- Open access
- Published: 09 September 2020
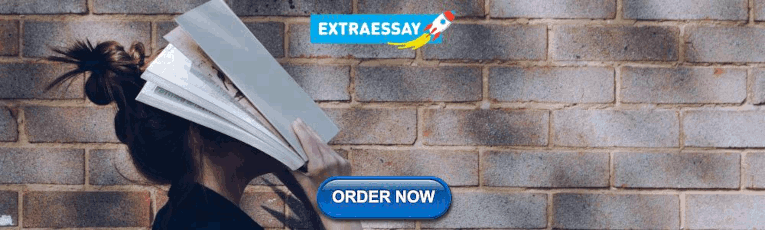
Electricity load forecasting: a systematic review
- Isaac Kofi Nti ORCID: orcid.org/0000-0001-9257-4295 1 ,
- Moses Teimeh 2 ,
- Owusu Nyarko-Boateng ORCID: orcid.org/0000-0003-0300-2469 3 &
- Adebayo Felix Adekoya ORCID: orcid.org/0000-0002-5029-2393 3
Journal of Electrical Systems and Information Technology volume 7 , Article number: 13 ( 2020 ) Cite this article
30k Accesses
121 Citations
Metrics details
The economic growth of every nation is highly related to its electricity infrastructure, network, and availability since electricity has become the central part of everyday life in this modern world. Hence, the global demand for electricity for residential and commercial purposes has seen an incredible increase. On the other side, electricity prices keep fluctuating over the past years and not mentioning the inadequacy in electricity generation to meet global demand. As a solution to this, numerous studies aimed at estimating future electrical energy demand for residential and commercial purposes to enable electricity generators, distributors, and suppliers to plan effectively ahead and promote energy conservation among the users. Notwithstanding, load forecasting is one of the major problems facing the power industry since the inception of electric power. The current study tried to undertake a systematic and critical review of about seventy-seven (77) relevant previous works reported in academic journals over nine years (2010–2020) in electricity demand forecasting. Specifically, attention was given to the following themes: (i) The forecasting algorithms used and their fitting ability in this field, (ii) the theories and factors affecting electricity consumption and the origin of research work, (iii) the relevant accuracy and error metrics applied in electricity load forecasting, and (iv) the forecasting period. The results revealed that 90% out of the top nine models used in electricity forecasting was artificial intelligence based, with artificial neural network (ANN) representing 28%. In this scope, ANN models were primarily used for short-term electricity forecasting where electrical energy consumption patterns are complicated. Concerning the accuracy metrics used, it was observed that root-mean-square error (RMSE) (38%) was the most used error metric among electricity forecasters, followed by mean absolute percentage error MAPE (35%). The study further revealed that 50% of electricity demand forecasting was based on weather and economic parameters, 8.33% on household lifestyle, 38.33% on historical energy consumption, and 3.33% on stock indices. Finally, we recap the challenges and opportunities for further research in electricity load forecasting locally and globally.
Electricity is the pivot in upholding highly technologically advanced industrialisation in every economy [ 1 , 2 , 3 ]. Almost every activity done in this modern era hinges on electricity. The demand and usage of electric energy increase globally as the years past [ 4 ]; however, the process of generating, transmitting, and distributing electrical energy remains complicated and costly. Hence, effective grid management is an essential role in reducing the cost of energy production and increased in generating the capacity to meet the growing demand in electric energy [ 5 ].
Accordingly, effective grid management involves proper load demand planning, adequate maintenance schedule for generating, transmission and distribution lines, and efficient load distribution through the supply lines. Therefore, an accurate load forecasting will go a long way to maximise the efficiency of the planning process in the power generation industries [ 5 , 6 ]. As a means to improve the accuracy of Electrical Energy Demand (EED) forecasting, several computational and statistical techniques have been applied to enhance forecast models [ 7 ].
EED forecasting techniques can be clustered into three (3), namely correlation, extrapolation, and a combination of both. The Extrapolation techniques (Trend analysis) involve fitting trend curves to primary historical data of electrical energy demand in a way to mirror the growth trend itself [ 7 , 8 ]. Here, the future value of electricity demand is obtained from estimating the trend curve function at the preferred future point. Despite its simplicity, its results are very realistic in some instances [ 8 ].
On the other hand, correlation techniques (End-use and Economic models) involve relating the system load to several economic and demographic factors [ 7 , 8 ]. Thus, the techniques ensure that the analysts capture the association existing between load increase patterns and other measurable factors. However, the disadvantage lies in the forecasting of economic and demographic factors, which is more complicated than the load forecast itself [ 7 , 8 ]. Usually, economic and demographic factors such as population, building permits, heating, employment, ventilation, air conditioning system information, weather data, building structure, and business are used in correlation techniques [ 7 , 8 , 9 ]. Nevertheless, some researchers group EED forecasting models into two, viz. data-driven (artificial intelligence) methods (same as the extrapolation techniques) and engineering methods (same as correlation the techniques) [ 9 ]. All the same, no single method is accepted scientifically superior in all situations.
Also, proper planning and useful applications of electric load forecasting require particular “forecasting intervals,” also referred to as “lead time”. Based on the lead time, load forecasting can be grouped into four (4), namely: very short-term load forecasting (VSTLF), short-term load forecasting (STLF), medium-term load forecasting (MTLF) and long-term load forecasting (LTLF) [ 6 , 7 , 10 ]. The VSTLF is applicable in real-time control, and its predicting period is within minutes to 1 h ahead. The STLF is for making forecasting within 1 h to 7 days or month ahead [ 11 ]. It is usually used for the day-to-day operations of the utility industry, such as scheduling the generation and transmission of electric energy. The MTLF is used for forecasting of fuel purchase, maintenance, utility assessments. Its forecasting period ranges from 1 week to 1 year. While the LTLF is for making forecasting beyond a year to 20 years ahead, it is suitable for forecasting the construction of new generations, strategic planning, and changes in the electric energy supply and delivery system [ 10 ].
Notwithstanding the above-mentioned techniques and approaches available, EED forecasting is seen to be complicated and cannot easily be solved with simple mathematical formulas [ 2 ]. Also, Hong and Fan [ 12 ] pointed out that electric load forecasting has been a primary problem for the electric power industries, since the inception of the electric power. Regardless of the difficulty in electric load forecasting, the optimal and proficient economic set-up of electric power systems has continually occupied a vital position in the electric power industries [ 13 ]. This exercise permits the utility industries to examine the dynamic growth in load demand patterns to facilitate continuity planning for a better and accurate power system expansion. Consequently, inaccurate prediction leads to power shortage, which can lead to “dumsor” and unneeded development in the power system leading to unwanted expenditure [ 7 , 14 ]. Besides, a robust EED forecasting is essential in developing countries having a low rate of electrification to facilitate a way for supporting the active development of the power systems [ 15 ].
Based on the sensitive nature of electricity demand forecasting in the power industries, there is a need for researchers and professionals to identify the challenges and opportunities in this area. Besides, as argued by Moher et al. [ 16 ], systematic reviews are the established reference for generating evidence in any research field for further studies. Our partial search of literature resulted in the following [ 10 , 12 , 17 , 18 , 19 , 20 , 21 ] papers that focused on comprehensive systematic review concerning the methods, models, and several methodologies used in electric load forecasting. Hammad et al. [ 10 ] compared forty-five (45) academic papers on electric load forecasting based on inputs, outputs, time frame, the scale of the project, and value. They revealed that despite the simplicity of regression models, they are mostly useful for long-term load forecasting compared with AI-based models such as ANN, Fuzzy logic, and SVM, which are appropriate for short-term forecasting.
Similarly, Hong and Fan [ 12 ] carried out a tutorial review of probabilistic EED forecasting. The paper focused on EED forecasting methodologies, special techniques, common misunderstandings and evaluation methods. Wang et al. [ 19 ] presented a comprehensive review of factors that affects EED forecasting, such as forecast model, evaluation metric, and input parameters. The paper reported that the commonly used evaluation metrics were the mean absolute error, MAPE, and RMSE. Likewise, Kuster et al. [ 22 ] presented a systematic review of 113 studies in electricity forecasting. The paper examined the timeframe, inputs, outputs, data sample size, scale, error type as criteria for comparing models aimed at identifying which model best suited for a case or scenario.
Also, Zhou et al. [ 17 ] presented a review of electric load classification in the smart-grid environment. The paper focused on the commonly used clustering techniques and well-known evaluation methods for EED forecasting. Another study in [ 21 ] presented a review of short-term EED forecasting based on artificial intelligence. Mele [ 20 ] presented an overview of the primary machine learning techniques used for furcating short-term EED. Gonzalez-Briones et al. [ 18 ] examined the critical machine learning models for EED forecasting using a 1-year dataset of a shoe store. Panda et al. [ 23 ] presented a comprehensive review of LTLF studies examining the various techniques and approaches adopted in LTLF.
The above-discussed works of literature show that two studies [ 20 , 21 ] address a comprehensive review on STLF, [ 23 ] addresses forecasting models based on LTLF. The study in [ 24 ] was entirely dedicated to STLF. Only a fraction (10%) of above systematic review studies included STLF, MTLF and LTLF papers in their review; however, as argued in [ 10 ], the lead time (forecasting interval) is a factor that positively influences the performance of a chosen model for EED forecasting studies. Again, a high percentage of these studies [ 10 , 12 , 17 , 18 , 19 , 20 , 21 , 22 , 24 ] concentrated on the methods (models), input parameter, and timeframe. Nevertheless, Wang et al. [ 19 ] revealed that the primary factors that influence EED forecasting models are property (characteristic) parameters of the building and weather parameters include. Besides, these parameters are territorial dependant and cultural bond. Thus, the weather pattern is not the same world-wide neither do we use the same building architecture and materials globally.
Notwithstanding, a higher percentage of previous systematic review studies overlooked the origin of studies and dataset of EED forecasting paper. Also, only a few studies [ 12 , 17 , 19 ] that examined the evaluation metrics used in EED forecasting models. However, as pointed out in [ 17 ], there is no single validity index that can correctly deal with any dataset and offer better performance always.
Despite all these review studies [ 10 , 12 , 17 , 18 , 19 , 20 , 21 , 22 , 24 ] on electricity load forecasting, a comprehensive systematic review of electricity load forecasting that takes into account all possible factors, such as the forecasting load (commercial, residential and combined), the forecast model (conventional and AI), model evaluation metrics and forecasting type (STLF, MTLF, and LTLF) that influences EED forecast models is still an open gate for research. Hence, to fill in the gap, this study presents an extensive systematic review of state-of-the-art literature based on electrical energy demand forecasting. The current review is classified according to the forecasting load (commercial, residential, and combined), the forecast model (conventional, AI and hybrids), model evaluation metrics, and forecasting type (STLF, MTLF, and LTLF). The Preferred-Reporting Items for Systematic-Review and Meta-Analysis (PRISMA) flow diagram was adopted for this study based on its ability to advance the value and quality of the systematic review as compared with other guidelines [ 16 , 25 , 26 ]. The current study contributes to knowledge as follows:
A comprehensive and detailed assessment of previous state-of-the-art studies on electricity demand forecasting; based on used methods, timeframe, the train and test split of data, error, and accuracy metrics applied to forecast.
We present a concise summary of the useful characteristics of compared techniques in electric load forecasting.
We identified the challenges and opportunities for further studies in electric load forecasting.
The remaining sections of the current paper are structured as follows. “ Methodology ” section presents the methods and materials used in the current study. “ Data collection ” section presents the results and a detailed discussion of the outcomes, and “ Study framework ” section presents the summary of findings and direction for future studies.
Methodology
The current study presents a systematic review of pertinent literature on electrical energy forecasting.
Data collection
A total of eighty-one (81) state-of-the-art research works published in journals, conferences, and magazines, and student’s thesis relevant to the scope of the current study were downloaded from the internet, thus using keywords and terms which included AI, Electricity Prediction (EP), Energy Forecasting (EF), Machine Learning (ML), and combination of AI and EP, AI and EF, ML and EP, ML and EF. Each downloaded literature was then carefully studied and categorised into the two methods of electrical load forecasting data-driven (artificial intelligence) methods and engineering methods.
Study framework
According to Moher et al. [ 16 ], the quality of every systematic review is based on building protocol, which outlines the justification, hypothesis, and planned methods of the investigation. However, only a few systematic review study reports of their framework. A detailed, well-described structure for systematic reviews facilitates the understanding and evaluation of the methods adopted. Hence, the PRISMA model [ 26 ] was adopted in this study (Fig. 1 ). As shown in Fig. 1 , the PRISMA presents the flow of information from one stage to another in a systematic review of the literature and gives the total number of the research identified, excluded, and included and the reasons for inclusion and exclusions.
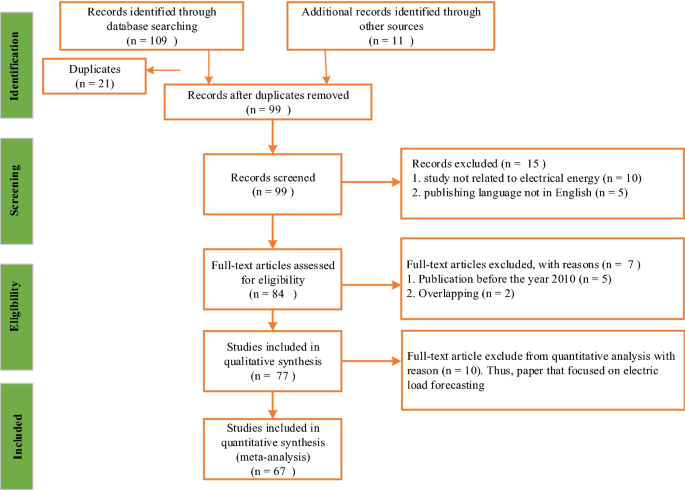
Source : Moher et al. [ 26 ]
The adopted PRISMA flow diagram.
The PRISMA flow diagram involved five (5) phases, as shown in Fig. 1 . Phase 1 consists of outlining the review scope, developing questions, and inclusion or exclusion. Phase 2 searches the literature with keywords to identify potential studies. Phase 3 includes determining the addition of a paper by screening its abstracts if it meets inclusion criteria. While phase 4 includes characterisation of paper for mapping by keywords. This review aimed to document an overview of research in the field of electric load forecasting to make way for future studies. As a result, a fifth (5) step offers an in-depth quantitative synthesis (meta-analysis) of studies included in the review.
Our search of literature retrieved one hundred and one (109) papers from online journals and eleven (11) from under sources, making one hundred and twelve (120) records in all (see Fig. 1 ). Of the 120 records, 21 were duplicates, hence, removed leaving ninety-one (99) record shortlisted for the screening stage. At the screening stage, fifteen (15) records were removed; thus, studies that were not related to electrical energy, and those that the primary publication language was not in English. Leaving eighty-four (84) records, of the 84 records, we further remove five (5) more records that were published before the year 2010, two (2) record omitted due to overlapping, this reduced eligible papers for analysis to seventy-seven (77). The 77 papers were used for the qualitative analysis. Ten (10) records that presented a review of electric load forecasting were also removed, and the remaining (67) were used for the quantitative analysis, as shown in Fig. 1 .
Results and discussion
Electrical energy consumption can be classified as residential (domestic), commercial (non-residential), or industrial. Residential or domestic refers to the home or a dwelling where people globally live from day-to-day. At the same time, commercial consumers are the business and industries that require massive supply than residential users for their businesses [ 27 ]. Selected literature was on electric load forecasting was classified into two main categories AI methods and engineering methods. However, each category was further grouped into residential and commercial or combined (residential and commercial), and the outcomes are presented. A total of seventy-seven (77) papers were eligible for the qualitative analysis, while sixty-seven (67) were included in the quantitative analysis, as already discussed above in this study.
AI methods used in electrical energy demand forecasting
This section presents the studies that were based on AI techniques.
Combined (commercial and residential)
Most works on EED forecasting sought to forecast the total load (residential and commercial) demand on the supplying authority. This section presents the selected studies that fell in this category of electric load forecasting.
Hybrid models
In a way to harness the strength in different machine learning techniques, some researchers sought to hybrid two or more ML techniques to improve the forecasting accuracy of their models.
A short-term (next-day) EED forecasting model based on the historical meteorological parameter to forecast the future load on the Greek Electric Network Grid using Support Vector Machine (SVM), ensemble XGBoost, Random Forest (RF), k-Nearest Neighbours (KNN), Neural Networks (NN) and Decision Trees (DT) was proposed by [ 28 ]. The mean absolute percentage error (MAPE) was used as a performance metric for comparison among selected models by the author. The study achieved a reduction in prediction error or + 4.74% compared with the Operator of the Electricity Market in Greece predictions. In other studies [ 29 ], long-term (10 years) EED forecasting using NN and Autoregressive Integrated Moving Average (ARIMA) was proposed to forecast the EED of Kuwait. Weather temperature and humidity, average salary, gross domestic, oil price, population, residence, passengers, currency earning rate, and economic factors like (total import and export in USD) were used as independent variables. The study concluded that NN outperformed ARIMA and weather parameters were found to be more significant than average salary, gross domestic and oil price.
A Particle Swarm Optimisation (PSO) and Differential Evolution (DE) for forecasting the Andhra Pradesh Grid using weather parameters were presented [ 6 ]. The reported concluded that better prediction accuracy was achieved with PSO and DE than the conventional time series forecast model. In another study, a Curve Fitting Algorithm (CFA) was proposed for forecasting electricity power demand for an hour/day/week/month [ 30 ]. Their study shows that future electricity demand can be effectively forecasted based on past demand. In a process to increase forecasting accuracy, historical electricity data combined with Twitter data was used as an input variable to hybrid ANN and SVM forecast model to forecast the electricity consumption in Dutch [ 14 ]. The authors compared the performance of ANN to SVM and concluded that the ANN outperformed the SVM. On the other side, the SVM performance in accuracy increases in long-term forecasting. The authors again admit that inclusion of weather data as input could not increase model performance. Similarly, in Ghana, an attempt to predict the 30-day ahead EED demand of the Bono region using a hybrid ML (MLP, SVM, and DT) based on historical weather and electricity demand was made [ 7 ]. The authors achieved 95% prediction accuracy; however, it affirms that the inclusion of household lifestyle as an input variable will improve prediction accuracy.
The hybrid of ML (SVM and RF) and time-series models Generalized Linear Model (GLM) and ARIMA forecast model was proposed for predict electricity consumption in South Africa based on the historical electricity price, load demand, and weather parameter [ 31 ]. The outcome of the study showed that the ARIMA-GLM combination performs better for long-term forecasting. Similarly, a combination of quantum search with SVM (quantum computing and the chaotic mechanics) for forecasting yearly EED in Taiwan was presented [ 32 ]. The empirical analysis revealed that the proposed model exhibits considerably enhanced forecasting performance than other SVM-based forecasting models. A hybrid of mode decomposition (EMD), PSO, and SVM model was present for forecasting short-term EED demand of the Australian electricity market [ 33 ]. Sulandari et al. [ 34 ] proposed a hybrid of ANN and Fuzzy algorithm and a recurrent formula (LRF) to predict electricity demand in Indonesian. The study results showed that the hybrid model performed well with low values of RMSE. Likewise, in [ 35 ], a hybrid of clustering technique (K-means) and ARIMA forecasting model was presented to forecast university buildings electricity demand. Paper revealed that the hybrid model outperformed the ARIMA model alone as a forecast model.
An artificial neural network (ANN)-based forecast model for short-term forecasting of Chhattisgarh State electricity demand was proposed [ 36 ], using historical weather data as input variables. The results conclude that ANN can efficiently forecast electricity demand. Likewise, an ANN model was applied to forecast the short-term electricity demand of the Iraqi National Grid [ 37 ]. The authors achieved high accuracy and a reasonable error margin. In a similar study, a DT algorithm forecast model was proposed for forecasting future EED on the Yola/Jimeta power transmission company using weather parameters. Again, a short to medium term EED forecasting using deep machine learning (ML) algorithm long short-term memory (LSTM)-based neural network enhanced with genetic algorithm (GA) for feature selection was proposed [ 9 ], to forecast France metropolitan’s electricity consumption. The mean absolute error (MAE) and root-mean-square error (RMSE) were used as the performance metrics, and the weather parameter was used in the independent variable. Their results affirm that weather parament is very useful in forecasting future electricity demand.
An enhanced Convolutional Neural Network (CNN) and enhanced SVM-based forecast model was presented for forecasting electricity price and load forecasting using [ 38 ]. Despite the enhancement made by authors, they recommended additional enhancement of classifiers to improve prediction. In a similar study, an ANN model to forecast consumer demand in North Cyprus was proposed [ 39 ]. Their results affirm the ability for ANN effective automatic modelling of electricity; however, the study concluded this could be achieved when the training and testing datasets are meaningful. A forecast model using a deep belief network using historical EED of Macedonian (2008–2014) was proposed to forecast a short-term (1 day) EED. The outcome of the forecast shows a reduction in MAPE by 8.6% by the proposed model compared with traditional techniques [ 2 ]. In Young et al. [ 40 ], an ANN with Bayesian regularisation algorithm-based model for short-term load forecasting of commercial building electricity usage was carried out. An ANN and hybrid methods to forecast electricity consumption of Turkey were proposed Aydogdu et al. [ 41 ]. The proposed model gave an average absolute prediction error of 2.25%.
Khwaja et al. [ 11 ] presented an ensemble ANN predictive model to enhance short-term electricity load forecasting. Different from existing studies, the authors combined both bagging and boosting techniques to train bagged-boosted ANNs. The study results showed that the proposed ensemble technique offered a reduction of both variance and bias compared to a bagged ANN, single ANN, and boosted ANN. Also, Ahmad et al. [ 42 ] combined Extreme Learning Machine and an enhanced Support Vector Machine to forecast short-term electricity demand. The study outcome showed that the proposed hybrid model outperformed other state-of-the-art predictive models in terms of performance and accuracy. Atef and Eltawil [ 43 ] proposed a deep-stacked LSTM forecasting model to forecast electricity demand. The paper reported that bidirectional (Bi-LSTM) networks outperformed unidirectional (Uni-LSTM) in terms of forecasting accuracy. Also, a generalised regression Neural Network (GRNN) predictive model was proposed in [ 44 ] to predict short-term electricity demand. The study results showed that a GRNN of 30 neurons offered better prediction accuracy in terms of MAPE and MAE than a GRNN of 10 neurons.
Fuzzy logic models
A Fuzzy Logic (FL)-based forecasting model for the next-day electricity demand in Albania was presented [ 45 ]. The time, the historical and forecasting value of the temperature and the previous day load (L) served as the independent variables for the forecast of the next-day consumption. The outcome of the study yielded accurate forecasting by the FL model. Motepe et al. [ 46 ] proposed an adaptive neuro-fuzzy inference system (ANFIS) model for forecasting South African electricity demand. The author concluded that adding temperature as an input parameter to the proposed model did not enhance forecast accuracy, as typically expected.
A new economy (stock indices) reflecting the STLF model for electricity demand forecasting was proposed [ 47 ]. The authors attempted to forecast the future demand for electricity based on the Taiwan Stock Exchange Capitalization Weighted Stock Index (TAIEX) data. The study revealed a significant relationship between stock market function and energy demand, which was helpful to financial analysis wanting to do reverse engineering.
Residential load forecasting
A survey in 2019 shows that EED forecasting, especially short-term load forecasting for individual (residential) electricity customers, plays an increasingly essential role in the future grid planning and operation Kong et al. [ 51 ]. Similarly, the outcome of Leahy and Lyons [ 48 ] affirms that water heating styled used by a household is even more essential than the number of electrical appliances when explaining domestic electrical energy usage. Furthermore, a study in Portugal shows that residential electricity consumption since 1990 has been increasing more rapidly than the Gross Domestic Product (GDP) per capita [ 49 ]. Therefore, studies that focused on forecasting residential EED is of necessity. However, the results revealed that eight (8) out of the sixty-seven (67) representing (11.94%) reviewed works focused on residential electricity forecasting, and a section of these studies is presented.
An hourly prediction of residential energy consumption using RF and SVM was proposed by Hedén [ 50 ], using 187 households in Austin. Error metrics are mean bias (MB), coefficient of variance (CV), and MAPE. The study achieved better forecasting accuracy compared with traditional time series models. Again, an LTSM for short-term residential load forecasting based on residential meter reading [ 51 ] and resident behaviour learning [ 52 ] was studied. The outcomes of these works showed the effectiveness of the LTSM in electricity demand forecasting.
In the same way, linear regression, RF, and SVM predictive model (data-driven) for estimation of city-scale energy use in buildings were proposed by Kontokosta and Tull [ 53 ]. The outcome of the study revealed that adequate electricity consumption in a building could be predicted using actual data from a moderately small subset of buildings. Likewise, unsupervised ML algorithms such as Self-Organising Maps (SOM), k-means, and k-medoid were used to cluster residential electricity based on their trend of electricity use within the day [ 54 ]. The study found that households and how they use electricity in the home can be categorised based on specific customer characteristics. Mcloughlin et al. [ 55 ] examined residential electricity consumption patterns in Irish based on occupant socio-economic and dwelling variables. The variable examined includes the number of bedrooms, dwelling type, age of household head, social class, household composition, and water heating.
The study outcome showed a positive association between maximum demand periods and several household appliances, especially dishwashers, electric cookers tumble dryers, with electric cook topping. The study further found that the time of use of electrical appliances was dependent on occupant characteristics, and younger occupants of a household tend to use more electricity than the older. Alike, an ARIMA, NN, and exponential smoothening forecast model were proposed for forecasting household electricity demand [ 56 ]. The authors concluded that forecasting accuracy varies considerably depending on the choice of forecasting techniques/tactic and configuration/selection of input parameters. Again, a neural network model for predicting residential building energy consumption was proposed by Biswas et al. [ 57 ]. The outcome of the study showed that models based on OWO-Newton algorithms and Levenberg–Marquardt outperformed previous literature.
Engineering and traditional electrical energy demand forecasting
This section presents the electrical energy demand forecasting study based on the traditional time-series and engineering models.
A predictive model for the prediction of medium-term (1-year) electricity consumption of general households based on the lifestyle of the household using Lasso and Group Lasso was proposed [ 58 ]. Their results showed that household lifestyle such as family composition, age, and house-type is good predictors of electricity consumption in a home. Similarly, in Nigeria, an attempt was made to estimate the electricity demand of residential users to support energy transitions using the engineering approach, such as calculating the total power consumed in a household based on the power rating of appliance and their duration of use [ 59 ]. The study concludes that the proposed system can serve policymaking in Nigeria to improve the financial sustainability of the energy supply structure. An extended Autoregressive Distributed Lag (ARDL) model was proposed to estimate residential electricity consumption per capita demand function, which depends on the GDP per capita in Algeria [ 60 ]. The study concluded that promoting financial growth among citizens of Algeria would reduce electricity consumption, since wealthier people (higher income earners) mighty use of more efficient appliances.
In Bogomolov et al. [ 61 ], authors used general public dynamics derived data from cellular network and energy consumption dataset to predict the next-day energy demand. The study could serve a model to enhance the energy meter to promote energy conservation. Likewise, the historical data of on–off times of residential appliances were used to predict the next-day electricity demand using Bayesian inference [ 62 ]. The study concluded that historical electricity consumption data only is not adequate for a decent eminence hourly forecast.
Also, an ARIMA and Holt-Winter model was proposed for forecasting the national electricity consumption of Pakistan from 1980 to 2011 [ 3 ]. The study revealed that the demand in household energy consumption would higher as compare with all other sectors. Jain et al. [ 63 ] proposed an ARIMA forecaster for forecasting electricity consumption. The proposed model achieved a MAPE of 6.63%. The authors concluded that the ARIMA model has the potential of computing in EED forecasting with other forecast techniques. Integration of three (3) forecasting model, long-range energy alternative planning (LEAP), ARIMA, and Holt-Winter, was proposed for forecasting long-term energy demand in Pakistan [ 64 ]. The study would be valid for energy supplies for accurate estimation of users’ demand in the future. The combination of Extreme Learning Machine and Multiple Regression for forecasting China’s electricity demand was proposed [ 65 ]. A quantile regression (QR) model for long-term electricity demand forecasting in South Africa within 2012 and 2015 was presented by Mokilane et al. [ 66 ]. The model was helpful to power distribution industries in the country.
An attempt was made to forecast the electricity consumption of Ghana by 2030 using ARIMA-based model. The study outcome projected that Ghana’s electricity consumption would grow from 8.5210 billion kWh in 2012 to 9.5597 billion kWh in 2030 [ 67 ]. However, in 2017, a report by the energy commission revealed that electricity consumption was 14,247 GWh [ 68 ]. A generalised additive model was adopted for forecasting medium-term electric energy demand in a South African power supply system [ 69 ]. The outcome of the study revealed a useful application of the proposed model in the power generation and distribution industries in the country. An investigation between the association of causal nexus and (environmental pollution, energy use, GDP per capita, and urbanisation) in an attempt to forecast Nigeria’s energy use by 2030 was carried out using the ARIMA and ETS models [ 70 ]. The study outcome showed better forecasting accuracy by both models, and a high rise in energy demand was observed.
Multiple regression analysis approaches for forecasting the yearly electricity demand in commercial sectors and electricity access rates in rural and urban households in some selected West African countries, which included Ghana, were carried out by Adeoye and Spataru [ 71 ]. The study showed that there is a very high variation in hourly electricity demand in the dry seasons. Their results affirm Nti et al. [ 7 ] report that the demand pattern of electricity in Ghana is highly dependent on the month of the year. In a way, one can say there is a partial agreement in these two studies. A time-series regression model for forecasting South African’s peak load demand was presented [ 72 ]. Experimental fallouts indicated that when the temperature is included as an input parameter, improvement in accuracy by the forecast model was realised. ARIMA-based predictive model for predicting both sectoral and total electrical energy consumption of Turkey for the next 15 years was proposed [ 73 ]. The study points out that the demand for electrical energy in agriculture sectors, transport, public service, residential, and commercial will keep increasing.
Similarly, partially linear additive quantile regression models for forecasting short-term electricity demand during the peak-demand periods (i.e., from 6:00 to 8:00 pm) were carried out in South African [ 74 ]. The authors found out that the use of the proposed system in power utility industries for the planning, scheduling, and dispatching of electricity activities will result in a minimal cost principally during the peak-period hours. Caro et al. [ 75 ] predicted the Spanish electricity demand using the ARIMA model. The study achieved an improvement in the short-term predictions of electricity demand with less computational time. A short-term electricity load forecasting model based on dynamic mode decomposition was proposed in [ 76 ], the proposed model showed better stability and accuracy compared with other predictive models.
Quantitative analysis of findings
The descriptive statistics of the study outcome is presented under this section with tables and charts.
Algorithms used for forecast models
As part of the aim of the review, we sought to find out the most used algorithms in electricity forecasting models. The most top nine (9) used algorithms found in the sixty-seven (67) article are presented (Fig. 2 ); this includes only algorithms that were used in more than a single paper. The study outcome revealed that 90% out of the top nine algorithms were AI-based, with ANN representing 28% of AI models used in electricity forecasting. Besides, the ANN models were primarily used for STLF where electrical energy consumption patterns are more intricate than LTLF. The traditional AFRIMA recorded 17.5% due to its efficiency in LTLF, where load fluctuations and periodicity are less critical.
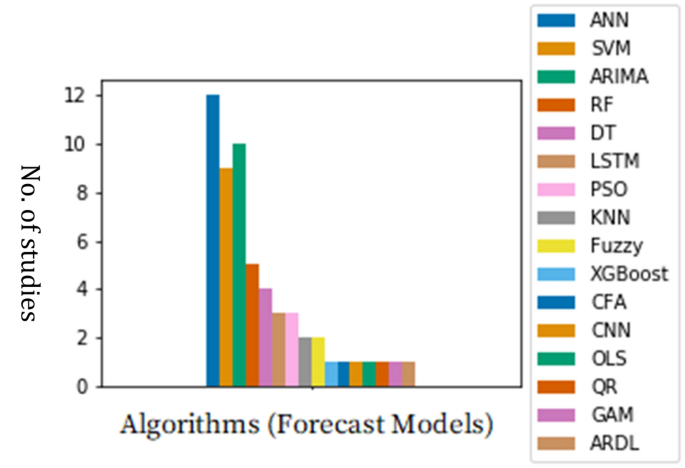
Top nine (9) most used algorithms for electricity forecasting
Additionally, a high percentage of regression models is used for LTLF prediction. The study outcome shows how AI is applied in various sectors of the economy to improve efficiency and profitability. Also, we observed that the SVM, PSO and Fuzzy are gaining more popularity in recent studies, a sign of increasing attention of researchers on these algorithms for EED forecasting.
Study origin
Table 1 shows the studies and their origin (countries). The origin of surveyed studies was examined, in order to find the linkage between the power crisis in the continent and studies on electrical energy demand forecasting. Concerning geographical coverage, it was found that a high number of studies (31.34%) were carried out in Europe, 17.91% in Africa and 19.40% in Asia. Making Africa the third-highest, however, interestingly, most of the studies in Africa were carried in South Africa (five representing 41.67%), three (3) representing 25% in Ghana, with the rest from Nigeria and other African countries. The energy crisis that hit Europe in 2008, reported by [ 77 ], can be attributed to the numerous studies in electricity forecast, as shown in Table 1 . It was further observed that 2 out of 3 studies in Ghana were based on national or regional electricity demand forecasting (one long-term, one short-term (1 month)). While the third study aimed at identifying the relationship between electricity demand and economic growth. Thus, it suggests that the limited number of studies in electricity demand forecasting by both academicians and professionals in this field might have partially contributed to the power shortages facing the nation, which in 2015 resulted in “Domsur”.
Used evaluation metrics
The performance of every forecasting model is examined based on the difference in error between the actual value \(\left( y \right)\) and the predicted value ( \(\hat{y}\) ). Several of these metrics were identified in the literature. However, we examined the most used in electrical energy demand forecasting to enable new beginners in the field of electricity demand forecasting to have a firm grip on which to apply in their study. Figure 3 shows the top four (4) most used error metrics in two or more studies. The results revealed that root-mean-square error RMSE (38%) was the most used error metric among EED forecasters, followed by mean absolute percentage error MAPE (35%). Due to the effectiveness in measure predictive model performance and their usefulness for short-term prediction, it was also observed that the MAPE is a standard primary metric as it is easy to both calculate and understand. The results affirm the findings in [ 12 , 19 , 22 ] that MAE, MAPE, and RMSE are the most commonly used evaluation metrics in EED forecasting model.
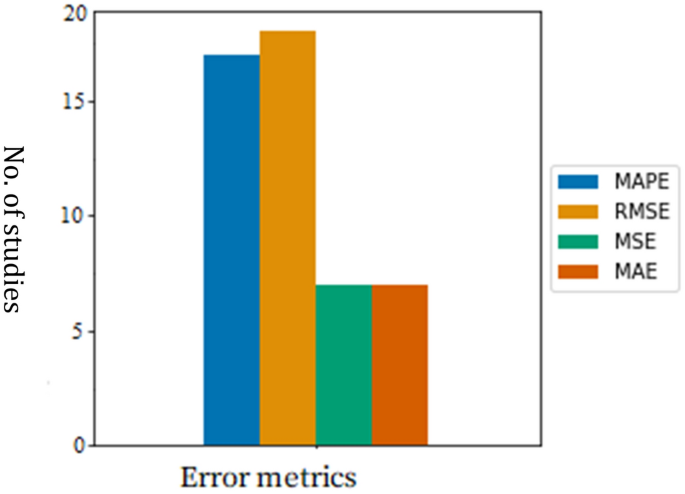
Most used error metrics in electricity load forecasting
Forecast type
Based on short-term load forecasting (STLF), medium-term load forecasting (MTLF), and long-term load forecasting (LTLF), it was observed that 80%, 15%, and 5% of the electrical energy demand forecasting were STLF, MTLF, and LTLF, respectively. The massive number of studies is based on STLF as compared with LTLF and MTLF call for further studies into the challenges associated with LTLF and MTLF electricity load forecasting. Again, 80% STLF forecasting affirms the 38% use of RMSE error metric, since it is for STLF forecasting. The results affirm the report in [ 22 ] that 43.6% of electricity forecasting are short-term prediction.
Model input parameters
The efficiency of every predictive model is believed to be partially dependent on the independent (input) variable [ 7 ]. At this level, the focus was to examine the different input variables used for electricity load forecasting. The type of independent variables (input features) used by electricity load forecasters was also examined. The current study observed that sixty (60) out of the sixty-seven (67) papers made known the input parameter to the proposed model. Table 2 presents the type of input variables and the percentage of studies that utilised it. It was observed that a high percentage (50%) of electricity demand forecasting was based on weather parameters; next was the historical electricity consumption pattern. The outcome exposed that little attention is given to household lifestyle in electricity demand forecasting. However, Nishida et al. [ 58 ] argue that residential (domestic) energy consumption differs depending on the lifestyle of the family. Family lifestyle, according to [ 56 ], cannot be undermined in electrical load forecasting. According to these studies, these factors include the life stage family composition, house type, age, home appliances possessed and their usage, family income, cultural background, social life, and lifestyle habits, which include how long to stay at home and how to spend holidays. The observations open the opportunity for further studies on the association between EED and household lifestyle.
Conclusions
The current study sought to reviewed state-of-the-art literature on electricity load forecasting to identify the challenges and opportunities for future studies. The outcome of the study revealed that electricity load forecasting is seen to be complicated for both engineers and academician and is still an ongoing area of research. The key findings are summarised as follows.
Several studies (90%) have applied AI in electrical energy demand forecasting as compared with traditional engineering and statistical method (10%) to address energy prediction problems; however, there are not enough studies benchmarking the performance of these methods.
There are few studies on EED forecasting in Africa countries (12 out of 67). Though the continent has progressive achievement in the creation of Regional Power Pools (PPP) over the last two decades, the continent still suffers from a lousy power network in most of its countries, leaving millions of people in Africa without electricity.
Temperature and rainfall as an input parameter to the EED forecasting model are seen to have a divergent view. At the same time, some sections of research recorded an improvement in accuracy and reported no improvement in accuracy when introduced and input. However, the current study attributes this to the difference in automorphic temperature globally and the different economic status among countries. An additional investigation will bring more clarity to the literature.
This study revealed that EED forecasting in the residential sector had seen little attention. On the other hand, Guo et al. [ 78 ] argue that the basic unit of electricity consumption is home.
It was observed that there had been a global increase in residential electricity demand, this according to the report in [ 49 ] can be attributed to the growing rate of buying electrical equipment and appliances of low quality due to higher living standards. However, a further probe into Soares et al. [ 49 ] assertion will bring clarity to literature because of the discrepancy in opinions in literature.
Lastly, the study revealed that there is a limited number of studies on load forecasting studies in Ghana. We, therefore, recommend rigorous researchers in this field in the country to enhance the economic growth of the country.
Our future study will focus on identifying the relationship between household lifestyle factors and electricity consumption in Ghana and predict load consumption based on identified factors since it is an area that has seen little or no attention in Ghana.
Availability of data and materials
All data generated or analysed during this study are included in this published article.
Abbreviations
- Electrical energy demand
- Artificial intelligence
Artificial neural network
Root-mean-square error
Mean absolute percentage error
Very short-term load forecasting
Short-term load forecasting
Medium-term load forecasting
Long-term load forecasting
Preferred-reporting items for systematic-review and meta-analysis
Electricity prediction
Energy forecasting
- Machine learning
Support vector machine
Random forest
k-nearest neighbours
Neural networks
Decision trees
Autoregressive integrated moving average
Particle swarm optimisation
Differential evolution
Curve fitting algorithm
Generalised linear model
Long short-term memory
Genetic algorithm
Mean absolute error
Convolutional neural network
Fuzzy logic
Adaptive neuro-fuzzy inference system
Generalised regression neural network
Taiwan stock exchange capitalisation-weighted stock index
Gross domestic product
Coefficient of variance
Self-organising maps
Autoregressive distributed lag
Long-range energy alternative planning
Quantile regression
Aung SS (2015) Electric power is the main driving force for industrialization. http://www.globalnewlightofmyanmar.com/electric-power-is-the-main-driving-force-for-industrialization/ . Accessed 2 Apr 2018
Dedinec A, Filiposka S, Dedinec A, Kocarev L (2016) Deep belief network based electricity load forecasting: an analysis of Macedonian case. Energy 115:1688–1700. https://doi.org/10.1016/j.energy.2016.07.090
Article Google Scholar
Hussain A, Rahman M, Memon JA (2016) Forecasting electricity consumption in Pakistan: the way forward. Energy Policy 90:73–80. https://doi.org/10.1016/j.enpol.2015.11.028
Jevgenijs S, Joeri deW, Kochnakyan A, Vivien F (2017) Forecasting electricity demand: an aid for practitioners. http://www.worldbank.org/energy/livewire . Accessed 15 Jun 2019
Zaman MU, Islam A, Sultana N (2018) Short term load forecasting based on internet of things (IoT). BRAC University, Dhaka
Google Scholar
Kumar CHJ, Veerakumari M (2012) Load forecasting of Andhra Pradesh grid using PSO, DE algorithms. Int J Adv Res Comput Eng Technol 1:179–184
Nti IK, Asafo-Adjei S, Agyemang M (2019) Predicting monthly electricity demand using soft-computing technique. Int Res J Eng Technol 06:1967–1973
Eeeguide.com (2014) Forecasting methodology. http://www.eeeguide.com/forecasting-methodology/ . Accessed 4 Jan 2019
Bouktif S, Fiaz A, Ouni A, Serhani MA (2018) Optimal deep learning LSTM model for electric load forecasting using feature selection and genetic algorithm: comparison with machine learning approaches †. Energies (MDPI) 11:1–20. https://doi.org/10.3390/en11071636
Hammad MA, Jereb B, Rosi B, Dragan D (2020) Methods and models for electric load forecasting: a comprehensive review. Logist Sustain Transp 11:51–76. https://doi.org/10.2478/jlst-2020-0004
Khwaja AS, Anpalagan A, Naeem M, Venkatesh B (2020) Joint bagged-boosted artificial neural networks: using ensemble machine learning to improve short-term electricity load forecasting. Electr Power Syst Res 179:106080. https://doi.org/10.1016/j.epsr.2019.106080
Hong T, Fan S (2016) Probabilistic electric load forecasting: a tutorial review. Int J Forecast 32:914–938. https://doi.org/10.1016/j.ijforecast.2015.11.011
Selvaraj KR, Sundararaj S, Ravi T (2013) Artificial neutral network based load forecasting and economic dispatch with particle swarm optimization. Int J Sci Eng Res 4:139–145
Stavast P (2014) Prediction of energy consumption using historical data and twitter. The University of Groningen, Groningen
Ouedraogo NS (2017) Modeling sustainable long-term electricity supply-demand in Africa. Appl Energy 190:1047–1067. https://doi.org/10.1016/j.apenergy.2016.12.162
Moher D, Shamseer L, Clarke M, Ghersi D, Liberati A, Petticrew M, Shekelle P, Stewart LA, Group P-P (2015) Preferred reporting items for systematic review and and explanation meta-analysis protocols (PRISMA-P) 2015: elaboration. Syst Rev 4:1–25
Zhou K, Yang S, Shen C (2013) A review of electric load classification in smart grid environment. Renew Sustain Energy Rev 24:103–110. https://doi.org/10.1016/j.rser.2013.03.023
Gonzalez-Briones A, Hernandez G, Corchado JM, Omatu S, Mohamad MS (2019) Machine learning models for electricity consumption forecasting: a review. In: 2019 2nd international conference on computer applications and information security, IEEE, pp 1–6. https://doi.org/10.1109/cais.2019.8769508
Wang Z, Li J, Zhu S, Zhao J, Deng S, Zhong S, Yin H, Li H, Qi Y, Gan Z (2019) A review of load forecasting of the distributed energy system. IOP Conf Ser Earth Environ Sci 237:042019. https://doi.org/10.1088/1755-1315/237/4/042019
Mele E (2019) A review of machine learning algorithms used for load forecasting at microgrid level. In: Sinteza 2019-International scientific conference on information technology and data related research, Singidunum University, pp 452–458. https://doi.org/10.15308/sinteza-2019-452-458
Shah RB (2019) A technological literature review on load forecasting in power system using artificial intelligence. Paripex-Indian J Res 8:14–15
Kuster C, Rezgui Y, Mourshed M (2017) Electrical load forecasting models: a critical systematic review. Sustain Cities Soc 35:257–270. https://doi.org/10.1016/j.scs.2017.08.009
Panda SK, Mohanty SN, Jagadev AK (2017) Long term electrical load forecasting: an empirical study across techniques and domains. Indian J Sci Technol 10:1–16
Jacob M, Neves C, Vukadinović-Greetham D (2020) Short term load forecasting. Forecast Assess Risk Individ Electr Peaks Math Planet Earth. https://doi.org/10.1007/978-3-030-28669-9_2
Article MATH Google Scholar
Appiahene P, Ussiph N, Missah YM (2018) Information technology impact on productivity. In: International conference on applied science and technology, Kumasi Technical University, Kumasi, Ghana, pp 122–133
Moher D, Liberati A, Tetzlaff J, Altman DG (2009) PRISMA Group, preferred reporting items for systematic reviews and meta-analyses: the PRISMA statement. Ann Intern Med 151:264–269. https://doi.org/10.7326/0003-4819-151-4-200908180-00135
Electricchoice.com (2016) Residential electricity for houses, apartments, condos, trailers, etc. https://www.electricchoice.com/residential-electricity . Accessed 10 Aug 2019
Kouroupetroglou P-N (2017) Machine learning techniques for short-term electric load forecasting. Aristotle University of Thessaloniki, Thessaloniki
Zakarya S, Abbas H, Belal M (2017) Long-term deep learning load forecasting based on social and economic factors in the Kuwait region. J Theor Appl Inf Technol 95:1524–1535
Soni A, Sharma AK (2013) Electricity load forecast for power system planning. Int Ref J Eng Sci 2:52–57
Angamuthu CR, Mukherjee A, Campion M, Salehfar H, Hansen T, Lin J, Ranganathan P (2018) A multi-stage price forecasting model for day-ahead electricity markets. Forecasting 1:3. https://doi.org/10.3390/forecast1010003
Lee CW, Lin BY (2016) Application of hybrid quantum tabu search with support vector regression (SVR) for load forecasting. Energies 9:1–16. https://doi.org/10.3390/en9110873
Hong WC, Fan GF (2019) Hybrid empirical mode decomposition with support vector regression model for short term load forecasting. Energies 12:1–16. https://doi.org/10.3390/en12061093
Sulandari W, Subanar MH, Lee PC (2020) Rodrigues, Indonesian electricity load forecasting using singular spectrum analysis, fuzzy systems and neural networks. Energy 190:116408. https://doi.org/10.1016/j.energy.2019.116408
Nepal B, Yamaha M, Yokoe A, Yamaji T (2020) Electricity load forecasting using clustering and ARIMA model for energy management in buildings. Jpn Archit Rev 3:62–76. https://doi.org/10.1002/2475-8876.12135
Ghore S, Goswami A (2015) Short term load forecasting of Chhattisgarh grid using artificial neural network. Int J Eng Dev Res 3:391–397
Kuhba H, Al-tamemi HAH (2016) Power system short-term load forecasting using artificial neural networks. Int J Eng Dev Res 4:78–87
Zahid M, Ahmed F, Javaid N, Abbasi R, Zainab KH, Javaid A, Bilal M, Akbar M, Ilahi M (2019) Electricity price and load forecasting using enhanced convolutional neural network and enhanced support vector regression in smart grids. Electronics 8:122. https://doi.org/10.3390/electronics8020122
Nwulu NI, Agboola OP (2012) Modelling and predicting electricity consumption using artificial neural networks. In: 2012 11th international conference on environmental and electrical engineering, pp 1059–1063
Young TC, Raya H, Youngdeok H, Young ML, (2016) Artificial neural network model for forecasting sub-hourly electricity usage in commercial buildings. Energy Buildings 111:184–194. https://doi.org/10.1016/j.enbuild.2015.11.045
Aydodu G, Yildiz O (2017) Forecasting the annual electricity consumption of Turkey using a hybrid model. In: 2017 25th signal processing and communication application conference, Antalya, Turkey, pp 1–4. https://doi.org/10.1109/SIU.2017.7960283
Ahmad W, Ayub N, Ali T, Irfan M, Awais M, Shiraz M, Glowacz A (2020) Towards short term electricity load forecasting using improved support vector machine and extreme learning machine. Energies 13:1–17. https://doi.org/10.3390/en13112907
Atef S, Eltawil AB (2020) Assessment of stacked unidirectional and bidirectional long short-term memory networks for electricity load forecasting. Electr Power Syst Res 187:106489. https://doi.org/10.1016/j.epsr.2020.106489
Azar AT, Khamis A, Kamal NA, Galli B (2020) Short term electricity load forecasting through machine learning. In: Hassanien A, Azar A, Gaber T, Oliva D, Tolba F (eds) International conference on artificial intelligence computer vision (AICV2020). AICV 2020. Advances in intelligent systems and computing, vol 1153, Springer, Cham. https://link.springer.com/chapter/10.1007/978-3-030-44289-7_40 . Accessed 5 July 2020
Konica JA, Hanelli L (2016) Forecasting next-day the electricity demand based on fuzzy logic method case for. J Multidiscip Eng Sci Technol 3:6172–6180
Motepe S, Hassan AN, Stopforth R (2019) South African distribution networks load forecasting using ANFIS. In: Proceedings of 2018 IEEE international conference power electronic drives energy system PEDES 2018, IEEE, Chennai, India, pp 1–6. https://doi.org/10.1109/pedes.2018.8707876
Lin CT, Der Chou L (2013) A novel economy reflecting short-term load forecasting approach. Energy Convers Manag 65:331–342. https://doi.org/10.1016/j.enconman.2012.08.001
Leahy E, Lyons S (2010) Energy use and appliance ownership in Ireland. Energy Policy 38:4265–4279. https://doi.org/10.1016/j.enpol.2010.03.056
Soares A, Gomes Á, Antunes CH (2014) Categorisation of residential electricity consumption as a basis for the assessment of the impacts of demand response actions. Renew Sustain Energy Rev 30:490–503. https://doi.org/10.1016/j.rser.2013.10.019
Hedén W (2016) Predicting hourly residential energy consumption using random forest and support vector regression an analysis of the impact of household clustering on the performance accuracy. KTH Royal Institute of Technology, Stockholm
Kong W, Dong ZY, Jia Y, Hill DJ, Xu Y, Zhang Y (2019) Short-term residential load forecasting based on LSTM recurrent neural network. IEEE Trans Smart Grid 10:841–851. https://doi.org/10.1109/TSG.2017.2753802
Kong W, Dong ZY, Hill DJ, Luo F, Xu Y (2018) Short-term residential load forecasting based on resident behaviour learning. IEEE Trans Power Syst 33:2016–2017. https://doi.org/10.1109/TPWRS.2017.2688178
Kontokosta CE, Tull C (2017) A data-driven predictive model of city-scale energy use in buildings. Appl Energy 197:303–317. https://doi.org/10.1016/j.apenergy.2017.04.005
McLoughlin F, Duffy A, Conlon M (2015) A clustering approach to domestic electricity load profile characterisation using smart metering data. Appl Energy 141:190–199. https://doi.org/10.1016/j.apenergy.2014.12.039
Mcloughlin F, Duffy A, Conlon M (2012) Characterising domestic electricity consumption patterns by dwelling and occupant socio-economic variables: an Irish case study. Energy Build 48:240–248. https://doi.org/10.1016/j.enbuild.2012.01.037
Veit A, Goebel C, Tidke R, Doblander C, Jacobsen H (2014) Household electricity demand forecasting-benchmarking state-of-the-art methods. arXiv:1603.00751 , pp 1–10
Biswas MAR, Robinson MD, Fumo N (2016) Prediction of residential building energy consumption: a neural network approach. Energy 117:84–92. https://doi.org/10.1016/j.energy.2016.10.066
Nishida K, Takeda A, Iwata S, Kiho M, Nakayama I (2017) Household energy consumption prediction by feature selection of lifestyle data. In: International conference on smart grid communication, Dresden, Germany, pp 235–240
Olaniyan K, Mclellan BC (2018) Estimating residential electricity consumption in Nigeria to support energy transitions. Sustain 10:1–22. https://doi.org/10.3390/su10051440
Bouznit M, Pablo-Romero MP (2018) Residential electricity consumption and economic growth in Algeria. Energies (MDPI). https://doi.org/10.3390/en11071656
Bogomolov A, Lepri B, Larcher R, Antonelli F, Pianesi F, Pentland A (2016) Energy consumption prediction using people dynamics derived from cellular network data. EPJ Data Sci. https://doi.org/10.1140/epjds/s13688-016-0075-3
Huber P, Gerber M, Rumsch A, Paice A (2018) Prediction of domestic appliances usage based on electrical consumption. Energy Inform 1:265–428
Jain PK, Quamer W, Pamula R (2018) Electricity consumption forecasting using time series analysis. In: Singh M, Gupta P, Tyagi V, Flusser J, Ören T (eds), Advanced computer data science ICACDS 2018. Communications in computer and information science, Springer, Singapore, Singapore. https://doi.org/10.1007/978-981-13-1813-9_33
Rehman USA, Cai Y, Fazal R, Das Walasai G, Mirjat NH (2017) An integrated modeling approach for forecasting long-term energy demand in Pakistan. Energies 10:1–23. https://doi.org/10.3390/en10111868
Liang Y, Niu D, Cao Y, Hong WC (2016) Analysis and modeling for China’s electricity demand forecasting using a hybrid method based on multiple regression and extreme learning machine: a view from carbon emission. Energies 9:1–22. https://doi.org/10.3390/en9110941
Mokilane P, Galpin J, Yadavalli VSS, Debba P, Koen R, Sibiya S (2018) Density forecasting for long-term electricity demand in South Africa using quantile regression. S Afr J Econ Manag Sci 21:1–14. https://doi.org/10.4102/sajems.v21i1.1757
Sarkodie SA (2017) Estimating Ghana’s electricity consumption by 2030: an ARIMA forecast, energy sources. Part B Econ Plan Policy 12:936–944. https://doi.org/10.1080/15567249.2017.1327993
Energy Commission, Energy Commission, Ghana 2018 Energy (Supply and Demand) Outlook for Ghana, Accra, 2018. http://www.energycom.gov.gh/planning/data-center/energy-outlook-for-ghana . Accessed 1 Feb 2020
Sigauke C (2017) Forecasting medium-term electricity demand in a South African electric power supply system. J Energy S Afr. https://doi.org/10.17159/2413-3051/2017/v28i4a2428
Asumadu-Sarkodie S, Owusu PA (2016) Forecasting Nigeria’s energy use by 2030, an econometric approach, Energy Sources. Part B Econ Plan Policy 11:990–997. https://doi.org/10.1080/15567249.2016.1217287
Adeoye O, Spataru C (2019) Modelling and forecasting hourly electricity demand in West African countries. Appl Energy 242:311–333. https://doi.org/10.1016/j.apenergy.2019.03.057
Sigauke C, Chikobvu D (2016) Peak electricity demand forecasting using time series regression models: an application to South African data. J Stat Manag Syst 19:567–586. https://doi.org/10.1080/09720510.2015.1086146
Ozturk S, Ozturk F (2018) Prediction of energy consumption of Turkey on sectoral bases by Arima model. Energy Econ Lett 5:23–30. https://doi.org/10.18488/journal.82.2018.51.23.30
Lebotsa ME, Sigauke C, Bere A, Fildes R, Boylan JE (2018) Short term electricity demand forecasting using partially linear additive quantile regression with an application to the unit commitment problem. Appl Energy 222:104–118. https://doi.org/10.1016/j.apenergy.2018.03.155
Caro E, Juan J, Cara J (2020) Periodically correlated models for short-term electricity load forecasting. Appl Math Comput 364:124642. https://doi.org/10.1016/j.amc.2019.124642
Article MathSciNet MATH Google Scholar
Kong X, Li C, Wang C, Zhang Y, Zhang J (2020) Short-term electrical load forecasting based on error correction using dynamic mode decomposition. Appl Energy 261:114368. https://doi.org/10.1016/j.apenergy.2019.114368
Auverlot D, Beeker É, Hossie G, Oriol L, Rigard-Cerison A (2014) The crisis of the european electricity system: diagnosis and possible ways forward. https://www.strategie.gouv.fr/sites/strategie.gouv.fr/files/archives/CGSP_Report_European_Electricity_System_030220141.pdf . Accessed 18 Apr 2019
Guo Z, Zhou K, Zhang C, Lu X, Chen W, Yang S (2018) Residential electricity consumption behavior: influencing factors, related theories and intervention strategies. Renew Sustain Energy Rev 81:399–412. https://doi.org/10.1016/j.rser.2017.07.046
Download references
Acknowledgements
Not applicable.
The authors declare that they did not receive any funding for this research.
Author information
Authors and affiliations.
Department of Computer Science, Sunyani Technical University, Box 206, Sunyani, Ghana
Isaac Kofi Nti
Department of Electrical and Electronic Engineering, Sunyani Technical University, Sunyani, Ghana
Moses Teimeh
Department of Computer Science and Informatics, University of Energy and Natural Resources, Sunyani, Ghana
Owusu Nyarko-Boateng & Adebayo Felix Adekoya
You can also search for this author in PubMed Google Scholar
Contributions
We, at this moment, declare that IKN and MT contributed to the design and implementation of the research. MT, IKN ON-B and AFA contributed to the analysis of the results and the writing of the manuscript. The manuscript has been read and approved by all named authors, and there are no other persons who satisfied the criteria for authorship but are not listed. All authors read and approved the final manuscript.
Corresponding author
Correspondence to Isaac Kofi Nti .
Ethics declarations
Competing interests.
The authors of the current study declare that they have no competing interests.
Additional information
Publisher's note.
Springer Nature remains neutral with regard to jurisdictional claims in published maps and institutional affiliations.
Rights and permissions
Open Access This article is licensed under a Creative Commons Attribution 4.0 International License, which permits use, sharing, adaptation, distribution and reproduction in any medium or format, as long as you give appropriate credit to the original author(s) and the source, provide a link to the Creative Commons licence, and indicate if changes were made. The images or other third party material in this article are included in the article's Creative Commons licence, unless indicated otherwise in a credit line to the material. If material is not included in the article's Creative Commons licence and your intended use is not permitted by statutory regulation or exceeds the permitted use, you will need to obtain permission directly from the copyright holder. To view a copy of this licence, visit http://creativecommons.org/licenses/by/4.0/ .
Reprints and permissions
About this article
Cite this article.
Nti, I.K., Teimeh, M., Nyarko-Boateng, O. et al. Electricity load forecasting: a systematic review. Journal of Electrical Systems and Inf Technol 7 , 13 (2020). https://doi.org/10.1186/s43067-020-00021-8
Download citation
Received : 06 April 2020
Accepted : 30 August 2020
Published : 09 September 2020
DOI : https://doi.org/10.1186/s43067-020-00021-8
Share this article
Anyone you share the following link with will be able to read this content:
Sorry, a shareable link is not currently available for this article.
Provided by the Springer Nature SharedIt content-sharing initiative
- Electricity load forecasting
Academia.edu no longer supports Internet Explorer.
To browse Academia.edu and the wider internet faster and more securely, please take a few seconds to upgrade your browser .
- We're Hiring!
- Help Center
Electricity
- Most Cited Papers
- Most Downloaded Papers
- Newest Papers
- Save to Library
- Last »
- Phisics Follow Following
- Home Power Follow Following
- Alternative Energy Follow Following
- Ingenieria y ciencias de los Materiales Follow Following
- Digital Electronics Follow Following
- Solar Energy Follow Following
- Wind Energy Follow Following
- Electricidad Follow Following
- Renewable Energy Follow Following
- Energy Follow Following
Enter the email address you signed up with and we'll email you a reset link.
- Academia.edu Publishing
- We're Hiring!
- Help Center
- Find new research papers in:
- Health Sciences
- Earth Sciences
- Cognitive Science
- Mathematics
- Computer Science
- Academia ©2024
wn1sdwk000IN4
- Conflicts of interest: the cost of investing in the energy transition in a high interest-rate era
Conflicts of interest
The cost of investing in the energy transition in a high interest-rate era
Peter Martin, Head of Economics, Macroeconomics
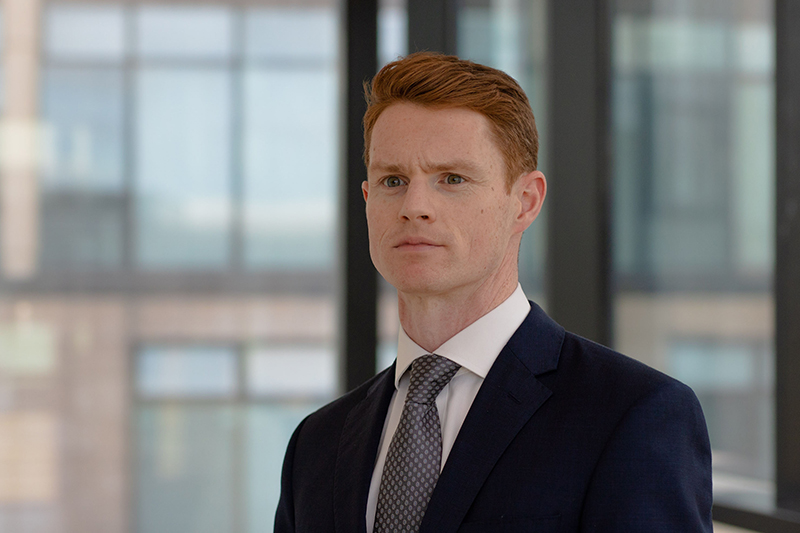
Join the debate. Contact Peter.
Peter Martin
Head of Economics, Macroeconomics
Peter joined Wood Mackenzie in 2010 to cover European energy markets and moved to the macroeconomics research team in 2011. He now leads the team responsible for producing our proprietary economic outlook to 2050, drawing on his experience in forecasting key metrics such as GDP, industrial production, FX and inflation.
Peter regularly develops macroeconomic scenarios and sensitivity analysis, including Brexit, banking crises, the Covid-19 pandemic and trade wars. He has also contributed to consulting projects, assessing the economic impact of energy and natural resources development. His special interests include the economics of energy transition and the fiscal stability of oil-producing economies in the Middle East and Africa.
Before joining Wood Mackenzie, Peter was an energy analyst at Iberdrola. In this role, he provided technical and fundamental analysis of European energy markets to the trading desk.
MA (Hons) Economics, the University of Glasgow
MSc Economic Management and Policy, Strathclyde Business School
James Whiteside, Head of Corporate, Metals & Mining
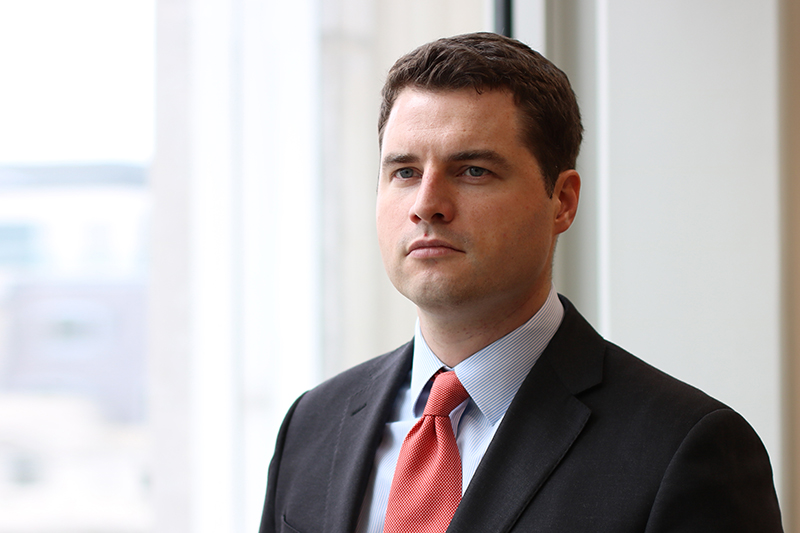
Join the debate. Contact James.
James Whiteside
Head of Corporate, Metals & Mining
James leads the development of corporate coverage for metals and mining. His current focus is on providing data-driven analysis of companies’ positioning for the energy transition and implications for valuation. He is based in London.
In previous leadership roles at Wood Mackenzie, James set the metals and mining product strategy. He led cross-commodity research initiatives and the development of new products including our metals and mining Emissions Benchmarking Tool.
James joined Wood Mackenzie in 2015 as a consultant. He has 15 years of experience in the metals and mining industry, spanning commercial, technical and financial roles. As a consultant, he managed several major transaction advisory engagements and was instrumental in developing our battery raw materials offerings.
MSc, Metals and Energy Finance, Imperial College London
MEng, Civil Engineering, University of Leeds
Fraser McKay, Head of Upstream Analysis
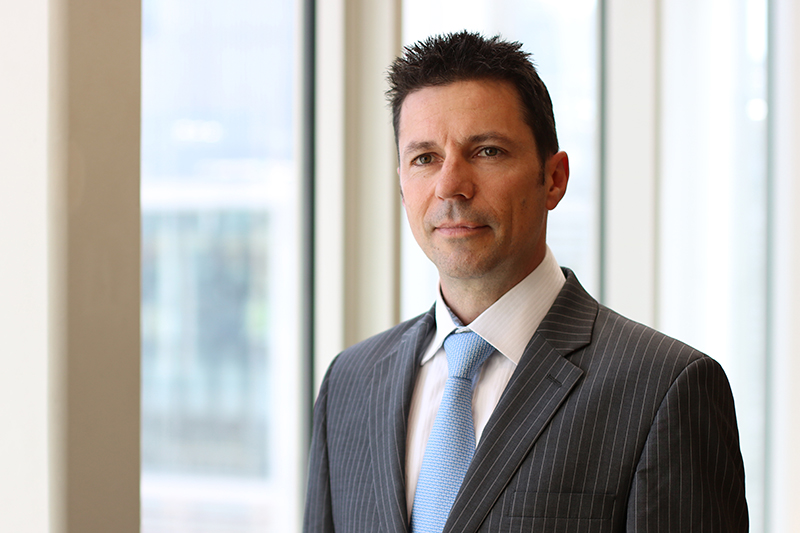
Join the debate. Contact Fraser.
Fraser McKay
Head of Upstream Analysis
As head of our Upstream Research team, Fraser is responsible for maximising the quality and impact of our analysis of key global upstream themes.
Fraser joined Wood Mackenzie in 2003, initially as an upstream analyst leading our offering in Canada and Alaska. Since then he has managed research teams focused on delivering in-depth, strategic corporate and upstream analysis and played a key role in building out our Americas upstream and corporate research capacity. Fraser has also played a key role in the development of a suite of products, including our M&A Service, Corporate Benchmarking Tool and Mid-Cap Corporate Coverage.
In his senior roles, Fraser has managed and contributed to major strategic consulting projects, interacted with client executive teams and government entities at the most senior level, and presented upstream and corporate material to global audiences.
Prior to joining Wood Mackenzie, Fraser worked for Schlumberger in various field and managerial positions in the oilfield services sector.
Srinivasan Santhakumar, Senior Research Analyst, Offshore Wind
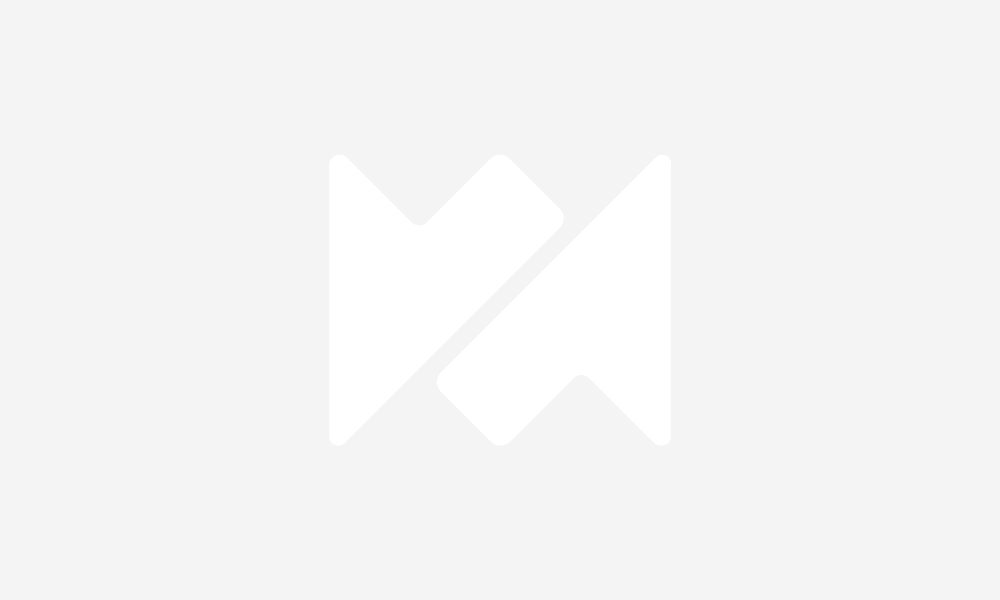
Join the debate. Contact Srinivasan .
Srinivasan Santhakumar
Senior Research Analyst, Offshore Wind
Srinivasan is a senior research analyst in our offshore wind team, involved in economic analysis with a particular focus on asset valuation, LCOE forecasts and market research. He also works on the cost modelling of offshore wind energy, analysing how technological advancements, performance and macroeconomic factors translate into cost impacts.
Srinivasan joined Wood Mackenzie in 2022 and is based in Amsterdam.
MSc, Sustainable Energy Engineering, KTH Royal Institute of Technology & Barcelona Tech
PhD, Offshore Renewable Energy Technologies, University of Groningen
The ‘zero era’ for interest rates has come to an end. In the past two years, rates have risen sharply as central banks have scrambled to fight inflationary pressures. Governments, companies and households face markedly higher market rates and bond yields, which could yet rise further. The increase in the cost of capital has profound implications for the energy and natural resources industries, particularly the cost and pace of the transition to low-carbon technologies.
The monetary environment over the next couple of decades is likely to remain much tighter than it was in the period from 2009 to 2022. In major economies, nominal and real interest rates could be as much as two percentage points higher, on average, than in the ‘zero era’. Companies, investors and policymakers should brace themselves: tougher financial conditions could persist for some time to come.
The higher cost of borrowing affects the energy and natural resources sectors unevenly. Highly capital intensive and often reliant on subsidies, low-carbon energy and nascent green technologies are most exposed. Debt accounts for a higher share of the capital structure for low-carbon energy sectors, too. The impact of higher interest rates grows as the capital expenditure (capex) share of total expenditure increases.
In contrast, the oil and gas industry, while also highly capital intensive, has far less exposure to the cost of debt, so is less affected by higher rates. The large metals and mining companies, with strong balance sheets, are also well positioned.
Transitioning to a net zero global economy is a monumental investment challenge. Meeting the challenge, already an outside bet, will have to happen against a less favourable monetary backdrop than the world has been used to since 2009.
Interest rates: higher for longer
Interest rates have normalised after the ‘zero era’, the period of loose monetary policy that followed the Great Recession. In 2023, policy rates in major developed economies hit their highest levels in decades after the most aggressive hiking cycle in 40 years. While inflation has fallen towards central banks’ targets of around 2%, rates may not come down as far or as quickly as markets anticipate.
Structural inflationary trends ‒ global trade reshuffling, deglobalisation and production onshoring ‒ are intensifying. Safeguarding the security of supply and protecting domestic industry and employment are being prioritised over economics. On the demand side, the energy transition will stimulate demand and, in some cases, even put upward pressure on inflation by shifting to higher-cost, low-carbon technologies.
To keep inflation averaging around 2%, therefore, higher nominal interest rates could persist. China is an exception, as its maturing economic development and lower growth are likely to translate into lower interest rates.
Figure 1. US and Eurozone real interest rates normalise
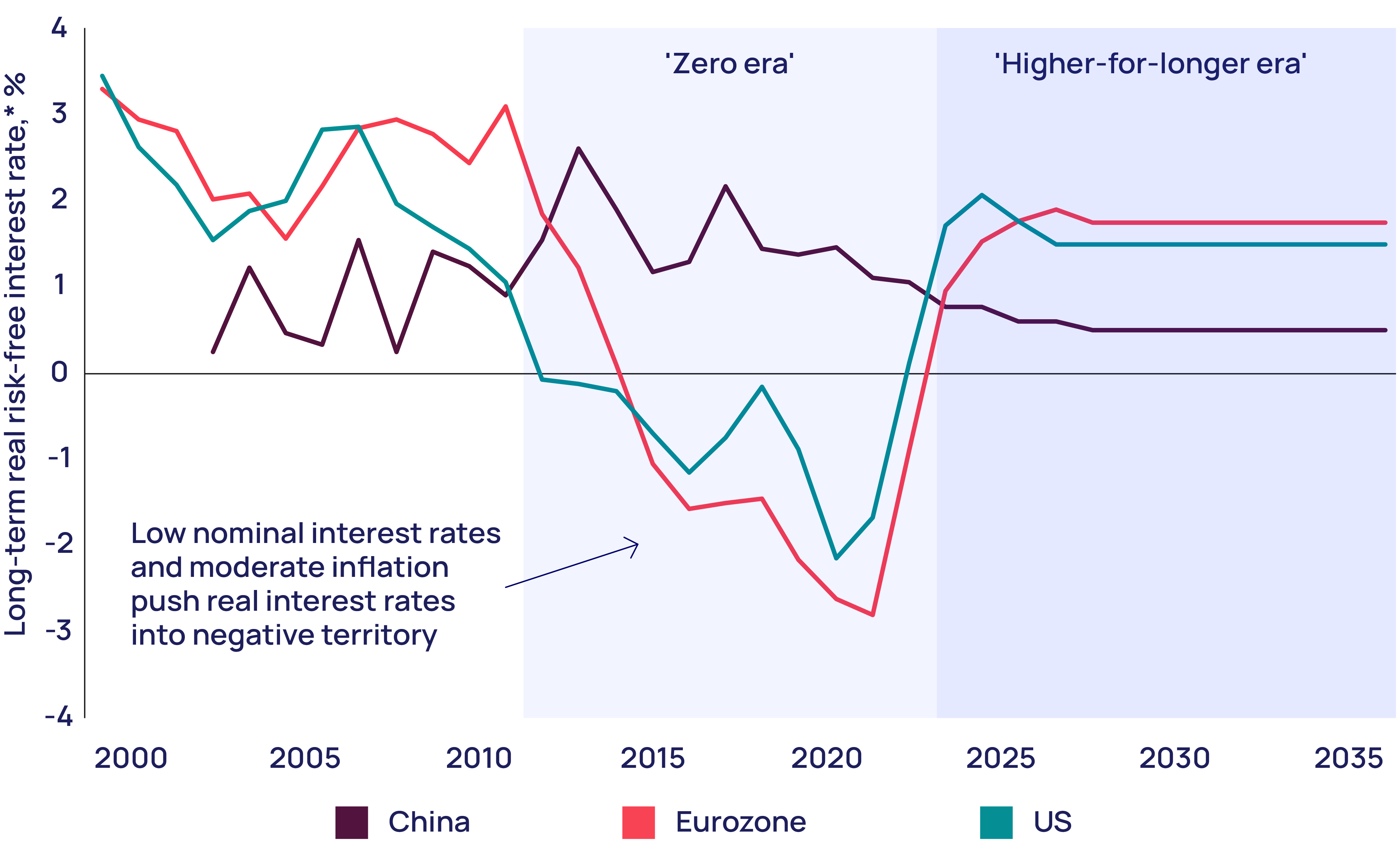
The energy transition to net zero could require US$75 trillion of investment by 2050. In a higher interest-rate scenario, achieving net zero will be even harder and more costly.
How do higher interest rates affect companies?
The higher cost of borrowing affects energy and natural resources sectors differently. Capital structure and balance-sheet resilience determine sensitivity to interest rates.
Thanks to their low gearing, many companies in the metals and mining and oil and gas sectors will be relatively unaffected by higher interest rates.
Gearing is higher for power and renewables firms. Debt from bonds and project finance, secured against long-term power purchasing agreements, has been used to fund rapid growth in renewables. Renewables and nuclear power, with their high capital intensity, are more exposed to interest rates . Project finance is less common in the metals and mining and oil and gas sectors, with the notable exceptions of liquefied natural gas (LNG) and midstream projects, where more stable revenues suit the financing model.
While power and renewables companies have higher gearing, they do compare favourably with other peer groups on a cost-of-debt basis. Mechanisms to reduce price and offtake risk enable power and renewables companies to obtain debt more cheaply than the relatively risky oil and gas and metals and mining sectors. The recent rise in interest rates, however, has a larger proportional impact on their cost of debt.
Figure 2. Cost of debt rising fastest for the highly geared power and renewables sector
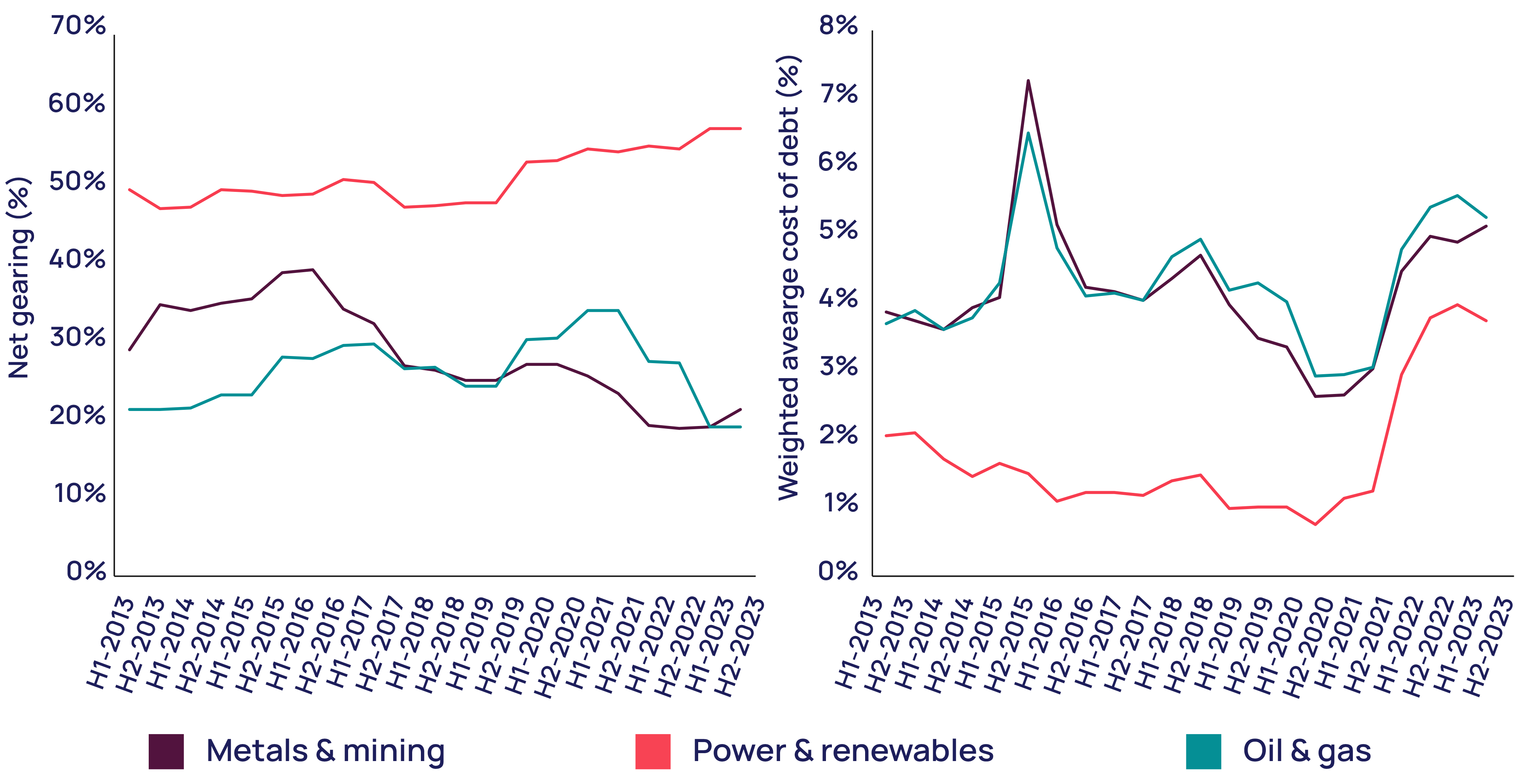
Renewables and green tech: feeling the squeeze
Higher interest rates disproportionately affect renewables and nuclear power. Their high capital intensity and low returns mean future projects will be at risk.
Renewable investments with subsidies and certainty on price and offtake can access cheaper finance, but the low cost of debt and low required returns are precisely what makes projects sensitive to interest rates. Higher interest rates affect required returns and the cost of capital more than other power generation projects that need higher returns in the first place.
In an illustrative example for the US, our analysis shows that a 2-percentage point increase in the risk-free interest rate pushes up the levelised cost of electricity (LCOE) by as much as 20% for renewables. The comparative increase in LCOE for a combined-cycle gas turbine plant is only 11%.
Figure 3: Renewables have highest capital intensity of US generation (New York, 2024)
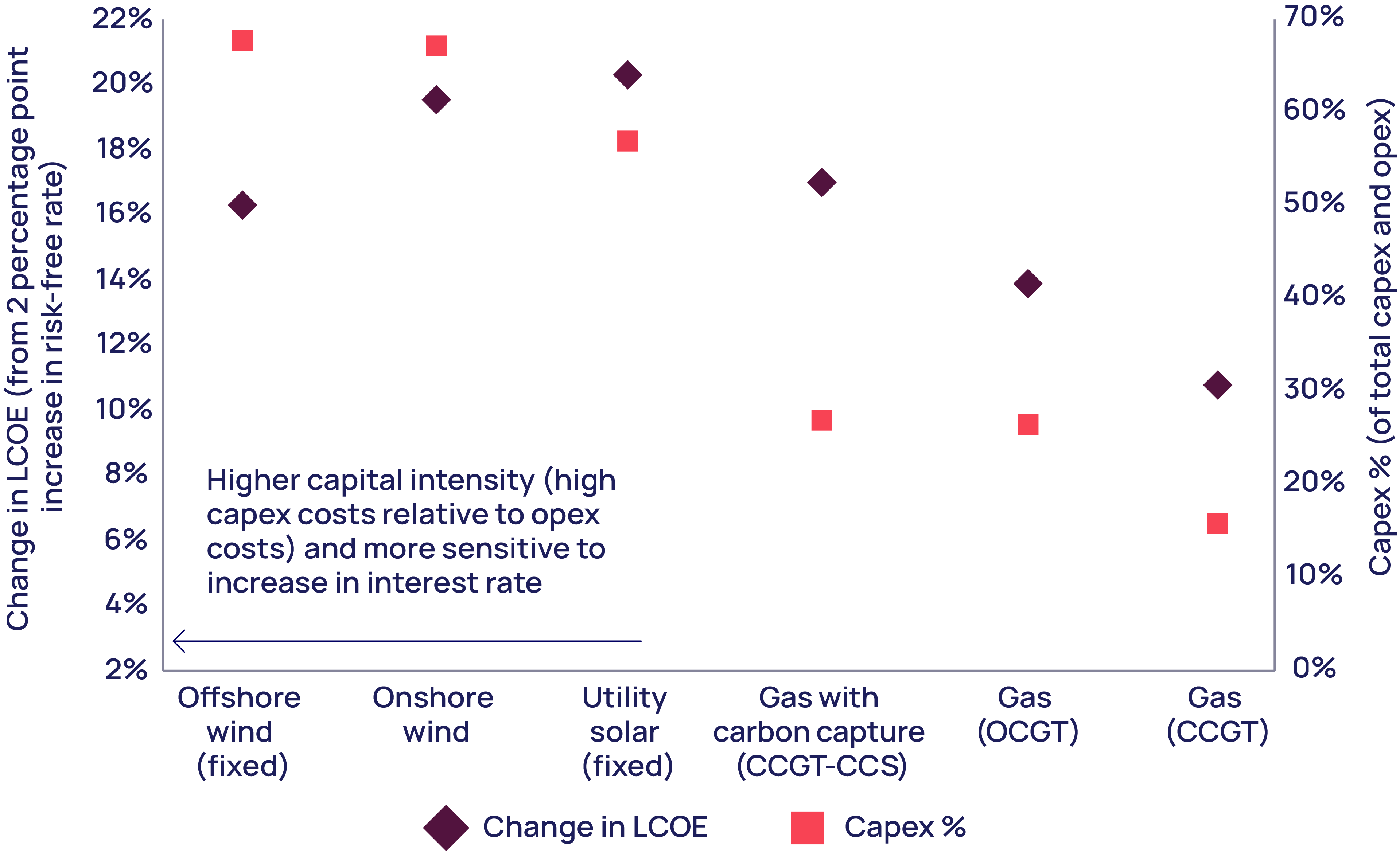
Assumptions: debt 55%, debt term 15 years
Definitions: levelised cost of electricity (lcoe), combined cycle gas turbine (ccgt), open cycle gas turbine (ocgt), carbon capture and storage (ccs), source: wood mackenzie.
The market structure matters, too. In the US and Australia, where renewables, including subsidies, must compete against the market price, higher interest rates will curb investment. In Europe, in contrast – where the mandate is to achieve decarbonisation targets and contracts for difference reduce price risk – investments are still likely to go ahead but result in higher prices.
We are seeing this play out. Offshore wind projects typically fix power purchasing agreements for 15 to 20 years ahead. Those projects that secured agreements three to four years ago are under pressure. Completed projects are booking impairments after razor-thin margins were squeezed by cost inflation, supply-chain constraints and the rising cost of capital. Some projects in development are being scrapped and some power contracts are being renegotiated.
How competitive are renewables? In many markets, onshore wind and solar have an economic advantage over hydrocarbon generation sources, even without subsidies in some cases. In the US, onshore wind can generate electricity at an LCOE of US$40/MWh, 50% of the cost of gas-fired generation. Higher interest rates, though, are eroding that advantage.
Figure 4. LCOE of power generation in the US (New York, 2024)
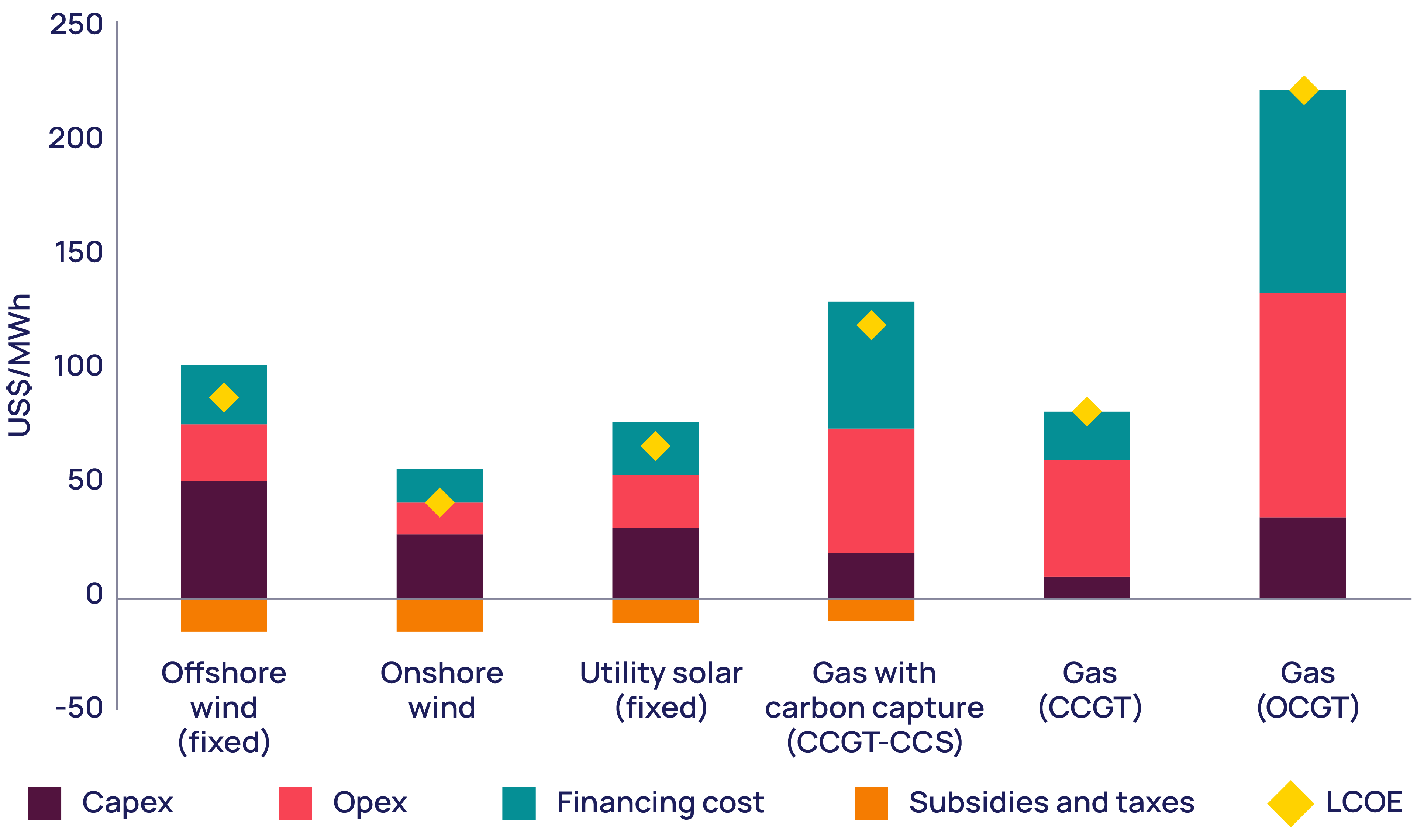
Definitions: Investment tax credit (ITC), levelised cost of electricity (LCOE), combined cycle gas turbine (CCGT), open cycle gas turbine (OCGT), carbon capture and storage (CCS)
Green tech under pressure.
Nascent technologies – low-carbon hydrogen, carbon capture, utilisation and storage (CCUS) and direct air capture (DAC) – will play an important role in the energy transition. However, they require major development and incentives to transform them into commercially viable, large-scale options for energy supply or decarbonising the economy.
With their remarkable levels of capital investment and high capital intensity, these projects are under threat amid higher interest rates. The capital intensity of hydrogen varies greatly by project, with capex ranging from 20% to 75% of total cost. At higher capital intensities, a 2-percentage point increase in interest rates lifts the levelised cost of hydrogen by around 10%.
The lack of economic incentives to capture carbon and the lack of a market for hydrogen are the most significant obstacles to investment in these sectors, but for projects that do progress, higher interest rates hurt the economics. This affects both smaller development companies that struggle to access debt and larger, credit-worthy emitters that rely on low-interest leverage to render projects attractive for shareholders.
Oil and gas: capital discipline puts the industry in a strong position
The oil and gas sector has less to fear in a tighter interest-rate environment. After record debt repayment in the last few years, the average balance sheet is healthy and gearing is low. Net debt for 25 of the largest international and national oil companies in Wood Mackenzie’s corporate coverage fell to US$150 billion in 2023 from US$390 billion in 2020. Gearing of 10% to 20% is already the ‘new normal’ for many and will be reinforced in a higher interest-rate era.
Figure 5: Oil and gas companies have cut debt sharply since 2020
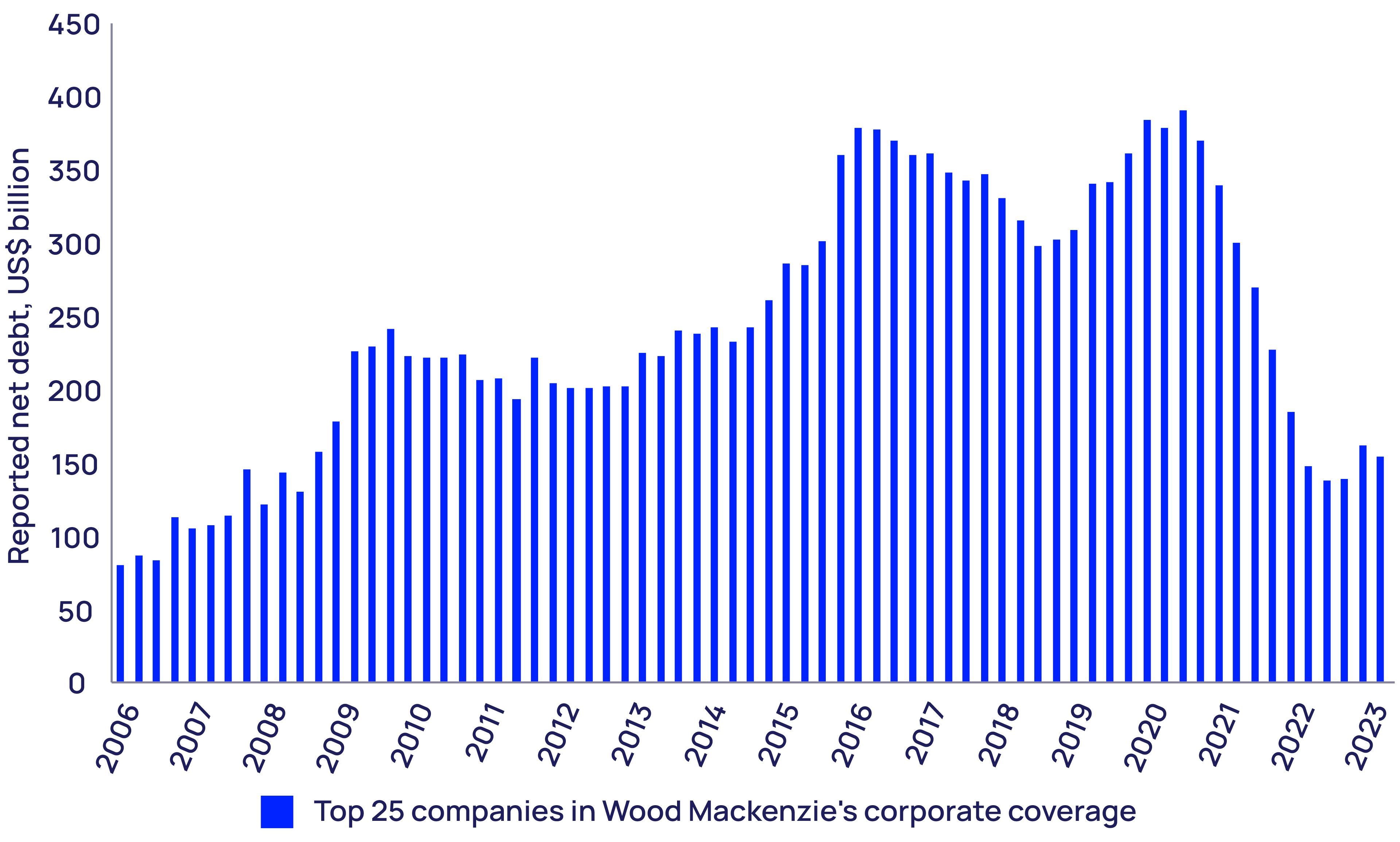
Our analysis shows that a 2-percentage point interest-rate increase has a similar effect on total corporate cash flow as a modest US$1/bbl change in the oil price. While the cost of capital is an ever-present consideration, interest rates are far from a primary concern. Rather, the availability of finance is a problem for small or financially stretched operators, with environmental, social and governance concerns contributing to an ever-shrinking list of lenders.
When it comes to decisions on capital allocation and project sanctioning, higher interest rates could impact investment sentiment. The cost of capital is baked into the 15%-plus return targets the industry’s biggest players expect from oil projects. The cost of equity is also a factor but, even in isolation, interest rates could impact investment models to a degree.
One area that embraces project financing and where rising interest rates have caused some concern is LNG. On a value basis, the sector accounts for 12% of the global oil and gas industry. Capital is raised with the project itself acting as collateral to access cheaper finance.
LNG projects already operating will have either partly or fully paid down or repackaged their project finance. New projects are more exposed. The burgeoning US LNG sector accounts for most of the new tolling capacity. Increasing development costs and higher interest rates have already caused a US$0.30-US$0.40/mmbtu increase in tolling fees ‒ around a 20% rise. A structural 2-percentage point increase in interest rates would permanently lock in more than half of that. These higher costs would mostly be passed through to the consumer.
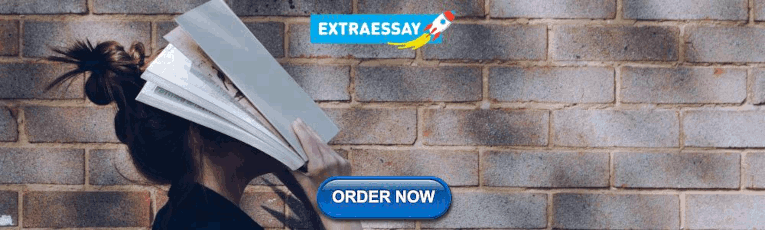
Metals and mining: accelerating a shift to growth?
Mining companies are running very low levels of debt and look well-positioned for a high-rate environment. High capital requirements are limiting new project sanctioning and hampering extraction growth, however.
For the mining Majors, we don’t think a higher-for-longer interest-rate environment will alter their approach. A focus on low-cost assets to protect margins, minimise earnings volatility and boost credit ratings is entrenched.
Things might start to get tricky for mining projects that require dedicated project finance. The scale of copper and aluminium projects presents a significant hurdle for independent developers. Debt interest payments will be higher, lowering the coverage ratio of projects and limiting the amount of borrowed capital available. This may force independents to consider alternative financing options or partner with the Majors to derisk project execution.
The mining industry faces a challenge in meeting metals demand in the energy transition. Capital intensity has reached a point where growing output is difficult. This is the conundrum for the mining industry.
By further suppressing output growth in the short term, higher rates may help underpin a shift to growth as the energy transition accelerates. Eventually as demand grows, price rises will kick in and support a shift to growth for the mining and metals sector.
How can policymakers offset the headwinds?
Governments need to subsidise the energy transition to encourage investment. But high interest rates put those subsidies at risk. With elevated debt and higher interest rates, governments’ debt servicing costs are increasing. This squeezes out other government spending and could restrict transition efforts by reducing supportive subsidies and tax incentives or cutting direct public capital investment in a low-carbon economy.
In the US, government expenditure on interest payments as a percentage of GDP has risen by 1.2 percentage points to 3.7% since the start of 2022; US$1 of every US$7 spent goes on interest. Budget trade-offs are a reality. The US Inflation Reduction Act could total US$1.8 trillion in subsidies to the power sector alone by 2050.
Figure 6. Elevated government debt in major economies
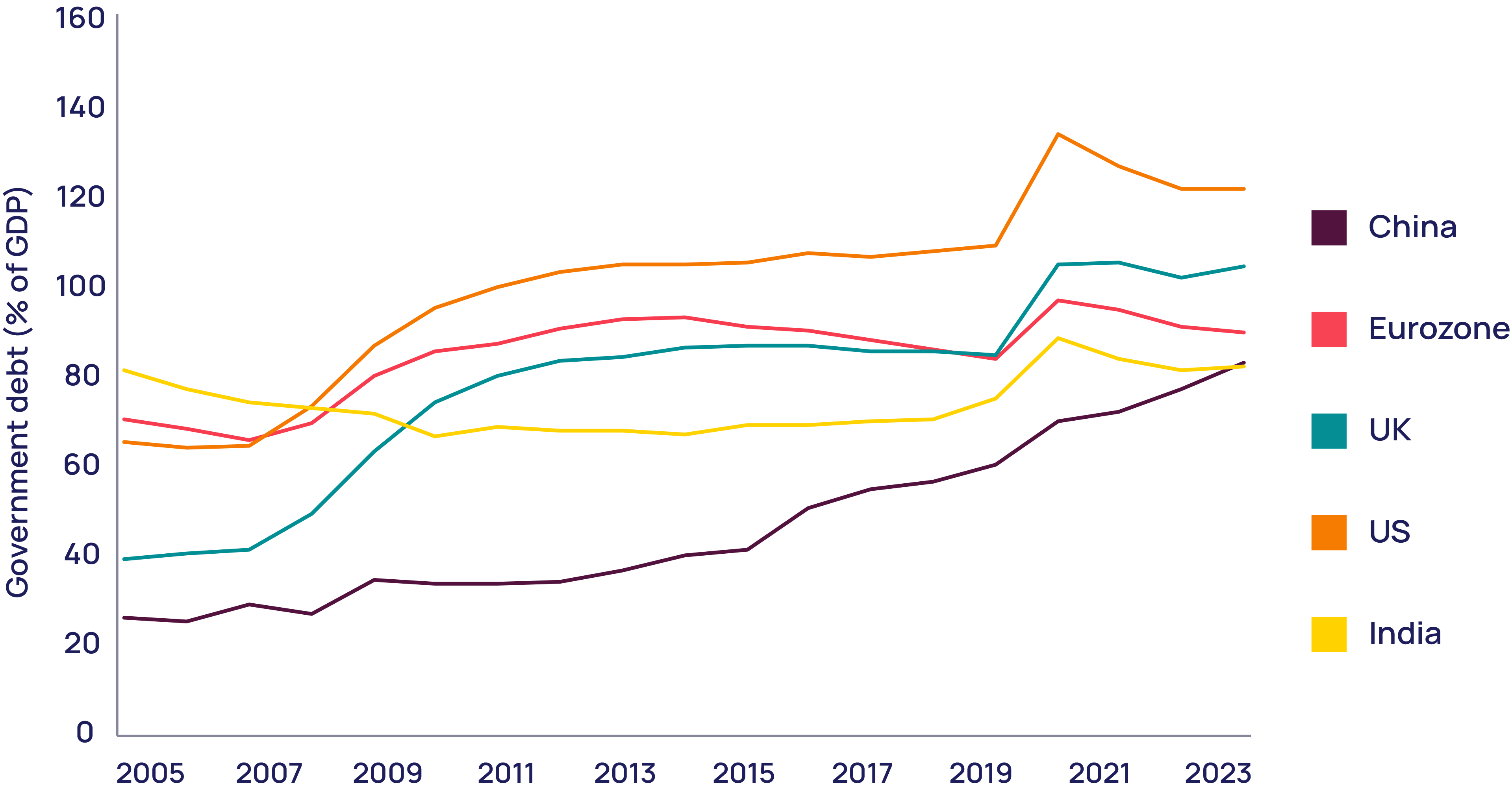
What’s playing out in China now may reflect the constraints other governments could soon experience. Public debt in China as a percentage of GDP has doubled over the past decade. The central government stopped subsidising new renewable power capacity in 2022, yet legacy subsidy payments to the renewables sector are climbing fast, rising from RMB40 billion in 2022 to RMB100 billion (US$14 billion) in 2023. As of 2021, subsidy arrears stood at RMB400 billion, as eligible projects outstripped available funding. China could struggle to support subsidies in future.
Three policy priorities
What can policymakers do? The higher-rate environment is a headwind to the energy transition globally, so it is imperative that they remove other barriers to transition. We see three priorities:
- Focus on subsidy efficiency. With government finances under pressure, subsidies need to have the maximum impact on decarbonising the global economy. Targeted and non-discriminatory subsidies are most efficient, minimising nationalistic subsidy battles that are counterproductive to global emissions targets.
- Bolster carbon markets. Article 6 of the Paris Agreement is the original ‘rulebook’ on carbon markets and non-market approaches to mitigating global emissions. For many countries, an operational Article 6 is likely to be necessary to meet their nationally determined contributions to reducing emissions. With governments having failed to conclude and sign off on the carbon crediting mechanism (Article 6.4) at the COP28 climate conference in Dubai in December 2023, the next opportunity will be at COP29 in Baku in November.
- Mobilise climate finance. Drumming up climate finance, be it from the private or the public sector, is critical to supporting green investment for climate change mitigation and adaptation in developed and developing economies. Developed economies committed to transferring US$100 billion per year to developing economies by 2020 and may have belatedly hit that target in 2022. However, it is a drop in the ocean compared with the trillions of dollars needed each year to steer the global economy onto a net zero path. Greater use of financial mechanisms and instruments to maximise private-sector investment is needed. Central banks could offer loans to commercial banks at preferential rates, specifically to be used to finance low-carbon investments. These models have been tried by central banks in Japan and China and advocated for Europe by President Emmanuel Macron of France.
Conclusion:
A call to action.
If higher interest rates persist, transitioning to net zero will be even more challenging. Nascent low-carbon technologies, exposed to higher rates, could cost more. Meanwhile, traditional hydrocarbon energy sources and the mining sector look to have a financing cost advantage.
Rising interest payments and debt constraints risk limiting the public sector’s ability to contribute to financing the energy transition, either directly by capital investment or through subsidies and tax credits.
Consumers and end users of energy and commodities are likely to pay more. And the transition to net zero will be delayed even further. Action is required to avoid, or at least mitigate, such an outcome. For investors and companies, strict capital discipline will remain in focus in a prolonged period of higher rates.
Policymakers need to act to offset the interest-rate headwinds. Removing obstacles such as slow permitting and project approval and offering clear, consistent and sustained incentives will support nascent low-carbon technologies. Strengthening global carbon markets, maximising subsidy efficiency and mobilising green finance are also essential. A higher interest-rate environment might be what it takes to get policymakers to spring into action.
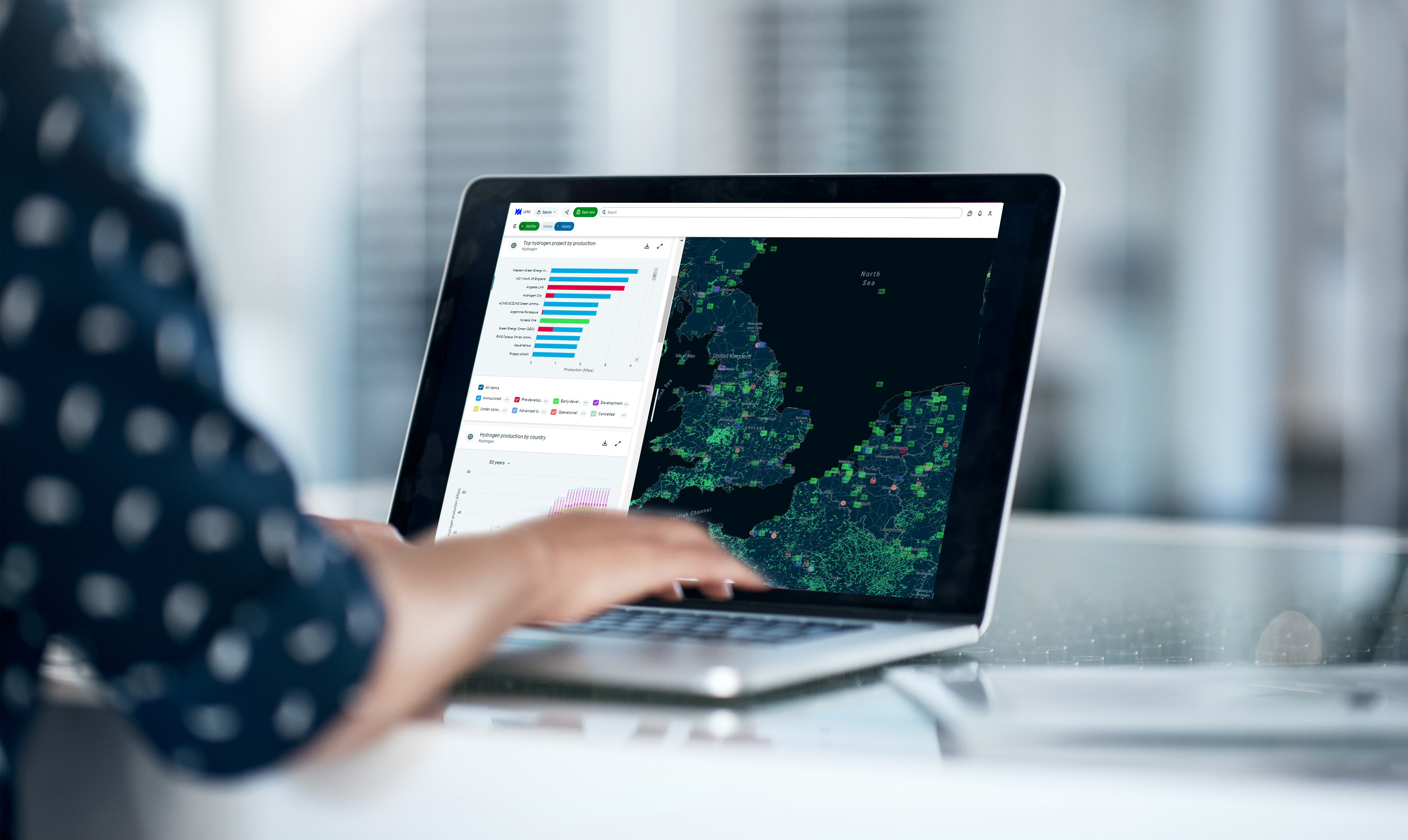
Sign up now to get insights like this in your inbox weekly
Stay updated with the freshest insights and news from Wood Mackenzie's team of global analysts.
Explore our latest thinking in Horizons
- Oil and Gas
- Oil Products
- Power and Renewables
- Metals costs
- Metals markets
- Metals concentrates and intermediates
- Power and renewables
- Downstream oil refining
- Midstream oil and gas
- Oil and gas markets
- Upstream oil and gas
- Macroeconomics, risk and global trends
- Latin America and the Caribbean
- North America
- Russia and the Caspian
- Middle East
- Commodity Analytics
- Corporate Analysis
- Exploration & Production
- Gas & LNG
- Government & NGO
- Institutional Investors
- Integrated Oil & Gas
- Investment Banks
- Macroeconomics & Energy Markets
- Metals & Mining
- National Oil Companies (NOCs)
- Power & Renewables
- Professional Services
- Refining & Marketing
- Technical Services
- Transportation
- Upstream oil & gas
- Industry Fundamentals
- Organisation
- Capital Allocation
- Government Strategy & Policy
- Commercial Advisory
- Procurement & Supply Chain
- Performance Improvement
- Due Diligence
- Opportunity Identification
- M&A & Divestments
- Capability Development
- Podcast CWIB
- LNG Big Chill podcast
- MENA in brief podcast
- SubSaharan Africa podcast
- Latin America podcast
- Digitalisation
- chemical reaction
- foresight 2020
- coronavirus
- Consulting case studies
- Oil market in crisis 2020
- Corporate distress
- M&M JK article series
- Women in energy
- wind solar O&M
- EU green deal
- Materials Transition
- US election 2020
- Chemicals - Mandarin
- Outlook 2021
- short term analytics
- Horizons Live
- Energy Gang
- Interchange
- Horizons podcast
- 2022 outlook
- partnered content
- Russia-Ukraine conflict
- Let's Share the Sun
- Data operations
- 2023 outlook
- ABM Utilities
- ABM M&M
- 2024 outlook
- careers content
- ABM Rosneft
- Podcast CFT
- Asia Pacific Energy Brief podcast
- The Inside Track
- energy transition
- electric vehicles
- apac energy buzz
Towards Sustainable Energy: A Systematic Review of Renewable Energy Sources, Technologies, and Public Opinions
Ieee account.
- Change Username/Password
- Update Address
Purchase Details
- Payment Options
- Order History
- View Purchased Documents
Profile Information
- Communications Preferences
- Profession and Education
- Technical Interests
- US & Canada: +1 800 678 4333
- Worldwide: +1 732 981 0060
- Contact & Support
- About IEEE Xplore
- Accessibility
- Terms of Use
- Nondiscrimination Policy
- Privacy & Opting Out of Cookies
A not-for-profit organization, IEEE is the world's largest technical professional organization dedicated to advancing technology for the benefit of humanity. © Copyright 2024 IEEE - All rights reserved. Use of this web site signifies your agreement to the terms and conditions.

HIST 212: History and Geopolitics of Energy in Eurasia
- Introduction
- In This Session (4/25/24)
- Getting Started
- Consider: Is this a book, article, or chapter level topic?
- Not Sure Where to Start?
- Searching Secondary Source Databases
- Policy Papers, Reports, and Grey Literature
- Advanced Searching
- Highlighted databases
- Stuck or need help? No problem!
Grey Literature
"Grey Literature" are scholarly or technical studies that are produced by experts or agencies but are outside of academic publications, and thus not captured in most databases. These are vital to understanding policies and politics, but are extremely difficult to find. Below are a few options on how t proceed.
- Policy Commons This link opens in a new window Policy Commons is a one-stop community platform for objective, fact-based research from the worlds leading policy experts, nonpartisan think tanks, IGOs and NGOs. The database provides users access to a variety of curated, high quality policy reports, briefs, analyses, working papers, and datasets from thousands of policy organizations covering disciplines such as agriculture, energy, pharmaceuticals, diversity, crime, and librarianship, among others.
- CIAO (Columbia International Affairs Online) This link opens in a new window International affairs. Working papers from universities, occasional papers from NGOs, foundation-funded research projects, conference proceedings, books, journals, policy briefs, case studies by international affairs experts, course packs for history and political science classes. Coverage: 1991-present.
- OECD (Organisation for Economic Co-Operation and Development): Economic, Environmental, and Social Statistics
- United Nations Digital LIbrary Search for documents, speeches, resolutions, and voting records.
- World Resources Institute Environmental and climatic (green house gas emission) statistics and reports.
Identifying Organizations
If you find an NGO or organization that publishes studies on your topic, search them as an Author in our databases.
Example: european atomic energy community in Worldcat or Euratom in Worldcat
The Organization's official website is also a good place to find reports. Ex
Other examples:
Provides world energy statistics. Covers energy supply and consumption for over 100 countries and regions.
Provided by The World Bank Group, Energydata.info is an open data platform that provides access to datasets and data analytics relevant to the energy sector. It was developed in support of the United Nations' sustainable development goal of ensuring "access to affordable, reliable, and sustainable modern energy for all" ( https://sdgs.un.org/goals ).
The world's first major carbon market, the EU ETS is "a cornerstone of the EU's policy to combat climate change and its key tool for reducing greenhouse gas emissions cost-effectively."
The World Bank Group provides the Global Solar Atlas, with data layers and maps, to support solar power in its client countries. Funded by the Energy Sector Management Assistance Program, it supports a global initiative on renewable energy resource mapping that includes biomass, small hydro, solar, and wind.
The IAEA is an intergovernmental organization for cooperation in the nuclear field. It provides information on nuclear applications and technology, nuclear safety and security, and related issues.
The International Energy Agency is comprised of 30 member countries (including the U.S.). It provides energy data and statistics on topics including coal, efficiency, electricity, emissions, prices, renewables, and more.
Provides information on development challenges, solutions, and strategic initiatives. Provides access to UNDP publications including the Human Development Report.
The United Nations Framework Convention on Climate Change has 197 members and works on supporting the global response to climate change through treaty agreements, including the Paris agreement and the Kyoto Protocol.
Provides reports and data on the U.S. government's international development and disaster assistance efforts.
Provides information on the World Bank's development projects, data by country and indicator, and research and publications.
An international organization that represents the global nuclear industry. It provides global trends reports, policy papers, and data on nuclear power, energy, and the environment.
Using Citations and Secondary Sources
Use Secondary Sources and bibliographies to identify papers and organizations of interest.
- Use Published Works (Ex) WOrks suggested by the EC
- << Previous: Searching Secondary Source Databases
- Next: Other Primary Sources: Interviews, Newspapers, Documents >>
- Last Updated: Apr 25, 2024 10:16 AM
- URL: https://guides.libraries.wm.edu/HIST212Spr2024
Thank you for visiting nature.com. You are using a browser version with limited support for CSS. To obtain the best experience, we recommend you use a more up to date browser (or turn off compatibility mode in Internet Explorer). In the meantime, to ensure continued support, we are displaying the site without styles and JavaScript.
- View all journals
- My Account Login
- Explore content
- About the journal
- Publish with us
- Sign up for alerts
- Open access
- Published: 24 April 2024
Probing entanglement in a 2D hard-core Bose–Hubbard lattice
- Amir H. Karamlou ORCID: orcid.org/0000-0001-7400-9604 1 , 2 nAff7 ,
- Ilan T. Rosen ORCID: orcid.org/0000-0001-8869-7364 1 ,
- Sarah E. Muschinske 1 , 2 ,
- Cora N. Barrett 1 , 3 ,
- Agustin Di Paolo 1 ,
- Leon Ding 1 , 4 ,
- Patrick M. Harrington 1 ,
- Max Hays ORCID: orcid.org/0000-0002-6241-1628 1 ,
- Rabindra Das 5 ,
- David K. Kim 5 ,
- Bethany M. Niedzielski 5 ,
- Meghan Schuldt 5 ,
- Kyle Serniak ORCID: orcid.org/0000-0003-3469-9166 1 , 5 ,
- Mollie E. Schwartz ORCID: orcid.org/0000-0003-4406-8974 5 ,
- Jonilyn L. Yoder 5 ,
- Simon Gustavsson ORCID: orcid.org/0000-0002-7069-1025 1 ,
- Yariv Yanay 6 ,
- Jeffrey A. Grover ORCID: orcid.org/0000-0001-6835-8517 1 &
- William D. Oliver ORCID: orcid.org/0000-0001-8041-0824 1 , 2 , 4 , 5
Nature ( 2024 ) Cite this article
58 Altmetric
Metrics details
- Quantum information
- Quantum simulation
Entanglement and its propagation are central to understanding many physical properties of quantum systems 1 , 2 , 3 . Notably, within closed quantum many-body systems, entanglement is believed to yield emergent thermodynamic behaviour 4 , 5 , 6 , 7 . However, a universal understanding remains challenging owing to the non-integrability and computational intractability of most large-scale quantum systems. Quantum hardware platforms provide a means to study the formation and scaling of entanglement in interacting many-body systems 8 , 9 , 10 , 11 , 12 , 13 , 14 . Here we use a controllable 4 × 4 array of superconducting qubits to emulate a 2D hard-core Bose–Hubbard (HCBH) lattice. We generate superposition states by simultaneously driving all lattice sites and extract correlation lengths and entanglement entropy across its many-body energy spectrum. We observe volume-law entanglement scaling for states at the centre of the spectrum and a crossover to the onset of area-law scaling near its edges.
Similar content being viewed by others
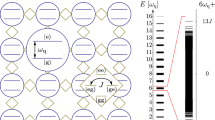
Two-dimensional hard-core Bose–Hubbard model with superconducting qubits
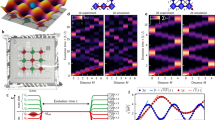
Quantum transport and localization in 1d and 2d tight-binding lattices
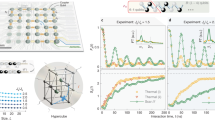
Many-body Hilbert space scarring on a superconducting processor
Entanglement is a uniquely quantum property that underpins descriptions of interacting quantum systems as statistical ensembles 4 , 5 , 6 , 7 . Within closed many-body quantum systems, entanglement among constituent subsystems introduces uncertainty to their individual states, even when the full system is in a pure state 1 , 15 . For this reason, entropy measures are commonly used to quantify quantum entanglement in many-body systems and have been directly probed in different platforms 8 , 9 , 10 , 11 , 12 , 13 , 14 . The study of entanglement in interacting many-body quantum systems is central to the understanding of a range of physical phenomena in condensed-matter systems 1 , quantum gravity 2 , 3 and quantum circuits 16 . The scaling of the entanglement entropy with subsystem size provides insight into classifying phases of quantum matter 17 , 18 , 19 and the feasibility of numerically simulating their dynamics 15 .
In a closed system, the bipartite entanglement entropy of a subsystem quantifies the amount of entanglement between the subsystem and the remainder of the system. For certain many-body states, such as the ground state of 1D local Hamiltonians 20 , the entanglement entropy is proportional to the boundary between a subsystem and the remaining system; this boundary is referred to as the area of a subsystem. Such states are said to have area-law entanglement scaling 15 . For other states, entanglement entropy increases proportionally to the bulk size (volume) of a subsystem, a behaviour referred to as volume-law scaling. To characterize the entanglement scaling in an interacting lattice, we consider the area and volume entanglement entropy per lattice site, represented by s A and s V , respectively. Disregarding logarithmic corrections, which are theoretically expected in certain contexts 21 , 22 , the entanglement entropy S ( ρ X ) of a subsystem X can be expressed using the ansatz
in which A X is the subsystem area and V X is the subsystem volume (see Fig. 1a for an example). The ratio of volume to area entropy per site, s V / s A , quantifies the extent to which that state obeys area-law or volume-law entanglement scaling 23 . Quantum states with area-law entanglement scaling have local correlations, whereas systems obeying volume-law entanglement scaling contain correlations that extend throughout the system and are therefore more challenging to study using classical numerical methods 24 , 25 .
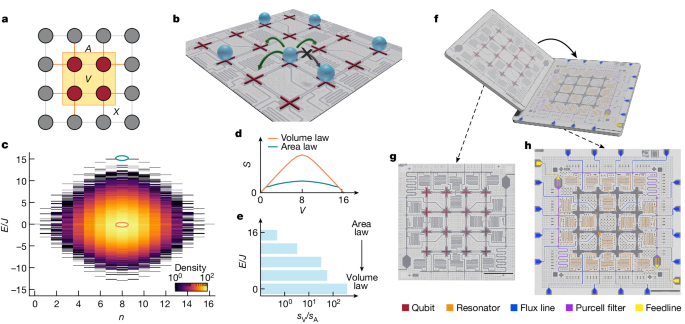
a , Schematic for an example subsystem X of four qubits within a 16-qubit lattice. The subsystem has a volume of 4 (maroon sites) and an area of 8 (orange lines). b , 2D HCBH lattice emulated by the superconducting quantum circuit. Each site can be occupied by, at most, a single particle. c , Energy E spectrum of the HCBH lattice emulated by our device, shown in the rotating frame resonant with the lattice sites. The energy spectrum is partitioned into distinct sectors defined by the total particle number n . d , Scaling of the entanglement entropy S with subsystem volume V for an eigenstate at the centre of the energy spectrum (orange line, corresponding to the energy eigenstate highlighted by the orange oval in c ) and an eigenstate at the edge of the energy spectrum (teal line, corresponding to the energy eigenstate highlighted by the teal oval in c ). e , Change in the entanglement behaviour, quantified by the geometric entropy ratio s V / s A , for states with n = 8. f , Schematic for the flip-chip sample consisting of 16 superconducting qubits. g , h , Optical images of the qubit tier ( g ) and the interposer tier ( h ) are illustrated with the qubits and the different signal lines false-coloured. Scale bars, 1 mm.
In this work, we study the entanglement scaling of states residing in different energetic regions of a 2D HCBH lattice. The Bose–Hubbard model is particle-number conserving, allowing its energy spectrum to be partitioned into sectors with definite particle number n . The ‘hard-core’ condition arises from strong on-site particle–particle interactions that mandate that each lattice site may be occupied by at most a single particle (Fig. 1b ). In 2D, the HCBH model is non-integrable and may exhibit eigenstate thermalization 5 , 26 , 27 and quantum information scrambling 28 . Highly excited many-body states—states residing near the centre of the energy spectrum (energy E ≈ 0; orange oval in Fig. 1c )—are expected to exhibit volume-law scaling of the entanglement entropy, following the Page curve 2 (orange line in Fig. 1d ). For subsystems smaller than half the size of the system, entropy grows linearly in subsystem size. Entropy is maximized when the subsystem comprises half of the entire system and decreases as the subsystem size is further increased. By contrast, the entanglement entropy of states residing near the edges of the HCBH energy spectrum does not follow the Page curve but instead grows less rapidly with subsystem volume (exemplified by the teal line in Fig. 1d showing the entropy of the eigenstate highlighted by a teal oval in Fig. 1c ). For states with intermediate energies, a crossover is expected from area-law scaling at the edges of the energy spectrum to volume-law scaling at its centre 22 , 23 (Fig. 1e ). Although Fig. 1e illustrates the crossover for the n = 8 particle-number manifold, the crossover similarly occurs in other manifolds (see Methods ).
The crossover from area-law to volume-law entanglement scaling can be observed by studying the exact eigenstates of the HCBH model. However, preparing a specific eigenstate generally requires a deep quantum circuit 29 or a slow adiabatic evolution 30 . Alternatively, we can explore the behaviour of the entanglement entropy by preparing superpositions of eigenstates across numerous particle-number manifolds 23 . Here we prepare such superposition states by simultaneously and weakly driving 16 superconducting qubits arranged in a 4 × 4 lattice. By varying the detuning of the drive frequency from the lattice frequency, we generate superposition states occupying different regions of the HCBH energy spectrum. Measurements of correlation lengths and entanglement entropies indicate volume-law entanglement scaling for states prepared at the centre of the spectrum and a crossover to the onset of area-law entanglement scaling for states prepared at the edges.
Experimental system
Superconducting quantum processors provide strong qubit–qubit interactions at rates exceeding individual qubit decoherence rates, making them a platform that is well suited for emulating many-body quantum systems 28 , 30 , 31 , 32 , 33 , 34 , 35 , 36 . In this experiment, we use a 2D lattice of 16 capacitively coupled superconducting transmon qubits 37 , fabricated in a flip-chip geometry 38 (Fig. 1f,g ; see details in Methods ). The circuit emulates the 2D Bose–Hubbard model, described by the Hamiltonian
in which \({\widehat{a}}_{i}^{\dagger }\) ( \({\widehat{a}}_{i}\) ) is the creation (annihilation) operator for qubit excitations at site i , \({\widehat{n}}_{i}={\widehat{a}}_{i}^{\dagger }{\widehat{a}}_{i}\) is the respective excitation number operator and qubit excitations correspond to particles in the Bose–Hubbard lattice. The first term represents the site energies ϵ i = ω i − ω r , in which the qubit transition frequencies ω i are written in a frame rotating at frequency ω r . The second term describes on-site interactions arising from the qubit anharmonicities U i , with an average strength U /2π = −218(6) MHz. The final term of the Hamiltonian describes particle-exchange interactions of strengths J i j between neighbouring lattice sites with an average strength of J /2π = 5.9(0.4) MHz at qubit frequency ω /2π = 4.5 GHz. Particle exchange during state preparation and readout is prevented by detuning the qubits to different frequencies (inset in Fig. 2a ). Our system features site-resolved, multiplexed single-shot dispersive qubit readout 39 with an average qubit state assignment fidelity of 93%, which—together with site-selective control pulses—allows us to perform simultaneous tomographic measurements of the qubit states.
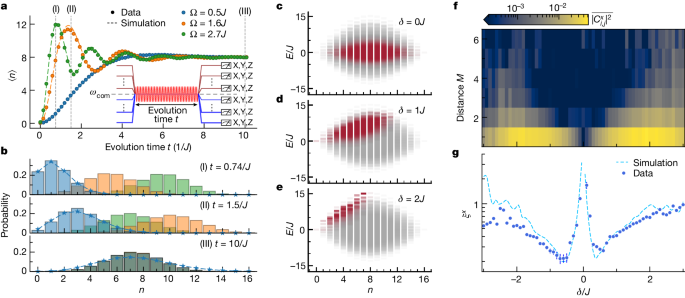
a , Total number of particles ⟨ n ⟩ in the uniform lattice while driving the system on resonance for time t . Simulations do not include decoherence. The lattice reaches half-filling at equilibrium. The experiments are executed using the pulse sequence shown in the inset. b , Probability of measuring different numbers of excitations in the lattice at three different times. The blue stars are from a Poisson fit to the excitation-number distribution for Ω = J /2, with the dashed lines as guides to the eye. c – e , Simulated overlap of the prepared coherent-like state in steady state ( t = 10/ J ) with drive strength Ω = J /2 and drive detuning δ = 0 J ( c ), δ = 1 J ( d ) and δ = 2 J ( e ) with the HCBH energy eigenstates. The different shades of red indicate the magnitude of the overlap between the prepared superposition states and energy eigenstates. Note that the spectra are shown in the rotating frame of the lattice sites and not of the drive. f , Average two-point correlator squared along the x basis, \(\overline{| {C}_{i,j}^{x}{| }^{2}}\) , between qubit pairs at distance M for drive duration t = 10/ J , strength Ω = J /2 and detuning δ from the lattice frequency. g , Correlation length ξ x extracted using the two-point correlators at different values of δ .
In our system, on-site interactions are much stronger than exchange interactions, J ≪ | U |. By restricting each site to two levels and mapping the bosonic operators to qubit operators, we transform the Hamiltonian in equation ( 2 ) to the hard-core limit
in which \({\widehat{\sigma }}_{i}^{+}\) ( \({\widehat{\sigma }}_{i}^{-}\) ) is the raising (lowering) operator for a qubit at site i and \({\widehat{\sigma }}_{i}^{z}\) is the Pauli Z operator. The energy relaxation rate Γ 1 and dephasing rate Γ ϕ are small compared with the particle-exchange rate, with Γ 1 ≈ 10 −3 J and Γ ϕ ≈ 10 −2 J , allowing us to prepare many-body states and probe their time dynamics faithfully.
Coherent-like states
We generate a superposition of many eigenstates of the lattice, which we refer to as a coherent-like state, by simultaneously driving all qubits through a common control line. Applied to the lattice initialized with no excitations, the drive acts as a displacement operation of the Fock basis defined by the number of excitations in the lattice 23 , hence, it will result in a state analogous to coherent states of light. Selecting the rotating-frame frequency ω r to be the frequency of the drive, the Hamiltonian of the driven lattice is
in which δ = ω r − ω com is the detuning between the drive and the qubit frequencies (all qubits are biased on resonance at ω com ). The drive strength Ω can be tuned by varying the amplitude of the applied drive pulse. The common drive couples independently to each qubit with a complex coefficient α j that depends on the geometric circuit parameters of the lattice.
We study the time dynamics of the average number of excitations ⟨ n ⟩ in the lattice under a resonant drive ( δ = 0) in Fig. 2a . The driven lattice reaches a steady state of half-filling, with an average particle number ⟨ n ⟩ = 8, after driving the lattice for time t ≈ 10/ J . Once in steady state, the drive adds and removes excitations coherently from the system at the same rate. Hamiltonian parameters J i j and α j were characterized through a procedure described in Section 4 of the Supplementary Information and the excellent agreement of numerical simulations of time evolution under equation ( 4 ) with experimental data confirms their accuracy. In Fig. 2b , we report the discrete probability distribution of measuring a different number of excitations in the lattice at three different times. The probability of a particular excitation number approximately follows a Poisson distribution for a weak drive Ω = J /2 (blue stars with dashed lines as guides to the eye), indicating that the quantum state is in a coherent-like superposition of excitation-number states.
The coherent-like state comprises a swath of the HCBH energy spectrum that depends on the drive detuning. Driving the lattice on resonance prepares a coherent-like state at the centre of the HCBH energy spectrum (Fig. 2c ). By varying the drive detuning, we can generate states that are a superposition of the eigenstates closer to the edge of the energy band (Fig. 2d,e ). The standard deviation in the energy of the state depends on the strength of the drive: a stronger drive increases the bandwidth of populated energy eigenstates within each particle-number subspace. Therefore, to probe the entanglement properties across the lattice energy spectrum, we choose a relatively weak drive with strength Ω = J /2 for the rest of the main text. We choose a drive duration t = 10/ J , which is short compared with the timescale of decoherence, yet long enough to allow the coherent-like state to reach its steady-state distribution (which occurs after roughly t = 6/ J according to simulations shown in Section 14 of the Supplementary Information ).
Correlation lengths
A system of interacting particles exhibits correlations between its constituent subsystems. The HCBH Hamiltonian is equivalent to an XY Hamiltonian, in which quantum order is reflected by the transverse correlations 40 . To quantify transverse correlations, for states generated with detuning δ , we measure the two-point correlators \({C}_{i,j}^{x}\equiv \langle {\widehat{\sigma }}_{i}^{x}{\widehat{\sigma }}_{j}^{x}\rangle -\langle {\widehat{\sigma }}_{i}^{x}\rangle \langle {\widehat{\sigma }}_{j}^{x}\rangle \) between different qubit pairs. In Fig. 2f , we show the magnitude-squared two-point correlator values \(\overline{| {C}_{i,j}^{x}{| }^{2}}\) , averaged over qubits at the same Manhattan distance M . When δ / J is small, generating a superposition state occupying the centre of the energy band, \(\overline{| {C}_{i,j}^{x}{| }^{2}}\) becomes small for all M , matching the expectation for states with volume-law entanglement scaling. The two-point correlator has an upper bound set by the mutual information between the sites I ( i : j ) = S ( ρ i ) + S ( ρ j ) − S ( ρ i j ). In a volume-law state, the entropy of small subsystems is equal to their volume 2 , hence we expect that the mutual information—and, in turn, the correlator between any qubit pair—will vanish.
Using the two-point correlators, we extract the correlation length ξ x by fitting \(| {C}_{i,j}^{x}{| }^{2}\propto \exp \left(-M/{\xi }^{x}\right)\) (Fig. 2g ). The correlation length quantifies the dependence of correlations on the distance between the subsystems within our system. As we increase the magnitude of the drive detuning δ , skewing the superposition state to the edge of the energy spectrum, we generally find that the correlation length increases. The states prepared with − J /2 ≲ δ ≲ J /2, however, follow the opposite trend, and the extracted correlation length diverges around δ = 0. In this regime in which the coherent-like state occupies the centre of the energy spectrum, the two-point correlators asymptotically reach zero, so the extracted two-point correlation length loses meaning. We attribute the slight asymmetry of the observed correlation lengths about δ = 0 to a slight offset of the energy spectrum of our lattice towards positive energies owing to next-nearest-neighbour exchange interactions (see Section 5 of the Supplementary Information ).
Entanglement scaling behaviour
To study the entanglement scaling of the coherent-like states, we reconstruct the density matrices of 163 unique subsystems using a complete set of tomography measurements. The measured subsystems contain up to six qubits (see Fig. 3a for examples). We quantify the entanglement entropy between subsystem X and the remaining system through the second Rényi entropy 41
in which ρ X is the reduced density matrix describing a subsystem X of the quantum system ρ .
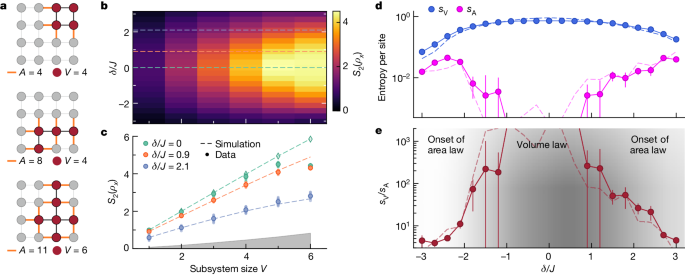
a , Examples of subsystems measured in our lattice. b , The average subsystem entanglement entropy S 2 ( ρ X ) for a subsystem with volume V for a state prepared with drive time t = 10/ J , strength Ω = J /2 and detuning δ from the lattice frequency. c , Subsystem entanglement entropy S 2 ( ρ X ) along the dashed lines in panel b for detunings δ / J = 0 (green), 0.9 (orange) and 2.1 (blue). Solid circles are experimental data averaged across all subsystems of each volume, with data for individual subsystems indicated by smaller, transparent circles. For δ / J = 0 (green), open diamonds are Monte Carlo simulations of 20,000 samples indicating that deviations at subsystem sizes 5 and 6 arise partially from insufficient sampling. The grey region indicates the estimated classical entropy from dephasing (see Supplementary Information for details). d , The volume entanglement entropy, s V , and area entanglement entropy, s A , per site extracted using 163 different subsystems of various volumes and areas for states prepared with drive detuning δ . The error bars indicate ±1 standard error of the fit parameter. e , The geometric entropy ratio s V / s A is used for quantifying the behaviour of entanglement. States prepared with δ = 0 exhibit a strong volume-law scaling and the states prepared with larger drive detuning values show a weaker volume-law scaling with an increasing area-law scaling. Dashed lines in c – e indicate results from numerical simulation.
We first study the scaling of the entanglement entropy with the subsystem volume V X , defined as the number of qubits within the subsystem, for states prepared with different drive detunings δ (Fig. 3b ). For states prepared with δ = 0, we observe nearly maximal scaling of the entropy with subsystem size S 2 ( ρ X ) ≈ V X , whereas with increasing δ , the entropy grows more slowly with subsystem size (Fig. 3c ).
There is excellent agreement between the expected and the measured entropy for subsystems of volume ≤4. Yet there is a discrepancy for the largest subsystems for which the coherent-like states are prepared near the centre of the spectrum (see Fig. 3c ). The discrepancy arises, in large part, from having a finite number of measurement samples when reconstructing subsystem density matrices. Tomographic reconstruction of a subsystem state requires measuring Pauli strings. When the system has volume-law entanglement, the measurement outcome distributions become more uniform, increasing sensitivity to sampling errors, especially for larger subsystems. Therefore, the reconstructed density matrices of the largest subsystems have less entropy than the actual states, as seen for V = 5 and 6 in Fig. 3c . Monte Carlo simulations of measurement outcomes confirm that a more accurate reconstruction of highly entangled states follows from larger numbers of measurement samples of each Pauli string (see Methods ). From our experimental data, we can extract 2,000 × 3 6− V measurement samples for each Pauli string describing a subsystem of volume V —sufficient for V = 1–4 but less so for V = 5 and 6. We could have obtained better agreement for V = 5 and 6 if we had used 20,000 samples (open diamonds in Fig. 3c ), which would have been straightforward to implement experimentally.
We next determine the scaling of entanglement entropy with subsystem volume s V and area s A as per equation ( 1 ). Using the Rényi entropies of the density matrices reconstructed from experimental data, we extract s V and s A by measuring the rate of change of S 2 ( ρ X ) with V X and the subsystem area A X , respectively, with the other held fixed. Here A X is defined as the number of nearest-neighbour bonds intersecting the boundary of the subsystem X . The linear fitting procedure used to determine s V and s A is detailed in Section 10 of the Supplementary Information . In Fig. 3d , we observe that, as the magnitude of δ becomes larger, s V decreases and s A increases. Although extraction of s V is reliable at all drive detunings, at small drive detuning values (− J < δ < J ), the entanglement entropy does not exhibit a notable dependence on the area of the subsystem in our finite lattice, hence we are not able to reliably fit s A . By considering the geometric entropy ratio s V / s A , we observe a change in the behaviour of entanglement entropy within our system (Fig. 3e ). The states prepared at the centre of the energy spectrum with δ = 0 exhibit a strong volume-law scaling and the states prepared closer to the edge of the energy spectrum show weaker volume-law scaling and increasing area-law scaling.
The entanglement spectrum, obtained through Schmidt decomposition, further quantifies the structure of entanglement across a bipartition of a closed quantum system 17 . The quantum state | ψ ⟩ can be represented as a sum of product states of the orthonormal Schmidt bases | k X ⟩ for a given subsystem X and \(\left|{k}_{\bar{X}}\right\rangle \) for the remaining lattice 42 :
in which positive scalars λ k are the Schmidt coefficients with \({\sum }_{k}{\lambda }_{k}^{2}=1\) . The Schmidt coefficients form the entanglement spectrum and provide a proxy for the degree of entanglement between the two subsystems. For a subsystem X maximally entangled with the remaining lattice, all of the Schmidt coefficients will have an equal value \({\lambda }_{k}=1/\sqrt{{2}^{{V}_{X}}}\) , in which V X is the volume of X (assuming that \({V}_{X} < {V}_{\bar{X}}\) ).
We obtain the entanglement spectrum for a bipartition of our lattice by diagonalizing the measured density matrix of a subsystem. In Fig. 4a , we study the entanglement formed within states prepared across the energy spectrum. We report the first 16 Schmidt coefficients squared \({\lambda }_{k}^{2}\) , in decreasing order, for a subsystem highlighted in maroon and the remaining lattice. We observe that, for states obeying volume-law entanglement scaling at the centre of the spectrum, the variation in coefficient magnitudes is small compared with states closer to the edge of the spectrum, in close agreement with numerical simulation. To quantify this difference, in Fig. 4b , we show the ratio of the largest and the k th largest Schmidt coefficient, \({\lambda }_{1}^{2}/{\lambda }_{k}^{2}\) , for k = 5, 10 and 14, of coherent-like states prepared with different drive detunings δ . We observe that a small number of Schmidt states contain nearly all the weight of the decomposition for the area-law-like states, whereas, for the volume-law states, the Schmidt coefficients are roughly equal. This variation signals a change in the extent of the entanglement distribution across the system.
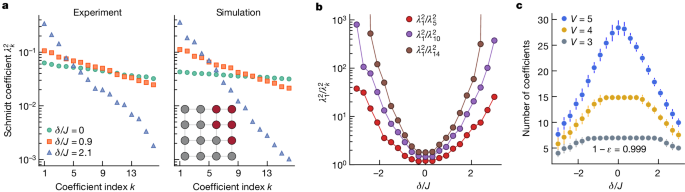
a , The Schmidt coefficients for the decomposition of a five-qubit subsystem (highlighted with maroon colour in the inset) and the remaining lattice. Coherent-like states are prepared with different drive detunings δ / J = 0, 0.9 and 2.1. The coefficients in the left panel are calculated from experimental data and agree well with the simulated coefficients in the right panel. b , The ratio of the largest and the k th largest Schmidt coefficient \({\lambda }_{1}^{2}/{\lambda }_{k}^{2}\) , for k = 5, 10 and 14, of coherent-like states prepared with different drive detunings δ in the experiment. c , The number of Schmidt coefficients required to represent the bipartition of the lattice with subsystem volume V = 3, 4 and 5 with accuracy 1 − ϵ = 0.999. Each data point is averaged over all the measured subsystems of the same size, with the error bars indicating ±1 standard deviation.
In Fig. 4c , we report the number of coefficients required to approximate the state of the lattice bipartition with accuracy 1 − ϵ = 0.999 (see Section 12 of the Supplementary Information ) for subsystems with volume V = 3, 4 and 5. We find that the states at the edge of the energy spectrum can be accurately represented with fewer coefficients, whereas the number of coefficients needed to approximate states at the centre of the band approaches the dimension of the Hilbert space of the subsystem, 2 V .
In this work, we study the entanglement scaling properties of the 2D HCBH model, emulated using a 16-qubit superconducting quantum processor. By simultaneously driving all qubits, we generate coherent-like superposition states that preferentially incorporate eigenstates from regions of the many-body energy spectrum that we tune between the centre and the edges. We probe the transverse quantum correlation lengths and the entanglement scaling behaviour of the superposition states. We observe a crossover from volume-law scaling of entanglement entropy near the centre of the band, coinciding with vanishing two-point correlators, to the onset of area-law entropy scaling at the edges of the energy band, accompanied by finite-range correlations.
The coherent-like superposition states comprise eigenstates across a swath of the HCBH energy spectrum. We can decrease the spectral width of these states by reducing the drive strength. However, the system under a weaker drive requires a longer evolution time to reach steady state. Improved processor coherence enables the creation of narrower-width states, providing a finer resolution for studying the area-to-volume-law crossover.
Although an analytical relation between entanglement and thermodynamic entropy exists for integrable systems 43 , the emergence of thermodynamic behaviour in non-integrable systems is less well understood 4 , 5 , 6 , 44 , 45 . In recent years, random-circuit protocols using digital quantum circuits have been applied to study this emergence 46 . The protocol introduced in this work is an analogue (that is, continuous-time evolution) counterpart to random-circuit experiments, one that is also capable of generating highly entangled states.
Our area-to-volume-law transition protocol applies to larger system sizes, despite the exponential time complexity of implementing state tomography, because we need not concomitantly increase the subsystem size (see simulations in Section 11 of the Supplementary Information ). A subsystem with V qubits can probe entanglement correlations up to a depth of 2 V qubits 47 . Therefore, a fixed subsystem volume and simultaneous readout ensure an essentially constant runtime and measure up to a fixed entanglement depth, even as the overall system size increases. Increasing the subsystem size does increase the accessible entanglement depth, but it comes with exponential cost. Therefore, our approach enables us to study emergent thermalization up to constant entanglement depth, even as we enter classically intractable regimes. The measurement time and depth are ultimately set by the subsystem size we are willing to accommodate.
Finally, the structure of entanglement within a quantum system determines the effective degrees of freedom required to accurately simulate the quantum states. Area-law states can generally be numerically simulated efficiently using tensor network methods 15 , 24 , 25 , whereas the computational complexity of classically simulating volume-law states scales exponentially with system size. It is the latter complexity that underpins the promise of quantum computational advantage. In this work, we demonstrated a hardware-efficient means to determine—to constant depth—the entanglement scaling and, thereby, the computational complexity of programs executed on quantum processors.
Note that, during the preparation of this manuscript, we became aware of related studies in a 1D trapped-ion simulator 48 .
Experimental setup
The experiment is performed in a dilution refrigerator at a base temperature of 20 mK. We study a superconducting processor with 16 transmon qubits arranged in a 4 × 4 square grid. The superconducting processor has aluminium circuit elements deposited on silicon substrates and is fabricated using a flip-chip process 38 , as depicted in Fig. 1f . The qubits are located on a qubit tier (Fig. 1g ) and the readout and control lines are located on a separate interposer tier (Fig. 1h ; see Supplementary Information for further device details).
Each transmon qubit in the superconducting processor represents one site in the Bose–Hubbard Hamiltonian. The site energy ω i is given by the transition frequency from the ground state to the first excited state of the qubit and can be controlled with an error of less than 300 kHz (5 × 10 −2 J ) (ref. 49 ). The on-site interaction U i arises from the anharmonicity of transmon qubit i , representing the energy cost for two particles to occupy the same site. For transmon qubits, the energy cost is negative. The particle-exchange interaction between neighbouring lattice sites is realized by capacitively coupling adjacent qubits. Although the coupling strengths between qubits are fixed, we effectively switch particle exchange off for state preparation and readout by detuning the qubits to different frequencies (inset in Fig. 2a ). The common drive we use to generate the coherent-like states is applied to the system by means of the readout feedlines and couples to each qubit through the respective readout resonator of the qubit.
To measure site populations and correlators, we make single-shot measurements of identically prepared systems and then determine the expectation value of each operator as its average value across all measurements. To measure X and Y Pauli operators, we apply site-selective control pulses immediately before measurement.
For tomography measurements, we simultaneously measured 163 subsystems up to volume V = 6 by taking 2,000 single-shot measurement samples for each of the 3 6 necessary Pauli strings, as visualized in Supplementary Fig. 14 . The set of 3 6 Pauli strings includes several copies of each of the 3 V Pauli strings needed to describe subsystems of volume V < 6. For subsystems of volume V , we can therefore extract 2,000 × 3 6− V measurement samples from our data. We extract density matrices using a standard maximum-likelihood estimator that is aware of individual single-shot outcomes. More detail is provided in the Supplementary Information .
Entanglement across the particle-number manifolds
For each constant-particle-number manifold of the HCBH Hamiltonian, we observe a variation in the geometric entanglement from the edge to the centre of the spectrum 23 . To illustrate this variation, we report the average subsystem entropy as a function of volume for states at the edge and at the centre of the energy band of subspaces with n = 5, 6, 7 and 8 particles in Extended Data Fig. 1a . The states at the centre of the energy band exhibit a distinct Page curve, whereas the entropy of the states at the edge of the energy band shows a weak dependence on volume. Furthermore, in Extended Data Fig. 1b , we show the geometric entanglement ratio s V / s A and notice the same trend between the states at the centre and at the edge of the energy band for the subspaces designated by the different number of particles. The geometric entanglement behaviour is consistent across different particle-number subspaces, allowing us to probe the entanglement scaling across the many-body spectrum using a superposition of different eigenstates.
Measurement sampling statistics
Full-state tomography of a subsystem X containing V X sites involves measurement of Pauli strings \({\prod }_{i\in X}{\sigma }_{i}^{{\alpha }_{i}}\) for all combinations of Pauli operators α i ∈ { x , y , z }. For each Pauli string, we aim to accurately determine the distribution of measurement outcomes, of which there are \({2}^{{V}_{X}}\) . For larger subsystems, the number of possible measurement outcomes is large, and as the state being measured approaches a volume-law state, the distribution of measurement outcomes approaches a uniform distribution. In this limit, the number of measurements required to accurately sample the outcome distribution becomes large.
The area-law states generated when | δ |/ J is larger feature far-from-uniform distributions of measurement outcomes. Reconstruction of these states is therefore less sensitive to finite sampling statistics. This observation is commensurate with the results of ref. 50 , in which only 3 × 10 3 samples per Pauli string were sufficient to accurately reconstruct ten-qubit Greenberger–Horne–Zeilinger states (which have area-law entanglement scaling).
To quantify the impact of the number of samples n s on the extracted entropy, we take a Monte Carlo approach. Here we consider the coherent-like state prepared at δ = 0 and Ω = J /2 (a volume-law state) and begin by obtaining the final state through a decoherence-free numerical simulation. For each subsystem and each Pauli string, we then sample from the distribution of bitstring measurement outcomes n s times. We reconstruct the subsystem density matrices from these samples and compute their entropy S 2 . Density-matrix reconstruction used the same maximum-likelihood estimation routine as was used to reconstruct density matrices from experimental data.
The results are shown in Extended Data Fig. 2 for n s ranging from 50 up to 2 × 10 4 . Density-matrix reconstruction without maximum-likelihood estimation is shown for comparison. For low n s , sampling bias causes a biased reconstruction of the distribution of measurement outcomes, resulting in a deficit of the extracted entropy. The extracted entropy increases and eventually saturates at the correct values as n s increases. The value of n s needed to accurately extract the subsystem entropy grows exponentially in subsystem volume. Although n s = 2 × 10 3 was used for V = 6 subsystems in the present experiment, these simulations show that n s ≳ 10 4 is needed to accurately extract the entropy of volume-law states for subsystems of volume 6.
The results from the Monte Carlo simulation of measurement sampling effects are compared with experimental data in Extended Data Fig. 2c . Owing to the simultaneous tomography of all subsystems, our data yield 2,000 × 3 6− V measurement samples for a volume V subsystem.
Data availability
The data that support the findings of this study are available from the corresponding author on reasonable request and with the cognizance of our US Government sponsors who financed the work.
Code availability
The code used for numerical simulations and data analyses is available from the corresponding author on reasonable request and with the cognizance of our US Government sponsors who financed the work.
Amico, L., Fazio, R., Osterloh, A. & Vedral, V. Entanglement in many-body systems. Rev. Mod. Phys. 80 , 517–576 (2008).
Article ADS MathSciNet CAS Google Scholar
Page, D. N. Average entropy of a subsystem. Phys. Rev. Lett. 71 , 1291–1294 (1993).
Article ADS MathSciNet CAS PubMed Google Scholar
Nishioka, T., Ryu, S. & Takayanagi, T. Holographic entanglement entropy: an overview. J. Phys. A Math. Theor. 42 , 504008 (2009).
Article MathSciNet Google Scholar
Deutsch, J. M. Quantum statistical mechanics in a closed system. Phys. Rev. A 43 , 2046–2049 (1991).
Article ADS CAS PubMed Google Scholar
Srednicki, M. Chaos and quantum thermalization. Phys. Rev. E 50 , 888–901 (1994).
Article ADS CAS Google Scholar
Rigol, M., Dunjko, V. & Olshanii, M. Thermalization and its mechanism for generic isolated quantum systems. Nature 452 , 854–858 (2008).
D’Alessio, L., Kafri, Y., Polkovnikov, A. & Rigol, M. From quantum chaos and eigenstate thermalization to statistical mechanics and thermodynamics. Adv. Phys. 65 , 239–362 (2016).
Article ADS Google Scholar
Islam, R. et al. Measuring entanglement entropy in a quantum many-body system. Nature 528 , 77–83 (2015).
Kaufman, A. M. et al. Quantum thermalization through entanglement in an isolated many-body system. Science 353 , 794–800 (2016).
Neill, C. et al. Ergodic dynamics and thermalization in an isolated quantum system. Nat. Phys. 12 , 1037–1041 (2016).
Article CAS Google Scholar
Linke, N. M. et al. Measuring the Rényi entropy of a two-site Fermi-Hubbard model on a trapped ion quantum computer. Phys. Rev. A 98 , 052334 (2018).
Lukin, A. et al. Probing entanglement in a many-body–localized system. Science 364 , 256–260 (2019).
Brydges, T. et al. Probing Rényi entanglement entropy via randomized measurements. Science 364 , 260–263 (2019).
Nakagawa, Y. O., Watanabe, M., Fujita, H. & Sugiura, S. Universality in volume-law entanglement of scrambled pure quantum states. Nat. Commun. 9 , 1635 (2018).
Article ADS PubMed PubMed Central Google Scholar
Eisert, J., Cramer, M. & Plenio, M. B. Colloquium: Area laws for the entanglement entropy. Rev. Mod. Phys. 82 , 277–306 (2010).
Article ADS MathSciNet Google Scholar
Choi, S., Bao, Y., Qi, X.-L. & Altman, E. Quantum error correction in scrambling dynamics and measurement-induced phase transition. Phys. Rev. Lett. 125 , 030505 (2020).
Li, H. & Haldane, F. D. M. Entanglement spectrum as a generalization of entanglement entropy: identification of topological order in non-Abelian fractional quantum Hall effect states. Phys. Rev. Lett. 101 , 010504 (2008).
Article ADS PubMed Google Scholar
Bauer, B. & Nayak, C. Area laws in a many-body localized state and its implications for topological order. J. Stat. Mech. Theory Exp. 2013 , P09005 (2013).
Khemani, V., Lim, S. P., Sheng, D. N. & Huse, D. A. Critical properties of the many-body localization transition. Phys. Rev. X 7 , 021013 (2017).
Google Scholar
Brandão, F. G. S. L. & Horodecki, M. An area law for entanglement from exponential decay of correlations. Nat. Phys. 9 , 721–726 (2013).
Article Google Scholar
Barthel, T., Chung, M.-C. & Schollwöck, U. Entanglement scaling in critical two-dimensional fermionic and bosonic systems. Phys. Rev. A 74 , 022329 (2006).
Miao, Q. & Barthel, T. Eigenstate entanglement: crossover from the ground state to volume laws. Phys. Rev. Lett. 127 , 040603 (2021).
Yanay, Y., Braumüller, J., Gustavsson, S., Oliver, W. D. & Tahan, C. Two-dimensional hard-core Bose–Hubbard model with superconducting qubits. npj Quantum Inf. 6 , 58 (2020).
Schollwöck, U. The density-matrix renormalization group. Rev. Mod. Phys. 77 , 259–315 (2005).
Perez-Garcia, D., Verstraete, F., Wolf, M. M. & Cirac, J. I. Matrix product state representations. Quantum Inf. Comput. 7 , 401–430 (2007).
MathSciNet Google Scholar
Santos, L. F. & Rigol, M. Onset of quantum chaos in one-dimensional bosonic and fermionic systems and its relation to thermalization. Phys. Rev. E 81 , 036206 (2010).
Nandkishore, R. & Huse, D. A. Many-body localization and thermalization in quantum statistical mechanics. Annu. Rev. Condens. Matter Phys. 6 , 15–38 (2015).
Braumüller, J. et al. Probing quantum information propagation with out-of-time-ordered correlators. Nat. Phys. 18 , 172–178 (2022).
Plesch, M. & Brukner, Č. Quantum-state preparation with universal gate decompositions. Phys. Rev. A 83 , 032302 (2011).
Saxberg, B. et al. Disorder-assisted assembly of strongly correlated fluids of light. Nature 612 , 435–441 (2022).
Roushan, P. et al. Spectroscopic signatures of localization with interacting photons in superconducting qubits. Science 358 , 1175–1179 (2017).
Xu, K. et al. Emulating many-body localization with a superconducting quantum processor. Phys. Rev. Lett. 120 , 050507 (2018).
Ma, R. et al. A dissipatively stabilized Mott insulator of photons. Nature 566 , 51–57 (2019).
Karamlou, A. H. et al. Quantum transport and localization in 1d and 2d tight-binding lattices. npj Quantum Inf. 8 , 35 (2022).
Mi, X. et al. Time-crystalline eigenstate order on a quantum processor. Nature 601 , 531–536 (2022).
Zhang, X., Kim, E., Mark, D. K., Choi, S. & Painter, O. A superconducting quantum simulator based on a photonic-bandgap metamaterial. Science 379 , 278–283 (2023).
Koch, J. et al. Charge-insensitive qubit design derived from the Cooper pair box. Phys. Rev. A 76 , 042319 (2007).
Rosenberg, D. et al. 3D integrated superconducting qubits. npj Quantum Inf. 3 , 42 (2017).
Blais, A., Huang, R.-S., Wallraff, A., Girvin, S. M. & Schoelkopf, R. J. Cavity quantum electrodynamics for superconducting electrical circuits: an architecture for quantum computation. Phys. Rev. A 69 , 062320 (2004).
Chen, C. et al. Continuous symmetry breaking in a two-dimensional Rydberg array. Nature 616 , 691–695 (2023).
Rényi, A. in Proc. Fourth Berkeley Symposium on Mathematical Statistics and Probability, Volume 1: Contributions to the Theory of Statistics Vol. 4.1 (ed. Neyman, J.) 547–562 (Univ. California Press, 1961).
Nielsen, M. A & Chuang, I. L. Quantum Computation and Quantum Information (Cambridge Univ. Press, 2000).
Alba, V. & Calabrese, P. Entanglement and thermodynamics after a quantum quench in integrable systems. Proc. Natl Acad. Sci. 114 , 7947–7951 (2017).
Article ADS MathSciNet CAS PubMed PubMed Central Google Scholar
Abanin, D. A., Altman, E., Bloch, I. & Serbyn, M. Colloquium: Many-body localization, thermalization, and entanglement. Rev. Mod. Phys. 91 , 021001 (2019).
Lami, L. & Regula, B. No second law of entanglement manipulation after all. Nat. Phys. 19 , 184–189 (2023).
CAS Google Scholar
Fisher, M. P. A., Khemani, V., Nahum, A. & Vijay, S. Random quantum circuits. Annu. Rev. Condens. Matter Phys. 14 , 335–379 (2023).
Sørensen, A. S. & Mølmer, K. Entanglement and extreme spin squeezing. Phys. Rev. Lett. 86 , 4431–4434 (2001).
Joshi, M. K. et al. Exploring large-scale entanglement in quantum simulation. Nature 624 , 539–544 (2023).
Barrett, C. N. et al. Learning-based calibration of flux crosstalk in transmon qubit arrays. Phys. Rev. Appl. 20 , 024070 (2023).
Song, C. et al. 10-qubit entanglement and parallel logic operations with a superconducting circuit. Phys. Rev. Lett. 119 , 180511 (2017).
Download references
Acknowledgements
We thank C. Tahan, E.-A. Kim, S. Choi, A. Harrow, S. Disseler, K. Seetharam, C. McNally, L. Li, L. Ateshian, D. Rower and K. Van Kirk for fruitful discussions and T. Orlando and A. Kaufman for careful reading of the manuscript. This material is based on work supported in part by the U.S. Department of Energy, Office of Science, National Quantum Information Science Research Centers, Quantum Systems Accelerator (QSA); in part by the Defense Advanced Research Projects Agency under the Quantum Benchmarking contract; in part by U.S. Army Research Office grant W911NF-18-1-0411; and by the Department of Energy and Under Secretary of Defense for Research and Engineering under Air Force contract no. FA8702-15-D-0001. A.H.K. acknowledges support from the NSF Graduate Research Fellowship Program. C.N.B. acknowledges support from the STC Center for Integrated Quantum Materials, NSF grant no. DMR-1231319 and the Wellesley College Samuel and Hilda Levitt Fellowship. S.E.M. is supported by a NASA Space Technology Research Fellowship. I.T.R., P.M.H. and M.H. are supported by an appointment to the Intelligence Community Postdoctoral Research Fellowship Program at the Massachusetts Institute of Technology administered by Oak Ridge Institute for Science and Education (ORISE) through an interagency agreement between the U.S. Department of Energy and the Office of the Director of National Intelligence (ODNI). Any opinions, findings, conclusions or recommendations expressed in this material are those of the author(s) and do not necessarily reflect the views of the Department of Energy, the Department of Defense or the Under Secretary of Defense for Research and Engineering.
Author information
Amir H. Karamlou
Present address: Google Quantum AI, Santa Barbara, CA, USA
Authors and Affiliations
Research Laboratory of Electronics, Massachusetts Institute of Technology, Cambridge, MA, USA
Amir H. Karamlou, Ilan T. Rosen, Sarah E. Muschinske, Cora N. Barrett, Agustin Di Paolo, Leon Ding, Patrick M. Harrington, Max Hays, Kyle Serniak, Simon Gustavsson, Jeffrey A. Grover & William D. Oliver
Department of Electrical Engineering and Computer Science, Massachusetts Institute of Technology, Cambridge, MA, USA
Amir H. Karamlou, Sarah E. Muschinske & William D. Oliver
Department of Physics, Wellesley College, Wellesley, MA, USA
Cora N. Barrett
Department of Physics, Massachusetts Institute of Technology, Cambridge, MA, USA
Leon Ding & William D. Oliver
MIT Lincoln Laboratory, Lexington, MA, USA
Rabindra Das, David K. Kim, Bethany M. Niedzielski, Meghan Schuldt, Kyle Serniak, Mollie E. Schwartz, Jonilyn L. Yoder & William D. Oliver
Laboratory for Physical Sciences, College Park, MD, USA
Yariv Yanay
You can also search for this author in PubMed Google Scholar
Contributions
A.H.K. performed the experiments and analysed the data with I.T.R. A.H.K. and I.T.R. performed the numerical simulations and analysis used for characterizing the system and validating the experimental results, with assistance from A.D.P. and Y.Y. A.H.K. and C.N.B. developed the experiment control tools used in this work. A.H.K., C.N.B., A.D.P., L.D., P.M.H., M.H. and J.A.G. contributed to the experimental setup. S.E.M., P.M.H. and M.S. designed the 4 × 4 qubit array. R.D., D.K.K. and B.M.N. fabricated the 4 × 4 flip-chip sample, with coordination from K.S., M.E.S. and J.L.Y. S.G., J.A.G. and W.D.O. provided experimental oversight and support. All authors contributed to the discussions of the results and to the development of the manuscript.
Corresponding authors
Correspondence to Amir H. Karamlou or William D. Oliver .
Ethics declarations
Competing interests.
The authors declare no competing interests.
Peer review
Peer review information.
Nature thanks Anurag Anshu, Alex Ma and the other, anonymous, reviewer(s) for their contribution to the peer review of this work.
Additional information
Publisher’s note Springer Nature remains neutral with regard to jurisdictional claims in published maps and institutional affiliations.
Extended data figures and tables
Extended data fig. 1 entanglement scaling at different particle-number subspaces..
a , Subsystem entropy versus volume of states at the centre and edge of the energy band for subspaces with n = 5, 6, 7 and 8 particles. b , Geometric entropy ratio s V / s A comparison for different particle-number subspaces.
Extended Data Fig. 2 Simulation of the measurement sampling statistics problem.
The dark markers present the second Rényi entropy extracted from simulated tomography of subsystems as a function of the number of measurement samples of each Pauli string used for density-matrix reconstruction. For each subsystem volume, the results were averaged over the same subsystems used in the experiment. The probability distribution of measurement outcomes was determined from the simulated state prepared at Ω = J /2 and δ = 0. The light dashed lines represent the exact entropy of the simulated state at each volume. a , Density matrices are reconstructed from the Stokes parameters obtained from Monte Carlo sampling of the probability distribution of eigenvalues of Pauli operator strings. b , Density matrices are reconstructed using maximum-likelihood estimation on the bitstrings obtained from Monte Carlo sampling of the probability distribution of measurement outcomes of each Pauli operator string. Maximum-likelihood estimation is not used in a , whereas the simulation in b uses the same reconstruction procedure that was used for experimental data. c , The entropy extracted from Monte Carlo sampling of the simulated state at Ω = J /2 and δ = 0 (crosses) is compared with the entropy extracted from experimental data (circles) (Fig. 3c ). The values show the mean entropy of all subsystems at each volume. The exact entropy of the simulated state is shown for comparison (dashed line).
Supplementary information
Supplementary information, rights and permissions.
Open Access This article is licensed under a Creative Commons Attribution 4.0 International License, which permits use, sharing, adaptation, distribution and reproduction in any medium or format, as long as you give appropriate credit to the original author(s) and the source, provide a link to the Creative Commons licence, and indicate if changes were made. The images or other third party material in this article are included in the article’s Creative Commons licence, unless indicated otherwise in a credit line to the material. If material is not included in the article’s Creative Commons licence and your intended use is not permitted by statutory regulation or exceeds the permitted use, you will need to obtain permission directly from the copyright holder. To view a copy of this licence, visit http://creativecommons.org/licenses/by/4.0/ .
Reprints and permissions
About this article
Cite this article.
Karamlou, A.H., Rosen, I.T., Muschinske, S.E. et al. Probing entanglement in a 2D hard-core Bose–Hubbard lattice. Nature (2024). https://doi.org/10.1038/s41586-024-07325-z
Download citation
Received : 09 June 2023
Accepted : 15 March 2024
Published : 24 April 2024
DOI : https://doi.org/10.1038/s41586-024-07325-z
Share this article
Anyone you share the following link with will be able to read this content:
Sorry, a shareable link is not currently available for this article.
Provided by the Springer Nature SharedIt content-sharing initiative
By submitting a comment you agree to abide by our Terms and Community Guidelines . If you find something abusive or that does not comply with our terms or guidelines please flag it as inappropriate.
Quick links
- Explore articles by subject
- Guide to authors
- Editorial policies
Sign up for the Nature Briefing newsletter — what matters in science, free to your inbox daily.

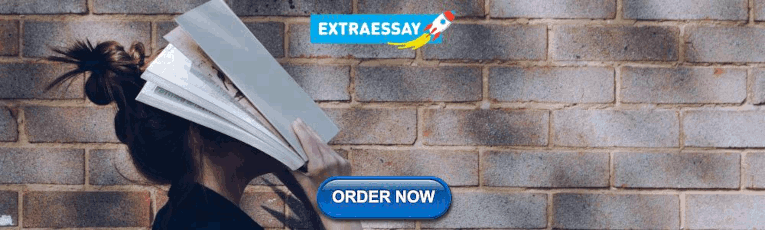
IMAGES
VIDEO
COMMENTS
Millions of people have limited or no access to electrical energy. A diverse group of scholars and activists, from individual researchers to nongovernmental organizations (NGOs), civil society organizations, UN, and increasingly to engineering and financial private sector firms, have been working on initiatives to address this issue, which is considered by many a human rights problem. Energy ...
The Electricity Journal is the leading journal in electric power policy. The journal deals primarily with fuel diversity and the energy mix needed for optimal energy market performance, and therefore covers the full spectrum of energy, from coal, nuclear, natural gas and oil, to renewable energy …. View full aims & scope.
Abstract and Figures. This paper presents a discussion of the future of the electric energy system, addressing the entire spectrum from power generation, through substations, to distribution and ...
electricity energy-saving behaviour were either experiments or surveys based. In this study, we seek to mitigate some of the shortcomings in the previous research are: first, the. experimental ...
A total of eighty-one (81) state-of-the-art research works published in journals, conferences, and magazines, and student's thesis relevant to the scope of the current study were downloaded from the internet, thus using keywords and terms which included AI, Electricity Prediction (EP), Energy Forecasting (EF), Machine Learning (ML), and combination of AI and EP, AI and EF, ML and EP, ML and EF.
Electric Power Systems Research is an international medium for the publication of original papers concerned with the generation, transmission, distribution and utilization of electrical energy. The journal aims at presenting important results of work in this field, whether in the form of applied research, development of new procedures or ...
Therefore, in this paper, qualitative methods are used to provide a more comprehensive understanding of the impacts of power sector policies and their effectiveness. ... It provided for the creation of a National Renewable Energy Authority, the promotion of research and development in the renewable energy sector, and the establishment of a ...
1 INTRODUCTION. Energy is inevitable for the development and improvement of our lifestyles. 1 The demand for energy is growing day by day. 2-4 In 2013, the use of energy all over the world was 532.9 × 10 18 J equivalent which was almost twice the energy utilization in 1980 (277.5 × 10 18 J). 5 In 2018, world energy consumption increases remarkably surging for continuous economic and ...
Feature papers represent the most advanced research with significant potential for high impact in the field. A Feature Paper should be a substantial original Article that involves several techniques or approaches, provides an outlook for future research directions and describes possible research applications. ... Electricity is an international ...
This paper preliminarily establishes the informational linkage between rock failure and energy release, analyzing the rock samples over time and space. ... provides an outlook for future research directions and describes possible research applications. Feature papers are submitted upon individual invitation or recommendation by the scientific ...
The aim of the paper is to ascertain if renewable energy sources are sustainable and examine how a shift from fossil fuel-based energy sources to renewable energy sources would help reduce climate change and its impact. A qualitative research was employed by reviewing peer-reviewed papers in the area of study.
1 INTRODUCTION. Due to the increase in world population, development in industrial activities, and enhancement in living standards, the human demand for electricity will grow in the future years. 1 Traditional fossil fuels such as oil and coal cause carbon dioxide emissions and global warming. 2 Thus, it is necessary to explore appropriate alternatives sources for electricity generation which ...
Forecasting has been an essential part of the power and energy industry. Researchers and practitioners have contributed thousands of papers on forecasting electricity demand and prices, and renewable generation (e.g., wind and solar power). This article offers a brief review of influential energy forecasting papers; summarizes research trends; discusses importance of reproducible research and ...
Electricity is a fundamental necessity in modern society, powering a wide range of daily activities and technologies [1], [2]. The generation of electrical energy involves various sources, such as ...
The appraising emanation and extension of renewable energy in contemporary scenario spatulation of electricity through natural resources (wind, solar, hydropower) has proliferate. In 2022, 41.4% of energy proportions stationed is through renewable sources. In accordance with statistics only 3.2% of the summation electricity is being generated by nuclear sources in 2021. Because of ...
This Review summarizes latest advancements in memristor-based hardware accelerators, an energy-efficient solution for computing-intensive artificial intelligence algorithms, covering crossbar ...
This review paper focuses on the connection between academic solar energy research and its practical real-world implications. It examines the current state of solar power and related academic solar energy research in different countries, aiming to provide valuable guidance for researchers, designers, and policymakers interested in incorporating ...
Research Papers Publications. OIES publishes full-length and in-depth Research Papers which take a longer view on the most important issues in international energy debates, aiming to contribute new policy ideas or new empirical work to the body of existing knowledge.
E Electricity stability is the key component in ensuring reliable power supply which is a major hurdle in most developing countries. ... The Research paper has to try to perceive the proposed ...
The sources of renewable energy which could serve as an alternative source of power include wind, solar, and biogas. These can also be combined together as an entity to form a hybrid renewable energy source. In this paper, a solar tree was designed and constructed to provide an alternative supply of electricity to farm settlements in Nigeria.
Srinivasan is a senior research analyst in our offshore wind team, involved in economic analysis with a particular focus on asset valuation, LCOE forecasts and market research. He also works on the cost modelling of offshore wind energy, analysing how technological advancements, performance and macroeconomic factors translate into cost impacts.
The use of renewable energy resources, such as solar, wind, and biomass will not diminish their availability. Sunlight being a constant source of energy is used to meet the ever-increasing energy need. This review discusses the world's energy needs, renewable energy technologies for domestic use, and highlights public opinions on renewable energy. A systematic review of the literature was ...
Provides world energy statistics. Covers energy supply and consumption for over 100 countries and regions. Energydata.info. Provided by The World Bank Group, Energydata.info is an open data platform that provides access to datasets and data analytics relevant to the energy sector.
2.1. Research questions. The key motivation of this systematic literature review is to gather, analyze and explore existing methods presented for electricity management all over the world. We summarize and use information obtained through this review for development of an electricity management system as a second step.
Abstract. Research Question: This paper presents a review of empirical methods used by authors to determine the influence of different groups of factors that influence households' electricity ...
This material is based on work supported in part by the U.S. Department of Energy, Office of Science, National Quantum Information Science Research Centers, Quantum Systems Accelerator (QSA); in ...
Paper study on the technological and economic opportunities to transition a retired coal plant to small nuclear plants or battery storage site. ... CX-030159: Integrated Education and Research in Energy Transition for Communities March 8, 2024. Office of NEPA Policy and Compliance;
waste to electricity area. Every year, approximat ely fift y-five million tons of. municipal stable waste (MSW) and 38 billion liters of sewage. are generated in the urban areas of India. B esides ...
The Pembina Institute, an energy research center, ... While this paper adopts a multi-pronged approach towards defining net-zero greenwashing in an effort to capture current practices, future research is warranted to examine whether an expanded conception of greenwashing is needed for future investigations. The current focus on advertising ...