Thank you for visiting nature.com. You are using a browser version with limited support for CSS. To obtain the best experience, we recommend you use a more up to date browser (or turn off compatibility mode in Internet Explorer). In the meantime, to ensure continued support, we are displaying the site without styles and JavaScript.
- View all journals
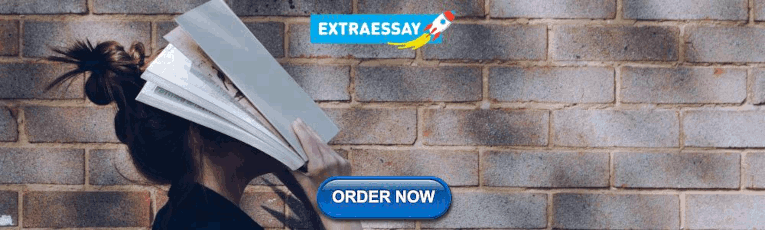
Functional genomics articles from across Nature Portfolio
Functional genomics uses genomic data to study gene and protein expression and function on a global scale (genome-wide or system-wide), focusing on gene transcription, translation and protein-protein interactions, and often involving high-throughput methods.
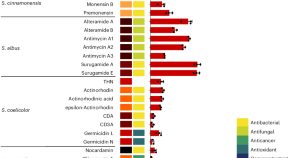
Co-evolved genes improve the biosynthesis of secondary metabolites
A new engineering strategy for improving the biosynthesis of secondary metabolites in Streptomyces has been developed through the analysis of genes co-evolved with biosynthetic gene clusters. This strategy has been verified in 11 Streptomyces strains to enhance production of 16,385 metabolites, showing potential applications in drug discovery and industrial production.
Related Subjects
- Gene expression profiling
- Mutagenesis
Latest Research and Reviews
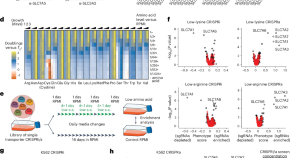
A CRISPRi/a screening platform to study cellular nutrient transport in diverse microenvironments
Chidley et al. report a CRISPR interference/activation screening platform to systematically interrogate the contribution of nutrient transporters to support cancer cell proliferation in environments of variable composition.
- Christopher Chidley
- Alicia M. Darnell
- Peter K. Sorger
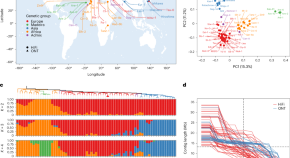
A pan-genome of 69 Arabidopsis thaliana accessions reveals a conserved genome structure throughout the global species range
A pan-genome of Arabidopsis thaliana constructed using chromosome-level genome assemblies of 69 diverse accessions reveals a conserved genome structure throughout the global species range.
- Qichao Lian
- Bruno Huettel
- Raphael Mercier
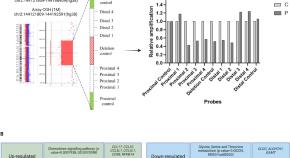
Further evidence supporting the role of GTDC1 in glycine metabolism and neurodevelopmental disorders
- Edoardo Errichiello
- Mauro Lecca
- Maria Clara Bonaglia
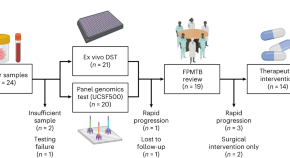
Feasibility of functional precision medicine for guiding treatment of relapsed or refractory pediatric cancers
In an observational study evaluating functional precision medicine in children and adolescents with relapsed or refractory solid and hematologic malignancies, it was feasible to provide personalized treatment recommendations to treating physicians on the basis of genomic profiling and ex vivo drug sensitivity testing within 4 weeks.
- Arlet M. Acanda De La Rocha
- Noah E. Berlow
- Diana J. Azzam
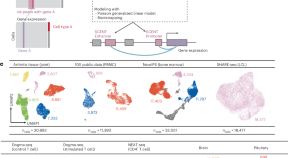
Tissue-specific enhancer–gene maps from multimodal single-cell data identify causal disease alleles
SCENT is a nonparametric method that models association between chromatin accessibility and gene expression in single-cell multimodal datasets, enabling construction of cell-type-specific enhancer–gene maps to aid mapping of candidate causal variants and genes for common diseases.
- Saori Sakaue
- Kathryn Weinand
- Soumya Raychaudhuri
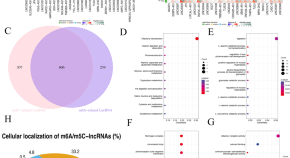
Identification of m6A/m5C-related lncRNA signature for prediction of prognosis and immunotherapy efficacy in esophageal squamous cell carcinoma
- Jianlin Wang
News and Comment
How transposable elements are spliced out.
- Chiara Anania
Transcription factor binding site affinity and the link to phenotype
- Michael Fletcher
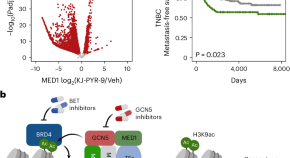
MYC activates transcriptional enhancers to drive cancer progression
We show that in addition to promoter activation, MYC drives cancer progression by activating transcriptional enhancers via a distinct mechanism. MYC cooperates with several other proteins at these cis -regulatory regions to change the epigenome and promote recruitment of RNA polymerase II and enhancer transcription.
Unveiling the expanding protein universe of life
In this Journal Club, Hajk-Georg Drost highlights a recent study by Pavlopoulos et al. that organizes proteins at tree-of-life scale using massively parallel graph-based clustering.
- Hajk-Georg Drost
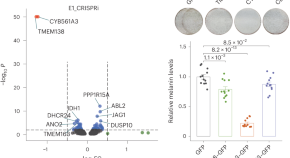
Unravelling the molecular mechanisms of skin color diversity in Africans
Skin color is highly variable in Africans, but the underlying molecular mechanisms remain poorly understood. Using population genetics and functional genomics, we identified key genetic variants, regulatory elements and genes that affect skin pigmentation, an adaptive trait, which provides valuable insights into the mechanisms underlying human skin color diversity and evolution.
Quick links
- Explore articles by subject
- Guide to authors
- Editorial policies

- Search Menu
- Advance articles
- Author Guidelines
- Submission Site
- Open Access
Learn more about submitting protocol articles
- About Briefings in Functional Genomics
- Journals Career Network
- Editorial Board
- Advertising and Corporate Services
- Self-Archiving Policy
- Journals on Oxford Academic
- Books on Oxford Academic

Editor-in-Chief
Paul J. Hurd
Consulting Editor
Andrew J. Bannister
Accepting Review and Protocol Papers
Briefings in Functional Genomics is accepting submissions for upcoming issues, including review and protocol articles. Articles range in scope and depth from the introductory level to specific details of protocols and analyses, encompassing bacterial, fungal, plant, animal, and human data.
Find out more about submitting and formatting your manuscript
Latest articles

Special Issues
Multi-omics approaches to therapeutic target identification
This special issue collects together 12 articles focused on omics technologies and their integration towards drug target identification, validation and subsequent therapeutic implications.
Functional Genomics of Ageing/Nutrigenomics
In this special issue, we compiled 6 reviews that aim to provide an overview on the current state of knowledge on functional genomics of ageing.
Biological functions of RNA modifications
In this special issue, we have compiled a collection of easy-to-read reviews focusing on key RNA modifications and their molecular and biological functions.

Special Issues Archive
Browse previous special issues from Briefings in Functional Genomics covering a wide range of subjects from across the discipline.

High-Impact Research Collection
Explore the most read, mot cited, and most discussed articles published in Briefings in Functional Genomics in recent years and discover what has caught the interest of your peers.
Browse the collection

Email alerts
Register to receive email alerts as soon as new content from Briefings in Functional Genomics is published online.

Read and Publish deals
Authors interested in publishing in Briefings in Functional Genomics may be able to publish their paper Open Access using funds available through their institution’s agreement with OUP.
Find out if your institution is participating
Editor's Choice Articles
Explore a collection of high quality articles published in Briefings in Functional Genomics , handpicked by the EIC of the journal. All the articles listed are freely available to read.
Browse all Editor's Choice Articles
Related Titles
- Recommend to your Library
Affiliations
- Online ISSN 2041-2657
- Copyright © 2024 Oxford University Press
- About Oxford Academic
- Publish journals with us
- University press partners
- What we publish
- New features
- Open access
- Institutional account management
- Rights and permissions
- Get help with access
- Accessibility
- Advertising
- Media enquiries
- Oxford University Press
- Oxford Languages
- University of Oxford
Oxford University Press is a department of the University of Oxford. It furthers the University's objective of excellence in research, scholarship, and education by publishing worldwide
- Copyright © 2024 Oxford University Press
- Cookie settings
- Cookie policy
- Privacy policy
- Legal notice
This Feature Is Available To Subscribers Only
Sign In or Create an Account
This PDF is available to Subscribers Only
For full access to this pdf, sign in to an existing account, or purchase an annual subscription.
- Introduction to Genomics
- Educational Resources
- Policy Issues in Genomics
- The Human Genome Project
- Funding Opportunities
- Funded Programs & Projects
- Division and Program Directors
- Scientific Program Analysts
- Contact by Research Area
- News & Events
- Research Areas
- Research investigators
- Research Projects
- Clinical Research
- Data Tools & Resources
- Genomics & Medicine
- Family Health History
- For Patients & Families
- For Health Professionals
- Jobs at NHGRI
- Training at NHGRI
- Funding for Research Training
- Professional Development Programs
- NHGRI Culture
- Social Media
- Broadcast Media
- Image Gallery
- Press Resources
- Organization
- NHGRI Director
- Mission & Vision
- Policies & Guidance
- Institute Advisors
- Strategic Vision
- Leadership Initiatives
- Diversity, Equity, and Inclusion
- Partner with NHGRI
- Staff Search
- Translational and Functional Genomics Branch
The Translational and Functional Genomics Branch (TFGB) studies how genome structure and function contribute to health by exploring the genetic, epigenetic and metagenomic basis of human disorders.
TFGB researchers have developed a wide range of experimental and computational techniques to study all aspects of the genomes of humans, microorganisms that live on humans and animal models of genetic disease. TFGB investigators catalyze technology development in genetics and computational genomics, including functional assessment, systematic mutagenesis, developmental genomics and computational analysis of both human and microbial DNA. By testing approaches and technologies in cell lines and animal models, TFGB investigators are making fundamental discoveries that will be critical to the success of human clinical trials. The long-term goal of their efforts is to successfully translate laboratory findings into improved diagnoses and therapeutics for human disorders.
Branch Staff
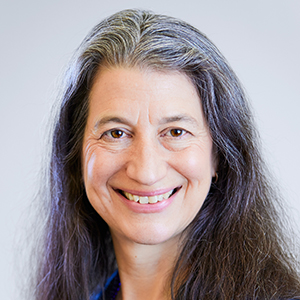
- Chief and NIH Distinguished Investigator
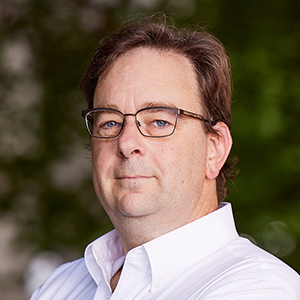
- Deputy Director
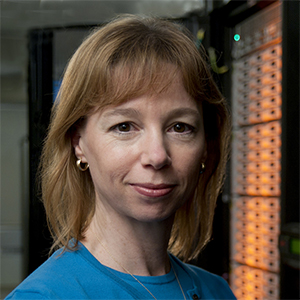
- Senior Investigator
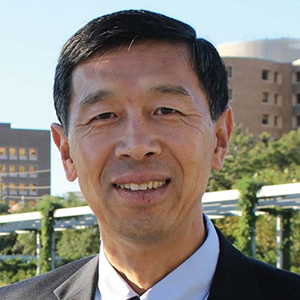
- Associate Investigator
- Microbial Genomics Section
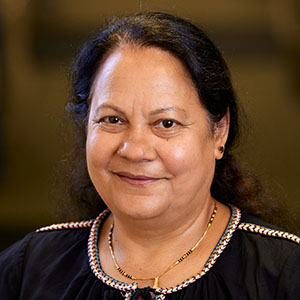
- Office of Scientific Core Facilities
Last updated: July 25, 2022
Functional Genomics Research
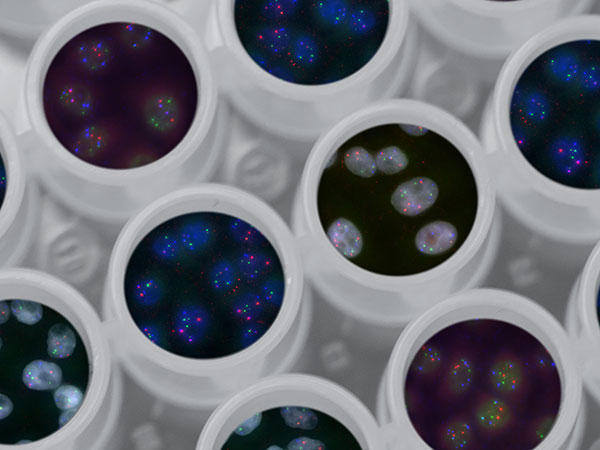
Cells with genes highlighted by florescent dyes grow in multi-well plates.
Functional genomics research examines the role of the genome in cancer. By testing hypotheses derived from structural genomics research , or by generating new ideas from experiments in cancer cells, functional genomics research reveals patterns in cancer biology that can sometimes be directly translated to precision cancer care. Studies like those from The Cancer Target Discovery and Development (CTD 2 ) Network, have already discovered genomic vulnerabilities in cancer that can be exploited through targeted treatments. Many of CCG’s functional genomics experiments further investigate insights from structural genomics studies, which are carried out by CCG’s Genome Characterization Pipeline .
Key Questions
- How do altered genes in cancer work together within pathways to promote abnormal proliferation and survival?
- Can molecular pathways affected by genetic abnormalities in cancer genes be targeted with available drugs or new compounds?
- Can tumor models generated from patient biopsies be used to understand mechanisms of therapeutic efficacy or resistance?
Tools and Methods
CCG’s functional genomics studies use models of cancer for high-throughput drug screens, gene perturbation experiments using RNA interference and CRISPR-Cas9 technology, and many other genome-wide techniques. Currently, CCG researchers use cancer cell lines , tumor organoid cultures that grow in petri dishes, or mice bearing grafts from human tumors to determine the effects of particular genetic alterations. Recognizing the power of new methods for generating cancer models, CCG is supporting the development of cutting-edge organoid and conditionally reprogrammed cell models to promote the safe and effective translation of functional cancer genomics findings to clinical care.
Programs and Collaborations
Cancer target discovery and development (ctd 2 ) network .
The CTD 2 Network bridges a major gap between cancer genomics and precision oncology by mining large-scale genomic datasets for alterations important in cancer development and translating those discoveries into treatment. The CTD² Network emphasizes collaborations between the funded Centers , which have expertise in various computational and functional genomic approaches. In addition to publishing novel results, the CTD 2 Network produces publicly available data , analytical tools , and reagents .
All data generated are open-access and can be obtained from the websites listed below:
- Raw and analyzed primary data are available through the CTD 2 Data Portal
- Network-generated observations and results, with associated supporting evidence, are compiled in a web interface known as the CTD 2 Dashboard
Human Cancer Models Initiative (HCMI)
HCMI is an international collaboration between the NCI, Cancer Research UK , the Wellcome Trust Sanger Institute , and the foundation Hubrecht Organoid Technology with the goal of generating a publicly available bank of 1,000 next-generation cancer models annotated with genomic and clinical data.
HCMI uses new, innovative technologies, including organoid and conditionally reprogrammed cell (CRC) culturing techniques, to create cancer models that more accurately represent the architecture and complexity of real tumors. The models also have associated genomic data and clinical data made available to the research community.
NCI is contributing to this international consortium by providing funding and support to two Cancer Model Development Centers (CMDCs), which will develop a subset of the HCMI's next-generation cancer models from patient tissues.
Next-Generation Technologies (NGT)
Next-Generation Technologies supports the development of technology tools to facilitate and accelerate research using next-generation cancer models, such as organoids and conditionally reprogrammed cells. The tools will focus on utilizing models developed by NCI's Human Cancer Models Initiative . Protocols, materials, and knowledge developed by the program will be shared broadly and expeditiously with the research community.
Plant genome information facilitates plant functional genomics
- Open access
- Published: 09 April 2024
- Volume 259 , article number 117 , ( 2024 )
Cite this article
You have full access to this open access article
- Judith Jazmin Bernal-Gallardo 1 &
- Stefan de Folter ORCID: orcid.org/0000-0003-4363-7274 1
175 Accesses
18 Altmetric
Explore all metrics
Main conclusion
In this review, we give an overview of plant sequencing efforts and how this impacts plant functional genomics research.
Plant genome sequence information greatly facilitates the studies of plant biology, functional genomics, evolution of genomes and genes, domestication processes, phylogenetic relationships, among many others. More than two decades of sequencing efforts have boosted the number of available sequenced plant genomes. The first plant genome, of Arabidopsis, was published in the year 2000 and currently, 4604 plant genomes from 1482 plant species have been published. Various large sequence initiatives are running, which are planning to produce tens of thousands of sequenced plant genomes in the near future. In this review, we give an overview on the status of sequenced plant genomes and on the use of genome information in different research areas.
Similar content being viewed by others
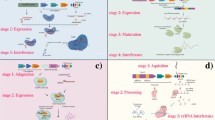
CRISPR/Cas genome editing in plants: mechanisms, applications, and overcoming bottlenecks
Delight Hwarari, Yasmina Radani, … Liming Yang
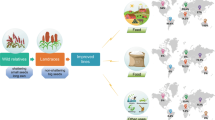
Sorghum breeding in the genomic era: opportunities and challenges
Huaiqing Hao, Zhigang Li, … Hai-Chun Jing
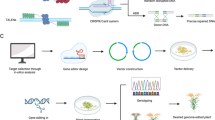
Crop bioengineering via gene editing: reshaping the future of agriculture
Mohamed Atia, Wenjun Jiang, … Magdy Mahfouz
Avoid common mistakes on your manuscript.
Introduction
The blueprint of living organisms sits in its DNA. It contains the instructions for an organism to grow and develop. In the last two decades, genome sequencing has greatly advanced. Currently, the NCBI database ( https://www.ncbi.nlm.nih.gov/ ) holds information on 30,530 eukaryotic genomes (representing 12,205 species), of which 5119 are complete or at chromosome level (accessed on 5 March 2024; Fig. 1 ). From these sequencing efforts, it became clear that the complexity of an organism is not necessary in the number of its genes. For instance, the number of genes of human (International Human Genome Sequencing Consortium 2001 ; Venter et al. 2001 ) or a roundworm (C. elegans Sequencing Consortium 1998 ) are not that far apart. A big part of the complexity is in how gene expression is regulated, and finally in how many proteins this can result. Genome information drives the discovery of biological insights on how organisms are functioning and their evolutionary history, and as well for biotechnological innovations. In the field of agriculture, genome information helps modern breeding, facilitates climate adaptation and food security, among others. Though it does not stop here, genome sequence efforts continue around the world. To highlight one large effort, the Earth BioGenome Project, which aims to sequence every living eukaryotic organism with a name on our planet, which is around 2 million species (Lewin et al. 2018 ; Ebenezer et al. 2022 ). A genomic tree of life is intended to aid in our understanding of how species change, adapt, and rely on one another across an ecosystem. Through these discoveries, long-standing problems in phylogenetics, evolution, ecology, conservation, agriculture, the bioindustry, and medicine will be resolved (Blaxter et al. 2022 ).
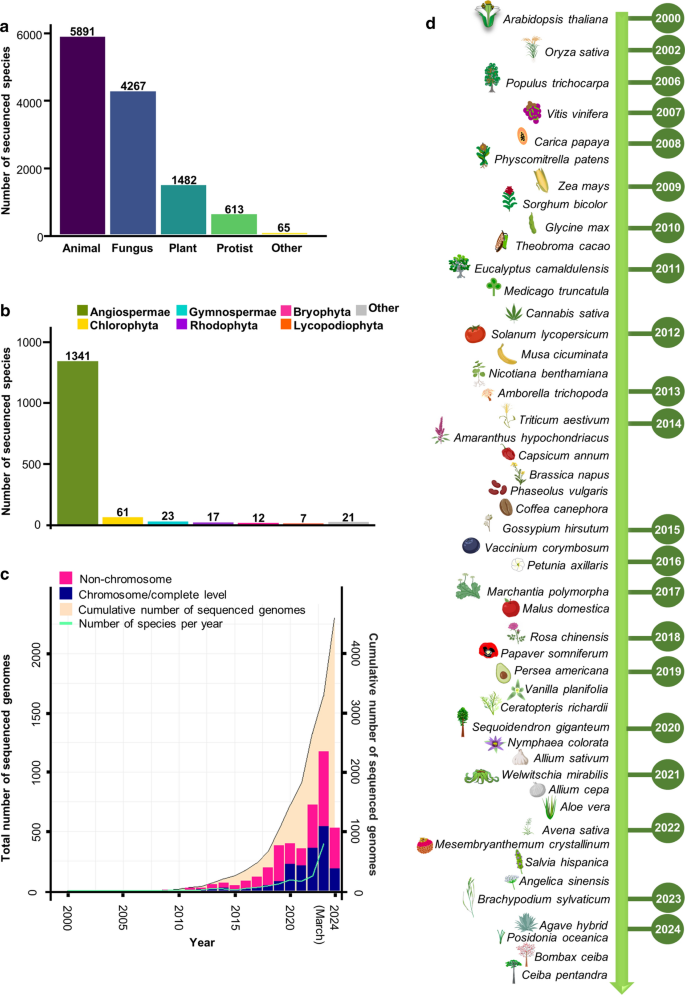
Sequenced genomes of plant species. a The plant kingdom stands as the third-most sequenced domain of life, as evidenced by the cumulative number of sequenced species. b Boxplot of sequenced species across the main clades of the Plant Kingdom. c Graphical representation of the progression in plant genome sequencing since 2000. The bars illustrate the distribution of plant genomes at both chromosomal and non-chromosomal levels. The green line tracks the annual sequencing rate of species, while the salmon shadowed area represents the cumulative count of sequences through March 2024. For the latter two, use values on the right y-axis. d Chronology of sequenced key plants of agriculturally and scientifically important plant species. In a , the data for animals, fungi, protists, and other domains of life were acquired from the NCBI database ( https://www.ncbi.nlm.nih.gov/ ). Sequenced plant species counts were obtained from https://www.plabipd.de/ , with the information updated on 19 February 2024. In b , species count data and genome sequencing details at both chromosomal and non-chromosomal levels were obtained from the NCBI database. Species counts were verified and updated using information from https://www.plabipd.de/ . In ( c ), the chronology was constructed using data obtained from the NCBI database and some of the images were generated using BioRender.com
In this review, we give an overview of the status of (nuclear) plant genome sequencing efforts and how this has helped for studies on plant functional genomics.
The status of sequenced plant genomes
Information on plant genome sequences enormously facilitates studies on plant biology, genetics, development, evolution, molecular biology, among many others. The first sequenced plant genome, Arabidopsis thaliana , was published in the year 2000 (Arabidopsis Genome Initiative 2000 ). This model plant is widely used worldwide and with the genome sequence, it opened the plant field into the genomics era. For a historical overview of Arabidopsis, we refer to other reviews (Meyerowitz 2001 ; Provart et al. 2016 , 2021 ; Somssich 2019 ). Arabidopsis has a genome size of around 135 Mb, and based on the latest Araport11 re-annotation, has 27,655 protein-coding loci with 48,359 transcripts (Cheng et al. 2017 ). Various dedicated websites house data for the community such as The Arabidopsis Information Resource (TAIR; Rhee et al. 2003 ), Araport (Cheng et al. 2017 ; Pasha et al. 2020 ), ThaleMine (Krishnakumar et al. 2017 ; Pasha et al. 2020 ), and Bio-Analytic Resource (BAR; Toufighi et al. 2005 ).
Nowadays, plant genome sequencing is a very active field (Michael and Jackson 2013 ; Chen et al. 2018 ; Kersey 2019 ; Marks et al. 2021 ; Kress et al. 2022 ; Sun et al. 2022 ). Since the publication of the Arabidopsis genome in December 2000 (Arabidopsis Genome Initiative 2000 ) 4604 nuclear plant genomes have been sequenced, corresponding to 1482 plant species, most of them being from angiosperms (90%) (Figs. 1 and 2 ). This genome data are based on information from the NCBI database (accessed on 5 March 2024; https://www.ncbi.nlm.nih.gov/genome/browse#!/overview/plants ), and from the website Published Plant Genomes that visualizes sequenced plant genomes over time ( https://www.plabipd.de/ ; R. Schwacke, personal communication, 19 February 2024). The second plant species to have a genome sequenced was rice, with two subspecies of rice ( Oryza Sativa subsp. japonica and subsp. indica ; Goff et al. 2002 ; Yu et al. 2002 ); in 2006 the first genome of a tree, from poplar ( Populus trichocarpa ; Tuskan et al. 2006 ); and in 2007 the genome of grape, the first genome of a fruit producing species ( Vitis vinifera ; Velasco et al. 2007 ). In the second decade of sequencing, the number of genome reports per year went up exponentially (Fig. 1 ).
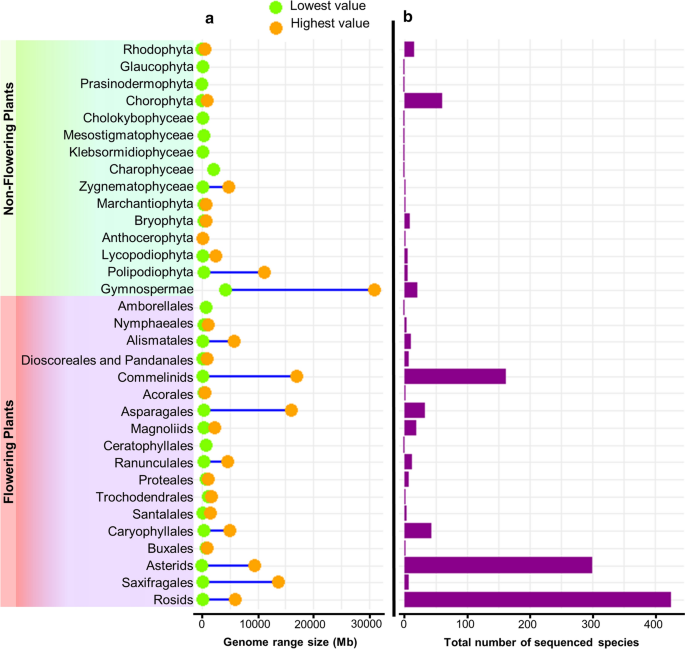
Genome size and species count across plant clades. a Range of genome size within each clade of plant classification, with data points denoting the minimum and maximum genome sizes for each clade. b Bars illustrating the distribution of the number of species within each clade of plant classification. The plant classification used is based on the taxonomy provided by https://www.plabipd.de/ , which was updated on 19 February 2024
Just in the last five year, numbers of sequenced nuclear plant genomes increased impressively from around 576 (reflecting 383 species) (Kersey 2019 ), 798 (reflecting 798 species) (Marks et al. 2021 ), 1031 (reflecting 788 species) (Sun et al. 2022 ), 1139 (reflecting 812 species) (Kress et al. 2022 ), to 4604 genome sequences (reflecting 1482 species) that have been reported (5 March 2024; Table S1 ). This has to do with improvements of sequence technologies and lower costs (Shendure et al. 2017 ; Michael and VanBuren 2020 ; Henry 2022 ). One of the descriptions of the quality of genome assemblies is the value of the Contig N50, which indicates the length of the shortest contig in the set of contigs containing at least 50% of the assembly length. This value greatly improved over the years (Fig. 3 a), which is low (< 1 kb or < 10 kb) when a short-read sequencing approach was used (e.g., Illumina), and nowadays, with the use of long-read sequencing approaches such as from Pacific Biosciences (PacBio) and Oxford Nanopore Technologies (ONT), the Contig N50 is hundreds of kb to several Mb, resulting in much higher quality genome assemblies (Michael and Jackson 2013 ; Belser et al. 2018 ; Kersey 2019 ; Michael and VanBuren 2020 ; Marks et al. 2021 ; Sharma et al 2021 ; Sun et al. 2022 ).
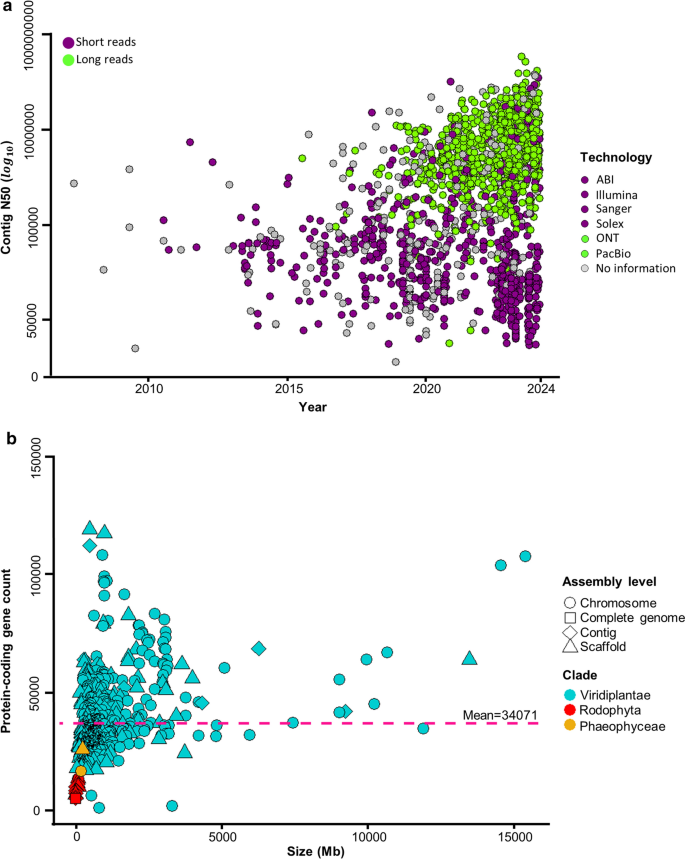
Comparative analysis of genome size and protein-coding genes in annotated plant genomes, and assembly statistics of contig N50 over time for sequenced plant species. a Distribution of assembly statistics: Contig N50 over time for the 1482 sequenced plant species; data obtained from the NCBI Database ( https://www.ncbi.nlm.nih.gov/ ). The green points represent assemblies based on long-read sequencing methods, while the purple points represent assemblies based on short-read sequencing methods. b The graph illustrates the distribution of the genome size and the number of protein-coding genes (the pink dashed line indicates the mean number of genes per genome: 34,071) in the 685 available annotated plant genomes, utilizing taxonomic classifications from the NCBI database ( https://www.ncbi.nlm.nih.gov/ ). Points are colored by assembly level, and the figure represents a clade of the Plant Kingdom
The estimated number of extant green plant species is around 450,000–500,000 (Corlett 2016 ; Lughadha et al. 2016 ). The number of green plant species with sequenced genomes (1482) represents around 0.26–0.29% of plant species, so only a fraction of them has been sequenced so far. Despite an uneven distribution, the reported genomes span around 500 million years of evolution and comprise the major clades of green plants (Viridiplantae) (Fig. 2 ). Nuclear plant genome size varies greatly among the sequenced species, from 9 Mb to 31 Gb (Fig. 2 ). In contrast to more than 3000-fold difference in genome size, the number of protein-coding genes per genome varies much less, only in the range of a few-fold difference (Fig. 3 b). Based on the 685 available annotated plant genomes depicted in Fig. 3 b, the mean number of protein-coding genes is 34,071 (Table S1 ). Large genome sizes are attributed in part to polyploidy events common in plants, but mainly to the activity of transposable elements (Michael and Jackson 2013 ; Michael 2014 ; Kersey 2019 ; Kress et al. 2022 ; Marks et al. 2021 ).
Furthermore, we can see that the model species and many agriculturally and economically important plant species have been sequenced (Figs. 1 and 2 ). Without doubt, the number of sequenced genomes and phylogenetic distributions of them will soon increase and expand, because of many current genome initiatives. Projects affiliated to the Earth BioGenome Project (Lewin et al. 2018 , 2022 ), is the Darwin Tree of Life Project that aims to sequence all 70,000 species in Britain and Ireland (Darwin Tree of Life Project Consortium 2022 ). Another example is the 10KP (10,000 Plants) Initiative, which aims to sequence genomes of 10,000 species representing every major clade of embryophytes (land plants), green algae (chlorophytes and streptophytes), and protists (photosynthetic and heterotrophic) (Cheng et al. 2018 ). Other initiatives are the African BioGenome Project (AfricaBP) aiming to sequence genomes of 105,000 endemic species, including plants (Ebenezer et al. 2022 ), the African Orphan Crops Consortium (AOCC) aiming to sequence 101 African orphan crops/trees (Hendre et al. 2019 ), and the Genomics for Australian Plants (GAP) consortium aiming to sequence representative Australian plant genomes across the plant tree of life (Genomics for Australian Plants Initiative 2018 ; McLay et al. 2022 ).
Mostly, when sequencing a genome, the genome of one individual species is sequenced, which will be used as the reference genome. However, this is unlikely to be the complete picture. Genetic differences among individual species may exist. To overcome this, the term pan-genome was coined. The first report was based on the sequencing of eight bacterial strains and the observation that not every gene was present in each strain (Tettelin et al. 2005 ). It refers to the ´whole´ genome within a species (Golicz et al. 2020 ; Bayer et al. 2020 ). A pan-genome can be made by sequencing different individuals, accessions, cultivars, or populations, and then by ´joining´ the information, the whole genetic diversity will be captured, in principle (Lei et al. 2021 ; Li et al. 2022 ). In plants, the first pan-genome was made for wild soybean ( Glycine soja ), by sequencing and de novo assembly of seven phylogenetically and geographically representative accessions (Li et al. 2014 ). To date, around 30 plant pan-genomes, mostly of crops, have been published (Li et al. 2022 ). To create pan-genomes, long read sequencing is used. Normally, for re-sequencing efforts, short read sequencing is used, which allows the detection of single nucleotide polymorphisms (SNPs), but structural variants (SVs) are more difficult to identify (Golicz et al. 2020 ).
For comparative plant genomics, we refer readers to the useful website Phytozome (Goodstein et al. 2012 ).
How plant genomes facilitate plant functional genomics
Gene function discovery using mutant collections.
With the availability of genome sequences, the identification of gene functions via mutant screens became much easier. To go from a phenotype to the probable casual mutation induced by ethyl methanesulfonate (EMS) mutagenesis using classical forward genetic screens involved long and laborious mapping strategies. Nowadays, mapping can be performed by sequencing the genomes of a population of backcrossed homozygous plants with the phenotype of interest, which allows the rapid identification of the casual mutation (Hartwig et al. 2012 ; Garcia et al. 2016 ).
In reverse genetic screens, starting with a gene of interest and determining the phenotype/function (Alonso and Ecker 2006 ), for 20 years the Arabidopsis community has used insertional T-DNA mutant collections where sequence information is available for most of the random T-DNA insertions in the genome, arguably, the most widely used is the SALK T-DNA collection (Alonso et al. 2003 ). Various other valuable sequenced collections of T-DNA, transposon insertion, or variations, are available for Arabidopsis (Samson et al. 2002 ; Sessions et al. 2002 ; Rosso et al. 2003 ; Woody et al. 2007 ), and for other model species such as rice (Wang et al. 2013 ; Wei et al. 2013 ), maize (Lu et al. 2018 ), and petunia (Vandenbussche et al. 2008 , 2016 ).
There are various other techniques available for gene function discovery where genome information is very useful. An example of a reverse genetics approach to find mutations is TILLING (Targeting Induced Local Lesions IN Genomes), which is a chemical random mutagenesis approach, followed by high-throughput screening of point mutations in targeted genomic regions. The screening part can be combined with high-throughput sequencing (Mccallum et al. 2000 ; Henikoff et al. 2004 ; Tadele 2016 ). Another frequently used approach is activation tagging to identify gain-of-function mutants. For this, a mutant population is made by random genome insertions of T-DNAs or transposons carrying an activation sequence, leading to the activation of nearby genes. Recovering the flanking sequence followed by the identification of the genome region leads to the discovery of the gene in question (Weigel et al. 2000 ; Marsch-Martinez et al. 2002 ; Tani et al. 2004 ).
Other reverse genetics approaches for gene function discovery, involve making dedicated constructs targeting genes of interests, which can be used to target one or more genes. RNA interference (RNAi) (Saurabh et al. 2014 ; Muhammad et al. 2019 ) or the fusion of a transcriptional repression domain (EAR domain) (Hiratsu et al. 2003 ; Mitsuda et al. 2011 ) can be used to obtain loss-of-function mutants. Another approach is the use of artificial miRNAs (amiRNAs) to silence genes. An amiRNA can be designed to silence one gene or a family of redundant genes (Schwab et al. 2006 ; Ossowski et al. 2008 ). A last example of an approach, still relatively new but already very actively used, is using a CRISPR-Cas system (Wada et al. 2020 ; Zhu et al. 2020 ; Gaillochet et al. 2021 ). The used guide RNAs (gRNAs) are typically directed towards coding regions, but can also be directed towards promoters or non-coding regions. Furthermore, multiple gRNAs can be cloned in the same vector to target different genes (Najera et al. 2019 ) or promoters (Rodríguez-Leal et al. 2017 ). Having the genome information, genome-wide screens can be made using pooled CRISPR libraries (Huang et al. 2022 ; Liu et al. 2023 ; Pan et al. 2023 ), and various reports have already been published such as in rice (Lu et al. 2017 ; Meng et al. 2017 ), tomato (Jacobs et al. 2017 ), soybean (Bai et al. 2020 ), maize (Liu et al. 2020 ), and canola (He et al. 2023 ).
The use of CRISPR systems, for ´traditional´ genome editing or for gene activation/repression, may fill the gap of functional genomics in plant species, beyond the model species currently used (Huang et al. 2022 ; Liu et al. 2023 ; Pan et al. 2023 ). With the use of pooled CRISPR libraries, massive plant transformation could be applied in different species. Sharing of whole genome gRNA library data, pooled libraries, and even complete transformed CRISPR mutant populations in the form of seeds could make a usage boost to functional studies. As mentioned above, 4,604 nuclear plant genomes have been sequenced, corresponding to 1482 plant species (Fig. 1 ), most functional genomics research is performed in a rough estimate of only 1–2% of plant species with genome information so far. The future holds interesting opportunities for the use of genome information.
OMICS technologies
In addition to genomics, there are now many other omics technologies available. All these technologies benefit greatly from genome information. Many efforts exist generating plant transcriptomes from model species but also non-model species, even from species with no genome information yet. For the latter, mapping of the sequence reads is done against the genome of the evolutionary closest species or reads can be mapped (and gene expression quantified) against a de novo assembled transcriptome from the target organism. In general, transcriptome information also helps to improve genome annotations. Many databases exist to explore transcriptome data such as BAR (Winter et al. 2007 ), Genevestigator (Zimmermann et al. 2004 ), and Plant Public RNA-seq Database (Yu et al. 2022 ). Other databases contain data from large initiatives like the 1KP (1000 Plants), where transcriptomes of 1124 species were sequenced to infer the phylogenomic relationships (Matasci et al. 2014 ; Leebens-Mack et al. 2019 ). Another initiative is the JGI Plant Gene Atlas, which contains almost 2100 RNA-Seq data sets collected from 18 plant species, with the aim to improve functional gene descriptions across the plant kingdom (Sreedasyam et al. 2023 ). Recently, a great number of specialized single cell and single nuclei transcriptome data sets are emerging (reviewed in: Seyfferth et al. 2021 ; Cervantes-Perez et al. 2022 ; Denyer and Timmermans 2022 ; Nolan and Shahan 2023 ; Zheng et al. 2023 ) and databases holding single cell transcriptome data (e.g., Ma et al. 2020 ; Wendrich et al. 2020 ; Chen et al. 2021a ; He et al. 2023 ).
Plant proteomics is also a large field and benefits from genome information, including transcriptome information, first to be able to predict all proteins and isoforms (Chen et al. 2021a , b ; Mergner and Kuster 2022 ). Many proteomic studies, from small studies to very large studies, and even pan-plant proteomes have been reported in the literature (e.g., McWhite et al. 2020 ; Mergner et al. 2020 ; van Wijk et al. 2021 , 2024 ).
An omics area that has a growing significance that can improve draft plant genomes, correct gene annotation, discover new translation initial sites, ORFs, and alternative splicing, and verify novel genes of the peptide/protein level is called proteogenomics (Nesvizhskii 2014 ; Song et al. 2023 ). The usefulness of proteogenomics has been illustrated for instance for the model organism Arabidopsis (e.g., Castellana et al. 2008 ; Zhu et al. 2017 ; Willems et al. 2017 , 2022 ). Recent examples of proteogenomics in other species are for sweet cherry and pear (Xanthopoulou et al. 2021; Wang et al. 2023 ).
Another big omics technology is metabolomics. Metabolomics is a good tool for functional genomics (Schauer and Fernie 2006 ). It is a powerful technique to analyze the metabolite content in plants and is less restricted to genome information or model species. Though limitations for metabolomics in some (non-model) plants are the lack of high-quality metabolite databases, such that some molecules cannot easily be unambiguously identified. On the other hand, combining different types of omics data can lead to the discovery of gene functions and help in future plant improvements (Kumar et al. 2017 ; Patel et al. 2021 ; Shen et al. 2023 ).
Evolution and domestication
Genome information facilitates the study of phylogenetic relationships among species. Furthermore, the importance of genes or gene families in the evolution of land plants can be studied (Yu et al. 2018 ; Leebens-Mack et al. 2019 ; Soltis and Soltis 2021 ; Guo et al. 2023 ). Another example facilitated by genome information is the study of domestication. Hundreds of plant species have been domesticated by humans by selecting for beneficial traits (Gepts 2004 ; Meyer and Purugganan 2013 ). Through candidate gene studies, quantitative trait locus (QTL) mapping and cloning, genome-wide association studies (GWASs), and whole-genome resequencing studies, a significant number of domestication or domestication-related genes have been discovered and isolated (Meyer and Purugganan 2013 ; Kantar et al. 2017 ). More recently, reports on pan-genomes also facilitate the study of evolution and domestication, and the identification of key genes associated with important agronomic traits (Li et al. 2022 ).
Interestingly, de novo domestication by genome editing has been used (Bartlett et al. 2023 ). For instance, using CRISPR-Cas9, this has been done in the wild tomato species (Li et al. 2018 ; Zsögön et al. 2018 ), in the Solanaceae species ´groundcherry´ (Lemmon et al. 2018 ), and in wild rice (Yu et al. 2021 ). Knowledge on domesticated genes was used to edit several of these genes at once, resulting directly in a ´crop´ with desirable agricultural traits.
Conclusion and perspective
In recent years, the number of sequenced plant genomes has increased at an incredible speed. It is clear that this will only continue, and in the near future we will have tens of thousands of sequenced plant genomes. This wealth of information will accelerate studies on plant biology, functional genomics, evolution of genomes and genes, domestication processes, phylogenetic relationships, among many others. In parallel, new and improved bioinformatics analysis methods will have to be developed.
The field of single cell genomics will also expand and will also come with technical challenges such as capturing more cells, capturing low-abundance cells, cell-type annotation, new sequencing and analysis methods (Efroni and Birnbaum 2016 ; Conde and Kirst 2022 ; Cuperus 2022 ). Moreover, this will not only apply to transcriptomics, but in all omics fields we are going to see a rapid expansion, from single cell omics , single cell multi- omics , spatial genomics and other omics , new omics analysis methods, and to inference of gene regulatory networks using single cell omics data, among others (Thibivilliers and Libault 2021 ; Clark et al. 2022 ; Yu et al. 2023 ; Baysoy et al. 2023 ).
The genome evolution and phylogenomic research field will have an ever-growing amount of data available for analyses. Furthermore, there is a great potential for the use of functional genomics data for genome-editing of crops and for the de novo domestication for future crops using this same technology (Fernie and Yan 2019 ; Zhou et al. 2020 ; Zaidi et al. 2020 ; Gao 2021 ; Kumar et al. 2022 ; Yu and Li 2022 ; Bartlett et al. 2023 ). Importantly, when it comes to crop yield, knowledge is required how to properly evaluate this (Khaipho-Burch et al. 2023 ).
Lastly, Artificial Intelligence (AI) is certainly going to play a role in the plant science fields discussed here. Predictive models or analysis methods are developed based on machine learning (ML) and deep learning (DL) (Wang et al. 2020 ; van Dijk et al. 2021 ; Xu et al. 2021 ; Holzinger et al. 2023 ). Besides ChatGPT as a tool to ask or write texts, among other tasks (OpenAI; https://chat.openai.com/chat ), probably one of the best-known tools now in life sciences, is AlphaFold and its successor Alphafold2, a model that can predict almost all protein tertiary structures (Senior et al. 2020 ; Jumper et al. 2021 ). Other examples are the use of AI in image analysis and image-based phenotyping, having autonomous robots and/or drones for plant phenotyping, pest management, fertilizer management, or harvesting (Harfouche et al. 2023 ; Holzinger et al. 2023 ; Murphy et al. 2024 ). Furthermore, AI can be applied in bioinformatic analysis, to improve genome annotations, predict with high accuracy specific motifs in regulatory regions, gene function prediction, or predict the import nucleotide region or gene(s) in EMS screens or QTL analysis, etc. These are just a few examples of the many possibilities of the use of AI now and in the near future.
In conclusion, plant genomics will undoubtedly remain a cornerstone, actively contributing to the ongoing advancement of plant science and its practical applications.
Data availability
The datasets generated during and/or analyzed during the current study are available from the corresponding author upon reasonable request.
Alonso JM, Ecker JR (2006) Moving forward in reverse: Genetic technologies to enable genome-wide phenomic screens in Arabidopsis. Nat Rev Genet 7:524–536. https://doi.org/10.1038/nrg1893
Article CAS PubMed Google Scholar
Alonso JM, Stepanova AN, Leisse TJ, Kim CJ, Chen H, Shinn P et al (2003) Genome-Wide Insertional Mutagenesis of Arabidopsis thaliana . Science 301:653–656. https://doi.org/10.1126/science.1086391
Article PubMed Google Scholar
Arabidopsis Genome Initiative (2000) Analysis of the genome sequence of the flowering plant Arabidopsis thaliana . Nature 408:796–815. https://doi.org/10.1038/35048692
Article Google Scholar
Bai M, Yuan J, Kuang H, Gong P, Li S, Zhang Z et al (2020) Generation of a multiplex mutagenesis population via pooled CRISPR-Cas9 in soya bean. Plant Biotechnol J 18:721–731. https://doi.org/10.1111/pbi.13239
Bartlett ME, Moyers BT, Man J et al (2023) The power and perils of De Novo domestication using genome editing. Annu Rev Plant Biol 74:727–750. https://doi.org/10.1146/annurev-arplant-053122
Bayer PE, Golicz AA, Scheben A et al (2020) Plant pan-genomes are the new reference. Nat Plants 6:914–920. https://doi.org/10.1038/s41477-020-0733-0
Baysoy A, Bai Z, Satija R, Fan R (2023) The technological landscape and applications of single-cell multi-omics. Nat Rev Mol Cell Biol 24:695–713. https://doi.org/10.1038/s41580-023-00615-w
Belser C, Istace B, Denis E et al (2018) Chromosome-scale assemblies of plant genomes using nanopore long reads and optical maps. Nat Plants 4:879–887. https://doi.org/10.1038/s41477-018-0289-4
Blaxter M, Archibald JM, Childers AK et al (2022) Why sequence all eukaryotes? Proc Natl Acad Sci U S A 119:1–9. https://doi.org/10.1073/pnas.2115636118
C. elegans Sequencing Consortium (1998) Genome Sequence of the Nematode C. elegans : a platform for investigating biology. Science 282:2012–2018. https://doi.org/10.1126/science.282.5396.2012
Castellana NE, Payne SH, Shen Z et al (2008) Discovery and revision of Arabidopsis genes by proteogenomics. Proc Natl Acad Sci U S A 105:21034–21038. https://doi.org/10.1073/PNAS.0811066106/SUPPL_FILE/ST5.XLS
Article CAS PubMed PubMed Central Google Scholar
Cervantes-Pérez SA, Thibivillliers S, Tennant S, Libault M (2022) Review: Challenges and perspectives in applying single nuclei RNA-seq technology in plant biology. Plant Sci 325:111486. https://doi.org/10.1016/J.PLANTSCI.2022.111486
Chen F, Dong W, Zhang J et al (2018) The sequenced angiosperm genomes and genome databases. Front Plant Sci. https://doi.org/10.3389/fpls.2018.00418
Article PubMed PubMed Central Google Scholar
Chen H, Yin X, Guo L et al (2021a) Plant scRNAdb: A database for plant single-cell RNA analysis. Mol Plant 14:855–857
Chen Y, Wang Y, Yang J et al (2021b) Exploring the diversity of plant proteome. J Integr Plant Biol 63:1197–1210
Cheng CY, Krishnakumar V, Chan AP et al (2017) Araport11: a complete reannotation of the Arabidopsis thaliana reference genome. Plant J 89:789–804. https://doi.org/10.1111/tpj.13415
Cheng S, Melkonian M, Smith SA et al (2018) 10KP: a phylodiverse genome sequencing plan. Gigascience 7:1–9. https://doi.org/10.1093/gigascience/giy013
Clark NM, Elmore JM, Walley JW (2022) To the proteome and beyond: advances in single-cell omics profiling for plant systems. Plant Physiol 188:726–737. https://doi.org/10.1093/plphys/kiab429
Conde D, Kirst M (2022) Decoding exceptional plant traits by comparative single-cell genomics. Trends Plant Sci 27:1095–1098. https://doi.org/10.1016/j.tplants.2022.08.006
Corlett RT (2016) Plant diversity in a changing world: Status, trends, and conservation needs. Plant Divers 38:10–16. https://doi.org/10.1016/j.pld.2016.01.001
Cuperus JT (2022) Single-cell genomics in plants: current state, future directions, and hurdles to overcome. Plant Physiol 188:749–755. https://doi.org/10.1093/plphys/kiab478
Darwin Tree of Life Project Consortium (2022) Sequence locally, think globally: The Darwin Tree of Life Project. PNAS 119:1–7. https://doi.org/10.1073/pnas.2115642118
Article CAS Google Scholar
Denyer T, Timmermans MCP (2022) Crafting a blueprint for single-cell RNA sequencing. Trends Plant Sci 27:92–103
Ebenezer TE, Muigai AWT, Nouala S et al (2022) Africa: sequence 100,000 species to safeguard biodiversity Setting the agenda in research. Nature 603:388–392
Efroni I, Birnbaum KD (2016) The potential of single-cell profiling in plants. Genome Biol 17:65. https://doi.org/10.1186/s13059-016-0931-2
Fernie AR, Yan J (2019) De novo domestication: an alternative route toward new crops for the future. Mol Plant 12:615–631. https://doi.org/10.1016/j.molp.2019.03.016
Gaillochet C, Develtere W, Jacobs TB (2021) CRISPR screens in plants: approaches, guidelines, and future prospects. Plant Cell 33:794–813. https://doi.org/10.1093/PLCELL/KOAB099
Gao C (2021) Genome engineering for crop improvement and future agriculture. Cell 184:1621–1635. https://doi.org/10.1016/j.cell.2021.01.005
Garcia V, Bres C, Just D et al (2016) Rapid identification of causal mutations in tomato EMS populations via mapping-by-sequencing. Nat Protoc 11:2401–2418. https://doi.org/10.1038/nprot.2016.143
Genomics for Australian Plants Initiative (2018) https://doi.org/10.25953/3108-3v82
Gepts P (2004) Crop domestication as a long-term selection experiment. In: Janick J (ed) Plant breeding reviews. Wiley. https://doi.org/10.1002/9780470650288.ch1
Chapter Google Scholar
Goff SA, Ricke D, Lan T-H et al (2002) A draft sequence of the rice genome ( Oryza sativa L. ssp. japonica). Science 296:92–100. https://doi.org/10.1126/science.1068275
Golicz AA, Bayer PE, Bhalla PL et al (2020) Pangenomics comes of age: from bacteria to plant and animal applications. Trends Genet 36:132–145. https://doi.org/10.1016/j.tig.2019.11.006
Goodstein DM, Shu S, Howson R, et al (2012) Phytozome: A comparative platform for green plant genomics. Nucleic Acids Res 40. https://doi.org/10.1093/nar/gkr944
Guo C, Luo Y, Gao LM, Yi T et al (2023) Phylogenomics and the flowering plant tree of life. J Integr Plant Biol 65:299–323. https://doi.org/10.1111/jipb.13415
Harfouche AL, Nakhle F, Harfouche AH et al (2023) A primer on artificial intelligence in plant digital phenomics: embarking on the data to insights journey. Trends Plant Sci 28:154–184. https://doi.org/10.1016/J.TPLANTS.2022.08.021
Hartwig B, James GV, Konrad K et al (2012) Fast isogenic mapping-by-sequencing of ethyl methanesulfonate-induced mutant bulks. Plant Physiol 160:591–600. https://doi.org/10.1104/pp.112.200311
He Z, Luo Y, Zhou X, Zhu T, Lan Y, Chen D (2023) scPlantDB: a comprehensive database for exploring cell types and markers of plant cell atlases. Nucleic Acids Res. https://doi.org/10.1093/nar/gkad706
Hendre PS, Muthemba S, Kariba R et al (2019) African Orphan Crops Consortium (AOCC): status of developing genomic resources for African orphan crops. Planta 250:989–1003. https://doi.org/10.1007/s00425-019-03156-9
Henikoff S, Till BJ, Comai L (2004) TILLING. Traditional mutagenesis meets functional genomics. Plant Physiol 135:630–636. https://doi.org/10.1104/pp.104.041061
Henry RJ (2022) Progress in plant genome sequencing. Appl Biosci 1:113–128. https://doi.org/10.3390/applbiosci1020008
Hiratsu K, Matsui K, Koyama T, Ohme-Takagi M (2003) Dominant repression of target genes by chimeric repressors that include the EAR motif, a repression domain, in Arabidopsis. Plant J 34:733–739. https://doi.org/10.1046/J.1365-313X.2003.01759.X
Holzinger A, Keiblinger K, Holub P et al (2023) AI for life: trends in artificial intelligence for biotechnology. N Biotechnol 74:16–24. https://doi.org/10.1016/J.NBT.2023.02.001
Huang Y, Shang M, Liu T, Wang K (2022) High-throughput methods for genome editing: the more the better. Plant Physiol 188:1731–1745. https://doi.org/10.1093/plphys/kiac017
International Human Genome Sequencing Consortium (2001) Initial sequencing and analysis of the human genome. Nature 409:860–921. https://doi.org/10.1038/35057062
Jacobs TB, Zhang N, Patel D, Martin GB (2017) Generation of a collection of mutant tomato lines using pooled CRISPR libraries. Plant Physiol 174:2023–2037. https://doi.org/10.1104/pp.17.00489
Jumper J, Evans R, Pritzel A et al (2021) Highly accurate protein structure prediction with AlphaFold. Nature 596:583. https://doi.org/10.1038/s41586-021-03819-2
Kantar MB, Nashoba AR, Anderson JE et al (2017) The genetics and genomics of plant domestication. Bioscience 67:971–982. https://doi.org/10.1093/biosci/bix114
Kersey PJ (2019) Plant genome sequences: past, present, future. Curr Opin Plant Biol 48:1–8. https://doi.org/10.1016/J.PBI.2018.11.001
Khaipho-Burch M, Cooper M, Crosssa J, de Leon N, Holland James LR et al (2023) Scale up trials to validate modified crops’ benefits. Nature 621:470–473
Kress WJ, Soltis DE, Kersey PJ et al (2022) Green plant genomes: what we know in an era of rapidly expanding opportunities. PNAS 119:1–9. https://doi.org/10.1073/pnas.2115640118
Krishnakumar V, Contrino S, Cheng CY, Belyaeva I, Ferlanti ES, Miller JR et al (2017) Thalemine: a warehouse for Arabidopsis data integration and discovery. Plant Cell Physiol 58:e4. https://doi.org/10.1093/pcp/pcw200
Kumar R, Bohra A, Pandey AK et al (2017) Metabolomics for plant improvement: Status and prospects. Front Plant Sci. https://doi.org/10.3389/fpls.2017.01302
Kumar K, Mandal SN, Pradhan B et al (2022) From evolution to revolution: accelerating crop domestication through genome editing. Plant Cell Physiol 63:1607–1623. https://doi.org/10.1093/PCP/PCAC124
Leebens-Mack JH, Barker MS, Carpenter EJ et al (2019) One thousand plant transcriptomes and the phylogenomics of green plants. Nature 574:679–685. https://doi.org/10.1038/s41586-019-1693-2
Lei L, Goltsman E, Goodstein D et al (2021) Plant pan-genomics comes of age. Annu Rev Plant Biol 72:411–413. https://doi.org/10.1146/annurev-arplant-080720
Lemmon ZH, Reem NT, Dalrymple J et al (2018) Rapid improvement of domestication traits in an orphan crop by genome editing. Nat Plants 4:766–770. https://doi.org/10.1038/s41477-018-0259-x
Lewin HA, Robinson GE, Kress WJ et al (2018) Earth BioGenome Project: Sequencing life for the future of life. R Bot Gardens 115:4325–4333. https://doi.org/10.1073/pnas.1720115115
Lewin HA, Richards S, Lieberman Aiden E et al (2022) The Earth BioGenome Project 2020: starting the clock. PNAS 119:1–7. https://doi.org/10.1073/pnas.2115635118
Li YH, Zhou G, Ma J et al (2014) De novo assembly of soybean wild relatives for pan-genome analysis of diversity and agronomic traits. Nat Biotechnol 32:1045–1052. https://doi.org/10.1038/nbt.2979
Li T, Yang X, Yu Y et al (2018) Domestication of wild tomato is accelerated by genome editing. Nat Biotechnol 36:1160–1163. https://doi.org/10.1038/nbt.4273
Li W, Liu J, Zhang H et al (2022) Plant pan-genomics: recent advances, new challenges, and roads ahead. J Genet Genom 49:833–846. https://doi.org/10.1016/j.jgg.2022.06.004
Liu HJ, Jian L, Xu J et al (2020) High-throughput CRISPR/Cas9 mutagenesis streamlines trait gene identification in maize. Plant Cell 32:1397–1413. https://doi.org/10.1105/tpc.19.00934
Liu T, Zhang X, Li K et al (2023) Large-scale genome editing in plants: approaches, applications, and future perspectives. Curr Opin Biotechnol 79:102875. https://doi.org/10.1016/J.COPBIO.2022.102875
Lu Y, Ye X, Guo R et al (2017) Genome-wide targeted mutagenesis in rice using the CRISPR/Cas9 system. Mol Plant 10:1242–1245. https://doi.org/10.1016/j.molp.2017.06.007
Lu X, Liu J, Ren W et al (2018) Gene-indexed mutations in maize. Mol Plant 11:496–504. https://doi.org/10.1016/j.molp.2017.11.013
Lughadha EN, Govaerts R, Belyaeva I et al (2016) Counting counts: Revised estimates of numbers of accepted species of flowering plants, seed plants, vascular plants and land plants with a review of other recent estimates. Phytotaxa 272:82–88. https://doi.org/10.11646/phytotaxa.272.1.5
Ma X, Denyer T, Timmermans MCP (2020) PscB: A browser to explore plant single cell RNA-sequencing data sets. Plant Physiol 183:464–467. https://doi.org/10.1104/pp.20.00250
Marks RA, Hotaling S, Frandsen PB, VanBuren R (2021) Representation and participation across 20 years of plant genome sequencing. Nat Plants 7:1571–1578. https://doi.org/10.1038/s41477-021-01031-8
Marsch-Martinez N, Greco R, Van Arkel G et al (2002) Activation tagging using the En-I maize transposon system in Arabidopsis. Plant Physiol 129:1544–1556. https://doi.org/10.1104/pp.003327
Matasci N, Hung LH, Yan Z et al (2014) Data access for the 1,000 Plants (1KP) project. Gigascience 3:17. https://doi.org/10.1186/2047-217X-3-17
Mccallum CM, Comai L, Greene EA, Henikoff S (2000) Targeted screening for induced mutations. Nat Biotechnol 18:455–457. https://doi.org/10.1038/74542
McLay TGB, Murphy DJ, Holmes GD, Mathews S, Brown GK et al (2022) A genome resource for Acacia, Australia’s Largest Plant Genus. PLoS ONE 17:e0274267. https://doi.org/10.1371/journal.pone.0274267
McWhite CD, Papoulas O, Drew K et al (2020) A pan-plant protein complex map reveals deep conservation and novel assemblies. Cell 181:460-474.e14. https://doi.org/10.1016/j.cell.2020.02.049
Meng X, Yu H, Zhang Y et al (2017) Construction of a genome-wide mutant library in rice using CRISPR/Cas9. Mol Plant 10:1238–1241. https://doi.org/10.1016/j.molp.2017.06.006
Mergner J, Kuster B (2022) Annual review of plant biology plant proteome dynamics. Annu Rev Plant Biol 73:67–92. https://doi.org/10.1146/annurev-arplant-102620
Mergner J, Frejno M, List M et al (2020) Mass-spectrometry-based draft of the Arabidopsis proteome. Nature 579:409–414. https://doi.org/10.1038/s41586-020-2094-2
Meyer RS, Purugganan MD (2013) Evolution of crop species: genetics of domestication and diversification. Nat Rev Genet 14:840–852. https://doi.org/10.1038/nrg3605
Meyerowitz EM (2001) Prehistory and history of arabidopsis research. Plant Physiol 125:15–19. https://doi.org/10.1104/pp.125.1.15
Michael TP (2014) Plant genome size variation: bloating and purging DNA. Brief Funct Genomics 13:308–317. https://doi.org/10.1093/BFGP/ELU005
Michael TP, VanBuren R (2020) Building near-complete plant genomes. Curr Opin Plant Biol 54:26–33. https://doi.org/10.1016/j.pbi.2019.12.009´
Michael TP, Jackson S (2013) The first 50 plant genomes. The Plant Genome 6:1–7. https://doi.org/10.3835/plantgenome2013.03.0001in
Mitsuda N, Takiguchi Y, Shikata M et al (2011) The new fioreDB database provides comprehensive information on plant transcription factors and phenotypes induced by CRES-T in ornamental and model plants. Plant Biotechnol 28:123–130. https://doi.org/10.5511/plantbiotechnology.11.0106a
Muhammad T, Zhang F, Zhang Y, Liang Y (2019) RNA interference: a natural immune system of plants to counteract biotic stressors. Cells 38(8):38. https://doi.org/10.3390/CELLS8010038
Murphy KM, Ludwig E, Gutierrez J, Gehan MA (2024) Deep learning in image-based plant phenotyping. Ann Rev Plant Biol. https://doi.org/10.1146/annurev-arplant-070523-042828
Najera VA, Twyman RM, Christou P, Zhu C (2019) Applications of multiplex genome editing in higher plants. Curr Opin Biotechnol 59:93–102. https://doi.org/10.1016/j.copbio.2019.02.015
Nesvizhskii AI (2014) Proteogenomics: concepts, applications and computational strategies. Nat Methods 11:1114–1125. https://doi.org/10.1038/nmeth.3144
Nolan TM, Shahan R (2023) Resolving plant development in space and time with single-cell genomics. Curr Opin Plant Biol 76:102444. https://doi.org/10.1016/j.pbi.2023.102444
Ossowski S, Schwab R, Weigel D (2008) Gene silencing in plants using artificial microRNAs and other small RNAs. Plant J 53:674–690. https://doi.org/10.1111/j.1365-313X.2007.03328.x
Pan C, Li G, Bandyopadhyay A, Qi Y (2023) Guide RNA library-based CRISPR screens in plants: opportunities and challenges. Curr Opin Biotechnol 79:102883. https://doi.org/10.1016/j.copbio.2022.102883
Pasha A, Shabari S, Cleary A, Chen X, Berardini T, Farmer A et al (2020) Araport lives: an updated framework for Arabidopsis bioinformatics. Plant Cell 32:2683–2686. https://doi.org/10.1105/tpc.20.00358
Patel MK, Pandey S, Kumar M et al (2021) Plants metabolome study: emerging tools and techniques. Plants 10:2409. https://doi.org/10.3390/plants10112409
Provart NJ, Alonso J, Assmann SM et al (2016) 50 years of Arabidopsis research: Highlights and future directions. New Phytol 209:921–944. https://doi.org/10.1111/nph.13687
Provart NJ, Brady SM, Parry G et al (2021) Anno genominis XX: 20 years of Arabidopsis genomics. Plant Cell 33:832–845. https://doi.org/10.1093/plcell/koaa038
Rhee SY, Beavis W, Berardini TZ et al (2003) The Arabidopsis Information Resource (TAIR): A model organism database providing a centralized, curated gateway to Arabidopsis biology, research materials and community. Nucleic Acids Res 31:224–228. https://doi.org/10.1093/nar/gkg076
Rodríguez-Leal D, Lemmon ZH, Man J et al (2017) Engineering quantitative trait variation for crop improvement by genome editing. Cell 171:470-480.e8. https://doi.org/10.1016/j.cell.2017.08.030
Rosso MG, Li Y, Strizhov N et al (2003) An Arabidopsis thaliana T-DNA mutagenized population (GABI-Kat) for flanking sequence tag-based reverse genetics. Plant Mol Biol 53:247–259. https://doi.org/10.1023/B:PLAN.0000009297.37235.4a
Samson F, Brunaud V, Balzergue S et al (2002) FLAGdb/FST: a database of mapped flanking insertion sites (FSTs) of Arabidopsis thaliana T-DNA transformants. Nucleic Acids Res 30:94–97. https://doi.org/10.1093/nar/30.1.94
Saurabh S, Vidyarthi AS, Prasad D (2014) RNA interference: Concept to reality in crop improvement. Planta 239:543–564. https://doi.org/10.1007/S00425-013-2019-5
Schauer N, Fernie AR (2006) Plant metabolomics: towards biological function and mechanism. Trends Plant Sci 11:508–516. https://doi.org/10.1016/j.tplants.2006.08.007
Schwab R, Ossowski S, Riester M et al (2006) Highly specific gene silencing by artificial microRNAs in Arabidopsis. Plant Cell 18:1121–1133. https://doi.org/10.1105/tpc.105.039834
Senior AW, Evans R, Jumper J et al (2020) Improved protein structure prediction using potentials from deep learning. Nature 577:706–710. https://doi.org/10.1038/s41586-019-1923-7
Sessions A, Burke E, Presting G et al (2002) A high-throughput Arabidopsis reverse genetics system. Plant Cell 14:2985–2994. https://doi.org/10.1105/tpc.004630
Seyfferth C, Renema J, Wendrich JR, Eekhout T, Seurinck R, Vandamme N et al (2021) Advances and opportunities in single-cell transcriptomics for plant research. Annu Rev Plant Biol 72:847–866. https://doi.org/10.1146/annurev-arplant-081720-010120
Sharma P, Al-Dossary O, Alsubaie B et al (2021) Improvements in the sequencing and assembly of plant genomes. GigaByte. https://doi.org/10.46471/gigabyte.24
Shen S, Zhan C, Yang C, Fernie AR, Luo J (2023) Metabolomics-centered mining of plant metabolic diversity and function: past decade and future perspectives. Mol Plant 16:43–63. https://doi.org/10.1016/j.molp.2022.09.007
Shendure J, Balasubramanian S, Church GM et al (2017) DNA sequencing at 40: Past, present and future. Nature 550:345–353. https://doi.org/10.1038/nature24286
Soltis PS, Soltis DE (2021) Plant genomes: markers of evolutionary history and drivers of evolutionary change. Plants, People, Planet 3:74–82. https://doi.org/10.1002/PPP3.10159
Somssich M (2019) A short history of Arabidopsis thaliana (L.) Heynh. Columbia-0. PeerJ Prepr. https://doi.org/10.7287/peerj.preprints.26931v5
Song YC, Das D, Zhang Y et al (2023) Proteogenomics-based functional genome research: approaches, applications, and perspectives in plants. Trends Biotechnol 41:1532–1548. https://doi.org/10.1016/J.TIBTECH.2023.05.010
Sreedasyam A, Plott C, Hossain MS et al (2023) JGI Plant Gene Atlas: an updateable transcriptome resource to improve functional gene descriptions across the plant kingdom. Nucleic Acids Res 51:8383–8401. https://doi.org/10.1093/nar/gkad616
Sun Y, Shang L, Zhu QH et al (2022) Twenty years of plant genome sequencing: achievements and challenges. Trends Plant Sci 27:391–401. https://doi.org/10.1016/j.tplants.2021.10.006
Tadele Z (2016) Mutagenesis and TILLING to dissect gene function in plants. Curr Genomics 17:499–508. https://doi.org/10.2174/138920291766616052010
Tani H, Chen X, Nurmberg P et al (2004) Activation tagging in plants: a tool for gene discovery. Funct Integr Genomics 4:258–266. https://doi.org/10.1007/s10142-004-0112-3
Tettelin H, Masignani V, Cieslewicz MJ et al (2005) Genome analysis of multiple pathogenic isolates of Streptococcus agalactiae : Implications for the microbial ‘pan-genome’. PNAS 102:13950–13955. https://doi.org/10.1073/pnas.0506758102
Thibivilliers S, Libault M (2021) Plant Single-cell multiomics: cracking the molecular profiles of plant cells. Trends Plant Sci 26:662–663. https://doi.org/10.1016/j.tplants.2021.03.001
Toufighi K, Brady SM, Austin R, Ly E, Provart NJ (2005) The botany array resource: e-Northerns, expression angling, and promoter analyses. Plant J 43:153–163. https://doi.org/10.1111/j.1365-313X.2005.02437.x
Tuskan GA, Difazio S, Jansson S et al (2006) The Genome of Black Cottonwood, Populus trichocarpa (Torr. & Gray). Science 313:1596–1604. https://doi.org/10.1126/science.1128691
van Dijk ADJ, Kootstra G, Kruijer W, de Ridder D (2021) Machine learning in plant science and plant breeding. iScience 24:101890. https://doi.org/10.1016/J.ISCI.2020.101890
Van Wijk KJ, Leppert T, Sun Q et al (2021) The Arabidopsis PeptideAtlas: harnessing worldwide proteomics data to create a comprehensive community proteomics resource. Plant Cell 33:3421–3453. https://doi.org/10.1093/PLCELL/KOAB211
van Wijk KJ, Leppert T, Sun Z et al (2024) Detection of the arabidopsis proteome and its post-translational modifications and the nature of the unobserved (Dark) proteome in PeptideAtlas. J Proteome Res 23:185–214. https://doi.org/10.1021/acs.jproteome.3c00536
Vandenbussche M, Janssen A, Zethof J et al (2008) Generation of a 3D indexed Petunia insertion database for reverse genetics. Plant J 54:1105–1114. https://doi.org/10.1111/j.1365-313X.2008.03482.x
Vandenbussche M, Chambrier P, Bento SR, Morel P (2016) Petunia, your next supermodel? Front Plant Sci. https://doi.org/10.3389/fpls.2016.00072
Velasco R, Zharkikh A, Troggio M et al (2007) A high quality draft consensus sequence of the genome of a heterozygous grapevine variety. PLoS ONE. https://doi.org/10.1371/journal.pone.0001326
Venter JC, Adams MD, Myers EW et al (2001) The sequence of the human genome. Science 291:1304–1351. https://doi.org/10.1126/science.1058040
Wada N, Ueta R, Osakabe Y, Osakabe K (2020) Precision genome editing in plants: state-of-the-art in CRISPR/Cas9-based genome engineering. BMC Plant Biol 20:234. https://doi.org/10.1186/s12870-020-02385-5
Wang N, Long T, Yao W et al (2013) Mutant resources for the functional analysis of the rice genome. Mol Plant 6:596–604. https://doi.org/10.1093/mp/sss142
Wang H, Cimen E, Singh N, Buckler E (2020) Deep learning for plant genomics and crop improvement. Curr Opin Plant Biol 54:34–41. https://doi.org/10.1016/J.PBI.2019.12.010
Wang P, Wu X, Shi Z et al (2023) A large-scale proteogenomic atlas of pear. Mol Plant 16:599–615. https://doi.org/10.1016/j.molp.2023.01.011
Wei FJ, Droc G, Guiderdoni E, Hsing YC (2013) International consortium of rice mutagenesis: resources and beyond. Rice. https://doi.org/10.1186/1939-8433-6-39
Weigel D, Ahn JH, Blàzquez MA et al (2000) Activation Tagging in Arabidopsis. Plant Physiol 122:1003–1013. https://doi.org/10.1104/pp.122.4.1003
Wendrich JR, Yang BJ, Vandamme N, Verstaen K, Smet W, Van de Velde C et al (2020) Vascular transcription factors guide plant epidermal responses to limiting phosphate conditions. Science 370:6518. https://doi.org/10.1126/science.aay4970
Willems P, Ndah E, Jonckheere V et al (2017) N-terminal proteomics assisted profiling of the unexplored translation initiation landscape in Arabidopsis thaliana . Mol Cell Proteom 16:1064–1080. https://doi.org/10.1074/mcp.M116.066662
Willems P, Ndah E, Jonckheere V et al (2022) To new beginnings: riboproteogenomics discovery of N-terminal proteoforms in Arabidopsis thaliana . Front Plant Sci 12:778804. https://doi.org/10.3389/FPLS.2021.778804/BIBTEX
Winter D, Vinegar B, Nahal H et al (2007) An “electronic fluorescent pictograph” Browser for exploring and analyzing large-scale biological data sets. PLoS ONE. https://doi.org/10.1371/journal.pone.0000718
Woody ST, Austin-Phillips S, Amasino RM, Krysan PJ (2007) The WiscDsLox T-DNA collection: An arabidopsis community resource generated by using an improved high-throughput T-DNA sequencing pipeline. J Plant Res 120:157–165. https://doi.org/10.1007/s10265-006-0048-x
Xanthopoulou A, Moysiadis T, Bazakos C et al (2022) The perennial fruit tree proteogenomics atlas: a spatial map of the sweet cherry proteome and transcriptome. Plant J 109:1319–1336. https://doi.org/10.1111/TPJ.15612
Xie L, Gong X, Yang K et al (2024) Technology-enabled great leap in deciphering plant genomes. Nat Plants. https://doi.org/10.1038/s41477-024-01655-6
Xu Y, Liu X, Cao X et al (2021) Artificial intelligence: a powerful paradigm for scientific research. The Innovation 2:100179. https://doi.org/10.1016/J.XINN.2021.100179
Yu H, Li J (2022) Breeding future crops to feed the world through de novo domestication. Nat Commun 13:1171. https://doi.org/10.1038/s41467-022-28732-8
Yu J, Hu S, Wang J et al (2002) A Draft Sequence of the Rice Genome ( Oryza sativa L. ssp. indica). Science 296:79–92. https://doi.org/10.1126/science.1068037
Yu X, Yang D, Guo C, Gao L (2018) Plant phylogenomics based on genome-partitioning strategies: progress and prospects. Plant Divers 40:158–164. https://doi.org/10.1016/J.PLD.2018.06.005
Yu H, Lin T, Meng X et al (2021) A route to de novo domestication of wild allotetraploid rice. Cell 184:1156-1170.e14. https://doi.org/10.1016/j.cell.2021.01.013
Yu Y, Zhang H, Long Y et al (2022) Plant public RNA-seq database: a comprehensive online database for expression analysis of ~45 000 plant public RNA-Seq libraries. Plant Biotechnol J 20:806–808. https://doi.org/10.1111/pbi.13798
Yu X, Liu Z, Sun X (2023) Single-cell and spatial multi-omics in the plant sciences: technical advances, applications, and perspectives. Plant Commun 4:100508. https://doi.org/10.1016/j.xplc.2022.100508
Zaidi SSEA, Mahas A, Vanderschuren H, Mahfouz MM (2020) Engineering crops of the future: CRISPR approaches to develop climate-resilient and disease-resistant plants. Genome Biol 21:289. https://doi.org/10.1186/s13059-020-02204-y
Zheng D, Xu J, Lu Y et al (2023) Recent progresses in plant single-cell transcriptomics. Crop Design 2:100041. https://doi.org/10.1016/j.cropd.2023.100041
Zhou J, Li D, Wang G et al (2020) Application and future perspective of CRISPR/Cas9 genome editing in fruit crops. J Integr Plant Biol 62:269–286. https://doi.org/10.1111/jipb.12793
Zhu FY, Chen MX, Ye NH et al (2017) Proteogenomic analysis reveals alternative splicing and translation as part of the abscisic acid response in Arabidopsis seedlings. Plant J 91:518–533. https://doi.org/10.1111/TPJ.13571
Zhu H, Li C, Gao C (2020) Applications of CRISPR–Cas in agriculture and plant biotechnology. Nat Rev Mol Cell Biol 21:661–677. https://doi.org/10.1038/s41580-020-00288-9
Zimmermann P, Hirsch-Hoffmann M, Hennig L, Gruissem W (2004) GENEVESTIGATOR. Arabidopsis microarray database and analysis toolbox. Plant Physiol 136:2621–2632. https://doi.org/10.1104/pp.104.046367
Zsögön A, Čermák T, Naves ER et al (2018) De novo domestication of wild tomato using genome editing. Nat Biotechnol 36:1211–1216. https://doi.org/10.1038/nbt.4272
Download references
We thank the Consejo Nacional de Humanidades, Ciencias y Tecnologías (CONAHCYT) from Mexico for a PhD fellowship to JJBG. The work in the SDF laboratory was financed by the CONAHCYT grants CB-2017–2018-A1-S-10126 and CF-2019–6360.
Author information
Authors and affiliations.
Unidad de Genómica Avanzada (UGA-Langebio), Centro de Investigación y de Estudios Avanzados del Instituto Politécnico Nacional (Cinvestav), Irapuato, Mexico
Judith Jazmin Bernal-Gallardo & Stefan de Folter
You can also search for this author in PubMed Google Scholar
Contributions
SDF conceived the study. JJBG analysed genome sequence information and made the figures. SDF wrote the manuscript. Both read and approved the final version.
Corresponding author
Correspondence to Stefan de Folter .
Ethics declarations
Conflict of interest.
The authors declare that the research was conducted in the absence of any commercial or financial relationships that could be construed as a potential conflict of interest. SDF is the EiC of this journal but was not involved in the evaluation of this manuscript.
Additional information
Communicated by Gerhard Leubner.
Publisher's Note
Springer Nature remains neutral with regard to jurisdictional claims in published maps and institutional affiliations.
Supplementary Information
Below is the link to the electronic supplementary material.
Supplementary file1 (XLSX 723 KB)
Rights and permissions.
Open Access This article is licensed under a Creative Commons Attribution 4.0 International License, which permits use, sharing, adaptation, distribution and reproduction in any medium or format, as long as you give appropriate credit to the original author(s) and the source, provide a link to the Creative Commons licence, and indicate if changes were made. The images or other third party material in this article are included in the article's Creative Commons licence, unless indicated otherwise in a credit line to the material. If material is not included in the article's Creative Commons licence and your intended use is not permitted by statutory regulation or exceeds the permitted use, you will need to obtain permission directly from the copyright holder. To view a copy of this licence, visit http://creativecommons.org/licenses/by/4.0/ .
Reprints and permissions
About this article
Bernal-Gallardo, J.J., de Folter, S. Plant genome information facilitates plant functional genomics. Planta 259 , 117 (2024). https://doi.org/10.1007/s00425-024-04397-z
Download citation
Received : 11 January 2024
Accepted : 20 March 2024
Published : 09 April 2024
DOI : https://doi.org/10.1007/s00425-024-04397-z
Share this article
Anyone you share the following link with will be able to read this content:
Sorry, a shareable link is not currently available for this article.
Provided by the Springer Nature SharedIt content-sharing initiative
- Plant genomes
- Plant development
- Find a journal
- Publish with us
- Track your research
An integrated toolkit for human microglia functional genomics
Affiliations.
- 1 Center for Translational and Computational Neuroimmunology, Columbia University Medical Center, New York, NY, USA.
- 2 Taub Institute for Research on Alzheimer's Disease and Aging Brain, Columbia University Medical Center, New York, NY, USA.
- 3 Department of Neurology, Columbia University Medical Center, New York, NY, USA.
- 4 Department of Physiology and Cellular Biophysics, Columbia University Medical Center, New York, NY, USA.
- 5 Neuroimmunology Core, Center for Translational & Computational Neuroimmunology, Division of Neuroimmunology, Department of Neurology, Columbia University Medical Center, New York, NY, USA.
- 6 Proteomics Core, Department of Pathology and Cell Biology, Columbia University Medical Center, New York, NY, USA.
- 7 Department of Medicine, Department of Systems Biology, Columbia University Irving Medical Center, New York, NY, 10032, USA.
- 8 Rush Alzheimer's Disease Center, Rush University Medical Center, Chicago, IL, USA.
- 9 Center for Translational and Computational Neuroimmunology, Columbia University Medical Center, New York, NY, USA. [email protected].
- 10 Taub Institute for Research on Alzheimer's Disease and Aging Brain, Columbia University Medical Center, New York, NY, USA. [email protected].
- 11 Department of Neurology, Columbia University Medical Center, New York, NY, USA. [email protected].
- PMID: 38600587
- PMCID: PMC11005142
- DOI: 10.1186/s13287-024-03700-9
Background: Microglia, the brain's resident immune cells, play vital roles in brain development, and disorders like Alzheimer's disease (AD). Human iPSC-derived microglia (iMG) provide a promising model to study these processes. However, existing iMG generation protocols face challenges, such as prolonged differentiation time, lack of detailed characterization, and limited gene function investigation via CRISPR-Cas9.
Methods: Our integrated toolkit for in-vitro microglia functional genomics optimizes iPSC differentiation into iMG through a streamlined two-step, 20-day process, producing iMG with a normal karyotype. We confirmed the iMG's authenticity and quality through single-cell RNA sequencing, chromatin accessibility profiles (ATAC-Seq), proteomics and functional tests. The toolkit also incorporates a drug-dependent CRISPR-ON/OFF system for temporally controlled gene expression. Further, we facilitate the use of multi-omic data by providing online searchable platform that compares new iMG profiles to human primary microglia: https://sherlab.shinyapps.io/IPSC-derived-Microglia/ .
Results: Our method generates iMG that closely align with human primary microglia in terms of transcriptomic, proteomic, and chromatin accessibility profiles. Functionally, these iMG exhibit Ca2 + transients, cytokine driven migration, immune responses to inflammatory signals, and active phagocytosis of CNS related substrates including synaptosomes, amyloid beta and myelin. Significantly, the toolkit facilitates repeated iMG harvesting, essential for large-scale experiments like CRISPR-Cas9 screens. The standalone ATAC-Seq profiles of our iMG closely resemble primary microglia, positioning them as ideal tools to study AD-associated single nucleotide variants (SNV) especially in the genome regulatory regions.
Conclusions: Our advanced two-step protocol rapidly and efficiently produces authentic iMG. With features like the CRISPR-ON/OFF system and a comprehensive multi-omic data platform, our toolkit equips researchers for robust microglial functional genomic studies. By facilitating detailed SNV investigation and offering a sustainable cell harvest mechanism, the toolkit heralds significant progress in neurodegenerative disease drug research and therapeutic advancement.
Keywords: CRISPR; Chromatin accessibility (ATAC-Seq); Functional genomics; Microglia; Neurodegenerative diseases; Proteomics; iPSC-derived microglia (iMG).
© 2024. The Author(s).
- Alzheimer Disease* / genetics
- Amyloid beta-Peptides
- Chromatin / genetics
- Chromatin / metabolism
- Microglia / metabolism
- Neurodegenerative Diseases*
Grants and funding
- R01AG070118/AG/NIA NIH HHS/United States
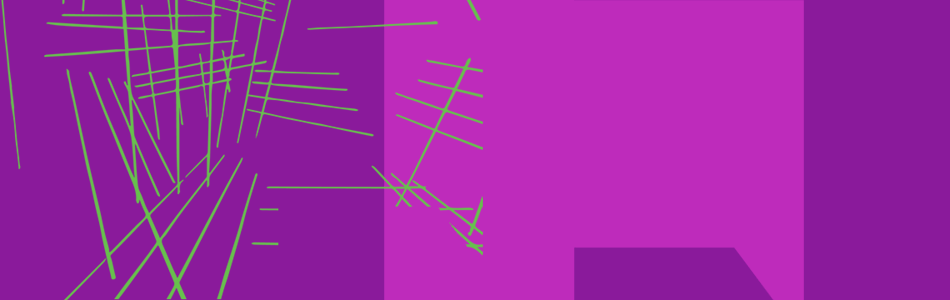
Funding opportunity: Human Functional Genomics Initiative clusters
Last updated: 31 August 2023 - see all updates
Apply for funding to lead a cluster as part of a coordinated Human Functional Genomics Initiative.
- be based at an organisation eligible for MRC funding
- meet individual eligibility requirements
Clusters could include:
- platforms using existing technologies at scale to interrogate functional readout of genetic variation
- development of novel tools, technologies and biological models for functional genomics research
- research focused on the interplay of genetic variance and physiological pathways, organs and systems in both healthy and disease states
The total fund is up to £16 million. MRC will fund up to 80% of the full economic cost (FEC) and funding will last for four years.
Please note the expression of interest stage (stage one) has closed with decisions communicated no later than 25 May 2023.
Full applications (stage two) are only permitted from invited applicants. The deadline for full applications is 27 July 2023.
Who can apply
Before applying to MRC for funding, please check the following:
- the MRC eligibility guidance for applicants
- the eligibility of your organisation
- your eligibility as an individual
- the MRC guidance for applicants , which sets out the MRC funding rules
Eligibility
To be eligible to apply for MRC funding you must:
- be a researcher or technologist employed by an eligible research organisation
- have at least a postgraduate degree, although we expect most applicants to have a PhD or medical degree and meet individual eligibility requirements
- show that you will direct the project and be actively engaged in the work
- have the relevant expertise and experience to lead an MRC project
Applications can be from a single eligible organisation or a partnership of organisations.
When there are two or more eligible organisations involved, for administrative purposes it is necessary to identify a single principal investigator who must be affiliated with the lead research organisation. However, the balance of activity and leadership across the partner organisations can be equally shared if desirable. What is critical is for the approach to leadership and decision making across multiple organisations to be clearly specified.
Where appropriate, we encourage you to include one or more project partners in your application, from industry, charities or the wider third sector.
While international organisations cannot lead an application, it is possible for an international researcher to apply, as a co-investigator. We expect international co-investigators to offer expertise or facilities not available in the UK and to provide clear indicators of commitment to the project.
Equality, diversity and inclusion (EDI)
MRC is committed to achieving equality of opportunity for all funding applicants. We encourage applications from a diverse range of researchers and technologists.
We support people to work in a way that suits their personal circumstances. This includes:
- career breaks
- support for people with caring responsibilities
- flexible working
- alternative working patterns
Read MRC’s guidance on flexible working and career breaks . You can also find out more about MRC’s current EDI initiatives and EDI at UK Research and Innovation .
Diversity is one of the core MRC values and we are committed to creating inclusive environments that encourage excellence in research through good equalities practice. We strongly encourage applications from currently underrepresented groups including female and ethnic minority researchers, and researchers with disabilities or long-term conditions. MRC expect funded projects and their leadership to be diverse. We encourage the leadership model to be inclusive, diverse, and creative, with rotation or succession of positions as appropriate.
Organisations that are not eligible to apply
These organisations cannot apply to host an award, but can participate as project partners on an application led by an eligible UK organisation:
- international research organisations
What we're looking for
The Human Functional Genomics Initiative will advance the UKRI ‘securing better health, ageing and wellbeing’ strategic priority, an initiative driven forward by all UKRI research councils.
The Human Functional Genomics Initiative aims to advance our understanding of the complexity of human physiology and how it changes over time and in disease, by exploiting recent advances in our ability to explore how genetic variation impacts complex phenotypes. This will enable the discovery and development of the next generation of genomically informed biomarkers, diagnostics, therapeutics, and preventative medicine strategies.
This is a timely opportunity to support functional genomics research as the convergence of recent advances in gene-editing technology, multimodal functional assessment at scale, artificial intelligence, and effective cell-based model systems allows us to answer questions that have previously been impossible.
In this initiative, we are seeking to fund a coordinated network of interdisciplinary clusters to drive a greater understanding of physiological pathways, organs and systems in both healthy and disease states through functional genomics research. The funded clusters are expected to complement each other, pro-actively coordinate across the initiative, work collaboratively, and provide access to the models, tools and data developed through the initiative on an open as possible, closed as necessary basis.
Read UK Research and Innovation’s (UKRI) guidance on making your research data open .
In addition to the clusters funded through this opportunity we will provide substantial ring-fenced funding to facilitate effective coordination across the initiative and with external partners, and support for platforms to curate, integrate and make available data generated via this initiative and related efforts.
Through this funding, we aim to:
- boost functional genomic capability
- develop causal models of biological processes in health and disease for functional assessment and validation
- develop new high throughput perturbation and readout technologies and tools for data analysis
This will be underpinned by support for data integration and findable, accessible, interoperable, and reusable (FAIR) data standards for data discoverability and accessibility.
Where relevant, each cluster proposal should articulate:
- the key biological and methodological challenges in functional genomics to be addressed
- health equity through population diversity of samples
- benefits of using diverse data in revealing new functional insights
- the limitations on the conclusions drawn from your own research and applicability of the research to UK and global populations
- how you will draw on or develop translatable models of human physiology across the life course for health and disease with a pathway, system, or organ focus. For example, this could include the use of longitudinal cohort or patient samples and data or development and validation of new technological approaches
You should also explain the intended outputs and impacts of the cluster proposal:
- how your work will drive deeper mechanistic understanding of the interplay of genetic variance in physiological function across the life course for healthy and disease states, and the potential impact. For example, new biomarkers, clinical positioning hypothesis or novel targets
- how the cluster will address a capability gap in the UK functional genomics research landscape
- why you are best placed to deliver it
- how what you are doing will connect with other relevant initiatives
- knowledge exchange
- sharing of tools, models and data
To be within scope, you must articulate an ambitious programme of work focused on human physiology in health and disease with clear potential to catalyse a step change in functional genomics research in a relevant biological context. The proposal could include, but is not limited to, the following:
- genome perturbation
- multiscale and multimodal functional analysis
- functional and regulatory screening
- development of novel and emerging tools and technologies to enable functional genomics research, for example, perturbation technologies, analytical methods or data methods
- bioinformatics
- computational biology and modelling
- artificial intelligence tools
- physiological and developmental states
- stage of the life course
- dynamic and spatial context
- the development of relevant translatable biological models that seek to delineate causal pathways. For example, models could include primary or induced pluripotent stem cells from healthy and patient donors
Where this is applicable to the research, we encourage the use of human biosamples and data to enhance the physiological and disease relevance and enable more rapid translation of the research.
You should also consider how the cluster will connect with and complement, rather than duplicate, existing infrastructure, initiatives and resources, including but not limited to:
- Joint AstraZeneca-Cancer Research Horizons Functional Genomics Centre
- Human Induced Pluripotent Stem Cells Initiative
- National Institute for Health and Care Research (NIHR) Bioresource
- NIHR-MRC Rare Disease Network
- Genomics England 100k Genomes Project
- UKRI artificial intelligence innovation to accelerate health research
We will not support:
- functional genomics approaches in oncology, as there is existing UK investment and expertise in this area. The greatest identified need is for investment in non-oncology approaches. The use of cancer cells lines in non-oncology projects is permitted, where appropriate
- proposals solely focused on high throughput arrayed or pooled clustered regularly interspaced short palindromic repeats (CRISPR) screening. The overall initiative will include a recent MRC investment in an industry-partnered functional genomics facility (details will be announced in due course). This will provide the UK research community with high throughput arrayed CRISPR screening capacity, expertise and technical support
- applications should primarily focus on human biological models and cells. Animal research that significantly enhances or complements activity may be considered, and, where appropriate, applicants are encouraged to engage with existing investments, for example, National Mouse Genetics Network
We also support functional genomics research focused on specific pathways, systems, or organs through MRC research boards, you should consider whether your proposed research is better aligned to these schemes:
- molecular and cellular medicine
- population and systems medicine
- infections and immunity
- neurosciences and mental health
We encourage you to contact us first at [email protected] to discuss your application.
Collaboration and principals of participation
Clusters should be organised around a defined research or methodological challenge and can include one or more eligible research organisations, as necessary. We strongly encourage applications from new groupings of researchers from multiple disciplines and research interests.
We strongly encourage collaboration with both small and large companies, including appropriate cash or in-kind contributions to the cluster, but it is not mandatory. Partnerships should be mutually beneficial and in line with MRC’s Industry Collaboration Framework .
We also encourage collaboration between academic and clinical researchers, where this is relevant to the cluster challenge.
We expect funded clusters to adopt an open and transparent approach to data sharing to enable access across the clusters, wider initiative and research community, as necessary. Read UKRI’s guidance on making your research data open .
Funded clusters are expected to champion a collaborative and open ethos to accelerate the collective impact and reach of the initiative and sustainability of its outputs.
Coordination and leadership
We will support an openly collaborative culture to enable the clusters to be outwardly facing, engaged with each other and relevant UK capabilities to allow the initiative to remain agile and responsive to emerging opportunities.
We will appoint a director of the initiative, and more information on the appointment process will be made available in due course. The role of the director will be to:
- oversee and champion the coordination of the clusters, both within the initiative and with the wider academic, clinical and industrial research communities
- advocate the principals of participation
- respond to emerging opportunities
- lead cluster engagement with an oversight board that reports to the funders
Additional funds will be available to the director to support these responsibilities.
The supporting data platform to curate, integrate and make available data generated via this initiative and related efforts will be commissioned separately by the funders
Funding and duration
- the total fund is up to £16 million and we anticipate funding four to five clusters
- we will fund 80% FEC
- projects should last four years
- projects should start by 1 April 2024
What costs we will fund
You can request funding for costs such as:
- a contribution to the salary of the principal investigator and co-investigators
- support for other posts such as research and technical
- research consumables
- travel costs
- data preservation, data sharing and dissemination costs
- estates and indirect costs
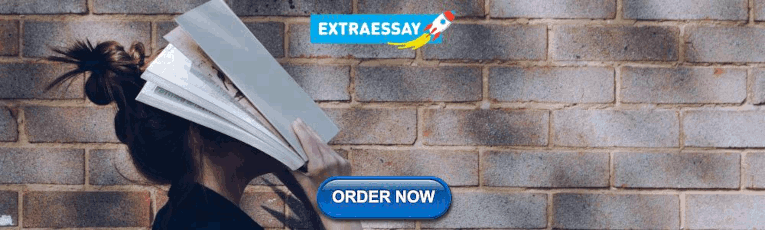
What costs we will not fund
- research involving randomised trials of clinical treatments
- costs for PhD studentships
- publication costs
Project partners
Where appropriate, we encourage the inclusion of project partners that will support the cluster through appropriate cash or in-kind contributions, such as:
- access to equipment
- sites or facilities
- the provision of data
- software or materials
We especially encourage collaboration between academic, clinical and industry researchers
At the full application stage, each project partner must provide a letter of support. If your application involves industry partners , they must provide a company letter of support if the project partner falls within the industry collaboration framework .
Find out more about subcontractors and dual roles .
Who cannot be included as a project partner
The individual named as the contact for the project partner organisation cannot also be named as staff.
How to apply
The Functional Genomics Initiative Clusters funding opportunity has two stages.
The expression of interest stage is mandatory. You must submit an expression of interest to MRC using the Joint Electronic Submission (Je-S) system and case for support template.
To manage demand, individuals may only submit one expression of interest as a principal investigator.
The expression for interest stage is open and will close on 4 May 2023 at 4:00pm UK time.
Following completion of your expression of interest, you will be notified and invited to apply to the full application stage (stage two). We will communicate expression of interest decisions no later than 25 May 2023. You can find advice on completing your Je-S expression of interest under the ‘How to apply stage one: expression of interest’ heading.
The full application stage will open on 4 April 2023, closing on 27 July 2023 at 4:00pm UK time.
How to apply stage one: expression of interest
We recommend you submit your expression of interest as soon as possible.
Your host organisation will also be able to provide advice and guidance.
Submitting your application
Before starting an application, you will need to log in or create an account in Je-S.
When applying:
- Select ‘documents’, then ‘new document’.
- Select ‘call search’.
- To find the opportunity, search for: Expression of Interest Functional genomics 2023.
This will populate:
- council: MRC
- document type: outline proposal
- scheme: standard outline
- call/type/mode: Expression of Interest Functional Genomics 2023
Once you have completed your application, make sure you ‘submit document’ to your research office for checking and approval.
You can save completed details in Je-S at any time and return to continue your application later.
MRC must receive your expression of interest application by 4 May 2023 at 4:00pm UK time.
You will not be able to apply after this time. Please leave enough time for your proposal to pass through your organisation’s Je-S submission route before this date.
You should ensure you are aware of and follow any internal institutional deadlines that may be in place.
What to include within your expression of interest application
You must follow the below guidance before accessing Je-S.
Je-S application
Project details section.
Search the database to add the lead research organisation and department, where the funded award will be held.
Project title
The title should be as informative as possible, capturing the essence of the research challenge. It should not exceed 150 characters. Avoid using specialist characters and symbols.
Start date and duration
The start date should be no later than 1 April 2024
The project duration will be 48 months.
Investigators
All applicants eligible to be included as part of the proposed leadership team, should be added to the Je-S application as an investigator.
If the application is to be delivered in partnership by two or more eligible organisations, then the principal investigator must be from the organisation which will lead the partnership (as detailed within project details section). All host organisations must be represented by an eligible co-investigator.
Each member of the leadership team will need an active Je-S account at the required level (proposal Je-S account type), to allow their inclusion as an investigator. The individual who will act as the grant holder with responsibilities to MRC at the start of the MRC award should be included within the application as principal investigator, this is for administrative purposes. Other leadership team members should be the application co-investigators (including international researchers if applicable).
The leadership team members’ application roles should not imply relative status or influence the leadership model which is for the applicants to propose.
These roles and people added do not limit who might be recruited to team at the full application.
If you are a researcher based in the UK, an overseas MRC unit or an international researcher who has not created a Je-S account, navigate to the Je-S home page and select ‘create an account’ .
Principal investigator
Search the Je-S database to add the principal investigator and select ‘save’.
Co-investigator
Search the Je-S database to add all other members of the leadership team as co-investigator and select ‘save’. Repeat process to add all new co-investigators (including any international researchers assisting with the leadership of the project).
Researcher co-investigator
Search the Je-S database to add all other members of the leadership team as researcher co-investigator and select ‘save’. Repeat process to add all researcher co-investigators (including any international researcher co-investigators assisting with the leadership of the project).
Researcher co-investigator status is aimed at researchers who are currently not eligible to be a principal investigator or a co-investigator on a grant but who provide significant intellectual input to grant writing and design.
To be considered suitable for the status of researcher co-investigator I, applicants are expected to not be eligible as a principal investigator or co-investigator of a research grant in their own right (for example, because they do not have a contract of employment with any of the participating research organisations for the duration of the grant prior to application). This could include, but is not limited to:
- postdoctoral researchers
- technology specialists
- clinical fellows
For further information related to researcher co-investigator eligibility.
If project partners will be involved in the project, please note, they are not required to be detailed at the expression of interest stage.
If your application involves one or more industrial partners, you should review the information published within the MRC Industry Collaboration Framework (ICF) to decide if you should submit your full application under the ICF.
Select the option to indicate research grant and save.
Case for support attachment
All applicants are required to use the expression of interest template (DOCX, 263KB) , designed for this expression of interest stage.
When you have downloaded and completed the case for support template, you are required to upload this to the attachments section of the Je-S expression of interest outline application.
How to apply stage two: full application
A full application is only permitted after receipt of your expression of interest application (stage one) and subsequent invitation to submit a full application (stage two).
All investigators involved in the project need to be registered on Je-S.
Any investigators who do not have a Je-S account must register for one at least seven working days before the funding opportunity deadline.
- To find the opportunity, search for: Functional Genomics clusters Initiative 2023.
- document type: standard proposal
- scheme: research grant
- call/type/mode: Functional Genomics clusters Initiative 2023
MRC must receive your full application by 27 July 2023 at 4:00pm UK time.
What to include with your application
In addition to the Je-S application, you will also need to include the following mandatory attachments:
- a curriculum vitae (CV) for each named researcher, including investigators and named researchers. Each CV should not exceed two sides of A4
- publications (should not exceed one side of A4 per named person)
- a case for support, which length should not exceed 12 pages
- a justification of resources (should not exceed two sides of A4)
- a data management plan (DMP). Page length can vary, see section 2.2.7 of our attachments guidance . You must use the available DMP template form
If your research includes excess treatment costs of studies involving human participants you will need to include a schedule of events cost attribution template (SoECAT). For details and access to the SoECAT form see the National Institute for Health and Care Research’s information on excess treatment costs .
If there will be a researcher co-investigator on your project you will need to include a statement of support for researcher co-investigators. This should not exceed two sides of A4 or equivalent on headed paper or a PDF of an email.
Optional attachments include:
- covering letter: this can be up to two sides of A4 using a sans-serif typeface (Arial or equivalent) and font size of 11pt
- letters of support: each letter should not exceed two sides of A4 or equivalent on headed paper or a PDF of an email
- Gantt chart: chart should not exceed one side of A4 or equivalent
You can find full details of what to include in mandatory and optional attachments in section 2.2 attachments guidance .
Guidance for applicants
The MRC guidance for applicants :
- helps you check your eligibility
- guides you through preparing an application
- shows you how to prepare a case for support
- provides details of any ethical and regulatory requirements that may apply
Industrial partner information
If you want to include one or more industry partners as a project partner, you must also complete the project partner section in Je-S .
Each project partner must provide a project partner letter of support, which should not exceed two pages of A4 on headed paper or a PDF of an email. The letter must:
- be an integral part of the application
- focus on the application it accompanies which includes the requirement to include a project partner letter of support
Full details of the content the project partner should include in their letter of support, are provided in section 2.2.6 of the MRC guidance for applicants .
If your application involves the collaboration of one or more industrial partners, you should review the information published within the MRC ICF to decide if you should submit your application under the ICF.
After reading the ICF information, if you decide that your application will include industry collaboration, you will need to include the following within your application for each collaborating industry partner:
- ICF company partner letter of support
The completed ICF form should be uploaded to the Je-S attachments section using the ‘MICA form’ document type. Please type ‘Industry Collaboration Framework form’ in the description box.
The company letter of support must use the available template and be uploaded to the relevant project partner entry you are required to add to your Je-S application.
Research disruption caused by COVID-19 pandemic
You have the option to include a one-page annex to the case for support. You can use it to provide additional information explaining any disruptions you have encountered to previous or current research, caused by the COVID-19 pandemic (where relevant to your pending application).
For further information relating to the annex, please see the MRC guidance for applicants (section 2.2.3.6) .
How we will assess your application
Stage one: expression of interest.
Information provided as part of the expression of interest will not be formally assessed. MRC head office will use this information to:
- check remit and fit to funding opportunity scope
- anticipate expected submission levels
- identify panel members
In the event of high demand for this funding opportunity, MRC reserves the right to introduce shortlisting during the assessment process.
Stage two: full application
Full applications will be assessed by an expert review panel on 14 to 15 September 2023. We expect to publish the membership of the panel on this web page before the full application deadline.
The panel will assess applications using MRC’s core research grant assessment criteria, within the context of the scope of this funding opportunity. Read our detailed assessment criteria .
In addition to the core assessment criteria, the panel will consider the following:
Collaboration
- is there convincing commitment to the principals of participation?
- is there evidence of previous collaborative and coordination activities?
- what are the outlined processes to enable sharing of models, tools and data developed through the initiative?
- Is the assembled team composed of a suitable breadth of researchers and partnering organisations?
- Is there alignment and complementarity with other proposed clusters and existing infrastructure, initiatives and resources?
Expert review panel
An expert review panel will review your application against the criteria, using the MRC scoring matrix . At the end of the meeting, the panel will discuss final funding recommendations, considering the score of each proposal and the potential complementarity of clusters, with the aim of supporting the strongest possible portfolio.
We aim to communicate funding decisions within two weeks of the full panel meeting in September 2023.
If your application was discussed by the expert review panel and they provided feedback, this will be sent to you within six weeks of the panel meeting.
Principles of assessment
UK Research and Innovation (UKRI) supports the San Francisco declaration on research assessment and recognises the relationship between research assessment and research integrity.
Find out about the UKRI principles of assessment and decision making .
Contact details
Get help with your application.
For help on costings and writing your application, contact your research office. Allow enough time for your organisation’s submission process.
Ask about this funding opportunity
Functional genomics team.
Email: [email protected]
Include ‘Functional Genomics’ in the subject line.
We aim to respond within five working days.
Get help with applying through Je-S
01793 444164
Opening times
Je-S helpdesk opening times
Additional info
Supporting documents.
Expression of interest template (DOCX, 263KB)
Webinar questions and answers (PDF, 76KB)
Webinar presentation (PDF, 1.1MB)
Panel membership (PDF, 85KB)
- 31 August 2023 Panel membership added under 'Supporting documents' in the 'Additional info' section. Panel meeting date changed from 'September 2023' to '14 to 15 September 2023'.
This is the website for UKRI: our seven research councils, Research England and Innovate UK. Let us know if you have feedback or would like to help improve our online products and services .

An official website of the United States government
The .gov means it’s official. Federal government websites often end in .gov or .mil. Before sharing sensitive information, make sure you’re on a federal government site.
The site is secure. The https:// ensures that you are connecting to the official website and that any information you provide is encrypted and transmitted securely.
- Publications
- Account settings
Preview improvements coming to the PMC website in October 2024. Learn More or Try it out now .
- Advanced Search
- Journal List
- Cancers (Basel)

Clinical Functional Genomics
Simple summary.
Functional genomics refers to the activity of the genome, that is, how the information contained in DNA (the book) is read and ‘acted upon’ in a biological context. Genes are turned ‘on’ (resulting in the synthesis of RNA that is translated into proteins) or ‘off’ during development and in response to environmental stimuli. Mis-regulation of these process can manifest as disease. Functional genomics are currently being developed clinically to improve patient care, with some clear potential future goals within the field. This commentary discusses rapidly evolving clinical functional genomic pathways and the underpinning technologies that have allowed for recent research and scientific advancements, and addresses challenges faced in the field.
Functional genomics is the study of how the genome and its products, including RNA and proteins, function and interact to affect different biological processes. The field of functional genomics includes transcriptomics, proteomics, metabolomics and epigenomics, as these all relate to controlling the genome leading to expression of particular phenotypes. By studying whole genomes—clinical genomics, transcriptomes and epigenomes—functional genomics allows the exploration of the diverse relationship between genotype and phenotype, not only for humans as a species but also in individuals, allowing an understanding and evaluation of how the functional genome ‘contributes’ to different diseases. Functional variation in disease can help us better understand that disease, although it is currently limited in terms of ethnic diversity, and will ultimately give way to more personalized treatment plans.
1. Introduction
The human genome is arguably the most useful information we currently possess to help improve patient health and is a key to medical advancement, as whole genome sequencing (WGS) of an individual maps out their entire unique genome, making it possible to pinpoint abnormalities or monitor patient improvement as a treatment is given.
The functional genome, namely the transcriptome, proteome, metabolome and epigenome, together contribute to specifying the phenotype of an organism. Information in genes as DNA is transcribed into RNA then translated into proteins; this, plus epigenetic ‘chemical tags’ induced by environmental factors, write the biological code that causes a specific phenotype. Clinical functional genomics [ 1 ] explores how these processes influence disease development and is starting to play in increasingly important role in both diagnostic and prognostic procedures. The advent of more sophisticated technologies to identify the expression profile of RNA molecules within individual cells, and spatially resolved in diseased tissues, offers a new paradigm in both hematology and pathology. This, coupled with the advent of gene editing technologies, is leading to a new era of understanding diseases, and from which new diagnostics and therapeutics will emerge.
2.1. Clinical Functional Genomic Pathway
Functional genomic analysis can be performed on a patient sample using high-throughput technologies like bulk RNA sequencing or newer methods, including spatial transcriptomics, to provide insight into the cellular transcriptome [ 2 ]. These are compatible with next generation sequencing (NGS) which is a massively parallel, high-throughput technology that rapidly determines the order of nucleotides in entire genomes or targeted regions [ 3 ]. It is also possible to use microarrays to profile multi-gene expression, however, while this method is more cost-effective, it does not give the same complete picture as RNA sequencing or spatial transcriptomics. If only a small number of genes need to be tested, real-time PCR provides a highly sensitive and cost-efficient method of choice. Downstream bioinformatic analysis of the patient’s unique functional genome can identify diseases or disorders, allowing for a tailored treatment plan specific to the patient. This more accurate diagnosis and treatment results in better prognosis for patients and is the fundamental basis of precision medicine.
2.1.1. Current Clinical Research
The field of functional genomics is of great relevance clinically to cancer patients, where most research and development is currently focused. Changes in the genome or epigenome can cause cancer by promoting uncontrolled cell growth or causing the immune system to fail to destroy tumors. Using a clinical functional genomics approach can allow for earlier and more accurate cancer diagnosis, leading to more accurate treatment options and better prognosis for patients. The UK government is hopeful about staying at the forefront of genomic research and new discoveries using the initiative ‘National NHS Genomic Medicine Service’. In the UK, WGS has already begun to enter clinical practice and it is the government’s aim to increase this and to offer elements of the functional genomic pathway for diagnosis of cancer. Using functional genomic methods such as RNA sequencing has been shown to successfully detect relapsing cancer up to 200 days before the relapse appears on a CT scan. This success has led to RNA sequencing being introduced more routinely in cancer diagnostics [ 4 ]. Functional genomic techniques open the door to personalized medicine, especially for cancer patients, and allow doctors to form personalized treatment plans. WGS and epigenomics for an individual patient with cancer will identify genetic mutations and epigenetic alterations as circulating biomarkers; in cancers, these are circulating tumor cells (CTCs) and circulating tumor DNA (ctDNA) which can originate from the primary tumor, providing early detection. Biomarkers from metastasizing tumors are also extremely difficult to detect as they are present in such low abundance and can only be detected using highly sensitive technologies.
The Functional Genomics Centre at AstraZeneca is currently working with Cancer Research UK using clustered regularly interspaced short palindromic repeats (CRISPR) technology to study cancer biology and create better, more appropriate biological models. Alongside this, they are developing informatic processes to analyze ever-increasing dataset numbers [ 5 ]. The clinical implications are the possibility of developing more effective cancer treatments. Similarly, the company GSK joined forces with the University of California to form the Laboratory for Genomic Research (LGR). The main focus of the LGR is to use functional genomics to improve drug discovery methods using CRISPR to knock out or alter multiple genes in one experiment at scale. This allows scientists to explore the function of genetic variants quickly and simply, with the ultimate goal of new and improved drug production, leading to more novel therapies since these methods increase clinical success [ 6 ].
2.1.2. Current Clinical Applications
The rapid development of functional genomics in the past two decades has allowed for breakthrough discoveries in certain cancer research, such as in acute myeloid leukemia (AML) and breast cancer studies. Novel molecular biology technologies, such as short hairpin RNA (shRNA) and CRISPR-Cas9, and advances in bioinformatics have allowed for wider research into the physiopathology of AML, resulting in a cure being within reach [ 7 ]. Furthermore, functional genomics have been used effectively to guide drug development, including one of the earliest examples; the discovery that the gene HER2 is overexpressed in certain types of breast cancers, which led to development of a drug, Herceptin [ 8 , 9 ]. Identifying drug targets within diseases allows for more rapid drug development or the repurposing of existing drugs. This is majorly beneficial to the pharmaceutical industry as it lowers costs and speeds up development times. If similar studies and clinical trials were to be conducted on other diseases, it could rapidly lead to deeper understanding of the diseases and improve drug discovery.
2.1.3. Benefits of This Field to Science and Public Health
Variant interpretation—deciding if a genetic change is pathogenic or benign—is a common issue caused by lack of understanding of biological functions within the genome [ 1 ]. Functional genomic studies investigate biological processes and how genes, RNA and proteins interact to form phenotypes, rather than just exploring the genome per se. These processes and pathways are complicated due to the ever-changing nature of the transcriptome, epigenome, proteome and metabolome. A clinical functional genomic approach can improve this issue by linking WGS datasets with functional omics datasets. Interpretation can be improved by widening datasets, such as increasing ethnical diversity within studies. This could lead to significant clinical results for patients by improving understanding of biological processes to potentially reveal insights that were not possible to achieve with the genome alone.
2.1.4. Challenges Currently Being Faced within the Field
High costs are currently one of the major factors limiting the clinical expansion of the field. Lowering the cost of sequencing whole genomes would make it more accessible and easier to integrate into healthcare systems around the world. According to pricing from the US National Human Genome Research Institute, the cost per megabase of sequence data has plateaued since 2016 and remains fairly high, at around USD 1000 per genome for sequencing alone. The cost of genome sequencing per cancer case is roughly GPB 6850 in the UK [ 10 ], therefore despite the service being made available to the NHS, certain health trusts/boards may not offer it routinely due to high costs. However, these hospitals should be encouraged to perform genome sequencing as an investment in healthcare since treatment cost are significantly lower if the cancer is diagnosed early [ 11 ]; the UK governments’ ‘Life Science Vision’ is focused on such early diagnosis and prevention [ 12 ].
Functional genomic studies should lead to equitable personalized medicine. However, a challenge faced within the research is that there is not enough population and demographic diversity. Sirugo et al. determined that most genome-wide association studies (GWAS) are performed in high income countries and the percentage of individuals in GWAS based on ethnicity were 78% European, 10% Asian, 2% African, and all other ethnicities represented 1% or less of GWAS [ 13 ]. This is not equitable representation, and this information disparity can cause clinical genome interpretation to be less reliable for the underrepresented minorities. As use of WGS and functional genomics begins to increase clinically, we must ensure that reference genomes are diverse enough to be used for all populations and ethnicities. If ethnical diversity in genome studies is not increased, this will block large groups of people from accessing this form of healthcare.
Since functional genomics looks at more than just the genome, it is currently uncertain whether some functional aspects will be tissue- or cell-dependent and therefore it is unclear how well functional data obtained from blood samples can compare directly to difficult-to-collect tissue samples, e.g., from the brain. Comprehensive functionally annotated genomes are beginning to be assembled, enabling scientists to compare vast amounts of genomic data to assess this issue in depth [ 1 ].
Most multi-omics studies have been performed on animal models rather than human cells or tissues, and this creates the issue of then translating this functional genomic data into data that are useful or applicable in human disease. This issue can be resolved in the future by performing clinical trials on humans or by using the engineered humanized physiologically relevant animal models in research studies.
Ethical restrictions impact most areas of scientific research, with this field being no exception. The rapid growth of technologies and knowledge in this field has allowed for many discoveries, however the time taken to assess risks and benefits of clinical genomic testing has and will continue to slow down research, although thoroughness is necessary to ensure the safety of patients. The discussion around the ethics of human gene editing has led to many prominent scientists insisting on banning human germline editing while research is conducted to prove it can be done safely and effectively [ 14 ]. While this does slow down progress, there is a broad societal consensus that this is an important step since the technology is not yet properly understood.
2.2. Functional Genomics Technology Platforms
The rapid development of new technologies over recent years has increased and continues to increase our ability to analyze the genome, transcriptome, and quantify the proteome and metabolome of single cells. Functional genomics uses single cell analysis technologies, like single cell sequencing, alongside omics datasets and high-throughput technologies, which enable simultaneous analysis of thousands of single cells, to study and understand how genetic variants can affect disease pathogenesis [ 12 ]. Other techniques such as mass spectrometry have recently reemerged as key analytical tools for proteomic and metabolomic analysis of single cells.
2.2.1. Single Cell Analysis
Single cell analysis ( Figure 1 ) was developed only a decade ago in 2011 and has become majorly beneficial to the field of functional genomics. It has enabled a view of cell-specific and cell-cell interactions at a single cell level. This in-depth view of cells allows molecular profiling that previously could not be revealed and, moving forward, will be particularly beneficial in hematological cancer diagnosis and monitoring, as well as in monitoring and understanding immune responses to, for example, SARS-CoV-2 in COVID-19. A single-cell multi-omics approach is perhaps the best way forward in the characterization of cancer functional genomes as it is the only technique that can achieve full resolution of tumor heterogeneity [ 14 ].

Single cell transcriptomics is a technology used to resolve RNA-seq data at a single cell level, and thereby all mRNAs (and miRNA and lncRNA where the technology permits). Cells may be derived from homogenized tissues, but also from the immune cell component (Buffy coat) from whole blood samples. This technique allows for gene activity measurements from all cells present in a sample, assuming highly efficient cell labeling. Cell samples are isolated, and each cell is individually labeled using sequencing bar code technologies, e.g., microfluidics. Labeled cells can then be re-pooled and sequenced. Finally, data are analyzed, and gene clusters are associated into cell types or tissue domains. Adapted from Single Cell Sequencing, by BioRender.com (2021). Retrieved from https://app.biorender.com/biorender-templates .
2.2.2. NanoString
NanoString is a type of DNA microarray originally developed for use in cancer diagnostics but now has many clinical applications alongside oncology, such as immunology research. The amplification-free NanoString nCounter single cell assay system is highly sensitive and measures nucleic acid content by counting molecules and directly profiling them in a highly multiplexed single reaction to profile gene expression. This eliminates amplification bias, meaning this technology presents a potentially more reliable way to analyze DNA or RNA [ 15 ]. NanoString should currently be viewed as complementary to NGS and not a full replacement in all settings [ 16 ].
2.2.3. Spatial Transcriptomics
Spatial transcriptomics ( Figure 2 ) is a molecular profiling method that allows gene activity to be mapped across a tissue sample. The main method used to perform this is by positioning the sample on an array of spatially barcoded reverse-transcription primers that attach to mRNA with oligo(dT) tails. The library product is compatible with NGS technologies, which allows for massive transcriptional profiling. This method is incredibly useful as it gives scientists insight into an individual’s entire tissue sample which could help diagnose a disease, for example, through determining cellular heterogeneity, and even towards cancer stem cell identification [ 17 ].

Spatial transcriptomics is a technology used to spatially resolve RNA-seq data, and thereby all mRNAs, in individual tissue sections. This technique allows for gene activity measurements and mapping in tissue samples. Tissue samples are prepared on glass slides and, by means of tissue-domain oligo primers, genes are encoded. Finally, data are analyzed, and gene clusters are associated with tissue domains. Reprinted from Spatial Transcriptomics, by BioRender.com (2021). Retrieved from https://app.biorender.com/biorender-templates , accessed on 3 September 2021.
2.2.4. The Use of CRISPR-Cas9 within Functional Genomic Studies
CRISPR-Cas9 ( Figure 3 ) is a revolutionary discovery that allows for the editing of genes that have been found to be, for example, mutated in diseases, though studying such mutations in model systems using a functional genomics approach will allow the consequences of genetic mutations to be determined. From this, approaches that will change the way we treat certain diseases and identify drug targets will be discovered.

CRISPR/Cas9 is a powerful tool for genome engineering. The Cas9 complex with a sgRNA recognizes a specific sequence, the protospacer. This is only possible if this sequence is followed by a Protospacer Adjacent Motif (PAM). When Cas9 binds, a dsDNA break is generated. Then, non-homologous end joining or homology-directed repair can occur, leading to mutations or gene changes, respectively. Reprinted from CRISPR/Cas9 Gene Editing, by BioRender.com (2021). Retrieved from https://app.biorender.com/biorender-templates , accessed on 3 September 2021.
CRISPR edits genes by releasing guide RNA (gRNA) that targets and attaches to a specific section of DNA within the genome. This allows the enzyme Cas9 nuclease to cut the DNA at that section which activates the cell’s own DNA repair process. Sequences within the cut gene can be edited or deleted and replaced with a new DNA sequence. This process is quick and easy, making it very cost-efficient. The ability to edit genes quickly and inexpensively allows for experiments to determine causes of diseases that were previously unknown [ 5 ]. CRISPR-Cas9 could become a key part of the genetic screening industry as it can be used to engineer embryos, although there are many ethical debates surrounding this topic currently. All programmable nucleases, including CRISPR nucleases, are in the process of being clinically investigated to gather enough evidence to suggest whether they are safe and effective. If they are proven to be effective and safe in clinical trials, they could be used to treat patients with a wide variety of diseases, from hereditary blindness to cancers.
Despite the potential benefits, there are issues with the programmable nucleases that have come to light during clinical trials. They can cause unwanted and dangerous mutations; this is a big issue with regards to using the technology with humans, as mutations may contribute to oncogenesis. The enzyme Cas9 can be immunogenic, therefore initial clinical trials have only been conducted in an immunologically privileged organ, the eye. Certain editing approaches have started to be developed that appear to overcome some of the challenges of working with nucleases to edit genomes. These editors use a Cas9 nickase, which unlike a wild-type nuclease produces DNA single-strand breaks, not double-strand breaks. This means the nickase editors are unlikely to cause large deletions or chromosomal rearrangements during the DNA repair process. Ultimately, this engineered gene editing nuclease appears to work more reliably and efficiently at correcting genes. Due to the reduced risk of unwanted mutations, these base and prime editors would be ideal for germline or in utero editing in the future if these processes become legal. These processes could eventually be used to correct pathogenic mutations in human embryos, since gene editing in newborns is generally inefficient. This ethical discussion may come around sooner than expected as society is always looking for ways to improve public health, both after and potentially even before birth. However, it is important not to rush this process as, despite the promising results of current base and prime editors, they can still be improved [ 14 ].
When CRISPR-Cas9 are developed enough to resolve all issues and the process is safe for use in humans, this could become a pivotal part of the clinical functional genomic pathway and be especially useful to the genetic screening industry because in the future in utero editing may be favored over preimplantation genetic diagnosis since it will not require destruction of human embryos, although this will remain a highly controversial approach.
2.3. The Functional Genomic Market
The success and importance of genome sequencing during the COVID-19 pandemic drew government attention to the already rapidly expanding field. This, along with increasing cancer cases, resulted in more government interest and funding, such as in the UK with the launch of the GBP 200 m life science investment program in summer 2021. This investment is expected to generate around GBP 600 m long-term capital for the industry in the UK [ 12 ].
North America accounted for the largest share of the genomics market in 2020, with established companies such as Illumina, Inc. and Thermo Fisher Scientific dominating the market [ 18 ]. The main driving force of this market is the sequencing technology: This segment accounted for the largest technology share of the genomics market in 2019 due to its rapid advancements and usefulness to a wide variety of sectors. The drug discovery and development sector, which uses many of these technologies, accounted for the largest share by application of the genomics market in 2019 [ 19 ].
The value of the global genomics market is expected to more than double by 2025 [ 19 ] and could create around 133,000 jobs by 2030 [ 20 ]. This may cause issues within the industry, as there is already a shortage of trained professionals [ 19 ], unless companies start investing now in training more scientists in this area to fulfill the demand.
2.4. Future Goals
Drug production and diagnostics are currently still developed in ‘traditional’, ways with a relatively small number of businesses such as GSK, Novartis and AstraZeneca beginning to experiment with and integrate functional genomic methods into their workflows. However, functional genomics is likely to become an essential part of all drug discovery and development pathways in the near future. The main hinderance during drug production for pharmaceutical companies is that over 50% of drugs fail at phase III clinical trials due to lack of efficacy [ 21 ]. Failures are costly and time consuming for the industry. Functional genomic techniques appear to improve success rates during drug discovery trails by mapping out potential targets within a disease, and targeting these generally results in more efficient drugs, therefore higher clinical trial success rates ultimately will lower the time taken to discover and produce vital drugs.
3. Conclusions
To date, functional genomics is not being used to its full potential for personalized medicine. More clinical investigations should be performed using functional genomic pathways to increase our understanding of genes and diseases and allow this knowledge to become integrated into mainstream medicine, as it is very much needed. Personalized medicine using functional genomics and genomics to determine the most effective medication at the right time based on an individual’s unique profile will transform medicine since molecular changes precede clinical manifestations; therefore, we can treat patients earlier, resulting in better and more accurate prognosis than ever before.
Clinical trials should be performed using functional genomics to track patient progress when different drugs and treatments are used. This will deepen the understanding of diseases and how treatments affect them by measuring molecular changes compared to clinical phenotypes.
Author Contributions
Conceptualization, R.S.C.; writing—review and editing, R.S.C., S.C. Both authors have read and agreed to the published version of the manuscript.
This research was funded by the Swansea University Employability Academy Internship Programme 2021 (S.C.).
Conflicts of Interest
The authors declare no conflict of interest.
Publisher’s Note: MDPI stays neutral with regard to jurisdictional claims in published maps and institutional affiliations.
- Open access
- Published: 05 April 2024
Yak genome database: a multi-omics analysis platform
- Hui Jiang 1 , 2 na1 ,
- Zhi-Xin Chai 3 na1 ,
- Xiao-Ying Chen 1 , 2 na1 ,
- Cheng-Fu Zhang 1 , 2 ,
- Yong Zhu 1 , 2 ,
- Qiu-Mei Ji 1 , 2 &
- Jin-Wei Xin 1 , 2
BMC Genomics volume 25 , Article number: 346 ( 2024 ) Cite this article
219 Accesses
Metrics details
The yak ( Bos grunniens ) is a large ruminant species that lives in high-altitude regions and exhibits excellent adaptation to the plateau environments. To further understand the genetic characteristics and adaptive mechanisms of yak, we have developed a multi-omics database of yak including genome, transcriptome, proteome, and DNA methylation data.
Description
The Yak Genome Database ( http://yakgenomics.com/ ) integrates the research results of genome, transcriptome, proteome, and DNA methylation, and provides an integrated platform for researchers to share and exchange omics data. The database contains 26,518 genes, 62 transcriptomes, 144,309 proteome spectra, and 22,478 methylation sites of yak. The genome module provides access to yak genome sequences, gene annotations and variant information. The transcriptome module offers transcriptome data from various tissues of yak and cattle strains at different developmental stages. The proteome module presents protein profiles from diverse yak organs. Additionally, the DNA methylation module shows the DNA methylation information at each base of the whole genome. Functions of data downloading and browsing, functional gene exploration, and experimental practice were available for the database.
This comprehensive database provides a valuable resource for further investigations on development, molecular mechanisms underlying high-altitude adaptation, and molecular breeding of yak.
Peer Review reports
Although single omics study provides information and insights into specific biological or molecular processes, it is hard to confirm the real molecular mechanisms underlying the functionality of an organism and the relationships between biological processes and environmental factors. Integrating and analyzing multiple omics data provide an effective and systematic approach to life science researchers. In general, genomics provides DNA sequence information, transcriptomics examines gene transcription patterns under specific conditions, proteomics explores the composition and expression levels of proteins in cells, and DNA methylation involves chemical modifications on DNA molecules [ 1 ]. Multi-omics analysis combines data at different levels to comprehensively explore biological processes. Multi-omics analysis reveals connections between genomics, transcriptomics, proteomics, and DNA methylation data, facilitating to understand how genomic variations impact gene transcription and protein expression, as well as the associations between DNA methylation and gene activities [ 2 ]. These pieces of information contribute novel information to the gene regulatory networks, which are important to molecular mechanisms underlying biological functions, development, metabolism, etiopathology, and environmental adaptation.
The yak ( Bos grunniens ) is a unique species in the Qinghai-Tibet Plateau, and widely distributes in high-altitude areas of Western China and neighboring regions. As a large mammal at the highest-altitude area, yak has survived and adapted to the harsh and cold environment after thousands of years of evolution [ 3 ]. Their unique biological features make them an ideal model for studying adaptive evolution and high-altitude ecosystems. Yak also plays important roles in agriculture and economic development. As a significant livestock species, yak provides meat, fur, and other economic resources. Their dung is also an important source of agricultural fertilizer and energy production. Moreover, yak positively impacts the ecological balance and vegetation restoration in the plateau grasslands through their grazing behaviors [ 4 ]. In recent years, we have analyzed yak using different omics approaches. These data preliminarily explored the yak genetic characteristics, gene transcription, protein expression, and DNA methylation patterns, as well as molecular regulatory mechanisms in response to different conditions [ 5 , 6 , 7 , 8 , 9 , 10 , 11 ], providing novel insights into the mechanisms underlying evolution, and high-altitude adaptation in yak.
Currently, the data resources of yak omics researches are generally stored in public databases in their raw data format, such as NCBI. These databases primarily provide storage and retrieval functions, but lack an integrated platform for data integration and in-depth analysis. Hu et al. [ 12 ] developed a yak genome database ( http://me.lzu.edu.cn/yak ), which incorporated genome sequences, predicted genes and associated annotations, non-coding RNA sequences, transposable elements, and single nucleotide variants of yak, as well as three-way whole-genome alignments between human, cattle and yak. However, this database did not include other omics datasets, such as transcriptome, proteome, and DNA methylation. Given the vast and diverse nature of omics data, the traditional database retrieval methods could not fully explore the relationship between different types of datasets [ 13 ]. Thus, an integrated platform of different omics data is crucial to facilitate data integration, interaction, and analysis. An integrated platform can also offer advanced data mining and machine learning algorithms to help researchers discover the complex relationships among yak genomics, transcriptomics, proteomics, and other omics levels, further deepening our understanding of biological processes and diseases in yak.
In this study, the Yak Genome Database ( http://yakgenomics.com/ ) was constructed, which successfully assembled a comprehensive yak fine-scale genome map at the chromosome level, using PacBio sequencing, Illumina sequencing, Bionano assembly, and Hi-C three-dimensional genome scaffolding. Moreover, this platform also integrated transcriptome, proteome, and DNA methylation data of yak, which were not available in Yak Genome Database developed by Hu et al. [ 12 ]. This database provides basic information for yak researches in future, such as molecular breeding, molecular evolution, disease prevention and control.
Construction and content
The Yak Genome Database was deployed in the Ubuntu 20.04 operation system using the AKKA 2.13 (web server), MySQL 8.0.30 (database server), Scala 2.13.2, and SBT 1.3.9. All data were managed and stored using the MySQL Database Management System. The query function was enforced based on Slick 3.3.2 middleware tier. The Jbrowse 1.16.11 was used to visualize the genome. The website interfaces were designed and implemented using the Bootstrap 4.6.0 and the Play Framework 2.8.7. The software versions and statistical tools used for data analyses and plot preparation have been presented in Xin et al. [ 6 , 7 , 8 , 9 , 10 , 11 ]. The boxplots, and heatmaps were prepared using R 4.2.1. The website has been tested in several popular web browsers, including Firefox, Google Chrome, and Internet Explorer.
Utility and discussion
The yak genome database content.
The multi-omics data in the Yak Genome Database are categorized into two central functional domains: data resources and navigation (Fig. 1 ). The data resources contain four main modules, including genome, transcriptome, proteome, and methylation information. The database contains 26,518 genes, 62 transcriptomes, 144,309 proteome spectra, and 22,478 methylation sites of yak. The navigation page consists of Browser, Jbrowse, Search and Blast functions. Currently, the database supports individual download of images and gene data. In the future, we will add functions such as one-click download of whole genome information.
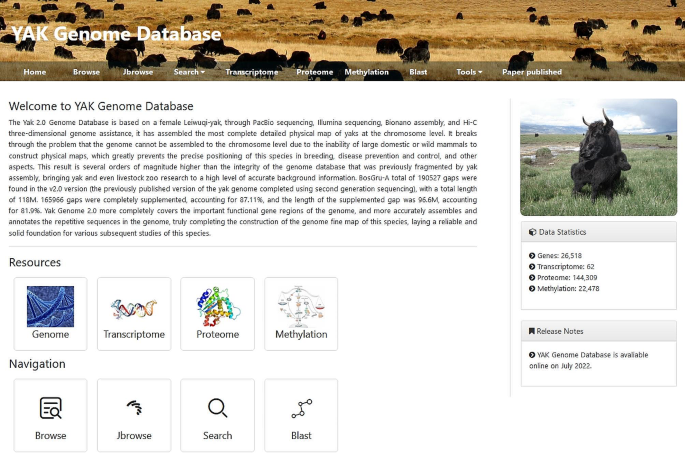
The homepage of yak genome database
Genome module
The Genome module incorporates the complete genomic DNA sequence of yaks obtained by the third-generation high-throughput sequencing platform (PacBio RSII) [ 14 ]. The yak genome was sequenced at a coverage of 70X, with the second-generation sequencing data used to correct errors. The Bionano assisted assembly technology was used for high-quality assembly, and analysis. Next, a refined physical map of the yak chromosome was generated, providing a more readable and complete genome database than the fragmented information in another Yak Genome Database (BosGru_v2.0) [ 15 ], and contributing a novel genome tool to yak researchers.
When accessing the ‘Genome’ section on the homepage, a new page will display information of genes at all locations, such as Gene ID, Chromosome, Start Position, End Position, Strand, GO (Gene Ontology) terms, Interpro, KEGG (Kyoto Encyclopedia of genes and Genomes), Swissprot, and Trembl in a user-friendly table format (Fig. 2 A). When clicking each gene, users can access detailed information of this gene, including annotations, transcriptional levels, proteome data, Jbrowse page, and nucleotide sequences associated with the gene (Fig. 2 B- 2 D). The ‘Annotation’ tav provides comprehensive gene annotation information, including GO terms, KEGG pathways, and Interpro annotations, which can be further explored by clicking them. The ‘Expression’ tab displays gene expression levels across different cattle breeds and tissues, and users can download the images in various formats by selecting the menu in the upper right corner of the image. ‘Jbrowse’ is used to display integrated information from annotated genomic datasets, while ‘Seqs’ provides the coding sequence (CDS) and protein sequence on the selected gene.
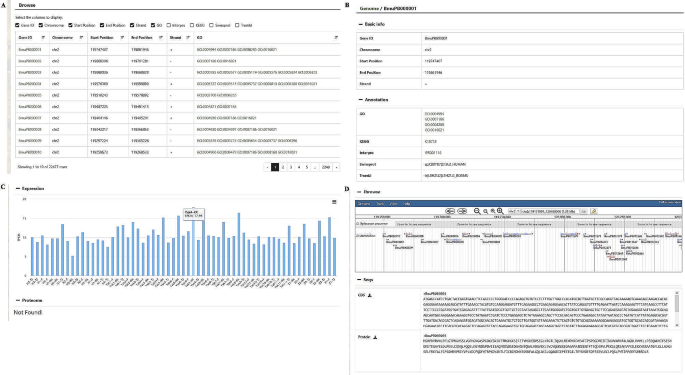
Features of the genome module. ( A ) Genome browse. ( B ) Basic information and annotation of a gene. ( C ) Gene expression. ( D ) Gene Jbrowse and sequences
Transcriptome module
Previously, comparative transcriptome sequencing was performed on lung, gluteal muscle, and mammary gland tissues of low-altitude cattle (Sanjiang and Holstein cattle), Tibetan cattle (living at a moderate altitude), and yaks (living at a high altitude). In addition, these tissues of yaks at different ages (6, 30, 60, and 90 months) were also subjected to transcriptome sequencing. These analyses identified the functional genes involved in the major biochemical, metabolic, and signal transduction pathways involved in yak development and high-altitude adaptation [ 10 , 11 ]. These data are included in the transcriptome module on the website, providing a valuable transcriptome database for specific tissue biomarkers, molecular research, and breeding of yaks. After clicking the “Transcriptome” button, users can select the strain in the ‘Sample’ dialog box, enter the gene ID in the ‘Gene ID’ dialog box, and then click ‘Search’ (Fig. 3 A), and then the website will return the transcriptional levels of the selected genes in selected samples in the forms of data table, Boxplot, Lineplot, and Heatmap (Fig. 3 B and D).
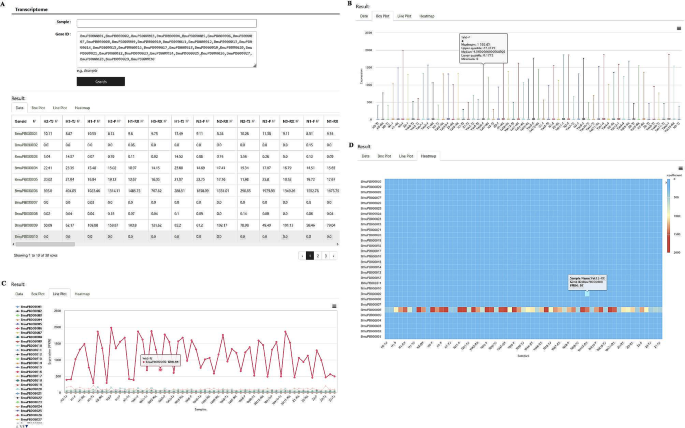
Features of the transcriptome module. ( A ) Transcriptome browse. ( B ) Box plot, ( C ) Line plot and ( D ) Heatmap of gene expression
Proteome module
Using the liquid chromatography-mass spectrometry (LC-MS) method, proteomic analyses were conducted for four specific tissues from four different species (yak, Tibetan cattle, Sanjiang cattle, and Holstein cattle) [ 7 , 8 , 9 ]. All the animals were female and 60 months of age. The proteome module provides two input dialog boxes. Users can select two samples and then click the “search” button. Next, the website will return the comparison results of the expression levels of all genes in the two selected samples, including log2(fold change) and statistical parameters (Fig. 4 ).
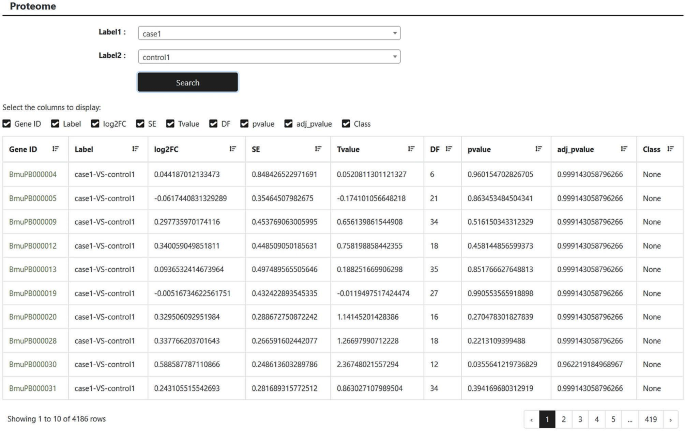
Browse of the proteome module
Methylation module
DNA methylation is a critical epigenetic modification that occurs in both animals and plants, playing pivotal roles in chromosome structure, gene expression and regulation [ 16 ]. The establishment of a comprehensive DNA methylation database for yak can significantly advance the comprehension of cellular gene expression and regulation, and provide deeper insights into the spatiotemporal specificity of DNA methylation across various developmental stages and organs [ 17 ]. The DNA methylation database of yak presents single-base methylation maps and tissue-specific methylation maps. The single-base methylation maps include: 1) DNA methylation levels at the single-base resolution, 2) DNA methylation levels specific to different base types, 3) DNA methylation levels specific to different gene structures, 4) DNA methylation levels in repetitive sequences, and 5) DNA methylation levels in non-coding sequences and regulatory regions. The tissue-specific methylation maps involve three tissues: mammary gland, lung, and muscle [ 6 ]. On the website, users can select ‘Sample’ and ‘Chromosome’ in the Methylation module, set the ‘Start Position’ and ‘End Position,’ and finally click ‘Search’ to obtain the corresponding DNA methylation results on the selected sequences (Fig. 5 ).
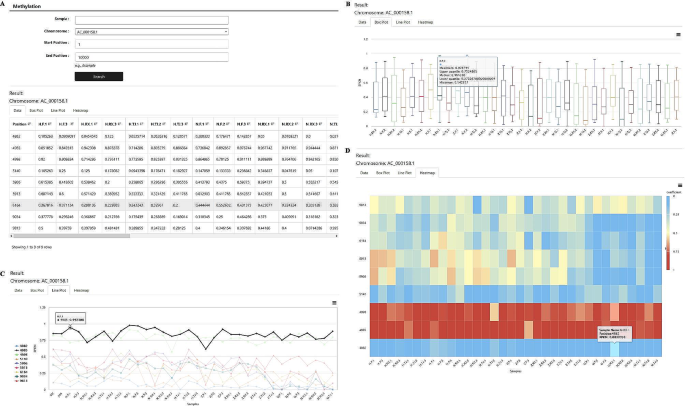
Features of the methylation module. ( B ) Box plot, ( C ) Line plot and ( D ) Heatmap of methylated gene expression
‘Browse’ allows users to read the yak genome directly. ‘JBrowse’ is a next-generation genome browser built with JavaScript and HTML5. The Jbrowse of Yak Genome Database includes tracks describing gene, gene sequence, mRNAs, structure, and other gene-related features, and provides a graphical display of annotations on the yak genome (Fig. 6 ). Users can browse gene models on chromosomes and unanchored contigs. For example, if user set the genomic region from 4,454,001 bp to 5,878,000 bp on Chr1 for browsing, all genes in this region will appear in order (Fig. 6 A). When clicking on ‘BmuPB021145’, an extra layer will appear with the detailed information, such as mRNAs, CDS and other features (Fig. 6 B). For more operational details, users can click the ‘Help’ button, which provides comprehensive instructions and guidance.
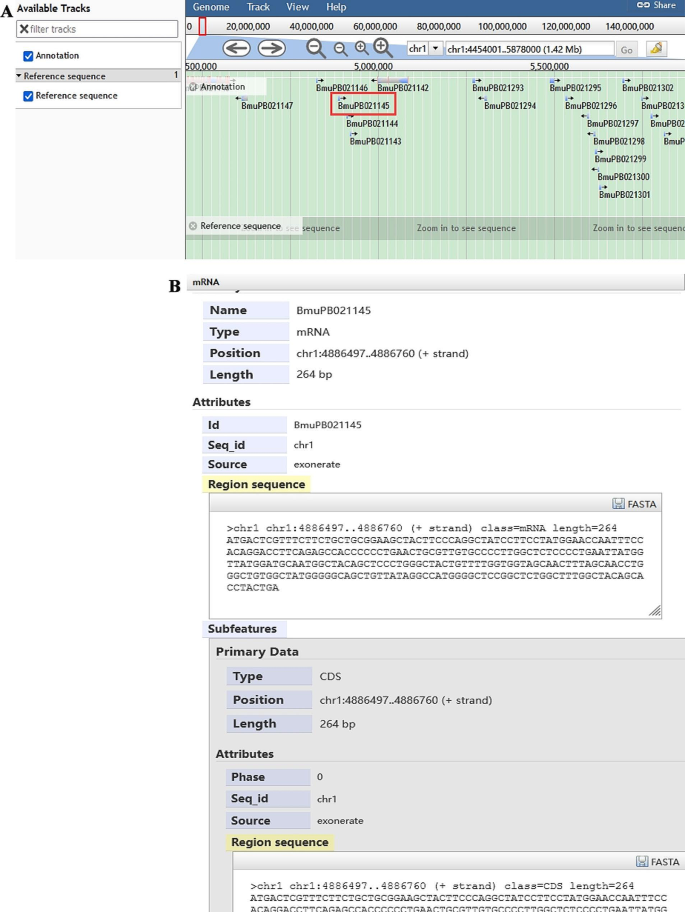
Regional view of the genome using Jbrowse. ( A ) A graphic view of the region 4,454,001 bp to 5,878,000 bp on Chr1. ( B ) The interface after clicking on ‘BmuPB021145’.
The ‘Search’ tab supplies users with two methods (search by gene ID or range) for genome searching. When users click on ‘Blast’, three options ‘Blastn Gene’, ‘Blastn Genome’ and ‘Blastp’ will display. Users can select the Blast type and enter a DNA or protein sequence, and set the parameters of ‘Evalue’, ‘Word size’ and ‘Max target seqs’. After clicking the ‘Search’ button, the nucleotide or protein sequence complying the search conditions will display and could be downloaded by the users.
Additional tools
The Yak Genome Database also provides users with several convenient online tools, including Primer designer, GO and KEGG enrichment. The ‘Primer designer’ tool offers primer design function to amplify a selected sequence. The ‘GO enrichment’ and ‘KEGG enrichment’ tools facilitate the users to obtain the GO and KEGG enrichment results of a set of genes.
Maintenance of the yak genome database in future
To ensure continuous operation of the Yak Genome Database, we would assign an administrator to manage the website regularly. We would keep omics studies on yak in future, and all the omics data we obtained would be uploaded to this database. In addition, we would keep cooperations with other investigators and find more cooperators who work on yak. Next, all the progresses on yak omics would also be encouraged to supplement in this database.
Conclusions
The Yak Genome Database is a comprehensive platform of genomic physical map, which integrates genome, transcriptome, proteome, and DNA methylation data. Information in the database can be downloaded, and shared through the Internet. Users who want to upload their own data can contact the administrator of the website. By providing timely updates on yak research progress, the Yak Genome Database enables efficient and interactive sharing of existing scientific data among researchers worldwide who are interested in yak, cattle, livestock, ruminant animals, and even medical research. Comparative analysis of multidimensional data from key yak tissues aims to uncover the mechanisms underlying high-altitude adaptation, disease resistance, cold tolerance, and starvation resistance of large animals in the plateau. These findings contribute to molecular breeding of livestock animals and the understanding of human responses to harsh environments.
Data availability
The datasets generated and analyzed in the current study are freely available on the Download page of Yak database with the web link: http://yakgenomics.com/ .
Abbreviations
Coding Sequence
Gene Ontology
Kyoto Encyclopedia of Genes and Genomes
National Center for Biotechnology Information
Manzoni C, Kia DA, Vandrovcova J, Hardy J, Wood NW, Lewis PA, et al. Genome, transcriptome and proteome: the rise of omics data and their integration in biomedical sciences. Brief Bioinform. 2018;19(2):286–302.
Article CAS PubMed Google Scholar
Liao Y, Wang J, Zou J, Liu Y, Liu Z, Huang Z. Multi-omics analysis reveals genomic, clinical and immunological features of SARS-CoV-2 virus target genes in pan-cancer. Front Immunol. 2023;14:1112704.
Article CAS PubMed PubMed Central Google Scholar
Ge Q, Guo Y, Zheng W, Zhao S, Cai Y, Qi X. Molecular mechanisms detected in yak lung tissue via transcriptome-wide analysis provide insights into adaptation to high altitudes. Sci Rep. 2021;11(1):7786.
Ayalew W, Chu M, Liang C, Wu X, Yan P. Adaptation mechanisms of Yak ( Bos grunniens ) to high-Altitude Environmental stress. Animals. 2021;11(8):2344.
Article PubMed PubMed Central Google Scholar
Gao X, Wang S, Wang YF, Li S, Wu SX, Yan RG, et al. Long read genome assemblies complemented by single cell RNA-sequencing reveal genetic and cellular mechanisms underlying the adaptive evolution of yak. Nat Commun. 2022;13(1):4887.
Xin J, Chai Z, Zhang C, Zhang Q, Zhu Y, Cao H, et al. Methylome and transcriptome profiles in three yak tissues revealed that DNA methylation and the transcription factor ZGPAT co-regulate milk production. BMC Genom. 2020;21(1):731.
Article CAS Google Scholar
Xin JW, Chai ZX, Zhang CF, Zhang Q, Zhu Y, Cao HW, et al. Signature of high altitude adaptation in the gluteus proteome of the yak. J Exp Zool B Mol Dev Evol. 2020;334(6):362–72.
Xin JW, Chai ZX, Zhang CF, Zhang Q, Zhu Y, Cao HW, et al. Differences in proteomic profiles between yak and three cattle strains provide insights into molecular mechanisms underlying high-altitude adaptation. J Anim Phys Anim Nutr. 2022;106(3):485–93.
Xin JW, Chai ZX, Zhang CF, Yang YM, Zhang Q, Zhu Y, et al. Comparative analysis of Skeleton muscle Proteome Profile between Yak and cattle provides insight into high-altitude adaptation. Curr Proteom. 2021;18(1):62–70.
Xin JW, Chai ZX, Zhang CF, Zhang Q, Zhu Y, Cao HW, et al. Transcriptome profiles revealed the mechanisms underlying the adaptation of yak to high-altitude environments. Sci Rep. 2019;9(1):7558.
Xin JW, Chai ZX, Zhang CF, Zhang Q, Zhu Y, Cao HW, et al. Comparisons of lung and gluteus transcriptome profiles between yaks at different ages. Sci Rep. 2019;9(1):14213.
Hu Q, Ma T, Wang K, Xu T, Liu J, Qiu Q. The yak genome database: an integrative database for studying yak biology and high-altitude adaption. BMC Genomics. 2012;13:600.
Tarazona S, Arzalluz-Luque A, Conesa A. Undisclosed, unmet and neglected challenges in multi-omics studies. Nat Comp Sci. 2021;1(6):395–402.
Article Google Scholar
Ji QM, Xin JW, Chai ZX, Zhang CF, Dawa Y, Luo S, et al. A chromosome-scale reference genome and genome-wide genetic variations elucidate adaptation in yak. Mol Ecol Res. 2021;21(1):201–11.
Jiangfeng F, Yuzhu L, Sijiu Y, Yan C, Gengquan X, Libin W, et al. Transcriptional profiling of two different physiological states of the yak mammary gland using RNA sequencing. PLoS ONE. 2018;13(7):e0201628.
Lucibelli F, Valoroso MC, Aceto S, Plant DNA, Methylation. An epigenetic Mark in Development, Environmental interactions, and evolution. Int j mol sci. 2022;23(15):8299.
Chai Z, Wu Z, Ji Q, Wang J, Wang J, Wang H, et al. Genome-wide DNA methylation and hydroxymethylation changes revealed epigenetic regulation of Neuromodulation and Myelination in Yak Hypothalamus. Front Genet. 2021;12:592135.
Download references
This work was supported by the Program of Provincial Department of Finance of the Tibet Autonomous Region, the Major Special Projects of Tibet Autonomous Region (XZ202101ZD0002N-01), the Second Tibetan Plateau Scientific Expedition and Research Program (2019QZKK0501), and the program National Beef Cattle and Yak Industrial Technology System (CARS-37).
Author information
Hui Jiang, Zhi-Xin Chai and Xiao-Ying Chen contributed equally to this work.
Authors and Affiliations
State Key Laboratory of Hulless Barley and Yak Germplasm Resources and Genetic Improvement, 850000, Lhasa, Tibet, China
Hui Jiang, Xiao-Ying Chen, Cheng-Fu Zhang, Yong Zhu, Qiu-Mei Ji & Jin-Wei Xin
Institute of Animal Science and Veterinary, Tibet Academy of Agricultural and Animal Husbandry Sciences, 850000, Lhasa, Tibet, China
Key Laboratory of Qinghai-Tibetan Plateau Animal Genetic Resource Reservation and Utilization, Sichuan Province and Ministry of Education, Southwest Minzu University, 610041, Chengdu, Sichuan, China
Zhi-Xin Chai
You can also search for this author in PubMed Google Scholar
Contributions
HJ and ZXC performed the analysis. XYC conducted the database. CFZ and YZ wrote the paper. QMJ supervised the database and JWX revised the manuscript. All authors have approved the final article.
Corresponding authors
Correspondence to Qiu-Mei Ji or Jin-Wei Xin .
Ethics declarations
Ethics approval and consent to participate.
All procedures and experiments involving animals followed the guidelines for the Care and Use of Laboratory Animals. The Ethics Committee at Institute of Animal Science and Veterinary, Tibet Academy of Agricultural and Animal Husbandry Sciences (Permit Number: 2015 − 216) approved this study.
Consent for publication
Not applicable.
Competing interests
The authors declare no competing interests.
Additional information
Publisher’s note.
Springer Nature remains neutral with regard to jurisdictional claims in published maps and institutional affiliations.
Rights and permissions
Open Access This article is licensed under a Creative Commons Attribution 4.0 International License, which permits use, sharing, adaptation, distribution and reproduction in any medium or format, as long as you give appropriate credit to the original author(s) and the source, provide a link to the Creative Commons licence, and indicate if changes were made. The images or other third party material in this article are included in the article’s Creative Commons licence, unless indicated otherwise in a credit line to the material. If material is not included in the article’s Creative Commons licence and your intended use is not permitted by statutory regulation or exceeds the permitted use, you will need to obtain permission directly from the copyright holder. To view a copy of this licence, visit http://creativecommons.org/licenses/by/4.0/ . The Creative Commons Public Domain Dedication waiver ( http://creativecommons.org/publicdomain/zero/1.0/ ) applies to the data made available in this article, unless otherwise stated in a credit line to the data.
Reprints and permissions
About this article
Cite this article.
Jiang, H., Chai, ZX., Chen, XY. et al. Yak genome database: a multi-omics analysis platform. BMC Genomics 25 , 346 (2024). https://doi.org/10.1186/s12864-024-10274-6
Download citation
Received : 30 October 2023
Accepted : 31 March 2024
Published : 05 April 2024
DOI : https://doi.org/10.1186/s12864-024-10274-6
Share this article
Anyone you share the following link with will be able to read this content:
Sorry, a shareable link is not currently available for this article.
Provided by the Springer Nature SharedIt content-sharing initiative
- Multi-omics
- Plateau environment
BMC Genomics
ISSN: 1471-2164
- Submission enquiries: [email protected]
- General enquiries: [email protected]
- Skip to main menu
- Skip to user menu
Scientist, Functional Genomics
- Research the potential functional impact of any given SNP on protein or cellular function: from RNA to protein to cell to systems-level analysis including work on known disease-associated SNPs and VUSs in the context of various human primary and iPSC-derived cell types
- Project manage out-sourced work with CROs to maximize your productivity
- Collaborate with other ICR scientists to solve complex human medical mysteries
- An advanced degree in biomedical science
- In-depth knowledge of and experience with modern molecular and cellular biology techniques, preferably experience doing functional genomics
- Experience project-managing out-sourced work with CROs
- A solid background in human genetics
- The ability to work in the United States without sponsorship
- To have an easygoing, hard-working personality
Share this job
Get job alerts
Create a job alert and receive personalised job recommendations straight to your inbox.
Before you apply - Turn on alerts for jobs like this!
We'll send them straight to your inbox :
When you create this job alert we will email you a selection of jobs matching your criteria. Our terms and conditions and privacy policy apply to this service and you can unsubscribe at any time.
By clicking to continue to apply below, your email address will be shared with the employer.
Office of Neuroscience Research
Thesis Defense: Ellie Wilson (Molecular Genetics and Genomics Program) – “Integrating DNA methylation and 3D-genome architecture to identify functional regulatory sequences in IDH mutant AML”
“Integrating DNA methylation and 3D-genome architecture to identify functional regulatory sequences in IDH mutant AML”
Thesis lab: David Spencer (WashU Medicine)
For inquiries contact Ellie at [email protected] .
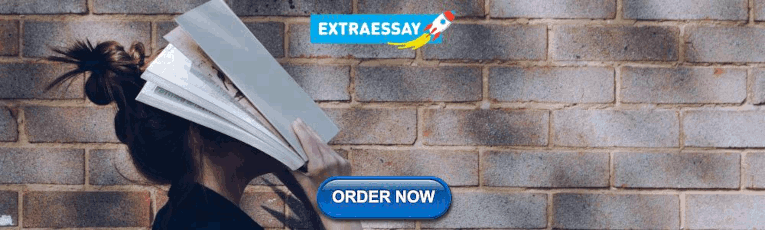
IMAGES
VIDEO
COMMENTS
Functional genomics uses genomic data to study gene and protein expression and function on a global scale (genome-wide or system-wide), focusing on gene transcription, translation and protein ...
Substantial information about functional genomics can be obtained through the analysis of the messenger RNA (mRNR) or cDNA, which is copied from the mRNA by reverse transcription PCR. Therefore researchers often choose to test the mRNR or cDNA rather than DNA, because RNA analysis may be more eligible for a gene that has many small exons and it ...
Functional Genomics. Michael Mannstadt, Marc N. Wein, in Genetics of Bone Biology and Skeletal Disease (Second Edition), 2018. Summary. The completion of the human genome project marked the beginning of the so-called postgenomic era, an exciting time that is delivering new insights into the complex regulation of genes in all fields of research, including bone biology.
Introduction. T he field of functional genomics attempts to describe the functions and interactions of genes and proteins by making use of genome-wide approaches, in contrast to the gene-by-gene approach of classical molecular biology techniques. It combines data derived from the various processes related to DNA sequence, gene expression, and protein function, such as coding and noncoding ...
Briefings in Functional Genomics is accepting submissions for upcoming issues, including review and protocol articles. Articles range in scope and depth from the introductory level to specific details of protocols and analyses, encompassing bacterial, fungal, plant, animal, and human data. Find out more about submitting and formatting your ...
Chapter 9 describes several big-picture challenges in functional genomics research: education and training, determining and defining "model organisms," and the social and ethical implications of functional genomics research. Chapter 10 offers a brief wrap-up of the workshop and a look to the future.
The era of functional genomics in neurodegenerative disease research has just begun There is no doubt that the integration of functional genomics with human genetics and single-cell profiling of human patient tissues will become a major engine for the discovery of disease mechanisms. The insights gained will help generate testable hypotheses ...
Functional genomics is a field of molecular biology that attempts to describe gene (and protein) functions and interactions. Functional genomics make use of the vast data generated by genomic and transcriptomic projects (such as genome sequencing projects and RNA sequencing ). Functional genomics focuses on the dynamic aspects such as gene ...
Functional genomics is a field of molecular biology that integrates genomic and transcriptomic data to describe gene (and protein) functions and interactions. Genomics is a study of the function and structure of genome, which comprise the complete set of all genes, regulatory sequences, and non-coding regions within an organism's DNA.
Functional genomics promises to rapidly narrow the gap between sequence and function and to yield new insights into the behavior of biological systems. Several recent studies fall under the operational definition of functional genomics. The recent completion ( 2) of the genome sequence of the budding yeast Saccharomyces cerevisiae (in other ...
Gene targeting has many applications in functional genomics research, such as precise gene modifications and epitope tagging of endogenous proteins. In addition, many agriculturally important traits are conferred by point mutations or indels at specific loci in either the gene coding region or promoter region, making gene targeting also useful ...
TFGB investigators catalyze technology development in genetics and computational genomics, including functional assessment, systematic mutagenesis, developmental genomics and computational analysis of both human and microbial DNA. By testing approaches and technologies in cell lines and animal models, TFGB investigators are making fundamental ...
Functional genomics research examines the role of the genome in cancer. By testing hypotheses derived from structural genomics research, or by generating new ideas from experiments in cancer cells, functional genomics research reveals patterns in cancer biology that can sometimes be directly translated to precision cancer care.Studies like those from The Cancer Target Discovery and Development ...
Here, we briefly review the advances in rice functional genomics research during the past 10 years, including a summary of functional genomics platforms, genes and molecular regulatory networks that regulate important agronomic traits, and newly developed tools for gene identification. These achievements made in functional genomics research ...
Welcome to the Functional Genomics Group, where we are dedicated to advancing the field of statistical genetics and bioinformatics through innovative research and cutting-edge techniques. Our group comprises a public-private collaboration between UMCG, Biogen, Roche and Takeda, aimed at drug-target identification and (in)validation. Our group ...
Main conclusion In this review, we give an overview of plant sequencing efforts and how this impacts plant functional genomics research. Abstract Plant genome sequence information greatly facilitates the studies of plant biology, functional genomics, evolution of genomes and genes, domestication processes, phylogenetic relationships, among many others. More than two decades of sequencing ...
This research has been conceived and developed within the activities of the working group 'AI for Functional Genomics' (AI4FG), which was launched in the framework of the CNR Observatory on Artificial Intelligence, an interdepartmental initiative established by the National Research Council of Italy in 2019.
There is a dearth of genetic and environmental diversity in functional genomics datasets. Research focused on the variant-to-function gap aims to identify molecular QTLs but lacks data from non-European-ancestry populations. We discuss the major barriers and pose actionable suggestions, which aim to empower research and researchers from underserved populations.
2 Taub Institute for Research on Alzheimer's Disease and Aging Brain, Columbia University Medical Center, New York, NY, USA. ... Methods: Our integrated toolkit for in-vitro microglia functional genomics optimizes iPSC differentiation into iMG through a streamlined two-step, 20-day process, producing iMG with a normal karyotype. We confirmed ...
development of novel tools, technologies and biological models for functional genomics research; research focused on the interplay of genetic variance and physiological pathways, organs and systems in both healthy and disease states; The total fund is up to £16 million. MRC will fund up to 80% of the full economic cost (FEC) and funding will ...
Current Clinical Research . The field of functional genomics is of great relevance clinically to cancer patients, where most research and development is currently focused. Changes in the genome or epigenome can cause cancer by promoting uncontrolled cell growth or causing the immune system to fail to destroy tumors. Using a clinical functional ...
The status of sequenced plant genomes and on the use of genome information in different research areas are given and how this impacts plant functional genomics research is given. Main conclusion In this review, we give an overview of plant sequencing efforts and how this impacts plant functional genomics research. Abstract Plant genome sequence information greatly facilitates the studies of ...
Background The yak (Bos grunniens) is a large ruminant species that lives in high-altitude regions and exhibits excellent adaptation to the plateau environments. To further understand the genetic characteristics and adaptive mechanisms of yak, we have developed a multi-omics database of yak including genome, transcriptome, proteome, and DNA methylation data. Description The Yak Genome Database ...
Scientist, Functional Genomics job in Leonia, New Jersey with InVitro Cell Research, LLC. Apply Today.
Office of Neuroscience Research. MSC 8111-96-07-7122. 4370 Duncan Ave. St. Louis, Missouri 63110. [email protected]