
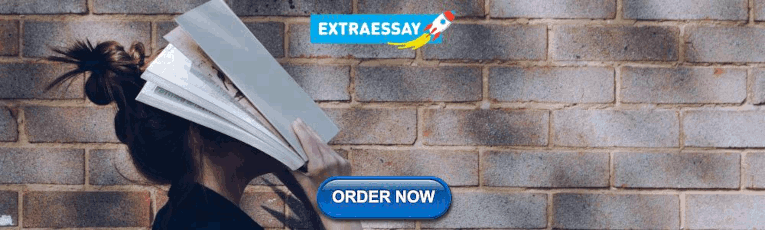
The best AI tools for research papers and academic research (Literature review, grants, PDFs and more)
As our collective understanding and application of artificial intelligence (AI) continues to evolve, so too does the realm of academic research. Some people are scared by it while others are openly embracing the change.
Make no mistake, AI is here to stay!
Instead of tirelessly scrolling through hundreds of PDFs, a powerful AI tool comes to your rescue, summarizing key information in your research papers. Instead of manually combing through citations and conducting literature reviews, an AI research assistant proficiently handles these tasks.
These aren’t futuristic dreams, but today’s reality. Welcome to the transformative world of AI-powered research tools!
This blog post will dive deeper into these tools, providing a detailed review of how AI is revolutionizing academic research. We’ll look at the tools that can make your literature review process less tedious, your search for relevant papers more precise, and your overall research process more efficient and fruitful.
I know that I wish these were around during my time in academia. It can be quite confronting when trying to work out what ones you should and shouldn’t use. A new one seems to be coming out every day!
Here is everything you need to know about AI for academic research and the ones I have personally trialed on my YouTube channel.
My Top AI Tools for Researchers and Academics – Tested and Reviewed!
There are many different tools now available on the market but there are only a handful that are specifically designed with researchers and academics as their primary user.
These are my recommendations that’ll cover almost everything that you’ll want to do:
Want to find out all of the tools that you could use?
Here they are, below:
AI literature search and mapping – best AI tools for a literature review – elicit and more
Harnessing AI tools for literature reviews and mapping brings a new level of efficiency and precision to academic research. No longer do you have to spend hours looking in obscure research databases to find what you need!
AI-powered tools like Semantic Scholar and elicit.org use sophisticated search engines to quickly identify relevant papers.
They can mine key information from countless PDFs, drastically reducing research time. You can even search with semantic questions, rather than having to deal with key words etc.
With AI as your research assistant, you can navigate the vast sea of scientific research with ease, uncovering citations and focusing on academic writing. It’s a revolutionary way to take on literature reviews.
- Elicit – https://elicit.org
- Litmaps – https://www.litmaps.com
- Research rabbit – https://www.researchrabbit.ai/
- Connected Papers – https://www.connectedpapers.com/
- Supersymmetry.ai: https://www.supersymmetry.ai
- Semantic Scholar: https://www.semanticscholar.org
- Laser AI – https://laser.ai/
- Inciteful – https://inciteful.xyz/
- Scite – https://scite.ai/
- System – https://www.system.com
If you like AI tools you may want to check out this article:
- How to get ChatGPT to write an essay [The prompts you need]
AI-powered research tools and AI for academic research
AI research tools, like Concensus, offer immense benefits in scientific research. Here are the general AI-powered tools for academic research.
These AI-powered tools can efficiently summarize PDFs, extract key information, and perform AI-powered searches, and much more. Some are even working towards adding your own data base of files to ask questions from.
Tools like scite even analyze citations in depth, while AI models like ChatGPT elicit new perspectives.
The result? The research process, previously a grueling endeavor, becomes significantly streamlined, offering you time for deeper exploration and understanding. Say goodbye to traditional struggles, and hello to your new AI research assistant!
- Consensus – https://consensus.app/
- Iris AI – https://iris.ai/
- Research Buddy – https://researchbuddy.app/
- Mirror Think – https://mirrorthink.ai
AI for reading peer-reviewed papers easily
Using AI tools like Explain paper and Humata can significantly enhance your engagement with peer-reviewed papers. I always used to skip over the details of the papers because I had reached saturation point with the information coming in.
These AI-powered research tools provide succinct summaries, saving you from sifting through extensive PDFs – no more boring nights trying to figure out which papers are the most important ones for you to read!
They not only facilitate efficient literature reviews by presenting key information, but also find overlooked insights.
With AI, deciphering complex citations and accelerating research has never been easier.
- Aetherbrain – https://aetherbrain.ai
- Explain Paper – https://www.explainpaper.com
- Chat PDF – https://www.chatpdf.com
- Humata – https://www.humata.ai/
- Lateral AI – https://www.lateral.io/
- Paper Brain – https://www.paperbrain.study/
- Scholarcy – https://www.scholarcy.com/
- SciSpace Copilot – https://typeset.io/
- Unriddle – https://www.unriddle.ai/
- Sharly.ai – https://www.sharly.ai/
- Open Read – https://www.openread.academy
AI for scientific writing and research papers
In the ever-evolving realm of academic research, AI tools are increasingly taking center stage.
Enter Paper Wizard, Jenny.AI, and Wisio – these groundbreaking platforms are set to revolutionize the way we approach scientific writing.
Together, these AI tools are pioneering a new era of efficient, streamlined scientific writing.
- Jenny.AI – https://jenni.ai/ (20% off with code ANDY20)
- Yomu – https://www.yomu.ai
- Wisio – https://www.wisio.app
AI academic editing tools
In the realm of scientific writing and editing, artificial intelligence (AI) tools are making a world of difference, offering precision and efficiency like never before. Consider tools such as Paper Pal, Writefull, and Trinka.
Together, these tools usher in a new era of scientific writing, where AI is your dedicated partner in the quest for impeccable composition.
- PaperPal – https://paperpal.com/
- Writefull – https://www.writefull.com/
- Trinka – https://www.trinka.ai/
AI tools for grant writing
In the challenging realm of science grant writing, two innovative AI tools are making waves: Granted AI and Grantable.
These platforms are game-changers, leveraging the power of artificial intelligence to streamline and enhance the grant application process.
Granted AI, an intelligent tool, uses AI algorithms to simplify the process of finding, applying, and managing grants. Meanwhile, Grantable offers a platform that automates and organizes grant application processes, making it easier than ever to secure funding.
Together, these tools are transforming the way we approach grant writing, using the power of AI to turn a complex, often arduous task into a more manageable, efficient, and successful endeavor.
- Granted AI – https://grantedai.com/
- Grantable – https://grantable.co/
Best free AI research tools
There are many different tools online that are emerging for researchers to be able to streamline their research processes. There’s no need for convience to come at a massive cost and break the bank.
The best free ones at time of writing are:
- Elicit – https://elicit.org
- Connected Papers – https://www.connectedpapers.com/
- Litmaps – https://www.litmaps.com ( 10% off Pro subscription using the code “STAPLETON” )
- Consensus – https://consensus.app/
Wrapping up
The integration of artificial intelligence in the world of academic research is nothing short of revolutionary.
With the array of AI tools we’ve explored today – from research and mapping, literature review, peer-reviewed papers reading, scientific writing, to academic editing and grant writing – the landscape of research is significantly transformed.
The advantages that AI-powered research tools bring to the table – efficiency, precision, time saving, and a more streamlined process – cannot be overstated.
These AI research tools aren’t just about convenience; they are transforming the way we conduct and comprehend research.
They liberate researchers from the clutches of tedium and overwhelm, allowing for more space for deep exploration, innovative thinking, and in-depth comprehension.
Whether you’re an experienced academic researcher or a student just starting out, these tools provide indispensable aid in your research journey.
And with a suite of free AI tools also available, there is no reason to not explore and embrace this AI revolution in academic research.
We are on the precipice of a new era of academic research, one where AI and human ingenuity work in tandem for richer, more profound scientific exploration. The future of research is here, and it is smart, efficient, and AI-powered.
Before we get too excited however, let us remember that AI tools are meant to be our assistants, not our masters. As we engage with these advanced technologies, let’s not lose sight of the human intellect, intuition, and imagination that form the heart of all meaningful research. Happy researching!
Thank you to Ivan Aguilar – Ph.D. Student at SFU (Simon Fraser University), for starting this list for me!

Dr Andrew Stapleton has a Masters and PhD in Chemistry from the UK and Australia. He has many years of research experience and has worked as a Postdoctoral Fellow and Associate at a number of Universities. Although having secured funding for his own research, he left academia to help others with his YouTube channel all about the inner workings of academia and how to make it work for you.
Thank you for visiting Academia Insider.
We are here to help you navigate Academia as painlessly as possible. We are supported by our readers and by visiting you are helping us earn a small amount through ads and affiliate revenue - Thank you!

2024 © Academia Insider


An official website of the United States government
The .gov means it’s official. Federal government websites often end in .gov or .mil. Before sharing sensitive information, make sure you’re on a federal government site.
The site is secure. The https:// ensures that you are connecting to the official website and that any information you provide is encrypted and transmitted securely.
- Publications
- Account settings
Preview improvements coming to the PMC website in October 2024. Learn More or Try it out now .
- Advanced Search
- Journal List
- Springer Nature - PMC COVID-19 Collection

Speeding up to keep up: exploring the use of AI in the research process
Jennifer chubb.
1 Department of Computer Science, Digital Creativity Labs, University of York, York, United Kingdom
Peter Cowling
2 Digital Creativity Labs, Queen Mary University of London, London, United Kingdom
Darren Reed
3 Department of Sociology, Digital Creativity Labs, University of York, York, United Kingdom
Associated Data
Anonymised data can be made available upon request via ethics approval.
There is a long history of the science of intelligent machines and its potential to provide scientific insights have been debated since the dawn of AI. In particular, there is renewed interest in the role of AI in research and research policy as an enabler of new methods, processes, management and evaluation which is still relatively under-explored. This empirical paper explores interviews with leading scholars on the potential impact of AI on research practice and culture through deductive, thematic analysis to show the issues affecting academics and universities today. Our interviewees identify positive and negative consequences for research and researchers with respect to collective and individual use . AI is perceived as helpful with respect to information gathering and other narrow tasks, and in support of impact and interdisciplinarity. However, using AI as a way of ‘speeding up—to keep up’ with bureaucratic and metricised processes, may proliferate negative aspects of academic culture in that the expansion of AI in research should assist and not replace human creativity. Research into the future role of AI in the research process needs to go further to address these challenges, and ask fundamental questions about how AI might assist in providing new tools able to question the values and principles driving institutions and research processes. We argue that to do this an explicit movement of meta-research on the role of AI in research should consider the effects for research and researcher creativity. Anticipatory approaches and engagement of diverse and critical voices at policy level and across disciplines should also be considered.
Introduction
The rise and convergence of technologies such as Artificial Intelligence (AI) is shaping the way we live our lives in profound ways (Brynjolfsson et al. 2019 ; Mazali 2018 ; Park 2018 ). Concerns over the efficacy of Machine Learning (ML) and AI approaches in a range of settings affecting the social world such as in healthcare and education (Baum 2017 ; Tsamados et al. 2021 ) make the ethicisation and governance of AI (Bryson 2019 ; Mittelstadt et al. 2016 ) a matter of pressing concern.
These concerns extend to university research. Major funders of academic research have begun to explore how AI could transform our world and the role they can play in utilising AI as an enabler of new methods, processes, management and evaluation in research (Cyranoki 2019 ; UKRI 2021 ). At the same time there is recognition of the potential for disruption to researchers and institutions (Procter et al. 2020 ; Royal Society 2019 ) and clear challenges ahead. The growing emphasis on AI creates space for empirical research to shed light on the possibilities and challenges for researchers who play a key role in developing and applying AI for wider society.
Within current debates about the future of AI and human society, AI is considered in education (Aiken and Epstein 2000 ; Serholt et al. 2017 ) and digital learning (Cope et al. 2020 ) but less is understood about the effects on research more broadly. Indeed, few have explored AI as an enabler of new research methods and processes, and forms of management and evaluation and there is little empirical investigation of the academic response.
The role of AI within research policy and practice is an interesting lens through which to investigate AI and society. Drawing on interviews with leading scholars, this paper reflects on the role of AI in the research process and its positive and negative implications. To do this, we reflect on the responses to the following questions; “what is the potential role of AI in the research process?” and “to what extent (to whom and in what ways) are the implications of AI in the research workplace positive or negative?”.
Contemporary research and AI
Research is global, fast paced, competitive and there is increased expectation to do more. The UK government targets investment of 2.4% of GDP in R&D by 2027 and 3% in the longer term. A new UK government roadmap 1 sets ambitious targets for UK science and there has been rapid growth in AI research. NESTA ( 2019 ) reports on the recent evolution of research that 77% of the papers they identified for their work on AI research were published in the last 5 years. 2 AI is implicated in researcher efficiency and productivity (Beer 2019 ). A performance focussed culture can use AI in pursuit of bureaucratic aims (Ball 2012 ) even though this might prove detrimental to individual identities and collective scholarly norms. While the deployment of AI might work toward satisfying funder expectations of research, increasing productivity, impact and interdisciplinarity (at least according to superficial metrics), it might also sacrifice the traditional roles of institutions and academic identities. With the advent of what was termed ‘Industry 4.0’ (Kagermann et al. 2013 )—a revolution in which AI will be central (Schwab 2017 )—now is the time for HE to seriously consider the responsible innovation and ethics of AI in research practice, culture and HEI governance (Samuel and Derrick 2020 ; Samuel et al. 2021 ).
Research is vital for the economy and its social characteristics also extend to creating benefits for wider society and culture (UKRI 2020 ; Bacevic 2017 ). Academic research shapes academic culture (Wellcome 2020 ), informs teaching (Ashwin et al. 2020 ), identifies new areas of knowledge (Gibbons 2000 ) , and fills gaps in existing knowledge (Holbrook and Hrotic 2013 ). The role AI could play in research adds a level of complexity to a system and the academics entrenched in its habits (Bourdieu 1988 ). AI potentially relieves researchers and institutions from mundane tasks, which saves time (AJE 2018 ) and arguably boosts speed and efficiency required in a (contested) market-driven university. Yet AI also presents concerns in how the use of AI in peer review introduces bias (Checco et al. 2021 ; Lee et al. 2013 ), how AI could miss nuance and surprise (Beer 2019 ) and how infrastructures developed for AI in research (such as in research management), could be used for surveillance and algorithmic management (Beer 2017 ; Williamson 2015 ). Building on Beer’s ( 2018 ) “visions of cultural speedup” as a result of the expansion of data-analytics and AI algorithms, we extend this to consider the effects for research creativity.
Current debates
The micro level of research has been less discussed and some similarities can be drawn from the effects of metrics (Wilsdon et al. 2015 ) and the need for responsible indicators e.g. The San Francisco Declaration on Responsible Metrics (DORA) and Leiden Manifesto (Hicks et al. 2015 ). The research funding community (e.g. Research Council of Norway) have been using Machine Learning (ML) and AI techniques within the research funding system (in grant management and research processes) to increase efficiency. However, further steps are needed to examine the effects and to understand what a responsible use of ML and AI would look like. The research policy community is aiming to develop and test different approaches to evaluation and allocation of research funding, such as randomisation and automated decision-making techniques.
A recent review by UKRI provides a very clear steer on the role that research can play in ensuring a beneficial future with AI, suggesting that there is potential for “AI to allow us to do research differently, radically accelerating the discovery process and enabling breakthroughs” (UKRI 2021 , p.19). The Royal Society and cross-party think-tank Demos ( 2020 ) have conducted work with The Turing Institute into ways in which AI approaches are being used to aid scientific research processes. Funders led by The Global Research on Research Institute 3 (RORI) convened to discuss AI as an enabler in research. Funders at the forefront of adoption of AI include the application of grant reviewers from the National Science Foundation (NSF) in China and the Russian Science Agency. The countries that have seized AI with the most enthusiasm are those with major issues in terms of scale and volume of research (Viglione 2020 ; Wilsdon 2021 ). In that context there is focus on positive outcomes and possibilities of AI. In addition, there is increased focus on the ethical pitfalls of AI across the world and in establishing design principles and guidelines (Bryson 2016 ; Hagendorff 2020 ; Jobin et al. 2019 ). It is easy for the focus on positive outcomes to be coloured in the West where there is an assumed preference for human based decision-making approaches through peer review—perhaps the least imperfect of a range of approaches (Wilsdon 2021 ). Bearing cultural factors in mind, little is actually asked about what changes would be beneficial. Care is needed to avoid approaching this question with the assumption that all is working well without pausing to criticise assessment, metrics, the application of narrow criteria in indicators for impact, research integrity, reproducibility for narrowing diversities, for encouraging systemic bias against types of research or researchers, or diverting attention toward only that which is valued or trackable rather than what is precious and valuable (Chubb 2017 ).
The debate is about what we mean by efficiency and productivity in research and whether speeding up—to keep up , is epistemically good. Reminiscent of an ‘accelerated academia’ (Martell 2017 ; Vostal 2016 ). While AI is seen as having huge potential to support interdisciplinary knowledge exchange, there may be deeper effects of using AI to further research policy and funders’ agendas. These may challenge traditional notions of a university and what it means to be an academic (Chubb and Watermeyer 2017 ; Clegg 2008 ; Harris 2005 ) which may or may not have ‘good’ consequences.
This empirical paper first provides context for the role of AI in the research landscape of the UK. A literature review of the existing research on AI in science and research is followed by an account of the methods. This paper reflects on the deductive thematic analysis of interviews with leading scholars who were asked about the role they could see AI playing in the research process. The paper aims to provide an empirical account of academic views on the potential deployment of AI in research. Authored by an interdisciplinary team of researchers (philosophy, computer science and social science) this paper aims to contribute to understanding about the broader societal and cultural impacts on higher education and research from which we hope to promote and engage academics and policy in a broader debate. The findings with respect to individual and collective benefits and concerns for research and researchers are presented and represent analysis of interviews of AI futures scholars from a range of fields. The implications for the thoughtful implementation of AI in the research process are discussed and suggestions for further research are then made.
Defining AI
AI is often described as an ‘umbrella’ term for a range of approaches to solve data-in-data-out problems which are usually presumed to require intelligence when solved by humans and other animals, distinct from deep and machine-learning techniques which are subsets of AI. AI operates on data to generate new data which solves a pre-specified problem. Hence, AI captures a very wide range of technologies applied to decision-making problems in natural language processing, forecasting, analysis and optimisation with a range of interpretations of data as things such as speech, video, robot movements, weather forecasting and human purchasing behaviour. AI does not include deeper human and animal abilities such as the creation of meaning, the connection with others and the ability to feel and think, except where aspects of meaning, connection, feeling and thinking can be encoded as data-in-data-out decision problems. Research such as Bostrom ( 2017 ) refer to AI developments to date as ‘narrow AI’, postulating a human level of decision making: Artificial General Intelligence (AGI) considering the (probably distant) possibility of Artificial Superintelligence. Many of our participants felt that speculation over whether the latter was possible was distracting from the pressing current issues of AI usage.
Exploring the use of AI as a research tool
The literature presents the use of AI in research as posing both opportunities and challenges. There is excitement about the opportunities AI brings for analysing large amounts of unstructured data (Van Belkon 2020 ) , increasing the amount of data-based research which can be performed, providing community impetus to the curation of big scientific datasets, and simplifying and speeding up the research process. At the same time, there is concern about the stability of academic skills and jobs, coupled with a sense that traditional norms of academic knowledge production might be at risk (Bryson 2016 ). Much literature relates to how AI will benefit or impede forms of productivity, collectively and individually. However, the meaning of “productivity” itself is debated and is not solely limited to notions of an audit culture in HE (Holmwood 2010 ). With respect to research, there is no doubt that there is an increasing expectation for researchers globally to publish quickly (Powell 2016 ). Debates about research productivity have shifted more toward considerations of quality rather than quantity and the scholarly communication process is said to be under strain (Checco et al. 2021 ). True, research productivity is seen to decrease in terms of the quantity of publications and academic output (Bloom et al. 2020 ) yet the literature also notes an increase in quality (Hill 2018 ). Additionally, there is a strong public perception that the ubiquitous growth of AI will impact the future of work (Royal Society 2018a , b ) and expert surveys have revealed widespread thinking that AI could outsmart humans by 2045, at least in a narrow sense (Bostrom 2017 ). This remains highly contested by commentators (Etzioni 2016 ). The rate that AI is accelerating and its potential to ‘replace’ human intelligence causes public fear (Arntz et al. 2016 ; Muller-Heyndyk 2018 ) about loss of jobs. More positively, AI could replace mundane tasks or those which are seen as narrow or repetitive. Hence, while some have associated this fear with the loss of lower skilled labour, others warn that white collar workers (such as academic roles) might also face competition from technology. Some argue that it is a mistake to fear the future of work and it is simply that jobs will change (Beyond Limits 2020 ; Reese 2019 ). In this scenario AI would replace only human ‘drudgery’. There is acceptance of AI if its role is limited to assisting in augmenting performance and task substitution (Grønsund and Aanestad 2020 ). For others, who share the opinion that AGI is imminent (and is as powerful as human intelligence 4 ), human capabilities, and thereby their work roles, will be rendered obsolete. While outside the scope of this paper, this could usher in a new era of creativity and good living for the human race, if we can collectively work out how to share the bounty from AI productivity. In the context of research, the threat of AI to jobs is feasible though there are distinct issues when we consider the nature of academic work, its history, and its values.
Research into how AI and digital technologies will impact research and science culture is relatively early stages (Checco et al. 2021 ). In addition to debates concerning the role of AI in productivity and the future of work, the use of tools to assist with other aspects of academic life is gaining traction. Publishers have piloted AI tools to select reviewers, check the efficacy of papers, summarise findings and flag plagiarism (Heaven 2018 ). Other tools like ‘AIRA’—an open access publisher’s AI assistant—generate recommendations to help assess the quality of manuscripts (Dhar 2020 ), and while AI to support journal editors has reduced the duration of peer review by 30% (Mrowinski et al. 2017 ) the outcome remains with the editor. Skepticism over the use of biased AI tools to conduct reviews is regularly described in the literature (Checco et al. 2021 ) whereas AI to identify discrepancies or errors is better received i.e. for use with respect to compliance or plagiarism. For instance, an AI tool 'statcheck' developed by Nuijten et al. ( 2016 ) revealed that roughly 50% of psychology papers included statistical errors. Such benefits continue to be debated alongside concerns that AI in peer review will simply reinforce existing biases (Checco et al. 2021 ; Derrick 2018 ) and the impact of using machine-learning in peer review or to guide research funding continues to be debated (Spezi et al. 2018 ; Weis and Jacobson 2021 ). There is some way to go before such tools replace a human evaluator. Studies consistently describe AI as a ‘risky fix’ and view it as a ‘runaway process’ in science (Hukkinen 2017 ). The Alan Turing Institute and The Royal Society ( 2019 ), raised a number of benefits and challenges arising from the increased use of AI in science. Notably, that AI combined with access to large amounts of data could be a ‘key enabler’ for a range of scientific fields ‘pushing the boundaries of science’, helping researchers to see patterns in data, find trends, clean and classify data, bridge gaps between datasets and make highly accurate predictions from complex data (2019, pp. 2–3). The near term benefits of AI seem wide ranging, but in the longer term, AI could prompt ‘unforeseen’ outcomes, potentially leading to a reframing of disciplines, modes and methods of knowledge production (Gibbons 2000 ; Nowotny et al. 2003 ). Our paper aims to contribute to the discussion about what developments in AI mean for a future scientific research culture which might rely more on digital technologies to enhance the research process and environment.
The paper reports on the findings from ( n = 25) interviews with leading academics working in AI futures or the applications of AI to human creativity from a range of disciplines (Table (Table1) 1 ) from the UK, Europe, Canada and the US. Scholars were contacted following a review of the literature for recently published works in the area of AI and futures. Their responses to a question on the role of AI in research from the perspective of scholars was then deductively and thematically analysed.
Participants grouped by cognate field and by discipline ( n = 25)
Interviews were conducted face-to-face online and analysed during the Covid-19 pandemic. Interviewees were identified following a comprehensive review of the literature on AI futures and the impact of AI, and a mapping of the AI research landscape institutes, centres and universities. Following the (non-exhaustive) mapping exercise of websites, university pages, and social media, and consultation across the research team and wider academic community within the institution, it was decided that it would be prudent to consider ‘futures research’ within the context of the domain of use of AI (Börjeson et al. 2006 ; Jenkins et al. 2010 ). A draft interview schedule was developed and piloted locally based on three categories: home, leisure and culture. Interviewees were asked to describe the role they personally could see AI having in their workplace (in this instance, the university environment). They were then promoted to consider its use in teaching, research and administration. Responses with respect to research were deductively coded and then thematically analysed. We combined thematic analysis (Braun and Clarke 2006 ) with qualitative thematic data analysis (Miles et al. 2014 ). This paper reports on the deductive findings from one aspect of our research: the role of AI in the university workplace, with a focus on research. The effects of AI on teaching, though described regularly by participants, is not considered in this paper (instead e.g. see Serholt et al. 2017 ).
Limitations
Interviews were conducted during a National Lockdown Summer, 2020 and this may have affected participants’ responses, at a time of multiple crisis (Simmel 2010 ). It can be difficult to develop a rapport with participants online and so, ahead of each interview we explained the session and offered an informal (non-recorded) chat before the main interview. We also offered interview timing flexibility, and made clear the option to withdraw or reschedule. The efficacy of such methodological practices during lockdown have been shared with the community (Jowett 2020 ). While generalization of an initial small scale qualitative study is difficult given the representation of disciplines, this research adds richness to existing issues and shows how AI can intersect with research from those at the cutting edge of its development and critique. The analysis was conducted across three disciplines.
Criteria for inclusion included proven expertise within AI through academic publication and current position within a research organisation/HEI. We aimed for a gender balance where possible, despite the preponderance of one gender in some disciplines. Stathoulopoulos and Matteos-Garcia ( 2019 ), report “while the share of female co-authors in AI papers is increasing, it has stagnated in disciplines related to computer science”. Despite this, 16/25 (64%) of our sample are female. Interviewees had expertise in the future of AI across a wide range of disciplines. We created a coding framework, identifying deductive and inductive codes. For the purposes of attributing participant involvement to verbatim quotation, we provide disciplinary field information and a unique numeric indicator. All interview data were anonymised at the time of analysis with individual identifiers used to denote verbatim quotations. Data were stored securely on a password protected computer with recordings deleted after use. Consent was gained for the audio-recording and transcription of interviews.
Interviewees all commented on the prevalence of AI in research and in most aspects of modern life. AI is seen by our interviewees to have huge potential in connecting knowledge and knowledge producers, while also presenting concerns with respect to the future of work and to equality and fairness across disciplines and career stages. Below, we analyse interviewee responses related to benefits for first individual and then collective use. Then, we look at the more challenging aspects identified by participants and consider how the role of AI might disrupt academic activities. Our interviewees provide compelling arguments that whilst AI has great potential in research, it is incumbent upon actors across the research ecosystem (academics, institutions, funders and governments) to ensure that it is not used as another bureaucratic tool which further metricises and destabilises research quality and academic identities and expertise.
AI for individual researcher use
The most commonly reported use for AI was to help with narrow, individual problems: to help researchers reveal patterns, increase speed and scale of data analysis and form new hypotheses. Many felt that the advent of web searching and online journal repositories had made it easier to ‘keep up’ with a fast moving research landscape. This was seen as transformative and was considered positive by most of our respondents. One participant argued that web searching enabled working across disciplines, enhancing their career:
...people get very good at using search algorithms and being discerning in the results that they choose, getting up to speed on a topic very, very fast, and then being able to digest and provide the information that was needed. So that’s [a] position that only really exists because of web search algorithms, because of AI. I would say all my working life I have done jobs that have only existed because of AI, that AI being the web searching algorithms (Arts and Humanities 15).
Almost all participants described how AI was already in use in the work environment, particularly that of higher education, in terms of enabling research practices and teaching. Several used the mode in which the interview was being conducted (online, using Zoom) to describe the everyday use of AI features:
…to make Zoom calls have blurred backgrounds, or to make pictures look as if they are taken with an expensive lens—that is AI heavy lifting. AI—things that are doing automatic voicemail transcription and transcribing research notes - AI is great for that kind of thing (Physical, Natural & Life Science 06).
Some commented on the role of AI and algorithms in in music or film research to boost creativity, or save time:
I think storytelling has a role to make us see the world differently and I would love AI to be used in that direction (Arts and Humanities 17). There’s lots of [AI] stuff out there now where you can press a button and you can get music in a certain style. Certain things that I use now that take me a long time, that I find clunky, so maybe certain pieces of software, I would like to be able to, you know, use in a more efficient manner (Arts and Humanities 13).
The growing use of algorithms in research in hard to reach environments was also described:
We’re using algorithms more now than we did even a few months ago because we can’t be present, you know, so lots of other things that we can’t check and verify, we are using models to check (Social Science 10).
While the role of algorithms is increasing because of the volume of data they can deal with, this is largely seen as a problem orientated narrative, whereby AI is employed to solve problems. There is also a need to think about how those algorithms are interacting in particular social spheres and crucially who they affect (Noble 2018).
AI and narrow tasks
With AI already used in research, some of which has been greatly accelerated because of the pandemic, over half of the participants then went on to describe how AI helps with narrow tasks and increases personal productivity.
I think thinking small, thinking narrow, what are the individual problems that AI could be helpful with, we can think of just personal productivity in terms of the AI that is in your computer, helping you with search functions (Physical, Natural and Life Science 06).
Here, AI is seen to reduce tedium and is welcomed if it is doing a very specific job or answering a specific question such as:
Has somebody looked at this chemical before and have they found whether it will oxidise aluminium nitrate or something? and then you type that sentence in and you get competent search results coming back nicely summarised (Physical, Natural and Life Science 06).
Several noted how AI could systematise the practice of literature searching by “trawling” through a lot of abstracts and then selecting those which might be relevant. Indeed, some reported the everyday use of such tools in their research team, to do ‘the heavy lifting for us’ (Engineering 06) e.g. see Paper Digest. Participants suggested that searching and summarising papers were the kinds of tasks that were ‘time consuming’, involving ‘endless drudgery’ (Physical, Natural and Life Science 21; Arts and Humanities 04) . While another felt that those same skills defined them as a researcher “some people dedicate their life to learning those skills (Psychology 12). Reflecting on Ewa Luger’s work on AI and accounting, one participant was clear in promoting understanding about the role of skills in particular professions; “it is not about some people liking it, it is how the skill is built up about being an accountant. It is the same with radiologists, they look at for example an x-ray every seven seconds, an x-ray goes by or a mammogram goes past them, and they build up the visceral skills of understanding what to do, and what the point is, the next generation of accountants will not have that.” Instead, Luger suggests that something else has to go in its place. They go on to suggest that “it is not just about deskilling, it is actually understanding what those skills are before you rip them away” (Physical, Natural and Life Science 17) .
One participant described how their subject area was so broad that filtering out the ‘wrong’ information would take years, affecting their ability to be ‘productive’.
Right now I’m working on a meta-analysis and I’ve been going through tons and tons of papers, and it’s so dumb… Still after months of trying I still don’t have a good way to narrow it down by idea … I mean you could imagine having an AI do some of that work for you. Wouldn’t that be nice if I could? (Physical, Natural and Life Science 02).
AI and emotional labour
Participants referred positively to AI as long as tasks did not require ‘emotional elements’, suggesting that a line is perceived in terms of how far we are willing to accept AI into our lives and work:
When it comes to looking at different articles etc, AI can do it way more quickly than humans can, but when it comes to making a sentence out of it or a verdict, then you also have like emotional elements attached...AI can augment human intelligence in different ways, but I don’t think we should make the mistake of trying to make them emotional because they will never really be emotional (Social Science 11).
Participants talked about AI as a personal aid tasked with mundane, everyday research tasks, such as information retrieval:
We are never going to have money to pay a research assistant to do this kind of [mundane information retrieval] work - it’s terrible - AI come here and do your magic (Physical, Natural and Life Science08).
Whilst many felt using AI to sift through large quantities of data and literature was positive, they had ‘mixed feelings because this might diminish the enjoyment and satisfaction of the job,
So, if something else could do it for me, I don’t know, but I’d feel like if somebody else could... if something else or somebody else could do that for me then I doubt that they would just stop there and not be able to do the second step as well and then I’m sort of not just out of job but out of a big passion, you know (Physical, Natural and Life Science 02).
Other respondents were positive about working with AI to save time in relation to a range of niche tasks, such catching up with what their peers are doing. This might be more effective than traditional methods such as conference attendance, yet “potentially less effective still than going to a team meeting or going for coffee with your colleagues” (Arts and Humanities 12) . Though much of the literature about the use of AI in research relates to peer review, most of our participants did not mention it explicitly. One, however, explained that where human oversight was required, they would not welcome the use of AI.
I cannot imagine good peer review done without humans... well, definitely human oversight but so much human intervention that it wouldn’t be that different from what’s happening now. I just can’t imagine it being possible for that to be done by a machine (Social Science 14).
Interestingly, not a single participant mentioned the use of AI in research evaluation, another process which relies on peer review and human judgement. Where AI is making judgments, all participants expressed concern about bias. In particular, participants warned against the use of AI for decision making when a system is reliant upon training that is built on historical data. When applied to the recruitment of students and staff in universities it becomes a social justice issue.
I would particularly worry about the application of AI in student admissions [...] I can see how there would be pressure to add AI to the mix and especially because it’s so time-consuming and its very people intensive, what I would really be afraid of is if a part of the process would be automated because what you always see when processes get automated the outliers disappear, the outliers get ignored or brushed over. I can understand that people hope to take out the personal bias of the interviewers, but it could also introduce a whole load of historical bias (Arts and Humanities 04).
Instead participants preferred that AI should augment and assist human judgement:
Instead of trying to copy human intelligence why not find ways and augment it instead of trying to substitute it (Social Science 11).
One participant explained how AI could be used in the near future to bypass traditional means of knowledge production:
I think there is certainly potential for significant speedup of research findings, I think there are certain fields that are genuinely amenable to just machine discovery of new theories, particularly very large-scale collection of new data and hypothesis generation from the data (Arts and Humanities 12).
Many talked about how research is proliferating so fast that it is difficult for researchers to keep up:
In academic life and in research [...] the rate at which we’re publishing stuff is exploding. We shouldn’t be doing that. But it is happening. So I think the key challenge for the future will be to navigate knowledge (Physical, Natural and Life Science 17).
Productivity is bound to the navigation of vast amounts of research. AI is helpful if it suggests useful literature. Researchers can then train the algorithm to do better next time around. Participants remarked on the variance of the effectiveness of such tools to get to the heart of the data or information, suggestive again of the need for human insight.
Increasing personal productivity
Participants explained that the main individual benefit of AI was the navigation of knowledge and streamlining the research process:
I would say one area that it could possibly be useful is just streamlining the research process and helping to maybe — for me, it would be helpful taking care of the more tedious aspects of the research process, like maybe the references of a paper for instance, or just recommending additional relevant articles in a way that is more efficient that what is being done now (Social Science 04).
AI was seen to help with accessing large amounts of data at speed and improving decision making by showing patterns at a scale difficult for humans to see:
In research - with all kinds of data aggregation, you can imagine being able to sort of let an AI loose on historical data and seeing what new kinds of things can be found. On the other hand, new hypotheses that can be tested, questions that can be asked simply because then the computing power and ability will be available (Arts and Humanities 04).
Participants referred to the fact that AI can free up time, enabling researchers to work on other things at the same time as conducting primary research. Here, participants strongly prefer AI that complements rather than replaces human expertise. This is particularly the case for certain disciplines when AI is used to discover new theories through large-scale data collection and hypothesis generation. The use of archival data in humanities research is one such area:
I mean if [AI] could go through all the archives I painstakingly try to go through and tell me where things are, organise stuff for me in much more convenient ways to analyse historical stuff - going through my own data without me having to programme things painfully? Oh gosh I would embrace it (Arts and Humanities 04).
Using AI technologies for individual use is where most benefit is perceived. We find that AI is welcome if it improves productivity and saves time. Specifically, AI is repeatedly viewed as beneficial for the navigation of knowledge, in the context of increased expectations to publish. When ‘let loose’ on large datasets AI has the potential to generate hypotheses and in turn increase collection and analysis capacity to streamline the research process:
There are certain fields that are genuinely amenable to just machine discovery of new theories, particularly very large-scale collection of new data and hypothesis generation from the data (Social Science12). Normally the scientific progress goes like this, so you have a hypothesis and then you collect data and try to verify or falsify the hypothesis, and now you have the data and the data, so to say, dictates you what hypothesis you can find. So, this is how methodologies, scientific methods are changing (Social Science 01).
As scientific methods embrace AI, one participant reports the potential for things to become more complex in an already overly-bureaucratic system:
It's a double edged sword because it has made it easier to increase the complexity of bureaucracies and forms and processes and procedures so it’s one step forward and maybe one step backwards in terms of the amount of time and energy it takes. I mean, you know, we see similar kinds of complexity (Physical, Natural and Life Science 03).
AI might be seen to add complexity because of the steps and processes that are inherent to its design. In order for it to be beneficial, participants stressed the need to be put in the conditions to be able to benefit from it and that requires that sort of social capabilities e.g. increased understanding of the remits and capabilities of current systems and transparency. Over half of our participants spoke to the need for explainable and transparent systems that take into account the social context:
There’s a bit of a tendency in the kind of engineering and computer sciences to sort of reduce what are quite complex things to quite simple things, like explainability, for example, or legibility. There’s a very kind of complicated social context that needs to be taken a bit more seriously, I would say (Social Science 23).
AI for collective use
Most participants welcomed the idea that AI could support collective activities that inform research culture and expectations, including citizen science, impact activities, and interdisciplinary working. The most cited benefits from AI were (1) its effects on modes of knowledge production, increasing freedom to conduct blue skies research; (2) facilitation of engagement and impact activities and (3) to act as a ‘bridge’ between research cultures, boosting interdisciplinarity.
Modes of knowledge production
Participants felt that AI could release researchers to pursue new areas of ‘blue skies’ research, conduct engagement and impact activities, and work across disciplines:
I feel the human mind and human curiosity will inevitably just be freer and more open, and quite honestly the cultural pursuits are one thing, but I really feel scientific pursuits will be another exploration, so suddenly if our world is running more efficiently, if we are not — you can imagine AI in policy making, eventually optimising how we use energy in the country. So, we will be able to focus more on human beauty and human knowledge. It feels like there will be more scientists who are doing basic research in trying to understand the world ourselves fundamentally (Arts and Humanities16).
Where AI is critical in the development of new modes of research and knowledge production the key benefits are noted with respect to career stage and discipline. One participant noted the potential for ECRs to benefit from AI because of access to big datasets.
I mean if you look at, you know, the sort of average postdoc of three years, how much data you can collect and analyse in that period could increase drastically (Physical, Natural and Life Science 11).
Similarly, with respect to academic discipline, another participant suggested that AI could collectively benefit the arts and humanities, from which one could see a new mode of critical engagement emerge:
I’m hoping that the idea that a text might be written by an AI rather than a human, over the next few years leads to a realisation and a new kind of critical engagement with texts where it just becomes much more the norm that people question the authorship of the texts that they read critically and that kind of critical reading is something that certainly is and should continue to be key in humanities education and I think many humanists would see AI as encroaching computers into the field, but I think there are lots of opportunities within digital humanities to use AI (Arts and Humanities 04).
Quite how far these new modes of production might encroach on traditional disciplinary norms is not yet known but there were moments in the data suggestive of disciplinary challenges, whereby scholars might be disadvantaged by virtue of their training to benefit from AI:
Being in a humanities department, one of the big challenges I see is helping people bridge between quite different areas of expertise. I think that’s a challenge that people are already trying to work on bringing the technology under the fingertips of students, or researchers who, by virtue of their background or interests, can’t use it themselves, but would be interested in using it. So, I see that as a major challenge (Arts and Humanities 13).
AI and impact
Several participants noted that AI could benefit multidisciplinary research teams with regards to open innovation, public engagement, citizen science and impact. When considering the role of AI in research, participants regularly referred to the idea that AI could act as a bridge beyond the university context and that boundaries could be expanded through greater participation in science. If used to support researchers to develop links with others and to build impact, AI could highlight the University’s civic role. As one participant described it “ communicating the potential benefits of our research to the wider world. AI can help us do that” (Arts and Humanities). One participant thought of AI as a kind of potential co-creation tool:
… There is a co-creation between a human author and AI that then creates a new type of story and what would that be and, more importantly, what are the conditions for this to be a real co-creation and not being one controlling the other or vice versa (Arts and Humanities 18).
The release from narrow individual research tasks, mentioned earlier, was also seen to result in the time to deliver impact activities more effectively. One explained how academic research takes too long to move beyond the academy “ we are used to doing research that always takes so much longer, we don’t work on the same timescale [as potential users of the research] and it’s super frustrating.” (Physical, Natural and Life Science08). Research impact takes time and effort and so AI’s ability to build connections could speed up the process. One participant suggested that AI could allow “ the vision of the open source community applied to AI” (Arts and Humanities12). However, these infrastructures, once established could also be used for many more negative and intrusive purposes. Some participants warned about potential threats to expertise where over-use of AI could render academics ‘ generalists ’, which could be both positive and negative. On the one hand, it could be unhelpful at a time when the role of expertise is challenged in the public and political sphere (on the other the contrasting view that enabling scientists as generalists might also be key to making societally useful advances in science and the emergence of real evidence about public attitudes towards expertise as positive e.g. see Dommett and Pearce 2019 ). Commenting that many researchers might become generalists, one participant expressed concern about working across disciplines they didn’t train in. Despite this, AI was mostly viewed positively for increasing potential to work across disciplines and with publics “the problems of discipline exclusivity and people not being able to talk across disciplines or understand each other and those barriers have really been broken down by AI” (Arts and Humanities 12).
Inequality, fairness and bias
Whilst impact is perceived as a benefit, some participants expressed concern over using AI to deliver impact activities with respect to inequalities and biases. One provided an example of where AI systems were used to promote awareness of HIV amongst high-risk populations. The AI selects peer leaders within communities of homeless youth to support awareness building. Concerns were expressed about “the potential intensification of existing inequalities that can happen (Social Science10). Several expressed concern over rising inequalities and felt that AI is only exacerbating unevenness in society; “the hugely and dramatically accelerating inequalities that are coming out of this” (Physical, Natural and Life Science 21), specifically that:
AI might amplify existing social inequalities among the youth of different genders, races, socio-economic statuses (Social Science 24).
One participant described AI as “a mirror to ourselves” (Futures 11) complete with biases. Generally, AI bias occurs because the data we put in are biased, due to human decisions in data curation and collection. Several interviewees suggested research to focus on explainability, trust and fairness. An important consideration therefore is who benefits from AI research and usage.
Even more important is the common understanding of how we can create AI systems that are ethically responsible (Social Science 11).
AI and interdisciplinarity
Our research suggests that AI has potential for boosting and supporting interdisciplinarity. Overwhelmingly, participants saw real potential for AI in bridging disciplines, which could also reorientate research priorities. For instance, AI can ‘match-make’ people across disciplines.
Some abolition of disciplinary boundaries, some significant massive participation of subjects of study in the design and carrying out of research that is affecting their lives and hopefully pretty soon a reorientation of research priorities to better match what people are generally interested in (Arts and Humanities12).
References to AI as match-maker were common in our interviews: “in a world where you’ve abundant and diverse interests and abundant and diverse high quality information sources, the trick is matchmaking” (Arts and Humanities 12). One participant notes that using AI to match-make academics, would require consideration of buy in, trust and privacy: “making such connections could happen but it requires engagement from different actors in the sector “a world that is more kind of embedded in a multistakeholder conversation, of course that’s the utopian vision, there is also a dystopian [side] to it” (Social Science 19). The opportunity is large, as AI could greatly extend the boundaries of collaboration (Lee and Bozeman 2005 ). Participants noted:
My positive vision is that we adopt some version of extreme citizen science where the boundary of who gets to contribute to research is significantly opened up, where everything is much more modular: there is a cloud of hypotheses, a cloud of data, a cloud of finding ways of connecting these to much better language, very good training, lots of ways of recruiting volunteers or collaborators to whatever interesting project that you have (Arts and Humanities 12). I suppose one of the benefits I’m already seeing which I think is really advancing quite quickly is the kind of interdisciplinary collaboration, working much more closely now with computer scientists and mathematicians and physicists in my university than I did before (Social Science 10).
This gives rise to the challenge of tailoring AI training to the needs of researchers with a wide range of disciplinary backgrounds.
The academic role
Another theme related to how AI could affect the academic role. One participant talked about how the shifting landscape of HE had the potential to challenge traditional roles:
If we are moving into a world where everyone is a continual learner and potentially everyone is a teacher (maybe not everyone but certainly many more people than we can think of as traditional professors), then the challenge becomes matchmaking. And having that matching be done by AI systems is probably the way you would need to go partly because this is not something that humans have traditionally been very good at and also because the scale is huge. (Arts and Humanities 12).
They go on to envision a rather exciting future, a “ trusted ecosystem … matchmaking learners and academics where the academic part will probably be heavily augmented with AI”.
Arguably, every skilled profession is in a state of evolution requiring continual learning, changing academic modes of interaction, roles and career trajectories. Some participants welcomed this enthusiastically and felt that AI will not take away from researchers and educators, but instead create new roles:
In AI-supported learning environments, there’ll be even more need for educators and teachers and teaching, even more need than ever before. In many more places, not just schools and universities, but workplaces, community organisations, so people who are…people who facilitate any community of practice in an online environment are involved in knowledge management, learning organisations, in a broad sense (Social Science 09).
The same participant went on to suggest that AI would lead to new forms of labour “a profoundly modern job… and a new economy” (Social Science 04) where AI could transform worklife for academics in positive ways. Another participant noted that whilst AI is often associated with low-skilled labour, many of those tasks which AI can and will perform are currently seen as skilled labour and higher-prestige white collar jobs are susceptible to automation: “it is interesting that some of the higher-prestige white collar jobs are maybe more susceptible to automation than something like a food delivery service” (Physical, Natural and Life Science 06) . Such comments are exemplified by suggestions for automating aspects of research. One participant suggested: “we should organise the university like a gym with an app” (Arts and Humanities 17). Participants referred regularly to the use of AI to help university functionality more generally such as building maintenance, cleaning, food selection, finances and logistics. All of these use cases have the potential to indirectly and positively affect research.
The future of academic labour
The potential of AI to alleviate work pressure comes with an associated paradox, in which personal gain requires a sacrifice of privacy through the gathering of large amounts of data on individuals:
You could imagine a university of the future where there would be much, much, much more data on people and much more understanding of how they learn… I have mixed feelings about it (Physical, Natural and Life Science 02).
When imagining a use for AI in the context of any domain of (human) work, there were concerns about the loss of jobs. In particular, this was seen to threaten certain groups, including early career researchers and researchers from the arts and humanities:
We’ve seen the hiring of fewer and fewer staff in terms of research within the humanities (Social Science 25).
Participants commenting on the use of AI in the humanities suggested that human knowledge will still be required alongside AI:
There will still be people who are studying urban planning, even though there are urban planning AI — there will be people doing that. If [the AI agents] are doing it better than us, fine — we will have scholars preserving the human knowledge and then pointing to why the AI knowledge is so much better. It just comes down to ego or not, in that case. (Arts and Humanities 16).
The implicit challenge of the word ‘better’ in this case provokes debate about the role of metrics in HE. The need to ensure that ‘unemotional’ AI only compliments and does not replace human knowledge with ‘deep information’ is particularly pertinent to certain collective groups, such as the arts and humanities who may find it difficult to objectively measure their cultural value and impact (Benneworth 2015 ; Belfiore 2015 ):
With budget cutting scenarios, I wonder to what extent various kinds of programmes that don’t fully work will be used to justify attrition of things that are currently done by people (Social Science 25).
Despite relative confidence among our participants that AI will not replace established academics, AI is seen to potentially challenge more precarious groups. Whilst AI is presented here both in positive and negative terms, it is already in use and we must now deal with the ‘hangover,’ as one participant aptly put it:
I joked on social media that we had our big party on Saturday night and now it’s Sunday morning and we got a hangover and we’re sobering up and we’re saying wow, there are some great potentials for bad and terrible uses of technology as well as very good ones (Physical, Natural and Life Science 03).
Anticipating the good and bad effects of AI will surely be key to better ensuring benefit which helps humanity to thrive, rather than impedes it. This is perhaps particularly pertinent during times of crisis.
If AI is let’s say replacing human capitalistic work in certain ways, the question I would like to ask is how is it making our lives better? And, one of the things that we will likely be holding on to — at least for the near future, near to medium-term future — is human creativity and culture. So, everything from religion to art and performance, the human spirit I feel will be the last thing for us to stop appreciating (Arts and Humanities 16).
We now draw together the findings in a discussion of the challenges and benefits perceived by our participants and explore their effects supported by argumentation in the literature.
We draw together the findings in a discussion of the challenges and benefits perceived by our participants and explore their effects as illustrated in Fig. 1 .

Individual and collective benefits of AI in research from thematic analysis of ( n = 25) participants. *AI in teaching was excluded from the discussion of this paper
The views and experiences of this study’s AI thought leaders help formulate an understanding of the current position of AI in research and likely routes forward. In general, our interviewees perceive the benefits of AI to be focussed on individual tasks such as the navigation of large data sets, particularly volumes of text, as well as providing collective benefits for groups across disciplines through facilitation of collaboration and impact. Primarily interviewees constructed their responses around the benefits, in line with the interview prompts to consider the opportunities for AI. Nevertheless, the possibilities of AI present challenges which require deep reflection, reminiscent of related debates in research about academic productivity, metrics and algorithmic allocation (Arruda et al. 2016 ; Bonn and Pinxton 2020 ; Bornmann and Haunschild 2018 ; Dix et al. 2020 ; Wilsdon et al. 2015 , 2017 ). There was optimism about the way AI might relieve tedium and open up new knowledge pathways. But this was coloured by concerns that AI tools used unthinkingly might promote bias and inequality. AI is seen to potentially challenge more precarious groups (Gruber 2014 ; Herschberg et al. 2018 ; Ivancheva et al. 2019 ). There is a preference for AI to play a role within research that assists rather than replaces human performance. In this section we discuss key themes which were discussed by our interviewees and relate them to the university context before using them to suggest a route forward.
The contemporary research context is a product of a complex set of factors, including the marketisation of the university and the multiplication and fragmentation of research areas. Asked to think about a future with AI in the workplace—in this case the research environment—participants described what the university of the future might look like and what role AI could play in it. Against a backdrop where increased investment in research demands greater output and impact, notions of productivity are increasingly tied to performance metrics and bureaucratic processes of a ‘performative’ university culture (Ball 2012 ). Thoughtless application of AI could “speed up” research to “keep up” with the metrics while negatively impacting the quality of research and the quality of life of researchers. Increasingly, research income is allocated at scale to underpin the UK ‘knowledge economy’ and to firm up the UK’s position in the world as a global leader of science and research (Olssen and Peters 2005 ). There is broad consensus that the total investment in research in the UK will increase (Royal Society 2019 5 ). The UK Government has committed to a target of 2.4% of GDP invested in research and development by 2027, with a longer term goal of 3%. Increased investment will flow into research through the science budget via research councils, the block grant resulting from the REF research audit and direct funding of large centres and projects, as well as through industry investment. Accountability and measured performance are a condition of funding, for instance, the requirement to demonstrate the impact of research through The Research Excellence Framework (REF) and grant funding enables institutions and funders to make ‘value’ visible to the government. Though rather knottily related to the question of how you measure research productivity, research grants and systems to assess the quality of UK research have been reported as damaging to traditional notions of what universities are for (Collini 2012 ; Martin 2011 ; Chubb and Watermeyer 2017 ). One consequence of AI undertaking narrow and mundane tasks is that it makes space and time to pursue other forms of collective knowledge production (Gibbons 2000 ). What Van Belkom ( 2020a ) refers to as “naive” but “breakthrough” research. Against this background, metric-driven AI tools will need thoughtful management to avoid a situation of rising scores and declining quality, value and researcher wellbeing.
Productivity
As with many areas of contemporary society, the university is reliant upon information technology. The incorporation of AI and machine learning are a logical next step. AI is an engine of productivity and the need for information navigation tools is a consequence of a rapid increase in research production and information accumulation. Productivity in this sense is an issue faced by contemporary academics. They are expected to be productive and at the same time cope with the increasing volumes of output of others. Interviewees referred to increased demands in academia to produce and to be seen to be productive. This begs the question as to what being “productive” means. The data points to a deeper issue about integrity and the need for academics to establish the true value of their work as opposed to what is likely to satisfy institutional and funder requirements (and of course universities and funders should continue to attempt to steer toward true value). Importantly, to approach the research system with the assumption that it is working diverts attention to only that which is valued, or trackable, rather than what is most precious to the researcher and to society. AI is perceived by our participants as reinforcing individual and selfish behaviours in pursuit of particular targets or measures (Elton 2004 ). There is a danger in considering productivity in terms of precisely-defined metrics (e.g. REF). AI is a very effective tool for ‘measuring’ productivity. However, our data supports the continued need for human judgement in decision making. A focus on efficiency and productivity— speeding up to keep up with a fast moving knowledge base—might therefore weaken output quality as it obscures the use of human judgement to produce unexpected interpretations of data and literature. In turn, this might encourage deleterious consequences for particular individual and epistemic identities (Chubb and Watermeyer 2017 ). One theme, explicitly and implicitly made in the interviews was that AI will never be emotional. Research roles that require emotional and nuanced judgement, such as forms of research evaluation, should avoid AI. The idea that research quality and productivity pull in different directions is a cause for concern, and a crucial issue for funders, research leaders and researchers in Science and Technology Studies, Research Policy, AI Futures and ethics to address.
Impact, engagement and AI
AI is beneficial when it supports information navigation and knowledge production. This occurs in the daily practices of researchers in web searches and communications technologies. It supports connections between researchers. Interviewees described how there may be collective benefit to using AI in research to connect researchers from different disciplines whilst others warned about how this may lead to academics being generalists and not experts. The impact agenda requires increased public engagement and interaction (Reed et al. 2014 ), which might in turn encourage generalism. But public intellectualism is perhaps better understood in terms of accessible specialisms (Collini 2012 ). At the same time, a new era of scientific generalists may spark a renaissance in science as ideas travel across disciplinary cliques and into public attention. While clear benefit can come from championing the role of AI to boost impact, disciplinary differences need to be sensitively considered. Some disciplines and groups, such as the Humanities and ECRs, may be disadvantaged, reducing the need of student support, and undermining valuable career experience. Recognition of AI as a component of ubiquitous computing systems, such as web search and recommender systems, is useful. The explicitness of naming something ‘AI’ reinforces the ‘good’ use of AI as its ability to speed up and make research more efficient. But this is problematic where a culture of performativity is damaging to individuals and groups. Responsibly employed AI could strengthen meaningful relationships within and between disciplines as well as between academia and the public.
Interpretations of work and emotion
The assumption that processing data is a large part of a researcher's job provides a reductionist approach to the academic role. A human reduced to any number of data points is a poor facsimile. The same being true for human organisations. Rather, humans are agents of meaning —the reader of a scientific article does not simply “process” the words of an article. Instead, they interpret, they experience. With this in mind, to assume that Science is about creating data, tells only a fraction of the story. Much more important is creativity and human understanding. We note how interviewees instead referred to AI as taking away or relieving them from the ‘drudgery’ of certain tasks, however, several warned that this was not reductionist and that a range of roles, research assistants, archivists etc., within research, and a range of skills gained through training as a researcher, could be lost or replaced by automation and AI. The skills accumulated when sifting through information might be critical to the person’s role as a generator of meaning. Luger’s ( 2021 ) current work ‘Exploring Ethical AI in Accounting’ provides an example. In her study of accountants Luger argues that the removal of mundane work—in the case of accounting looking through receipts, for example—reduces professional skills development. Instead there should be greater consideration of understanding skills rather than just blindly taking them away or replacing them. AI should then not necessarily take those mundane tasks away from researchers, because it would take away a fundamental skill relevant to a profession. Our interviewees prefer for AI to be limited toward the factual as opposed to the interpretative, chiming with views commonly held that AI ought to assist in the workplace, i.e. ‘IA instead of AI’ (Pasquale 2020 ). Interviewees expressed concern about loss of jobs, particularly for those whose roles demanded more repetitive tasks. At the same time, some noted how computation could increase demand for labour. While a counter position to this may err toward a more reductionist view of the scientific enterprise, the preferred view of science, knowledge and discovery is that it is precious and should not be reduced to a series of tasks and measured by metrics. The extent to which this relates only to AI, is debatable, but a form of anticipatory governance—motivating foresight and engagement at all levels, vis-à-vis the implementation of AI within professional roles—seems appropriate (Fuller 2009 ).
Whilst our participants identified a clear beneficial role for AI in navigating large bodies of knowledge, information and data, there is also potential for generating impact and nurturing interdisciplinarity. AI, alongside human creativity and insight, could yield extraordinary benefits through research. At the same time, there are threats to groups of researchers where a reliance on technology destabilises certain kinds of knowledge production and producers. The replacement of human capabilities in collective activities such as peer review, where human judgement is deemed vital, is considered undesirable by our participants.
Operationalising values in research
The interview data echoes macro level debates about human ‘values’ in research. Interviewees foresaw issues with the ways in which AI might reflect and further existing inequalities and bias. A large amount of research and regulation has targeted the minimisation of this bias (Caliskan et al. 2017 ; Röösli et al. 2021 ; Zajko 2021 ), but the hidden consequences of AI adoption with respect to research have yet to be fully addressed. If AI challenges institutional and academic identities and helps shape the future role of the academy, it may be pertinent to ask how technology can support, rather than impede, the process. But as AI bias originates in human beings, there is an important consideration to address e.g. as AI emerges from communities (developers and technologists, etc.), which themselves hold certain values and perspectives and priorities, which may be distinct from the users—here, academia and scholars, then the technology itself cannot be seen as value free or neutral in this process. Rather, as much of the literature shows, AI bias ought to tackle the very stories and narratives which are propagated from the fairly homogenous group from which they often come. Narratives and story can pervade public and policy perception (Cave et al. 2018 ; Cave and Dihal 2019 ). A study by the Royal Society suggests there is urgency to take AI narratives seriously to improve public reasoning and narrative evidence (Dillon and Craig 2022 ). What is required, they suggest, is ‘story-listening’—an active engagement and anticipation with narratives as a form of public participation. Issues of social justice emerge as key concerns within AI. As gendered AIs populate the media (Yee 2017 ; Cave et al. 2020 ) and our homes (Alexa and Cortana, etc.), questions are rightly focussed on who is telling the stories that are informing our sense-making about AI. Indeed, the extent to which these fictional narratives inform and engage with issues of race (Cave and Dihal 2020 ; Cave et al. 2019 ) and ascribe a view of ‘whiteness’ is of ongoing concern and debate, not only through stories, but in wider attempts to decolonize AI (Noble 2018; Benjamin 2019 ). Shining a light on these design issues, where AI might embody such values and principles needs to be evaluated with society and culture at the heart. In the case of academia, a diverse community, attention as to how these stories and perspectives are shaping this diverse community or holding back areas of science which already struggle to be so.
The 2021 UKRI report Transforming our World with AI (UKRI 2021 ) suggests that the “profound impact of AI… has not yet been realised”, and that AI “can open up new avenues of scientific study and exploration”. This paper strongly supports these views by providing insights from leading AI Futures scholars. Through this we have a better understanding of the questions to be asked and actions taken to achieve outcomes that balance research quality and researcher quality of life with the demands for impact, measurement and added bureaucracy.
The effects of AI tools in scientific research are already profound. Our interviewees discussed individual and cultural factors, seeing AI opportunities in areas where change might be appreciated, alongside a desire for stability for more entrenched habitus. Some comparisons on the micro level of research policy can be made with the debate within research and responsible metrics and how to foster responsible applications of AI. AI strategy can learn from wider discussion on metrics, aiming to avoid further worsening the impact of metrics in higher education . Multidisciplinary academic teams should test the reliability of systems, whatever their domain of use, and this could encourage a fairer, more just, use of AI. Each potential application of AI will give rise to positives and negatives. Identifying what these positives and negatives are at a high level (e.g. their impact on early career researchers) is urgent. It is here that multiple stakeholders across the research system must exercise their agency (by deciding which systems to buy, build and use) and implement with care conscious of the lack of neutrality in technologies they seek to deploy in an already diverse community. There is also a need for futures research, anticipatory governance, and forecasting to develop a beneficial and supportive research culture where AI is part.
While AI is a tool for solving problems modelled as data-in-data-out processes, such problems represent a small fraction of human experience. The participants express concern about removing the ‘human’ from future research. Issues of interpretation, value, and principle ring out in discussions of emotional investment, fairness, and care for colleagues. It is critical to achieve quality over quantity. These concerns reassert the human character of research where research is much more about curiosity, exploration and fascination than it is about solving data-in-data-out problems. The danger and fear is that the desire for measurable research products will eclipse human considerations. We are therefore left with a choice as to how far AI is incorporated into future research and to what end. Currently, there is no clear strategy. As tools are developed they are embraced by some, rejected by others. There is insufficient information to guide best practice or consideration as to what the limits of AI application should be. It is unsurprising that the result is excitement and fear in equal measure. We need, perhaps, to step away from the relentless push towards greater measured productivity and ask more important questions about what we want for the future. These rest on a realistic view of what AI can and cannot do and a decoupling of truly effective research practice from measured research outcomes.
AI presents a challenge for research and researchers. Whilst AI may have a positive impact, the realisation of benefits relies on the actions and decisions of human users, and the research cultures in which it is designed and deployed. To find a useful and beneficial role for AI, wider stakeholder discussions are needed on the challenges posed by introducing AI into the research process and to reflect on where its use is inappropriate or disadvantageous for research or researchers. If AI is to be deployed responsibly, incentives need to be provided and there needs to be acceptance of the potential for disruption. AI might, as one of our participants stated, ‘ rock the boat ’ and there will be a divide between those that do and do not have the mindset that AI can assist in true productivity rather than simply replacing human academic labour. As discussed, knowledge production is entrenched in long standing scholarly norms and ideals and change can manifest fear among researchers, stimulating a drain of AI early career researchers to industry (and models motivated ultimately by questions of profit). There is work to do in terms of developing a research culture in which AI supports academic tasks in a way that is meaningful and edifying for researchers, and enriches the knowledge systems within which they operate. To do so, there is a need to interrogate and invoke the values and principles driving institutions. Universities can consider ways to improve the conditions for researchers to retain them.
AI can help research and researchers, but a deeper debate is required at all levels so as to avoid unintended negative consequences. This requires key players, e.g. funders, HE institutions, publishers and researchers to participate in leading and informing this debate, even leveraging initiatives such as those used to promote the responsible use of metrics in research (e.g. DORA and others), to extend into these areas. Alongside this, Global actors and signatories of consortia in Research on Research and meta-research, as well as scholars interested in the social implications of AI, must work with AI and Machine Learning scholars at all career stages and from varying backgrounds to help deepen and adapt the Responsible Research and Innovation discourse. The understanding of how AI can transform society has itself become a new multidisciplinary research field: AI Futures. Our explicit aims lay the ground for further much-needed exploration. More research is needed to establish a clear view of the role of AI in research. There is, then, a need for new narratives about the ways in which AI can support academic labour and help make sense of its introduction (Felt 2017 ). This includes addressing the systemic issues of research and HE and requires deeper foresight and futures research (Van Belkom 2020b ).
It will be pertinent to ask if AI can help to foster change and enable responsible practices in research and how AI can help us address long standing issues in research. Stakeholders across the research ecosystem will need to identify the values and virtues they wish to see in their institutions and turn inward to address the assumptions they make and the biases they propagate. Research is needed now to avoid a situation where AI simply allows us to “speed up” to “keep up” with an ever-increasing focus on measured research outputs. In an environment of increased research power, the human capacity for deciding what questions are worth pursuing becomes more valuable than ever.
Acknowledgements
We are grateful to Professor James Wilsdon at The University of Sheffield, Research on Research Institute for his critical feedback on this paper and to our interviewees who kindly gave up their time.
Author contributions
This paper’s lead author is Dr Jennifer Chubb.
This work was supported by the Digital Creativity Labs ( www.digitalcreativity.ac.uk ), jointly funded by EPSRC/AHRC/InnovateUK under Grant No. EP/M023265/1.
Data availability
Code availability, declarations.
This project has ethics approval from the University of York Ref: Chubb20200323.
All participants consented to participate following review from the University of York ethics committee for the Department of Computer Science Chubb20200323.
Not applicable.
1 https://www.gov.uk/government/publications/uk-research-and-development-roadmap .
2 https://www.nesta.org.uk/report/semantic-analysis-recent-evolution-ai-research/ .
3 The Research on Research Institute (RORI) consortium includes 21 partners, drawn from 13 countries or regions https://researchonresearch.org/ .
4 AGI. A contested term, https://intelligence.org/2013/08/11/what-is-agi/ , broadly AI with human level performance.
5 https://royalsociety.org/-/media/policy/projects/investing-in-uk-r-and-d/2019/investing-in-UK-r-and-d-may-2019.pdf .
Publisher's Note
Springer Nature remains neutral with regard to jurisdictional claims in published maps and institutional affiliations.
Contributor Information
Jennifer Chubb, Email: [email protected] .
Peter Cowling, Email: [email protected] .
Darren Reed, Email: [email protected] .
- Aiken RM, Epstein RG. Ethical guidelines for AI in education: starting a conversation. Int J Artif Intell Educ. 2000; 11 :163–176. [ Google Scholar ]
- AJE (2018) Peer review: how we found 15 million hours of lost time. https://www.aje.com/arc/peer-review-process-15-million-hours-lost-time/ . Accessed 18 Aug 2021
- Arntz M, Gregory T, Zierahn U (2016) The risk of automation for jobs in OECD countries: a comparative analysis. In: OECD social, employment and migration working papers, no. 189, OECD Publishing, Paris, 10.1787/5jlz9h56dvq7-en.
- Arruda JRF, Champieux R, Cook C, Davis MEK, Gedye R, Goodman L, et al. The journal impact factor and its discontents: steps toward responsible metrics and better research assessment. Open Scholarsh Initiat Proc. 2016 doi: 10.13021/G88304. [ CrossRef ] [ Google Scholar ]
- Ashwin P, Boud D, Calkins S, Coate K, Hallett F, Light G, et al. Reflective teaching in higher education. Bloomsbury Academic; 2020. [ Google Scholar ]
- Bacevic J. Universities in the neoliberal era. London: Palgrave Macmillan; 2017. Beyond the third mission: toward an actor-based account of universities’ relationship with society; pp. 21–39. [ Google Scholar ]
- Ball SJ. Performativity, commodification and commitment: an I-spy guide to the neoliberal university. Br J Educ Stud. 2012; 60 (1):17–28. doi: 10.1080/00071005.2011.650940. [ CrossRef ] [ Google Scholar ]
- Baum SD. On the promotion of safe and socially beneficial artificial intelligence. AI Soc. 2017; 32 (4):543–551. doi: 10.1007/s00146-016-0677-0. [ CrossRef ] [ Google Scholar ]
- Beer D. The social power of algorithms. Inf Commun Soc. 2017; 20 (1):1–13. doi: 10.1080/1369118X.2016.1216147. [ CrossRef ] [ Google Scholar ]
- Beer D. The data gaze: capitalism, power and perception. Sage; 2018. [ Google Scholar ]
- Beer D (2019) Should we use AI to make us quicker and more efficient researchers. https://blogs.lse.ac.uk/impactofsocialsciences/2019/10/30/should-we-use-ai-to-make-us-quicker-and-more-efficient-researchers/ . Accessed 18 Nov 2019
- Belfiore E. ‘Impact’, ‘value’ and ‘bad economics’: making sense of the problem of value in the arts and humanities. Arts Humanit High Educ. 2015; 14 (1):95–110. doi: 10.1177/1474022214531503. [ CrossRef ] [ Google Scholar ]
- Benjamin R. Race after technology: abolitionist tools for the new jim code. Social Forces. 2019; 98 :1–4. doi: 10.1093/sf/soz162. [ CrossRef ] [ Google Scholar ]
- Benneworth P. Putting impact into context: the Janus face of the public value of arts and humanities research. Arts Humanit High Educ. 2015; 14 (1):3–8. doi: 10.1177/1474022214533893. [ CrossRef ] [ Google Scholar ]
- Beyond Limits (2020) https://www.beyond.ai/news/artificial-intelligence-creates-more-jobs/ . Accessed 16 Nov 2020
- Bloom N, Jones CI, Van Reenen J, Webb M. Are ideas getting harder to find? Am Econ Rev. 2020; 110 (4):1104–44. doi: 10.1257/aer.20180338. [ CrossRef ] [ Google Scholar ]
- Bonn NA, Pinxten W. Advancing science or advancing careers? Researchers’ opinions on success indicator. PLoS ONE. 2020 doi: 10.1101/2020.06.22.165654. [ PMC free article ] [ PubMed ] [ CrossRef ] [ Google Scholar ]
- Börjeson L, Höjera M, Dreborgb K-H, Ekvallc T, Finnvedena G. Scenario types and techniques: towards a user’s guide. Futures. 2006; 38 :723–739. doi: 10.1016/j.futures.2005.12.002. [ CrossRef ] [ Google Scholar ]
- Bornmann L, Haunschild R. Alternative article-level metrics: the use of alternative metrics in research evaluation. EMBO Rep. 2018; 19 (12):e47260. doi: 10.15252/embr.201847260. [ PMC free article ] [ PubMed ] [ CrossRef ] [ Google Scholar ]
- Bostrom N. Superintelligence. Dunod; 2017. [ Google Scholar ]
- Bourdieu P. Homo academicus. Stanford University Press; 1988. [ Google Scholar ]
- Braun V, Clarke V. Using thematic analysis in psychology. Qual Res Psychol. 2006; 3 (2):77–101. doi: 10.1191/1478088706qp063oa. [ CrossRef ] [ Google Scholar ]
- Brynjolfsson E, Rock D, Syverson C. 1. Artificial intelligence and the modern productivity paradox: a clash of expectations and statistics. University of Chicago Press; 2019. pp. 23–60. [ Google Scholar ]
- Bryson J (2016) What are academics for? Can we be replaced by AI?. https://joanna-bryson.blogspot.com/2016/01/what-are-academics-for-can-we-be.html . Accessed 16 Nov 2020
- Bryson JJ. The Oxford handbook of ethics of artificial intelligence. Oxford University Press; 2019. The artificial intelligence of the ethics of artificial intelligence: an introductory overview for law and regulation. [ Google Scholar ]
- Caliskan A, Bryson JJ, Narayanan A. Semantics derived automatically from language corpora contain human-like biases. Science. 2017; 356 (6334):183–186. doi: 10.1126/science.aal4230. [ PubMed ] [ CrossRef ] [ Google Scholar ]
- Cave S, Craig C, Dihal K, Dillon S, Montgomery J, Singler B, Taylor L. Portrayals and perceptions of AI and why they matter. University of Cambridge; 2018. [ Google Scholar ]
- Cave S, Dihal K. Hopes and fears for intelligent machines in fiction and reality. Nat Mach Intell. 2019; 1 (2):74–78. doi: 10.1038/s42256-019-0020-9. [ CrossRef ] [ Google Scholar ]
- Cave S, Coughlan K, Dihal K (2019) “Scary Robots” examining public responses to AI. In: Proceedings of the 2019 AAAI/ACM conference on AI, ethics, and society, pp 331–337
- Cave S, Dihal K. The whiteness of AI. Philos Technol. 2020; 33 (4):685–703. doi: 10.1007/s13347-020-00415-6. [ CrossRef ] [ Google Scholar ]
- Cave S, Dihal K, Dillon S, editors. AI narratives: a history of imaginative thinking about intelligent machines. Oxford University Press; 2020. [ Google Scholar ]
- Checco A, Bracciale L, Loreti P, Pinfield S, Bianchi G. AI-assisted peer review. Hum Soc Sci Commun. 2021; 8 (1):1–11. [ Google Scholar ]
- Chubb J (2017) Academics fear the value of knowledge for its own sake is diminishing. https://theconversation.com/academics-fear-the-value-of-knowledge-for-its-own-sake-is-diminishing-75341 . Retrieved 4 Mar 2021
- Chubb J, Watermeyer R. Artifice or integrity in the marketization of research impact? Investigating the moral economy of (pathways to) impact statements within research funding proposals in the UK and Australia. Stud High Educ. 2017; 42 (12):2360–2372. doi: 10.1080/03075079.2016.1144182. [ CrossRef ] [ Google Scholar ]
- Clegg S. Academic identities under threat? Br Edu Res J. 2008; 34 (3):329–334. doi: 10.1080/01411920701532269. [ CrossRef ] [ Google Scholar ]
- Collini S. What are universities for? London: Penguin; 2012. [ Google Scholar ]
- Cope B, Kalantzis M, Searsmith D. Artificial intelligence for education: knowledge and its assessment in AI-enabled learning ecologies. Educ Philos Theory. 2020 doi: 10.1080/00131857.2020.1728732. [ CrossRef ] [ Google Scholar ]
- Cyranoki (2019) Artificial intelligence is selecting grant reviewers in China. https://www.nature.com/articles/d41586-019-01517-8 . Accessed 3 Mar 2021 [ PubMed ]
- Derrick G. The evaluators’ eye: impact assessment and academic peer review. Springer; 2018. [ Google Scholar ]
- Dhar (2020) Peer review of scholarly research gets an AI boost. https://spectrum.ieee.org/tech-talk/robotics/artificial-intelligence/peer-review-of-scholarly-research-gets-an-ai-boost . Accessed 16 Nov 2020
- Dillon S, Craig C (2022) Storylistening. https://www.routledge.com/Storylistening-Narrative-Evidence-and-Public-Reasoning/Dillon-Craig/p/book/9780367406738 . Accessed 18 August
- Dix G, Kaltenbrunner W, Tijdink JK, Valkenburg G, De Rijcke S. Algorithmic allocation: untangling rival conceptions of fairness in research management. Politics Gov. 2020; 8 (2):15–25. doi: 10.17645/pag.v8i2.2594. [ CrossRef ] [ Google Scholar ]
- Dommett K, Pearce W. What do we know about public attitudes towards experts? Reviewing survey data in the United Kingdom and European Union. Public Underst Sci. 2019; 28 (6):669–678. doi: 10.1177/0963662519852038. [ PubMed ] [ CrossRef ] [ Google Scholar ]
- Elton L. Goodhart’s law and performance indicators in higher education. Eval Res Educ. 2004; 18 (1–2):120–128. doi: 10.1080/09500790408668312. [ CrossRef ] [ Google Scholar ]
- Etzioni O (2016) AI impacts. https://aiimpacts.org/etzioni-2016-survey/ . Accessed 18 Aug 2021
- Felt U. Responsible innovation. Cham: Springer; 2017. “Response-able practices” or “new bureaucracies of virtue”: the challenges of making RRI work in academic environments; pp. 49–68. [ Google Scholar ]
- Fuller S. Review of the handbook of science and technology studies. Isis. 2009; 100 (1):207–209. doi: 10.1086/599701. [ CrossRef ] [ Google Scholar ]
- Gibbons M. Mode 2 society and the emergence of context-sensitive science. Sci Public Policy. 2000; 27 (3):159–163. doi: 10.3152/147154300781782011. [ CrossRef ] [ Google Scholar ]
- Grønsund T, Aanestad M. Augmenting the algorithm: emerging human-in-the-loop work configurations. J Strateg Inf Syst. 2020; 29 (2):101614. doi: 10.1016/j.jsis.2020.101614. [ CrossRef ] [ Google Scholar ]
- Grove J (2016) Robot-written reviews fool academics. In Times Higher Education. https://www.timeshighereducation.com/news/robot-written-reviews-fool-academics . Accessed 16 Nov 2021
- Gruber T. Academic sell-out: how an obsession with metrics and rankings is damaging academia. J Mark High Educ. 2014; 24 (2):165–177. doi: 10.1080/08841241.2014.970248. [ CrossRef ] [ Google Scholar ]
- Hagendorff T. The ethics of AI ethics: an evaluation of guidelines. Mind Mach. 2020; 30 (1):99–120. doi: 10.1007/s11023-020-09517-8. [ CrossRef ] [ Google Scholar ]
- Harris S. Rethinking academic identities in neo-liberal times. Teach High Educ. 2005; 10 (4):421–433. doi: 10.1080/13562510500238986. [ CrossRef ] [ Google Scholar ]
- Heaven D (2018) AI peer reviewers unleashed to ease publishing grind. https://www.nature.com/articles/d41586-018-07245-9 . Accessed 18 Aug 2021 [ PubMed ]
- Herschberg C, Benschop Y, Van den Brink M. Precarious postdocs: a comparative study on recruitment and selection of early-career researchers. Scand J Manag. 2018; 34 (4):303–331. doi: 10.1016/j.scaman.2018.10.001. [ CrossRef ] [ Google Scholar ]
- Hicks et al. (2015) https://www.nature.com/news/bibliometrics-the-leiden-manifesto-for-research-metrics-1.17351 . Accessed 12 Mar 2021
- Hill S (2018) Loomming REF deadlines lead to a rush in publication of lower quality research. https://blogs.lse.ac.uk/impactofsocialsciences/2018/03/15/looming-ref-deadlines-lead-to-a-rush-inpublication-of-lower-quality-research/ . Accessed 18 Aug 2021
- Holbrook JB, Hrotic S. Blue skies, impacts, and peer review. RT J Res Policy Eval. 2013 doi: 10.3130/2282-5398/2914. [ CrossRef ] [ Google Scholar ]
- Holmwood J. Sociology’s misfortune: disciplines, interdisciplinarity and the impact of audit culture. Br J Sociol. 2010; 61 (4):639–658. doi: 10.1111/j.1468-4446.2010.01332.x. [ PubMed ] [ CrossRef ] [ Google Scholar ]
- Hukkinen J (2017) Peer review has its shortcomings, but AI is a risky fix. https://www.wired.com/2017/01/peer-review-shortcomings-ai-risky-fix/ . Accessed 18 Aug 2021
- Ivancheva M, Lynch K, Keating K. Precarity, gender and care in the neoliberal academy. Gend Work Organ. 2019; 26 (4):448–462. doi: 10.1111/gwao.12350. [ CrossRef ] [ Google Scholar ]
- Jenkins N, Bloor M, Fischer J, Berney L, Neale J. Putting it in context: the use of vignettes in qualitative interviewing. Qual Res. 2010; 10 (2):175–198. doi: 10.1177/1468794109356737. [ CrossRef ] [ Google Scholar ]
- Jobin A, Ienca M, Vayena E. The global landscape of AI ethics guidelines. Nat Mach Intell. 2019; 1 (9):389–399. doi: 10.1038/s42256-019-0088-2. [ CrossRef ] [ Google Scholar ]
- Jowett A (2020) Carrying out qualitative research under lockdown—practical and ethical considerations. https://blogs.lse.ac.uk/impactofsocialsciences/2020/04/20/carrying-out-qualitative-research-under-lockdown-practical-and-ethical-considerations/ . Accessed 16 Nov 2020
- Kagermann H, Wahlster W, Helbig J (Eds) Recommendations for implementing the strategic initiative Industrie 4.0. In: Final report of the Industrie 4.0 Working Group, 2013.
- Lee S, Bozeman B. The impact of research collaboration on scientific productivity. Soc Stud Sci. 2005; 35 (5):673–702. doi: 10.1177/0306312705052359. [ CrossRef ] [ Google Scholar ]
- Lee CJ, Sugimoto CR, Zhang G, et al. Bias in peer review. J Am Soc Inform Sci Technol. 2013; 64 (1):2–17. doi: 10.1002/asi.22784. [ CrossRef ] [ Google Scholar ]
- Luger E (2021). https://www.designinformatics.org/person/ewa-luger/ . Accessed 18 Aug 2021
- Martell L. LSE review of books. LSE; 2017. Book review: accelerating academia: the changing structure of academic time by Filip Vostal. [ Google Scholar ]
- Martin BR. The research excellence framework and the ‘impact agenda’: are we creating a Frankenstein monster? Res Eval. 2011; 20 (3):247–254. doi: 10.3152/095820211X13118583635693. [ CrossRef ] [ Google Scholar ]
- Mazali T. From industry 4.0 to society 4.0, there and back. AI Soc. 2018; 33 (3):405–411. doi: 10.1007/s00146-017-0792-6. [ CrossRef ] [ Google Scholar ]
- McCarthy J, Minsky ML, Rochester N, Shannon CE. A proposal for the dartmouth summer research project on artificial intelligence. AI Mag. 1955; 27 (4):12. [ Google Scholar ]
- Miles MB, Huberman AM, Saldaña J. Qualitative data analysis: a methods sourcebook. 3. SAGE Publications; 2014. [ Google Scholar ]
- Mittelstadt BD, Allo P, Taddeo M, Wachter S, Floridi L (2016) The ethics of algorithms: Mapping the debate. Big Data Soc 3(2). 10.1177/2053951716679679
- Mrowinski MJ, Fronczak P, Fronczak A, Ausloos M, Nedic O. Artificial intelligence in peer review: how can evolutionary computation support journal editors? PLoS ONE. 2017; 12 (9):e0184711. doi: 10.1371/journal.pone.0184711. [ PMC free article ] [ PubMed ] [ CrossRef ] [ Google Scholar ]
- Muller-Heyndyk (2018) Workers fear losing their jobs to AI. from https://www.hrmagazine.co.uk/article-details/workers-fear-losing-their-jobs-to-ai#:~:text=Over%20a%20third%20of%20workers,needed%2C%20according%20to%20YouGov%20research . Accessed 18 Aug 2021
- NESTA (2019) A semantic analysis of the recent evolution of AI research. https://www.nesta.org.uk/report/semantic-analysis-recent-evolution-ai-research/ . Accessed 8 Aug 2021
- Nowotny H, Scott P, Gibbons M. Introduction: ‘Mode 2’ revisited: the new production of knowledge. Minerva. 2003; 41 (3):179–194. doi: 10.1023/A:1025505528250. [ CrossRef ] [ Google Scholar ]
- Nuijten MB, Hartgerink CH, Van Assen MA, Epskamp S, Wicherts JM. The prevalence of statistical reporting errors in psychology (1985–2013) Behav Res Methods. 2016; 48 (4):1205–1226. doi: 10.3758/s13428-015-0664-2. [ PMC free article ] [ PubMed ] [ CrossRef ] [ Google Scholar ]
- Olssen M, Peters MA. Neoliberalism, higher education and the knowledge economy: from the free market to knowledge capitalism. J Educ Policy. 2005; 20 (3):313–345. doi: 10.1080/02680930500108718. [ CrossRef ] [ Google Scholar ]
- Park SC. The fourth industrial revolution and implications for innovative cluster policies. AI Soc. 2018; 33 (3):433–445. doi: 10.1007/s00146-017-0777-5. [ CrossRef ] [ Google Scholar ]
- Pasquale F. New laws of robotics: defending human expertise in the age of AI. Belknap Press; 2020. [ Google Scholar ]
- Powell K. Does it take too long to publish research? Nature. 2016; 530 :148–151. doi: 10.1038/530148a. [ PubMed ] [ CrossRef ] [ Google Scholar ]
- Procter R, Glover B, Jones E (2020) Research 4.0—research in the age of automation. Demos, September 2020. https://demos.co.uk/wp-content/uploads/2020/09/Research-4.0-Report.pdf
- Reed MS, Stringer LC, Fazey I, Evely AC, Kruijsen JH. Five principles for the practice of knowledge exchange in environmental management. J Environ Manag. 2014; 146 :337–345. doi: 10.1016/j.jenvman.2014.07.021. [ PubMed ] [ CrossRef ] [ Google Scholar ]
- Reese B (2019) AI will create millions more jobs than it will destroy. Here’s How. https://singularityhub.com/2019/01/01/ai-will-create-millions-more-jobs-than-it-will-destroy-heres-how/ . Accessed 16 Nov 2020
- Röösli E, Rice B, Hernandez-Boussard T. Bias at warp speed: how AI may contribute to the disparities gap in the time of COVID-19. J Am Med Inf Assoc. 2021; 28 (1):190–192. doi: 10.1093/jamia/ocaa210. [ PMC free article ] [ PubMed ] [ CrossRef ] [ Google Scholar ]
- Samuel G, Derrick G. Defining ethical standards for the application of digital tools to population health research. Bull World Health Organ. 2020; 98 (4):239. doi: 10.2471/BLT.19.237370. [ PMC free article ] [ PubMed ] [ CrossRef ] [ Google Scholar ]
- Samuel G, Chubb J, Derrick G. Boundaries between research ethics and ethical research use in artificial intelligence health research. J Empir Res Hum Res Ethics. 2021 doi: 10.1177/15562646211002744. [ PMC free article ] [ PubMed ] [ CrossRef ] [ Google Scholar ]
- Schwab K. The fourth industrial revolution. Currency; 2017. [ Google Scholar ]
- Serholt S, Barendregt W, Vasalou A, Alves-Oliveira P, Jones A, Petisca S, Paiva A. The case of classroom robots: teachers’ deliberations on the ethical tensions. AI Soc. 2017; 32 (4):613–631. doi: 10.1007/s00146-016-0667-2. [ CrossRef ] [ Google Scholar ]
- Simmel G. The view of life: four metaphysical essays with journal aphorisms. Chicago: Chicago University Press; 2010. [ Google Scholar ]
- Spezi V, Wakeling S, Pinfield S, et al. “Let the community decide”? The vision and reality of soundness-only peer review in open-access mega-journals. J Doc. 2018; 74 (1):137–161. doi: 10.1108/JD-06-2017-0092. [ CrossRef ] [ Google Scholar ]
- Stathoulopoulos K, Mateos-Garcia JC (2019) Gender diversity in AI research. Available at SSRN 3428240.
- The Royal Society (2018a) The AI revolution in scientific research. https://royalsociety.org/-/media/policy/projects/ai-and-society/AI-revolution-in-science.pdf?la=en-GB&hash=5240F21B56364A00053538A0BC29FF5F . Retrieved 16 Nov 2020
- The Royal Society (2018b) The impact of artificial intelligence on work. https://royalsociety.org/-/media/policy/projects/ai-and-work/evidence-synthesis-the-impact-of-AI-on-work.PDF . Accessed 16 Nov 2020
- The Royal Society (2019) The AI revolution in science. https://royalsociety.org/-/media/policy/projects/ai-and-society/AI-revolution-in-science.pdf?la=en-GB&hash=5240F21B56364A00053538A0BC29FF5F . Accessed 8 Aug 2021
- The Royal Society (2020) Research culture. https://royalsociety.org/topics-policy/projects/research-culture/#:~:text=Research%20culture%20encompasses%20the%20behaviours,research%20is%20conducted%20and%20communicated . Retrieved 16 Nov 2020
- Tsamados A, Aggarwal N, Cowls J, Morley J, Roberts H, Taddeo M, Floridi L. The ethics of algorithms: key problems and solutions. AI Soc. 2021 doi: 10.1007/s00146-021-01154-8. [ CrossRef ] [ Google Scholar ]
- UKRI (2020) Corporate plan, 2020–21. https://www.ukri.org/wp-content/uploads/2020/10/UKRI-091020-CorporatePlan2020-21.pdf . Accessed 17 Nov 2020
- UKRI (2021) Transforming our world with AI. https://www.ukri.org/wp-content/uploads/2021/02/UKRI-120221-TransformingOurWorldWithAI.pdf . Accessed 16 Mar 2021
- Van Belkom R. The impact of artificial intelligence on the activities of a futurist. World Futur Rev. 2020; 12 (2):156–168. doi: 10.1177/1946756719875720. [ CrossRef ] [ Google Scholar ]
- Van Belkon R (2020b) AI no longer has a plug: about ethics in the design process. https://www.researchgate.net/publication/343106745_AI_no_longer_has_a_plug_about_ethics_in_the_design_process . Accessed 16 Nov 2020
- Viglione G. China is closing gap with United States on research spending. Nature. 2020 doi: 10.1038/d41586-020-00084-7. [ PubMed ] [ CrossRef ] [ Google Scholar ]
- Vostal F. Accelerating academia: the changing structure of academic time. Springer; 2016. [ Google Scholar ]
- Weis JW, Jacobson JM. Learning on knowledge graph dynamics provides an early warning of impactful research. Nat Biotechnol. 2021 doi: 10.1038/s41587-021-00907-6. [ PubMed ] [ CrossRef ] [ Google Scholar ]
- Wellcome Trust (2020) What researchers think about the culture they work in. https://wellcome.org/sites/default/files/what-researchers-think-about-the-culture-they-work-in.pd . Accessed 17 Nov 2020
- Williamson B. Governing software: networks, databases and algorithmic power in the digital governance of public education. Learn Media Technol. 2015; 40 (1):83–105. doi: 10.1080/17439884.2014.924527. [ CrossRef ] [ Google Scholar ]
- Wilsdon J (2021) AI & machine learning in research assessment: can we draw lessons from debates over responsible metrics? In: RoRI & RCN workshop, Act One, 11 January 2021. https://figshare.shef.ac.uk/account/articles/14258495 . Accessed 22 Mar 2021
- Wilsdon J, Allen L, Belfiore E, Campbell P, Curry S, Hill S et al. (2015) The metric tide. In: Report of the independent review of the role of metrics in research assessment and management.
- Wilsdon JR, Bar-Ilan J, Frodeman R, Lex E, Peters I, Wouters P (2017) Next-generation metrics: responsible metrics and evaluation for open science.
- Yee S. “You bet she can fuck”–trends in female AI narratives within mainstream cinema: Ex Machina and Her. Ekphrasis. 2017; 17 (1):85–98. doi: 10.24193/ekphrasis.17.6. [ CrossRef ] [ Google Scholar ]
- Zajko M. Conservative AI and social inequality: conceptualizing alternatives to bias through social theory. AI Soc. 2021 doi: 10.1007/s00146-021-01153-9. [ CrossRef ] [ Google Scholar ]
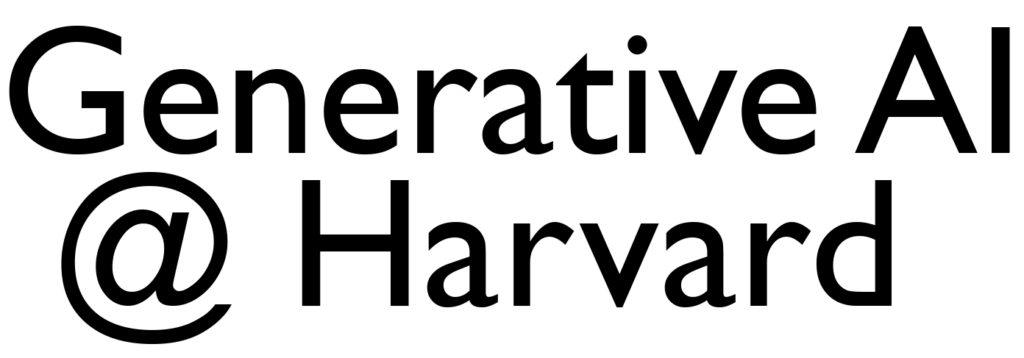
Research with Generative AI
Resources for scholars and researchers
Generative AI (GenAI) technologies offer new opportunities to advance research and scholarship. This resource page aims to provide Harvard researchers and scholars with basic guidance, information on available resources, and contacts. The content will be regularly updated as these technologies continue to evolve. Your feedback is welcome.
Leading the way
Harvard’s researchers are making strides not only on generative AI, but the larger world of artificial intelligence and its applications. Learn more about key efforts.
The Kempner Institute
The Kempner Institute is dedicated to revealing the foundations of intelligence in both natural and artificial contexts, and to leveraging these findings to develop groundbreaking technologies.
Harvard Data Science Initiative
The Harvard Data Science Initiative is dedicated to understanding the many dimensions of data science and propelling it forward.
More AI @ Harvard
Generative AI is only part of the fascinating world of artificial intelligence. Explore Harvard’s groundbreaking and cross-disciplinary academic work in AI.
funding opportunity
GenAI Research Program/ Summer Funding for Harvard College Students 2024
The Office of the Vice Provost for Research, in partnership with the Office of Undergraduate Research and Fellowships, is pleased to offer an opportunity for collaborative research projects related to Generative AI between Harvard faculty and undergraduate students over the summer of 2024.
Learn more and apply
Frequently asked questions
Can i use generative ai to write and/or develop research papers.
Academic publishers have a range of policies on the use of AI in research papers. In some cases, publishers may prohibit the use of AI for certain aspects of paper development. You should review the specific policies of the target publisher to determine what is permitted.
Here is a sampling of policies available online:
- JAMA and the JAMA Network
- Springer Nature
How should AI-generated content be cited in research papers?
Guidance will likely develop as AI systems evolve, but some leading style guides have offered recommendations:
- The Chicago Manual of Style
- MLA Style Guide
Should I disclose the use of generative AI in a research paper?
Yes. Most academic publishers require researchers using AI tools to document this use in the methods or acknowledgements sections of their papers. You should review the specific guidelines of the target publisher to determine what is required.
Can I use AI in writing grant applications?
You should review the specific policies of potential funders to determine if the use of AI is permitted. For its part, the National Institutes of Health (NIH) advises caution : “If you use an AI tool to help write your application, you also do so at your own risk,” as these tools may inadvertently introduce issues associated with research misconduct, such as plagiarism or fabrication.
Can I use AI in the peer review process?
Many funders have not yet published policies on the use of AI in the peer review process. However, the National Institutes of Health (NIH) has prohibited such use “for analyzing and formulating peer review critiques for grant applications and R&D contract proposals.” You should carefully review the specific policies of funders to determine their stance on the use of AI
Are there AI safety concerns or potential risks I should be aware of?
Yes. Some of the primary safety issues and risks include the following:
- Bias and discrimination: The potential for AI systems to exhibit unfair or discriminatory behavior.
- Misinformation, impersonation, and manipulation: The risk of AI systems disseminating false or misleading information, or being used to deceive or manipulate individuals.
- Research and IP compliance: The necessity for AI systems to adhere to legal and ethical guidelines when utilizing proprietary information or conducting research.
- Security vulnerabilities: The susceptibility of AI systems to hacking or unauthorized access.
- Unpredictability: The difficulty in predicting the behavior or outcomes of AI systems.
- Overreliance: The risk of relying excessively on AI systems without considering their limitations or potential errors.
See Initial guidelines for the use of Generative AI tools at Harvard for more information.
- Initial guidelines for the use of Generative AI tools at Harvard
Generative AI tools
- Explore Tools Available to the Harvard Community
- System Prompt Library
- Request API Access
- Request a Vendor Risk Assessment
- Questions? Contact HUIT
Copyright and intellectual property
- Copyright and Fair Use: A Guide for the Harvard Community
- Copyright Advisory Program
- Intellectual Property Policy
- Protecting Intellectual Property
Data security and privacy
- Harvard Information Security and Data Privacy
- Data Security Levels – Research Data Examples
- Privacy Policies and Guidelines
Research support
- University Research Computing and Data (RCD) Services
- Research Administration and Compliance
- Research Computing
- Research Data and Scholarship
- Faculty engaged in AI research
- Centers and initiatives engaged in AI research
- Degree and other education programs in AI
Thank you for visiting nature.com. You are using a browser version with limited support for CSS. To obtain the best experience, we recommend you use a more up to date browser (or turn off compatibility mode in Internet Explorer). In the meantime, to ensure continued support, we are displaying the site without styles and JavaScript.
- View all journals
- Explore content
- About the journal
- Publish with us
- Sign up for alerts
- Published: 24 February 2023
Artificial intelligence in academic writing: a paradigm-shifting technological advance
- Roei Golan ORCID: orcid.org/0000-0002-7214-3073 1 na1 ,
- Rohit Reddy 2 na1 ,
- Akhil Muthigi 2 &
- Ranjith Ramasamy 2
Nature Reviews Urology volume 20 , pages 327–328 ( 2023 ) Cite this article
3816 Accesses
26 Citations
61 Altmetric
Metrics details
- Preclinical research
- Translational research
Artificial intelligence (AI) has rapidly become one of the most important and transformative technologies of our time, with applications in virtually every field and industry. Among these applications, academic writing is one of the areas that has experienced perhaps the most rapid development and uptake of AI-based tools and methodologies. We argue that use of AI-based tools for scientific writing should widely be adopted.
This is a preview of subscription content, access via your institution
Relevant articles
Open Access articles citing this article.
How artificial intelligence will affect the future of medical publishing
- Jean-Louis Vincent
Critical Care Open Access 06 July 2023
Access options
Access Nature and 54 other Nature Portfolio journals
Get Nature+, our best-value online-access subscription
24,99 € / 30 days
cancel any time
Subscribe to this journal
Receive 12 print issues and online access
195,33 € per year
only 16,28 € per issue
Buy this article
- Purchase on Springer Link
- Instant access to full article PDF
Prices may be subject to local taxes which are calculated during checkout
Checco, A., Bracciale, L., Loreti, P., Pinfield, S. & Bianchi, G. AI-assisted peer review. Humanit. Soc. Sci. Commun. 8 , 25 (2021).
Article Google Scholar
Hutson, M. Could AI help you to write your next paper? Nature 611 , 192–193 (2022).
Article CAS PubMed Google Scholar
Krzastek, S. C., Farhi, J., Gray, M. & Smith, R. P. Impact of environmental toxin exposure on male fertility potential. Transl Androl. Urol. 9 , 2797–2813 (2020).
Article PubMed PubMed Central Google Scholar
Khullar, D. Social media and medical misinformation: confronting new variants of an old problem. JAMA 328 , 1393–1394 (2022).
Article PubMed Google Scholar
Reddy, R. V. et al. Assessing the quality and readability of online content on shock wave therapy for erectile dysfunction. Andrologia 54 , e14607 (2022).
Khodamoradi, K., Golan, R., Dullea, A. & Ramasamy, R. Exosomes as potential biomarkers for erectile dysfunction, varicocele, and testicular injury. Sex. Med. Rev. 10 , 311–322 (2022).
Stone, L. You’ve got a friend online. Nat. Rev. Urol. 17 , 320 (2020).
PubMed Google Scholar
Pai, R. K. et al. A review of current advancements and limitations of artificial intelligence in genitourinary cancers. Am. J. Clin. Exp. Urol. 8 , 152–162 (2020).
PubMed PubMed Central Google Scholar
You, J. B. et al. Machine learning for sperm selection. Nat. Rev. Urol. 18 , 387–403 (2021).
Stone, L. The dawning of the age of artificial intelligence in urology. Nat. Rev. Urol. 18 , 322 (2021).
Download references
Acknowledgements
The manuscript was edited for grammar and structure using the advanced language model ChatGPT. The authors thank S. Verma for addressing inquiries related to artificial intelligence.
Author information
These authors contributed equally: Roei Golan, Rohit Reddy.
Authors and Affiliations
Department of Clinical Sciences, Florida State University College of Medicine, Tallahassee, FL, USA
Desai Sethi Urology Institute, University of Miami Miller School of Medicine, Miami, FL, USA
Rohit Reddy, Akhil Muthigi & Ranjith Ramasamy
You can also search for this author in PubMed Google Scholar
Corresponding author
Correspondence to Ranjith Ramasamy .
Ethics declarations
Competing interests.
R.R. is funded by the National Institutes of Health Grant R01 DK130991 and the Clinician Scientist Development Grant from the American Cancer Society. The other authors declare no competing interests.
Additional information
Related links.
ChatGPT: https://chat.openai.com/
Cohere: https://cohere.ai/
CoSchedule Headline Analyzer: https://coschedule.com/headline-analyzer
DALL-E 2: https://openai.com/dall-e-2/
Elicit: https://elicit.org/
Penelope.ai: https://www.penelope.ai/
Quillbot: https://quillbot.com/
Semantic Scholar: https://www.semanticscholar.org/
Wordtune by AI21 Labs: https://www.wordtune.com/
Writefull: https://www.writefull.com/
Rights and permissions
Reprints and permissions
About this article
Cite this article.
Golan, R., Reddy, R., Muthigi, A. et al. Artificial intelligence in academic writing: a paradigm-shifting technological advance. Nat Rev Urol 20 , 327–328 (2023). https://doi.org/10.1038/s41585-023-00746-x
Download citation
Published : 24 February 2023
Issue Date : June 2023
DOI : https://doi.org/10.1038/s41585-023-00746-x
Share this article
Anyone you share the following link with will be able to read this content:
Sorry, a shareable link is not currently available for this article.
Provided by the Springer Nature SharedIt content-sharing initiative
This article is cited by
Techniques for supercharging academic writing with generative ai.
- Zhicheng Lin
Nature Biomedical Engineering (2024)
Critical Care (2023)
What do academics have to say about ChatGPT? A text mining analytics on the discussions regarding ChatGPT on research writing
- Rex Bringula
AI and Ethics (2023)
Quick links
- Explore articles by subject
- Guide to authors
- Editorial policies
Sign up for the Nature Briefing: Translational Research newsletter — top stories in biotechnology, drug discovery and pharma.

A free, AI-powered research tool for scientific literature
- Barbara L. Fredrickson
- Group Dynamics
New & Improved API for Developers
Introducing semantic reader in beta.
Stay Connected With Semantic Scholar Sign Up What Is Semantic Scholar? Semantic Scholar is a free, AI-powered research tool for scientific literature, based at the Allen Institute for AI.
Research & Innovation
Office of the vice president for research & innovation (ovpri), generative ai in academic research: perspectives and cultural norms.
Download the full report as a PDF: Generative AI in Academic Research: Perspectives and Cultural Norms ( PDF)
- Executive Summary
- Introduction
- Framework for Using Generative AI in Research
- GenAI for Literature Review
- GenAI for Research Infrastructure
- GenAI for Data Collection and Generation
- GenAI for Ideation & Hypothesis Generation
B. Research Dissemination Stage
C. research translation stage, d. research funding & funding agreement compliance stage, considerations for the cornell research community.
- Considerations for Cornell Leadership
- A. Research Ideation and Execution Stage
D. Research Funding and Funding Agreement Compliance Stage
- Appendix 1. Existing Community Publication Policies
- Appendix 2. References Consulted or Cited
- Appendix 3. Task Force Charge
E xecutive Summary
This report offers perspectives and practical guidelines to the Cornell community, specifically on the use of Generative Artificial Intelligence (GenAI) in the practice and dissemination of academic research. As emphasized in the charge to a Cornell task force representing input across all campuses, the report aims to establish the initial set of perspectives and cultural norms for Cornell researchers, research team leaders, and research administration staff. It is meant as internal advice rather than a set of binding rules. As GenAI policies and guardrails are rapidly evolving, we stress the importance of staying current with the latest developments, and updating procedures and rules governing the use of GenAI tools in research thoughtfully over time. This report was developed within the same 12-month period that GenAI became available to a much wider number of researchers (and citizens) than AI specialists who help create such tools. While the Cornell community is the intended audience, this report is publicly available as a resource for other research communities to use or adapt. No endorsement of specific tools is implied, but specific examples are referenced to illustrate concepts.
Recognizing many potential benefits and risks of GenAI tools, we address the use of GenAI at four stages of the research process: (i) research conception and execution stage, (ii) research dissemination stage, (iii) research translation stage, and (iv) research funding and funding agreement compliance stage. We further outline coordinating duties by researchers that apply across these stages: duty of discretion, duty of verification, and duty of disclosure; identify categories of GenAI use in research; and illustrate how these duties apply to specific categories and situations in the research process. We emphasize the importance of clearly defining individual and collective/communal responsibilities for meeting these duties throughout the research process. We conclude by offering a set of guidelines for Cornell researchers in varied faculty, staff, and student roles, as well as considerations for Cornell leadership. It is important that Cornell offers its research community access to appropriate GenAI tools and resources, particularly to improve our “AI literacy” regarding the limits of the appropriate use of specific public and commercial GenAI tools and the risks involved in their use for academic research. It is equally important that researchers have Cornell-facilitated access to licensed GenAI tools with privacy/confidentiality provisions, and thus important that Cornell researchers from varied communities understand the value, limitations, and trade-offs of using such tools in research.
The report also contains responses to anticipated questions about best practices and use cases for each of the four stages of research (Appendix 0) that may serve as discussion starters for research communities. Finally, we offer a summary of existing community publication policies regarding the use of GenAI in research from funders, journals, professional societies, and peers, which we surveyed as part of the preparation of this report (Appendix 1); references consulted and cited that include a list of recommended resources (Appendix 2); and task force charge (Appendix 3). Notably, the task force included Cornell faculty and staff quite familiar with GenAI tools and uses, and the task force elected to not use GenAI in drafting the structure, text, or figures of this report.
I ntroduction
Generative Artificial Intelligence (GenAI) offers transformative capabilities, but we must strike a balance between exploring the potential of these tools and ensuring that research meets standards of veracity, validity, originality, and reproducibility. Briefly, GenAI has the capacity to generate new content (new text or images or audio), typically by computer-generated pattern recognition gleaned from access to large volumes of prior examples. These prior examples, data collectively called training sets or training data, can be provided by the GenAI user or provided by others, with or without their explicit awareness or consent.
This exciting capability to spark new ideas from prior knowledge, perhaps now connected in unexpected new ways, can now be accessed by the masses via online tools and for-fee apps. These users include the masses of academic researchers with a shared sense of research integrity, but with widely varied experience in computer programming and in cultural norms for creation, authorship, and invention. Many of these tools, whether “free to the user” or fee-based, have been released by for-profit companies that maintain the model details as proprietary (i.e., do not disclose details of the trained models or the training data sets that serve as the basis for the GenAI output). Open-source approaches for GenAI development can be a counterpoint that provides a more transparent toolset, but are not an automatic panacea to responsible development or use of such tools in academic research. Thus, we need to develop common ground and guardrails that prioritize research integrity, accelerate innovation, address obvious issues like data privacy and security, and reflect on non-obvious issues like how practices and expertise of research communities will evolve for better and worse. No one policy can cover the range of research carried out at a university, from archives to surveys to experimental labs to pure math and the visual arts. GenAI capabilities and affordances are also changing from month to month, only a year into publicly available and initially free versions, but for the cost of providing your own email address or mobile phone number to a for-profit company. External to Cornell, GenAI policies and guardrails are rapidly evolving, and procedures and rules governing the use of GenAI tools in the research enterprise should be regularly updated to stay current with the latest developments. Internal to Cornell, aligning practices for GenAI use with existing policies on research data and with our institutional values will also remain a work in progress for years to come.
GenAI has many potential benefits for researchers at all stages of their research career, for administrative staff who provide key support to but do not participate in research and translation activities directly, and for other users in the course of conducting and administering research. These include:
- Abstractions. Many systems for data analysis and document retrieval have been available only to those with substantial programming experience. Likewise, systems for creative audiovisual generation have been limited to specialists or those with years of content production experience. GenAI tools can provide powerful results with interfaces accessible to anyone.
- Efficiency. Even when researchers, administrative staff, and other users have the capability to perform a task, GenAI may be able to produce comparable output in dramatically less time, allowing users to focus on more difficult, human interaction-intensive, and/or rewarding tasks.
- Scale . GenAI may allow users to perform a task such as coding/annotating documents or generating infographic images on a larger scale than would be possible with manual effort. This ability may allow users to explore larger or broader data sets that were previously limited.
At the same time, we have identified several concerns that apply across the wide range of research disciplines at Cornell and represented internationally. These include:
- Blind spots and potential bias. All current generative language models are entirely defined by their training data, and thus perpetuate the omissions and biases of that training data. The model behind ChatGPT has no access to information that was not presented during training, and can only access that information through learned combinations of parameters. There is no explicit and verifiable representation of data or text encoded in the model. Other GenAI systems, such as GPT4, may increasingly have access to web searches and databases to retrieve verifiable sources, and the underlying language model may be able to interact with text returned from those searches (in the same way it interacts with user queries), but the model itself still has no “knowledge” beyond the statistical regularities of its training data.
- Validation and responsibility. There is a risk that systems are good enough that users will become trusting and complacent, but bad enough that there are serious problems that have profound consequences. A system that produces seemingly plausible answers, yet is prone to false and biased information, can cause researchers to lower their guard. Therefore, we emphasize the crucial role of researcher validation of research outputs produced with the help of GenAI tools. Responsibility is an area that is particularly sensitive to discipline-specific variation. Most fields have tacit understandings of roles and responsibilities (e.g., principal investigator, Ph.D. student, corresponding author), which may differ substantially from those in even closely related fields.
- Transparency and documentation. Guidelines for the use of GenAI in research vary greatly across journals, funding agencies, and professional associations, from blanket bans to restrictions on certain outputs to permissions with disclosure (most with the emphasis that AI-generated outputs should be original, i.e., reflecting the authors’ actual work). Laws and regulations for patents, copyright and data use are evolving and vary among countries. As the policies regarding the use of GenAI continue to evolve, maintaining documentation and reporting transparency will remain critical to ensure the reproducibility and replicability of research findings produced with the help of GenAI tools.
- Data privacy and protection. GenAI tools should not be assumed a priori to be private or secure. Users must understand the potential risks associated with inputting sensitive, private, confidential, or proprietary data into these tools, and that doing so may violate legal or contractual requirements, or expectations for privacy.
- Resource utilization tradeoffs. Because GenAI users perceive the output of such tools to be a “free good” or at least generated “in the cloud” even when user fee-based, as with research travel impacts the resource utilization of this computational output can be out of sight and out of mind. However, the magnitude of computational resources operating on Earth to create models based on large volumes of training data, and the electricity use and potential cooling water use associated with such computational processing, can be in tension with values associated with sustainability. A recent study posted on arXiv and currently under peer review attempts to quantify this resource tradeoff in terms of carbon dioxide emissions, equating the carbon cost for you to generate a single image using a specific energy-intensive GenAI model to that required to fully charge your mobile phone (Luccioni et al., 2023 preprint). (CITE: https://arxiv.org/pdf/2311.16863.pdf ). Certainly, other research-related activities may contribute more significantly on a per-instance basis (e.g., a research group flying to present at an overseas conference). However, Cornell’s public commitment to climate action and our individual sense of responsibility for our own resource use choices benefit from our shared awareness that the use phase (or inference phase) of GenAI can be estimated, is non-zero and will remain so without concerted effort, and naturally scales with access to computational resources.
F ramework for Using Generative AI in Research
The epochal developments of the past five years in GenAI have enabled systems to generate complex, recognizable outputs such as text, programming code, images, and voices. AI as a field has been around for decades, but the output of systems has often been narrow, binary predictions: whether an email is spam, or whether a transaction is likely to be fraudulent. GenAI offers dramatic
new capabilities, generating output in response to prompts (i.e., questions, requests, instructions) from the user. See inset: What are generative uses of AI?
GenAI provides the user a sense of power in its apparent intellectual assistance on demand, which unsurprisingly also vests the user with a need to take responsibility. Academic research groups and projects often include multiple users with different stages of contribution, different degrees of experience and leadership, and different responsibilities to research integrity and translation of research results to societal impact. Thus, we begin with the following general framework describing categories of uses of GenAI in research and categories of duties that researchers may have.
There are many levels of potential uses in research, ranging from surface level adjustments to applications that blur the boundary of authorship. At one extreme, we might consider systems that simulate a copyeditor, correcting spelling or grammar, which are already integrated in many word processing systems. At the other extreme might be a system that acts as a ghost writer, converting vague descriptions and specifications into polished final presentations. In between, systems might act more like research assistants, collecting and collating information, writing short computer programs, or filling in paragraph bodies from thesis statements. Other uses might be more like reviewers or editors, enabling researchers to “bounce ideas” or summarize a passage of text to ensure that it reads correctly.
These uses imply corresponding duties by researchers. Most high-performance GenAI systems are currently available as third-party (i.e., company product, not university-managed resource) cloud (i.e., using remotely located computers) applications, so there is a researcher duty of discretion in what data should be uploaded. GenAI, while usually convincing and fluent (at least in English), is often factually incorrect or lacking in attribution, so verification is another key duty to ensure accuracy and validity of research outputs. Researchers may also have a duty to provide transparency and disclosure to identify how and where GenAI contributed. Finally, we need clear lines of individual and collective responsibility to ensure that the other duties are actually executed.
For the remainder of this report we will identify specific situations in the research process, and describe how they relate to these categories of use and what duties we believe apply in academic research – and are consistent with Cornell shared values. In all of these research stages, we consider GenAI to be a useful research tool that can and should be explored and used to great scholarly advantage. As with all tools, the user is responsible for understanding how to use such tools wisely. As with all academic research, the responsibilities are shared, but the research leader – called principal investigator in some fields and contexts, and lead author, corresponding author, or lead inventor in others – is considered responsible for communicating expectations to their research colleagues and students, and ultimately bearing consequences of intentional or incidental errors in tool use.
G enerative AI Use across Research Stages
We considered four stages of research, each of which may receive different emphasis among Cornell’s impressive breadth of research and scholarship areas. Figure 1 illustrates these four stages where GenAI can be used to great advantage, with appropriate sense of duty by the researcher(s).
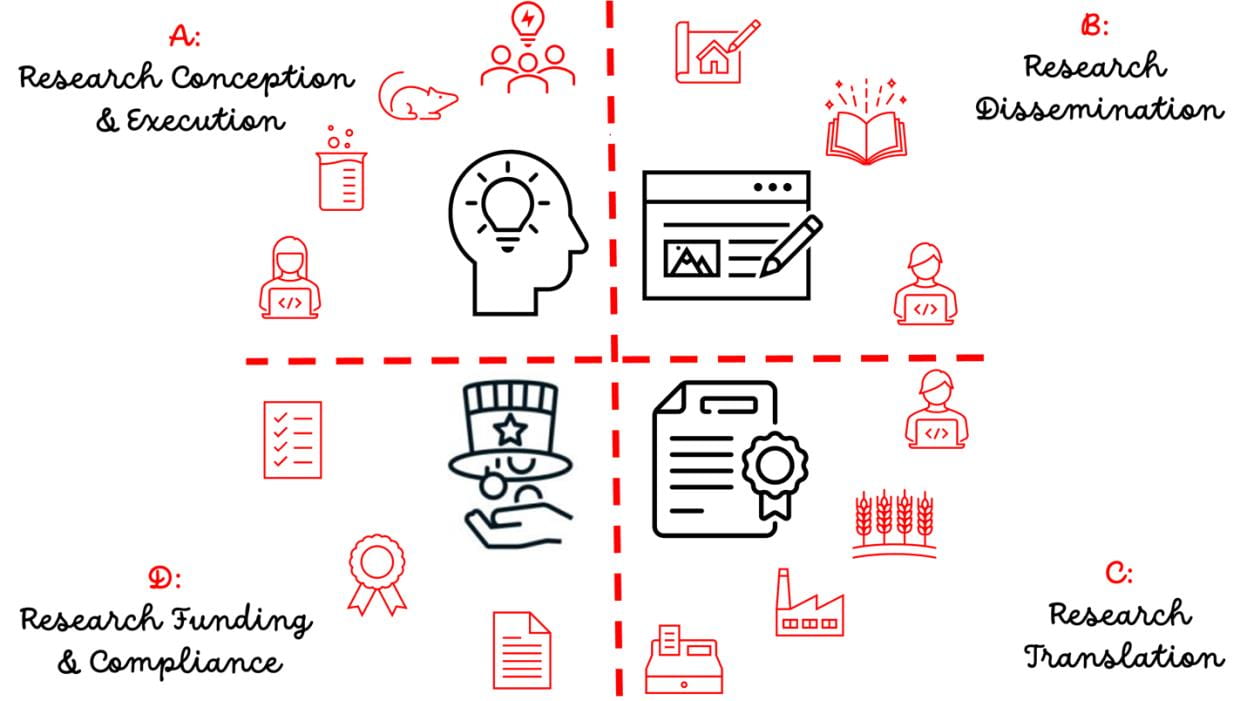
These four stages that can be considered in the life cycle of research include:
- Research Conception and Execution Stage: Includes ideation by the individual and research team, prior to any public dissemination of ideas or research results.
- Research Dissemination Stage: Includes public sharing of research ideas and results, including peer-reviewed journal publications, manuscripts and books, and other creative works.
- Research Translation Stage: Includes reducing research findings or results to practice, which may be in the form of patented inventions or copyrights, for products or processes or policies.
- Research Funding and Funding Agreement Compliance Stage: Includes proposals seeking funding of research plans, as well as compliance with expectations of sponsors or the US government policies relevant to Cornell as an institution of higher education and a research university.
A. Research Conception and Execution Stage
In this section we discuss uses of GenAI for the “internal” research process prior to the preparation of public documents. Research conception and execution includes literature review, research infrastructure, research ideation, and hypothesis generation.
G enAI for Literature Review
The volume of published research literature and data have been expanding exponentially, accelerating with technology advances such as movable type and publishing changes such as electronic journal proliferation and public databases. It is widely assumed that there are pockets of information in distinct fields that, if combined, could lead to breakthroughs. But despite the volume of published information, those serendipitous connections are infrequent because fields are mutually inaccessible due to technical language, and no one from either field knows to look for the other (e.g., epidemiologists and aerosol physicists). In fact, interdisciplinary research often espouses the mixing of existing information in new ways, implying that GenAI systems which can keep track of vastly more information than any person may find connections that might be missed entirely by humans. Acting as “state-of-the-art information retrieval” systems (Extance, 2018), they go beyond conventional databases, such as Google Scholar and PubMed, by being able to retrieve, synthesize, visualize, and summarize massive amounts of existing knowledge (e.g., Semantic Scholar, Scopus AI, Microsoft Academic, Iris.ai, Scite, Consensus). As such, they have the potential to help overcome the problem of “undiscovered public knowledge” (Swanson, 1986; Davies, 1989), which may exist within the published literature, and break through disciplinary silos, facilitating the discovery of relevant research across diverse academic disciplines.
In this context, suggested practices for using GenAI in the literature review phase of research conception are that:
- GenAI can be used to triage, organize, summarize, and quickly get directionally oriented, in the context of an exponentially growing base of reported claims and established knowledge.
- GenAI can be used to assist with drafting literature reviews, although researchers should fact-check and be aware of incomplete, biased, or even false GenAI outputs. In some cases, it can help to provide GenAI with explicit prompt text to try to guard against the use of fake references (e.g., Dowling & Lucey, 2023), although it still does not guarantee accurate results.
- Subject to authorship, citation, and fact-checking considerations, it can be helpful to use GenAI to ideate and iterate on the quality of a literature review. Examples include (a) refining the review to include both prior research and its connection to the new research idea, (b) rewriting the style of the literature review, and (c) refining the literature review to emphasize the contribution of the new research, such as is relevant to other gaps in literature, uncertainties, or even market sentiments.
Duty of verification . The reliability and quality of AI-powered literature review tools are limited by the databases they search, which can affect the comprehensiveness and accuracy of the results. Therefore, it is advisable to use these tools in conjunction with other methods. Another major concern when using these tools is plagiarism, as they can produce verbatim copies of existing work without proper attribution or introduce ideas and results from actual published work but provide incorrect or missing citations. To minimize the risk of unintentional plagiarism, it is best to start with original text and then use GenAI assistance to refine it, in line with the distinction between AI-assisted and AI-written text (van Dis, Bollen, Zuidema et al., 2023). This will help ensure that AI-generated text is original and reflects the authors’ own work, as also emphasized in journals and professional societies that permit the use of GenAI tools (but note that some journals prohibit the use of GenAI for any part of the research process entirely; see Appendix 1 for a summary of existing community publication policies, noting that such policies are subject to change by those communities and publishers). Finally, depending on the extent of GenAI assistance with information search and literature review production (specifically, when it is used beyond grammatical polishing of authorwritten text), researchers may have a duty of disclosure for this research stage.
G enAI for Research Infrastructure
One of the more benign possible uses of AI is in improving workflows and research processes. Collecting and processing data often involve custom software, using complicated APIs (application programming interface, or software with a specific function) that may be poorly documented. Code generation tools such as Copilot have become powerful and successful, leading to significant improvements in users’ ability to create software to collate and analyze data. Other ways might involve using GenAI to help construct or critique survey questions or interview templates. In each case, the AI is not involved in producing or recording data, but in building the infrastructure that is itself used to produce data. A second category of infrastructure might include code or language generation for presentation of research results. APIs for generating figures, such as matplotlib or ggplot2, are notoriously complicated, with innumerable options for modifying the appearance and layout of graphics. Code generation may help in producing programs to generate graphics from data sets, without being directly involved in the construction of data sets themselves. Similarly, language models might assist in generating alt-text for image accessibility. Duty of verification. As with any other use of GenAI, infrastructure-building uses require careful checking to ensure that outputs are correct. There should be clear responsibility for who will do this verification. We see less need to disclose the use of GenAI in these “back office” contexts relative to other uses, though still with care for potential implications at later research stages.
G enAI for Data Collection and Generation
A subtle but important distinction is when we move from using GenAI to help develop tools that we use in research to using GenAI as a tool for research, specifically for data collection and generation. In principle, the potential for data collection is enormous. GenAI can be used to help construct data sets from unstructured data, such as descriptions of patents, job vacancies, SEC (US Security and Exchange Commission) filings, banker speeches, etc. GenAI tools can also be used to synthesize information coming from text, or images. They can be employed to self-assess (predict accuracy) and augment which coding tasks are conducted by human iteration. Advantages for data collection and generation using GenAI as a tool for research include:
- Collecting and organizing data. Consider the Cornell Lab of Ornithology’s example of eBird as one data-rich source: Through this global application platform, birdwatchers have submitted a large amount of bird observations that have already informed development of species distribution models (Sullivan et al., 2014).
- Generating data out of unstructured information.
- Summarizing data coming from various sources. Data related to human clinical trials or patient outcomes hold different and important data privacy concerns, but the collection and organization/cleaning of such data is a key step in inference for patient-centered health outcomes (Waitman et al., 2023).
- Scaling up data collection with GenAI by conducting faster and less resource-intensive experiments.
The challenges of using GenAI tools for data collection and generation primarily relate to the duties of verification and disclosure :
- Issues with performance and accuracy: Large language models like ChatGPT are currently not fundamentally trained to speak accurately or stay faithful to some ground truth.
- The reliance on large amounts of data may be challenging, and the needed data may not always be available.
- Bias (King and Zenil, 2023): AI is traditionally trained on data that has been processed by humans. Example: In using ML to categorize different types of astronomical images, humans might need to feed the system with a series of images they have already categorized and labeled. This would allow the system to learn the differences between the images. However, those doing the labeling might have different levels of competence, make mistakes and so on. GenAI could be used to detect and to some extent redress such biases.
- Attribution: Data sources may not always be tracked. There is a need for ensuring correct attribution of data sources.
Given these challenges, the use of GenAI tools for data generation and collection must be carefully documented and disclosed to facilitate research assessment, transparency, and reproducibility.
G enAI for Ideation & Hypothesis Generation
While the use of GenAI for idea generation is under early consideration by most academic researchers, it is important to weigh its strengths and weaknesses in the early phases of research. If we think of the idea generation process as a creative process (as opposed to fact-checking or verification), then complementing ideation with GenAI can potentially offset human weaknesses, such as comparatively poorer memory recall versus recognition processes and narrower breadth of knowledge bases. In this sense, GenAI can complement individual researchers during the idea generation process and democratize access to research assistants. On the other hand, scientific knowledge relies on the ability to reason rationally, do abstract modeling and make logical inferences. However, these abilities are handled poorly by statistical machine learning (ML). Humans do not need a large amount of data or observations to generate a hypothesis, while statistical ML relies on vast amounts of data. As a consequence, computers are still unable to formulate impactful research questions, design proper experiments, and understand and describe their limitations.
Furthermore, assessing the scientific value of a hypothesis requires in-depth, domain-specific, and subject-matter expertise. An example is the potential of “Language Based Discovery” (LBD) as the possibility to create entirely new, plausible and scientifically non-trivial hypotheses by combining findings or assertions across multiple documents. If one article asserts that “A affects B” and another that “B affects C,” then “A affects C” is a natural hypothesis. The challenge is for LBD to identify which assertions of the type “A affects C” are novel, scientifically plausible, non-trivial and sufficiently interesting that a scientist would find them worthy of study (Smalheiser et al. 2023). Whereas GenAI does well in identifying and retrieving potential data constructs, researcher domain expertise remains critical for determining the quality of output (Dowling and Lucey, 2023).
While considering the possibilities of GenAI-human collaboration for research ideation, it is essential to emphasize the duty of discretion to prevent the leakage of proprietary, sensitive, and confidential information into public information space. Furthermore, since using GenAI tools is an evolving space, academics should learn more about GenAI technologies and stay abreast of potentially useful ways for hypothesis generation. For example, as food for thought, one of the GenAI prompts used by Dowling and Lucey (2023) for idea generation: “You [the GenAI tool] created this research idea, and I’d like you to improve it. Could you see if there is an additional article that can be added, to improve the research idea. Can you also talk about the novel contribution of the idea. Please keep it to about 100 words.” Moreover, as we humans gain experience and familiarity with new tools, we do well to be observant to the expectation that they can also change how we conduct research and interact with researchers at this ideation and hypothesis generation stage – in ways that are not always easy to identify a priori.
Finally, we note that research execution includes expectations of responsible conduct of research, which for some studies and disciplines includes prior approval of data use and management, animal welfare and procedures, and human subjects. Use of GenAI in research will likely augment considerations of these approvals per expectations of sponsors or federal agencies through research integrity review processes of the university. Those considerations related to research compliance are expanded in Section D. Next, we consider the stage where research of any type is disseminated through public disclosure including peer-reviewed publications.
GenAI offers new affordances that support both positive and negative outcomes for research dissemination (Nordling, 2003). On the positive side there is the potential to level the playing field for non-native speakers of English; to provide writing assistance resulting in improved clarity; and for new tools that aid in more equitable discovery of related work (improving on common practices of searching for well-known authors, for example). On the negative side there are serious and reasonable concerns such as erroneous information being disseminated because of inadequate verification; easier plagiarism (either intentional or accidental); lack of appropriate attribution because current LLM-based tools are unable to indicate the source of information; bias and ideological influence; and inappropriate use of GenAI as a lazy peer review tool. Additionally, we must be aware that careless use of GenAI may entrench biases in scholarly communication and dissemination, such as reinforcing the positions of prominent scholars and preferring sources in English as the dominant language of the initial trained models available to the general public. However, future GenAI tools may also provide new interventions to oppose existing biases that are entrenched in current practice. As such, the following is less focused on specific GenAI tools available today, but more on general recommendations for the responsible use of GenAI tools in research dissemination that upholds research integrity as a principal value at Cornell.
In this section and research stage, we do not discuss questions of copyright, confidentiality or intellectual property (see Section C), but instead focus on the conceptual impact that GenAI can have on producing research output. Following from the definition of GenAI from above, a key distinction is whether the tool produces output for dissemination that contains concepts and interpretations that the author did not supply . From this perspective, GenAI tools that fill in concepts or interpretations for the authors can fundamentally change the research methodology, they can provide authors with “shortcuts” that lead to a breakdown of rigor, and they can introduce bias. This makes it imperative that users of GenAI tools are well aware of the limitations of these tools, and that it is clear who is responsible for the integrity of the research and the output that is produced.
Below we discuss these issues in more detail and provide a minimal set of norms that we recommend across all disciplines. However, we recognize that the methodology and standards are differentially impacted by GenAI across disciplines (e.g., humanities as well as engineering), and that community norms around the use of GenAI may be stricter than what is outlined below.
Authorship : We posit that GenAI tools do not deserve author credit and cannot take on author responsibility. This means that authors of research outputs, not any GenAI tools used in the process, carry the responsibility for checking the correctness of any statements. Authors must be aware that GenAI tools can and do produce erroneous results including “hallucinated” citations. The content will be viewed as statements made by the authors. Indeed, there are emerging concerns on impact to scientific publishing with which publishers and AI ethicists are now grappling (Conroy 2023), but the responsibility of authentic authorship is a component of research integrity that will continue to rest with the human authors.
Impact on Concepts and Interpretations : Researchers need to be aware that GenAI tools can have a substantial impact on the research output depending on how they are used to fill in concepts and add interpretations. If the impact is substantial, we recommend that the use of GenAI is disclosed and detailed so that readers are aware of its potential impact. What constitutes substantial impact depends on the type of publication (e.g., journal articles, books, talks, reports, reviews, research proposals) and community norms in the respective discipline. An example that is probably considered to have a substantial impact in any discipline is the use of GenAI to draft a related work section.
Impact on Methodology : Writing and other dissemination activities typically cannot be separated from conducting the research, and the act of writing is explicitly part of the research methodology in some disciplines. A key concern is that the use of GenAI as a “shortcut” can lead to a degradation of methodological rigor. If the use of GenAI tools can be viewed as part of the research methodology, then we recommend disclosure so that readers can assess the rigor of the methodology. Indeed, there may be collective impact on methodology at the scale of the research community’s practices. Whether GenAI becomes a tool that sharpens our minds or a blunt instrument that dulls them is a question that Cornell (and other communities of research scholars) must address actively over time. Historically, human imagination sees most tools as helpful implements to move on to harder problems and more creative discovery and analysis, if one masters the tool instead of the other way around. But we can also recognize from past experiences that zeal for rapid development of exciting new research-enabling capabilities – especially when these provide competitive advantage over peers that can relate to economic competition or even national security – can shift even the best intentioned individuals to start to behave collectively as a group that focuses sharply on the benefits without openly discussing the costs and trade-offs.
Potential for Bias : Just as authors need to be aware of human biases in their sources, authors using GenAI tools need to be aware that these tools have biases and may reflect specific ideologies. It is the authors’ responsibility to counteract or avoid these biases, and authors cannot offload responsibility for bias in their work on the AI system. For example, use of a GenAI tool to create a hospital scene might result in an image in which the nurses are female and the doctors are male. Changing the prompt could address this bias. Another issue is that GenAI tools may reflect a particular ideology, or they may perpetuate historical biases because GenAI tools are trained on historical data. This may be compounded by particular algorithms such as citation analysis which has an inherent time lag, and might further bias recommendations back in time or towards a dominant group or language.
Acceptable Use : There are many different forms and venues of research dissemination: journal articles, books, talks, reports, reviews, research proposals, etc. What is acceptable use of GenAI in one form of communication is not necessarily acceptable in other forms, and authors must adhere to community standards. To take an extreme example, having a GenAI tool draft a peer review from scratch runs counter to the idea of peer review and has an extremely high impact on the review, even if the author checks and edits the review. This is likely unacceptable in most communities. Even within communities, different publication venues (e.g., journal, conference) may have different policies, and authors must check and follow these if more stringent than what is outlined here.
AI Literacy to support Research Integrity : Rigorous and ambitious use of GenAI tools requires a good understanding of the strengths and weaknesses of these tools. Furthermore, as GenAI has become part of the integrity of research and its dissemination, then research leaders such as principal investigators and faculty supervising student research should now make the appropriate use of GenAI part of their mentoring. In particular, part of their mentoring is to communicate the standards and the norms in their specific fields to the researchers and students they lead – just as they mentor on other topics of research conduct (e.g., plagiarism, co-authorship, privacy regulations).
Regulations : Any use of GenAI tools needs to be compliant with regulations (e.g., copyright law, privacy regulations such as HIPAA and FERPA, confidentiality agreements, and intellectual property). In particular, users must be aware that use of GenAI tools may disclose sensitive information to a third party, which may be in violation of regulations and confidentiality norms (Lauer et al. 2023; Conroy 2023). This extension to implications for subsequent research translation to policies, processes, and products of all types is discussed further in Section C.
C . Research Translation Stage
The use of GenAI in any stage of the research process may impact the translation, protection and licensing of intellectual property (IP), commercialization of technology, open-source release of software and other uses of the research output downstream. Interpretations of laws and new regulations regarding GenAI are major topics for governments in many countries including the US. There may be new government agencies and international organizations created for AI regulation and coordination in the near future. In fact, while the European Union recently announced new regulations on artificial intelligence, current understanding is that most of this EU policy effort to create these “first rules” preceded widespread use of GenAI (European Union Commission 2023).
We can draw no immediate conclusions on how the EU’s risk-based approach may impact GenAI development and uses specifically. The nature of the impact of GenAI is still evolving and may change in coming years, with legislation and guidelines expected to lag the use of GenAI and its shorter term implications, which may be inadequately addressed under current laws and regulations. The following are important areas for researchers to consider for translation when they use GenAI in their research process:
Inventorship and Patentability: Recent US case law has held that inventors of patents must be human beings under the US Patent Act. Documentation of human contribution and disclosure of the nature of GenAI utilization are essential for patent eligibility. Key information needs to be carefully documented, such as:
- Specific GenAI tools used and rationale for their use;
- Detailed input into and output of the GenAI tool;
- Whether the outputs lead to any aspects of the conception of the invention;
- Contributions of individual inventors in the inventive idea, and how they directed and refined the GenAI output; and
- For research done in teams, delineate the role of GenAI for each inventor.
Copyright and Authorship : Under current US copyright law, copyright can protect only material that is the product of human creativity and authors of copyrighted materials must be human beings. When incorporating GenAI-generated content, the authors should:
- Clearly document the boundary between human-created and GenAI-created content with clear annotations.
- According to guidance published by US Copyright Office, if copyright registration is sought, the nature and extent of the use of GenAI, if containing more than de minimis AI-generated material, must be disclosed with clarifications of what part of the work was created by the researchers and what part of the work was created by the GenAI.
- Specifically for computational algorithms and code, where research code can be further translated to wider use through copyright and various licensing types including open-source licensing, considerations attach at a time of active discussion. We note emerging considerations of copyright infringement, not only for creative works such as songs but also for computational code. For example, it is possible that code generated by a LLM reflects code reproduced verbatim from the LLM training set unbeknownst to the user. When such code is part of a research outcome that may be made available to licensees (even open-source licensees), it is possible but not yet well understood how use of such code, even when unintentionally plagiarized from other original sources, may violate copyright or invalidate licenses.
Commercialization and Fair Use : For research that leads to commercialization and publications with financial benefits, to mitigate risks of potential infringement claims, the inventors and authors should:
- Prioritize the use of GenAI tools that are trained using only public domain works, which is a small but growing area of development. For example, the recently announced AI Alliance coalition that includes Cornell as a founding member and anchored by two for-profit companies, IBM and Meta, advocates for development of open-source software tools including those enabling GenAI ( https://thealliance.ai/ ; Lin, B., 2023).
- Understand that the commercial intent can significantly impact fair use considerations. Consult with relevant university offices, such as the Center for Technology Licensing or General Counsel’s office, when there are questions.
- Stay informed of ongoing litigation that may influence the use of copyrighted materials in GenAI training data set. There are pending class action copyright suits by authors against entities owning GenAI tools for training without compensation to the authors.
Data Privacy and Protection : For data that researchers enter into GenAI themselves, it is important that researchers follow Cornell Policy 4.21 on Research Data Retention. Note that this is an existing policy and practice, simply extended to GenAI. Private, confidential, or proprietary data owned or controlled by Cornell may have certain contractual or legal restrictions or limitations, such as those to sponsors or collaborators, that would preclude their use in GenAI research projects, and it is a researcher’s responsibility to verify/determine whether any such data sets have restrictions on such use before inputting them into public-facing GenAI and ensuring compliance with any restrictions mandated by contract, law, or governing body (e.g., IRB, IAUCC). Any use of patient or human derived data should be disclosed to such governing body during the approval process and any such data set should only be used in research projects upon the explicit approval of the relevant governing body on campus.
Training : Specific to this stage of research translation, it is recommended that the university provide ongoing workshops on campus or through online platforms, and offer training materials through websites and other distribution channels, on topics related to the use of GenAI and its impact on patent rights, copyrighted materials, commercialization, open-source release and other uses to aid the researchers in understanding their rights, obligations, best practices and landscapes of relevant laws and regulations. Indeed, Cornell includes faculty and staff experts that can facilitate and co-develop such resources as part of their scholarly practice.
During the research funding and funding-agreement compliance stage, there are many potential applications of GenAI. For example, these tools can be leveraged to assist in the writing of technical, science-related information for a proposal to a sponsor or a donor, such as the technical scope and anticipated impact. On the non-technical side, they can also be used to draw appropriate data from multiple data sources to develop information for a biosketch, a report of Current and Pending Support, and other documentation relevant at this stage of the research process.
Work conducted during the Research Funding and Funding Agreement Compliance stage is poised to benefit from the use of GenAI tools, for example, due to efficiency improvements and reductions in the time taken to produce previously time-consuming work. However, the use of these tools also comes with risks. GenAI may produce outputs that include incorrect or incomplete information. These tools also may lack sufficient security and privacy protections, both in the tools themselves, and in any plug-ins and add-ons to the tools.
Note that we and many federal agency sponsors refer to the person of primary responsibility in research as the PI , or principal investigator, and pronounced pee-I, for shorthand. We acknowledge that this term is common for research in the sciences and engineering with cultures of team-based research and that other fields have a tradition of independent scholarship and authorship even when enrolled as graduate students.
Responsibility: As with the earlier stages of research, users of GenAI hold some burden of responsibility (or duty ) in the Research Funding and Funding Agreement Compliance stage. In this stage, however, it is common to attach the primary responsibility of compliance to the leader of the research effort. For example, the accuracy of any information contained in a proposal for funding is ultimately the responsibility of the PI, and so if the PI uses GenAI in the development of materials for that proposal, they must review the information in those materials and correct any omissions, errors, or otherwise inaccurate information. The PI must also understand that although resources (for example, research administration staff professionals in Cornell departments, colleges/schools, or research & innovation office units) are available to help them during this stage of the research process, these resources cannot certify to the accuracy of much of the information provided to them, and therefore cannot be expected to identify mistakes in that information, such as those generated by GenAI. The PI must also understand that they are responsible for the activities of students and research personnel working on funded projects under their supervision or mentorship, and for ensuring the appropriate use of GenAI tools by those individuals. See Appendix 0 , Prompts on Gen AI in Research for suggested discussion starters.
During this stage, individuals may desire to input information into GenAI to assist in the production of their research proposals, reports to sponsors, or even the public dissemination and translation stage documents that may have specific restrictions placed by the sponsors. Because some of this information will be highly sensitive, such as unpublished technical information or private funding data, users of GenAI tools must understand their responsibility for protecting the privacy and security of any information they input into these tools, and must seek approval to do so from the owner (such as the PI) of any such information. In fact, even in the peer review of sponsored research proposals (e.g., faculty serving on review panels for NSF or study sections for NIH), the use of GenAI may not be allowable by the sponsor (NSF Notice to Research Community, Dec 2023).
In this stage of the research process, it is also important for those who are responsible for making decisions regarding the use of sponsored funds to consider whether, and under what circumstances, it is appropriate to charge the use of GenAI tools to a research account, and to ensure their awareness with each sponsor’s requirements. Although some sponsors are clear on whether and how funds may be applied to the use of GenAI, others are not.
Guidance and Training: The nature of these tools, their potential applications, and the associated benefits and pitfalls will continue to develop and change over time, and thus, so will the appropriate guidance on how to use them. Although information and guidance should be shared with users about the risks of the use of GenAI and about which tools to avoid, it is also important to share information and training on how users can make use of these tools, how to navigate security and privacy concerns with confidence, and to provide access to tools that have been vetted and found to be aligned with the university’s expectations for security and privacy.
In this context, we suggest the following considerations as resources developed by and for the research community, including staff professionals experienced in research integrity, information systems, and user experience.
- Broad communications and outreach about GenAI in responsible conduct of research. These communications should include guidance and resources on the use of GenAI, as well as information about training, what tools to use or avoid, and references to offices and units that are available to provide support. When appropriate, this outreach should be shared by central offices and posted to central web pages – such as the recently developed Artificial Intelligence website hosted by Cornell Information Technology (CIT) that links to Cornell’s GenAI in Education report and other resources – rather than from individual units or departments, to create consistent understanding and information access across campus. Providing this type of outreach from central offices can help ensure that the university as a whole is looking to the same resources; that inquiries and concerns come to the appropriate offices; that approaches, advice, and guidance given are consistent across units; and that gaps in accessibility of information and learning are kept to a minimum.As with training on the use of, for example, animals, human participants, or biological agents in research, centralized training should be provided on the use of GenAI. This training should not only focus on risks and concerns, but on how to get the most out of these types of tools, and how to use them better. “Hackathons as Training” should make it enjoyable for researchers to gain new skills, while also contributing to the safe and responsible use of GenAI.
- Guidance on navigating mistakes made and security breaches should be communicated university-wide. It is important to acknowledge that with these new tools comes some anxiety about making mistakes in using them appropriately or even safely. To an extent, inadvertent mistakes present opportunities for education and training. However, it is also important that any mistakes that lead to security, privacy, or other concerns are handled correctly and in a timely manner. Information should be shared university-wide about Cornell’s expectations and processes with regard to what to do in the case of a potential security or other risk related to the use of GenAI, and which responsible offices should be notified.
Additional tools and resources should be developed to provide guidance. It would be beneficial to researchers and administrative staff alike to develop a GenAI-enabled tool (e.g., a form of a chatbot) that would respond to common inquiries about the use of GenAI in research. For example, “Can I use GenAI to edit my scope of work?” This tool could be populated with responses to common questions, so that consistent answers could be communicated broadly – even while appreciating that perspectives and cultural norms and even sponsor requirements and expectations may be changing fluidly in the coming years. Because such a tool would be automated and would provide immediate access to answers to these types of common questions, it would both reduce wait times and delays associated with other means of gathering this information, and reduce administrative workload in responding to these types of common requests. Similarly, resources that facilitate awareness of resource use (e.g., estimated carbon dioxide emissions associated with tool use; see Section A) can be made available at the Artificial Intelligence website and/or developed by Cornellians whose research and translation focus includes sustainability practices (e.g., Cornell Atkinson Center for Sustainability).
P erspectives and Cultural Norms
Having framed the use of GenAI in research across the stages of research above, we here summarize the perspectives that can inform our cultural norms. The widespread availability of GenAI tools offers new opportunities of creativity and efficiency, and as with any new tool depends on humans for responsible and ethical deployment in research and society. Thus, it is important that Cornell anticipates that researchers can and should use such tools appropriately, facilitates researcher access to appropriate GenAI tools and to resources to improve researchers’ “AI literacy.” It is also important that we develop shared understanding of the limits of appropriate use of specific publicly available and commercial GenAI tools, as well as the tradeoffs or risks involved in their use.
While these perspectives and cultural norms will vary reasonably among different research communities, and likely vary over time in the coming years, we offer the following summary considerations. These are considerations of both opportunity (ambitious use that may create new knowledge, insights, and impact for the world) and accountability or responsibility (duty grounded in research integrity of individuals, research teams, and institutions including Cornell). We consider these to be peer-to-peer guidelines, not a suggestion of any formalized university policy. However, we remind our fellow Cornellians that two existing policies naturally extend to use of GenAI tools in research:
- As noted in the University Privacy Statement , Cornell strives to honor the Privacy Principles : Notice, Choice, Accountability for Onward Transfer, Security, Data Integrity and Purpose Limitation, Access, and Recourse. This is noted on Cornell’s Artificial Intelligence website, along with preliminary guidelines of accountability that are discussed in this report in the context of researcher duties and research integrity.
- Cornell Policy 4.21 on Research Data Retention . Private, confidential, or proprietary data owned or controlled by Cornell may have certain contractual or legal restrictions or limitations, such as those to sponsors or collaborators, that would preclude their use in GenAI research projects. It is the researcher’s responsibility to verify/determine whether any such data sets have restrictions on such use before inputting them into public-facing GenAI and ensuring governing body compliance (e.g., IRB, IAUCC). Relatedly, any use of patient or human derived data should be disclosed to such governing body during the approval process, and any such data set should only be used in research projects upon the explicit approval of the relevant governing body on campus.
We as colleagues encourage faculty, research and administrative staff, and students to help develop the norms, technology, and public reflection on GenAI use in research, to both shape and stay current on these uses and scholarly practices. These five areas of consideration for the Cornell research community are summarized below, as part of responsible experimentation.
HELP DEVELOP THE NORMS, TECHNOLOGY, and PUBLIC LITERACY around GenAI.
- Actively develop the norms and best practices around the use of GenAI in their disciplines.
- Develop GenAI technology that is particularly suited for research (e.g., improved attribution). GenAI development for academic use should not be left solely to for-profit companies.
- Engage in GenAI public literacy efforts to foster responsible and ethical use of GenAI tools. Using at least one of these tools is enormously helpful to being part of that conversation and process, and many are freely and publicly available with associated caveats on risks of use. Table 1 provides examples of currently available GenAI tools that can be accessed (denoted as “free” to indicate no financial charge to the user). We emphasize user awareness and appropriate caution: only publicly available data should be included, and the user should assume that any entry of information by the user can be absorbed into that tool’s training set and potentially exposed to others.
STAY UP-TO-DATE with GenAI Uses and Practices
- Each research subcommunity (whether a faculty member’s research group, a department, interdisciplinary research center or institute, or college/school as those researchers see fit) gather relevant information on relevant policies by professional associations, journals and funding institutions to stay up-to-date with evolving policies, practices, and requirements in your field . Appendix 0 may serve as a discussion starter.
- Train in how to use GenAI tools in a safe, effective, and productive manner in research and innovation. Develop expertise in the potential limitations and risks of GenAI tools.
Further, when acting as well-informed academic researchers with access to this research tool among others, consider the individual and shared duties of verification, disclosure, and discretion across the stages of research ideation and execution, public disclosure, translation, and funding expectations:
Duty OF VERIFICATION
- DO verify the accuracy and validity of GenAI outputs. The responsibility for research accuracy remains with researchers.
- DO check for unintentional plagiarism. GenAI can produce verbatim copies of existing work, or more subtly, introduce ideas and results from other sources but provide incorrect or missing citations.
Duty OF DISCLOSURE
- DO keep documentation and provide disclosure of GenAI use in all aspects of a research process, in accordance with the principles of research reproducibility, research transparency, authorship and inventorship.
Duty of DISCRETION
X DO NOT assume that GenAI is private. GenAI systems run on training examples, and user input and behavior are a prime source. Even if organizations that provide GenAI tools do not currently claim to use data in this way, there is no guarantee that they may not in the future.
X DO NOT share confidential, sensitive, proprietary, and export-controlled information with publicly available GenAI tools.
X DO NOT assume that GenAI output is already considered part of the public domain (e.g., not legally encumbered by copyright). GenAI tools can “memorize” their training data and repeat it with a level of verbatim accuracy that violates copyright. Even material that is not copyrighted may produce liability for corporate partners in sponsored research, if it is derived from data generated by a competitor.
C onsiderations for Cornell Leadership
We also provide considerations for Cornell leadership, particularly for aspects of GenAI preparedness and facilitated use in research and innovation that can be implemented collectively across Cornell’s colleges, schools, and campuses.
- Develop a knowledge base module, perhaps as part of responsible research conduct training resources, for rigorous, ethical and responsible use of GenAI in research and related activities. Users of GenAI tools need to understand their strengths and weaknesses, as well as regulations around privacy, special data considerations such as personally identifiable, human subject, or proprietary commercial data, and confidentiality and commercialization.
- Consider procurement of Cornell-licensed GenAI tools with data and privacy protection as facilities for research, as well as for administrative and teaching uses. Text generation and chat, program code generation, streamlined processes, and image generation would likely all be of value.
- Consider development or co-development of GenAI tools that are particularly suited for academic research use cases, including use cases in research administration services.
- Identify relevant central offices responsible for providing university-wide communications, guidance, outreach, and training to all GenAI users on various aspects of uses. To the extent that it is possible and relevant, information on the use of GenAI should be shared from central locations to encourage consistent access and understanding across the university, and to avoid siloed, inconsistent, or incorrect information.
- In support of Cornell’s public engagement mission, recognize Cornell efforts that improve GenAI public literacy beyond the university-affiliated community.
- Consider periodic updates to Cornell guidance, through a task force or other appropriate mechanisms, given the rapidly changing landscape of generative AI tools, uses, and considerations in academic research and translation of research outcomes.
A ppendix 0. Prompts on GenAI in Research (Discussion Starters or Frequently Asked Questions)
We further consider best practices and use cases in response to questions (prompts) for each of the research stages. During the task force, we simply used these prompts to stimulate early conversations and perspectives among Cornell colleagues from different fields of research and at different stages of research. The responses to such questions provided below are not prescriptive or complete, but share our initial, collective responses to such prompts as a diverse group of faculty and staff began shared discussion of this topic.
These same questions could be used within Cornell research group discussions or at department faculty meetings. For researchers to generate familiarity or insights into how these tools work to generate text or images or audio, these same prompts could be entered into multiple GenAI-enabled programs, or entered multiple times in the same GenAI-enabled program, or variations on these prompts.
A. Re search Ideation and Execution Stage
When using a tool such as ChatGPT to generate research ideas for a research project sponsored by NSF, how do the researcher and principal investigator decide on which information and ideas to enter and “share” with ChatGPT?
Any information entered into public versions of ChatGPT involves sending that data to a third-party that is under limited confidentiality and privacy restrictions (if any) with end users and not party to agreements with the NSF via data entry by other users, and the information that is entered can eventually become public. As such, where the use of ChatGPT for research idea generation does not currently violate any known general NSF policies, users should also be sure not to violate any other agreements that may exist relative to their funding sponsorship agreements, such as confidentiality, intellectual property, and entity identification clauses (e.g., mentioning NSF in any input data may be discouraged to the extent that it is in conflict with an agreement).
When using a tool such as ChatGPT to brainstorm solutions for research sponsored by a company (e.g., Samsung, Boeing, Johnson and Johnson, Google), how do the researcher and principal investigator decide what information about the project can be entered and shared?
Again, any information entered into public versions of ChatGPT involves sending that data to a third-party that is under limited confidentiality and privacy restrictions (if any) with end users and not party to agreements with corporate sponsors via data entry by other users. Furthermore, any information that is entered can eventually become public. As such, where the use of ChatGPT for brainstorming solutions does not currently violate any known general policies, users should also be sure not to violate any other agreements that may exist relative to their funding sponsorship agreements, especially including but not limited to, confidentiality and intellectual property clauses.
When using generative AI tools to summarize the literature for the introduction or discussion of a peer-reviewed article, how should the researcher and corresponding author attribute or disclose this section of a manuscript or thesis?
In general, authors and/or principal investigators have ultimate responsibility for works (including their accuracy), and furthermore, summaries should not violate plagiarism rules and regulations. Citation style guides and support websites (e.g., for APA, Chicago, Harvard) are being updated to reflect proper citations for verbatim output from generative AI and other uses. As a general practice, authors should be transparent and fully disclose uses of generative AI technologies, consistent with publication outlet, department, or area policies.
What are the conditions, if any, that a researcher should not use GenAI to generate research ideas? Examples may vary among research fields, sources of information included in a prompt including FERPA or HIPAA data, and collaborating or sponsoring organizations.
There are no general rules that prohibit the user of generative AI to generate research ideas. However, because inputs into public generative AI platforms are not confidential and data can also become public, sensitive information and individual data should never be entered for any phase of a research project, pursuant to personal data identification, re-identification, and/or chain of custody FERPA and HIPAA requirements.
In the process of research publication development, can tools such as Bard or ChatGPT be used: To summarize responses to online surveys of income level of state residents? To summarize preclinical research animal model histology? To summarize patients’ blood oxygen levels in a registered NIH study? How can the differences among these use cases be distinguished in the responsible conduct of research?
The summary of online survey data and/or other data sets can be assisted by Bard or ChatGPT to the extent that the researcher 1) does not enter confidential or data protected by other laws (e.g., HIPAA), 2) does not violate a broader agreement (e.g., between researcher, institution, host, and/or funder), and 3) has responsibility for the accuracy of the summary.
When a figure for a publication or presentation or patent disclosure is generated by AI tools (e.g., Midjourney; DeepAI), how should the principal investigator (who is typically the corresponding author or communicating inventor) verify the accuracy and intellectual ownership over the data or content of that image?
We see a distinction between cases where the author supplies all semantics and uses a tool for layout and rendering, and when a tool is used to introduce semantics such as organization of ideas or the generation of structure. Where the author supplies all semantics the use is akin to PowerPoint style suggestions or an automated layout tool, and acknowledgement is generally unnecessary unless publication policies require it. However, when GenAI introduces new semantics then we advise acknowledging its use, and checking the output carefully for accuracy. Norms around intellectual ownership are in flux at this time, and we caution authors that this poses a substantial risk.
Can images used in publications and theses be created wholly by generative AI? How does this expectation change if the GenAI-drafted images are edited by the authors? How does that vary among research disciplines? 24
Uses include generation of a cover image for a book or presentation, or images similar to clipart. We advise that authors should generally acknowledge the use of GenAI in this case, and they should carefully check images for bias and accuracy. Authors should be aware that, in the US and many other jurisdictions, it is not possible to claim copyright in non-human created works even if any human additions/edits may be copyrightable. There are unresolved legal questions regarding possible copyright infringement both as a result of the training of GenAI programs on works under copyright, and as a result of output that might closely resemble works under copyright (see, for example, the US Congressional Research Service, “Generative Artificial Intelligence and Copyright Law”, Updated September 29, 2023, https://crsreports.congress.gov/product/pdf/LSB/LSB10922 ). There is also significant variability in the acceptability of GenAI-generated images based on the publication venue.
When and how should the corresponding author inform a journal of manuscript elements created by GenAI, if not explicitly required to disclose by the publisher and when not obviously using or studying GenAI? Examples may be a proposed cover image, a graphical depiction of a new method, a graph containing research-generated data, a set of test data, generation of derivative data, etc.
GenAI technology is increasingly being built into services that provide grammar checks, polish language, and proofreading. General purpose tools such as ChapGPT are also effective for these tasks. It is not usual to acknowledge the use of checking and suggestion tools. GenAI tools, like human proofreaders who may not understand the subject matter in detail, can suggest changes that change the intended meaning so authors must still verify suggestions with care. Commercial checking and suggestion tools are being extended with GenAI features to draft entire sections or articles, or summarize texts, so authors should consider when their use crosses the line into generative use as defined above.
When and how should research group leaders (e.g., faculty) communicate these expectations of appropriate/ethical/responsible use of GenAI in research to researchers who are undergraduate students? Graduate students? Postdoctoral researchers? Other research staff?
Educating about the responsible use of GenAI should become part of the regular training on research methodology and norms of the respective discipline. This includes training that research leaders provide, but it also is a responsibility of Cornell to educate faculty and students on the affordances and pitfalls of GenAI tools.
If an invention is reduced to practice in part by use of generative AI, how should the inventors document and inform others when considering a disclosure of invention or copyright?
Any use of GenAI in the conception and reduction to practice of an invention or generation of copyrighted materials should be carefully documented and disclosed to the Center For Technology Licensing by the inventors/authors.
For example, as to the conception and reduction to practice:
- What was the GenAI tool used?
- What were the inputs to the GenAI? Do you have rights to the data used for input?
- What were the outputs of the GenAI?
- How did the outputs of the GenAI, if at all, lead to the conception of any aspect of the invention?
- Were there any difficulties encountered in using the GenAI to yield the outputs desired and, if so, which researchers updated use of the GenAI, model and/or data to yield the desired outputs?
- Which researchers substantively contributed to/controlled the development of the input and output corresponding to the invention?
If the research outcome is open-source licensable and/or posted on an open-source repository (e.g., code or algorithm or app), should and how should the researcher disclose use of GenAI in creation of the “open source” item?
Disclosure of the use of a specific GenAI tool and possibly even the origins of, and the rights to use, the input data used will likely be viewed as the standard for ethical behavior over time. Currently there are no hard and fast rules.
If the research outcome is a creative work (e.g., book, play, sculpture, musical score, multimedia exhibit) that used GenAI in the creation of that work, how should the researcher disclose that contribution in discussions of copyright?
According to Guidance published by USCO, if copyright registration is sought, the nature and extent of the use of GenAI, if containing more than de minimis AI-generated material, must be disclosed, and what part of the work was created by the researchers and what part of the work was created by the GenAI.
How should the researcher inform themselves of the uncompensated contributions of others to the GenAI output used in their own creative and/or copyrighted work or invention? How does this responsibility depend on whether the researcher derives personal financial benefit (e.g., royalties on published book) from the research outcome?
There are pending class action copyright suits by authors against entities owning GenAI tools. In those suits, GenAI tools are alleged to utilize existing copyrighted works for training without compensation to the authors. Commercial purpose is an important factor in the determination of fair use. For research that leads to commercialization and publications with financial benefits, it will be safer to use GenAI tools that are trained using only public domain works. For data that the researchers put into GenAI themselves, it’s important that they make sure they have the rights to do so regardless of whether they expect financial benefits from the output of GenAI.
If grant proposal information related to science (technical scope) and non-science (biosketch, current & pending funding) components are generated by generative AI, who is responsible for editing them before submission to a potential sponsor? Who is responsible if there are omissions or errors in those work products?
The PI is responsible for the accuracy of information related to the science, as well as for omissions and errors in that information. On the non-science side, the PI is, again, primarily responsible for the information contained in their proposal. There are resources available to help them (such as research administration staff), but those resources cannot certify the accuracy of the information provided to them, or identify mistakes in information provided to them that occurred as a result of the use of GenAI.
It is also important for the PI and their less experienced collaborators (mentees, supervised students) to discuss concerns about inputting information into GenAI tools. This information can be highly sensitive (unpublished technical information, for example) or personal to an individual (Current & Pending funding that must be disclosed to employers and sponsors but not to peers or the general public). To an extent, whether information is considered sensitive may depend on the context of the use of GenAI or of the research field itself. Consensus of this task force was that the PI is responsible for the security of his or her research information, but that anyone who intended to input information into GenAI would need to seek approval to do so from the owner of that information (such as the PI).
Should the costs of Generative AI be charged to a research account, assuming this is not disallowed by the corresponding funding agreement (i.e., not disallowed by a research sponsor)?
The appropriate source of funds for GenAI in research may depend on how the GenAI is being used. If such use is categorized in such a way that other things falling under the same type of use could be charged to a research account (e.g., software services), then it is plausible that the use of GenAI may be acceptable. In some cases, sponsors note definitively whether such charges to sponsored project accounts are allowed, but this is not always the case.
If a principal investigator becomes aware that her graduate student queried a generative AI tool (e.g., ChatGPT) with proprietary data obtained appropriately from a company when summarizing research team meeting notes, what should her next steps be? Who is responsible for notifying the company? Who is responsible for remedying the action if the company has grounds to sue for breach of the data use agreement?
The PI is responsible for what their students do in the course of their Cornell work, and is therefore responsible for ensuring that these individuals use GenAI resources appropriately. That said, mistakes are bound to happen, and they present great opportunities for education and training of both the faculty and of the students.
Further, in these situations when proprietary information is input into GenAI inappropriately, it is reasonable that the PI may feel compelled to directly report this issue to their technical contact at the company, but doing so may not align with Cornell’s processes for resolution. Therefore, we should educate faculty about the appropriate way to resolve something like this, which Cornell resources are available to them, and what offices – such as OSP or Counsel’s Office – are available to help.
What tools or approaches might Cornell researchers find useful for shared awareness of responsible GenAI use?
The use of GenAI comes with significant privacy and security concerns, and it may be important for the university to gain an understanding of the privacy policies of GenAI companies in order to determine whether they are safe to use. Also of concern are plug‑ins to GenAI programs, which may come with their own privacy and security issues.
Although Cornell should provide guidance on risks and tools to avoid, it would also be very useful to provide researchers with information about what tools and resources they can or should use, as well as access to those tools, and confirmation that they’ve been vetted and found to be secure.
The university could also provide information about the use of GenAI through other means:
- Creation of a tool – “Asking for a Friend” – which could be used to answer questions researchers may have (ex.: “Can I use GenAI to edit my scope of work?”).
- Training should not only focus on risks and concerns, we should also provide training on how to get the most out of these types of tools, and how to use them better. “Hackathons as Training” – could make it fun for researchers to gain new skills, while also contributing to the safe and responsible use of GenAI.
- The IT@Cornell web page is a centralized location that can be used to post preliminary guidelines, general information about GenAI, and what researchers need to know about it.
In order to educate researchers on the use of GenAI, communication and outreach are key. We should educate researchers about the central offices that issue training, guidance, etc. that can help them, rather than leaving them to rely on potentially siloed offices in the units that may not provide consistent advice. If the university as a whole is looking to the same resources, and inquiries consistently come to the same/appropriate offices, approaches/advice/guidance given is more likely to be consistent university-wide.
Finally, much like training on the use of other things in research (animals, human participants, biological agents, etc.), education and training should be provided on how to use GenAI safely.
A ppendix 1. Existing Community Publication Policies
We surveyed current policies regarding the use of GenAI in research from funders, journals, professional societies, and peers. We found that most of these examples were stated by journals, professional societies, and research funders, and centered around the research dissemination phase. These include the authorship and review of publications. As of fall 2023, we found relatively little policy about the “private” phases of research, such as ideation or data analysis in what Fig. 1 of our report describes as research stage A, the ideation and execution phase. In these policies, institutions tend to be cautious rather than eager to embrace the possibilities of AI.
Current policies on AI use are often cited in the context of publication, through journals, funding agencies, or professional societies that run peer-reviewed conferences. Many express an openness to the use of GenAI as a tool for writing and editing, especially in so far as it “levels the playing field” for researchers who are not native English speakers. But many also express serious concerns about generation of text beyond grammatical polishing of author-written text. Potential harms usually fall into two categories. First, AI can produce plausible-sounding information that is not, in fact, correct. The published record could become increasingly polluted by unfounded information that is extremely difficult to detect. Second, AI can produce verbatim copies of existing work, possibly causing unintentional plagiarism. More subtly, AI could introduce ideas and findings from actual published work but omit or provide inaccurate citations.
There is significant concern about responsibility. We can find no example of a journal that allows non-human authors, and several that explicitly ban such a practice, as it cannot meet the authorship criteria of accountability for the work. But there are also more subtle questions of duty. Given that the use of generative AI provides substantial risk of inappropriate output (either false or inadequately cited) and may require substantial work to fact-check, who should carry out that work, and who should be punished if it is not done adequately? There is unlikely to be a single policy throughout academia as there are many distinct cultures of collaboration and responsibility. Some fields make strong distinctions between a principal investigator (PI)/advisor’s work and PhD student work. In this case a PI may have comparatively little responsibility to check an advisee’s use of GenAI. In other fields PIs and students work collaboratively on multi-author publications, where a senior or last author may be expected to have a more supervisory (and therefore responsible) role.
Some agencies also raise the issue of sensitive data. The best current language models are beyond the capabilities of typical laptop hardware, so they are often available as a cloud-based service. While there have been differing statements about what OpenAI or Google might do with information uploaded to the systems, the bottom line is that using such tools exposes potentially sensitive information to third parties. Therefore, institutions explicitly ban entering confidential, sensitive, proprietary, and export-controlled information into publicly available GenAI tools. Similarly, grant agencies and publications prohibit using AI tools in the peer review process to avoid the confidentiality breach.
When AI tools are permitted, there is a consensus about documenting their use in research conception and execution for reporting transparency and reproducibility/replicability purposes. Most publications require disclosure of GenAI use in the Materials and Methods section of a submitted manuscript as well as in a disclosure to editors, except when AI is used as an editorial assistant for author-written text.
Living guidelines for generative AI published in Nature, https://www.nature.com/articles/d41586-023-03266-1 :
“For Researchers, reviewers and editors of scientific journals
- Interpretation of data analysis;
- Writing of manuscripts;
- Evaluating manuscripts (journal editors);
- Peer review;
- Identifying research gaps;
- Formulating research aims;
- Developing hypotheses.
- Researchers should always acknowledge and specify for which tasks they have used generative AI in (scientific) research publications or presentations.
- Researchers should acknowledge which generative AI tools (including which versions) they used in their work.
- To adhere to open-science principles, researchers should preregister the use of generative AI in scientific research (such as which prompts they will use) and make the input and output of generative AI tools available with the publication.
- Researchers who have extensively used a generative AI tool in their work are recommended to replicate their findings with a different generative AI tool (if applicable).
- Scientific journals should acknowledge their use of generative AI for peer review or selection purposes.
- Scientific journals should ask reviewers to what extent they used generative AI for their review.”
A ppendix 2. References Consulted or Cited
Conroy, G. (2023). How ChatGPT and other AI tools could disrupt scientific publishing, Nature 622 , 234-236.
Current Cornell guidance on Gen AI for use in research, education, and administration (2023): https://it.cornell.edu/ai
Current Cornell guidance on Gen AI in teaching (Nov 2023): Available for download at https://teaching.cornell.edu/sites/default/files/2023-08/Cornell-GenerativeAIForEducation-Report_2.pdf and accessible online at https://teaching.cornell.edu/generative-artificial-intelligence/cu-committee-report-generative-artificial-intelligence-education .
Bockting, C. L., van Dis, E. A. M., van Rooij, R., Zuidema, W., & Bollen, J. (2023). Living guidelines for generative AI—why scientists must oversee its use. Nature , 622 (7984), 693-696.
Davies, R. (1989). The creation of new knowledge by information retrieval and classification. Journal of documentation , 45 (4), 273-301.
Dwivedi, Y. K., Kshetri, N., Hughes, L., Slade, E. L., Jeyaraj, A., Kar, A. K., … & Wright, R. (2023). “So what if ChatGPT wrote it?” Multidisciplinary perspectives on opportunities, challenges and implications of generative conversational AI for research, practice and policy. International Journal of Information Management , 71 , 102642.
Dowling, M., & Lucey, B. (2023). ChatGPT for (finance) research: The Bananarama conjecture. Finance Research Letters , 53 , 103662. 30
European Union Commission (2023). Press release, Dec 9, 2023: Commission welcomes political agreement on Artificial Intelligence Act. https://ec.europa.eu/commission/presscorner/detail/%20en/ip_23_6473
Figure 1: Subfigure component sources were obtained from three sources: Microsoft Powerpoint; Freepix free license (patent certificate icon): https://www.flaticon.com/free-icons/certificate” title=”certificate icons . Certificate icons created by Freepik – Flaticon; and iStock (istockphoto.com) standard license with subscription (top hat icon).
Free GenAI to write your research paper with/for you: https://jenni.ai
Free GenAI to assist with literature review: https://www.semanticscholar.org/ (among many available AI-powered tools for literature review, such as Iris.ai, Microsoft Academic, Scopus AI, Elicit, Scite, and Consensus).
Glossary of GenAI-related terms. Steven Rosenbush, Isabelle Bousquette and Belle Lin, “Learn these AI basics,” the Wall Street Journal https://www.wsj.com/story/learn-these-ai-basics-39247aaf
Institutional Review Board considerations: Some research centers consider these implications as part of the scholarly effort of the research practice, such as the Center for Precision Nutrition and Health: https://www.cpnh.cornell.edu/bond-kids-1 .
King, R., & Zenil, H. (2023). Artificial Intelligence in Science: Artificial intelligence in scientific discovery: Challenges and opportunities. OECD Publishing, Paris, https://doi.org/10.1787/a8d820bd-en .
Lauer, M., Constant, S., & Wernimont, A. (2023). Using AI in peer review is a breach of confidentiality. National Institutes of Health , 23 .
Lin, B. (2023). Meta and IBM Launch AI Alliance. Dec 5, 2023, Wall Street Journal .
Luccioni, A.S., Jernite, Y., & Strubell, E. (28 Nov 2023). Power Hungry Processing: Watts Driving the Cost of AI Deployment? https://arxiv.org/pdf/2311.16863.pdf
National Science Foundation (NSF) Notice to research community: Use of generative artificial intelligence technology in the NSF merit review process (Dec 14, 2023): https://new.nsf.gov/news/notice-to-the-research-community-on-ai?utm_medium=email&utm_source=govdelivery
Nordling, L. (2023). How ChatGPT is transforming the postdoc experience. Nature , 622 (7983), 655-657.
Smalheiser, N. R., Hahn-Powell, G., Hristovski, D., & Sebastian, Y. (2023). From knowledge discovery to knowledge creation: How can literature-based discovery accelerate progress in science?. In Artificial Intelligence in Science: Challenges, Opportunities and the Future of Research . OECD Publishing. 31
Swanson, D. R. (1986). Undiscovered public knowledge. The Library Quarterly , 56 (2), 103-118.
Table 1: Content summarized in tabular form by N. Bazarova as Associate Vice Provost, Research & Innovation; and Z. Jacques as Director, Research Administration Information Services for Cornell’s Ithaca and Cornell Tech campuses, with format suggested by B. Maddox, Chief Information Officer at Cornell’s Ithaca campus.
Van Dis, E. A., Bollen, J., Zuidema, W., van Rooij, R., & Bockting, C. L. (2023). ChatGPT: five priorities for research. Nature , 614 (7947), 224-226.
Van Noorden, R., & Perkel, J. M. (2023). AI and science: what 1,600 researchers think. Nature , 621 (7980), 672-675.
Verma, P. and Ormeus, W. These lawyers used ChatGPT to save time. They got fired and fined. Washington Post , published 11/16/2023.
Waitman, L.R., Bailey, L.C., Becich, M.J., Chung-Bridges, K., Dusetzina, S.B., Espino, J.U., Hogan, R., Kaushal, R., McClay, J.C., Merritt, J.G., Rothman, R.L., Shenkman, E.A., Song, X., & Nauman, E. (2023). Avenues for strengthening PCORnet’s capacity to advance patient-centered economic outcomes in patient-centered outcomes research (PCOR). Medical Care 61(12), S153-S160.
Zewe, A. Explained: What is Generative AI? MIT News, published 11/9/2023.
A ppendix 3. Task Force Charge
The following charge was provided to the task force by Cornell’s vice president for research & innovation, Krystyn J. Van Vliet, who worked with the task force comprising membership across Cornell’s several campuses of research communities in New York state (Ithaca and Geneva, Cornell Tech, and Weill Cornell Medicine) to finalize the report prior to public release. The task force engaged a wider cross-section of the research community’s departments and disciplines through discussions during the report development, and a Cornell-internal comment period on a draft version of the report in fall 2023 engaged faculty and staff from additional departments, colleges, interdisciplinary research centers, and offices.
Charge on Generative AI in Academic Research: Perspectives and Cultural Norms
Cornell’s leadership recognizes the opportunity and challenge of generative artificial intelligence (GenAI) on academic research, as well as the communication and translation of research outcomes to research peers and broader society. The Vice President for Research & Innovation charges this task force to discuss and offer guidelines and practices for GenAI in the practice and dissemination of research. The outcome of this ad hoc task force provides clarity in establishing perspectives and cultural norms for Cornell researchers and research team leaders, as internal advice, and is not meant to be a set of binding rules.
Charge to Task Force
Generative artificial intelligence is a tool that is now widely available to the research community. Such capabilities can provide new efficiencies and insights in research, and can also introduce new quandaries for the responsible conduct of research. Faculty and senior research scientists (also called principal investigators of externally funded research) are leaders of research projects, and are thus ultimately responsible for setting and adhering to such norms – particularly when formal guidelines are nascent or disparate. Cornell now has the opportunity to discuss and establish these cultural and professional norms, consistent with our wider institutional values in responsible research across many fields.
This group of staff and faculty is charged to consider any guidelines and best practices on appropriate use and attribution of generative AI that should be shared with the Cornell research community of students, staff and faculty. This task force should identify the range of cultural norms consistent with Cornell values when using this class of tools for research. These recommendations should be communicated in a brief (<10 pages written), internal advisory report by Monday 6 November 2023.
The task force should not include extensions to Cornell education or admissions or hiring practices or institutional communications; those use cases are under consideration elsewhere.
Task Force Roster (listed alphabetically by family name)
- Natalie Bazarova Department of Communication, College of Agriculture and Life Sciences
- Michèle Belot Department of Economics, School of Industrial and Labor Relations 33
- Olivier Elemento Department of Physiology and Biophysics, Weill Cornell Medicine
- Thorsten Joachims Departments of Computer Science and Information Science, Cornell Bowers CIS
- Alice Li Cornell Center for Technology Licensing, OVPRI
- Bridget MacRae Office of Research Integrity Assurance, OVPRI
- David Mimno Information Science, Cornell Bowers CIS
- Lisa Placanica Cornell Center for Technology Licensing, OVPRI and Weill Cornell Medicine
- Alexander (Sasha) M. Rush Cornell Tech and Department of Computer Science, Cornell Bowers CIS
- Stephen Shu Dyson School, SC Johnson College of Business
- Simeon Warner Cornell University Library
- Fengqi You Smith School of Chemical and Biomolecular Engineering, College of Engineering
Generative AI Can Supercharge Your Academic Research
Explore more.
- Artificial Intelligence
- Perspectives
C onducting relevant scholarly research can be a struggle. Educators must employ innovative research methods, carefully analyze complex data, and then master the art of writing clearly, all while keeping the interest of a broad audience in mind.
Generative AI is revolutionizing this sometimes tedious aspect of academia by providing sophisticated tools to help educators navigate and elevate their research. But there are concerns, too. AI’s capabilities are rapidly expanding into areas that were once considered exclusive to humans, like creativity and ingenuity. This could lead to improved productivity, but it also raises questions about originality, data manipulation, and credibility in research. With a simple prompt, AI can easily generate falsified datasets, mimic others’ research, and avoid plagiarism detection.
As someone who uses generative AI in my daily work, both in academia and beyond, I have spent a lot of time thinking about these potential benefits and challenges—from my popular video to the symposium I organized this year, both of which discuss the impact of AI on research careers. While AI can excel in certain tasks, it still cannot replicate the passion and individuality that motivate educators; however, what it can do is help spark our genius.
Below, I offer several ways AI can inspire your research, elevating the way you brainstorm, analyze data, verify findings, and shape your academic papers.
AI’s potential impact on research, while transformative, does heighten ethical and existential concerns about originality and academic credibility. In addition to scrutiny around data manipulation and idea plagiarism, educators using AI may face questions about the style, or even the value, of their research.
However, what truly matters in academic research is not the tools used, but educators’ approach in arriving at their findings. Transparency, integrity, intellectual curiosity, and a willingness to question and challenge one’s previous beliefs and actions should underpin this approach.
Despite potentially compounding these issues, generative AI can also play a pivotal role in addressing them. For instance, a significant problem in research is the reliance on patterns and correlations without understanding the “why” behind them. We can now ask AI to help us understand causality and mechanisms that are most likely. For example, one could inquire, “ What are the causal explanations behind these correlations? What are the primary factors contributing to spurious correlations in this data? How can we design tests to limit spurious correlations? ”
AI has the potential to revolutionize research validation, ensuring the reliability of findings and bolstering the scientific community’s credibility. AI’s ability to process massive amounts of data efficiently makes it ideal for generating replication studies. Instructions such as “ Suggest a replication study design and provide detailed instructions for independent replication ,” or “ Provide precise guidance for configuring a chatbot to independently replicate these research findings ” can guide educators in replicating and verifying study results.
ChatGPT-4 , OpenAI’s latest and paid version of the large language model (LLM), plays a vital role in enhancing my daily research process; it has the capacity to write, create graphics, analyze data, and browse the internet, seemingly as a human would. Rather than using predefined prompts, I conduct generative AI research in a natural and conversational manner, using prompts that are highly specific to the context.
To use ChatGPT-4 as a valuable resource for brainstorming, I ask it prompts such as, “ I am thinking about [insert topic], but this is not a very novel idea. Can you help me find innovative papers and research from the last 10 years that has discussed [insert topic]? ” and “ What current topics are being discussed in the business press? ” or “ Can you create a table of methods that have and have not been used related to [insert topic] in recent management research? ”
The goal is not to have a single sufficient prompt, but to hone the AI’s output into robust and reliable results, validating each step along the way as a good scholar would. Perhaps the AI sparks an idea that I can then pursue, or perhaps it does not help me at all. But sometimes just asking the questions furthers my own process of getting “unstuck” with hard research problems.
There is still a lot of work to be done after using these prompts, but having an AI research companion helps me quickly get to a better answer. For example, the prompt “ Explore uncharted areas in organizational behavior and strategy research ” led to the discovery of promising niches for future research projects. You might think that this will result in redundant projects, but all you have to do is write, “ I don’t like that, suggest more novel ideas ” or “ I like the second point, suggest 10 ideas related to it and make them more unique ” to come up with some interesting projects.
2. Use AI to gather and analyze data
Although the AI is far from perfect, iterative feedback can help its output become more robust and valuable. It is like an intelligent sounding board that adds clarity to your own ideas. I do not necessarily have a set of similar prompts that I always use to gather data, but I have been able to leverage ChatGPT-4’s capabilities to assist in programming tasks, including writing and debugging code in various programming languages.
Additionally, I have used ChatGPT-4 to craft programs designed for web scraping and data extraction. The tool generates code snippets that are easy to understand and helps find and fix errors, which makes it useful for these tasks. Prior to AI, I would spend far more time debugging software programs than I did writing. Now, I simply ask, “ What is the best way to collect data on [insert topic]? What is the best software to use for this? Can you help get that data? How do I build the code to get this data? What is the best way to analyze this data? If you were a skeptical reviewer, what would you also control for with this analysis? ”
“While the initial results may not be on point, starting from scratch without AI is still more difficult.”
When the AI generates poor responses, I ask, “ That did not work. Here is my code, can you help me find the problem? Can you help me debug this code? Why did that not work? ” or “ No, that is incorrect. Can you suggest two alternative ways to generate the result? ” There have been many occasions when the AI suggests that data will exist; however, like inspiration in the absence of AI, the data is not practically accessible or useful upon further examination. In those situations, I write, “ That data is too difficult to get, can you suggest good substitutes? ” or “ That is not real data, can you suggest more novel data or a data source where I can find the proper data? ”
While the initial results may not be on point, starting from scratch without AI is still more difficult. By incorporating AI into this data gathering and analysis process, researchers can gain valuable insights and solve difficult problems that often have ambiguous and equivocal solutions. For instance, learning how to program more succinctly or think of different data sources can help discovery. It also makes the process much less frustrating and more effective.
3. Use AI to help verify your findings and enhance transparency
AI tools can document the evolution of research ideas, effectively serving as a digital audit trail. This trail is a detailed record of a research process, including queries, critical decision points, alternative hypotheses, and refinements throughout the entire research study creation process. One of the most significant benefits of maintaining a digital audit trail is the ability to provide clear and traceable evidence of the research process. This transparency adds credibility to research findings by demonstrating the methodical steps taken to reach conclusions.
For example, when I was writing some code to download data from an external server, I asked, “ Can you find any bugs or flaws in this software program? ” and “ What will the software program’s output be? ” One of the problems I ran into was that the code was inefficient and required too much memory, taking several days to complete. When I asked, “ Could you write it in simpler and more efficient code? ” the generated code provided an alternative method for increasing data efficiency, significantly reducing the time it took.
“Prior to AI, I would spend far more time debugging software programs than I did writing.”
What excites me the most is the possibility of making it easier for other researchers to replicate what I did. Because writing up these iterations takes time, many researchers skip this step. With generative AI, we can ask it to simplify many of these steps so that others can understand them. For example, I might ask the following:
Can you write summarized notations of this program or of the previous steps so that others can understand what I did here?
Can we reproduce these findings using a different statistical technique?
Can you generate a point-by-point summary diary of what I did in the previous month from this calendar?
Can you create a step-by-step representation of the workflow I used in this study?
Can you help generate an appendix of the parameters, tests, and configuration settings for this analysis?
In terms of qualitative data, I might ask, “ Can you identify places in this text where this idea was discussed? Please put it in an easy-to-understand table ” or “ Can you find text that would negate these findings? What conditions do you believe generated these counterfactual examples? ”
You could even request that the AI create a database of all the prompts you gave it in order for it to generate the results and data. With the advent of AI-generated images and videos, we may soon be able to ask it to generate simple video instructions for recreating the findings or to highlight key moments in a screen recording of researchers performing their analyses. This not only aids validation but also improves the overall reliability and credibility of the research. Furthermore, because researchers incur little cost in terms of time and resources, such demands for video instructions may eventually be quite reasonable.
4. Use AI to predict and then parse reviewer feedback
I try to anticipate reviewer concerns before submitting research papers by asking the AI, “ As a skeptical reviewer who is inclined to reject papers, what potential flaws in my paper do you see? How can I minimize those flaws? ” The results help me think through areas where my logic or analysis may be flawed, and what I might want to refine before submitting my paper to a skeptical scientific audience. The early detection of problems in a competitive scientific arena with high time pressure can be effective and time saving.
Once I receive reviewer feedback, I also like to use ChatGPT to better understand what reviewers expect of me as an author. I’ll ask, “ Help me identify key points in this review, listing them from the easiest and quickest comments to address, up to the most challenging and time-consuming reviewer comments. ” It’s surprising how much more enjoyable the review process becomes once I have a more holistic understanding of what the reviewer or editor is asking.
Balancing AI’s strengths and weaknesses to improve academic research
As educators, we must learn to coexist and co-create with these technological tools. LLMs have the potential to accelerate and improve research, resulting in ground-breaking ideas that push the limits of current possibilities.
But we must be careful. When used incorrectly, AI can speed up the process of achieving surface-level learning outcomes at the expense of a deeper understanding. Educators should approach generative AI with skepticism and curiosity, like they would with any other promising tool.
AI can also democratize research by making it accessible to people of all abilities and levels of expertise. This only makes our human essence—passions, interests, and complexities—even more important. After all, AI might be great at certain tasks, but the one thing it can’t take away is what makes you, well, you.
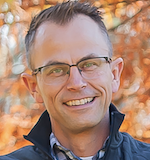
David Maslach is an associate professor at Florida State University specializing in organizational learning and innovation. He holds a PhD from the Ivey School of Business and serves on multiple academic journal boards. Maslach is also the founder of the R3ciprocity Project , a platform that provides solutions and hope to the global research community.
AI Tools For Academic Research: Top 10
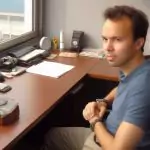
The world of academic research is constantly evolving, and artificial intelligence (AI) is playing a significant role in transforming the research landscape. From finding sources to analyzing data, AI-powered tools are making the research process more efficient and accurate. This article provides our roundup of the top 10 AI tools that are revolutionizing academic research .
Reviewing AI Software for Academic Researchers
What’s the toughest part of advancing in your postgraduate studies? For a multitude of students, the challenge lies in tackling the enormous amount of research required. As well as structuring it and putting all observations on paper.
The sheer number of research articles one needs to go through can be intimidating. Additionally, research material tends to be complex, making it difficult to extract the necessary information. This process demands a significant amount of time and effort. Organizing your insights and articulating them in a coherent, insightful, and scholarly manner presents yet another obstacle in postgraduate research. While AI tools for academic research can help with that issue, the essay writing sites can facilitate the process of finalizing the findings.
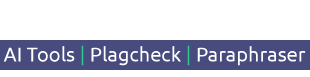
The Choice of The Number 1 AI Tool for Academia: Best Solution
Postdoctoral researcher Mushtaq Bilal believes that ChatGPT will revolutionize academic research, but acknowledges that many academics don’t know how to use it effectively. Academia is split between early AI adopters and those concerned about its impact on academic integrity . Bilal, an early adopter, believes that AI language models can democratize education and promote greater knowledge if used thoughtfully.
Top List of the Academic Research Software
Several experts have raised concerns about the reliability of language models like ChatGPT, noting that their output, when used as a best AI text generator , can sometimes be biased, limited, or inaccurate. However, Bilal argues that being aware of these limitations and adopting the right approach can enable language models to perform valuable work, particularly in academia.
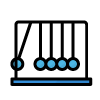
You can ask Consensus about relationships between concepts, or even cause and effect, like whether immigration improves the economy. It’ll give you an answer based on academic research, even listing the papers and summarizing the top ones.
But it’s got a limited scope, though. It only covers six areas: economics, sleep, social policy, medicine, mental health, and health supplements. Still, it seems like a handy resource for those topics.
It saves time by providing quick access to research-backed answers on the covered topics. Consensus bases its answers on academic research, increasing the credibility of the information. The tool provides summaries of the top articles it analyzes, making it easier to understand complex research.
It’s an easily accessible way for users to gain knowledge about specific topics without needing extensive research skills. By providing research-backed answers, Consensus promotes evidence-based thinking.
- Efficient research
- Credible sources
- Encourages evidence-based decision making
- Limited scope
- Potential bias
- AI limitations
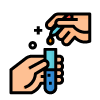
Elicit is like a research assistant that uses language models to answer questions, but it’s entirely based on research. This makes it a solid source for having “intelligent conversations” and brainstorming sessions.
What’s cool is that it can find relevant papers even without exact keyword matches, and it can summarize them, making it a handy paper typer that pulls out the key details.
Elicit’s knowledge is solely based on research, which ensures a more reliable and verified source of information. It can find relevant papers without needing perfect keyword matches, making it easier to discover important research.
- Research-based knowledge
- Flexible search
- Supports creative thinking
- Limited to research
- Accessibility
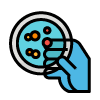
Scite uses AI to provide detailed citation information for research papers, helping researchers evaluate the credibility of their sources. This service is really helpful for getting real citations from actual published papers. It’s great for improving workflows. Scite gives you answers to questions with a detailed list of cited papers. Plus, it tells you the exact number of times a claim has been refuted or corroborated in various journals, making it a powerful tool.
- Accurate citations
- Improves workflow
- Fact-checking capability
- Potential for user error
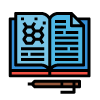
Research Rabbit
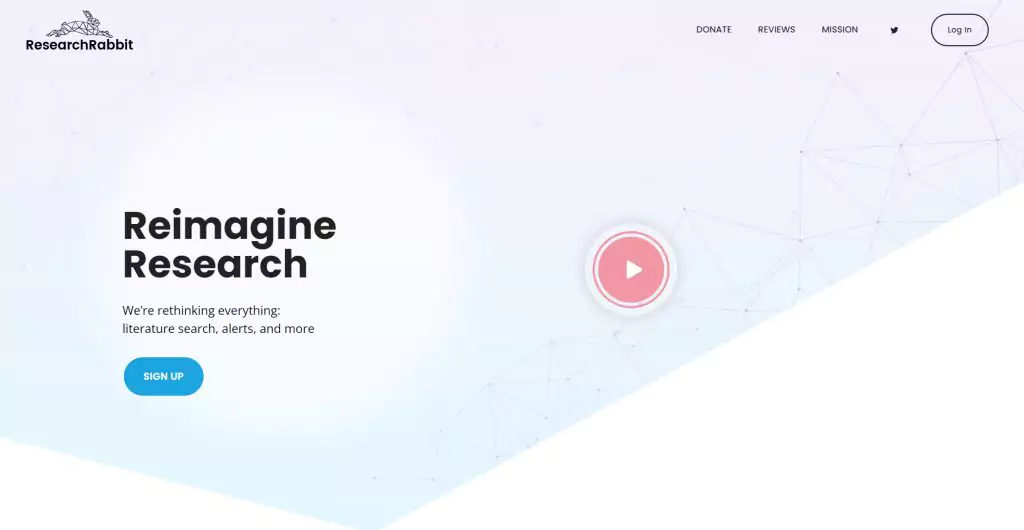
Research Rabbit is a tool for fast-tracking research, and the best part is that it’s free!
It can be called “Spotify of research.” Users can create collections of academic papers that the software can learn from to give them relevant recommendations. Plus, it even visualizes scholarly networks in graphs, so it’s possible follow the work of specific authors or topics.
- Time-saving
- Personalized recommendations
- Limited to academic papers
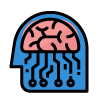
ChatPDF = “like ChatGPT, but for research papers”. It could be useful for reading and analyzing journal articles.
Basically, you start by uploading a PDF of the research paper into the app, and then you can start asking it questions. ChatPDF will then generate a short summary of the paper and provide examples of questions that it can answer based on the full article.
This could really speed up the process of reading and analyzing research papers, which can be a time-consuming task.
- Summarization capabilities
- Question examples
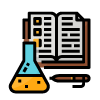
Perplexity AI
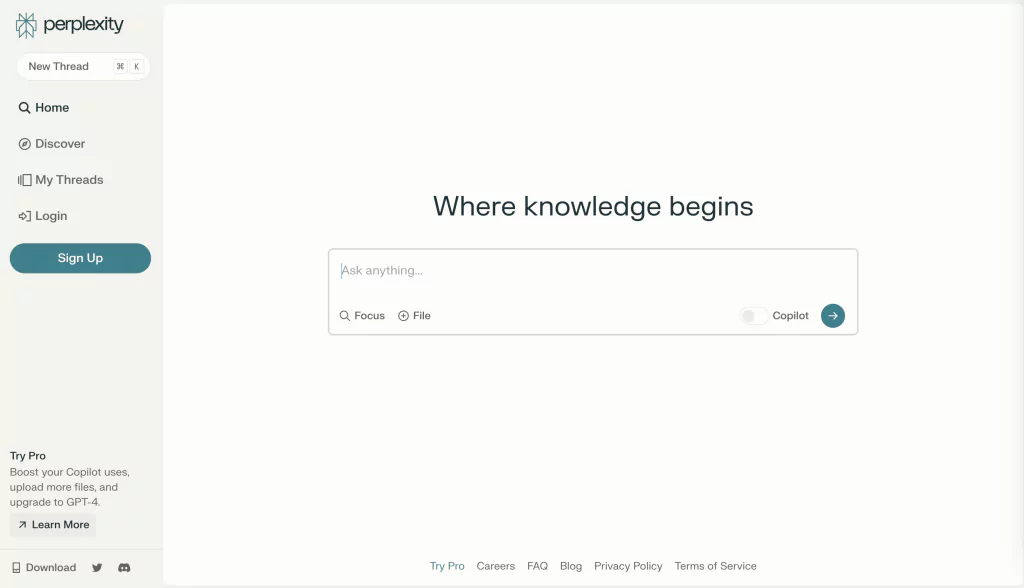
Perplexity AI can potentially become a number 1 tool for academic research. Arguably better than ChatGPT, Perplexity boasts functions that its famous rival doesn’t have. So what exactly is Perplexity AI?
It’s another AI search engine with powerful academic research abilities. PerplexityAI has access to a variety of different sources, which makes information completely up-to-date. The tool can draw information not only from the Internet, which most other services do well, but also from scholarly sources, WolframAlpha, YouTube, Reddit, news, and Wikipedia. After typing in a search query, a user can specify where exactly they want to receive the information from, and the tool will do the task. Additionally, it can search across individual domains or websites or summarize their content.
A significant advantage of Perplexity is its advanced functionality when searching for academic materials. Not only does it give you the result, but it also offers a list of related questions and references. This is an edge compared to ChatGPT, especially GPT-3.5, which often makes users question the relevance or even existence of the references it provides.
With all these features onboard, apps for essay , and Chrome extension, Perplexity is an excellent AI tool for academic research.
- Actual scholar references
- Available for iPhone (Android app is coming)
- Many credible sources of data
- Clunky functionality with PDFs
- Lacks AI conversational skills
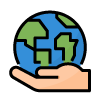
Semantic Scholar
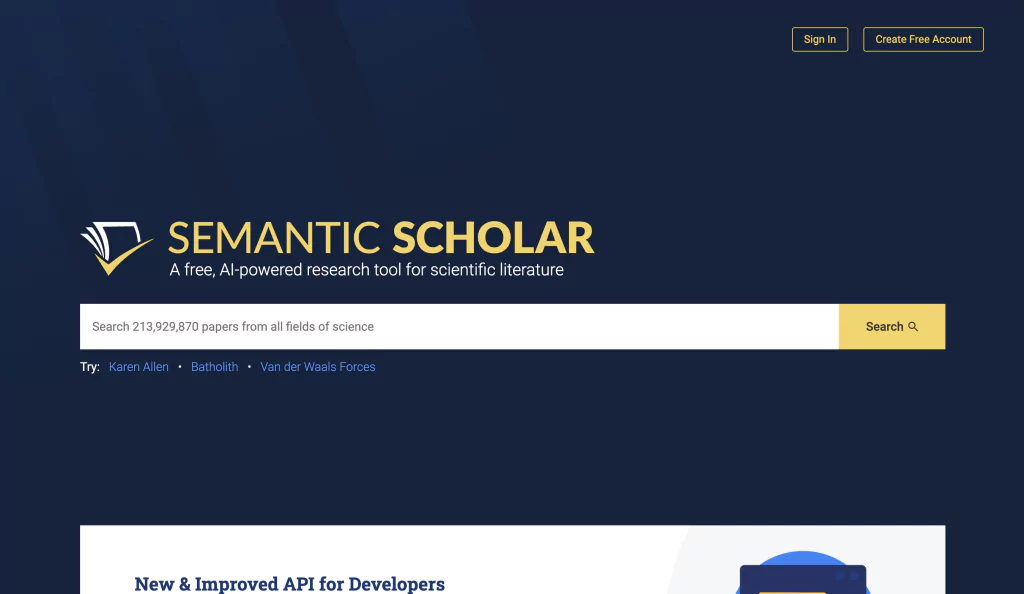
Semantic Scholar is an academic search engine driven by artificial intelligence that enables users to filter through millions of scholarly pieces for educationally appropriate content related to their research subject. It integrates artificial intelligence, machine learning, and language processing with semantic analysis to provide users with precise search outcomes.
Using machine learning methodologies, Semantic Scholar deciphers significance and discovers links within academic papers. It then presents these findings, facilitating scholars in rapidly acquiring a comprehensive understanding.
- Highlights the most crucial elements of a paper
- Free to use
- The limited scope of fields for research
- Narrow evaluation metrics of scholarly articles
- Does not search for material behind a paywall
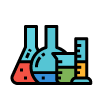
Iris.ai is an artificial intelligence tool designed to aid researchers in scientific discoveries. The service uses natural language processing and machine learning algorithms to comprehend the context of a research project and suggest pertinent literature. It helps navigate and find data sources without relying on specific keywords, making it significantly more efficient than traditional search engines.
Content-based search, context and data filtering, and extracting and systematizing data are just a few of the many other functions of this versatile research tool.
- Fast and easy to use
- Has access to vast databases of research articles, including open access papers
- Dependent on the accuracy and quality of the AI algorithms
- Not the best choice for marketing research or economics

Paper Digest
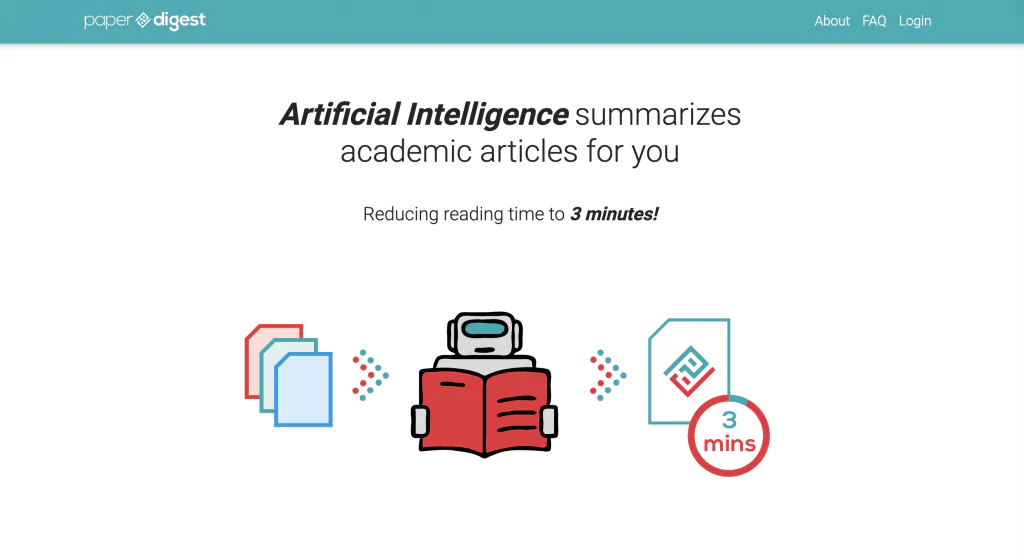
Updated August 28, 2023: he service has stopped operations and the website is no longer maintained. Paper Digest is an AI-based academic article summarization service that aims to help researchers quickly grasp the core ideas of a paper without reading the whole thing. It automatically lists out the key sentences of a paper, taking about 10 seconds to do so. It can also reduce reading time to 3 minutes. The service also imitates researcher behavior by automatically summarizing the paper and helping them decide whether it is worth reading. However, Paper Digest may not be suitable for researchers who require more detailed summaries or who need to capture all the nuances of the original paper.
- Can be accessed from any device
- Can be used for free
- Works only with open-access papers
- The summaries may not capture all the nuances of the original paper
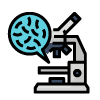
SciSpace is an AI-powered platform that aims to modernize scientific research workflows and collaboration. It offers a suite of tools to discover, read, write, collaborate, and publish research papers. It also links users to more than 45,000 verified journal formats that researchers can select from, making it more suitable than Word for research writing.
SciSpace is a useful service for those looking for an easy and quick way to understand scholar papers. Its AI-powered features and personalized suggestion engine can help researchers stay on track while gaining a comprehensive understanding of the topic.
- Personalized suggestion engine
- 40,000+ journal templates and processes 30,000+ papers per month
- Has a grammar and spell-checking systems
- Issues with exporting to different journal formats
- The free plan is quite basic and lacks major features
AI-powered tools are transforming the way we approach academic research, making it more efficient, accurate, and accessible. By leveraging the capabilities of these top 10 AI tools, researchers can save time, improve the quality of their work, and contribute to groundbreaking discoveries in their respective fields .
Best Tips How to Make the Most of AI Tools as a PhD student
PhD students employ AI-chatbots, such as ChatGPT, to enhance their studies and boost efficiency. Some ways they use AI-chatbots include:
- Summarizing texts for quicker reading and idea mapping.
- Checking the validity of individual arguments on different aspects.
- Exploring comprehensiveness by discussing generated options and seeking additional possibilities.
- Testing counterfactuals by presenting arguments and asking for opposing viewpoints.
- Preparing for a jury by sharing arguments and requesting ten related questions.
- Requesting critiques on arguments for improvement.
PhD students can also:
- Direct prompts from the perspective of a renowned book on the topic.
- Use ChatGPT as a writing mentor for thesis and research papers.
- Utilize it for basic proofreading of academic texts, adjusting tone and voice as needed, and rating the original text on a scale of 1 to 10.
- Generate an outline of a dissertation’s main chapter using ChatGPT-generated prompts.
- Employ the “freewriting” technique, writing down unfiltered thoughts, then prompting ChatGPT to refine the text for a scientific publication using appropriate language.
Why academichelp.net is a credible source of information:
Stay curious with us. Academichelp.net has been a reliable educational resource since 2011, providing students with the latest news, assignment samples, and other valuable materials. Even with the extensive information we process, our quality remains consistent. Each team member has experience in education, allowing us to evaluate new sector offerings critically. Our reviews are up-to-date and relevant, with impartiality ensured by the A*Help score methodology from mystery shopping. We aren’t affiliated with any listed service providers. Our focus remains on providing our audience with reliable and unbiased data.
What is the best AI academic research tool?
Choosing the best AI platform for academic research depends on your priorities and preferences. Our experience suggests that the top choice is often a website that combines various services in one place.
Is there a free AI academic research tool?
Yes, there are free AI academic research tools. Many platforms do not require subscriptions or additional payments. Some websites also provide new clients with a free trial to test certain features. Most of these solutions are mentioned in our top list, so be sure to check it out to find the AI service that suits you best.
Which AI model supports academic research?
Academic research is primarily facilitated by language models that use NLP (natural language processing) and deep learning to gather relevant data and generate different types of content. One of the major companies developing language models is OpenAI, with its latest releases including GPT-3, GPT-3.5, and GPT-4 AI. Other popular language models are LamDa, used by Google, and LLaMA, adopted by Meta and its social media companies.
What is the best free AI for academic research?
To identify the best free AI-powered platform for academic research, you need to determine your specific needs, such as text generation, reference finder, rephrasing tool, or other services.
Students also ask
Can ai be used for research.
Absolutely! AI is a valuable tool for research, aiding in data analysis, pattern recognition, and simulations. So, when you feel a little stuck when doing research for your assignments, you can try out an AI helper.
Which AI is best for research?
The ideal AI for research purposes depends on the specific field’s needs and goals! Different AI models excel in various tasks, so there is no definitive answer to that question unless you do a bit of research first.
How is AI used in scientific research?
AI is very often used in scientific research for a number of reasons. It can easily analyze vast datasets, simulate different experiments, and assist the person, helping researchers uncover new insights and continue their work.
Is there an AI that can read scientific papers?
Yes, there are AI systems capable of doing so. They can extract the necessary information from scientific papers according to the prompt you give the system.
What is the smartest AI today?
Determining the smartest AI is subjective since it can be used for a variety of needs. However, models like GPT-3 and GPT-4 have demonstrated remarkable language processing and comprehension abilities, which can significantly ease the process of writing or compiling information.
Can AI discover new knowledge?
AI plays a crucial role in discovering new knowledge by identifying correlations, suggesting hypotheses, and aiding in data analysis. It helps researchers to make certain discoveries. Although AI is getting more and more popular each day, it cannot replace humans and should rather be used as a tool.
Is AI going to replace scientists?
AI is not poised to replace scientists, writers, or anyone for that matter. Instead, it enhances their capabilities, enabling more efficient and effective research, which leads to accelerated progress. Human impact is undeniably necessary when working with AI, even though it may seem the other way.
Can AI discover new things?
Certainly! AI sometimes can discover new things by analyzing data and detecting patterns. This way AI is contributing to scientific advancements and expanding our understanding of the world.
Useful AI solution articles
- Khanmigo: Khan Academy’s AI Solution to Enhance Learning and Tackle Classroom Challenges
- Doctrina AI: Artificial Intelligence for Learning
- Introducing Wisdolia: The AI-Powered Flashcard Generator
- StudyWand: An AI-Powered Tool for Exam Preparation
AI trends and latest news
- Malaysia’s Ministry of Higher Education Developing Guidelines for ChatGPT Usage in Universities
- OpenAI Expert, Anna Bernstein, Shares Tips for Crafting Effective AI Chatbot Prompts
Follow us on Reddit for more insights and updates.
Comments (1)
Welcome to A*Help comments!
We’re all about debate and discussion at A*Help.
We value the diverse opinions of users, so you may find points of view that you don’t agree with. And that’s cool. However, there are certain things we’re not OK with: attempts to manipulate our data in any way, for example, or the posting of discriminative, offensive, hateful, or disparaging material.
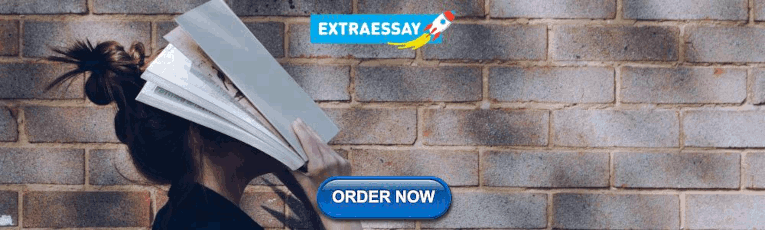
Cancel reply
Your email address will not be published. Required fields are marked *
Save my name, email, and website in this browser for the next time I comment.
What’s the guarantee that inputs provided is not being snooped by someone at the backend? In other words,
Remember Me
Is English your native language ? Yes No
What is your profession ? Student Teacher Writer Other
Forgotten Password?
Username or Email
share this!
May 13, 2024
This article has been reviewed according to Science X's editorial process and policies . Editors have highlighted the following attributes while ensuring the content's credibility:
fact-checked
trusted source
written by researcher(s)
AI-assisted writing is quietly booming in academic journals—here's why that's OK
by Julian Koplin, The Conversation
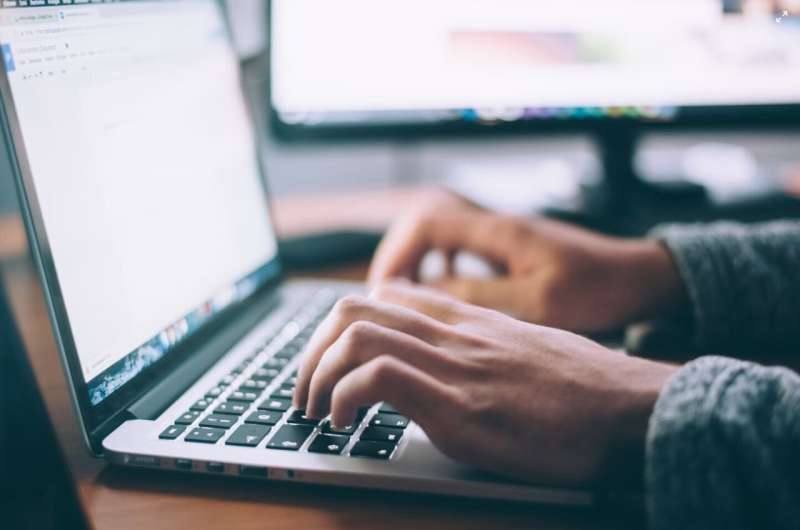
If you search Google Scholar for the phrase " as an AI language model ," you'll find plenty of AI research literature and also some rather suspicious results. For example, one paper on agricultural technology says,
"As an AI language model, I don't have direct access to current research articles or studies. However, I can provide you with an overview of some recent trends and advancements …"
Obvious gaffes like this aren't the only signs that researchers are increasingly turning to generative AI tools when writing up their research. A recent study examined the frequency of certain words in academic writing (such as "commendable," "meticulously" and "intricate"), and found they became far more common after the launch of ChatGPT—so much so that 1% of all journal articles published in 2023 may have contained AI-generated text.
(Why do AI models overuse these words? There is speculation it's because they are more common in English as spoken in Nigeria, where key elements of model training often occur.)
The aforementioned study also looks at preliminary data from 2024, which indicates that AI writing assistance is only becoming more common. Is this a crisis for modern scholarship, or a boon for academic productivity?
Who should take credit for AI writing?
Many people are worried by the use of AI in academic papers. Indeed, the practice has been described as " contaminating " scholarly literature.
Some argue that using AI output amounts to plagiarism. If your ideas are copy-pasted from ChatGPT, it is questionable whether you really deserve credit for them.
But there are important differences between "plagiarizing" text authored by humans and text authored by AI. Those who plagiarize humans' work receive credit for ideas that ought to have gone to the original author.
By contrast, it is debatable whether AI systems like ChatGPT can have ideas, let alone deserve credit for them. An AI tool is more like your phone's autocomplete function than a human researcher.
The question of bias
Another worry is that AI outputs might be biased in ways that could seep into the scholarly record. Infamously, older language models tended to portray people who are female, black and/or gay in distinctly unflattering ways, compared with people who are male, white and/or straight.
This kind of bias is less pronounced in the current version of ChatGPT.
However, other studies have found a different kind of bias in ChatGPT and other large language models : a tendency to reflect a left-liberal political ideology.
Any such bias could subtly distort scholarly writing produced using these tools.
The hallucination problem
The most serious worry relates to a well-known limitation of generative AI systems: that they often make serious mistakes.
For example, when I asked ChatGPT-4 to generate an ASCII image of a mushroom, it provided me with the following output.
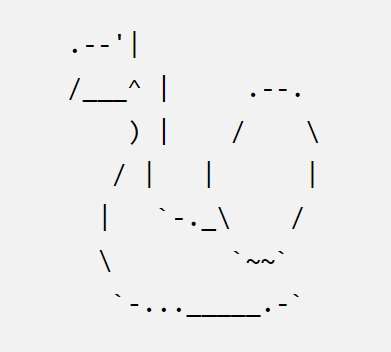
It then confidently told me I could use this image of a "mushroom" for my own purposes.
These kinds of overconfident mistakes have been referred to as "AI hallucinations" and " AI bullshit ." While it is easy to spot that the above ASCII image looks nothing like a mushroom (and quite a bit like a snail), it may be much harder to identify any mistakes ChatGPT makes when surveying scientific literature or describing the state of a philosophical debate.
Unlike (most) humans, AI systems are fundamentally unconcerned with the truth of what they say. If used carelessly, their hallucinations could corrupt the scholarly record.
Should AI-produced text be banned?
One response to the rise of text generators has been to ban them outright. For example, Science—one of the world's most influential academic journals—disallows any use of AI-generated text .
I see two problems with this approach.
The first problem is a practical one: current tools for detecting AI-generated text are highly unreliable. This includes the detector created by ChatGPT's own developers, which was taken offline after it was found to have only a 26% accuracy rate (and a 9% false positive rate ). Humans also make mistakes when assessing whether something was written by AI.
It is also possible to circumvent AI text detectors. Online communities are actively exploring how to prompt ChatGPT in ways that allow the user to evade detection. Human users can also superficially rewrite AI outputs, effectively scrubbing away the traces of AI (like its overuse of the words "commendable," "meticulously" and "intricate").
The second problem is that banning generative AI outright prevents us from realizing these technologies' benefits. Used well, generative AI can boost academic productivity by streamlining the writing process. In this way, it could help further human knowledge. Ideally, we should try to reap these benefits while avoiding the problems.
The problem is poor quality control, not AI
The most serious problem with AI is the risk of introducing unnoticed errors, leading to sloppy scholarship. Instead of banning AI, we should try to ensure that mistaken, implausible or biased claims cannot make it onto the academic record.
After all, humans can also produce writing with serious errors, and mechanisms such as peer review often fail to prevent its publication.
We need to get better at ensuring academic papers are free from serious mistakes, regardless of whether these mistakes are caused by careless use of AI or sloppy human scholarship. Not only is this more achievable than policing AI usage, it will improve the standards of academic research as a whole.
This would be (as ChatGPT might say) a commendable and meticulously intricate solution.
Provided by The Conversation
Explore further
Feedback to editors

A devastating fire 2,200 years ago preserved a moment of life and war in Iron Age Spain, down to a single gold earring
7 hours ago

Airborne technology brings new hope to map shallow aquifers in Earth's most arid deserts
13 hours ago

First-generation medical students face unique challenges and need more targeted support, say researchers
14 hours ago

Thermoelectric materials approach boosts band convergence to avoid time-consuming trial-and-error approach

Ion swap dramatically improves performance of CO₂-defeating catalyst
15 hours ago

Military rank affects medical care, offering societal insights: Study

Mystery CRISPR unlocked: A new ally against antibiotic resistance?
16 hours ago

Researchers develop a detector for continuously monitoring toxic gases

Sea otter study finds tool use allows access to larger prey, reduces tooth damage
17 hours ago

Accelerated discovery research unveils 21 novel materials for advanced organic solid-state laser technology
Relevant physicsforums posts, physics education is 60 years out of date.
12 hours ago
Is "College Algebra" really just high school "Algebra II"?
May 14, 2024
Plagiarism & ChatGPT: Is Cheating with AI the New Normal?
Physics instructor minimum education to teach community college.
May 11, 2024
Studying "Useful" vs. "Useless" Stuff in School
Apr 30, 2024
Why are Physicists so informal with mathematics?
Apr 29, 2024
More from STEM Educators and Teaching
Related Stories

AI-generated academic science writing can be identified with over 99% accuracy
Jun 7, 2023

ChatGPT maker fields tool for spotting AI-written text
Feb 1, 2023

Is the genie out of the bottle? Can you trust ChatGPT in scientific writing?
Oct 19, 2023

What is ChatGPT: Here's what you need to know
Feb 16, 2023

Tool detects AI-generated text in science journals
Nov 7, 2023

Could artificial intelligence help or hurt medical research articles?
Feb 6, 2024
Recommended for you

Investigation reveals varied impact of preschool programs on long-term school success
May 2, 2024

Training of brain processes makes reading more efficient
Apr 18, 2024

Researchers find lower grades given to students with surnames that come later in alphabetical order
Apr 17, 2024

Earth, the sun and a bike wheel: Why your high-school textbook was wrong about the shape of Earth's orbit
Apr 8, 2024

Touchibo, a robot that fosters inclusion in education through touch
Apr 5, 2024
Let us know if there is a problem with our content
Use this form if you have come across a typo, inaccuracy or would like to send an edit request for the content on this page. For general inquiries, please use our contact form . For general feedback, use the public comments section below (please adhere to guidelines ).
Please select the most appropriate category to facilitate processing of your request
Thank you for taking time to provide your feedback to the editors.
Your feedback is important to us. However, we do not guarantee individual replies due to the high volume of messages.
E-mail the story
Your email address is used only to let the recipient know who sent the email. Neither your address nor the recipient's address will be used for any other purpose. The information you enter will appear in your e-mail message and is not retained by Phys.org in any form.
Newsletter sign up
Get weekly and/or daily updates delivered to your inbox. You can unsubscribe at any time and we'll never share your details to third parties.
More information Privacy policy
Donate and enjoy an ad-free experience
We keep our content available to everyone. Consider supporting Science X's mission by getting a premium account.
E-mail newsletter

Oxford University Press's Academic Insights for the Thinking World

Are academic researchers embracing or resisting generative AI? And how should publishers respond?

Oxford Academic
Learn more about the world of academic publishing—from open access to peer review, accessibility to getting published—with our Publishing 101 series on the OUPblog.
- By David Clark
- May 13 th 2024
The most interesting thing about any technology is how it affects humans: how it makes us more or less collaborative, how it accelerates discovery and communication, or how it distracts and frustrates us. We saw this in the 1990s. As the internet became more ubiquitous, researchers began experimenting with collaborative writing tools that allowed multiple authors to work on a single document simultaneously, regardless of their physical locations. Some of the earliest examples were the Collaboratories launched by researchers in the mid-1990s at the University of Michigan. These platforms enabled real-time co-authoring, annotation, and discussion, streamlining the research process and fostering international collaborations that would have been unimaginable just a few years earlier.
Most people, but not all, would agree that the internet has benefitted research and researchers’ working lives. But can we be so sure about the role of new technologies today, and, most immediately, generative AI?
Anyone with a stake in research—researchers, societies, and publishers, to name a few—should be considering an AI-enabled future and their role in it. As the largest not-for-profit research publisher, OUP is beginning to define the principles on which we are engaging with companies creating Large Language Models (LLMs). I wrote about this more extensively in the Times Higher Education , but important considerations for us include: a respect for intellectual property, understanding the importance of technology to support pedagogy and research, appropriate compensation and routes to attribution for authors, and robust escalation routes with developers to address errors or problems.
Ultimately, we want to understand what researchers consider important in the decision to engage with generative AI—what excites or concerns them, how they are using or imagining using AI tools, and the role they believe publishers (among other institutional stakeholders) can play in supporting and protecting their published research.
We recently carried out a global survey of researchers to explore how they felt about all aspects of AI—we heard from thousands of researchers across geographies, disciplines, and career stages. The results are revealing in many important ways, and we will be sharing these findings in more detail soon, but the point that struck me immediately was that many researchers are looking for guidance from their institutions, their scholarly societies, and publishers on how to make best use of AI.
Publishers like OUP are uniquely positioned to advocate for the protection of researchers and their research within LLMs. And we are beginning to do so in important ways, because Gen AI and LLM providers want unbiased, high-quality scholarly data to train their models, and the most responsible providers appreciate that seeking permission (and paying for that) is the most sustainable way of building models that will beat the competition. LLMs are not being built with the intention of replacing researchers, and nor should they be. However, such tools should benefit from using high quality scholarly literature, in addition to much of what sits on the public web. And since the Press, and other publishers, will use Gen AI technologies to make its own products and services better and more usable, we want LLMs to be as neutral and unbiased as possible.
As we enter discussions with LLM providers, we have important considerations to guide us. For example, we’d need assurances that there will be no intended verbatim reproduction rights or citation in connection with display (this includes not surfacing the content itself); that the content would not be used for the creation of substantially similar content, including reverse engineering; and that no services or products would be created for the sole purpose of creating original scholarship. The central theme guiding all of these discussions and potential agreements is to protect research authors against plagiarism in any of its forms.
We know this is a difficult challenge, particularly given how much research content has already been ingested into LLMs by users engaging with these conversational AI tools. But publishers like OUP are well positioned to take this on, and I believe we can make a difference as these tools evolve. And by taking this approach, we hope to ensure that researchers can either begin or continue to make use of the best of AI tools to improve their research outcomes.
Featured image by Alicia Perkins for OUP.
David Clark , Managing Director, Academic Division, Oxford University Press
- Online products
- Publishing 101
- Series & Columns
Our Privacy Policy sets out how Oxford University Press handles your personal information, and your rights to object to your personal information being used for marketing to you or being processed as part of our business activities.
We will only use your personal information to register you for OUPblog articles.
Or subscribe to articles in the subject area by email or RSS
Related posts:

Recent Comments
There are currently no comments.
Leave a Comment
Your email address will not be published. Required fields are marked *
Save my name, email, and website in this browser for the next time I comment.
Information Technology Services
- All Services and Software
- Computers and Devices
- Services for Students
- Services for Staff
- Services for Faculty
- Services for Researchers
- Services for IT Professionals
- Services for Guests
- Training and Events
- Online Training Videos (LinkedIn Learning)
- Technology Consulting
- IT Communities/Networking
- Support Information
- IT Service Alerts
- ITS Organization
- IT Strategic Plan
- IT Communities
You are here
Using ai in academics and research.
Generative AI is rapidly transforming the academic and research landscape, offering new opportunities for discovery and innovation. It is important to approach generative AI with a thoughtful approach, balancing the benefits with potential risks and ethical considerations. In this page, we collect information for using generative AI in an academic and research settings.
- The ITS Research Services group has provided Using Artificial Intelligence (AI) Tools in Research to explain the benefits and limitations of AI tools and to provide guidance on using AI tools in research at the University of Iowa.
- The Office of the Executive Vice President and Provost created a page with steps and tips for instructors to provide guidance in responding to AI in the classroom. This page includes suggestions of strategies for creating AI-resistant assignments, recent developments and challenges associated with AI in higher education, and the encouragement for instructors to provide students with clear instructions and ongoing discussion about what uses of AI are permissible within a course’s context.
- The Center for Teaching and the Office of Teaching, Learning, and Technology has written the page Artificial Intelligence Tools and Teaching to provide answers to some frequently asked questions about AI tools and teaching, such as how to address generative AI in a syllabus, how to have conversations with students, and how to learn more about AI’s impact on education.
Guidance on Student Facing Chatbots
As chatbots have become easier and less expensive to deploy, interest in them has increased and many faculty are starting to experiment with their use. Chatbots can provide information at any time, answering questions quickly, and can be an effective tool for both faculty and students.
To enable informed decisions about the uses and potential applications of chatbots in work, teaching and learning, and more, we ask faculty to consider the following guidance.
- Make sure you understand what goals you have for the chatbot. Is your goal to provide students with basic information or individualized learning opportunities? Is your chatbot being created to automate administrative tasks? A clear understanding of your goals will help you design and implement your chatbot.
- Adjust the chatbot responses to match your goals. Many chatbots allow you to control the tone, or voice, of the chatbot conversations. You should choose a style that matches the purpose for implementing the chatbot. For example, a chatbot that responds to questions in a formal manner may not be the best tone for a chatbot with a conversational purpose. A chatbot with a humorous or strongly emotional character may not be a good fit for a professional setting.
- Make sure that any chatbot that is student- or private-facing is introduced with reminders to users that private or sensitive information should not be shared with the chatbots because, in general, the university cannot protect the privacy of information shared with chatbots.
- Share a link to the University’s data classification guidelines to help users make informed decisions about their interactions with a chatbot.
- The capabilities and limitations of chatbots used in academic settings should be clearly communicated to students. Students should understand that chatbots are incapable of understanding, emotions, or subjective opinions. Any output from the chatbot should be treated as supplemental or a convenience. Students should verify essential information with an authoritative human source. These reminders about chatbot limitations could be shared with students in an introductory message or prompt that appears each time they access the chatbot tool.
- To avoid potential harm, users should be explicitly advised not to rely on any chatbot for emergencies, medical advice, or mental health support. Chatbots cannot assess emergencies or urgent situations and may give inaccurate, incomplete, or misleading information. Chatbots cannot replace trained professionals and using chatbots for health matters could have severe consequences.
- Chatbots will not be able to answer all questions. It is important that chatbots let users know when they don’t know an answer, instead of “hallucinating” or providing incorrect information in its responses. This is especially true for when users ask personal, self-harm, bullying, or food insecurity types of questions.
- To ensure validity of interactions with users, chatbots should be able to record their interactions so they can be reviewed later. Chatbots should also be able to share when they cannot produce an answer. Identify a person responsible for the chatbot and establish a regular schedule of review for this information to ensure the performance of the chatbot.
- The knowledge base and programming behind any chatbot will need ongoing maintenance and updates to expand its capabilities over time. Plan for allocating time and money, not just to the initial implementation of a chatbot, but also for continuous improvement to support the chatbot’s continued use.
- Students may use chatbots to get individualized help, to review content, to research a topic, and to find answers at any time and any location. Ask others, have a focus group, or whatever method works best to understand how people will use the chatbot before implementing the chatbot with students. Consider the types of questions the chatbot will be asked and see what the answers generated by the chatbot are.
Campus Resources:
For further information on developing messaging/communication that would resonate with students, contact Academic Support & Retention at [email protected] .
For more information on the role of generative AI at the University of Iowa, visit the ITS website here .
As we continue to explore AI, generative AI in particular, we’re open to learning more about how you are using AI. Faculty and staff can email their AI experiences, questions, and suggestions to [email protected] .
Generative AI & Research
- What is Generative AI?
- Examples of Gen AI Tools
- Uses in Research
- Citing Generative AI
- Example - Evaluate a Tool (ChatGPT)
- Example - Content Evaluation (ChatGPT)
- Use in Academic Publishing
- Faculty Resources
Publishing & Generative AI
Generative AI tools raise questions and concerns around authorship, research integrity, and more. At the same time, among other uses, these tools can ease and accelerate the editing process. Scholarly publishers have released policies and guidance for the use of Generative AI, for both authors and sometimes reviewers. As both the technology and its use evolve, publishers will review and adapt their policies.
Practical Takeaways for Authors
- Review the Generative AI policies of potential publication outlets prior to any Generative AI use.
- Document Generative AI use and err on the side of more documentation than less.
- Remember plagiarism, copyright, and attribution concerns during use.
- Ensure disclosure of Generative AI use upon submission.
A Survey of Publisher Policies
Policies for Authors
Publisher policies often contain common elements related to the scope of permitted AI use, authorship, recommendations for responsible AI use, and the duty to disclose AI use. Below is a partial, but not fully representative, survey of these elements across several notable publishing associations, publishers, and journals.
Adapted from: Lin, Zhicheng. “Towards an AI Policy Framework in Scholarly Publishing.” Trends in Cognitive Sciences , 2024, https://doi.org/10.1016/j.tics.2023.12.002.
Policies for Reviewers
Where publishers have policies for reviewers, they usually forbid any submission of the manuscript to a Generative AI tool.
A Changing Landscape
As the Generative AI landscape evolves, so will related policies. For example, over the course of 2023, Science released and then significantly revised its Generative AI policy, moving from something more restrictive to less. The full impact of Generative AI on scholarly publishing lies ahead.
- << Previous: Example - Content Evaluation (ChatGPT)
- Next: Faculty Resources >>
- Last Updated: May 16, 2024 10:19 AM
- URL: https://researchguides.dartmouth.edu/GenAI
Clarivate AI for Academia
Pushing the boundaries of research and learning with AI you can trust.
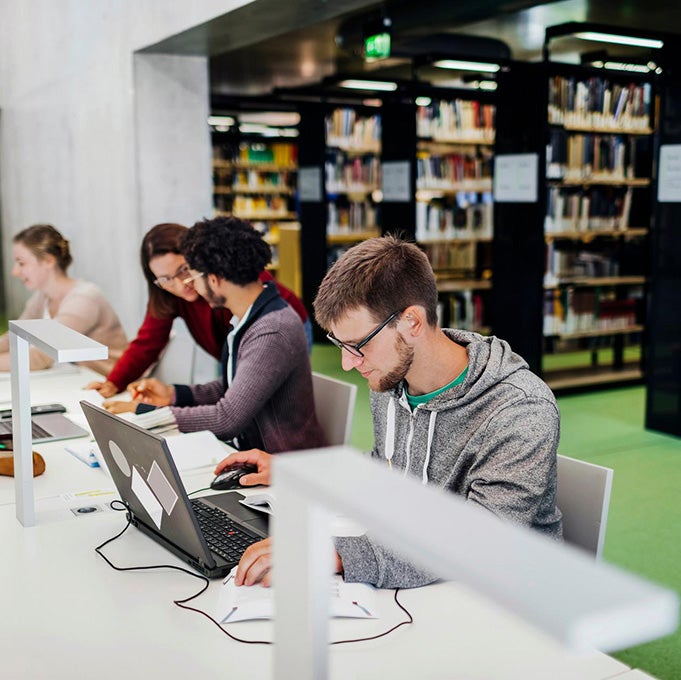
Artificial intelligence (AI) is transforming research, teaching and learning. Clarivate makes sure you can safely and responsibly navigate this new landscape, driving research excellence and student learning outcomes.
Trusted Academic AI
Clarivate AI-based solutions provide users with intelligence grounded in trustworthy sources and embedded in academic workflows, thus reducing the risks of misinformation, bias, and IP abuse.
- A wealth of expertly curated content
- Deep understanding of academic processes
- Rigorous testing and validation of results
- Close partnership with the academic community
- Strong governance, driven by academic principles
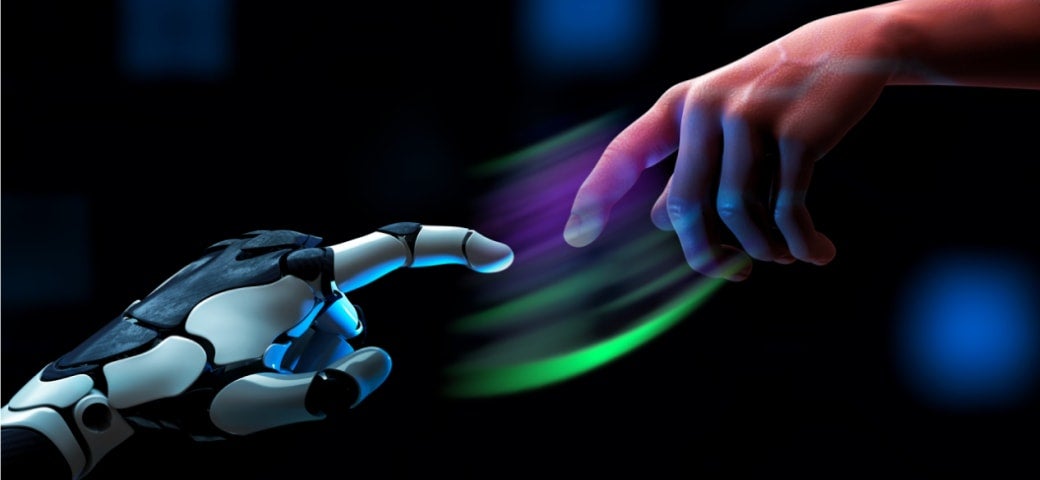
Think forward with Clarivate AI
A monthly newsletter that will keep you informed on our latest AI news and product developments
Web of Science™ Research Assistant
Discover a new, conversational way to understand topics, gain insight, locate must-read papers, and connect the dots between articles in the world’s most trusted citation index.
- Natural language search of documents, in multiple languages.
- Guided workflows and contextual data visualizations.
- Responses to scholarly questions and commentaries on relevant articles.
- Links to Web of Science articles and result sets for further exploration.
Watch the demo Learn more
ProQuest Research Assistant
A new way to navigate millions of full text academic works within ProQuest and easily find the high quality, trusted sources to accelerate your research and learning.
- Discover documents with natural language queries
- Get narrated responses to research questions
- Find trusted content, based on curated academic works
- Combines ChatGPT-like convenience with academic integrity
Watch highlights Learn more
Alethea Academic Coach
Nurture students learning skills and critical thinking with Alethea. The AI-based coach guides students to the core of their course readings, helping them distill takeaways and prepare for effective class discussion.
- Chat-based interactions, questions and prompts
- Combined proven learning principles and GenAI
- Insights to support students at risk of falling behind
- Built into your academic environment

In partnership with the community
Clarivate Academia AI Advisory Council is being formed to ensure that generative AI is developed in collaboration with the academic community. The council will help foster responsible design and application of GenAI for academic settings, including best practices, recommendations and guardrails.
arrow_forward Contact us
Committed to responsible application of AI
At Clarivate, we’ve been using AI and machine learning for years, guided by our AI principles .
- Deliver trusted content and data, based on authoritative academic sources
- Ensure proper attribution and easy access to cited works
- Collaborate with publishers, ensuring clear usage rights
- Do not use licensed content to train public LLM and AI
- Adhere to evolving global regulations

Frequently Asked Questions
Are you training public large language models (llm).
We do not train public LLMs. We use commercially pre-trained Large Language Models as part of our information retrieval and augmentation framework. Currently, this includes the use of a Retrieval Augmented Generation (RAG) architecture among other advanced techniques. While we are using the pre-trained LLMs to support the creation of narrative content, the facts in this content are generated from our trusted academic sources. We test this setup rigorously to ensure academic integrity and alignment with the academic ecosystem. Testing includes validation of responses through academic subject matter experts who evaluate the outputs for accuracy and relevance. Additionally, we conduct extensive user testing that involve real-world research and learning scenarios to further refine accuracy and performance.
We are committed to the highest standards of user privacy and security. We do not share or pass any publisher content, library-owned materials, or user data to large language models (LLMs) for any purpose.
What sources are used to generate content?
How are you ensuring against 'ai content hallucinations' and bad information.
We strongly believe that we have a critical responsibility to the academic community to mitigate AI-induced inaccuracies. We continuously test our solutions and the results they produce, including through dedicated beta programs and close collaboration with customers and subject matter experts. Our data science expertise helps ensure system accuracy, fairness, robustness and interpretability. Pairing this with our trustworthy, curated content, we significantly reduce the risk of ‘hallucinations’ and misinformation.
What transparency do you offer regarding your AI-based responses?
How is customer data protected and kept secured, how do your ai-based discovery tools rank and prioritize the sources listed.
The ranking and prioritization of sources by our AI-based discovery tools will vary according to the specific characteristics of the user’s query, the user persona, and the context of each query.
The approach to ranking and prioritization is similar to the way it is traditionally done in our discovery solutions. This understanding enables us to present the most relevant and valuable sources first, ensuring that the information provided matches the user’s needs as closely as possible.
Speak to our team
Learn how Clarivate can help you advance innovation.
Your Writing Assistant for Research
Unlock Your Research Potential with Jenni AI
Are you an academic researcher seeking assistance in your quest to create remarkable research and scientific papers? Jenni AI is here to empower you, not by doing the work for you, but by enhancing your research process and efficiency. Explore how Jenni AI can elevate your academic writing experience and accelerate your journey toward academic excellence.

Loved by over 1 million academics

Academia's Trusted Companion
Join our academic community and elevate your research journey alongside fellow scholars with Jenni AI.

Effortlessly Ignite Your Research Ideas
Unlock your potential with these standout features
Boost Productivity
Save time and effort with AI assistance, allowing you to focus on critical aspects of your research. Craft well-structured, scholarly papers with ease, backed by AI-driven recommendations and real-time feedback.
Get started

Overcome Writer's Block
Get inspiration and generate ideas to break through the barriers of writer's block. Jenni AI generates research prompts tailored to your subject, sparking your creativity and guiding your research.
Unlock Your Full Writing Potential
Jenni AI is designed to boost your academic writing capabilities, not as a shortcut, but as a tool to help you overcome writer's block and enhance your research papers' quality.

Ensure Accuracy
Properly format citations and references, ensuring your work meets academic standards. Jenni AI offers accurate and hassle-free citation assistance, including APA, MLA, and Chicago styles.
Our Commitment: Academic Honesty
Jenni AI is committed to upholding academic integrity. Our tool is designed to assist, not replace, your effort in research and writing. We strongly discourage any unethical use. We're dedicated to helping you excel in a responsible and ethical manner.
How it Works
Sign up for free.
To get started, sign up for a free account on Jenni AI's platform.
Prompt Generation
Input your research topic, and Jenni AI generates comprehensive prompts to kickstart your paper.
Research Assistance
Find credible sources, articles, and relevant data with ease through our powerful AI-driven research assistant.
Writing Support
Draft and refine your paper with real-time suggestions for structure, content, and clarity.
Citation & References
Let Jenni AI handle your citations and references in multiple styles, saving you valuable time.
What Our Users Say
Discover how Jenni AI has made a difference in the lives of academics just like you

· Aug 26
I thought AI writing was useless. Then I found Jenni AI, the AI-powered assistant for academic writing. It turned out to be much more advanced than I ever could have imagined. Jenni AI = ChatGPT x 10.

Charlie Cuddy
@sonofgorkhali
· 23 Aug
Love this use of AI to assist with, not replace, writing! Keep crushing it @Davidjpark96 💪

Waqar Younas, PhD
@waqaryofficial
· 6 Apr
4/9 Jenni AI's Outline Builder is a game-changer for organizing your thoughts and structuring your content. Create detailed outlines effortlessly, ensuring your writing is clear and coherent. #OutlineBuilder #WritingTools #JenniAI

I started with Jenni-who & Jenni-what. But now I can't write without Jenni. I love Jenni AI and am amazed to see how far Jenni has come. Kudos to http://Jenni.AI team.

· 28 Jul
Jenni is perfect for writing research docs, SOPs, study projects presentations 👌🏽

Stéphane Prud'homme
http://jenni.ai is awesome and super useful! thanks to @Davidjpark96 and @whoisjenniai fyi @Phd_jeu @DoctoralStories @WriteThatPhD
Frequently asked questions
How much does jenni ai cost, how can jenni ai assist me in writing complex academic papers, can jenni ai handle different types of academic papers, such as essays, research papers, and dissertationss jenni ai maintain the originality of my work, how does artificial intelligence enhance my academic writing with jenni ai.
Can Jenni AI help me structure and write a comprehensive literature review?
Will using Jenni AI improve my overall writing skills?
Can Jenni AI assist with crafting a thesis statement?
What sets Jenni AI apart as an AI-powered writing tool?
Can I trust Jenni AI to help me maintain academic integrity in my work?
Choosing the Right Academic Writing Companion
Get ready to make an informed decision and uncover the key reasons why Jenni AI is your ultimate tool for academic excellence.
Feature Featire
COMPETITORS
Enhanced Writing Style
Jenni AI excels in refining your writing style and enhancing sentence structure to meet academic standards with precision.
Competitors may offer basic grammar checking but often fall short in fine-tuning the nuances of writing style.
Academic Writing Process
Jenni AI streamlines the academic writing process, offering real-time assistance in content generation and thorough proofreading.
Competitors may not provide the same level of support, leaving users to navigate the intricacies of academic writing on their own.
Scientific Writing
Jenni AI is tailored for scientific writing, ensuring the clarity and precision needed in research articles and reports.
Competitors may offer generic writing tools that lack the specialized features required for scientific writing.
Original Content and Academic Integrity
Jenni AI's AI algorithms focus on producing original content while preventing plagiarism, ensuring academic integrity.
Competitors may not provide robust plagiarism checks, potentially compromising academic integrity.
Valuable Tool for Technical Writing
Jenni AI extends its versatility to technical writing, aiding in the creation of clear and concise technical documents.
Some competitors may not be as well-suited for technical writing projects.
User-Friendly Interface
Jenni AI offers an intuitive and user-friendly interface, making it easy for both novice and experienced writers to utilize its features effectively.
Some competitors may have steeper learning curves or complex interfaces, which can be time-consuming and frustrating for users.
Seamless Citation Management
Jenni AI simplifies the citation management process, offering suggestions and templates for various citation styles.
Competitors may not provide the same level of support for correct and consistent citations.
Ready to Revolutionize Your Research Writing?
Sign up for a free Jenni AI account today. Unlock your research potential and experience the difference for yourself. Your journey to academic excellence starts here.
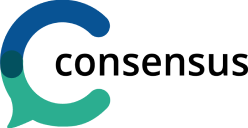
- Universities & students
- How to search
- How it works
- Start a new search
- Blog & updates
AI Search Engine for Research
Consensus is a search engine that uses AI to find insights in research papers
& start searching now!
Why Consensus?
Consensus responsibly uses AI to help you conduct effective research
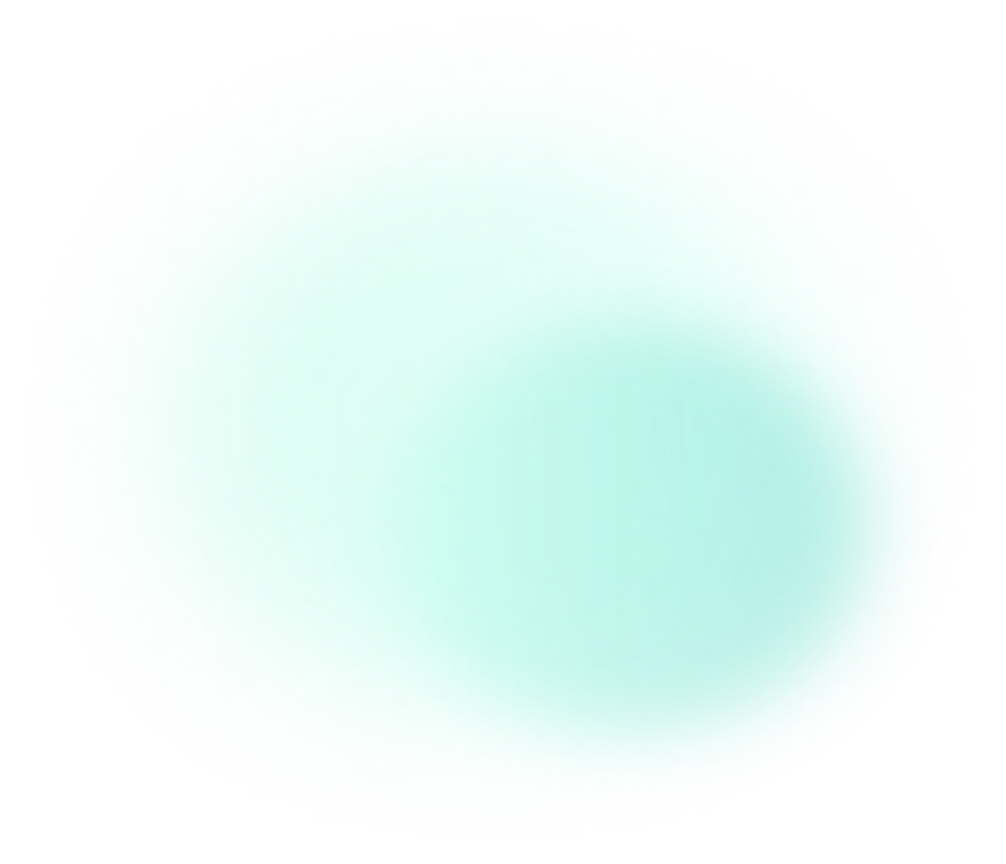
Search through over 200 million scientific papers without having to keyword match
All of our results are tied to actual studies, we cite our sources and we will never show you ads
Proprietary and purpose-built features that leverage GPT4 and other LLMs to summarize results for you
Used by researchers at the world’s top institutions
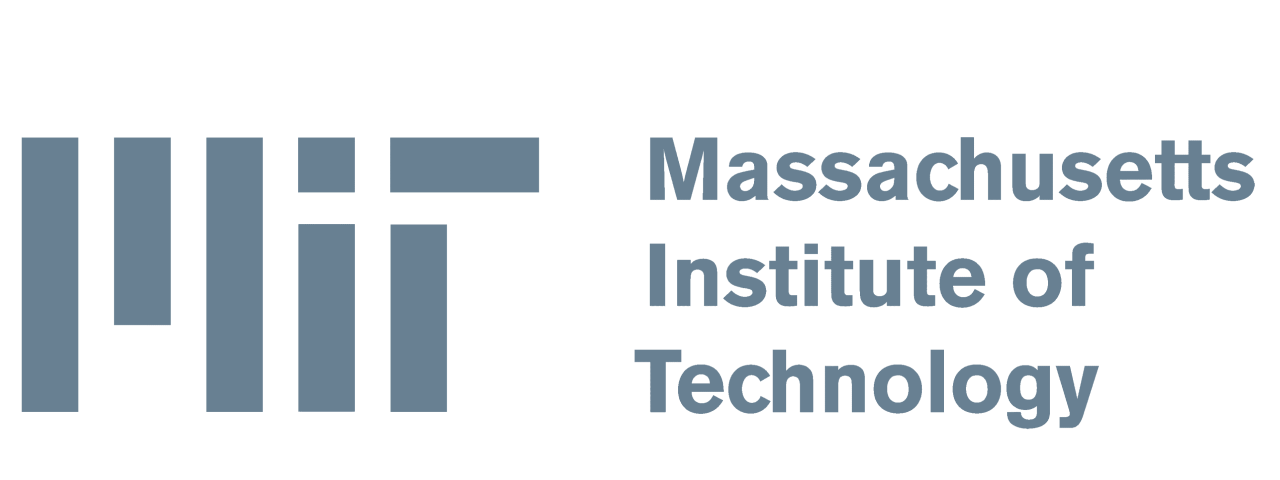
researchers, students, doctors, professionals and evidence-conscious consumers choose Consensus
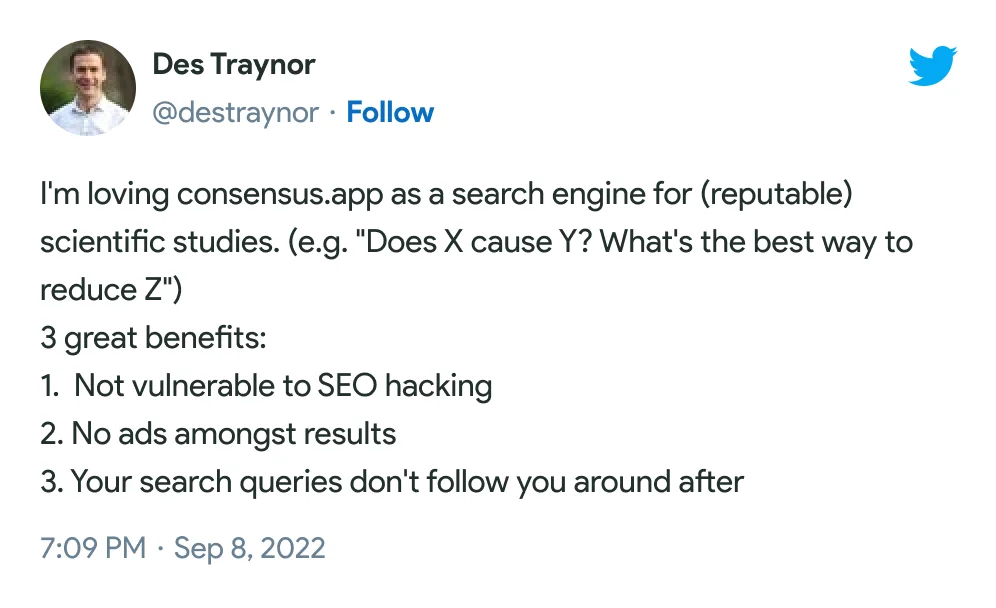
Consensus has been Featured in
Consensus helps.
Find supporting evidence for your paper
Researchers
Efficiently conduct literature reviews
Quickly find answers to patients’ questions
Instantly find expert quotes for presentations
Content Creators
Source peer-reviewed insights for your blog
Health and fitness enthusiasts
Check the viability of supplements and routines
Consensus vs ChatGPT
ChatGPT is built to have a conversation with you. Consensus is purpose-built to help you conduct effective research.
Results pulled directly from peer-reviewed studies
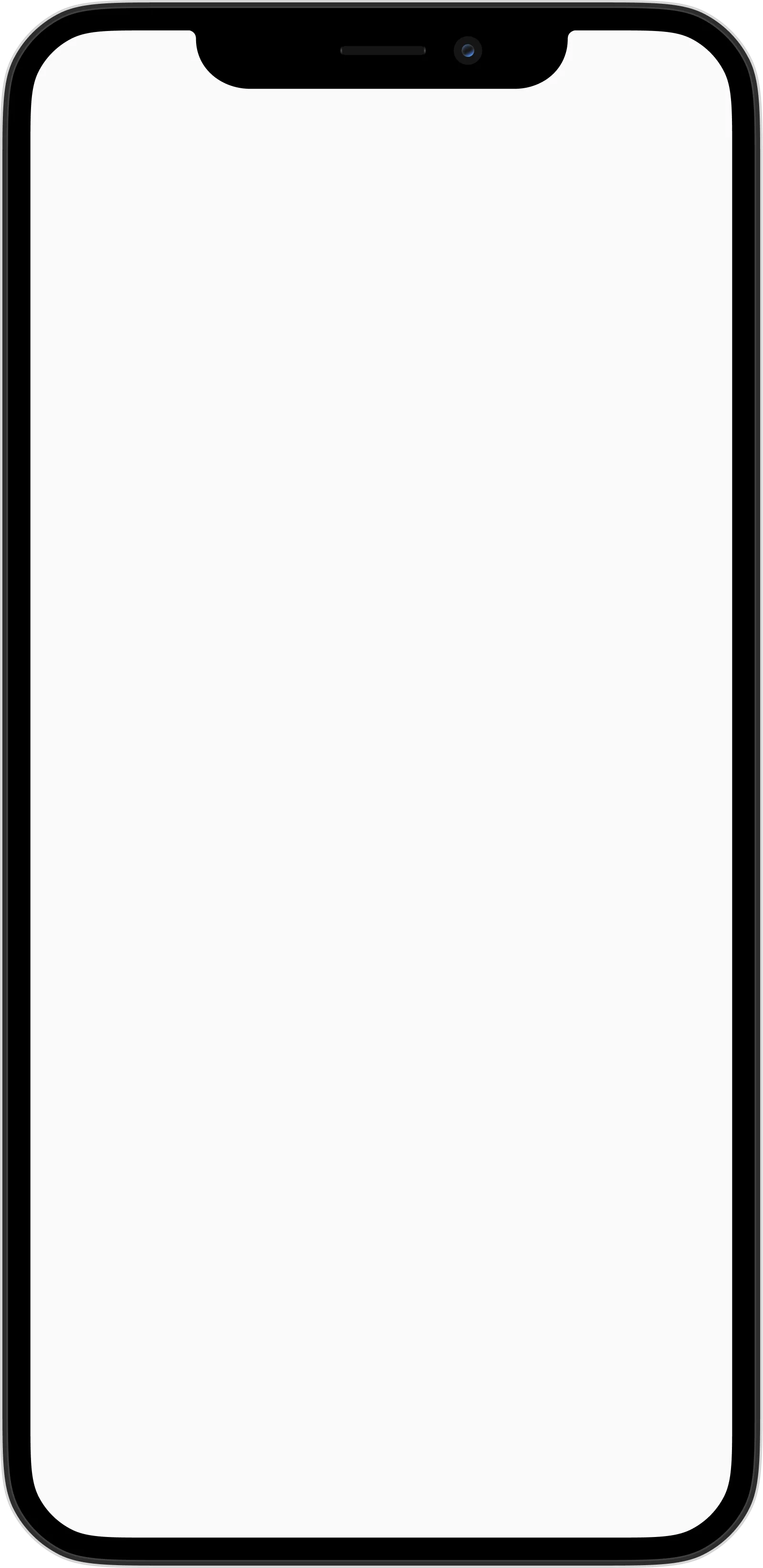
Fully machine-generated, trained on the entire internet
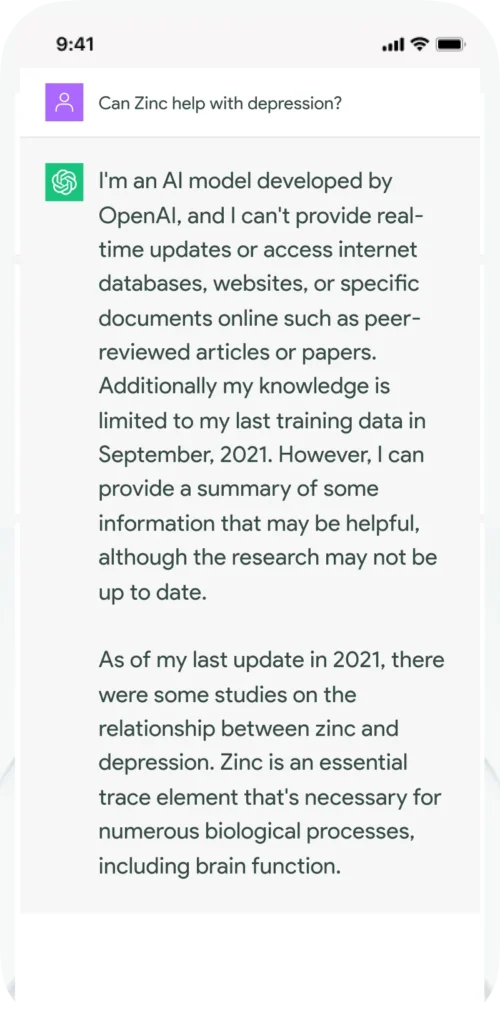
Our mission is to democratize expert knowledge
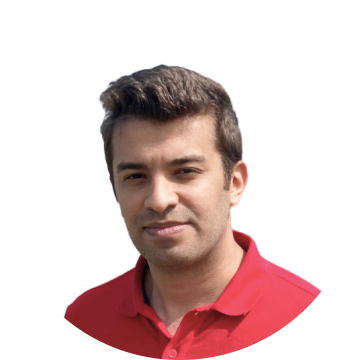
Sign up for our free BETA
Welcome to the MIT CISR website!
This site uses cookies. Review our Privacy Statement.
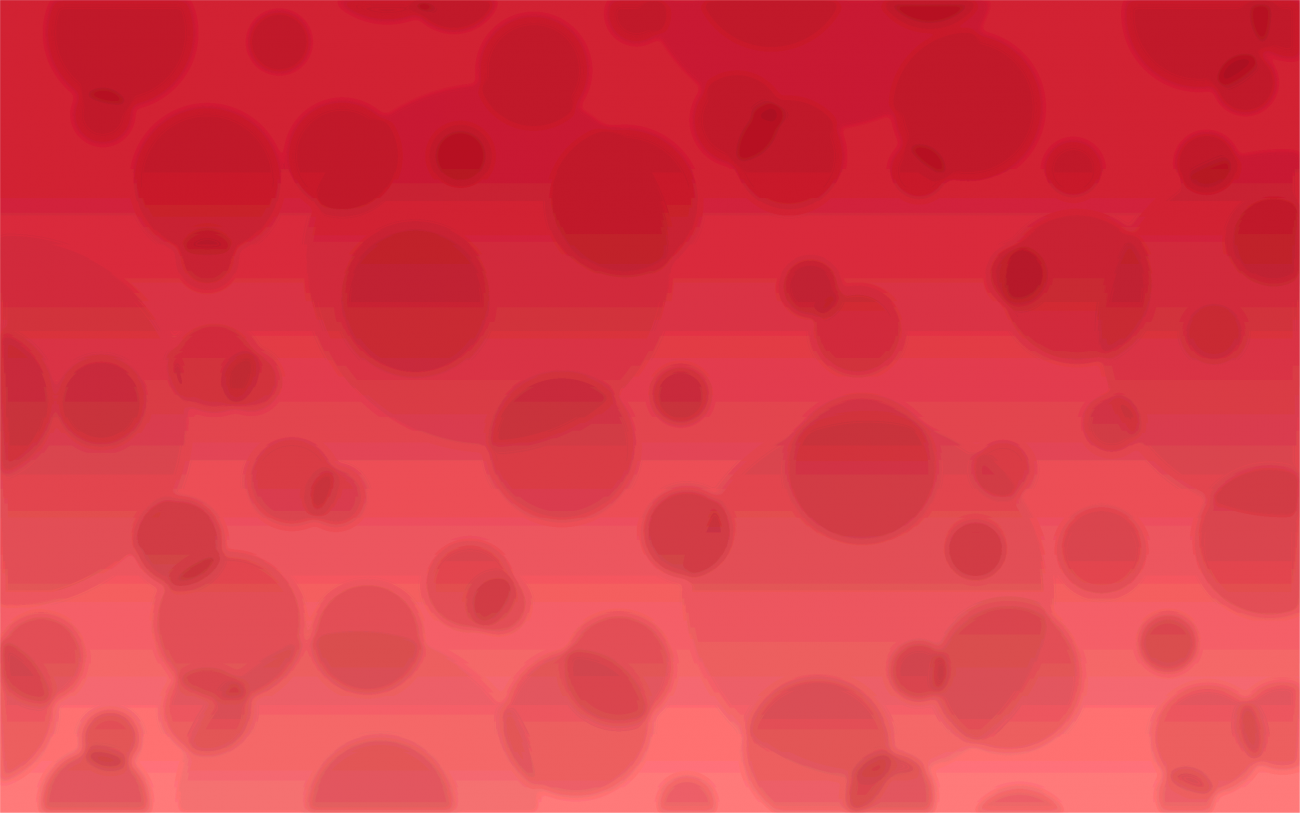
AI Is Everybody’s Business
This briefing presents three principles to guide business leaders when making AI investments: invest in practices that build capabilities required for AI, involve all your people in your AI journey, and focus on realizing value from your AI projects. The principles are supported by the MIT CISR data monetization research, and the briefing illustrates them using examples from the Australia Taxation Office and CarMax. The three principles apply to any kind of AI, defined as technology that performs human-like cognitive tasks; subsequent briefings will present management advice distinct to machine learning and generative tools, respectively.
Access More Research!
Any visitor to the website can read many MIT CISR Research Briefings in the webpage. But site users who have signed up on the site and are logged in can download all available briefings, plus get access to additional content. Even more content is available to members of MIT CISR member organizations .
Author Barb Wixom reads this research briefing as part of our audio edition of the series. Follow the series on SoundCloud.
DOWNLOAD THE TRANSCRIPT
Today, everybody across the organization is hungry to know more about AI. What is it good for? Should I trust it? Will it take my job? Business leaders are investing in massive training programs, partnering with promising vendors and consultants, and collaborating with peers to identify ways to benefit from AI and avoid the risk of AI missteps. They are trying to understand how to manage AI responsibly and at scale.
Our book Data Is Everybody’s Business: The Fundamentals of Data Monetization describes how organizations make money using their data.[foot]Barbara H. Wixom, Cynthia M. Beath, and Leslie Owens, Data Is Everybody's Business: The Fundamentals of Data Monetization , (Cambridge: The MIT Press, 2023), https://mitpress.mit.edu/9780262048217/data-is-everybodys-business/ .[/foot] We wrote the book to clarify what data monetization is (the conversion of data into financial returns) and how to do it (by using data to improve work, wrap products and experiences, and sell informational solutions). AI technology’s role in this is to help data monetization project teams use data in ways that humans cannot, usually because of big complexity or scope or required speed. In our data monetization research, we have regularly seen leaders use AI effectively to realize extraordinary business goals. In this briefing, we explain how such leaders achieve big AI wins and maximize financial returns.
Using AI in Data Monetization
AI refers to the ability of machines to perform human-like cognitive tasks.[foot]See Hind Benbya, Thomas H. Davenport, and Stella Pachidi, “Special Issue Editorial: Artificial Intelligence in Organizations: Current State and Future Opportunities , ” MIS Quarterly Executive 19, no. 4 (December 2020), https://aisel.aisnet.org/misqe/vol19/iss4/4 .[/foot] Since 2019, MIT CISR researchers have been studying deployed data monetization initiatives that rely on machine learning and predictive algorithms, commonly referred to as predictive AI.[foot]This research draws on a Q1 to Q2 2019 asynchronous discussion about AI-related challenges with fifty-three data executives from the MIT CISR Data Research Advisory Board; more than one hundred structured interviews with AI professionals regarding fifty-two AI projects from Q3 2019 to Q2 2020; and ten AI project narratives published by MIT CISR between 2020 and 2023.[/foot] Such initiatives use large data repositories to recognize patterns across time, draw inferences, and predict outcomes and future trends. For example, the Australian Taxation Office (ATO) used machine learning, neural nets, and decision trees to understand citizen tax-filing behaviors and produce respectful nudges that helped citizens abide by Australia’s work-related expense policies. In 2018, the nudging resulted in AUD$113 million in changed claim amounts.[foot]I. A. Someh, B. H. Wixom, and R. W. Gregory, “The Australian Taxation Office: Creating Value with Advanced Analytics,” MIT CISR Working Paper No. 447, November 2020, https://cisr.mit.edu/publication/MIT_CISRwp447_ATOAdvancedAnalytics_SomehWixomGregory .[/foot]
In 2023, we began exploring data monetization initiatives that rely on generative AI.[foot]This research draws on two asynchronous generative AI discussions (Q3 2023, N=35; Q1 2024, N=34) regarding investments and capabilities and roles and skills, respectively, with data executives from the MIT CISR Data Research Advisory Board. It also draws on in-progress case studies with large organizations in the publishing, building materials, and equipment manufacturing industries.[/foot] This type of AI analyzes vast amounts of text or image data to discern patterns in them. Using these patterns, generative AI can create new text, software code, images, or videos, usually in response to user prompts. Organizations are now beginning to openly discuss data monetization initiative deployments that include generative AI technologies. For example, used vehicle retailer CarMax reported using OpenAI’s ChatGPT chatbot to help aggregate customer reviews and other car information from multiple data sets to create helpful, easy-to-read summaries about individual used cars for its online shoppers. At any point in time, CarMax has on average 50,000 cars on its website, so to produce such content without AI the company would require hundreds of content writers and years of time; using ChatGPT, the company’s content team can generate summaries in hours.[foot]Paula Rooney, “CarMax drives business value with GPT-3.5,” CIO , May 5, 2023, https://www.cio.com/article/475487/carmax-drives-business-value-with-gpt-3-5.html ; Hayete Gallot and Shamim Mohammad, “Taking the car-buying experience to the max with AI,” January 2, 2024, in Pivotal with Hayete Gallot, produced by Larj Media, podcast, MP3 audio, https://podcasts.apple.com/us/podcast/taking-the-car-buying-experience-to-the-max-with-ai/id1667013760?i=1000640365455 .[/foot]
Big advancements in machine learning, generative tools, and other AI technologies inspire big investments when leaders believe the technologies can help satisfy pent-up demand for solutions that previously seemed out of reach. However, there is a lot to learn about novel technologies before we can properly manage them. In this year’s MIT CISR research, we are studying predictive and generative AI from several angles. This briefing is the first in a series; in future briefings we will present management advice specific to machine learning and generative tools. For now, we present three principles supported by our data monetization research to guide business leaders when making AI investments of any kind: invest in practices that build capabilities required for AI, involve all your people in your AI journey, and focus on realizing value from your AI projects.
Principle 1: Invest in Practices That Build Capabilities Required for AI
Succeeding with AI depends on having deep data science skills that help teams successfully build and validate effective models. In fact, organizations need deep data science skills even when the models they are using are embedded in tools and partner solutions, including to evaluate their risks; only then can their teams make informed decisions about how to incorporate AI effectively into work practices. We worry that some leaders view buying AI products from providers as an opportunity to use AI without deep data science skills; we do not advise this.
But deep data science skills are not enough. Leaders often hire new talent and offer AI literacy training without making adequate investments in building complementary skills that are just as important. Our research shows that an organization’s progress in AI is dependent on having not only an advanced data science capability, but on having equally advanced capabilities in data management, data platform, acceptable data use, and customer understanding.[foot]In the June 2022 MIT CISR research briefing, we described why and how organizations build the five advanced data monetization capabilities for AI. See B. H. Wixom, I. A. Someh, and C. M. Beath, “Building Advanced Data Monetization Capabilities for the AI-Powered Organization,” MIT CISR Research Briefing, Vol. XXII, No. 6, June 2022, https://cisr.mit.edu/publication/2022_0601_AdvancedAICapabilities_WixomSomehBeath .[/foot] Think about it. Without the ability to curate data (an advanced data management capability), teams cannot effectively incorporate a diverse set of features into their models. Without the ability to oversee the legality and ethics of partners’ data use (an advanced acceptable data use capability), teams cannot responsibly deploy AI solutions into production.
It’s no surprise that ATO’s AI journey evolved in conjunction with the organization’s Smarter Data Program, which ATO established to build world-class data analytics capabilities, and that CarMax emphasizes that its governance, talent, and other data investments have been core to its generative AI progress.
Capabilities come mainly from learning by doing, so they are shaped by new practices in the form of training programs, policies, processes, or tools. As organizations undertake more and more sophisticated practices, their capabilities get more robust. Do invest in AI training—but also invest in practices that will boost the organization’s ability to manage data (such as adopting a data cataloging tool), make data accessible cost effectively (such as adopting cloud policies), improve data governance (such as establishing an ethical oversight committee), and solidify your customer understanding (such as mapping customer journeys). In particular, adopt policies and processes that will improve your data governance, so that data is only used in AI initiatives in ways that are consonant with your organization's values and its regulatory environment.
Principle 2: Involve All Your People in Your AI Journey
Data monetization initiatives require a variety of stakeholders—people doing the work, developing products, and offering solutions—to inform project requirements and to ensure the adoption and confident use of new data tools and behaviors.[foot]Ida Someh, Barbara Wixom, Michael Davern, and Graeme Shanks, “Configuring Relationships between Analytics and Business Domain Groups for Knowledge Integration, ” Journal of the Association for Information Systems 24, no. 2 (2023): 592-618, https://cisr.mit.edu/publication/configuring-relationships-between-analytics-and-business-domain-groups-knowledge .[/foot] With AI, involving a variety of stakeholders in initiatives helps non-data scientists become knowledgeable about what AI can and cannot do, how long it takes to deliver certain kinds of functionality, and what AI solutions cost. This, in turn, helps organizations in building trustworthy models, an important AI capability we call AI explanation (AIX).[foot]Ida Someh, Barbara H. Wixom, Cynthia M. Beath, and Angela Zutavern, “Building an Artificial Intelligence Explanation Capability,” MIS Quarterly Executive 21, no. 2 (2022), https://cisr.mit.edu/publication/building-artificial-intelligence-explanation-capability .[/foot]
For example, at ATO, data scientists educated business colleagues on the mechanics and results of models they created. Business colleagues provided feedback on the logic used in the models and helped to fine-tune them, and this interaction helped everyone understand how the AI made decisions. The data scientists provided their model results to ATO auditors, who also served as a feedback loop to the data scientists for improving the model. The data scientists regularly reported on initiative progress to senior management, regulators, and other stakeholders, which ensured that the AI team was proactively creating positive benefits without neglecting negative external factors that might surface.
Given the consumerization of generative AI tools, we believe that pervasive worker involvement in ideating, building, refining, using, and testing AI models and tools will become even more crucial to deploying fruitful AI projects—and building trust that AI will do the right thing in the right way at the right time.
Principle 3: Focus on Realizing Value From Your AI Projects
AI is costly—just add up your organization’s expenses in tools, talent, and training. AI needs to pay off, yet some organizations become distracted with endless experimentation. Others get caught up in finding the sweet spot of the technology, ignoring the sweet spot of their business model. For example, it is easy to become enamored of using generative AI to improve worker productivity, rolling out tools for employees to write better emails and capture what happened in meetings. But unless those activities materially impact how your organization makes money, there likely are better ways to spend your time and money.
Leaders with data monetization experience will make sure their AI projects realize value in the form of increased revenues or reduced expenses by backing initiatives that are clearly aligned with real challenges and opportunities. That is step one. In our research, the leaders that realize value from their data monetization initiatives measure and track their outcomes, especially their financial outcomes, and they hold someone accountable for achieving the desired financial returns. At CarMax, a cross-functional team owned the mission to provide better website information for used car shoppers, a mission important to the company’s sales goals. Starting with sales goals in mind, the team experimented with and then chose a generative AI solution that would enhance the shopper experience and increase sales.
Figure 1: Three Principles for Getting Value from AI Investments
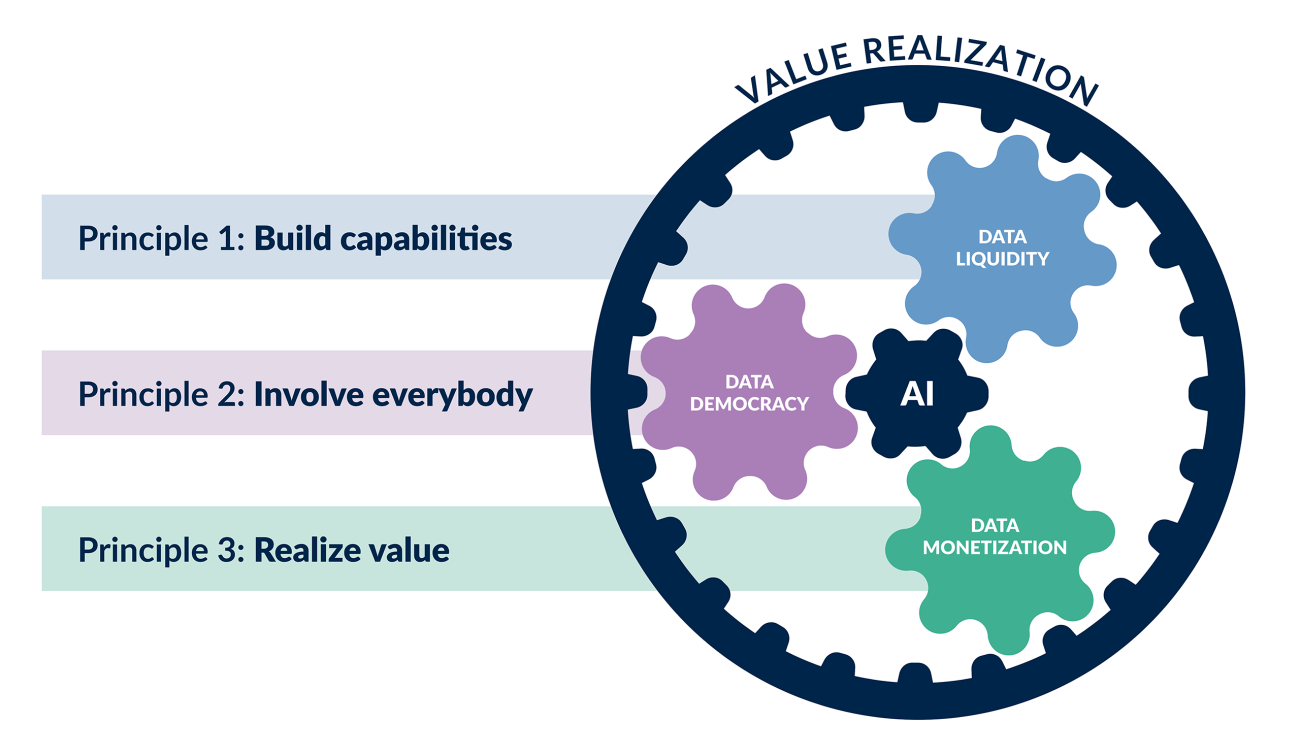
The three principles are based on the following concepts from MIT CISR data research: 1. Data liquidity: the ease of data asset recombination and reuse 2. Data democracy: an organization that empowers employees in the access and use of data 3. Data monetization: the generation of financial returns from data assets
Managing AI Using a Data Monetization Mindset
AI has and always will play a big role in data monetization. It’s not a matter of whether to incorporate AI, but a matter of how to best use it. To figure this out, quantify the outcomes of some of your organization’s recent AI projects. How much money has the organization realized from them? If the answer disappoints, then make sure the AI technology value proposition is a fit for your organization’s most important goals. Then assign accountability for ensuring that AI technology is applied in use cases that impact your income statements. If the AI technology is not a fit for your organization, then don’t be distracted by media reports of the AI du jour.
Understanding your AI technology investments can be hard if your organization is using AI tools that are bundled in software you purchase or are built for you by a consultant. To set yourself up for success, ask your partners to be transparent with you about the quality of data they used to train their AI models and the data practices they relied on. Do their answers persuade you that their tools are trustworthy? Is it obvious that your partner is using data compliantly and is safeguarding the model from producing bad or undesired outcomes? If so, make sure this good news is shared with the people in your organization and those your organization serves. If not, rethink whether to break with your partner and find another way to incorporate the AI technology into your organization, such as by hiring people to build it in-house.
To paraphrase our book’s conclusion: When people actively engage in data monetization initiatives using AI , they learn, and they help their organization learn. Their engagement creates momentum that initiates a virtuous cycle in which people’s engagement leads to better data and more bottom-line value, which in turn leads to new ideas and more engagement, which further improves data and delivers more value, and so on. Imagine this happening across your organization as all people everywhere make it their business to find ways to use AI to monetize data.
This is why AI, like data, is everybody’s business.
© 2024 MIT Center for Information Systems Research, Wixom and Beath. MIT CISR Research Briefings are published monthly to update the center’s member organizations on current research projects.
Related Publications
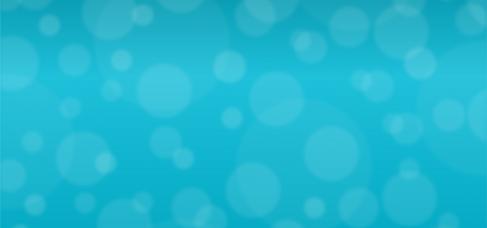
Talking Points
Ai, like data, is everybody's business.
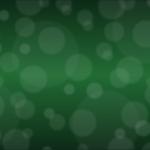
Working Paper: Vignette
The australian taxation office: creating value with advanced analytics.
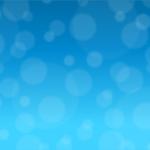
Research Briefing
Building advanced data monetization capabilities for the ai-powered organization.
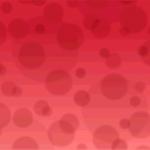
Building AI Explanation Capability for the AI-Powered Organization
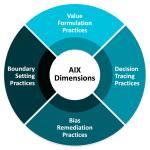
What is Data Monetization?
About the researchers.
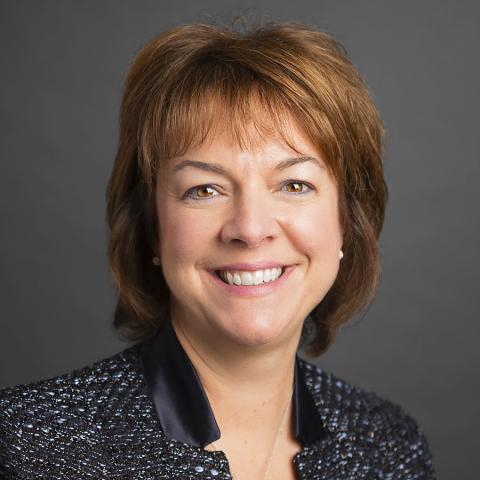
Barbara H. Wixom, Principal Research Scientist, MIT Center for Information Systems Research (CISR)
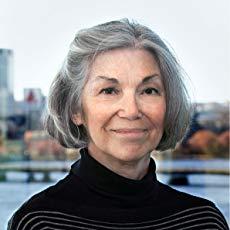
Cynthia M. Beath, Professor Emerita, University of Texas and Academic Research Fellow, MIT CISR
Mit center for information systems research (cisr).
Founded in 1974 and grounded in MIT's tradition of combining academic knowledge and practical purpose, MIT CISR helps executives meet the challenge of leading increasingly digital and data-driven organizations. We work directly with digital leaders, executives, and boards to develop our insights. Our consortium forms a global community that comprises more than seventy-five organizations.
MIT CISR Associate Members
MIT CISR wishes to thank all of our associate members for their support and contributions.
MIT CISR's Mission Expand
MIT CISR helps executives meet the challenge of leading increasingly digital and data-driven organizations. We provide insights on how organizations effectively realize value from approaches such as digital business transformation, data monetization, business ecosystems, and the digital workplace. Founded in 1974 and grounded in MIT’s tradition of combining academic knowledge and practical purpose, we work directly with digital leaders, executives, and boards to develop our insights. Our consortium forms a global community that comprises more than seventy-five organizations.
Microsoft Research Blog
Microsoft at chi 2024: innovations in human-centered design.
Published May 15, 2024
Share this page
- Share on Facebook
- Share on Twitter
- Share on LinkedIn
- Share on Reddit
- Subscribe to our RSS feed

The ways people engage with technology, through its design and functionality, determine its utility and acceptance in everyday use, setting the stage for widespread adoption. When computing tools and services respect the diversity of people’s experiences and abilities, technology is not only functional but also universally accessible. Human-computer interaction (HCI) plays a crucial role in this process, examining how technology integrates into our daily lives and exploring ways digital tools can be shaped to meet individual needs and enhance our interactions with the world.
The ACM CHI Conference on Human Factors in Computing Systems is a premier forum that brings together researchers and experts in the field, and Microsoft is honored to support CHI 2024 as a returning sponsor. We’re pleased to announce that 33 papers by Microsoft researchers and their collaborators have been accepted this year, with four winning the Best Paper Award and seven receiving honorable mentions.
This research aims to redefine how people work, collaborate, and play using technology, with a focus on design innovation to create more personalized, engaging, and effective interactions. Several projects emphasize customizing the user experience to better meet individual needs, such as exploring the potential of large language models (LLMs) to help reduce procrastination. Others investigate ways to boost realism in virtual and mixed reality environments, using touch to create a more immersive experience. There are also studies that address the challenges of understanding how people interact with technology. These include applying psychology and cognitive science to examine the use of generative AI and social media, with the goal of using the insights to guide future research and design directions. This post highlights these projects.
Microsoft Research Podcast

AI Frontiers: AI for health and the future of research with Peter Lee
Peter Lee, head of Microsoft Research, and Ashley Llorens, AI scientist and engineer, discuss the future of AI research and the potential for GPT-4 as a medical copilot.
Best Paper Award recipients
DynaVis: Dynamically Synthesized UI Widgets for Visualization Editing Priyan Vaithilingam, Elena L. Glassman, Jeevana Priya Inala , Chenglong Wang GUIs used for editing visualizations can overwhelm users or limit their interactions. To address this, the authors introduce DynaVis, which combines natural language interfaces with dynamically synthesized UI widgets, enabling people to initiate and refine edits using natural language.
Generative Echo Chamber? Effects of LLM-Powered Search Systems on Diverse Information Seeking Nikhil Sharma, Q. Vera Liao , Ziang Xiao Conversational search systems powered by LLMs potentially improve on traditional search methods, yet their influence on increasing selective exposure and fostering echo chambers remains underexplored. This research suggests that LLM-driven conversational search may enhance biased information querying, particularly when the LLM’s outputs reinforce user views, emphasizing significant implications for the development and regulation of these technologies.
Piet: Facilitating Color Authoring for Motion Graphics Video Xinyu Shi, Yinghou Wang, Yun Wang , Jian Zhao Motion graphic (MG) videos use animated visuals and color to effectively communicate complex ideas, yet existing color authoring tools are lacking. This work introduces Piet, a tool prototype that offers an interactive palette and support for quick theme changes and controlled focus, significantly streamlining the color design process.
The Metacognitive Demands and Opportunities of Generative AI Lev Tankelevitch , Viktor Kewenig, Auste Simkute, Ava Elizabeth Scott, Advait Sarkar , Abigail Sellen , Sean Rintel Generative AI systems offer unprecedented opportunities for transforming professional and personal work, yet they present challenges around prompting, evaluating and relying on outputs, and optimizing workflows. This paper shows that metacognition—the psychological ability to monitor and control one’s thoughts and behavior—offers a valuable lens through which to understand and design for these usability challenges.
Honorable Mentions
B ig or Small, It’s All in Your Head: Visuo-Haptic Illusion of Size-Change Using Finger-Repositioning Myung Jin Kim, Eyal Ofek, Michel Pahud , Mike J. Sinclair, Andrea Bianchi This research introduces a fixed-sized VR controller that uses finger repositioning to create a visuo-haptic illusion of dynamic size changes in handheld virtual objects, allowing users to perceive virtual objects as significantly smaller or larger than the actual device.
LLMR: Real-time Prompting of Interactive Worlds Using Large Language Models Fernanda De La Torre, Cathy Mengying Fang, Han Huang, Andrzej Banburski-Fahey, Judith Amores , Jaron Lanier Large Language Model for Mixed Reality (LLMR) is a framework for the real-time creation and modification of interactive mixed reality experiences using LLMs. It uses novel strategies to tackle difficult cases where ideal training data is scarce or where the design goal requires the synthesis of internal dynamics, intuitive analysis, or advanced interactivity.
Observer Effect in Social Media Use Koustuv Saha, Pranshu Gupta, Gloria Mark, Emre Kiciman , Munmun De Choudhury This work investigates the observer effect in behavioral assessments on social media use. The observer effect is a phenomenon in which individuals alter their behavior due to awareness of being monitored. Conducted over an average of 82 months (about 7 years) retrospectively and five months prospectively using Facebook data, the study found that deviations in expected behavior and language post-enrollment in the study reflected individual psychological traits. The authors recommend ways to mitigate the observer effect in these scenarios.
Reading Between the Lines: Modeling User Behavior and Costs in AI-Assisted Programming Hussein Mozannar, Gagan Bansal , Adam Fourney , Eric Horvitz By investigating how developers use GitHub Copilot, the authors created CUPS, a taxonomy of programmer activities during system interaction. This approach not only elucidates interaction patterns and inefficiencies but can also drive more effective metrics and UI design for code-recommendation systems with the goal of improving programmer productivity.
SharedNeRF: Leveraging Photorealistic and View-dependent Rendering for Real-time and Remote Collaboration Mose Sakashita, Bala Kumaravel, Nicolai Marquardt , Andrew D. Wilson SharedNeRF, a system for synchronous remote collaboration, utilizes neural radiance field (NeRF) technology to provide photorealistic, viewpoint-specific renderings that are seamlessly integrated with point clouds to capture dynamic movements and changes in a shared space. A preliminary study demonstrated its effectiveness, as participants used this high-fidelity, multi-perspective visualization to successfully complete a flower arrangement task.
Understanding the Role of Large Language Models in Personalizing and Scaffolding Strategies to Combat Academic Procrastination Ananya Bhattacharjee, Yuchen Zeng, Sarah Yi Xu, Dana Kulzhabayeva, Minyi Ma, Rachel Kornfield, Syed Ishtiaque Ahmed, Alex Mariakakis, Mary P. Czerwinski , Anastasia Kuzminykh, Michael Liut, Joseph Jay Williams In this study, the authors explore the potential of LLMs for customizing academic procrastination interventions, employing a technology probe to generate personalized advice. Their findings emphasize the need for LLMs to offer structured, deadline-oriented advice and adaptive questioning techniques, providing key design insights for LLM-based tools while highlighting cautions against their use for therapeutic guidance.
Where Are We So Far? Understanding Data Storytelling Tools from the Perspective of Human-AI Collaboration Haotian Li, Yun Wang , Huamin Qu This paper evaluates data storytelling tools using a dual framework to analyze the stages of the storytelling workflow—analysis, planning, implementation, communication—and the roles of humans and AI in each stage, such as creators, assistants, optimizers, and reviewers. The study identifies common collaboration patterns in existing tools, summarizes lessons from these patterns, and highlights future research opportunities for human-AI collaboration in data storytelling.
Learn more about our work and contributions to CHI 2024, including our full list of publications , on our conference webpage .
Related publications
Llmr: real-time prompting of interactive worlds using large language models, reading between the lines: modeling user behavior and costs in ai-assisted programming, observer effect in social media use, where are we so far understanding data storytelling tools from the perspective of human-ai collaboration, the metacognitive demands and opportunities of generative ai, piet: facilitating color authoring for motion graphics video, dynavis: dynamically synthesized ui widgets for visualization editing, generative echo chamber effects of llm-powered search systems on diverse information seeking, understanding the role of large language models in personalizing and scaffolding strategies to combat academic procrastination, sharednerf: leveraging photorealistic and view-dependent rendering for real-time and remote collaboration, big or small, it’s all in your head: visuo-haptic illusion of size-change using finger-repositioning, continue reading.
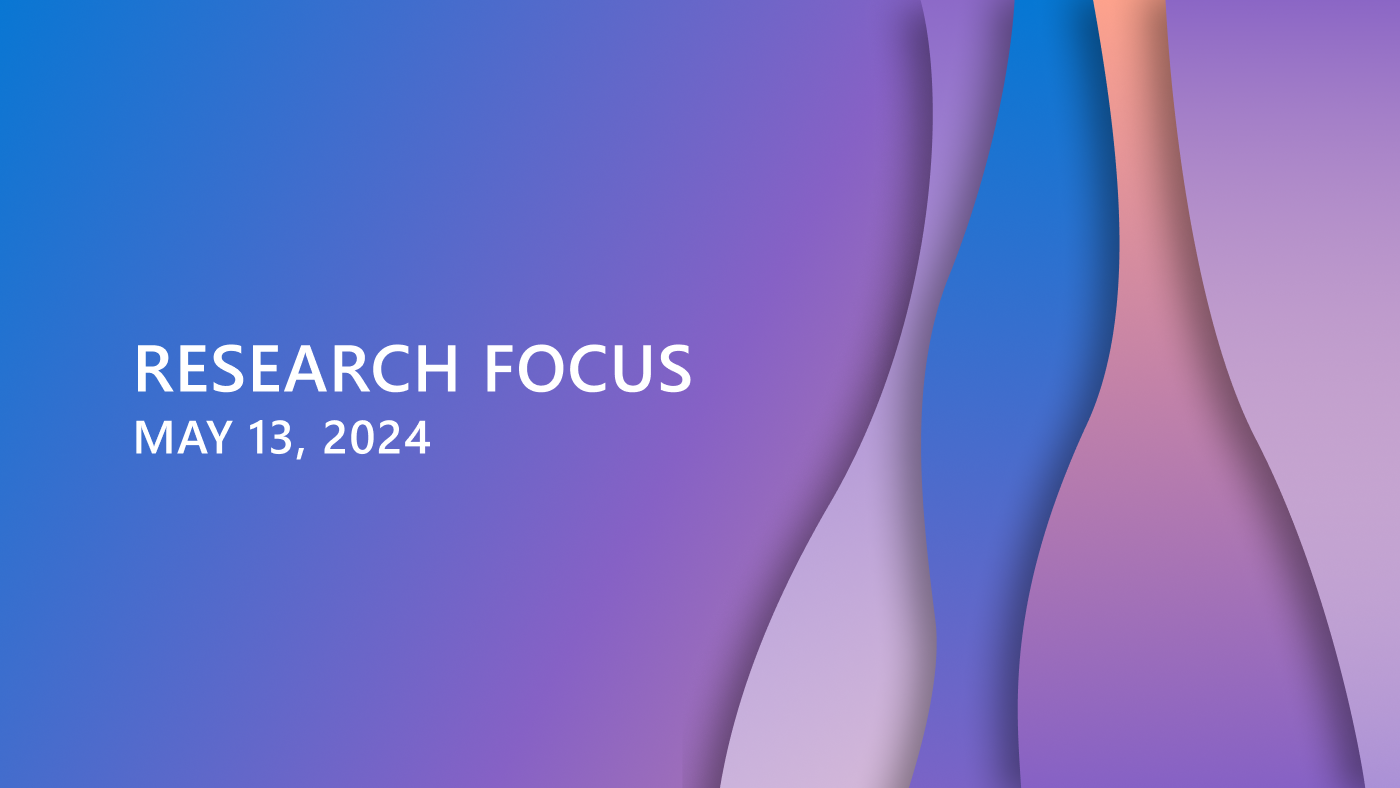
Research Focus: Week of May 13, 2024

Research Focus: Week of April 15, 2024

Research Focus: Week of March 18, 2024

Advancing human-centered AI: Updates on responsible AI research
Research areas.

Related events
- Microsoft at CHI 2024
Related labs
- Microsoft Research Lab - Asia
- Microsoft Research Lab - Cambridge
- Microsoft Research Lab - Redmond
- Microsoft Research Lab – Montréal
- AI Frontiers
- Follow on Twitter
- Like on Facebook
- Follow on LinkedIn
- Subscribe on Youtube
- Follow on Instagram
Share this page:
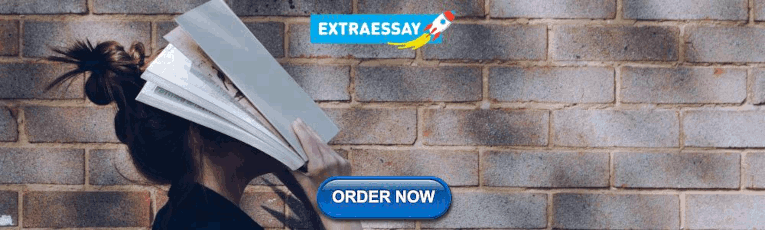
IMAGES
VIDEO
COMMENTS
AI-powered research tools and AI for academic research. AI research tools, like Concensus, offer immense benefits in scientific research. Here are the general AI-powered tools for academic research. These AI-powered tools can efficiently summarize PDFs, extract key information, and perform AI-powered searches, and much more.
Literature review. Artificial Intelligence (AI) has dramatically altered the landscape of academic research, acting as a catalyst for both methodological innovation and broader shifts in scholarly paradigms (Pal, Citation 2023).Its transformative power is evident across multiple disciplines, enabling researchers to engage with complex datasets and questions at a scale previously unimaginable ...
Results. The search identified 24 studies through which six core domains were identified where AI helps academic writing and research: 1) facilitating idea generation and research design, 2) improving content and structuring, 3) supporting literature review and synthesis, 4) enhancing data management and analysis, 5) supporting editing, review, and publishing, and 6) assisting in communication ...
Here are other AI-driven software to help your academic efforts, handpicked by Bilal. 1. Consensus. In Bilal's own words: "If ChatGPT and Google Scholar got married, their child would be ...
Artificial intelligence (AI) is being increasingly integrated into scientific discovery to augment and accelerate research, helping scientists to generate hypotheses, design experiments, collect ...
AI was implemented and examined in a wide variety of subject areas, such as science, medicine, arts, sports, engineering, mathematics, technologies, foreign language, business, history and more (See Table 3).The largest number of AIEd research studies (n = 14) were in engineering, computer science, information technology (IT), or informatics, followed by mathematics (n = 8), foreign language ...
The role of AI within research policy and practice is an interesting lens through which to investigate AI and society. Drawing on interviews with leading scholars, this paper reflects on the role of AI in the research process and its positive and negative implications. To do this, we reflect on the responses to the following questions; "what ...
Gemma Conroy, Hepeng Jia, Benjamin Plackett &. Andy Tay. As artificial intelligence (AI) becomes ubiquitous in fields such as medicine, education and security, there are significant ethical and ...
Academic publishers have a range of policies on the use of AI in research papers. In some cases, publishers may prohibit the use of AI for certain aspects of paper development. You should review the specific policies of the target publisher to determine what is permitted.
The integration of AI into academic writing streamlines the creative and writing process, increasing productivity and content. The research process can present challenges, particularly for ...
Semantic Reader is an augmented reader with the potential to revolutionize scientific reading by making it more accessible and richly contextual. Try it for select papers. Semantic Scholar uses groundbreaking AI and engineering to understand the semantics of scientific literature to help Scholars discover relevant research.
Artificial Intelligence (AI) is increasingly adopted by organizations to innovate, and this is ever more reflected in scholarly work. To illustrate, assess and map research at the intersection of AI and innovation, we performed a Systematic Literature Review (SLR) of published work indexed in the Clarivate Web of Science (WOS) and Elsevier Scopus databases (the final sample includes 1448 ...
This report offers perspectives and practical guidelines to the Cornell community, specifically on the use of Generative Artificial Intelligence (GenAI) in the practice and dissemination of academic research. As emphasized in the charge to a Cornell task force representing input across all campuses, the report aims to establish the initial set ...
The report, "Generative AI in Academic Research: Perspectives and Cultural Norms," was published Dec. 15 and highlights best practices in the current landscape, how university policies impact the Cornell community and considerations for other faculty members or researchers navigating the new tech tools. The background: The university ...
AI tools can be used to support different aspects of the research process, including: Hypotheses Generation: AI can automatically generate research questions based on a given dataset or topic that can serve as starting points for researchers to refine and develop into hypotheses. Literature Review: AI can accelerate the literature review process by analyzing and summarizing a body of ...
Artificial intelligence (AI) has developed into a powerful tool that Academics can exploit for their research, writing, and cooperation in recent years. AI can speed up the writing and research ...
1. Use AI to help you brainstorm. ChatGPT-4, OpenAI's latest and paid version of the large language model (LLM), plays a vital role in enhancing my daily research process; it has the capacity to write, create graphics, analyze data, and browse the internet, seemingly as a human would.Rather than using predefined prompts, I conduct generative AI research in a natural and conversational manner ...
The Choice of The Number 1 AI Tool for Academia: Best Solution. Postdoctoral researcher Mushtaq Bilal believes that ChatGPT will revolutionize academic research, but acknowledges that many academics don't know how to use it effectively. Academia is split between early AI adopters and those concerned about its impact on academic integrity.
Many people are worried by the use of AI in academic papers. Indeed, the practice has been described as "contaminating" scholarly literature. Some argue that using AI output amounts to plagiarism ...
Ultimately, we want to understand what researchers consider important in the decision to engage with generative AI—what excites or concerns them, how they are using or imagining using AI tools, and the role they believe publishers (among other institutional stakeholders) can play in supporting and protecting their published research.
For a more 'human' touch in machine-assisted research, AI-driven peer review platforms like HeyScience offer constructive feedback, ensuring a scholar's work resonates with academic communities.
This reignited AI research, and DL algorithms have become one of the most active fields of AI research. DL is a subset of ML based on multiple layers of neural networks with representation learning, 5 while ML is a part of AI that a computer or a program can use to learn and acquire intelligence without human intervention. Thus, "learn" is ...
Using AI in academics and research. Generative AI is rapidly transforming the academic and research landscape, offering new opportunities for discovery and innovation. It is important to approach generative AI with a thoughtful approach, balancing the benefits with potential risks and ethical considerations. In this page, we collect information ...
Publishing & Generative AI. Generative AI tools raise questions and concerns around authorship, research integrity, and more. At the same time, among other uses, these tools can ease and accelerate the editing process. Scholarly publishers have released policies and guidance for the use of Generative AI, for both authors and sometimes reviewers.
Clarivate makes sure you can safely and responsibly navigate this new landscape, driving research excellence and student learning outcomes. Trusted Academic AI. Clarivate AI-based solutions provide users with intelligence grounded in trustworthy sources and embedded in academic workflows, thus reducing the risks of misinformation, bias, and IP ...
Our Commitment: Academic Honesty. Jenni AI is committed to upholding academic integrity. Our tool is designed to assist, not replace, your effort in research and writing. We strongly discourage any unethical use. We're dedicated to helping you excel in a responsible and ethical manner.
Consensus responsibly uses AI to help you conduct effective research. Extensive Coverage. Search through over 200 million scientific papers without having to keyword match. Results you can trust. All of our results are tied to actual studies, we cite our sources and we will never show you ads.
The new version adds to the tsunami of interest in generative artificial intelligence since ChatGPT's launch in Nov. 2022. Over the last two years, some in higher education have shunned AI, while others embraced it, and the majority have cautiously begun tinkering.. Ajjan said she immediately thought the new vocal and video capabilities could allow GPT to serve as a personalized tutor.
Figure 1: Three Principles for Getting Value from AI Investments. The three principles are based on the following concepts from MIT CISR data research: 1. Data liquidity: the ease of data asset recombination and reuse. 2. Data democracy: an organization that empowers employees in the access and use of data. 3.
Understanding the Role of Large Language Models in Personalizing and Scaffolding Strategies to Combat Academic Procrastination Ananya Bhattacharjee, Yuchen Zeng, Sarah Yi ... summarizes lessons from these patterns, and highlights future research opportunities for human-AI collaboration in data storytelling. Learn more about our work and ...