- Skip to main content
- Skip to primary sidebar
- Skip to footer
- QuestionPro

- Solutions Industries Gaming Automotive Sports and events Education Government Travel & Hospitality Financial Services Healthcare Cannabis Technology Use Case NPS+ Communities Audience Contactless surveys Mobile LivePolls Member Experience GDPR Positive People Science 360 Feedback Surveys
- Resources Blog eBooks Survey Templates Case Studies Training Help center

Home Market Research
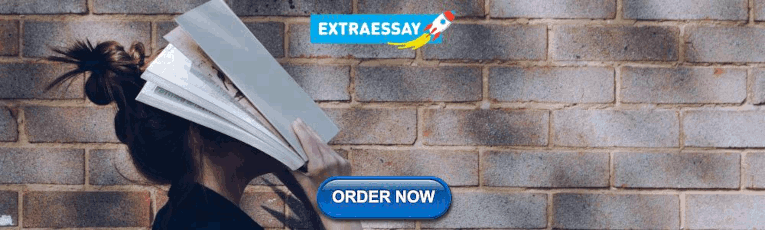
Empirical Research: Definition, Methods, Types and Examples
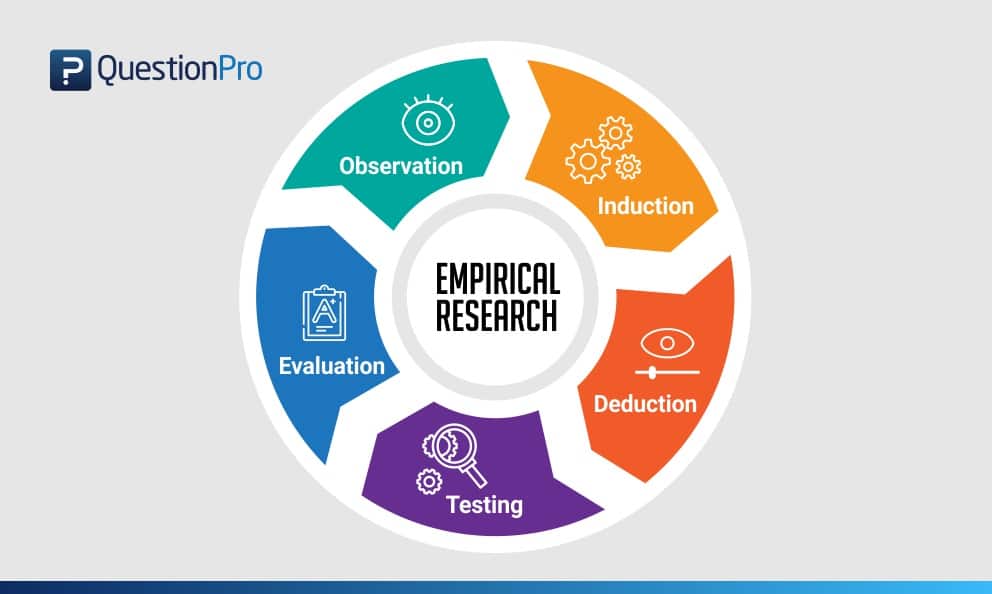
Content Index
Empirical research: Definition
Empirical research: origin, quantitative research methods, qualitative research methods, steps for conducting empirical research, empirical research methodology cycle, advantages of empirical research, disadvantages of empirical research, why is there a need for empirical research.
Empirical research is defined as any research where conclusions of the study is strictly drawn from concretely empirical evidence, and therefore “verifiable” evidence.
This empirical evidence can be gathered using quantitative market research and qualitative market research methods.
For example: A research is being conducted to find out if listening to happy music in the workplace while working may promote creativity? An experiment is conducted by using a music website survey on a set of audience who are exposed to happy music and another set who are not listening to music at all, and the subjects are then observed. The results derived from such a research will give empirical evidence if it does promote creativity or not.
LEARN ABOUT: Behavioral Research
You must have heard the quote” I will not believe it unless I see it”. This came from the ancient empiricists, a fundamental understanding that powered the emergence of medieval science during the renaissance period and laid the foundation of modern science, as we know it today. The word itself has its roots in greek. It is derived from the greek word empeirikos which means “experienced”.
In today’s world, the word empirical refers to collection of data using evidence that is collected through observation or experience or by using calibrated scientific instruments. All of the above origins have one thing in common which is dependence of observation and experiments to collect data and test them to come up with conclusions.
LEARN ABOUT: Causal Research
Types and methodologies of empirical research
Empirical research can be conducted and analysed using qualitative or quantitative methods.
- Quantitative research : Quantitative research methods are used to gather information through numerical data. It is used to quantify opinions, behaviors or other defined variables . These are predetermined and are in a more structured format. Some of the commonly used methods are survey, longitudinal studies, polls, etc
- Qualitative research: Qualitative research methods are used to gather non numerical data. It is used to find meanings, opinions, or the underlying reasons from its subjects. These methods are unstructured or semi structured. The sample size for such a research is usually small and it is a conversational type of method to provide more insight or in-depth information about the problem Some of the most popular forms of methods are focus groups, experiments, interviews, etc.
Data collected from these will need to be analysed. Empirical evidence can also be analysed either quantitatively and qualitatively. Using this, the researcher can answer empirical questions which have to be clearly defined and answerable with the findings he has got. The type of research design used will vary depending on the field in which it is going to be used. Many of them might choose to do a collective research involving quantitative and qualitative method to better answer questions which cannot be studied in a laboratory setting.
LEARN ABOUT: Qualitative Research Questions and Questionnaires
Quantitative research methods aid in analyzing the empirical evidence gathered. By using these a researcher can find out if his hypothesis is supported or not.
- Survey research: Survey research generally involves a large audience to collect a large amount of data. This is a quantitative method having a predetermined set of closed questions which are pretty easy to answer. Because of the simplicity of such a method, high responses are achieved. It is one of the most commonly used methods for all kinds of research in today’s world.
Previously, surveys were taken face to face only with maybe a recorder. However, with advancement in technology and for ease, new mediums such as emails , or social media have emerged.
For example: Depletion of energy resources is a growing concern and hence there is a need for awareness about renewable energy. According to recent studies, fossil fuels still account for around 80% of energy consumption in the United States. Even though there is a rise in the use of green energy every year, there are certain parameters because of which the general population is still not opting for green energy. In order to understand why, a survey can be conducted to gather opinions of the general population about green energy and the factors that influence their choice of switching to renewable energy. Such a survey can help institutions or governing bodies to promote appropriate awareness and incentive schemes to push the use of greener energy.
Learn more: Renewable Energy Survey Template Descriptive Research vs Correlational Research
- Experimental research: In experimental research , an experiment is set up and a hypothesis is tested by creating a situation in which one of the variable is manipulated. This is also used to check cause and effect. It is tested to see what happens to the independent variable if the other one is removed or altered. The process for such a method is usually proposing a hypothesis, experimenting on it, analyzing the findings and reporting the findings to understand if it supports the theory or not.
For example: A particular product company is trying to find what is the reason for them to not be able to capture the market. So the organisation makes changes in each one of the processes like manufacturing, marketing, sales and operations. Through the experiment they understand that sales training directly impacts the market coverage for their product. If the person is trained well, then the product will have better coverage.
- Correlational research: Correlational research is used to find relation between two set of variables . Regression analysis is generally used to predict outcomes of such a method. It can be positive, negative or neutral correlation.
LEARN ABOUT: Level of Analysis
For example: Higher educated individuals will get higher paying jobs. This means higher education enables the individual to high paying job and less education will lead to lower paying jobs.
- Longitudinal study: Longitudinal study is used to understand the traits or behavior of a subject under observation after repeatedly testing the subject over a period of time. Data collected from such a method can be qualitative or quantitative in nature.
For example: A research to find out benefits of exercise. The target is asked to exercise everyday for a particular period of time and the results show higher endurance, stamina, and muscle growth. This supports the fact that exercise benefits an individual body.
- Cross sectional: Cross sectional study is an observational type of method, in which a set of audience is observed at a given point in time. In this type, the set of people are chosen in a fashion which depicts similarity in all the variables except the one which is being researched. This type does not enable the researcher to establish a cause and effect relationship as it is not observed for a continuous time period. It is majorly used by healthcare sector or the retail industry.
For example: A medical study to find the prevalence of under-nutrition disorders in kids of a given population. This will involve looking at a wide range of parameters like age, ethnicity, location, incomes and social backgrounds. If a significant number of kids coming from poor families show under-nutrition disorders, the researcher can further investigate into it. Usually a cross sectional study is followed by a longitudinal study to find out the exact reason.
- Causal-Comparative research : This method is based on comparison. It is mainly used to find out cause-effect relationship between two variables or even multiple variables.
For example: A researcher measured the productivity of employees in a company which gave breaks to the employees during work and compared that to the employees of the company which did not give breaks at all.
LEARN ABOUT: Action Research
Some research questions need to be analysed qualitatively, as quantitative methods are not applicable there. In many cases, in-depth information is needed or a researcher may need to observe a target audience behavior, hence the results needed are in a descriptive analysis form. Qualitative research results will be descriptive rather than predictive. It enables the researcher to build or support theories for future potential quantitative research. In such a situation qualitative research methods are used to derive a conclusion to support the theory or hypothesis being studied.
LEARN ABOUT: Qualitative Interview
- Case study: Case study method is used to find more information through carefully analyzing existing cases. It is very often used for business research or to gather empirical evidence for investigation purpose. It is a method to investigate a problem within its real life context through existing cases. The researcher has to carefully analyse making sure the parameter and variables in the existing case are the same as to the case that is being investigated. Using the findings from the case study, conclusions can be drawn regarding the topic that is being studied.
For example: A report mentioning the solution provided by a company to its client. The challenges they faced during initiation and deployment, the findings of the case and solutions they offered for the problems. Such case studies are used by most companies as it forms an empirical evidence for the company to promote in order to get more business.
- Observational method: Observational method is a process to observe and gather data from its target. Since it is a qualitative method it is time consuming and very personal. It can be said that observational research method is a part of ethnographic research which is also used to gather empirical evidence. This is usually a qualitative form of research, however in some cases it can be quantitative as well depending on what is being studied.
For example: setting up a research to observe a particular animal in the rain-forests of amazon. Such a research usually take a lot of time as observation has to be done for a set amount of time to study patterns or behavior of the subject. Another example used widely nowadays is to observe people shopping in a mall to figure out buying behavior of consumers.
- One-on-one interview: Such a method is purely qualitative and one of the most widely used. The reason being it enables a researcher get precise meaningful data if the right questions are asked. It is a conversational method where in-depth data can be gathered depending on where the conversation leads.
For example: A one-on-one interview with the finance minister to gather data on financial policies of the country and its implications on the public.
- Focus groups: Focus groups are used when a researcher wants to find answers to why, what and how questions. A small group is generally chosen for such a method and it is not necessary to interact with the group in person. A moderator is generally needed in case the group is being addressed in person. This is widely used by product companies to collect data about their brands and the product.
For example: A mobile phone manufacturer wanting to have a feedback on the dimensions of one of their models which is yet to be launched. Such studies help the company meet the demand of the customer and position their model appropriately in the market.
- Text analysis: Text analysis method is a little new compared to the other types. Such a method is used to analyse social life by going through images or words used by the individual. In today’s world, with social media playing a major part of everyone’s life, such a method enables the research to follow the pattern that relates to his study.
For example: A lot of companies ask for feedback from the customer in detail mentioning how satisfied are they with their customer support team. Such data enables the researcher to take appropriate decisions to make their support team better.
Sometimes a combination of the methods is also needed for some questions that cannot be answered using only one type of method especially when a researcher needs to gain a complete understanding of complex subject matter.
We recently published a blog that talks about examples of qualitative data in education ; why don’t you check it out for more ideas?
Since empirical research is based on observation and capturing experiences, it is important to plan the steps to conduct the experiment and how to analyse it. This will enable the researcher to resolve problems or obstacles which can occur during the experiment.
Step #1: Define the purpose of the research
This is the step where the researcher has to answer questions like what exactly do I want to find out? What is the problem statement? Are there any issues in terms of the availability of knowledge, data, time or resources. Will this research be more beneficial than what it will cost.
Before going ahead, a researcher has to clearly define his purpose for the research and set up a plan to carry out further tasks.
Step #2 : Supporting theories and relevant literature
The researcher needs to find out if there are theories which can be linked to his research problem . He has to figure out if any theory can help him support his findings. All kind of relevant literature will help the researcher to find if there are others who have researched this before, or what are the problems faced during this research. The researcher will also have to set up assumptions and also find out if there is any history regarding his research problem
Step #3: Creation of Hypothesis and measurement
Before beginning the actual research he needs to provide himself a working hypothesis or guess what will be the probable result. Researcher has to set up variables, decide the environment for the research and find out how can he relate between the variables.
Researcher will also need to define the units of measurements, tolerable degree for errors, and find out if the measurement chosen will be acceptable by others.
Step #4: Methodology, research design and data collection
In this step, the researcher has to define a strategy for conducting his research. He has to set up experiments to collect data which will enable him to propose the hypothesis. The researcher will decide whether he will need experimental or non experimental method for conducting the research. The type of research design will vary depending on the field in which the research is being conducted. Last but not the least, the researcher will have to find out parameters that will affect the validity of the research design. Data collection will need to be done by choosing appropriate samples depending on the research question. To carry out the research, he can use one of the many sampling techniques. Once data collection is complete, researcher will have empirical data which needs to be analysed.
LEARN ABOUT: Best Data Collection Tools
Step #5: Data Analysis and result
Data analysis can be done in two ways, qualitatively and quantitatively. Researcher will need to find out what qualitative method or quantitative method will be needed or will he need a combination of both. Depending on the unit of analysis of his data, he will know if his hypothesis is supported or rejected. Analyzing this data is the most important part to support his hypothesis.
Step #6: Conclusion
A report will need to be made with the findings of the research. The researcher can give the theories and literature that support his research. He can make suggestions or recommendations for further research on his topic.
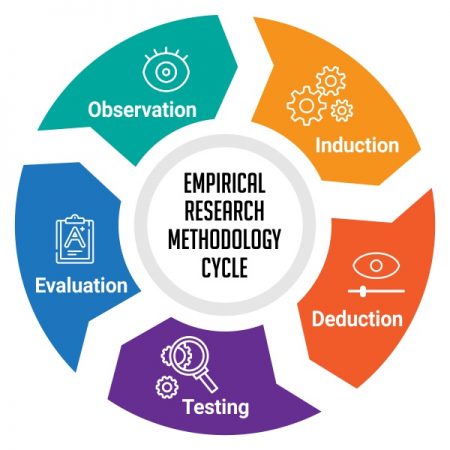
A.D. de Groot, a famous dutch psychologist and a chess expert conducted some of the most notable experiments using chess in the 1940’s. During his study, he came up with a cycle which is consistent and now widely used to conduct empirical research. It consists of 5 phases with each phase being as important as the next one. The empirical cycle captures the process of coming up with hypothesis about how certain subjects work or behave and then testing these hypothesis against empirical data in a systematic and rigorous approach. It can be said that it characterizes the deductive approach to science. Following is the empirical cycle.
- Observation: At this phase an idea is sparked for proposing a hypothesis. During this phase empirical data is gathered using observation. For example: a particular species of flower bloom in a different color only during a specific season.
- Induction: Inductive reasoning is then carried out to form a general conclusion from the data gathered through observation. For example: As stated above it is observed that the species of flower blooms in a different color during a specific season. A researcher may ask a question “does the temperature in the season cause the color change in the flower?” He can assume that is the case, however it is a mere conjecture and hence an experiment needs to be set up to support this hypothesis. So he tags a few set of flowers kept at a different temperature and observes if they still change the color?
- Deduction: This phase helps the researcher to deduce a conclusion out of his experiment. This has to be based on logic and rationality to come up with specific unbiased results.For example: In the experiment, if the tagged flowers in a different temperature environment do not change the color then it can be concluded that temperature plays a role in changing the color of the bloom.
- Testing: This phase involves the researcher to return to empirical methods to put his hypothesis to the test. The researcher now needs to make sense of his data and hence needs to use statistical analysis plans to determine the temperature and bloom color relationship. If the researcher finds out that most flowers bloom a different color when exposed to the certain temperature and the others do not when the temperature is different, he has found support to his hypothesis. Please note this not proof but just a support to his hypothesis.
- Evaluation: This phase is generally forgotten by most but is an important one to keep gaining knowledge. During this phase the researcher puts forth the data he has collected, the support argument and his conclusion. The researcher also states the limitations for the experiment and his hypothesis and suggests tips for others to pick it up and continue a more in-depth research for others in the future. LEARN MORE: Population vs Sample
LEARN MORE: Population vs Sample
There is a reason why empirical research is one of the most widely used method. There are a few advantages associated with it. Following are a few of them.
- It is used to authenticate traditional research through various experiments and observations.
- This research methodology makes the research being conducted more competent and authentic.
- It enables a researcher understand the dynamic changes that can happen and change his strategy accordingly.
- The level of control in such a research is high so the researcher can control multiple variables.
- It plays a vital role in increasing internal validity .
Even though empirical research makes the research more competent and authentic, it does have a few disadvantages. Following are a few of them.
- Such a research needs patience as it can be very time consuming. The researcher has to collect data from multiple sources and the parameters involved are quite a few, which will lead to a time consuming research.
- Most of the time, a researcher will need to conduct research at different locations or in different environments, this can lead to an expensive affair.
- There are a few rules in which experiments can be performed and hence permissions are needed. Many a times, it is very difficult to get certain permissions to carry out different methods of this research.
- Collection of data can be a problem sometimes, as it has to be collected from a variety of sources through different methods.
LEARN ABOUT: Social Communication Questionnaire
Empirical research is important in today’s world because most people believe in something only that they can see, hear or experience. It is used to validate multiple hypothesis and increase human knowledge and continue doing it to keep advancing in various fields.
For example: Pharmaceutical companies use empirical research to try out a specific drug on controlled groups or random groups to study the effect and cause. This way, they prove certain theories they had proposed for the specific drug. Such research is very important as sometimes it can lead to finding a cure for a disease that has existed for many years. It is useful in science and many other fields like history, social sciences, business, etc.
LEARN ABOUT: 12 Best Tools for Researchers
With the advancement in today’s world, empirical research has become critical and a norm in many fields to support their hypothesis and gain more knowledge. The methods mentioned above are very useful for carrying out such research. However, a number of new methods will keep coming up as the nature of new investigative questions keeps getting unique or changing.
Create a single source of real data with a built-for-insights platform. Store past data, add nuggets of insights, and import research data from various sources into a CRM for insights. Build on ever-growing research with a real-time dashboard in a unified research management platform to turn insights into knowledge.
LEARN MORE FREE TRIAL
MORE LIKE THIS
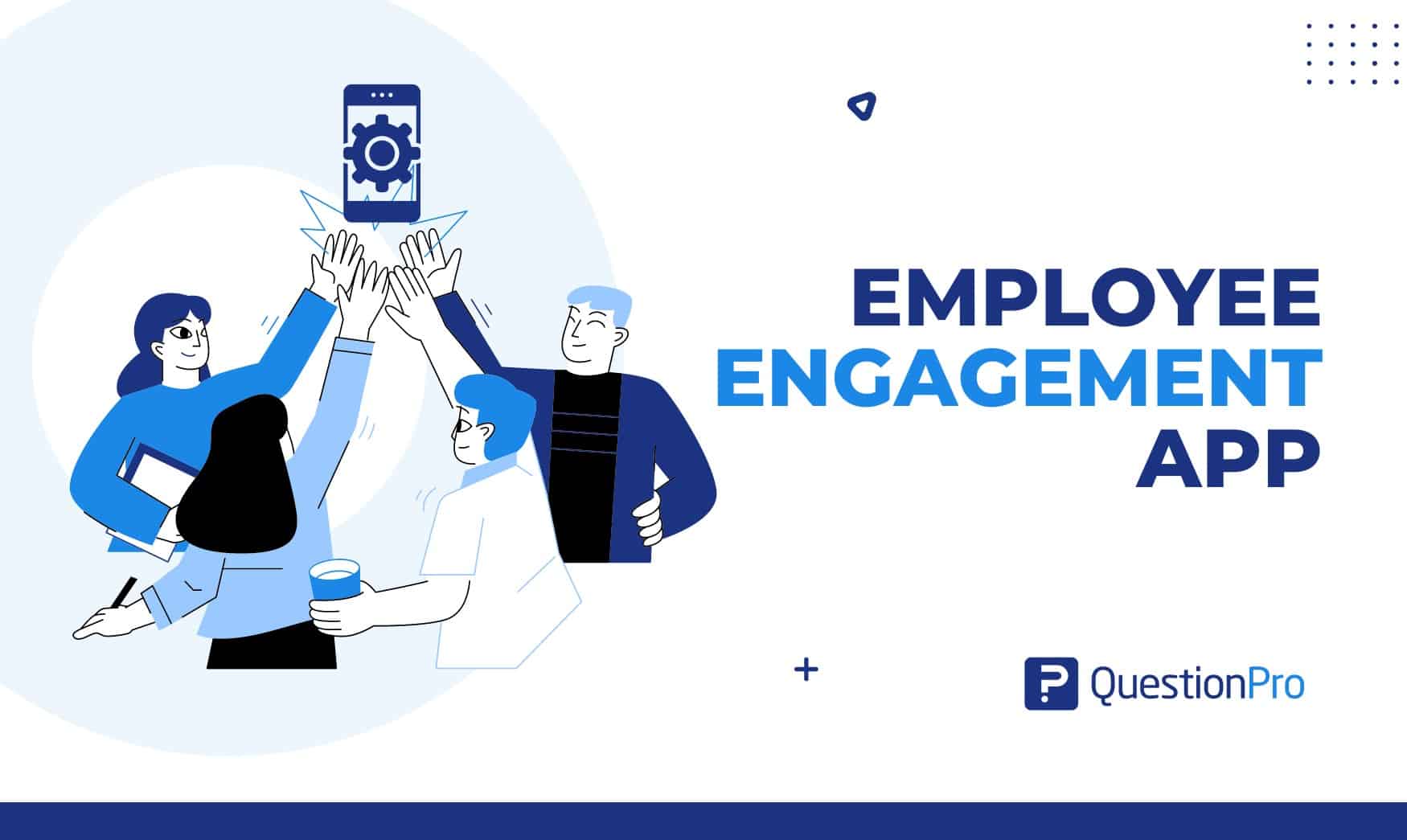
Employee Engagement App: Top 11 For Workforce Improvement
Apr 10, 2024
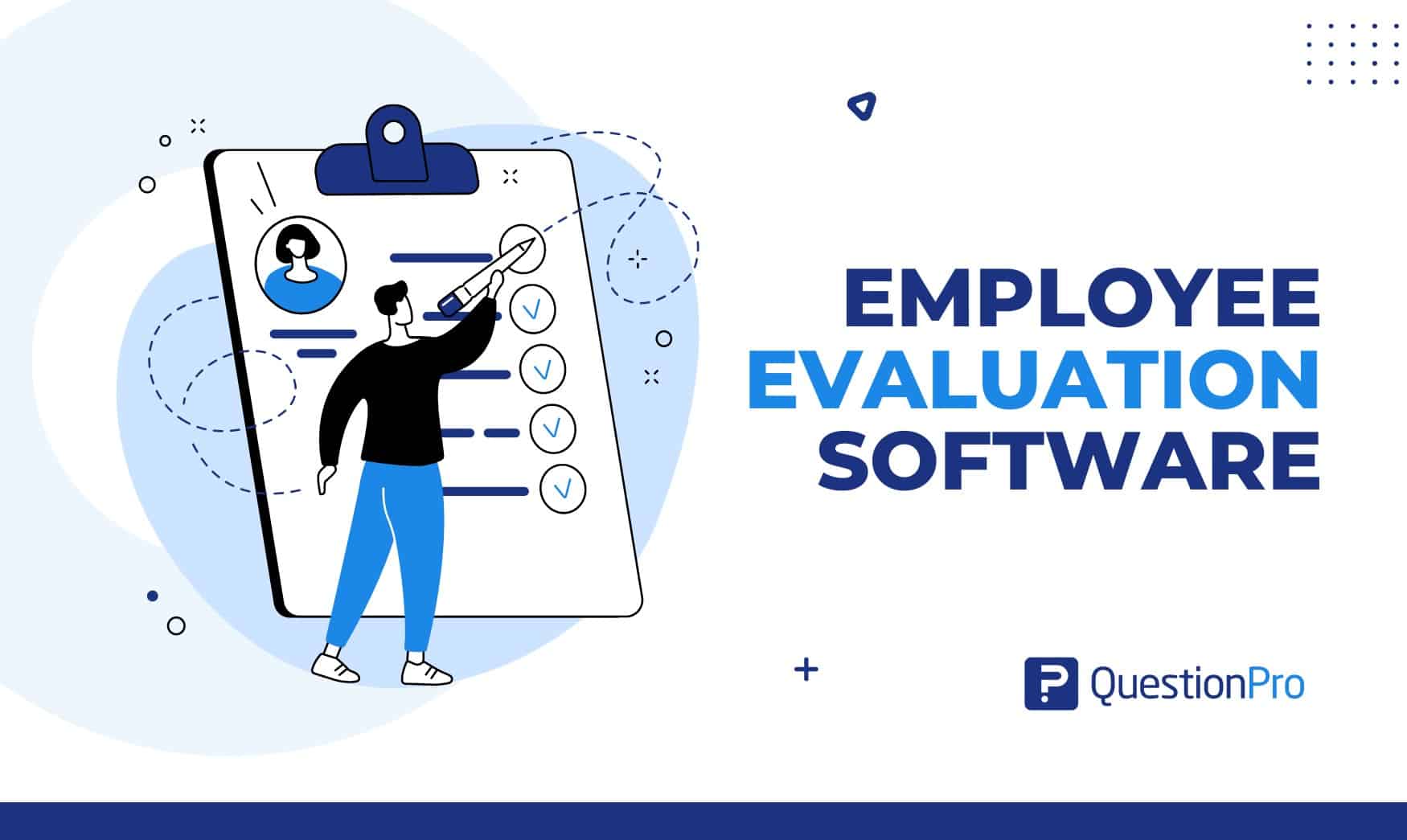
Top 15 Employee Evaluation Software to Enhance Performance
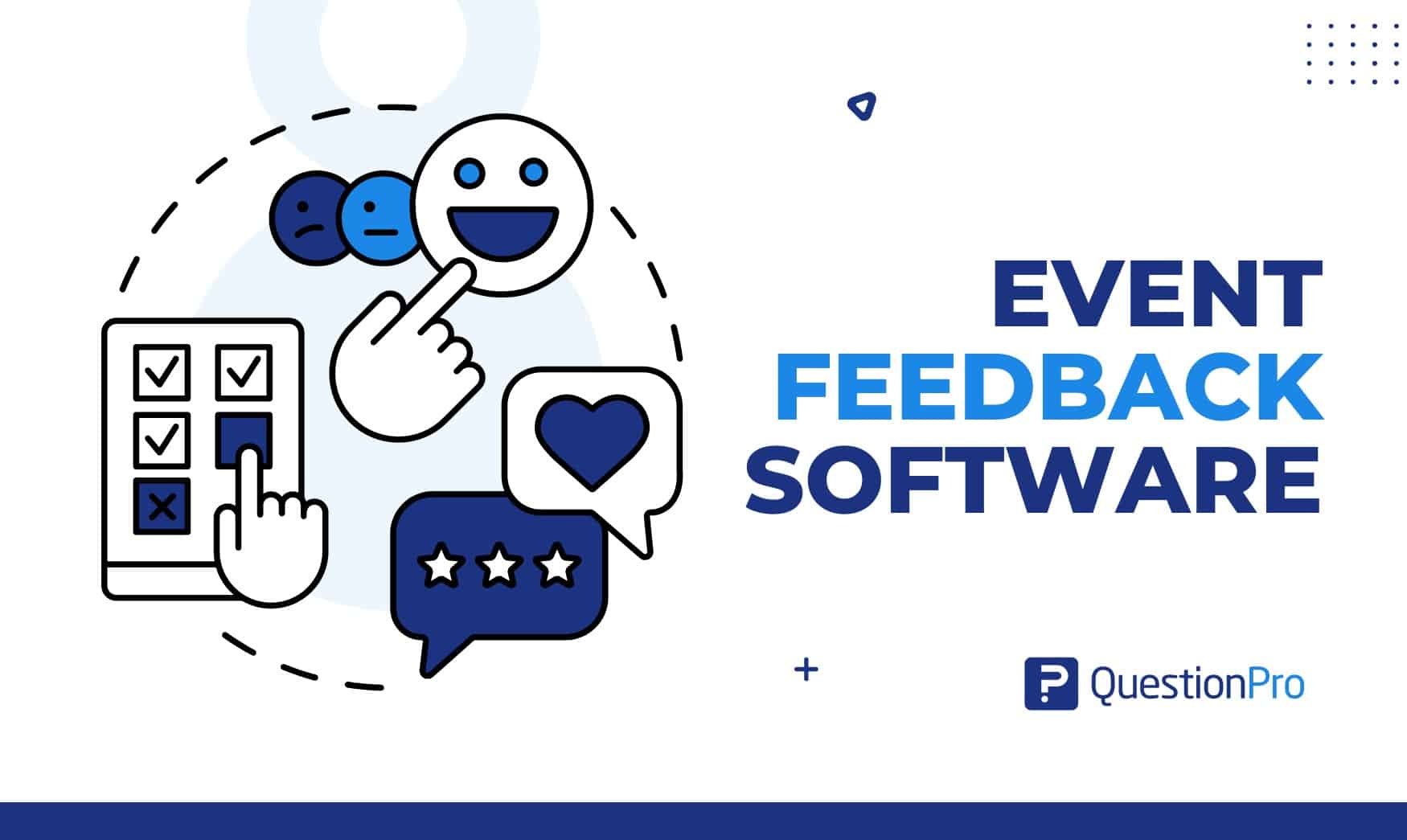
Event Feedback Software: Top 11 Best in 2024
Apr 9, 2024
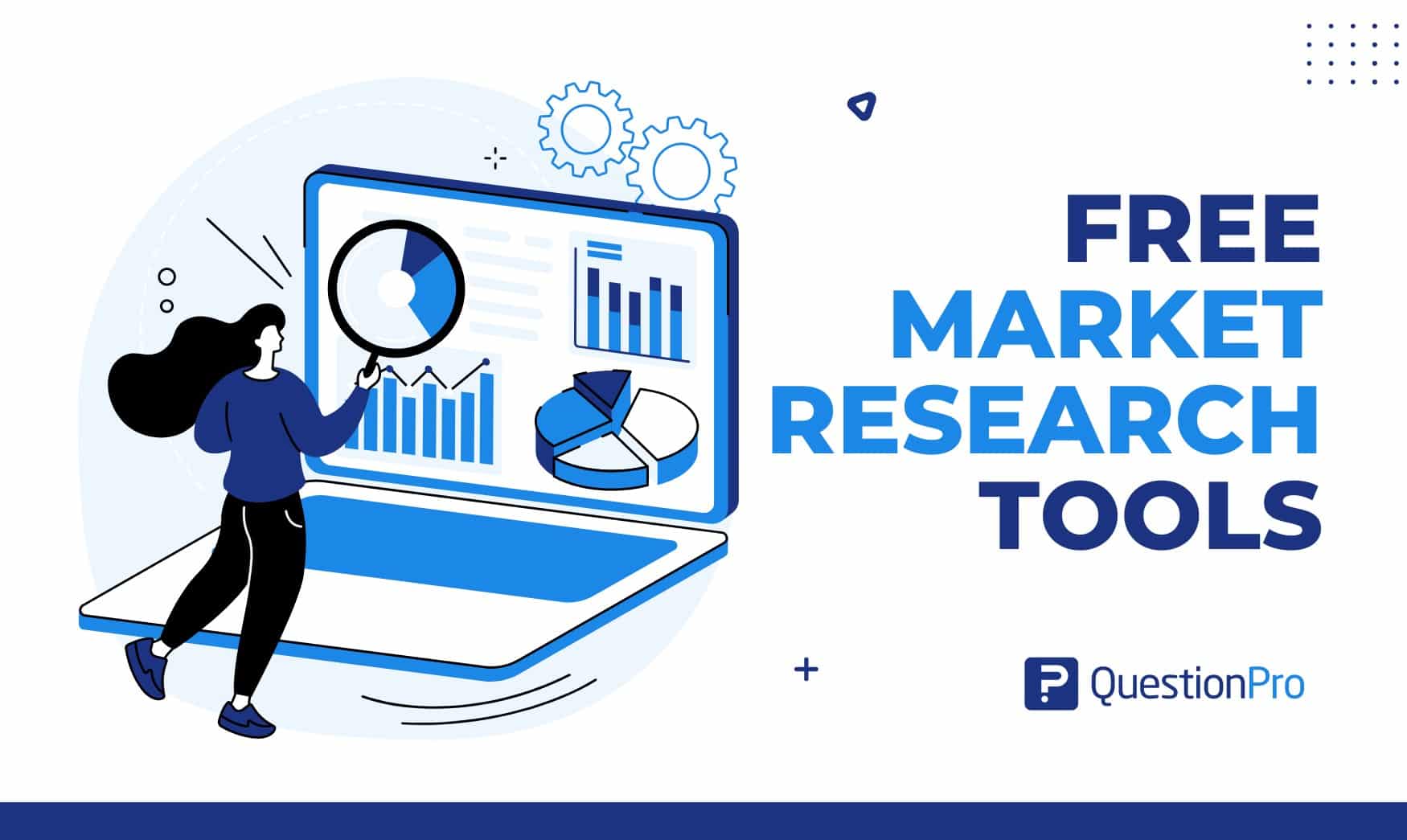
Top 10 Free Market Research Tools to Boost Your Business
Other categories.
- Academic Research
- Artificial Intelligence
- Assessments
- Brand Awareness
- Case Studies
- Communities
- Consumer Insights
- Customer effort score
- Customer Engagement
- Customer Experience
- Customer Loyalty
- Customer Research
- Customer Satisfaction
- Employee Benefits
- Employee Engagement
- Employee Retention
- Friday Five
- General Data Protection Regulation
- Insights Hub
- Life@QuestionPro
- Market Research
- Mobile diaries
- Mobile Surveys
- New Features
- Online Communities
- Question Types
- Questionnaire
- QuestionPro Products
- Release Notes
- Research Tools and Apps
- Revenue at Risk
- Survey Templates
- Training Tips
- Uncategorized
- Video Learning Series
- What’s Coming Up
- Workforce Intelligence

- Ask a Librarian
Research: Overview & Approaches
- Getting Started with Undergraduate Research
- Planning & Getting Started
- Building Your Knowledge Base
- Locating Sources
- Reading Scholarly Articles
- Creating a Literature Review
- Productivity & Organizing Research
- Scholarly and Professional Relationships
Introduction to Empirical Research
Databases for finding empirical research, guided search, google scholar, examples of empirical research, sources and further reading.
- Interpretive Research
- Action-Based Research
- Creative & Experimental Approaches
Your Librarian

- Introductory Video This video covers what empirical research is, what kinds of questions and methods empirical researchers use, and some tips for finding empirical research articles in your discipline.

- Guided Search: Finding Empirical Research Articles This is a hands-on tutorial that will allow you to use your own search terms to find resources.

- Study on radiation transfer in human skin for cosmetics
- Long-Term Mobile Phone Use and the Risk of Vestibular Schwannoma: A Danish Nationwide Cohort Study
- Emissions Impacts and Benefits of Plug-In Hybrid Electric Vehicles and Vehicle-to-Grid Services
- Review of design considerations and technological challenges for successful development and deployment of plug-in hybrid electric vehicles
- Endocrine disrupters and human health: could oestrogenic chemicals in body care cosmetics adversely affect breast cancer incidence in women?
- << Previous: Scholarly and Professional Relationships
- Next: Interpretive Research >>
- Last Updated: Apr 5, 2024 9:55 AM
- URL: https://guides.lib.purdue.edu/research_approaches
What is Empirical Research? Definition, Methods, Examples
Appinio Research · 09.02.2024 · 35min read
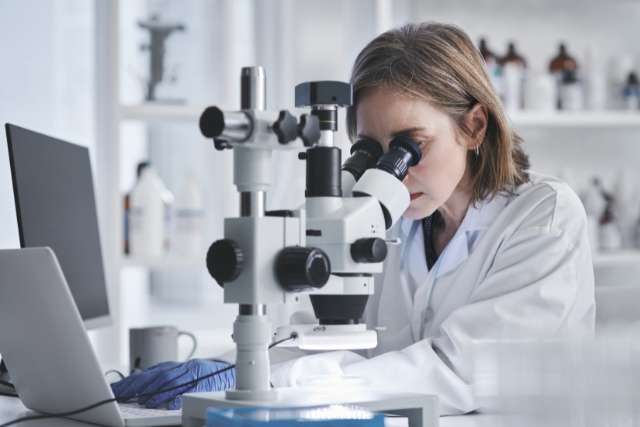
Ever wondered how we gather the facts, unveil hidden truths, and make informed decisions in a world filled with questions? Empirical research holds the key.
In this guide, we'll delve deep into the art and science of empirical research, unraveling its methods, mysteries, and manifold applications. From defining the core principles to mastering data analysis and reporting findings, we're here to equip you with the knowledge and tools to navigate the empirical landscape.
What is Empirical Research?
Empirical research is the cornerstone of scientific inquiry, providing a systematic and structured approach to investigating the world around us. It is the process of gathering and analyzing empirical or observable data to test hypotheses, answer research questions, or gain insights into various phenomena. This form of research relies on evidence derived from direct observation or experimentation, allowing researchers to draw conclusions based on real-world data rather than purely theoretical or speculative reasoning.
Characteristics of Empirical Research
Empirical research is characterized by several key features:
- Observation and Measurement : It involves the systematic observation or measurement of variables, events, or behaviors.
- Data Collection : Researchers collect data through various methods, such as surveys, experiments, observations, or interviews.
- Testable Hypotheses : Empirical research often starts with testable hypotheses that are evaluated using collected data.
- Quantitative or Qualitative Data : Data can be quantitative (numerical) or qualitative (non-numerical), depending on the research design.
- Statistical Analysis : Quantitative data often undergo statistical analysis to determine patterns , relationships, or significance.
- Objectivity and Replicability : Empirical research strives for objectivity, minimizing researcher bias . It should be replicable, allowing other researchers to conduct the same study to verify results.
- Conclusions and Generalizations : Empirical research generates findings based on data and aims to make generalizations about larger populations or phenomena.
Importance of Empirical Research
Empirical research plays a pivotal role in advancing knowledge across various disciplines. Its importance extends to academia, industry, and society as a whole. Here are several reasons why empirical research is essential:
- Evidence-Based Knowledge : Empirical research provides a solid foundation of evidence-based knowledge. It enables us to test hypotheses, confirm or refute theories, and build a robust understanding of the world.
- Scientific Progress : In the scientific community, empirical research fuels progress by expanding the boundaries of existing knowledge. It contributes to the development of theories and the formulation of new research questions.
- Problem Solving : Empirical research is instrumental in addressing real-world problems and challenges. It offers insights and data-driven solutions to complex issues in fields like healthcare, economics, and environmental science.
- Informed Decision-Making : In policymaking, business, and healthcare, empirical research informs decision-makers by providing data-driven insights. It guides strategies, investments, and policies for optimal outcomes.
- Quality Assurance : Empirical research is essential for quality assurance and validation in various industries, including pharmaceuticals, manufacturing, and technology. It ensures that products and processes meet established standards.
- Continuous Improvement : Businesses and organizations use empirical research to evaluate performance, customer satisfaction, and product effectiveness. This data-driven approach fosters continuous improvement and innovation.
- Human Advancement : Empirical research in fields like medicine and psychology contributes to the betterment of human health and well-being. It leads to medical breakthroughs, improved therapies, and enhanced psychological interventions.
- Critical Thinking and Problem Solving : Engaging in empirical research fosters critical thinking skills, problem-solving abilities, and a deep appreciation for evidence-based decision-making.
Empirical research empowers us to explore, understand, and improve the world around us. It forms the bedrock of scientific inquiry and drives progress in countless domains, shaping our understanding of both the natural and social sciences.
How to Conduct Empirical Research?
So, you've decided to dive into the world of empirical research. Let's begin by exploring the crucial steps involved in getting started with your research project.
1. Select a Research Topic
Selecting the right research topic is the cornerstone of a successful empirical study. It's essential to choose a topic that not only piques your interest but also aligns with your research goals and objectives. Here's how to go about it:
- Identify Your Interests : Start by reflecting on your passions and interests. What topics fascinate you the most? Your enthusiasm will be your driving force throughout the research process.
- Brainstorm Ideas : Engage in brainstorming sessions to generate potential research topics. Consider the questions you've always wanted to answer or the issues that intrigue you.
- Relevance and Significance : Assess the relevance and significance of your chosen topic. Does it contribute to existing knowledge? Is it a pressing issue in your field of study or the broader community?
- Feasibility : Evaluate the feasibility of your research topic. Do you have access to the necessary resources, data, and participants (if applicable)?
2. Formulate Research Questions
Once you've narrowed down your research topic, the next step is to formulate clear and precise research questions . These questions will guide your entire research process and shape your study's direction. To create effective research questions:
- Specificity : Ensure that your research questions are specific and focused. Vague or overly broad questions can lead to inconclusive results.
- Relevance : Your research questions should directly relate to your chosen topic. They should address gaps in knowledge or contribute to solving a particular problem.
- Testability : Ensure that your questions are testable through empirical methods. You should be able to gather data and analyze it to answer these questions.
- Avoid Bias : Craft your questions in a way that avoids leading or biased language. Maintain neutrality to uphold the integrity of your research.
3. Review Existing Literature
Before you embark on your empirical research journey, it's essential to immerse yourself in the existing body of literature related to your chosen topic. This step, often referred to as a literature review, serves several purposes:
- Contextualization : Understand the historical context and current state of research in your field. What have previous studies found, and what questions remain unanswered?
- Identifying Gaps : Identify gaps or areas where existing research falls short. These gaps will help you formulate meaningful research questions and hypotheses.
- Theory Development : If your study is theoretical, consider how existing theories apply to your topic. If it's empirical, understand how previous studies have approached data collection and analysis.
- Methodological Insights : Learn from the methodologies employed in previous research. What methods were successful, and what challenges did researchers face?
4. Define Variables
Variables are fundamental components of empirical research. They are the factors or characteristics that can change or be manipulated during your study. Properly defining and categorizing variables is crucial for the clarity and validity of your research. Here's what you need to know:
- Independent Variables : These are the variables that you, as the researcher, manipulate or control. They are the "cause" in cause-and-effect relationships.
- Dependent Variables : Dependent variables are the outcomes or responses that you measure or observe. They are the "effect" influenced by changes in independent variables.
- Operational Definitions : To ensure consistency and clarity, provide operational definitions for your variables. Specify how you will measure or manipulate each variable.
- Control Variables : In some studies, controlling for other variables that may influence your dependent variable is essential. These are known as control variables.
Understanding these foundational aspects of empirical research will set a solid foundation for the rest of your journey. Now that you've grasped the essentials of getting started, let's delve deeper into the intricacies of research design.
Empirical Research Design
Now that you've selected your research topic, formulated research questions, and defined your variables, it's time to delve into the heart of your empirical research journey – research design . This pivotal step determines how you will collect data and what methods you'll employ to answer your research questions. Let's explore the various facets of research design in detail.
Types of Empirical Research
Empirical research can take on several forms, each with its own unique approach and methodologies. Understanding the different types of empirical research will help you choose the most suitable design for your study. Here are some common types:
- Experimental Research : In this type, researchers manipulate one or more independent variables to observe their impact on dependent variables. It's highly controlled and often conducted in a laboratory setting.
- Observational Research : Observational research involves the systematic observation of subjects or phenomena without intervention. Researchers are passive observers, documenting behaviors, events, or patterns.
- Survey Research : Surveys are used to collect data through structured questionnaires or interviews. This method is efficient for gathering information from a large number of participants.
- Case Study Research : Case studies focus on in-depth exploration of one or a few cases. Researchers gather detailed information through various sources such as interviews, documents, and observations.
- Qualitative Research : Qualitative research aims to understand behaviors, experiences, and opinions in depth. It often involves open-ended questions, interviews, and thematic analysis.
- Quantitative Research : Quantitative research collects numerical data and relies on statistical analysis to draw conclusions. It involves structured questionnaires, experiments, and surveys.
Your choice of research type should align with your research questions and objectives. Experimental research, for example, is ideal for testing cause-and-effect relationships, while qualitative research is more suitable for exploring complex phenomena.
Experimental Design
Experimental research is a systematic approach to studying causal relationships. It's characterized by the manipulation of one or more independent variables while controlling for other factors. Here are some key aspects of experimental design:
- Control and Experimental Groups : Participants are randomly assigned to either a control group or an experimental group. The independent variable is manipulated for the experimental group but not for the control group.
- Randomization : Randomization is crucial to eliminate bias in group assignment. It ensures that each participant has an equal chance of being in either group.
- Hypothesis Testing : Experimental research often involves hypothesis testing. Researchers formulate hypotheses about the expected effects of the independent variable and use statistical analysis to test these hypotheses.
Observational Design
Observational research entails careful and systematic observation of subjects or phenomena. It's advantageous when you want to understand natural behaviors or events. Key aspects of observational design include:
- Participant Observation : Researchers immerse themselves in the environment they are studying. They become part of the group being observed, allowing for a deep understanding of behaviors.
- Non-Participant Observation : In non-participant observation, researchers remain separate from the subjects. They observe and document behaviors without direct involvement.
- Data Collection Methods : Observational research can involve various data collection methods, such as field notes, video recordings, photographs, or coding of observed behaviors.
Survey Design
Surveys are a popular choice for collecting data from a large number of participants. Effective survey design is essential to ensure the validity and reliability of your data. Consider the following:
- Questionnaire Design : Create clear and concise questions that are easy for participants to understand. Avoid leading or biased questions.
- Sampling Methods : Decide on the appropriate sampling method for your study, whether it's random, stratified, or convenience sampling.
- Data Collection Tools : Choose the right tools for data collection, whether it's paper surveys, online questionnaires, or face-to-face interviews.
Case Study Design
Case studies are an in-depth exploration of one or a few cases to gain a deep understanding of a particular phenomenon. Key aspects of case study design include:
- Single Case vs. Multiple Case Studies : Decide whether you'll focus on a single case or multiple cases. Single case studies are intensive and allow for detailed examination, while multiple case studies provide comparative insights.
- Data Collection Methods : Gather data through interviews, observations, document analysis, or a combination of these methods.
Qualitative vs. Quantitative Research
In empirical research, you'll often encounter the distinction between qualitative and quantitative research . Here's a closer look at these two approaches:
- Qualitative Research : Qualitative research seeks an in-depth understanding of human behavior, experiences, and perspectives. It involves open-ended questions, interviews, and the analysis of textual or narrative data. Qualitative research is exploratory and often used when the research question is complex and requires a nuanced understanding.
- Quantitative Research : Quantitative research collects numerical data and employs statistical analysis to draw conclusions. It involves structured questionnaires, experiments, and surveys. Quantitative research is ideal for testing hypotheses and establishing cause-and-effect relationships.
Understanding the various research design options is crucial in determining the most appropriate approach for your study. Your choice should align with your research questions, objectives, and the nature of the phenomenon you're investigating.
Data Collection for Empirical Research
Now that you've established your research design, it's time to roll up your sleeves and collect the data that will fuel your empirical research. Effective data collection is essential for obtaining accurate and reliable results.
Sampling Methods
Sampling methods are critical in empirical research, as they determine the subset of individuals or elements from your target population that you will study. Here are some standard sampling methods:
- Random Sampling : Random sampling ensures that every member of the population has an equal chance of being selected. It minimizes bias and is often used in quantitative research.
- Stratified Sampling : Stratified sampling involves dividing the population into subgroups or strata based on specific characteristics (e.g., age, gender, location). Samples are then randomly selected from each stratum, ensuring representation of all subgroups.
- Convenience Sampling : Convenience sampling involves selecting participants who are readily available or easily accessible. While it's convenient, it may introduce bias and limit the generalizability of results.
- Snowball Sampling : Snowball sampling is instrumental when studying hard-to-reach or hidden populations. One participant leads you to another, creating a "snowball" effect. This method is common in qualitative research.
- Purposive Sampling : In purposive sampling, researchers deliberately select participants who meet specific criteria relevant to their research questions. It's often used in qualitative studies to gather in-depth information.
The choice of sampling method depends on the nature of your research, available resources, and the degree of precision required. It's crucial to carefully consider your sampling strategy to ensure that your sample accurately represents your target population.
Data Collection Instruments
Data collection instruments are the tools you use to gather information from your participants or sources. These instruments should be designed to capture the data you need accurately. Here are some popular data collection instruments:
- Questionnaires : Questionnaires consist of structured questions with predefined response options. When designing questionnaires, consider the clarity of questions, the order of questions, and the response format (e.g., Likert scale, multiple-choice).
- Interviews : Interviews involve direct communication between the researcher and participants. They can be structured (with predetermined questions) or unstructured (open-ended). Effective interviews require active listening and probing for deeper insights.
- Observations : Observations entail systematically and objectively recording behaviors, events, or phenomena. Researchers must establish clear criteria for what to observe, how to record observations, and when to observe.
- Surveys : Surveys are a common data collection instrument for quantitative research. They can be administered through various means, including online surveys, paper surveys, and telephone surveys.
- Documents and Archives : In some cases, data may be collected from existing documents, records, or archives. Ensure that the sources are reliable, relevant, and properly documented.
To streamline your process and gather insights with precision and efficiency, consider leveraging innovative tools like Appinio . With Appinio's intuitive platform, you can harness the power of real-time consumer data to inform your research decisions effectively. Whether you're conducting surveys, interviews, or observations, Appinio empowers you to define your target audience, collect data from diverse demographics, and analyze results seamlessly.
By incorporating Appinio into your data collection toolkit, you can unlock a world of possibilities and elevate the impact of your empirical research. Ready to revolutionize your approach to data collection?
Book a Demo
Data Collection Procedures
Data collection procedures outline the step-by-step process for gathering data. These procedures should be meticulously planned and executed to maintain the integrity of your research.
- Training : If you have a research team, ensure that they are trained in data collection methods and protocols. Consistency in data collection is crucial.
- Pilot Testing : Before launching your data collection, conduct a pilot test with a small group to identify any potential problems with your instruments or procedures. Make necessary adjustments based on feedback.
- Data Recording : Establish a systematic method for recording data. This may include timestamps, codes, or identifiers for each data point.
- Data Security : Safeguard the confidentiality and security of collected data. Ensure that only authorized individuals have access to the data.
- Data Storage : Properly organize and store your data in a secure location, whether in physical or digital form. Back up data to prevent loss.
Ethical Considerations
Ethical considerations are paramount in empirical research, as they ensure the well-being and rights of participants are protected.
- Informed Consent : Obtain informed consent from participants, providing clear information about the research purpose, procedures, risks, and their right to withdraw at any time.
- Privacy and Confidentiality : Protect the privacy and confidentiality of participants. Ensure that data is anonymized and sensitive information is kept confidential.
- Beneficence : Ensure that your research benefits participants and society while minimizing harm. Consider the potential risks and benefits of your study.
- Honesty and Integrity : Conduct research with honesty and integrity. Report findings accurately and transparently, even if they are not what you expected.
- Respect for Participants : Treat participants with respect, dignity, and sensitivity to cultural differences. Avoid any form of coercion or manipulation.
- Institutional Review Board (IRB) : If required, seek approval from an IRB or ethics committee before conducting your research, particularly when working with human participants.
Adhering to ethical guidelines is not only essential for the ethical conduct of research but also crucial for the credibility and validity of your study. Ethical research practices build trust between researchers and participants and contribute to the advancement of knowledge with integrity.
With a solid understanding of data collection, including sampling methods, instruments, procedures, and ethical considerations, you are now well-equipped to gather the data needed to answer your research questions.
Empirical Research Data Analysis
Now comes the exciting phase of data analysis, where the raw data you've diligently collected starts to yield insights and answers to your research questions. We will explore the various aspects of data analysis, from preparing your data to drawing meaningful conclusions through statistics and visualization.
Data Preparation
Data preparation is the crucial first step in data analysis. It involves cleaning, organizing, and transforming your raw data into a format that is ready for analysis. Effective data preparation ensures the accuracy and reliability of your results.
- Data Cleaning : Identify and rectify errors, missing values, and inconsistencies in your dataset. This may involve correcting typos, removing outliers, and imputing missing data.
- Data Coding : Assign numerical values or codes to categorical variables to make them suitable for statistical analysis. For example, converting "Yes" and "No" to 1 and 0.
- Data Transformation : Transform variables as needed to meet the assumptions of the statistical tests you plan to use. Common transformations include logarithmic or square root transformations.
- Data Integration : If your data comes from multiple sources, integrate it into a unified dataset, ensuring that variables match and align.
- Data Documentation : Maintain clear documentation of all data preparation steps, as well as the rationale behind each decision. This transparency is essential for replicability.
Effective data preparation lays the foundation for accurate and meaningful analysis. It allows you to trust the results that will follow in the subsequent stages.
Descriptive Statistics
Descriptive statistics help you summarize and make sense of your data by providing a clear overview of its key characteristics. These statistics are essential for understanding the central tendencies, variability, and distribution of your variables. Descriptive statistics include:
- Measures of Central Tendency : These include the mean (average), median (middle value), and mode (most frequent value). They help you understand the typical or central value of your data.
- Measures of Dispersion : Measures like the range, variance, and standard deviation provide insights into the spread or variability of your data points.
- Frequency Distributions : Creating frequency distributions or histograms allows you to visualize the distribution of your data across different values or categories.
Descriptive statistics provide the initial insights needed to understand your data's basic characteristics, which can inform further analysis.
Inferential Statistics
Inferential statistics take your analysis to the next level by allowing you to make inferences or predictions about a larger population based on your sample data. These methods help you test hypotheses and draw meaningful conclusions. Key concepts in inferential statistics include:
- Hypothesis Testing : Hypothesis tests (e.g., t-tests, chi-squared tests) help you determine whether observed differences or associations in your data are statistically significant or occurred by chance.
- Confidence Intervals : Confidence intervals provide a range within which population parameters (e.g., population mean) are likely to fall based on your sample data.
- Regression Analysis : Regression models (linear, logistic, etc.) help you explore relationships between variables and make predictions.
- Analysis of Variance (ANOVA) : ANOVA tests are used to compare means between multiple groups, allowing you to assess whether differences are statistically significant.
Inferential statistics are powerful tools for drawing conclusions from your data and assessing the generalizability of your findings to the broader population.
Qualitative Data Analysis
Qualitative data analysis is employed when working with non-numerical data, such as text, interviews, or open-ended survey responses. It focuses on understanding the underlying themes, patterns, and meanings within qualitative data. Qualitative analysis techniques include:
- Thematic Analysis : Identifying and analyzing recurring themes or patterns within textual data.
- Content Analysis : Categorizing and coding qualitative data to extract meaningful insights.
- Grounded Theory : Developing theories or frameworks based on emergent themes from the data.
- Narrative Analysis : Examining the structure and content of narratives to uncover meaning.
Qualitative data analysis provides a rich and nuanced understanding of complex phenomena and human experiences.
Data Visualization
Data visualization is the art of representing data graphically to make complex information more understandable and accessible. Effective data visualization can reveal patterns, trends, and outliers in your data. Common types of data visualization include:
- Bar Charts and Histograms : Used to display the distribution of categorical or discrete data.
- Line Charts : Ideal for showing trends and changes in data over time.
- Scatter Plots : Visualize relationships and correlations between two variables.
- Pie Charts : Display the composition of a whole in terms of its parts.
- Heatmaps : Depict patterns and relationships in multidimensional data through color-coding.
- Box Plots : Provide a summary of the data distribution, including outliers.
- Interactive Dashboards : Create dynamic visualizations that allow users to explore data interactively.
Data visualization not only enhances your understanding of the data but also serves as a powerful communication tool to convey your findings to others.
As you embark on the data analysis phase of your empirical research, remember that the specific methods and techniques you choose will depend on your research questions, data type, and objectives. Effective data analysis transforms raw data into valuable insights, bringing you closer to the answers you seek.
How to Report Empirical Research Results?
At this stage, you get to share your empirical research findings with the world. Effective reporting and presentation of your results are crucial for communicating your research's impact and insights.
1. Write the Research Paper
Writing a research paper is the culmination of your empirical research journey. It's where you synthesize your findings, provide context, and contribute to the body of knowledge in your field.
- Title and Abstract : Craft a clear and concise title that reflects your research's essence. The abstract should provide a brief summary of your research objectives, methods, findings, and implications.
- Introduction : In the introduction, introduce your research topic, state your research questions or hypotheses, and explain the significance of your study. Provide context by discussing relevant literature.
- Methods : Describe your research design, data collection methods, and sampling procedures. Be precise and transparent, allowing readers to understand how you conducted your study.
- Results : Present your findings in a clear and organized manner. Use tables, graphs, and statistical analyses to support your results. Avoid interpreting your findings in this section; focus on the presentation of raw data.
- Discussion : Interpret your findings and discuss their implications. Relate your results to your research questions and the existing literature. Address any limitations of your study and suggest avenues for future research.
- Conclusion : Summarize the key points of your research and its significance. Restate your main findings and their implications.
- References : Cite all sources used in your research following a specific citation style (e.g., APA, MLA, Chicago). Ensure accuracy and consistency in your citations.
- Appendices : Include any supplementary material, such as questionnaires, data coding sheets, or additional analyses, in the appendices.
Writing a research paper is a skill that improves with practice. Ensure clarity, coherence, and conciseness in your writing to make your research accessible to a broader audience.
2. Create Visuals and Tables
Visuals and tables are powerful tools for presenting complex data in an accessible and understandable manner.
- Clarity : Ensure that your visuals and tables are clear and easy to interpret. Use descriptive titles and labels.
- Consistency : Maintain consistency in formatting, such as font size and style, across all visuals and tables.
- Appropriateness : Choose the most suitable visual representation for your data. Bar charts, line graphs, and scatter plots work well for different types of data.
- Simplicity : Avoid clutter and unnecessary details. Focus on conveying the main points.
- Accessibility : Make sure your visuals and tables are accessible to a broad audience, including those with visual impairments.
- Captions : Include informative captions that explain the significance of each visual or table.
Compelling visuals and tables enhance the reader's understanding of your research and can be the key to conveying complex information efficiently.
3. Interpret Findings
Interpreting your findings is where you bridge the gap between data and meaning. It's your opportunity to provide context, discuss implications, and offer insights. When interpreting your findings:
- Relate to Research Questions : Discuss how your findings directly address your research questions or hypotheses.
- Compare with Literature : Analyze how your results align with or deviate from previous research in your field. What insights can you draw from these comparisons?
- Discuss Limitations : Be transparent about the limitations of your study. Address any constraints, biases, or potential sources of error.
- Practical Implications : Explore the real-world implications of your findings. How can they be applied or inform decision-making?
- Future Research Directions : Suggest areas for future research based on the gaps or unanswered questions that emerged from your study.
Interpreting findings goes beyond simply presenting data; it's about weaving a narrative that helps readers grasp the significance of your research in the broader context.
With your research paper written, structured, and enriched with visuals, and your findings expertly interpreted, you are now prepared to communicate your research effectively. Sharing your insights and contributing to the body of knowledge in your field is a significant accomplishment in empirical research.
Examples of Empirical Research
To solidify your understanding of empirical research, let's delve into some real-world examples across different fields. These examples will illustrate how empirical research is applied to gather data, analyze findings, and draw conclusions.
Social Sciences
In the realm of social sciences, consider a sociological study exploring the impact of socioeconomic status on educational attainment. Researchers gather data from a diverse group of individuals, including their family backgrounds, income levels, and academic achievements.
Through statistical analysis, they can identify correlations and trends, revealing whether individuals from lower socioeconomic backgrounds are less likely to attain higher levels of education. This empirical research helps shed light on societal inequalities and informs policymakers on potential interventions to address disparities in educational access.
Environmental Science
Environmental scientists often employ empirical research to assess the effects of environmental changes. For instance, researchers studying the impact of climate change on wildlife might collect data on animal populations, weather patterns, and habitat conditions over an extended period.
By analyzing this empirical data, they can identify correlations between climate fluctuations and changes in wildlife behavior, migration patterns, or population sizes. This empirical research is crucial for understanding the ecological consequences of climate change and informing conservation efforts.
Business and Economics
In the business world, empirical research is essential for making data-driven decisions. Consider a market research study conducted by a business seeking to launch a new product. They collect data through surveys, focus groups, and consumer behavior analysis.
By examining this empirical data, the company can gauge consumer preferences, demand, and potential market size. Empirical research in business helps guide product development, pricing strategies, and marketing campaigns, increasing the likelihood of a successful product launch.
Psychological studies frequently rely on empirical research to understand human behavior and cognition. For instance, a psychologist interested in examining the impact of stress on memory might design an experiment. Participants are exposed to stress-inducing situations, and their memory performance is assessed through various tasks.
By analyzing the data collected, the psychologist can determine whether stress has a significant effect on memory recall. This empirical research contributes to our understanding of the complex interplay between psychological factors and cognitive processes.
These examples highlight the versatility and applicability of empirical research across diverse fields. Whether in medicine, social sciences, environmental science, business, or psychology, empirical research serves as a fundamental tool for gaining insights, testing hypotheses, and driving advancements in knowledge and practice.
Conclusion for Empirical Research
Empirical research is a powerful tool for gaining insights, testing hypotheses, and making informed decisions. By following the steps outlined in this guide, you've learned how to select research topics, collect data, analyze findings, and effectively communicate your research to the world. Remember, empirical research is a journey of discovery, and each step you take brings you closer to a deeper understanding of the world around you. Whether you're a scientist, a student, or someone curious about the process, the principles of empirical research empower you to explore, learn, and contribute to the ever-expanding realm of knowledge.
How to Collect Data for Empirical Research?
Introducing Appinio , the real-time market research platform revolutionizing how companies gather consumer insights for their empirical research endeavors. With Appinio, you can conduct your own market research in minutes, gaining valuable data to fuel your data-driven decisions.
Appinio is more than just a market research platform; it's a catalyst for transforming the way you approach empirical research, making it exciting, intuitive, and seamlessly integrated into your decision-making process.
Here's why Appinio is the go-to solution for empirical research:
- From Questions to Insights in Minutes : With Appinio's streamlined process, you can go from formulating your research questions to obtaining actionable insights in a matter of minutes, saving you time and effort.
- Intuitive Platform for Everyone : No need for a PhD in research; Appinio's platform is designed to be intuitive and user-friendly, ensuring that anyone can navigate and utilize it effectively.
- Rapid Response Times : With an average field time of under 23 minutes for 1,000 respondents, Appinio delivers rapid results, allowing you to gather data swiftly and efficiently.
- Global Reach with Targeted Precision : With access to over 90 countries and the ability to define target groups based on 1200+ characteristics, Appinio empowers you to reach your desired audience with precision and ease.
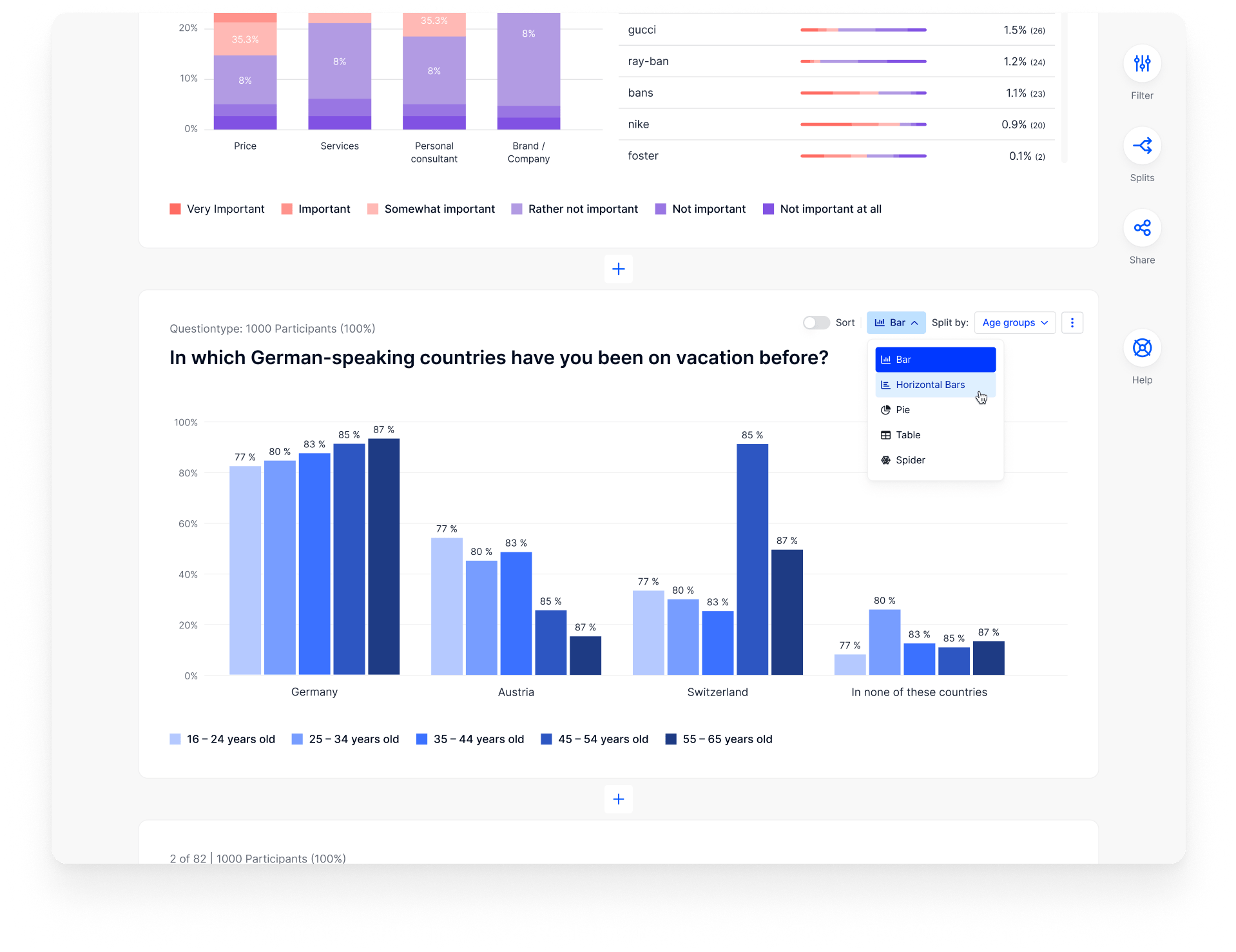
Get free access to the platform!
Join the loop 💌
Be the first to hear about new updates, product news, and data insights. We'll send it all straight to your inbox.
Get the latest market research news straight to your inbox! 💌
Wait, there's more
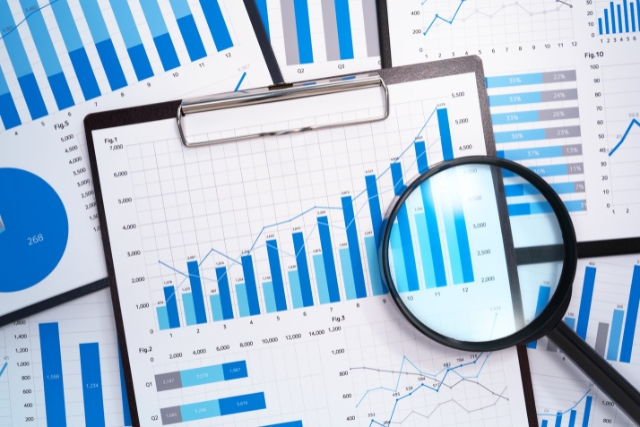
11.04.2024 | 34min read
What is Data Analysis? Definition, Tools, Examples
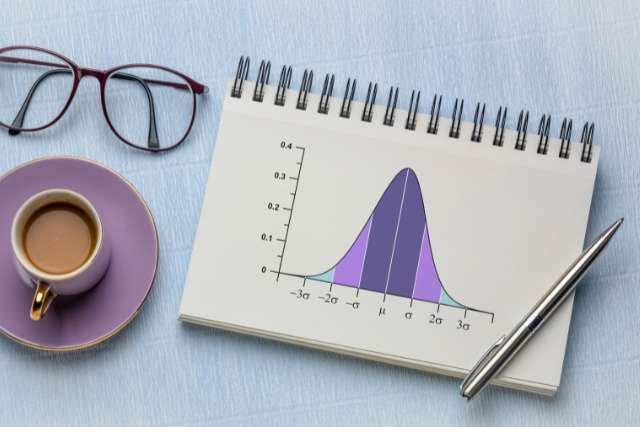
09.04.2024 | 29min read
What is a Confidence Interval and How to Calculate It?
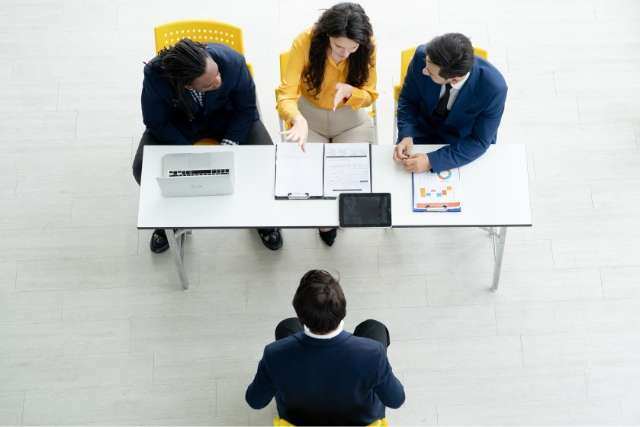
05.04.2024 | 28min read
What is Field Research? Definition, Types, Methods, Examples
Get science-backed answers as you write with Paperpal's Research feature
Empirical Research: A Comprehensive Guide for Academics
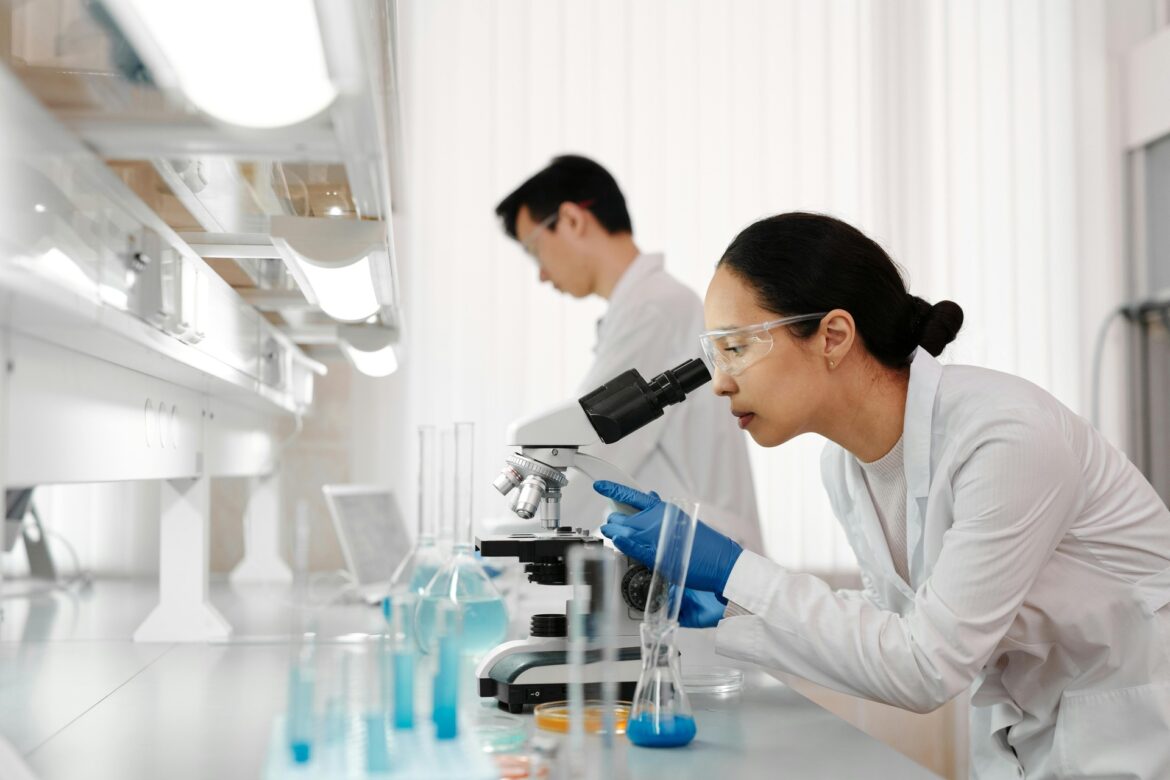
Empirical research relies on gathering and studying real, observable data. The term ’empirical’ comes from the Greek word ’empeirikos,’ meaning ‘experienced’ or ‘based on experience.’ So, what is empirical research? Instead of using theories or opinions, empirical research depends on real data obtained through direct observation or experimentation.
Why Empirical Research?
Empirical research plays a key role in checking or improving current theories, providing a systematic way to grow knowledge across different areas. By focusing on objectivity, it makes research findings more trustworthy, which is critical in research fields like medicine, psychology, economics, and public policy. In the end, the strengths of empirical research lie in deepening our awareness of the world and improving our capacity to tackle problems wisely. 1,2
Qualitative and Quantitative Methods
There are two main types of empirical research methods – qualitative and quantitative. 3,4 Qualitative research delves into intricate phenomena using non-numerical data, such as interviews or observations, to offer in-depth insights into human experiences. In contrast, quantitative research analyzes numerical data to spot patterns and relationships, aiming for objectivity and the ability to apply findings to a wider context.
Steps for Conducting Empirical Research
When it comes to conducting research, there are some simple steps that researchers can follow. 5,6
- Create Research Hypothesis: Clearly state the specific question you want to answer or the hypothesis you want to explore in your study.
- Examine Existing Research: Read and study existing research on your topic. Understand what’s already known, identify existing gaps in knowledge, and create a framework for your own study based on what you learn.
- Plan Your Study: Decide how you’ll conduct your research—whether through qualitative methods, quantitative methods, or a mix of both. Choose suitable techniques like surveys, experiments, interviews, or observations based on your research question.
- Develop Research Instruments: Create reliable research collection tools, such as surveys or questionnaires, to help you collate data. Ensure these tools are well-designed and effective.
- Collect Data: Systematically gather the information you need for your research according to your study design and protocols using the chosen research methods.
- Data Analysis: Analyze the collected data using suitable statistical or qualitative methods that align with your research question and objectives.
- Interpret Results: Understand and explain the significance of your analysis results in the context of your research question or hypothesis.
- Draw Conclusions: Summarize your findings and draw conclusions based on the evidence. Acknowledge any study limitations and propose areas for future research.
Advantages of Empirical Research
Empirical research is valuable because it stays objective by relying on observable data, lessening the impact of personal biases. This objectivity boosts the trustworthiness of research findings. Also, using precise quantitative methods helps in accurate measurement and statistical analysis. This precision ensures researchers can draw reliable conclusions from numerical data, strengthening our understanding of the studied phenomena. 4
Disadvantages of Empirical Research
While empirical research has notable strengths, researchers must also be aware of its limitations when deciding on the right research method for their study.4 One significant drawback of empirical research is the risk of oversimplifying complex phenomena, especially when relying solely on quantitative methods. These methods may struggle to capture the richness and nuances present in certain social, cultural, or psychological contexts. Another challenge is the potential for confounding variables or biases during data collection, impacting result accuracy.
Tips for Empirical Writing
In empirical research, the writing is usually done in research papers, articles, or reports. The empirical writing follows a set structure, and each section has a specific role. Here are some tips for your empirical writing. 7
- Define Your Objectives: When you write about your research, start by making your goals clear. Explain what you want to find out or prove in a simple and direct way. This helps guide your research and lets others know what you have set out to achieve.
- Be Specific in Your Literature Review: In the part where you talk about what others have studied before you, focus on research that directly relates to your research question. Keep it short and pick studies that help explain why your research is important. This part sets the stage for your work.
- Explain Your Methods Clearly : When you talk about how you did your research (Methods), explain it in detail. Be clear about your research plan, who took part, and what you did; this helps others understand and trust your study. Also, be honest about any rules you follow to make sure your study is ethical and reproducible.
- Share Your Results Clearly : After doing your empirical research, share what you found in a simple way. Use tables or graphs to make it easier for your audience to understand your research. Also, talk about any numbers you found and clearly state if they are important or not. Ensure that others can see why your research findings matter.
- Talk About What Your Findings Mean: In the part where you discuss your research results, explain what they mean. Discuss why your findings are important and if they connect to what others have found before. Be honest about any problems with your study and suggest ideas for more research in the future.
- Wrap It Up Clearly: Finally, end your empirical research paper by summarizing what you found and why it’s important. Remind everyone why your study matters. Keep your writing clear and fix any mistakes before you share it. Ask someone you trust to read it and give you feedback before you finish.
References:
- Empirical Research in the Social Sciences and Education, Penn State University Libraries. Available online at https://guides.libraries.psu.edu/emp
- How to conduct empirical research, Emerald Publishing. Available online at https://www.emeraldgrouppublishing.com/how-to/research-methods/conduct-empirical-research
- Empirical Research: Quantitative & Qualitative, Arrendale Library, Piedmont University. Available online at https://library.piedmont.edu/empirical-research
- Bouchrika, I. What Is Empirical Research? Definition, Types & Samples in 2024. Research.com, January 2024. Available online at https://research.com/research/what-is-empirical-research
- Quantitative and Empirical Research vs. Other Types of Research. California State University, April 2023. Available online at https://libguides.csusb.edu/quantitative
- Empirical Research, Definitions, Methods, Types and Examples, Studocu.com website. Available online at https://www.studocu.com/row/document/uganda-christian-university/it-research-methods/emperical-research-definitions-methods-types-and-examples/55333816
- Writing an Empirical Paper in APA Style. Psychology Writing Center, University of Washington. Available online at https://psych.uw.edu/storage/writing_center/APApaper.pdf
Paperpal is an AI writing assistant that help academics write better, faster with real-time suggestions for in-depth language and grammar correction. Trained on millions of research manuscripts enhanced by professional academic editors, Paperpal delivers human precision at machine speed.
Try it for free or upgrade to Paperpal Prime , which unlocks unlimited access to premium features like academic translation, paraphrasing, contextual synonyms, consistency checks and more. It’s like always having a professional academic editor by your side! Go beyond limitations and experience the future of academic writing. Get Paperpal Prime now at just US$19 a month!
Related Reads:
- How to Write a Scientific Paper in 10 Steps
- What is a Literature Review? How to Write It (with Examples)
- What is an Argumentative Essay? How to Write It (With Examples)
- Ethical Research Practices For Research with Human Subjects
Ethics in Science: Importance, Principles & Guidelines
Presenting research data effectively through tables and figures, you may also like, what is hedging in academic writing , how to use ai to enhance your college..., ai + human expertise – a paradigm shift..., how to use paperpal to generate emails &..., ai in education: it’s time to change the..., is it ethical to use ai-generated abstracts without..., do plagiarism checkers detect ai content, word choice problems: how to use the right..., how to avoid plagiarism when using generative ai..., what are journal guidelines on using generative ai....
Canvas | University | Ask a Librarian
- Library Homepage
- Arrendale Library
Empirical Research: Quantitative & Qualitative
- Empirical Research
Introduction: What is Empirical Research?
Quantitative methods, qualitative methods.
- Quantitative vs. Qualitative
- Reference Works for Social Sciences Research
- Contact Us!
Call us at 706-776-0111
Chat with a Librarian
Send Us Email
Library Hours
Empirical research is based on phenomena that can be observed and measured. Empirical research derives knowledge from actual experience rather than from theory or belief.
Key characteristics of empirical research include:
- Specific research questions to be answered;
- Definitions of the population, behavior, or phenomena being studied;
- Description of the methodology or research design used to study this population or phenomena, including selection criteria, controls, and testing instruments (such as surveys);
- Two basic research processes or methods in empirical research: quantitative methods and qualitative methods (see the rest of the guide for more about these methods).
(based on the original from the Connelly LIbrary of LaSalle University)
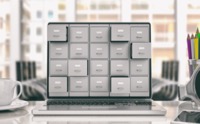
Empirical Research: Qualitative vs. Quantitative
Learn about common types of journal articles that use APA Style, including empirical studies; meta-analyses; literature reviews; and replication, theoretical, and methodological articles.
Academic Writer
© 2024 American Psychological Association.
- More about Academic Writer ...
Quantitative Research
A quantitative research project is characterized by having a population about which the researcher wants to draw conclusions, but it is not possible to collect data on the entire population.
- For an observational study, it is necessary to select a proper, statistical random sample and to use methods of statistical inference to draw conclusions about the population.
- For an experimental study, it is necessary to have a random assignment of subjects to experimental and control groups in order to use methods of statistical inference.
Statistical methods are used in all three stages of a quantitative research project.
For observational studies, the data are collected using statistical sampling theory. Then, the sample data are analyzed using descriptive statistical analysis. Finally, generalizations are made from the sample data to the entire population using statistical inference.
For experimental studies, the subjects are allocated to experimental and control group using randomizing methods. Then, the experimental data are analyzed using descriptive statistical analysis. Finally, just as for observational data, generalizations are made to a larger population.
Iversen, G. (2004). Quantitative research . In M. Lewis-Beck, A. Bryman, & T. Liao (Eds.), Encyclopedia of social science research methods . (pp. 897-898). Thousand Oaks, CA: SAGE Publications, Inc.
Qualitative Research
What makes a work deserving of the label qualitative research is the demonstrable effort to produce richly and relevantly detailed descriptions and particularized interpretations of people and the social, linguistic, material, and other practices and events that shape and are shaped by them.
Qualitative research typically includes, but is not limited to, discerning the perspectives of these people, or what is often referred to as the actor’s point of view. Although both philosophically and methodologically a highly diverse entity, qualitative research is marked by certain defining imperatives that include its case (as opposed to its variable) orientation, sensitivity to cultural and historical context, and reflexivity.
In its many guises, qualitative research is a form of empirical inquiry that typically entails some form of purposive sampling for information-rich cases; in-depth interviews and open-ended interviews, lengthy participant/field observations, and/or document or artifact study; and techniques for analysis and interpretation of data that move beyond the data generated and their surface appearances.
Sandelowski, M. (2004). Qualitative research . In M. Lewis-Beck, A. Bryman, & T. Liao (Eds.), Encyclopedia of social science research methods . (pp. 893-894). Thousand Oaks, CA: SAGE Publications, Inc.
- Next: Quantitative vs. Qualitative >>
- Last Updated: Mar 22, 2024 10:47 AM
- URL: https://library.piedmont.edu/empirical-research
- Ebooks & Online Video
- New Materials
- Renew Checkouts
- Faculty Resources
- Friends of the Library
- Library Services
- Request Books from Demorest
- Our Mission
- Library History
- Ask a Librarian!
- Making Citations
- Working Online

Arrendale Library Piedmont University 706-776-0111
Empirical Research
- Living reference work entry
- First Online: 22 May 2017
- Cite this living reference work entry
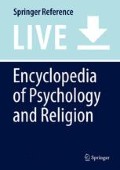
- Emeka Thaddues Njoku 2
491 Accesses
This is a preview of subscription content, log in via an institution to check access.
Access this chapter
Institutional subscriptions
Bibliography
Bhattacherjee, A. (2012). Social science research: Principles, methods, and practices. Textbooks Collection . Book 3.
Google Scholar
Comte, A., & Bridges, J. H. (Tr.) (1865). A general view of positivism . Trubner and Co. (reissued by Cambridge University Press , 2009).
Dilworth, C. B. (1982). Empirical research in the literature class. English Journal, 71 (3), 95–97.
Article Google Scholar
Heisenberg, W. (1971). Positivism, metaphysics and religion. In R. N. Nanshen (Ed.), Werner Heisenberg – Physics and beyond – Encounters and conversations , World Perspectives. 42. Translator: Arnold J. Pomerans. New York: Harper and Row.
Hossain, F. M. A. (2014). A critical analysis of empiricism. Open Journal of Philosophy, 2014 (4), 225–230.
Kant, I. (1783). Prolegomena to any future metaphysic (trans: Bennett, J.). Early Modern Texts. www.earlymoderntexts.com
Koch, S. (1992). Psychology’s Bridgman vs. Bridgman’s Bridgman: An essay in reconstruction. Theory and Psychology, 2 (3), 261–290.
Matin, A. (1968). An outline of philosophy . Dhaka: Mullick Brothers.
Mcleod, S. (2008). Psychology as science. http://www.simplypsychology.org/science-psychology.html
Popper, K. (1963). Conjectures and refutations: The growth of scientific knowledge . London: Routledge.
Simmel, G. (1908). The problem areas of sociology in Kurt H. Wolf: The sociology of Georg Simmel . London: The Free Press.
Weber, M. (1991). The nature of social action. In W. G. Runciman (Ed.), Weber: Selections in translation . Cambridge: Cambridge University Press.
Download references
Author information
Authors and affiliations.
Department of Political Science, University of Ibadan, Ibadan, Oyo, 200284, Nigeria
Emeka Thaddues Njoku
You can also search for this author in PubMed Google Scholar
Corresponding author
Correspondence to Emeka Thaddues Njoku .
Editor information
Editors and affiliations.
Rhinebeck, New York, USA
David A. Leeming
Rights and permissions
Reprints and permissions
Copyright information
© 2017 Springer-Verlag GmbH Germany
About this entry
Cite this entry.
Njoku, E.T. (2017). Empirical Research. In: Leeming, D. (eds) Encyclopedia of Psychology and Religion. Springer, Berlin, Heidelberg. https://doi.org/10.1007/978-3-642-27771-9_200051-1
Download citation
DOI : https://doi.org/10.1007/978-3-642-27771-9_200051-1
Received : 01 April 2017
Accepted : 08 May 2017
Published : 22 May 2017
Publisher Name : Springer, Berlin, Heidelberg
Print ISBN : 978-3-642-27771-9
Online ISBN : 978-3-642-27771-9
eBook Packages : Springer Reference Behavioral Science and Psychology Reference Module Humanities and Social Sciences Reference Module Business, Economics and Social Sciences
- Publish with us
Policies and ethics
- Find a journal
- Track your research
- quicklinks Academic admin council Academic calendar Academic stds cte Admission Advising African studies Alumni engagement American studies Anthropology/sociology Arabic Arboretum Archives Arcus center Art Assessment committee Athletics Athletic training Biology Biology&chem center Black faculty&staff assoc Bookstore BrandK Business office Campus event calendar Campus safety Catalog Career & prof dev Health science Ctr for civic engagement Ctr for international pgrms Chemistry Chinese Classics College communication Community & global health Community council Complex systems studies Computer science Copyright Counseling Council of student reps Crisis response Critical ethnic studies Critical theory Development Dining services Directories Disability services Donor relations East Asian studies Economics and business Educational policies cte Educational quality assmt Engineering Environmental stewardship Environmental studies English Experiential education cte Facilities management Facilities reservations Faculty development cte Faculty executive cte Faculty grants Faculty personnel cte Fellowships & grants Festival playhouse Film & media studies Financial aid First year experience Fitness & wellness ctr French Gardens & growing spaces German Global crossroads Health center Jewish studies History Hornet hive Hornet HQ Hornet sports Human resources Inclusive excellence Index (student newspaper) Information services Institutional research Institutional review board Intercultural student life International & area studies International programs Intramural sports Japanese LandSea Learning commons Learning support Lgbtqai+ student resources Library Mail and copy center Math Math/physics center Microsoft Stream Microsoft Teams Moodle Movies (ch 22 online) Music OneDrive Outdoor programs Parents' resources Payroll Phi Beta Kappa Philharmonia Philosophy Physics Physical education Political science Pre-law advising Provost Psychology Public pol & urban affairs Recycling Registrar Religion Religious & spiritual life Research Guides (libguides) Residential life Safety (security) Sexual safety Shared passages program SharePoint online Sophomore experience Spanish Strategic plan Student accounts Student development Student activities Student organizations Study abroad Support staff Sustainability Teaching and learning cte Teaching commons Theatre arts Title IX Webmail Women, gender & sexuality Writing center
PSYC 301: Intro to Research Methods
- Advanced Search Strategies
- Tracking the Research Process
- Annotations
- Article Cards
- Organizing Sources
- Writing an Outline
- Citing Sources

Finding Empirical Research
Empirical research is published in books and in scholarly, peer-reviewed journals. PsycInfo offers straightforward ways to identify empirical research, unlike most other databases.
Finding Empirical Research in PsycInfo
- PsycInfo Choose "Advanced Search" Scroll down the page to "Methodology," and choose "Empirical Study" Type your keywords into the search boxes Choose other limits, such as publication date, if needed Click on the "Search" button
Slideshow showing how to find empirical research in APA PsycInfo
Video of finding empirical articles in psycinfo.
- Searching for Peer-Reviewed Empirical Articles (YouTube Video) Created by the APA
What is Empirical Research?
Empirical research is based on observed and measured phenomena and derives knowledge from actual experience rather than from theory or belief.
How do you know if a study is empirical? Read the subheadings within the article, book, or report and look for a description of the research "methodology." Ask yourself: Could I recreate this study and test these results?
Key characteristics to look for:
- Specific research questions to be answered
- Definition of the population, behavior, or phenomena being studied
- Description of the process used to study this population or phenomena, including selection criteria, controls, and testing instruments (such as surveys)
Another hint: some scholarly journals use a specific layout, called the "IMRaD" format, to communicate empirical research findings. Such articles typically have 4 components:
- Introduction : sometimes called "literature review" -- what is currently known about the topic -- usually includes a theoretical framework and/or discussion of previous studies
- Methodology: sometimes called "research design" -- how to recreate the study -- usually describes the population, research process, and analytical tools
- Results : sometimes called "findings" -- what was learned through the study -- usually appears as statistical data or as substantial quotations from research participants
- Discussion : sometimes called "conclusion" or "implications" -- why the study is important -- usually describes how the research results influence professional practices or future studies
Adapted from PennState University Libraries, Empirical Research in the Social Sciences and Education
Using PsycInfo
- Narrowing a Search (Canva Slideshow) Created by K Librarians
- Searching with the Thesaurus and Index Terms (YouTube Video) Created by the APA
- << Previous: Home
- Next: Advanced Search Strategies >>
- Last Updated: Apr 4, 2024 4:11 PM
- URL: https://libguides.kzoo.edu/psyc301
Penn State University Libraries
Empirical research in the social sciences and education.
- What is Empirical Research and How to Read It
- Finding Empirical Research in Library Databases
- Designing Empirical Research
- Ethics, Cultural Responsiveness, and Anti-Racism in Research
- Citing, Writing, and Presenting Your Work
Contact the Librarian at your campus for more help!

Introduction: What is Empirical Research?
Empirical research is based on observed and measured phenomena and derives knowledge from actual experience rather than from theory or belief.
How do you know if a study is empirical? Read the subheadings within the article, book, or report and look for a description of the research "methodology." Ask yourself: Could I recreate this study and test these results?
Key characteristics to look for:
- Specific research questions to be answered
- Definition of the population, behavior, or phenomena being studied
- Description of the process used to study this population or phenomena, including selection criteria, controls, and testing instruments (such as surveys)
Another hint: some scholarly journals use a specific layout, called the "IMRaD" format, to communicate empirical research findings. Such articles typically have 4 components:
- Introduction : sometimes called "literature review" -- what is currently known about the topic -- usually includes a theoretical framework and/or discussion of previous studies
- Methodology: sometimes called "research design" -- how to recreate the study -- usually describes the population, research process, and analytical tools used in the present study
- Results : sometimes called "findings" -- what was learned through the study -- usually appears as statistical data or as substantial quotations from research participants
- Discussion : sometimes called "conclusion" or "implications" -- why the study is important -- usually describes how the research results influence professional practices or future studies
Reading and Evaluating Scholarly Materials
Reading research can be a challenge. However, the tutorials and videos below can help. They explain what scholarly articles look like, how to read them, and how to evaluate them:
- CRAAP Checklist A frequently-used checklist that helps you examine the currency, relevance, authority, accuracy, and purpose of an information source.
- IF I APPLY A newer model of evaluating sources which encourages you to think about your own biases as a reader, as well as concerns about the item you are reading.
- Credo Video: How to Read Scholarly Materials (4 min.)
- Credo Tutorial: How to Read Scholarly Materials
- Credo Tutorial: Evaluating Information
- Credo Video: Evaluating Statistics (4 min.)
- Next: Finding Empirical Research in Library Databases >>
- Last Updated: Feb 18, 2024 8:33 PM
- URL: https://guides.libraries.psu.edu/emp

Yearly paid plans are up to 65% off for the spring sale. Limited time only! 🌸
- Form Builder
- Survey Maker
- AI Form Generator
- AI Survey Tool
- AI Quiz Maker
- Store Builder
- WordPress Plugin

HubSpot CRM

Google Sheets

Google Analytics

Microsoft Excel

- Popular Forms
- Job Application Form Template
- Rental Application Form Template
- Hotel Accommodation Form Template
- Online Registration Form Template
- Employment Application Form Template
- Application Forms
- Booking Forms
- Consent Forms
- Contact Forms
- Donation Forms
- Customer Satisfaction Surveys
- Employee Satisfaction Surveys
- Evaluation Surveys
- Feedback Surveys
- Market Research Surveys
- Personality Quiz Template
- Geography Quiz Template
- Math Quiz Template
- Science Quiz Template
- Vocabulary Quiz Template
Try without registration Quick Start
Read engaging stories, how-to guides, learn about forms.app features.
Inspirational ready-to-use templates for getting started fast and powerful.
Spot-on guides on how to use forms.app and make the most out of it.

See the technical measures we take and learn how we keep your data safe and secure.
- Integrations
- Help Center
- Sign In Sign Up Free
- What is empirical research: Methods, types & examples
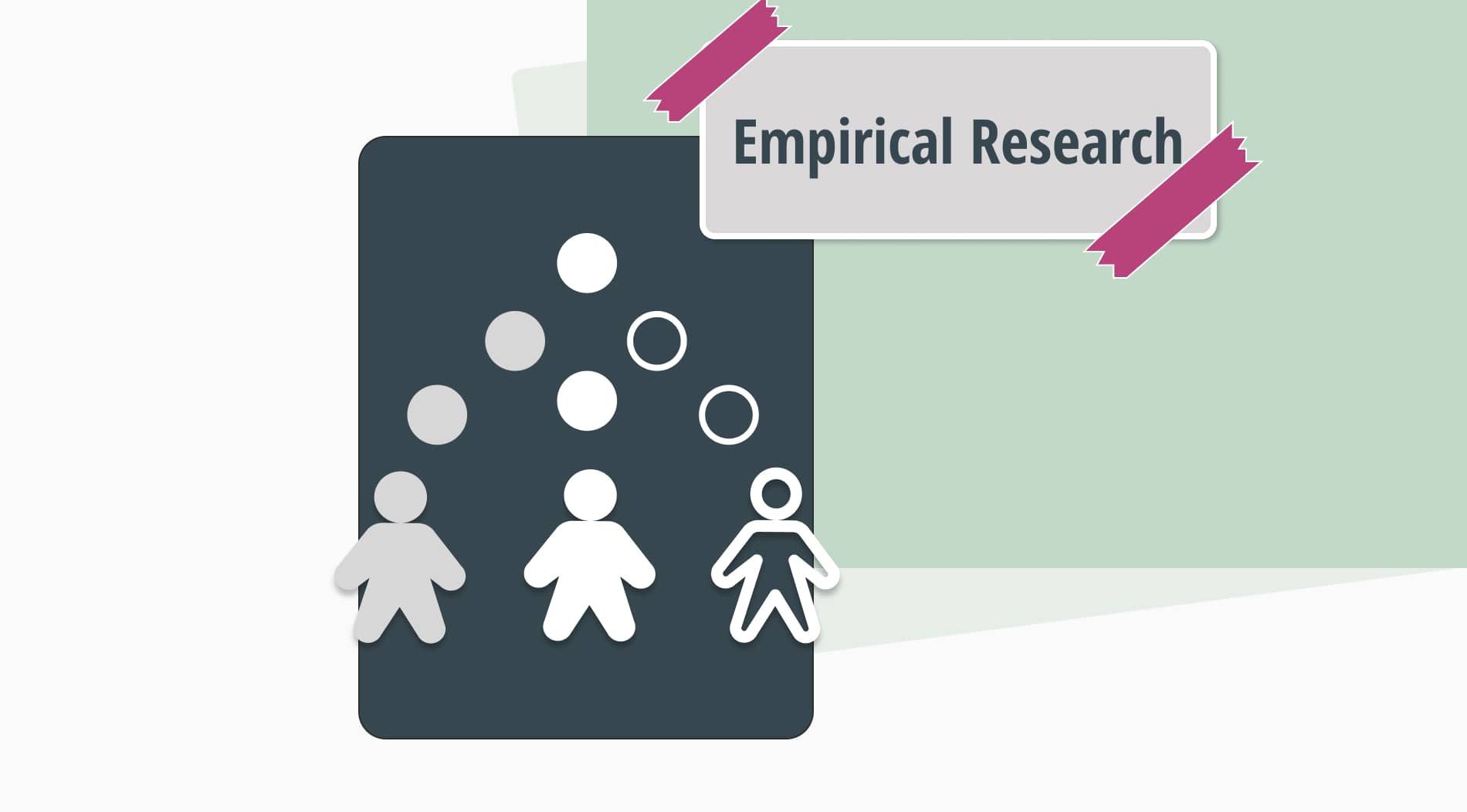
Defne Çobanoğlu
Having opinions on matters based on observation is okay sometimes. Same as having theories on the subject you want to solve. However, some theories need to be tested. Just like Robert Oppenheimer says, “Theory will take you only so far .”
In that case, when you have your research question ready and you want to make sure it is correct, the next step would be experimentation. Because only then you can test your ideas and collect tangible information. Now, let us start with the empirical research definition:
- What is empirical research?
Empirical research is a research type where the aim of the study is based on finding concrete and provable evidence . The researcher using this method to draw conclusions can use both quantitative and qualitative methods. Different than theoretical research, empirical research uses scientific experimentation and investigation.
Using experimentation makes sense when you need to have tangible evidence to act on whatever you are planning to do. As the researcher, you can be a marketer who is planning on creating a new ad for the target audience, or you can be an educator who wants the best for the students. No matter how big or small, data gathered from the real world using this research helps break down the question at hand.
- When to use empirical research?
Empirical research methods are used when the researcher needs to gather data analysis on direct, observable, and measurable data. Research findings are a great way to make grounded ideas. Here are some situations when one may need to do empirical research:
1. When quantitative or qualitative data is needed
There are times when a researcher, marketer, or producer needs to gather data on specific research questions to make an informed decision. And the concrete data gathered in the research process gives a good starting point.
2. When you need to test a hypothesis
When you have a hypothesis on a subject, you can test the hypothesis through observation or experiment. Making a planned study is a great way to collect information and test whether or not your hypothesis is correct.
3. When you want to establish causality
Experimental research is a good way to explore whether or not there is any correlation between two variables. Researchers usually establish causality by changing a variable and observing if the independent variable changes accordingly.
- Types of empirical research
The aim of empirical research is to collect information about a subject from the people by doing experimentation and other data collection methods. However, the methods and data collected are divided into two groups: one collects numerical data, and the other one collects opinion-like data. Let us see the difference between these two types:
Quantitative research
Quantitative research methods are used to collect data in a numerical way. Therefore, the results gathered by these methods will be numbers, statistics, charts, etc. The results can be used to quantify behaviors, opinions, and other variables. Quantitative research methods are surveys, questionnaires, and experimental research.
Qualitiative research
Qualitative research methods are not used to collect numerical answers, instead, they are used to collect the participants’ reasons, opinions, and other meaningful aspects. Qualitative research methods include case studies, observations, interviews, focus groups, and text analysis.
- 5 steps to conduct empirical research
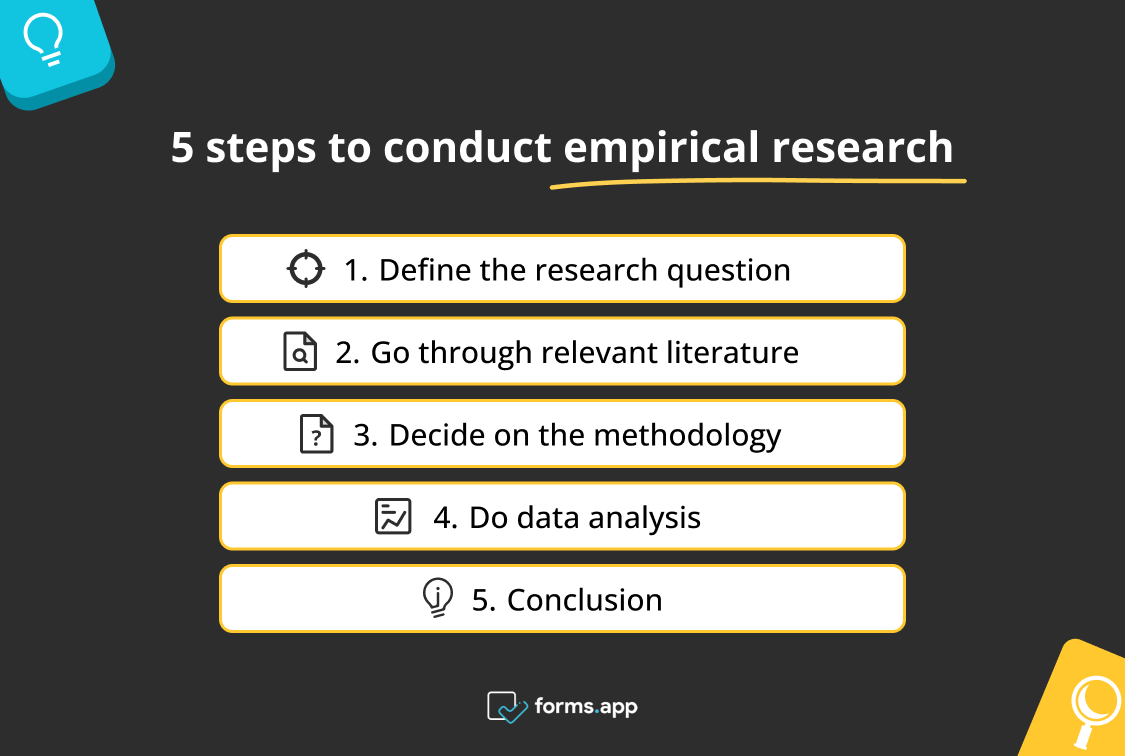
Necessary steps for empirical research
When you want to collect direct and concrete data on a subject, empirical research is a great way to go. And, just like every other project and research, it is best to have a clear structure in mind. This is even more important in studies that may take a long time, such as experiments that take years. Let us look at a clear plan on how to do empirical research:
1. Define the research question
The very first step of every study is to have the question you will explore ready. Because you do not want to change your mind in the middle of the study after investing and spending time on the experimentation.
2. Go through relevant literature
This is the step where you sit down and do a desk research where you gather relevant data and see if other researchers have tried to explore similar research questions. If so, you can see how well they were able to answer the question or what kind of difficulties they faced during the research process.
3. Decide on the methodology
Once you are done going through the relevant literature, you can decide on which method or methods you can use. The appropriate methods are observation, experimentation, surveys, interviews, focus groups, etc.
4. Do data analysis
When you get to this step, it means you have successfully gathered enough data to make a data analysis. Now, all you need to do is look at the data you collected and make an informed analysis.
5. Conclusion
This is the last step, where you are finished with the experimentation and data analysis process. Now, it is time to decide what to do with this information. You can publish a paper and make informed decisions about whatever your goal is.
- Empirical research methodologies
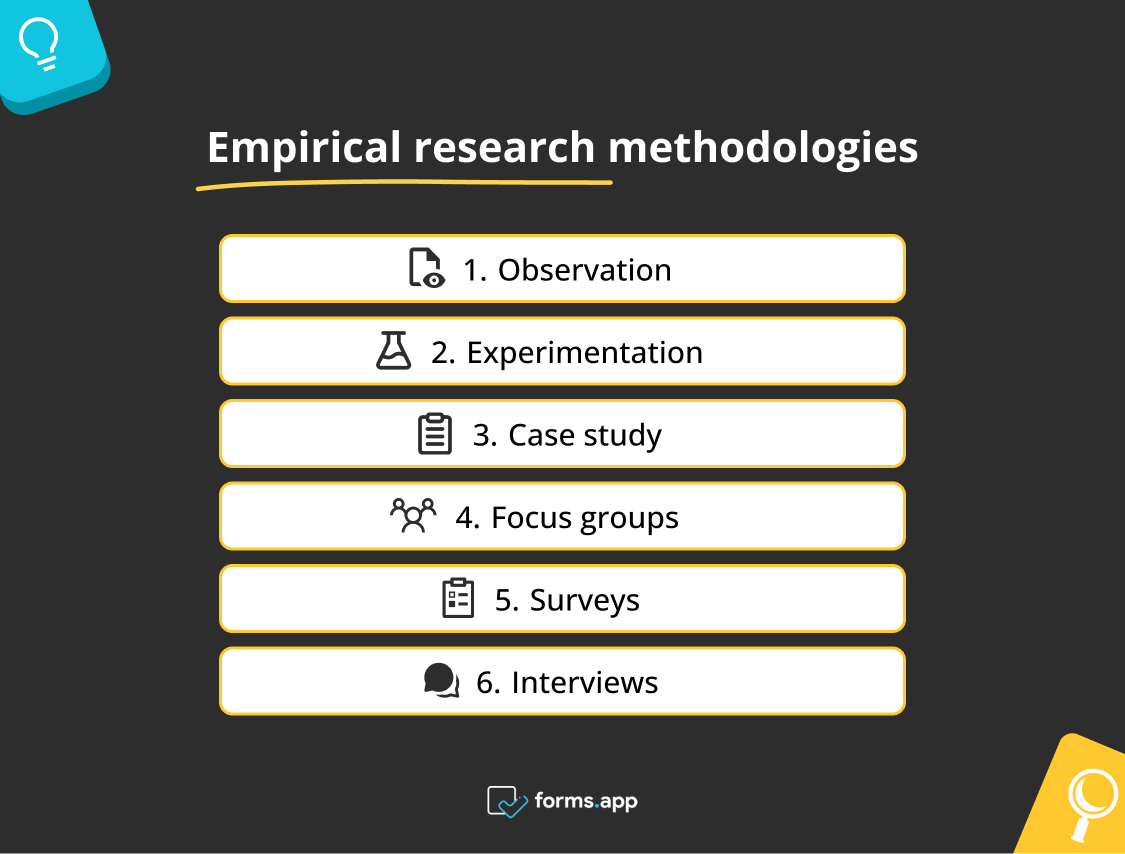
Some essential methodologies to conduct empirical research
The aim of this type of research is to explore brand-new evidence and facts. Therefore, the methods should be primary and gathered in real life, directly from the people. There is more than one method for this goal, and it is up to the researcher to use which one(s). Let us see the methods of empirical research:
- Observation
The method of observation is a great way to collect information on people without the effect of interference. The researcher can choose the appropriate area, time, or situation and observe the people and their interactions with one another. The researcher can be just an outside observer or can be a participant as an observer or a full participant.
- Experimentation
The experimentation process can be done in the real world by intervening in some elements to unify the environment for all participants. This method can also be done in a laboratory environment. The experimentation process is good for being able to change the variables according to the aim of the study.
The case study method is done by making an in-depth analysis of already existing cases. When the parameters and variables are similar to the research question at hand, it is wise to go through what was researched before.
- Focus groups
The case study method is done by using a group of individuals or multiple groups and using their opinions, characteristics, and responses. The scientists gather the data from this group and generalize it to the whole population.
Surveys are an effective way to gather data directly from people. It is a systematic approach to collecting information. If it is done in an online setting as an online survey , it would be even easier to reach out to people and ask their opinions in open-ended or close-ended questions.
Interviews are similar to surveys as you are using questions to collect information and opinions of the people. Unlike a survey, this process is done face-to-face, as a phone call, or as a video call.
- Advantages of empirical research
Empirical research is effective for many reasons, and helps researchers from numerous fields. Here are some advantages of empirical research to have in mind for your next research:
- Empirical research improves the internal validity of the study.
- Empirical evidence gathered from the study is used to authenticate the research question.
- Collecting provable evidence is important for the success of the study.
- The researcher is able to make informed decisions based on the data collected using empirical research.
- Disadvantages of empirical research
After learning about the positive aspects of empirical research, it is time to mention the negative aspects. Because this type may not be suitable for everyone and the researcher should be mindful of the disadvantages of empirical research. Here are the disadvantages of empirical research:
- As it is similar to other research types, a case study where experimentation is included will be time-consuming no matter what. It has more steps and variables than concluding a secondary research.
- There are a lot of variables that need to be controlled and considered. Therefore, it may be a challenging task to be mindful of all the details.
- Doing evidence-based research can be expensive if you need to complete it on a large scale.
- When you are conducting an experiment, you may need some waivers and permissions.
- Frequently asked questions about empirical research
Empirical research is one of the many research types, and there may be some questions in mind about its similarities and differences to other research types.
Is empirical research qualitative or quantitative?
The data collected by empirical research can be qualitative, quantitative, or a mix of both. It is up to the aim of researcher to what kind of data is needed and searched for.
Is empirical research the same as quantitative research?
As quantitative research heavily relies on data collection methods of observation and experimentation, it is, in nature, an empirical study. Some professors may even use the terms interchangeably. However, that does not mean that empirical research is only a quantitative one.
What is the difference between theoretical and empirical research?
Empirical studies are based on data collection to prove theories or answer questions, and it is done by using methods such as observation and experimentation. Therefore, empirical research relies on finding evidence that backs up theories. On the other hand, theoretical research relies on theorizing on empirical research data and trying to make connections and correlations.
What is the difference between conceptual and empirical research?
Conceptual research is about thoughts and ideas and does not involve any kind of experimentation. Empirical research, on the other hand, works with provable data and hard evidence.
What is the difference between empirical vs applied research?
Some scientists may use these two terms interchangeably however, there is a difference between them. Applied research involves applying theories to solve real-life problems. On the other hand, empirical research involves the obtaining and analysis of data to test hypotheses and theories.
- Final words
Empirical research is a good means when the goal of your study is to find concrete data to go with. You may need to do empirical research when you need to test a theory, establish causality, or need qualitative/quantitative data. For example, you are a scientist and want to know if certain colors have an effect on people’s moods, or you are a marketer and want to test your theory on ad places on websites.
In both scenarios, you can collect information by using empirical research methods and make informed decisions afterward. These are just the two of empirical research examples. This research type can be applied to many areas of work life and social sciences. Lastly, for all your research needs, you can visit forms.app to use its many useful features and over 1000 form and survey templates!
Defne is a content writer at forms.app. She is also a translator specializing in literary translation. Defne loves reading, writing, and translating professionally and as a hobby. Her expertise lies in survey research, research methodologies, content writing, and translation.
- Form Features
- Data Collection
Table of Contents
Related posts.
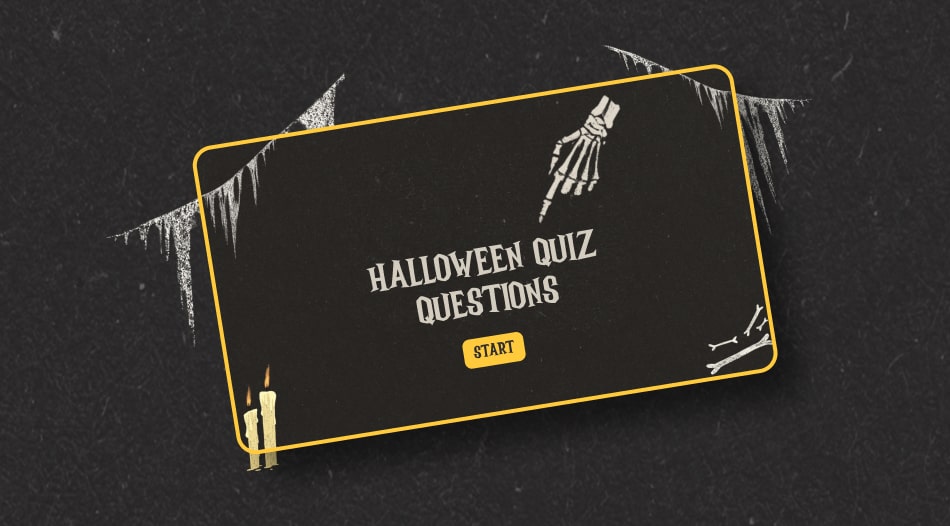
50+ Best Halloween quiz questions & answers
Şeyma Beyazçiçek
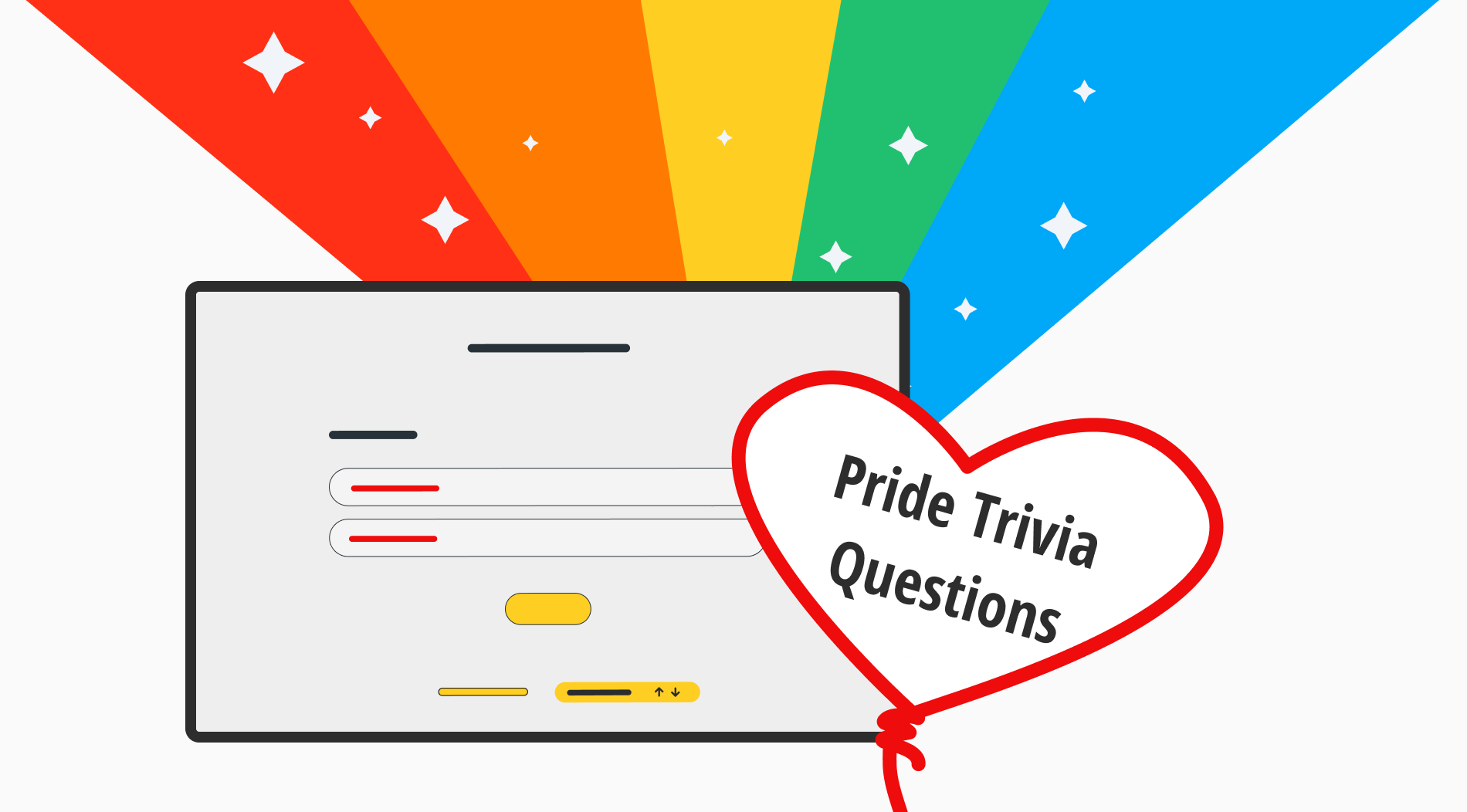
25+ Fun LGBTQ+ quiz questions & answers
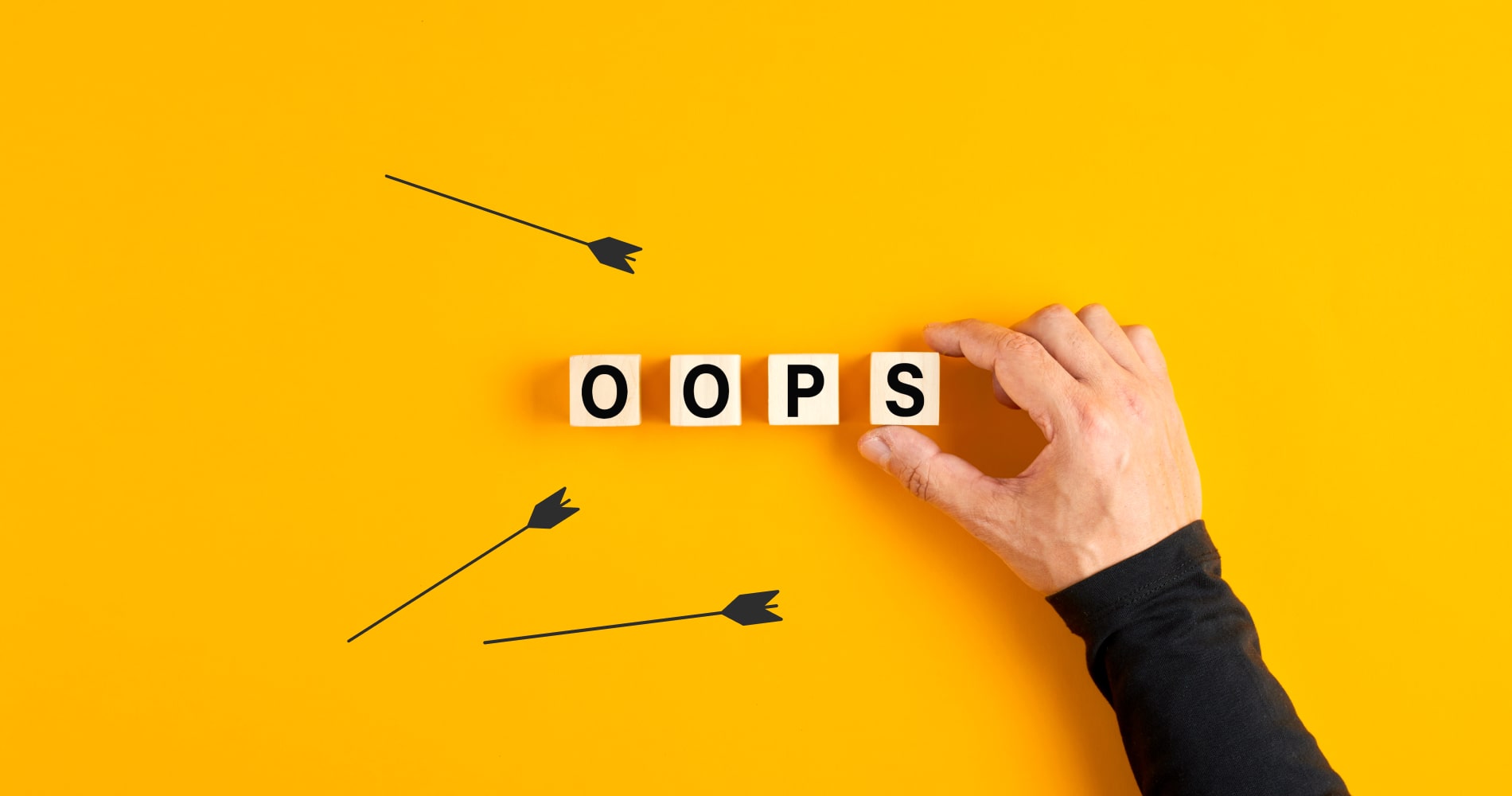
7 Common mistakes to avoid in your customer satisfaction surveys
Sophia Young
- What is Empirical Research Study? [Examples & Method]

The bulk of human decisions relies on evidence, that is, what can be measured or proven as valid. In choosing between plausible alternatives, individuals are more likely to tilt towards the option that is proven to work, and this is the same approach adopted in empirical research.
In empirical research, the researcher arrives at outcomes by testing his or her empirical evidence using qualitative or quantitative methods of observation, as determined by the nature of the research. An empirical research study is set apart from other research approaches by its methodology and features hence; it is important for every researcher to know what constitutes this investigation method.
What is Empirical Research?
Empirical research is a type of research methodology that makes use of verifiable evidence in order to arrive at research outcomes. In other words, this type of research relies solely on evidence obtained through observation or scientific data collection methods.
Empirical research can be carried out using qualitative or quantitative observation methods , depending on the data sample, that is, quantifiable data or non-numerical data . Unlike theoretical research that depends on preconceived notions about the research variables, empirical research carries a scientific investigation to measure the experimental probability of the research variables
Characteristics of Empirical Research
- Research Questions
An empirical research begins with a set of research questions that guide the investigation. In many cases, these research questions constitute the research hypothesis which is tested using qualitative and quantitative methods as dictated by the nature of the research.
In an empirical research study, the research questions are built around the core of the research, that is, the central issue which the research seeks to resolve. They also determine the course of the research by highlighting the specific objectives and aims of the systematic investigation.
- Definition of the Research Variables
The research variables are clearly defined in terms of their population, types, characteristics, and behaviors. In other words, the data sample is clearly delimited and placed within the context of the research.
- Description of the Research Methodology
An empirical research also clearly outlines the methods adopted in the systematic investigation. Here, the research process is described in detail including the selection criteria for the data sample, qualitative or quantitative research methods plus testing instruments.
An empirical research is usually divided into 4 parts which are the introduction, methodology, findings, and discussions. The introduction provides a background of the empirical study while the methodology describes the research design, processes, and tools for the systematic investigation.
The findings refer to the research outcomes and they can be outlined as statistical data or in the form of information obtained through the qualitative observation of research variables. The discussions highlight the significance of the study and its contributions to knowledge.
Uses of Empirical Research
Without any doubt, empirical research is one of the most useful methods of systematic investigation. It can be used for validating multiple research hypotheses in different fields including Law, Medicine, and Anthropology.
- Empirical Research in Law : In Law, empirical research is used to study institutions, rules, procedures, and personnel of the law, with a view to understanding how they operate and what effects they have. It makes use of direct methods rather than secondary sources, and this helps you to arrive at more valid conclusions.
- Empirical Research in Medicine : In medicine, empirical research is used to test and validate multiple hypotheses and increase human knowledge.
- Empirical Research in Anthropology : In anthropology, empirical research is used as an evidence-based systematic method of inquiry into patterns of human behaviors and cultures. This helps to validate and advance human knowledge.
Discover how Extrapolation Powers statistical research: Definition, examples, types, and applications explained.
The Empirical Research Cycle
The empirical research cycle is a 5-phase cycle that outlines the systematic processes for conducting and empirical research. It was developed by Dutch psychologist, A.D. de Groot in the 1940s and it aligns 5 important stages that can be viewed as deductive approaches to empirical research.
In the empirical research methodological cycle, all processes are interconnected and none of the processes is more important than the other. This cycle clearly outlines the different phases involved in generating the research hypotheses and testing these hypotheses systematically using the empirical data.
- Observation: This is the process of gathering empirical data for the research. At this stage, the researcher gathers relevant empirical data using qualitative or quantitative observation methods, and this goes ahead to inform the research hypotheses.
- Induction: At this stage, the researcher makes use of inductive reasoning in order to arrive at a general probable research conclusion based on his or her observation. The researcher generates a general assumption that attempts to explain the empirical data and s/he goes on to observe the empirical data in line with this assumption.
- Deduction: This is the deductive reasoning stage. This is where the researcher generates hypotheses by applying logic and rationality to his or her observation.
- Testing: Here, the researcher puts the hypotheses to test using qualitative or quantitative research methods. In the testing stage, the researcher combines relevant instruments of systematic investigation with empirical methods in order to arrive at objective results that support or negate the research hypotheses.
- Evaluation: The evaluation research is the final stage in an empirical research study. Here, the research outlines the empirical data, the research findings and the supporting arguments plus any challenges encountered during the research process.
This information is useful for further research.
Learn about qualitative data: uncover its types and examples here.
Examples of Empirical Research
- An empirical research study can be carried out to determine if listening to happy music improves the mood of individuals. The researcher may need to conduct an experiment that involves exposing individuals to happy music to see if this improves their moods.
The findings from such an experiment will provide empirical evidence that confirms or refutes the hypotheses.
- An empirical research study can also be carried out to determine the effects of a new drug on specific groups of people. The researcher may expose the research subjects to controlled quantities of the drug and observe research subjects to controlled quantities of the drug and observe the effects over a specific period of time to gather empirical data.
- Another example of empirical research is measuring the levels of noise pollution found in an urban area to determine the average levels of sound exposure experienced by its inhabitants. Here, the researcher may have to administer questionnaires or carry out a survey in order to gather relevant data based on the experiences of the research subjects.
- Empirical research can also be carried out to determine the relationship between seasonal migration and the body mass of flying birds. A researcher may need to observe the birds and carry out necessary observation and experimentation in order to arrive at objective outcomes that answer the research question.
Empirical Research Data Collection Methods
Empirical data can be gathered using qualitative and quantitative data collection methods. Quantitative data collection methods are used for numerical data gathering while qualitative data collection processes are used to gather empirical data that cannot be quantified, that is, non-numerical data.
The following are common methods of gathering data in empirical research
- Survey/ Questionnaire
A survey is a method of data gathering that is typically employed by researchers to gather large sets of data from a specific number of respondents with regards to a research subject. This method of data gathering is often used for quantitative data collection , although it can also be deployed during quantitative research.
A survey contains a set of questions that can range from close-ended to open-ended questions together with other question types that revolve around the research subject. A survey can be administered physically or with the use of online data-gathering platforms like Formplus.
Empirical data can also be collected by carrying out an experiment. An experiment is a controlled simulation in which one or more of the research variables is manipulated using a set of interconnected processes in order to confirm or refute the research hypotheses.
An experiment is a useful method of measuring causality; that is cause and effect between dependent and independent variables in a research environment. It is an integral data gathering method in an empirical research study because it involves testing calculated assumptions in order to arrive at the most valid data and research outcomes.
T he case study method is another common data gathering method in an empirical research study. It involves sifting through and analyzing relevant cases and real-life experiences about the research subject or research variables in order to discover in-depth information that can serve as empirical data.
- Observation
The observational method is a method of qualitative data gathering that requires the researcher to study the behaviors of research variables in their natural environments in order to gather relevant information that can serve as empirical data.
How to collect Empirical Research Data with Questionnaire
With Formplus, you can create a survey or questionnaire for collecting empirical data from your research subjects. Formplus also offers multiple form sharing options so that you can share your empirical research survey to research subjects via a variety of methods.
Here is a step-by-step guide of how to collect empirical data using Formplus:
Sign in to Formplus
In the Formplus builder, you can easily create your empirical research survey by dragging and dropping preferred fields into your form. To access the Formplus builder, you will need to create an account on Formplus.
Once you do this, sign in to your account and click on “Create Form ” to begin.
Unlock the secrets of Quantitative Data: Click here to explore the types and examples.
Edit Form Title
Click on the field provided to input your form title, for example, “Empirical Research Survey”.
Edit Form
- Click on the edit button to edit the form.
- Add Fields: Drag and drop preferred form fields into your form in the Formplus builder inputs column. There are several field input options for survey forms in the Formplus builder.
- Edit fields
- Click on “Save”
- Preview form.
Customize Form
Formplus allows you to add unique features to your empirical research survey form. You can personalize your survey using various customization options. Here, you can add background images, your organization’s logo, and use other styling options. You can also change the display theme of your form.
- Share your Form Link with Respondents
Formplus offers multiple form sharing options which enables you to easily share your empirical research survey form with respondents. You can use the direct social media sharing buttons to share your form link to your organization’s social media pages.
You can send out your survey form as email invitations to your research subjects too. If you wish, you can share your form’s QR code or embed it on your organization’s website for easy access.
Empirical vs Non-Empirical Research
Empirical and non-empirical research are common methods of systematic investigation employed by researchers. Unlike empirical research that tests hypotheses in order to arrive at valid research outcomes, non-empirical research theorizes the logical assumptions of research variables.
Definition: Empirical research is a research approach that makes use of evidence-based data while non-empirical research is a research approach that makes use of theoretical data.
Method: In empirical research, the researcher arrives at valid outcomes by mainly observing research variables, creating a hypothesis and experimenting on research variables to confirm or refute the hypothesis. In non-empirical research, the researcher relies on inductive and deductive reasoning to theorize logical assumptions about the research subjects.
The major difference between the research methodology of empirical and non-empirical research is while the assumptions are tested in empirical research, they are entirely theorized in non-empirical research.
Data Sample: Empirical research makes use of empirical data while non-empirical research does not make use of empirical data. Empirical data refers to information that is gathered through experience or observation.
Unlike empirical research, theoretical or non-empirical research does not rely on data gathered through evidence. Rather, it works with logical assumptions and beliefs about the research subject.
Data Collection Methods : Empirical research makes use of quantitative and qualitative data gathering methods which may include surveys, experiments, and methods of observation. This helps the researcher to gather empirical data, that is, data backed by evidence.
Non-empirical research, on the other hand, does not make use of qualitative or quantitative methods of data collection . Instead, the researcher gathers relevant data through critical studies, systematic review and meta-analysis.
Advantages of Empirical Research
- Empirical research is flexible. In this type of systematic investigation, the researcher can adjust the research methodology including the data sample size, data gathering methods plus the data analysis methods as necessitated by the research process.
- It helps the research to understand how the research outcomes can be influenced by different research environments.
- Empirical research study helps the researcher to develop relevant analytical and observation skills that can be useful in dynamic research contexts.
- This type of research approach allows the researcher to control multiple research variables in order to arrive at the most relevant research outcomes.
- Empirical research is widely considered as one of the most authentic and competent research designs.
- It improves the internal validity of traditional research using a variety of experiments and research observation methods.
Disadvantages of Empirical Research
- An empirical research study is time-consuming because the researcher needs to gather the empirical data from multiple resources which typically takes a lot of time.
- It is not a cost-effective research approach. Usually, this method of research incurs a lot of cost because of the monetary demands of the field research.
- It may be difficult to gather the needed empirical data sample because of the multiple data gathering methods employed in an empirical research study.
- It may be difficult to gain access to some communities and firms during the data gathering process and this can affect the validity of the research.
- The report from an empirical research study is intensive and can be very lengthy in nature.
Conclusion
Empirical research is an important method of systematic investigation because it gives the researcher the opportunity to test the validity of different assumptions, in the form of hypotheses, before arriving at any findings. Hence, it is a more research approach.
There are different quantitative and qualitative methods of data gathering employed during an empirical research study based on the purpose of the research which include surveys, experiments, and various observatory methods. Surveys are one of the most common methods or empirical data collection and they can be administered online or physically.
You can use Formplus to create and administer your online empirical research survey. Formplus allows you to create survey forms that you can share with target respondents in order to obtain valuable feedback about your research context, question or subject.
In the form builder, you can add different fields to your survey form and you can also modify these form fields to suit your research process. Sign up to Formplus to access the form builder and start creating powerful online empirical research survey forms.
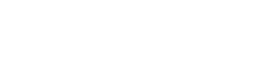
Connect to Formplus, Get Started Now - It's Free!
- advantage of empirical research
- disadvantages of empirical resarch
- empirical research characteristics
- empirical research cycle
- empirical research method
- example of empirical research
- uses of empirical research
- busayo.longe
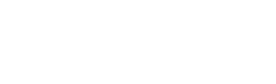
You may also like:
Extrapolation in Statistical Research: Definition, Examples, Types, Applications
In this article we’ll look at the different types and characteristics of extrapolation, plus how it contrasts to interpolation.

Research Questions: Definitions, Types + [Examples]
A comprehensive guide on the definition of research questions, types, importance, good and bad research question examples
Recall Bias: Definition, Types, Examples & Mitigation
This article will discuss the impact of recall bias in studies and the best ways to avoid them during research.
What is Pure or Basic Research? + [Examples & Method]
Simple guide on pure or basic research, its methods, characteristics, advantages, and examples in science, medicine, education and psychology
Formplus - For Seamless Data Collection
Collect data the right way with a versatile data collection tool. try formplus and transform your work productivity today..

- University of Memphis Libraries
- Research Guides
Empirical Research: Defining, Identifying, & Finding
- Defining Empirical Research
- Introduction
The Methods Section
- Database Tools
- Search Terms
- Image Descriptions
The Methods section exists to explain to the reader how the researchers collected and analyzed their data. The authors need to convince the reader that the methods used can provide an answer to the research question and that the reader can trust the results.
What Criteria to Look For
Since the "Methods" section describes how the research is being conducted, it is probably the most important section for identifying empirical research. It is where you are likely to find many criteria, including the
- Methodology, and
The Methods section is also connected to how to recreate the study. By providing sufficient information about their design, methodology, and sample, the authors of the research let other researchers know what they would need to do to recreate it. Additionally, the authors may also discuss how the methodology affects how much you can generalize from their results.
Finding the Criteria
When looking for the sample, look for where the authors discuss who was included in the research and why the authors wanted that group. Design and methodology tend to mix together. Look for where the researchers discuss how they identified the sample, how they performed the research, and how they analyzed their results.
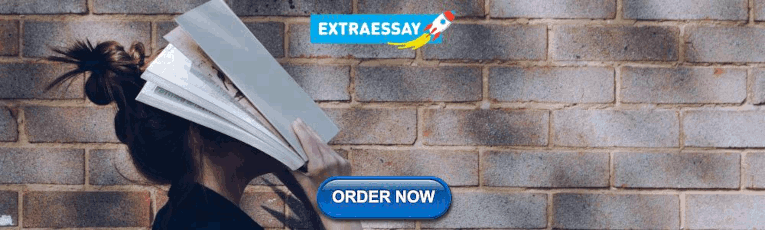
What the Section Might Be Called
The Methods section has a few common heading labels:
- Methodology
You might see "research" or "study" added to any of the above headings. The section may also be broken down into headings or subheadings for specific aspects of the methods, such as "participants," "sample," "measures," or "data analysis."
- Has a single "Methods" heading for the section which starts on page 1004.
- The first paragraph of the section discusses the sample of "24 LGBTQ students attending a public university in the southeastern United States" with a detailed breakdown of the demographics of that sample, which is then also conveyed in Table 1 on page 1005.
- The first paragraph also introduces broadly that the methodology will be interviews, and then in the second paragraph, which stretches from page 1004 to page 1005, the authors further explain methodology and design decisions including how participants were recruited, how the interviews were conducted, what types of questions were asked, how the data was collected, and how the authors analyzed that data.
- The last paragraph of the section, spanning the end of page 1005 and beginning of page 1006, begins to discuss how readers might be able to generalize the results based on limitations in the sample.
- Section labeled "Method" and begins on page 540.
- Has subheadings: Sample (page 540), Measures (page 541), and Data Analysis (page 541).
- "Sample" subheading covers the sample while "Measures" and "Data Analysis" cover methodology and design.
- Section labeled "Method" and begins on page 573.
- Has subheadings: Participants, Procedure, and Measures.
- "Participants" subheading covers sample while "Procedure" and "Measures" cover methodology and design.
- << Previous: Introduction
- Next: Results >>
- Last Updated: Apr 2, 2024 11:25 AM
- URL: https://libguides.memphis.edu/empirical-research
Table of Contents
Collaboration, information literacy, writing process, empirical research methods.
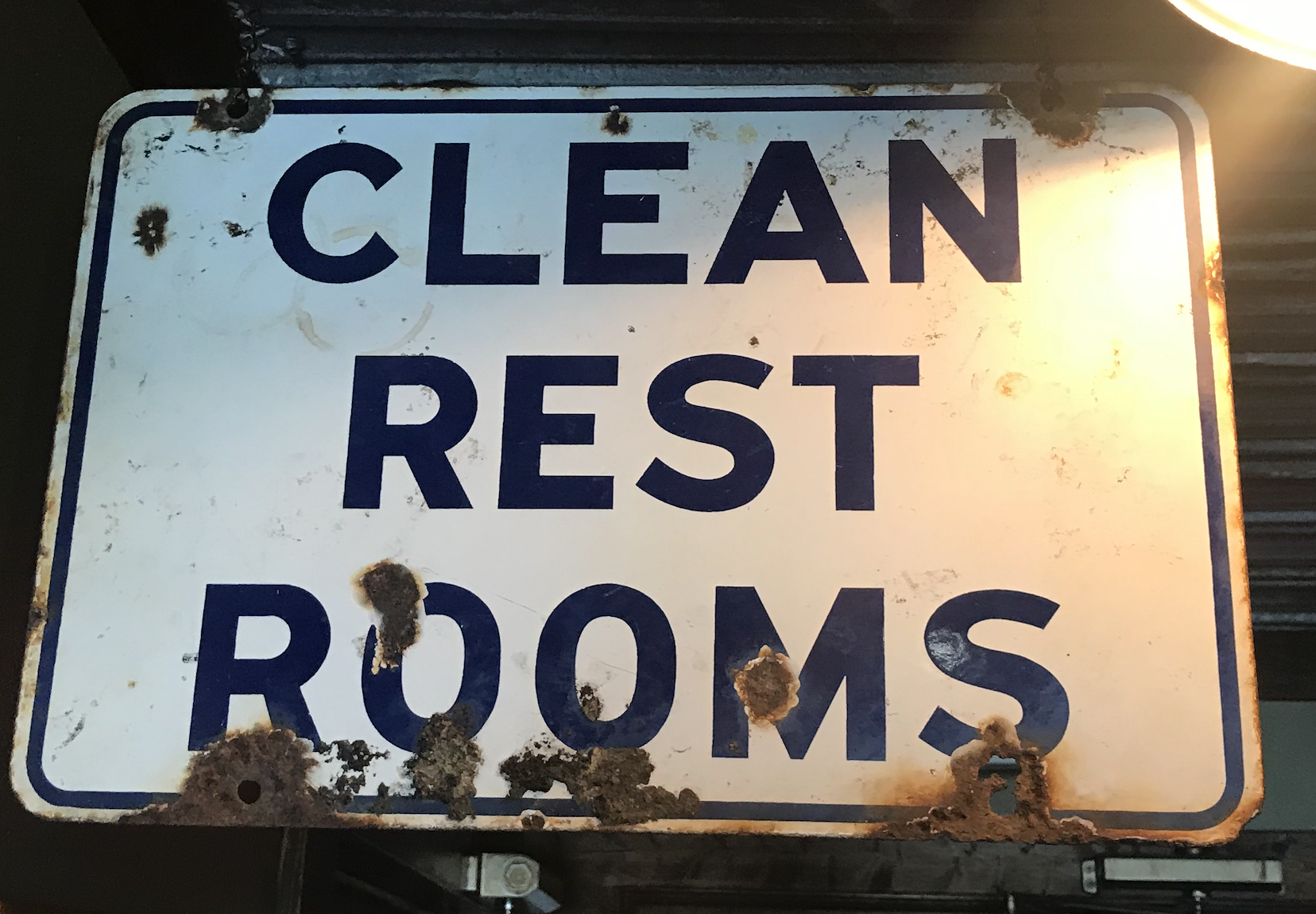
Alternative Article Title: Primary Research, Scientific Research , or Field Research .
- Empirical Research may be called Primary Research, Scientific Research , or Field Research . People who conduct empirical research are typically called investigators , but they may also be called knowledge workers, scientists, empiricists, or researchers.
Empirical research is a research method that investigators use to test knowledge claims and develop new knowledge .
Empirical methods focus on observation and experimentation .
Investigators observe and conduct experiments in systematic ways
is largely determined by their rhetorical contexts. Different workplace contexts and academic disciplines have developed unique tools and techniques for gathering and interpreting information .
professions and business organizations—i.e., discourse communities , especially methodological communities.
Professions and workplaces develop unique tools and technique
Empirical research is informed by
- empiricism , a philosophy that assumes knowledge is grounded in what you can see, hear, or experience
- positivism , a philosophy that assumes the universe is an orderly place; a nonrandom order of the universe exists; events have causes and occur in regular patterns that can be determined through observation.
Investigators and discourse communities use empirical research methods
- to create new knowledge (e.g., Basic Research )
- to solve a problem at work, school, or personal life (e.g., Applied Research ).
- to conduct replication studies–i.e., repeat a study with the same methods (or with slight variations, such as changes in subjects and experimenters).
Textual research plays an important role in empirical research . Empiricists engage in some textual research in order to understand scholarly conversations around the topics that interest them. Empiricists consult archives to learn methods for conducting empirical studies. However, there are important distinctions between how scholars weight claims in textual research and how scientists weigh claims in empirical studies.
Unlike investigators who use primarily textual methods , empiricists do not consider “claims of authority, intuition, imaginative conjecture, and abstract, theoretical, or systematic reasoning as sources of reliable belief” (Duignan, Fumerton, Quinton, Quinton 2020).
Instead of relying on logical reasoning and Following Most contemporary empiricists would acknowledge that any act of observation and experimentation are somewhat subjective processes.
There are three major types of empirical research :
- e.g., numbers, mathematical equations).
- Mixed Methods (a mixture of Quantitative Methods and Qualitative Methods .
Empirical research aims to be as objective as possible by being RAD —
- (sufficient details about the research protocol is provided so the study can be repeated)
- (the results and implications of the study can be extended in future research)
- ( quantitative evidence and/or Qualitative evidence are provided to substantiate claims, results, interpretations, implications).
Key Terms: positivism ; research methods ; research methodologies .
As humans, we learn about the world from experience, observation and experimentation. Even as babies we conduct informal research: what happens when we cry and complain? If we do x , does it cause y ? Over time, we invariably learn from our experience that our actions have consequences. We sharpen our abilities to identify commons patterns (e.g., whenwe write a lot, we are more creative). Invariably, as we evolve during our lives, we come to trust our experiences, our senses, and our procedural knowledge and declarative knowledge evolves.
In work and school settings, systematic engagement at efforts of observaion are called empirical or scientific research.
Investigators conduct empirical research when the answers to research questions are not readily available from informal research or textual research , when the occasion is kairotic , when personal or financial gains are on the table. That said, most empirical research is informed by textual research: investigators review the conclusions and implications of previously published research past studies—they analyze scholarly conversations and research methods—prior to engaging in empirical studies.
Informally, as humans, we engage routinely in the intellectual strategies that inform empirical research:
- we talk with others and listen to their stories to better understand their perceptions and experiences,
- we make observations,
- we survey friends, peers, coworkers
- we cross cultures and learn about difference, and
- we make predictions about future events based on our experiences and observations.
These same intellectual strategies we use to reason from our observations and experiences also undergird empirical research methods. For example,
- a psychologist might develop a case study based on interviews
- an anthropologist or sociologist might engage in participant observation to write an ethnographic study
- a political science researcher might survey voter trends
- a stock trader may project a stock bounce based on a 30-day moving average.
The main difference between informal and formal empirical research is intentionality : Formal empirical research presupposes a Research Plan , which is sometimes referred to as as Research Protocol . When investigators want their results to be taken seriously they have to employ the research methods a methodological community has for vetting knowledge claims .
Different academic communities (e.g., Natural Sciences, Social Science, Humanities, Arts) have unique ideas about how to conduct empirical research. Professionals in the workplace — e.g., geologists, anthropologists, biologists — use entirely different tools to gather and interpret data. Being credentialed in a particular discipline or profession is tied to mastery of unique methodological practices.
Across disciplines, however, empiricists share a number of operating assumptions: Empiricists
- develop a research plan prior to engaging in research.
- seek approval from Ethics Committees when human subjects or animal testing is involved
- explain how subjects/research participants are chosen and given opportunities to opt in or opt out of studies.
Empiricists are meticulous about how they collect data because their research must be verifiable if they want other empiricists to take their work seriously. In other words, their research plan needs to be so explicit that subsequent researchers can conduct the same study.
Empirical Research is a Rhetorical Practice
Empiricists develop their research question and their research methods by considering their audience and purpose . Prior to initiating a study, researchers conduct secondary research–especially Searching as Strategic Exploration –to identify the current knowledge about a topic. As a consequence of their deep understanding of pertinent scholarly conversations on the topic, empiricists identify gaps in knowledge.
Duignan, B., Fumerton, R., Quinton, A. M., & Quinton, B. (2020). Empiricism. Encyclopedia Britannica. https://www.britannica.com/topic/empiricism
Haswell, R. (2005). NCTE/CCCC’s recent war on scholarship. Written Communication, 22 (2), 198-223.
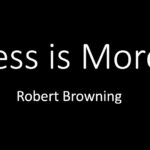
Brevity - Say More with Less
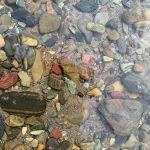
Clarity (in Speech and Writing)
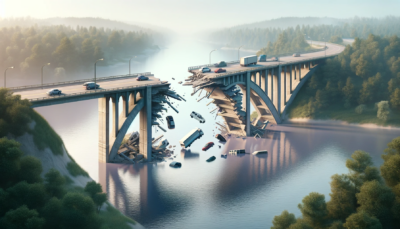
Coherence - How to Achieve Coherence in Writing
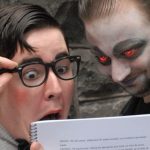
Flow - How to Create Flow in Writing
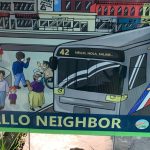
Inclusivity - Inclusive Language
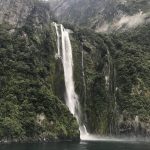
The Elements of Style - The DNA of Powerful Writing
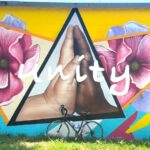
Suggested Edits
- Please select the purpose of your message. * - Corrections, Typos, or Edits Technical Support/Problems using the site Advertising with Writing Commons Copyright Issues I am contacting you about something else
- Your full name
- Your email address *
- Page URL needing edits *
- Phone This field is for validation purposes and should be left unchanged.
Other Topics:
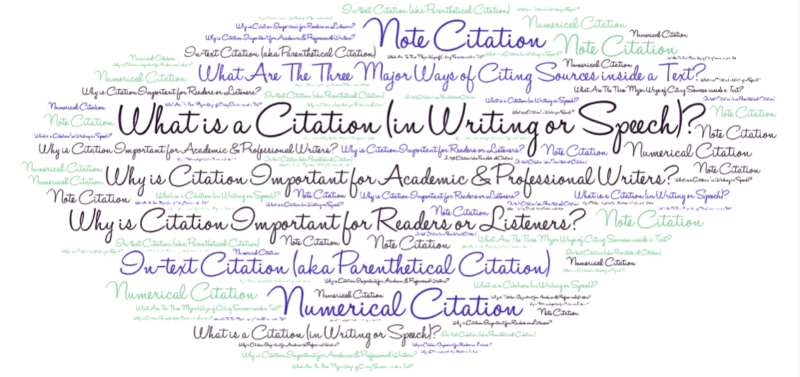
Citation - Definition - Introduction to Citation in Academic & Professional Writing
- Joseph M. Moxley
Explore the different ways to cite sources in academic and professional writing, including in-text (Parenthetical), numerical, and note citations.
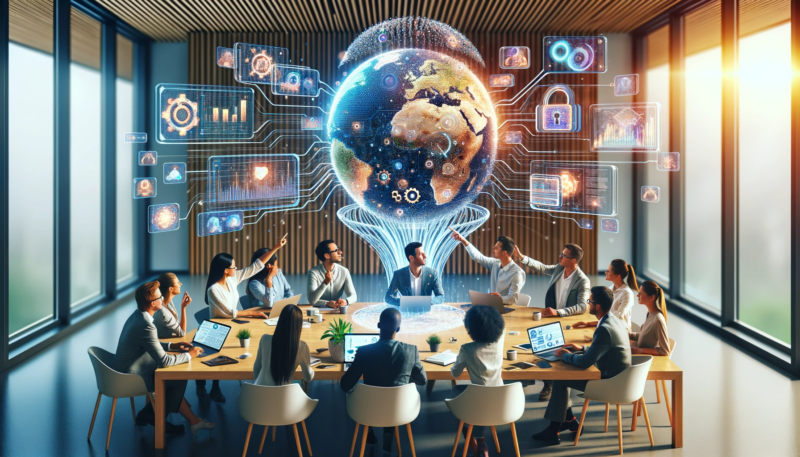
Collaboration - What is the Role of Collaboration in Academic & Professional Writing?
Collaboration refers to the act of working with others or AI to solve problems, coauthor texts, and develop products and services. Collaboration is a highly prized workplace competency in academic...
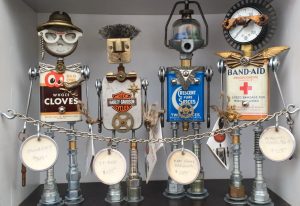
Genre may reference a type of writing, art, or musical composition; socially-agreed upon expectations about how writers and speakers should respond to particular rhetorical situations; the cultural values; the epistemological assumptions...
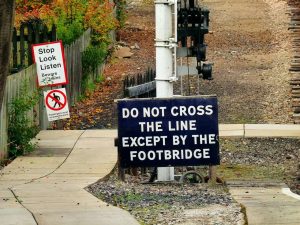
Grammar refers to the rules that inform how people and discourse communities use language (e.g., written or spoken English, body language, or visual language) to communicate. Learn about the rhetorical...
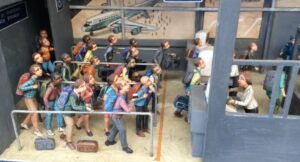
Information Literacy - Discerning Quality Information from Noise
Information Literacy refers to the competencies associated with locating, evaluating, using, and archiving information. In order to thrive, much less survive in a global information economy — an economy where information functions as a...
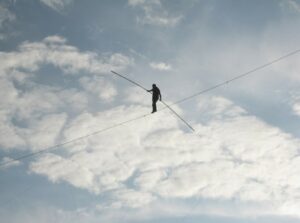
Mindset refers to a person or community’s way of feeling, thinking, and acting about a topic. The mindsets you hold, consciously or subconsciously, shape how you feel, think, and act–and...
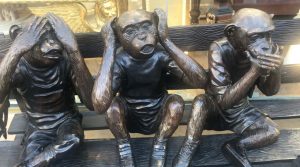
Rhetoric: Exploring Its Definition and Impact on Modern Communication
Learn about rhetoric and rhetorical practices (e.g., rhetorical analysis, rhetorical reasoning, rhetorical situation, and rhetorical stance) so that you can strategically manage how you compose and subsequently produce a text...
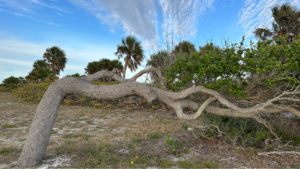
Style, most simply, refers to how you say something as opposed to what you say. The style of your writing matters because audiences are unlikely to read your work or...
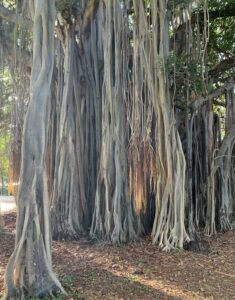
The Writing Process - Research on Composing
The writing process refers to everything you do in order to complete a writing project. Over the last six decades, researchers have studied and theorized about how writers go about...
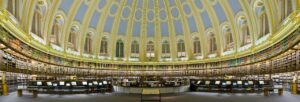
Writing Studies
Writing studies refers to an interdisciplinary community of scholars and researchers who study writing. Writing studies also refers to an academic, interdisciplinary discipline – a subject of study. Students in...
Featured Articles
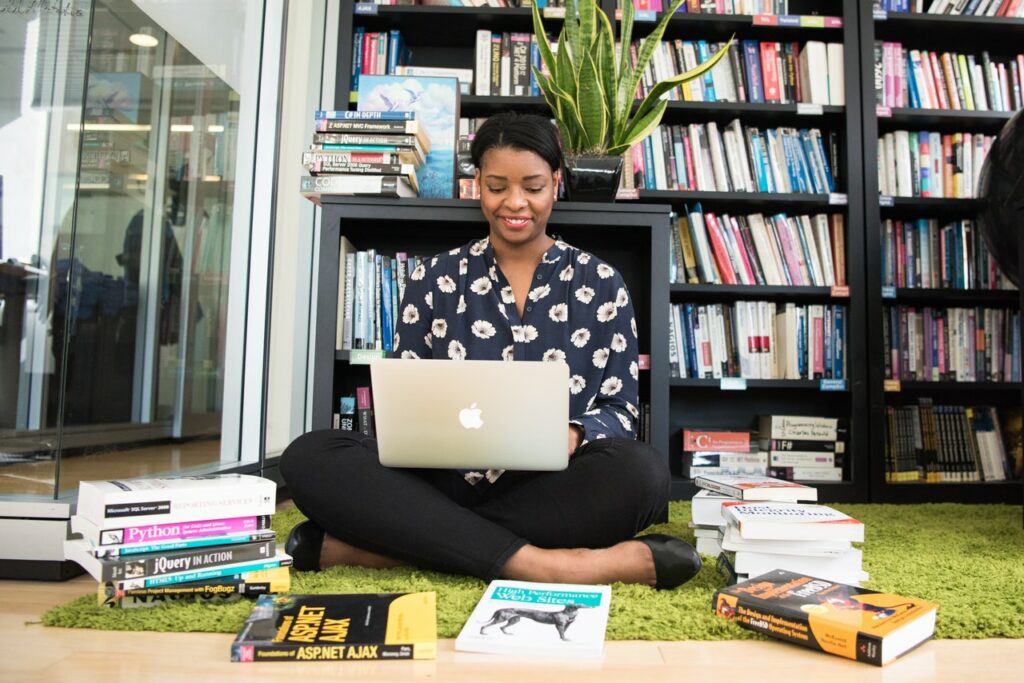
Academic Writing – How to Write for the Academic Community
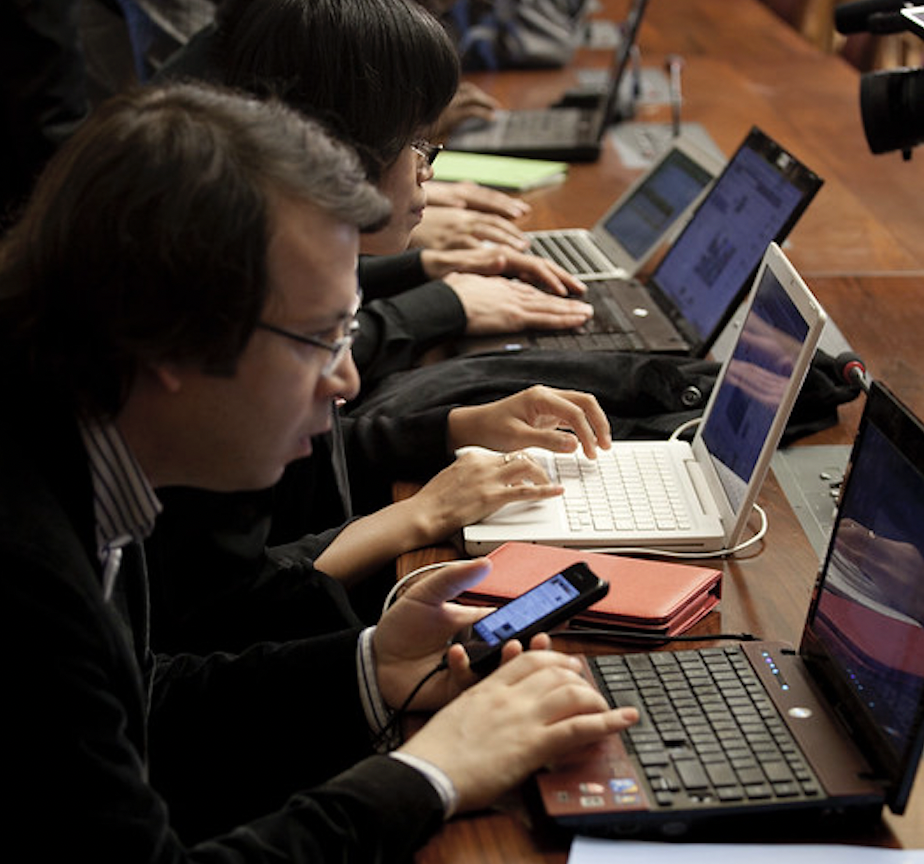
Professional Writing – How to Write for the Professional World
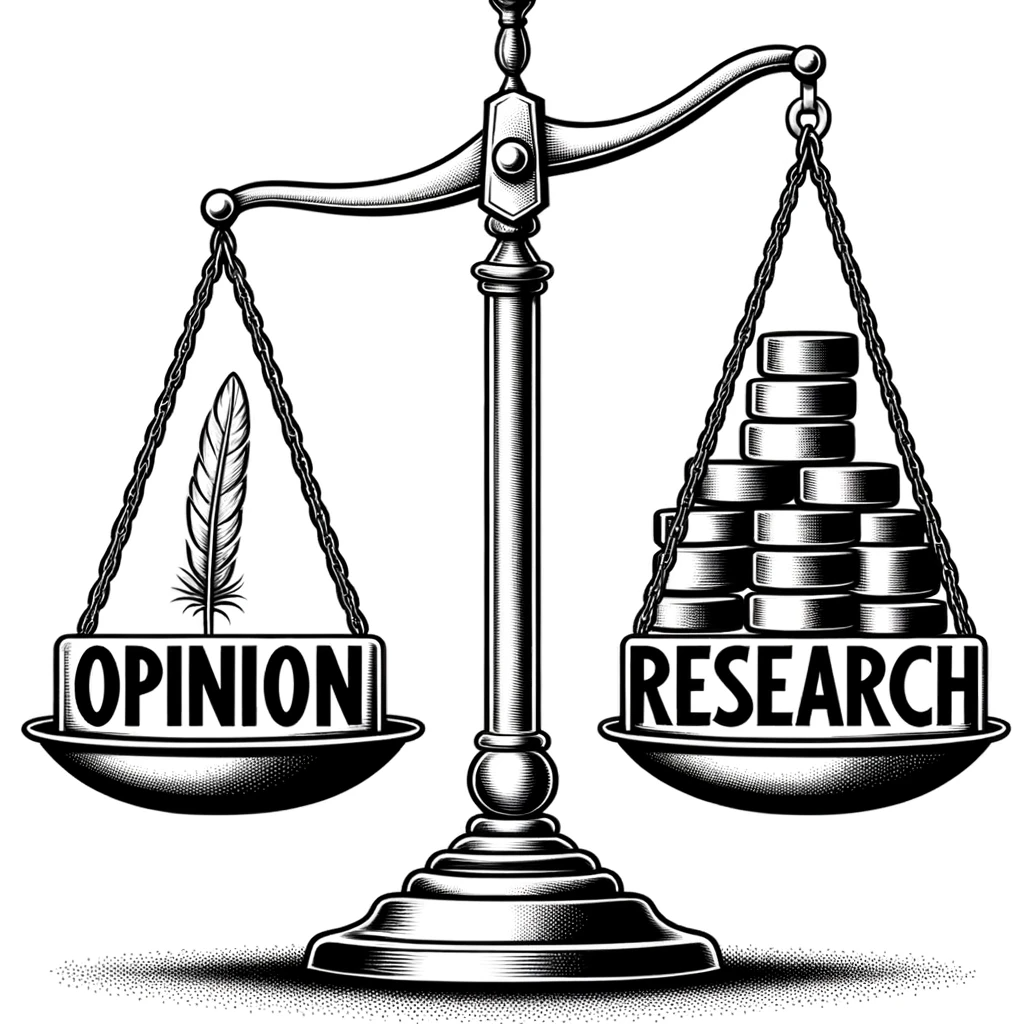
Authority – How to Establish Credibility in Speech & Writing

An official website of the United States government
The .gov means it’s official. Federal government websites often end in .gov or .mil. Before sharing sensitive information, make sure you’re on a federal government site.
The site is secure. The https:// ensures that you are connecting to the official website and that any information you provide is encrypted and transmitted securely.
- Publications
- Account settings
Preview improvements coming to the PMC website in October 2024. Learn More or Try it out now .
- Advanced Search
- Journal List
- BMC Med Res Methodol

A tutorial on methodological studies: the what, when, how and why
Lawrence mbuagbaw.
1 Department of Health Research Methods, Evidence and Impact, McMaster University, Hamilton, ON Canada
2 Biostatistics Unit/FSORC, 50 Charlton Avenue East, St Joseph’s Healthcare—Hamilton, 3rd Floor Martha Wing, Room H321, Hamilton, Ontario L8N 4A6 Canada
3 Centre for the Development of Best Practices in Health, Yaoundé, Cameroon
Daeria O. Lawson
Livia puljak.
4 Center for Evidence-Based Medicine and Health Care, Catholic University of Croatia, Ilica 242, 10000 Zagreb, Croatia
David B. Allison
5 Department of Epidemiology and Biostatistics, School of Public Health – Bloomington, Indiana University, Bloomington, IN 47405 USA
Lehana Thabane
6 Departments of Paediatrics and Anaesthesia, McMaster University, Hamilton, ON Canada
7 Centre for Evaluation of Medicine, St. Joseph’s Healthcare-Hamilton, Hamilton, ON Canada
8 Population Health Research Institute, Hamilton Health Sciences, Hamilton, ON Canada
Associated Data
Data sharing is not applicable to this article as no new data were created or analyzed in this study.
Methodological studies – studies that evaluate the design, analysis or reporting of other research-related reports – play an important role in health research. They help to highlight issues in the conduct of research with the aim of improving health research methodology, and ultimately reducing research waste.
We provide an overview of some of the key aspects of methodological studies such as what they are, and when, how and why they are done. We adopt a “frequently asked questions” format to facilitate reading this paper and provide multiple examples to help guide researchers interested in conducting methodological studies. Some of the topics addressed include: is it necessary to publish a study protocol? How to select relevant research reports and databases for a methodological study? What approaches to data extraction and statistical analysis should be considered when conducting a methodological study? What are potential threats to validity and is there a way to appraise the quality of methodological studies?
Appropriate reflection and application of basic principles of epidemiology and biostatistics are required in the design and analysis of methodological studies. This paper provides an introduction for further discussion about the conduct of methodological studies.
The field of meta-research (or research-on-research) has proliferated in recent years in response to issues with research quality and conduct [ 1 – 3 ]. As the name suggests, this field targets issues with research design, conduct, analysis and reporting. Various types of research reports are often examined as the unit of analysis in these studies (e.g. abstracts, full manuscripts, trial registry entries). Like many other novel fields of research, meta-research has seen a proliferation of use before the development of reporting guidance. For example, this was the case with randomized trials for which risk of bias tools and reporting guidelines were only developed much later – after many trials had been published and noted to have limitations [ 4 , 5 ]; and for systematic reviews as well [ 6 – 8 ]. However, in the absence of formal guidance, studies that report on research differ substantially in how they are named, conducted and reported [ 9 , 10 ]. This creates challenges in identifying, summarizing and comparing them. In this tutorial paper, we will use the term methodological study to refer to any study that reports on the design, conduct, analysis or reporting of primary or secondary research-related reports (such as trial registry entries and conference abstracts).
In the past 10 years, there has been an increase in the use of terms related to methodological studies (based on records retrieved with a keyword search [in the title and abstract] for “methodological review” and “meta-epidemiological study” in PubMed up to December 2019), suggesting that these studies may be appearing more frequently in the literature. See Fig. 1 .

Trends in the number studies that mention “methodological review” or “meta-
epidemiological study” in PubMed.
The methods used in many methodological studies have been borrowed from systematic and scoping reviews. This practice has influenced the direction of the field, with many methodological studies including searches of electronic databases, screening of records, duplicate data extraction and assessments of risk of bias in the included studies. However, the research questions posed in methodological studies do not always require the approaches listed above, and guidance is needed on when and how to apply these methods to a methodological study. Even though methodological studies can be conducted on qualitative or mixed methods research, this paper focuses on and draws examples exclusively from quantitative research.
The objectives of this paper are to provide some insights on how to conduct methodological studies so that there is greater consistency between the research questions posed, and the design, analysis and reporting of findings. We provide multiple examples to illustrate concepts and a proposed framework for categorizing methodological studies in quantitative research.
What is a methodological study?
Any study that describes or analyzes methods (design, conduct, analysis or reporting) in published (or unpublished) literature is a methodological study. Consequently, the scope of methodological studies is quite extensive and includes, but is not limited to, topics as diverse as: research question formulation [ 11 ]; adherence to reporting guidelines [ 12 – 14 ] and consistency in reporting [ 15 ]; approaches to study analysis [ 16 ]; investigating the credibility of analyses [ 17 ]; and studies that synthesize these methodological studies [ 18 ]. While the nomenclature of methodological studies is not uniform, the intents and purposes of these studies remain fairly consistent – to describe or analyze methods in primary or secondary studies. As such, methodological studies may also be classified as a subtype of observational studies.
Parallel to this are experimental studies that compare different methods. Even though they play an important role in informing optimal research methods, experimental methodological studies are beyond the scope of this paper. Examples of such studies include the randomized trials by Buscemi et al., comparing single data extraction to double data extraction [ 19 ], and Carrasco-Labra et al., comparing approaches to presenting findings in Grading of Recommendations, Assessment, Development and Evaluations (GRADE) summary of findings tables [ 20 ]. In these studies, the unit of analysis is the person or groups of individuals applying the methods. We also direct readers to the Studies Within a Trial (SWAT) and Studies Within a Review (SWAR) programme operated through the Hub for Trials Methodology Research, for further reading as a potential useful resource for these types of experimental studies [ 21 ]. Lastly, this paper is not meant to inform the conduct of research using computational simulation and mathematical modeling for which some guidance already exists [ 22 ], or studies on the development of methods using consensus-based approaches.
When should we conduct a methodological study?
Methodological studies occupy a unique niche in health research that allows them to inform methodological advances. Methodological studies should also be conducted as pre-cursors to reporting guideline development, as they provide an opportunity to understand current practices, and help to identify the need for guidance and gaps in methodological or reporting quality. For example, the development of the popular Preferred Reporting Items of Systematic reviews and Meta-Analyses (PRISMA) guidelines were preceded by methodological studies identifying poor reporting practices [ 23 , 24 ]. In these instances, after the reporting guidelines are published, methodological studies can also be used to monitor uptake of the guidelines.
These studies can also be conducted to inform the state of the art for design, analysis and reporting practices across different types of health research fields, with the aim of improving research practices, and preventing or reducing research waste. For example, Samaan et al. conducted a scoping review of adherence to different reporting guidelines in health care literature [ 18 ]. Methodological studies can also be used to determine the factors associated with reporting practices. For example, Abbade et al. investigated journal characteristics associated with the use of the Participants, Intervention, Comparison, Outcome, Timeframe (PICOT) format in framing research questions in trials of venous ulcer disease [ 11 ].
How often are methodological studies conducted?
There is no clear answer to this question. Based on a search of PubMed, the use of related terms (“methodological review” and “meta-epidemiological study”) – and therefore, the number of methodological studies – is on the rise. However, many other terms are used to describe methodological studies. There are also many studies that explore design, conduct, analysis or reporting of research reports, but that do not use any specific terms to describe or label their study design in terms of “methodology”. This diversity in nomenclature makes a census of methodological studies elusive. Appropriate terminology and key words for methodological studies are needed to facilitate improved accessibility for end-users.
Why do we conduct methodological studies?
Methodological studies provide information on the design, conduct, analysis or reporting of primary and secondary research and can be used to appraise quality, quantity, completeness, accuracy and consistency of health research. These issues can be explored in specific fields, journals, databases, geographical regions and time periods. For example, Areia et al. explored the quality of reporting of endoscopic diagnostic studies in gastroenterology [ 25 ]; Knol et al. investigated the reporting of p -values in baseline tables in randomized trial published in high impact journals [ 26 ]; Chen et al. describe adherence to the Consolidated Standards of Reporting Trials (CONSORT) statement in Chinese Journals [ 27 ]; and Hopewell et al. describe the effect of editors’ implementation of CONSORT guidelines on reporting of abstracts over time [ 28 ]. Methodological studies provide useful information to researchers, clinicians, editors, publishers and users of health literature. As a result, these studies have been at the cornerstone of important methodological developments in the past two decades and have informed the development of many health research guidelines including the highly cited CONSORT statement [ 5 ].
Where can we find methodological studies?
Methodological studies can be found in most common biomedical bibliographic databases (e.g. Embase, MEDLINE, PubMed, Web of Science). However, the biggest caveat is that methodological studies are hard to identify in the literature due to the wide variety of names used and the lack of comprehensive databases dedicated to them. A handful can be found in the Cochrane Library as “Cochrane Methodology Reviews”, but these studies only cover methodological issues related to systematic reviews. Previous attempts to catalogue all empirical studies of methods used in reviews were abandoned 10 years ago [ 29 ]. In other databases, a variety of search terms may be applied with different levels of sensitivity and specificity.
Some frequently asked questions about methodological studies
In this section, we have outlined responses to questions that might help inform the conduct of methodological studies.
Q: How should I select research reports for my methodological study?
A: Selection of research reports for a methodological study depends on the research question and eligibility criteria. Once a clear research question is set and the nature of literature one desires to review is known, one can then begin the selection process. Selection may begin with a broad search, especially if the eligibility criteria are not apparent. For example, a methodological study of Cochrane Reviews of HIV would not require a complex search as all eligible studies can easily be retrieved from the Cochrane Library after checking a few boxes [ 30 ]. On the other hand, a methodological study of subgroup analyses in trials of gastrointestinal oncology would require a search to find such trials, and further screening to identify trials that conducted a subgroup analysis [ 31 ].
The strategies used for identifying participants in observational studies can apply here. One may use a systematic search to identify all eligible studies. If the number of eligible studies is unmanageable, a random sample of articles can be expected to provide comparable results if it is sufficiently large [ 32 ]. For example, Wilson et al. used a random sample of trials from the Cochrane Stroke Group’s Trial Register to investigate completeness of reporting [ 33 ]. It is possible that a simple random sample would lead to underrepresentation of units (i.e. research reports) that are smaller in number. This is relevant if the investigators wish to compare multiple groups but have too few units in one group. In this case a stratified sample would help to create equal groups. For example, in a methodological study comparing Cochrane and non-Cochrane reviews, Kahale et al. drew random samples from both groups [ 34 ]. Alternatively, systematic or purposeful sampling strategies can be used and we encourage researchers to justify their selected approaches based on the study objective.
Q: How many databases should I search?
A: The number of databases one should search would depend on the approach to sampling, which can include targeting the entire “population” of interest or a sample of that population. If you are interested in including the entire target population for your research question, or drawing a random or systematic sample from it, then a comprehensive and exhaustive search for relevant articles is required. In this case, we recommend using systematic approaches for searching electronic databases (i.e. at least 2 databases with a replicable and time stamped search strategy). The results of your search will constitute a sampling frame from which eligible studies can be drawn.
Alternatively, if your approach to sampling is purposeful, then we recommend targeting the database(s) or data sources (e.g. journals, registries) that include the information you need. For example, if you are conducting a methodological study of high impact journals in plastic surgery and they are all indexed in PubMed, you likely do not need to search any other databases. You may also have a comprehensive list of all journals of interest and can approach your search using the journal names in your database search (or by accessing the journal archives directly from the journal’s website). Even though one could also search journals’ web pages directly, using a database such as PubMed has multiple advantages, such as the use of filters, so the search can be narrowed down to a certain period, or study types of interest. Furthermore, individual journals’ web sites may have different search functionalities, which do not necessarily yield a consistent output.
Q: Should I publish a protocol for my methodological study?
A: A protocol is a description of intended research methods. Currently, only protocols for clinical trials require registration [ 35 ]. Protocols for systematic reviews are encouraged but no formal recommendation exists. The scientific community welcomes the publication of protocols because they help protect against selective outcome reporting, the use of post hoc methodologies to embellish results, and to help avoid duplication of efforts [ 36 ]. While the latter two risks exist in methodological research, the negative consequences may be substantially less than for clinical outcomes. In a sample of 31 methodological studies, 7 (22.6%) referenced a published protocol [ 9 ]. In the Cochrane Library, there are 15 protocols for methodological reviews (21 July 2020). This suggests that publishing protocols for methodological studies is not uncommon.
Authors can consider publishing their study protocol in a scholarly journal as a manuscript. Advantages of such publication include obtaining peer-review feedback about the planned study, and easy retrieval by searching databases such as PubMed. The disadvantages in trying to publish protocols includes delays associated with manuscript handling and peer review, as well as costs, as few journals publish study protocols, and those journals mostly charge article-processing fees [ 37 ]. Authors who would like to make their protocol publicly available without publishing it in scholarly journals, could deposit their study protocols in publicly available repositories, such as the Open Science Framework ( https://osf.io/ ).
Q: How to appraise the quality of a methodological study?
A: To date, there is no published tool for appraising the risk of bias in a methodological study, but in principle, a methodological study could be considered as a type of observational study. Therefore, during conduct or appraisal, care should be taken to avoid the biases common in observational studies [ 38 ]. These biases include selection bias, comparability of groups, and ascertainment of exposure or outcome. In other words, to generate a representative sample, a comprehensive reproducible search may be necessary to build a sampling frame. Additionally, random sampling may be necessary to ensure that all the included research reports have the same probability of being selected, and the screening and selection processes should be transparent and reproducible. To ensure that the groups compared are similar in all characteristics, matching, random sampling or stratified sampling can be used. Statistical adjustments for between-group differences can also be applied at the analysis stage. Finally, duplicate data extraction can reduce errors in assessment of exposures or outcomes.
Q: Should I justify a sample size?
A: In all instances where one is not using the target population (i.e. the group to which inferences from the research report are directed) [ 39 ], a sample size justification is good practice. The sample size justification may take the form of a description of what is expected to be achieved with the number of articles selected, or a formal sample size estimation that outlines the number of articles required to answer the research question with a certain precision and power. Sample size justifications in methodological studies are reasonable in the following instances:
- Comparing two groups
- Determining a proportion, mean or another quantifier
- Determining factors associated with an outcome using regression-based analyses
For example, El Dib et al. computed a sample size requirement for a methodological study of diagnostic strategies in randomized trials, based on a confidence interval approach [ 40 ].
Q: What should I call my study?
A: Other terms which have been used to describe/label methodological studies include “ methodological review ”, “methodological survey” , “meta-epidemiological study” , “systematic review” , “systematic survey”, “meta-research”, “research-on-research” and many others. We recommend that the study nomenclature be clear, unambiguous, informative and allow for appropriate indexing. Methodological study nomenclature that should be avoided includes “ systematic review” – as this will likely be confused with a systematic review of a clinical question. “ Systematic survey” may also lead to confusion about whether the survey was systematic (i.e. using a preplanned methodology) or a survey using “ systematic” sampling (i.e. a sampling approach using specific intervals to determine who is selected) [ 32 ]. Any of the above meanings of the words “ systematic” may be true for methodological studies and could be potentially misleading. “ Meta-epidemiological study” is ideal for indexing, but not very informative as it describes an entire field. The term “ review ” may point towards an appraisal or “review” of the design, conduct, analysis or reporting (or methodological components) of the targeted research reports, yet it has also been used to describe narrative reviews [ 41 , 42 ]. The term “ survey ” is also in line with the approaches used in many methodological studies [ 9 ], and would be indicative of the sampling procedures of this study design. However, in the absence of guidelines on nomenclature, the term “ methodological study ” is broad enough to capture most of the scenarios of such studies.
Q: Should I account for clustering in my methodological study?
A: Data from methodological studies are often clustered. For example, articles coming from a specific source may have different reporting standards (e.g. the Cochrane Library). Articles within the same journal may be similar due to editorial practices and policies, reporting requirements and endorsement of guidelines. There is emerging evidence that these are real concerns that should be accounted for in analyses [ 43 ]. Some cluster variables are described in the section: “ What variables are relevant to methodological studies?”
A variety of modelling approaches can be used to account for correlated data, including the use of marginal, fixed or mixed effects regression models with appropriate computation of standard errors [ 44 ]. For example, Kosa et al. used generalized estimation equations to account for correlation of articles within journals [ 15 ]. Not accounting for clustering could lead to incorrect p -values, unduly narrow confidence intervals, and biased estimates [ 45 ].
Q: Should I extract data in duplicate?
A: Yes. Duplicate data extraction takes more time but results in less errors [ 19 ]. Data extraction errors in turn affect the effect estimate [ 46 ], and therefore should be mitigated. Duplicate data extraction should be considered in the absence of other approaches to minimize extraction errors. However, much like systematic reviews, this area will likely see rapid new advances with machine learning and natural language processing technologies to support researchers with screening and data extraction [ 47 , 48 ]. However, experience plays an important role in the quality of extracted data and inexperienced extractors should be paired with experienced extractors [ 46 , 49 ].
Q: Should I assess the risk of bias of research reports included in my methodological study?
A : Risk of bias is most useful in determining the certainty that can be placed in the effect measure from a study. In methodological studies, risk of bias may not serve the purpose of determining the trustworthiness of results, as effect measures are often not the primary goal of methodological studies. Determining risk of bias in methodological studies is likely a practice borrowed from systematic review methodology, but whose intrinsic value is not obvious in methodological studies. When it is part of the research question, investigators often focus on one aspect of risk of bias. For example, Speich investigated how blinding was reported in surgical trials [ 50 ], and Abraha et al., investigated the application of intention-to-treat analyses in systematic reviews and trials [ 51 ].
Q: What variables are relevant to methodological studies?
A: There is empirical evidence that certain variables may inform the findings in a methodological study. We outline some of these and provide a brief overview below:
- Country: Countries and regions differ in their research cultures, and the resources available to conduct research. Therefore, it is reasonable to believe that there may be differences in methodological features across countries. Methodological studies have reported loco-regional differences in reporting quality [ 52 , 53 ]. This may also be related to challenges non-English speakers face in publishing papers in English.
- Authors’ expertise: The inclusion of authors with expertise in research methodology, biostatistics, and scientific writing is likely to influence the end-product. Oltean et al. found that among randomized trials in orthopaedic surgery, the use of analyses that accounted for clustering was more likely when specialists (e.g. statistician, epidemiologist or clinical trials methodologist) were included on the study team [ 54 ]. Fleming et al. found that including methodologists in the review team was associated with appropriate use of reporting guidelines [ 55 ].
- Source of funding and conflicts of interest: Some studies have found that funded studies report better [ 56 , 57 ], while others do not [ 53 , 58 ]. The presence of funding would indicate the availability of resources deployed to ensure optimal design, conduct, analysis and reporting. However, the source of funding may introduce conflicts of interest and warrant assessment. For example, Kaiser et al. investigated the effect of industry funding on obesity or nutrition randomized trials and found that reporting quality was similar [ 59 ]. Thomas et al. looked at reporting quality of long-term weight loss trials and found that industry funded studies were better [ 60 ]. Kan et al. examined the association between industry funding and “positive trials” (trials reporting a significant intervention effect) and found that industry funding was highly predictive of a positive trial [ 61 ]. This finding is similar to that of a recent Cochrane Methodology Review by Hansen et al. [ 62 ]
- Journal characteristics: Certain journals’ characteristics may influence the study design, analysis or reporting. Characteristics such as journal endorsement of guidelines [ 63 , 64 ], and Journal Impact Factor (JIF) have been shown to be associated with reporting [ 63 , 65 – 67 ].
- Study size (sample size/number of sites): Some studies have shown that reporting is better in larger studies [ 53 , 56 , 58 ].
- Year of publication: It is reasonable to assume that design, conduct, analysis and reporting of research will change over time. Many studies have demonstrated improvements in reporting over time or after the publication of reporting guidelines [ 68 , 69 ].
- Type of intervention: In a methodological study of reporting quality of weight loss intervention studies, Thabane et al. found that trials of pharmacologic interventions were reported better than trials of non-pharmacologic interventions [ 70 ].
- Interactions between variables: Complex interactions between the previously listed variables are possible. High income countries with more resources may be more likely to conduct larger studies and incorporate a variety of experts. Authors in certain countries may prefer certain journals, and journal endorsement of guidelines and editorial policies may change over time.
Q: Should I focus only on high impact journals?
A: Investigators may choose to investigate only high impact journals because they are more likely to influence practice and policy, or because they assume that methodological standards would be higher. However, the JIF may severely limit the scope of articles included and may skew the sample towards articles with positive findings. The generalizability and applicability of findings from a handful of journals must be examined carefully, especially since the JIF varies over time. Even among journals that are all “high impact”, variations exist in methodological standards.
Q: Can I conduct a methodological study of qualitative research?
A: Yes. Even though a lot of methodological research has been conducted in the quantitative research field, methodological studies of qualitative studies are feasible. Certain databases that catalogue qualitative research including the Cumulative Index to Nursing & Allied Health Literature (CINAHL) have defined subject headings that are specific to methodological research (e.g. “research methodology”). Alternatively, one could also conduct a qualitative methodological review; that is, use qualitative approaches to synthesize methodological issues in qualitative studies.
Q: What reporting guidelines should I use for my methodological study?
A: There is no guideline that covers the entire scope of methodological studies. One adaptation of the PRISMA guidelines has been published, which works well for studies that aim to use the entire target population of research reports [ 71 ]. However, it is not widely used (40 citations in 2 years as of 09 December 2019), and methodological studies that are designed as cross-sectional or before-after studies require a more fit-for purpose guideline. A more encompassing reporting guideline for a broad range of methodological studies is currently under development [ 72 ]. However, in the absence of formal guidance, the requirements for scientific reporting should be respected, and authors of methodological studies should focus on transparency and reproducibility.
Q: What are the potential threats to validity and how can I avoid them?
A: Methodological studies may be compromised by a lack of internal or external validity. The main threats to internal validity in methodological studies are selection and confounding bias. Investigators must ensure that the methods used to select articles does not make them differ systematically from the set of articles to which they would like to make inferences. For example, attempting to make extrapolations to all journals after analyzing high-impact journals would be misleading.
Many factors (confounders) may distort the association between the exposure and outcome if the included research reports differ with respect to these factors [ 73 ]. For example, when examining the association between source of funding and completeness of reporting, it may be necessary to account for journals that endorse the guidelines. Confounding bias can be addressed by restriction, matching and statistical adjustment [ 73 ]. Restriction appears to be the method of choice for many investigators who choose to include only high impact journals or articles in a specific field. For example, Knol et al. examined the reporting of p -values in baseline tables of high impact journals [ 26 ]. Matching is also sometimes used. In the methodological study of non-randomized interventional studies of elective ventral hernia repair, Parker et al. matched prospective studies with retrospective studies and compared reporting standards [ 74 ]. Some other methodological studies use statistical adjustments. For example, Zhang et al. used regression techniques to determine the factors associated with missing participant data in trials [ 16 ].
With regard to external validity, researchers interested in conducting methodological studies must consider how generalizable or applicable their findings are. This should tie in closely with the research question and should be explicit. For example. Findings from methodological studies on trials published in high impact cardiology journals cannot be assumed to be applicable to trials in other fields. However, investigators must ensure that their sample truly represents the target sample either by a) conducting a comprehensive and exhaustive search, or b) using an appropriate and justified, randomly selected sample of research reports.
Even applicability to high impact journals may vary based on the investigators’ definition, and over time. For example, for high impact journals in the field of general medicine, Bouwmeester et al. included the Annals of Internal Medicine (AIM), BMJ, the Journal of the American Medical Association (JAMA), Lancet, the New England Journal of Medicine (NEJM), and PLoS Medicine ( n = 6) [ 75 ]. In contrast, the high impact journals selected in the methodological study by Schiller et al. were BMJ, JAMA, Lancet, and NEJM ( n = 4) [ 76 ]. Another methodological study by Kosa et al. included AIM, BMJ, JAMA, Lancet and NEJM ( n = 5). In the methodological study by Thabut et al., journals with a JIF greater than 5 were considered to be high impact. Riado Minguez et al. used first quartile journals in the Journal Citation Reports (JCR) for a specific year to determine “high impact” [ 77 ]. Ultimately, the definition of high impact will be based on the number of journals the investigators are willing to include, the year of impact and the JIF cut-off [ 78 ]. We acknowledge that the term “generalizability” may apply differently for methodological studies, especially when in many instances it is possible to include the entire target population in the sample studied.
Finally, methodological studies are not exempt from information bias which may stem from discrepancies in the included research reports [ 79 ], errors in data extraction, or inappropriate interpretation of the information extracted. Likewise, publication bias may also be a concern in methodological studies, but such concepts have not yet been explored.
A proposed framework
In order to inform discussions about methodological studies, the development of guidance for what should be reported, we have outlined some key features of methodological studies that can be used to classify them. For each of the categories outlined below, we provide an example. In our experience, the choice of approach to completing a methodological study can be informed by asking the following four questions:
- What is the aim?
A methodological study may be focused on exploring sources of bias in primary or secondary studies (meta-bias), or how bias is analyzed. We have taken care to distinguish bias (i.e. systematic deviations from the truth irrespective of the source) from reporting quality or completeness (i.e. not adhering to a specific reporting guideline or norm). An example of where this distinction would be important is in the case of a randomized trial with no blinding. This study (depending on the nature of the intervention) would be at risk of performance bias. However, if the authors report that their study was not blinded, they would have reported adequately. In fact, some methodological studies attempt to capture both “quality of conduct” and “quality of reporting”, such as Richie et al., who reported on the risk of bias in randomized trials of pharmacy practice interventions [ 80 ]. Babic et al. investigated how risk of bias was used to inform sensitivity analyses in Cochrane reviews [ 81 ]. Further, biases related to choice of outcomes can also be explored. For example, Tan et al investigated differences in treatment effect size based on the outcome reported [ 82 ].
Methodological studies may report quality of reporting against a reporting checklist (i.e. adherence to guidelines) or against expected norms. For example, Croituro et al. report on the quality of reporting in systematic reviews published in dermatology journals based on their adherence to the PRISMA statement [ 83 ], and Khan et al. described the quality of reporting of harms in randomized controlled trials published in high impact cardiovascular journals based on the CONSORT extension for harms [ 84 ]. Other methodological studies investigate reporting of certain features of interest that may not be part of formally published checklists or guidelines. For example, Mbuagbaw et al. described how often the implications for research are elaborated using the Evidence, Participants, Intervention, Comparison, Outcome, Timeframe (EPICOT) format [ 30 ].
Sometimes investigators may be interested in how consistent reports of the same research are, as it is expected that there should be consistency between: conference abstracts and published manuscripts; manuscript abstracts and manuscript main text; and trial registration and published manuscript. For example, Rosmarakis et al. investigated consistency between conference abstracts and full text manuscripts [ 85 ].
In addition to identifying issues with reporting in primary and secondary studies, authors of methodological studies may be interested in determining the factors that are associated with certain reporting practices. Many methodological studies incorporate this, albeit as a secondary outcome. For example, Farrokhyar et al. investigated the factors associated with reporting quality in randomized trials of coronary artery bypass grafting surgery [ 53 ].
Methodological studies may also be used to describe methods or compare methods, and the factors associated with methods. Muller et al. described the methods used for systematic reviews and meta-analyses of observational studies [ 86 ].
Some methodological studies synthesize results from other methodological studies. For example, Li et al. conducted a scoping review of methodological reviews that investigated consistency between full text and abstracts in primary biomedical research [ 87 ].
Some methodological studies may investigate the use of names and terms in health research. For example, Martinic et al. investigated the definitions of systematic reviews used in overviews of systematic reviews (OSRs), meta-epidemiological studies and epidemiology textbooks [ 88 ].
In addition to the previously mentioned experimental methodological studies, there may exist other types of methodological studies not captured here.
- 2. What is the design?
Most methodological studies are purely descriptive and report their findings as counts (percent) and means (standard deviation) or medians (interquartile range). For example, Mbuagbaw et al. described the reporting of research recommendations in Cochrane HIV systematic reviews [ 30 ]. Gohari et al. described the quality of reporting of randomized trials in diabetes in Iran [ 12 ].
Some methodological studies are analytical wherein “analytical studies identify and quantify associations, test hypotheses, identify causes and determine whether an association exists between variables, such as between an exposure and a disease.” [ 89 ] In the case of methodological studies all these investigations are possible. For example, Kosa et al. investigated the association between agreement in primary outcome from trial registry to published manuscript and study covariates. They found that larger and more recent studies were more likely to have agreement [ 15 ]. Tricco et al. compared the conclusion statements from Cochrane and non-Cochrane systematic reviews with a meta-analysis of the primary outcome and found that non-Cochrane reviews were more likely to report positive findings. These results are a test of the null hypothesis that the proportions of Cochrane and non-Cochrane reviews that report positive results are equal [ 90 ].
- 3. What is the sampling strategy?
Methodological reviews with narrow research questions may be able to include the entire target population. For example, in the methodological study of Cochrane HIV systematic reviews, Mbuagbaw et al. included all of the available studies ( n = 103) [ 30 ].
Many methodological studies use random samples of the target population [ 33 , 91 , 92 ]. Alternatively, purposeful sampling may be used, limiting the sample to a subset of research-related reports published within a certain time period, or in journals with a certain ranking or on a topic. Systematic sampling can also be used when random sampling may be challenging to implement.
- 4. What is the unit of analysis?
Many methodological studies use a research report (e.g. full manuscript of study, abstract portion of the study) as the unit of analysis, and inferences can be made at the study-level. However, both published and unpublished research-related reports can be studied. These may include articles, conference abstracts, registry entries etc.
Some methodological studies report on items which may occur more than once per article. For example, Paquette et al. report on subgroup analyses in Cochrane reviews of atrial fibrillation in which 17 systematic reviews planned 56 subgroup analyses [ 93 ].
This framework is outlined in Fig. 2 .

A proposed framework for methodological studies
Conclusions
Methodological studies have examined different aspects of reporting such as quality, completeness, consistency and adherence to reporting guidelines. As such, many of the methodological study examples cited in this tutorial are related to reporting. However, as an evolving field, the scope of research questions that can be addressed by methodological studies is expected to increase.
In this paper we have outlined the scope and purpose of methodological studies, along with examples of instances in which various approaches have been used. In the absence of formal guidance on the design, conduct, analysis and reporting of methodological studies, we have provided some advice to help make methodological studies consistent. This advice is grounded in good contemporary scientific practice. Generally, the research question should tie in with the sampling approach and planned analysis. We have also highlighted the variables that may inform findings from methodological studies. Lastly, we have provided suggestions for ways in which authors can categorize their methodological studies to inform their design and analysis.
Acknowledgements
Abbreviations, authors’ contributions.
LM conceived the idea and drafted the outline and paper. DOL and LT commented on the idea and draft outline. LM, LP and DOL performed literature searches and data extraction. All authors (LM, DOL, LT, LP, DBA) reviewed several draft versions of the manuscript and approved the final manuscript.
This work did not receive any dedicated funding.
Availability of data and materials
Ethics approval and consent to participate.
Not applicable.
Consent for publication
Competing interests.
DOL, DBA, LM, LP and LT are involved in the development of a reporting guideline for methodological studies.
Publisher’s Note
Springer Nature remains neutral with regard to jurisdictional claims in published maps and institutional affiliations.
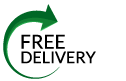
The Plagiarism Checker Online For Your Academic Work
Start Plagiarism Check
Editing & Proofreading for Your Research Paper
Get it proofread now
Online Printing & Binding with Free Express Delivery
Configure binding now
- Academic essay overview
- The writing process
- Structuring academic essays
- Types of academic essays
- Academic writing overview
- Sentence structure
- Academic writing process
- Improving your academic writing
- Titles and headings
- APA style overview
- APA citation & referencing
- APA structure & sections
- Citation & referencing
- Structure and sections
- APA examples overview
- Commonly used citations
- Other examples
- British English vs. American English
- Chicago style overview
- Chicago citation & referencing
- Chicago structure & sections
- Chicago style examples
- Citing sources overview
- Citation format
- Citation examples
- College essay overview
- Application
- How to write a college essay
- Types of college essays
- Commonly confused words
- Definitions
- Dissertation overview
- Dissertation structure & sections
- Dissertation writing process
- Graduate school overview
- Application & admission
- Study abroad
- Master degree
- Harvard referencing overview
- Language rules overview
- Grammatical rules & structures
- Parts of speech
- Punctuation
- Methodology overview
- Analyzing data
- Experiments
- Observations
- Inductive vs. Deductive
- Qualitative vs. Quantitative
- Types of validity
- Types of reliability
- Sampling methods
- Theories & Concepts
- Types of research studies
- Types of variables
- MLA style overview
- MLA examples
- MLA citation & referencing
- MLA structure & sections
- Plagiarism overview
- Plagiarism checker
- Types of plagiarism
- Printing production overview
- Research bias overview
- Types of research bias
- Example sections
- Types of research papers
- Research process overview
- Problem statement
- Research proposal
- Research topic
- Statistics overview
- Levels of measurment
- Frequency distribution
- Measures of central tendency
- Measures of variability
- Hypothesis testing
- Parameters & test statistics
- Types of distributions
- Correlation
- Effect size
- Hypothesis testing assumptions
- Types of ANOVAs
- Types of chi-square
- Statistical data
- Statistical models
- Spelling mistakes
- Tips overview
- Academic writing tips
- Dissertation tips
- Sources tips
- Working with sources overview
- Evaluating sources
- Finding sources
- Including sources
- Types of sources
Your Step to Success
Plagiarism Check within 10min
Printing & Binding with 3D Live Preview
Empirical Research – Characteristics & Advantages
How do you like this article cancel reply.
Save my name, email, and website in this browser for the next time I comment.
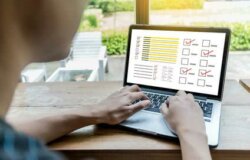
Many people ascribe to the quote, “I will not believe it unless I see it.” What most don’t know is that this quote came from empirical research enthusiasts in the renaissance period and is currently a cornerstone for modern science and research. The word empirical has a Greek origin from empeirikos, meaning experienced.

Inhaltsverzeichnis
- 1 Empirical Research – FAQs
- 2 Empirical Research: Definition
- 3 Characteristics of Empirical Research
- 4 Uses of Empirical Research
- 5 Empirical Research Methods
- 6 Empirical vs Non-Empirical Research
- 7 Advantages of Empirical Research
- 8 In a Nutshell
Empirical Research – FAQs
What is empirical research.
This is a type of research whose findings and conclusions are based on valid data or evidence. These pieces of evidence are collected using either or both qualitative research and quantitative research . In philosophy, empiricism is concluding direct observations and assessment instead of using logic alone.
What are examples of Empirical Research?
An excellent example of empirical research is the process of DNA testing and its findings.
What is Empirical and Non-Empirical Research?
While empirical research focuses on individual pieces of evidence and facts, the non-empirical study considers personal judgement and authoritative experience as necessary as the data collected.
What are the three types of research questions?
A research project can use either descriptive, relational, or causal questions to collect and analyse data.
What's the need for Empirical Research?
This form of research is quite useful in science, history, and business as it validates hypothesis, thus increasing human knowledge with tangible pieces of evidence.
Empirical Research: Definition
Empirical Resarch is a type of research where the conclusions and decisions are based on valid data or evidence. Empirical research can be analyzed quantitatively or qualitatively. Instead of using only logic, in philosophy empiricism is concluding direct observations and assessment.
Characteristics of Empirical Research
The distinctive feature of empirical research is its strictness in collecting and observing data and experiences to collect useful information that is used to create a provable analysis. For this reason, the research has six main characteristics, better known as the steps to complete empirical research.

Abstract/Purpose
The first step involves coming up with the foundational purpose of the research. The researcher answers questions like:
- What do I want to find out?
- How do I define the problem statement?
- Is the data readily available?
- Are there challenges that might limit the collection of data in terms of time, resources, language barrier, etc.?
- What is the ratio between the research itself and the finding in terms of profitability? In short, is it worth the effort?
This is the area where the researcher goes deep into why he/she wants to perform it and what he/she seeks to find or prove from the outcome.

Introduction
The second step involves having an initial report for the research. Here, any facts ever documented on the same are highlighted plus the statement of hypotheses for the current study. Also, the parties involved describe tolerable errors, measurements, and acceptable methods in this section.

This is where the actual research takes place, plus the recording of the process and data. Researchers decide whether they will use experimental or non-experimental methods for data collection in relation t the hypotheses. Depending on the field of research, they will then carve out a research design that can maximise their resources and methods of data collection. Data collection techniques are then used, which might include sampling and questionnaire methods.

Some researchers merge this part with step 3, while others handle them separately. This is where the findings from the data collection methods are put together. The data is then cleaned and analysed.

Data analysis methods include qualitative or quantitative methods. From either or both of these methods, researchers check their findings to support or reject their hypothesis. A discussion on the interpretation and implications of the findings plus the study as a whole is then documented for formal use.

Finally, credit is given to whomever the credit is due. Citations or a bibliography with materials used in the report are also included.
Uses of Empirical Research
Thanks to it insisting on having tangible pieces of evidence, empirical research comes in handy in various disciplines. The most prevalent uses are in medicine, anthropology and law. In medicine, the research helps to prove several hypotheses that increase human knowledge on the body’s behaviour and adaptability to new methods of handling diseases.
In the legal field, this type of research helps to study how rules and regulations impact the society or a certain institution understudy while in anthropology, it follows human patterns to understand cultures.
Empirical Research Methods
The two main methods used in empirical research are quantitative and qualitative. In quantitative research , numerical data is used to collect information or any other quantifiable process. The most common examples are polls, longitudinal studies and surveys.
On the other hand, qualitative research focuses more on meaning, underlying reasons and opinions from the data collected. The method takes on an unstructured approach or semi-structured. By the nature of its data, the method provides in-depth information and is often a smaller sample. Its examples include interviews, groups, and experiments.
Empirical vs Non-Empirical Research
While empirical research stops at verifiable data, non-empirical research includes authoritative opinions and logic. This is to add some meaning to the data for further elaboration. In short, data from the non-empirical study is theorised. While empirical research uses qualitative and quantitative methods, a researcher using the non-empirical method will use a lot of systematic reviews, meta-analysis, and critical studies to conclude the matter at hand.
GOOD TO KNOW: Read our article about Research Methodology !
Advantages of Empirical Research
First and foremost, this form of research has increased the credibility of findings, making it easy for someone to make an argument out of facts instead of theoretical assumptions. It keeps works authentic, formal, and verifiable, thus increasing the quality depth of information.
Secondly, empirical research is quite flexible. The researcher has the authority to adjust methodologies and also the sample size to suit any form of necessity. All in all, it is easy and the most reliable type of research.
In a Nutshell
Empirical research is, in short, an essential approach to building up information and making provable arguments to support specific implications. To wrap this up:
- Empirical research is a valid form of research that leaves no room for misinterpretation of information.
- Both qualitative and quantitative methods help to draw viable conclusions and are useful in this form of research.
- A researcher is free to explore the best approach to reach a viable conclusion within the empirical survey.
We use cookies on our website. Some of them are essential, while others help us to improve this website and your experience.
- External Media
Individual Privacy Preferences
Cookie Details Privacy Policy Imprint
Here you will find an overview of all cookies used. You can give your consent to whole categories or display further information and select certain cookies.
Accept all Save
Essential cookies enable basic functions and are necessary for the proper function of the website.
Show Cookie Information Hide Cookie Information
Statistics cookies collect information anonymously. This information helps us to understand how our visitors use our website.
Content from video platforms and social media platforms is blocked by default. If External Media cookies are accepted, access to those contents no longer requires manual consent.
Privacy Policy Imprint

Understanding the Empirical Method in Research Methodology

Table of Contents
Have you ever wondered how scientists gather evidence to support their theories? Or what steps researchers take to ensure that their findings are reliable and not just based on speculation? The answer lies in a cornerstone of scientific investigation known as the empirical method . This approach to research is all about collecting data and observing the world to form solid, evidence-based conclusions. Let’s dive into the empirical method’s fascinating world and understand why it’s so critical in research methodology.
What is the empirical method?
The empirical method is a way of gaining knowledge by means of direct and indirect observation or experience. It’s fundamentally based on the idea that knowledge comes from sensory experience and can be acquired through observation and experimentation. This method stands in contrast to approaches that rely solely on theoretical or logical means.
The role of observation in the empirical method
Observation is at the heart of the empirical method. It involves using your senses to gather information about the world. This could be as simple as noting the color of a flower or as complex as using advanced technology to observe the behavior of microscopic organisms. The key is that the observations must be systematic and replicable, providing reliable data that can be used to draw conclusions.
Data collection: qualitative and quantitative
Different types of data can be collected using the empirical method:
- Qualitative data – This data type is descriptive and conceptual, often collected through interviews, observations, and case studies.
- Quantitative data – This involves numerical data collected through methods like surveys, experiments, and statistical analysis.
Empirical vs. experimental methods
While the empirical method is often associated with experimentation, it’s important to distinguish between the two. Experimental methods involve controlled tests where the researcher manipulates one variable to observe the effect on another. In contrast, the empirical method doesn’t necessarily involve manipulation. Instead, it focuses on observing and collecting data in natural settings, offering a broader understanding of phenomena as they occur in real life.
Why the distinction matters
Understanding the difference between empirical and experimental methods is crucial because it affects how research is conducted and how results are interpreted. Empirical research can provide a more naturalistic view of the subject matter, whereas experimental research can offer more control over variables and potentially more precise outcomes.
The significance of experiential learning
The empirical method has deep roots in experiential learning, which emphasizes learning through experience. This connection is vital because it underlines the importance of engaging with the subject matter at a practical level, rather than just theoretically. It’s a hands-on approach to knowledge that has been valued since the time of Aristotle.
Developing theories from empirical research
One of the most significant aspects of the empirical method is its role in theory development . Researchers collect and analyze data, and from these findings, they can formulate or refine theories. Theories that are supported by empirical evidence tend to be more robust and widely accepted in the scientific community.
Applying the empirical method in various fields
The empirical method is not limited to the natural sciences. It’s used across a range of disciplines, from social sciences to humanities, to understand different aspects of the world. For instance:
- In psychology , researchers might use the empirical method to observe and record behaviors to understand the underlying mental processes.
- In sociology , it could involve studying social interactions to draw conclusions about societal structures.
- In economics , empirical data might be used to test the validity of economic theories or to measure market trends.
Challenges and limitations
Despite its importance, the empirical method has its challenges and limitations. One major challenge is ensuring that observations and data collection are unbiased. Additionally, not all phenomena are easily observable, and some may require more complex or abstract approaches.
The empirical method is a fundamental aspect of research methodology that has stood the test of time. By relying on observation and data collection, it allows researchers to ground their theories in reality, providing a solid foundation for knowledge. Whether it’s used in the hard sciences, social sciences, or humanities, the empirical method continues to be a critical tool for understanding our complex world.
How do you think the empirical method affects the credibility of research findings? And can you think of a situation where empirical methods might be difficult to apply but still necessary for advancing knowledge? Let’s discuss these thought-provoking questions and consider the breadth of the empirical method’s impact on the pursuit of understanding.
How useful was this post?
Click on a star to rate it!
Average rating / 5. Vote count:
No votes so far! Be the first to rate this post.
We are sorry that this post was not useful for you!
Let us improve this post!
Tell us how we can improve this post?
Submit a Comment Cancel reply
Your email address will not be published. Required fields are marked *
Save my name, email, and website in this browser for the next time I comment.
Submit Comment
Research Methodology
1 Introduction to Research in General
- Research in General
- Research Circle
- Tools of Research
- Methods: Quantitative or Qualitative
- The Product: Research Report or Papers
2 Original Unity of Philosophy and Science
- Myth Philosophy and Science: Original Unity
- The Myth: A Spiritual Metaphor
- Myth Philosophy and Science
- The Greek Quest for Unity
- The Ionian School
- Towards a Grand Unification Theory or Theory of Everything
- Einstein’s Perennial Quest for Unity
3 Evolution of the Distinct Methods of Science
- Definition of Scientific Method
- The Evolution of Scientific Methods
- Theory-Dependence of Observation
- Scope of Science and Scientific Methods
- Prevalent Mistakes in Applying the Scientific Method
4 Relation of Scientific and Philosophical Methods
- Definitions of Scientific and Philosophical method
- Philosophical method
- Scientific method
- The relation
- The Importance of Philosophical and scientific methods
5 Dialectical Method
- Introduction and a Brief Survey of the Method
- Types of Dialectics
- Dialectics in Classical Philosophy
- Dialectics in Modern Philosophy
- Critique of Dialectical Method
6 Rational Method
- Understanding Rationalism
- Rational Method of Investigation
- Descartes’ Rational Method
- Leibniz’ Aim of Philosophy
- Spinoza’ Aim of Philosophy
7 Empirical Method
- Common Features of Philosophical Method
- Empirical Method
- Exposition of Empiricism
- Locke’s Empirical Method
- Berkeley’s Empirical Method
- David Hume’s Empirical Method
8 Critical Method
- Basic Features of Critical Theory
- On Instrumental Reason
- Conception of Society
- Human History as Dialectic of Enlightenment
- Substantive Reason
- Habermasian Critical Theory
- Habermas’ Theory of Society
- Habermas’ Critique of Scientism
- Theory of Communicative Action
- Discourse Ethics of Habermas
9 Phenomenological Method (Western and Indian)
- Phenomenology in Philosophy
- Phenomenology as a Method
- Phenomenological Analysis of Knowledge
- Phenomenological Reduction
- Husserl’s Triad: Ego Cogito Cogitata
- Intentionality
- Understanding ‘Consciousness’
- Phenomenological Method in Indian Tradition
- Phenomenological Method in Religion
10 Analytical Method (Western and Indian)
- Analysis in History of Philosophy
- Conceptual Analysis
- Analysis as a Method
- Analysis in Logical Atomism and Logical Positivism
- Analytic Method in Ethics
- Language Analysis
- Quine’s Analytical Method
- Analysis in Indian Traditions
11 Hermeneutical Method (Western and Indian)
- The Power (Sakti) to Convey Meaning
- Three Meanings
- Pre-understanding
- The Semantic Autonomy of the Text
- Towards a Fusion of Horizons
- The Hermeneutical Circle
- The True Scandal of the Text
- Literary Forms
12 Deconstructive Method
- The Seminal Idea of Deconstruction in Heidegger
- Deconstruction in Derrida
- Structuralism and Post-structuralism
- Sign Signifier and Signified
- Writing and Trace
- Deconstruction as a Strategic Reading
- The Logic of Supplement
- No Outside-text
13 Method of Bibliography
- Preparing to Write
- Writing a Paper
- The Main Divisions of a Paper
- Writing Bibliography in Turabian and APA
- Sample Bibliography
14 Method of Footnotes
- Citations and Notes
- General Hints for Footnotes
- Writing Footnotes
- Examples of Footnote or Endnote
- Example of a Research Article
15 Method of Notes Taking
- Methods of Note-taking
- Note Book Style
- Note taking in a Computer
- Types of Note-taking
- Notes from Field Research
- Errors to be Avoided
16 Method of Thesis Proposal and Presentation
- Preliminary Section
- Presenting the Problem of the Thesis
- Design of the Study
- Main Body of the Thesis
- Conclusion Summary and Recommendations
- Reference Material
Share on Mastodon
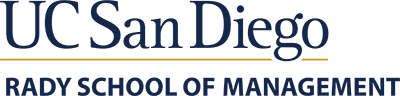
- Search This Site All UCSD Sites Faculty/Staff Search Term
- Business is a Science
- Dean’s Office
- UC San Diego's Business School
- 20th Anniversary
- Career Impact
- Student Experience
- Centers of Excellence
- Our Partnership With San Diego
- Equity Diversity, and Inclusion
- Explore by Program
- Full-Time MBA
- Part-Time MBA (FlexEvening)
- Executive MBA
- Full-Time MSBA
- Part-Time MSBA (FlexMSBA)
Master of Quantitative Finance (MQF)
- Master of Professional Accountancy (MPAc)
- Ph.D. Program
- Undergraduate Programs
- Executive Education
- Faculty & Research Overview
- Research Faculty
- Other Faculty
- What We Are Working On
- Research Seminars
- Faculty Recruitment
- Engage with Rady
- Recruit Rady Talent
- Hiring Rady Graduates
- Where Our Graduates Work
- Corporate Projects
- Connect with Rady
- Connect with us
Upcoming Events
- Request Information
- Venue Rental
Take a Virtual Tour
- Incoming Rady Students
Current Students
- Rady Alumni
- Join the Rady Network
- Career Development
- Get Involved
Master of Quantitative Finance
Distinctly quantitative, combining data science and financial modeling.
The Rady Master of Quantitative Finance prepares you to analyze the large — and often multiple — real-time data sets that drive financial decision-making and risk management. Many of the empirical methods you’ll use have emerged from UC San Diego’s own world-renowned econometrics research. This emphasis on empirical data-driven methods sets the Rady degree apart from other finance master programs.
You’ll graduate technically proficient and ready to be a sought-after colleague, collaborator and incisive decision-maker.
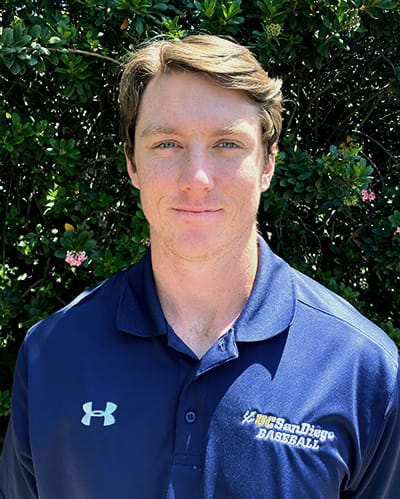
“My Life at Rady has been amazing. I’ve had the chance to meet some fantastic people who have a passion for finance and are extremely smart.” - Noah Conlon , MFin '22
Key Differentiators
Stem designated.
Our STEM designation ensures that you will be equipped with the strong quantitative and analytical skills in demand by top employers.
CFA and GARP affiliate
Scholarships for exams and topical industry seminars and meetings are available to MQF students as a benefit of the program’s academic affiliate status.
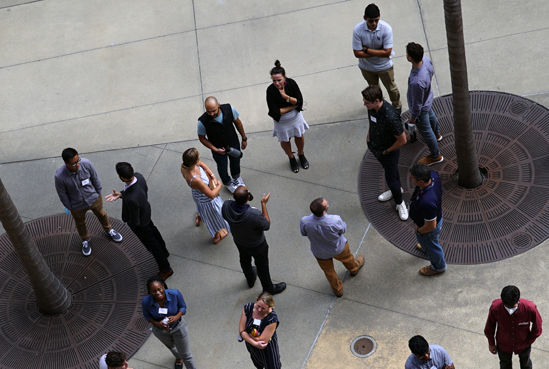
- Stats & Stories
Meet our students and learn about their experience in the MQF program.
Your coursework emphasizes cutting-edge methods for analyzing big data for financial decision-making and risk management.
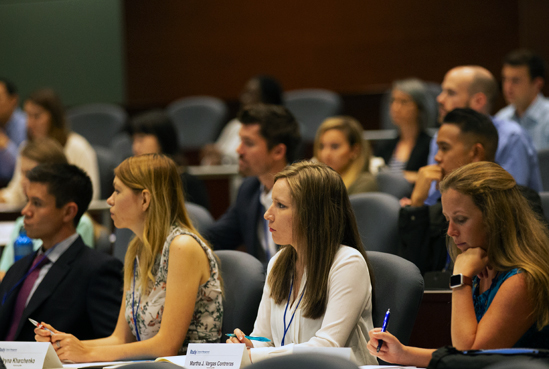
Meet a Few of our Master of Quantitative Finance Students and Alumni
Read first-hand stories about their Life at Rady, and how the data-driven coursework and support from professors helped them land amazing roles.
Meet our Students
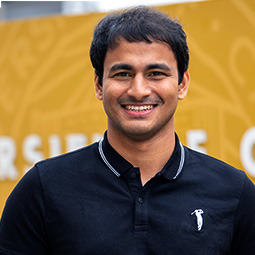
Career Impact See where a MQF degree can take you — and where our graduates work now.
Student Experience Our community is genuinely inclusive and collaborative — relationships have real staying power. Learn more about the Rady School student experience .
Admissions Learn more about program qualifications , the admissions process , and program deadlines, or contact us to meet an admissions advisor.
Tuition and Aid Everything you need to know about costs and fellowship and scholarship opportunities.
Donors & Investors
Military/Veterans
Companies and Recruiters
Contact Admissions
Schedule a Visit
Maps & Directions
- Annual Security and Fire Safety Report
- Open Staff Positions
- Tuition & Aid
- Program FAQs
Thank you for visiting nature.com. You are using a browser version with limited support for CSS. To obtain the best experience, we recommend you use a more up to date browser (or turn off compatibility mode in Internet Explorer). In the meantime, to ensure continued support, we are displaying the site without styles and JavaScript.
- View all journals
- My Account Login
- Explore content
- About the journal
- Publish with us
- Sign up for alerts
- Open access
- Published: 10 April 2024
The impact of environmental regulation on green investment efficiency of thermal power enterprises in China-based on a three-stage exogenous variable model
- Fang-rong Ren 1 ,
- Tao-feng Wu 1 ,
- Yang-jun Ren 2 ,
- Xiao-yan Liu 1 &
- Xiaomei Yuan 3
Scientific Reports volume 14 , Article number: 8400 ( 2024 ) Cite this article
Metrics details
- Energy and society
- Environmental economics
- Sustainability
Due to the increased frequency of extreme weather events and the implementation of the China’s dual-carbon target, thermal power companies have been under pressure to construct green infrastructure and to actively pursue low-carbon transformation in response to stricter environmental regulations. This research thus selects 30 listed thermal power enterprises in China as study objects and assesses their green investment efficiency in the low-carbon transition process using three-stage DEA evaluation model with environmental regulation as an exogenous variable. Based on this, a benchmark regression model is used to corroborate the relationship between environmental regulation and green investment. Simultaneously, we carry out analysis to compare the correlation between thermal power firms’ green investment efficiency and their focus on green investments. The results show in terms of total efficiency that environmental regulation significantly improves the total efficiency of 80% of thermal power enterprises compared to the absence of this exogenous variable. With the addition of environmental regulation, firms’ total efficiency declines gradually in general from 2018 to 2022, with the mean value of efficiency falling by 0.068. In terms of stage-specific efficiency, the efficiency of the green investment stage of the majority of firms is between 0.3 and 0.6, which is much lower than that of the operational stage and the market performance stage. In terms of sub-indicator efficiency, both green investment efficiency and social donation efficiency among thermal power enterprises show obvious polarization, with 30% of them having an efficiency of 1 and 30% less than 0.1. In terms of green investment focus, thermal power unit renovation has a more obvious role in boosting the green investment efficiency of thermal power enterprises than do wind power and photovoltaic projects. Therefore, both governmental departments and thermal power enterprises need to take active measures in order to achieve green transformation from the perspective of green investment efficiency. Through the segmentation of important projects of green investment, this paper provides a reasonable investment direction reference for the sustainable transformation of China’s thermal power industry. It also provides a rich and novel theoretical basis for the Chinese government to further improve the relevant environmental protection laws and regulations of thermal power industry.
Introduction
With environmental degradation and resource depletion becoming key impediments to global economic development, the green transformation of energy firms is on the horizon. According to the Energy Institute of the United Kingdom’s 2023 Statistical Yearbook of World Energy, worldwide energy demand increased by 1% in 2022. The historic increase of renewable energy has not altered the dominance of fossil fuels, which continue to supply 82% of global energy. Governments and other international organizations, such as the World Health Organization and the United Nations Environment Programme, have been actively encouraging research into environmental pollution and its effects on development in this respect. With coordinated efforts, the share of renewable energy in the world’s energy consumption from 2021 to 2022 would be 7.5%, or up around 1% from the year before. Indeed, 84% of the increase in net electricity consumption is met by a record 12% rise in wind and solar power generation.
Thermal power generation in China has accounted for 66.6% of total power generation in 2022, or growing 1.4% year on year. As a key energy supplier, thermal power firms must undergo green transformation in order to achieve sustainable development, and achieving this goal necessitates significant green investments. Global green investment increased from US$7 billion in 2000 to US$154 billion in 2010 1 , with the majority of that growth occurring in China. According to the United Nations Framework Convention on Climate Change (UNFCC), by 2050, $125 trillion in green investments will be needed to achieve carbon neutrality. According to the United Nations climate change annual report 2021, China is the largest contributor to green investment, particularly in the energy sector, valued at $266 billion. Among them, China's thermal power industry has also made large breakthroughs in the field of green investment. For example, according to the latest analysis of the China Electricity Council, the installed non-fossil energy generation capacity of the thermal power industry exceeded the size of the installed thermal power capacity for the first time in 2023, accounting for the first time for the proportion of the total installed capacity to exceed 50%.
To promote green investment, the China government has also continued to improve the environmental regulatory system by levying environmental protection taxes and opening up the carbon emissions trading market and green subsidy system. China is gradually forming a comprehensive environmental regulatory system combining command and control, market-led, and public participation 2 , 3 . According to the China Electricity Council, between 2016 and 2021 the power industry has already reduced carbon dioxide emissions by approximately 21.51 billion tons through non-fossil energy sources, reduced coal usage in power supply, and lower line-loss rate. China has now constructed the world’s largest ultra-low emission thermal power plant cluster.
As thermal power firms are critical to energy savings and emission reduction, green investment can help drive their sustainable development and also meet market and government requirements by enhancing energy cleanliness and low carbon. Thermal power companies typically boost their green investments in the early stages of tighter environmental rules in order to enhance their brand value and company image. However, because pollution control costs are rising and profit margins are narrowing due to stricter environmental restrictions, green investments have decreased rather than increased (Fig. 1 ). Therefore, it is necessary for this study to dig deeper into the reasons behind the phenomenon—that is, the trade-off between costs and benefits of green investment in thermal power enterprises as well as the relationship between enterprise green investment efficiency and the choice of green investment focus.
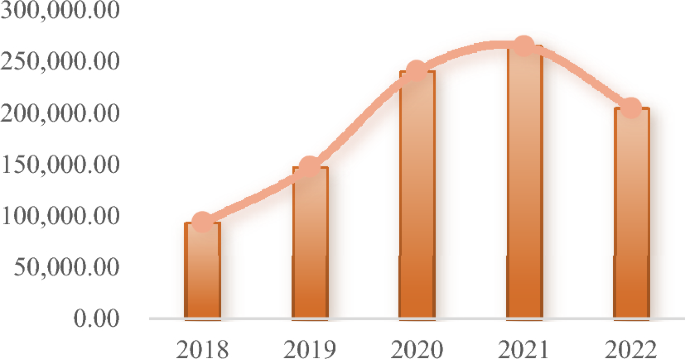
China’s thermal power industry annual green investment.
Summarizing the research conducted by scholars at home and abroad in this field, there are three main limitations. First, the analysis of existing research in this field mainly focuses on developed regions or areas, and lacks research on developing countries 1 , 4 . However, the impact of environmental regulation on green investment has a strong geographical nature, and the differences in policies across countries can lead to different implementation strengths of environmental regulation by local governments. Second, due to the difficulty of obtaining data on corporate green investment, scholars' studies on green investment have mainly focused on the national or city cluster level, and lacked analysis on the corporate level 5 , 6 . Even the studies on corporate green investment are too broad in terms of industries, ignoring the fact that the impact of environmental regulations on green investment is limited by industry attributes 3 , 7 . Third, most of the existing literature uses the amount of green investments made by firms or cities, which is rather one-sided 8 , 9 , 10 , 11 . The input–output indicator system constructed with multiple variables is more comprehensive to measure the efficiency of green investment.
Therefore, this study overcomes the above shortcomings with the following three main contributions. First, it considers that changes in both the ESG index and media attention affect the financial support received by enterprises, which in turn influences the size of the funds used by enterprises for green investment projects. Therefore, this study incorporates the enterprise ESG index and media attention into the index system for assessing the green investment efficiency of thermal power enterprises.
Second, there are fewer studies on the green investment efficiency of energy enterprises in developing countries, especially Chinese thermal power enterprises. This study analyzes the impact of environmental regulations on the green investment efficiency of Chinese thermal power enterprises in the context of the country’s social system, taking into account the reality of its economic development.
Third, other studies have analyzed the efficiency of green investment using mainly econometric models, which can only deal with a single efficiency assessment. This study adopts the DEA model, which is not only able to deal with multiple inputs and multiple outputs, but also avoids subjectivity due to the assignment of weights.
Literature review
Impact of green investment.
The literature on the impact of green investment of thermal power companies on their market value is divided into two main views: the promotion theory and the inhibition theory. Green investment is a form of resource allocation in thermal power companies, which can improve their environmental quality and corporate reputation by reducing the necessary investment in generating pollutants in their operations 1 . Taking listed energy companies in China as an example, some scholars have found that green investment improves their environmental performance through the reduction of environmental violations. This enhances the impact of green investment on the long-term market value of energy companies 12 . In addition, incorporating green investments into energy business strategy formulation helps reduce corporate environmental risks and prompts companies to create value in terms of social sustainability, ultimately leading to an increase in their market value 7 , 13 , 14 .
Some scholars have put forward opposite views on the impact of green investment on firm value, arguing that green investment has a negative or zero impact on firm performance. This is because green investment is relatively costly, takes away resources that companies use for normal production and operations, and usually requires a long payback period. Therefore, when enterprises conduct green investment operations, they incur a certain negative impact on cash flow and financial performance 15 . In short, green investment may have a certain inhibitory effect on the financial performance of enterprises. However, in the long term, green investments can help improve the environmental performance of energy companies, which in turn contributes to financial performance. Therefore, energy companies need to weigh inputs and outputs when making green investments as part of a long-term development strategy to achieve sustainable development.
Factors affecting green investment
The literature on green investment has a variety of research angles. Some scholars have studied the influencing factors and development trends of green investment in the energy industry in developed countries by using econometric models from the perspective of macroeconomics 5 , 9 . The results show that green investment is influenced by economic growth, interest rates, and fuel prices 1 . At the same time, policy intervention of the national government, such as an environmental tax burden, also has a positive impact on green investment 10 , 11 . In view of the impact of an environmental tax burden, some scholars have used a variety of advanced panel data methods to conduct more detailed research. The results show although taxes have a weak catalytic effect compared to other factors that well-structured environmental regulation can still significantly promote green investment by firms 6 . Therefore, governments can provide tax incentives for companies to undergo green innovation, so that companies can get help with innovative projects.
Some scholars have studied the relationship between corporate ESG ratings and green investment using linear mixed models. The results show that there is a positive correlation between green investment and corporate ESG ratings, which has a significant impact on corporate sustainable operations 8 . In addition, some studies start from the perspective of public attention. Through the use of panel data and econometric models, research has found that strict environmental regulations prompt enterprises to increase green investment 5 . Given the high energy intensity of countries in the Asia–Pacific region, some economists have examined the relationship between green investment and renewable energy deployment from a renewable energy perspective. Using Panel Pooled Mean Group (PMG) technique, the study found that green investment potential positively affects renewable energy deployment 16 . Finally, from the perspective of R&D and innovation, some scholars use multiple regression models to conduct empirical analyses, and the results show that environmental regulation, by increasing green investment, not only benefits green innovation in the region, but also contributes to the neighboring region's 17 .
No matter from which viewpoint, scholars’ analysis of green investment mainly focuses on the motivation, and the conclusions are controversial. Therefore, the reasons for the dispute need to be further analyzed. Moreover, research has mainly focused on the green investment of enterprises, lacking any analysis and evaluation on green investment efficiency.
Influence of environmental regulation on green investments
As an important means to coordinate social development and environmental protection, environmental regulation has played an important role in environmental governance in recent years by effectively guiding the environmental behavior of enterprises and individuals. Studies mainly measure environmental regulation by the cost of pollution control, capital expenditure on pollution reduction 18 , pollutant discharge 19 , environmental tax burden 6 , 20 , 21 , and so on. Based on the differences in the above measurement standards, the impact of environmental regulations on green investment can be divided into three perspectives.
Traditional economists have argued that environmental regulations inhibit firms' green investments. They believe that environmental regulation inhibits firms' green investment. This group of scholars argues that environmental regulation can inhibit green investment by imposing unnecessary costs on firms and having a crowding-out effect on investment in innovation, which reduces the productivity of firms 22 , 23 , 24 . However, some scholars, led by Porter, have challenged that view. Porter believes that environmental regulation can bring “innovation compensation effect”, which is conducive to the realization of the enterprise's environmental performance and economic performance of the joint improvement 25 . Therefore, this group of economists point out that environmental regulation will stimulate enterprises to break the inherent business model and product structure, and put pressure on enterprises to consider environmental issues, so as to realize energy saving and emission reduction 26 , 27 . In addition, a small number of scholars, based on the “factor endowment hypothesis”, believes in a non-linear relationship between environmental regulation and corporate green investment.
No matter using regression analysis or the SBM-DDF model, studies have shown an inverted U-type non-linear relationship between environmental regulation and corporate green investment 3 , 28 . In the early stage of strengthening environmental regulations, enterprises increase green investment and change production methods due to legal requirements. However, with increasing environmental regulation, there will be an inflection point in the factor endowment advantage, when the costs of green investment outweigh the benefits of complying with environmental regulations. This is also contrary to the Poter hypothesis, where high pollution control costs lead to "crowding out" of R&D investment 29 , and firms prefer to accept penalties for non-compliance rather than make more green investments.
Most of the literature acknowledges the significant impact of environmental regulation on enterprises’ green investment, but due to differences in indicators and methods adopted by each research institute, the conclusions are inconsistent. A higher level of environmental tax burden closely relates to strict environmental regulations 30 . As an economic means, an environmental tax burden can regulate the environmental behavior of thermal power enterprises by directly affecting their production and operation. Therefore, this study uses environmental tax burden to measure environmental regulation and studies its impact on the green investment efficiency of China’s thermal power enterprises. Doing so provides a theoretical basis for the government to formulate relevant policies for the sustainable development of thermal power enterprises.
- Green investment efficiency
As green investment efficiency has gradually become a crucial factor affecting the sustainable development of energy enterprises, empirical research based on DEA theory has also been widely used by scholars. Some scholars have analyzed the relationship between enterprise green investment and performance by the DEA method and panel vector autoregression method (VAR). Findings show that enterprises’ green investment inhibits productivity improvement 31 . From the perspective of environmental regulation, some scholars have introduced the learning curve theory into the traditional DEA model to describe the dynamic changes of power enterprises under different policy scenarios. The results show an interactive relationship between environmental regulation, environmental protection investment, and the sustainable development of power enterprises 32 . Environmental regulation motivates enterprises to pay attention to environmental performance, thus improving the efficiency of their green investment 33 .
Some scholars hold a different view, with some quantifying the green investment efficiency of heavy polluting enterprises through the SBM-DEA model. The results show an inverted U-shape non-linear relationship between environmental regulation and green investment efficiency of Chinese polluting enterprises 34 . Some scholars have also utilized the Tobit model and found a double effect of environmental regulation on the green efficiency of thermal power enterprises. It is specifically manifested as a U-shape non-linear relationship of first inhibition and then promotion 35 . Therefore, local governments must pay attention to the differentiation of environmental regulations in order to encourage energy enterprises to improve green investment efficiency.
From the perspective of digital empowerment, some scholars have used the SBM-DEA method to conduct quantitative analysis of the green investment efficiency of heavy polluting enterprises. Research has found that digital empowerment promotes green efficiency through increased analyst attention and greater R&D investment 36 .
In short, the green investment efficiency evaluation method based on DEA has wide application prospects in the current global low-carbon environmental protection era. These theories also provide a theoretical basis for government departments to guide the environmental management of energy enterprises.
Although there is a growing body of literature on the relationship between environmental regulation, green investment efficiency, and firm performance, there are still some limitations in this research field. In fact, most studies admit under strict environmental regulations that green investment of enterprises positively impacts their performance. However, the existing studies on the relationship between green investment and environmental regulation are relatively broad, mostly focusing on city clusters or all enterprises, and rarely focusing on a particular industry, especially the thermal power industry. As a result, the conclusions and policy recommendations are not fully applicable to all industries, and it is difficult for the government to improve environmental policies accordingly. In addition, the existing literature on green investment at the enterprise level is mainly limited to econometric methods, and there is less literature on the use of DEA models to study the efficiency of green investment in energy enterprises.
Research method
Based on the fact that the evaluation performance of the DDF non-ray distance function is better and provides more accurate estimation results, this study amends the traditional DDF model, combines the dynamic DEA model of Tone and Tsutsui 37 , and considers the exogenous problem, so as to solve the deficiencies of one-, two-, and three-stage Dynamic DDF under an exogenous DEA model. The formula runs as follows.
Assume that a decision-making unit ( \({DMU}_{j},j=1,\dots ,J\) ) has \(t (t=1,\dots ,T)\) time periods. Within each time period there are three stages: Stage 1, Stage 2, and Stage 3.
In the first stage, there are D inputs \({x}_{ij}^{t}\left(i=1,\dots ,m\right)\) that produce P intermediate products \({z}_{Pj}^{t}\left(p=1,\dots ,P\right)\) and Q desired outputs \({o}_{qj}^{t}\left(q=1,\dots ,Q\right)\) .
The second stage uses P intermediate products \({z}_{Pj}^{t}\left(p=1,\dots ,P\right)\) and F inputs \({a}_{fj}^{t}\left(f=1,\dots ,F\right)\) to create R desired outputs \({y}_{rj}^{t}\left(r=1,\dots ,R\right)\) and S intermediate products \({u}_{sj}^{t}\left(s=1,\dots ,S\right)\) .
The third stage uses S intermediate products \({u}_{sj}^{t}\left(s=1,\dots ,S\right)\) and G inputs \({f}_{gj}^{t}\left(g=1,\dots ,G\right)\) to create L desired outputs \({n}_{lj}^{t}\left(l=1,\dots ,L\right)\) .
Stage 1 (operation stage) inputs are number of employees and thermal power installed capacity. Output is operating revenue. Stage 1 links to Stage 2 via R&D expenses.
Stage 2 (green investment stage) inputs are green investment, proportion of installed renewable energy capacity, and social donation. Output is the ESG index. Stage 2 links to stage 3 via media attention.
Stage 3 (market performance stage) input is operating costs. Outputs are enterprise market value and market share. Exogenous variable \(={A}_{vj}\left(v=1\dots V\right)\) is environmental regulation, and carry-over \({= c}_{hj}^{t}\left(h=1,\dots ,H\right)\) is fixed assets.
Here, \(j\) represents the number of each DMU, i.e., the 30 thermal power enterprises in this paper, \(t\) represents the stage, and \(i,p,q,f,r,s,g,v,h,l\) represent the order of each variable. For example, \({x}_{ij}^{t}\) stands for the i ’th input of enterprise \(j\) in stage \(t\) .
Objective function
If there is an n dimension \(DMU\) set denoted as j , where \({DMU}_{o}\) represents the \(DMU\) under evaluation and \({DMU}_{o}\in j,\) then the mathematical model is formulated as follows.
Formula ( 1 ) calculates the efficiency of \({DMU}_{o}\) . Of these, formula ( 1 ) is primarily referenced to Chiu et al 38 .
If there is an n dimension \(DMU\) set denoted as \(j\) , where \({DMU}_{o}\) represents the \(DMU\) under evaluation and \({DMU}_{o}\in j.\)
Here, GFE represents Global-Factor Efficiency. \({\gamma }_{t}\) is the weight assigned to period t, and \({w}_{1}^{t}\) , \({w}_{2}^{t}\) , and \({w}_{3}^{t}\) are the weights assigned to Stage 1 efficiency, Stage 2 efficiency, and Stage 3 efficiency, respectively. Therefore, \({w}_{1}^{t}\) , \({w}_{2}^{t}\) , and \({w}_{3}^{t}\) and \(\sum_{t=1}^{T}{\gamma }_{t}=1\) .
Exogenous variable and links of stages
The exogenous variable is formula ( 2 ). Of these, formula ( 2 ) is primarily referenced to Li et al 39 .
Here, \( \lambda_{j}^{t} ,\;\mu_{j}^{t} ,\;\rho_{j}^{t}\) denote the weights of the benchmarking for \({DMU}_{o}\) in the first, second and three stages, respectively.
Stage 1 and Stage 2 links are formula ( 3 ). Stage 2 and Stage 3 links are formula ( 4 ). The two periods of links are formula ( 5 ). Of these, formula ( 3 ), (4), (5) is primarily referenced to Lu et al 40 .
Overall efficiency, period efficiency, stage efficiency, and period stage efficiency can be obtained from the above results.
Sub-efficiency values
The sub-efficiency values of the variables in this study are calculated in accordance with the Total-Factor Efficiency (TFE) indicator published by Hu and Wang 41 via the following equation.
Input variables and good output variables are formulae (6) and (7), respectively.
If the value of total factor efficiency is 1, then the efficiency target has been achieved; conversely, it means that there is an excess of inputs or a shortage of outputs, indicating that there is room for improvement.
Empirical analysis
Data description.
This paper selects data from 30 listed thermal power companies in China from 2018 to 2022. The selection is based on thermal power listed companies that are ranked among the top 30 in market capitalization on the Flush Financial Data Platform as of the end of 2022 and have been listed for more than five years. The abbreviations of the sample companies are in “ Appendix A ”. Since the green investment path of listed thermal power companies is not unique, different companies illustrate differentiated transformation by combining their own advantages.
Studies vary in their division of green investments. According to the green financial products invested, they can be divided into green credit, green securities, and green insurance 42 . According to the use of green investment project funds, they can be divided into expenditures for environmental pollution control and expenditures for environmental infrastructure construction 43 . As the research object of this paper is thermal power listed enterprises, power generation projects are the key aspects of their main business and green investments. Therefore, this paper classifies the green investments of enterprises into three types according to the energy type of the project by analyzing the important ongoing projects of thermal power enterprises: the transformation of thermal power units, photovoltaic projects and wind power projects (Table 1 ).
A new energy project is a national key support project that can effectively reduce the carbon emissions of enterprises. From the viewpoint of power generation cost, the cost of photovoltaic power generation has been reduced to parity and even lower than the cost of thermal power. From the viewpoint of geographical adaptability, wind power projects can be built in cities, suburbs, villages, and coastal areas with strong geographical adaptability. However, the instability of a wind project determines that thermal power units need to be used as a peaking power source to ensure stable operation of the grid. A new energy project construction cycle is longer, and it is difficult to achieve results in the short term. Therefore, thermal power companies still carry out thermal power unit renovation to maintain their stable operation.
Among the enterprises in the sample of this study, there are 25, 18, and 11 carrying out thermal power unit renovation, Photovoltaic projects, and wind power projects, respectively. There are 5 enterprises carrying out all three types of projects at the same time. Finally, 13 enterprises carry out both types of projects at the same time.
This study evaluates the number of employees, installed thermal power capacity, operating revenue, R&D expenses, green investment, proportion of installed renewable energy capacity, social donations, ESG index, media attention, operating costs, enterprise market value, market share, fixed assets, and environmental regulation of the sample companies from 2018 to 2022. Among them, Min–Max normalization is performed on the raw data of the proportion of installed renewable energy capacity and market share. The research framework based on the three-stage parallel DEA model with relevant indicators is given in Fig. 2 .
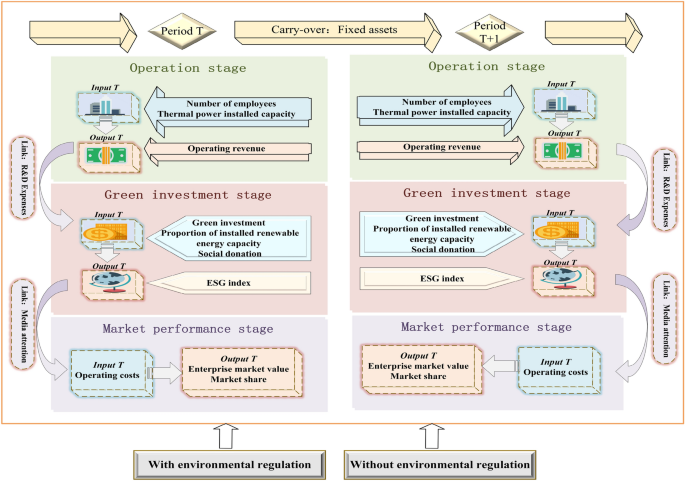
Research framework based on three-stage parallel DEA modeling.
The main design ideas of the three-stage DEA model in this paper are as follows. First, the process of assessing the green investment efficiency of thermal power enterprises is divided into operation stage (Stage 1), green investment stage (Stage 2), and market performance stage (Stage 3). The first stage is operation. Thermal power enterprises through the normal operation and profitability in this stage lay a good foundation for subsequent green investment. Thermal power installed capacity and the number of employees is mainly selected as inputs, and operating revenue and R&D expenses are outputs. The R&D expenses are continuously invested into the second stage as supportive funds for green investment.
Second, the second stage is green investment. Green investment, the proportion of installed renewable energy capacity, and social donations are taken as inputs. The ESG index and media attention are taken as outputs.
Finally, the third stage is market performance. Media attention is used as an input in the third stage to characterize its important role in a firm’s market performance. Operating costs are an input. Market value and market share are outputs. The T and T + 1 stages are connected through the carry-over variable of fixed assets. Table 2 below specifically explains each indicator.
Descriptive statistics
Due to space limitation, only major variables are selected for statistical description in this paper. Green investment stage is a key turning point for thermal power enterprises to achieve sustainable development and plays an important role in the steady operation of enterprises. Therefore, the selected indicators are categorized into two types for statistical description herein: operation and market indicators and sustainability indicators. Their mean, maximum, and standard deviation are calculated respectively, and the results are rounded to two decimal places. Figures 3 and 4 show the statistical description of the two categories of variables by year.
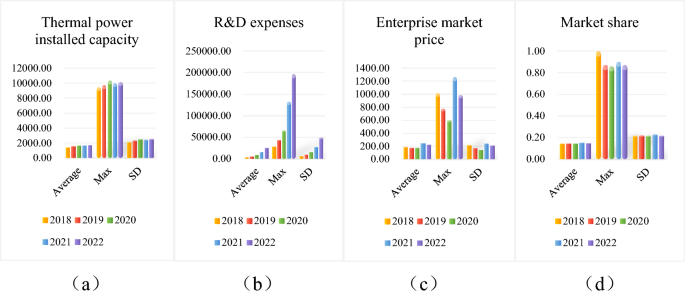
( a – d ) Descriptive statistics of operating and marketability variables.
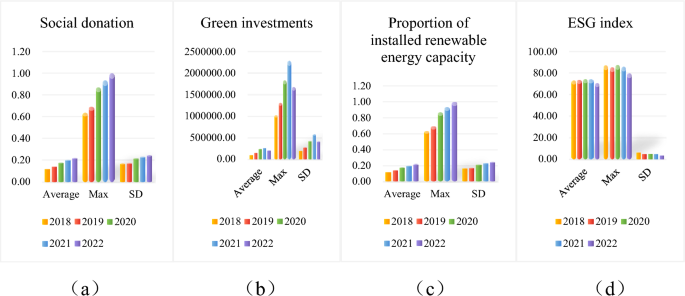
( a – d ) Descriptive statistics of sustainability variables.
In terms of the operation and market performance of thermal power enterprises, their installed capacity (Fig. 3 a) has been relatively stable in recent years. R&D expenses (Fig. 3 b) have increased year by year, and the growth rate is also increasing year by year. By raising R&D expenses, thermal power enterprises can carry out technological innovation to develop new products or new energy technologies, thus improving their production and operational efficiency. In addition, although the market value of enterprises (Fig. 3 c) has obvious fluctuations, the market share (Fig. 3 d) is generally stable and does not show large changes.
Looking at the sustainability of thermal power firms, first, social donation (Fig. 4 a) is far more volatile than the other variables, with 2019 leading the five-year period in terms of this factor. Second, green investment (Fig. 4 b), which had been trending upward in the previous four years, suddenly declined in 2022, possibly due to increased environmental regulations. Finally, the steady growth trend in the proportion of installed renewable energy capacity (Fig. 4 c) and the high level of the ESG index (Fig. 4 d) reflect that companies are actively pursuing a green and low-carbon transition.
Empirical result analysis
Total efficiency analysis.
This study considers the inclusion of exogenous variables and the absence of exogenous variables separately when assessing each DMU. According to the empirical results of this paper, after adding exogenous variables, the green investment efficiencies of 24 out of 30 firms significantly improve. Without considering exogenous factors, the average value of the overall efficiency of enterprises is 0.553, and 10 enterprises have total efficiency greater than 0.6. After considering exogenous factors, the mean value of overall efficiency is 0.591, and 13 thermal power enterprises have total efficiency greater than 0.6. Obviously, environmental regulation as an exogenous variable in the model significantly improves the underestimation of enterprises’ green investment efficiency.
As can be seen from Fig. 5 , the green investment efficiency of thermal power enterprises was at its lowest in 2019 at 0.501 and hit its peak in 2021 at 0.590. The overall trend is that it first declines, then improves, and finally declines again. In 2018–2019, at the beginning of the strengthening of environmental regulations, the increased cost of thermal power enterprises for pollution control had a crowding out effect on innovation investment. This also led to a decline in the green investment efficiency of thermal power enterprises, from 0.553 to 0.501. With the implementation of environmental policies, enterprises are forced to develop cleaner and more environmentally friendly production methods due to pressure from the environmental tax burden. For example, the renovation of thermal power units and the development of new energy projects spurred the green investment efficiency of enterprises to increase by 8.9% in two years. However, as the proportion of new energy connected to the grid increases, the power system is not flexible enough, and the problem of consumption gradually emerges. The green investment efficiency of enterprises thus exhibits a significant decrease.
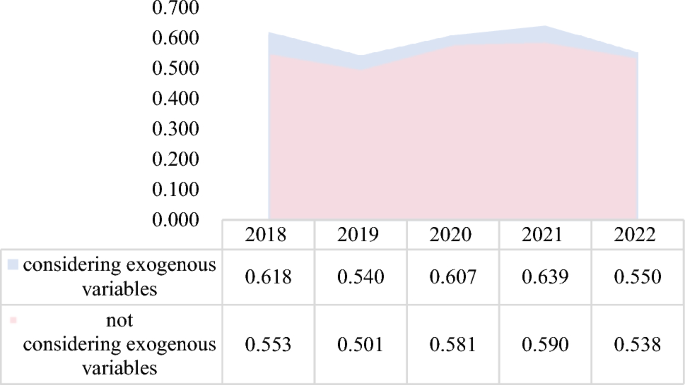
Average green investment efficiency (2018–2022).
Of the 24 thermal power companies that have improved after adhering to the environmental regulation, 18 companies are carrying out retrofitting of thermal power units and 15 are carrying out photovoltaic projects. Relatively fewer firms, less than half, are targeting the construction of wind power projects. Among the top 10 enterprises with the strongest improvement effect, 80% of them have carried out thermal power unit renovation. Obviously, enterprises prefer to realize the improvement of their green investment efficiency by retrofitting thermal power units. On the one hand, retrofitting thermal power units is flexible and has a low cost compared to new energy construction. Thermal power units can operate stably for a long period of time and adjust their output according to power demand. On the other hand, photovoltaic projects and wind power projects have strong volatility, homogeneity and require flexible resource packages to solve the consumption problem. In the case of a significant increase in electricity load, wind and solar clean resources are difficult to provide enough controllable power.
In China there are still provinces with a significant problem of abandoned wind or abandoned light. They include Inner Mongolia, Qinghai, Gansu, and other wind power provinces. Some areas of wind power or photovoltaic power only have a utilization rate of 90%. Therefore, compared with Photovoltaic projects and wind power projects, companies can more feasibly improve their total efficiency through the renovation of thermal power units.
By comparing the green investment efficiency of the top 5 and bottom 5 thermal power enterprises, this paper finds that efficiency significantly improves after considering exogenous variables. As seen from Fig. 6 , the green investment efficiency value of each thermal power enterprise varies greatly. Among them, DHEP has the lowest green investment efficiency value of 0.341, which is only 1% higher than the case without considering environmental regulation. DLPC has the highest efficiency value of 0.960, which is an increase of nearly 10% over the case without taking into account exogenous factors. The improvement is significant. Therefore, the impact of environmental regulations on DLPC is much greater than that on DHEP. This may be due to the fact that DHEP is higher than DLPC in terms of enterprise size, capital cost, and technical feasibility. Its investment in green innovation has already reached a high level, and so the improvement rate is not as high as DLPC. In addition, DHEP’s fuel costs increased by U$$2.953 billion year-over-year in 2021 due to the increase in the unit price of standard coal used for power generation. The significant increase in operating costs leads to a company’s lower gross margin and continued losses. In turn, the focus on green investments continues to diminish, and the efficiency of green investments is bound to decrease.
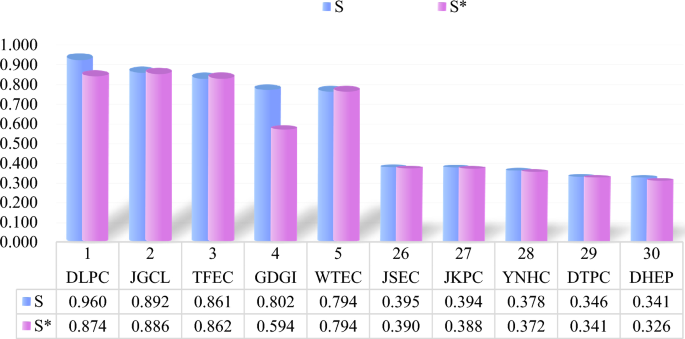
Total efficiency values of thermal power enterprises (top and bottom 5). Notes : The ranking is based on the efficiency value after adding exogenous variables. S means considering exogenous variables. S* means not considering exogenous variables.
In terms of the magnitude of improvement, the impact of environmental regulation on GDGI ranks first among the sample firms, with a 20.8% improvement in green investment efficiency. On the one hand, GDGI's earnings continue to be high, and there is enough capital to build on green investments. On the other hand, environmental regulations have been strengthened, and significant results have been achieved in green investments. Therefore, a company can decide to continue to invest in green investments in the future and continue to invest in research and development. From thermal power generation to multiple energy sources, GDGI has always adhered to the direction of clean energy development. The improvement of its green investment efficiency is also an inevitable trend.
In conclusion, environmental regulations have a more significant impact on the green investment efficiency of Chinese thermal power companies. During the period from 2018 to 2022, the average value of the environmental tax burden paid by enterprises is generally increasing. Environmental regulations force thermal power enterprises to improve their production and operation methods by increasing their environmental management costs. Moreover, thermal power enterprises combine their own advantages to carry out green transformation of industrial structure. While realizing green and high-quality development, they also promote the improvement of green investment efficiency.
Stage efficiency analysis
Efficiency analysis of operation stage (stage 1).
In the operation stage, this study includes the number of employees, installed thermal power capacity, and operating revenue in the input–output index system of this stage. According to the empirical results of this paper, during the period from 2018 to 2022 the efficiency of thermal power enterprises in the operation stage performs well with an overall mean value of 0.613. However, there are still some enterprises with low efficiency in this stage, such as DTPC, whose efficiency value fluctuates around 0.2 or less than one-third of the average value.
As seen from Fig. 7 , the inclusion of environmental regulation can significantly increase the efficiency of thermal power enterprises in the operation stage. The collection of environmental taxes raises the production costs of highly polluting enterprises, thus promoting the optimal allocation of resources and industrial restructuring. For thermal power enterprises, the collection of environmental taxes pushes them to pay more attention to the development of clean energy and to increase investment in renewable energy. By optimizing the industrial structure, the energy efficiency of thermal power enterprises will improve. In response to the role of exogenous variables in this stage, BNEC is most strongly affected, mainly because BNEC is driven by environmental regulations and focuses on the level of cost optimization and energy savings. It is a commitment to operational efficiency improvement whose improvement effect is as high as 0.462 in 2019.
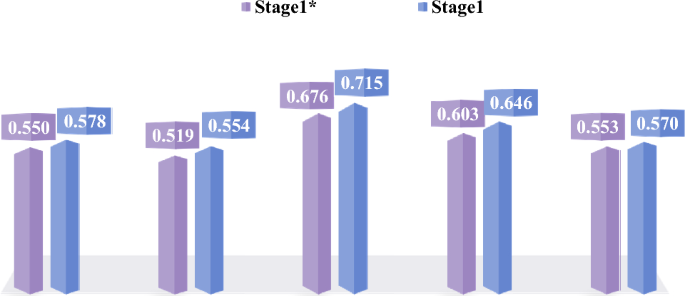
Average value of efficiency in operation stage (2018–2022). Notes : Stage1 means considering exogenous variables. Stage1* means not considering exogenous variables.
After 2020 in the late period of strengthening environmental regulations, the operational efficiency of thermal power enterprises has significantly weakened. The average efficiency fell by 14.5% in two years, perhaps due to the need for thermal power companies to invest more resources and funds in technology upgrades in order to meet more stringent environmental requirements. Therefore, for improving energy efficiency and reducing pollutant emissions, there will be some technical or economic restrictions that result in the decline of efficiency at this stage. In summary, environmental regulations can, to some extent, lead to higher efficiency values by inducing improvements in energy use efficiency and reductions in pollutant emissions during the operational stage of an enterprise. However, with increased pressure on profitability, thermal power companies may encounter some constraints in this regard, leading to a slowdown or decline in the rate of improvement of efficiency values.
Efficiency analysis of green investment stage (Stage 2)
The green investment stage is an indispensable part of thermal power enterprises to realize sustainable development. This study includes green investment, the proportion of installed renewable energy capacity, social donations, and the ESG index into the input–output index system of this stage. Table 3 shows the efficiency value of green investment stage of thermal power enterprises selected by this paper with more typical performance in this stage.
The inclusion of environmental regulations has somewhat increased the efficiency value of the green investment stage of thermal power companies during the period from 2018 to 2022. This may be due to the fact that environmental regulation incentivizes firms to approach the green investment business in a more prudent and proactive manner, which in turn promotes green investment efficiency. However, nearly one-third of the thermal power firms, such as HDEC and TFEC (Table 3 ), have reached the optimal efficiency value before the addition of the exogenous variables, and thus there is no significant improvement.
With the increase of environmental regulation pressure, the efficiency value of thermal power companies in the green investment stage shows a large decline. It falls from 0.566 in 2018 to 0.307 in 2022 or a drop of 25.9% (Fig. 8 ). Even though there is a slight rebound during the period, it again shows a more substantial fall in the following year. This is mainly due to the thermal power enterprises in the process of accelerating the development and utilization of clean energy illustrating that new energy generation will have difficulties in sending out and consuming. The wind turbines and photovoltaic equipment in many areas have been left idle for a long time.
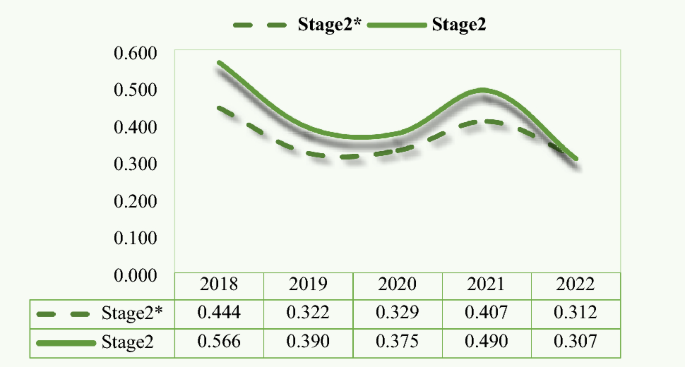
Average efficiency of green investment stage (2018–2022). Notes : Stage2 means considering exogenous variables. Stage2* means not considering exogenous variables.
The phenomenon of abandoning light and wind is serious. Moreover, supply chain issues and downward economic pressure due to COVID-19 could have also hindered green investments by thermal power companies between 2021 and 2022. However, some companies have bucked the trend. For example, GEPC’s green investment stage efficiency grew from 0.047 to 0.112, or an improvement of nearly three times.
Compared with the other two stages, the green investment stage has the lowest average efficiency value, between 0.3 and 0.6, and the largest difference in efficiency between enterprises. This is reflected in the serious bifurcation of the efficiency values of the sample enterprises in this stage. The difference between high-efficiency and low-efficiency enterprises is even close to 100%, which is also directly linked to the green investment focus of thermal power companies. Retrofitting thermal power units not only has greater potential and lower investment costs, but also smoothes out the impact of new energy power on the grid.
Thermal power units have a stronger role in improving the efficiency of the green investment stage. For example, JGCL’s green investment projects during this five-year period were dominated by the renovation of thermal power units and supplemented by the construction of new energy projects (Table 3 ). Thus, JGCL has always maintained a high level of efficiency in the green investment stage. By contrast, HDPC focuses on the construction of new energy projects, such as photovoltaic projects and wind power projects. Therefore, its efficiency in this stage is lower (Table 3 ).
Efficiency analysis of market performance stage (Stage 3)
Thermal power firms have the highest efficiency values in the market performance stage compared to the efficiency values in the other two stages. There is also not much difference in the efficiency values among the firms. Except for a few firms such as JNPC, NMHD, and SNPC, most of the other firms have efficiency values fluctuating around 0.7 in this stage. At this stage, the inclusion of environmental regulations had a relatively small effect on improving efficiency, with most firms improving by less than 5%. Overall, the market performance stage efficiency increased year-on-year with the strengthening of environmental regulations and rose much higher than the operational stage (Fig. 9 ). The unusual performance in 2018 was due to the multiple adjustments in the domestic refined petroleum product market along with the increase in coal prices during the year. They had a dampening effect on the efficiency of most thermal power companies, especially JGCL, in the market performance stage.
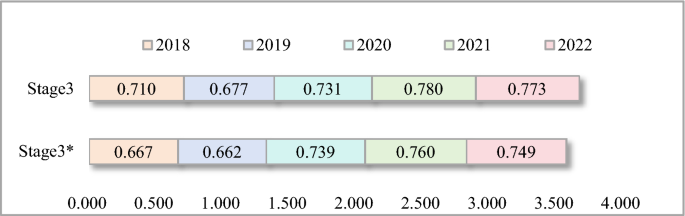
Average efficiency of market performance stage (2018–2022). Notes : Stage3 means considering exogenous variables. Stage3* means not considering exogenous variables.
The collection of environmental taxes will prompt thermal power enterprises to increase their investment in and development of clean energy. Thus, it will guide enterprises toward restructuring, transformation, and upgrading. For example, GDGI has not only raised its R&D investment year by year, but also continued to increase mergers and acquisitions of high-quality assets and clean energy to optimize its industrial layout.
There are some firms that are not sensitive to environmental regulation. For example, DTPC and HNPC, whose efficiency values in the market performance stage did not improve more significantly from the environmental tax burden (Table 4 ). The pressure of environmental regulations burden increases the cost of highly polluting and inefficient thermal power firms. For firms adopting clean energy and high efficiency technologies, their costs will be relatively lower. This leads to the exit of inefficient thermal power firms from the market. In turn, high-efficiency firms have the opportunity to expand their market share and increase their efficiency value in the market performance stage.
Sub-index efficiency analysis
Due to space limitations, only the main indicators are selected for sub-indicator efficiency analysis in this paper. Table 5 reflects the average values of the efficiency of the main indicators of Chinese thermal power enterprises from 2018 to 2022 with and without considering exogenous variables.
First, the efficiency of installed thermal power capacity directly affects the power generation capacity and capacity level of the enterprise. Hence, it is an important factor for thermal power enterprises to maintain normal business management. After considering the exogenous variable of environmental regulation, the average value of thermal power firms’ installed capacity efficiency increases in most years and peaks at 0.730 in 2020 (Table 5 ). A few firms are insensitive to the role of environmental regulation, such as JKPC and JNPC. Most companies show a very clustered thermal power installed capacity efficiency of 0.5 or more from 2018 to 2022. For highly polluting and inefficient thermal power units, environmental regulation will increase the operating costs of thermal power enterprises. Therefore, companies will actively adopt energy saving and emission reduction measures and emphasize technological innovation, such as vigorously renovating thermal power units and developing clean energy projects. For example, SNCL has invested in a number of hydrogen energy projects, driven by environmental regulation.
Second, enterprises’ market value efficiency stays at a high level from 2018 to 2021, yet declines substantially in 2022, as in the case of AHWC and GEPC. The inclusion of environmental regulations improves the average market value efficiency of thermal power firms in all years except 2022. In particular, in 2018 the average market value efficiency improves by as much as 15.9%. Environmental regulations can incentivize firms to adopt more environmental protection measures to reduce pollutant emissions, such as ultra-low emissions and desulfurization and denitrification technologies. However, in the late stage of environmental regulation strengthening, the obstruction of new energy construction and excessive cost burden can inhibit the market value efficiency of enterprises. Their average value of efficiency decreases by two-thirds from 0.931 in 2018 to 0.619 in 2022 (Table 5 ). For example, in 2018 to 2021, DTPC and DLPC have reached the optimal point of their enterprises’ market value indicator efficiency in yearly increments under the pressure of the environmental regulation. In 2022, however, excessive cost burdens led to a significant decline in firm market efficiency, or far less than half of what it was in 2021.
Third, with the strengthening of environmental regulations, the average green investment efficiency value of thermal power enterprises has been declining in fluctuation over the five-year period. The average green investment efficiency value is the highest in 2018 at 0.595, while 2022 has the lowest efficiency at 0.274 or less than half of that in 2018 (Table 5 ). Green investment efficiency rebounds considerably in 2021, but then deteriorates sharply in the following year, going from 54.2 to 27.4%. SEPC and GDGI have seen a typical plunge in the efficiency of their green investments, with a decline of almost 100%. This is mainly due to the fact that companies pay more attention to compliance in order to avoid being fined or facing other legal risks. This ultimately results in companies not being able to fully utilize the benefits of green investment.
After adding exogenous variables, the average green investment efficiency of the 30 thermal power companies has improved in the previous four years. Especially for BNEC, the addition of environmental regulation directly doubles its efficiency value several times to reach optimal efficiency. During this period, the average social donation efficiency value has fluctuated slightly, but overall, it is still slowly decreasing. The average social donation efficiency value in 2018 ranked first among the five years at 0.520 (Table 5 ). It is worth noting that both green investment efficiency and social donation efficiency of thermal power enterprises are bifurcated. High-efficiency enterprises, such as DLPC and HDEC, achieve an optimal efficiency of 1 for both green investment efficiency and social donation efficiency. However, more than half of the enterprises have both efficiencies of almost 0. This phenomenon may relate more to the enterprises’ view of social responsibility and their own operation situation.
Finally, the ESG index efficiency of most thermal power companies is decreasing year by year, from 0.907 to 0.486. This is mainly due to the fact that companies will reduce the financial pressure from an environmental tax burden by lowering environmental protection investment, which leads to the inability to fully utilize the benefits of ESG index efficiency. In the first two years, the inclusion of exogenous variables can improve the average ESG index efficiency of thermal power enterprises. However, in the latter three years, environmental regulation shows an inhibitory effect on it, and the inhibitory impact increases year by year. In 2022 the inhibitory effect of environmental regulations on the ESG index efficiency of thermal power companies hit 22.8%. This causes the average ESG index efficiency of firms to fall from 0.714 to 0.486 (Table 5 ). However, there are also firms that show an abnormal rise. For example, CEPC’s ESG index efficiency has been positively affected by environmental regulation with a small increase in efficiency values.
Regression analysis
where i denotes the individual company, t denotes the year, \({Green investment}_{it}\) denotes the amount of green investment of the thermal power enterprise, \({Environmental\; regulation}_{it}\) denotes the environmental tax burden paid by the thermal power enterprise, \({\gamma }_{t}\) denotes the time fixed effect, and \({\varepsilon }_{it}\) is the random perturbation term.
In order to further verify the impact of environmental regulation on green investment, this paper carries out a benchmark regression for formula ( 8 ). The regression results show that environmental regulation is significantly positive at the 1% level, indicating that environmental regulation can promote the green investment of thermal power enterprises. On the one hand, under the pressure of environmental regulation, thermal power enterprises will actively make green investment for tax incentives. On the other hand, environmental regulation will increase the cost pressure on thermal power enterprises, which will encourage them to make green investment to reduce their pollutant emissions. This result further confirms the accuracy of the above DEA model evaluation (Table 6 ).
Conclusions and suggestions
The above analysis shows that environmental regulation improves the green investment efficiency of thermal power enterprises. This is similar to the view of some scholars 26 , 27 , 33 , 44 . Environmental regulation improves the green investment efficiency of enterprises by encouraging them to use low-carbon technologies, which have a positive effect in promoting the sustainable development of enterprises. Some studies have also suggested that the relationship between environmental regulation and green investment is nonlinear 45 , 46 , 47 , mainly because the relationship is affected by industry and regional differences. Most thermal power enterprises are polluting enterprises, which are more sensitive to the role of environmental regulation and also bear a higher degree of pressure to spend on environmental protection compared to their own environmental pollution.
With the continuous strengthening of environmental regulation, the green investment efficiency of thermal power enterprises will gradually decrease. This is slightly different from some of the scholars’ research on heavily polluting enterprises 4 , 34 , 48 . It may be due to the fact that our study just selects the relevant data of thermal power enterprises within 5 years. Only some of the old power plants in thermal power enterprises are heavy polluters, and so there is some difference for the future trend of green investment efficiency.
Although the results of this study show a correlation between the green investment focus of thermal power enterprises and their green investment efficiency, there may still be some errors. Future research should explore the specific reasons for the changes in green investment efficiency in depth, taking into account the geographical location of thermal power enterprises and the impact of the policy environment. This will provide a more comprehensive analysis for the literature on green investment efficiency.
Conclusions
This paper draws the following conclusions by evaluating the operational, green investment and market performance efficiencies of 30 listed thermal power companies from 2018 to 2022, and regressing environmental regulation on green investment.
In terms of total efficiency, 80% of the sample firms show a significant improvement in total efficiency with the addition of environmental regulations compared to without exogenous variables. Among them, the improvement of GDGI is the most prominent. The addition of environmental regulations can force high-polluting enterprises to emphasize green innovation through the R&D mechanism, which turn plays a positive role in promoting the total efficiency of thermal power enterprises. However, with the addition of environmental regulations, the total efficiency of thermal power firms exhibits a slight decreasing trend between 2018 and 2022. This is associated with cost pressures on firms and the long-term nature of the reduction in green investments.
In terms of stage-specific efficiency, more than 60% of thermal power firms’ green investment stages are less efficient as a result of stronger environmental regulations. Compared with the operation and market performance stages, the green investment stage has the lowest efficiency at an overall average value between 0.3 and 0.6. This suggests that the green investment stage is a more difficult stage in the development process of thermal power enterprises and needs to be emphasized by enterprises.
In terms of indicative efficiency, there is a serious bifurcation in the green investment efficiency and social donation efficiency of thermal power enterprises. The difference between high-efficiency enterprises and low-efficiency enterprises even reaches 100%. This is strongly linked to the low-carbon transition strategy and operational financial status among thermal power enterprises.
In the benchmark regression analysis, environmental regulation is significantly positive at the 1% level, indicating that environmental regulation promotes green investment in electric utilities. This further confirms the accuracy of the DEA assessment.
In terms of the green investment focus of thermal power companies, compared to new energy construction, such as photovoltaic and wind power projects, thermal power unit retrofits are more effective in improving green investments in terms of economics and dexterity. Among the top 10 thermal power companies with the strongest improvement effect, eight of them have undertaken thermal power unit retrofits. Thermal unit retrofits are not only cheaper, but can also promote coal power and renewable energy interconnections. Therefore, their effect on energy utilization efficiency and green investment efficiency is more obvious.
Suggestions
Suggestions for thermal power enterprises.
First, thermal power enterprises should pay particular attention to green investment in the process of low-carbon transformation. Enterprises should not only target the expansion and upgrading of thermal power units, but also promote mature energy and low-carbon technologies.
Second, thermal power enterprises should achieve differentiated transformation according to their own situation. Due to wind power, photovoltaic and other new energy generation efficiencies are not high, and in the short term they cannot provide reliable power support. Thus, coal power installed capacity also needs to maintain reasonable growth.
Finally, thermal power companies should strengthen information disclosure. Through open and transparent information, investors can understand the enterprise’s green investment situation and the progress of low-carbon transformation as well as enhance investor confidence. In addition, through information disclosure, thermal power enterprises can also learn from the successful experience and lessons of other enterprises in order to improve their own green investment efficiency.
Suggestions for government departments
First, government departments should formulate medium- and long-term plans for the transformation of the thermal power industry as early as possible. The formulation of environmental regulations should be coordinated with market operation mechanisms. They can guide thermal power enterprises to form a market competition pattern for green investment. Governments can also provide policy preferences to enterprises that meet environmental protection standards and formulate and implement further incentive policies.
Second, in view of the phenomenon of increasing wind and solar curtailment in some local areas, government departments should coordinate solutions to the large-scale development and high-level consumption of new energy. When implementing unified dispatch across the entire grid, the State Grid Corporation should break through inter-provincial barriers, accelerate the construction of a new power system, and minimize the start-up period of thermal power as much as possible. At the same time, it can implement mandatory consumption of new energy across the entire grid. When wind and solar energy are curtailed, it can obligatorily reduce the output of matched thermal power and prioritize the transmission of new energy.
Data availability
All data generated or analyzed during this study are included in this published article and its supplementary information files.
Eyraud, L., Clements, B. & Wane, A. Green investment: Trends and determinants. Energy Pol. 60 , 852–865 (2013).
Article Google Scholar
Zhang, J. X., Cai, N. & Yang, C. Impact of environmental regulation on green growth index of industry in China. China Popul. Resour. Environ. 25 (1), 24–31 (2015).
CAS Google Scholar
Huang, L. & Lei, Z. How environmental regulation affect corporate green investment: Evidence from China. J. Clean. Prod. 279 , 123560 (2021).
Leiter, A. M., Parolini, A. & Winner, H. Environmental regulation and investment: Evidence from European industry data. Ecol. Econ. 70 (4), 759–770 (2011).
Liao, X. Public appeal, environmental regulation and green investment: Evidence from China. Energy Pol. 119 , 554–562 (2018).
Sharif, A., Kocak, S., Khan, H. H. A., Uzuner, G. & Tiwari, S. Demystifying the links between green technology innovation, economic growth, and environmental tax in ASEAN-6 countries: The dynamic role of green energy and green investment. Gondwana Res. 115 , 98–106 (2023).
Article ADS Google Scholar
Zhang, D. & Kong, Q. Renewable energy policy, green investment, and sustainability of energy firms. Renew. Eng. 192 , 118–133 (2022).
Cao, M., Duan, K. & Ibrahim, H. Green investments and their impact on ESG ratings: An evidence from China. Econ. Lett. 232 , 111365 (2023).
Zhang, H., Shao, Y., Han, X. & Chang, H. L. A road towards ecological development in China: The nexus between green investment, natural resources, green technology innovation, and economic growth. Res. Pol. 77 , 102746 (2022).
Shen, F. et al. The effect of economic growth target constraints on green technology innovation. J. Environ. Manag. 292 , 112765 (2021).
Ramanathan, R., He, Q., Black, A., Ghobadian, A. & Gallear, D. Environmental regulations, innovation and firm performance: A revisit of the Porter hypothesis. J. Clean. Prod. 155 , 79–92 (2017).
Chen, Y. & Ma, Y. Does green investment improve energy firm performance?. Energy Pol. 153 , 112252 (2021).
Tang, M., Walsh, G., Lerner, D., Fitza, M. A. & Li, Q. Green innovation, managerial concern and firm performance: An empirical study. Bus. Strateg. Environ. 27 (1), 39–51 (2018).
Saunila, M., Ukko, J. & Rantala, T. Sustainability as a driver of green innovation investment and exploitation. J. Clean. Prod. 179 , 631–641 (2018).
Wu, W., Liu, Y., Wu, C. H. & Tsai, S. B. An empirical study on government direct environmental regulation and heterogeneous innovation investment. J. Clean. Prod. 254 , 120079 (2020).
Wang, Y. & Xu, A. Green investments and development of renewable energy projects: Evidence from 15 RCEP member countries. Renew. Eng. 211 , 1045–1050 (2023).
Li, C. J., Razzaq, A., Irfan, M. & Luqman, A. Green innovation, environmental governance and green investment in China: Exploring the intrinsic mechanisms under the framework of COP26. Technol. Forecast. Soc. Change 194 , 122708 (2023).
Gray, W. B., Shadbegian, R. J., Wang, C. & Meral, M. Do EPA regulations affect labor demand? Evidence from the pulp and paper industry. J. Environ. Econ. Manag. 68 (1), 188–202 (2014).
Xing, Y. & Kolstad, C. D. Do lax environmental regulations attract foreign investment?. Environ. Resour. Econ. 21 , 1–22 (2002).
Krass, D., Nedorezov, T. & Ovchinnikov, A. Environmental taxes and the choice of green technology. Prod. Oper. Manag. 22 (5), 1035–1055 (2013).
Hieu, V. M. Influence of green investment, environmental tax and sustainable environment: Evidence from ASEAN countries. Int. J. Energy Econ. Policy 12 (3), 227–235 (2022).
Yuan, B. & Xiang, Q. Environmental regulation, industrial innovation and green development of Chinese manufacturing: Based on an extended CDM model. J. Clean. Prod. 176 , 895–908 (2018).
Orsato, R. J. Competitive environmental strategies: When does it pay to be green?. Calif. Manag. Renew. 48 (2), 127–143 (2006).
Arouri, M. E. H., Caporale, G. M., Rault, C., Sova, R. & Sova, A. Environmental regulation and competitiveness: Evidence from Romania. Ecol Econ. 81 , 130–139 (2012).
Porter, M. E. & Linde, C. V. D. Toward a new conception of the environment-competitiveness relationship. J. Econ. Perspect. 9 (4), 97–118 (1995).
Lv, C., Shao, C. & Lee, C. C. Green technology innovation and financial development: Do environmental regulation and innovation output matter?. Energy Econ. 98 , 105237 (2021).
Zhao, L., Zhang, Y., Sadiq, M., Hieu, V. M. & Ngo, T. Q. Testing green fiscal policies for green investment, innovation and green productivity amid the COVID-19 era. Econ. Change Restruct. 56 (5), 2943–2964 (2023).
Zhang, J. et al. The impact of environmental regulations on urban Green innovation efficiency: The case of Xi’an. Sust. Cities Soc. 57 , 102123 (2020).
Ouyang, X., Li, Q. & Du, K. How does environmental regulation promote technological innovations in the industrial sector? Evidence from Chinese provincial panel data. Energy Pol. 139 , 111310 (2020).
Dean, J. M., Lovely, M. E. & Wang, H. Are foreign investors attracted to weak environmental regulations? Evaluating the evidence from China. J. Dev. Econ. 90 (1), 1–13 (2009).
Lundgren, T. & Zhou, W. Firm performance and the role of environmental management. J. Environ. Manag. 203 , 330–341 (2017).
Ji, X., Li, G. & Wang, Z. Impact of emission regulation policies on Chinese power firms’ reusable environmental investments and sustainable operations. Energy Pol. 108 , 163–177 (2017).
Iyer, G. C., Clarke, L. E., Edmonds, J. A., Hultman, N. E. & McJeon, H. C. Long-term payoffs of near-term low-carbon deployment policies. Energy Pol. 86 , 493–505 (2015).
Chen, Y. & Feng, J. Do corporate green investments improve environmental performance? Evidence from the perspective of efficiency. China J. Account. Stud. 7 (1), 62–92 (2019).
Bai, Y., Hua, C., Jiao, J., Yang, M. & Li, F. Green efficiency and environmental subsidy: Evidence from thermal power firms in China. J. Clean. Prod. 188 , 49–61 (2018).
Liao, F., Hu, Y., Sun, Y. & Ye, S. Does digital empowerment affect corporate green investment efficiency?. Environ. Dev. Sustain. https://doi.org/10.1007/s10668-023-03591-5 (2023).
Article PubMed PubMed Central Google Scholar
Tone, K. & Tsutsui, M. Network DEA: A slacks-based measure approach. Eur. J. Oper. Res. 197 (1), 243–252 (2009).
Chiu, Y. H., Huang, C. W. & Ma, C. M. Assessment of China transit and economic efficiencies in a modified value-chains DEA model. Eur. J. Oper. Res. 209 (2), 95–103 (2011).
Li, L. B., Liu, B. L., Liu, W. L. & Chiu, Y. H. Efficiency evaluation of the regional high-tech industry in China: A new framework based on meta-frontier dynamic DEA analysis. Socio-Econ-Plan-Sci. 60 , 24–33 (2017).
Article CAS Google Scholar
Lu, L. C., Chiu, S. Y., Chiu, Y. H. & Chang, T. H. Three-stage circular efficiency evaluation of agricultural food production, food consumption, and food waste recycling in EU countries. J. Clean. Prod. 343 , 130870 (2022).
Hu, J. L. & Wang, S. C. Total-factor energy efficiency of regions in China. Energy pol. 34 (17), 3206–3217 (2006).
He, L. Y., Zang, L. H., Zhong, Z. Q., Wang, D. Q. & Wang, F. Green credit, renewable energy investment and green economy development: Empirical analysis based on 150 listed companies of China. J. Clean. Prod. 208 , 363–372 (2019).
Han, Y. Impact of environmental regulation policy on environmental regulation level: A quasi-natural experiment based on carbon emission trading pilot. Environ. Sci. Pollut. Res. 27 , 23602–23615 (2020).
Kesidou, E. & Demirel, P. On the drivers of eco-innovations: Empirical evidence from the UK. Res. Pol. 41 (5), 862–870 (2012).
Ai, Y. H., Peng, D. Y. & Xiong, H. H. Impact of environmental regulation intensity on green technology innovation: From the perspective of political and business connections. Sustainability. 13 (9), 4862 (2021).
Perino, G. & Requate, T. Does more stringent environmental regulation induce or reduce technology adoption? When the rate of technology adoption is inverted U-shaped. J. Environ. Econ. 64 (3), 456–467 (2012).
Google Scholar
Wang, Y. & Yu, L. Can the current environmental tax rate promote green technology innovation? Evidence from China’s resource-based industries. J. Clean. Prod. 278 , 123443 (2021).
Oggioni, G., Riccardi, R. & Toninelli, R. Eco-efficiency of the world cement industry: A data envelopment analysis. Energy Pol. 39 (5), 2842–2854 (2011).
Download references
This study was supported by Jiangsu Province Social Science Foundation Project (22GLD019), Major Project of Philosophy and Social Science Research in Universities of Jiangsu Province (2022SJZD053).
Author information
Authors and affiliations.
College of Economics and Management, Nanjing Forestry University, Nanjing, 210037, People’s Republic of China
Fang-rong Ren, Tao-feng Wu & Xiao-yan Liu
Changzhou Vocational Institute of Textile and Garment, Changzhou, 213164, People’s Republic of China
Yang-jun Ren
Shaanxi Rural Revitalization Institute, Xi’an Innovation College of Yan’an University, Xi’an, 710100, People’s Republic of China
Xiaomei Yuan
You can also search for this author in PubMed Google Scholar
Contributions
F.-rR.: conceptualization, methodology and software resources. T.-fW.: formal analysis, writing—original draft preparation. Y.-jR.: visualization and investigation. X.-yL.: data curation, writing—review and editing. X.-mY.: project administration. All authors read and contributed to the manuscript. All authors have read and agreed to the published version of the manuscript.
Corresponding author
Correspondence to Xiaomei Yuan .
Ethics declarations
Competing interests.
The authors declare no competing interests.
Additional information
Publisher's note.
Springer Nature remains neutral with regard to jurisdictional claims in published maps and institutional affiliations.
Supplementary Information
Supplementary information., supplementary information 1., rights and permissions.
Open Access This article is licensed under a Creative Commons Attribution 4.0 International License, which permits use, sharing, adaptation, distribution and reproduction in any medium or format, as long as you give appropriate credit to the original author(s) and the source, provide a link to the Creative Commons licence, and indicate if changes were made. The images or other third party material in this article are included in the article's Creative Commons licence, unless indicated otherwise in a credit line to the material. If material is not included in the article's Creative Commons licence and your intended use is not permitted by statutory regulation or exceeds the permitted use, you will need to obtain permission directly from the copyright holder. To view a copy of this licence, visit http://creativecommons.org/licenses/by/4.0/ .
Reprints and permissions
About this article
Cite this article.
Ren, Fr., Wu, Tf., Ren, Yj. et al. The impact of environmental regulation on green investment efficiency of thermal power enterprises in China-based on a three-stage exogenous variable model. Sci Rep 14 , 8400 (2024). https://doi.org/10.1038/s41598-024-58396-x
Download citation
Received : 16 January 2024
Accepted : 28 March 2024
Published : 10 April 2024
DOI : https://doi.org/10.1038/s41598-024-58396-x
Share this article
Anyone you share the following link with will be able to read this content:
Sorry, a shareable link is not currently available for this article.
Provided by the Springer Nature SharedIt content-sharing initiative
- Environmental regulation
- Thermal power enterprises
- Three-stage model
- Exogenous variable
By submitting a comment you agree to abide by our Terms and Community Guidelines . If you find something abusive or that does not comply with our terms or guidelines please flag it as inappropriate.
Quick links
- Explore articles by subject
- Guide to authors
- Editorial policies
Sign up for the Nature Briefing newsletter — what matters in science, free to your inbox daily.

- Reference Manager
- Simple TEXT file
People also looked at
Original research article, how has the rural digital economy influenced agricultural carbon emissions agricultural green technology change as a mediated variable.
- 1 Guiyang Institute of Humanities and Technology, Guiyang, China
- 2 Binary University of Management and Entrepreneurship, Selangor, Malaysia
- 3 Business School, Nanjing Normal University, Nanjing, China
- 4 College of Economics and Management, Nanjing University of Aeronautics and Astronautics, Nanjing, China
- 5 School of Politics and Economic Administration, Guizhou Minzu University, Guiyang, China
Digital economy is being closely integrated with agricultural development and tapping into its unique potential to alleviate agriculture’s carbon emissions To explore the mechanism of how digital economy reduce the agricultural carbon emissions, this paper constructs a systematic evaluation method with extend STIRPAT model and panel data drawn from 29 provinces (or municipalities and autonomous regions) in the Chinese mainland from 2013–2020. The results show that the development of the rural digital economy has a significant negative influence on agricultural CEs, and this result is still valid given robustness tests. Second, the alleviation of CEs based on the rural digital economy is more significant in the higher technological investment zones than that in the lower technological investment zones, and the central and eastern regions also have more significant CEs reduction effect. Third, the influence mechanism analysis shows that agricultural green technology change is an effective means to promote the rural digital economy’s CEs reduction effect. This paper not only provide new empirical evidence for understanding nexus between digital economy and agricultural carbon reduction, but also give constructive policy implication to improve agricultural green development.
1 Introduction
Alleviating carbon emission is receiving more and more attention globally ( Ma S. et al., 2022 ). To maintain harmonious coexistence between humans and natures and realize the United Nations’ Sustainable Development Goals, Chinese central government pledged the global stakeholders that the Chinese people will try their best to have CEs peak before 2030 and achieve carbon neutrality before 2060, which demonstrates a strong determination to solve the problem of climate change. Activities of agricultural sector not only release CO 2 but also hold carbon sequestration function, and the CEs and sequestration function make agricultural production activities have function of maintaining the carbon balance in the atmospheric. However, agricultural CEs have obvious spatial heterogeneity ( Charkovska et al., 2019 ). Faced with issues such as global economic instability, rising energy demand, frequent adverse weather conditions, and expanding food demand ( Fahad et al., 2022 ), the Chinese government should attach importance to cutting agricultural CEs. China is a large and longstanding agricultural country with widespread and extensive agricultural production activities. In the traditional agricultural production mode, the overuse of pesticides and chemical fertilizers, land ploughing and irrigation, as well as the problems of low production efficiency and unreasonable resource allocation in the agricultural production process, will directly or indirectly lead to more agricultural CEs and their higher intensity, thereby seriously restricting the development of low-carbon and high-quality agriculture. The 14th Five-Year Plan for National Agricultural Green Development emphasizes building an agricultural industry system with characteristic of green, low-carbon, and circular, while the 2023 Government Work Report further emphasizes the need to continuously improve the ecological environment and achieve low-carbon and sustainable development.
The digital economy plays an important role in promoting the full and balanced development between urban and rural areas, and its development has driven the economic development of agricultural and rural areas ( Zhao et al., 2023 ). In China, the digital transformation of agriculture sector has shown initial results. According to the Information Center of the Ministry of Agriculture and Rural Affairs, the informatization level of national agricultural production in 2020 was 22.5% and the national level of agricultural product quality and safety traceability informatization was 22.1%. In 2021, the online retail sales agricultural production nationwide has reached 2.05 trillion Yuan, with growth rate of 11.3% compared to the level of the previous year. The construction of digital rural areas has been promoted extensively, with 117 digital rural pilot projects established nationwide, nine agricultural IoT demonstration provinces delineated, and 100 digital agriculture pilot projects established. Alongside these tremendous achievements, the digital economy has a positive impact on carbon emissions from agricultural production ( Zhao et al., 2023 ). Thus, the problem is how to realize the coordinated relationship between them. Would the rural digital economy development bring fresh momentum to reducing agricultural CEs? Meanwhile, how can the rural digital economy empower the reduction of agricultural CEs? Exploring these issues has important practical value for the development of the rural digital economy and improving the reduction of agricultural CEs while also contributing to policy enlightenment in terms of achieving the great mission of China’s “Carbon Peak and Carbon Neutrality”.
The main contribution of this paper comparing to the existing literature are as following. First, we use the extend STIRPAT model to explore the influence mechanism of agricultural digital economy on the agricultural carbon emission. Second, the agricultural green production efficiency is used as a proxy for agricultural green technology change, which not only considering the quantity of the agricultural green development, but also capture the quality of agricultural green development. Third, this paper use three dimensions to measure the agricultural digital economy. Digital infrastructure in rural areas, digitalization of agriculture, and rural digital finance).
The rest of this paper is organized as follows. Section 2 is the literature review. The theory base and research hypnosis are showed in Section 3 . Section 4 describes the models and data used in this paper. Section 5 analysis the estimation results. Section 6 gave the conclusion and presents the policy implications.
2 Literature review
So far, the relevant studies relating to CEs focus on the challenges faced by China in realizing its CEs reduction strategy and corresponding countermeasures. Hu (2021) , OuYang (2021) and others have analyzed the severe challenges faced by China in realizing the goals of dual carbon strategy in terms of international and domestic perspectives, respectively. Liu et al. (2021) and others have analyzed the problems that exist in China in the context of carbon neutrality from on the viewpoint of energy structure, and have put forward countermeasures such as energy conservation and efficiency improvement, while accelerating the transformation and further promotion of energy structures. Adopting another approach, some scholars have conducted empirical analysis on the CEs reduction effect of the carbon trading pilot policy implemented by the Chinese government through the synthetic control method ( Li et al., 2021 ; Yang et al., 2021 ), and have argued that China’s carbon trading pilot policy has played a significant role in the reduction of CEs, but there are problems such as insufficient market driving force for low-carbon innovation, poor pilot policy incentives, and regional heterogeneity. At the same time, Chen et al. (2016) have emphasized that increasing CEs reduces green total factor productivity (GTFP) based on studying the relationship between CEs and GTFP and economic development, and Wang et al. (2019) have also reached the same conclusion in relation to GTFP in agriculture economy development.
In addition, many researchers have devoted attention to agricultural CEs and carried out relevant research on the characteristics and calculation of agricultural CEs, agricultural CEs reduction policies, and influencing factors. Jin and other authors (2021) have explored the structural characteristics of China’s agricultural CEs, and drawn the conclusion that agricultural CEs in China have a phased upward trend alongside regional and provincial heterogeneity. In terms of policy research, Zhang et al. (2001) compared different environmental and economic instruments and argued that the environmental tax system has been more advantageous; Zheng et al. (2011) elaborated on a number of low-carbon special plans and proposed relevant recommendations, such as the establishment of a Chinese low-carbon agricultural model. Based on evolutionary game theory, Fan et al. (2011) suggested that government support and intervention can guide agricultural source farmers to choose CEs reduction strategies. In terms of influencing factors, the empirical studies of Xu et al. (2022a) and Xu et al. (2022b) have suggested that agricultural mechanization and the rural finance service have significant preventative effects on agricultural CEs. Furthermore, He et al. (2020) have discussed the status and role of green production efficiency in agriculture in various provinces.
The digital economy, a new engine of high-quality economic growth, has also attracted extensive attention and discussion in the academic community in recent years. On the one hand, there is research on the definition of the digital economy. Li et al. (2021a) characterize the digital economy on macro, meso- and micro-levels, asserting it includes four levels, namely, broad, middle, narrow and narrowest, and explored the mechanism and evolution process involved in data becoming a production factor ( Li et al., 2021b ). On the other hand, researches about digital economy are mainly about the comprehensive effect of digital economy, and they have put forward the argument that the digital economy can reduce environmental pollution ( Deng, 2022 ), while driving high-quality urban development and promoting a specific economic pattern, which aim to coordinate development between regions ( Zhao et al., 2020 ).
Especially since the strategy “Carbon Peak and carbon neutrality” was put forward, the relationship between the digital economy and carbon emission has become an important topic, and academia has also carried out extensive research ( Yu et al., 2022 ). While researchers hold different conclusion on the nexus between digital economy and carbon emissions. Most studies show that the digital economy has improved the environmental situation, and provided impetus for emission reduction, Wang (2022) point out the digital economy is helpful to reducing the carbon emissions. Zhang (2022a) find that the digital economy plays a significant role in carbon emission reduction. They all conduct their research based on China’s urban data. However, some studies hold that the digital economy has a heterogeneous influence on CEs. Some scholars ( Salahuddin et al., 2015 ; Avom et al., 2020 ) believe that, as the core foundation of the digital economy, the development of digital technology will lead to a large amount of power consumption and energy consumption, thereby increasing carbon emissions.
Furthermore, there are many researches focusing on the development of the digital economy in rural areas. According to theoretical analysis, the existing literature mainly pays attention to the mechanisms or development paths of the rural digital economy. Wang et al. (2021) , Yin and others (2020) and others have explored the significance, practice mode and mechanism of the digital economy development in agriculture production and rural regions, and believe that it should be promoted by, respectively, accelerating the construction of rural digital infrastructure, promoting agricultural digitalization, and developing rural e-commerce. Some researches on digital inclusive finance (DIF) have argued that DIF can push the regional convergence of green economic growth while less developed regions experience a more significant convergence effect ( Wang et al., 2022 ).
Many studies have also been carried out focusing on the influence of digital economy on CEs, mainly adopting the empirical analysis method with panel data based on province- or city-level contexts in China, and have found that digital economy growth can significantly alleviate the intensity of CEs ( Xu et al., 2022 ; Guo et al., 2023 ), however, there exist certain regional differences ( Miao et al., 2022 ; Xie, 2022 ).
A few researches have focused on the correlation between digital economy growth and agricultural CEs in China or foreign countries, and these literature mainly concentrate on the introduction of information and communications technology (ICT) into the field of smart agriculture, the promotion of sustainable agriculture, and the reduction of chemical use on the basis of embedding artificial intelligence ( Patrício and Rieder, 2018 ), sensors ( Basnet and Bang, 2018 ), robotics, and remote sensing technologies ( Huang et al., 2018 ) into agricultural modernization processes. ICT, as a main focus of advanced technology trends, can promote comprehensive productivity efficiency, total factor efficiency (TFP) and agricultural sustainability ( Dlodlo and Kalezhi, 2015 ). The prevalence of ICT not only promotes agricultural productivity and TFP, but also improves the progress of sustainable agricultural development. Ma S. Z. et al. (2022) focus on the nexus between the development of the agricultural digital economy and agricultural CEs; their conclusions emphasize that digital economy development inhibits agricultural CEs. In addition, advances in agricultural technologies, the optimization of agricultural industrial structure, and improvements in rural education all significantly inhibit the agricultural CEs in the research area. Adding to the influence factors outlined above, Zhang J. et al. (2022) emphasize that the development of DIF has significantly reduced agricultural CEs. Unlike other countries or regions, China’s agricultural digital economy mostly stresses the digital transformation of rural industrial models ( Wu, 2021 ), agricultural industries ( Zhao MJ. et al., 2022 ; Zhao YL. et al., 2022 ) and the effectiveness of the digital economy ( Xie, 2020 ). These studies all pay attention to the innovative developments in digital agriculture ( Wang et al., 2020 ). Through the systematic review of the literature outlined above, three main shortcomings can be found in the existing research: First, although many researchers have devoted attention to the correlation between the digital economy and CEs, more of them have studied this on urban level, and rarely extended this correlation to the rural development context, hence there is a lack of research that directly and empirically tests the correlations between the rural digital economy and agricultural CEs. Second, when analyzing heterogeneity, most existing studies only conduct sub-sample studies by region, and consider to a lesser extent the role of R&D in leading the high-quality development of the digital economy. Third, the path or mechanisms of the digital economy in rural areas in relation to the reduction of agricultural CEs is unclear, hence this requires further research. Considering the three points mentioned above, this article measures the intensity and amount of agricultural CEs, the progress in agricultural green technology and the development level of rural digital economy at a provincial level in China and tests empirically the nexus between rural digital economy and agricultural CEs. Meanwhile, this study not only examines the regional heterogeneity of the rural digital economy on agricultural CEs, it also analyzes the heterogeneity of this in relation to the science and technology investment level.
3 The mechanism and research hypotheses
The digital economy is an advanced economic mode with data as the important production factor and its development depends on the ability to obtain data. The establishment of a digital infrastructure not only realizes the utilization and transmission of data information, but also improves the efficiency of data circulation, thereby accelerating the process of digital infrastructure construction, the latter having become an indispensable foundation for the promotion of the growth of the digital economy. China has ascribed importance to the construction of digital infrastructure, and since 2018, the Politburo of the Central Committee has repeatedly stressed the need to accelerate the roll out and promotion of new digital infrastructure and its construction. At the same time, the construction of digital infrastructure is an important prerequisite for the integration of the digital and rural economies; whether it is agricultural informatization, agricultural product trading e-commerce, or the rural digital finance development, the prerequisite is it must be a complete rural digital infrastructure construction.
The reports of the China Academy of Information and Communications Technology believe that the definition of the digital economy can be divided into industrial digitization and digital industrialization, whereby industrial digitalization means the output and efficiency improvement brought about by the introduction of ICT into traditional industries. With the empowerment of digital technology, an environmental monitoring system for agricultural pre-production and production can be established, while new formats such as rural e-commerce goods can be formed after production, thereby realizing the transformation of traditional agriculture into a scientifically based and efficient modern model.
The integration of the digital and rural economies has improved the practice model of digital financial services in China’s “San Nong” field. The development of the digital economy has spawned updated financial models while the innovative development of digital finance has continuously added new momentum to the digital economy. The integration of ICT and traditional finance provides the possibility of opening up the farmers’ “last mile”. Furthermore, digital finance enables rural areas to address difficulties in accessing affordable financing at a low cost, fully leveraging the inclusive and the sharing advantages of digital finance, thereby contributing to the rural revitalization strategy while promoting the in-depth and comprehensive growth of the digital economy in today’s China.
Based on these insights, this article mainly explores the effect and mechanism of the rural digital economy growth level (explained from three aspects: rural digital infrastructure construction, agricultural digitalization, and development of the rural digital finance development) on agricultural CEs while also examining the intermediary effect of green technologies progress, which was measured by the agricultural green technological efficiency (see Figure 1 ).
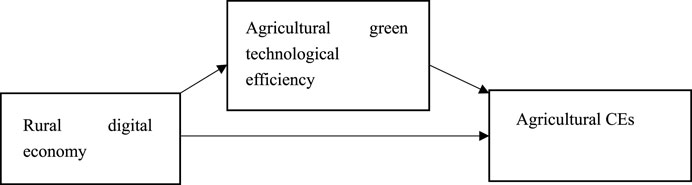
Figure 1 . Model of the impact of the rural digital economy on agricultural CEs.
3.1 Digital infrastructure in rural areas
Digital infrastructure as a foundation for the development of the digital economy plays an important role in realizing agricultural digitalization and rural digital finance. It contributes to promoting the deep development of the digital economy while limiting the digital economy’s CEs. The agricultural CEs reduction effect of rural digital infrastructure construction is mainly manifested in the following two aspects: First, rural digital infrastructure construction can guide residents in rural areas to form green environmental protection concepts. The development of ICT enables rural residents to accelerate their access to the online environment, understand news and public opinion related to environmental pollution, and develop green and environmental protection concepts, thereby promoting the formation of informal environmental regulations on the Internet ( Xu, 2014 ) while helping to alleviate agricultural CEs and reshaping patterns of rural environmental governance. Second, the establishment of perfect rural digital infrastructures can reduce the limitations of geographical space, promote information interconnections and sharing, and help achieve a rational allocation of resources, thereby reducing the energy consumption caused by spatial and time factors in production and life, improving energy efficiency while unleashing CEs reduction effects.
3.2 Digitalization of agriculture
In terms of agricultural production management, the technology of big data analysis can promote the establishment of large-scale and standardized agricultural production bases, realize scientific analysis and reasonable predictions of crop sowing, output and demand, while reducing the imbalance between supply and demand and the waste of resources caused by insufficient and asymmetric information. In addition, through modern information processing technologies such as remote sensing satellites, real-time data collection, monitoring and analysis of agricultural production can be realized, and a scientific environmental monitoring system can be established so as to improve the allocation efficiency of production factor, grasp changes in the ecological environment, accurately measure CEs and trace them in time, thereby promoting effective governance and green development.
Digital technology can also continuously enrich the marketing methods of agricultural products, forming new sales models, i.e., rural e-commerce and live streaming. The continuous popularization of the rural Internet has connected farmers to online consumption cyberspace, realized “point-to-point” transactions, and reduced resource waste and CEs caused by the problems of information asymmetry and high transaction costs in traditional agricultural sales models. In terms of logistics and distribution, low-carbon logistics has become an important future development direction. The Vision 2035 Plan points out that green and low-carbon development should be promoted in the transportation industry while low-carbon freight logistics should also be realized. Aim to achieve development of the low-carbon logistics, relying on digital technology, the logistics and distribution industry is gradually replacing traditional fuel vehicles with clean energy electric vehicles, and accelerating the application of drones in rural areas for logistics distribution to reduce CEs. Regarding the latter, Jingdong drones have been used in some rural areas of Suqian City, Jiangsu Province, and this has already achieved normalized delivery ( Lin et al., 2020 ). Relying on artificial intelligence technology can also promote the intelligence of agricultural product logistics systems, while the establishment of rural smart logistics information platform can optimize distribution routes, achieve resource intensification, continuously save costs, improve efficiency, and deepen the digital economy’s Carbon reduction effect.
3.3 Rural digital finance
The development of rural digital finance has promoted the establishment of rural environmental protection service platforms. Participation in environmental governance and other activities has effectively increased farmers’ enthusiasm for engaging in environmental protection and has helped to improve their sense of social responsibility ( Meng et al., 2022 ; Dong et al., 2023 ). Taking the “Ant Forest” in Alipay’s personal carbon account platform as an example, users collect online energy and plant virtual trees to achieve real afforestation projects in reality, which attracts lots of subscribers to participate in environmental protection actions. In addition, it not only provides a sense of gain for the masses, but also promotes agricultural green development and reduces CEs. Furthermore, the rural environmental protection service platform built by relying on the digital finance development can also analyze the information of platform users through big data technology while rationally allocating resources, thereby reducing agricultural CEs. For example, Alipay’s “garbage sorting and recycling platform” is specially set up for problems such as the low recycling rate of domestic waste, supporting door-to-door collection of waste items so that the resource recycling rate is improved. Digital finance promotes green growth and green technological significantly ( Wu et al., 2022 ; Razzaq and Yang, 2023 ). Mobile payment and online financial services can continuously reduce farmers’ dependence on financial institutions, not only reducing the transaction costs of paper money but also promoting the rational layout of financial business outlets, lowering resource consumption, while uniting both economic and environmental benefits.
In addition, digital finance can effectively compensate for the neglect of traditional finance in rural areas. In the traditional financial environment, farmers have difficulty in financing and own single source of funds, which is not conducive to introduce new agricultural technologies and form the extensive production methods, resulting in more agricultural CEs, hence more serious agricultural pollution problems. The promotion and application of digital finance has broadened the channels of farmers’ capital sources, assisted them to introduce efficient and low-carbon new agricultural technologies, and formed a green agricultural business model, thereby continuously reducing agricultural CEs’ intensity and promoting green agricultural development. Besides, digital finance can also alleviate the misallocation of financial resources and provide more career options for rural residents.
3.4 The progress of agricultural green technology
Generally speaking, a valuable way to achieve high-quality agricultural development is via green agricultural technological change ( Deng et al., 2022 ).
In the existing agricultural economics research, more studies focus on green technological change or environmental technological change using different methods to assess agricultural green technology’s efficiency or that of environmentally friendly technology’s efficiency. According to the existing study on agricultural green technology change (AGTC) of China, the improvement of China’s agricultural productivity is overestimated due to ignoring the influence of environmental factors. Considering the regional heterogeneity of environmental conditions, agricultural technological change in rural China shows an increase trend, while there is a descending trend in the eastern, western, and central regions respectively. The northeast region has experienced an obvious decline in levels of technological change, while technological change without environmental constraints has exhibited a descending trend from eastern to western China ( Jiang et al., 2022 ). He et al. (2021) have identified some important factors affecting agricultural green innovation efficiency, such as the level of agricultural technologies’ diffusion, absorption, implementation, and informatization, the amounts of agricultural extension workers, the average schooling of households, and levels of agricultural mechanization.
To estimate the green efficiency of agricultural production, Korhonen and Luptacik (2004) developed and extended the DEA considering environmental aspects. Existing literature usually through two ways to calculate the green efficiency, one is choosing the environmental factors as the inputs, the other is taking the environmental factors, especially the bad environmental results as bad outputs. The SBM-DEA taking account undesirable outputs is a widely used model to deal with economic and ecological issues ( Liu et al., 2022 ). In this paper, we also chose the SBM-DEA model to estimate the agricultural green production efficiency, taking the carbon emission as the bad output in the DEA model.
In view of the above analysis regarding how the rural digital economy influences agricultural CEs, this article puts forward two research hypothesizes.
Hypothesis 1:. The rural digital economy may reduce the level and intensity of agricultural CEs significantly.
Hypothesis 2:. The rural digital economy may reduce CEs through green technological innovation efficiencies.
4 Research design
4.1 constructing the modelling.
The STIRPAT (Stochastic Impacts by Regression on Population, Affluence, and Technology) model initially proposed by Dietz and Rosa (1994) explores the factors influencing atmospheric emissions, such as socioeconomic, demographic, and technological issues. In the existing literature, the STIRPAT model mainly has been introduced to explore the causes of CEs in different industries, countries or cross-government economic organizations. These researches have concluded that certain factors such as rising population and affluence levels, the growth of urbanization, the structure of economic development and energy consumption as well as the energy mix and related technological issues are all responsible for increasing emissions. The STIRPAT model is in introduced in our study and is extended from a base IPAT model, which was initially proposed by Ehrlich and Holdren (1971) . The advantage of this is that it allows for appropriate decomposition of population, technology, and wealth, while also adding other issues when analyzing environmental impact factors. The expression is:
where I i is the influence in observational unit i from population P , affluence A and technology T . μ i is the random error term, α、η、κ and φ are the parameters.
The fixed-effects model can be used to control regional invisible differentiation, so the endogeneity issue generated by invisible or unchanging is addressed ( Liu et al., 2024 ). Because of the advantages of fixed-effects, here we choose the fixed-effects model.
To effectively avoid the heteroscedasticity of the model, this article converts the terms in Equation 1 into their logarithms as follows:
where i indicates province; t indicates time; λ i indicates provincial fixed effects; and ε i t represents random error terms. β is the coefficient that this article focuses on, and it is expected to be negative.
A E i t stands for the agricultural CEs intensity of the i th province (city) in the t year; A D I G i t represents the comprehensive level of rural digital economy growth in the t year of i th province (city), which is the core explanatory variable of this paper. In York et al. (2003) , the STIRPAT model was introduced to interpret the technology term, which can be composed of more than one variable considering the needs of a given study. In the STIRPAT model, the estimated coefficients of core explanatory variables can be clarified as environmental effect elasticities, which means the percentage change of CEs for one percentage change in digital economy growth.
Thus in our paper we choose certain control variables, including urbanization rate ( U R B A N i t ), level of agricultural mechanization ( M E C H i t ), planting structure ( S T R U i t ), agrochemical input intensity ( C H E M i t ), traffic ( T R A N i t ), rural electricity use ( E L E C i t ) to represent the population, affluence and technology of a given rural area.
Digital agriculture is conducive to the green transformation of agricultural industry, meanwhile, the progress of green technologies can decrease the CEs level of agricultural production. Thus, the influence path of digital agricultural economy on CEs can be expressed as the following models, as shown in (3) to (5) .
Here, Eq. 5 is the total effect model, Eq. 4 is the estimated model of the agricultural digital economy on agricultural green production efficiency, and Eq. 3 is the estimated model that considers both the agricultural digital economy and the mediating mechanism. Where, the mediator variable is the variable GTFP, the green agricultural production efficiency. The coefficient ω 1 in the formula (5) reflects the overall effect of the digital economy on the agricultural CEs, the coefficient λ 2 represents the direct effect of digital economy on the agricultural CEs, and the magnitude of the mediating effect can be determined by ω 1 − λ 2 . If the coefficient ω 1 , λ 2 and ζ 1 are all significant, and λ 2 < ω 1 or the significance of λ 2 is lower than ω 1 , it can be inferred that the mediating effect exists.
4.2 Variable selection
1. Variable to be explained: Agricultural carbon intensity (AE). In this study, agricultural CEs intensity is chosen to measure the level of agricultural CEs in provinces. Agricultural CEs intensity is expressed by the ratio of total agricultural CEs to agricultural added value. The total amounts of agricultural CEs of each province were calculated from six dimensions: agricultural fertilizer, pesticide, farm PE film, agricultural diesel, tilling and irrigation ( Li et al., 2011 ).
The CEs estimation formula is:
where variable E is the total CEs generated by agriculture production. E i stands for the CEs amount of various carbon sources, T i is the amount of i th carbon source, and δ i is the CEs coefficient of i th carbon source. The CEs coefficients of different carbon sources are listed as follows: 0.896 kg kg -1 for agricultural fertilizers, 4.934 kg kg -1 for pesticides, 5.180 kg kg -1 for agricultural film, 0.593 kg kg -1 for agricultural diesel, and 312.600 kg km -2 for ploughing. Agricultural irrigation is 25 kg hm -2 ( Dubey and Lal, 2009 ). After calculating the total agricultural CEs of each province, divide by the agricultural added value of each province to get the agricultural CEs intensity of each province (kg/10,000 yuan). The average values of total agricultural CEs and agricultural CEs’ intensity from 2013–2020 in each province (municipality) are shown in Figure 2 . The top five average agricultural CEs are Henan, Shandong, Heilongjiang, Hebei and Anhui, mainly in the major agricultural provinces. Nearly half of whole country have agricultural carbon emissions exceeding five million tons. From the viewpoint of agricultural CEs’ intensity, the top five areas are Gansu, Jilin, Inner Mongolia, Shanxi and Xinjiang, which produce large volumes of CEs per 10,000 yuan of agricultural added value, all exceeding 180kg, on the one hand because they may be dominated by extensive agricultural production methods, while on the other hand it is also related to the less development level of the agricultural digital economy.
2. Core explanatory variable: Rural Digital Economy Development Index (ADIG). Based on the existing research, this paper selects 10 indicators such as rural Internet penetration rate and agricultural meteorological observation stations from the three aspects of rural digital economy infrastructure construction, agricultural digitalization, and rural digital services, and constructs an evaluation index system for the growth level of the digital economy in rural areas, as shown in Table 1 . The Internet penetration rate in rural areas is assessed using the proportion of rural Internet broadband access users to the rural population in an area, while the number of Taobao villages is taken from the Ali Research Institute’s China Taobao Village Research Report , 1 the DIF coverage breadth index is obtained from the digital inclusive financial index data of Peking University ( Guo et al., 2020 ) measured by account coverage status, including the number of Alipay accounts per 10,000 people, the ratio of Alipay card users, and the average amounts of bank cards bound to an Alipay account. Other metric data is available directly. Among these, the average population served by postal outlets is a negative indicator while the others are positive indicators. In this research, the entropy method is introduced to measure 10 indicators of rural digital economy growth at three dimensions in order to get the rural digital economy development index of each province (city).
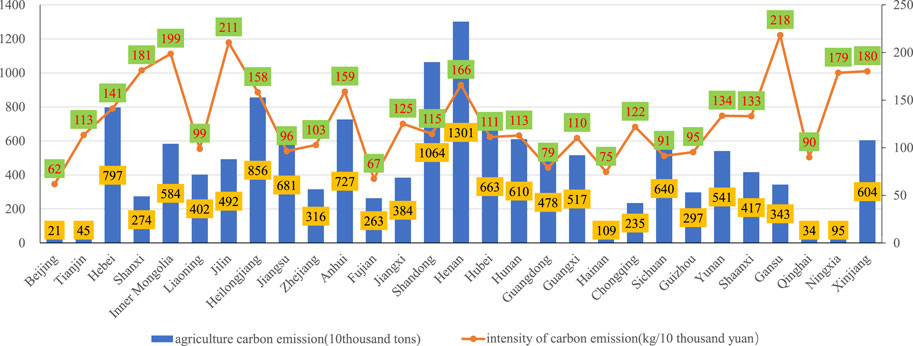
Figure 2 . Average level of total agricultural CEs amounts and intensity in each province (city), 2013–2020.
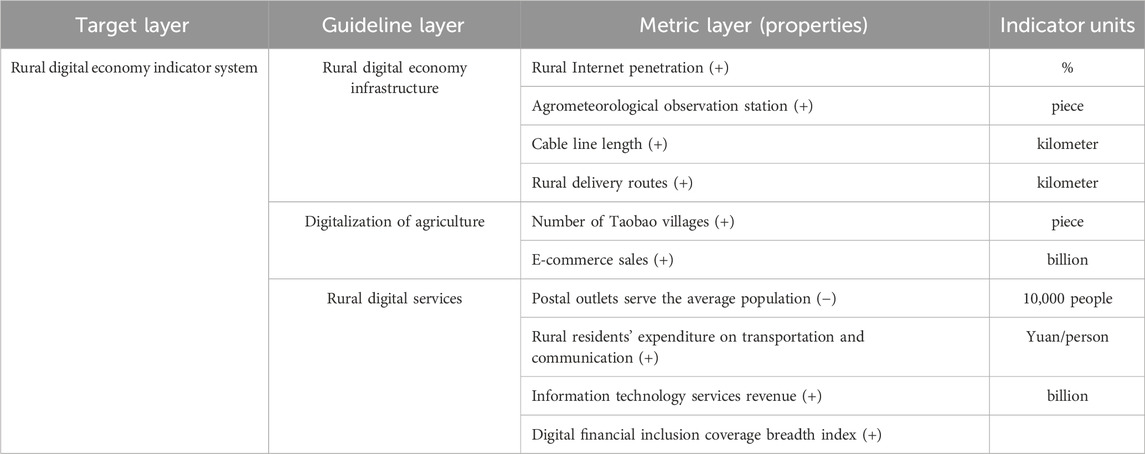
Table 1 . Evaluation index system of rural digital economy development.
The growth level of the rural digital economy in every province (city) in 2013 and 2020 are shown in Figure 3 . It is found that there is significant heterogeneity in the growth level of the rural digital economy between different regions and different years.
3. Mediated variables: Green efficiency agricultural development (GE). In the existing literature, the total factor productivity (TFP) calculated by DEA-Malmquist index is always used to measure the technological change, while using the Malmquist index will sacrifice time information. Thus, this paper uses agricultural green technological efficiency with environmental constraints. In the DEA model of this paper, agricultural added value was defined as the good output, agricultural CEs constitute the bad output, meanwhile the sown area of crops, fixed capital investment and the agricultural workers were set as the input variables.
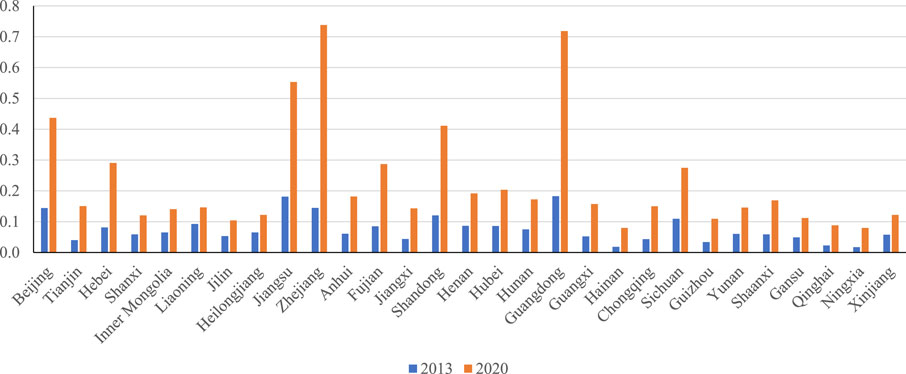
Figure 3 . Comparison of comprehensive scores of rural digital economy development in 29 provinces (municipalities and districts) in China, 2013–2020.
From Figure 4 , it is obvious that the green agricultural technological efficiency of less than half province is more than 1, which means that more than half of provinces have less efficient green agricultural technologies. Thus, for China, there is still more space to improve the green technologies. In this paper, we use GE to stand for green technological efficiency.
4. Control variables. Due to the complexity of factors influencing the agricultural carbon emission, considering only the impact of the agricultural digital economy on agricultural CEs might lead to bias, and even serious endogeneity issues. Therefore, the following variables are selected to ensure the comprehensiveness and accuracy of empirical analysis. Is complexity and variables: 1) Urbanization rate (URBAN), measured by the proportion of urban population in a region to total population in the same area; 2) The level of agricultural mechanization (MECH), expressed as the total power of agricultural machinery; 3) Planting structure (STRU), expressed as the ratio of the grain sown area to the crop sown area; 4) Agricultural chemical input intensity (CHEM), expressed as the ratio of fertilizer use to the crop sown area; 5) Traffic conditions (TRAN), expressed as the sum of railway operating mileage and highway mileage; 6) Rural electricity consumption (ELEC), expressed in terms of agricultural power generation. The above variables are logarithmic.
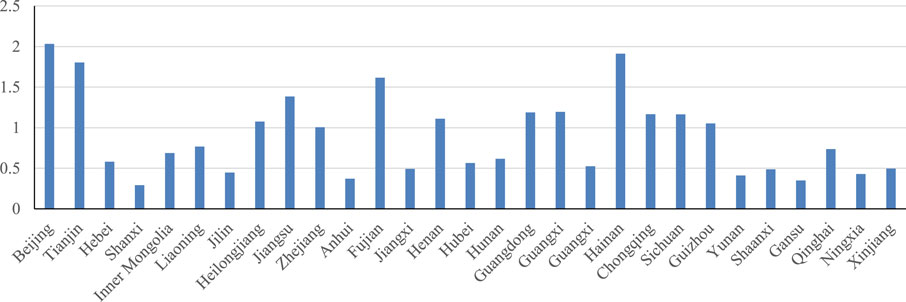
Figure 4 . Average green agricultural technological efficiency of 29 provinces, 2013–2020.
Considering the availability of data, the Institute uses all data for 29 provinces (cities) in China from 2013–2020 (excluding Shanghai, Tibet, Taiwan, Hong Kong and Macao), which are derived from the China Statistical Yearbook (2014–2021) 2 and China Rural Statistical Yearbook (2014–2021), the EPS data platform, the Ali Research Institute Report, and the Peking University Digital Inclusive Finance Index (2011–2020). The descriptive results for all variables chosen are shown in Table 2 .
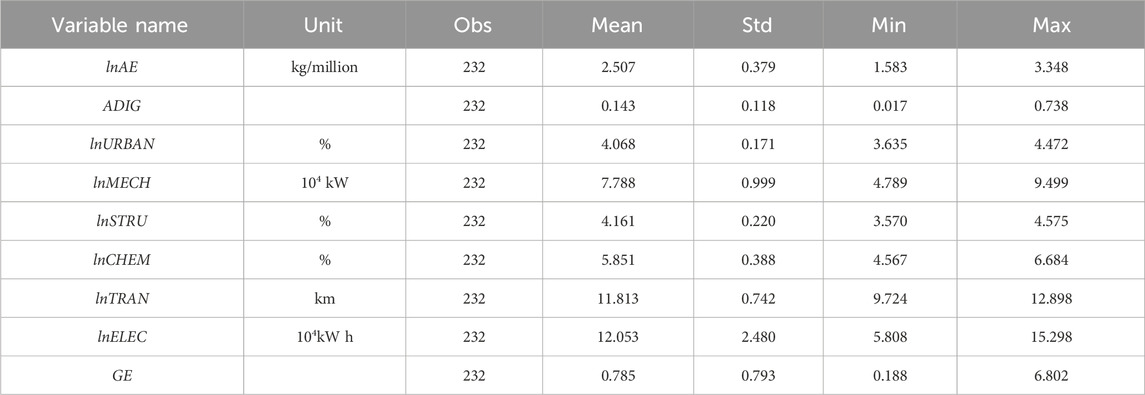
Table 2 . Description of main variables and descriptive statistical analysis.
As shown in Table 2 , except for lnELEC , all other variables have very small fluctuation trends, namely, less than 1.
5 Empirical results and analysis
5.1 estimates of basic regression model.
Firstly, only the core explanatory variable, namely, rural digital economy development composite score (ADIG) is considered, while the mixed-, fixed- and random-effects model is selected, and the F-test is 25.04 and the p -value is 0.0000, and the fixed-effect model should be selected. The Hausmann test shows that χ 2 is 4.77 and the p -value is 0.029, choosing a fixed-effect model. The other control variables were then added, and mixed-, fixed-, and random-effects models were selected, and the F-test was 42.79 and the p -value was 0.0000, and the fixed-effect model should be selected. The Hausmann test showed that χ 2 was 17.29 and the p -value was 0.0156, choosing a fixed-effect model.
Table 3 reports the baseline estimation of the influence effect of the rural digital economy development on the intensity of agricultural CEs. 1) considers only the core explanatory variable, and finds that the rural digital economy growth significantly reduces agricultural CEs intensity at the 1% level. Adding control variables to column 2), it is found that for every 1 unit increase in the growth level of rural digital economy, agricultural CEs intensity decreases by 40.01%, and this negative impact is still significant at the 1% level, thus validating the research hypothesis. For one thing, the development of the rural digital economy accelerates rural residents’ access to the network environment, not only promoting information interconnection and sharing while realizing the rational allocation of resources, but also helps rural residents establish the concept of green consumption and to develop informal network environment regulations, thereby reducing agricultural CEs intensity. And for another, the close combination of digital technology and agriculture helps farmers to, respectively, grasp agricultural production data accurately, improve production efficiency, and effectively reduce agricultural pollution caused by waste of resources. In addition, in an environment marked by the continuous development of rural digital finance, rural residents can broaden financing channels, introduce efficient and low-carbon new agricultural technologies, form a green business model, and promote the transformation of traditional extensive agricultural production methods to intensive ones, thereby realizing the agricultural CEs reduction effect of the rural digital economy.
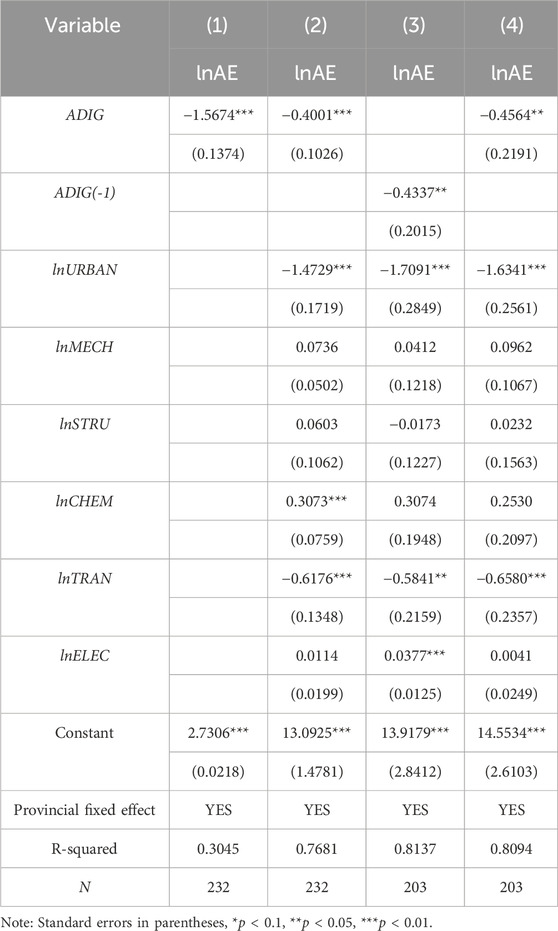
Table 3 . Baseline regression results.
5.2 Endogeneity test
To alleviate the impact of endogeneity on empirical results, this article also verifies the relationship between agricultural digital economy with a lag of one period and agricultural CEs, the results are in the column 3) in Table 3 . The results of Table 3 have verified the negative impact of agricultural digital economy on agricultural carbon emissions. If the digital economy is an endogenous variable, then the estimation results in this paper are biased. This paper will test the core explanatory variable and each control variable with a lag of one period to overcome the possible reverse causal relationship between contemporaneous variables. The corresponding empirical results are shown in column 4) of Table 3 . The regression results show that the coefficient of the core explanatory variable is −0.4564, with a p -value of 0.047, excluding the possibility that agricultural digital economy is an endogenous variable.
5.3 Robustness test
1. Replace the explanatory variable. In the baseline regression, the logarithmic form of agricultural CEs intensity was used as the explanatory variable. In order to further enhance the robustness of the conclusion, the dependent variable was replaced with the total amounts of agricultural CEs (logarithmic value) for robustness testing, and the results are shown in columns 1) and 2), Table 4 . With the variables to be replaced, the growth of the rural digital economy still has a significant negative impact on agricultural CEs.
2. Exclude part of sampling. Considering substantial heterogeneity in the levels digital economy growth among Chinese provinces, in order to further strength the robustness of the conclusions, the data of two provinces with a digital economy scale of more than 15 trillion yuan and 12 provinces (cities) with a digital economy scale of more than one trillion yuan of 2020 are excluded. The results in column 3) and column 4) of Table 4 show that the development of rural digital economy still has a significant negative impact on agricultural CEs, and this negative impact has become stronger, which may be due to the fact that the digital economy in these provinces is on the rise, with accelerated development speed and greater development potential, so it is easier to reduce agricultural CEs intensity.
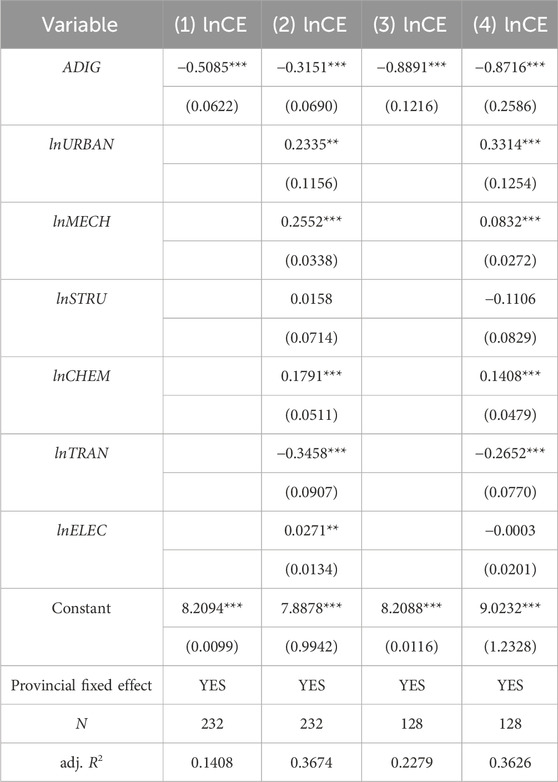
Table 4 . Robustness test results.
5.4 Heterogeneity analysis
1. Regional heterogeneity. This study categorizes the samples into four parts: eastern, central, western and northeastern regions for sub-sample regression, and discusses the regional heterogeneous impact of rural digital economy development on agricultural CEs intensity in the four parts. The estimations of regional heterogeneity analysis are shown in Table 5 ; for the eastern and central China, the development of rural digital economy still has a significant negative impact on agricultural CEs intensity and the central China have greater influence than their eastern counterparts while the western China is not significant. Possible explanations are: the eastern region has a good economic development foundation; the digital economy came early; it has a relatively complete rural digital economy infrastructure; and the integration and development of digital technology and agriculture is higher. Meanwhile, the central region is China’s most important agricultural production zone, the central government places greater focus on agricultural input, especially its green agricultural policy and finance support, which may lead to a larger and more significant negative impact on the intensity of agricultural CEs. The development and application of digital technology in the western region started late, that is might the reason why the impact is not significant. But it is not rational to deny its rapid upward phase and the low-carbon development potential of agriculture. The results also show that the coefficient of the rural digital economy development in the northeast region is positive, indicating that the development of the rural digital economy may increase the intensity of agricultural CEs. The development of the digital economy in northeast China is relatively backward, its digital infrastructure is not yet perfect, the coverage of rural digital finance is small, the proportion of secondary industry is large, while the integration of digital technology and agriculture is not complete.
2. Heterogeneity of scientific investment. As the primary productive and innovative force, the increased science and technology investment plays an important supporting role in the reduction of CEs and the growth of the digital economy. On the one hand, advances in science and technology have a direct impact on CEs’ reduction. At present, technological progress is an important driving force for the reduction of CEs and green development, while investment in science and technology helps to promote green technology innovations ( Yang et al., 2019 ; Gu et al., 2022 ), saving production costs, promoting the professional division of labor in various fields, and improving productivity, thereby directly reducing CEs. On the other hand, the progress of science and technology will also promote the progress of digital technologies such as AI and big data, accelerating the development process of industrial digitalization and digital industrialization, thereby promoting the high-quality development of the digital economy, thus further reducing CEs.
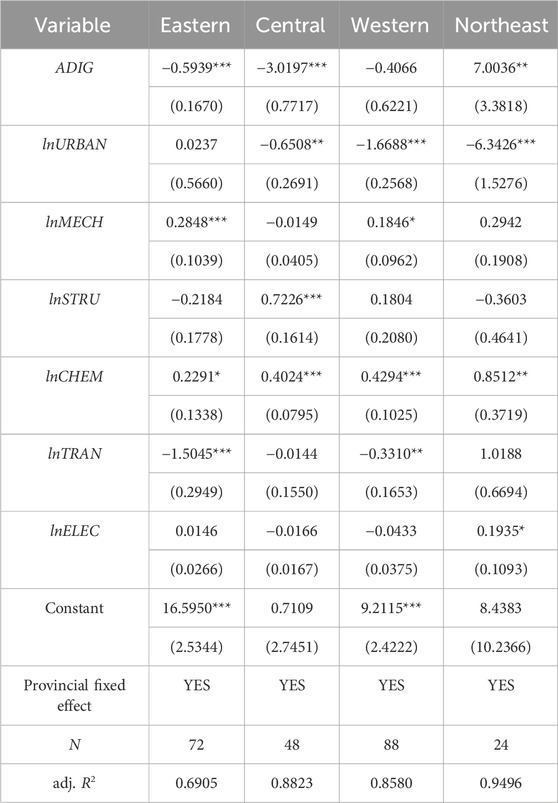
Table 5 . Results of regional heterogeneity analysis.
To examine the impact of rural digital economy development on agricultural CEs’ intensity against the background of different scientific and technological inputs, this paper divides 29 provinces (municipalities) into high and low sample groups for heterogeneity analysis based on the average science and technology expenditures in each province (municipality) over 2013–2020, and the results are shown in Table 6 . For the high-tech input group, the development of the rural digital economy still had a significant negative impact on the intensity of agricultural CEs, while the low-tech input group was not significantly negative. This shows that high scientific and technological investment can help promote the green development of agriculture while reducing the intensity of agricultural CEs. The development of the rural digital economy is premised on the completion and improvement of rural digital infrastructure as well as the production, transportation, sales of agricultural products, as well as the supervision, measurement, and traceability of CEs in the whole process of agricultural digitalization, which depends on sound digital infrastructure. High levels of investment in science and technology is conducive to promoting scientific and technological innovation and building a higher quality digital economy infrastructure, thereby providing the realization method and technical guarantee required for the close integration of digital technology and agriculture while promoting the reduction of agricultural CEs. At the same time, the continuous inflow of high-tech labor as a result of government investment in science and technology in the form of subsidies can enhance the level of local innovation, thereby promoting the sustainable and high-quality development of the digital economy and realizing the digital economy’s capacity to reduce CEs. Therefore, local governments should vigorously promote innovation-driven development strategies, increase financial support for science and technology, establish a sound incentive system, and encourage applied research and technological innovation in key fields. In addition, local governments can also increase the weight and proportion of indicators such as scientific and technological investment and their application in the government assessment index system, design a sound talent introduction system, and pay attention to cultivating high-quality talent ( Bian et al., 2020 ), so as to achieve high-quality development and deepen the digital economy’s CEs reduction effects.
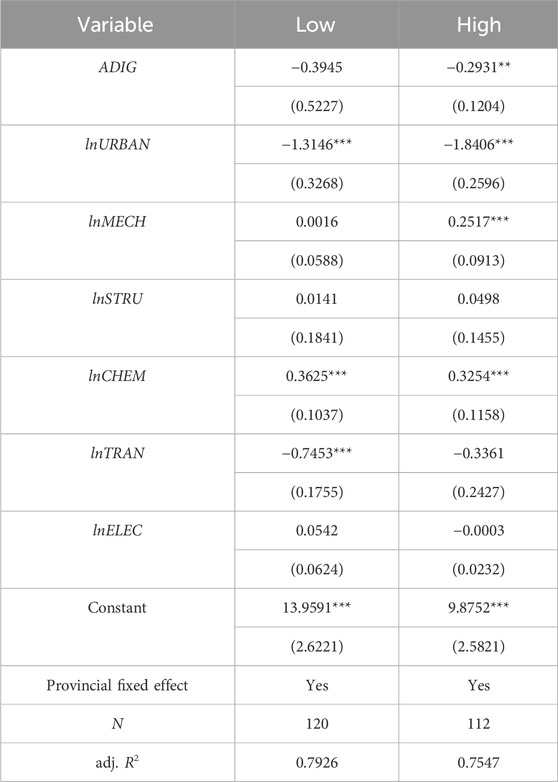
Table 6 . Analysis results of scientific and technological inputs’ heterogeneity.
5.5 Mediated effect analysis
From above analysis, it is obvious that the digital economy development has ability to decrease the agriculture CEs intensity and amounts. Further to explore the influence mechanism of the digital economy development on the agriculture CEs, the model 3) and model 4) mentioned in Section 4.1 is run using Stata software. To directly and conveniently compare the mediating effects with the estimates of the basic model of digital economy influence on agricultural CEs’ intensity, the baseline regression results in Table 3 were listed again in column 1), Table 7 . The dependent variable in column 2) is the mediator variable agriculture green efficiency (GE), while the explanatory variable focused on in this paper, agricultural digital economy (ADIG), is significantly positive, consistent with expectations. The dependent variable in column 3) is the agricultural CEs intensity (lnAE). After adding the mediating variable GE, the explanatory variable agricultural digital economy (ADIG) remained significantly negative at the 1% level, while the mediating variable agricultural green efficiency (GE) was significantly negative.
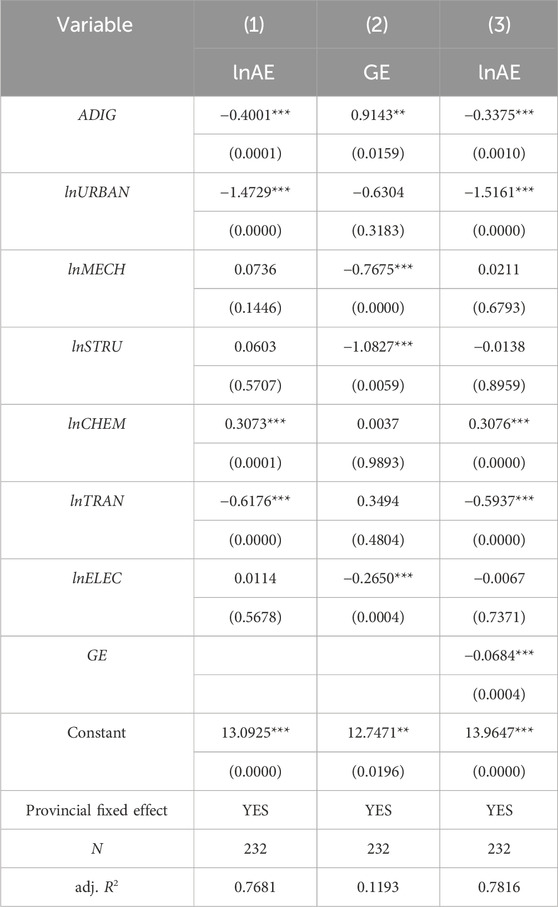
Table 7 . Analysis results of mediating effect.
Comparing the results of Table 3 and Table 7 , the coefficient β = 0.4001 with 1% significance, the coefficient λ 2 = -0.3375 is significant at 1% level, besides the coefficient ζ 1 = 0.9143 is significant at 5% level, the mediating effect is β − λ 2 = -0.0626, and the mediating effect of green agricultural technology exists through the empirically analysis. The coefficient −0.4001 show the total effect, and means when the agricultural digital economy increases one unit, the agricultural CEs will decrease 40.01%. The coefficient −0.3375 is the direct effect of agricultural digital economy with one unit increase on the agricultural CEs reduction is 33.75%. The gap between the total and direct effect is the mediating effect.
6 Discussion
6.1 the construction of agricultural digital economy indicators.
Based on the existing researches, this paper mainly focuses on the three aspects of rural digital economy infrastructure, digitalization of agriculture and rural digital services to construct the indicator of agricultural digital economy. This indicator not only consider the hardware and software agricultural digital economy level, but also digital service level. In Zhao et al. (2023) study, the indicators of digitalization level mainly focus on two aspects of digital economy infrastructure and digital economy service level, while they choose the digitization levels to substitute the rural digitalization index. In our study, we use the agricultural digital economy, which is closely related to the development agriculture and rural areas, and can better reflect the digitization level of agriculture.
6.2 The main effect of agricultural digital economy on agricultural carbon emission
In the existing studies, the level of digitalization can significantly reduce the agricultural carbon emission ( Zhao et al., 2023 ), although their research chose the carbon emission intensity of different agricultural sector, cropping and livestock sector respectively. Even in the city level or other sector of China, most studies also hold the same conclusion as our study, such as Wang et al. (2022) , Zhang W. et al. (2022) . And our study also support the carbon emission reduction effect of digital economy.
6.3 The mediating effect of agricultural digital economy on agricultural carbon emission intensity
Through the mediating effect analysis, it is obvious that the agricultural green production technology is an important mechanism for the development of the digital economy’s capacity to alleviate agricultural CEs. The same results are also evident in the research of Rong et al. (2023) . They emphasize that green technology can effectively suppress agricultural CEs directly, which has significantly negative spatial spillover effects on agricultural CEs in both the short and long term. Except for the influence mechanism, Guo et al. (2023) underline that the role of agricultural green technology in reducing agricultural CEs is particularly dominant in the main grain-producing areas. Zhao et al. (2023) emphasis digitalization can reduce China’s carbon intensity by promoting the agricultural technological input. This can support our influence mechanism of agricultural digital economy on the agricultural carbon emission. Except for the agricultural technology inputs, Zhao et al. (2023) also emphasis the role of human capital level and urbanization rate. In our research we use the agricultural green production efficiency as the mediating variable, which both considering the input and output of agricultural technology, and considering the agricultural green transformation.
6.4 Discussion of heterogeneity in the impact of agricultural digital economy on the agricultural carbon emissions
In Zhao et al. (2023) study, the carbon reduction effect is slightly greater in the central and western regions than that in the eastern regions, which is slightly different with our results, one reason is the different research period, the former chose the 2006–2018, while we chose the 2013–2020, considering the fact China’s digital economy has entered a mature period since the year 2013, thus we choose the 2013 is more rational for agricultural digital economy. Other reasons such as the region and province chosen difference also would lead to the less reduction effect of west region.
7 Conclusion and policy implications
This study uses the data of 29 provinces (cities) in China from 2013–2020 in order to measure the intensity of agricultural CEs as well as the development level of rural digital economy in each province. On this basis, the influence of the development of the rural digital economy on agricultural CEs is empirically estimated. The results show that: 1) the development of the rural digital economy could significantly reduce the intensity of agricultural CEs, a conclusion which is still valid after robustness test such as replacing the explanatory variables and removing some samples. The overall environmental effect is 40.01%, which means the agricultural CEs would decrease 40.01% when the agricultural digital economy increase one unit, the direct effect of digital economy on the agricultural CEs reduction is 33.75%; 2) The alleviation of CEs based on the rural digital economy is more significant in the higher technological investment zones than that in the lower technological investment zones, and the central and eastern regions also have more significant CEs reduction effect. 3) The influence mechanism analysis shows that agricultural green technology change is an effective means to promote the rural digital economy’s CEs reduction effect, and the mediating effect is −6.26%, which means the agricultural CEs would decrease 6.26% for one unit agricultural digital economy increase, through mediating effect of the agricultural green technology. Based on the above conclusions, this article puts forward the policy recommendations as follows.
Firstly, continuously improve the level of agricultural digital economy. Including build a complete rural digital economy infrastructure, strength the agricultural digitalization and promote the agricultural finance service. Further promote the full coverage of rural Internet, accelerate the construction of rural 5G networks, realize the in-depth application of agricultural Internet, and establish a smart agricultural technology system. Accelerate information interconnection and sharing, build a unified Big Data platform for agricultural and rural development, and provide solid information infrastructure support for the rural digital economy and agricultural digitalization, so as to accelerate the agricultural CEs reduction effect of the rural digital economy. Besides, increase the accessibility and coverage of agricultural finance is crucial for the green transformation of agricultural industry. The agricultural green development balances the agricultural industry growth and the sustainability of the rural environment.
Secondly, focus on achieving the balanced the rural digital economy development in various regions and better effect of agricultural CEs reduction. On the one hand, it is necessary to strengthen the interconnection and information sharing of various regions while deepening cooperation to promote the establishment of data sharing platforms. On the other hand, it is necessary to raise financial investment in the central, western and northeast regions, implement coordinated and sustainable digital economy development policies in accordance with local conditions, strive to eliminate the digital divide between regions, and bring into play the CEs reduction effect of digital economy. Meanwhile, the central China and western China can also take the initiative to expand foreign cooperation, such as introducing information technology to empower agriculture through free trade zone cooperation, thereby giving full scope to local comparative advantages, hence accelerating the digitization transformation of agriculture ( Guo, 2021 ) while realizing the coordinated the digital economy development between regions.
Thirdly, the government should pay attention to agricultural green development, because the agricultural carbon reduction effect of digital economy needs to be achieved through the mediating variable of agricultural green technology change. Considering the peculiarity of agricultural development, there is a need to increase financial support and incentives for science and technology, set up special funds to encourage agricultural green technology R&D and innovation levels, continuously strengthen the scientific and technological research and technology research capacity of low-carbon technologies, while promoting agriculture’s turn to low-carbon and green development.
8 Limitations
This paper has some shortcomings and can be further analyzed. The assessment of agricultural digital economy has consistently constituted an important issue and challenge in related research. Although this paper assesses the agricultural digital economy by establishing a novel evaluation framework, because of the availability and measurability of data, some regions and some indicators cannot be included in the evaluation system in this paper. Thus, there is still space to further improve the evaluation methodology in the future, to enhance the comprehensiveness and scientific rigor of the research. Furthermore, since the agricultural digitalization and CEs are highly influence by the grassroots government, the role of township-level government played in the agricultural green development and agricultural digital economy is very direct and important. While the related data on the grassroots government is relatively incomplete, which would not provide sufficient evidence for our study. If we would get enough data of township level government, we would conduct more comprehensive research in this area.
Data availability statement
The raw data supporting the conclusions of this article will be made available by the authors, without undue reservation.
Author contributions
HZ: Writing–original draft, Conceptualization, Funding acquisition, Investigation, Resources. KG: Conceptualization, Data curation, Formal Analysis, Methodology, Writing–original draft, Resources. ZL: Conceptualization, Funding acquisition, Investigation, Writing–original draft, Data curation, Formal Analysis, Methodology, Validation. ZJ: Data curation, Formal Analysis, Methodology, Project administration, Resources, Visualization, Writing–original draft. JY: Data curation, Formal Analysis, Software, Writing–review and editing.
The author(s) declare that financial support was received for the research, authorship, and/or publication of this article. This work was supported by Guizhou Planning Office of Philosophy and Social Science grant numbers 22GZQN28.
Conflict of interest
The authors declare that the research was conducted in the absence of any commercial or financial relationships that could be construed as a potential conflict of interest.
Publisher’s note
All claims expressed in this article are solely those of the authors and do not necessarily represent those of their affiliated organizations, or those of the publisher, the editors and the reviewers. Any product that may be evaluated in this article, or claim that may be made by its manufacturer, is not guaranteed or endorsed by the publisher.
1 Taobao Village: The Alibaba Research Institute’s recognition criteria for “Taobao Village” mainly includes: 1) business premises (in rural areas, administrative villages are the unit); 2) sales scale (the annual sales volume of e-commerce reaches 10 million yuan); 3) scale of online merchants (the number of active online stores in this village reaches 100, or the number of active online stores reaches 10% of the local household size).
2 The China Statistical Yearbook (2014) shows the development of whole economic and social status in the year 2013, the rest can be done in the same manner.
Avom, D., Nkengfack, H., Fotio, H. K., and Totouom, A. (2020). ICT and environmental quality in Sub-Saharan Africa: effects and transmission channels. Technol. Forecast. Soc. Change 155, 120028. doi:10.1016/j.techfore.2020.120028
CrossRef Full Text | Google Scholar
Basnet, B., and Bang, J. (2018). The state-of-the-art of knowledge-intensive agriculture: a review on applied sensing systems and data analytics. J. Sensors 2018, 1–13. doi:10.1155/2018/3528296
Bian, Y., Wu, L., and Bai, J. (2020). Does the competition of fiscal S&T expenditure improve the regional innovation performance? ——based on the perspective of R&D factor flow. Public Finance Res. (1), 45–58. [In Chinese]. doi:10.19477/j.cnki.11-1077/f.2020.01.004
Charkovska, N., Horabik-Pyzel, J., Bun, R., Danylo, O., Nahorski, Z., Jonas, M., et al. (2019). High-resolution spatial distribution and associated uncertainties of greenhouse gas emissions from the agricultural sector. Mitig. Adapt. Strategies Glob. Change 24 (6), 881–905. doi:10.1007/s11027-017-9779-3
Chen, C. (2016). China’s industrial green total factor productivity and its determinants—an empirical study based on ML index and dynamic panel data model. Stat. Res. 33 (3), 53–62. [in Chinese]. doi:10.19343/j.cnki.11-1302/c.2016.03.007
Deng, R., and Zhang, A. (2022). Research on the impact of urban digital economy development on environmental pollution and its mechanism. South China J. Econ. (2), 18–37. [In Chinese]. doi:10.19592/j.cnki.scje.390724
Deng, Y., Cui, Y., Khan, S. U., Zhao, M., and Lu, Q. (2022). The spatiotemporal dynamic and spatial spillover effect of agricultural green technological progress in China. Environ. Sci. Pollut. Res. 29 (19), 27909–27923. doi:10.1007/s11356-021-18424-z
Dietz, T., and Rosa, E. A. (1994). Rethinking the environmental impacts of population, affluence and technology. Hum. Ecol. Rev. 1 (2), 277–300.
Google Scholar
Dlodlo, N., and Kalezhi, J. (2015). “The internet of things in agriculture for sustainable rural development,” in 2015 International Conference on Emerging Trends in Networks and Computer Communications (ETNCC) , Windhoek, Namibia , 17-20 May 2015 , 13–18.
Dong, H., Zhang, Y., and Chen, T. (2023). A study on farmers' participation in environmental protection in the context of rural revitalization: the moderating role of policy environment. Int. J. Environ. Res. public health 20 (3), 1768. doi:10.3390/ijerph20031768
PubMed Abstract | CrossRef Full Text | Google Scholar
Dubey, A., and Lal, R. (2009). Carbon footprint and sustainability of agricultural production systems in Punjab, India, and Ohio, USA. J. Crop Improv. 23, 332–350. doi:10.1080/15427520902969906
Ehrlich, P. R., and Holdren, J. P. (1971). Impact of population growth. Science 171 (3977), 1212–1217. doi:10.1126/science.171.3977.1212
Fahad, S., Bai, D., Liu, L., and Baloch, Z. A. (2022). Heterogeneous impacts of environmental regulation on foreign direct investment: do environmental regulation affect FDI decisions? Environ. Sci. Pollut. Res. 29 (4), 5092–5104. doi:10.1007/s11356-021-15277-4
Fan, D., and Liao, J. (2011). Evolutionary game analysis of agricultural carbon emission reduction. Statistics Decis. 4 (1), 40–42. [In Chinese]. doi:10.13546/j.cnki.tjyjc.2011.01.013
Gu, H., Yang, W., and Chen, W. (2022). Effect of green technology innovation on urban carbon emission reduction. Acad. Explor. (3), 120–132. [In Chinese]. doi:10.3969/j.issn.1006-723X.2022.03.014
Guo, F., Wang, J., Wang, F., Kong, T., Zhang, X., and Cheng, Z. (2020). Measuring China’s digital financial inclusion: index compilation and spatial characteristics. China Econ. Q. 19 (4), 1401–1418. [In Chinese]. doi:10.13821/j.cnki.ceq.2020.03.12
Guo, K. (2021). A new path for solving regional differences in digital economy development from the perspective of opening to the outside world—taking free trade area as an example. Serv. Sci. Manag. 10 (6), 151–156. [In Chinese]. doi:10.12677/ssem.2021.106021
Guo, Z., and Zhang, X. (2023). Carbon reduction effect of agricultural green production technology: a new evidence from China. Sci. Total Environ. 874, 162483. doi:10.1016/j.scitotenv.2023.162483
He, P., Zhang, J., He, K., and Chen, Z. (2020). Why there is a low-carbon efficiency illusion in agricultural production: evidence from Chinese provincial panel data in 1997-2016. J. Nat. Resour. 35 (9), 2205–2217. [In Chinese]. doi:10.31497/zrzyxb.20200913
He, W., Li, E., and Cui, Z. (2021). Evaluation and influence factor of green efficiency of China’s agricultural innovation from the perspective of technical transformation. Chin. Geogr. Sci. 31 (2), 313–328. doi:10.1007/s11769-021-1192-x
Hong, M., Tian, M., and Wang, J. (2023). The impact of digital economy on green development of agriculture and its spatial spillover effect. China Agric. Econ. Rev. 15 (4), 708–726. doi:10.1108/caer-01-2023-0004
Hu, A. (2021). China’s goal of achieving Carbon Peak by 2030 and its main approaches. J. Beijing Univ. Technol. Sci. Ed. 21 (3), 1–15. [in Chinese]. doi:10.12120/bjutskxb202103001
Huang, Y., Chen, Z.-x., Yu, T., Huang, X.-z., and Gu, X.-f. (2018). Agricultural remote sensing big data: management and applications. J. Integr. Agric. 17 (9), 1915–1931. doi:10.1016/s2095-3119(17)61859-8
Jiang, Q., Li, J., Si, H., and Su, Y. (2022). The impact of the digital economy on agricultural green development: evidence from China. Agriculture 12, 1107. doi:10.3390/agriculture12081107
Jin, S., Lin, Y., and Niu, K. (2021). Driving green transformation of agriculture with low carbon: characteristics of agricultural carbon emissions and its emission reduction path in China. Reform 5, 29–37. [in Chinese].
Korhonen, P. J., and Luptacik, M. (2004). Eco-efficiency analysis of power plants: an extension of data envelopment analysis. Eur. J. Operational Res. 154 (2), 437–446. doi:10.1016/s0377-2217(03)00180-2
Li, B., Zhang, J., and Li, H. (2011). Research on spatial-temporal characteristics and affecting factors decomposition of agricultural carbon emission in China. China Popul. Resour. Environ. 21 (8), 80–86. [In Chinese]. doi:10.3969/j.issn.1002-2104.2011.08.013
Li, H., and Zhang, J. (2021a). Some understanding on definition of digital economy. Enterp. Econ. 40 (7), 13–22. [In Chinese]. doi:10.13529/j.cnki.enterprise.economy.2021.07.002
Li, H., and Zhao, L. (2021b). Data becomes a factor of production: characteristics, mechanisms, and the evolution of value form. Shanghai J. Econ. (8), 48–59. [In Chinese]. doi:10.19626/j.cnki.cn31-1163/f.2021.08.005
Li, Z., and Wang, J. (2021). Spatial emission reduction effects of China’s carbon emission trading: quasi-natural experiments and policy spillovers. China Population,Resources Environ. 31 (1), 26–36. [in Chinese]. doi:10.12062/cpre.20200907
Lin, Y., Lyu, J., and Jiang, Y. (2020). Research on optimization of drone delivery based on urban-rural transportation considering time-varying characteristics of traffic. Appl. Res. Comput. 37 (10), 2984–2989. [In Chineses]. doi:10.19734/j.issn.1001-3695.2019.07.0210
Liu, L., Zhang, L., Li, B., Wang, Y., and Wang, M. (2024). Can financial agglomeration curb carbon emissions reduction from agricultural sector in China? Analyzing the role of industrial structure and digital finance. J. Clean. Prod. 440, 140862. doi:10.1016/j.jclepro.2024.140862
Liu, S., Lei, P., Li, X., and Li, Y. (2022). A nonseparable undesirable output modified three-stage data envelopment analysis application for evaluation of agricultural green total factor productivity in China. Sci. Total Environ. 838, 155947. doi:10.1016/j.scitotenv.2022.155947
Liu, X., Cui, L., Li, B., and Du, X. (2021). Research on the high-quality development path of China’s Energy Industry under the target of Carbon Neutralization. J. Beijing Inst. Technol. Sci. Ed. 23 (3), 1–8. [in Chinese]. doi:10.15918/j.jbitss1009-3370.2021.7522
Ma, S., Li, J., and Wei, W. (2022a). The carbon emission reduction effect of digital agriculture in China. Environ. Sci. Pollut. Res . doi:10.1007/s11356-022-24404-8
Ma, S. Q., Dai, J., and Wen, H. D. (2019). Trade openness, environmental regulation and green technology progress: spatial Econometric analysis based on Provincial data in China. J. Int. Trade (10), 132–145. [In Chinese]. doi:10.13510/j.cnki.jit.2019.10.009
Ma, S. Z., He, G., and Guo, J. W. (2022b). Welfare effects of digital agriculture: deconstruction from the perspective of value re-creation and redistribution. Issues Agric. Econ. (5), 10–26. [In Chinese]. doi:10.13246/j.cnki.iae.2022.05.006
Meng, F., Chen, H., Yu, Z., Xiao, W., and Tan, Y. (2022). What drives farmers to participate in rural environmental governance? Evidence from villages in sandu town, eastern China. Sustainability 14, 3394. doi:10.3390/su14063394
Miao, L., Chen, J., Fan, T., and Lv, Y. (2022). The impact of Digital economy development on carbon emission: a panel data analysis of 278 prefecture-level cities. South China Finance 2, 45–57. [In Chinese]. doi:10.3969/j.issn.1007-9041.2022.02.004
Ouyang, Z., Shi, Z., Shi, M., Yang, D., Long, R., Zhou, H., et al. (2021). Challenges and countermeasures of “carbon peak and carbon neutrality”. J. Hebei Univ. Econ. Bus. 42 (5), 1–11. [In Chinese]. doi:10.14178/j.cnki.issn1007-2101.20210826.001
Patrício, D. I., and Rieder, R. (2018). Computer vision and artificial intelligence in precision agriculture for grain crops: a systematic review. Comput. Electron. Agric. 153, 69–81. doi:10.1016/j.compag.2018.08.001
Razzaq, A., and Yang, X. (2023). Digital finance and green growth in China: appraising inclusive digital finance using web crawler technology and big data. Technol. Forecast. Soc. Change 188, 122262. doi:10.1016/j.techfore.2022.122262
Rong, J., Hong, J., Guo, Q., Fang, Z., and Chen, S. (2023). Path mechanism and spatial spillover effect of green technology innovation on agricultural CO2 emission intensity: a case study in Jiangsu Province, China. Ecol. Indic. 157, 111147. doi:10.1016/j.ecolind.2023.111147
Salahuddin, M., and Alam, K. (2015). Internet usage, electricity consumption and economic growth in Australia: a time series evidence. Telematics Inf. 32 (4), 862–878. doi:10.1016/j.tele.2015.04.011
Wang, J., Luo, X., and Zhu, J. (2022). Does the digital economy contribute to carbon emissions reduction? A city-level spatial analysis in China. Chin. J. Popul. Resour. Environ. 20 (2), 105–114. doi:10.1016/j.cjpre.2022.06.001
Wang, L., Yao, H., and Niu, K. (2019). Carbon emission, green total factor productivity and agricultural economic Growth. Inq. into Econ. Issues (2), 142–149. [In Chinese].
Wang, S., Yu, N., and Fu, R. (2021). Digital rural construction: action mechanism, realistic challenge and implementation strategy. Reform 4, 45–59. [In Chinese].
Wang, X., Zhu, Y., Ren, X., and Gozgor, G. (2023). The impact of digital inclusive finance on the spatial convergence of the green total factor productivity in the Chinese cities. Appl. Econ. 55 (42), 4871–4889. doi:10.1080/00036846.2022.2131721
Wang, X. H., Zhao, B., and Wang, X. (2020). Research on digital agriculture model innovation based on the case of Net Ease Weiyang Pig. Issues Agric. Econ. (8), 115–130. [In Chinese]. doi:10.13246/j.cnki.iae.2020.08.009
Wu, M., Guo, J., Tian, H., and Hong, Y. (2022). Can digital finance promote peak carbon dioxide emissions? Evidence from China. Int. J. Environ. Res. public health 19, 14276. doi:10.3390/ijerph192114276
Wu, X. X. (2021). Research on the integration of digital economy and rural industry. Southwest Finance 10, 78–88. [In Chinese].
Xie, L. (2020). Rural digital inclusive finance innovation model analysis under the development of digital agriculture and rural areas. Agric. Econ. 11, 12–14. [In Chinese].
Xie, Y. (2022). The effect and mechanism of digital economy on regional carbon emission intensity. Contemp. Econ. Manag. 44 (2), 68–78. [In Chinese]. doi:10.13253/j.cnki.ddjjgl.2022.02.008
Xu, Q., and Zhang, G. (2022a). Spatial spillover effect of agricultural mechanization on agricultural carbon emission intensity: an empirical analysis of panel data from 282 cities. China Population,Resources Environ. 32 (4), 23–33. [In Chinese]. doi:10.12062/cpre.20220411
Xu, W., Mao, Y., and Qu, X. (2022b). Research on the impact of rural financial development on agricultural carbon emissions -- a case study of 17 provincial cities in Henan Province. Credit. Ref. 40 (7), 86–92. [In Chinese]. doi:10.3969/j.issn.1674-747X.2022.07.013
Xu, W., Zhou, J., and Liu, C. (2022). The impact of digital economy on urban carbon emissions: based on the analysis of spatial effects. Geogr. Res. 41 (1), 111–129. [In Chinese]. doi:10.11821/dlyj020210459
Xu, Y. (2014). Whether informal environmental regulation from social pressure constraints on China’s industrial pollution? Finance Trade Res. 25 (2), 7–15. [In Chinese]. doi:10.19337/j.cnki.34-1093/f.2014.02.002
Yang, L., Zhu, J., and Jia, Z. (2019). Influencing factors and current challenges of CO2 emission reduction in China: a perspective based on technological progress. Econ. Res. J. 54 (11), 118–132. [In Chinese].
Yang, X., Li, J., and Guo, X. (2021). The impact of carbon trading pilots on emission mitigation in China: empirical evidence from synthetic control method. J. Xi'an Jiaot. Univ. Sci. 41 (3), 93–104. [in Chinese]. doi:10.15896/j.xjtuskxb.202103010
Yin, H., Hou, P., and Wang, S. (2020). Agricultural and rural digital transformation: realistic representation, impact mechanism and promotion strategy. Reform 12, 48–56. [In Chinese].
York, R., Rosa, E. A., and Dietz, T. (2003). STIRPAT, IPAT and ImPACT: analytic tools for unpacking the driving forces of environmental impacts. Ecol. Econ. 46 (3), 351–365. doi:10.1016/s0921-8009(03)00188-5
Yu, Z. X., Liu, S., and Zhu, Z. C. (2022). Has the digital economy reduced carbon emissions? analysis based on panel data of 278 cities in China. Int. J. Environ. Res. public health 19 (18), 11814. doi:10.3390/ijerph191811814
Zhang, B. Y., Liu, J. Y., and Zhu, R. B. (2022a). Digital agriculture development: international experience, emission reduction effects and financial support: based on the case study on Chengdu. Southwest Finance (1), 28–39. [In Chinese].
Zhang, J., Lyu, Y., Li, Y., and Geng, Y. (2022b). Digital economy: an innovation driving factor for low-carbon development. Environ. Impact Assess. Rev. 96, 106821. doi:10.1016/j.eiar.2022.106821
Zhang, S., He, H., and Cao, J. (2001). Environmental policy innovation: discussion on implementing environmental tax in China. Acta Sci. Nat. Univ. Pekin. 37 (4), 550–556. [In Chinese]. doi:10.13209/j.0479-8023.2001.100
Zhang, W., Liu, X., Wang, D., and Zhou, J. (2022c). Digital economy and carbon emission performance: evidence at China's city level. Energy Policy 165, 112927. doi:10.1016/j.enpol.2022.112927
Zhao, L., Rao, X., and Lin, Q. (2023). Study of the impact of digitization on the carbon emission intensity of agricultural production in China. Sci. Total Environ. 903, 166544. doi:10.1016/j.scitotenv.2023.166544
Zhao, M. J., Shi, R., and Yao, L. Y. (2022a). Analysis on the goals and paths of carbon neutral agriculture in China. Issues Agric. Econ. 9, 1–11. [In Chinese]. doi:10.13246/j.cnki.iae.20220913.002
Zhao, T., Zhang, Z., and Liang, S. (2020). Digital economy, entrepreneurship, and high-quality economic development: empirical evidence from urban China. J. Manag. World 36 (10), 65–76. [in Chinese]. doi:10.19744/j.cnki.11-1235/f.2020.0154
Zhao, Y. L., Zhang, Z. W., Wei, L. H., and Luo, S. (2022b). Digital agriculture in the whole industry chain promotes industrial digital transformation and upgrading. Yunnan Agric. 3, 17–19. [in Chinese]. doi:10.3969/j.issn.1005-1627.2022.3.ynny202203007
Zheng, H., and Li, Y. (2011). The research of low carbon agriculture model. Issues Agric. Econ. Mon. 32 (6), 26–29. [In Chinese]. doi:10.13246/j.cnki.iae.2011.06.005
Keywords: agricultural carbon emissions, agricultural green technology efficiency, rural digital economy, rural digital finance, digitalization of agriculture
Citation: Zhang H, Guo K, Liu Z, Ji Z and Yu J (2024) How has the rural digital economy influenced agricultural carbon emissions? Agricultural green technology change as a mediated variable. Front. Environ. Sci. 12:1372500. doi: 10.3389/fenvs.2024.1372500
Received: 18 January 2024; Accepted: 20 March 2024; Published: 08 April 2024.
Reviewed by:
Copyright © 2024 Zhang, Guo, Liu, Ji and Yu. This is an open-access article distributed under the terms of the Creative Commons Attribution License (CC BY). The use, distribution or reproduction in other forums is permitted, provided the original author(s) and the copyright owner(s) are credited and that the original publication in this journal is cited, in accordance with accepted academic practice. No use, distribution or reproduction is permitted which does not comply with these terms.
*Correspondence: Jinna Yu, [email protected]
This article is part of the Research Topic
Low-Carbon Economy and Sustainable Development: Driving Force, Synergistic Mechanism, and Implementation Path
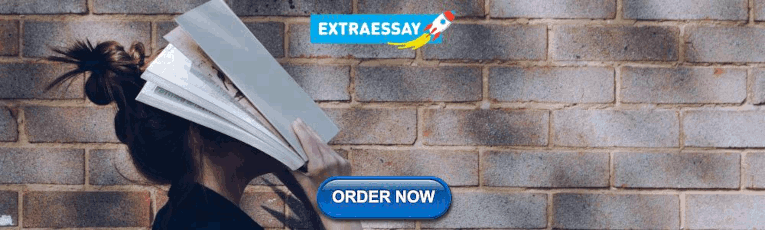
IMAGES
VIDEO
COMMENTS
Empirical research: Definition. Empirical research is defined as any research where conclusions of the study is strictly drawn from concretely empirical evidence, and therefore "verifiable" evidence. This empirical evidence can be gathered using quantitative market research and qualitative market research methods.
The result of empirical research using statistical hypothesis testing is never proof. It can only support a hypothesis, reject it, or do neither. These methods yield only probabilities. Among scientific researchers, empirical evidence (as distinct from empirical research) refers to objective evidence that appears the same regardless of the ...
Strategies for Empirical Research in Writing is a particularly accessible approach to both qualitative and quantitative empirical research methods, helping novices appreciate the value of empirical research in writing while easing their fears about the research process. This comprehensive book covers research methods ranging from traditional ...
II. Types and Methodologies of Empirical Research. Empirical research is done using either qualitative or quantitative methods. Qualitative research Qualitative research methods are utilized for gathering non-numerical data. It is used to determine the underlying reasons, views, or meanings from study participants or subjects.
Empirical research is characterized by several key features: Observation and Measurement: It involves the systematic observation or measurement of variables, events, or behaviors. Data Collection: Researchers collect data through various methods, such as surveys, experiments, observations, or interviews.
Empirical research methodologies can be described as quantitative, qualitative, or a mix of both (usually called mixed-methods). Ruane (2016) (UofM login required) gets at the basic differences in approach between quantitative and qualitative research: Quantitative research -- an approach to documenting reality that relies heavily on numbers both for the measurement of variables and for data ...
Disadvantages of Empirical Research. While empirical research has notable strengths, researchers must also be aware of its limitations when deciding on the right research method for their study.4 One significant drawback of empirical research is the risk of oversimplifying complex phenomena, especially when relying solely on quantitative methods.
Description of the methodology or research design used to study this population or phenomena, including selection criteria, controls, and testing instruments (such as surveys); Two basic research processes or methods in empirical research: quantitative methods and qualitative methods (see the rest of the guide for more about these methods).
The third development is in empirical methods: thanks to parallel advances in data science, network science, artificial intelligence and econometrics, researchers can study relationships, make ...
Hence, empirical research is a method of uncovering empirical evidence. Through the process of gathering valid empirical data, scientists from a variety of fields, ranging from the social to the natural sciences, have to carefully design their methods. This helps to ensure quality and accuracy of data collection and treatment.
Another hint: some scholarly journals use a specific layout, called the "IMRaD" format, to communicate empirical research findings. Such articles typically have 4 components: Introduction : sometimes called "literature review" -- what is currently known about the topic -- usually includes a theoretical framework and/or discussion of previous ...
Empirical research is based on observed and measured phenomena and derives knowledge from actual experience rather than from theory or belief. How do you know if a study is empirical? Read the subheadings within the article, book, or report and look for a description of the research "methodology."
The dividing line between empirical and nonempirical methods is marked by scholars' approach to knowledge gain (i.e., epistemology). Empirical methods typically involve systematic collection and analysis of data (i.e., observation and evidence). They are used primarily in quantitative research involving original collection of data, but also in ...
Empirical research is a research type where the aim of the study is based on finding concrete and provable evidence. The researcher using this method to draw conclusions can use both quantitative and qualitative methods. Different than theoretical research, empirical research uses scientific experimentation and investigation.
Empirical research is a type of research methodology that makes use of verifiable evidence in order to arrive at research outcomes. In other words, this type of research relies solely on evidence obtained through observation or scientific data collection methods. Empirical research can be carried out using qualitative or quantitative ...
The authors need to convince the reader that the methods used can provide an answer to the research question and that the reader can trust the results. What Criteria to Look For. Since the "Methods" section describes how the research is being conducted, it is probably the most important section for identifying empirical research.
Typically, empirical research embodies the following elements: A research question, which will determine research objectives. A particular and planned design for the research, which will depend on the question and which will find ways of answering it with appropriate use of resources. The gathering of primary data, which is then analysed.
Empirical evidence, information gathered directly or indirectly through observation or experimentation that may be used to confirm or disconfirm a scientific theory or to help justify, or establish as reasonable, a person's belief in a given proposition. ... It can be obtained by methods such as experiments, surveys, correlational research ...
Empirical research is a research method that investigators use to test knowledge claims and develop new knowledge.. Empirical methods focus on observation and experimentation.. Investigators observe and conduct experiments in systematic ways. is largely determined by their rhetorical contexts. Different workplace contexts and academic disciplines have developed unique tools and techniques for ...
The dividing line between empirical and non -empirical methods is marked by scholars' approach to knowledge. gain (i.e., epistemology). Empirical methods typically involve syste matic collection ...
Even though methodological studies can be conducted on qualitative or mixed methods research, this paper focuses on and draws examples exclusively from quantitative research. ... Previous attempts to catalogue all empirical studies of methods used in reviews were abandoned 10 years ago . In other databases, a variety of search terms may be ...
Empirical Research Methods. The two main methods used in empirical research are quantitative and qualitative. In quantitative research, numerical data is used to collect information or any other quantifiable process. The most common examples are polls, longitudinal studies and surveys.
The empirical method, central to scientific inquiry, relies on data collection and observation over theoretical speculation. It contrasts with experimental methods by focusing on natural data aggregation rather than controlled experiments, highlighting its roots in experiential learning and its significance in developing theories or conclusions.
The Rady Master of Quantitative Finance prepares you to analyze the large — and often multiple — real-time data sets that drive financial decision-making and risk management. Many of the empirical methods you'll use have emerged from UC San Diego's own world-renowned econometrics research. This emphasis on empirical data-driven methods ...
As green investment efficiency has gradually become a crucial factor affecting the sustainable development of energy enterprises, empirical research based on DEA theory has also been widely used ...
The above theoretical framework can be used to summarize the many research suggestions about the relationship between population growth and economic growth: there is a theoretical literature view in both pessimistic and optimistic views that supports population control policy, especially in developing countries, while optimistic views do not support population control policies, because ...
This paper not only provide new empirical evidence for understanding nexus between digital economy and agricultural carbon reduction, but also give constructive policy implication to improve agricultural green development. ... In this research, the entropy method is introduced to measure 10 indicators of rural digital economy growth at three ...
At the current stage, >100 cities in China are in the process of constructing "Zero-waste Cities" (ZCs). However, the absence of a unified quantitative evaluation method hinders the real-time display, assessment, and analysis of the progress and effectiveness of construction in various cities. Based on China's "Zero-waste City" (ZC) construction index system, a five-dimensional evaluation ...
In contrast, time-frequency processing methods based on empirical mode decomposition (EMD) and Hilbert-Huang transform (HHT) can adaptively decompose signals into independent components, overcoming the limitations of wavelet transform. ... Liu, H.; Qu, X. Research on indirect monitoring method of wave bending moment for large semi ...