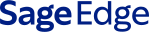
- Request new password
- Create a new account
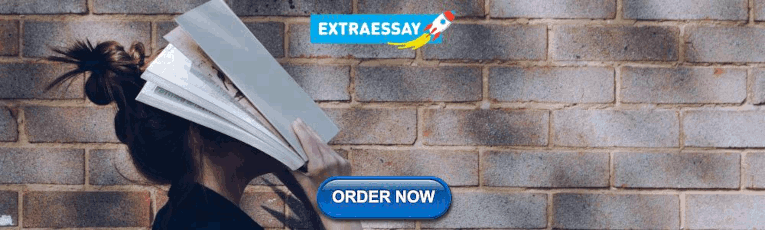
Introducing Communication Research: Paths of Inquiry
Student resources.
Welcome to the SAGE edge site for Introducing Communication Research, Fourth Edition .
The SAGE edge site for Introducing Communication Research by Donald Treadwell and Andrea Davis offers a robust online environment you can access anytime, anywhere, and features an impressive array of free tools and resources to keep you on the cutting edge of your learning experience.
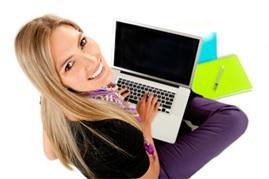
Introducing Communication Research: Paths of Inquiry teaches students the basics of communication research in an accessible manner by using interesting real-world examples, engaging application exercises, and up-to-date resources. Best-selling author Donald Treadwell and new co-author Andrea Davis guide readers through the process of conducting communication research and presenting findings for scholarly, professional, news/media, and web audiences. The Fourth Edition continues to emphasize the Internet and social media as topics of, and tools for, communication research, and incorporates new content on online methodologies, qualitative research, critical methodologies, and ethics.
Acknowledgments
We gratefully acknowledge Donald Treadwell and Andrea Davis for writing an excellent text. Special thanks are also due to Hailey Gillen Hoke of Weber State University, Vicki Karns of Suffolk University, and Thomas Wright of Temple University for developing the ancillaries on this site.
For instructors
Access resources that are only available to Faculty and Administrative Staff.
Want to explore the book further?
Order Review Copy
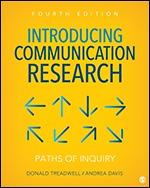
Breadcrumbs Section. Click here to navigate to respective pages.

Communication Research Methodology
DOI link for Communication Research Methodology
Get Citation
This introduction to communication research methods takes the student from the conceptual beginnings of a research project through the design and analysis. Emphasizing the correct questions to ask and how to approach the answers, authors Gary Petty, Cheryl Campanella Bracken, and Elizabeth Babin approach social science methods as a language to be learned, requiring multiple sessions and reinforcement through practice. They explain the basics of conducting communication research, facilitating students’ understanding of the operation and roles of research so that they can better critique and consume the materials in their classes and in the media.
The book takes an applied methods approach, introducing students to the conceptual elements of communication science and then presenting these elements in a single study throughout the text, articulating the similarities and differences of individual methods along the way. The study is presented as a communication campaign, involving multiple methodologies. The approach highlights how one method can build upon another and emphasizes the fact that, given the nature of methodology, no single study can give complete answers to our research questions.
Unique features of the text:
- It introduces students to research methods through a conceptual approach, and the authors demonstrate that the statistics are a tool of the concepts.
- It employs an accessible approach and casual voice to personalize the experience for the readers, leading them through the various stages and steps.
- The presentation of a communication campaign demonstrates each method discussed in the text. This campaign includes goals and objectives that will accompany the chapters, demonstrates each individual methodology, and includes research questions related to the communication campaign.
The tools gained herein will enable students to review, use, understand, and critique research, including the various aspects of appropriateness, sophistication and utility of research they encounter.
TABLE OF CONTENTS
Part i | 7 pages, a new language, chapter 1 | 11 pages, reality, what a concept: definition is everything, chapter 2 | 19 pages, six of one, half a dozen of the other: explication, validity, reliability, and measurement, chapter | 15 pages, ladies and gentlemen, place your bets: probability, sampling theory, and hypothesis testing, chapter 4 | 12 pages, ask it in the form of a question, please: developing questions and creating groups, chapter 5 | 14 pages, are they apples and oranges, or fruit: describing groups, part ii | 55 pages, methodologies/making observations, chapter 6 | 11 pages, getting to know you: empirical qualitative approaches, chapter 7 | 10 pages, intelligent design: basic approaches to design, chapter | 12 pages, kids, don’t try this at home: experimental design, chapter 9 | 11 pages, survey says: survey methodology, chapter 10 | 9 pages, is it a boy or a girl are those the only choices: content analysis, part iii | 2 pages, data analysis, chapter 11 | 9 pages, and what does it all mean: analytic principles, chapter | 11 pages, the count says: quantitative approaches, who are you calling a deviate: group differences, anova and the f, chapter | 13 pages, we need to have a talk about relationships: correlation, simple regression, multiple regression, chapter 15 | 8 pages, nobody got hurt: human subjects, institutional review boards, and ethics.
- Privacy Policy
- Terms & Conditions
- Cookie Policy
- Taylor & Francis Online
- Taylor & Francis Group
- Students/Researchers
- Librarians/Institutions
Connect with us
Registered in England & Wales No. 3099067 5 Howick Place | London | SW1P 1WG © 2024 Informa UK Limited
- Words, Language & Grammar
Sorry, there was a problem.

Download the free Kindle app and start reading Kindle books instantly on your smartphone, tablet, or computer - no Kindle device required .
Read instantly on your browser with Kindle for Web.
Using your mobile phone camera - scan the code below and download the Kindle app.

Image Unavailable

- To view this video download Flash Player
Follow the author

Media and Communication Research Methods: An Introduction to Qualitative and Quantitative Approaches 5th Edition
This step-by-step introduction to conducting media and communication research offers practical insights along with Arthur Asa Berger’s signature lighthearted style to make discussion of qualitative and quantitative methods easy to comprehend. The Fifth Edition of Media and Communication Research Methods includes a new chapter on discourse analysis; expanded discussion of social media, including discussion of the ethics of Facebook experiments; and expanded coverage of the research process with new discussion of search strategies and best practices for analyzing research articles. Ideal for research students at both the graduate and undergraduate level, this proven book is clear, concise, and accompanied by just the right number of detailed examples, useful applications, and valuable exercises to help students to understand, and master, media and communication research.
- ISBN-10 1544332688
- ISBN-13 978-1544332680
- Edition 5th
- Publication date February 1, 2019
- Language English
- Dimensions 6 x 1 x 8.75 inches
- Print length 488 pages
- See all details
Products related to this item

Editorial Reviews
About the author.
Arthur Asa Berger is Professor Emeritus of Broadcast and Electronic Communication Arts at San Francisco State University, where he taught between 1965 and 2003. He has published more than 100 articles, numerous book reviews, and more than 60 books. Among his latest books are the third edition of Media and Communication Research Methods: An Introduction to Qualitative and Quantitative Approaches (2013), The Academic Writer’s Toolkit: A User’s Manual (2008), What Objects Mean: An Introduction to Material Culture (2009), Bali Tourism (2013), Tourism in Japan: An Ethno-Semiotic Analysis (2010), The Culture Theorist’s Book of Quotations (2010), and The Objects of Our Affection: Semiotics and Consumer Culture (2010). He has also written a number of academic mysteries such as Durkheim is Dead: Sherlock Holmes is Introduced to Sociological Theory (2003) and Mistake in Identity: A Cultural Studies Murder Mystery (2005). His books have been translated into eight languages and thirteen of his books have been translated into Chinese.
Product details
- Publisher : SAGE Publications, Inc; 5th edition (February 1, 2019)
- Language : English
- Paperback : 488 pages
- ISBN-10 : 1544332688
- ISBN-13 : 978-1544332680
- Item Weight : 1.46 pounds
- Dimensions : 6 x 1 x 8.75 inches
- #142 in Media Studies (Books)
- #899 in Communication Reference (Books)
- #2,095 in Communication & Media Studies
About the author
Arthur asa berger.
ARTHUR ASA BERGER
I am the author of more than 100 articles and more than 90 books on popular culture, media, humor, advertising and tourism. I have also written some academic murder mysteries in which I bump off academics various ways and write nasty things about academia. I was born in Boston, Mass. I went to school at the University of Massachusetts in Amherst, MA for my BA degree in literature and philosophy. I went to the University of Iowa in Iowa City for my MA degree in journalism (but I also studied at the Writers' Workshop there)and I went to the University of Minnesota in Minneapolis for my Ph.D degree in American Studies. I wrote my dissertation on the comic strip Li'L Abner. After I received my MA degree I was drafted into the US Army and I did public information in Washington DC for two years. I also wrote high school sports for the Washington Post weekends while I was in the Army. I lived in Italy for a year in 1963 and taught American literature at the University of Milan. I also lived in England for a year in 1973 doing research on pop culture there. I taught at San Francisco State University from 1965 until 2003, when I retired. My books have been translated into Russian, Italian, German, Arabic, Indonesia, Swedish, Korean and Chinese. These Chinese like my books and have translated 12 of my books. Not all reviewers like my books. A review of my book THE TV-GUIDED AMERICAN, published in the early 1970s read "Berger is to the study of television what Idi Amin is to tourism in Uganda." I've lectured in many different countries, such as England, France, Germany, Italy, Norway, Denmark, Sweden, Thailand, Vietnam, Peru, and Tunisia. I was a visiting professor in Hong Kong for two months (in a school of tourism)in 2008 and I taught media criticism for six weeks in 2009 at Jinan University in Guangzhou and Tsing Hua University in Beijing. I spent a month in Argentina in September and October of 2012 lecturing on semiotics, media criticism and post modernism. In the course of my career I've lectured in around 20 foreign countries. I have continued to write and love to travel. I've visited more than 60 countries over the years. I live in Mill Valley, California.
I have some new books I've written are being published that will be of interest: Everyday Life in the Postmodern World (Springer), Crowds in American Culture (Anthem), Searching for a Self (Anthem), Shakespeare's Comedy of Errors (Anthem),
The Political Animal (Cambridge Scholars).
Here is a complete list of my books and their publishers.
1. Li'l Abner, 1970 (Twayne), 1994 (Univ. of Mississippi Press)
2. The Evangelical Hamburger, 1970 (MSS Publications)
3. Pop Culture, 1973 (Pflaum)
4. About Man, 1974 (Pflaum)
5. The Comic Stripped American, 1974 (Walker & Co., Penguin, Milano Libri)
6. The TV Guided American, 1975 (Walker & Co.)
7. Language in Thought and Action (in collaboration with S.I.Hayakawa, 1974, 1978) (HBJ)
8. Film in SOCIETY, 1978 (Transaction)
9. Television as an Instrument of Terror, 1978 (Transaction)
10. Media Analysis Techniques, 1982, 2nd Edition 1998 (SAGE)
11. Signs in Contemporary Culture, 1984 (Longman); 2nd edition, Sheffield, 1998. (Indonesian edition, 2003)
12. Television in SOCIETY, l986 (Transaction)
13. Semiotics of Advertising, 1987 (Herodot)
14. Media USA, 1988, (Longman 2nd Edition, 1991
15. Seeing is Believing: An Introduction to Visual Communication, 1989 (McGraw-Hill).
16. Political Culture and Public Opinion, 1989 (Transaction)
17. Agitpop: Political Culture and Communication Theory, 1989 (Transaction)
18. Scripts: Writing for Radio and Television, 1990 (SAGE)
19. Media Research Techniques, 1991, 2nd edition 1998 (SAGE)
20. Reading Matter, 1992 (Transaction)
21. Popular Culture Genres, 1992 (SAGE)
22. An Anatomy of Humor, 1993. (Transaction)
23. Improving Writing Skills, 1993 (SAGE)
24. Blind Men & Elephants: Perspectives on Humor, 1995 (Transaction)
25. Cultural Criticism: A Primer of Key Concepts, 1995 (SAGE
26. Essentials of Mass Communication Theory, 1995 (SAGE)
27. Manufacturing Desire: Media, Popular Culture & Everyday Life, 1996 (Transaction)
28. Narratives in Popular Culture, Media & Everyday Life, 1997 (SAGE)
29. The Genius of the Jewish Joke, 1997 (Jason Aronson)
30. Bloom’s Morning, 1997 (Westview/HarperCollins)
31. The Art of Comedy Writing, 1997 (Transaction)
32. Postmortem for a Postmodernist, 1997 (AltaMira).
33. The Postmodern Presence, 1998. (AltaMira)
34. Media & Communication Research Methods, 2000. (SAGE)
35. Ads, Fads & Consumer Culture, 2000. (Rowman & Littlefield)
36. Jewish Jesters, 2001. (Hampton Press)
37. The Mass Comm Murders: Five Media Theorists Self-Destruct. 2002 (Rowman & Littlefield).
38. The Agent in the Agency. 2003 (Hampton Press)
39. The Portable Postmodernist, 2003 (AltaMira Press)
40. Durkheim is Dead: Sherlock Holmes is Introduced to Social Theory, 2003 (AltaMira Press)
41. Media and Society, 2003 (Rowman & Littlefield)
42. Games and Activities for Media, Communication and Cultural Studies Students. 2004. (Rowman & Littlefield)
43. Ocean Travel and Cruising, 2004 (Haworth)
44. Deconstructing Travel: A Cultural Perspective, 2004 (AltaMira Press)
45. Making Sense of Media: Key Texts in Media and Cultural Studies, 2004 (Blackwell)
46. Shop Till You Drop: Perspectives on American Consumer Culture. 2004. (Rowman & Littlefield)
47. The Kabbalah Killings. 2004. (PulpLit)
48. Vietnam Tourism. 2005. (Haworth)
49. Mistake in Identity: A Cultural Studies Murder Mystery. 2005. (AltaMira)
50. 50 Ways to Understand Communication. 2006. Rowman & Littlefield.
51. Thailand Tourism. 2008. (Haworth Hospitality and Tourism Press)
52. The Golden Triangle. 2008. (Transaction Books).
53. The Academic Writer’s Toolkit: A User’s Manual. 2008. (LeftCoast Press)
54. What Objects Mean: An Introduction to Material Culture 2009. (LeftCoast Press)
55. Tourism in Japan: An Ethno-Semiotic Analysis. 2010 (Channel View Publications)
56. The Cultural Theorist’s Book of Quotations. 2010. (Left Coast Press)
57. The Objects of Affection: Semiotics and Consumer Culture. 2010. (Palgrave)
58. Understanding American Icons: An Introduction to Semiotics. 2012. (Left Coast Press).
59. Media, Myth and Society. 2012. (Palgrave Pivot)
60. Theorizing Tourism. 2012. (Left Coast Press).
61. Bali Tourism. 2013. (Haworth).
62. A Year Amongst the UK: Notes on Character and Culture in England 1973-1974. Marin Arts Press.
63. Dictionary of Advertising and Marketing Concepts. 2013 (Left Coast Press)
64. Messages: An Introduction to Communication. 2015. (Left Coast Press)
65. Gizmos, or The Electronic Imperative. 2015. (Palgrave)
66. Applied Discourse Analysis. 2016. (Palgrave)
67. Marketing and American Consumer Culture. 2016 (Palgrave)
68. Perspectives on Everyday Life. 2018 (Palgrave)
69. Cultural Perspectives on Millennials. 2018 (Palgrave)
70. Freud is Fixated: Sherlock Holmes and Psychoanalytic Theory. 2018. (Marin Arts Press)
71. Semiotics of Sport. 2018. (Marin Arts Press)
72. Signs and Society: 2019 (Nanjing Normal University Press)
73. Three Tropes on Trump. 2019. (Peter Lang)
74. Brands and Cultural Analysis. 2019 (Palgrave)
75. Shopper’s Paradise: Retail and American Consumer Culture 2019 (Brill)
76. Humor, Psyche, and Society. 2020. (Vernon)
77. Saussure Suspects. 2020. (Marin Arts)
78. Travel Notes. 2020. (Marin Arts)
79. My Name is Sherlock Holmes. 2020 (Marin Arts)
80. Trump’s Followers. 2020. (Peter Lang)
81. USA POP. 2020. (Cambridge Scholars)
82. LUXE: Luxury in American Consumer Culture. 2020 (Cambridge Scholars)
83. The Creative Process. 2021. (Cambridge Scholars)
84. Smooth Sailing. 2022. (Brill) in press
85. Why We Love Westerns. 2022. (DSP)
86. Searching for a Self. 2022. (Vernon)
87. Everyday Life in the Postmodern World. 2022. (Springer) in press
88. Shakespeare’s The Comedy of Errors. 2022. (Anthem) in press
89. Semiotics of Sport. 2022. (Brill) in press
90. The Political Animal. 2022?
91. The New Crowd: An American Variation. 2022?
92. Taste: Why We Like What We Like. 2022?

Customer reviews
- 5 star 4 star 3 star 2 star 1 star 5 star 75% 10% 5% 7% 3% 75%
- 5 star 4 star 3 star 2 star 1 star 4 star 75% 10% 5% 7% 3% 10%
- 5 star 4 star 3 star 2 star 1 star 3 star 75% 10% 5% 7% 3% 5%
- 5 star 4 star 3 star 2 star 1 star 2 star 75% 10% 5% 7% 3% 7%
- 5 star 4 star 3 star 2 star 1 star 1 star 75% 10% 5% 7% 3% 3%
Customer Reviews, including Product Star Ratings help customers to learn more about the product and decide whether it is the right product for them.
To calculate the overall star rating and percentage breakdown by star, we don’t use a simple average. Instead, our system considers things like how recent a review is and if the reviewer bought the item on Amazon. It also analyzed reviews to verify trustworthiness.
- Sort reviews by Top reviews Most recent Top reviews
Top reviews from the United States
There was a problem filtering reviews right now. please try again later..

Top reviews from other countries

- About Amazon
- Investor Relations
- Amazon Devices
- Amazon Science
- Sell products on Amazon
- Sell on Amazon Business
- Sell apps on Amazon
- Become an Affiliate
- Advertise Your Products
- Self-Publish with Us
- Host an Amazon Hub
- › See More Make Money with Us
- Amazon Business Card
- Shop with Points
- Reload Your Balance
- Amazon Currency Converter
- Amazon and COVID-19
- Your Account
- Your Orders
- Shipping Rates & Policies
- Returns & Replacements
- Manage Your Content and Devices
- Conditions of Use
- Privacy Notice
- Consumer Health Data Privacy Disclosure
- Your Ads Privacy Choices

1st Edition
Communication Research Methodology A Strategic Approach to Applied Research
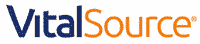
- Taylor & Francis eBooks (Institutional Purchase) Opens in new tab or window
Description
This introduction to communication research methods takes the student from the conceptual beginnings of a research project through the design and analysis. Emphasizing the correct questions to ask and how to approach the answers, authors Gary Petty, Cheryl Campanella Bracken, and Elizabeth Babin approach social science methods as a language to be learned, requiring multiple sessions and reinforcement through practice. They explain the basics of conducting communication research, facilitating students’ understanding of the operation and roles of research so that they can better critique and consume the materials in their classes and in the media. The book takes an applied methods approach, introducing students to the conceptual elements of communication science and then presenting these elements in a single study throughout the text, articulating the similarities and differences of individual methods along the way. The study is presented as a communication campaign, involving multiple methodologies. The approach highlights how one method can build upon another and emphasizes the fact that, given the nature of methodology, no single study can give complete answers to our research questions. Unique features of the text: It introduces students to research methods through a conceptual approach, and the authors demonstrate that the statistics are a tool of the concepts. It employs an accessible approach and casual voice to personalize the experience for the readers, leading them through the various stages and steps. The presentation of a communication campaign demonstrates each method discussed in the text. This campaign includes goals and objectives that will accompany the chapters, demonstrates each individual methodology, and includes research questions related to the communication campaign. The tools gained herein will enable students to review, use, understand, and critique research, including the various aspects of appropriateness, sophistication and utility of research they encounter.
Table of Contents
Gary Pettey, Cheryl Campanella Bracken, Elizabeth B. Pask
About VitalSource eBooks
VitalSource is a leading provider of eBooks.
- Access your materials anywhere, at anytime.
- Customer preferences like text size, font type, page color and more.
- Take annotations in line as you read.
Multiple eBook Copies
This eBook is already in your shopping cart. If you would like to replace it with a different purchasing option please remove the current eBook option from your cart.
Book Preview

The country you have selected will result in the following:
- Product pricing will be adjusted to match the corresponding currency.
- The title Perception will be removed from your cart because it is not available in this region.
Pardon Our Interruption
As you were browsing something about your browser made us think you were a bot. There are a few reasons this might happen:
- You've disabled JavaScript in your web browser.
- You're a power user moving through this website with super-human speed.
- You've disabled cookies in your web browser.
- A third-party browser plugin, such as Ghostery or NoScript, is preventing JavaScript from running. Additional information is available in this support article .
To regain access, please make sure that cookies and JavaScript are enabled before reloading the page.
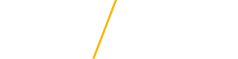
- Chapter One: Introduction
In this chapter, we first describe how developing a command of research methods can assist you in your careers and personal lives. Second, we provide a brief definition of our topic of study in this book – communication research. Third we identify the predominant research and creative methods used in the field of Communication Studies. Fourth, we explain the academic roots of the diverse methods used in communication studies: the humanities and social sciences. Fifth, we explain the implicit and explicit relationships between theory and research methods. Sixth, we describe how the choice of research methods influences the results of a study. Sixth, we provide a preview of the remainder of the book, and finally, seventh, we describe our approach to writing this book.
- Chapter Two: Understanding the distinctions among research methods
- Chapter Three: Ethical research, writing, and creative work
- Chapter Four: Quantitative Methods (Part 1)
- Chapter Four: Quantitative Methods (Part 2 - Doing Your Study)
- Chapter Four: Quantitative Methods (Part 3 - Making Sense of Your Study)
- Chapter Five: Qualitative Methods (Part 1)
- Chapter Five: Qualitative Data (Part 2)
- Chapter Six: Critical / Rhetorical Methods (Part 1)
- Chapter Six: Critical / Rhetorical Methods (Part 2)
- Chapter Seven: Presenting Your Results
How Will Research Methods Help in My Life?
If you want to learn practical skills relevant to your professional, personal and community life, learn research methods. Given that daily life is full of decision-making opportunities and challenges, knowing how to effectively do research is essential. Ideally, any decision you make is based on research, and rigorous methods enable you to conduct better research and make better decisions. People who know how to ethically use research methods quickly become leaders in their workplaces and communities. Research also can inform creative expression. If you understand why things work the way they do, you can make more thoughtful, creative choices.
Consider how you make choices in everyday life such as the following:
- Which route to take to get to class on time
- What to eat for lunch
- How to make a major purchasing decision
Or, how you address more complex questions such as whether dishonesty is ever warranted, or if there is a God?
Brainstorm all the ways in which you think you know something for one or more of the examples listed above.
If you are like previous students in this course, you may have responded: "read," "observe," "intuit," "faith," "advice," "physical senses," "test it out," "compare," "Google it" and more.
What does this activity reveal about how you come to know something?
We hope the activity above reveals you already are a researcher, and use some informal research methods every day of your life. You likely use more than one way to know something. Multiple methods construct knowledge. And being educated includes questioning the results of each method. For example, if you use Google or Wikipedia to find information, how do you know the source is reliable? What clues should you look for?
Research methods will help you be a better ....
- Critical Consumer — You will find you look at the world of information through a more refined lens. You may ask questions about information you never thought to ask before, such as: "What evidence is this conclusion based on?" "Why did the researcher interview rather than survey a larger number of people?" and "Would the results have been different if the participants were more ethnically and racially diverse?
- Competent Contributor — When an organization you belong to wants to attain a group's input on a program, product or service, you will know how to construct, administer and statistically analyze survey results. Or if the project warrants small focus group discussions for information gathering, you will know how to facilitate them as well as how to identify themes from the discussions.
- Problem-Solver — Research methods skills are nearly synonymous with problem- solving skills. You will learn how to synthesize information, assess a current state of knowledge, think creatively, and make a plan of action for original research gathering and application.
- Strategic Planner – Knowing research methods can teach you how to gather the necessary information to forecast and plan tactically rather than only react to situations, whether it is in your work place or personal life.
- Decision-Maker — As you cross through life transitions and major decisions stare you in the face, such as how to keep a job, give the best care for aging parents, or select the least invasive medical treatment, you will have coping skills to help you break down the decision into manageable parts and approach the decision making process from more than one perspective.
- Informed Citizen — As a person educated in how knowledge is constructed, you will have the skills needed to be vigilant for your community and to identify and address potential problems, be they environmental, political, social, educational, and/or about quality of community life.
For more specific ideas about how a command of research methods can broaden your life options, see the examples of practical research at Communication Currents: Knowledge for Communicating Well . It is a reader-friendly magazine where communication scholars discuss research about current social problems ( The National Communication Association - Communication Currents ). Also check out the National Communication Association website http://www.natcom.org/ for careers in communication.
The Topic of Study: Communication Research
You may have noticed we, the authors, use the singular form of the term communication to refer to the academic field of study on a wide variety of message types, rather than the plural form: communications. The distinction is a quick way to tell who understands communication is one specific field of study and who does not, so you will want to use the proper, singular form when referring to the field of study. Communications – plural is used only when referring to multiple media sources, as in "the communications news media" (Korn, Morreale, & Boileau, 2000).
The forms communication can take are nearly endless. They include, but are not limited to: language, nonverbal communication, one-on-one interpersonal communication, organizational communication, film, oral interpretations of prose or poetry, theatre, public speeches, public events, political campaigns, public relations campaigns, news media, Internet, social media, photography, television, social movements, performance in everyday life, journalistic writing, and more. Yet, the theme that runs through almost all Communication Studies research is that communication is more than a means to transmit information. Although it is used to transmit information and get things done, more importantly, communication is the means through which people make meaning and come to understand each other and the world.
Because of this, communication scholars tend to operate with the assumption that reality is a social construction, constructed through human beings' use of communication, both verbal and visual (Gergen, 1994). Thus, when communication scholars conduct research, they ask questions not only about how to make communication more precise and/or effective, but they also ask questions about how communication is being used in a particular context to shape individuals' and groups' world views.
Research, as a form of communication, contributes to the social construction of knowledge. Knowledge does not come out of a vacuum that is free of cultural values. Instead, research results, or what society calls knowledge, is influenced by the values, beliefs, methods choices, and interpretations of those in a given culture doing the research. Knowledge and one's reality are constructed through an interactive, interpretive process. Although scholars from a more traditional natural science view might argue there are absolute truths and set realities, in the study of human interaction, there are few universal truths about communication and what is seen as knowledge changes across cultures and over time. Unique cultural contexts, social roles, and inequities create a wide spectrum of behaviors ( Kim , http://plato.stanford.edu/entries/weber/ ). That is what makes miscommunication common and why research in our field is so much in demand. It is highly practical and relevant work.
Research refers to the systematic study of a topic and can include social science and creative work. Research, quite simply, refers to people's intellectual work of gathering, organizing, and analyzing data, which enables them to create meaning they can then present to others. Research is conducted to answer questions or solve problems in a systematic way. Being systematic means that the steps of the study are guided by principles and theory, rather than just chaotic wandering; the data used is representative and not just anecdotal or random. Being systematic in a way that can be replicated is usually emphasized more in natural and social science research, such as organizational and interpersonal communication research, than the humanities and fine arts, such as rhetorical studies and performance studies, but, rhetoric scholars and artists also rely on methods and theoretical training to guide their work.
Communication Studies research has several unique characteristics:
- Communication research is the study of how people make meaning. If one thinks of communication as the process of making meaning, then the study of communication is the study of this meaning making process.
- Communication research is the study of patterns (Keyton, 2011). Communication and meaning are made possible through the creation of patterns. For example, languages are rule-based and construct recognizable patterns (such as sentences). Conversations have social norms of politeness to enable participants to build on each party's turns at talk; social media have unique patterns of interaction (such as the abbreviations used in text messaging on cell phones or the emoticons used in e-mail and social networks); and persuasive messages are built on patterns of communication strategies (such as advertisements showing sequences of visual appeals for destitute children to solicit donations).
- Communication research is practical knowledge construction. The field of communication is highly applied. Scholars and practitioners try to do work that matters. Work that improves the quality of people's lives, that solves problems, and that is needed. Research in the field is pragmatic. Film makers tell a story that they believe needs to be told, performance studies students create interactive scenarios to draw the audience into needed cultural discussion, and public relations practitioners conduct market analyses as a basis for planning a client's communication strategies.
- A ll research builds an argument. Whether it is a creative, rhetorical, qualitative or quantitative project, the author necessarily has a point to make. The introductory rationale for a project, the choices the researcher makes in methods selection, the interpretations offered, and the significance she or he claims for the results are all a part of building an argument. If all knowledge is socially constructed, then all research or scholarship is a persuasive process.
Whether one is doing Creative, qualitative, rhetorical or quantitative work, the methods share the above characteristics, as they are inherent in the very communication process being studied.
Research Methods
The term method refers to the processes that govern scholarly and creative work. Methods provide a framework for collecting, organizing, analyzing and presenting data. Scholars use a range of methods in Communication Studies: quantitative, qualitative, critical/rhetorical, and creative. This text focuses on the first three, but the authors note connections to creative work when relevant.
Quantitative Studies reduce data into measurable numerical units (quantities). An example would be a survey administered to determine the number of times first-year college students use social networking sites and for what purposes. Such a survey could provide general statistics on frequency and purpose of use. But, such a study also could be set up to determine if first and fourth year college students use social networks differently, or if students with smart phones spend more time on Facebook than students who rely on computers to check Facebook.
Qualitative Studies use more natural observations and interviews as data. An example would be a study about a workplace organization's leadership and communication patterns. A researcher could interview all the members of the business, and then also observe the members in action in their place of work. The researcher would then analyze the data to see if themes emerge, and if the interview and observational data results are similar. The researcher might then propose changes to the organization to enhance communication and performance for the organization.
Critical/Rhetorical Studies focus on texts as sources for data. The term texts is used loosely here to refer to any communication artifact --films, speeches, historical monuments, news stories, letters, tattoos, photos, etc. Here, the data collected is the text, and it is used by the researcher to support an argument about how the text participates in the construction of people's understanding of the world. An example would be an analysis of a presidential inaugural address to understand how the speech writers and speaker are attempting to reunite the nation after a hotly contested election and invest the new president with the powers of the office.
Creative Scholarship in the field of Communication Studies refers most often to work done in performance studies, film making, and computer digital imagery, such as Dreamweaver and Photoshop (e.g. Camp Multimedia Begins Two-Week Run ). Performance Studies is a wide umbrella term used to refer to several methods and products of scholarship. It is distinct from theater in that it is the study of performances in everyday life. It involves students in script writing, acting, and directing productions based upon oral history and ethnographic qualitative research, as well as personal experience and creative performance techniques used to tell a story more evocatively. Film Making can also include interviews, oral histories, and ethnographies, as well as learning aesthetic methods to effectively present verbal and visual images. Our colleague Karen Mitchell has used qualitative methods of interviewing and ethnography to script performances on topics from the lives of undocumented immigrants in the U.S. to romance novel readers (1996).
Communication Studies Bridges the Humanities and Social Sciences
How is it that Communication Studies as an academic field came to embrace so many different methods, given most other disciplines tend to use only one, or maybe two? The answer lies in the history of the field.
Communication Studies is different from other academic fields because it is rooted in one of the oldest areas of scholarship (rhetoric is one of the original four liberal arts) and in several of the newest areas of scholarship (such as electronic media and intercultural communication). The study of rhetoric dates back to 350 B.C.E, the time of Aristotle and the formation of democratic governance in Greece. The study of intercultural communication dates back to the 1940s and emerged out of the commerce and political needs in the U.S. after World War II (Leeds-Hurwitz, 1990). The study of the Internet took shape in the 1990s as it became a popular medium for communication (Campbell, Martin & Fabos, 2010). Because the discipline of Communication Studies includes research on all forms of communication, the method of study needs to fit as the form of communication. However, just because new forms emerge (like social media), old forms (like public speaking) do not disappear. Thus, as students, future practitioners and scholars, we need to employ a wide range of scholarly approaches. (for more about the history of the field see: Communication Scholarship and the Humanities ) .
The diverse origins of Communication Studies mean its scholars use a range of methods from the humanities (e.g., rhetorical criticism and performance) and the social sciences (e.g., quantitative and qualitative research). Both focus on the study of society, but the humanities embrace a more holistic approach to knowledge and creativity. The humanities are those fields of study that focus on analytic and interpretive studies of human stories, ideas and words (rather than numbers), and include philosophy, English, religion, modern and classical languages, and Communication Studies. When Communication Studies scholars analyze how communicative acts (like speeches or photographs or letters) create social meaning, they do so from a perspective that emphasizes interpretation.
The social sciences use research methods borrowed from previously established and recognized fields of natural science study, such as biology and chemistry. Scientific methods of knowledge construction are accomplished through controlled observation and measurement or laboratory experiments, and generally use statistics to form conclusions (Kim, 2007). The social sciences apply scientific methods to study human behavior, for example scholars use surveys to find out about people's communication patterns or create laboratory experiments to observe interruption patterns in conversation. In addition to Communication Studies, examples of other social science fields include economics, geography, psychology, sociology, and political science.
Studying human communication from the perspective of the social sciences differs in important ways from studying human communication from the perspective of the humanities. Social scientists typically are interested in studying shared everyday life experiences, such as turn-taking norms in conversation, how people build relationships through self-disclosure, and what behaviors contribute to a successful group, family or organizational culture. Social science researchers attempt to find generalizations about human behaviors based on extensive research that may be used to make predictions about that behavior. Take, for instance, research on communication in heterosexual married couples. Based on over twenty years of research, psychologist John Gottman found in 1994 he could predict with 94% accuracy which marriages will fail based on patterns of only five negative conflict behaviors among couples who ended in divorce(for updates on his work visit his website ( Research FAQs ). (Of course exceptions exist to generalizations, but for a social scientist, the exception to the rule may be ignored as an insignificant outliers, a random error.
Instead of seeking out generalizations about communication, scholars in the humanities tend to focus on the outliers, or what are considered distinctive human creations, such as Abraham Lincoln's "Gettysburg Address," Elizabeth Cady Stanton's "Solitude of Self," Shakespeare's Romeo and Juliet , Lorraine Hansberry's Raisin in the Sun , Beethoven's Fifth Symphony, or Ani diFranco's "Dilate." Humanities scholars tend to focus on understanding how something happened or how someone attempted to evoke meanings and aesthetic reactions in the receivers of a message, rather than describing what occurred and predicting what will occur.
As an example of how diverse methods have been used to research a topic, consider how researchers who want to try to reduce intimate partner violence have approached the problem drawing on methods from across fields of study.
Quantitative researchers administered the National Survey on Violence Against Women and found 1.5 million women are physically or sexually assaulted by their domestic partners annually in the U.S. (Tjaden & Thoennes, 2000). The survey identified the difficult reality about the enormous extent of the problem. Qualitative researcher, Loren Olson (2010), wrote an autoethnography of her personal experience as a battered woman. By doing so she put a face on the problem and demonstrated a way in which she was able to reconstruct her identity after the abuse.
Performance studies scholar M. Heather Carver and ethnographic folklorist Elaine Lawless (2009) conducted a qualitative study with women who are surviving intimate violence and generated a creative performance script from their observations. The theatrical performance developed with creative methods literally help to give voice to the experiences of the women in the qualitative study, raises awareness about the problem, and may motivate audience members to address the problem in their personal or community lives.
Researchers also have critically analyzed the way domestic violence is communicated in various media. For example, Cathy Ferrand Bullock (2008) studied media framing in domestic violence news stories in Utah newspapers and rhetoric scholar Nathan Stormer (2003) studied the play, A Jury of Her Peers , to explore how collective memory is formed about acts of domestic violence. These samples of research into the complex social problem of domestic violence demonstrate how both humanities and social science approaches to scholarship are needed and valued. Because the social sciences and humanities provide different contributions to the construction of knowledge, together they create a fuller picture of a social problem or issue of study.
Given its multi-methodological research, Communication Studies is uniquely positioned to contribute to both of the two most prominent approaches to knowledge construction: humanistic and social scientific approaches. This is why, as the authors of this book, we believe Communication Studies provides a well-rounded education to prepare students to respond to the challenges and opportunities of the culturally, technologically, and economically complex 21st century.
The Interdependence of Theory and Research Methods
Whether you approach a topic of study from a humanistic or social science perspective, you will necessarily work with two-components: theory (explanations that guide or evolve from a study) and methods (application of tools to analyze texts or data). Even though the two serve distinct research functions, there is great interdependence between theory and method. Theory informs methods, and methods enable theory construction and revision.
At its essence, a theory is simply a person's attempt to explain or understand something. Individuals use theories to help make sense of their world and everyday lives. An academic theory is different from everyday theories only in the degree of rigor and research used to develop it and the depth of explanation it provides. Academic theories are more formal, with detailed explanations of the parts that make up the theory, and are usually tested (West & Turner, 2010). But as with theories for everyday life, they are subject to change and refinement. DeFrancisco and Palczewski (2007) emphasize, "A theory is not an absolute truth, but an argument to see, order, and explain the world in a particular way" (p. 27). For any topic of study, multiple theories could explain it, and research can be used to determine which theory offers the best explanation. Communication theories tend to focus on helping explain how and why people interact as they do in interpersonal relationships, small groups, organizations, cultures, nations, publics, and mediated contexts. Theories can help people understand their own and others' communication.
When you make decisions in daily life, you probably use an informal theory. You might collect some data (or try and recall what information you have), you might discard data that comes from non-credible sources, and then you might assess your options. You will likely make your assessment based on hunches or underlying assumptions you have about what makes sense. Those hunches or assumptions are a lay person's theory. They help you make sense of things and inform your decisions.
Activity Consider the following questions to determine if you use theories in your daily life:
- What is your advice for how to live on a college student's budget?
- Do you think advertising influences your purchasing decisions? If so, how?
- What is your approach to making a good first impression on a person to whom you are attracted?
- How do you know someone is attracted to you?
- Why do you think people tend to avoid relationships with others they perceive as different from them?
If you have ideas on the above topics, you are a theorist.
Now ask yourself: what do your answers to the specific questions consist of?
- Are they attempts to explain a phenomenon?
- How did you form the explanations?
- Are they based on prior experience, advice from others, and/or informal research?
Likely your answers are a little of each.
A further question to ask yourself is:
- Are other explanations possible besides the ones you developed?
Students have developed more than one way to survive on a college student's budget. For one thing, not all college students are living on a tight budget, many will survive through student loans and jobs, others may get allowances from their parents, have spouses or partners who are supporting them, etc. Some will delay gratification of purchases such as cars, I-Pads, smartphones, spring break trips, and more. Others may argue, "You only live once," and use credit cards to charge for their pleasures or life necessities. The point is people develop multiple theories for any topic of interest, and many are useful.
People develop theories through testing, academic debates, and scholarly/creative work. Natural science and social science researchers, in particular, believe that the best research is directed or driven by academic theory. This means the research methods chosen are not random but are firmly based in a credible theoretical approach that has been tested over time.
Theories often guide research. When studying presidential campaigns for example, scholars often use Thomas Burke's theories on how speakers create identification to explore the ways in which candidates create connections with their audience (Burke, 2002).
Sometimes the research will extend or challenge the legitimacy of the theory. For example, intercultural communication scholar, William Gudykunst extended Berger and Calabrese's (1975) assumed universal Uncertainty Reduction Theory (URT) regarding what people do to reduce uncertainty anxiety when communicating with strangers. During 30 years of research, Gudykunst tested URT in cross cultural interactions and developed a new intercultural theory, Anxiety/Uncertainty Management (AUM) with 47 axioms or specific distinctions that help explain the universal and cultural variances he found (2005). Contrary to the original URT, Gudykunst now proclaims cultures vary in terms of comfort with uncertainty and the methods they use to manage it. These cultural differences contribute to unique cultural identities and help explain communication problems with other groups.
In the field of gender studies in communication there are countless examples of research that has disproven the commonly held theoretical assumption that universal gender differences exist between all women and men (e.g. Tannen, 1990; or in the popular press: Men are from Mars and Women are from Venice (Gray, 1992). In fact, communication scholars Kathryn Dindia and Dan Canary (2006) published a series of quantitative meta-analyses (a statistical way to control for differences across studies to directly compare the results) on just about every presumed communication difference previous researchers have studied. What did they find? While some differences were present, the variances among women's behaviors and among men's behaviors were greater than those between the sexes, and furthermore, women and men communicate in many more ways that are similar rather than different. Finally, they found that the assumption of two distinct sets of behavior is far too simplistic. It ignores the fact that people have the ability to adjust their behaviors according to situational needs and that gender identity does not affect one's behavior alone. It is also influenced by one's race, ethnic, age, nationality, sexual orientation and more.
A useful way to think of the relationship between theory and scholarly/creative work is that it is synergistic – each influences the other, almost simultaneously. As the illustration below shows, the theories selected direct the types of research questions posed to guide a study, the questions dictate the appropriate research methods needed, which then affect the results produced, which in turn contributes to theory building, thus the cycle repeats.
The General Research Process: Circular and Interdependent
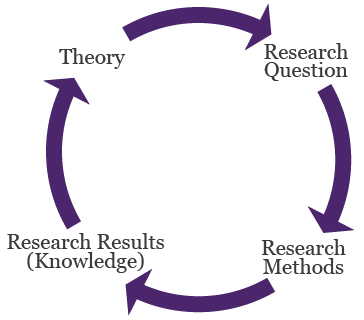
Knowledge generally refers to a command of facts, theory and practical information. There is not one agreed upon approach for constructing knowledge as is illustrated in the above discussion of diverse research methods. Indeed, there is an entire field of philosophy, epistemology , which focuses on debates about how knowledge is attained. Epistemologists ask "how does one know something?" Is knowledge found or created? These are questions we encourage you to ask as you learn about the various research methods. The methods researchers use to construct knowledge are generally called methodology . The term simply means an approach being used to form knowledge is assumed to have both a theory and a method. Here again the interdependence between theory and method are evident.
Finally, throughout the research process the ability to think critically is essential. To be critical means to examine material in more depth, to peel back layers of meaning, to look beyond chunks of information to the context in which the information is presented, to look for multiple interpretations, to attempt to identify why a piece of information or perspective is important and/or not important. It requires doing a close reading or investigation of the topic of study in a more nuanced, systematic way. It does not mean to always be negative, but rather to question even common assumptions.
Research Methods Influence Results
The research methods one chooses for a study are critical. The methods will largely determine the results or what is called knowledge. The influence of methods choices is more visible when comparing social science and humanities approaches to the construction of knowledge, as will be discussed in chapter 2. The two are designed to answer different types of research questions. Together, they will offer you a wealth of methods choices.
For example, consider the relatively simple task of measuring the floor area of a room. We assigned small groups of students to measure the square footage in a room. Each group was provided different measurement tools. One group used a tape measure 12 feet long, another used a tape measure 40 feet long, another group used their own feet, and another used a metric tape measure. As you can imagine, the groups' results differed every time. Some used feet rather than inches to calculate square feet, some did not measure the same exact places in the room, metric measurements produced different results than the U.S. measuring system, and human feet produced varying results. The point here is not that one method was superior to another or that the groups made errors. The point is that even a slight change in methods can produce significant changes in results (Turman, personal communication, January 27, 2010). (If you would like to see more on metrics versus U.S. units conversion, see for example, Metric to U.S. units conversion .
If diverse results can be produced when measuring the floor area of a room, imagine how different research methods may influence the study of processes as complex as human communication. Leslie Baxter has studied interpersonal relationship development and maintenance for nearly 20 years. Most of her early research was based on quantitative surveys of romantic partners in an attempt to identify the specific tensions or stresses in their relationship. By using standardized surveys she was able to identify three dominant tensions most couples struggled with: connection/independence, openness/closedness, and predictability/spontaneity. From this she developed what is now a well known theory in the field, Dialectic Tensions Theory. However, more recently she revised her theory based on qualitative studies of relational partners' conversations. Baxter now argues that by examining tensions in actual discourse rather than surveys, she is not only able to identify common tensions, but move beyond identification to see why some relationships successfully negotiate the tensions and why others do not (2011). We offer this example not to argue qualitative methods are superior to quantitative ones, but simply to make the point that the two serve different functions.
As you will learn in the coming chapters, each method used to collect data carries with it a different implied theory about how knowledge should be, or is formed. When researchers use surveys they value the ability to solicit a larger number of people and make generalizations from the responses. When researchers analyze conversations or use interviews, they value the ability to probe individual perspectives in more depth and are less concerned with generalizations. As teachers, scholars and practitioners, the authors of this book believe a command of research methods is central to developing one's unique expertise.
Preview of Chapters
In this book, three general research approaches are included: quantitative social science research methods, qualitative social science research methods, and critical rhetorical research methods. This does not mean these three are the only approaches to knowledge construction used in the field of Communication Studies or that they are necessarily independent or opposite of each other. Communication Studies is a wonderfully diverse field of study. In addition to rhetorical methods, other humanities scholarship include performance studies and film making. Because of the extreme interdisciplinary nature of film-making and performance studies, no one research method or chapter is dedicated to them. Instead we integrate examples throughout the collection, and readers should keep in mind how such work pushes the boundaries of traditional academic fields. Below are summaries/previews of the remaining chapters in this book.
Chapter One Summary : In the present chapter, we overviewed the interdisciplinary nature of the field of Communication Studies and demonstrated how this provides a broader choice of research methods for students and faculty members in the field. We introduced basic concepts necessary to have a foundation for the study of research methods. Even though scholars use diverse research methods in the field, they are built on common premises. One is that knowledge is constructed. The way it is constructed is influenced by the theoretical approaches used and the related research methods chosen. Understanding these fundamental relationships will help students be more informed critical consumers and contributors to the field of Communication Studies, their chosen professions, and society.
Chapter Two: General Comparisons . In chapter two, we offer basic points of comparison for the research methods taught in this book. This comparison should help provide a structure to understand how the diverse methods are distinct from each other before you are introduced to the specifics of conducting research in each method in subsequent chapters. The comparison is based on the two general orientations to knowledge construction introduced in chapter one: humanistic and social scientific.
Chapter Three: Ethical Research, Writing, and Creative Work . In this chapter, we discuss the importance of researcher ethics. This chapter is placed at the front of the book to stress this importance. Regardless of the method chosen, researchers have ethical choices to make in writing honestly, citing other sources, and treating human subjects fairly. Good research is, at its core, based on ethical principles.
Chapter Four: Quantitative Methods . The first research approach presented is quantitative research methods from the social sciences. The rules involved in doing quantitative methods are very clear, with a linear research process. Reading this chapter will teach you how to plan and conduct a quantitative study, and make sense of your findings once you have collected your data.
Chapter Five: Qualitative Methods . Qualitative research methods can be placed in the middle of a continuum of research methods from the scientific to the humanistic. Qualitative methods are usually considered to be a social science approach, but in more recent years researchers have been pushing these boundaries to embrace multiple ways of knowing.
Chapter Six: Critical/Rhetorical Methods . The core assumption of rhetorical criticism is that symbolic action (the use of words, images, stories, and argument) are more than a means to transmit information, but actually construct social reality, or people's understanding of the world. Learning methods of rhetorical criticism enable you to critique the use of symbolic action and understand how it constructs a particular understanding of the world by framing a concept in one way rather than another. The more adept you become at analyzing others' messages, the more skilled you become at constructing your own.
Chapter Seven: Presenting Your Results . This chapter teaches you how to present the results of your study, regardless of the choice made among the three methods. Writing in academics has a basic form and style that you will want to learn not only to report your own research, but also to enhance your skills at reading original research published in academic journals. Beyond the basic academic style of report writing, there are specific, often unwritten assumptions about how quantitative, qualitative, and rhetorical studies should be organized and the information they should contain. In this chapter students will learn about the functions of each part of a report (e.g. introduction, methods and data description, and critical conclusion) and find useful criteria to help guide the writing of each part in a research report.
Approach to Writing this Resource Book
When the faculty in the UNI Department of Communication Studies decided to make research methods a required course for all students majoring in the department (starting Fall, 2010), we searched for a textbook that equitably covered methods used in the humanities and social sciences. We could not find one, so we decided to write our own. This resource book is the product of a collaborative effort by faculty in the department. Although five of us wrote and organized the chapters, everyone in the department was invited to contribute ideas and examples.
The result is not a traditional textbook. For one thing, rather than one voice, the authors hope you will hear their distinct voices in each chapter influenced, in part, by the methods chosen and the values these methods reflect. The differing styles should help prepare you for the differing writing styles you will find when you read original research in journals that feature quantitative, qualitative, or critical/rhetorical studies. Consequently, the citation systems we use to document sources differ across chapters. In chapters on social science research (quantitative and qualitative research methods) we use the American Psychological Association (APA) (2010) style, because it is the format of choice for most journals publishing social science research. In the chapters on ethics and rhetorical methods we use the format prescribed by the Modern Language Association (MLA) (2009) because rhetoric is rooted in the Humanities, and rhetorical research often is published in journals that also include scholarship from performance studies, English literature and the fine arts. As one reads the coming chapters, it can be insightful to attempt to identify how the methods and values are reflected in the writing styles.
Another distinction is that because the text is digital, rather than paper, we are able to make the book more interactive, including additional websites and other resources to hopefully help make the methods come alive. Perhaps most importantly for you as a student, using a digital delivery system means far less expense. The digital delivery system also means we have the ability to update material continuously.
Through this book, we hope you will become excited by the possibilities of participating in the construction of knowledge in Communication Studies. We also hope to help demystify the research process and reveal underlying assumptions of each process. Contrary to what some public figures, educators and media sources would have the public believe, most knowledge is not absolute. We invite your critical voice to this learning process.
American Psychological Association. (2010). Publication manual of the American Psychological Association (6th ed.). Washington, DC: American Psychological Association.
Baxter, L. (2011). Voicing relationships: A dialogic perspective . Thousand Oaks, CA: Sage Publications, Inc.
Berger, C. R., & Calabrese, R. (1975). Some explorations in initial interactions and beyond: Toward a development theory of interpersonal communication. Human Communication Research , 1, 99-112.
Bullock, C. F. (2008). Official sources dominate domestic violence reporting. Newspaper Research Journal , 29(2), 6-22.
Burke, K. (1966). Language as symbolic action: Essays on life, literature, and method . Berkeley, CA: University of California Press.
Burke, T. (2002). Lawyers, lawsuits and legal rights: The battle over litigation in American society . Berkeley, CA: University of California.
Campbell, R., Martin, C. R., & Fabos, B. (2010). Media & culture: An introduction to mass communication (8th ed.). Boston, MA: Bedford/St. Martin's.
Carver, M. H., & Lawless, E. J. (2009). Troubling violence: A performance project . Jackson, MI: University of Mississippi Press.
DeFrancisco, V. P., & Palczewski, C. H. (2007). Communicating gender diversity: A critical approach . Thousand Oaks, CA: Sage.
Dindia, K., & Canary, D. J. (Eds.). (2006). Sex differences and similarities in communication (2nd edition). Mahwah, NJ: Erlbaum.
Dues, M., & Brown, M. (2004). Boxing Plato' s shadow: An introduction to the study of human communication . Boston, MA: McGraw Hill.
Gergen, K. J. (1994). Realities and relationships: Soundings in social construction . Cambridge, MA: Harvard University Press.
Gray, J. (1992). Men are from Mars, women are from Venus: A practical guide for improving communication and getting what you want in your relationship . New York: HarperCollins.
Gottman, J. M. (1994). What predicts divorce? The relationship between marital processes and marital outcomes . Hillsdale, NJ: Erlbaum.
Gudykunst, W. B. (2005). An anxiety/uncertainty management (AUM) theory of effective communication: Making the mesh of the net finer. In W. B. Gudykunst (Ed.), Theorizing about intercultural communication (pp. 281-322). Thousand Oaks, CA: Sage.
Keyton, J. (2011). Communicating research: Asking questions, finding answers (3rd ed). New York: McGraw Hill.
Kim, B. (n.d.) Social constructivism. In M. Orey (Ed.), Emerging perspectives on learning, teaching, and technology . Department of Educational Psychology and Instructional Technology, University of Georgia. Retrieved from http://projects.coe.uga.edu/epltt/index.php?title=Social_Constructivism
Kim, S. H. (2007). Max Weber. Stanford encyclopedia of philosophy . Retrieved from http://plato.stanford.edu/entries/weber/
Korn, C.J., Morreale, S.P., and Boileau, D.M. (2000). Defining the field: Revisiting the ACA 1995 definition of communication studies. Journal of the Association for Communication Administration , 29, 40-52.
Leeds-Hurwitz, W. (1990). Notes in the history of intercultural communication: The foreign service institute and the mandate for intercultural training. Quarterly Journal of Speech , 76, 262-281.
Merriam-Webster's Word of the Year 2006. (2006). Merriam-Webster Online. Retrieved from http://www.merriam-webster.com/info/06words.htm
Mitchell, K. S. (1996). Ever after: Reading the women who read (and re-write) romance. Theatre Topics 6.1 (1996) 51-69
The Modern Language Association. (2009). MLA handbook for writers of research papers (7th ed.). New York: The Modern Language Association of America.
National Communication Association. (2007). Communication scholarship and the humanities: A white paper sponsored by the National Communication Association. Retrieved from http://www.natcom.org/uploadedFiles/Resources_For/Policy_Makers/PDF-Communication_Scholarship_and_the_Humanities_A_White_Paper_by_NCA.pdf
Olson, L. N. (2010). The role of voice in the (re)construction of a battered woman's identity: An autoethnography of one woman's experiences of abuse. Women's Studies in Communication 27(1), 1-33. DOI: 10.1080/0749/409-2004.10162464
Stormer, N. (2003). To remember, to act, to forget: Tracing collective remembrance through "A Jury of Her Peers". Communication Studies , 54(4), 510-529.
Sunstein, C. (2001). Republic.com . Princeton, NJ: Princeton University Press.
Tannen, D. (1990). You just don't understand: Women and men in conversation . New York: William Morrow.
Tjaden, P. & Thoennes, N. (2000, July). Extent, nature and consequences of intimate partner violence: Findings from the National Violence Against Women Survey. (NCJ 181867). Washington, DC: National Institute of Justice/Centers for Disease Control and Prevention. Retrieved from http://www.ncjrs.gov/pdffiles1/nij/181867.pdf
Walker, L. (1979). The battered woman . New York: Harper & Row Publishers.
West, R., & Turner, L. H. (2010). Introducing communication theory: Analysis and application . Boston, MA: McGraw-Hill.
- Corpus ID: 60602524
Investigating Communication: An Introduction to Research Methods
- L. Frey , Carl H. Botan , Gary L. Kreps
- Published 5 November 1999
1,069 Citations
Communication research methods, teaching engaged research literacy: a description and assessment of the research ripped from the headlines project, methodological issues in advertising research: current status, shifts, and trends.
- Highly Influenced
Course info
Comm2201 introduction to communication research methods.
This course is an introduction to research as it relates to media and communication. Emphasis will be on the theory and practice of media and communication research. The course is intended to equip students with theoretical knowledge and practical skills needed to conduct communication research in a variety of professional and academic settings. Students who intend to pursue graduate studies in communication should be interested in this course. The course is really designed for the student who has no basic knowledge of research.
Thank you for visiting nature.com. You are using a browser version with limited support for CSS. To obtain the best experience, we recommend you use a more up to date browser (or turn off compatibility mode in Internet Explorer). In the meantime, to ensure continued support, we are displaying the site without styles and JavaScript.
- View all journals
- Explore content
- About the journal
- Publish with us
- Sign up for alerts
- Open access
- Published: 01 September 2024
Robust identification of perturbed cell types in single-cell RNA-seq data
- Phillip B. Nicol 1 ,
- Danielle Paulson 1 ,
- Gege Qian 2 ,
- X. Shirley Liu ORCID: orcid.org/0000-0003-4736-7339 3 ,
- Rafael Irizarry ORCID: orcid.org/0000-0002-3944-4309 3 &
- Avinash D. Sahu ORCID: orcid.org/0000-0002-2193-6276 4
Nature Communications volume 15 , Article number: 7610 ( 2024 ) Cite this article
Metrics details
- Bioinformatics
- Computational models
- Data mining
- Statistical methods
- Transcriptomics
Single-cell transcriptomics has emerged as a powerful tool for understanding how different cells contribute to disease progression by identifying cell types that change across diseases or conditions. However, detecting changing cell types is challenging due to individual-to-individual and cohort-to-cohort variability and naive approaches based on current computational tools lead to false positive findings. To address this, we propose a computational tool, scDist , based on a mixed-effects model that provides a statistically rigorous and computationally efficient approach for detecting transcriptomic differences. By accurately recapitulating known immune cell relationships and mitigating false positives induced by individual and cohort variation, we demonstrate that scDist outperforms current methods in both simulated and real datasets, even with limited sample sizes. Through the analysis of COVID-19 and immunotherapy datasets, scDist uncovers transcriptomic perturbations in dendritic cells, plasmacytoid dendritic cells, and FCER1G+NK cells, that provide new insights into disease mechanisms and treatment responses. As single-cell datasets continue to expand, our faster and statistically rigorous method offers a robust and versatile tool for a wide range of research and clinical applications, enabling the investigation of cellular perturbations with implications for human health and disease.
Introduction
The advent of single-cell technologies has enabled measuring transcriptomic profiles at single-cell resolution, paving the way for the identification of subsets of cells with transcriptomic profiles that differ across conditions. These cutting-edge technologies empower researchers and clinicians to study human cell types impacted by drug treatments, infections like SARS-CoV-2, or diseases like cancer. To conduct such studies, scientists must compare single-cell RNA-seq (scRNA-seq) data between two or more groups or conditions, such as infected versus non-infected 1 , responders versus non-responders to treatment 2 , or treatment versus control in controlled experiments.
Two related but distinct classes of approaches exist for comparing conditions in single-cell data: differential abundance prediction and differential state analysis 3 . Differential abundance approaches, such as DA-seq, Milo, and Meld 4 , 5 , 6 , 7 , focus on identifying cell types with varying proportions between conditions. In contrast, differential state analysis seeks to detect predefined cell types with distinct transcriptomic profiles between conditions. In this study, we focus on the problem of differential state analysis.
Past differential state studies have relied on manual approaches involving visually inspecting data summaries to detect differences in scRNA data. Specifically, cells were clustered based on gene expression data and visualized using uniform manifold approximation (UMAP) 8 . Cell types that appeared separated between the two conditions were identified as different 1 . Another common approach is to use the number of differentially expressed genes (DEGs) as a metric for transcriptomic perturbation. However, as noted by ref. 9 , the number of DEGs depends on the chosen significance level and can be confounded by the number of cells per cell type because this influences the power of the corresponding statistical test. Additionally, this approach does not distinguish between genes with large and small (yet significant) effect sizes.
To overcome these limitations, Augur 9 uses a machine learning approach to quantify the cell-type specific separation between the two conditions. Specifically, Augur trains a classifier to predict condition labels from the expression data and then uses the area under the receiver operating characteristic (AUC) as a metric to rank cell types by their condition difference. However, Augur does not account for individual-to-individual variability (or pseudoreplication 10 ), which we show can confound the rankings of perturbed cell types.
In this study, we develop a statistical approach that quantifies transcriptomic shifts by estimating the distance (in gene expression space) between the condition means. This method, which we call scDist , introduces an interpretable metric for comparing different cell types while accounting for individual-to-individual and technical variability in scRNA-seq data using linear mixed-effect models. Furthermore, because transcriptomic profiles are high-dimensional, we develop an approximation for the between-group differences, based on a low-dimensional embedding, which results in a computationally convenient implementation that is substantially faster than Augur . We demonstrate the benefits using a COVID-19 dataset, showing that scDist can recover biologically relevant between-group differences while also controlling for sample-level variability. Furthermore, we demonstrated the utility of the scDist by jointly inferring information from five single-cell immunotherapy cohorts, revealing significant differences in a subpopulation of NK cells between immunotherapy responders and non-responders, which we validated in bulk transcriptomes from 789 patients. These results highlight the importance of accounting for individual-to-individual and technical variability for robust inference from single-cell data.
Not accounting for individual-to-individual variability leads to false positives
We used blood scRNA-seq from six healthy controls 1 (see Table 1 ), and randomly divided them into two groups of three, generating a negative control dataset in which no cell type should be detected as being different. We then applied Augur to these data. This procedure was repeated 20 times. Augur falsely identified several cell types as perturbed (Fig. 1 A). Augur quantifies differences between conditions with an AUC summary statistic, related to the amount of transcriptional separation between the two groups (AUC = 0.5 represents no difference). Across the 20 negative control repeats, 93% of the AUCs (across all cell typess) were >0.5, and red blood cells (RBCs) were identified as perturbed in all 20 trials (Fig. 1 A). This false positive result was in part due to high across-individual variability in cell types such as RBCs (Fig. 1 B).
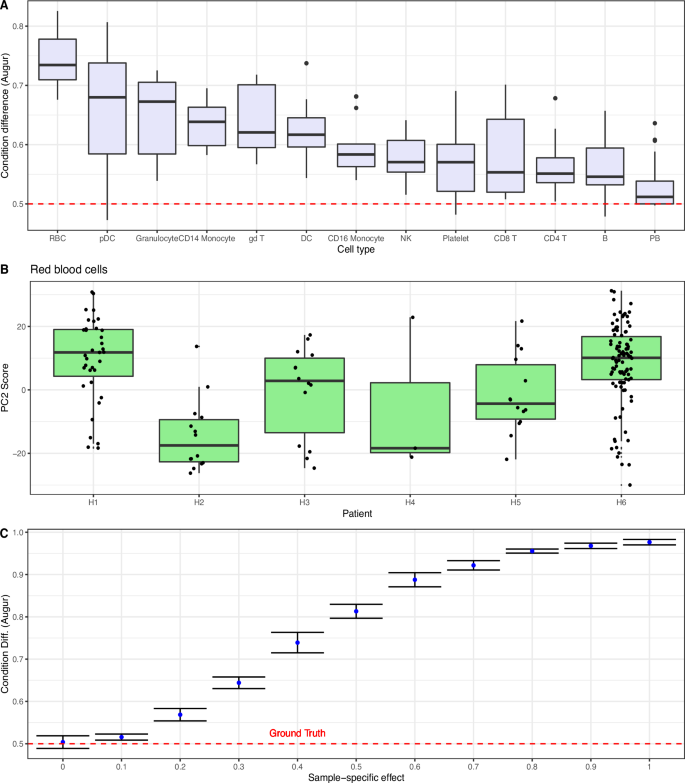
A AUCs achieved by Augur on 20 random partitions of healthy controls ( n = 6 total patients divided randomly into two groups of 3), with no expected cell type differences (dashed red line indicates the null value of 0.5). B Boxplot depicting the second PC score for red blood cells from healthy individuals, highlighting high across-individual variability (each box represents a different individual). The boxplots display the median and first/third quartiles. C AUCs achieved by Augur on simulated scRNA-seq data (10 individuals, 50 cells per individual) with no condition differences but varying patient-level variability (dashed red line indicates the ground truth value of no condition difference, AUC 0.5), illustrating the influence of individual-to-individual variability on false positive predictions. Points and error bands represent the mean ±1 SD. Source data are provided as a Source Data file.
We confirmed that individual-to-individual variation underlies false positive predictions made by Augur using a simulation. We generated simulated scRNA-seq data with no condition-level difference and varying patient-level variability (Methods). As patient-level variability increased, differences estimated by Augur also increased, converging to the maximum possible AUC of 1 (Fig. 1 C): Augur falsely interpreted individual-to-individual variability as differences between conditions.
Augur recommends that unwanted variability should be removed in a pre-processing step using batch correction software. We applied Harmony 11 to the same dataset 1 , treating each patient as a batch. We then applied Augur to the resulting batch corrected PC scores and found that several cell types still had AUCs significantly above the null value of 0.5 (Fig. S1 a). On simulated data, batch correction as a pre-processing step also leads to confounding individual-to-individual variability as condition difference (Fig. S1 b).
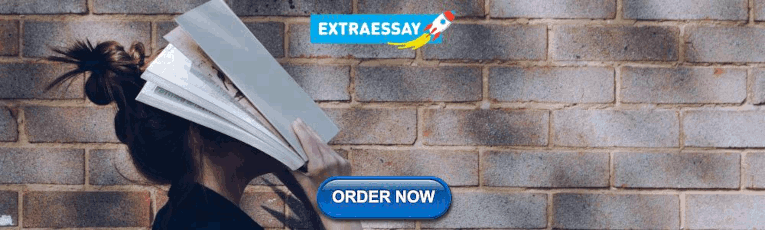
A model-based distance metric controls for false positives
To account for individual-to-individual variability, we modeled the vector of normalized counts with a linear mixed-effects model. Mixed models have previously been shown to be successful at adjusting for this source of variability 10 . Specifically, for a given cell type, let z i j be a length G vector of normalized counts for cell i and sample j ( G is the number of genes). We then model
where α is a vector with entries α g representing the baseline expression for gene g , x j is a binary indicator that is 0 if individual j is in the reference condition, and 1 if in the alternative condition, β is a vector with entries β g representing the difference between condition means for gene g , ω j is a random effect that represents the differences between individuals, and ε i j is a random vector (of length G ) that accounts for other sources of variability. We assume that \({{{\boldsymbol{\omega }}}}_{j}\mathop{ \sim }\limits^{{{\rm{i}}}.{{\rm{i}}}.{{\rm{d}}}}{{\mathcal{N}}}(0,{\tau }^{2}I)\) , \({{{\boldsymbol{\varepsilon }}}}_{ij}\mathop{ \sim }\limits^{{{\rm{i}}}.{{\rm{i}}}.{{\rm{d}}}}{{\mathcal{N}}}(0,{\sigma }^{2}I)\) , and that the ω j and ε i j are independent of each other.
To obtain normalized counts, we recommend defining z i j to be the vector of Pearson residuals obtained from fitting a Poisson or negative binomial GLM 12 , the normalization procedure is implemented in the scTransform function 13 . However, our proposed approach can be used with other normalization methods for which the model is appropriate.
Note that in model ( 18 ), the means for the two conditions are α and α + β , respectively. Therefore, we quantify the difference in expression profile by taking the 2 − norm of the vector β :
Here, D can be interpreted as the Euclidean distance between condition means (Fig. 2 A).
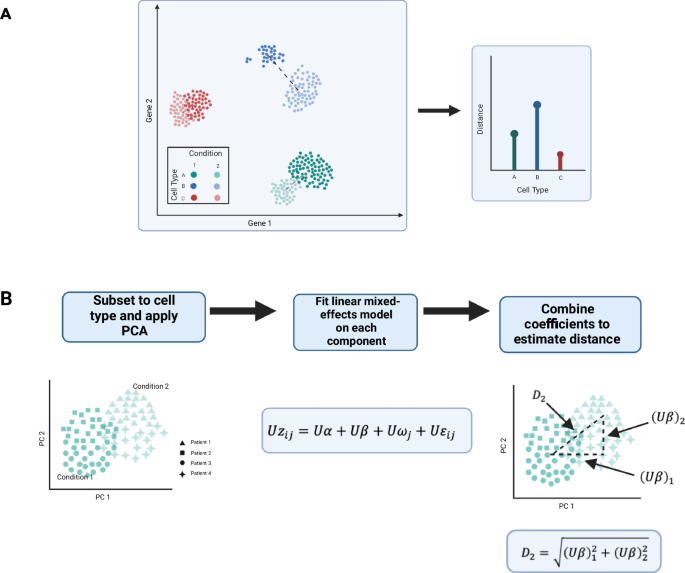
A scDist estimates the distance between condition means in high-dimensional gene expression space for each cell type. B To improve efficiency, scDist calculates the distance in a low-dimensional embedding space (derived from PCA) and employs a linear mixed-effects model to account for sample-level and other technical variability. This figure is created with Biorender.com, was released under a Creative Commons Attribution-NonCommercial-NoDerivs 4.0 International license.
Because we expected the vector of condition differences β to be sparse, we improved computational efficiency by approximating D with a singular value decomposition to find a K × G matrix U , with K much smaller than G , and
With this approximation in place, we fitted model equation ( 18 ) by replacing z i j with U z i j to obtain estimates of ( U β ) k . A challenge with estimating D K is that the maximum likelihood estimator can have a significant upward bias when the number of patients is small (as is typically the case). For this reason, we employed a post-hoc Bayesian procedure to shrink \({{(U{\boldsymbol{\beta}} )}_{k}^{2}}\) towards zero and compute a posterior distribution of D K 14 . We also provided a statistical test for the null hypothesis that D K = 0. We refer to the resulting procedure as scDist (Fig. 2 B). Technical details are provided in Methods.
We applied scDist to the negative control dataset based on blood scRNA-seq from six healthy used to show the large number of false positives reported by Augur (Fig. 1 ) and found that the false positive rate was controlled (Fig. 3 A, B). We then applied scDist to the data from the simulation study and found that, unlike Augur , the resulting distance estimate does not grow with individual-to-individual variability (Fig. 3 C). scDist also accurately estimated distances on fully simulated data (Fig. S2 ).
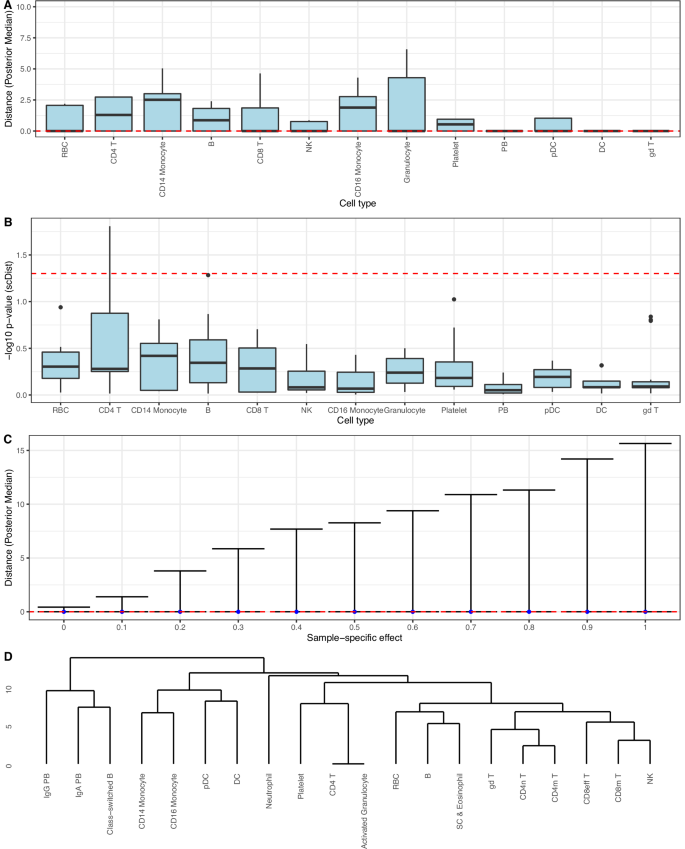
A Reanalysis of the data from Fig. 1 A using distances calculated with scDist ; the dashed red line represents ground truth value of 0. B As in A , but for p-values computed with scDist ; values above the dashed red line represent p < 0.05. C Null simulation from Fig. 1 C reanalyzed using distances calculated by scDist ; the dashed red line represents the ground truth value of 0. Points and error bands represent the mean ±1 SD. D Dendrogram generated by hierarchical clustering based on distances between pairs of cell types estimated by scDist . In all panels the boxplots display median and first/third quartiles. Source data are provided as a Source Data file.
The Euclidean distance D measures perturbation by taking the sum of squared differences across all genes. To show that this measure is biologically meaningful, we applied scDist to obtain estimated distances between pairs of known cell types in the above dataset and then applied hierarchical clustering to these distances. The resulting clustering is consistent with known relationships driven by cell lineages (Fig. 3 D). Specifically, Lymphoid cell types T and NK cells clustered together, while B cells were further apart, and Myeloid cell types DC, monocytes, and neutrophils were close to each other.
Though the scDist distance D assigns each gene an equal weight (unweighted), scDist includes an option to assign different weights w g to each gene (Methods). Weighting could be useful in situations where certain genes are known to contribute more to specific phenotypes. We conducted a simulation to study the impact of using the weighted distance. These simulations show that when a priori information is available, using the correct weighting leads to a slightly better estimation of the distance. However, incorrect weighting leads to significantly worse estimation compared to the unweighted distance (Fig. S3 ). Therefore, the unweighted distance is recommended unless strong a priori information is available.
Challenges in cell type annotations are expected to impact scDist ’s interpretation, much like it does for other methods reliant on a priori cell type annotation such as 3 , 9 . Our simulations (see Methods), reveal scDist ’s vulnerability to false-negatives when annotations are confounded by condition- or patient-specific factors. However, when clusters are annotated using data where such differences have been removed, scDist’s predictions become more reliable (Fig. S23 ). Thus, we recommend removing these confounders before annotation. As potential issues could occur when the inter-condition distance exceeds the inter-cell-type distance, scDist provides a diagnostic plot (Fig. S6 ) to compare these two distances. scDist also incorporates an additional diagnostic feature (Fig. S24 ) to identify annotation issues, utilizing a cell-type tree to evaluate cell relationships at different hierarchical levels. Inconsistencies in scDist ’s output signal potential clustering or annotation errors.
Comparison to counting the number of DEGs
We also compared scDist to the approach of counting the number of differentially expressed genes (nDEG) on pseudobulk samples 3 . Given that the statistical power to detect DEGs is heavily reliant on sample size, we hypothesized that nDEG could become a misleading measure of perturbation in single-cell data with a large variance in the number of cells per cell type. To demonstrate this, we applied both methods to resampled COVID-19 data 1 where the number of cells per cell type was artificially varied between 100 and 10,000. nDEG was highly confounded by the number of cells (Fig. 4 A), whereas the scDist distance remained relatively constant despite the varying number of cells (Fig. 4 B). When the number of subsampled cells is small, the ranking of cell types (by perturbation) was preserved by scDist but not by nDEG (Fig. S5 a–c). Additionally, scDist was over 60 times faster than nDEG since the latter requires testing all G genes as opposed to K ≪ G PCs (Fig. S4 ).
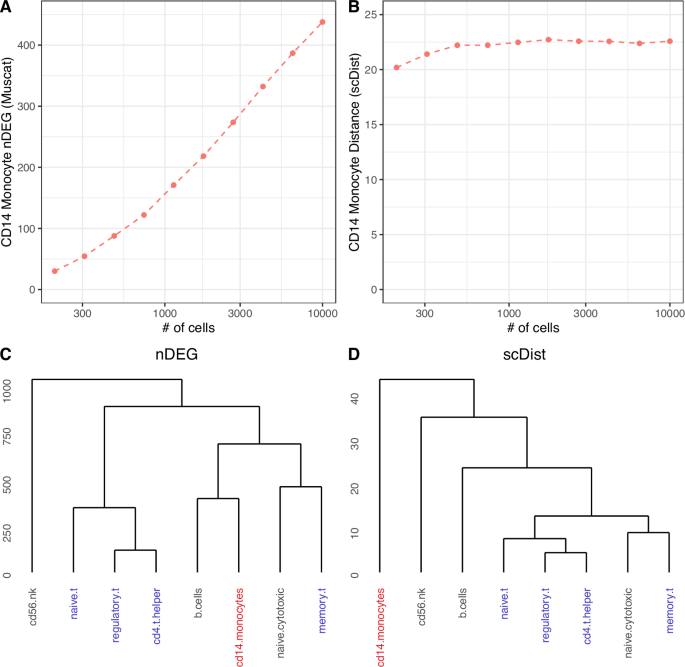
A Sampling with replacement from the COVID-19 dataset 1 to create datasets with a fixed number of cells per cell type, and then counting the number of differentially expressed genes (nDEG) for the CD14 monocytes. B Repeating the previous analysis with the scDist distance. C Comparing all pairs of cell types on the downsampled 15 dataset and applying hierarchical clustering to the pairwise perturbations. Leaves corresponding to T cells are colored blue while the leaf corresponding to monocytes is colored red. D The same analysis using the scDist distances. Source data are provided as a Source Data file.
An additional limitation of nDEG is that it does not account for the magnitude of the differential expression. We illustrated this with a simple simulation that shows the number of DEGs between two cell types can be the same (or less) despite a larger transcriptomic perturbation in gene expression space (Fig. S7 a, b). To demonstrate this on real data, we considered a dataset consisting of eight sorted immune cell types (originally from ref. 15 and combined by ref. 16 ) where scDist and nDEG were applied to all pairs of cell types, and the perturbation estimates were visualized using hierarchical clustering. Although both nDEG and scDist performed well when the sample size was balanced across cell types (Fig. S8 ), nDEG provided inconsistent results when the CD14 Monocytes were downsampled to create a heterogeneous cell type size distribution. Specifically, scDist produced the expected result of clustering the T cells together, whereas nDEG places the Monocytes in the same cluster as B and T cells (Fig. 4 C, D) despite the fact that these belong to different lineages. Thus by taking into account the magnitude of the differential expression, scDist is able to produce results more in line with known biology.
We also considered varying the number of patients on simulated data with a known ground truth. Again, the nDEG (computed using a mixed model, as recommended by ref. 10 ) increases as the number of patients increases, whereas scDist remains relatively stable (Fig. S9 a). Moreover, the correlation between the ground truth perturbation and scDist increases as the number of patients increases (Fig. S9 b). Augur was also sensitive to the number of samples and had a lower correlation with the ground truth than both nDEG and scDist .
scDist detects cell types that are different in COVID-19 patient compared to controls
We applied scDist to a large COVID-19 dataset 17 consisting of 1.4 million cells of 64 types from 284 PBMC samples from 196 individuals consisting of 171 COVID-19 patients and 25 healthy donors. The large number of samples of this dataset permitted further evaluation of our approach using real data rather than simulations. Specifically, we defined true distances between the two groups by computing the sum of squared log fold changes (across all genes) on the entire dataset and then estimated the distance on random samples of five cases versus five controls. Because Augur does not estimate distances explicitly, we assessed the two methods’ ability to accurately recapitulate the ranking of cell types based on established ground truth distances. We found that scDist recovers the rankings better than Augur (Fig. 5 A, S10 ). When the size of the subsample is increased to 15 patients per condition, the accuracy of scDist to recover the ground truth rank and distance improves further (Fig. S25 ).
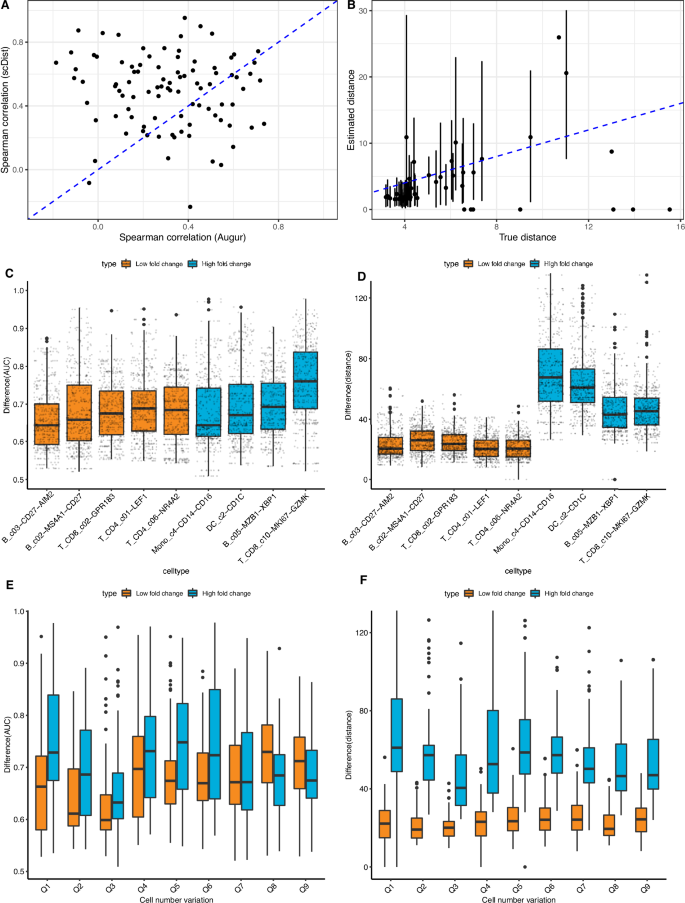
A Correlation between estimated ranks (based on subsamples of 5 cases and 5 controls) and true ranks for each method, with points above the diagonal line indicate better agreement of scDist with the true ranking. B Plot of true distance vs. distances estimated with scDist (dashed line represents y = x ). Poins and error bars represent mean, and 5/95th percentile. C AUC values achieved by Augur , where color represents likely true (blue) or false (orange) positive cell types. D Same as C , but for distances estimated with scDist . E AUC values achieved by Augur against the cell number variation in subsampled-datasets (of false positive cell types). F Same as E , but for distances estimated with scDist . In all boxplots the median and first/third quartiles are reported. For C – E , 1000 random subsamples were used. Source data are provided as a Source Data file.
To evaluate scDist ’s accuracy further, we defined a new ground truth using the entire COVID-19 dataset, consisting two groups: four cell types with differences between groups (true positives) and five cell types without differences (false positives) (Fig. S11 , Methods). We generated 1000 random samples with only five individuals per cell type and estimated group differences using both Augur and scDist . Augur failed to accurately separate the two groups (Fig. 5 C); median difference estimates of all true positive cell types, except MK167+ CD8+T, were lower than median estimates of all true negative cell types (Fig. 5 C). In contrast, scDist showed a separation between scDist estimates between the two groups (Fig. 5 D).
Single-cell data can also exhibit dramatic sample-specific variation in the number of cells of specific cell types. This imbalance can arise from differences in collection strategies, biospecimen quality, and technical effects, and can impact the reliability of methods that do not account for sample-to-sample or individual-to-individual variation. We measured the variation in cell numbers within samples by calculating the ratio of the largest sample’s cell count to the total cell counts across all samples (Methods). Augur ’s predictions were negatively impacted by this cell number variation (Figs. 5 E, S12 ), indicating its increased susceptibility to false positives when sample-specific cell number variation was present (Fig. 1 C). In contrast, scDist ’s estimates were robust to sample-specific cell number variation in single-cell data (Fig. 5 F).
To further demonstrate the advantage of statistical inference in the presence of individual-to-individual variation, we analyzed the smaller COVID-19 dataset 1 with only 13 samples. The original study 1 discovered differences between cases and controls in CD14+ monocytes through extensive manual inspection. scDist identified this same group as the most significantly perturbed cell type. scDist also identified two cell types not considered in the original study, dendritic cells (DCs) and plasmacytoid dendritic cells (pDCs) ( p = 0.01 and p = 0.04, Fig. S13 a), although pDC did not remain significant after adjusting for multiple testing. We note that DCs induce anti-viral innate and adaptive responses through antigen presentation 18 . Our finding was consistent with studies reporting that DCs and pDCs are perturbed by COVID-19 infection 19 , 20 . In contrast, Augur identified RBCs, not CD14+ monocytes, as the most perturbed cell type (Fig. S14 ). Omitting the patient with the most RBCs dropped the perturbation between infected and control cases estimated by Augur for RBCs markedly (Fig. S14 ), further suggesting that Augur predictions are clouded by patient-level variability.
scDist enables the identification of genes underlying cell-specific across-condition differences
To identify transcriptomic alteration, scDist assigns an importance score to each gene based on its contribution to the overall perturbation (Methods). We assessed this importance score for CD14+ monocytes in small COVID-19 datasets. In this cell type, scDist assigned the highest importance score to genes S100 calcium-binding protein A8 ( S100A8 ) and S100 calcium-binding protein A9 ( S100A9 ) ( p < 10 −3 , Fig. S13 b). These genes are canonical markers of inflammation 21 that are upregulated during cytokine storm. Since patients with severe COVID-19 infections often experience cytokine storms, the result suggests that S100A8/A9 upregulation in CD14+ monocyte could be a marker of the cytokine storm 22 . These two genes were reported to be upregulated in COVID-19 patients in the study of 284 samples 17 .
scDist identifies transcriptomic alterations associated with immunotherapy response
To demonstrate the real-world impact of scDist , we applied it to four published dataset used to understand patient responses to cancer immunotherapy in head and neck, bladder, and skin cancer patients, respectively 2 , 23 , 24 , 25 . We found that each individual dataset was underpowered to detect differences between responders and non-responders (Fig. S15 ). To potentially increase power, we combined the data from all cohorts (Fig. 6 A). However, we found that analyzing the combined data without accounting for cohort-specific variations led to false positives. For example, responder-non-responder differences estimated by Augur were highly correlated between pre- and post-treatments (Fig. 6 B), suggesting a confounding effect of cohort-specific variations. Furthermore, Augur predicted that most cell types were altered in both pre-treatment and post-treatment samples (AUC > 0.5 for 41 in pre-treatment and 44 in post-treatment out of a total of 49 cell types), which is potentially due to the confounding effect of cohort-specific variations.
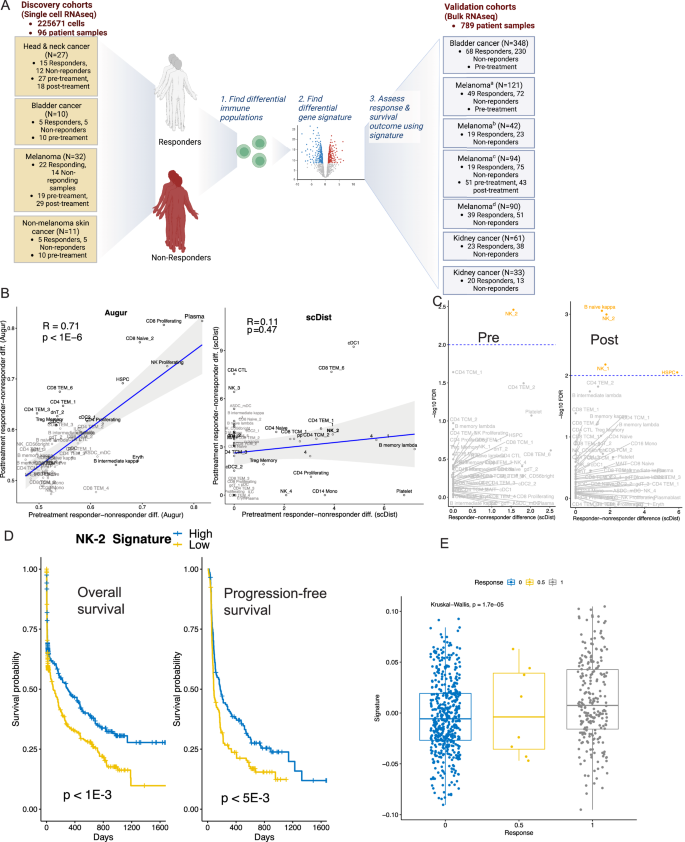
A Study design: discovery cohorts of four scRNA cohorts (cited in order as shown 2 , 23 , 24 , 25 ) identify cell-type-specific differences and a differential gene signature between responders and non-responders. This signature was evaluated in validation cohorts of six bulk RNA-seq cohorts (cited in order as shown 32 , 33 , 34 , 35 , 36 , 37 , 38 ). B Pre-treatment and post-treatment sample differences were estimated using Augur and scDist (Spearman correlation is reported on the plot). The error bars represent 95% confidence interval for the fitted linear regression line. C Significance of the estimated differences ( scDist ). D Kaplan–Meier plots display the survival differences in anti-PD-1 therapy patients, categorized by low-risk and high-risk groups using the median value of the NK-2 signature value; overall and progression-free survival is shown. E NK-2 signature levels in non-responders, partial-responders, and responders (Bulk RNA-seq cohorts). The boxplots report the median and first/third quartiles. A created with BioRender.com, released under a Creative Commons Attribution-NonCommercial-NoDerivs 4.0 International license. Source data are provided as a Source Data file.
To account for cohort-specific variations, we ran scDist including an explanatory variable to the model ( 18 ) to account for cohort effects. With this approach, distance estimates were not correlated significantly between pre- and post-treatment (Fig. 6 B). Removal of these variables re-established correlation (Fig. S16 ). scDist predicted CD4-T and CD8-T altered pre-treatment (Fig. S17 a), while NK, CD8-T, and B cells altered post-treatment (Fig. S17 b). Analysis of subtypes revealed FCER1G+NK cells (NK-2) were changed in both pre-treatment and post-treatment samples (Fig. 6 C). To validate this finding, we generated an NK-2 signature differential between responders and non-responders (Fig. S18 Methods) and evaluated these signatures in bulk RNA-seq immunotherapy cohorts, composing 789 patient samples (Fig. 6 A). We scored each of the 789 patient samples using the NK-2 differential signature (Methods). The NK-2 signature scores were significantly associated with overall and progression-free survival (Fig. 6 D) as well as radiology-based response (Fig. 6 E). We similarly evaluated the top Augur prediction. Differential signature from plasma, the top predicted cell type by Augur , did not show an association with the response or survival outcomes in 789 bulk transcriptomes (Fig. S19 , Methods).
scDist is computationally efficient
A key strength of the linear modeling framework used by scDist is that it is efficient on large datasets. For instance, on the COVID-19 dataset with 13 samples 1 , scDist completed the analysis in around 50 seconds, while Augur required 5 minutes. To better understand how runtime depends on the number of cells, we applied both methods to subsamples of the dataset that varied in size and observed that scDist was, on average, five-fold faster (Fig. S20 ). scDist is also capable of scaling to millions of cells. On simulated data, scDist required approximately 10 minutes to fit a dataset with 1,000,000 cells (Fig. S21 ). We also tested the sensitivity of scDist to the number of PCs used by comparing D K for various values of K . We observed that the estimated distances stabilize as K increases (Fig. S22 ), justifying K = 20 as a reasonable choice for most datasets.
The identification of cell types influenced by infections, treatments, or biological conditions is crucial for understanding their impact on human health and disease. We present scDist , a statistically rigorous and computationally fast method for detecting cell-type specific differences across multiple groups or conditions. By using a mixed-effects model, scDist estimates the difference between groups while quantifying the statistical uncertainty due to individual-to-individual variation and other sources of variability. We validated scDist through the unbiased recapitulation of known relationships between immune cells and demonstrated its effectiveness in mitigating false positives from patient-level and technical variations in both simulated and real datasets. Notably, scDist facilitates biological discoveries from scRNA cohorts, even when the number of individuals is limited, a common occurrence in human scRNA-seq datasets. We also pointed out how the detection of cell-type specific differences can be obscured by batch effects or other confounders and how the linear model used by our approach permits accounting for these.
Since the same expression data is used for annotation and by scDist , there are potential issues associated with “double dipping.” Our simulation highlighted this issue by showing that condition-specific effects can result in over-clustering and downward bias in the estimated distances (Methods, Fig. S23 ). Users can avoid these false negatives by using annotation approaches that can control for patient and condition-specific effects. scDist provides two diagnostic tools to help users identify potential issues in their annotation (Figs. S24 and S6 . Despite this, significant errors in clustering and annotation could cause unavoidable bias in scDist , and thus designing a cluster-free extension of scDist is an area for future work. scDist also provides a diagnostic tool that estimates distances at multiple resolutions to help users identify potential issues in their annotation (Fig. S24 ). Another point of sensitivity for scDist is the choice of the number of principal components used to estimate the distance. Although in practice we observed that the distance estimate is stable as the number of PCs varies between 20 and 50 (Fig. S22 ), an adaptive approach for selecting K could improve performance and maximize power. Finally, although Pearson residual-based normalized counts 12 , 13 is recommended input for scDist , if the data available was normalized by another, sub-optimal, approach, scDist ’s performances could be affected. A future version could adapt the model and estimation procedure so that scDist can be directly applied to the counts, and avoid potential problems introduced by normalization.
We believe that scDist will have extensive utility, as the comparison of single-cell experiments between groups is a common task across a range of research and clinical applications. In this study, we have focused on examining discrete phenotypes, such as infected versus non-infected (in COVID-19 studies) and responders vs. non-responders to checkpoint inhibitors. However, the versatility of our framework allows for extension to experiments involving continuous phenotypes or conditions, such as height, survival, and exposure levels, to name a few. As single-cell datasets continue to grow in size and complexity, scDist will enable rigorous and reliable insights into cellular perturbations with implications for human health and disease.
Normalization
Our method takes as input a normalized count matrix (with corresponding cell type annotations). We recommend using scTransform 13 to normalize, although the method is compatible with any normalization approach. Let y i j g be the UMI counts for gene 1 ≤ g ≤ G in cell i from sample j . scTransform fits the following model:
where r i j is the total number of UMI counts for the particular cell. The normalized counts are given by the Pearson residuals of the above model:
Distance in normalized expression space
In this section, we describe the inferential procedure of scDist for cases without additional covariates. However, the procedure can be generalized to the full model ( 18 ) with arbitrary covariates (design matrix) incorporating random and fixed effects, as well as nested-effect mixed models. For a given cell type, we model the G -dimensional vector of normalized counts as
where \({\boldsymbol{\alpha}},{\boldsymbol{\beta}} \in {{\mathbb{R}}}^{G}\) , x i j is a binary indicator of condition, \({\boldsymbol{\omega }}_{j} \sim {{\mathcal{N}}}(0,{\tau }^{2}{I}_{G})\) , and \({\boldsymbol{\varepsilon }}_{ij} \sim {{\mathcal{N}}}(0,{\sigma }^{2}{I}_{G})\) . The quantity of interest is the Euclidean distance between condition means α and α + β :
If \(U\in {{\mathbb{R}}}^{G\times G}\) is an orthonormal matrix, we can apply U to equation ( 6 ) to obtain the transformed model :
Since U is orthogonal, U ω j and U ε i j still have spherical normal distributions. We also have that
This means that the distance in the transformed model is the same as in the original model. As mentioned earlier, our goal is to find U such that
with K ≪ G .
Let \(Z\in {{\mathbb{R}}}^{n\times G}\) be the matrix with rows z i j (where n is the total number of cells). Intuitively, we want to choose a U such that the projection of z i j onto the first K rows of U ( \({u}_{1},\ldots,{u}_{K}\in {{\mathbb{R}}}^{G}\) ) minimizes the reconstruction error
where \(\mu \in {{\mathbb{R}}}^{G}\) is a shift vector and \(({v}_{ik})\in {{\mathbb{R}}}^{n\times K}\) is a matrix of coefficients. It can be shown that the PCA of Z yields the (orthornormal) u 1 , …, u K that minimizes this reconstruction error 26 .
Given an estimator \(\widehat{{(U{\boldsymbol{\beta}} )}_{k}}\) of ( U β ) k , a naive estimator of D K is given by taking the square root of the sum of squared estimates:
However, this estimator can have significant upward bias due to sampling variability. For instance, even if the true distance is 0, \(\widehat{{(U\beta )}_{k}}\) is unlikely to be exactly zero, and that noise becomes strictly positive when squaring.
To account for this, we apply a post-hoc Bayesian procedure to the \({\widehat{U\beta }}_{k}\) to shrink them towards zero before computing the sum of squares. In particular, we adopt the spike slab model of 14
where \({{\rm{Var}}}[\widehat{{(U{\boldsymbol{\beta}} )}_{k}}]\) is the variance of the estimator \(\widehat{{(U{\boldsymbol{\beta}} )}_{k}}\) , δ 0 is a point mass at 0, and π 0 , π 1 , … π T are mixing weights (that is, they are non-negative and sum to 1). 14 provides a fast empirical Bayes approach to estimate the mixing weights and obtain posterior samples of ( U β ) k . Then samples from the posterior of D K are obtained by applying the formula ( 12 ) to the posterior samples of ( U β ) k . We then summarize the posterior distribution by reporting the median and other quantiles. Advantage of this particular specification is that the amount of shrinkage depends on the uncertainty in the initial estimate of ( U β ) k .
We use the following procedure to obtain \({\widehat{U\beta }}_{k}\) :
Use the matrix of PCA loadings as a plug in estimator for U . Then U z i j is the vector of PC scores for cell i in sample j .
Estimate ( U β ) k by using lme4 27 to fit the model ( 6 ) using the PC scores corresponding to the k -th loading (i.e., each dimension is fit independently).
Note that only the first K rows of U need to be stored.
We are particularly interested in testing the null hypothesis of D K = 0 against the alternative D d > 0. Because the null hypothesis corresponds to ( U β ) k = 0 for all 1 ≤ k ≤ d , we can use the sum of individual Wald statistics as our test statistic:
Under the null hypothesis that ( U β ) k = 0, W k can be approximated by a \({F}_{{\nu }_{k},1}\) distribution. ν k is estimated using Satterthwaite’s approximation in lmerTest . This implies that
under the null. Moreover, the W k are independent because we have assumed that covariance matrices for the sample and cell-level noise are multiples of the identity. Equation ( 16 ) is not a known distribution but quantiles can be approximated using Monte Carlo samples. To make this precise, let W 1 , …, W M be draws from equation ( 16 ), where M = 10 5 and let W * be the value of equation ( 15 ) (i.e., the actual test statistic). Then the empirical p -value 28 is computed as
Controlling for additional covariates
Because scDist is based on a linear model, it is straightforward to control for additional covariates such as age or sex of a patient in the analysis. In particular, model ( 18 ) can be replaced with
where \({w}_{ijk}\in {\mathbb{R}}\) is the value of the k th covariate for cell i in sample j and \({\boldsymbol{\gamma }}_{k}\in {{\mathbb{R}}}^{G}\) is the corresponding gene-specific effect corresponding to the k th covariate.
Choosing the number of principal components
An important choice in scDist is the number of principal components d . If d is chosen too small, then estimation accuracy may suffer as the first few PCs may not capture enough of the distance. On the other hand, if d is chosen too large then the power may suffer as a majority of the PCs will simply be capturing random noise (and adding to degrees of freedom to the Wald statistic). Moreover, it is important that d is chosen a priori, as choosing the d that produces the lowest p values is akin to p -hacking.
If the model is correctly specified then it is reasonable to choose d = J − 1, where J is the number of samples (or patients). To see why, notice that the mean expression in sample 1 ≤ j ≤ J is
In particular, the J sample means lie on a ( J − 1)-dimensional subspace in \({{\mathbb{R}}}^{G}\) . Under the assumption that the condition difference and sample-level variability is larger than the error variance σ 2 , we should expect that the first J − 1 PC vectors capture all of the variance due to differences in sample means.
In practice, however, the model can not be expected to be correctly specified. For this reason, we find that d = 20 is a reasonable choice when the number of samples is small (as is usually the case in scRNA-seq) and d = 50 for datasets with a large number of samples. This is line with other single-cell methods, where the number of PCs retained is usually between 20 and 50.
Cell type annotation and “double dipping”
scDist takes as input an annotated list of cells. A common approach to annotate cells is to cluster based on gene expression. Since scDist also uses the gene expression data to measure the condition difference there are concerns associated with “double-dipping” or using the data twice. In particular, if the condition difference is very large and all of the data is used to cluster it is possible that the cells in the two conditions would be assigned to different clusters. In this case scDist would be unable to estimate the inter-condition distance, leading to a false negative. In other words, the issue of double dipping could cause scDist to be more conservative. Note that the opposite problem occurs when performing differential expression between two estimated clusters; in this case, the p -values corresponding to genes will be anti-conservative 29 .
To illustrate, we simulated a normalized count matrix with 4000 cells and 1000 genes in such a way that there are two “true” cell types and a true condition distance of 4 for both cell types (Fig. S23 a). To cluster (annotate) the cells, we applied k -means with various choices of k and compared results by taking the median inter-condition distance across all clusters. As the number of clusters increases, the median distance decays towards 0, which demonstrates that scDist can produce false negatives when the data is over-clustered (Fig. S23 b). To avoid this issue, one possible approach is to begin by clustering the data for only one condition and then to assign cells in the other condition by finding the nearest centroid in the existing clusters. When applied to the simulated data this approach is able to correctly estimate the condition distance even when the number of clusters k is larger than the true value.
On real data, one approach to identify possible over-clustering is to apply scDist at various cluster resolutions. We used the expression data from the small COVID-19 data 1 to construct a tree \({{\mathcal{T}}}\) with leaf nodes corresponding to the cell types in the original annotation provided by the authors (Fig. S24 , see Appendix A for a description of how the tree is estimated). At each internal node \(v\in {{\mathcal{T}}}\) , we applied scDist to the cluster containing all children of v . We can then visualize the estimated distances by plotting the tree (Fig. S24 ). Situations where the child nodes have a small distance but the parent node has a large distance could be indicative of over-clustering. For example, PB cells are almost exclusiviely found in cases (1977 cells in cases and 86 cells in controls), suggesting that it is reasonable to consider PB and B cells as a single-cell type when applying scDist .
Feature importance
To better understand the genes that drive the observed difference in the CD14+ monocytes, we define a gene importance score . For 1 ≤ k ≤ d and 1 ≤ g ≤ G , the k -th importance score for gene g is ∣ U k g ∣ β g . In other words, the importance score is the absolute value of the gene’s k -th PC loading times its expression difference between the two conditions. Note that the gene importance score is 0 if and only if β g = 0 or U k g = 0. Since the U k g are fixed and known, significance can be assigned to the gene importance score using the differential expression method used to estimate β g .
Simulated single-cell data
We test the method on data generated from model equation ( 6 ). To ensure that the “true” distance is D , we use the R package uniformly 30 to draw β from the surface of the sphere of radius D in \({{\mathbb{R}}}^{G}\) . The data in Figs. 1 C and 3 C are obtained by setting β = 0 and σ 2 = 1 and varying τ 2 between 0 and 1.
Weighted distance
By default, scDist uses the Euclidean distance D which treats each gene equally. In cases where a priori information is available about the relevance of each gene, scDist provides the option to estimate a weighted distance D w , where \(w\in {{\mathbb{R}}}^{G}\) has non-negative components and
The weighted distance can be written in matrix form by letting \(W\in {{\mathbb{R}}}^{G\times G}\) be a diagonal matrix with W g g = w g , so that
Thus, the weighted distance can be estimated by instead considered the transformed model where \(U\sqrt{W}\) is applied to each z i j . After this different transformed model is obtained, estimation and inference of D w proceeds in exactly the same way as the unweighted case.
To test the accuracy of the weighted distance estimate, we considered a simulation where each gene had only a 10% chance of having β g ≠ 0 (otherwise \({\beta }_{g} \sim {{\mathcal{N}}}(0,1)\) ). We then considered three scenarios: w g = 1 if β g ≠ 0 and w g = 0 otherwise (correct weighting), w g = 1 for all g (unweighted), and w g = 1 randomly with probability 0.1 (incorrect weights). We then quantified the performance by taking the absolute value of the error between \({\sum }_{g}{\beta }_{g}^{2}\) and the estimated distance. Figure S3 shows that correct weighting slightly outperforms unweighted scDist but random weights are significantly worse. Thus, the unweighted version of scDist should be preferred unless strong a priori information is available.
Robustness to model misspecification
The scDist model assumes that the cell-specific variance σ 2 and sample-specific variance τ 2 are shared across genes. The purpose of this assumption is to ensure that the noise in the transformed model follows a spherical normal distribution. Violations of this assumption could lead to miscalibrated standard errors and hypothesis tests but should not effect estimation. To demonstrate this, we considered simulated data where each gene has σ g ~ Gamma( r , r ) and τ g ~ Gamma( r /2, r ). As r varies, the quality of the distance estimates does not change significantly (Fig. S26 ).
Semi-simulated COVID-19 data
COVID-19 patient data for the analysis was obtained from ref. 17 , containing 1.4 million cells of 64 types from 284 PBMC samples collected from 196 individuals, including 171 COVID-19 patients and 25 healthy donors.
Ground truth
We define the ground truth as the cell-type specific transcriptomic differences between the 171 COVID-19 patients and the 25 healthy controls. Specifically, we used the following approach to define a ground truth distance:
For each gene g , we computed the log fold changes L g between COVID-19 cases and controls, with L g = E g ( C o v i d ) − E g ( C o n t r o l ), where E g denotes the log-transformed expression data \(\log (1+x)\) .
The ground truth distance is then defined as \(D={\sum }_{g}{L}_{g}^{2}\) .
Subsequently, we excluded any cell types not present in more than 10% of the samples from further analysis. For true negative cell types, we identified the top 5 with the smallest fold change and a representation of over 20,000 cells within the entire dataset. When attempting similar filtering based on cell count alone, no cell types demonstrated a sufficiently large true distance. Consequently, we chose the top four cell types with over 5000 cells as our true positives Fig. S11 .
Using the ground truth, we performed two separate simulation analyses:
1: Simulation analyses I (Fig. 5 A, B): Using one half of the dataset (712621 cells, 132 case samples, 20 control samples), we created 100 subsamples consisting of 5 cases and 5 controls. For each subsample, we applied both scDist and Augur to estimate perturbation/distance between cases and controls for each cell type. Then we computed the correlation between the ground truth ranking (ordering cells by sum of log fold changes on the whole dataset) and the ranking obtained by both methods. For scDist , we restricted to cell types that had a non-zero distance estimate in each subsample, and for Augur we restricted to cell types that had an AUC greater than 0.5 (Fig. 5 A). For Fig. 5 B, we took the mean estimated distance across subsamples for which the given cell type had a non-zero distance estimate. This is because in some subsamples a given cell type could be completely absent.
2: Simulation analyses II (Fig. 5 C–F): We subsampled the COVID-19 cohort with 284 samples (284 PBMC samples from 196 individuals: 171 with COVID-19 infection and 25 healthy controls) to create 1,000 downsampled cohorts, each containing samples from 10 individuals (5 with COVID-19 and 5 healthy controls). We randomly selected each sample from the downsampled cohort, further downsampled the number of cells for each cell type, and selected them from the original COVID-19 cohort. This downsampling procedure increases both cohort variability and cell-number variations.
Performance Evaluation in Subsampled Cohorts : We applied scDist and Augur to each subsampled cohort, comparing the results for true positive and false positive cell types. We partitioned the sampled cohorts into 10 groups based on cell-number variation, defined as the number of cells in a sample with the highest number of cells for false-negative cell types divided by the average number of cells in cell types. This procedure highlights the vulnerability of computational methods to cell number variation, particularly in negative cell types.
Analysis of immunotherapy cohorts
Data collection.
We obtained single-cell data from four cohorts 2 , 23 , 24 , 25 , including expression counts and patient response information.
Pre-processing
To ensure uniform processing and annotation across the four scRNA cohorts, we analyzed CD45+ cells (removing CD45− cells) in each cohort and annotated cells using Azimuth 31 with reference provided for CD45+ cells.
Model to account for cohort and sample variance
To account for cohort-specific and sample-specific batch effects, scDist modeled the normalized gene expression as:
Here, Z represents the normalized count matrix, X denotes the binary indicator of condition (responder = 1, non-responder = 0); γ and ω are cohort and sample-level random effects, and (1 ∣ γ : ω ) models nested effects of samples within cohorts. The inference procedure for distance, its variance, and significance for the model with multiple cohorts is analogous to the single-cohort model.
We estimated the signature in the NK-2 cell type using differential expression between responders and non-responders. To account for cohort-specific and patient-specific effects in differential expression estimation, we employed a linear mixed model described above for estimating distances, performing inference for each gene separately. The coefficient of X inferred from the linear mixed models was used as the estimate of differential expression:
Here, Z represents the normalized count matrix, X denotes the binary indicator of condition (responder = 1, non-responder = 0); γ and ω are cohort and sample-level random effects, and (1 ∣ γ : ω ) models nested effects of samples within cohorts.
Bulk RNA-seq cohorts
We obtained bulk RNA-seq data from seven cancer cohorts 32 , 33 , 34 , 35 , 36 , 37 , 38 , comprising a total of 789 patients. Within each cohort, we converted counts of each gene to TPM and normalized them to zero mean and unit standard deviation. We collected survival outcomes (both progression-free and overall) and radiologic-based responses (partial/complete responders and non-responders with stable/progressive disease) for each patient.
Evaluation of signature in bulk RNA-seq cohorts
We scored each bulk transcriptome (sample) for the signature using the strategy described in ref. 39 . Specifically, the score was defined as the Spearman correlation between the normalized expression and differential expression in the signature. We stratified patients into two groups using the median score for patient stratification. Kaplan–Meier plots were generated using these stratifications, and the significance of survival differences was assessed using the log-rank test. To demonstrate the association of signature levels with radiological response, we plotted signature levels separately for non-responders, partial-responders, and responders.
Evaluating Augur Signature in Bulk RNA-Seq Cohorts
A differential signature was derived for Augur ’s top prediction, plasma cells, using a procedure analogous to the one described above for scDist . This plasma signature was then assessed in bulk RNA-seq cohorts following the same evaluation strategy as applied to the scDist signature.
Statistics and reproducibility
No statistical method was used to predetermine sample size. No data were excluded from the analyses. The experiments were not randomized. The Investigators were not blinded to allocation during experiments and outcome assessment.
Reporting summary
Further information on research design is available in the Nature Portfolio Reporting Summary linked to this article.
Data availability
Table 1 gives a list of the datasets used in each figure, as well as details about how the datasets can be obtained. Source data are provided with this paper.
Code availability
scDist is available as an R package and can be downloaded from GitHub 40 : github.com/phillipnicol/scDist . The repository also includes scripts to replicate some of the figures and a demo of scDist using simulated data.
Wilk, A. J. et al. A single-cell atlas of the peripheral immune response in patients with severe covid-19. Nat. Med. 26 , 1070–1076 (2020).
Article PubMed PubMed Central Google Scholar
Yuen, K. C. et al. High systemic and tumor-associated il-8 correlates with reduced clinical benefit of pd-l1 blockade. Nat. Med. 26 , 693–698 (2020).
Crowell, H. L. et al. Muscat detects subpopulation-specific state transitions from multi-sample multi-condition single-cell transcriptomics data. Nat. Commun. 11 , 6077 (2020).
Article ADS PubMed PubMed Central Google Scholar
Helmink, B. A. et al. B cells and tertiary lymphoid structures promote immunotherapy response. Nature 577 , 549–555 (2020).
Zhao, J. et al. Detection of differentially abundant cell subpopulations in scrna-seq data. Proc. Natl. Acad. Sci. 118 , e2100293118 (2021).
Dann, E., Henderson, N. C., Teichmann, S. A., Morgan, M. D. & Marioni, J. C. Differential abundance testing on single-cell data using k-nearest neighbor graphs. Nat. Biotechnol. 40 , 245–253 (2022).
Article PubMed Google Scholar
Burkhardt, D. B. et al. Quantifying the effect of experimental perturbations at single-cell resolution. Nat. Biotechnol. 39 , 619–629 (2021).
McInnes, L., Healy, J. & Melville, J. Umap: uniform manifold approximation and projection for dimension reduction. arXiv https://arxiv.org/abs/1802.03426 (2018).
Skinnider, M. A. et al. Cell type prioritization in single-cell data. Nat. Biotechnol. 39 , 30–34 (2021).
Zimmerman, K. D., Espeland, M. A. & Langefeld, C. D. A practical solution to pseudoreplication bias in single-cell studies. Nat. Commun. 12 , 1–9 (2021).
Article Google Scholar
Korsunsky, I. et al. Fast, sensitive and accurate integration of single-cell data with harmony. Nat. Methods 16 , 1289–1296 (2019).
Townes, F. W., Hicks, S. C., Aryee, M. J. & Irizarry, R. A. Feature selection and dimension reduction for single-cell rna-seq based on a multinomial model. Genome Biol. 20 , 1–16 (2019).
Hafemeister, C. & Satija, R. Normalization and variance stabilization of single-cell RNA-seq data using regularized negative binomial regression. Genome Biol. 20 , 1–15 (2019).
Stephens, M. False discovery rates: a new deal. Biostatistics 18 , 275–294 (2017).
MathSciNet PubMed Google Scholar
Zheng, G. X. et al. Massively parallel digital transcriptional profiling of single cells. Nat. Commun. 8 , 14049 (2017).
Duò, A., Robinson, M. D. & Soneson, C. A systematic performance evaluation of clustering methods for single-cell RNA-seq data. F1000Res. 7 , 1141 (2018).
Ren, X. et al. Covid-19 immune features revealed by a large-scale single-cell transcriptome atlas. Cell 184 , 1895–1913 (2021).
Galati, D., Zanotta, S., Capitelli, L. & Bocchino, M. A bird’s eye view on the role of dendritic cells in sars-cov-2 infection: Perspectives for immune-based vaccines. Allergy 77 , 100–110 (2022).
Pérez-Gómez, A. et al. Dendritic cell deficiencies persist seven months after sars-cov-2 infection. Cell. Mol. Immunol. 18 , 2128–2139 (2021).
Upadhyay, A. A. et al. Trem2+ and interstitial macrophages orchestrate airway inflammation in sars-cov-2 infection in rhesus macaques. bioRxiv https://www.biorxiv.org/content/10.1101/2021.10.05.463212v1 (2021).
Wang, S. et al. S100a8/a9 in inflammation. Front. Immunol. 9 , 1298 (2018).
Mellett, L. & Khader, S. A. S100a8/a9 in covid-19 pathogenesis: impact on clinical outcomes. Cytokine Growth Factor Rev. 63 , 90–97 (2022).
Luoma, A. M. et al. Tissue-resident memory and circulating t cells are early responders to pre-surgical cancer immunotherapy. Cell 185 , 2918–2935 (2022).
Yost, K. E. et al. Clonal replacement of tumor-specific t cells following pd-1 blockade. Nat. Med. 25 , 1251–1259 (2019).
Sade-Feldman, M. et al. Defining T cell states associated with response to checkpoint immunotherapy in melanoma. Cell 175 , 998–1013 (2018).
Pearson, K. Liii. on lines and planes of closest fit to systems of points in space. Lond. Edinb. Dublin Philos. Mag. J. Sci. 2 , 559–572 (1901).
Bates, D., Mächler, M., Bolker, B. & Walker, S. Fitting linear mixed-effects models using lme4. J. Stat. Softw. 67 , 1–48 (2015).
North, B. V., Curtis, D. & Sham, P. C. A note on the calculation of empirical p values from Monte Carlo procedures. Am. J. Hum. Genet. 71 , 439–441 (2002).
Neufeld, A., Gao, L. L., Popp, J., Battle, A. & Witten, D. Inference after latent variable estimation for single-cell RNA sequencing data. arXiv https://arxiv.org/abs/2207.00554 (2022).
Laurent, S. uniformly: uniform sampling. R package version 0.2.0 https://CRAN.R-project.org/package=uniformly (2022).
Hao, Y. et al. Integrated analysis of multimodal single-cell data. Cell 184 , 3573–3587 (2021).
Mariathasan, S. et al. Tgf β attenuates tumour response to pd-l1 blockade by contributing to exclusion of T cells. Nature 554 , 544–548 (2018).
Weber, J. S. et al. Sequential administration of nivolumab and ipilimumab with a planned switch in patients with advanced melanoma (checkmate 064): an open-label, randomised, phase 2 trial. Lancet Oncol. 17 , 943–955 (2016).
Liu, D. et al. Integrative molecular and clinical modeling of clinical outcomes to pd1 blockade in patients with metastatic melanoma. Nat. Med. 25 , 1916–1927 (2019).
McDermott, D. F. et al. Clinical activity and molecular correlates of response to atezolizumab alone or in combination with bevacizumab versus sunitinib in renal cell carcinoma. Nat. Med. 24 , 749–757 (2018).
Riaz, N. et al. Tumor and microenvironment evolution during immunotherapy with nivolumab. Cell 171 , 934–949 (2017).
Miao, D. et al. Genomic correlates of response to immune checkpoint therapies in clear cell renal cell carcinoma. Science 359 , 801–806 (2018).
Van Allen, E. M. et al. Genomic correlates of response to ctla-4 blockade in metastatic melanoma. Science 350 , 207–211 (2015).
Sahu, A. et al. Discovery of targets for immune–metabolic antitumor drugs identifies estrogen-related receptor alpha. Cancer Discov. 13 , 672–701 (2023).
Nicol, P. scdist https://doi.org/10.5281/zenodo.12709683 (2024).
Download references
Acknowledgements
P.B.N. is supported by NIH T32CA009337. A.D.S. received support from R00CA248953, the Michelson Foundation, and was partially supported by the UNM Comprehensive Cancer Center Support Grant NCI P30CA118100. We express our gratitude to Adrienne M. Luoma, Shengbao Suo, and Kai W. Wucherpfennig for providing the scRNA data 23 . We also thank Zexian Zeng for assistance with downloading and accessing the bulk RNA-seq dataset.
Author information
Authors and affiliations.
Harvard University, Cambridge, MA, USA
Phillip B. Nicol & Danielle Paulson
University of California San Diego School of Medicine, San Diego, CA, USA
Dana-Farber Cancer Institute, Boston, MA, USA
X. Shirley Liu & Rafael Irizarry
University of New Mexico Comprehensive Cancer Center, Albuquerque, NM, USA
Avinash D. Sahu
You can also search for this author in PubMed Google Scholar
Contributions
P.B.N., D.P., G.Q., X.S.L., R.I., and A.D.S. conceived the study. P.B.N. and A.D.S. implemented the method and performed the experiments. P.B.N., R.I., and A.D.S. wrote the manuscript.
Corresponding authors
Correspondence to Rafael Irizarry or Avinash D. Sahu .
Ethics declarations
Competing interests.
X.S.L. conducted the work while being on the faculty at DFCI, and is currently a board member and CEO of GV20 Therapeutics. P.B.N., D.P., G.Q., R.I., and A.D.S. declare no competing interests.
Peer review
Peer review information.
Nature Communications thanks the anonymous reviewer(s) for their contribution to the peer review of this work. A peer review file is available.
Additional information
Publisher’s note Springer Nature remains neutral with regard to jurisdictional claims in published maps and institutional affiliations.
Supplementary information
Supplementary information, peer review file, reporting summary, source data, source data, rights and permissions.
Open Access This article is licensed under a Creative Commons Attribution 4.0 International License, which permits use, sharing, adaptation, distribution and reproduction in any medium or format, as long as you give appropriate credit to the original author(s) and the source, provide a link to the Creative Commons licence, and indicate if changes were made. The images or other third party material in this article are included in the article’s Creative Commons licence, unless indicated otherwise in a credit line to the material. If material is not included in the article’s Creative Commons licence and your intended use is not permitted by statutory regulation or exceeds the permitted use, you will need to obtain permission directly from the copyright holder. To view a copy of this licence, visit http://creativecommons.org/licenses/by/4.0/ .
Reprints and permissions
About this article
Cite this article.
Nicol, P.B., Paulson, D., Qian, G. et al. Robust identification of perturbed cell types in single-cell RNA-seq data. Nat Commun 15 , 7610 (2024). https://doi.org/10.1038/s41467-024-51649-3
Download citation
Received : 14 December 2023
Accepted : 09 August 2024
Published : 01 September 2024
DOI : https://doi.org/10.1038/s41467-024-51649-3
Share this article
Anyone you share the following link with will be able to read this content:
Sorry, a shareable link is not currently available for this article.
Provided by the Springer Nature SharedIt content-sharing initiative
By submitting a comment you agree to abide by our Terms and Community Guidelines . If you find something abusive or that does not comply with our terms or guidelines please flag it as inappropriate.
Quick links
- Explore articles by subject
- Guide to authors
- Editorial policies
Sign up for the Nature Briefing newsletter — what matters in science, free to your inbox daily.

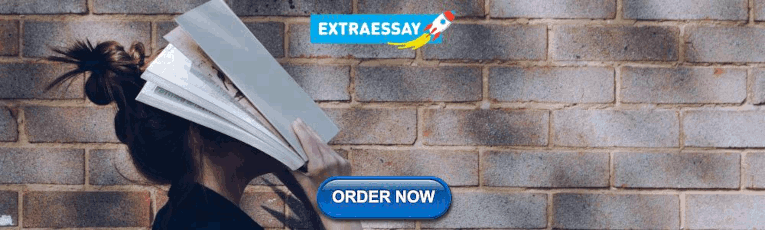
IMAGES
VIDEO
COMMENTS
conducting communication research is an enormous. field of study that requires very skilled and knowl-. edgeable researchers who are aware of its many. areas, approaches, designs, methods, tools ...
COM 365 Course Syllabus ~ Fall 2010. "The best research is driven by theory, validates a theory, further explains a theory, challenges an existing theory, or aids in the creation of theory. Theoretically driven research is built on the results of previous researchers, and it provides a foundation for subsequent researchers.
Ideal for beginning research students at both the graduate and undergraduate level, this proven book is clear, concise, and accompanied by just the right number of detailed examples, useful applications, and valuable exercises that are sure to get your students to want to understand, and master, media and communication research.
Communication Department Office: 859.257.3622 Monday and Wednesday 1:00 p.m. - 2:00 p.m. (And by appointment) COURSE DESCRIPTION & OBJECTIVES. Introduction to Communication Research Methods provides an overview of the concepts, methods, and tools by which communication research is designed, conducted, interpreted, and critically evaluated.
Purpose of Course: This course is designed to introduce you to the scientific, interpretive, rhetorical, and critical research methods we use to study communication problems and processes. We will focus on the critical analysis, evaluation, and use of quantitative and qualitative communication research methods.
This step-by-step introduction to conducting media and communication research offers practical insights along with Arthur Asa Berger's signature lighthearted style to make discussion of qualitative and quantitative methods easy to comprehend. The Fifth Edition of Media and Communication Research Methods includes a new chapter on discourse analysis; expanded discussion of social media ...
Communication Research Methods Communication 206 Fall Quarter, 1999. Professor Shanto Iyengar McClatchy 440 3-5509 [email protected]. ... An Introduction to Survey Research, Chs. 1-4; Sudman and Bradburn, Response Effects in Surveys, 1-19; Loftus et al., "A tale of two questions"; Bassili and Fletcher, "Response-time measurement in survey ...
The SAGE edge site for Introducing Communication Research by Donald Treadwell and Andrea Davis offers a robust online environment you can access anytime, anywhere, and features an impressive array of free tools and resources to keep you on the cutting edge of your learning experience. Introducing Communication Research: Paths of Inquiry teaches ...
This third edition is again a practical introduction to communication research methods, foregrounding the role research plays in communication and media industry careers. Covering major methodologies such as surveys, experiments, focus groups, in-depth interviews, content analysis, and others, the book takes the reader through the research process from beginning to end. The text continues to ...
This book provides a comprehensive, balanced introduction to communication research methods. Designed for readers who have little or no familiarity with research methods, this book helps one become a more knowledgeable and competent consumer (and limited producer) of scholarly research and of the everyday research to which they are exposed by the mass media. Praised for its accessible writing ...
This introduction to communication research methods takes the student from the conceptual beginnings of a research project through the design and analysis. Emphasizing the correct questions to ask and how to approach the answers, authors Gary Petty, Cheryl Campanella Bracken, and Elizabeth Babin approach social science methods as a language to ...
The Fifth Edition of Media and Communication Research Methods includes a new chapter on discourse analysis; expanded discussion of social media, including discussion of the ethics of Facebook experiments; and expanded coverage of the research process with new discussion of search strategies and best practices for analyzing research articles ...
This book provides a comprehensive, balanced introduction to communication research methods. Designed for readers who have little or no familiarity with research methods, this book helps one become a more knowledgeable and competent consumer (and limited producer) of scholarly research and of the everyday research to which they are exposed by the mass media.
This introduction to communication research methods takes the student from the conceptual beginnings of a research project through the design and analysis. Emphasizing the correct questions to ask and how to approach the answers, authors Gary Petty, Cheryl Campanella Bracken, and Elizabeth Babin approach social science methods as a language to be learned, requiring multiple sessions and ...
Communication research is important because it focuses on a common goal—to enhance our interactions with others. In this chapter we highlighted how research is done and the basic steps that guide most research projects—identify the topic, write a research question, define key terms, select a methodology, establish a sample, gather and analyze the data, and finally, interpret and share the ...
Chapter One: Introduction. In this chapter, we first describe how developing a command of research methods can assist you in your careers and personal lives. Second, we provide a brief definition of our topic of study in this book - communication research. Third we identify the predominant research and creative methods used in the field of ...
What is research? -- Library searches. Part II. Methods of textual analysis. Semiotic analysis -- Rhetorical analysis -- Ideological criticism -- Psychoanalytic criticism. Part III. Qualitative research methods. Interviews -- Historical analysis -- Ethnomethodological research -- Participant observation. Part IV. Quantitative research methods.
This chapter introduces the Research Culture, and discusses the importance of Knowing Research Methods, and the process of Analyzing and Reporting Qualitative Data in Communication Research. All chapters end with "Conclusion." Preface. I.CONCEPTUALIZING COMMUNICATION RESEARCH. 1.Introduction to the Research Culture. The Importance of Knowing Research Methods.
Introduction to Qualitative Communication Research Two Researchers, Two Scenes of Research READING COMMUNITY IN A POST OFFICE The people of Burgin, Kentucky, and surrounding countryside pick up ... 2 Qualitative Communication Research Methods Lindlof01.qxd 8/13/02 5:01 PM Page 2. Monica found the people she worked with in the college to be ...
Investigating Communication: An Introduction to Research Methods Lawrence R. Frey , Carl H. Botan , Paul G. Friedman , Gary L. Kreps Pearson Education Canada , 1991 - Language Arts & Disciplines
COM 365 Course Syllabus ~ Fall 2011. "The best research is driven by theory, validates a theory, further explains a theory, challenges an existing theory, or aids in the creation of theory. Theoretically driven research is built on the results of previous researchers, and it provides a foundation for subsequent researchers.
COMM2201 Introduction to Communication Research Methods. This course is an introduction to research as it relates to media and communication. Emphasis will be on the theory and practice of media and communication research. The course is intended to equip students with theoretical knowledge and practical skills needed to conduct communication ...
Single-cell transcriptomics has emerged as a powerful tool for understanding how different cells contribute to disease progression by identifying cell types that change across diseases or conditions.