- S&P Dow Jones Indices
- S&P Global Market Intelligence
- S&P Global Mobility
- S&P Global Commodity Insights
- S&P Global Ratings
- S&P Global Sustainable1
- Investor Relations Overview
- Investor Presentations
- Investor Fact Book
- News Releases
- Quarterly Earnings
- SEC Filings & Reports
- Executive Committee
- Corporate Governance
- Merger Information
- Stock & Dividends
- Shareholder Services
- Contact Investor Relations
- Email Subscription Center
- Media Center
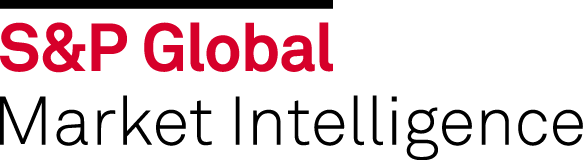
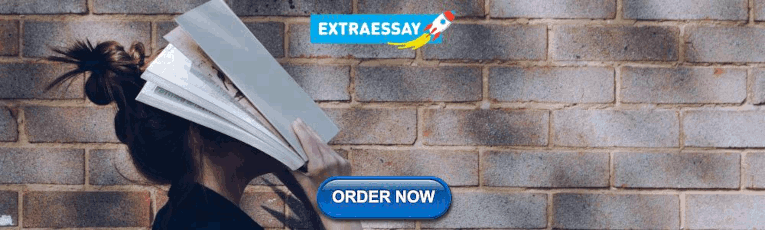
Analytical Credit Risk Case Studies
Our credit and risk specialists leverage Credit Analytics, our suite of cutting-edge analytical models to provide you with credit risk insights and real-life case studies on the topics that are important to you and your business.
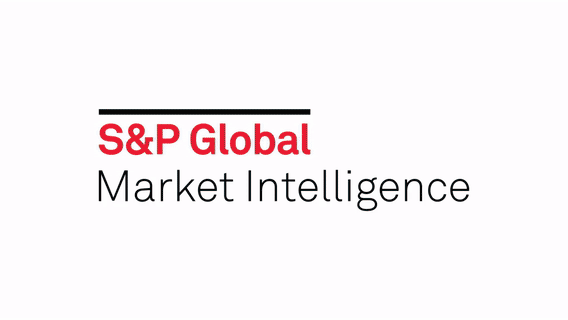
- on this page
Market Volatility
Customer risk, supply chain, industry assessment, transfer pricing.
We’ve recently experienced some of the most extraordinary events in our lifetime, a full year of the Russia-Ukraine conflict, global monetary interventions to fight one of the most significant inflations in recent history, and a continuing threat from global COVID containment policies that continue to disrupt supply chains today.
It goes without saying that the importance of monitoring and embedding macroeconomic factors into credit risk assessments is critical.
Read the results from our recent research where we assessed the credit risk of 22 industry sectors (Corporates and Banks) in the United States and compare historical trends based on the probability of default score generated by the RiskGauge™ Model, with forecasted trends based on this score conditioned with macroeconomic scenarios.
READ THE FULL REPORT
The Continued Evolution of Credit Risk in the European Union and the United Kingdom
Anticipate the Unknown: Insights to Turn Market Risks into Business Opportunities
European Industries Most Impacted by the Russia-Ukraine War from a Probability of Default Perspective
A Fundamentals Approach to Detect Early Signs of Private Company Credit Deterioration
Go Beyond Fundamentals to Uncover Early Signs of Private Company Credit Deterioration
Credit Score Implications of Russia-Ukraine War
Silicon Valley Bank: Uncovering Regional Bank Stress with Equity-Driven Credit Models
It’s different this time: a banking rhyme, how to assess risk appetite in trade credit, bed bath and bankruptcy using quantitative credit models to predict corporate defaults.
Christopher & Banks Corporation – tracking the early-warning signals of credit risk
Default Insights: Avianca Holdings S.A. - Tracking the early warning signals of credit risk
SAS AB Tracking the early-warning signals of credit risk
Car rental industry’s credit risk: a bouncy ride during the pandemic
Understanding Drivers of Credit Risk: Differences and Similarities in the Credit Risk Assessment of a Non-Financial Corporation via a PD Scoring Model
Tracking Credit Risk of a Major U.S. Retailer

In the past, only large financial institutions have ventured into deploying credit risk automation and workflow systems due to the volume of resources and investments required and the need to lock down processes. However, it is not uncommon today, to meet with a corporate credit risk officer who is looking to discuss the options, benefits, and risks of automating customer onboarding and credit risk management and monitoring processes.
Read about the risks and opportunities of credit risk automation here.
READ THE BLOG
Data That Delivers: Automating the Credit Risk Workflow
Machine Learning and Credit Risk Modelling
Managing Credit Risk Automation during Economic Uncertainty
Get a deeper view of your credit risk exposure.
Continuous financial health management of suppliers, minimizing counterparty risk in supply chains, gauging supply chain risk in volatile times, covid-19’s wake-up call for supply chain credit risk.
SEE THE FULL BLOG
Corporate Credit Risk Macroeconomic Recovery Projections Post-COVID-19
In the U.S., continuous government stimulus payments and efficient vaccine rollouts have further revived both supply and demand for goods and services. However, although public companies began publishing financial reports for the second quarter of 2021, the results did not show the full extent of the global recovery, due to the intrinsic lag effect of financial performance.
To help navigate these transition times, we used our Macro-Scenario model to analyze how the credit risk of public and private firms in the U.S. may change under different macroeconomic projections.
Highlights include:
- The degree of economic recovery in different industries is mostly driven by the characteristics of post COVID-19 positive macroeconomic projections.
- Energy, industrial products, and construction materials, as well as retail and media industries, exhibit the best recovery over the Q1 2022 outlook when compared with the baseline scenario.
- The real estate and construction materials industries also show strong recovery and transition in credit risk.
The Bankruptcy Outlook and a Trifecta of Factors: Global Risk, Inflation, Rising Rates
COVID-19: Company Fundamental Scars and the Path to Recovery in Asian Economies
Corporate Credit Risk Trends in Developing Markets An Expected Credit Loss ECL Perspective
Corporate Credit Risk Trends in Developing Markets: A Probability of Default Perspective
Uncertainties Impact Credit Risk in the European Union and the UK
How to Take Control of Intercompany Financing
A large accounting firm automates its credit assessments for transfer pricing, successfully navigating the complexity of intercompany financing, law firm leverages scorecard approach to support transfer pricing engagements.
Credit Risk Analysis Using EDA
- Conference paper
- First Online: 16 September 2022
- Cite this conference paper
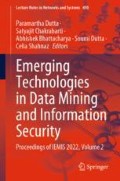
- Prakriti Arora 14 ,
- Siddharth Gautam 15 ,
- Anushka Kalra 16 ,
- Ashish Negi 14 &
- Nitin Tyagi 14
Part of the book series: Lecture Notes in Networks and Systems ((LNNS,volume 490))
489 Accesses
Organizations or banks are providing funds to support people monetarily, keeping assets in return till the amount is repaid to the company with interest encounter loss at many instances when the borrower or the client fails to repay the loan appearing to be a defaulter. Also, when the firm disapproves the loan of an applicant who is likely to repay the sum, the loss is again withstood by the firm. Therefore, to avoid this loss, this research is performed deeply analyzing the factors using exploratory data analysis, affecting the trend of defaulters as well as non-defaulters, helping the firm recognize the defaulters, and disapproving their request to borrow. The exploratory data analysis is performed by visually performing univariate, bivariate, and multivariate analysis on almost all the aspects of the two credit history datasets. The patterns and learnings were noted based on the visual as well as statistical analysis to determine creditworthiness of a client.
This is a preview of subscription content, log in via an institution to check access.
Access this chapter
- Available as PDF
- Read on any device
- Instant download
- Own it forever
- Available as EPUB and PDF
- Compact, lightweight edition
- Dispatched in 3 to 5 business days
- Free shipping worldwide - see info
Tax calculation will be finalised at checkout
Purchases are for personal use only
Institutional subscriptions
Huang S-H, Tu W-P, Yeh H-H, Chi MC (2013) An EDA course module for the topic of reliability using automotive electronics as applications. In: 2013 3rd Interdisciplinary engineering design education conference. https://doi.org/10.1109/iedec.2013.6526768
Malik A, Gautam S, Khatoon N, Sharma N, Kaushik I, Kumar S (2020) Analysis of black-hole attack with its mitigation techniques in Ad-hoc network. In: Deep learning strategies for security enhancement in wireless sensor networks advances in information security, privacy, and ethics, pp 211–232. https://doi.org/10.4018/978-1-7998-5068-7.ch011
Schiantarelli F, Stacchini M, Strahan P (2016) Bank quality, judicial efficiency and borrower runs: Loan repayment delays in Italy. https://doi.org/10.3386/w22034
Pulakkazhy (2013) Data mining in banking and its Applications-A Review. J Comput Sci 9(10):1252–1259. https://doi.org/10.3844/jcssp.2013.1252.1259
Rustagi A, Manchanda C, Sharma N (2020) IoE: a boon & threat to the mankind. In: 2020 IEEE 9th international conference on communication systems and network technologies (CSNT). https://doi.org/10.1109/csnt48778.2020.9115748
Arif M, Khatak A, Hussain M (2015) A framework for Data Warehouse using data mining and knowledge discovery for a network of hospitals in Pakistan. Int J Bio-Sci Bio-Technol 7(3):217–222. https://doi.org/10.14257/ijbsbt.2015.7.3.23
Tiwari A, Sharma N, Kaushik I, Tiwari R (2019) Privacy issues & security techniques in big data. In: 2019 International conference on computing, communication, and intelligent systems (ICCCIS). https://doi.org/10.1109/icccis48478.2019.8974511
Predicting student’s academic performance using data mining techniques (2020) Int J Eng Adv Technol 9(3)”215–219. https://doi.org/10.35940/ijeat.b4521.029320
Zurada J, Zurada M (2002) How secure are good loans: validating loan-granting decisions and predicting default rates on consumer loans. Rev Bus Inf Syst (RBIS) 6(3):65–84. https://doi.org/10.19030/rbis.v6i3.4563
Zurada J (2002) Data mining techniques in predicting default rates on customer loans. Databases Inf Syst II:285–296. https://doi.org/10.1007/978-94-015-9978-8_22
Article MATH Google Scholar
Xin L, Guozi S, Huakang L (2017) Overdue prediction of bank loans based on Deep Neural Network. In: International symposium on computer science and artificial intelligence (ISCSAI). https://doi.org/10.26480/iscsai.01.2017.26.28
Comparative analysis and study of data mining techniques used for IOT based Smart Healthcare System (2020) Int J Emerg Trends Eng Res 8(9):6131–6138. https://doi.org/10.30534/ijeter/2020/198892020
Data mining techniques for analysing employment data (2019) Int J Eng Adv Technol 9(2):555–556. https://doi.org/10.35940/ijeat.b3311.129219
Purohit SU, Mahadevan V, Kulkarni AN (2012) Credit evaluation model of loan proposals for Indian Banks. Int J Model Optim 529–534. https://doi.org/10.7763/ijmo.2012.v2.176
Yuanyuan L (2008) Research on personal credit evaluation system of commercial banks. In: First International Workshop on Knowledge Discovery and Data Mining (WKDD 2008). https://doi.org/10.1109/wkdd.2008.147
Jafar Hamid A, Ahmed TM (2016) Developing prediction model of loan risk in banks using data mining. Mach Learn Appl Int J 3(1):1–9. https://doi.org/10.5121/mlaij.2016.3101
Article Google Scholar
Li W, Liao J (2011) An empirical study on credit scoring model for credit card by using Data Mining Technology. In: 2011 Seventh international conference on computational intelligence and security. https://doi.org/10.1109/cis.2011.283
Jayasree (2013) A review on data mining in banking sector. Am J Appl Sci 10(10):1160–1165. https://doi.org/10.3844/ajassp.2013.1160.1165
Kamatchi K, Siva Balan A (2013) Multiphase text mining predictor for market analysis. In: 2013 International conference on current trends in engineering and technology (ICCTET). https://doi.org/10.1109/icctet.2013.6675990
Samanta D, Dutta S, Galety MG, Pramanik S (2022) A novel approach for web mining taxonomy for high-performance computing. In: Tavares JMRS, Dutta P, Dutta S, Samanta D (eds) Cyber intelligence and information retrieval. Lecture Notes in Networks and Systems, vol 291. Springer, Singapore. https://doi.org/10.1007/978-981-16-4284-5_37
Grover M, Sharma N, Bhushan B, Kaushik I, Khamparia A (2020) Malware threat analysis of IoT devices using deep learning neural network methodologies. In: Security and Trust Issues in Internet of Things, pp 123–143. https://doi.org/10.1201/9781003121664-6
Goel A, Tyagi N, Gautam S (2019) Comparative analysis of 3-D password using various techniques (June 16, 2019). ' Comparative analysis of 3-D password using various techniques '. Int J Emerg Technol Innov Res, 6(6):711–718 ( www.jetir.org ), ISSN:2349-5162. http://www.jetir.org/papers/JETIR1907Q08.pdf
Goyal S, Sharma N, Kaushik I, Bhushan B (2021) Industrial revolution: blockchain as a wave for industry 4.0 and iiot. In: Advances in computing communications and informatics, pp 108–130. https://doi.org/10.2174/9781681088624121010008
Kaushik I, Sharma N (2020) Black hole attack and its security measure in wireless sensors networks. In: Advances in intelligent systems and computing handbook of wireless sensor networks: issues and challenges in current scenarios, pp 401–416. https://doi.org/10.1007/978-3-030-40305-8_20
An exploratory analysis of corporate governance using supervised data mining learning (2019) Int J Recent Technol Eng 8(3):3546–3557. https://doi.org/10.35940/ijrte.c5279.098319
Kaieski N, Oliveira LP, Villamil MB (2016) Vis-health: Exploratory analysis and visualization of dengue cases in Brazil. In: 2016 49th Hawaii international conference on system sciences (HICSS). https://doi.org/10.1109/hicss.2016.385
Tyagi N, Gautam S, Goel A, Mann P (2021) A framework for blockchain technology including features. In: Hassanien AE, Bhattacharyya S, Chakrabati S, Bhattacharya A, Dutta S (eds) Emerging technologies in data mining and information security. Advances in Intelligent Systems and Computing, vol 1286. Springer, Singapore. https://doi.org/10.1007/978-981-15-9927-9_62
Gautam S, Malik A, Singh N, Kumar S (2019) Recent advances and countermeasures against various attacks in IoT environment. In: 2019 2nd international conference on signal processing and communication (ICSPC), pp 315–319. https://doi.org/10.1109/ICSPC46172.2019.8976527
Singh G, Gautam S, Prachi VA, Kaushal T (2021) Analysis of blockchain induced cryptocurrency: regulations and challenges of cryptocurrencies. In: Hassanien AE, Bhattacharyya S, Chakrabati S, Bhattacharya A, Dutta S (eds) Emerging technologies in data mining and information security. Advances in intelligent systems and computing, vol 1286. Springer, Singapore. https://doi.org/10.1007/978-981-15-9927-9_54
Sharma N, Kaushik I, Bhushan B, Gautam S, Khamparia A (2020) Applicability of WSN and biometric models in the field of healthcare. In: Deep learning strategies for security enhancement in wireless sensor networks advances in information security, privacy, and ethics, pp 304–329. https://doi.org/10.4018/978-1-7998-5068-7.ch016
Sharma N, Kaushik I, Rathi R, Kumar S (2020) Evaluation of accidental death records using hybrid genetic algorithm. SSRN Electron J. https://doi.org/10.2139/ssrn.3563084
Gurung A, Gautam S, Garg T, Bhardwaj Y, Gupta H (2021) Virtual numeric authentication system using contour detection of color-banded fingertips. In: Tavares JMRS, Chakrabarti S, Bhattacharya A, Ghatak S (eds) Emerging technologies in data mining and information security. Lecture notes in networks and systems, vol 164. Springer, Singapore. https://doi.org/10.1007/978-981-15-9774-9_32
Rustagi A, Manchanda C, Sharma N, Kaushik I (2020) Depression anatomy using combinational deep neural network. In: Advances in intelligent systems and computing international conference on innovative computing and communications, pp 19–33. https://doi.org/10.1007/978-981-15-5148-2_3
Goel A, Gautam S, Tyagi N, Sharma N, Sagayam M (2021) Securing biometric framework with cryptanalysis. In: Intelligent data analytics for terror threat prediction, pp 181–208. https://doi.org/10.1002/9781119711629.ch9
Kathuria RS, Gautam S, Singh A, Khatri S, Yadav N (2019) Real time sentiment analysis on twitter data using deep learning (Keras). In: 2019 international conference on computing, communication, and intelligent systems (ICCCIS), pp 69–73. https://doi.org/10.1109/ICCCIS48478.2019.8974557
Download references
Author information
Authors and affiliations.
HMRITM, Delhi, India
Prakriti Arora, Ashish Negi & Nitin Tyagi
NSUT, Delhi, India
Siddharth Gautam
Mahavir Swami Institute of Technology, Sonipat, India
Anushka Kalra
You can also search for this author in PubMed Google Scholar
Corresponding author
Correspondence to Nitin Tyagi .
Editor information
Editors and affiliations.
Department of Computer and System Sciences, Visva-Bharati University, Santiniketan, West Bengal, India
Paramartha Dutta
Department of Computer Science and Engineering, Institute of Engineering and Management, Kolkata, West Bengal, India
Satyajit Chakrabarti
Department of Computer Application and Science, Institute of Engineering and Management, Kolkata, West Bengal, India
Abhishek Bhattacharya
Soumi Dutta
Department of Electrical and Electronic Engineering, Bangladesh University of Engineering and Technology, Dhaka, Bangladesh
Celia Shahnaz
Rights and permissions
Reprints and permissions
Copyright information
© 2023 The Author(s), under exclusive license to Springer Nature Singapore Pte Ltd.
About this paper
Cite this paper.
Arora, P., Gautam, S., Kalra, A., Negi, A., Tyagi, N. (2023). Credit Risk Analysis Using EDA. In: Dutta, P., Chakrabarti, S., Bhattacharya, A., Dutta, S., Shahnaz, C. (eds) Emerging Technologies in Data Mining and Information Security. Lecture Notes in Networks and Systems, vol 490. Springer, Singapore. https://doi.org/10.1007/978-981-19-4052-1_56
Download citation
DOI : https://doi.org/10.1007/978-981-19-4052-1_56
Published : 16 September 2022
Publisher Name : Springer, Singapore
Print ISBN : 978-981-19-4051-4
Online ISBN : 978-981-19-4052-1
eBook Packages : Intelligent Technologies and Robotics Intelligent Technologies and Robotics (R0)
Share this paper
Anyone you share the following link with will be able to read this content:
Sorry, a shareable link is not currently available for this article.
Provided by the Springer Nature SharedIt content-sharing initiative
- Publish with us
Policies and ethics
- Find a journal
- Track your research
Search code, repositories, users, issues, pull requests...
Provide feedback.
We read every piece of feedback, and take your input very seriously.
Saved searches
Use saved searches to filter your results more quickly.
To see all available qualifiers, see our documentation .
- Notifications
This case study aims to give you an idea of applying EDA in a real business scenario. In this case study, apart from applying the techniques that you have learnt in the EDA module, you will also develop a basic understanding of risk analytics in banking and financial services and understand how data is used to minimise the risk of losing money w…
Gaurav-Gilalkar/Credit_EDA_case_study_upgrad
Folders and files, repository files navigation, credit_eda_case_study_upgrad.
This case study aims to give you an idea of applying EDA in a real business scenario. In this case study, apart from applying the techniques that you have learnt in the EDA module, you will also develop a basic understanding of risk analytics in banking and financial services and understand how data is used to minimise the risk of losing money while lending to customers.
Business Understanding
The loan providing companies find it hard to give loans to the people due to their insufficient or non-existent credit history. Because of that, some consumers use it as their advantage by becoming a defaulter. Suppose you work for a consumer finance company which specialises in lending various types of loans to urban customers. You have to use EDA to analyse the patterns present in the data. This will ensure that the applicants capable of repaying the loan are not rejected. When the company receives a loan application, the company has to decide for loan approval based on the applicant’s profile. Two types of risks are associated with the bank’s decision:
If the applicant is likely to repay the loan, then not approving the loan results in a loss of business to the company
If the applicant is not likely to repay the loan, i.e. he/she is likely to default, then approving the loan may lead to a financial loss for the company.
The data given below contains the information about the loan application at the time of applying for the loan. It contains two types of scenarios:
The client with payment difficulties: he/she had late payment more than X days on at least one of the first Y instalments of the loan in our sample,
All other cases: All other cases when the payment is paid on time.
When a client applies for a loan, there are four types of decisions that could be taken by the client/company):
Approved : The Company has approved loan Application
Cancelled : The client cancelled the application sometime during approval. Either the client changed her/his mind about the loan or in some cases due to a higher risk of the client he received worse pricing which he did not want.
Refused : The company had rejected the loan (because the client does not meet their requirements etc.).
Unused offer : Loan has been cancelled by the client but on different stages of the process.
In this case study, you will use EDA to understand how consumer attributes and loan attributes influence the tendency of default.
Business Objectives
This case study aims to identify patterns which indicate if a client has difficulty paying their installments which may be used for taking actions such as denying the loan, reducing the amount of loan, lending (to risky applicants) at a higher interest rate, etc. This will ensure that the consumers capable of repaying the loan are not rejected. Identification of such applicants using EDA is the aim of this case study.
- Jupyter Notebook 100.0%
Your browser is not supported
Sorry but it looks as if your browser is out of date. To get the best experience using our site we recommend that you upgrade or switch browsers.
Find a solution
We use cookies to improve your experience on this website. To learn more, including how to block cookies, read our privacy policy .
- Skip to main content
- Skip to navigation
- Collaboration Platform
- Data Portal
- Reporting Tool
- PRI Academy
- PRI Applications

- Back to parent navigation item
- What are the Principles for Responsible Investment?
- PRI 2021-24 strategy
- A blueprint for responsible investment
- About the PRI
- Annual report
- Public communications policy
- Financial information
- Procurement
- PRI sustainability
- Diversity, Equity & Inclusion for our employees
- Meet the team
- Board members
- Board committees
- 2023 PRI Board annual elections
- Signatory General Meeting (SGM)
- Signatory rights
- Serious violations policy
- Formal consultations
- Signatories
- Signatory resources
- Become a signatory
- Get involved
- Signatory directory
- Quarterly signatory update
- Multi-lingual resources
- Espacio Hispanohablante
- Programme Francophone
- Reporting & assessment
- R&A Updates
- Public signatory reports
- Progression pathways
- Showcasing leadership
- The PRI Awards
- News & events
- The PRI podcast
- News & press
- Upcoming events
- PRI in Person 2024
- All events & webinars
- Industry events
- Past events
- PRI in Person 2023 highlights
- PRI in Person & Online 2022 highlights
- PRI China Conference: Investing for Net-Zero and SDGs
- PRI Digital Forums
- Webinars on demand
- Investment tools
- Introductory guides to responsible investment
- Principles to Practice
- Stewardship
- Collaborative engagements
- Active Ownership 2.0
- Listed equity
- Passive investments
- Fixed income
- Credit risk and ratings
- Private debt
- Securitised debt
- Sovereign debt
- Sub-sovereign debt
- Private markets
- Private equity
- Real estate
- Climate change for private markets
- Infrastructure and other real assets
- Infrastructure
- Hedge funds
- Investing for nature: Resource hub
- Asset owner resources
- Strategy, policy and strategic asset allocation
- Mandate requirements and RfPs
- Manager selection
- Manager appointment
- Manager monitoring
- Asset owner DDQs
- Sustainability issues
- Environmental, social and governance issues
- Environmental issues
- Circular economy
- Social issues
- Social issues - case studies
- Social issues - podcasts
- Social issues - webinars
- Social issues - blogs
- Cobalt and the extractives industry
- Clothing and Apparel Supply Chain
- Human rights
- Human rights - case studies
- Modern slavery and labour rights
- Just transition
- Governance issues
- Tax fairness
- Responsible political engagement
- Cyber security
- Executive pay
- Corporate purpose
- Anti-corruption
- Whistleblowing
- Director nominations
- Climate change
- The PRI and COP28
- Inevitable Policy Response
- UN-convened Net-Zero Asset Owner Alliance
- Sustainability outcomes
- Sustainable Development Goals
- Sustainable markets
- Sustainable financial system
- Driving meaningful data
- Private retirement systems and sustainability
- Academic blogs
- Academic Seminar series
- Introduction to responsible investing academic research
- Our policy approach
- Policy reports
- Consultations and letters
- Global policy
- Policy toolkit
- Policy engagement handbook
- Regulation database
- A Legal Framework for Impact
- Fiduciary duty
- Australia policy
- Canada Policy
- China policy
- Stewardship in China
- EU taxonomy
- Japan policy
- SEC ESG-Related Disclosure
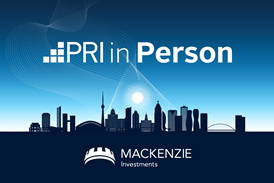
- More from navigation items
ESG, credit risk and ratings: part 3 - from disconnects to action areas
- 1 Executive summary
- 2 Fostering CRA-investor dialogue
- 3 From disconnects to action areas
- 4 A transparent and systematic framework
- 5 Applying theory to practice
- 6 Next steps: Connecting the dots
- 7 Regional colour from the forums
- 8 Sovereign versus corporate credit risk
- 9 CRA examples
- 10 Investor case studies
- 11 Case study: AXA Group
- 12 Case study: BlueBay Asset Management LLP
- 13 Case study: Futuregrowth Asset Management
- 14 Case study: HSBC Global Asset Management
- 15 Case study: Legal & General Investment Management
- 16 Case study: Nikko Asset Management
- 17 Case study: NN Investment Partners
- 18 Case study: Nomura Asset Management
- 19 Case study: Triodos Investment Management
- 20 Case study: Aegon Asset Management
- 21 Case study: Caisse des Depots
- 22 Case study: Colchester Global Investors
- 23 Case study: Insight Investment
- 24 Case study: PIMCO
- 25 Case study: Templeton Global Macro
Case study: HSBC Global Asset Management
2019-01-30T17:49:00+00:00
Case study by HSBC Global Asset Management
Action areas:
- Organisational approach
- Communication and transparency
The investment approach
We believe that ESG issues can have a long-term material impact on company fundamentals, and that they are linked to opportunities and risks which financial markets may not price appropriately.
The integration of ESG factors within our investment process is led by the FI investment team and is not a standalone process. The team comprises 177 members who rely on the support of the Global Credit Research platform, comprising 46 sector and regional analysts including 11 ESG champions, and a separate team of ESG specialists who support the process by providing ESG data, sector knowledge and thematic research.
The current approach is the result of a process (see below) starting in 2002 with the launch of our first sustainable fund. We integrated ESG factors more systematically across our FI process starting in 2007, using external ESG data providers for our research and analysis.
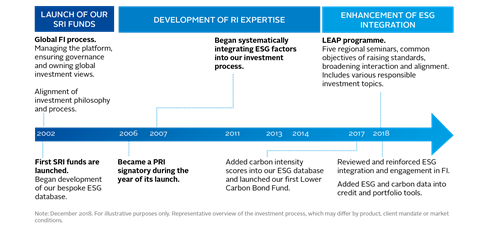
ESG integration throughout the investment decision-making process
Source: HSBC Global Asset Management
Taking the learnings from managing successful sustainable funds, backed by analytical research showing that considering ESG factors is beneficial to the investment process and rarely has a negative impact, we reviewed our process in 2017 and subsequently reinforced our ESG integration and engagement process. This involved the launch of a FI ESG thematic “university” to promote awareness, training and guidance on ESG subject matter, and recruiting a dedicated FI responsible investment advisor.
The investment process
The investment process starts with the selection of our investment universe, involving issuer-level screening in line with our controversial weapons exclusion policy and any other client or strategy exclusions.
We then consider the composite ESG score and summary for each issuer provided by our global ESG database, using data from third-party ESG data providers. The highest-risk names per sector (categorised by emerging and developed markets) are highlighted in the database and require a more detailed level of due diligence by the credit research team before any investment.
Our fundamental research framework for all companies incorporates ESG analysis as specific inputs, including a business profile detailing components of management, governance and strategy, and liabilities (legal, social and environmental). This analysis highlights – firstly and most importantly – any potential negative impacts on the operating profile of the company and, secondly, financial metrics such as revenue and debt/EBITDA. This is supplemented with issuer meetings by the credit research team where further ESG questions specific to the issuer or sector are raised.
CRA reports and external ratings are one of many inputs into our credit analysis, but we do not rely solely on them; in fact, we produce our own proprietary credit ratings. However, we use external credit ratings to define investment universes for funds and mandates. They are also among the second-party certifiers of green bonds we use for green bond assessment.
The credit process ensures we only select issuers whose operating and financial metrics we are comfortable with and exclude those that are viewed as unreliable or have potential idiosyncratic risks. The credit analyst approves each issuer with oversight from the Global Head of Credit Research.
Credit analysts communicate directly with their investment colleagues globally at sector and country level as well as through groups such as the ESG Analyst Group that communicates and shares ESG sector-level research, investment views and engagement findings between analysts in the credit and equity teams. One of the group’s main outputs of 2018 was the production of 24 ESG sector checklists, summarising each industry’s ESG issues and suggesting engagement questions, enabling analysts to focus on the most financially-material dimensions for credit. We monitor engagement activities on a quarterly basis, covering the contact that we have with issuers to ensure that we raise ESG-related questions with them and share our findings across the investment platform. We also monitor funds’ ESG and carbon intensity scores, providing feedback into the portfolio construction process (see below).
One of the main challenges in integrating ESG factors in FI is data availability, partly due to the dominance of data providers using equity indicators. All ESG data needs to be mapped internally to our issuer universe. Coverage of unlisted or sovereign-owned enterprises has yet to be developed.
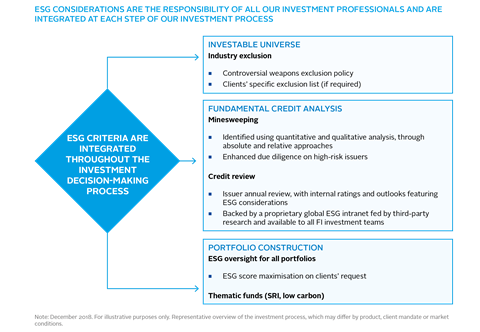
The development of the global approach to responsible investment
The investment outcomes
As part of the fundamental credit process, we require a full understanding of the balance sheet including any potential ESG risks that could impact cash flow, debt/EBITDA and other credit metrics. For privately-held companies, the required financial and ESG information is often unavailable or insufficient to complete a credit review. When this happens, we engage with the issuer, requesting information from the treasurer, CFO or investor relations team.
In a recent example concerning a European unlisted company (with an external credit rating of AA and a low governance rating by an ESG service provider), we were unable to confirm the existence of policies related to anti-corruption. This information was required to complete the liabilities (legal, social and environmental) component of our credit analysis and was potentially financially material due to the issuer’s high level of government-regulated income and involvement with government procurement. We contacted the company and spoke with the treasurer in 2017, when we requested the required policy. The company understood our requirements and agreed to disclose the policy. We were able to approve the credit and invest in its upcoming bond issue.
In another example, in the European unlisted market, a corporate with a complex financial structure and limited public disclosure meant we were unable to form a comprehensive view on the credit. This led to uncertainty about the potential for stable future cash flows and credit metrics. We attempted several engagements with company management to mitigate these concerns, which were unsuccessful and therefore further amplified our concerns. As a result, we felt that this risk was not being priced into the company’s bonds. Based on this limited information, we internally downgraded the credit, and informed the company that we would not be able to participate in any new issues unless it increased its willingness to engage with debt investors.
In both examples, we also considered carbon risk in our credit analysis. However, given the lack of carbon intensity disclosure data in the unlisted corporate market, we are unable to complete our carbon risk calculations and may be increasingly restricted in the size of positions we can hold.
Key takeaways
Although we have been integrating ESG in our investment process for many years, the PRI’s workstreams on FI and on CRAs has highlighted the requirements for clearer explanations of the investment process, particularly in how we consider ESG data and risks and opportunities in our credit research process, as well as the importance of issuer engagement.
Our recent enhancements have increased ESG knowledge and dialogue within the investment team, leading to better evidence of our ESG integration process to clients. We are also planning to introduce a public quarterly report on integrating ESG in FI to enhance our transparency.
Within our propriety tools, we can measure the outcome of our ESG integration through improved portfolio ESG and carbon scores. We believe this will lead to more sustainable risk-adjusted returns for clients in the long term.
Future plans include systematically embedding sector-specific ESG criteria directly into proprietary quantitative credit ratings. This will further enhance our ability to consider ESG data in the credit process.
Download the report

Shifting perceptions: ESG, credit risk and ratings: part 3 - from disconnects to action areas
January 2019
- Corporate debt
- HQ: Developed Markets
- Shifting perceptions: ESG, credit risk and ratings - part 3: from disconnects to action areas
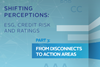
Executive summary
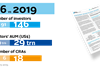
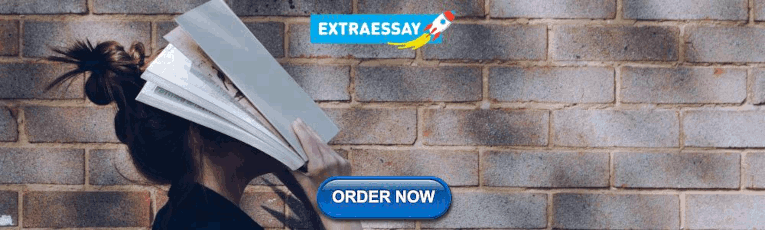
Fostering CRA-investor dialogue
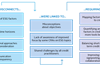
From disconnects to action areas
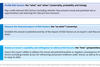
A transparent and systematic framework
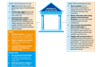
Applying theory to practice
Next steps: connecting the dots.
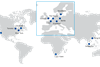
Regional colour from the forums
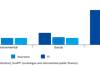
Sovereign versus corporate credit risk
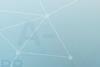
CRA examples
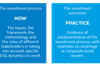
Investor case studies
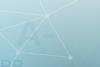
Case study: AXA Group
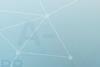
Case study: BlueBay Asset Management LLP
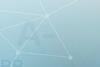
Case study: Futuregrowth Asset Management
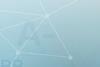
Case study: Legal & General Investment Management
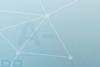
Case study: Nikko Asset Management
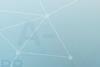
Case study: NN Investment Partners
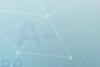
Case study: Nomura Asset Management
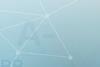
Case study: Triodos Investment Management
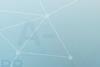
Case study: Aegon Asset Management
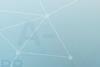
Case study: Caisse des Depots
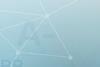
Case study: Colchester Global Investors
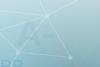
Case study: Insight Investment
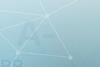
Case study: PIMCO
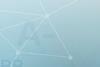
Case study: Templeton Global Macro
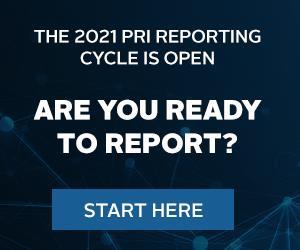
Related content
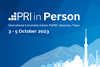
Labelled bonds and loans: Do they do what they say on the tin?
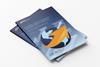
Global responsible investment trends: Inside PRI reporting data
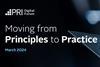
PRI Digital Forum: Moving from Principles to Practice
2024-03-07T07:00:00Z 2024-03-26T21:00:00Z
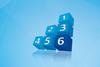
PRI asset class and asset owner guidance advisory committees: Call for new members
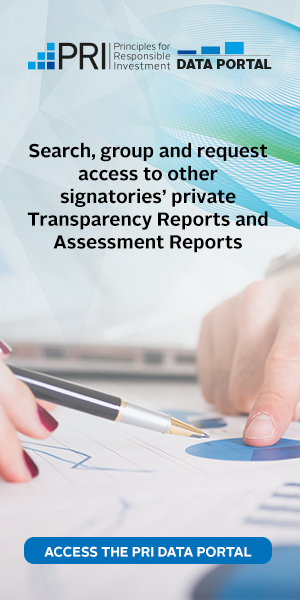
More from Credit risk and ratings
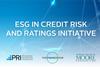
ESG in Credit Risk and Ratings initiative - phase two: Watch the video
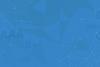
ESG, credit risk and ratings: part 4 - deepening the dialogue between investors, issuers, and CRAs
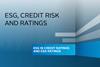
ESG in credit ratings and ESG ratings
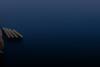
ESG in credit risk: Workshop with insurance companies
- News and press
- Annual Report
- PRI governance
- Privacy policy
- The PRI is an investor initiative in partnership with UNEP Finance Initiative and UN Global Compact .
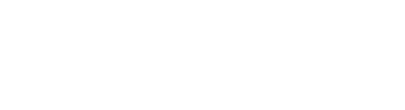
- PRI Association, 25 Camperdown Street, London, E1 8DZ, UK
- Company no: 7207947
- +44 (0)20 3714 3141
- [email protected]
- PRI DISCLAIMER The information contained on this website is meant for the purposes of information only and is not intended to be investment, legal, tax or other advice, nor is it intended to be relied upon in making an investment or other decision. All content is provided with the understanding that the authors and publishers are not providing advice on legal, economic, investment or other professional issues and services. PRI Association is not responsible for the content of websites and information resources that may be referenced. The access provided to these sites or the provision of such information resources does not constitute an endorsement by PRI Association of the information contained therein. PRI Association is not responsible for any errors or omissions, for any decision made or action taken based on information on this website or for any loss or damage arising from or caused by such decision or action. All information is provided “as-is” with no guarantee of completeness, accuracy or timeliness, or of the results obtained from the use of this information, and without warranty of any kind, expressed or implied. Content authored by PRI Association For content authored by PRI Association, except where expressly stated otherwise, the opinions, recommendations, findings, interpretations and conclusions expressed are those of PRI Association alone, and do not necessarily represent the views of any contributors or any signatories to the Principles for Responsible Investment (individually or as a whole). It should not be inferred that any other organisation referenced endorses or agrees with any conclusions set out. The inclusion of company examples does not in any way constitute an endorsement of these organisations by PRI Association or the signatories to the Principles for Responsible Investment. While we have endeavoured to ensure that information has been obtained from reliable and up-to-date sources, the changing nature of statistics, laws, rules and regulations may result in delays, omissions or inaccuracies in information. Content authored by third parties The accuracy of any content provided by an external contributor remains the responsibility of such external contributor. The views expressed in any content provided by external contributors are those of the external contributor(s) alone, and are neither endorsed by, nor necessarily correspond with, the views of PRI Association or any signatories to the Principles for Responsible Investment other than the external contributor(s) named as authors.
Site powered by Webvision Cloud
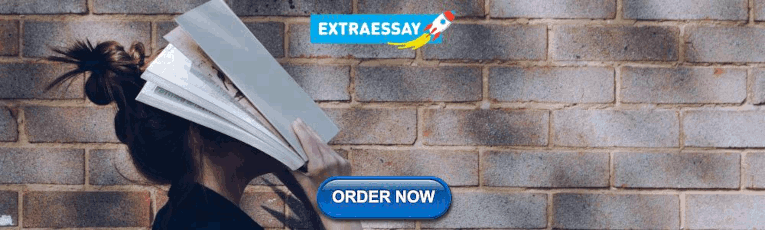
IMAGES
VIDEO
COMMENTS
Analytical Credit Risk Case Studies. Our credit and risk specialists leverage Credit Analytics, our suite of cutting-edge analytical models to provide you with credit risk insights and real-life case studies on the topics that are important to you and your business. Request Follow Up.
Credit Risk Analysis & Modeling: A Case Study DOI: 10.9790/5933-0802026981 www.iosrjournals.org 71 | Page Research Methodology: This study shows how to create a credit score card object, bin data, display and plot binned data information through MATLAB. This study also shows how to fit a logistic regression model, obtain a score for the ...
2.1.1 Conceptual framework of credit risk management This study adapts the credit risk management framework by Watchira (2017) as shown in Figure 1. According to Wachira (2017), there are four independent variables that constitute credit risk management practices. The dependent variable is banks performance as measured by ratio of Non-
PAGE 37. Credit Risk Analysis of Canara Bank - A Case Study. Shaila Kamath 1 & Ramesh Pai 2. 1 Research Scholar, Institute of Management and Commerce, Srinivas University, Mangaluru- 575001 ...
Keywords Credit risk analysis ·Loan EDA case study ... effective study [4]. The data are filtered from all the outliers, and any imbalances present in the dataset are eradicated completely before the analysis for a better accu-racy. Figure 1 depicts the process of how the analysis of the credibility of the person
In particular, the above case study is an example of how ESG considerations can be a source of risk mitigation in fixed income investing. Material ESG risks should be factored into credit valuations, otherwise investors can be exposed to negative events (such as a credit rating downgrade) and their investments could subsequently underperform ...
To date, there has been relatively little research in the field of credit risk analysis that compares all of the well known statistical, optimization technique (heuristic methods) and machine learning based approaches in a single article. Review on credit risk assessment using sixteen well-known approaches has been conducted in this work. The accuracy of the machine learning approaches in ...
The investment approach. The launch of a specialised investment-grade corporate debt strategy led Nomura Asset Management to develop a quantitative ESG risk and portfolio analysis framework focused on corporate sustainability issues material to credit investors. Our research showed that credit portfolios of higher ESG quality have fewer rating ...
Investor case studies. 30 January 2019. This section contains 15 investor case studies. Contributors are asset owners and investment managers that support the ESG in Credit Ratings Statement and have actively engaged with CRAs through the forums that the PRI has organised as part of the initiative. The PRI asked the contributors to describe how ...
A Comparative Assessment of Credit Risk Model Based on Machine Learning ——a case study of bank loan data. Author links open overlay panel Yuelin Wang a, Yihan Zhang a, Yan Lu a, Xinran Yu a. ... a logistic regression model is constructed. 4.Experimental results and analysis Table1: Definition: Positive Negative True TP TN False FP FN ...
Commercial Banking & Credit Analyst (CBCA)® Certification. RockCrusher Rentals is part of the Commercial Banking & Credit Analyst (CBCA)® certification, which includes 59 courses. Skills Learned Financial Analysis, Credit Structuring, Risk Management. Career Prep Commercial Banking, Credit Analyst, Private Lending.
Crediting represents one of the biggest risks faced by the banking sector, and especially by commercial banks. In the literature, there have been a number of studies concerning credit risk management, often involving credit scoring systems making use of machine learning (ML) techniques. However, the specificity of individual banks' datasets means that choosing the techniques best suited to ...
The money lending companies experience financial loss in two ways, by providing and not providing credit to their clients in a way that if the client is likely to repay loan, then not approving the loan will out-turn to be a loss, while if the client is likely to default, then approving would bring in loss again [].The research work, with the help of credit risk analysis using the current and ...
The investment approach. Insight believes that investing effectively in sovereign debt requires in-depth analysis of ESG matters. However, most ESG analysis and research focuses on corporates - not countries. As a result, awareness of the materiality of risks, and the availability of tools to help make informed decisions, are lacking for ...
The aim of this group case study is to allow participants to apply the framework and tools of credit analysis to a company and make a concise and conclusive presentation to the group on the final day of the course: • Preparation and presentation of a complete credit analysis and critique of a financing proposal in small groups of 2 to 5 ...
Credit Risk - Predictive Modelling Radek Lastovicka ... Model Development Model Validation Methodological Reviews Asset Quality Reviews Data Analysis ... We recommend teams for case study Menti.com -14 13 31 65 Credit Risk -Predictive Modelling 1. Credit Risk & Banking W 09:15-09:50 2. Underwriting & Scoring W 09:50-10:25
Keywords: Credit Risk, Non-Performing Loans, Credit Worthiness, Bankruptcy, Systematic Risk 1. INTRODUCTION: Credit risk is the oldest form of risk that is faced by the bankers across the globe. It is the risk of default on loans. Credit risk is the biggest risk the bank face by the virtue of nature of business, inherits.
Proprietary ratings model: PIMCO's proprietary sovereign credit ratings model incorporates many quantitative ESG indicators, which include near and long-term drivers of credit risk, as well as variables that may be more slow moving and have more diffuse effects. These include measures of political stability, voice and accountability, rule of ...
The use of survival analysis techniques to study credit risk, and more particularly to model PD, can be motivated via Figure 1. It presents three common situations that may occur in practice when a credit company observes the "lifetime" of a credit. Let us consider the interval [0,τ] as the horizon of the study.
In particular, the above case study is an example of how ESG considerations can be a source of risk mitigation in fixed income investing. Material ESG risks should be factored into credit valuations, otherwise investors can be exposed to negative events (such as a credit rating downgrade) and their investments could subsequently underperform the
These two works confirm the interest of SVM and neural methods. Below, we will be focusing on two ML methods, namely SVM and RF. Our choice of these two methods derives from a careful study of the literature and from our own analysis of the case in hand, as we will explain in the remainder of the paper. 2.2.
This case study aims to give you an idea of applying EDA in a real business scenario. In this case study, apart from applying the techniques that you have learnt in the EDA module, you will also develop a basic understanding of risk analytics in banking and financial services and understand how data is used to minimise the risk of losing money while lending to customers.
This led to uncertainty about the potential for stable future cash flows and credit metrics. We attempted several engagements with company management to mitigate these concerns, which were unsuccessful and therefore further amplified our concerns. As a result, we felt that this risk was not being priced into the company's bonds.