medicine
- Haematology
- History of Medicine
- Browse content in Medical Dentistry
- Oral and Maxillofacial Surgery
- Paediatric Dentistry
- Restorative Dentistry and Orthodontics
- Surgical Dentistry
- Browse content in Medical Skills
- Clinical Skills
- Communication Skills
- Nursing Skills
- Surgical Skills
- Medical Ethics
- Medical Statistics and Methodology
- Browse content in Neurology
- Clinical Neurophysiology
- Neuropathology
- Nursing Studies
- Browse content in Obstetrics and Gynaecology
- Gynaecology
- Occupational Medicine
- Ophthalmology
- Otolaryngology (ENT)
- Browse content in Paediatrics
- Neonatology
- Browse content in Pathology
- Chemical Pathology
- Clinical Cytogenetics and Molecular Genetics
- Histopathology
- Medical Microbiology and Virology
- Patient Education and Information
- Browse content in Pharmacology
- Psychopharmacology
- Browse content in Popular Health
- Caring for Others
- Complementary and Alternative Medicine
- Self-help and Personal Development
- Browse content in Preclinical Medicine
- Cell Biology
- Molecular Biology and Genetics
- Reproduction, Growth and Development
- Primary Care
- Professional Development in Medicine
- Browse content in Psychiatry
- Addiction Medicine
- Child and Adolescent Psychiatry
- Forensic Psychiatry
- Learning Disabilities
- Old Age Psychiatry
- Psychotherapy
- Browse content in Public Health and Epidemiology
- Epidemiology
- Public Health
- Browse content in Radiology
- Clinical Radiology
- Interventional Radiology
- Nuclear Medicine
- Radiation Oncology
- Reproductive Medicine
- Browse content in Surgery
- Cardiothoracic Surgery
- Gastro-intestinal and Colorectal Surgery
- General Surgery
- Neurosurgery
- Paediatric Surgery
- Peri-operative Care
- Plastic and Reconstructive Surgery
- Surgical Oncology
- Transplant Surgery
- Trauma and Orthopaedic Surgery
- Vascular Surgery
- Browse content in Science and Mathematics
- Browse content in Biological Sciences
- Aquatic Biology
- Biochemistry
- Bioinformatics and Computational Biology
- Developmental Biology
- Ecology and Conservation
- Evolutionary Biology
- Genetics and Genomics
- Microbiology
- Molecular and Cell Biology
- Natural History
- Plant Sciences and Forestry
- Research Methods in Life Sciences
- Structural Biology
- Systems Biology
- Zoology and Animal Sciences
- Browse content in Chemistry
- Analytical Chemistry
- Computational Chemistry
- Crystallography
- Environmental Chemistry
- Industrial Chemistry
- Inorganic Chemistry
- Materials Chemistry
- Medicinal Chemistry
- Mineralogy and Gems
- Organic Chemistry
- Physical Chemistry
- Polymer Chemistry
- Study and Communication Skills in Chemistry
- Theoretical Chemistry
- Browse content in Computer Science
- Artificial Intelligence
- Computer Architecture and Logic Design
- Game Studies
- Human-Computer Interaction
- Mathematical Theory of Computation
- Programming Languages
- Software Engineering
- Systems Analysis and Design
- Virtual Reality
- Browse content in Computing
- Business Applications
- Computer Security
- Computer Games
- Computer Networking and Communications
- Digital Lifestyle
- Graphical and Digital Media Applications
- Operating Systems
- Browse content in Earth Sciences and Geography
- Atmospheric Sciences
- Environmental Geography
- Geology and the Lithosphere
- Maps and Map-making
- Meteorology and Climatology
- Oceanography and Hydrology
- Palaeontology
- Physical Geography and Topography
- Regional Geography
- Soil Science
- Urban Geography
- Browse content in Engineering and Technology
- Agriculture and Farming
- Biological Engineering
- Civil Engineering, Surveying, and Building
- Electronics and Communications Engineering
- Energy Technology
- Engineering (General)
- Environmental Science, Engineering, and Technology
- History of Engineering and Technology
- Mechanical Engineering and Materials
- Technology of Industrial Chemistry
- Transport Technology and Trades
- Browse content in Environmental Science
- Applied Ecology (Environmental Science)
- Conservation of the Environment (Environmental Science)
- Environmental Sustainability
- Environmentalist Thought and Ideology (Environmental Science)
- Management of Land and Natural Resources (Environmental Science)
- Natural Disasters (Environmental Science)
- Nuclear Issues (Environmental Science)
- Pollution and Threats to the Environment (Environmental Science)
- Social Impact of Environmental Issues (Environmental Science)
- History of Science and Technology
- Browse content in Materials Science
- Ceramics and Glasses
- Composite Materials
- Metals, Alloying, and Corrosion
- Nanotechnology
- Browse content in Mathematics
- Applied Mathematics
- Biomathematics and Statistics
- History of Mathematics
- Mathematical Education
- Mathematical Finance
- Mathematical Analysis
- Numerical and Computational Mathematics
- Probability and Statistics
- Pure Mathematics
- Browse content in Neuroscience
- Cognition and Behavioural Neuroscience
- Development of the Nervous System
- Disorders of the Nervous System
- History of Neuroscience
- Invertebrate Neurobiology
- Molecular and Cellular Systems
- Neuroendocrinology and Autonomic Nervous System
- Neuroscientific Techniques
- Sensory and Motor Systems
- Browse content in Physics
- Astronomy and Astrophysics
- Atomic, Molecular, and Optical Physics
- Biological and Medical Physics
- Classical Mechanics
- Computational Physics
- Condensed Matter Physics
- Electromagnetism, Optics, and Acoustics
- History of Physics
- Mathematical and Statistical Physics
- Measurement Science
- Nuclear Physics
- Particles and Fields
- Plasma Physics
- Quantum Physics
- Relativity and Gravitation
- Semiconductor and Mesoscopic Physics
- Browse content in Psychology
- Affective Sciences
- Clinical Psychology
- Cognitive Psychology
- Cognitive Neuroscience
- Criminal and Forensic Psychology
- Developmental Psychology
- Educational Psychology
- Evolutionary Psychology
- Health Psychology
- History and Systems in Psychology
- Music Psychology
- Neuropsychology
- Organizational Psychology
- Psychological Assessment and Testing
- Psychology of Human-Technology Interaction
- Psychology Professional Development and Training
- Research Methods in Psychology
- Social Psychology
- Browse content in Social Sciences
- Browse content in Anthropology
- Anthropology of Religion
- Human Evolution
- Medical Anthropology
- Physical Anthropology
- Regional Anthropology
- Social and Cultural Anthropology
- Theory and Practice of Anthropology
- Browse content in Business and Management
- Business Strategy
- Business Ethics
- Business History
- Business and Government
- Business and Technology
- Business and the Environment
- Comparative Management
- Corporate Governance
- Corporate Social Responsibility
- Entrepreneurship
- Health Management
- Human Resource Management
- Industrial and Employment Relations
- Industry Studies
- Information and Communication Technologies
- International Business
- Knowledge Management
- Management and Management Techniques
- Operations Management
- Organizational Theory and Behaviour
- Pensions and Pension Management
- Public and Nonprofit Management
- Strategic Management
- Supply Chain Management
- Browse content in Criminology and Criminal Justice
- Criminal Justice
- Criminology
- Forms of Crime
- International and Comparative Criminology
- Youth Violence and Juvenile Justice
- Development Studies
- Browse content in Economics
- Agricultural, Environmental, and Natural Resource Economics
- Asian Economics
- Behavioural Finance
- Behavioural Economics and Neuroeconomics
- Econometrics and Mathematical Economics
- Economic Systems
- Economic History
- Economic Methodology
- Economic Development and Growth
- Financial Markets
- Financial Institutions and Services
- General Economics and Teaching
- Health, Education, and Welfare
- History of Economic Thought
- International Economics
- Labour and Demographic Economics
- Law and Economics
- Macroeconomics and Monetary Economics
- Microeconomics
- Public Economics
- Urban, Rural, and Regional Economics
- Welfare Economics
- Browse content in Education
- Adult Education and Continuous Learning
- Care and Counselling of Students
- Early Childhood and Elementary Education
- Educational Equipment and Technology
- Educational Strategies and Policy
- Higher and Further Education
- Organization and Management of Education
- Philosophy and Theory of Education
- Schools Studies
- Secondary Education
- Teaching of a Specific Subject
- Teaching of Specific Groups and Special Educational Needs
- Teaching Skills and Techniques
- Browse content in Environment
- Applied Ecology (Social Science)
- Climate Change
- Conservation of the Environment (Social Science)
- Environmentalist Thought and Ideology (Social Science)
- Natural Disasters (Environment)
- Social Impact of Environmental Issues (Social Science)
- Browse content in Human Geography
- Cultural Geography
- Economic Geography
- Political Geography
- Browse content in Interdisciplinary Studies
- Communication Studies
- Museums, Libraries, and Information Sciences
- Browse content in Politics
- African Politics
- Asian Politics
- Chinese Politics
- Comparative Politics
- Conflict Politics
- Elections and Electoral Studies
- Environmental Politics
- European Union
- Foreign Policy
- Gender and Politics
- Human Rights and Politics
- Indian Politics
- International Relations
- International Organization (Politics)
- International Political Economy
- Irish Politics
- Latin American Politics
- Middle Eastern Politics
- Political Methodology
- Political Communication
- Political Philosophy
- Political Sociology
- Political Behaviour
- Political Economy
- Political Institutions
- Political Theory
- Politics and Law
- Public Administration
- Public Policy
- Quantitative Political Methodology
- Regional Political Studies
- Russian Politics
- Security Studies
- State and Local Government
- UK Politics
- US Politics
- Browse content in Regional and Area Studies
- African Studies
- Asian Studies
- East Asian Studies
- Japanese Studies
- Latin American Studies
- Middle Eastern Studies
- Native American Studies
- Scottish Studies
- Browse content in Research and Information
- Research Methods
- Browse content in Social Work
- Addictions and Substance Misuse
- Adoption and Fostering
- Care of the Elderly
- Child and Adolescent Social Work
- Couple and Family Social Work
- Developmental and Physical Disabilities Social Work
- Direct Practice and Clinical Social Work
- Emergency Services
- Human Behaviour and the Social Environment
- International and Global Issues in Social Work
- Mental and Behavioural Health
- Social Justice and Human Rights
- Social Policy and Advocacy
- Social Work and Crime and Justice
- Social Work Macro Practice
- Social Work Practice Settings
- Social Work Research and Evidence-based Practice
- Welfare and Benefit Systems
- Browse content in Sociology
- Childhood Studies
- Community Development
- Comparative and Historical Sociology
- Economic Sociology
- Gender and Sexuality
- Gerontology and Ageing
- Health, Illness, and Medicine
- Marriage and the Family
- Migration Studies
- Occupations, Professions, and Work
- Organizations
- Population and Demography
- Race and Ethnicity
- Social Theory
- Social Movements and Social Change
- Social Research and Statistics
- Social Stratification, Inequality, and Mobility
- Sociology of Religion
- Sociology of Education
- Sport and Leisure
- Urban and Rural Studies
- Browse content in Warfare and Defence
- Defence Strategy, Planning, and Research
- Land Forces and Warfare
- Military Administration
- Military Life and Institutions
- Naval Forces and Warfare
- Other Warfare and Defence Issues
- Peace Studies and Conflict Resolution
- Weapons and Equipment
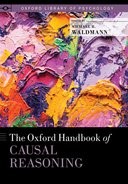
- < Previous chapter
- Next chapter >
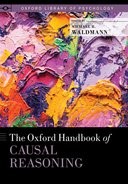
22 Causal Explanation
Department of Psychology University of California, Berkeley Berkeley, California, USA
- Published: 10 May 2017
- Cite Icon Cite
- Permissions Icon Permissions
Explanation and causation are intimately related. Explanations often appeal to causes, and causal claims are often answers to implicit or explicit questions about why or how something occurred. This chapter considers what we can learn about causal reasoning from research on explanation. In particular, it reviews an emerging body of work suggesting that explanatory considerations—such as the simplicity or scope of a causal hypothesis—can systematically influence causal inference and learning. It also discusses proposed distinctions among types of explanations and reviews the effects of each explanation type on causal reasoning and representation. Finally, it considers the relationship between explanations and causal mechanisms and raises important questions for future research.
A doctor encounters a patient: Why does she have a fever and a rash? An engineer investigates a failure: Why did the bridge collapse? A parent wonders about her child: Why did she throw a tantrum? In each of these cases, we seek an explanation for some event—an explanation that’s likely to appeal to one or more antecedent causes . The doctor might conclude that a virus caused the symptoms, the engineer that defects in cast iron caused the collapse, and the parent that the toy’s disappearance caused the tantrum.
Not all explanations are causal, and not all causes are explanatory. Explanations in mathematics, for example, are typically taken to be non-causal, and many causal factors are either not explanatory at all, or only explanatory under particular circumstances. (Consider, for instance, appealing to the big bang as an explanation for today’s inflation rates, or the presence of oxygen as an explanation for California wildfires.) Nonetheless, causation and explanation are closely related, with many instances of causal reasoning featuring explanations and explanatory considerations, and many instances of abductive inference and explanation appealing to causes and causal considerations. The goal of the present chapter is to identify some of the connections between explanation and causation, with a special focus on how the study of explanation can inform our understanding of causal reasoning.
The chapter is divided into five sections. In the first three, we review an emerging body of work on the role of explanation in three types of causal reasoning: drawing inferences about the causes of events, learning novel causal structures, and assigning causal responsibility. In the fourth section, we consider different kinds of explanations, including a discussion of whether each kind is properly “causal” and how different kinds of explanations can differentially influence causal judgments. In the fifth section, we focus on causal explanations that appeal to mechanisms, and consider the relationship between explanation, causal claims, and mechanisms. Finally, we conclude with some important questions for future research.
Causal Inference and Inference to the Best Explanation
Consider a doctor who infers, on the basis of a patient’s symptoms, that the patient has a particular disease—one known to cause that cluster of symptoms. We will refer to such instances of causal reasoning as “causal inference,” and differentiate them from two other kinds of causal reasoning that we will discuss in subsequent sections: causal learning (which involves learning about novel causes and relationships at the type level) and assigning causal responsibility (which involves attributing an effect to one or more causes, all of which could have occurred and could have contributed to the effect).
How might explanation influence causal inference? One possibility is that people engage in a process called “inference to the best explanation” (IBE). IBE was introduced into the philosophical literature by Gilbert Harman in a 1965 paper, but the idea is likely older, and closely related to what is sometimes called “abductive inference” ( Douven, 2011 ; Lombrozo, 2012 , 2016 ; Peirce, 1955 ). The basic idea is that one infers that a hypothesis is likely to be true based on the fact that it best explains the data. To borrow vocabulary from another influential philosopher of explanation, Peter Lipton, one uses an explanation’s “loveliness” as a guide to its “likeliness” ( Lipton, 2004 ).
A great deal of work has aimed to characterize how people go about inferring causes from patterns of evidence ( Cheng, 1997 ; Cheng & Novick, 1990 , 1992 ; Glymour & Cheng, 1998 ; Griffiths & Tenenbaum, 2005 ; Kelley, 1973 ; Perales & Shanks, 2003 ; Shanks & Dickinson, 1988 ; Waldmann & Hagmayer, 2001 ; see Buehner, 2005 ; Holyoak & Cheng, 2011 ; Waldmann & Hagmayer, 2013 , for reviews), and this work is summarized in other chapters of this volume (see Part I , “Theories of Causal Cognition,” and Meder & Mayrhofer, Chapter 23 , on diagnostic reasoning). Thus a question that immediately presents itself is whether IBE is distinct from the kinds of inference these models typically involve, such as analyses of covariation or Bayesian inference. For most advocates of IBE, the answer is “yes”: IBE is a distinct inferential process, where the key commitment is that explanatory considerations play a role in guiding judgments. These considerations can include the simplicity, scope, or other “virtues” of the explanatory hypotheses under consideration.
To provide evidence for IBE as a distinctly explanatory form of inference, it is thus important to identify explanatory virtues, and to demonstrate their role in inference. The most direct evidence of this form comes from research on simplicity ( Bonawitz & Lombrozo, 2012 ; Lombrozo, 2007 ; Pacer & Lombrozo, in preparation), scope ( Khemlani, Sussman, & Oppenheimer, 2011 ), and explanatory power (Douven & Schupbach, 2015a , 2015b ). We focus on this research for the remainder of the section.
In one study from Lombrozo (2007) , participants learned novel causal structures describing the relationships between diseases and symptoms on an alien planet. For example, the conjunction of two particular symptoms—say “sore minttels” and “purple spots”—could be explained by appeal to a single disease that caused both symptoms (Tritchet’s syndrome), or by appeal to the conjunction of two diseases that each caused one symptom (Morad’s disease and a Humel infection). Lombrozo set out to test whether participants would favor the explanation that was simpler in the sense that it invoked a single common cause over two independent causes, and whether they would do so even when probabilistic evidence, in the form of disease base rates, favored the more complex explanation. Lombrozo found that participants’ explanation choices were a function of both simplicity and probability, with a substantial proportion of participants selecting the simpler explanation even when it was less likely than the complex alternative. This is consistent with the idea that an explanation’s “loveliness”—in this case, its simplicity—is used as a basis for inferring its “likeliness.”
In subsequent work, Bonawitz and Lombrozo (2012) replicated the same basic pattern of results with 5-year-old children in a structurally parallel task: children observed a toy generating two effects (a light and a spinning fan), and had to infer whether one block (which generated both effects) or two blocks (which each generated one effect) fell into the toy’s activator bin. In this case, probabilistic information was manipulated across participants by varying the number of blocks of each type and the process by which they fell into the bin. Interestingly, adults did not show a preference for simplicity above and beyond probability in this task, while the 5-year-olds did. Bonawitz and Lombrozo suggest that in the face of probabilistic uncertainty—of the kind that is generated by a more complex task like the alien diagnosis problems used in Lombrozo (2007) —adults rely on explanatory considerations such as simplicity to guide assessments of probability. But when a task involves a transparent and seemingly deterministic causal system, and when the numbers involved are small (as was the case for the task developed for young children in Bonawitz and Lombrozo, 2012 ), adults may engage in more explicit probabilistic reasoning, and may bypass explanatory considerations altogether. Consistent with this idea, adults in Lombrozo (2007) also ceased to favor simplicity when they were explicitly told that the complex hypothesis was most likely to be true.
In more recent work, Pacer and Lombrozo (in preparation) provide a more precise characterization of how people assess an explanation’s simplicity. They differentiate two intuitive metrics for causal explanations, both of which are consistent with prior results: “node simplicity,” which involves counting the number of causes invoked in an explanation; and “root simplicity,” which involves counting the number of unexplained causes invoked in an explanation. For example, suppose that Dr. Node explains a patient’s symptoms by appeal to pneumonia and sarcoma—two diseases. And that Dr. Root explains the symptoms by appeal to pneumonia, sarcoma, and HIV , where HIV is a cause (or at least a contributing factor) for both pneumonia and sarcoma. Dr. Root has invoked more causes than Dr. Node (three versus two), and so her explanation is less simple according to node simplicity. But Dr. Root has explained the symptoms by appeal to only one unexplained cause (HIV), as opposed to Dr. Node’s two (pneumonia and sarcoma), so her explanation is simpler according to root simplicity. Extending the basic method developed by Lombrozo (2007) , Pacer and Lombrozo found strong evidence that people favor explanations with low root simplicity (above and beyond what is warranted on the basis of the frequency information which they were provided), but no evidence that people are sensitive to node simplicity. By using appropriate causal structures, they were able to rule out alternative explanations for these results (e.g., that people prefer explanations that involve intervening variables).
These findings suggest that in drawing causal inferences, people do not simply engage in probabilistic inference on the basis of frequency information. In addition to frequency information, they use explanatory considerations (in this case, low root simplicity) to guide their judgments, at least in the face of probabilistic uncertainty. The findings therefore suggest that IBE plays a role in inferences concerning causal events. But is this effect restricted to simplicity, or do other explanatory considerations play a role as well? Research to date supports a role for two additional factors: narrow latent scope and explanatory power .
An explanation’s “latent scope” refers to the number of unverified effects that the explanation predicts. For example, an observed symptom could be explained by appeal to a disease that predicts that single symptom, or by appeal to a disease that additionally predicts an effect that has not yet been tested for and is hence unobserved (e.g., whether the person has low blood levels of some mineral). In this case, the former explanation has narrower latent scope. Khemlani, Sussman, and Oppenheimer (2011) found that people favor explanations with narrow latent scope, even if the two diseases are equally prevalent. Importantly, they also find that latent scope affects probability estimates: explanations with narrow latent scope are judged more likely than those with broader latent scope (see also Johnson, Johnston, Toig, & Keil, 2014 , for evidence that explanatory scope informs causal strength inferences, and Johnston, Johnson, Koven, & Keil, 2015 , for evidence of latent scope bias in children). Thus latent scope appears to be among the cues to explanatory “loveliness” that affects the perceived “likeliness” of explanatory hypotheses.
Finally, recent work by Douven and Schupbach ( 2015a , 2015b ) provides further evidence of a role for explanatory considerations in inference, with hints that the relevant consideration is “explanatory power.” Employing a quite different paradigm, Douven and Schupbach demonstrate that people’s explanatory judgments better predict their estimates of posterior probability than do objective probabilities on their own. In a study reported in Douven and Schupbach (2015a) , participants observed 10 balls successively drawn from one of two urns, which was selected by a coin flip. One urn contained 30 black balls and 10 white balls, and the other contained 15 black balls and 25 white ones. After each draw, participants were asked to consider the evidence so far, and to rate the “explanatory goodness” of each of two hypotheses: the hypothesis that the balls were drawn from the 30/10 urn, or the hypothesis that the balls were drawn from the 15/25 urn. Participants were also asked to estimate a posterior probability for each hypothesis after each draw. In a series of models, Douven and Schupbach tested whether people’s judgments of the explanatory “goodness” of each hypothesis improved model predictions of their subjective posterior probabilities, above and beyond the objective posteriors calculated on the basis of the data presented to each participant. They found that models incorporating these explanatory judgments outperformed alternatives, even when appropriately penalized for using additional predictors.
Douven and Schupbach’s (2015a) results suggest that explanatory considerations do inform assessments of probability, and that these considerations diverge from posterior probability. However, the findings do not pinpoint the nature of the explanatory considerations themselves. On what basis were participants judging one hypothesis more or less explanatory than the other? Additional analyses of these data, reported in Douven and Schupbach (2015b) , provide some hints: models that took into account some measure of “explanatory power”—computed on the basis of the objective probabilities—outperformed the basic model that only considered posteriors. The best-performing model employed a measure based on Good (1960) that roughly tracks confirmation : it takes the log of the ratio of the probability of the data given the hypothesis to the probability of the data. In other work, Schupbach (2011) finds evidence that people’s judgments of an explanation’s “goodness” are related to another measure of explanatory power, proposed by Schupbach and Sprenger (2011) , which is also related to Bayesian measures of confirmation.
These findings suggest that explanatory considerations—in the form of root simplicity, latent scope, and explanatory power—inform causal inference, and in so doing reveal something potentially surprising: that while people’s responses to evidence are systematic, they do not (always) lead to causal inferences that track the posterior probabilities of each causal hypothesis. This not only supports a role for explanatory considerations in causal inference, but also challenges the idea that identifying causes to explain effects is essentially a matter of conditionalizing on the effects to infer the most likely cause. Further challenging this idea, Pacer, Williams, Chen, Lombrozo, and Griffiths (2013) compare judgments of explanatory goodness from human participants to those generated by four distinct computational models of explanation in causal Bayesian networks, and find that models that compute measures of evidence or information considerably outperform those that compute more direct measures of (posterior) probability.
In sum, there is good evidence that people engage in a process like IBE when drawing inferences about causal events: they use explanatory considerations to guide their assessments of which causes account for observed effects, and of how likely candidate hypotheses are to be true. The most direct evidence to date concerns root simplicity, latent scope, and explanatory power, but there is indirect evidence that other explanatory considerations, such as coherence, completeness, and manifest scope, may play a similar role ( Pennington & Hastie, 1992 ; Read & Marcus-Newhall, 1993 ; Preston & Epley, 2005 ; Thagard, 1989 ; Williams & Lombrozo, 2010 ).
Before concluding this section on IBE in causal inference, it is worth considering the normative implications of this work. It is typically assumed that Bayesian updating provides the normatively correct procedure for revising belief in causal hypotheses in light of the evidence. Do the findings reported in this section describe a true departure from Bayesian inference, and therefore a systematic source of error in human judgment? This is certainly one possibility. For example, it could be that IBE describes an imperfect algorithm by which people approximate Bayesian inference. If this is the case, it becomes an interesting project to spell out when and why explanatory considerations ever succeed in approximating more direct probabilistic inference.
There are other possibilities, however. In particular, an appropriately specified Bayesian model could potentially account for these results. In fact, some have argued that IBE-like inference could simply fall out of hierarchical Bayesian inference with suitably assigned priors and likelihoods ( Henderson, 2014 ), in which case there could be a justified, Bayesian account of this behavior. It could also be that the Bayesian models implicit in the comparisons between people’s judgments and posterior probabilities fail to describe the inference that people are actually making. In their chapter in this volume on diagnostic reasoning, for example, Meder and Mayrhofer (Chapter 23 ) make the important point that there can be more than one “Bayesian” model for a given inference, and in fact find different patterns of inference for models that make different assumptions when it comes to elemental diagnostic reasoning: inferring the value of a single binary cause from a single binary effect, which has clear parallels to the cases considered here. In particular, they argue for a model that takes into account uncertainty in causal structures over one that simply computes the empirical conditional probability of a cause given an effect. Similarly, it could be that the “departures” from Bayesian updating observed here reflect the consequences of a Bayesian inference that involves more than a straight calculation of posteriors.
Finally, some argue that IBE corresponds to a distinct but normatively justifiable alternative to Bayesianism (e.g., Douven & Schupbach, 2015a ). In particular, while Bayesian inference may be the best approach for minimizing expected inaccuracy in the long run, it could be that a process like IBE dominates Bayesian inference when the goal is, say, to get things mostly right in the short term, or to achieve some other aim ( Douven, 2013 ). It could also be that explanation judgments take considerations other than accuracy into account, such as the ease with which the explanation can be communicated, remembered, or used in subsequent processing. These are all important possibilities to explore in future research.
Causal Learning and the Process of Explaining
Consider a doctor who, when confronted with a recurring pattern of symptoms, posits a previously undocumented disease, or a previously unknown link between some pathogen and those symptoms. In each case, the inference involves a change in the doctor’s beliefs about the causal structure of the world, not only about the particular patient’s illness. This kind of inference, which we will refer to as causal model learning , differs from the kinds of causal inferences considered in the preceding section in that the learner posits a novel cause or causal relation, not (only) a new token of a known type.
Just as explanatory considerations can influence causal inference, it is likely that a process like IBE can guide causal model learning. In fact, “Occam’s Razor,” the classic admonition against positing unnecessary types of entities ( Baker, 2013 ), is typically formulated and invoked in the context of positing novel types, not tokens of known types. However, research to date has not (to our knowledge) directly explored IBE in the context of causal model learning. Doing so would require assessing whether novel causes or causal relations are more likely to be inferred when they provide better explanations.
What we do know is that engaging in explanation— the process —can affect the course of causal learning. In particular, a handful of studies with preschool-aged children suggest that being prompted to explain, even without feedback on the content or quality of explanations, can promote understanding of number conservation ( Siegler, 1995 ) and of physical phenomena (e.g., a balance beam; Pine & Siegler, 2003 ), and recruit causal beliefs that are not invoked spontaneously to guide predictions ( Amsterlaw & Wellman, 2006 ; Bartsch & Wellman, 1995 ; Legare, Wellman, & Gelman, 2009 ). Prompts to explain can also accelerate children’s understanding of false belief ( Amsterlaw & Wellman, 2006 ; Wellman & Lagattuta, 2004 ; see Wellman & Liu, 2007 , and Wellman, 2011 , for reviews), which requires a revision from one causal model of behavior to a more complex model involving an unobserved variable (belief) and a causal link between beliefs and behavior (e.g., Goodman et al., 2006 ). Finally, there is evidence that prompting children to explain can lead them to preferentially learn about and remember causal mechanisms over causally irrelevant perceptual details ( Legare & Lombrozo, 2014 ), and that prompting children to explain makes them more likely to generalize internal parts and category membership from some objects to others on the basis of shared causal affordances as opposed to perceptual similarity ( Walker, Lombrozo, Legare, & Gopnik, 2014 ; see also Muentener & Bonawitz, Chapter 33 in this volume, for more on children’s causal learning).
To better understand the effects of explanation on children’s causal learning, Walker, Lombrozo, Williams, Rafferty, and Gopnik (2016) set out to isolate effects of explanation on two key factors in causal learning: evidence and prior beliefs. Walker et al. used the classic “blicket detector” paradigm ( Gopnik & Sobel, 2000 ), in which children observe blocks placed on a machine, where some of the blocks make the machine play music. Children have to learn which blocks activate the machine, which can involve positing a novel kind corresponding to a subset of blocks, and/or positing a novel causal relationship between those blocks (or some of their features) and the machine’s activation.
In Walker et al.’s studies, 5-year-old children observed eight blocks successively placed on the machine, where four activated the machine and four did not. Crucially, half the children were prompted to explain after each observation (“Why did [didn’t] this block make my machine play music?”), and the remaining children, in the control condition, were asked to report the outcome (“What happened to my machine when I put this block on it? Did it play music?”). This control task was intended to match the explanation condition in eliciting a verbal response and drawing attention to the relaionship between each block and the machine, but without requiring that the child explain.
Across studies, Walker et al. (2016) varied the properties of the blocks to investigate whether prompting children to explain made them more likely to favor causal hypotheses that were more consistent with the data (i.e., one hypothesis accounted for 100% of observations and the other for 75%) and/or more consistent with prior beliefs (i.e., one hypothesis involved heavier blocks activating the machine, which matched children’s initial asumptions; the other involved blocks of a given color activating the machine). When competing causal hypotheses were matched in terms of prior beliefs but varied in the evidence they accounted for, children who were prompted to explain were significantly more likely than controls to favor the hypothesis with stronger evidence. And when competing causal hypotheses were matched in terms of evidence but varied in their consistency with prior beliefs, children who were prompted to explain were significantly more likely than controls to favor the hypothesis with a higher prior. In other words, explaining made children more responsive to both crucial ingredients of causal learning: evidence and prior beliefs.
In their final study, Walker et al. (2016) considered a case in which evidence and prior beliefs came into conflict: a hypothesis that accounted for 100% of the evidence (“blue blocks activate the machine”) was pitted against a hypothesis favored by prior beliefs (“big blocks activate the machine”), but that only accounted for 75% of the evidence. In this case, children who were prompted to explain were significantly more likely than controls to go with prior beliefs, guessing that a novel big block rather than a novel blue block would activate the machine. This pattern of responses was compared against the predictions of a Bayesian model that incorporated children’s own priors and likelihoods as estimated from an independent task. The results suggested that children who were prompted to explain were less likely than children in the control condition to conform to Bayesian inference. This result may seem surprising in light of explainers’ greater sensitivity to both evidence and prior beliefs, which suggests that explaining results in “better” performance. However, it is less surprising in light of the findings reported in the previous section, which consistently point to a divergence between explanation-based judgments and assessments of posterior probability.
While the evidence summarized thus far is restricted to preschool-aged children, it is likely that similar processes operate in older children and adults. For instance, Kuhn and Katz (2009) had fourth-grade children engage in a causal learning task that involved identifying the causes of earthquakes by observing evidence. The children subsequently participated in a structurally similar causal learning task involving an ocean voyage, where half were instructed to explain the basis for each prediction that they made, and those in a control group were not. When the same students completed the earthquake task in a post-test, those who had explained generated a smaller number of evidence-based inferences; instead, they seemed to rely more heavily on their (mistaken) prior beliefs, in line with the findings from Walker et al. (2016) . In a classic study with eighth-grade students, Chi, De Leeuw, Chiu, and LaVancher (1994) prompted students to “self-explain” as they read a passage about the circulatory system, with students in the control condition instead prompted to read the text twice. Students who explained were significantly more likely to acquire an accurate causal model of the circulatory system, in part, they suggest, because explaining “involved the integration of new information into existing knowledge”—that is, the coordination of evidence with prior beliefs. Finally, evidence with adults investigating the effects of explanation in categorization tasks mirrors the findings from Walker et al. (2016) , with participants who explain both more responsive to evidence ( Williams & Lombrozo, 2010 ) and more likely to recruit prior beliefs ( Williams & Lombrozo, 2013 ).
Why does the process of explaining affect causal learning? One possibility is that explaining simply leads to greater attention or engagement. This is unlikely for a variety of reasons. Prior work has found that while explaining leads to some improvements in performance, it also generates systematic impairments. In one study, children prompted to explain were significantly less likely than controls to remember the color of a gear in a gear toy ( Legare & Lombrozo, 2014 ); in another, they were significantly less likely to remember which sticker was placed on a block ( Walker et al., 2014 ). Research with adults has also found that a prompt to explain can slow learning and increase error rates in a category learning task ( Williams, Lombrozo, & Rehder, 2013 ). Moreover, the findings from the final study of Walker et al. (2016) suggest that prompting children to explain makes them look less, not more, like ideal Bayesian learners. Far from generating a global boost in performance, explanation seems to generate highly selective benefits.
A second possibility is that explaining plays a motivational role that is specifically tied to causal learning. In a provocatively titled paper (“Explanation as Orgasm and the Drive for Causal Understanding”), Gopnik (2000) argues that the phenomenological satisfaction that accompanies a good explanation is part of what motivates us to learn about the causal structure of the world. Prompting learners to explain could potentially ramp up this motivational process, directing children and adults to causal relationships over causally irrelevant details (consistent with Legare & Lombrozo, 2014 ; Walker et al., 2014 ). Explaining could also affect the course of causal inquiry itself, with effects on which data are acquired and how they inform beliefs (see Legare, 2012 , for preliminary evidence that explanation guides exploration).
Finally (and not mutually exclusively), it could be that effects of explanation on learning are effectively a consequence of IBE—that is, that in the course of explaining, children generate explanatory hypotheses, and these explanatory hypotheses are evaluated with “loveliness” as a proxy for “likeliness.” For instance, in Walker et al. (2016) , children may have favored the hypothesis that accounted for more evidence because it had greater scope or coverage, and the hypothesis consistent with prior knowledge because it provided a specification of mechanism or greater coherence. We suspect that this is mostly, but only mostly, correct. Some studies have found that children who are prompted to explain outperform those in control conditions even when they fail to generate the right explanation , or any explanation at all ( Walker et al., 2014 ). This suggests the existence of some effects of engaging in explanation that are not entirely reducible to the effects of having generated any particular explanation.
While such findings are puzzling on a classic interpretation of IBE, they can potentially be accommodated with a modified and augmented version (Lombrozo, 2012 , 2016 ; Wilkenfeld & Lombrozo, 2015 ). Wilkenfeld and Lombrozo (2015) argue for what they call “explaining for the best inference” (EBI), an inferential practice that differs from IBE in focusing on the process of explaining as opposed to candidate explanations themselves. While IBE and EBI are likely to go hand in hand, there could be cases in which the explanatory processes that generate the best inferences are not identical with those promoted by possessing the best explanations, and EBI allows for this possibility.
In sum, there is good evidence that the process of engaging in explanation influences causal learning. This is potentially driven by effects of explanation on the evaluation of both evidence and prior beliefs ( Walker et al., 2016 ). One possibility is that by engaging in explanation, learners are more likely to favor hypotheses that offer “lovely” explanations (Lombrozo, 2012 , 2016 ), and to engage in cognitive processes that affect learning even when a lovely or accurate explanation is not acquired ( Wilkenfeld & Lombrozo, 2015 ). It is not entirely clear, however, whether and when these effects of explanation lead to “better” causal learning. The findings from Amsterlaw and Wellman (2006) and Chi et al. (1994) suggest that effects can be positive, accelerating conceptual development and learning. Other findings are more mixed (e.g., Kuhn & Katz, 2009 ), with the modeling result from Walker et al. (2016) suggesting that prompting children to explain makes them integrate evidence and prior beliefs in a manner that corresponds less closely to Bayesian inference. Better delineating the contours of explanation’s beneficial and detrimental effects will be an important step for future research. It will also be important to investigate how people’s tendency to engage in explanation spontaneously corresponds to these effects. That is, are the conditions under which explaining is beneficial also the conditions under which people tend to spontaneously explain?
Assigning Causal Responsibility
The previous sections considered two kinds of causal reasoning, one involving novel causal structures and the other causal events generated by known structures. Another important class of causal judgments involves the assignment of causal responsibility : to which cause(s) do we attribute a given effect? For instance, a doctor might attribute her patient’s disease to his weak immune system or to a cold virus, when both are in fact present and play a causal role.
Causal attribution has received a great deal of attention within social psychology, with the classic conundrum concerning the attribution of some behavior to a person (“she’s so clumsy!”) versus a situation (“the staircase is so slippery!”) (for reviews, see Fiske & Taylor, 2013 ; Kelley & Michela, 1980 ; Malle, 2004 ). While this research is often framed in terms of causation, it is natural to regard attribution in terms of explanation, with attributions corresponding to an answer to the question of why some event occurred (“Why did Ava slip?”). In his classic “ANOVA model,” Kelley ( 1967 , 1973 ) proposed that people effectively carry out an analysis of covariation between the behavior and a number of internal and external factors, such as the person, stimulus, and situation. For example, to explain why Ava slipped on the staircase yesterday, one would consider how this behavior fares along the dimensions of consensus (did other people slip?), the distinctiveness of the stimulus (did she slip only on that staircase?), and consistency across situations (does she usually slip, or was it the only time she did so?). Subsequent work, however, has identified a variety of additional factors that influence people’s attributions (e.g., Ahn, Kalish, Medin, & Gelman, 1995 ; Försterling, 1992 ; Hewstone & Jaspars, 1987 ; McArthur, 1972 ), and some have challenged the basic dichotomy on which the person-versus-situation analysis is based (Malle, 1999 , 2004 ; Malle, Knobe, O’Laughlin, Pearce, & Nelson, 2000 ). (We direct readers interested in social attribution to Hilton, Chapter 32 in this volume.)
Assignments of causal responsibility also arise in the context of what is sometimes called “causal selection”: the problem of deciding which cause or causes in a chain or other causal structure best explain or account for some effect. Such judgments are especially relevant in moral and legal contexts, where they are closely tied to attributions of blame. For example, suppose that someone steps on a log, which pushes a boulder onto a picnic blanket, crushing a chocolate pie. The person, the log, and the boulder all played a causal role in the pie’s destruction, but various factors might influence our assignment of causal responsibility, including the location of each factor in the chain, whether and by how much it increased the probability of the outcome, and whether the person intended and foresaw the culinary catastrophe (see, e.g., Hart & Honoré, 1985 ; Hilton, McClure, & Sutton, 2009 ; Lagnado & Channon, 2008 ; McClure, Hilton, & Sutton, 2007 ; Spellman, 1997 ). (Chapter 29 in this volume, in which Lagnado and Gerstenberg discuss moral and legal reasoning, explores these issues in detail; also relevant is Chapter 12 by Danks on singular causation.)
While research has not (to our knowledge) investigated whether explanatory considerations such as simplicity and explanatory power influence judgments of causal responsibility, ideas from the philosophy and psychology of explanation can usefully inform research on this topic. For example, scholars of explanation often emphasize the ways in which an explanation request is underspecified by a why-question itself. When we ask, “Why did Ava slip on the stairs?” the appropriate response is quite different if we’re trying to get at why Ava slipped (as opposed to Boris) than if we’re trying to get at why Ava slipped on the stairs (as opposed to the landing). These questions involve a shift in what van Fraassen (1980) calls a “contrast class,” that is, the set of alternatives to the target event that the explanation should differentiate from the target via some appropriate relation (see also Cheng & Novick, 1991 ).
McGill (1989) showed in a series of studies that a number of previously established effects in causal attribution—effects of perspective (actor vs. observer; Jones & Nisbett, 1971 ), covariation information (consensus and distinctiveness; Kelley, 1967 ), and the valence of the behavior being explained (positive vs. negative; Weiner, 1985 )—are related to shifts in the contrast class. Specifically, by manipulating the contrast class adopted by participants, McGill was able to eliminate the actor–observer asymmetry, interfere with the roles of consensus and distinctiveness information, and counteract self-serving attributions of positive versus negative performance. These findings underscore the close relationship between attribution and explanation.
Focusing on explanation is also helpful in bringing to the foreground questions of causal relevance as distinct from probability . In a 1996 paper, Hilton and Erb presented a set of studies designed to clearly differentiate these notions. In one study, Hilton and Erb showed that contextual information can influence the perceived “goodness” and relevance of an explanation without necessarily affecting its probability. For example, participants were asked to rate the following explanation of why a watch broke (an example adapted from Einhorn & Hogarth, 1986 ): “the watch broke because the hammer hit it.” This explanation was rated as fairly good, relevant, and likely to be true; however, after learning that the hammer hit the watch during a routine testing procedure at a watch factory, participants’ ratings of explanation quality and relevance dropped. In contrast, ratings of probability remained high, suggesting that causal relevance and the probability of an explanation can diverge, and that these two factors differ in their susceptibility to this contextual manipulation. It is possible that these effects were generated by a shift in contrast, from “Why did this watch break now (as opposed to not breaking now)?” to “Why did this watch break (as opposed to some other watch breaking)?”
More recently, Chin-Parker and Bradner (2010) showed that effects of background knowledge and implicit contrasts extend to the generation of explanations. They manipulated participants’ background assumptions by presenting a sequence of causal events that either did or did not seem to unfold toward a particular functional outcome (when it did, the sequence appeared to represent a closed-loop system functioning in a self-sustaining manner). Participants’ explanations of an ambiguous observation at the end of the sequence tended to invoke a failure of a system to perform its function in the former case, but featured proximal causes in the latter case. (In contrast to prior research, context did not affect explanation evaluation in this design.)
Taken together, these studies offer another set of examples of how explanatory considerations (in this case, the contextually determined contrast class) can influence causal judgments, and suggest that ascriptions of causal responsibility may vary depending on how they are framed: in terms of causal relevance and explanation, or in terms of probability and truth. It is also possible that considerations such as simplicity and scope play a role in assigning causal responsibility, above and beyond their roles in causal inference and learning. These are interesting questions for future research.
The Varieties of Causal Explanation
There is no agreed-upon taxonomy for explanations; in fact, even the distinction between causal and non-causal explanation generates contested cases. For instance, consider an example from Putnam (1975) . A rigid board has a round hole and a square hole. A peg with a square cross-section passes through the square hole, but not the round hole. Why? Putnam suggests that this can be explained by appeal to the geometry of the rigid objects (which is not causal), without appeal to lower-level physical phenomena (which are presumably causal). Is this a case of non-causal explanation? Different scholars provide different answers.
One taxonomy that has proven especially fruitful in the psychological study of explanation has roots in Aristotle’s four causes (efficient, material, final, and formal), which are sometimes characterized not as causes per se, but in terms of explanation—as distinct answers to a “why?” question ( Falcon, 2015 ). Efficient causes, which identify “the primary source of the change or rest” (e.g., a carpenter who makes a table), seem like the most canonically causal. Material causes, which specify “that out of which” something is made (e.g., wood for a table), are not causal in a narrow sense (for instance, we wouldn’t say that the wood causes or is a cause of the table), but they nonetheless play a clear causal role in the production of an object. Final and formal causes are less clearly causal; but, as we consider in the following discussion, there are ways in which each could be understood causally, as well.
First, consider final causes, which offer “that for the sake of which a thing is done.” Final cause explanations (or perhaps more accurately, their contemporary counterparts) are also known as teleological or functional explanations, as they offer a goal or a function. For instance, we might explain the detour to the café by appeal to a goal (getting coffee), or the blade’s sharpness by appeal to its function (slicing vegetables). On the face of it, these explanations defy the direction of causal influence: they explain a current event (the detour) or property (the sharpness) by appeal to something that occurs only later (the coffee acquisition or the vegetable slicing). Nonetheless, some philosophers have argued that teleological explanations can be understood causally (e.g., Wright, 1976 ), and there is evidence that adults ( Lombrozo & Carey, 2006 ) and children ( Kelemen & DiYanni, 2005 ) treat them causally, as well (see also Chaigneau, Barsalou, & Sloman, 2004 , and Lombrozo & Rehder, 2012 , for more general investigations of the causal structure of functions).
How can teleological explanations be causal? On Wright’s view, teleological explanations do not explain the present by appeal to the future—rather, the appeal to an unrealized goal or function is a kind of shorthand for a complex causal process that brought about (and hence preceded ) what is being explained. In cases of intentional action, the function or goal could be a shorthand for the corresponding intention that came first: the detour to the café was caused by a preceding intention to get coffee, and the blade’s sharpness was caused by the designer’s antecedent intention to create a tool for slicing vegetables. Other cases, however, can be more complex. For instance, we might explain this zebra’s stripes by appeal to their biological function (camouflage) because its ancestors had stripes that produced effective camouflage, and in part for that reason, stripes were increased or maintained in the population. If past zebra stripes didn’t produce camouflage, then this zebra wouldn’t have stripes (indeed, this zebra might not exist at all). In this case, the function can be explanatory because it was produced by “a causal process sensitive to the consequences of changes it produces” ( Lombrozo & Carey, 2006 ; Wright, 1976 ), even in the absence of a preceding intention to realize the function.
Lombrozo and Carey (2006) tested these ideas as a descriptive account of the conditions under which adults accept teleological explanations. In one study, they presented participants with causal stories in which a functional property did or did not satisfy Wright’s conditions. For example, participants learned about genetically engineered gophers that eat weeds, and whose pointy claws damage the roots of weeds as they dig, making them popular among farmers. The causal role of “damaging roots” in bringing about the pointy claws varied across conditions, from no role (the genetic engineer accidentally introduced a gene sequence that resulted in gophers with pointy claws), to a causal role stemming from an intention to damage roots (the genetic engineer intended to help eliminate weeds, and to that end engineered pointy claws), to a causal role without an intention to damage roots (the genetic engineer didn’t realize that pointy claws damaged weed roots, but did notice that the pointy claws were popular and decided to create all of his gophers with pointy claws). Participants then rated the acceptability and quality of teleological (and other) explanations. For the vignette involving genetically engineered gophers, they were asked why the gophers had pointy claws, and rated “because the pointy claws damage weed roots” as a response.
In this and subsequent studies, Lombrozo and Carey (2006) found that teleological explanations are understood causally in the sense that participants only accepted teleological explanations when the function or goal invoked in the explanation played an appropriate causal role in bringing about what was being explained. More precisely, this causal requirement was necessary for teleological explanations to be accepted, but not sufficient . In the preceding examples, teleological explanations were accepted at high levels when the function was intended, at moderate levels when the function played a non-intentional causal role, and at low levels when the function played no causal role at all. Lombrozo and Carey suggest (and provide evidence) that in addition to satisfying certain causal requirements, teleological explanations might call for the existence of a general pattern that makes the function predictively useful.
Kelemen and DiYanni (2005) conducted a study with elementary school children (6–7 and 9–10-year-olds) investigating the relationship between their acceptance and generation of teleological explanations for natural phenomena, on the one hand, and their causal commitments concerning their origins, on the other hand—specifically, whether they believed that an intentional designer of some kind (“someone or something”) made them or they “just happened.” The tendency to endorse and generate teleological explanations of natural events, non-living natural objects, and animals was significantly correlated with belief in the existence of an intentional creator of some kind, be it God, a human, or an unspecified force or agent. While these findings do not provide direct support for the idea that teleological explanations are grounded in a preceding intention to produce the specific function in question, the link between teleological explanations and intentional design more generally is consistent with the idea that teleological explanations involve some basic causal commitments. Along the same lines, Kelemen, Rottman, and Seston (2013) found that adults (including professional scientists) who believe in God or “Gaia” are more likely to accept scientifically unwarranted teleological explanations (see also ojalehto, Waxman, & Medin, 2013 , for a relevant discussion). Thus, the findings to date suggest that teleological explanations are understood causally by both adults and children.
What about formal explanations? Within Aristotle’s framework, a formal explanation offers “the form” of something or “the account of what-it-is-to-be.” Within psychology, what little work there is on formal explanation has focused on explanations that appeal to category membership. For example, Prasada and Dillingham (2006) define formal explanations as stating that tokens of a type have certain properties because they are the kinds of things they are (i.e., tokens of the respective type): we can say that Zach diagnoses ailments because he is a doctor , or that a particular object is sharp because it is a knife .
In their original paper and in subsequent work, Prasada and Dillingham ( 2006 , 2009 ) argue that formal explanations are not causal, but instead are explanatory by virtue of a part–whole relationship. They show that only properties that are considered to be aspects of the kind support formal explanations, in contrast to “statistical” properties that are merely reliably associated with the kind. For example, people accepted a formal explanation of why something has four legs by reference to its category (“because it’s a dog”), and also accepted the claim that “having four legs” is one aspect of being a dog. In contrast, participants rejected formal explanations such as “that (pointing to a barn) is red because it’s a barn,” and also denied that being red is one aspect of being a barn (even though most barns are red). Prasada and Dillingham (2009) argue that the relationship underlying such formal explanation is constitutive (not causal): aspects are connected to kinds via a part–whole relationship, and such relationships are explanatory because the “existence of a whole presupposes the existence of its parts, and thus the existence of a part is rendered intelligible by identifying the whole of which it is a part” (p. 421).
Prasada and Dillingham offer two additional pieces of evidence for the proposal that formal explanations are constitutive, and not causal. First, they demonstrate the explanatory potential of the part–whole relationship by showing that when this relationship is made explicit, even statistical features can support formal explanations. For example, we can explain, “Why is that (pointing to a barn) red? Because it is a red barn,” where being red is understood as part of being a red barn ( Prasada & Dillingham, 2009 ). This explanation isn’t great, but neither is it tautological: it identifies the source of the redness in something about the red barn, as opposed, for instance, to the light that happens to be shining on it (see also Cimpian & Salomon, 2014 , on “inherent” explanations). Less convincingly, they attempt to differentiate formal explanations from causal-essentialist explanations. On causal-essentialist accounts, a category’s essence is viewed as the cause of the category members’ properties ( Gelman, 2003 ; Gelman & Hirschfeld, 1999 ; Medin & Ortony, 1989 ), which could ground formal explanations in a causal relationship. To test this, Prasada and Dillingham had participants evaluate explanations such as “Why does that (pointing to a dog) have four legs? Because it has the essence of a dog which causes it to have four legs” ( Prasada & Dillingham, 2006 ). While there was a trend for formal explanations to be rated more highly than causal-essentialist explanations for properties that were taken to be aspects of a given kind, the results were inconclusive. As Prasada and Dillingham acknowledge, the wording of the causal-essentialist explanations was awkward, which could partially account for their middling acceptance. It thus remains a possibility that at least some formal explanations are understood causally, as pointers to some category-associated essence or causal factor responsible for the properties being explained.
One reason it is valuable to recognize the diversity of explanations is that different kinds of explanations lead to systematically different patterns of causal judgment. For example, Lombrozo (2009) investigated the relationship between different kinds of causal explanations and the relative importance of features in classification (see also Ahn, 1998 ). Participants learned about novel artifacts and organisms with three causally related features. To illustrate, one item involved “holings,” a type of flower with “brom” compounds in its stem, which makes it bend over as it grows, which means its pollen can be spread to other flowers by wandering field mice. Participants were asked a why-question about the middle feature (e.g., “Why do holings typically bend over?”), which was ambiguous as a request for a mechanistic explanation (e.g., “Because of the brom compounds”) or a teleological explanation (e.g., “In order to spread their pollen”). Participants provided an explanation and were subsequently asked to decide whether novel flowers were holings, where some shared the mechanistic feature (brom compounds) and some shared the functional feature (bending over). Lombrozo found that participants who provided functional explanations in response to the ambiguous why-question were significantly more likely than participants who did not to then privilege the functional feature relative to the mechanistic feature when it came to classification. Similarly, a follow-up study found that experimentally prompting participants to generate a particular explanation type by disambiguating the why-question (“In other words, what purpose might bending over serve?”) had the same effect (see also Lombrozo & Rehder, 2012 , for additional evidence about the relationship between functions and kind classification).
Additional studies suggest that the effects of mechanistic versus functional explanations extend beyond judgments of category membership. Lombrozo and Gwynne (2014) employed a method similar to Lombrozo (2009) , presenting participants with causal chains consisting of three elements, such as a certain gene that causes a speckled pattern in a plant, which attracts butterflies that play a role in pollination. Participants explained the middle feature (the speckled pattern) and generalized a number of aspects of that feature (e.g., its density, contrast, and color) to novel entities that shared either a causal or a functional feature with the original. Lombrozo and Gwynne found that explaining a property functionally (versus mechanistically) promoted the corresponding type of generalization.
Vasilyeva and Coley (2013) demonstrated a similar link between explanation and generalization in an open-ended task. Participants learned about plants and animals possessing novel but informative properties (e.g., ducks have parasite X [or X-cells ]) and generated hypotheses about which other organisms might share the property. In the course of generating these hypotheses, participants spontaneously produced formal, causal, and teleological explanations in a manner consistent with the property they reasoned about. Of most importance the type of explanation predicted the type of generalization: for example, people were most likely to generalize properties to entities related via causal interactions (e.g., plants and insects that ducks eat, or things that eat ducks) after generating causal explanations (e.g., they got it from their food). In a separate set of studies, Vasilyeva and Coley (in preparation) ruled out an alternative account based exclusively on the direct effects of generalized properties on generalizations.
Beyond highlighting some causal relationships over others, different kinds of explanations could change the way participants represent and reason about causal structure. Indeed, findings from Lombrozo (2010) suggest that this is the case. In a series of studies, Lombrozo presented participants with causal structures drawn from the philosophical literature and intended to disambiguate two accounts of causation: those based on some kind of dependence relationship (see Le Pelley, Griffiths, and Beesley, Chapter 2 in this volume) and those based on some kind of transference (see Wolff and Thorstad, Chapter 9 in this volume). According to one version of the former view, C is a cause of E if it is the case that had C not occurred, E would not have occurred. In other words, E depends upon C in the appropriate way, in this case counterfactually. According to one version of transference views, C is a cause of E if there was a physical connection between C and E—some continuous mechanism or conserved physical quantity, such as momentum.
While dependence and transference often go hand in hand, they can come apart in cases of “double prevention” and “overdetermination.” Lombrozo presented participants with such cases and found that judgments were more closely aligned with dependence views than transference views when the causal structures were directed toward a function or goal, and therefore supported a teleological explanation. Lombrozo (2010) explains this result, in part, by appeal to the idea of equifinality : when a process is goal-directed, the end may be achieved despite variations in the means. To borrow William James’s famous example, Romeo will find his way to Juliet whatever obstacle is placed in his path ( James, 1890 ). He might scale a fence or wade through a river, but the end—reaching Juliet—will remain the same. When participants reason about a structure in teleological or goal-directed terms, they may similarly represent it as means- or mechanism-invariant, and therefore focus on dependence relationships irrespective of the specific transference that happened to obtain.
In sum, pluralism has long been recognized as a feature of explanation, with Aristotle’s taxonomy providing a useful starting point for charting variation in explanations (although it is by no means the only taxonomy of explanation; see, for example, Cimpian & Salomon, 2014 , on inherent versus extrinsic explanations). We have reviewed evidence that teleological explanations are causal explanations, but that they are nonetheless treated differently from mechanistic explanations, which do not appeal to functions or goals. The evidence concerning formal explanations is less conclusive, but points to a viable alternative to a causal interpretation, with formal explanation instead depending on constitutive part–whole relations.
Recognizing explanatory pluralism can provide a useful road map for thinking about pluralism when it comes to causation and causal relations. In fact, as we have seen, different kinds of explanations do lead to systematic differences in classification and inference, with evidence that causal relationships themselves may be represented differently under different “explanatory modes.” In the following section, we take a closer look at mechanistic explanations and their relationship to causation and mechanisms.
Explanation and Causal Mechanisms
The “mechanistic explanations” considered in the previous section concerned the identification of one or more causes that preceded some effect. Often, however, causal explanations do not simply identify causes, but instead aim to articulate how the cause brought about the effect. That is, they involve a mechanism . But what, precisely, is a mechanism? Are all mechanisms causal? And do mechanisms have a privileged relationship to explanation? In this section, we begin to address these questions about the relationship between mechanisms and explanations. For a more general discussion of mechanisms, we direct readers to the chapter on mechanisms by Johnson and Ahn (Chapter 8 in this volume).
Within psychology, there is growing interest in the role of mechanisms in causal reasoning. For example, Ahn, Kalish, Medin, and Gelman (1995) found that people seek “mechanistic” information in causal attribution. Park and Sloman (2013) found that people’s violations of the Markov assumption depended on their “mechanistic” beliefs about the underlying causal structure. Buehner and McGregor (2006) showed that beliefs about mechanism type moderate effects of temporal contiguity in causal judgments (see also Ahn & Bailenson, 1996 ; Buehner & May, 2004 ; Fugelsang & Thompson, 2000 ; Koslowski & Okagaki, 1986 ; Koslowski, Okagaki, Lorenz, & Umbach, 1989 ; for reviews, see Ahn & Kalish, 2000 ; Johnson & Ahn, Chapter 8 in this volume; Koslowski, 1996 , 2012 ; Koslowski & Masnik, 2010 ; Sloman & Lagnado, 2014 ; Waldmann & Hagmayer, 2013 ). Despite these frequent appeals to mechanisms and mechanistic information, however, there is no explicitly articulated and widely endorsed conception of “mechanism.”
Most often, a mechanism is taken to spell out the intermediate steps between some cause and some effect. For example, Park and Sloman (2014) define a mechanism as “the set of causes, enablers, disablers, and preventers that are directly involved in producing an effect, along with information about how the effect comes about, including how it unfolds over time” (p. 807). Research that adopts a perspective along these lines often goes further in explicitly identifying such mechanisms as explanations (and these terms are often used interchangeably, as in Koslowski & Masnik, 2010 ). Other work operationalizes mechanisms using measures of explanation, implicitly suggesting a correspondence. For example, to validate a manipulation of mechanism, Park and Sloman asked participants whether the same explanation applies to both effects in a common-cause structure (see also Park & Sloman, 2013 ). Similarly, in a study examining mental representations of mechanisms, Johnson and Ahn (2015) considered (but did not ultimately endorse) an “explanatory” sense of mechanism, which they operationalized by asking participants to rate the extent to which some event B explains why event A led to event C.
Shifting from psychology to philosophy, we find a class of accounts of explanation that likewise associate explanations with a specification of mechanisms (e.g., Bechtel & Abrahamsen, 2005 ; Glennan, 1996 , 2002 ; Machamer, Darden, & Craver, 2000 ; Railton, 1978 ; Salmon, 1984 ). Consistent with the empirical work reviewed earlier, some of these accounts (e.g., Railton, 1978 ; Salmon, 1984 ) consider mechanisms to be “sequences of interconnected events” ( Glennan, 2002 , p. S345). Canonical examples include causal chains or networks of events leading to a specific outcome, such as a person who kicks a ball, which bounces off a pole, which breaks a window. On these views, explanation, causation, and mechanisms are not only intimately related, but potentially interdefined.
A second view of mechanisms within philosophy, however, departs more dramatically from work in psychology, and also suggests a more circumscribed role for causation. These views analyze mechanisms as complex systems that involve a (typically hierarchical) structure and arrangement of parts and processes, such as that exhibited by a watch, a cell, or a socioeconomic system (e.g., Bechtel & Abrahamsen, 2005 ; Glennan, 1996 , 2002 ; Machamer, Darden, & Craver, 2000 ). Within this framework, Craver and Bechtel (2007) offer an insightful analysis of causal and non-causal relationships within a multilevel mechanistic system. Specifically, they suggest that interlevel (i.e., “vertical”) relationships within a mechanism are not causal, but constitutive . For instance, a change in rhodopsin in retinal cells can partially explain how signal transduction occurs, but we wouldn’t say that this change causes signal transduction; it arguably is signal transduction (or one aspect of it). Craver and Bechtel point out that constitutive relations conflict with many common assumptions about event causation: that causes and effects must be distinct events, that causes precede their effects, that the causal relation is asymmetrical, and so on. Unlike causation, explanation can accommodate both causal (intralevel) relationships and constitutive (interlevel) relationships, of the kind documented by Prasada and Dillingham’s (2009) work on formal explanation.
Although Craver and Bechtel convincingly argue that the causal reading of interlevel relationships is erroneous (see also Glennan, 2010 , for related claims), as a descriptive matter, it could be that laypeople nonetheless interpret them in causal terms. An example from the Betty Crocker Cookbook , discussed by Patricia Churchland (1994) , illustrates the temptation. In the book, Crocker is correct to explain that microwave ovens work by accelerating the molecules comprising the food, but she wrongly states that the excited molecules rub against one another and that their friction generates heat. Crocker assumes that the increase in mean kinetic energy of the molecules causes heat, when in fact heat is constituted by the mean kinetic energy of the molecules ( Craver & Bechtel, 2007 ). A study by Chi, Roscoe, Slotta, Roy, and Chase (2012) showed that eighth and ninth graders, like Crocker, tended to misconstrue non-sequential, emergent processes as direct sequential causal relationships. It’s possible that adults might make similar errors as well, assimilating non-causal explanations to a causal mold.
There are thus many open questions about how best to define mechanisms for the purposes of psychological theory, and about the extent to which mechanisms are represented in terms of strictly causal relationships. What we do know, however, is that explanations and mechanisms seem to share a privileged relationship. More precisely, there is evidence that the association between mechanisms and explanation claims is closer than that between mechanisms and corresponding causal claims ( Vasilyeva & Lombrozo, 2015 ).
The studies by Vasilyeva and Lombrozo (2015) used “minimal pairs”: causal and explanatory claims that were matched as closely as possible. For example, participants read about a person, PK, who spent some time in the portrait section of a museum and made an optional donation to the museum. They were then asked to evaluate how good they found an explanation for the donation (“Why did PK make an optional donation to the museum? Because PK spent some time in the portrait section”), or how strongly they endorsed a causal relationship (“Do you think there exists a causal relationship between PK spending some time in a portrait section and PK making an optional donation to the museum?”).
Vasilyeva and Lombrozo varied two factors across items and participants: the strength of covariation evidence between the candidate cause and effect, and knowledge of a mediating mechanism. In the museum example, some participants learned the speculative hypothesis that “being surrounded by many portraits (as opposed to other kinds of paintings) creates a sense that one is surrounded by watchful others. This reminds the person of their social obligations, which in turn encourages them to donate money to the public museum.” Both explanation and causal judgments were affected by these manipulations of covariation and mechanism information. However, they were not affected equally: specifying a mechanism had a stronger effect on explanation ratings than on causal ratings, while the strength of covariation evidence had a stronger effect on causal ratings than on explanation ratings.
The findings from Vasilyeva and Lombrozo (2015) support a special relationship between explanations and mechanisms. They also challenge views that treat explanations as equivalent to identifying causal relationships, since matched explanation and causal claims were differentially sensitive to mechanisms and covariation. The findings thus raise the possibility that explanatory and causal judgments are tuned to support different cognitive functions. For example, explanation could be especially geared toward reliable and broad generalizations ( Lombrozo & Carey, 2006 ), which can benefit from mechanistic information: when we understand the mechanism by which some cause generates some effect, we can more readily infer whether the same relationship will obtain across variations in circumstances. By learning the mechanism that mediates the relationship between visiting a portrait gallery and making an optional museum donation, for example, we are in a better position to predict whether visiting a figurative versus an abstract sculpture garden will have the same effect. This benefit can potentially be realized with quite skeletal mechanistic ( Rozenblit & Keil, 2002 ) or functional understanding ( Alter, Oppenheimer, & Zemla, 2010 ); people need not understand a mechanism in full detail to gain some inferential advantage. Causal claims, by contrast, could more closely track the evidence concerning a particular event or relationship, rather than the potential for broad generalization.
In sum, the picture that emerges is one of partial overlap between causality, explanation, and mechanisms. Work in philosophy offers a variety of proposals emphasizing different aspects of mechanisms: structure, functions, temporally unfolding processes connecting starting conditions to the end state, and so on. Explanatory and causal judgments could track different aspects of mechanisms, resulting in the patterns of association and divergence observed. We suspect that adopting more explicit and sophisticated notions of mechanism will help research in this area move forward. On a methodological note, we think the strategy adopted in Vasilyeva and Lombrozo (2015) —of contrasting the characteristics of causal explanation claims with “matched” causal claims—could be useful in driving a wedge between different kinds of judgments, thus shedding light on their unique characteristics and potentially unique roles in human cognition. This strategy can also generalize to other kinds of judgments. For example, Dehghani, Iliev, and Kaufmann (2012) and Rips and Edwards (2013) both report systematic patterns of divergence between explanations and counterfactual claims, another judgment with a potentially foundational relationship to both explanation and causation.
Conclusions
Throughout the chapter, we have presented good evidence that explanatory considerations affect causal reasoning, with implications for causal inference, causal learning, and attribution. We have also considered different kinds of explanations, including their differential effects on causal generalizations and causal representation, and the role of mechanisms in causal explanation. However, many questions remain open. We highlight four especially pressing questions here.
First, we have observed many instances in which explanation leads to departures from “normative” reasoning, at least on the assumption that one ought to infer causes and causal relationships by favoring causal hypotheses with the highest posterior probabilities. Are these departures truly errors? Or have we mischaracterized the relevant competence? In particular, could it be that explanatory judgments are well-tuned to some cognitive end, but that end is not the approximation of posterior probabilities?
Second, we have focused on a characterization of explanations and the effects of engaging in explanation, with little attention to underlying cognitive mechanisms. How do people actually go about generating and evaluating causal explanations? How do the mental representations that support explanation relate to those that represent causal structure? And how do explanatory capacities arise over the course of development?
Third, what is the relationship between causal and non-causal explanations? Are they both explanatory by virtue of some shared explanatory relationship, or are causal explanations explanatory by virtue of being causal, with non-causal explanations explanatory for some other reason (for instance, because they embody a part–whole relationship)? On each view, what are the implications for causation?
Finally, we have seen how debates in explanation (from both philosophy and psychology) can inform the study of causation, with examples including inference to the best explanation, the idea of a “contrast class,” and pluralism about explanatory kinds. Can the literature on levels of explanation (e.g., Potochnik, 2010 ) perhaps inspire some new debates about levels of causation (as in, e.g., Woodward, 2010 )? Recent work on hierarchical Bayesian models and hierarchical causal structures is beginning to move in this direction, with the promise of a richer and more powerful way to understand humans’ remarkable ability to reason about and explain the causal structure of the world.
Acknowledgments
The preparation of this chapter was partially supported by the Varieties of Understanding Project funded by the Templeton Foundation, as well as an NSF CAREER award to the first author (DRL-1056712). We are also grateful to David Danks, Samuel Johnson, and Michael Waldmann for helpful comments on a previous draft if this chapter.
Ahn, W. ( 1998 ). Why are different features central for natural kinds and artifacts? The role of causal status in determining feature centrality. Cognition , 69 (2), 135–178.
Google Scholar
Ahn, W. K. , & Bailenson, J. ( 1996 ). Causal attribution as a search for underlying mechanisms: An explanation of the conjunction fallacy and the discounting principle. Cognitive Psychology , 31 (1), 82–123.
Ahn, W. K. , & Kalish, C. ( 2000 ). The role of mechanism beliefs in causal reasoning. In F. C. Keil (Ed.), Explanation and cognition . Cambridge, MA: MIT Press.
Google Preview
Ahn, W. K. , Kalish, C. W. , Medin, D. L. , & Gelman, S. A. ( 1995 ). The role of covariation versus mechanism information in causal attribution. Cognition , 54 , 299–352.
Alter, A. L. , Oppenheimer, D. M. , & Zemla, J. C. ( 2010 ). Missing the trees for the forest: A construal level account of the illusion of explanatory depth. Journal of Personality and Social Psychology , 99 , 436–451.
Amsterlaw, J. , & Wellman, H. M. ( 2006 ). Theories of mind in transition: A microgenetic study of the development of false belief understanding. Journal of Cognition and Development , 7 (2), 139–172.
Baker, A. ( 2013 ). Simplicity. In E. N. Zalta (Ed.), The Stanford encyclopedia of philosophy (Fall 2013 ed.). http://plato.stanford.edu/archives/fall2013/entries/simplicity/ .
Bartsch, K. , & Wellman, H. M. ( 1995 ). Children talk about the mind . Oxford: Oxford University Press.
Bechtel, W. , & Abrahamsen, A. ( 2005 ). Explanation: A mechanist alternative. Studies in History and Philosophy of Science Part C :Studies in History and Philosophy of Biological and Biomedical Sciences , 36 (1995), 421–441.
Bonawitz, E. B. , & Lombrozo, T. ( 2012 ). Occam’s rattle: Children’s use of simplicity and probability to constrain inference. Developmental Psychology , 48 (4), 1156–1164.
Buehner, M. J. ( 2005 ). Contiguity and covariation in human causal inference. Learning & Behavior: A Psychonomic Society Publication , 33 (2), 230–238.
Buehner, M. J. , & May, J. ( 2004 ). Abolishing the effect of reinforcement delay on human causal learning. The Quarterly Journal of Experimental Psychology , 57 B , 179–191.
Buehner, M. J. , & McGregor, S. ( 2006 ). Temporal delays can facilitate causal attribution: Towards a general timeframe bias in causal induction. Thinking & Reasoning , 12 , 353–378.
Chaigneau, S. E. , Barsalou, L. W. , & Sloman, S. A. ( 2004 ). Assessing the causal structure of function. Journal of Experimental Psychology: General , 133 (4), 601–25.
Cheng, P. W. ( 1997 ). From covariation to causation: A causal power theory. Psychological Review , 104 , 367–405.
Cheng, P. W. , & Novick, L. R. ( 1990 ). A probabilistic contrast model of causal induction. Journal of Personality and Social Psychology , 58 (4), 545.
Cheng, P. W. , & Novick, L. R. ( 1991 ). Causes versus enabling conditions. Cognition , 40 (1–2), 83–120.
Cheng, P. W. , & Novick, L. R. ( 1992 ). Covariation in natural causal induction. Psychological Review , 99 (2), 365–382.
Chi, M. T. H. , De Leeuw, N. , Chiu, M.-H. , & Lavancher, C. ( 1994 ). Eliciting self-explanations improves understanding. Cognitive Science , 18 (3), 439–477.
Chi, M. T. H. , Roscoe, R. D. , Slotta, J. D. , Roy, M. , & Chase, C. C. ( 2012 ). Misconceived causal explanations for emergent processes. Cognitive Science , 36 (1), 1–61.
Chin-Parker, S. , & Bradner, A. ( 2010 ). Background shifts affect explanatory style: How a pragmatic theory of explanation accounts for background effects in the generation of explanations. Cognitive Processing , 11 (3), 227–249.
Churchland, P. S. ( 1994 ). Can neurobiology teach us anything about consciousness? Proceedings and Addresses of the American Philosophical Association , 67 (4), 23–40.
Cimpian, A. , & Salomon, E. ( 2014 ). The inherence heuristic: An intuitive means of making sense of the world, and a potential precursor to psychological essentialism. Behavioral and Brain Sciences , 37 (5), 461–480.
Craver, C. F. , & Bechtel, W. ( 2007 ). Top-down causation without top-down causes. Biology and Philosophy , 22 , 547–563.
Dehghani, M. , Iliev, R. , & Kaufmann, S. ( 2012 ). Causal explanation and fact mutability in counterfactual reasoning. Mind & Language , 27 (1), 55–85.
Douven, I. ( 2011 ). Abduction. In E. N. Zalta (Ed.), The Stanford encyclopedia of philosophy . (Spring 2011 ed.). http://plato.stanford.edu/archives/spr2011/entries/abduction/ .
Douven, I. ( 2013 ). Inference to the best explanation, Dutch books, and inaccuracy minimisation. Philosophical Quarterly , 63 (252), 428–444.
Douven, I. , & Schupbach, J. N. ( 2015 a). The role of explanatory considerations in updating. Cognition , 142 , 299–311.
Douven, I. , & Schupbach, J. N. ( 2015 b). Probabilistic alternatives to Bayesianism: The case of explanationism. Frontiers in Psychology , 6 , 1–9.
Einhorn, H. J. , & Hogarth, R. M. ( 1986 ). Judging probable cause. Psychological Bulletin , 99 (1), 3–19.
Falcon, A. ( 2015 ). Aristotle on causality. In E. N. Zalta (Ed.), The Stanford encyclopedia of philosophy (Spring 2015 Edition). http://plato.stanford.edu/archives/spr2015/entries/aristotle-causality/ .
Fiske, S. T. , & Taylor, S. E. ( 2013 ). Social cognition: From brains to culture . Thousand Oaks, CA: Sage.
Försterling, F. ( 1992 ). The Kelley model as an analysis of variance analogy: How far can it be taken? Journal of Experimental Social Psychology , 28 (5), 475–490.
Fugelsang, J. A. , & Thompson, V. A. ( 2000 ). Strategy selection in causal reasoning: When beliefs and covariation collide. Canadian Journal of Experimental Psychology , 54 , 15–32.
Gelman, S. A. ( 2003 ). The essential child: Origins of essentialism in everyday thought . Oxford: Oxford University Press.
Gelman, S. A. , & Hirschfeld, L. A. ( 1999 ). How biological is essentialism. Folkbiology , 9 , 403–446.
Glennan, S. ( 1996 ). Mechanisms and the nature of causation. Erkenntnis , 44 (1), 49–71.
Glennan, S. ( 2002 ). Rethinking mechanistic explanation. Philosophy of Science , 69 (3), S342–S353.
Glennan, S. ( 2010 ). Mechanisms, causes, and the layered model of the world. Philosophy and Phenomenological Research , 81 (2), 362–381.
Glymour, C. , & Cheng, P. W. ( 1998 ). Causal mechanism and probablity: A normative approach. In M. Oaksford & N. Chater (Eds.), Rational models of cognition (pp. 295–313). Oxford: Oxford University Press.
Good, I. J. ( 1960 ). Weight of evidence, corroboration, explanatory power, information and the utility of experiments. Journal of the Royal Statistical Society: Series B (Methodological) , 22 (2), 319–331.
Goodman, N. D. , Baker, C. L. , Bonawitz, E. B. , Mansinghka, V. K. , Gopnik, A. , Wellman, H. , et al. ( 2006 ). Intuitive theories of mind: A rational approach to false belief. In R. Sun & N. Miyake (Eds.), Proceedings of the 28th annual conference of the Cognitive Science Society (pp. 1382–1387). Mahwah, NJ: Lawrence Erlbaum Associates.
Gopnik, A. ( 2000 ). Explanation as orgasm and the drive for causal knowledge: The function, evolution, and phenomenology of the theory formation system. In F. Keil & R. A. Wilson (Eds.), Explanation and cognition (pp. 299–323). Cambridge, MA: MIT Press.
Gopnik, A. , & Sobel, D. M. ( 2000 ). Detecting blickets: How young children use information about novel causal powers in categorization and induction. Child Development , 71 (5), 1205–1222.
Griffiths, T. L. , & Tenenbaum, J. B. ( 2005 ). Structure and strength in causal induction. Cognitive Psychology , 51 (4), 334–384.
Harman, G. H. ( 1965 ). The inference to the best explanation. Philosophical Review , 74 (1), 88–95.
Hart, H. L. A. , & Honoré, T. ( 1985 ). Causation in the Law . Oxford: Oxford University Press.
Henderson, L. ( 2014 ). Bayesianism and inference to the best explanation. The British Journal for the Philosophy of Science , 65 , 687–715.
Hewstone, M. , & Jaspars, J. ( 1987 ). Covariation and causal attribution: A logical model of the intuitive analysis of variance. Journal of Personality and Social Psychology , 53 (4), 663–672.
Hilton, D. J. , & Erb, H.-P. ( 1996 ). Mental models and causal explanation: Judgments of probable cause and explanatory relevance. Thinking and Reasoning , 2 (4), 273–308.
Hilton, D. J. , McClure, J. , & Sutton, R. M. ( 2009 ). Selecting explanations from causal chains: Do statistical principles explain preferences for voluntary causes? European Journal of Social Psychology , 39 , 1–18.
Holyoak, K. J. , & Cheng, P. W. ( 2011 ). Causal learning and inference as a rational process: The new synthesis. Annual Review of Psychology , 62 , 135–163.
James, W. ( 1890 ). The principles of psychology . New York: H. Holt.
Johnson, S. G. B. , & Ahn, W. ( 2015 ). Causal networks or causal islands? The Rrepresentation of mechanisms and the transitivity of causal judgment. Cognitive Science , 1–36.
Johnson, S. G. B. , Johnston, A. M. , Toig, A. E. , & Keil, F. C. ( 2014 ). Explanatory scope informs causal strength inferences. In P. Bello , M. Guarini , M. McShane , & B. Scassellati (Eds.), Proceedings of the 36th annual conference of the Cognitive Science Society (pp. 2453–2458). Austin, TX: Cognitive Science Society.
Johnston, A. M. , Johnson, S. G. B. , Koven M. L. , & Keil, F. C. ( 2015 ). Probabilistic versus heuristic accounts of explanation in children: Evidence from a latent scope bias. In D. C. Noelle , R. Dale , A. S. Warlaumont , J. Yoshimi , T. Matlock , C. D. Jennings , & P. P. Maglio (Eds.), Proceedings of the 37th annual conference of the Cognitive Science Society (pp. 1021–1026). Austin, TX: Cognitive Science Society.
Jones, E. E. , & Nisbett, R. E. ( 1971 ). The actor and the observer: Divergent perceptions of the causes of behavior. In E. E. Jones et al. (Eds.), Attribution: Perceiving the causes of behavior . Morristown, N.J.: General Learning Press.
Kelemen, D. , & DiYanni, C. ( 2005 ). Intuitions about origins: Purpose and intelligent design in children’s reasoning about nature. Journal of Cognition and Development , 6 , 3–31.
Kelemen, D. , Rottman, J. , & Seston, R. ( 2013 ). Professional physical scientists display tenacious teleological tendencies: Purpose-based reasoning as a cognitive default. Journal of Experimental Psychology: General , 142 (4), 1074–1083.
Kelley, H. H. ( 1967 ). Attribution theory in social psychology. Nebraska Symposium on Motivation , 15 , 192–238.
Kelley, H. H. ( 1973 ). The process of causal attributions. American Psychologist , 28 , 107–128.
Kelley, H. H. , & Michela, J. L. ( 1980 ). Attribution theory and research. Annual Review of Psychology , 31 , 457–501.
Kemp, C. , Goodman, N. , & Tenenbaum, J. ( 2010 ). “ http://www.psy.cmu.edu/%7Eckemp/papers/kempgt10_learningtolearncausalmodels.pdf ” Learning to learn causal models. Cognitive Science , 34 (7), 1185–1243.
Khemlani, S. S. , Sussman, A. B. , & Oppenheimer, D. M. ( 2011 ). Harry Potter and the sorcerer’s scope: Latent scope biases in explanatory reasoning. Memory & Cognition , 39 (3), 527–535.
Koslowski, B. ( 1996 ). Theory and evidence: The development of scientific reasoning . Cambridge, MA: MIT Press.
Koslowski, B. ( 2012 ). Scientific reasoning: Explanation, confirmation bias, and scientific practice. In G. Feist & M. Gorman (Eds.), Handbook of the psychology of science . New York: Springer.
Koslowski, B. , & Masnick, A. ( 2010 ). Causal reasoning and explanation. In U. C. Goswami (Ed.), The Wiley-Blackwell handbook of childhood cognitive development (2nd ed., pp. 377–398). Malden, MA: Wiley-Blackwell.
Koslowski, B. , & Okagaki, L. ( 1986 ). Non-Humean indices of causation in problem-solving situations: Causal mechanism, analogous effects, and the status of rival alternative accounts. Child Development , 57 (5), 1100–1108.
Koslowski, B. , Okagaki, L. , Lorenz, C. , & Umbach, D. ( 1989 ). When covariation is not enough: The role of causal mechanism, sampling method, and sample size in causal reasoning. Child Development , 60 (6), 1316–1327.
Kuhn, D. , & Katz, J. ( 2009 ). Are self-explanations always beneficial? Journal of Experimental Child Psychology , 103 (3), 386–394.
Lagnado, D. A. , & Channon, S. ( 2008 ). Judgments of cause and blame: the effects of intentionality and foreseeability. Cognition , 108 (3), 754–70.
Legare, C. H. ( 2012 ). Exploring explanation: Explaining inconsistent evidence informs exploratory, hypothesis-testing behavior in young children. Child Development , 83 (1), 173–85.
Legare, C. H. , & Lombrozo, T. ( 2014 ). Selective effects of explanation on learning during early childhood. Journal of Experimental Child Psychology , 126 , 198–212.
Legare, C. H. , Wellman, H. M. , & Gelman, S. A. ( 2009 ). Evidence for an explanation advantage in naïve biological reasoning. Cognitive Psychology , 58 (2), 177–94.
Lipton, P. ( 2004 ). Inference to the best explanation . London: Routledge.
Lombrozo, T. ( 2007 ). Simplicity and probability in causal explanation. Cognitive Psychology , 55 (3), 232–257.
Lombrozo, T. ( 2009 ). Explanation and categorization: How “why?” informs “what?.” Cognition , 110 (2), 248–53.
Lombrozo, T. ( 2010 ). Causal-explanatory pluralism: How intentions, functions, and mechanisms influence causal ascriptions. Cognitive Psychology , 61 (4), 303–32.
Lombrozo, T. ( 2012 ). Explanation and abductive inference. In Oxford handbook of thinking and reasoning (pp. 260–276). Oxford: Oxford University Press.
Lombrozo, T. ( 2016 ). Explanatory preferences shape learning and inference. Trends in Cognitive Sciences , 20 , 748–759.
Lombrozo, T. , & Carey, S. ( 2006 ). Functional explanation and the function of explanation. Cognition , 99 (2), 167–204.
Lombrozo, T. , & Gwynne, N. Z. (2014). Explanation and inference: Mechanistic and functional explanations guide property generalization. Frontiers in Human Neuroscience , 8 (September), 700.
Lombrozo, T. , & Rehder, B. ( 2012 ). Functions in biological kind classification. Cognitive Psychology , 65 (4), 457–485.
Machamer, P. , Darden, L. , & Craver, C. F. ( 2000 ). Thinking about mechanisms. Philosophy of Science , 67 (1), 1–25.
Malle, B. F. ( 1999 ). How people explain behavior: A new theoretical framework. Personality and Social Psychology Review , 3 (1), 23–48.
Malle, B. F. ( 2004 ). How the mind explains behavior: Folk explanations, meaning, and social interaction . Cambridge, MA: MIT Press.
Malle, B. F. , Knobe, J. , O’Laughlin, M. J. , Pearce, G. E. , & Nelson, S. E. ( 2000 ). Conceptual structure and social functions of behavior explanations: Beyond person-situation attributions. Journal of Personality and Social Psychology , 79 (3), 309–326.
Mansinghka, V. K. , Kemp, C. , Tenenbaum, J. B. , & Griffiths, T. L. (2006). Structured priors for structure learning. Proceedings of the Twenty-Second Conference on Uncertainty in Artificial Intelligence (UAI 2006).
McArthur, L. A. ( 1972 ). The how and what of why: Some determinants and consequences of causal attribution. Journal of Personality and Social Psychology , 22 (2), 171–193.
McClure, J. , Hilton, D. J. , & Sutton, R. M. ( 2007 ). Judgments of voluntary and physical causes in causal chains: Probabilistic and social functionalist criteria for attributions. European Journal of Social Psychology , 37 , 879–901.
McGill, A. L. ( 1989 ). Context effects in judgments of causation. Journal of Personality and Social Psychology , 57 (2), 189–200.
Medin, D. L. , & Ortony, A. ( 1989 ). Psychological essentialism. In S. Vosniadou & A. Ortony (Eds.), Similarity and Analogical Reasoning (pp. 179–195). Cambridge: Cambridge University Press.
ojalehto, b. , Waxman, S. R. , & Medin, D. L. ( 2013 ). Teleological reasoning about nature: Intentional design or relational perspectives? Trends in Cognitive Sciences , 17 (4), 166–171.
Pacer, M. , & Lombrozo, T. ( 2015 ). Ockham’s Razor cuts to the root: simplicity in causal explanation. Manuscript in revision.
Pacer, M. , Williams, J. J. , Chen, X. , Lombrozo, T. , & Griffiths, T. L. ( 2013 ). Evaluating computational models of explanation using human judgments. In A. Nicholson & P. Smyth (Eds.), Proceedings of the Twenty-Ninth Conference on Uncertainty in Artificial Intelligence (pp. 498–507). Corvallis, Oregon: AUAI Press.
Park, J. , & Sloman, S. A. ( 2014 ). Causal explanation in the face of contradiction. Memory & Cognition , 42 (5), 806–820.
Park, J. , & Sloman, S. A. ( 2013 ). Mechanistic beliefs determine adherence to the Markov property in causal reasoning. Cognitive Psychology , 67 (4), 186–216.
Peirce, C. S. ( 1955 ). Abduction and induction. In Philosophical writings of Peirce (Vol. 11). New York: Dover.
Pennington, N. , & Hastie, R. ( 1992 ). Explaining the evidence: Tests of the Story Model for juror decision making. Journal of Personality and Social Psychology , 62 (2), 189–206.
Perales, J. C. , & Shanks, D. R. ( 2003 ). Normative and descriptive accounts of the influence of power and contingency on causal judgement. The Quarterly Journal of Experimental Psychology. A: Human Experimental Psychology , 56 (6), 977–1007.
Pine, K. J. , & Siegler, R. S. (2003). The role of explanatory activity in increasing the generality of thinking. Paper presented at the biennial meeting of the Society for Research in Child Development, Tampa, FL.
Potochnik, A. ( 2010 ). Levels of explanation reconceived. Philosophy of Science , 77 (1), 59–72.
Prasada, S. , & Dillingham, E. M. ( 2006 ). Principled and statistical connections in common sense conception. Cognition , 99 (1), 73–112.
Prasada, S. , & Dillingham, E. M. ( 2009 ). Representation of principled connections: A window onto the formal aspect of common sense conception. Cognitive Science , 33 (3), 401–48.
Preston, J. , & Epley, N. ( 2005 ). Explanations versus applications: The explanatory power of valuable beliefs. Psychological Science , 16 (10), 826–832.
Putnam, H. ( 1975 ). Philosophy and our mental life. In H. Putnam , Mind, Language and Reality: Philosophical Papers (Vol. 2). New York: Cambridge University Press.
Railton, P. ( 1978 ). A deductive-nomological model of probabilistic explanation. Philosophy of Science , 44 (2), 206–226.
Read, S. J. , & Marcus-Newhall, A. ( 1993 ). Explanatory coherence in social explanations: A parallel distributed processing account. Journal of Personality and Social Psychology , 65 (3), 429.
Rips, L. J. , & Edwards, B. J. ( 2013 ). Inference and explanation in counterfactual reasoning. Cognitive Science , 37 (6), 1107–35.
Rozenblit, L. , & Keil, F. ( 2002 ). The misunderstood limits of folk science: An illusion of explanatory depth. Cognitive Science , 26 , 521–562.
Salmon, W. ( 1984 ). Scientific explanation and the causal structure of the world . Princeton, NJ: Princeton University Press.
Schupbach, J. N. ( 2011 ). Comparing probabilistic measures of explanatory power. Philosophy of Science , 78 (5), 813–829.
Schupbach, J. N. , & Sprenger, J. ( 2011 ). The logic of explanatory power. Philosophy of Science , 78 (1), 105–127.
Shanks, D. R. , & Dickinson, A. ( 1988 ). Associative accounts of causality judgment. Psychology of Learning and Motivation: Advances in Research and Theory , 21 (C), 229–261.
Siegler, R. S. ( 1995 ). How does change occur: A microgenetic study of number conservation. Cognitive Psychology , 28 , 225–273.
Sloman, S. A , & Lagnado, D. ( 2014 ). Causality in thought. Annual Review of Psychology , 66 , 223–247.
Spellman, B. A. ( 1997 ). Crediting causality. Journal of Experimental Psychology: General , 126 (4), 323–348.
Thagard, P. ( 1989 ). Explanatory coherence. Behavioral and Brain Sciences , 12 , 435–502.
van Fraassen, B. C. ( 1980 ). The scientific image . Oxford University Press.
Vasilyeva, N. , & Coley, J.C. ( 2013 ). Evaluating two mechanisms of flexible induction: Selective memory retrieval and evidence explanation. In M. Knauff , M. Pauen , N. Sebanz , & I. Wachsmuth (Eds.), Proceedings of the 35th annual conference of the Cognitive Science Society (pp. 3645–3650). Austin, TX: Cognitive Science Society.
Vasilyeva, N. , & Lombrozo, T. ( 2015 ). Explanation and causal judgments are differentially sensitive to covariation and mechanism information. In Proceedings of the 37th annual conference of the Cognitive Science Society (pp. 2663–2668). Austin, TX: Cognitive Science Society.
Waldmann, M. R. , & Hagmayer, Y. ( 2001 ). Estimating causal strength: The role of structural knowledge and processing effort. Cognition , 82 (1), 27–58.
Waldmann, M. R. , & Hagmayer, Y. ( 2013 ). Causal reasoning. In D. Reisberg (Ed.), Oxford handbook of cognitive psychology (pp. 733–752). New York: Oxford University Press.
Walker, C. M. , Lombrozo, T. , Legare, C. H. , & Gopnik, A. ( 2014 ). Explaining prompts children to privilege inductively rich properties. Cognition , 133 (2), 343–57.
Walker, C.M. , Lombrozo, T. , Williams, J. J. , Rafferty, A. , & Gopnik, A. ( 2016 ). Explaining constrains causal learning in childhood. Child Development .
Weiner, B. ( 1985 ). An attributional theory of achievement motivation and emotion. Psychological Review , 92 (4), 548–573.
Wellman, H. M. ( 2011 ). Reinvigorating explanations for the study of early cognitive development. Child Development Perspectives , 5 (1), 33–38.
Wellman, H. M. , & Lagattuta, K. H. ( 2004 ). Theory of mind for learning and teaching: The nature and role of explanation. Cognitive Development , 19 , 479–497.
Wellman, H. M. , & Liu, D. ( 2007 ). Causal reasoning as informed by the early development of explanations. In A. Gopnik & L. Schulz (Eds.), Causal Learning: Psychology, Philosophy, and Computation (pp. 261–279). Oxford: Oxford University Press.
Wilkenfeld, D. A. , & Lombrozo, T. ( 2015 ). Infernece to the Best Explanation (IBE) vs. Explaining for the Best Inference (EBI). Science and Education , 24 (9–10), 1059–1077.
Williams, J. J. , & Lombrozo, T. ( 2010 ). The role of explanation in discovery and generalization: Evidence from category learning. Cognitive Science , 34 (5), 776–806.
Williams, J. J. , & Lombrozo, T. ( 2013 ). Explanation and prior knowledge interact to guide learning. Cognitive Psychology , 66 (1), 55–84.
Williams, J. J. , Lombrozo, T. , & Rehder, B. ( 2013 ). The hazards of explanation: Overgeneralization in the face of exceptions. Journal of Experimental Psychology. General , 142 (4), 1006–14.
Woodward, J. ( 2010 ). Causation in biology: Stability, specificity, and the choice of levels of explanation. Biology & Philosophy , 25 (3), 287–318.
Wright, L. ( 1976 ). Teleological explanations: An etiological analysis of goals and functions . Berkeley: University of California Press.
- About Oxford Academic
- Publish journals with us
- University press partners
- What we publish
- New features
- Open access
- Institutional account management
- Rights and permissions
- Get help with access
- Accessibility
- Advertising
- Media enquiries
- Oxford University Press
- Oxford Languages
- University of Oxford
Oxford University Press is a department of the University of Oxford. It furthers the University's objective of excellence in research, scholarship, and education by publishing worldwide
- Copyright © 2024 Oxford University Press
- Cookie settings
- Cookie policy
- Privacy policy
- Legal notice
This Feature Is Available To Subscribers Only
Sign In or Create an Account
This PDF is available to Subscribers Only
For full access to this pdf, sign in to an existing account, or purchase an annual subscription.
Start Writing a Causal Analysis Essay
- Essay Writing Guides
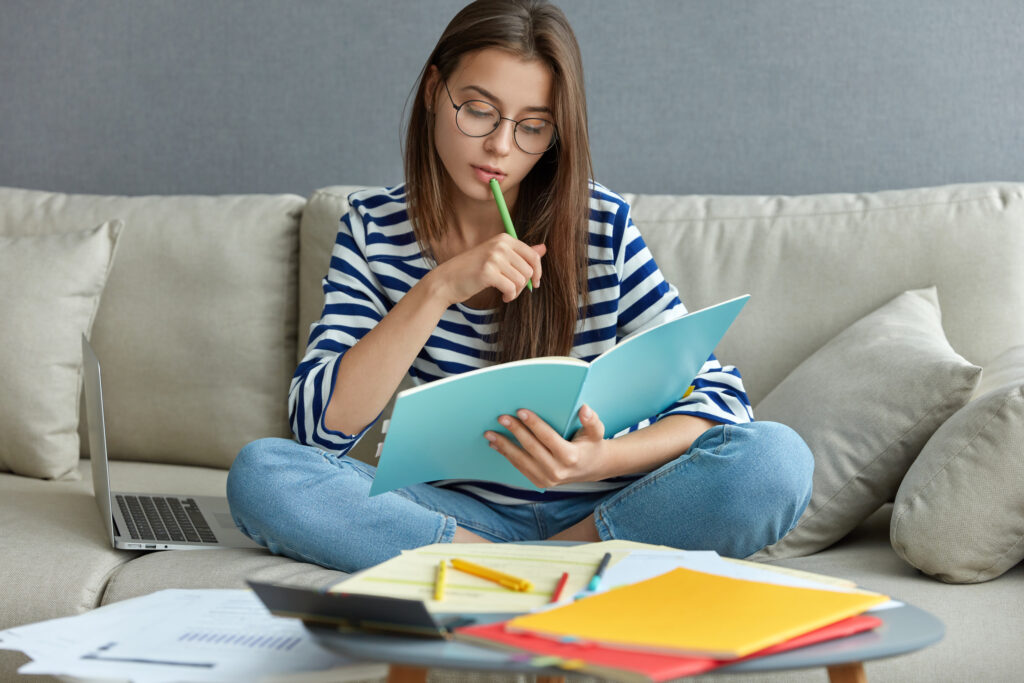
A causal analysis essay is a research paper that examines the causes and effects of a particular phenomenon, event, or trend. It aims to uncover the relationships between various factors and their outcomes, providing insight into complex issues. It typically involves critical thinking, analysis, and logical reasoning to explore causality. A causal analysis essay example includes examining the causes of obesity, the effects of climate change, or the reasons behind economic fluctuations.
Writing a causal analysis paper is crucial for clear communication, honing analytical skills, and fostering academic and professional development. Our article offers a comprehensive step-by-step guide to writing a causal analysis essay , providing practical tips and strategies. The guide emphasizes practicality, offering actionable advice that writers can apply to enhance their writing skills. The guide aims to empower writers to tackle causal analysis essays with confidence, equipping them with the necessary tools and knowledge to write proficiently.
Definition of Causal Analysis Essay
Causal analysis essays are academic writings that examine the causal relationships between factors or events, aiming to explain why certain outcomes occur. They analyze complex phenomena, identify underlying causes and effects, explain the connections between elements and their impact on outcomes, and illuminate patterns by examining causality. They prioritize analytical thinking and critical evaluation of causation, providing an objective analysis without advocating for a specific viewpoint.
Understanding causality allows for targeted interventions, better planning and decision-making, and the development of critical thinking skills. They also foster intellectual growth, empowering individuals to engage more deeply with complex issues in various disciplines. After answering your question, “ What is a causal analysis essay?” let’s understand how to write it properly.
Pre-Writing Stage
The pre-writing stage involves selecting relevant topics for causal analysis essays , conducting thorough research, and organizing and analyzing data. This involves choosing a topic that aligns with your interests, is significant in your field, and is narrowed down to a specific aspect. Thorough research involves identifying reliable sources, diversifying perspectives, and critically evaluating them. Keeping detailed notes of key findings and arguments helps in later analysis. The organization and analysis of data involve creating an outline, analyzing causality, prioritizing relevant information, and refining the thesis statement. This ensures your essay is specific, debatable, and supported by evidence.
Causal Analysis Essay Topics
A causal analysis essay provides an opportunity to explore the underlying reasons behind various phenomena. Below are some compelling causal essay topics:
- Impact of Social Media on Adolescents: Examine the impact of social media use on mental health.
- Causes of Climate Change on Global Weather Patterns: Investigate the factors contributing to climate change and its global weather effects.
- Effects of Parental Divorce on Children’s Emotional Well-being: Analyze the emotional consequences of parental divorce.
- Causes of Income Inequality in Modern Societies: Investigate the factors contributing to income disparities.
- Impact of Technology on Human Communication Skills: Explore how technology affects interpersonal relationships and communication abilities.
- Factors Contributing to the Rise of Obesity Rates in Developed Countries: Examine the factors driving the rise in obesity prevalence.
- Effects of Video Games on Behavior in Adolescents: Investigate the potential link between exposure to violent video games and aggressive behavior.
- Consequences of the Gender Pay Gap in the Workplace: Analyze the reasons behind the gender wage disparity.
These causal essay topic ideas offer rich avenues for analysis, allowing for a deeper understanding of complex societal issues and their underlying dynamics.
Causal Analysis Essay Outline
Let’s discuss what causal analysis essay outline should include.
- Introduction: Hook/attention grabber, background information on the causal argument topic , thesis statement.
- Body Paragraphs: Identifying causes, analyzing effects, establishing connections.
- Conclusion: Restate the thesis, summary of key points, final thoughts, and references.
This causal essay outline provides a structured approach to writing a causal analysis essay, guiding the writer through the process of identifying causes, analyzing effects, establishing connections, and drawing conclusions based on the analysis conducted.
Causal Analysis Essays Writing Process
The writing process involves crafting a strong thesis statement, which should be clear, specific, and reflective of the causal relationship being explored. It should also be open to debate, encouraging critical engagement from readers.
The causal analysis essay format includes an introduction, body paragraphs, identifying causes, analysis effects, establishing connections, comparative analysis, synthesizing causal factors, and ensuring a logical progression from one paragraph to the next. The conclusion should restate the statement, summarise key points, and offer final thoughts on the broader implications of the analysis.
Incorporating evidence and examples is crucial, and it should be relevant and reliable. Use credible sources, such as scholarly articles, statistics, and expert opinions. Incorporate a variety of evidence, including quantitative data, case studies, anecdotes, and real-life examples, to provide depth and context on how to write a causal analysis essay . Analyze the evidence thoroughly, explaining its relevance to the argument and interpreting its implications for the causal relationships being examined.
Citation and attribution are also essential, as proper citation ensures credit to the original authors and avoids plagiarism. For example, the rising prevalence of social media addiction among teenagers can be attributed to excessive screen time, peer pressure, and the dopamine-driven feedback loop created by ‘likes’ and notifications.
Tips and Tricks
Practical causal analysis essay writing tips include using clear and concise language, defining key terms, removing redundant words, providing examples, and utilizing transitions effectively. Identifying common logical fallacies, evaluating arguments for flaws or weaknesses, providing evidence and reasoning, and seeking feedback is essential for improving the overall quality of your essay.
To revise and proofread, take breaks, focus on different aspects, read aloud, and use tools like spelling and grammar checkers, style guides, and writing handbooks. Listen for awkward phrasing, unclear sentences, or grammatical errors. Seek feedback from mentors to gain additional insights and perspectives on your writing.
Causal Analysis Essay Example and Illustration
Causal analysis essay examples are essential tools for analyzing causal relationships and understanding the underlying mechanisms driving phenomena. These essays can be found in academic journals, textbooks, or online writing repositories. The structure of each essay, including the introduction, body paragraphs, and conclusion, is crucial for presenting causal relationships. Evidence, such as statistical data, case studies, expert opinions, or anecdotal evidence, is also essential. The causal chains presented in essays are crucial for understanding the connections between causes and effects.
Effective writing techniques, such as persuasive language, descriptive imagery, or logical reasoning, are employed in the sample essays. The effectiveness of each technique is evaluated to engage the reader, convey the author’s message, and support the overall argument. Comparing the writing techniques used in different sample essays helps identify patterns and trends in effective causal analysis writing.
Afterthoughts on Writing Causal Essays
In summary, developing the ability to write a causal analysis essay is an important life skill that not only improves academic performance but also develops the critical thinking and analytical reasoning needed to handle challenging situations in different contexts.
To unravel causal relationships, shed light on the complexities of our world, and make significant contributions to knowledge and understanding, writers can confidently set out on their journey by adhering to the helpful advice provided in this guide and finding inspiration from sample essays and successful writing techniques. Thus, take on the task of writing a causal analysis essay with diligence, inventiveness, and a dedication to perfection, regardless of whether you’re a professional looking to hone your analytical skills or a student hoping to succeed academically.
- Academic Writing Guides
- Citation Guides
- Essay Samples
- Essay Topics
- Research Paper Topics
- Research Paper Writing Guides
- Study Tips and Tricks
Featured articles
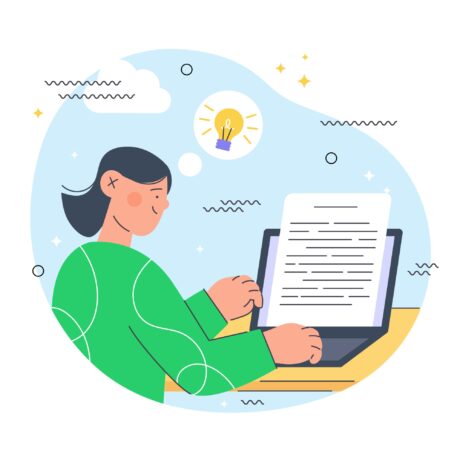
Strategies for Writing a Great Problem Solution Essay
Composing an assignment on a problem and solution essay topic allows you to demonstrate your ability to resolve human challenges. It helps you understand human problems and how to be a part of the solution instead of allocating blame and pointing fingers. So, how do you compose a great problem solutions essay? This post has […]
Author: Marina Kean
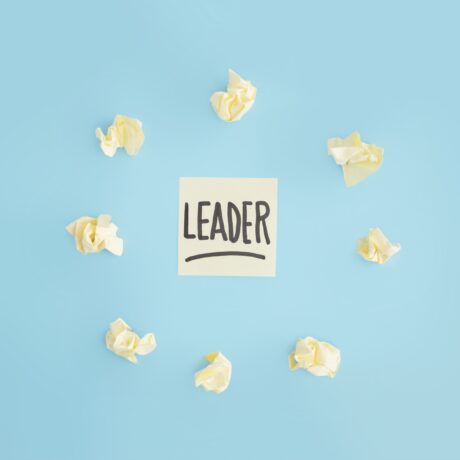
Your Complete Guide to Writing a Compelling Leadership Essay
True leadership lies at the heart of human well-being and success. Every positive step humanity ever took required great and responsible leadership. Therefore, people have studied leadership for many edges to unravel its different underlying factors. But how do you write a great essay that demonstrates your grasp of this sacred call? This post shares […]
How to Write a Causal Analysis Essay and Master Cause and Effect

If you find yourself grappling with the task of writing your essay, fear not. We're here to assist you through the process with a comprehensive guide from our essay writing services . This resource is meticulously crafted to ensure clarity and effectiveness, offering you valuable insights into starting and completing your essay efficiently. Dive into the guide, follow the steps, and conquer your essay with confidence.
.png)
Check out another articles: cause and effect essay , and how to write an analytical essay at EssayHub blog.
What Is Causal Analysis Essay?
Causal analysis essay writing explores the cause-and-effect relationships inherent in a particular subject or phenomenon. In essence, it delves into the reasons behind an occurrence and its subsequent outcomes. The primary goal is to scrutinize the connections between various elements, shedding light on how one factor influences another.
In simpler terms, imagine investigating the roots of a specific event or situation and examining the ripple effects it generates. This analytical process involves identifying the contributing factors and understanding how they interconnect to produce a particular result.
For instance, if the chosen essay topic is the impact of technology on education, the essay would dissect the various aspects of technology (the causes) and elucidate how they shape, enhance, or perhaps impede the educational system (the effects). Whether you choose to send us your ' write an essay for me ' request or tackle it yourself, it's important to know that it's essentially a methodical exploration into the 'why' and 'how' behind a given phenomenon, offering a deeper understanding of the relationships at play.
Causal Analysis Essay Outline
Crafting a roadmap, or what we like to call a causal essay outline, can be a game-changer for your writing journey. Having one makes the writing process smoother and even a bit speedier; everything just falls into place more seamlessly when you know where you're headed.
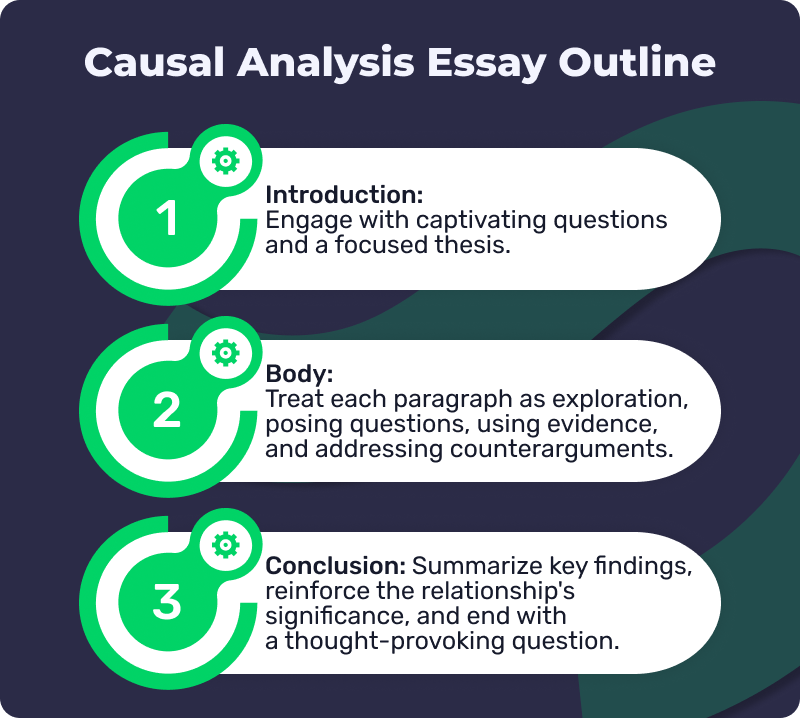
Introduction
In this initial phase, consider framing the causal analysis essay introduction as a captivating entry point, similar to opening the door to a thought-provoking space. Are there questions that can intrigue your reader, sparking curiosity about the phenomena under scrutiny?
Craft a thesis statement that serves as a compass, providing direction for the forthcoming analysis. How might this thesis encapsulate the essence of the cause-and-effect relationship to be unraveled?
Transitioning into the causal analysis essay body, envision each paragraph as a point of exploration, similar to uncovering layers of complex terrain. Can you pose questions that guide the reader through the nuanced aspects of the cause-and-effect relationship? Start each paragraph with a topic sentence that acts as a waypoint, introducing a distinct facet of the phenomenon.
How can you substantiate these points with evidence and examples, ensuring a comprehensive examination? Consider incorporating counterarguments—what potential detours in understanding might merit acknowledgment and resolution?
As the essay culminates, view the causal analysis essay conclusion as a reflective summit, offering panoramic views of the explored landscape. How can you succinctly recapitulate the key findings without introducing novel elements? Reinforce the significance of the cause-and-effect relationship—what enduring insights should linger in the reader's contemplation? Conclude with a thought-provoking query, inviting the reader to ponder the implications of the journey.
5 PRO Tips on How to Write a Causal Analysis Essay
Alright, let's dive into the nitty-gritty of crafting a top-notch essay. Here are five practical tips to make writing causal analysis essay a breeze:
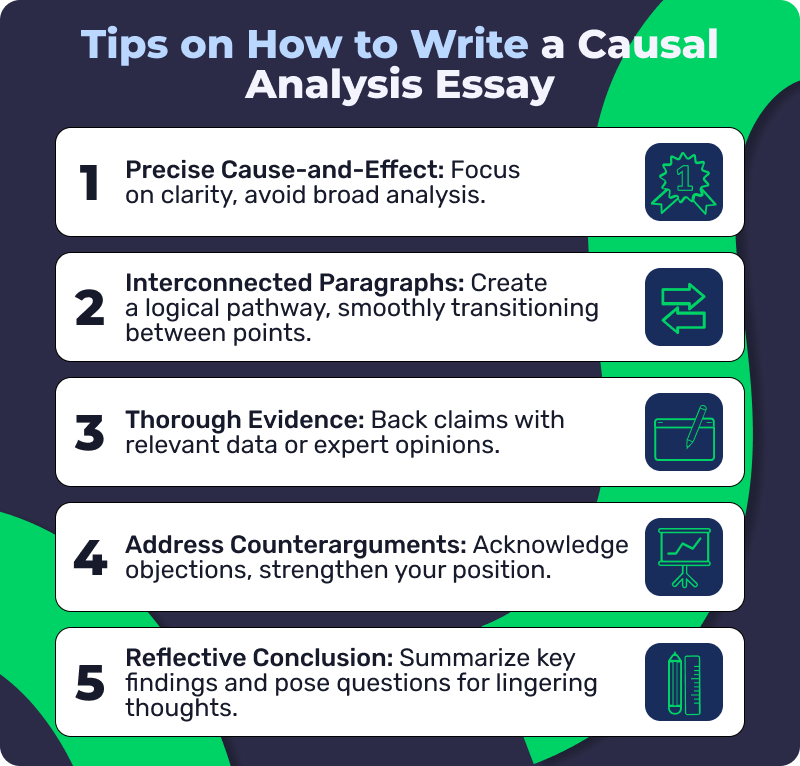
1. Precision in Cause-and-Effect Selection : Start by pinpointing the exact cause-and-effect relationship you want to explore. It's like aiming a spotlight on a specific intersection of events. The more precise you are, the clearer your analysis becomes. Don't cast too wide a net; focus on a particular aspect to dissect thoroughly.
2. Interconnected Paragraphs : Think of your causal analysis example as a chain reaction—each paragraph should seamlessly connect to the next. Begin with a strong cause or effect statement, then smoothly transition to the next point. It's like creating a logical pathway for your readers, guiding them through your analysis step by step. Avoid abrupt jumps; make it a smooth journey.
3. Thorough Evidence Exploration : Back up your cause-and-effect claims with solid evidence. Dig into data, examples, or expert opinions that reinforce your points. It's similar to building a case – the stronger your evidence, the more convincing your analysis becomes. Ensure your evidence is directly related to the cause-and-effect relationship under scrutiny.
4. Anticipate and Address Counterarguments : Don't shy away from potential objections. It's like preparing for a friendly debate—acknowledge counterarguments and demonstrate why your analysis holds water. This not only strengthens your position but also showcases a comprehensive understanding of the subject. Consider it an opportunity to showcase the depth of your analysis.
5. Reflective Conclusion : Wrap it up with a reflective conclusion that echoes the essence of your cause-and-effect exploration. Summarize your key findings without introducing new elements—it's like tying a neat bow on your analysis. Pose questions or suggest implications to leave your reader pondering. Make it a conclusion that lingers in their thoughts after they've finished reading.
Causal Analysis Essay Topics: An In-Depth Compilation
Here, we've curated an extensive list of causal analysis essay topic ideas, thoughtfully organized into different categories. This ensures that students, depending on their field of study, can swiftly pinpoint topics that resonate with their academic pursuits. Our diverse selection guarantees an array of engaging essay argument topics for every interest.
Environmental Issues
- The Effect of Urban Green Spaces on Mitigating Heat Islands
- Analyzing the Causal Factors Behind the Decline of Bee Populations
- Investigating the Impact of Microplastics on Freshwater Ecosystems
- The Role of Deforestation in the Spread of Infectious Diseases
- Examining the Causal Links Between Air Pollution and Respiratory Health in Urban Areas
- The Impact of Light Pollution on Nocturnal Wildlife Behavior
- Assessing the Influence of Land Use Changes on Local Climate Patterns
- Causal Analysis of the Relationship Between Pesticide Use and Decline in Pollinator Populations
- Exploring the Connection Between Household Waste Management Practices and Environmental Sustainability
- The Role of Industrial Chemicals in Coral Bleaching Events
Technology and Society
- Analyzing the Impact of Social Media on Political Polarization
- Causal Links Between Online Gaming and Its Influence on Academic Performance
- Investigating the Role of Technology in Shaping Modern Dating Trends
- The Effect of E-books on Traditional Publishing Industries
- Examining the Causal Relationship Between Screen Time and Mental Health in Adolescents
- Assessing the Impact of Artificial Intelligence on Job Market Dynamics
- The Influence of Smartphone Use on Interpersonal Communication Skills
- Analyzing the Causal Factors Behind the Digital Gender Divide
- The Effect of Wearable Fitness Technology on Personal Health and Behavior
- Investigating the Relationship Between Online Learning Platforms and Educational Inequality
Education and Learning
- The Impact of Virtual Reality in Enhancing Classroom Engagement
- Analyzing the Causal Factors Behind the Gender Gap in STEM Education
- Investigating the Influence of Outdoor Education on Academic Performance
- Examining the Relationship Between Teacher Expectations and Student Achievement
- The Effect of Inclusive Education Practices on Social Development
- Assessing the Impact of Standardized Testing on Curriculum Design
- Causal Links Between Parental Involvement and Student Motivation
- The Role of Early Childhood Education in Preventing Learning Disabilities
- Exploring the Influence of Technology in Transforming Learning Environments
- Analyzing the Causal Factors Behind Educational Inequality in Urban Settings
Economics and Finance
- The Effect of Cryptocurrency Adoption on Traditional Banking Systems
- Investigating the Causal Relationship Between Income Inequality and Economic Growth
- Analyzing the Impact of Global Supply Chain Disruptions on Local Economies
- The Role of Government Policies in Shaping Economic Stability
- Examining the Causal Links Between Consumer Behavior and Economic Trends
- Assessing the Influence of Entrepreneurship on Job Creation and Economic Development
- The Effect of Financial Education Programs on Personal Savings Habits
- Investigating the Causal Factors Behind Economic Recessions
- Analyzing the Relationship Between Corporate Social Responsibility and Financial Performance
- The Impact of Technological Advancements on the Gig Economy
Psychology and Human Behavior
- The Causal Links Between Childhood Trauma and Adult Attachment Styles
- Investigating the Influence of Social Media on Body Image and Self-Esteem
- Analyzing the Impact of Parenting Styles on Adolescent Mental Health
- Examining the Relationship Between Sleep Deprivation and Cognitive Functioning
- The Effect of Social Isolation on the Development of Anxiety and Depression
- Assessing the Causal Factors Behind the Rise in Smartphone Addiction
- The Influence of Cultural Background on Personality Traits: A Causal Analysis
- Analyzing the Relationship Between Music Preferences and Emotional Well-being
- Investigating the Impact of Exercise on Stress Reduction and Mental Health
- Causal Links Between Early Attachment Patterns and Adult Relationship Satisfaction
Causal Analysis Essay Example
As we've touched upon earlier, a cause and effect essay involves making a claim and supporting it with solid facts and arguments. Good causal analysis essay examples in this genre link various issues with their underlying problems, providing a comprehensive understanding. Let's jump into a sample essay to find out the ins and outs of how sleep impacts our academic journey.

How to End a Causal Analysis Essay Effectively?
What is the structure of a causal essay, how do you start analysis essay, what is a causal analysis essay example, what is a causal analysis essay.

- Plagiarism Report
- Unlimited Revisions
- 24/7 Support
Opening statements in Trump's historic trial set to begin Monday after tense day of jury selection
Opening statements are set to begin next week in Donald Trump’s historic criminal trial after the final members of the jury were seated Friday, following a dramatic day in which two prospective jurors broke down in tears, an appeals court judge rejected Trump's request for a stay, and a man set himself on fire in front of the courthouse.
“We’re going to have opening statements on Monday morning. This trial is starting,” Judge Juan Merchan said towards the end of the day, after successfully seating the remaining five alternate jurors that were needed.
The case — the first-ever criminal trial of a former president —will be heard by a panel of 12 jurors and a total of six alternates. It's expected to last roughly six weeks.
The five alternates ultimately selected Friday include an unemployed married woman who’s into art and described herself as not political, an audio professional, a contract specialist, a clothing company executive and a construction company project manager. It took four days of jury selection to find the 18 jurors.
Around the same time the judge declared, "we have our full panel" inside the courtroom in the early afternoon, a man set himself on fire outside the courthouse. The NYPD said the man, identified as Max Azzarello of Florida, later died. He appeared to have had pamphlets describing a conspiracy involving cryptocurrency that he threw around before setting himself ablaze, police said.
Later in the afternoon, Trump's attorneys were in a state appeals court trying again to get an emergency stay of the trial. Trump attorney Cliff Robert argued his client could not get a fair trial in Manhattan, which had been Trump's longtime home before moving to Florida after he was elected president in 2016.
Steven Wu of Manhattan District Attorney Alvin Bragg's office countered that "what the last week has shown is that the jury selection has worked."
"We have 18 ordinary New Yorkers who are ready to serve. It would be unfair to them and the public for this to be delayed further," he argued. The judge rejected Trump's stay request a short time later.
The jury selection process Friday was especially intense, some potential jurors breaking down in tears and others saying they were too anxious to serve.
The day began with the judge calling up the 22 remaining potential jurors from the previous pool of 96 to answer questions designed to indicate whether they could be fair and impartial about the divisive real estate mogul and presumptive Republican nominee for president.
The first of those potential jurors was dismissed after she said she didn’t think she could be fair. “I have really, really bad anxiety and people have found out where I am,” she told the judge. A short time later, two other potential jurors were dismissed after each told the judge that upon further reflection, “I don’t think I can be impartial.”
Other potential jurors included a married father who said he listens to a podcast called “Order of Man,” which is described on Apple’s website as discussions about “reclaiming what it means to be a man.” Some past guests of the podcast include people who’ve been outspoken in their support of Trump and were highly critical of the civil fraud case New York Attorney General Letitia James brought against the former president. The man, an audio specialist, was chosen as one of the alternates.
Another potential juror was a married fund manager who said he’d done “get-out-the-vote” work for former Secretary of State Hillary Clinton, Trump’s 2016 presidential opponent. Trump and his attorney Todd Blanche passed notes back and forth while that juror was speaking. He was later dismissed after being asked about a 2020 Facebook post where he apparently called Trump “the devil and a sociopath.”
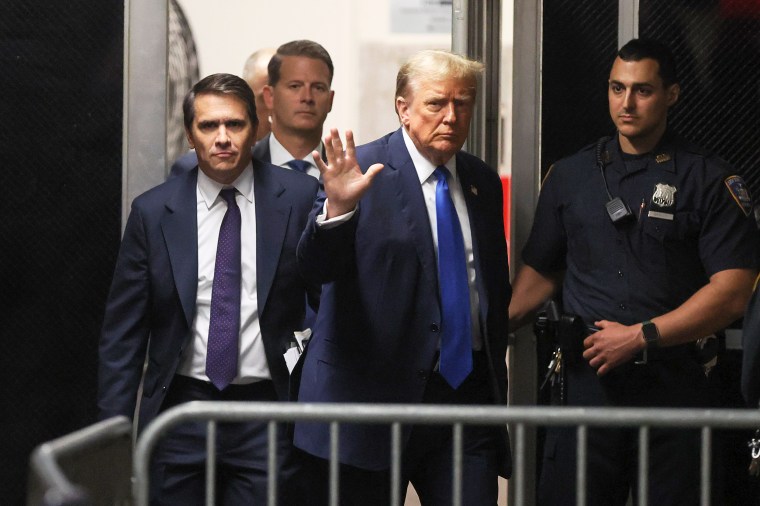
Trump appeared most interested in jurors whose answers offer ambiguity around their personal political views. When one prospective juror said they were a Fox News viewer, Trump cocked his head, then quickly conferred with his lawyer, Todd Blanche.
Another potential juror was a woman who became emotional as she disclosed she'd served two years in prison on drug-related charges, but said she could be "fair and impartial."
During a morning break, Merchan — who'd chided reporters on Thursday for disclosing too much information about potential jurors — said the woman had shared "very personal things about her life" and was "very brave." “I just wanted to encourage the press to please be kind. Please be kind to this person,” the judge said. He later dismissed her, saying she needed a certificate of release to be qualified for service going forward. On her way out, she cheerfully called out, "Good luck!"
Following that juror's departure, the DA's office began its individual questioning of the jurors. One woman, who'd disclosed that her father is lifelong friends with Trump ally turned critic Chris Christie, broke down in tears when prosecutor Susan Hoffinger asked her an innocuous question about the burden of proof in the case. "I feel so nervous and anxious right now. I’m sorry," she responded, bursting out into tears. "I thought I could do this," she said, adding "I wouldn’t want someone who feels this way to judge my case." She was dismissed.
Hoffinger's questioning was followed by Trump attorney Susan Necheles, who asked a potential juror who'd started their own business how she would assess a witness's credibility. The woman then asked to speak to the judge, saying she was "getting anxiety and self-doubt” from Necheles's line of questioning. She was dismissed.
Necheles later asked another woman — who previously said she was a victim of sexual assault — whether she would hold it against Trump that women outside this case have accused Trump of sexual assault. She said she would not have a problem setting those accusations aside but the judge ultimately excused her, saying, "It’s best to err on the side of caution."
Another man said he has some differences from Trump on his policies but thinks he's “usually awesome.” He was not chosen for the jury.
On his way into court in the morning, Trump again complained the case against him is "unfair," and that the partial gag order preventing him from lashing out at witnesses, prosecutors, court staffers and jurors is not "constitutional." "Everyone else can say whatever they want about me. They can say anything they want. They can continue to make up lies and everything else. They lie. They’re real scum. But you know what? I’m not allowed to speak," he told reporters.
Prosecutors this week asked the judge to fine Trump and hold him in contempt for social media posts that they said violate the gag order. A hearing on the matter is scheduled for Tuesday.
The m a in pa nel of 12 is made up of seven men and five women, including two lawyers, a teacher, a retired wealth manager, a product development manager, a security engineer, a software engineer, a speech therapist and a physical therapist. The foreman — the juror who essentially acts as the leader and spokesperson for the panel — is a married man who works in sales and gets his news from The New York Times, MSNBC and Fox News.
The lone alternate selected Thursday is a woman who works as an asset manager.
Trump vented about the speed of the process in a post on social media shortly after the final jurors were selected, claiming the judge is “‘railroading’ me, at breakneck speed, in order to completely satisfy his ‘friends’.”
Later in the day, Merchan held what's known as a Sandoval hearing . That's a type of hearing designed to let defendants know the scope of questions they could face from prosecutors on cross-examination so they can make informed decisions about whether to take the witness stand in their own defense.
Leaving court on Friday, Trump was asked whether he was still planning to testify and he said he was.
Manhattan District Attorney Alvin Bragg's office disclosed in a court filing that it would like to ask Trump about several items, among them the $464 million civil judgment against him and his company for fraud , the total $88 million verdicts and liability findings for sexual abuse and defamation in lawsuits brought by writer E. Jean Carroll and a number of other adverse court rulings over the past few years.
Trump has denied wrongdoing in all the cases and is appealing the fraud judgment and the Carroll verdicts.
Prosecutors said they want to be able to bring those findings up “to impeach the credibility of the defendant” if he takes the witness stand.
Discussing the findings in the fraud case, prosecutor Matthew Colangelo told the judge it was "hard to think of something that is more squarely in the wheelhouse” for the DA to ask Trump about "than a finding by a judge of persistent and repeated fraud and illegality."
Trump's attorney Emil Bove countered that prosecutors shouldn't be able to breach the topic at all because Trump's appeal is still pending. He made similar arguments over the DA's contention that they should be allowed to ask about a judge's finding that he was untruthful on the witness stand during the fraud trial and had violated a gag order in the case.
“Is it your position that because a case is being appealed or might be appealed, that therefore it can not be used?" Merchan asked the lawyer. "Not necessarily," Bove replied.
The judge said he'd issue his ruling on the dispute on Monday morning.
Trump said last week he “absolutely” plans to testify , but he is under no obligation to do so.
Asked by Necheles at the end of the day who the DA's first witness would be, prosecutor Joshua Steinglass said they wouldn't inform Trump's team of the person's identity until Sunday, given that Trump has been criticizing some witnesses on social media despite the partial gag order in the case. “And if that should be tweeted, that’ll be the last time we provide that courtesy,” Steinglass said.
Merchan called the DA's position "understandable" and told Necheles "I will not compel them to do anything."
Trump has pleaded not guilty to 34 counts of falsifying business records and faces up to four years in prison if he is convicted.
Bragg alleges that Trump falsified records to hide money he was paying his former lawyer Michael Cohen to reimburse him for $130,000 he paid adult film actor Stormy Daniels near the end of the 2016 presidential campaign. Daniels has claimed she had a sexual encounter with Trump in 2006. Trump has denied that he slept with Daniels, but he has acknowledged repaying Cohen.
The DA’s office also alleges that as part of a scheme to boost Trump, National Enquirer publisher American Media Inc. paid $150,000 to model and actor Karen McDougal , who appeared in Playboy magazine and claimed that she had a nine-month affair with Trump before he was elected president “in exchange for her agreement not to speak out about the alleged sexual relationship,” according to a statement of facts filed by Bragg.
Trump has also denied having a sexual relationship with McDougal.

Adam Reiss is a reporter and producer for NBC and MSNBC.

Lisa Rubin is an MSNBC legal correspondent and a former litigator.

Dareh Gregorian is a politics reporter for NBC News.
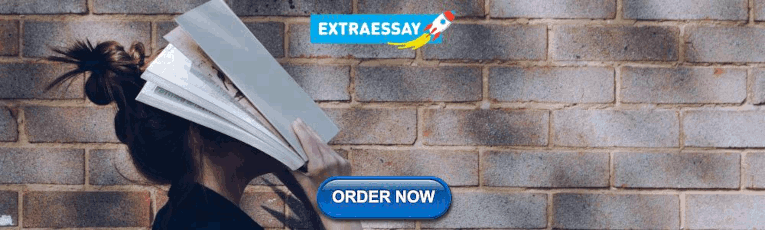
IMAGES
VIDEO
COMMENTS
A causal argument is an important argument type, as people are often looking for reasons as to why things have happened but may not be sure or have all of the necessary information. In your causal argument, you get the chance to make these things clear. ... End with a thesis statement that makes an assertion about causes or what led to something.
Because the causal argument can take several different forms, a single example of a causal argument can't illustrate organization for most of you. Consequently, this web page presents information and short samples of different approaches to causality, depending upon your thesis. For full-length samples of causal arguments, see Ramage and Bean's ...
Here he relies on experts to support the idea of causation.) Without action to control emissions, carbon dioxide might reach 0.1% of the atmosphere by 2100, more than triple the level before the Industrial Revolution. This would be a faster change than transitions in Earth's past that had huge consequences.
Sample annotated causal argument. The article "Climate Explained: Why Carbon Dioxide Has Such Outsized Influence on Earth's Climate" by Jason West, published in The Conversation, can serve as an example. Annotations point out how the author uses several causal argument strategies.
13. Key Concept. Causal Argument: argues that there is a cause and effect relationship between certain conditions. Sometimes the cause and effect relationship between certain things can seem really clear cut. For example, if someone drops a vase, and the vase hits the floor and breaks, obviously that happened because the person dropped it.
Causal explanations are useful as long as they are not confused with either arguments or excuses. 1. A causal claim says that one thing causes another; a hypothesis is an initial speculation about a causal claim. 2. Informal causal reasoning follows one of two main patterns: relevant difference and common thread.
However, you may want to avoid explicit thesis sentences and forecasting statements if your subject is likely to threaten the beliefs of your audience or if it is an inherently emotional subject. You may occasionally find it important to establish a credible persona first by reviewing what your readers are likely to believe about a causal ...
Attribution: Parts of this section on proposal arguments are original content by Anna Mills and Darya Myers. Parts were adapted from Technical Writing, which was derived in turn by Annemarie Hamlin, Chris Rubio, and Michele DeSilva, Central Oregon Community College, from Online Technical Writing by David McMurrey—CC BY 4.0.It has been further edited and re-mixed by Dr. Adam Falik and Dr ...
Nevertheless, there are a number of independent strands of research involving causation and argument. The goal of this chapter is to bring these currently separate bodies of work together in order to provide a coherent basis for future empirical research on causal argument that elucidates the dialectical and inferential role of cause-effect relationships in reasoned discourse.
Causal Arguments. Search for: Sample Causal Argument. Now that you have had the chance to learn about writing a causal argument, it's time to see what one might look like. Linked, you'll see a sample causal argument essay written following MLA formatting guidelines.
14.2: Organizing the Causal Analysis Essay. Page ID. The causal analysis essay can be split into four basic sections: introduction, body, conclusion, and Works Cited page. There are three basic formats for writing a cause/effect: Single effect with multiple causes-air pollution is the effect, and students would identify several causes;
Placement of the thesis statement. Step 1: Start with a question. Step 2: Write your initial answer. Step 3: Develop your answer. Step 4: Refine your thesis statement. Types of thesis statements. Other interesting articles. Frequently asked questions about thesis statements.
The argument essay should adhere to the following guidelines: The essay meets requirements for the type of argument it is intended to convey (definition argument, proposal argument, or causal argument). The essay is based on a clearly stated, arguable thesis statement. The thesis is appropriately developed and supported: the writer has provided ...
In most cases, it will need to make causal arguments about the roots of the problem and the good effects of the proposed solution. Common elements of proposal arguments Background on the problem, opportunity, or situation ... the claim you make in your thesis statement. This explanation can appear in one or more of the following forms ...
Thesis statement (State the central argument you will be discussing in your essay) II. Body paragraph 1. Topic sentence (State the strongest argument (the first cause) in support of your thesis) ... The statement should explain what your entire essay will be focusing on. A typical thesis statement for a causal analysis essay sounds like this ...
A causal chain can help you organize your essay and explain your thesis. If you have done the appropriate research and have found causality among events, you can see whether a causal chain has developed. A causal chain occurs when one event leads to a result and that result leads to another result.
Abstract. Explanation and causation are intimately related. Explanations often appeal to causes, and causal claims are often answers to implicit or explicit questions about why or how something occurred. This chapter considers what we can learn about causal reasoning from research on explanation.
1. Causal Argument What it does: Examines a cause and its effects, states an effect and traces the effect back to its causes, or debunks an existing cause-and-effect argument. Include in your thesis: Topic (cause OR effect) and statement of the effects OR the causes; or an existing argument and the reasons why it is invalid.
Let's discuss what causal analysis essay outline should include. Introduction: Hook/attention grabber, background information on the causal argument topic, thesis statement. Body Paragraphs: Identifying causes, analyzing effects, establishing connections. Conclusion: Restate the thesis, summary of key points, final thoughts, and references.
For example, if a chosen topic is harm of alcohol, then an argument is "Alcohol consumption (A) causes XYZ failure (B)" where A is a cause and B is an effect. ... In causal analysis essay, thesis statement should be one or two sentences that sum up main points of your paper. Thesis should not simply include certain statements, but show causal ...
Here are five practical tips to make writing causal analysis essay a breeze: 1. Precision in Cause-and-Effect Selection: Start by pinpointing the exact cause-and-effect relationship you want to explore. It's like aiming a spotlight on a specific intersection of events. The more precise you are, the clearer your analysis becomes.
Opening statements are set to begin next week in Donald Trump's historic criminal trial after the final members of the jury were seated Friday, following a dramatic day in which two prospective ...