
An official website of the United States government
The .gov means it’s official. Federal government websites often end in .gov or .mil. Before sharing sensitive information, make sure you’re on a federal government site.
The site is secure. The https:// ensures that you are connecting to the official website and that any information you provide is encrypted and transmitted securely.
- Publications
- Account settings
Preview improvements coming to the PMC website in October 2024. Learn More or Try it out now .
- Advanced Search
- Journal List
- PLoS Comput Biol
- v.19(12); 2023 Dec
- PMC10703290

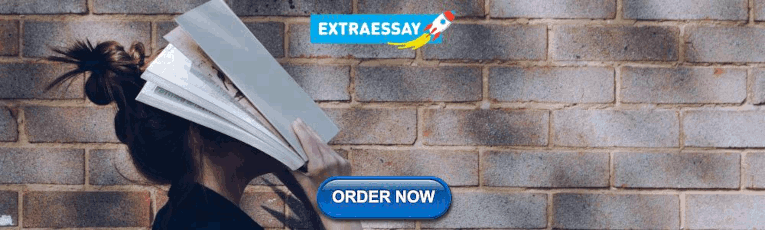
Ten simple rules for managing laboratory information
Casey-tyler berezin.
1 Department of Chemical and Biological Engineering, Colorado State University, Fort Collins, Colorado, United States of America
Luis U. Aguilera
Sonja billerbeck.
2 Molecular Microbiology Unit, Faculty of Science and Engineering, University of Groningen, Groningen, the Netherlands
Philip E. Bourne
3 School of Data Science, University of Virginia, Charlottesville, Virginia, United States of America
4 Department of Biomedical Engineering, University of Virginia, Charlottesville, Virginia, United States of America
Douglas Densmore
5 College of Engineering, Boston University, Boston, Massachusetts, United States of America
Paul Freemont
6 Department of Infectious Disease, Imperial College, London, United Kingdom
Thomas E. Gorochowski
7 School of Biological Sciences, University of Bristol, Bristol, United Kingdom
8 BrisEngBio, University of Bristol, Bristol, United Kingdom
Sarah I. Hernandez
Nathan j. hillson.
9 Biological Systems and Engineering Division, Lawrence Berkeley National Laboratory, Berkeley, California, United States of America
10 US Department of Energy Agile BioFoundry, Emeryville, California, United States of America
11 US Department of Energy Joint BioEnergy Institute, Emeryville, California, United States of America
Connor R. King
Michael köpke.
12 LanzaTech, Skokie, Illinois, United States of America
13 Center for Global Infectious Disease Research, Seattle Children’s Hospital, University of Washington Medicine, Seattle, Washington, United States of America
Katie M. Miller
Tae seok moon.
14 Department of Energy, Environmental & Chemical Engineering, Washington University in St. Louis, St. Louis, Missouri, United States of America
Jason H. Moore
15 Department of Computational Biomedicine, Cedars-Sinai Medical Center, Los Angeles, California, United States of America
Brian Munsky
Chris j. myers.
16 Department of Electrical, Computer & Energy Engineering, University of Colorado Boulder, Boulder, Colorado, United States of America
Dequina A. Nicholas
17 Department of Molecular Biology & Biochemistry, University of California Irvine, Irvine, California, United States of America
Samuel J. Peccoud
18 Department of Electrical and Computer Engineering, Colorado State University, Fort Collins, Colorado, United States of America
19 Department of Statistics, Colorado State University, Fort Collins, Colorado, United States of America
Jean Peccoud
Author summary.
Information is the cornerstone of research, from experimental (meta)data and computational processes to complex inventories of reagents and equipment. These 10 simple rules discuss best practices for leveraging laboratory information management systems to transform this large information load into useful scientific findings.
Introduction
The development of mathematical models that can predict the properties of biological systems is the holy grail of computational biology [ 1 , 2 ]. Such models can be used to test biological hypotheses [ 3 ], quantify the risk of developing diseases [ 3 ], guide the development of biomanufactured products [ 4 ], engineer new systems meeting user-defined specifications, and much more [ 4 , 5 ]. Irrespective of a model’s application and the conceptual framework used to build it, the modeling process proceeds through a common iterative workflow. A model is first evaluated by fitting its parameters such that its behavior matches experimental data. Models that fit previous observations are then further validated by comparing the model predictions with the results of new observations that are outside the scope of the initial data set ( Fig 1 ).

Our 10 simple rules for managing laboratory information (green) augment the cycle of hypothesis formulation, design, data analysis, modeling, and decision-making (gray). The experimental design phase is improved by carefully tracking your inventory, samples, parameters, and variables. Proactive data management and the thoughtful use of databases facilitate statistical and exploratory analyses as well as the development of conclusions that inform the next round of experiments. Frequent reevaluation of project, team, and workflow success is a critical component of refining experimental processes, developing a common culture, and positioning your research group in the greater scientific context.
Historically, the collection of experimental data and the development of mathematical models were performed by different scientific communities [ 6 ]. Computational biologists had little control over the nature and quality of the data they could access. With the emergence of systems biology and synthetic biology, the boundary between experimental and computational biology has become increasingly blurred [ 6 ]. Many labs and junior scientists now have expertise in both producing and analyzing large volumes of digital data produced by high-throughput workflows and an ever-expanding collection of digital instruments [ 7 ]. In this context, it is critically important to properly organize the exponentially growing volumes of experimental data to ensure they can support the development of models that can guide the next round of experiments [ 8 ].
We are a group of scientists representing a broad range of scientific specialties, from clinical research to industrial biotechnology. Collectively, we have expertise in experimental biology, data science, and mathematical modeling. Some of us work in academia, while others work in industry. We have all faced the challenges of keeping track of laboratory operations to produce high-quality data suitable for analysis. We have experience using a variety of tools, including spreadsheets, open-source software, homegrown databases, and commercial solutions to manage our data. Irreproducible experiments, projects that failed to meet their goals, datasets we collected but never managed to analyze, and freezers full of unusable samples have taught us the hard way lessons that have led to these 10 simple rules for managing laboratory information.
This journal has published several sets of rules regarding best practices in overall research design [ 9 , 10 ], as well as the computational parts of research workflows, including data management [ 11 – 13 ] and software development practices [ 14 – 16 ]. The purpose of these 10 rules ( Fig 1 ) is to guide the development and configuration of lab information management systems (LIMS). LIMS typically offer lab notebook, inventory, workflow planning, and data management features, allowing users to connect data production and data analysis to ensure that useful information can be extracted from experimental data and increase reproducibility [ 17 , 18 ]. These rules can also be used to develop training programs and lab management policies. Although we all agree that applying these rules increases the value of the data we produce in our laboratories, we also acknowledge that enforcing them is challenging. It relies on the successful integration of effective software tools, training programs, lab management policies, and the will to abide by these policies. Each lab must find the most effective way to adopt these rules to suit their unique environment.
Rule 1: Develop a common culture
Data-driven research projects generally require contributions from multiple stakeholders with complementary expertise. The project’s success depends on the entire team developing a common vision of the project objectives and the approaches to be used [ 19 – 21 ]. Interdisciplinary teams, in particular, must establish a common language as well as mutual expectations for experimental and publication timelines [ 19 ]. Unless the team develops a common culture, one stakeholder group can drive the project and impose its vision on the other groups. Although interdisciplinary (i.e., wet-lab and computational) training is becoming more common in academia, it is not unusual for experimentalists to regard data analysis as a technique they can acquire simply by hiring a student with computer programming skills. In a corporate environment, research informatics is often part of the information technology group whose mission is to support scientists who drive the research agenda. In both situations, the research agenda is driven by stakeholders who are unlikely to produce the most usable datasets because they lack sufficient understanding of data modeling [ 20 ]. Perhaps less frequently, there is also the situation where the research agenda is driven by people with expertise in data analysis. Because they may not appreciate the subtleties of experimental methods, they may find it difficult to engage experimentalists in collaborations aimed at testing their models [ 20 ]. Alternatively, their research may be limited to the analysis of disparate sets of previously published datasets [ 19 ]. Thus, interdisciplinary collaboration is key to maximizing the insights you gain from your data.
The development of a common culture, within a single laboratory or across interdisciplinary research teams, must begin with a thorough onboarding process for each member regarding general lab procedures, research goals, and individual responsibilities and expectations [ 21 , 22 ]. Implementing a LIMS requires perseverance by users, thus a major determinant of the success of a LIMS is whether end-users are involved in the development process [ 17 , 23 ]. When the input and suggestions of end-users are considered, they are more likely to engage with and upkeep the LIMS on a daily basis [ 23 ]. The long-term success of research endeavors then requires continued training and reevaluation of project goals and success [ 19 , 21 ] ( Fig 1 ).
These 10 simple rules apply to transdisciplinary teams that have developed a common culture allowing experimentalists to gain a basic understanding of the modeling process and modelers to have some familiarity with the experimental processes generating the data they will analyze [ 19 ]. Teams that lack a common vision of data-driven research are encouraged to work toward acquiring this common vision through frequent communication and mutual goal setting [ 19 , 20 ]. Discussing these 10 simple rules in group meetings may aid in initiating this process.
Rule 2: Start with what you purchase
All the data produced by your lab are derived from things you have purchased, including supplies (consumables), equipment, and contract manufactured reagents, such as oligonucleotides or synthetic genes. In many cases, (meta)data on items in your inventory may be just as important as experimentally derived data, and as such, should be managed according to the Findability, Accessibility, Interoperability, and Reuse (FAIR) principles for (meta)data management ( https://www.go-fair.org/fair-principles/ ) [ 24 ]. Assembling an inventory of supplies and equipment with their associated locations can be handled in a few weeks by junior personnel without major interruption of laboratory operations, although establishing a thorough inventory may be more difficult and time-consuming for smaller labs with fewer members. Nevertheless, managing your lab inventory provides an immediate return on investment by positively impacting laboratory operations in several ways. People can quickly find the supplies and equipment they need to work, supplies are ordered with appropriate advance notice to minimize work stoppage, and data variation is reduced due to standardized supplies and the ability to track lot numbers easily [ 17 , 25 , 26 ] ( Fig 1 ).
Many labs still use Excel to keep track of inventory despite the existence of several more sophisticated databases and LIMS (e.g., Benchling , Quartzy , GenoFAB , LabWare , LabVantage , TeselaGen ) [ 25 ]. These can facilitate real-time inventory tracking unlike a static document, increasing the Findability and Accessibility of inventory data. While some systems are specialized for certain types of inventories (e.g., animal colonies or frozen reagents), others are capable of tracking any type of reagent or item imaginable [ 25 ]. When considering what items to keep track of, there are 3 main considerations: expiration, maintenance, and ease of access.
Most labs manage their supplies through periodic cleanups of the lab, during which they sort through freezers, chemical cabinets, and other storage areas; review their contents; and dispose of supplies that are past their expiration date or are no longer useful. By actively tracking expiration dates and reagent use in a LIMS, you can decrease the frequency of such cleanups since the LIMS will alert users when expiration dates are approaching or when supplies are running low. This can prevent costly items from being wasted because they are expired or forgotten, and furthermore, the cost of products can be tracked and used to inform which experiments are performed.
LIMS can also support the use and service of key laboratory equipment. User manuals, service dates, warranties, and other identifying information can be attached directly to the equipment record, which allows for timely service and maintenance of the equipment. Adding equipment to the inventory can also prevent accidental losses in shared spaces where it is easy for people to borrow equipment without returning it. The label attached to the equipment (Rule 5) acts as an indication of ownership that limits the risk of ownership confusion when almost identical pieces of equipment are owned by neighboring laboratories. As the laboratory inventory should focus on larger, more expensive equipment and supplies, inexpensive and easily obtained equipment (i.e., office supplies) may not need to be inventoried. An additional benefit of inventory management in a LIMS is the ability to create a record connecting specific equipment and supplies to specific people and projects, which can be used to detect potential sources of technical bias and variability (Rules 4 and 5).
Rule 3: Focus on your current projects first
After establishing an inventory of supplies and equipment, it is natural to consider using a similar approach with the samples that have accumulated over the years in freezers or other storage locations. This can be overwhelming because the number of samples will be orders of magnitude larger than the number of supplies. In addition, documenting them is likely to require more effort than simply retrieving a product documentation from a vendor’s catalog.
Allocating limited resources to making an inventory of samples generated by past projects may not benefit current and future projects. A more practical approach is to prioritize tracking samples generated by ongoing projects and document samples generated by past projects on an as-needed basis.
Inventory your samples before you generate them
It is a common mistake to create sample records well after they were produced in the lab. The risks of this retroactive approach to recordkeeping include information loss, as well as selective recordkeeping, in which only some samples are deemed important enough to document while most temporary samples are not, even though they may provide critical information.
A more proactive approach avoids these pitfalls. When somebody walks into a lab to start an experiment, the samples that will be generated by this experiment should be known. It is possible to create the computer records corresponding to these samples before initiating the laboratory processes that generates the physical samples. The creation of a sample record can therefore be seen as part of the experiment planning process ( Fig 1 ). This makes it possible to preemptively print labels that will be used to track samples used at different stages of the process (Rule 5).
It may also be useful to assign statuses to samples as they progress through different stages of their life cycle, such as “to do,” “in progress,” “completed,” or “canceled,” to differentiate active works in progress from the backlog and samples generated by previous experiments. As the experimental process moves forward, data can be continually appended to the sample computer record. For example, the field to capture the concentration of a solution would be filled after the solution has been prepared. Thus, the success, or failure, of experiments can be easily documented and used to inform the next round of experiments.
Develop sample retention policies
It is always unpleasant to have to throw away poorly documented samples. The best strategy to avoid this outcome is to develop policies to discard only samples that will not be used in the future, a process rendered more objective and straightforward with adequate documentation. Properly structured workflows (Rule 8) should define criteria for which samples should be kept and for how long. All lab members should be trained in these policies to ensure consistency, and policies should be revisited as new research operating procedures are initiated.
It can be tempting to keep every tube or plate that still contains something as a backup. This conservative strategy generates clutter, confusion, and reproducibility issues, especially in the absence of documentation. While it makes sense to keep some intermediates during the execution of a complex experimental workflow, the successful completion of the experiment should trigger the elimination of intermediates that have lost their purpose, have limited shelf life, and/or are not reusable. During this intermediate step, samples that are deemed as critical backup should be stored in a separate location from the working sample to minimize the likelihood of loss of both samples in case of electrical failure, fire, etc. Using clear labels (Rules 4 and 5) and storing intermediate samples in dedicated storage locations can help with the enforcement of sample disposal policies.
Rule 4: Use computer-generated sample identification numbers
Generating sample names is probably not the best use of scientists’ creativity. Many labs still rely on manually generated sample names that may look something like “JP PCR 8/23 4.” Manually generated sample names are time-consuming to generate, difficult to interpret, and often contain insufficient information. Therefore, they should not be the primary identifier used to track samples.
Instead, computer-generated sample identification numbers (Sample ID) should be utilized as the primary ID as they are able to overcome these limitations. Rather than describing the sample, a computer-generated sample ID provides a link between a physical sample and a database entry that contains more information associated with the sample. The Sample ID is the only piece of information that needs to be printed on the sample label (Rule 5) because it allows researchers to retrieve all the sample information from a database. A sample tracking system should rely on both computer-readable and human-readable Sample IDs.
Computer-readable IDs
Since the primary purpose of a sample ID is to provide a link between a physical sample and the computer record that describes the sample, it saves time to rely on Sample IDs that can be scanned by a reader or even a smartphone [ 27 , 28 ] ( Fig 2 ). Barcodes are special fonts to print data in a format that can be easily read by an optical sensor [ 29 ]. There are also newer alternatives, such as quick response (QR) codes, data matrices, or radio-frequency identification (RFID), to tag samples [ 30 , 31 ]. QR codes and data matrices are 2D barcodes that are cheaper to generate than RFID tags and store more data than traditional barcodes [ 27 ]. Nevertheless, these technologies encode a key that points to a database record.

The first line includes a unique computer-readable barcode as well as a human-readable computer-generated sample identification number. The second and third lines include a description of the sample content, the date, and the identity of the inoculum.
Uniqueness is the most important property of the data encoded in barcodes, and the use of unique and persistent identifiers is a critical component of the Findability of your (meta)data [ 24 ]. Several vendors now offer products with 2D barcodes printed on the side or bottom of the tube. It is common for such products, as well as lab-made reagents, to be split across multiple tubes or moved from one tube to another. In these cases, each of these “new” samples should have unique barcodes. A barcoding system can therefore facilitate the accurate identification of “parent” samples (e.g., a stock solution with ID X) and the unique “child” samples derived from them (e.g., aliquots of the stock solution with IDs Y and Z).
Human-readable IDs
While computer-readable IDs should be the main ID used when tracking a sample or supply, it is sometimes necessary for laboratory personnel to have a secondary sample ID they can read without the use of any equipment or while doing manual operations (i.e., handling samples).
To make an identifier readable by humans, it is best to keep the ID short and use their structure to provide contextual information. For example, the use of a prefix may help interpret the ID. For example, the ID #CHEM1234 would naturally be interpreted as a chemical or #MCUL2147 as a mammalian culture ( Fig 2 ).
Since these identifiers do not need to map to a unique database entry, human-readable IDs do not have the same uniqueness requirements as computer-readable IDs. For example, it may be acceptable to allow 2 labs using the same software environment to use the same human-readable ID, because this ID will only be seen in the context of a single lab. The software system should maintain the integrity of the relationships between the human-readable ID and the computer-readable ID by preventing users from editing these identifiers.
Rule 5: Label everything
Print labels to identify supplies, equipment, samples, storage locations, and any other physical objects used in your lab. Many labs are extensively relying on handwritten markings that create numerous problems [ 17 ]. A limited amount of information can be written on small sample containers, and like manually generated sample names, handwritten labels can be difficult to read or interpret.
Some labels are self-contained. For example, shipping labels include all the information necessary to deliver a package. However, in a laboratory environment, a sample label must not only identify a physical sample but also establish a connection to a record describing the sample and the data associated with it ( Fig 2 ).
Content of a label
Only 2 pieces of information are necessary on a label: a computer-readable Sample ID printed as a barcode and a human-readable Sample ID to make it easier for the researcher to work with the sample. If there is enough space to print more information on the label, your research needs should inform your label design. Ensure you have sufficient space to meet regulatory labeling requirements (e.g., biosafety requirements, hazards) and if desired, information such as the sample type, sample creator, date (e.g., of generation or expiration), or information about related samples (e.g., parent/child samples).
Label printing technology
Putting in place a labeling solution requires the integration of several elements, but once configured, proper use of label printing technologies makes it much faster and easier to print labels than to label tubes manually.
There are many types of label printers on the market today and most are compatible with the Zebra Programming Language (ZPL) standard [ 32 ]. Labeling laboratory samples can be challenging due to harsh environmental conditions: exposure to liquid nitrogen or other chemicals, as well as ultra-low or high temperatures, will require specialized labels. For labeling plastic items, thermal transfer will print the most durable labels, especially if used with resin ribbon instead of wax, while inkjet printers can print durable labels for use on paper [ 33 – 35 ]. Furthermore, laboratory samples can be generated in a broad range of sizes, so labels should be adapted to the size of the object they are attached to. A high-resolution printer (300 dpi or greater) will make it possible to print small labels that will be easy to read by humans and scanners. Finally, select permanent or removable labels based on the application. Reusable items should be labeled with removable labels, whereas single-use containers are best labeled with permanent labels.
Label printing software applications can take data from a database or a spreadsheet and map different columns to the fields of label templates, helping to standardize your workflows (Rule 8). They also support different formats and barcode standards. Of course, the label printing software needs to be compatible with the label printer. When selecting a barcode scanner, consider whether it supports the barcode standards that will be used in your label, as well as the size and shape of the barcodes it can scan. Inexpensive barcode scanners will have difficulty reading small barcodes printed on curved tubes with limited background, whereas professional scanners with high performance imagers will effectively scan more challenging labels. When used, barcode scanners transmit a unique series of characters to the computer. How these characters are then used depends on the software application in which the barcode is read. Some applications will simply capture the content of the barcode. Other applications will process barcoded data in real-time to retrieve the content of the corresponding records.
Rule 6: Manage your data proactively
Many funding agencies now require investigators to include a data management and sharing plan with their research proposals [ 36 , 37 ], and journals have data-sharing policies that authors need to uphold [ 38 ]. However, the way many authors share their data indicates a poor understanding of data management [ 39 , 40 ]. Data should not be managed only when publishing the results of a project, they should be managed before the data collection starts [ 41 ]. Properly managed data will guide project execution by facilitating analysis as data gets collected ( Fig 1 ). Projects that do not organize their data will face difficulties during analysis, or worse, a loss of critical information that will negatively impact progress.
Use databases to organize your data
It can be tempting to only track data files through notebook entries or dump them in a shared drive (more in Rule 9). That simple data management strategy makes it very difficult to query data that may be spread across multiple files or runs, especially because a lot of contextual information must be captured in file names and directory structures using conventions that are difficult to enforce. Today, most data are produced by computer-controlled instruments that export tabular data (i.e., rows and columns) that can easily be imported into relational databases. Data stored in relational databases (e.g., MySQL ) are typically explored using standard query language (SQL) and can be easily analyzed using a variety of statistical methods ( Table 1 ). There are also no-code and low-code options, such as the Open Science Framework ( https://osf.io/ ) [ 42 ], AirTable , and ClickUp , which can also be used to track lab processes, develop standardized workflows, manage teams, etc.
*These technologies have been dubbed “lakehouses” that integrate components of data lakes and data warehouses.
In the age of big data applications enabled by cloud computing infrastructures, there are more ways than ever to organize data. Today, NoSQL (not only SQL) databases [ 43 – 45 ], data lakes [ 46 – 48 ], and data warehouses [ 49 , 50 ] provide additional avenues to manage complex sets of data that may be difficult to manage in relational databases ( Table 1 ). All these data management frameworks make it possible to query and analyze data, depending on the size, type, and structure of your data as well as your analysis goals. NoSQL databases can be used to store and query data that is unstructured or otherwise not compatible with relational databases. Different NoSQL databases implement different data models to choose from depending on your needs ( Table 1 ). Data lakes are primarily used for storing large-scale data with any structure. It is easy to input data into a data lake, but metadata management is critical for organizing, accessing, and interrogating the data. Data warehouses are best suited for storing and analyzing large-scale structured data. They are often SQL-like and are sometimes optimized for specific analytical workflows. These technologies are constantly evolving and the overlap between them is growing as captured in the idea of “lakehouses” such as Databricks and Snowflake Data Cloud ( https://www.snowflake.com/en/ ) ( Table 1 ).
When choosing a data management system, labs must consider the trade-off between the cost of the service and the accessibility of the data (i.e., storage in a data lake may be cheaper than in a data warehouse, but retrieving/accessing the data may be more time-consuming or costly) [ 51 ]. Many companies offer application programming interfaces (API) to connect their instruments and/or software to databases. In addition, new domain-specific databases continue to be developed [ 52 ]. If necessary, it is also possible to develop your own databases for particular instruments or file types [ 53 ]. Nevertheless, when uploading your data to a database, it is recommended to import them as interoperable nonproprietary file types (e.g., .csv instead of .xls for tabular data; .gb (GenBank flat file https://www.ncbi.nlm.nih.gov/genbank/ ) instead of .clc (Qiagen CLC Sequence Viewer format [ 54 ]) for gene annotation data; see Rule 4 of [ 51 ] for more), so that the data can be accessed if a software is unavailable for any reason and to facilitate date sharing using tools such as git (Rule 10) [ 14 , 24 ].
Link data to protocols
One of the benefits of data organization is the possibility of capturing critical metadata describing how the data were produced. Many labs have spent years refining protocols to be used in different experiments. Many of these protocols have minor variations that can significantly alter the outcome of an experiment. If not properly organized, this can cause major reproducibility issues and can be another uncontrolled source of technical variation. By linking protocol versions to the associated data that they produced (ideally all the samples generated throughout the experiment), it is possible to use this metadata to inform data reproducibility and analysis efforts.
Capture context in notebook entries
Organizing data in databases and capturing essential metadata describing the data production process can greatly simplify the process of documenting research projects in laboratory notebooks [ 55 ]. Instead of needing to include copies of the protocols and the raw data produced by the experiment, the notebook entry can focus on the context, purpose, and results of the experiment. In the case of electronic lab notebooks (ELNs; e.g., SciNote , LabArchives , and eLabJournal ), entries can benefit from providing links to previous notebook entries, the experimental and analytical protocols used, and the datasets produced by the workflows. ELNs also bring additional benefits like portability, standardized templates, and improved reproducibility. Finally, notebook entries should include the interpretation of the data as well as a conclusion pointing to the next experiment. The presence of this rich metadata and detailed provenance is critical to ensuring the FAIR principles are being met and your experiments are reproducible [ 24 ].
Rule 7: Separate parameters and variables
Not all the data associated with an experiment are the same. Some data are controlled by the operator (i.e., parameters), whereas other data are observed and measured (i.e., variables). It is necessary to establish a clear distinction between set parameters and observed variables to improve reproducibility and analysis.
When parameters are not clearly identified, lab personnel may be tempted to change parameter values every time they perform experiments, which will increase the variability of observations. If, instead, parameter values are clearly identified and defined, then the variance of the observations produced by this set of parameters should be smaller than the variance of the observations produced using different parameter values.
Separating and recording the parameters and variables associated with an experiment makes it possible to build statistical models that compare the observed variables associated with different parameter values [ 41 , 56 ]. It also enables researchers to identify and account for both the underlying biological factors of interest (e.g., strain, treatment) and the technical and random (noise) sources of variation (e.g., batch effects) in an experiment [ 56 ].
Utilizing metadata files is a convenient way of reducing variability caused by parameter value changes. A metadata file should include all the parameters needed to perform the same experiment with the same equipment. In an experimental workflow, pairing a metadata file with the quantified dataset is fundamental to reproducing the same experiment later [ 51 , 55 , 57 ]. Additionally, metadata files allow the user to assess whether multiple experiments were performed using the same parameters.
Rule 8: Standardize your workflows
Track your parameters from beginning to end.
Experimental parameters have a direct influence on observations. However, some factors may have indirect effects on observations or affect observations further downstream in a pipeline. For example, the parameters of a DNA purification process may indirectly influence the quality of sequencing data derived from the extracted DNA.
To uncover such indirect effects, it is necessary to capture the sequence of operations in workflows. For the above example, this would include the DNA extraction, preparation of the sequencing library, and the sequencing run itself. When dealing with such workflows, it is not possible to use a single Sample ID as the key connecting different datasets as in Rule 4. The workflow involves multiple samples (i.e., the biological sample or tissue, the extracted DNA, the sequencing library) that each have their own identifier. Comprehensive inventory and data management systems will allow you to track the sample lineage and flows of data produced at different stages of an experimental process.
Recording experimental parameters and workflows is especially critical when performing new experiments, since they are likely to change over time. As they are finalized, this information can be used to develop both standardized templates for documenting your workflow, as well as metrics for defining the success of each experiment, which can help you to optimize your experimental design and data collection efforts ( Fig 1 ).
Document your data processing pipeline
After the experimental data are collected, it is important to document the different steps used to process and analyze the data, such as if normalization was applied to the data, or if extreme values were not considered in the analyses. The use of ELNs and LIMS can facilitate standardized documentation: creating templates for experimental and analysis protocols can ensure that all the necessary information is collected, thereby improving reproducibility and publication efforts [ 55 , 58 ].
Similarly, thorough source-code documentation is necessary to disseminate your data and ensure that other groups can reproduce your analyses. There are many resources on good coding and software engineering practices [ 14 – 16 , 59 ], so we only touch on a few important points. Developing a “computational narrative” by writing comments alongside your code or using interfaces that allow for markdown (e.g., Jupyter notebooks, R Markdown) can make code more understandable [ 60 – 62 ]. Additionally, using syntax conventions and giving meaningful names to your code variables increases readability (i.e., use average_mass = 10 instead of am = 10). Furthermore, documenting the libraries or software used and their versions is necessary to achieve reproducibility. Finally, implementing a version control system, such as git, protects the provenance of your work and enables collaboration [ 63 ].
Rule 9: Avoid data silos
Depending on your workflows, you may collect information from different instruments or use several databases to store and interact with different types of data. Care must be taken to prevent any of these databases from becoming data silos: segregated groups of data that restrict collaboration and make it difficult to capture insights resulting from data obtained by multiple instruments [ 47 , 49 , 64 ]. Data lakes and data warehouses are good solutions for integrating data silos [ 47 , 49 , 64 ].
Data silos not only stymie research efforts but also raise significant security issues when the silo is the sole storage location. Keeping your data management plan up-to-date with your current needs and utilizing the right databases for your needs can prevent this issue (Rule 6). Regardless, it is crucial to back up your data in multiple places for when a file is corrupted, a service is unavailable, etc. Optimally, your data should always be saved in 3 different locations: 2 on-site and 1 in the cloud [ 51 ]. Of course, precautions should always be taken to ensure the privacy and security of your data online and in the cloud [ 65 , 66 ].
Never delete data
As projects develop and data accumulates, it may be tempting to delete data that no longer seems relevant. Data may also be lost as computers are replaced, research personnel leave, and storage limits are reached. Poorly managed data can be easily lost simply because it is unorganized and difficult to query. However, while data collection remains expensive, data storage continues to get cheaper, so there is little excuse for losing or deleting data today. The exception may be intermediary data that is generated by reproducible data processing pipelines, which can be easily regenerated if and when necessary. Most data files can also be compressed to combat limitations on storage capacity.
Properly organized data is a treasure trove of information waiting to be discovered. By using computer-generated sample IDs (Rule 4) and data lakes/warehouses (Rule 6) to link data collected on different instruments, it is possible to extract and synthesize more information than originally intended in the project design. Data produced by different projects using common workflows (Rule 8) can be analyzed to improve workflow performance. Data from failed experiments can be used to troubleshoot a problem affecting multiple projects.
Rule 10: Place your lab in context
Once you have developed a common culture (Rule 1), inventoried your laboratory (Rules 2 and 3), labeled your samples and equipment with computer-generated IDs (Rules 4 and 5), standardized your parameters and workflows (Rules 7 and 8), and backed up your data in several databases (Rules 6 and 9), what comes next?
Track processes occurring outside the lab
Laboratory operations and the data they produce are increasingly dependent on operations that take place outside of the lab. For example, the results of a PCR assay will be affected by the primer design algorithm and the values of its parameters. They will also be affected by the quality of the primers manufactured by a specialized service provider. Even though the primer design and primer synthesis are not taking place in the lab, they are an integral part of the process of generating PCR data. They should therefore be captured in data flows (Rule 8). Furthermore, the software and computational steps used to design experiments and analyze data they produce must also be properly recorded, to identify as many factors that may affect the data produced in the lab as possible.
Increase the accessibility of your work
There are several ways to place your lab in the greater scientific context and increase reproducibility. As discussed, using standardized, non-proprietary file types can increase ease of access within a lab and across groups [ 14 , 51 ]. You may also choose to make your data and source code public in an online repository to comply with journal requirements, increase transparency, or allow access to your data by other groups [ 67 ]. In addition, data exchange standards, such as the Synthetic Biology Open Language [ 68 , 69 ], increase the accessibility and reproducibility of your work.
Practice makes perfect
Whereas traditional data management methods can restrict your analyses to limited subsets of data, centralized information management systems (encompassing relational and NoSQL databases, metadata, sample tracking, etc.) facilitate the analysis of previously disparate datasets. Given the increasing availability and decreasing cost of information management systems, it is now possible for labs to produce, document, and track a seemingly endless amount of samples and data, and use these to inform their research directions in previously impossible ways. When establishing your LIMS, or incorporating new experiments, it is better to capture more data than less. As you standardize your workflows (Rule 8), you should be able to establish clear metrics defining the success of an experiment and to scale the amount of the data you collect as needed.
While there are plenty of existing ELN and LIMS services to choose from (see Rule 6 and Rule 2, respectively), none are a turnkey solution (S1 Supporting information). All data management systems require configuration and optimization for an individual lab. Each service has its own benefits and limitations your group must weigh. Coupled with the need to store your data with multiple backup options, thoughtful management practices are necessary to make any of these technologies work for your lab. The 10 rules discussed here should provide both a starting place and continued resource in the development of your lab information management system. Remember that developing a LIMS is not a one-time event; all lab members must contribute to the maintenance of the LIMS and document their supplies, samples, and experiments in a timely manner. Although it might be an overwhelming process to begin with, careful data management will quickly benefit the data, users, and lab through saved time, standardized practices, and more powerful insights [ 17 , 18 ].
Conclusions
Imparting a strong organizational structure for your lab information can ultimately save you both time and money if properly maintained. We present these 10 rules to help you build a strong foundation in managing your lab information so that you may avoid the costly and frustrating mistakes we have made over the years. By implementing these 10 rules, you should see some immediate benefits of your newfound structure, perhaps in the form of extra fridge space or fewer delays waiting for a reagent you did not realize was exhausted. In time, you will gain deep insights into your workflows and more easily analyze and report your data. The goal of these rules is also to spur conversation about lab management systems both between and within labs as there is no one-size-fits-all solution for lab management. While these rules provide a great starting point, the topic of how to manage lab information is something that must be a constant dialogue. The lab needs to discuss what is working and what is not working to assess and adjust the system to meet the needs of the lab. This dialogue must also be extended to all new members of the lab as many of these organizational steps may not be intuitive. It is critical to train new members extensively and to ensure that they are integrated into the lab’s common culture or else you risk falling back into bad practices. If properly trained, lab members will propagate and benefit from the organizational structure of the lab.
Funding Statement
J.P., S.H., C.K., K.M., and C.-T.B. are supported by the National Science Foundation (award #2123367) and the National Institutes of Health (R01GM147816, T32GM132057). T.S.M. is supported by the Defense Advanced Research Projects Agency (N660012324032), the Office of Naval Research (N00014-21-1-2206), U.S. Environmental Protection Agency (84020501), the National Institutes of Health (R01 AT009741), and the National Science Foundation (MCB-2001743 and EF-2222403). C.J.M. is supported by the National Science Foundation (MCB-2231864) and the National Institutes Standards and Technology (70NANB21H103). D.A.N. is supported by the National Institutes of Health (R00HD098330 and DP2AI171121). B.M. and L.U.A. are supported by the National Institutes of Health (R35 GM124747). T.E.G. was supported by a Royal Society University Research Fellowship grant UF160357, a Turing Fellowship from The Alan Turing Institute under the EPSRC grant EP/N510129/1. W.Z. is supported by the National Institutes of Health (R01GM144961) and the National Science Foundation (IOS1922701). This work was part of the Agile BioFoundry (agilebiofoundry.org) supported by the U.S. Department of Energy, Energy Efficiency and Renewable Energy, Bioenergy Technologies Office, and was part of the DOE Joint BioEnergy Institute (jbei.org) supported by the U.S. Department of Energy, Office of Science, Office of Biological and Environmental Research, through contract DE-AC02-05CH11231 between Lawrence Berkeley National Laboratory and the U.S. Department of Energy. The views and opinions of the authors expressed herein do not necessarily state or reflect those of the United States Government or any agency thereof. Neither the United States Government nor any agency thereof, nor any of their employees, makes any warranty, expressed or implied, or assumes any legal liability or responsibility or the accuracy, completeness, or usefulness of any information, apparatus, product, or process disclosed or represents that its use would not infringe privately owned rights. The United States Government retains and the publisher, by accepting the article for publication, acknowledges that the United States Government retains a nonexclusive, paid-up, irrevocable, worldwide license to publish or reproduce the published form of this manuscript, or allow others to do so, for United States Government purposes. The Department of Energy will provide public access to these results of federally sponsored research in accordance with the DOE Public Access Plan ( http://energy.gov/downloads/doe-public-access-plan ). The funders had no role in study design, data collection and analysis, decision to publish, or preparation of the manuscript.
Home — Essay Samples — Information Science and Technology — Information Systems — The Laboratory Information Management System

The Laboratory Information Management System
- Categories: Information Systems Project Management
About this sample

Words: 1222 |
Published: Dec 12, 2018
Words: 1222 | Pages: 3 | 7 min read
- Impact of the migration on the continuity of business.
- Managing the transition and user acceptance.
- Configuring and customizing the LIMS.
- The time and cost required for validation and testing of the data and system.
- Workforce requirement for the LIMS.
- Retrieval of the migrated data.
- What is the reason or the motive behind the migration?
- What should be the dedicated period of the migration?
- What should be the optimal costing dedicated towards migration?
- What could be the possible outcomes of the migration?
- How is the migration going to benefit the overall business development and continuity?
- How to select the suitable data for migration?
- Is it necessary to group the data before migration? If yes, on what basis should the grouping be processed?
- Who and how many are going to be the involved workforce?
- Who are the points of contact for both sides of the migration (the vendor and the company)?
- How is the system or transfer going to occur, including the planning, performance and the testing of the new LIMS?
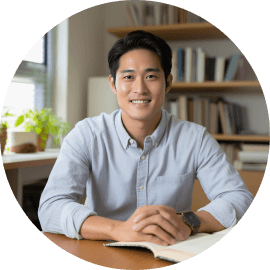
Cite this Essay
Let us write you an essay from scratch
- 450+ experts on 30 subjects ready to help
- Custom essay delivered in as few as 3 hours
Get high-quality help
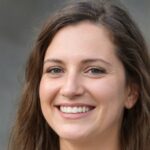
Verified writer
- Expert in: Information Science and Technology Business
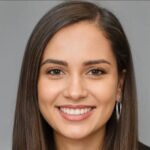
+ 120 experts online
By clicking “Check Writers’ Offers”, you agree to our terms of service and privacy policy . We’ll occasionally send you promo and account related email
No need to pay just yet!
Related Essays
3 pages / 1370 words
3 pages / 1146 words
4 pages / 1806 words
4 pages / 1802 words
Remember! This is just a sample.
You can get your custom paper by one of our expert writers.
121 writers online
Still can’t find what you need?
Browse our vast selection of original essay samples, each expertly formatted and styled
Related Essays on Information Systems
The evolution of the internet has witnessed the transformation of information systems from rudimentary tools of data management to indispensable assets driving organizational competitiveness. Information systems, as a set of [...]
One of the main advantages offered is technology mobility because it allows access to the employees to the organization data, wherever they are and the service flexibility enables business development without the appeal to [...]
An electronic flight instrument system (EFIS) is a flight deck instrument display system located at the flight cockpit in the lower deck after the windshield. It is powered electronically and produce digital output. The [...]
Nowaday’s demand for power generation is increasing. Therefore control system requirements are also increasing. In this paper boiler is operated using many control strategies so that resultant operation will be practically [...]
The wide of knowledge which deals with the creation and use of technical means and their interrelation with life, society ,and the environment and studies such as engineering ,arts, applied science and information [...]
Malware is commonly found a headache in almost all the mobile phones, laptops, memory cards etc. The most common technique used by malware to avoid detection is binary obfuscation either by either using encryption. One of the [...]
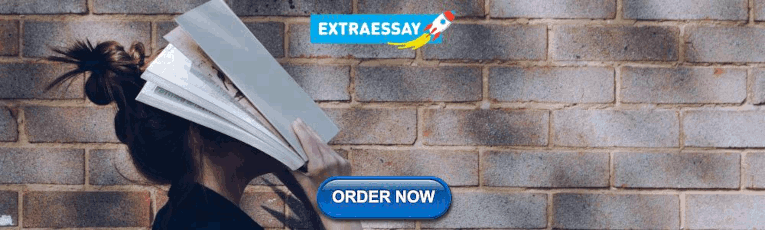
Related Topics
By clicking “Send”, you agree to our Terms of service and Privacy statement . We will occasionally send you account related emails.
Where do you want us to send this sample?
By clicking “Continue”, you agree to our terms of service and privacy policy.
Be careful. This essay is not unique
This essay was donated by a student and is likely to have been used and submitted before
Download this Sample
Free samples may contain mistakes and not unique parts
Sorry, we could not paraphrase this essay. Our professional writers can rewrite it and get you a unique paper.
Please check your inbox.
We can write you a custom essay that will follow your exact instructions and meet the deadlines. Let's fix your grades together!
Get Your Personalized Essay in 3 Hours or Less!
We use cookies to personalyze your web-site experience. By continuing we’ll assume you board with our cookie policy .
- Instructions Followed To The Letter
- Deadlines Met At Every Stage
- Unique And Plagiarism Free
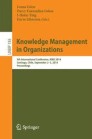
International Conference on Knowledge Management in Organizations
KMO 2014: Knowledge Management in Organizations pp 281–287 Cite as
Laboratory Information Management Systems – Challenges of Implementation
- Hilary Berger 10
- Conference paper
- First Online: 01 January 2014
1867 Accesses
Part of the book series: Lecture Notes in Business Information Processing ((LNBIP,volume 185))
For many organizations, their Laboratory Information Management System (LIMS) is a mission-critical component of their overall corporate Information Systems (IS) and is thus directly linked to performance. However there is some debate regarding the challenges of effective implementation. Using ten companies involved in refining hydrocarbons operating within the Petrochemical Industry we evaluate the implementation of a LIMS system, and examine the problems experienced. In practice we found a critical need for both user involvement and established, well defined training programs during implementation. Moreover, provision of transparent communication mechanisms that feed back up the management chain was recognized. We propose best practice guidelines for implementing the LIMS system to address the key challenges identified.
- Effective implementation
This is a preview of subscription content, log in via an institution .
Buying options
- Available as PDF
- Read on any device
- Instant download
- Own it forever
- Available as EPUB and PDF
- Compact, lightweight edition
- Dispatched in 3 to 5 business days
- Free shipping worldwide - see info
Tax calculation will be finalised at checkout
Purchases are for personal use only
Bentley, D.: Analysis of a Laboratory Information Management System (LIMS). http://www.umsl.edu
Bryman, A., Bell, E.: Business Research Methods. Oxford University Press, Oxford (2007)
Google Scholar
Cresswell, J.W., Plano Clark, V.L.: Designing and Conducting Mixed Methods Research. Sage Publications, Thousand Oaks (2011)
Farrimond, H.: Doing Ethical Research. Palgrave Macmillan, Hants (2013)
Fowler, F.J.: Survey Research Methods. Sage Publications, Thousand Oaks (2002)
Gibbon, G.A.: A brief history of LIMS. Lab. Autom. Inf. Manage. 32 , 1–5 (1996)
Article Google Scholar
Gonzalez, J.M.C., Cortes, O.F.: LIMS Standardization –The Real Benefits for the Process Industry. http://www.thermo.com
Klein, H.K., Myers, M.D.: A set of principles for conducting and evaluating interpretive field studies in information systems. MIS Q. 23 , 67–94 (1999)
Murray, M.: Laboratory Information Management Systems (LIMS) (2014). http://logistics.about.com
Myers, M.D.: Qualitative Research in Business and Management. Sage Publications, Thousand Oaks (2009)
O’Leary, K.M.: Selecting the Right LIMS: Critiquing technological strengths and limitations. Scientific Computing (2011)
Paszko, C., Turner, E., Hinton, M.D.: Laboratory Information Management Systems. CRC Press, New York (2002)
Raese, J., Rime, D.: Nevada District partners with NWQL to help investigate Fallon cancer cluster. National Water Quality Laboratory Newsletter. 9 (3) (2001)
Ritchie, J., Lewis, J.: Qualitative Research Practice – A Guide for Social Science Students and Researchers. Sage Publications, London (2004)
Robson, D.: LIMS aids industrial production (2008). http://www.scientific-computing.com
Sapio Sciences, Laboratory Information Management: So what is a LIMS? (2011). http://sapiosciences.blogspot.com
Saunders, M., Lewis, P., Thornhill, A.: Research Methods for Business Students. Prentice Hall, England (2007)
Silverman, D.: Doing Qualitative Research. Sage Publications, London (2010)
Simpson, G.: The software driving the petrochemicals industry. Scientific Computing World (2008). http://www.scientific-computing.com
Smith, K., Thurston, C.: LIMS Integration (2009). http://www.thermo.com
Thermo Fisher Scientific Inc., The Enterprise LIMS for Chemical and Petrochemical Companies. https://www.thermo.com
Thurston, C., Carlini, A.: IT and Automation Systems in the Petrochemical Industry (2008). http://www.petro-online.com
Thurston, C.: Why a LIMS is Critical to ISO 17025 Compliance (2013). http://www.scientificcomputing.com
Tomiello, K.: Regulatory compliance drives LIMS (2007). www.designworld.online.com
Vaughan, A.: LIMS: The Laboratory ERP (2011). http://www.limsfinder.com
Wauquier, J.P., Favennec, J.P., Trambouze, P., Baker, R.: Petroleum Refining: Refinery operation and management. Editions TECHNIP, France (2001)
Yin, R.: Case Study Research: Design and Methods. Sage Publications, Thousand Oaks (2009)
Download references
Author information
Authors and affiliations.
Cardiff School of Management, Cardiff Metropolitan University, Western Avenue, Cardiff, CF5 2YB, UK
Hilary Berger
You can also search for this author in PubMed Google Scholar
Corresponding author
Correspondence to Hilary Berger .
Editor information
Editors and affiliations.
Staffordshire University, Staffordshire, United Kingdom
Universidad Santa Maria, Santiago, Chile
Darcy Fuenzaliza Oshee
National University of Kaohsiung, Kaohsiung City, Taiwan
I-Hsien Ting
Dario Liberona
Rights and permissions
Reprints and permissions
Copyright information
© 2014 Springer International Publishing Switzerland
About this paper
Cite this paper.
Berger, H. (2014). Laboratory Information Management Systems – Challenges of Implementation. In: Uden, L., Fuenzaliza Oshee, D., Ting, IH., Liberona, D. (eds) Knowledge Management in Organizations. KMO 2014. Lecture Notes in Business Information Processing, vol 185. Springer, Cham. https://doi.org/10.1007/978-3-319-08618-7_27
Download citation
DOI : https://doi.org/10.1007/978-3-319-08618-7_27
Published : 26 August 2014
Publisher Name : Springer, Cham
Print ISBN : 978-3-319-08617-0
Online ISBN : 978-3-319-08618-7
eBook Packages : Computer Science Computer Science (R0)
Share this paper
Anyone you share the following link with will be able to read this content:
Sorry, a shareable link is not currently available for this article.
Provided by the Springer Nature SharedIt content-sharing initiative
- Publish with us
Policies and ethics
- Find a journal
- Track your research
- Efficacy and Safety of Tirzepatide
- Vascular Leak in Sepsis
- AsymMirai: New Mammography-based Deep Learnin
- Evidence-Based Medicine and the Practice...
Top 10 Patient Safety Concerns 2024: How...
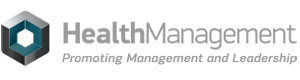
Implementing DLR in MRI Routines Could Lead...
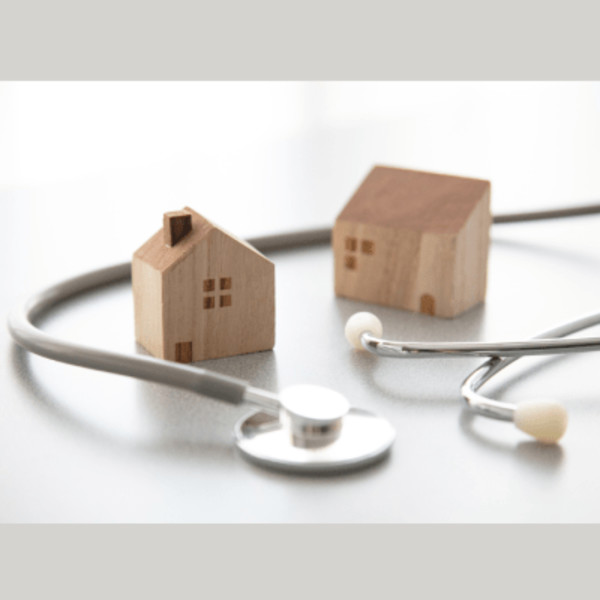
Transforming Healthcare Delivery: Navigating...
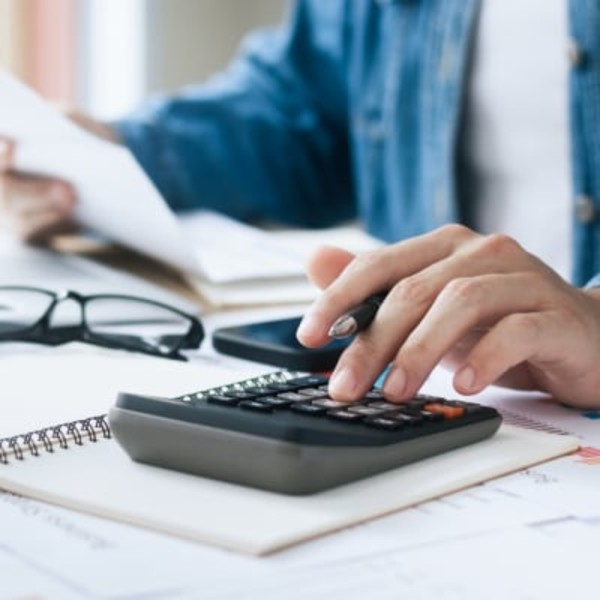
Financial Outlook for Healthcare: Optimistic...
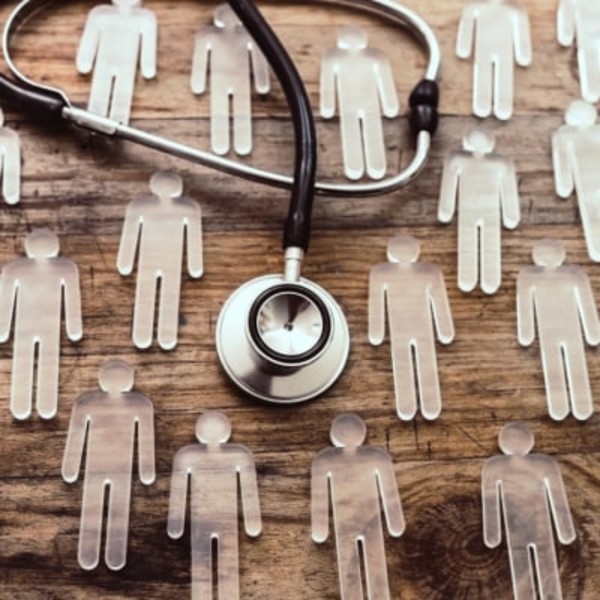
Laboratory Information Management System
- Sun, 3 Feb 2008
HealthManagement, Volume 3 / Issue 2 / 2008
The Core Laboratory for Clinical Studies (CLCS) at Washington University School of Medicine in Saint Louis, USA, conducts testing on Phase 3 and 4 clinical trial samples. In addition to pharmaceutical sponsors, the CLCS also performs testing for Washington University School of Medicine researchers, and for clinicians who service patients. In early 2002 it was determined that the legacy Laboratory Information Management System (LIMS) was to a more robust, data driven system.
David A. Mulvihill
is Manager of Information
Systems, Core Laboratory
for Clinical Studies,
Washington University
School of Medicine, USA.
The IT department was tasked with designing a solution that would meet business, regulatory, user and client requirements. Determining where the legacy system failed was helpful in planning a system that would be extendable and maintainable. The legacy system was written in dBase 4, an application development language, and was architectured in a way that required significant re-coding of the LIMS to implement basic enhancements (such as a new test or bringing new pharmaceutical studies online). Performing these updates and subsequent testing and implementation on a rigorous schedule became overwhelming, and led to programming inconsistencies and system errors. In addition, the legacy LIMS database was not normalised, resulting in significant data duplication. When a patient’s name was updated, for example, numerous tables needed to be updated in a cascading fashion. This caused inconsistencies in the database and was to be avoided in the development of the new system.
We determined that the best architecture for the new LIMS would be a data driven n-Tier system that would utilise the reusability of classes in an object-oriented programming language to ease in performing system updates or enhancements. Due to the separation of business logic, user interface and the data store, n-Tiering permits modification of one tier without necessarily impacting the others. For example, a database upgrade
or a modification to allow web access can minimise the need for heavy reworking. Microsoft Visual FoxPro 6 (VFP) was selected as the development language because there was in-house expertise in the use of an x-based language (dBase 4). VFP is an object-oriented language offering an integrated development environment for both user interface and database. In addition, the VFP database is easily scalable to an enterprise database management system such as Oracle eor MS SQL Server.
The next step was to map all the legacy processes to the new n-tier system. This involved creating logical maps of laboratory processes and determining the impact the new system would have on these processes throughout the laboratory. Key process changes were identified and noted so standard operating procedures and training material could be developed prior to implementation. Physical maps of the database were developed to classify how data from the legacy system would be stored. Legacy entities, attributes, indexes and data were scrutinised to maximise performance and eliminate data duplication in the new normalised database schema. The data conversion programming was developed from these maps.
The CLCS utilises numerous automated analysers to perform testing. In order to speed data entry into the LIMS and minimise the risk of data entry errors, these instruments were targeted for electronic data interfaces. Most laboratory analysers use an ASTM International or subset protocol for messaging between the LIMS and the instrument. There were two scenarios that were considered when designing instrument interfaces. In the first, the instrument only sent sample results, uni-directionally, to the LIMS. In the other, the instrument queried the LIMS for pending test orders and sent samples results to the LIMS, bi-directionally. Both scenarios used barcoded IDs to uniquely identify the sample. Instrument interfaces for each analyser were created using Microsoft C#.Net to pass data between the instrument and the LIMS. Data import processes were created for other laboratory instrumentation that did not support a messaging protocol but allowed for exporting the data in some fashion (.txt, .csv, etc.).
Since the CLCS performs laboratory testing for multi-site international clinical trials as well as physician offices, we needed to create the flexibility to route the report to clients via printed, faxed or PDF copy. In addition, we needed to mimic the report format from the legacy system so the LIMS upgrade would be transparent to clients. This was accomplished by designing a helper application that monitored the LIMS for reports to create. When the helper application found all testing was complete on a patient, it created an ASCII text file with an identical format to the legacy systems report. The LIMS user would then be able to review the report and subsequently authorise it to be delivered via the preferred client method. An important business critical requirement that was addressed was the detection of laboratory results that fell outside reasonable limits. The LIMS was designed to compare patient results to known extreme, physician alert and client requested values. If outside defined limits, the result was flagged for resolution by the technologist. This insured that no questionable values were released to clients without being double checked for accuracy. An additional delta check, added to compare a patient’s current result against a previous one, aided in identifying non-analytical errors such as switched or mislabelled samples.
Due to special data handling requests from the CLCS client base, it is difficult to predict data formats and attributes required for the client data set. In these cases, data handling applications are developed independent of the LIMS. They are controlled by a formal change control process and validated as standalone applications.
After realisation of the full scope of the development project, we began formal development of the system. Throughout the development lifecycle, numerous iterations of unit, module and system testing phases were performed to internal quality assurance standards.
The impact of the new LIMS on workflow and lab-level operations was foreseen to be minimal. The impact on users, however, was expected to be extensive and posed a significant business risk. Standard Operating Procedures (SOPs) were created to address, in detail, all aspects of the use and maintenance of the LIMS and associated applications. Group training sessions were conducted to give users a general overview of the systems functionality. Specialised sessions targeted smaller user groups; they focused on tasks specific to particular laboratory positions and were conducted close to the ‘go-live’ date to provide comfort to users during transition to the new LIMS.
A new server was installed and workstation hardware upgraded or replaced prior to deployment of the LIMS. The network operating system (NOS) was updated from Novell Netware 4.0 to Windows 2000 Server and all workstation operating systems were upgraded from Windows 98 to Windows XP. The system was tested side-by-side with the legacy system before being fully implemented.
In order to bring the LIMS into compliance with the US Regulations mandated by our pharmaceutical clients, a retrospective validation was performed three years later. Such a process establishes documented evidence, which ensures that the system not only offers users the requisite functional capability but will continue to do so through the LIMS lifecycle. System validation is considered a critical instrument to ensure data quality. By improving regulatory compliance and reliability, it can help ensure that patient risks, error rates and related costs can be controlled. Costs can therefore be reduced throughout the lifecycle, as it becomes easier to perform system modifications and re-validations.
System Upgrades
Since the CLCS operates in a regulated environment, all computer devices that capture, enter or modify data are required to follow change control procedures. These allow system modifications to be traced to their origins and permit ‘rollback’ to a previous system state if required. A change control SOP was developed to provide an organised and consistent plan to ensure integrity and stability throughout the LIMS lifecycle.
Conclusion: Build or Buy ?
There are many commercial LIMS systems that could have performed well for the CLCS and we took account of many factors– among them, flexibility, scalability, quality, security and cost – before deciding to build a custom system. The CLCS also sought to avoid a rigid LIMS framework which required a prolonged waiting period for upgrades or entailed dependence for maintenance on an external vendor.
Our new system blends the productivity and throughput of commercial systems with the flexibility of a custom system built around the core business which we, as users, know best.
«« Healthcare IT Providers Need To Do More To Solicit User Feedback
Latest Articles
Pharmacist in the icu.
- Journal Article
Pharmacists play a crucial role in the ICU, where patients often require complex medication regimens, including multiple medications and intravenous therapies. The role of a critical care pharmacist is multifaceted and vital to ensuring optimal patient care in the critical care setting. Cr
Defining Medicines Optimisation in the Intensive Care Unit
Complex polypharmacy and pathophysiology are common in the intensive care unit (ICU). Medicines optimisation is essential to deliver safe, effective, and individualised pharmacotherapy. This is ideally performed by a specialised ICU pharmacist. Introduction Medicines ar
Critical Care Pharmacists Save Lives
The purpose of this review is to discuss the role of critical care pharmacists on the interprofessional healthcare team in the care of critically ill patients and explore current gaps in the provision of comprehensive medication management. Introduction “The patient’s
Latest News
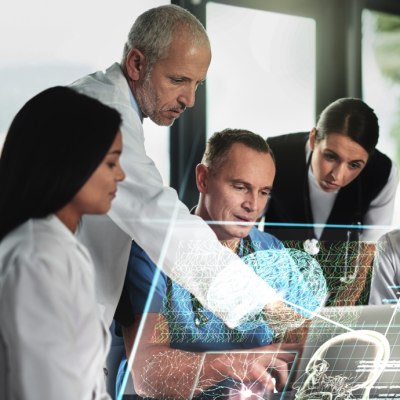
Bridging the Gap: Navigating AI's Promise and Perils for Health Equity
The HIMSS Global Health Conference & Exhibition highlighted Artificial Intelligence (AI) as a central theme, emphasising its potential to revolutionise healthcare by
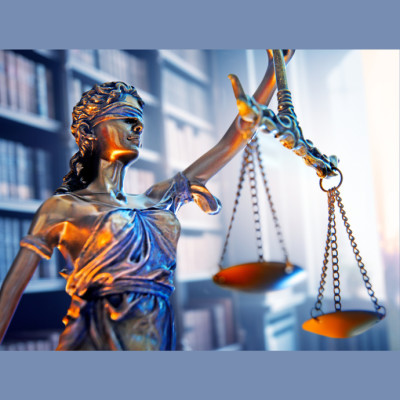
AI and Real-World Data in Healthcare: A Call for Fairness Assessment
Recent advancements in artificial intelligence (AI), particularly machine learning (ML), have been successfully integrated into healthcare applications, showing promise i
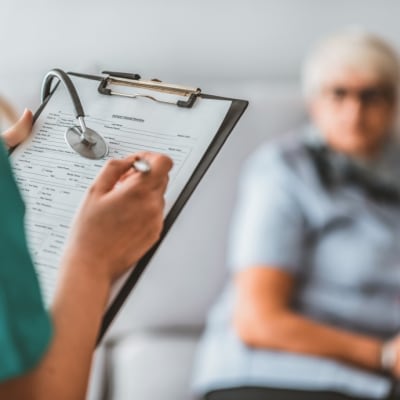
Machine Learning Model to Identify Social Needs From Patient Medical Notes
Social needs and social determinants of health (SDOH) are significant factors influencing clinical outcomes, yet they are often under-documented in healthcare systems' el
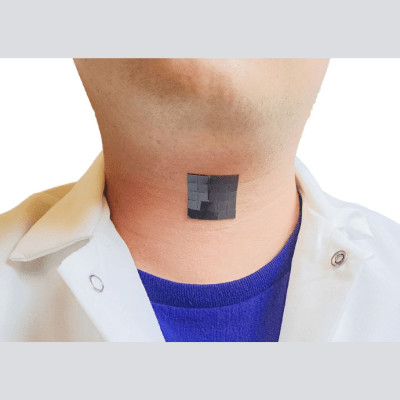
AI-Supported Wearable Device Offers Hope for Patients with Voice Disorders
A groundbreaking invention by a team of engineers at UCLA offers hope to individuals grappling with voice disorders, whether stemming from pathological vocal cord conditi
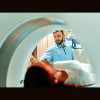
- Movers & Shakers
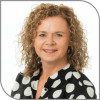
Gill Rooke Assumes Deputy Director Role at...
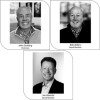
BRIJ Medical Bolsters Leadership Team
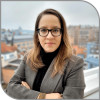
European Health Management Association Welcom
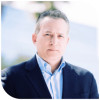
Applied BioCode Announces Retirement of CEO...
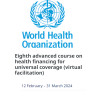
Eighth advanced course on health financing...
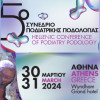
5th Hellenic Congress Of Podiatry - Podology...
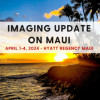
Imaging Update On Maui 2024
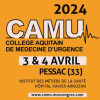
C@MU 2024 Congress
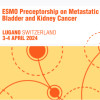
ESMO Preceptorship on Metastatic Bladder...
- Advertising
- Submit Article
- Author Guide
- Privacy Policy
- Cookie Policy
- Terms and conditions
- Copyright and permissions
- Editorial Board
- White Papers & Case Studies
- IMAGING Highlights
- ICU Highlights
- EXEC Highlights
- IT Highlights
- CARDIO Highlights
- HealthManagement
- ICU Management
- (E)Hospital
- Imaging Management
- Imaging Management French
- Healthcare IT
- Cardiology Management
- IQ - Interventional Quarter
- List your event
- Past Events
- International Association
- National Association
- Universities - Institutions
- Paid Guest Posts
- I-I-I DigiFlash
Communities
- Decision Support
- Women's Health
- Enterprise Imaging
- Artificial Intelligence
- Finance Management
- Cybersecurity
- Sustainability
- Digital Transformation
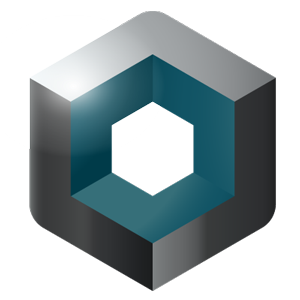
Rue Villain XIV 53-55
B-1050 brussels, belgium, tel: +357 86 870 007, e-mail: [email protected], emea & row office, 166, agias filaxeos, cy-3083, limassol, cyprus, headquarters, kosta ourani, 5, petoussis court, 5th floor, cy-3085 limassol, cyprus.
The Role of Laboratory Information System in Improving the Delivery of Laboratory Services: A Recent Systematic Review
Affiliations.
- 1 Department of Public Health, College of Health Sciences, Saudi Electronic University, Riyadh, Saudi Arabia.
- 2 Pathology and Laboratory Department, King Abdulaziz Medical City, Ministry of National Guard, Riyadh, Saudi Arabia.
- 3 Department of Public Health, College of Health Sciences, Saudi Electronic University, Dammam, Saudi Arabia.
- PMID: 36111772
- DOI: 10.2174/1386207325666220914112713
Background: Recently, laboratory information systems (LIS) have become necessary for every laboratory to improve the decision-making process and achieve better treatment and diagnostic results. By standardizing laboratory's tests, procedures, and workflows, the software enables laboratories to improve patient care, reduce human error, and constructively lower operating costs. Implementing LIS has a multidimensional impact on improving the delivery of laboratory services.
Objectives: This paper aims to investigate how patient services can be improved by laboratory information system.
Methods: This paper is based on a review conducted by searching PubMed, Google Scholar, Saudi Digital Library and Research Gate for English language articles published from 2015 to 2021 and focused primarily on laboratory information systems.
Results: The literature searches yielded a total of 30 articles that were then initially screened based on the titles and abstracts. Seven articles were excluded because they did not primarily address LIMS for biosafety, automated verification of test results in the core clinical laboratory, clinical biochemistry, or the impact of health information technology on patient safety, or were not written in English. The remaining 23 articles were then screened in full text.
Conclusion: Advanced laboratory information systems may eliminate diagnostic errors in the preanalytical, analytical, and postanalytical phases. In addition, they can incorporate genomic data at the analytical stage to generate useful reports for providers and patients.
Keywords: Laboratory; health services; information system; multidimensional impact; postanalytical phases; service delivery.
Copyright© Bentham Science Publishers; For any queries, please email at [email protected].
Publication types
- Systematic Review
- Clinical Laboratory Information Systems*
Academia.edu no longer supports Internet Explorer.
To browse Academia.edu and the wider internet faster and more securely, please take a few seconds to upgrade your browser .
Enter the email address you signed up with and we'll email you a reset link.
- We're Hiring!
- Help Center
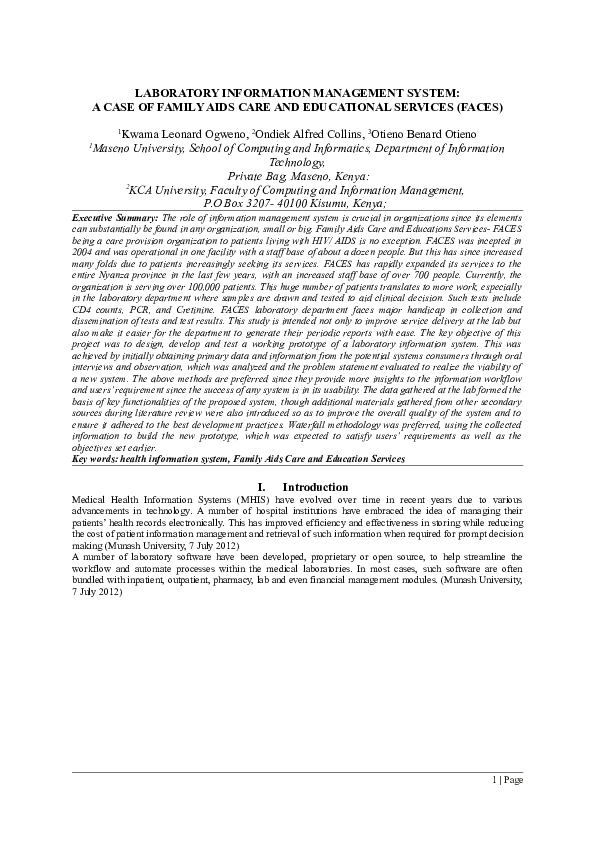
LABORATORY INFORMATION MANAGEMENT SYSTEM: A CASE OF FAMILY AIDS CARE AND EDUCATIONAL SERVICES (FACES) 1
Related Papers
Fardanto Setyatama
The use of information technology has transformed laboratory service from conventional way (by using paper/manually) into using computer-based Information System (IS). The output of computer-based IS could also be used for management as service quality improvement. Patient data along with their laboratory test result stored electronically in IS become a valuable asset for the clinical laboratory as well as the patient itself. PT. Populer Sarana Medika is trying to utilize information technology as their competitive strategy to improve interoperability with agencies, to integrate information systems between their departments, and also to improve their overall quality of service. This paper mainly discuss about the use of Rapid Application Development (RAD) method in the development of Clinical Laboratory Information System (CLIS). RAD method could accelerate the development of IS which is normally done in 180 days (minimum) to only 30-90 days. By using RAD method, it is expected that...
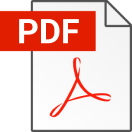
International Journal of Online and Biomedical Engineering (iJOE)
Somchat Taertulakarn
In the medical laboratory, equipment and material management is well real-ized as one of the high-priority tasks in handling of information about the periodic quality inspection and maintenance of test equipment and other in-strument monitoring in the laboratory. Lack of managing and neglecting the equipment can result into malfunction that ends up costing more time and resources. This paper proposed a designed web-based information system in order to address the issues of managing the expensive medical equipment (ME) and various consumable medical materials (CMM) in the laboratories. In regard to data administration improvement of medical laboratories' day-to-day operations, the objective of this study is to design to complete the func-tionality and evaluate the information system based on the user specifica-tion. More than 200 initiate materials list and 50 medical equipment records were collected and transferred into the system. Three independent laborato-ries utilized the de...
International Journal for Pharmaceutical Research Scholars (IJPRS)
Laboratory Information System (LIS) streamlines workflow in the laboratory and eliminates time-consuming paperwork. The business today is getting increasingly pressured to be more productive and efficient with less traditional resources. If we look at a typical production process, for instance, the level of automation and optimization that has permeated into the production floor is quite astounding. Many industries have had a tremendous impact in bringing to the market the automation and optimization solutions to streamline -the production floor. The other ends of the spectrum are the back-office transaction oriented processes like Inventory Management, Materials Management, Financials and so on, Therefore a lab’s ability to process data quickly and its ability to disseminate that information efficiently throughout the plant is vital for the success of the production process. Laboratory Information Management System (LIMS) can help in managing the information flow within the lab and can be the modus operandi of connecting the lab with the rest of the organization. For instance, imagine all the information that is generated in a lab.
Ika Menarianti
In 2021 PGRI Semarang University have at least 64 laboratories. This makes it a challenge for a private university to obtain funding independently in managing and maintaining assets of that size. It is necessary to develop an information system that could provide information input and information output facilities managed by the faculty and laboratory managers at the unit level of study programs and faculties, to be further reported as information to institutions and stakeholders. The researcher wants to make it a research goal, by designing and developing a Laboratory Information System (SILab) at the University of PGRI Semarang, which is validly tested according to the design results and is reliable or proven to be used well by users through user response tests.
Journal of Physics: Conference Series
Purwiro Harjati
School laboratories have an essential role in helping the development of students’ thinking processes. However, until now many school laboratories have not functioned well in supporting the learning process. One of the causes that there is no practical and efficient laboratory management system. Laboratory work now feels very complex which requires persistent. On the other, technology is developing very rapidly in the current era of the 4.0 industrial revolution, which is demonstrated by cyber technology. This cyber system provides an opportunity to develop a laboratory management system that is easier both in administration and management. Therefore the purpose of this study is the development of management systems for industrial revolutionary school laboratories 4.0. This development research method includes needs analysis (implemented), design, development, and implementation stages. Data analysis uses a qualitative approach through documentation, focus group discourse and questi...
Kumbirai Chigudu
Anastasia Maukar
The main objective of this research is to improve the current flow of information in the laboratory as it is found to be ineffective to reach the customers. This research is necessary and demanded to be finished as soon as possible as it may affect the product development in Toy Manufacturing company. To solve the information flow problem, it is then initiated the idea to develop a new progress tracking system, which is supposed to be able to provide real-time information to customers and make them able to react faster to problems. Lack of information in the problem could lead to longer development lead time. T The research conducted during the project was basically involving literature review, online journals review, questionnaire, case study, and discussion. This research covers the whole development process of the system, up to the implementation and evaluation. The result shows that there is improvement of 42.47% in delivering tracking information to the customers.
Chemometrics and Intelligent Laboratory Systems
Maiara Cancian
The development of health care information systems has been shown to be complex and costly. A primary concern is the thorough analysis of stakeholders’ information, knowledge and their needs. Traditional processes have to be adapted in order maximize their efficiency and effectiveness. In this paper, we describe a requirements development process in alignment with CMMI-DEV and ISO/IEC 15504, which has been defined and applied for the development of new clinical laboratory information system for the Central Laboratory of the Santa Catarina State Public Health Department (LACEN)/Brazil. The system is currently being applied statewide for more than 75 types of clinical analyses involving 7 facilities. We present our experiences in applying the requirement development process and lessons learned.
Analytica Chimica Acta
Elizabeth Turner
RELATED PAPERS
Gerald Katsenga
US Patent 7,984,537
Jack Champaigne
Alireza Alishahi
IOSR Journal of Applied Chemistry
MOHAMED AHMED Shaban
Colloids and Surfaces B: Biointerfaces
Anal K A N T Jha
Optics Express
Tevhit karacalı
Archivos Latinoamericanos de Producción Animal
Antonio Santana
Physical Review Letters
M. Feigelman
Melilah: Manchester Journal of Jewish Studies (1759-1953)
Kenneth Seeskin
ITL - International Journal of Applied Linguistics
Dmitri Leontjev
Revista Do Cepa
Sergio Klamt
International Journal for Parasitology
Adriana Goncalves Ranulfo Dos Santos
Revista Diálogos Mediterrânicos
Marcella Lopes Guimarães
Progress in Oceanography
Javier Ruiz
Journal of Personalized Medicine
Petronela Naghi
Rachel Midura
Journal of Architecture and Planning (Transactions of AIJ)
nanae nakao
DOAJ (DOAJ: Directory of Open Access Journals)
Indira Franco
Hector Fabio Restrepo
Springer Seminars in Immunopathology
Antonio Coutinho
Miloje Rakocevic
Journal of Radioanalytical and Nuclear Chemistry Letters
Hidde Brongersma
Information and Learning Sciences
Kuldeep Gurjar
Muzeum: Muzejní a vlastivědná práce
Michaela Smidová
See More Documents Like This
- We're Hiring!
- Help Center
- Find new research papers in:
- Health Sciences
- Earth Sciences
- Cognitive Science
- Mathematics
- Computer Science
- Academia ©2024
- Free Samples
- Premium Essays
- Editing Services Editing Proofreading Rewriting
- Extra Tools Essay Topic Generator Thesis Generator Citation Generator GPA Calculator Study Guides Donate Paper
- Essay Writing Help
- About Us About Us Testimonials FAQ
- Studentshare
- Laboratory Information Management Systems
Laboratory Information Management Systems - Research Proposal Example
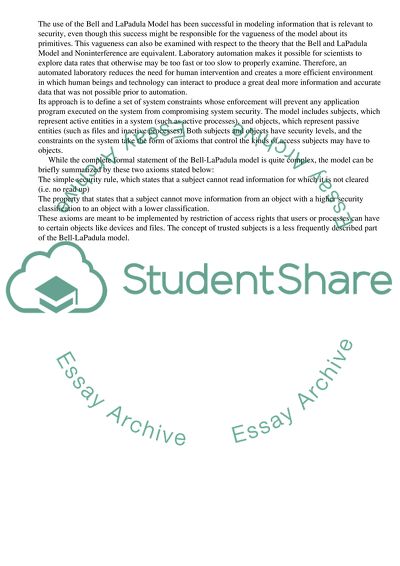
- Subject: Management
- Type: Research Proposal
- Level: College
- Pages: 5 (1250 words)
- Downloads: 3
- Author: uokeefe
Extract of sample "Laboratory Information Management Systems"
- Cited: 0 times
- Copy Citation Citation is copied Copy Citation Citation is copied Copy Citation Citation is copied
CHECK THESE SAMPLES OF Laboratory Information Management Systems
Clinical laboratory improvement act, the impact of the us federal regulatory agencies on the lims, the effects of regulatory agencies on the laboratory information management system software, healthcare information systems, resources that support information management systems in healthcare, management of information systems, laboratory information systems to make critical decisions, evolution of laboratory quality management systems.
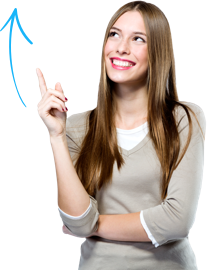
- TERMS & CONDITIONS
- PRIVACY POLICY
- COOKIES POLICY
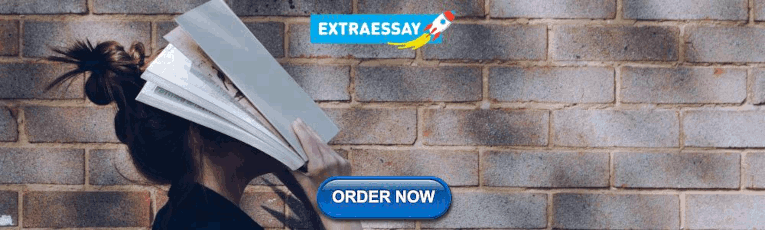
COMMENTS
Open Document. Laboratory information management system (LIMS) is a software system that leads records and reserves data for laboratories. It sends laboratory test orders to laboratory materials, tracking its responsible and records the results to a searchable database. Also LIMS supports the operations of public health organizations such as ...
Laboratory Information Management Systems (LIMS) The LIMS is the core backbone of a laboratory's operations. An ideal LIMS can. drive laboratory work o ws and allows for maximum throughout the ...
Fig 1. Information management enhances the experimental and modeling cycle. Our 10 simple rules for managing laboratory information (green) augment the cycle of hypothesis formulation, design, data analysis, modeling, and decision-making (gray). The experimental design phase is improved by carefully tracking your inventory, samples, parameters ...
Published: Dec 12, 2018. LIMS or the Laboratory Information Management System is an efficient method to handle large amounts of data produced in the modern laboratory. The efficiency comes from the fact that LIMS automates the process of laboratory data storage as well as the data retrieval. One of the biggest challenges a LIMS faces is the ...
A Laboratory Information Management System (LIMS) is a software-based information management system with features that facilitate efficient laboratory operations. Quality management practices are put in place to ensure every aspect of the system functions harmoniously. The data generated by these practices are best managed and maintained in a ...
Laboratory information management systems (LIMS) is designed considering the need of analytical laboratories to carry out the research in fast, efficient and transparent manner with better accessibility of the instruments. ... LIMS brings accuracy and accessibility to the flow of samples and data laboratory. As discussed in the papers [32], [33
Abstract. Laboratory information management systems belong to the class of application software intended for storage and management of information obtained in the course of the work of the ...
Laboratory Information Management System (LIMS) is software that is designed to administer samples, acquire and manipulate data, and report results via a database. ... Based on title and abstract ...
To this point, we have examined many of the concepts and practices related to the use of computers in analytical laboratories. We have stated that a LIMS provides the information management tools that lab analysts require to manage the tremendous amount of information which they are required to handle. In Chapter 5 we begin looking in detail at ...
The approach shown in the paper presents a system's model based on modern web oriented software technologies that includes planning tools, a virtualized lab, and on-line process adaptation as well as an interface for computational data processing and data mining. This paper discusses different aspects of laboratory information management systems in life science applications.
Cardiff School of Management, Cardiff Metropolitan University, Western Avenue, Cardiff CF5 2YB, UK [email protected] Abstract. For many organizations, their Laboratory Information Management System (LIMS) is a mission-critical component of their overall corporate Information Systems (IS) and is thus directly linked to performance. However
Gibbon /Laboratory Automation and Information Management 32 (/996) l-S System, and Purvis Systems - Computerized LIS. 1982 was the watershed year! Perkin-Elmer announced LIMS 2000, Purvis Systems offered a `Turnkey LIMS', and Spectrogram Corporation introduced a LMIS (laboratory management information system) [9].
Laboratory Information Management System (LIMS) makes it possible for laboratory technologists to trace and track all samples or specimens through a laboratory process. Steps in such a process would likely include data analysis and storage, tracking system analysts, and recording date and time of each step in the analysis process.
David A. Mulvihill. is Manager of Information. Systems, Core Laboratory. for Clinical Studies, Washington University. School of Medicine, USA. Design. The IT department was tasked with designing a solution that would meet business, regulatory, user and client requirements. Determining where the legacy system failed was helpful in planning a ...
This robust laboratory management tool satisfies Namibia's information obligations under international quality standard ISO 17025:2005. The Laboratory Information Management System (LIMS) for Africa was designed to collect and manage all necessary information on samples, tests and test results.
Background: Recently, laboratory information systems (LIS) have become necessary for every laboratory to improve the decision-making process and achieve better treatment and diagnostic results. By standardizing laboratory's tests, procedures, and workflows, the software enables laboratories to improve patient care, reduce human error, and ...
A recent electronic laboratory information system assessment reflected the need for this three-step approach. When beginning an evaluation design, multiple factors must be considered. One ...
Fig 4.6 Sample Test report Conclusion The purpose of this project was to develop a prototype of a laboratory management system for FACES laboratory and to ascertain their adequacy in managing the patients' tests information this was because Medical Health Information Systems (MHIS) have evolved over time in recent years due to various ...
Nowadays, the labs management under the background of JAVA,designing effectively the management system is on the basis of B/S model and does effective experiments. With the fast development of the science and information technology, the social level of China has developed and people's living standard has also been improved. The development of information technology has made the computers be ...
Download. Essay, Pages 2 (449 words) Views. 759. The LIMS system allows laboratories and other industries, to insert information in the electronic format that is required in order for it to function for it to function and generate the working system. This allows other users and participants to insert relevant data in mandatory fields, this ...
Scope This dissertation covers the applicability of the bell-lapadula model in Laboratory Information Management Systems, and the limitations involved in the use of the Bell-LaPadula model, including an absence of policies for changing user access rights. Also to be covered is the relationship that this model has with other existing security ...
Instruments are also part of the core laboratory items whose records are essential. The storage of all this information can be a daunting task for laboratory personnel. Thus, storage becomes easier through the assistance provided by laboratory information management systems (Voegele et al., 2007).