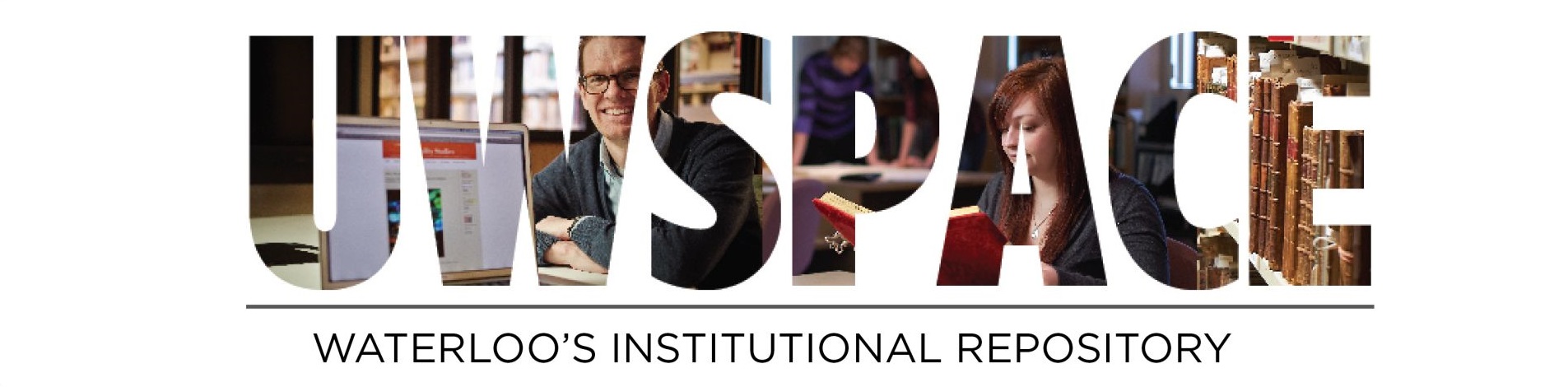
- UWSpace Home
- University of Waterloo
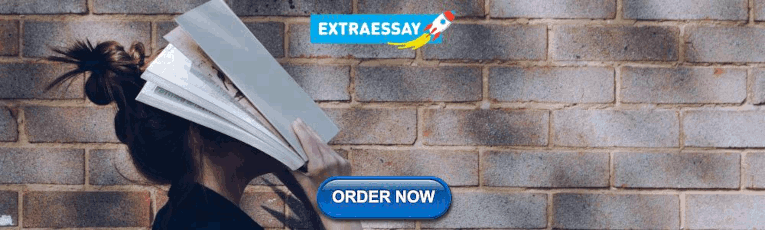
Investigating the current approach to developing data governance in the Canadian smart city

View Google statistics
Collections
- Geography and Environmental Management
Cite this version of the work
Other formats
Search UWSpace
Information.

- < Previous
Home > Graduate Theses, Dissertations, and Capstone Projects > All Graduate Theses, Dissertations, and Capstones > 1126
All Graduate Theses, Dissertations, and Other Capstone Projects
Proposed data governance framework for small and medium scale enterprises (smes).
Rejoice Okoro , Minnesota State University, Mankato
Data governance is not a one size fits all, instead, it should be an evolutionary process that can be started small and measurable along the way. This research aims at proposing a data governance framework by ensuring data management processes, data security and control are compliant with laws and policies. This article also presents the first results of a comparative analysis between three data privacy laws and outlines five components which together form a data governance framework for SMEs. The data governance model documents data quality roles and their type of interaction with data quality management activities exploring how data is perceived and applicable to SMEs providing best practices for proper data management which includes roles and responsibilities about the use of data for automated decision making, privacy, compliance to data laws, the intersection of data governance and data science in the digital era.
Salivia Guarionex
Committee Member
Veltsos Christophe
Kruse Sarah
Date of Degree
Document type.
Master of Science (MS)
Computer Information Science
Science, Engineering and Technology
Recommended Citation
Okoro, R. (2021). Proposed data governance framework for small and medium scale enterprises (SMEs) [Master’s thesis, Minnesota State University, Mankato]. Cornerstone: A Collection of Scholarly and Creative Works for Minnesota State University, Mankato. https://cornerstone.lib.mnsu.edu/etds/1126/
Creative Commons License

Since June 02, 2021
Included in
Databases and Information Systems Commons , Data Science Commons , Information Security Commons
Rights Statement
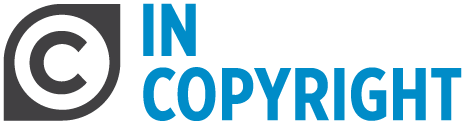
Advanced Search
- Notify me via email or RSS
Author Corner
- All Authors
- Submit Research
University Resources
- Digital Exhibits
- ARCH: University Archives Digital Collections
- Library Services
- Minnesota State University, Mankato
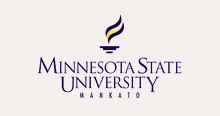
Home | About | FAQ | My Account | Accessibility Statement
Privacy Copyright
Designing data governance that delivers value
Executives in every industry know that data is important. Without it, there can be no digital transformation to propel the organization past competitors . There are no analytics driving new sources of revenue . Even running the basic business well isn’t possible. But for data to fuel these initiatives, it must be readily available, of high quality, and relevant. Good data governance ensures data has these attributes, which enable it to create value.
The problem is that most governance programs today are ineffective. The issue frequently starts at the top, with a C-suite that doesn’t recognize the value-creation potential in data governance. As a result, it becomes a set of policies and guidance relegated to a support function executed by IT and not widely followed—rendering the initiatives that data powers equally ineffective. In other cases, organizations try to use technology to solve the problem. While technology solutions such as data lakes and data-governance platforms can help, they aren’t a panacea.
Without quality-assuring governance, companies not only miss out on data-driven opportunities; they waste resources. Data processing and cleanup can consume more than half of an analytics team’s time, including that of highly paid data scientists, which limits scalability and frustrates employees. Indeed, the productivity of employees across the organization can suffer: respondents to our 2019 Global Data Transformation Survey reported that an average of 30 percent of their total enterprise time was spent on non-value-added tasks because of poor data quality and availability (Exhibit 1).
While it’s challenging to directly attribute value to data governance, there are multiple examples of its significant indirect value. Leading firms have eliminated millions of dollars in cost from their data ecosystems and enabled digital and analytics use cases worth millions or even billions of dollars. Data governance is one of the top three differences between firms that capture this value and firms that don’t. In addition, firms that have underinvested in governance have exposed their organizations to real regulatory risk , which can be costly.
Building the foundation for effective governance
While many organizations struggle to effectively scale data governance, some have excelled. For example, a leading global retailer, whose data governance was managed within IT, struggled to capture value from data for years. Then, as part of an enterprise-wide analytics transformation, it invested in educating and involving the entire senior-executive leadership team in data governance. It assigned to each executive leader (CFO, CMO, and so on) several data domains, or business-data subject areas, some of which, such as consumer transactions and employee data, spanned multiple functions or lines of business.
Once these leaders grasped the value of data governance, they became its champions. Within their domains, they selected representatives to act as data-domain owners and stewards and directly linked data-governance efforts to priority analytics use cases. They then worked in sprints to identify priority data based on the value they could deliver, checking in with the CEO and senior leadership team every few weeks. These efforts have begun to pay off, allowing the organization to stand up priority data domains over the course of a few months (versus years) and reduce the amount of time data scientists spend on data cleanup, accelerating analytics use-case delivery. The program continues to grow over time.
As the example demonstrates, effective data governance requires rethinking its organizational design. A typical governance structure includes three components:
- a central data management office (DMO), typically led by a chief data officer (CDO) , with a targeted data strategy and governance leaders who set the overall direction and standards
- governance roles organized by data domain where the day-to-day work is done
- a data council that brings domain leaders and the DMO together to connect the data strategy and priorities to the corporate strategy, approve funding, and address issues
This structure serves as the foundation for data governance, balancing central oversight, proper prioritization, and consistency while ensuring that the employees creating and using data are the ones leading its management (Exhibit 2).
Would you like to learn more about McKinsey Digital ?
Six ways to drive data-governance excellence.
The organizational foundation alone, however, is not enough. Six critical practices are needed to ensure data governance creates value.
1. Secure top management’s attention
As the aforementioned example highlights, success with data governance requires buy-in from business leadership. The first step is for the DMO to engage with the C-suite to understand their needs, highlight the current data challenges and limitations, and explain the role of data governance. The next step is to form a data-governance council within senior management (including, in some organizations, leaders from the C-suite itself), which will steer the governance strategy toward business needs and oversee and approve initiatives to drive improvement—for example, the appropriate design and deployment of an enterprise data lake—in concert with the DMO.
The DMO and the governance council should then work to define a set of data domains and select the business executives to lead them. These leaders drive governance efforts day-to-day by defining data elements and establishing quality standards. Companies need to invest the time to introduce these leaders to their new roles, which are typically added to their primary responsibilities. They should understand the value they will generate in these roles and be armed with the skills they need, including an understanding of the relevant regulations and core elements of the data architecture.
Critically, having top-down business-leadership buy-in will avoid the usual challenges around role clarity and empowerment. Data stewards on the business side will understand that the effort is an enterprise priority and make time to address it (which might be facilitated by a shift in their performance metrics or an adjustment in their other responsibilities). Top-down mandates also make it possible to immediately address conflicts over data ownership.
Leading organizations also create tangible ways to track progress and value creation. For example, they can measure the amount of time data scientists spend finding, curating, or enabling data for priority use cases, or the dollar losses associated with poor-quality data and associated business errors. Tracking impact metrics like these helps ensure the attention and continuing support of top management.
2. Integrate with primary transformation themes
To ensure that governance efforts create value, link them directly to continuing transformation efforts that already have CEO attention, such as digitization, omnichannel enablement, or enterprise-resource-planning modernization. These efforts typically depend on data availability and quality.
Linking governance to transformation themes simplifies senior leadership buy-in and changes the organizational construct. Rather than governance running on its own, such initiatives shift data responsibility and governance toward product teams, integrating it at the point of production and consumption.
For example, a European retailer embarked on a digital transformation of its core business and a rapid extension of its online business, which required significant redevelopment of the e-commerce stack, including back-end platforms. Data was identified as a critical enabler, and a DMO and a data council were set up to develop the core framing on the future ecosystem, as well as the structure of data domains, including the strategic goals on managing data in the future.
Lead product owners, who were heading several digital-transformation squads in dedicated functional areas, became data leaders within their area of responsibility. Product owners became data-domain owners. For example, the product owner working to drive process improvements around in-store checkout owned the sales and payment domains. This structure ensured that governance efforts were oriented primarily to enabling business needs and that the leaders creating and consuming data were actively shepherding it.
3. Prioritize data assets and focus data leadership accordingly
Many organizations approach data governance in a holistic manner, looking at all data assets at once. But such a large scope means slow relative progress in any given area and a risk that efforts aren’t linked directly to business needs. To succeed, data assets should be prioritized in two ways: by domains and by data within each domain.
The data council, supported by the DMO, should prioritize domains based on transformational efforts, regulatory requirements, and other inputs to create a road map for domain deployment. Then the organization should rapidly roll out priority domains, starting with two to three initially, and aim for each domain to be fully functional in several months.
For example, a North American retailer set a bold aspiration to transform the company over three years with advanced analytics. The company quickly realized that its current data would hold it back and established a DMO and data domains to scale governance. It identified ten domains across the enterprise and prioritized deployment of the first two—transactional data (logging in-store purchases) and product data (establishing a clear hierarchy of products and their details). This helped accelerate priority use cases around in-store assortment and inventory.
In addition to prioritizing domains, prioritize data assets within each domain by defining a level of criticality (and associated care) for each data element. Critical data typically represents no more than 10 to 20 percent of total data in most organizations. Critical elements, such as customer name or address, should receive a high level of care, including ongoing quality monitoring and clear tracking of flow across the organization, whereas for elements that are used less often in analytics, reporting, or business operations (such as a customer’s academic degree), ad hoc quality monitoring without tracking may suffice. This significantly narrows the scope of governance efforts and ensures that they are focused on the most important data.
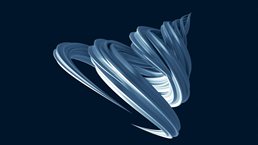
How chief data officers can navigate the COVID-19 response and beyond
4. apply the right level of governance.
Data-governance programs can vary dramatically across organizations and industries. Leading organizations take a “needs-based” approach, adopting the level of governance sophistication appropriate to their organization and then adjusting the level of rigor by data set.
It’s important to realize that data governance was largely first championed by banks under pressure from BCBS 239 1 Basel Committee on Banking Supervision’s standard number 239: “Principles for effective risk data aggregation and risk reporting.” and other regulations that required sophisticated governance models. Most other industries and organizations don’t face the same level of regulatory pressure, so the design of their programs should align with the level of regulation they uniquely face and the level of their data complexity. Organizations with multiple, distinct businesses spanning many geographies have more complex needs than those with a business in only one geography; similarly, a high pace of data change or low level of technology automation increases data complexity (Exhibit 3).
The most comprehensive governance model— say, for a global bank—will have a robust data-governance council (often with C-suite leaders involved) to drive it; a high degree of automation with metadata recorded in an enterprise dictionary or data catalog; data lineage tracked back to the source for many data elements; and a broader domain scope with ongoing prioritization as enterprise needs shift.
In contrast, targeted data governance for a regional technology company might have a data council that meets less frequently and includes C-suite leaders only periodically; metadata tracking that could even start in Excel; limited lineage tracking; and narrower domain scope, at least initially, to enable priority use cases.
In parallel with establishing the right level of governance for the organization as a whole, adjust the level of governance rigor across data sets. Many organizations’ legacy data standards set conservative restrictions on quality and access across the board. This minimizes risk but can stifle innovation. Leading organizations consciously balance opportunities and risks and differentiate governance by data set.
For example, organizations can apply light governance for data that is used only in an exploration setting and not beyond the boundaries of the science team. The team may also not need perfectly prepared and integrated data with full metadata available. Data masking may be appropriate to ensure privacy, together with strict internal non-disclosure agreements (NDAs). However, as soon as such data is used in a broader setting, such as in interactions with customers, stronger governance principles need to be applied.
An Asian financial institution took an aggressive approach to “free the data” using these principles. It agreed on the sensitivity level for each data set and was able to free the roughly 60 percent of enterprise data that was low risk, giving all employees access to use and explore it. On the other hand, highly sensitive data, such as personally identifiable information, was highly restricted both in terms of who could access it and how.
As organizations mature and their governance capabilities and technology continue to advance, scope becomes less important. A suite of tools is beginning to automate data-governance activities, and its coverage and cost-effectiveness will only improve over time. Both newer platforms, such as Octopai and erwin, and established organizations, such as Informatica and Collibra, are rolling out capabilities for automated metadata harvesting, lineage creation, data-quality management, and other governance functions.
5. Choose iterative and focused implementation
To ensure that data governance creates value fast, tailor governance priorities to the domain, and use iteration to adapt quickly. This goes beyond integrating governance with business needs, prioritizing use cases and domains, and applying needs-based governance; the key is to adopt iterative principles in day-to-day governance. For example, if there is a backlog of known data-quality issues, review and reprioritize daily, working to maximize the benefit to the business as priorities shift.
Push to enable priority use cases quickly even if the solution isn’t perfect. Longer-term development to make use cases production ready (by integrating with the core customer-relationship-management and operational customer master data) can occur once value has been demonstrated. For example, enhancing customer campaigns may not require a fully integrated set of data across the entire enterprise, but rather a tailored approach in a dedicated platform. Data governance should support and accelerate this tailored approach, focusing on solving issues around availability and quality in addition to establishing strong master-data management.
6. Generate excitement for data
When people are excited and committed to the vision of data enablement , they’re more likely to help ensure that data is high quality and safe. Leading organizations invest in change management to build data supporters and convert the skeptics. This can be the most difficult part of the program, as it requires motivating employees to use data and encouraging producers to share it (and ideally improve its quality at the source).
Successful organizations use a combination of interventions to drive the right behavior. These can include role modeling from the CEO and other senior leaders, recognition for high quality, responsive sources, and new demonstrated-use cases. Some organizations also offer training and qualifications, often as part of a larger academy approach , together with communicating about career opportunities in data jobs. Others have used successes in data and analytics to create excitement in the form of events, publications, or even data art. 2 TED compiled a series of talks on data art: ted.com/playlists/201/art_from_data. What works is highly dependent on the culture of the organization.
Getting started
Companies should begin their new data-governance approach by asking these six questions:
- What is the opportunity cost of not getting data governance right in terms of missed upside, extensive time lost in manually cleaning data, or incorrect and suboptimal business decisions?
- Who is leading governance efforts today, and what would it look like to elevate the conversation to the C-suite? Who should be involved?
- Where is governance most important? What domains and parts of domains does the organization most need right now?
- What governance archetype best fits the organization, and are current efforts aligned to that level of need?
- How can governance be accelerated by adjusting its focus and injecting iterative working concepts?
- Do you have the in-house capabilities to manage such a shift?
Data governance is critical to capturing value through analytics, digital, and other transformative opportunities. While many companies struggle to get it right, every company can succeed by shifting its mindset from thinking of data governance as frameworks and policies to embedding it strategically into the way the organization works every day.
Bryan Petzold is an associate partner in McKinsey’s Silicon Valley office, Matthias Roggendorf is a partner in the Berlin office, Kayvaun Rowshankish is a partner in the New York office, and Christoph Sporleder is a partner in the Frankfurt office.
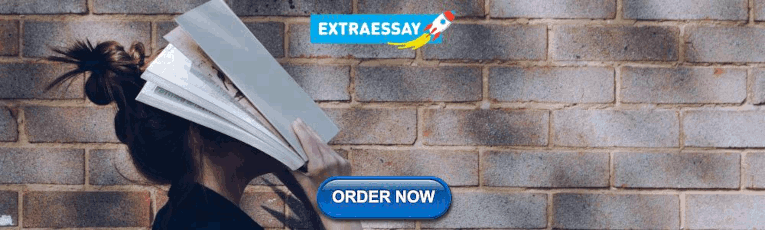
Explore a career with us
Related articles.
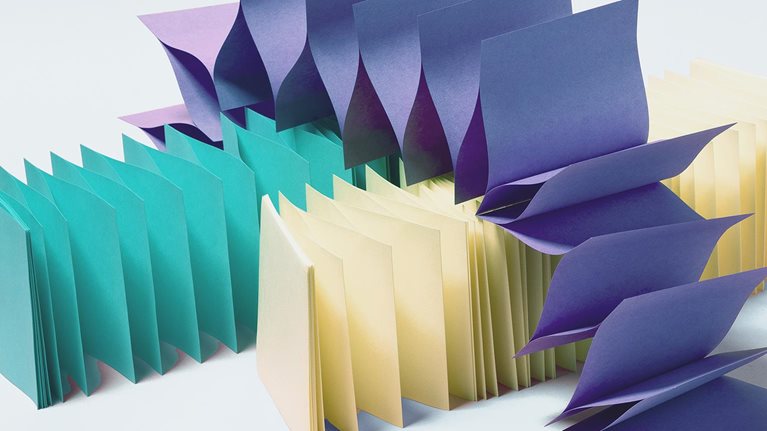
How to build a data architecture to drive innovation—today and tomorrow
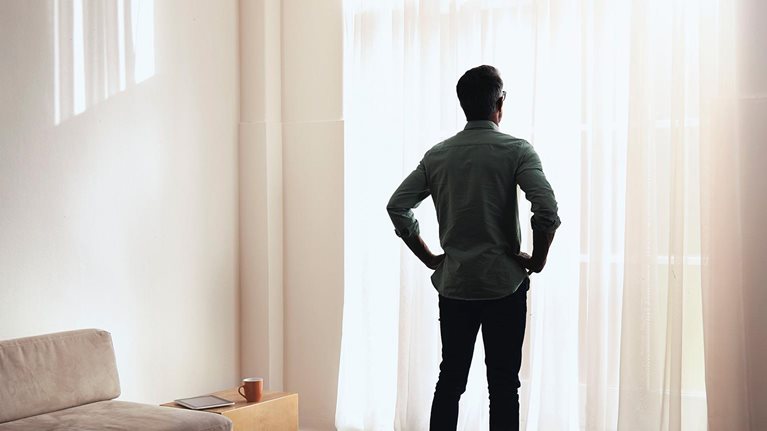
The digital-led recovery from COVID-19: Five questions for CEOs
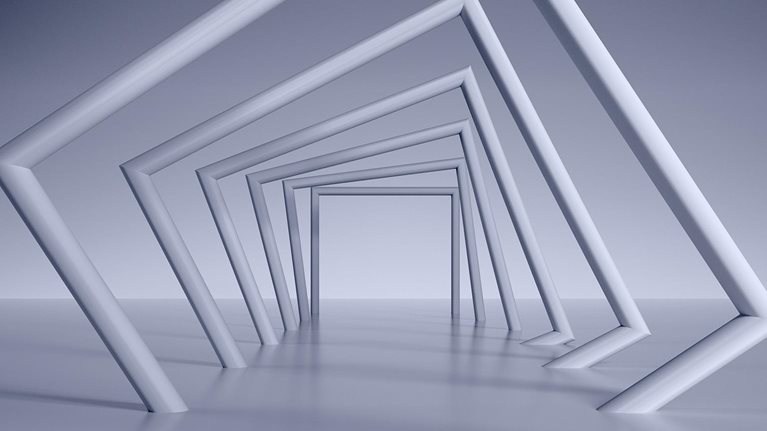
How to make the most of AI? Open up and share data
Developing an effective data governance framework to deliver African digital potentials
Subscribe to africa in focus, adedeji adeniran adedeji adeniran director of research - center for the study of the economies of africa @adedeji_adenira.
March 21, 2022
Below is a governance viewpoint from the Foresight Africa 2022 report, which explores top priorities for the region in the coming year. Read the full chapter on technological innovations .

The intersections of these threats could significantly affect the transformational impacts of digitalization; hence, there is a need for a data governance framework that maximizes the potential gains (through enablers) and limits the threats (through safeguards).
State of data governance in Africa
- Proliferation of laws and regulations on data protection. Between 2012 and 2021, the number of African countries with at least one form of data protection law tripled from 12 to 28 . Though the current adoption rate is 52 percent for the African region, it is still the lowest relative to other regions. Notably, the data governance framework tends to show more emphasis on fostering safeguards (e.g., data protection, privacy), and less focus on enablers (e.g., data portability, localization)—but both efforts are crucial.
- Implementation remains a challenge. Institutions charged with regulating data governance have not evolved with the dynamic needs and peculiarities of the digital space. Regulatory inertia and capacity challenges that characterize broader governance in Africa are manifesting in data governance implementation, given that this structure is embedded within the existing public institutional framework. The implementation gap is further worsened by the limitations in human and financial capacity of regulatory agencies, and power and knowledge asymmetry between platform firms and mostly small and resource-constrained African countries.
- There is more progress at the national level than through regional and multilateral frameworks. Given the fragmented data policy environment and capacity gap in Africa’s digital space, scaling up regional efforts is crucial for addressing the inadequacies in the national efforts: Even developed countries like those in Europe have found a regional approach to be an effective means of addressing power and knowledge asymmetry. Currently, only eight African countries have ratified the Malabo Convention , the regional-led approach for data protection and cybercrime law. Similarly, only six African countries are participating in the World Trade Organization’s e-commerce plurilateral negotiations , which will set up new global trading rules for electronic commerce and trade.
Policymakers must build checks and balances into the data governance framework as a monopoly in data governance, either by government or the private sector, will hinder digital development.
Recommendations
- Policymakers should scale up efforts on “enabler” laws, especially around e-commerce, for safe and secure financial settlements, improved and better enforcement without recourse to data protectionism, and enabling cross-border data flows. In general, enhancing the implementation capacity with investment in finance, manpower, and technical skills of data regulatory authorities is important to ensuring data governance supports development outcomes.
- Policymakers must build checks and balances into the data governance framework as a monopoly in data governance, either by government or the private sector, will hinder digital development. Civil society accountability and regional cooperation are also needed to limit state abuses as well as platform companies’ abuse resulting from their dominance and gatekeeper roles in the digital space.
- National and regional leaders must enhance collaboration and coordination around strengthening regional data governance, sharing mechanisms and experiences for best practices in regulating the digital space, and committing more to multilateral frameworks for data governance.
Related Content
Mike Ogbalu III
February 11, 2022
Tara Nathan
February 9, 2022
Lesly Goh, Buhle Goslar
February 1, 2022
Global Economy and Development
Sub-Saharan Africa
Africa Growth Initiative
Jacob Taylor
May 20, 2024
Mark Schoeman
May 16, 2024
Online Only
9:30 am - 10:30 am EDT
The healthcare industry faces arguably the highest stakes when it comes to data governance. For starters, healthcare organizations constantly encounter vast (and ever-increasing) amounts of highly regulated personal data.
The impact of healthcare data usage on people’s lives lies at the heart of why data governance in healthcare is so crucial.In healthcare, managing the accuracy, quality and integrity of data is the focus of data governance. When healthcare organizations excel at this, it can lead to better clinical decision-making, improved patient outcomes and prevention of medical errors.
Despite this, many healthcare organizations face challenges. Healthcare organizations need a strong data governance framework to help ensure compliance with regulations like the Health Insurance Portability and Accountability Act of 1996 (HIPAA) in the US and the General Data Protection Regulation (GDPR) in the EU.
How can a healthcare provider improve its data governance strategy, especially considering the ripple effect of small changes? Data lineage can help.With data lineage, your team establishes a strong data governance strategy, enabling them to gain full control of your healthcare data pipeline.
Understanding data governance in healthcare
The need for a strong data governance framework is undeniable in any highly-regulated industry, but the healthcare industry is unique because it collects and processes massive amounts of personal data to make informed decisions about patient care. One broken or incomplete piece of data can trigger not only noncompliance and audit issues but also harm real people. For example:
- Healthcare providers regularly rely on medical records to diagnose and establish treatment plans for patients. Inaccuracies in these medical records leading to misdiagnoses, medication errors or delayed care can have serious consequences.
- Inaccuracies might also lead to more delays or complications with insurance coverage.
- Healthcare organizations must adhere to data privacy regulations like HIPAA and GDPR. Noncompliance with these laws is costly and can damage your reputation, besides posing a danger to patients and practitioners when data breaches occur.
Conversely, confidence in the accuracy and consistency of your data can minimize the risk of adverse health outcomes, rather than merely reacting to or causing them. Also, using predictive analytics can help identify trends, patterns and potential future health risks in your patients.
It’s worth noting that most electronic health records (EHR) systems offer predictive analytics capabilities. The accuracy of these analytics is limited by the accuracy of the data used.
Thus, having a comprehensive understanding of the data environment and a clear chain of custody becomes imperative. Detecting leaks and pressure points depends on implementing a strong data governance strategy, with data lineage as a critical component.
Challenges in data governance for healthcare and how data lineage can help
Data governance can help healthcare organizations maximize the accuracy and security of their data assets. At the same time, implementing a data governance framework poses some challenges, such as data quality issues, data silos security and privacy concerns.
1. Data quality issues
Positive business decisions and outcomes rely on trustworthy, high-quality data. However, healthcare facilities continue to face data quality issues despite the best efforts of business leaders, primarily due to the sheer number of people inputting data and the high-pressure situations in which data entry often occurs.
A study conducted by the Journal of the American Medical Association (JAMA) revealed that errors were found in the records of one-fifth of patients with access to ambulatory care notes. Among those patients, 21% identified the errors as critical, with common issues including diagnostic errors, medication data errors and incomplete or inaccurate EHR data conversions. These errors are crucial and can occur daily. To prevent these errors, it’s critical for data flows to be mapped out and for issues with data quality to be flagged by using root-cause analysis, thereby reducing the impact on patients.
2. Data silos
In the healthcare industry, where an estimated 30% of the world’s total data is generated, patient data often remains unstructured and scattered across disparate systems. The consequence? An incomplete picture of patient health and multiple sources of truth prevents you from achieving the benefits of data visibility such as informed patient care. Issues with compliance and audit conduct also arise due to these scattered data sources.
The solution lies in the ability to visualize patient data from different sources in one place. That’s exactly what enterprise-wide data lineage does. Data lineage extends throughout your data environment to create a comprehensive map of all your data flows and dependencies, eliminating data silos effectively.
However, not all data lineage solutions can visualize data from different silos. Some platforms only allow you to see data stored within their specific catalog. Opting for a catalog-agnostic solution helps you address this issue.
3. Security concerns and chain of custody
Healthcare organizations are in a unique position because both depend on cross-departmental information sharing to facilitate patient care and are bound by strict regulations to help ensure secure data transmission.
As part of both HIPAA and GDPR compliance, healthcare organizations need to provide auditors with details regarding the chain of custody of patient records. This includes information on who accessed the records and the time and location of access. Establishing a chain of custody for data stored in an EHR system accessible across several devices within a medical facility can be laborious and time-intensive, especially when dealing with so many records that exist in a paper format or have been manually entered or scanned.
Data lineage significantly reduces the amount of effort needed to establish a chain of custody within healthcare information systems . By mapping data flows, you can trace the journey of your data backward to see where and when it was changed in your systems. Combined with your governance efforts that establish the meaning, quality and stewardship of the data stores in this chain of custody, you can deliver the crucial data pipeline information required by your auditors.
Better patient care and predictive analytics
With high-quality data, you can provide well-informed, cross-collaborative and personalized patient care. You also place deeper trust in the predictive analytics within your EHR system to predict patient conditions, disease progression, hospital overstays, readmissions, and more. All this relies on reliable data and requires data lineage for governance.
Enhanced regulatory compliance
If you’re struggling with data silos, data quality or proving chain of custody, you might also be finding it difficult to establish and prove compliance with healthcare-related regulations like HIPAA and GDPR. Data lineage can help you establish your chain of information flow and dependencies to auditors clearly and quickly, which is key to compliance.
Increased data security and privacy
In the healthcare industry, data privacy is integral. When data lineage creates a map of your data environment, it does so without sharing or processing any private information. Instead, it uses active metadata . That means that you can create a strong data governance framework without sacrificing patient privacy.
Improved operational efficiency and cost savings
Mapping out data flows manually is a time and resource-intensive process, especially in the highly-complex healthcare industry. Among the top advantages of automated data lineage for data governance are its operational efficiency and cost-effectiveness. You can save money and time on labor costs and focus your efforts on what matters most to your organization.
We’re 90% faster
“Our ETL teams can identify the impacts of planned ETL process changes 90% faster than before.” Robert D, BI Team Leader at GEMU
90% increase in analyzing source system changes
“Effort for analyzing the impact of a source system change has decreased by at least 90%, from hours to minutes (or seconds).” Michael L., BI Manager at Schumacher Clinical
Data governance and compliance
In the healthcare industry, ensuring compliance with regulations like HIPAA and GDPR is another important piece of data governance, crucial for both protecting patient privacy and facilitating secure information-sharing critical for the highest level of patient care.
Some healthcare organizations today still struggle to maintain compliance with HIPAA and GDPR. Meanwhile, the world’s regulatory landscape is becoming increasingly complex. In fact, Gartner® predicts that by the end of 2024, 75% of the world will have its data protected under modern privacy regulations. Given that the healthcare industry is generating new regulated patient data by the second, now is the time to kickstart an effective data governance strategy.
It’s worth noting that these regulations don’t just apply to patient care-focused organizations. Nearly every area of healthcare processed large quantities of protected data, including:
- Biotechnology companies
- Health insurance providers
- Medical device manufacturers
- Pharmaceutical companies
With data lineage, you get a detailed map of your data flows that help ensure that you are processing and securing data within the strict requirements of regulatory frameworks like HIPAA and GDPR. You can also more easily prove the chain of custody to auditors, who will need to see who has had access to your regulated data assets and apply stricter controls around who has access.
Next steps to increase data security and enhance compliance
The modern healthcare industry is undeniable in its complexity, with the emergence of EHR systems, the proliferation of healthcare data, and an increasingly complex regulatory landscape contributing to this complexity.
To keep up, healthcare companies today need to implement data governance. A strong data governance framework helps ensure that you can verify that the data you’re collecting, processing and using is accurate, consistent and dependable. Without it, you risk making poorly informed decisions about patient care based on erroneous data or inaccurate predictive insights. These decisions can have serious or even fatal outcomes for patients.
Data governance is also integral when complying with healthcare data privacy regulations like HIPAA and GDPR. Any healthcare organization processing protected data needs to have a data governance strategy in place to remain compliant with these regulations and be prepared for any new regulations that might arise.
Despite challenges like data quality issues, data silos, security concerns and proving chain of custody, there is a solution: automated data lineage. By using automated data lineage, your organization can overcome common data governance barriers, improve patient care, enhance regulatory compliance, increase data security and privacy and enhance operational efficiency while reducing costs.
More from Artificial intelligence
In preview now: ibm watsonx bi assistant is your ai-powered business analyst and advisor.
3 min read - The business intelligence (BI) software market is projected to surge to USD 27.9 billion by 2027, yet only 30% of employees use these tools for decision-making. This gap between investment and usage highlights a significant missed opportunity. The primary hurdle in adopting BI tools is their complexity. Traditional BI tools, while powerful, are often too complex and slow for effective decision-making. Business decision-makers need insights tailored to their specific business contexts, not complex dashboards that are difficult to navigate. Organizations…
Introducing the watsonx platform on Microsoft Azure
4 min read - Artificial intelligence (AI) is revolutionizing industries by enabling advanced analytics, automation, and personalized experiences. According to The business value of AI, from the IBM Institute of Business Value, AI adoption has more than doubled since 2017. Enterprises are taking an intentional design approach to hybrid cloud and AI to drive technology decisions and enable adoption of Generative AI. According to the McKinsey report, The economic potential of generative AI: The next productivity frontier, generative AI is projected to add $2.6…
Democratizing Large Language Model development with InstructLab support in watsonx.ai
5 min read - There is no doubt that generative AI is changing the game for many industries around the world due to its ability to automate and enhance creative and analytical processes. According to McKinsey, generative AI has a potential to add $4 trillion to the global economy. With the advent of generative AI and, more specifically, Large Language Models (LLMs), driving tremendous opportunities and efficiencies, we’re finding that the path to success for organizations to effectively use and scale their generative AI…
IBM Newsletters
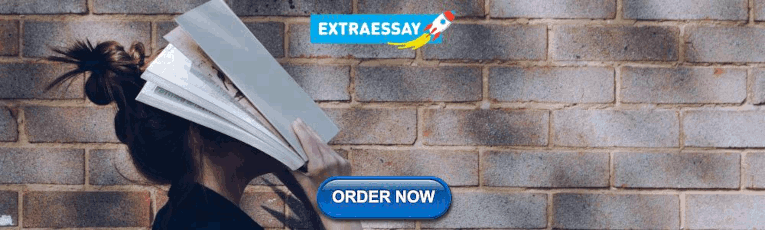
IMAGES
VIDEO
COMMENTS
Data Gove rnance: A conceptual framework, structured review, and. r esearch agenda. 1. Abstract. Data governance refers to the exercise of authority and control over the management of data. The ...
First, data governance is a cross-functional effort. It enables collaboration across functional boundaries and data subject areas. Second, data governance is a framework, which provides structure and formalization for the management of data. Third, data governance focuses on data as a strategic enterprise asset.
Data governance depicts a structured framework for decision-making, rights, and responsibilities regarding the use of data in an enterprise [15]. Generally, data only generate value if it is being used and analyzed. Data quality refers to data "fitness for use" [16].
Data governance is a success factor for BDAS (Brous, Janssen, & Krans, 2020) and has an overall positive effect on the performance of organizations that apply BDAS (Zhang, Zhao, & Kumar, 2016). The purpose is to increase the value of data and minimize data-related costs and risks (Abraham, Schneider, & vom Brocke, J., 2019). Given the ...
Master's thesis Subject: Information Systems Science Author: Miikka Luosmaa Title: Data governance on data platforms - designing playbook for data platforms Supervisor(s): Ph. D. Tiina Nokkala Number of pages: 50 pages + appendices seven pages Date: 20.2.2022 Abstract The amount and value of data are increasing (Nokkala 2020). Data can be seen as one of the key
This thesis develops a Values-Based Data Governance (VBDG) theory by understanding the political, cultural, ideological, and historical contexts for governance of data in social economy organizations (SEOs) with a focus on Ontario, and more broadly Canada.
Data governance standards are complex and lack implementation details. This paper proposes Data Governance Model used in a financial institution. Data Governance Model is based on BCBS 239 and DAMA methodology. The Model enhances practices of DAMA and tailors them to the needs of financial institution. The scope of the Data Governance Model is ...
The findings address challenges of data governance in inter organizational settings and help practitioners to define their own data governance. Additionally, the thesis highlights the potential for future research. Keywords: Data Governance, Digital Platform, Taxonomy, Building Sector. 1. Introduction.
Data governance is necessary to smart cities to ensure integrity, accessibility, and accountability of data. There is also a growing concern about having proper data governance to protect citizens' digital rights and democracy. ... This thesis aims to address this gap by understanding the current state of data governance of proposed Canadian ...
Data governance is not a one size fits all, instead, it should be an evolutionary process that can be started small and measurable along the way. This research aims at proposing a data governance framework by ensuring data management processes, data security and control are compliant with laws and policies. This article also presents the first results of a comparative analysis between three ...
Data governance background. Data governance is defined as 'A companywide framework for assigning decision-related rights and duties in order to be able to adequately handle data as a company asset' (Otto, Citation 2011b; p. 47).The main driver for data governance is considering data as an asset of the firm (Panian, Citation 2010).Horne (Citation 1995) connected governance with optimal uses ...
Data Governance for an effective Data Management. The purpose of this thesis is to introduce a road map about how to develop a framework for the Data Governance of Big Data. Since most of the knowledge that exists nowadays comes from IT Governance, this thesis aims to separate those two fields and focus on Data Governance itself. 1.1Problem Area
Data Governance Maturity Model (DGMM): Based on the literature a maturity model is designed with relevant dimensions, levels, qualifications and criteria to grow in data governance. A translation ...
features of the GDPR, the de facto reality is that data privacy regulation is a tool of state control. The United States case shows how a changing global environ-ment forced the U.S. legislators to retreat from their market-driven approach to data governance in the direction of GDPR-like regulation. Primary Reader & Advisor: Matthias Matthijs, PhD
For instance, 47 percent of nations with a trade secret law did not give firms using data analytics explicit control over data they analyzed using a mechanism protected under trade secrets, while 52.9 percent of nations did give these firms such control. Significant convergence on data governance in trade agreements: 77 percent of our cases ...
The Role of Data Protection and Cybersecurity Regulations in Artificial Intelligence Global Governance: A Comparative Analysis of the European Union, the United States, and China Regulatory Framework By Dalia Alic In Partial Fulfillment of the Requirements for the Master of Arts in Human Rights Master of Arts in Human Rights Final Thesis
This thesis is trying to point out the background for this problem, find out what companies can do to make situation better and what are the advantages to business that can be achieved with better data management. KEYWORDS: Data, information, data governance, data management
3. Prioritize data assets and focus data leadership accordingly. Many organizations approach data governance in a holistic manner, looking at all data assets at once. But such a large scope means slow relative progress in any given area and a risk that efforts aren't linked directly to business needs.
Brown ( 2010) see Data Governance as. referring to the assignment of decision-. making rights with regard to an enter-. prise's " data assets". Data Governance aims at maximizing. the value ...
ABSTRACT. Data Trusts are an important emerging approach to enabling the much wider sharing of data from many different sources and for many different purposes, backed by the confidence of clear and unambiguous data governance. Data Trusts combine the technical infrastructure for sharing data with the governance framework of a legal trust.
Notably, the data governance framework tends to show more emphasis on fostering safeguards (e.g., data protection, privacy), and less focus on enablers (e.g., data portability, localization)—but ...
The impact of healthcare data usage on people's lives lies at the heart of why data governance in healthcare is so crucial.In healthcare, managing the accuracy, quality and integrity of data is the focus of data governance. When healthcare organizations excel at this, it can lead to better clinical decision-making, improved patient outcomes ...