We can help you reset your password using the email address linked to your Project Euclid account.

- Subscription and Access
- Library Resources
- Publisher Tools
- Researcher Resources
- About Project Euclid
- Advisory Board
- News & Events
- SUPPLEMENTAL CONTENT
- DOWNLOAD PAPER SAVE TO MY LIBRARY
The study of networks leads to a wide range of high-dimensional inference problems. In many practical applications, one needs to draw inference from one or few large sparse networks. The present paper studies hypothesis testing of graphs in this high-dimensional regime, where the goal is to test between two populations of inhomogeneous random graphs defined on the same set of $n$ vertices. The size of each population $m$ is much smaller than $n$, and can even be a constant as small as 1. The critical question in this context is whether the problem is solvable for small $m$.
We answer this question from a minimax testing perspective. Let $P$, $Q$ be the population adjacencies of two sparse inhomogeneous random graph models, and $d$ be a suitably defined distance function. Given a population of $m$ graphs from each model, we derive minimax separation rates for the problem of testing $P=Q$ against $d(P,Q)>\rho $. We observe that if $m$ is small, then the minimax separation is too large for some popular choices of $d$, including total variation distance between corresponding distributions. This implies that some models that are widely separated in $d$ cannot be distinguished for small $m$, and hence, the testing problem is generally not solvable in these cases.
We also show that if $m>1$, then the minimax separation is relatively small if $d$ is the Frobenius norm or operator norm distance between $P$ and $Q$. For $m=1$, only the latter distance provides small minimax separation. Thus, for these distances, the problem is solvable for small $m$. We also present near-optimal two-sample tests in both cases, where tests are adaptive with respect to sparsity level of the graphs.
Debarghya Ghoshdastidar. Maurilio Gutzeit. Alexandra Carpentier. Ulrike von Luxburg. "Two-sample hypothesis testing for inhomogeneous random graphs." Ann. Statist. 48 (4) 2208 - 2229, August 2020. https://doi.org/10.1214/19-AOS1884
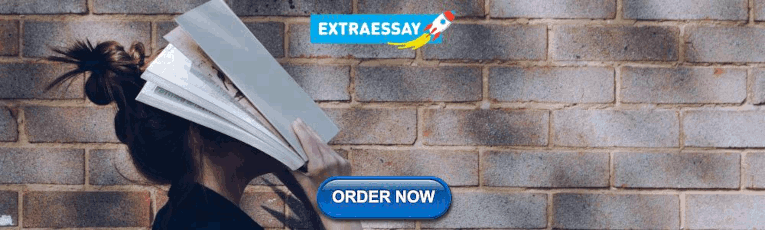
Information
MathSciNet: MR4134792 Digital Object Identifier: 10.1214/19-AOS1884
Subjects: Primary: 62H15 Secondary: 05C80 , 60B20 , 62C20
Keywords: inhomogeneous Erdős–Rényi model , minimax testing , two-sample test
Rights: Copyright © 2020 Institute of Mathematical Statistics

KEYWORDS/PHRASES
Publication title:, publication years.
Two-sample hypothesis testing for inhomogeneous random graphs
- Related Documents
The Fréchet Mean of Inhomogeneous Random Graphs
Big jobs arrive early: from critical queues to random graphs.
We consider a queue to which only a finite pool of n customers can arrive, at times depending on their service requirement. A customer with stochastic service requirement S arrives to the queue after an exponentially distributed time with mean S-α for some [Formula: see text]; therefore, larger service requirements trigger customers to join earlier. This finite-pool queue interpolates between two previously studied cases: α = 0 gives the so-called [Formula: see text] queue and α = 1 is closely related to the exploration process for inhomogeneous random graphs. We consider the asymptotic regime in which the pool size n grows to infinity and establish that the scaled queue-length process converges to a diffusion process with a negative quadratic drift. We leverage this asymptotic result to characterize the head start that is needed to create a long period of activity. We also describe how this first busy period of the queue gives rise to a critically connected random forest.
Parameterized clique on inhomogeneous random graphs
The largest component in subcritical inhomogeneous random graphs.
We study the ‘rank 1 case’ of the inhomogeneous random graph model. In the subcritical case we derive an exact formula for the asymptotic size of the largest connected component scaled to log n. This result complements the corresponding known result in the supercritical case. We provide some examples of applications of the derived formula.
Duality in inhomogeneous random graphs, and the cut metric
Diffusion approximation for the components in critical inhomogeneous random graphs of rank 1., geometric inhomogeneous random graphs, limits of multiplicative inhomogeneous random graphs and lévy trees: limit theorems.
AbstractWe consider a natural model of inhomogeneous random graphs that extends the classical Erdős–Rényi graphs and shares a close connection with the multiplicative coalescence, as pointed out by Aldous (Ann Probab 25:812–854, 1997). In this model, the vertices are assigned weights that govern their tendency to form edges. It is by looking at the asymptotic distributions of the masses (sum of the weights) of the connected components of these graphs that Aldous and Limic (Electron J Probab 3:1–59, 1998) have identified the entrance boundary of the multiplicative coalescence, which is intimately related to the excursion lengths of certain Lévy-type processes. We, instead, look at the metric structure of these components and prove their Gromov–Hausdorff–Prokhorov convergence to a class of (random) compact measured metric spaces that have been introduced in a companion paper (Broutin et al. in Limits of multiplicative inhomogeneous random graphs and Lévy trees: the continuum graphs. arXiv:1804.05871, 2020). Our asymptotic regimes relate directly to the general convergence condition appearing in the work of Aldous and Limic. Our techniques provide a unified approach for this general “critical” regime, and relies upon two key ingredients: an encoding of the graph by some Lévy process as well as an embedding of its connected components into Galton–Watson forests. This embedding transfers asymptotically into an embedding of the limit objects into a forest of Lévy trees, which allows us to give an explicit construction of the limit objects from the excursions of the Lévy-type process. The mains results combined with the ones in the other paper allow us to extend and complement several previous results that had been obtained via model- or regime-specific proofs, for instance: the case of Erdős–Rényi random graphs obtained by Addario-Berry et al. (Probab Theory Relat Fields 152:367–406, 2012), the asymptotic homogeneous case as studied by Bhamidi et al. (Probab Theory Relat Fields 169:565–641, 2017), or the power-law case as considered by Bhamidi et al. (Probab Theory Relat Fields 170:387–474, 2018).
Critical behavior in inhomogeneous random graphs
A phase transition regarding the evolution of bootstrap processes in inhomogeneous random graphs, export citation format, share document.
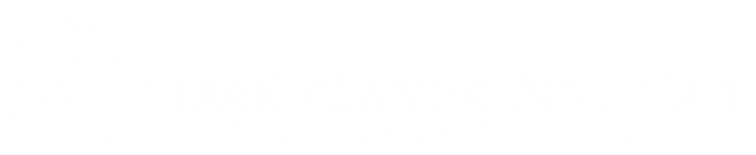
Two-sample Hypothesis Testing for Inhomogeneous Random Graphs
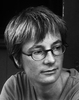
Subscribe to the PwC Newsletter
Join the community, edit social preview.

Add a new code entry for this paper
Remove a code repository from this paper, mark the official implementation from paper authors, add a new evaluation result row.
- TWO-SAMPLE TESTING
- VOCAL BURSTS VALENCE PREDICTION
Remove a task

Add a method
Remove a method, edit datasets, two-sample hypothesis testing for inhomogeneous random graphs.
4 Jul 2017 · Debarghya Ghoshdastidar , Maurilio Gutzeit , Alexandra Carpentier , Ulrike Von Luxburg · Edit social preview
The study of networks leads to a wide range of high dimensional inference problems. In many practical applications, one needs to draw inference from one or few large sparse networks. The present paper studies hypothesis testing of graphs in this high-dimensional regime, where the goal is to test between two populations of inhomogeneous random graphs defined on the same set of $n$ vertices. The size of each population $m$ is much smaller than $n$, and can even be a constant as small as 1. The critical question in this context is whether the problem is solvable for small $m$. We answer this question from a minimax testing perspective. Let $P,Q$ be the population adjacencies of two sparse inhomogeneous random graph models, and $d$ be a suitably defined distance function. Given a population of $m$ graphs from each model, we derive minimax separation rates for the problem of testing $P=Q$ against $d(P,Q)>\rho$. We observe that if $m$ is small, then the minimax separation is too large for some popular choices of $d$, including total variation distance between corresponding distributions. This implies that some models that are widely separated in $d$ cannot be distinguished for small $m$, and hence, the testing problem is generally not solvable in these cases. We also show that if $m>1$, then the minimax separation is relatively small if $d$ is the Frobenius norm or operator norm distance between $P$ and $Q$. For $m=1$, only the latter distance provides small minimax separation. Thus, for these distances, the problem is solvable for small $m$. We also present near-optimal two-sample tests in both cases, where tests are adaptive with respect to sparsity level of the graphs.
Code Edit Add Remove Mark official
Tasks edit add remove, datasets edit, results from the paper edit add remove, methods edit add remove.
Two-sample Hypothesis Testing for Inhomogeneous Random Graphs
The study of networks leads to a wide range of high dimensional inference problems. In many practical applications, one needs to draw inference from one or few large sparse networks. The present paper studies hypothesis testing of graphs in this high-dimensional regime, where the goal is to test between two populations of inhomogeneous random graphs defined on the same set of n 𝑛 n vertices. The size of each population m 𝑚 m is much smaller than n 𝑛 n , and can even be a constant as small as 1. The critical question in this context is whether the problem is solvable for small m 𝑚 m .
We also show that if m > 1 𝑚 1 m>1 , then the minimax separation is relatively small if d 𝑑 d is the Frobenius norm or operator norm distance between P 𝑃 P and Q 𝑄 Q . For m = 1 𝑚 1 m=1 , only the latter distance provides small minimax separation. Thus, for these distances, the problem is solvable for small m 𝑚 m . We also present near-optimal two-sample tests in both cases, where tests are adaptive with respect to sparsity level of the graphs.
1707.00833 \startlocaldefs \endlocaldefs
t1Partially supported by the Deutsche Forschungsgemeinschaft (DFG, FOR1735), the Institutional Strategy of the University of Tübingen (DFG, ZUK 63) and by the Baden-Württemberg Stiftung through the Eliteprogramm for Postdocs. t2Supported by the Deutsche Forschungsgemeinschaft (DFG) Emmy Noether grant MuSyAD (CA 1488/1-1). t3Partially supported by the DFG Emmy Noether grant MuSyAD (CA 1488/1-1), by the DFG - 314838170, GRK 2297 MathCoRe, by the DFG GRK 2433 DAEDALUS (384950143/GRK2433), by the DFG CRC 1294 ‘Data Assimilation’, Project A03, and by the UFA-DFH through the French-German Doktorandenkolleg CDFA 01-18. t4Partially supported by the DFG FOR1735, the Institutional Strategy of the University of Tübingen (DFG, ZUK 63) and DFG Cluster of Excellence “Machine Learning – New Perspectives for Science” EXC 2064/1, project number 390727645.
1 Introduction
Analysis of random graphs has piqued the curiosity of probabilists since its inception decades ago, but the widespread use of networks in recent times has made statistical inference from random graphs a topic of immense interest for both theoretical and applied researchers. This has caused a fruitful interplay between theory and practice leading to deep understanding of statistical problems that, in turn, has led to advancements in applied research. Significant progress is clearly visible in problems related to network modelling (Albert and Barabási, 2002 ; Lovász, 2012 ) , community detection (Decelle et al., 2011 ; Abbe and Sandon, 2016 ) , network dynamics (Berger et al., 2005 ) among others, where statistically guaranteed methods have emerged as effective practical solutions. Quite surprisingly, the classical problem of hypothesis testing of random graphs is yet to benefit from such joint efforts from theoretical and applied researchers. It should be noted the problem itself is actively studied in both communities. Testing between brain or ‘omics’ networks have surfaced as a crucial challenge in the context of both modelling and decision making (Ginestet et al., 2017 ; Hyduke, Lewis and Palsson, 2013 ) . On the other hand, phase transitions are now known for the problems of detecting high-dimensional geometry or strongly connected groups in large random graphs (Bubeck et al., 2016 ; Arias-Castro and Verzelen, 2014 ) . However, little progress has been made in the design of consistent tests for general models of random graphs. The present paper takes a step towards addressing this general concern.
While research on testing large random graphs has been limited, hypothesis testing in large dimension is an integral part of modern statistics literature. In fact, Bai and Saranadasa ( 1996 ) demonstrated the need for studying high dimensional statistics through a two-sample testing problem, where one tests between two n 𝑛 n -variate normal distributions with different means by accessing m 𝑚 m i.i.d. observations from either distributions, where m ≪ n much-less-than 𝑚 𝑛 m\ll n (we denote the dimension by n 𝑛 n since, in the context of graphs, the number of vertices n 𝑛 n governs the dimensionality of the problem). More recent works in this direction provide tests and asymptotic guarantees as m → ∞ → 𝑚 m\to\infty and n m → ∞ → 𝑛 𝑚 \frac{n}{m}\to\infty (Chen and Qin, 2010 ; Cai, Liu and Xia, 2014 ; Ramdas et al., 2015 ) . Similar studies also exist in the context of testing whether a n 𝑛 n -dimensional covariance matrix is identity, where only m ≪ n much-less-than 𝑚 𝑛 m\ll n i.i.d. observations of the data is available (Ledoit and Wolf, 2002 ; Berthet and Rigollet, 2013 ; Arias-Castro, Bubeck and Lugosi, 2015 ) . It is also known that the computational complexity of this problem is closely related to the clique detection problem in random graphs (Berthet and Rigollet, 2013 ) . An extreme version of high dimensional testing arises in the signal detection literature, where one observes a high-dimensional (Gaussian) signal, and tests the nullity of its mean. Subsequently, asymptotics of the problem is considered for n → ∞ → 𝑛 n\to\infty but fixed sample size ( m = 1 𝑚 1 m=1 or a constant), and minimax separation rates between the null and the alternative hypotheses are derived (Ingster and Suslina, 2003 ; Baraud, 2002 ; Verzelen and Arias-Castro, 2017 ; Mukherjee, Pillai and Lin, 2015 ) . The signal detection problem has also been extended in the case of matrices in the context of trace regression (Carpentier and Nickl, 2015 ) and sub-matrix detection (Sun and Nobel, 2008 ) among others, where the latter generalises the planted clique detection problem.
In practice, the problem of testing random graphs comes in a wide range of flavours. For instance, while dealing with graphs associated with chemical compounds (Shervashidze et al., 2011 ) or brain networks of several patients collected at multiple laboratories (Ginestet et al., 2017 ) , one has access to a large number of graphs (large m 𝑚 m ). This scenario is more amenable as one can resort to the vast literature of non-parametric hypothesis testing that can even be applied to random graphs. A direct approach to this problem is to use kernel based tests (Gretton et al., 2012 ) in conjunction with graph kernels (Vishwanathan et al., 2010 ; Kondor and Pan, 2016 ) , which does not require any structural assumptions on the network models. However, known guarantees for such tests depend crucially on the sample size, and one cannot conclude about the fidelity of such tests for very small m 𝑚 m . A more challenging situation arises when m 𝑚 m is small, and unfortunately, this is often the case in network analysis. For example, if the graphs correspond to brain networks collected from patients in a single lab setup ( m < 20 ) 𝑚 20 (m<20) , brain networks of one individual obtained from test-retest MRI scans (Landman et al., 2011 ) , or molecular interaction networks arising from genomic or proteomic data (Hyduke, Lewis and Palsson, 2013 ) . Test-retest data of a patient provide only m = 2 𝑚 2 m=2 networks, while omics data typically result in one large interaction network, that is, m = 1 𝑚 1 m=1 . Hence, designing two-sample tests for small populations for graphs is a problem of immense significance, and yet, practical tests with statistical guarantees are rather limited.
From a theoretical perspective, only a handful of results on testing large random graphs are known. While finding hidden cliques have been a long-standing open problem, Arias-Castro and Verzelen ( 2014 , 2015 ) for the first time provide a characterisation of the more basic problem of detecting a planted clique in an Erdős-Rényi graph. Gao and Lafferty ( 2017 ) and Lei ( 2016 ) consider generalised variants of this problem while designing tests to distinguish a stochastic block model from an Erdős-Rényi graph, or to estimate the number of communities in a stochastic block model, respectively. In a different direction, Bubeck et al. ( 2016 ) study the classical problem of testing whether a given graph corresponds to a neighbourhood graph in a high-dimensional space, or it is generated from Erdős-Rényi model. This result is in fact a specific instance of the generic problem of detecting whether a network data has a dependence structure or is unstructured (Ryabko, 2017 ; Bresler and Nagaraj, 2018 ; Daskalakis, Dikkala and Kamath, 2018 ) . The first study on two-sample testing of graphs, under a relatively broad framework is by Tang et al. ( 2017a ) , where the authors test between a pair of random dot product graphs which are undirected graphs on a common set of n 𝑛 n vertices with mutually independent edges and low-rank population adjacency matrices. A test statistic based on the difference in adjacency spectral embeddings is shown to be asymptotically consistent as n → ∞ → 𝑛 n\to\infty provided that the rank of the population adjacencies is fixed and known. Tang et al. ( 2017b ) study a more general problem of comparing two random dot product graphs defined on different vertex sets, where the vertices have latent Euclidean representations. The latent representation can be recovered from the adjacency spectral embedding, and kernel two-sample testing for Euclidean data can be employed to solve the testing problem. Ghoshdastidar et al. ( 2017 ) provide a framework to formulate the graph two-sample problem with minimal structural restrictions, and show that this general framework can be used to prove minimax optimality of tests based on triangle counts and spectral properties under special cases.
In this paper, we restrict ourselves to graphs on a common vertex set of size n 𝑛 n and sampled from an inhomogeneous Erdős-Rényi (IER) model (Bollobas, Janson and Riordan, 2007 ) , that is, we consider undirected and unweighted random graphs where the edges occur independently, but there is no structural assumption on the population adjacency matrix. We study the problem of testing between two IER models, where m 𝑚 m i.i.d. graphs are observed for each model. Apparently, allowing m ≥ 1 𝑚 1 m\geq 1 appears to be a slight generalisation of the m = 1 𝑚 1 m=1 case (Tang et al., 2017a ) , but we show that in some situations, the testing problem behaves differently in the m = 1 𝑚 1 m=1 and m > 1 𝑚 1 m>1 cases. It is also well established that many graph learning problems have different behaviour in the case of dense and sparse graphs. This is indeed true in the context of testing for geometric structures (Bubeck et al., 2016 ) and community detection (Arias-Castro and Verzelen, 2015 ) . Bearing this in mind, we study the two-sample problem at different levels of sparsity of the graph. A formal description of the problem is presented in Section 2 .
Given the above framework, one may resort to a variety of testing procedures. A classical approach involves viewing the problem as an instance of closeness testing for high dimensional discrete distributions (Chan et al., 2014 ; Daskalakis, Dikkala and Kamath, 2018 ) and using variants of the χ 2 superscript 𝜒 2 \chi^{2} -test or related localisation procedures. On the other hand, exploiting the independence of edges, one may even view the problem as a instance of multiple testing, and may resort to tests based on higher criticism (Donoho and Jin, 2004 , 2015 ) . A more direct approach may be to simply compare the adjacency spectral embeddings (Tang et al., 2017a ) , or other network statistics (Ghoshdastidar et al., 2017 ) or even the raw adjacency matrices. Sections 3 – 5 present a variety of testing problems, which differ in terms of the distance d ( P , Q ) 𝑑 𝑃 𝑄 d(P,Q) , that is, how we quantify the separation between the two models. We show that some of the above principles are not useful for small m 𝑚 m since the associated testing problems are generally unsolvable in these cases. However, in some cases, one can construct uniformly consistent tests that work with a small number of observation, even m = 1 𝑚 1 m=1 . Section 6 discusses the practicality of graph two-sample testing and also presents minimax separation under special cases of the IER model, such as Erdős-Rényi or stochastic block models, or under different notions of sparsity in graphs. The detailed proofs are provided in the appendix.
2 Problem statement
In this section, we formally state the generic two-sample graph testing problem studied in this paper. We also present the minimax framework that forms the basis of our theoretical analysis.
We use the notations ≲ less-than-or-similar-to \lesssim and ≳ greater-than-or-equivalent-to \gtrsim to denote the standard inequalities but ignoring absolute constants. Further, we use ≍ asymptotically-equals \asymp to denote that two quantities are same up to possible difference in constant scaling. We use ∧ \wedge and ∨ \vee (or ⋀ \bigwedge and ⋁ \bigvee ) to denote minimum and maximum, respectively. We also need several standard norms and distances. For two discrete distributions, we denote the total variation distance by T V ( ⋅ , ⋅ ) 𝑇 𝑉 ⋅ ⋅ TV(\cdot,\cdot) and the symmetric Kullback-Leibler (KL) divergence by S K L ( ⋅ , ⋅ ) 𝑆 𝐾 𝐿 ⋅ ⋅ SKL(\cdot,\cdot) . The latter is a symmetrized version of KL-divergence (Daskalakis, Dikkala and Kamath, 2018 ) . We use the following quantities for any matrix: (i) Frobenius norm, ∥ ⋅ ∥ F \|\cdot\|_{F} , is the root of sum of squares of all entries, (ii) max norm, ∥ ⋅ ∥ m a x \|\cdot\|_{max} , is largest absolute entry of the matrix, (iii) zero norm, ∥ ⋅ ∥ 0 \|\cdot\|_{0} , is the number of non-zero entries, (iv) operator norm, ∥ ⋅ ∥ o p \|\cdot\|_{op} , is the largest singular value of the matrix, and (v) row sum norm, ∥ ⋅ ∥ r o w \|\cdot\|_{row} , (or, the induced ∞ \infty -norm) is the maximum absolute row sum of the matrix.
2.1 The model and the testing problem
Throughout the paper, V = { 1 , 2 , … , n } 𝑉 1 2 … 𝑛 V=\{1,2,\ldots,n\} denotes a set of n 𝑛 n vertices, and we consider undirected graphs defined on V 𝑉 V . Any such graph can be expressed as G = ( V , E G ) 𝐺 𝑉 subscript 𝐸 𝐺 G=(V,E_{G}) , where E G subscript 𝐸 𝐺 E_{G} is the set of undirected edges. We use the symmetric matrix A G ∈ { 0 , 1 } n × n subscript 𝐴 𝐺 superscript 0 1 𝑛 𝑛 A_{G}\in\{0,1\}^{n\times n} to denote the adjacency matrix of G 𝐺 G , where ( A G ) i j = 1 subscript subscript 𝐴 𝐺 𝑖 𝑗 1 (A_{G})_{ij}=1 if ( i , j ) ∈ E G 𝑖 𝑗 subscript 𝐸 𝐺 (i,j)\in E_{G} , and 0 otherwise. The class of inhomogeneous random graphs, or more precisely inhomogeneous Erdős-Rényi (IER) graphs, on V 𝑉 V can be described as follows. Let 𝕄 n ⊂ [ 0 , 1 ] n × n subscript 𝕄 𝑛 superscript 0 1 𝑛 𝑛 \mathbb{M}_{n}\subset[0,1]^{n\times n} be the set of symmetric matrices with zero diagonal, and off-diagonal entries in [ 0 , 1 ] 0 1 [0,1] . For any P ∈ 𝕄 n 𝑃 subscript 𝕄 𝑛 P\in\mathbb{M}_{n} , we say that G 𝐺 G is an IER graph with population adjacency P 𝑃 P , denoted by G ∼ IER ( P ) similar-to 𝐺 IER 𝑃 G\sim\textup{IER}(P) , if the adjacency matrix A G subscript 𝐴 𝐺 A_{G} is a symmetric random matrix such that ( A G ) i j ∼ Bernoulli 0 − 1 ( P i j ) similar-to subscript subscript 𝐴 𝐺 𝑖 𝑗 subscript Bernoulli 0 1 subscript 𝑃 𝑖 𝑗 (A_{G})_{ij}\sim\text{Bernoulli}_{0-1}(P_{ij}) , and ( ( A G ) i j ) 1 ≤ i < j ≤ n subscript subscript subscript 𝐴 𝐺 𝑖 𝑗 1 𝑖 𝑗 𝑛 \left((A_{G})_{ij}\right)_{1\leq i<j\leq n} are independent.
for some specified distance function d 𝑑 d and a threshold ρ ≥ 0 𝜌 0 \rho\geq 0 . At this stage, note that the distribution IER ( P ) IER 𝑃 \textup{IER}(P) is completely characterised by the expected adjacency matrix P 𝑃 P . Hence, ℋ 0 subscript ℋ 0 \mathcal{H}_{0} in ( 1 ) is similar both under the mean difference alternative and the general difference alternative (Ramdas et al., 2015 ) . Hence, one may assume d 𝑑 d to be either a distance between the distributions IER ( P ) IER 𝑃 \textup{IER}(P) and IER ( Q ) IER 𝑄 \textup{IER}(Q) , or a matrix distance between P 𝑃 P and Q 𝑄 Q . Different examples of d 𝑑 d are considered in Sections 3 – 5 , which result in specific instances of the testing problems.
2.2 Minimax framework
which is the sum of maximum possible Type-I and Type-II error rates incurred by the test. Here, we use θ 𝜃 \theta to denote any tuple ( P , Q ) 𝑃 𝑄 (P,Q) . The minimax risk for the problem in ( 2 )–( 3 ) is defined as
3 Challenges of testing with small sample size
The theme of this paper is to test between two populations of sparse graphs, where the sample size m 𝑚 m is much smaller than the number of vertices n 𝑛 n . Our main interest is in cases where m 𝑚 m is a small constant, or may grow very slowly with n 𝑛 n . In this section, we show that in case of some popular distance functions, the testing problem is nearly unsolvable if m 𝑚 m is small.
We formalise the notion of unsolvability in the following way. For any instance of the two-sample testing problem ( 2 )–( 3 ), there is a trivial upper bound for ρ ∗ superscript 𝜌 \rho^{*} which is the maximal possible value that can be attained by d ( P , Q ) 𝑑 𝑃 𝑄 d(P,Q) (diameter with respect to d 𝑑 d ). As an example, if d ( P , Q ) = T V ( IER ( P ) , IER ( Q ) ) 𝑑 𝑃 𝑄 𝑇 𝑉 IER 𝑃 IER 𝑄 d(P,Q)=TV\big{(}\textup{IER}(P),\textup{IER}(Q)\big{)} , then ρ ∗ ≤ 1 superscript 𝜌 1 \rho^{*}\leq 1 trivially. Similarly, if d ( P , Q ) = ‖ P − Q ‖ F 𝑑 𝑃 𝑄 subscript norm 𝑃 𝑄 𝐹 d(P,Q)=\|P-Q\|_{F} , a trivial upper bound is ρ ∗ ≤ n δ superscript 𝜌 𝑛 𝛿 \rho^{*}\leq n\delta . On the other hand, for small m 𝑚 m , if there is a lower bound ρ ℓ ≤ ρ ∗ subscript 𝜌 ℓ superscript 𝜌 \rho_{\ell}\leq\rho^{*} such that ρ ℓ subscript 𝜌 ℓ \rho_{\ell} is equal or close to the trivial upper bound, then there exist model pairs such that d ( P , Q ) 𝑑 𝑃 𝑄 d(P,Q) is nearly as large as the diameter and yet cannot be distinguished for small m 𝑚 m . Hence, we may conclude that the problem ( 2 )–( 3 ) with the specific choice of d 𝑑 d is unsolvable for small m 𝑚 m under a worst-case (minimax) analysis.
We present the first instance of such an impossibility result for the case of total variation distance. For any two probability mass functions p 𝑝 p and q 𝑞 q , defined on the space of undirected n 𝑛 n -vertex graphs,
where the summation is over all unweighted undirected graphs on n 𝑛 n vertices. We present the following minimax rate in the small sample regime.
Proposition 3.1 ( ρ ∗ superscript 𝜌 \rho^{*} for total variation distance) .
Consider the problem in ( 2 )–( 3 ) with d ( P , Q ) = T V ( IER ( P ) , IER ( Q ) ) 𝑑 𝑃 𝑄 𝑇 𝑉 IER 𝑃 IER 𝑄 d(P,Q)=TV\big{(}\textup{IER}(P),\textup{IER}(Q)\big{)} and δ ∈ ( 0 , 1 ) 𝛿 0 1 \delta\in(0,1) . Let η ∈ ( 0 , 1 ) 𝜂 0 1 \eta\in(0,1) be the allowable risk. For any δ ≥ C ′ ln n n 2 𝛿 superscript 𝐶 ′ 𝑛 superscript 𝑛 2 \delta\geq C^{\prime}\frac{\ln n}{n^{2}} ,
where C ∈ ( 0 , 1 ) 𝐶 0 1 C\in(0,1) and C ′ > 1 superscript 𝐶 ′ 1 C^{\prime}>1 are absolute constants.
In particular, for large n 𝑛 n and m ≲ n ln n less-than-or-similar-to 𝑚 𝑛 𝑛 m\lesssim\frac{n}{\ln n} , we have ρ ∗ ≈ 1 superscript 𝜌 1 \rho^{*}\approx 1 .
Proof sketch.
The main idea is to find an appropriate choice of ( P , Q ) 𝑃 𝑄 (P,Q) such that T V ( IER ( P ) , IER ( Q ) ) ≥ 1 − 1 n 𝑇 𝑉 IER 𝑃 IER 𝑄 1 1 𝑛 TV\big{(}\textup{IER}(P),\textup{IER}(Q)\big{)}\geq 1-\frac{1}{n} , and yet they cannot be distinguished using m ≲ n ln n less-than-or-similar-to 𝑚 𝑛 𝑛 m\lesssim\frac{n}{\ln n} samples. For this, we use standard approaches to derive minimax lower bounds (Baraud, 2002 ) . This is described in the present context of two-sample testing of IER graphs in the appendix.
𝛿 2 𝛾 \{\frac{\delta}{2}-\gamma,\frac{\delta}{2}+\gamma\} . An appropriate choice of γ ≤ δ 2 𝛾 𝛿 2 \gamma\leq\frac{\delta}{2} leads to the result. ∎
The stated lower bound shows that at least m ≳ n ln n greater-than-or-equivalent-to 𝑚 𝑛 𝑛 m\gtrsim\frac{n}{\ln n} samples are needed to test for separation in total variation distance, which is beyond the small sample regime that we are interested in. In fact, in the case of constant m 𝑚 m , one can improve the stated bound as 1 − e − C ′′ n ≤ ρ ∗ ≤ 1 1 superscript 𝑒 superscript 𝐶 ′′ 𝑛 superscript 𝜌 1 1-e^{-C^{\prime\prime}n}\leq\rho^{*}\leq 1 , where C ′′ superscript 𝐶 ′′ C^{\prime\prime} is a constant that depends on m 𝑚 m .
An impossibility result also holds in the case of symmetric KL-divergence, which has been effectively used for high dimensional discrete distributions, particularly Ising models (Daskalakis, Dikkala and Kamath, 2018 ) . For any two probability mass functions p 𝑝 p and q 𝑞 q , defined on the space of undirected n 𝑛 n -vertex graphs, the symmetric KL-divergence is given by
where the summation is over all unweighted undirected graphs on n 𝑛 n vertices. Note that the above distance is unbounded even for finite n 𝑛 n since S K L ( p , q ) = ∞ 𝑆 𝐾 𝐿 𝑝 𝑞 SKL(p,q)=\infty if there exists G 0 subscript 𝐺 0 G_{0} such that p ( G 0 ) = 0 𝑝 subscript 𝐺 0 0 p(G_{0})=0 but q ( G 0 ) ≠ 0 𝑞 subscript 𝐺 0 0 q(G_{0})\neq 0 . We present the following result that demonstrates the impossibility of testing with respect to the symmetric KL-divergence when sample size m 𝑚 m is small.
Proposition 3.2 ( ρ ∗ superscript 𝜌 \rho^{*} for symmetric KL-divergence) .
Let η ∈ ( 0 , 1 ) 𝜂 0 1 \eta\in(0,1) and consider the problem in ( 2 )–( 3 ) with d ( P , Q ) = S K L ( IER ( P ) , IER ( Q ) ) 𝑑 𝑃 𝑄 𝑆 𝐾 𝐿 IER 𝑃 IER 𝑄 d(P,Q)=SKL\big{(}\textup{IER}(P),\textup{IER}(Q)\big{)} and any δ ∈ ( 0 , 1 ) 𝛿 0 1 \delta\in(0,1) . Then
The basic technique for the proof is similar to Proposition 3.1 , but we choose P 𝑃 P and Q 𝑄 Q such that S K L ( IER ( P ) , IER ( Q ) ) = ∞ 𝑆 𝐾 𝐿 IER 𝑃 IER 𝑄 SKL\big{(}\textup{IER}(P),\textup{IER}(Q)\big{)}=\infty , and yet the models are indistinguishable for small m 𝑚 m .
To be precise, under ℋ 0 subscript ℋ 0 \mathcal{H}_{0} we set P = Q 𝑃 𝑄 P=Q corresponding to an ER model. Under ℋ 1 subscript ℋ 1 \mathcal{H}_{1} , we set Q 𝑄 Q to be the same as P 𝑃 P except for a randomly chosen entry, for which Q i j = 0 ≠ P i j subscript 𝑄 𝑖 𝑗 0 subscript 𝑃 𝑖 𝑗 Q_{ij}=0\neq P_{ij} . This implies that IER ( P ) IER 𝑃 \textup{IER}(P) and IER ( Q ) IER 𝑄 \textup{IER}(Q) do not have a common support, which leads to S K L ( IER ( P ) , IER ( Q ) ) = ∞ 𝑆 𝐾 𝐿 IER 𝑃 IER 𝑄 SKL\big{(}\textup{IER}(P),\textup{IER}(Q)\big{)}=\infty . However, if the sample size is small (small m 𝑚 m ) or the graphs are sparse (small δ 𝛿 \delta ), then the models cannot be distinguished. ∎
The above result shows that testing for separation in symmetric KL-divergence is impossible for m ≲ ln n less-than-or-similar-to 𝑚 𝑛 m\lesssim\ln n sample even when the graphs are dense ( δ ≍ 1 ) asymptotically-equals 𝛿 1 (\delta\asymp 1) . However, the situation is worse in the case of sparse graphs. If δ ≍ 1 n asymptotically-equals 𝛿 1 𝑛 \delta\asymp\frac{1}{n} , then at least m ≳ n ln n greater-than-or-equivalent-to 𝑚 𝑛 𝑛 m\gtrsim n\ln n samples are necessary, which is worse than the condition for total variation distance.
Both Propositions 3.1 and 3.2 suggest that achieving a small sample complexity (small m 𝑚 m ) could be difficult under general difference alternatives, that is, if d 𝑑 d corresponds to distributional distances. Hence, subsequent discussions focus only on matrix distances. However, even in this case, the two-sample problem is not necessarily easily solvable for all distances or dissimilarities.
Proposition 3.3 ( ρ ∗ superscript 𝜌 \rho^{*} for zero norm / effect rarity) .
Let η ∈ ( 0 , 1 ) 𝜂 0 1 \eta\in(0,1) and consider the problem in ( 2 )–( 3 ) with d ( P , Q ) = ‖ P − Q ‖ 0 𝑑 𝑃 𝑄 subscript norm 𝑃 𝑄 0 d(P,Q)=\|P-Q\|_{0} and any δ ∈ ( 0 , 1 ) 𝛿 0 1 \delta\in(0,1) . Then
The proof is straightforward since the entries P 𝑃 P and Q 𝑄 Q can be arbitrarily close but still be unequal. Hence, the models may not be distinguishable though ‖ P − Q ‖ 0 = n ( n − 1 ) subscript norm 𝑃 𝑄 0 𝑛 𝑛 1 \|P-Q\|_{0}=n(n-1) , which is the trivial upper bound. ∎
Proposition 3.3 may be viewed as a trivial extremity of the rare/weak effect studied in the context of multiple testing (Donoho and Jin, 2015 ) . To put it simply, here we view the problem as testing P i j = Q i j subscript 𝑃 𝑖 𝑗 subscript 𝑄 𝑖 𝑗 P_{ij}=Q_{ij} or P i j ≠ Q i j subscript 𝑃 𝑖 𝑗 subscript 𝑄 𝑖 𝑗 P_{ij}\neq Q_{ij} for every i < j 𝑖 𝑗 i<j , and the edge independence in IER graphs leads to a problem of multiple independent comparisons. Proposition 3.3 states that if min P i j ≠ Q i j | P i j − Q i j | subscript subscript 𝑃 𝑖 𝑗 subscript 𝑄 𝑖 𝑗 subscript 𝑃 𝑖 𝑗 subscript 𝑄 𝑖 𝑗 \min\limits_{P_{ij}\neq Q_{ij}}|P_{ij}-Q_{ij}| is arbitrarily small, that is, the individual effects are arbitrarily weak, then they cannot be detected even when the effects are dense ‖ P − Q ‖ 0 = n ( n − 1 ) subscript norm 𝑃 𝑄 0 𝑛 𝑛 1 \|P-Q\|_{0}=n(n-1) . A more detailed analysis of the rare/weak effect in the sparse Bernoulli setting may be done by imposing a threshold min P i j ≠ Q i j | P i j − Q i j | subscript subscript 𝑃 𝑖 𝑗 subscript 𝑄 𝑖 𝑗 subscript 𝑃 𝑖 𝑗 subscript 𝑄 𝑖 𝑗 \min\limits_{P_{ij}\neq Q_{ij}}|P_{ij}-Q_{ij}| that characterises weakness of the effect. We do not discuss further on this effect, and instead, we proceed to other instances of ( 2 )–( 3 ), where the problem can be solved for small m 𝑚 m .
4 Testing for separation in Frobenius norm
The previous section focused on impossibility results, where ρ ∗ superscript 𝜌 \rho^{*} is typically large (close to trivial upper bound) when m 𝑚 m is small. In this section and the next one, we study two instances of the problem ( 2 )–( 3 ) where tests can be constructed even for small m 𝑚 m . Formally, we show that the lower bound for ρ ∗ superscript 𝜌 \rho^{*} can be much smaller than the trivial upper bound, and subsequently, we propose two-sample tests to derive nearly matching upper bounds for ρ ∗ superscript 𝜌 \rho^{*} .
We first quantify the separation in terms of Frobenius norm, that is, d ( P , Q ) = ‖ P − Q ‖ F 𝑑 𝑃 𝑄 subscript norm 𝑃 𝑄 𝐹 d(P,Q)=\|P-Q\|_{F} . This is equivalent to viewing the adjacencies as ( n 2 ) binomial 𝑛 2 \binom{n}{2} -dimensional Bernoulli vectors, and using two-sample test for high dimensional vectors — a well-studied problem in the Gaussian case (Chen and Qin, 2010 ) . We state the following bounds for the minimax separation ρ ∗ superscript 𝜌 \rho^{*} .
Theorem 4.1 ( ρ ∗ superscript 𝜌 \rho^{*} for Frobenius norm separation) .
n δ 4 ≤ ρ ∗ ≤ n δ 𝑛 𝛿 4 superscript 𝜌 𝑛 𝛿 \displaystyle\frac{n\delta}{4}\leq\rho^{*}\leq n\delta\; for m = 1 𝑚 1 m=1 ,
( 1 4 ⋀ η 2 ℓ η 8 C 1 ) n δ ≤ ρ ∗ ≤ n δ 1 4 superscript 𝜂 2 subscript ℓ 𝜂 8 subscript 𝐶 1 𝑛 𝛿 superscript 𝜌 𝑛 𝛿 \displaystyle\bigg{(}\frac{1}{4}\bigwedge\sqrt{\frac{\eta^{2}\ell_{\eta}}{8C_{1}}}\bigg{)}n\delta\leq\rho^{*}\leq n\delta\; for m > 1 𝑚 1 m>1 and δ ≤ C 1 η 2 m n 𝛿 subscript 𝐶 1 superscript 𝜂 2 𝑚 𝑛 \delta\leq\displaystyle\frac{C_{1}}{\eta^{2}mn} , and
ℓ η 8 n δ m ≤ ρ ∗ ≤ C 2 η n δ m subscript ℓ 𝜂 8 𝑛 𝛿 𝑚 superscript 𝜌 subscript 𝐶 2 𝜂 𝑛 𝛿 𝑚 \displaystyle\sqrt{\frac{\ell_{\eta}}{8}\frac{n\delta}{m}}\leq\rho^{*}\leq\sqrt{\frac{C_{2}}{\eta}\frac{n\delta}{m}}\; for m > 1 𝑚 1 m>1 and δ ≥ C 1 η 2 m n 𝛿 subscript 𝐶 1 superscript 𝜂 2 𝑚 𝑛 \delta\geq\displaystyle\frac{C_{1}}{\eta^{2}mn}\; ,
1 4 superscript 1 𝜂 2 \ell_{\eta}=\sqrt{\ln\left(1+4(1-\eta)^{2}\right)} . Hence, assuming the allowable risk η 𝜂 \eta is fixed, we have ρ ∗ ≍ n δ asymptotically-equals superscript 𝜌 𝑛 𝛿 \rho^{*}\asymp n\delta for m = 1 𝑚 1 m=1 and ρ ∗ ≍ n δ ∧ n δ m asymptotically-equals superscript 𝜌 𝑛 𝛿 𝑛 𝛿 𝑚 \rho^{*}\asymp n\delta\wedge\sqrt{\frac{n\delta}{m}}\, for m ≥ 2 𝑚 2 m\geq 2 .
Theorem 4.1 provides a clear characterisation of the minimax separation ρ ∗ superscript 𝜌 \rho^{*} (up to factors of η 𝜂 \eta ) when the distance between models is in terms of Frobenius norm. The second and third statements deal with the case of m > 1 𝑚 1 m>1 . In the ultra-sparse regime, that is δ ≲ 1 m n less-than-or-similar-to 𝛿 1 𝑚 𝑛 \delta\lesssim\frac{1}{mn} , one observes a total of only O ( n ) 𝑂 𝑛 O(n) edges from the entire population of 2 m 2 𝑚 2m graphs generated from either models. This information is insufficient for testing equality of models, and hence, it is not surprising that ρ ∗ ≍ n δ asymptotically-equals superscript 𝜌 𝑛 𝛿 \rho^{*}\asymp n\delta , which is the trivial upper bound. On the other hand, when δ ≳ 1 m n greater-than-or-equivalent-to 𝛿 1 𝑚 𝑛 \delta\gtrsim\frac{1}{mn} , we find a non-trivial separation rate indicating that the problem is solvable in this case.
The surprising finding of Theorem 4.1 is that ρ ∗ ≍ n δ asymptotically-equals superscript 𝜌 𝑛 𝛿 \rho^{*}\asymp n\delta for m = 1 𝑚 1 m=1 , which informally means that the problem is not solvable when one observes only m = 1 𝑚 1 m=1 sample from each model. This result is significant since it shows that the problem of testing for separation in Frobenius norm is unsuitable in the setting of comparing between two large networks, for instance, the case of testing between two omics networks.
4.1 Proof of Theorem 4.1
We provide an outline of the proof of the above result highlighting the key technical lemmas. We sketch their proofs here, and the detailed proofs can be found in the appendix. To prove the lower bounds, we have the following result.
Lemma 4.2 (Necessary conditions for detecting Frobenius norm separation) .
For the testing problem ( 2 )–( 3 ) with d ( P , Q ) = ‖ P − Q ‖ F 𝑑 𝑃 𝑄 subscript norm 𝑃 𝑄 𝐹 d(P,Q)=\|P-Q\|_{F} and for any η ∈ ( 0 , 1 ) 𝜂 0 1 \eta\in(0,1) , the minimax risk ( 4 ) is at least η 𝜂 \eta if either of the following conditions hold:
𝛿 2 𝛾 \{\frac{\delta}{2}-\gamma,\frac{\delta}{2}+\gamma\} . One can easily see that Q 𝑄 Q is chosen uniformly from a set of 2 n ( n − 1 ) / 2 superscript 2 𝑛 𝑛 1 2 2^{n(n-1)/2} matrices, but for each choice of Q 𝑄 Q , we have ‖ P − Q ‖ F ≈ n γ subscript norm 𝑃 𝑄 𝐹 𝑛 𝛾 \|P-Q\|_{F}\approx n\gamma . Hence, a choice of γ ∈ ( ρ n , δ 2 ] 𝛾 𝜌 𝑛 𝛿 2 \gamma\in\left(\frac{\rho}{n},\frac{\delta}{2}\right] implies that the pair of ( P , Q ) 𝑃 𝑄 (P,Q) for every choice of Q 𝑄 Q lies in Ω 1 subscript Ω 1 \Omega_{1} .
Subsequently, we use the techniques of Baraud ( 2002 ) to show that for m ≥ 2 𝑚 2 m\geq 2 and δ ≳ 1 m n greater-than-or-equivalent-to 𝛿 1 𝑚 𝑛 \delta\gtrsim\frac{1}{mn} , there is an appropriate choice γ < δ 2 𝛾 𝛿 2 \gamma<\frac{\delta}{2} for which the random choice of ( P , Q ) ∈ Ω 1 𝑃 𝑄 subscript Ω 1 (P,Q)\in\Omega_{1} cannot be distinguished from the null case. If δ ≲ 1 m n less-than-or-similar-to 𝛿 1 𝑚 𝑛 \delta\lesssim\frac{1}{mn} , then the same situation occurs even for the choice γ = δ 2 𝛾 𝛿 2 \gamma=\frac{\delta}{2} . Finally for m = 1 𝑚 1 m=1 , we observe that the same proof leads to the conclusion that the random choice of ( P , Q ) ∈ Ω 1 𝑃 𝑄 subscript Ω 1 (P,Q)\in\Omega_{1} is indistinguishable from the null case for any choice of γ ≤ δ 2 𝛾 𝛿 2 \gamma\leq\frac{\delta}{2} . In particular, γ = δ 2 𝛾 𝛿 2 \gamma=\frac{\delta}{2} leads to the claim in (ii).
and consider the test
Lemma 4.3 (Sufficient conditions for detecting Frobenius norm separation) .
then R ( Ψ F , n , m , d , ρ , δ ) ≤ η 𝑅 subscript Ψ 𝐹 𝑛 𝑚 𝑑 𝜌 𝛿 𝜂 R(\Psi_{F},n,m,d,\rho,\delta)\leq\eta .
The proof is based on concentration statements for μ ^ ^ 𝜇 \widehat{\mu} and σ ^ ^ 𝜎 \widehat{\sigma} derived via Chebyshev’s inequality using
𝑃 𝑄 𝐹 \|P+Q\|_{F} . For the type-I-error, i.e. under ℋ 0 subscript ℋ 0 \mathcal{H}_{0} , these concentration bounds lead to large enough choices for t 1 subscript 𝑡 1 t_{1} and t 2 subscript 𝑡 2 t_{2} such that at least one of the events
has small probability, which bounds the probability of the event { Ψ F = 1 } subscript Ψ 𝐹 1 \{\Psi_{F}=1\} . On the other hand, by construction, in order to control the type-II-error rate we want to ensure that both the events
have small probability under ℋ 1 subscript ℋ 1 \mathcal{H}_{1} . Now, the requirement in ( 8 ) on ρ 𝜌 \rho guarantees that
which allows us to rewrite these two events as large deviation statements of μ ^ ^ 𝜇 \widehat{\mu} and σ ^ 2 superscript ^ 𝜎 2 \widehat{\sigma}^{2} from their means as required. ∎
4.2 Further remarks on Theorem 4.1
As part of the proof of Theorem 4.1 , we propose a test in ( 7 ) which provides the non-trivial upper bound in the third statement of Theorem 4.1 . Though this bound matches the corresponding lower bound up to factors of η 𝜂 \eta , the difference is rather large with respect to η 𝜂 \eta . This is an artefact of the proof of Lemma 4.3 which is based on Chebyshev’s inequality, and its effect can also be seen in the two thresholds t 1 η subscript 𝑡 1 𝜂 \frac{t_{1}}{\sqrt{\eta}} and t 2 η 3 / 2 subscript 𝑡 2 superscript 𝜂 3 2 \frac{t_{2}}{\eta^{3/2}} defined in ( 7 ), which can be very high for small η 𝜂 \eta limiting the practical usefulness of the test. Below, we show that this can be improved using more refined concentration inequalities, but provides a sufficient condition that is weaker by a factor of ln n 𝑛 \ln n .
Proposition 4.4 (Improving dependence on η 𝜂 \eta ) .
Consider the two sample problem of Theorem 4.1 and assume m ≥ 2 𝑚 2 m\geq 2 . Define the test
Proof Sketch.
The proof is very close in spirit to that of Lemma 4.3 above, but it is based on a much more involved concentration statement than Chebyshev’s inequality (stated in the appendix). As a result, the test is based on the same test statistic μ ^ σ ^ ^ 𝜇 ^ 𝜎 \frac{\widehat{\mu}}{\widehat{\sigma}} and differs from ( 7 ) only in the choice of thresholds.
More specifically, due to the fact that μ ^ ^ 𝜇 \widehat{\mu} and σ ^ 2 superscript ^ 𝜎 2 \widehat{\sigma}^{2} are sums of products of sums with strong independence properties, we can derive concentration inequalities with logarithmic dependence on η 𝜂 \eta for them by repeated application of Bernstein’s inequality. However, this comes with the additional ln n 𝑛 \ln n factor which can be seen in equation ( 10 ) above. ∎
A key feature of both tests is that they are adaptive, that is, they do not require specification of the sparsity parameter δ 𝛿 \delta . We highlight the importance of this property in the following remark.
Remark 4.5 (Adaptivity of proposed tests) .
𝑃 𝑄 𝐹 \|P+Q\|_{F} , which is a lower bound for 2 n δ 2 𝑛 𝛿 2n\delta .
5 Testing for separation in operator norm
In this section, we study the two sample testing problem where d ( P , Q ) = ‖ P − Q ‖ o p 𝑑 𝑃 𝑄 subscript norm 𝑃 𝑄 𝑜 𝑝 d(P,Q)=\|P-Q\|_{op} , and provide bounds on the minimax separation ρ ∗ superscript 𝜌 \rho^{*} for all m ≥ 1 𝑚 1 m\geq 1 . An interesting finding of this section is that one can obtain a non-trivial minimax separation rate even for m = 1 𝑚 1 m=1 , that is, the problem is indeed solvable even with a single observation from each model. Our main result is the following.
Theorem 5.1 ( ρ ∗ superscript 𝜌 \rho^{*} for operator norm separation) .
- ′ 2 𝜂 16 𝑚 𝑛 \delta\leq\displaystyle\frac{\ell^{\prime 2}_{\eta}}{16mn}\; , and
ℓ η ′ n δ m ≤ ρ ∗ ≤ n δ m ( C ln ( n η ) ⋁ 4 C ′ ℓ η ′ ln ( n η ) ) subscript superscript ℓ ′ 𝜂 𝑛 𝛿 𝑚 superscript 𝜌 𝑛 𝛿 𝑚 𝐶 𝑛 𝜂 4 superscript 𝐶 ′ subscript superscript ℓ ′ 𝜂 𝑛 𝜂 \displaystyle\ell^{\prime}_{\eta}\sqrt{\frac{n\delta}{m}}\leq\rho^{*}\leq\sqrt{\frac{n\delta}{m}}\left(C\sqrt{\ln\left(\frac{n}{\eta}\right)}~{}\bigvee~{}\frac{4C^{\prime}}{\ell^{\prime}_{\eta}}\ln\left(\frac{n}{\eta}\right)\right)\; otherwise.
The theorem shows that the problem is not solvable in the ultra-sparse regime, that is, δ ≲ 1 m n less-than-or-similar-to 𝛿 1 𝑚 𝑛 \delta\lesssim\frac{1}{mn} . However, beyond this regime there is a non-trivial separation rate, which Theorem 5.1 finds up to a factor of ln n 𝑛 \ln n . It is natural to ask whether the additional logarithmic factor is necessary. Later in this section, we refine Theorem 5.1 to remove the ln n 𝑛 \ln n term in the upper bound (see Corollary 5.5 ). This is achieved by using a non-adaptive test, which has prior knowledge of δ 𝛿 \delta (see Proportion 5.4 ).
5.1 Proof of Theorem 5.1
The lower bounds in the theorem are due to the following necessary condition.
Lemma 5.2 (Necessary condition for detecting operator norm separation) .
For the testing problem ( 2 )–( 3 ) with d ( P , Q ) = ‖ P − Q ‖ o p 𝑑 𝑃 𝑄 subscript norm 𝑃 𝑄 𝑜 𝑝 d(P,Q)=\|P-Q\|_{op} , δ ∈ ( 0 , 1 ) 𝛿 0 1 \delta\in(0,1) and m ≥ 1 𝑚 1 m\geq 1 , and for any η ∈ ( 0 , 1 ) 𝜂 0 1 \eta\in(0,1) , the minimax risk ( 4 ) is at least η 𝜂 \eta if
One can see that for each choice of Q 𝑄 Q , we have ‖ P − Q ‖ o p = γ ( n − 1 ) subscript norm 𝑃 𝑄 𝑜 𝑝 𝛾 𝑛 1 \|P-Q\|_{op}=\gamma(n-1) . Hence, a choice of γ ∈ ( ρ n − 1 , δ 2 ] 𝛾 𝜌 𝑛 1 𝛿 2 \gamma\in\left(\frac{\rho}{n-1},\frac{\delta}{2}\right] implies that the pair of ( P , Q ) 𝑃 𝑄 (P,Q) for every choice of Q 𝑄 Q lies in Ω 1 subscript Ω 1 \Omega_{1} . Now similar to the proof of Lemma 4.2 , we show for any m ≥ 1 𝑚 1 m\geq 1 and δ ≳ 1 m n greater-than-or-equivalent-to 𝛿 1 𝑚 𝑛 \delta\gtrsim\frac{1}{mn} , there is an appropriate choice γ < δ 2 𝛾 𝛿 2 \gamma<\frac{\delta}{2} for which the random choice of ( P , Q ) ∈ Ω 1 𝑃 𝑄 subscript Ω 1 (P,Q)\in\Omega_{1} cannot be distinguished from the null case. If δ ≲ 1 m n less-than-or-similar-to 𝛿 1 𝑚 𝑛 \delta\lesssim\frac{1}{mn} , then the same situation occurs even for the choice γ = δ 2 𝛾 𝛿 2 \gamma=\frac{\delta}{2} , which leads to the claim. ∎
We now prove the upper bounds in Theorem 5.1 . For this, we note that ‖ P − Q ‖ o p ≤ ‖ P − Q ‖ r o w ≤ n δ subscript norm 𝑃 𝑄 𝑜 𝑝 subscript norm 𝑃 𝑄 𝑟 𝑜 𝑤 𝑛 𝛿 \|P-Q\|_{op}\leq\|P-Q\|_{row}\leq n\delta is the trivial upper bound for ρ ∗ superscript 𝜌 \rho^{*} . To prove the non-trivial upper bound, we consider the following test:
We have the following sufficient condition for the two-sample test Ψ o p subscript Ψ 𝑜 𝑝 \Psi_{op} .
Lemma 5.3 (Sufficient condition for detecting operator norm separation) .
The proof mainly controls the type-I and type-II error rates for Ψ o p subscript Ψ 𝑜 𝑝 \Psi_{op} . We achieve this through the matrix Bernstein inequality (Tropp, 2012 ; Oliveira, 2009 ) , which in the present case guarantees that
𝑟 𝑜 𝑤 𝑛 \|S^{+}\|_{row}\lesssim\ln n otherwise.
We bound the type-I error by noting that under ℋ 0 subscript ℋ 0 \mathcal{H}_{0} , that is P = Q 𝑃 𝑄 P=Q , the above concentration results imply that with high probability:
𝑃 𝑄 𝑟 𝑜 𝑤 𝑛 𝑚 \|P+Q\|_{row}\gtrsim\frac{\ln n}{m} , and
𝑃 𝑄 𝑟 𝑜 𝑤 𝑛 𝑚 \|P+Q\|_{row}\lesssim\frac{\ln n}{m} .
On the other hand, we control the type-II error in the following way. Under ℋ 1 subscript ℋ 1 \mathcal{H}_{1} , we have ‖ P − Q ‖ o p > ρ subscript norm 𝑃 𝑄 𝑜 𝑝 𝜌 \|P-Q\|_{op}>\rho with ρ ≥ C ′ m ln ( n η ) 𝜌 superscript 𝐶 ′ 𝑚 𝑛 𝜂 \rho\geq\frac{C^{\prime}}{m}\ln\left(\frac{n}{\eta}\right) , which implies that
So the second indicator in Ψ o p subscript Ψ 𝑜 𝑝 \Psi_{op} is guaranteed to be 1 for C ′ superscript 𝐶 ′ C^{\prime} large enough. This condition also allows us to use the matrix Bernstein inequality which, along with the reverse triangle inequality, gives
𝑃 𝑄 𝑟 𝑜 𝑤 \|S^{+}\|_{row}\asymp m\|P+Q\|_{row} to prove that the first indicator in Ψ o p subscript Ψ 𝑜 𝑝 \Psi_{op} is also true with high probability for large enough C 𝐶 C . This bounds the type-II error rate. ∎
5.2 An optimal non-adaptive test
One of the objectives of this work is to determine whether it is possible to test between two large graphs, that is, the case of m = 1 𝑚 1 m=1 . Theorem 5.1 provides an affirmative answer to this question, but our proposed test Ψ o p subscript Ψ 𝑜 𝑝 \Psi_{op} ( 11 ) is not optimal since the sufficient condition on ρ 𝜌 \rho is worse than the necessary condition by a factor of ln n 𝑛 \ln n . As we note above, this is a consequence of our use of matrix Bernstein inequality in the proof of Lemma 5.3 , and leads to the question whether one can improve the result by using more sharp concentration techniques known in the case of random graphs. We show that this is indeed true, at least for m = 1 𝑚 1 m=1 , and can be shown using concentration of trimmed or regularised adjacency matrices (Le, Levina and Vershynin, 2017 ) .
Assume m = 1 𝑚 1 m=1 , and let G ∼ IER ( P ) similar-to 𝐺 IER 𝑃 G\sim\textup{IER}(P) and H ∼ IER ( Q ) similar-to 𝐻 IER 𝑄 H\sim\textup{IER}(Q) be the two random graphs. Also assume that δ 𝛿 \delta is specified. For some constant c ≥ 6 𝑐 6 c\geq 6 , let A G ′ subscript superscript 𝐴 ′ 𝐺 A^{\prime}_{G} be the adjacency matrix of the graph obtained by deleting all edges in G 𝐺 G that are incident on vertices with degree larger than c n δ ln ( 2 η ) 𝑐 𝑛 𝛿 2 𝜂 cn\delta\ln(\frac{2}{\eta}) . Similarly, we obtain A H ′ subscript superscript 𝐴 ′ 𝐻 A^{\prime}_{H} from H 𝐻 H . Define a test as
for some constant t > 0 𝑡 0 t>0 . We have the following guarantee for Ψ o p ′ subscript superscript Ψ ′ 𝑜 𝑝 \Psi^{\prime}_{op} .
Proposition 5.4 (Optimality for m = 1 𝑚 1 m=1 ) .
Hence, assuming η 𝜂 \eta is fixed, ρ ∗ ≍ n δ asymptotically-equals superscript 𝜌 𝑛 𝛿 \rho^{*}\asymp\sqrt{n\delta} for δ > 10 n 𝛿 10 𝑛 \delta>\frac{10}{n} whereas ρ ∗ ≍ n δ asymptotically-equals superscript 𝜌 𝑛 𝛿 \rho^{*}\asymp n\delta for δ ≤ 10 n 𝛿 10 𝑛 \delta\leq\frac{10}{n} .
The proof differs from that of Lemma 5.3 in the use of a different matrix concentration inequality. We rely on the concentration of the trimmed adjacency matrix (Le, Levina and Vershynin, 2017 ) . In the present context, the result states that for δ > 10 n 𝛿 10 𝑛 \delta>\frac{10}{n} and G ∼ IER ( P ) similar-to 𝐺 IER 𝑃 G\sim\textup{IER}(P) with ‖ P ‖ m a x ≤ δ subscript norm 𝑃 𝑚 𝑎 𝑥 𝛿 \|P\|_{max}\leq\delta ,
with probability 1 − η 4 1 𝜂 4 1-\frac{\eta}{4} for some absolute constant C > 0 𝐶 0 C>0 .
For P = Q 𝑃 𝑄 P=Q , we have with probability 1 − η 2 1 𝜂 2 1-\frac{\eta}{2} ,
and hence, setting t = 2 C 𝑡 2 𝐶 t=2C ensures that the type-I error rate is smaller than η 2 𝜂 2 \frac{\eta}{2} . Similarly, it follows that under ℋ 1 subscript ℋ 1 \mathcal{H}_{1} ,
with probability 1 − η 2 1 𝜂 2 1-\frac{\eta}{2} . Hence, ‖ A G ′ − A H ′ ‖ o p subscript norm subscript superscript 𝐴 ′ 𝐺 subscript superscript 𝐴 ′ 𝐻 𝑜 𝑝 \|A^{\prime}_{G}-A^{\prime}_{H}\|_{op} exceeds the threshold for ρ > 4 C n δ ln 2 ( 2 η ) 𝜌 4 𝐶 𝑛 𝛿 superscript 2 2 𝜂 \rho>4C\sqrt{n\delta}\ln^{2}\left(\frac{2}{\eta}\right) , and we have a similar bound on the type-II error rate. ∎
While Ψ o p ′ subscript superscript Ψ ′ 𝑜 𝑝 \Psi^{\prime}_{op} indeed achieves optimality, it comes at the cost of prior knowledge of δ 𝛿 \delta . As we note in Remark 4.5 , this is an unreasonable restriction and hence, the sub-optimal test Ψ o p subscript Ψ 𝑜 𝑝 \Psi_{op} ( 11 ) may be preferable due to its adaptivity. We do not know if an adaptive optimal operator norm based test can be constructed since existing concentration bounds for sparse IER graphs are typically in terms of the largest edge probability, which is hard to estimate.
5.3 Improving the bounds in Theorem 5.1
As discussed above, the non-adaptive test Ψ o p ′ subscript superscript Ψ ′ 𝑜 𝑝 \Psi^{\prime}_{op} helps in improving the upper bound on ρ ∗ superscript 𝜌 \rho^{*} for m = 1 𝑚 1 m=1 . We have not studied whether Ψ o p ′ subscript superscript Ψ ′ 𝑜 𝑝 \Psi^{\prime}_{op} ( 13 ) and Proposition 5.4 extend to the case of m > 1 𝑚 1 m>1 . However, the following corollary shows that the results of Sections 4 and 5 can be combined to remove the undesirable ln n 𝑛 \ln n factor in the upper bound of ρ ∗ superscript 𝜌 \rho^{*} in Theorem 5.1 for any m ≥ 1 𝑚 1 m\geq 1 . For convenience, let the allowable risk η 𝜂 \eta be a constant, which we ignore in the statement of the result.
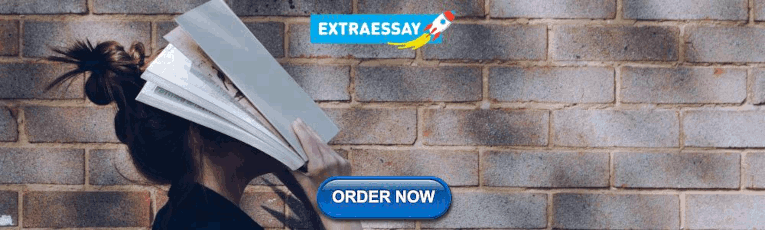
Corollary 5.5 (Improved bounds on ρ ∗ superscript 𝜌 \rho^{*} for operator norm separation) .
Consider the two-sample problem ( 2 )–( 3 ) with d ( P , Q ) = ‖ P − Q ‖ o p 𝑑 𝑃 𝑄 subscript norm 𝑃 𝑄 𝑜 𝑝 d(P,Q)=\|P-Q\|_{op} , and any m ≥ 1 𝑚 1 m\geq 1 , δ ∈ ( 0 , 1 ) 𝛿 0 1 \delta\in(0,1) . There exists a constant C > 0 𝐶 0 C>0 such that:
ρ ∗ ≍ n δ asymptotically-equals superscript 𝜌 𝑛 𝛿 \displaystyle\rho^{*}\asymp n\delta\; for δ ≤ C m n , 𝛿 𝐶 𝑚 𝑛 \delta\leq\displaystyle\frac{C}{mn}\;,\qquad and ρ ∗ ≍ n δ m asymptotically-equals superscript 𝜌 𝑛 𝛿 𝑚 \qquad\displaystyle\rho^{*}\asymp\sqrt{\frac{n\delta}{m}}\; otherwise.
The lower bounds on ρ ∗ superscript 𝜌 \rho^{*} follow from Theorem 5.1 , and hence it remains to prove that
We claim that this is a direct consequence of the upper bounds in Proposition 5.4 and Theorem 4.1 . To see this, consider the following test:
Ψ = Ψ o p ′ Ψ subscript superscript Ψ ′ 𝑜 𝑝 \Psi=\Psi^{\prime}_{op} for m = 1 𝑚 1 m=1 , and Ψ = Ψ F Ψ subscript Ψ 𝐹 \qquad\Psi=\Psi_{F} for m ≥ 2 𝑚 2 m\geq 2 .
For m = 1 𝑚 1 m=1 , Proposition 5.4 leads to the above stated upper bound.
For m ≥ 2 𝑚 2 m\geq 2 , note that given δ 𝛿 \delta and ρ 𝜌 \rho , the two-sample problems under Frobenius and operator norm separation have the same null set Ω 0 subscript Ω 0 \Omega_{0} ( 3 ), whereas the sets under alternative hypotheses are
We use the relation ‖ P − Q ‖ F ≥ ‖ P − Q ‖ o p subscript norm 𝑃 𝑄 𝐹 subscript norm 𝑃 𝑄 𝑜 𝑝 \|P-Q\|_{F}\geq\|P-Q\|_{op} to observe that Ω 1 o p ⊂ Ω 1 F superscript subscript Ω 1 𝑜 𝑝 superscript subscript Ω 1 𝐹 \Omega_{1}^{op}\subset\Omega_{1}^{F} . As a consequence, the maximum risk of Ψ F subscript Ψ 𝐹 \Psi_{F} under the two different distances can be related as
that is, if ρ 𝜌 \rho is fixed and Ψ F subscript Ψ 𝐹 \Psi_{F} is used for the problem of testing difference in operator norm, then it achieves a smaller worst-case risk than what it achieves for the the problem of testing difference in Frobenius norm. This implies that the minimax separation for operator norm is at most the minimax separation for Frobenius norm. The claim now follows from the upper bounds in Theorem 4.1 for m ≥ 2 𝑚 2 m\geq 2 . ∎
6 Discussion
In this section, we discuss related graph two-sample problems, and comment on practical two-sample tests.
6.1 A note on the sparsity condition
The notion of sparsity has no formal definition in the context of random graphs, unlike sparsity in the signal detection literature. The informal definition of a sparse graph is a graph where the number of edges are not arbitrarily large compared to the number of vertices n 𝑛 n . The sparsity condition used in ( 3 ), that is ‖ P ‖ m a x ≤ δ subscript norm 𝑃 𝑚 𝑎 𝑥 𝛿 \|P\|_{max}\leq\delta , is one approach to define sparse graphs. More precisely, this condition implies that the expected number of edges is at most n 2 δ superscript 𝑛 2 𝛿 n^{2}\delta , and in particular, for δ ≍ 1 n asymptotically-equals 𝛿 1 𝑛 \delta\asymp\frac{1}{n} , we obtain graphs where the number of edges grows linearly with n 𝑛 n . However, one can induce sparsity through alternative restrictions on P 𝑃 P :
among others. We note the δ i subscript 𝛿 𝑖 \delta_{i} ’s are of different order than δ 𝛿 \delta used in ( 3 ). The first condition bounds the expected number of edges, while condition (iii) provides graphs with bounded expected degrees. The last restriction is along the lines of the signal detection literature since it implies that at most δ 4 subscript 𝛿 4 \delta_{4} entries in P 𝑃 P are non-zero, and results in random graphs with absolutely bounded number of edges (not only in expectation).
It is natural to ask to whether our results also extend to the case where sparsity is controlled through any one of the conditions. We do not provide a complete characterisation in each case, but present two corollaries of Theorems 4.1 and 5.1 which show that some of our results easily extend to alternative notions of sparsity.
Corollary 6.1 ( ρ ∗ superscript 𝜌 \rho^{*} under Frobenius norm sparsity) .
Consider the problem of testing between the following two hypotheses sets
The bounds on minimax separation stated in Theorem 4.1 hold in this case with the substitution δ 2 = n δ subscript 𝛿 2 𝑛 𝛿 \delta_{2}=n\delta .
The proof of the corollary follows immediately from that of Theorem 4.1 . The substitution δ 2 = n δ subscript 𝛿 2 𝑛 𝛿 \delta_{2}=n\delta is a consequence of the relation ‖ P ‖ F ≤ n ‖ P ‖ m a x subscript norm 𝑃 𝐹 𝑛 subscript norm 𝑃 𝑚 𝑎 𝑥 \|P\|_{F}\leq n\|P\|_{max} . ∎
We also have a result in the case of graphs with bounded expected degrees, which can be similarly derived from Theorem 5.1 . We do not know if the more precise rates given in Corollary 5.5 can be extended to this setting.
Corollary 6.2 ( ρ ∗ superscript 𝜌 \rho^{*} under row sum norm sparsity) .
The bounds on minimax separation stated in Theorem 5.1 hold in this case with the substitution δ 3 = n δ subscript 𝛿 3 𝑛 𝛿 \delta_{3}=n\delta .
6.2 On the practicality of proposed tests
The theme of this paper has been to explore different separation criteria for which the graph two sample testing problem can be solved for a small population size. In the process of addressing this question, we suggest adaptive tests Ψ F subscript Ψ 𝐹 \Psi_{F} ( 7 ), Ψ F ′ subscript superscript Ψ ′ 𝐹 \Psi^{\prime}_{F} ( 9 ) and Ψ o p subscript Ψ 𝑜 𝑝 \Psi_{op} ( 11 ) that also turn out to be near-optimal for the problems of detecting separation in Frobenius or operator norms. However, the practical applicability of these tests have not been discussed so far.
We note that in practice, one is more interested in the testing problem P = Q 𝑃 𝑄 P=Q vs. P ≠ Q 𝑃 𝑄 P\neq Q , and hence, a more basic question that needs to be addressed is — Which separation criterion should be used? The findings of this paper suggest that for m = 1 𝑚 1 m=1 , operator norm separation is a possible choice, whereas other distances like total variation distance and Frobenius norm should not be considered. For m > 1 𝑚 1 m>1 but small, we show that one could detect separation in Frobenius norm using Ψ F subscript Ψ 𝐹 \Psi_{F} ( 7 ) or detect separation in operator norm using Ψ o p subscript Ψ 𝑜 𝑝 \Psi_{op} ( 11 ). We compare the relative merits of both tests in terms of sample complexity in the following way.
Remark 6.3 (Comparison between Ψ F subscript Ψ 𝐹 \Psi_{F} and Ψ o p subscript Ψ 𝑜 𝑝 \Psi_{op} ) .
However, the tests Ψ F subscript Ψ 𝐹 \Psi_{F} , Ψ F ′ subscript superscript Ψ ′ 𝐹 \Psi^{\prime}_{F} and Ψ o p subscript Ψ 𝑜 𝑝 \Psi_{op} require n 𝑛 n to be very large or the graphs to be dense to achieve a small risk, and hence have limited applicability in moderate-sized problems. It is known that the practical applicability of concentration based tests can be improved by using bootstrapped variants (Gretton et al., 2012 ) , which approximate the null distribution by generating bootstrap samples through random mixing of the two populations. Simulations, not included in this paper, show that permutation based bootstrapping provides a reasonable rejection rate for moderate sample size ( m ≥ 10 ) 𝑚 10 (m\geq 10) , but such bootstrapping is not effective for smaller m 𝑚 m , for instance m = 2 𝑚 2 m=2 . Furthermore, the relative merit Ψ F subscript Ψ 𝐹 \Psi_{F} (or Ψ F ′ subscript superscript Ψ ′ 𝐹 \Psi^{\prime}_{F} ) over Ψ o p subscript Ψ 𝑜 𝑝 \Psi_{op} , as suggested by Remark 6.3 , could not be verified in case of the bootstrapped variants.
When m = 1 𝑚 1 m=1 and the population adjacency is of low rank, Tang et al. ( 2017a ) suggest an alternative bootstrapping principle based on estimation of the population adjacency P 𝑃 P and then drawing bootstrap samples from estimate of P 𝑃 P . Simulations in Ghoshdastidar and von Luxburg ( 2018 ) show that this procedure works to some extent for dense IER graphs but only when P 𝑃 P has a small (known) rank. When the rank is unknown or, more generally, if P 𝑃 P does not have a low rank, such bootstrapped tests are not necessarily reliable.
6.3 Minimax separation under structural assumptions
The present paper studies the two-sample problem for IER graphs, where the population adjacency matrices do not have any structural restriction. In other words, we study a hypothesis testing problem in a dimension of ( n 2 ) binomial 𝑛 2 \binom{n}{2} with a sample size of m 𝑚 m . Under this broad framework, Theorem 4.1 shows that the minimax separation in Frobenius norm ρ F ∗ subscript superscript 𝜌 𝐹 \rho^{*}_{F} is given by
Similarly, Corollary 5.5 shows that the minimax separation in operator norm
It is natural to ask if these rates decrease if we further impose structural assumptions on the population adjacencies thereby effectively reducing the problem dimension. In this section, we provide some initial results in this direction. We impose the structural assumptions by restricting the possible values for the population adjacency matrices. Formally, we define 𝕄 ~ n ⊂ 𝕄 n subscript ~ 𝕄 𝑛 subscript 𝕄 𝑛 \widetilde{\mathbb{M}}_{n}\subset\mathbb{M}_{n} as the set of symmetric matrices in [ 0 , 1 ] n × n superscript 0 1 𝑛 𝑛 [0,1]^{n\times n} , whose diagonal entries are zero and satisfy additional structural assumptions (specified below). Subsequently, the graph two-sample problem, restricted to a special graph class, can be stated as the problem of testing between
where Ω 0 subscript Ω 0 \Omega_{0} and Ω 1 subscript Ω 1 \Omega_{1} are as defined in the original problem ( 2 )–( 3 ).
We begin with the most simple case of Erdős-Rényi (ER) graphs, where the restricted set 𝕄 ~ n subscript ~ 𝕄 𝑛 \widetilde{\mathbb{M}}_{n} corresponds to the symmetric matrices whose diagonal entries are zero and all off-diagonal entries are identical. Note that each distribution is modelled by a single parameter, and hence, we have a hypothesis testing problem in one dimension. We present following result on minimax separation for Frobenius and operator norms in this setting. We simplify the statement by ignoring absolute constants including the allowable risk η 𝜂 \eta , which is assumed to be fixed.
Proposition 6.4 (Minimax separation for testing ER graphs) .
In particular, in the sparsity regime δ ≳ 1 n 2 m greater-than-or-equivalent-to 𝛿 1 superscript 𝑛 2 𝑚 \delta\gtrsim\frac{1}{n^{2}m} , the minimax separation is much smaller than the corresponding rates for I E R 𝐼 𝐸 𝑅 IER graphs.
The proof is relatively simple, and borrows ideas from the Theorem 4.1 . Hence, we only provide a brief sketch of the proof.
𝑝 𝛾 \{p-\gamma,p+\gamma\} for some γ ≤ δ 2 𝛾 𝛿 2 \gamma\leq\frac{\delta}{2} . It turns out that γ ≍ δ 2 ∧ 1 n δ m asymptotically-equals 𝛾 𝛿 2 1 𝑛 𝛿 𝑚 \gamma\asymp\frac{\delta}{2}\wedge\frac{1}{n}\sqrt{\frac{\delta}{m}} is an appropriate choice, which corresponds to the claimed lower bound for minimax separation.
The upper bound is obtained by using a simple test that compares the edge densities estimated from the two population. Define a test
𝑛 2 n+2 parameters, and the problem has a dimension much smaller than ( n 2 ) binomial 𝑛 2 \binom{n}{2} -dimensional IER problem. However, the following result shows that the minimax separation does not decrease in this case compared to the general IER setting.
Proposition 6.5 (Minimax separation for testing 2-SBM graphs) .
For minimax separation in Frobenius norm ρ F ∗ subscript superscript 𝜌 𝐹 \rho^{*}_{F} , the above rate hold only for m ≥ 2 𝑚 2 m\geq 2 . For m = 1 𝑚 1 m=1 , we have loose bounds n δ ∧ n δ ≲ ρ F ∗ ≲ n δ less-than-or-similar-to 𝑛 𝛿 𝑛 𝛿 subscript superscript 𝜌 𝐹 less-than-or-similar-to 𝑛 𝛿 n\delta\wedge\sqrt{n\delta}\lesssim\rho^{*}_{F}\lesssim n\delta .
Hence, the minimax separation for 2-SBM is similar to the corresponding rates for I E R 𝐼 𝐸 𝑅 IER graphs (with possible exception of ρ F ∗ subscript superscript 𝜌 𝐹 \rho^{*}_{F} for m = 1 𝑚 1 m=1 ).
We first note that the testing problem is a restriction of the original IER testing problem, and hence, the upper bounds of ρ F ∗ subscript superscript 𝜌 𝐹 \rho^{*}_{F} and ρ o p ∗ subscript superscript 𝜌 𝑜 𝑝 \rho^{*}_{op} , derived in Theorem 4.1 and Corollary 5.5 respectively, also hold in this case. Hence, we only need to prove the lower bounds
for all m ≥ 1 𝑚 1 m\geq 1 . This lower bound on ρ o p ∗ subscript superscript 𝜌 𝑜 𝑝 \rho^{*}_{op} follows directly from the proof of Lemma 5.2 . Recall that the construction used to prove Lemma 5.2 was essentially a case of distinguishing a 2-SBM from an ER, where the latter is also a special case of a 2-SBM. Hence, the same proof works in the present case as well, and the claimed lower bound for ρ o p ∗ subscript superscript 𝜌 𝑜 𝑝 \rho^{*}_{op} holds.
The lower bound for ρ F ∗ subscript superscript 𝜌 𝐹 \rho^{*}_{F} also follows from the same construction and computing the Frobenius norm distance between the choice of population adjacency matrices used in proof of Lemma 5.2 . ∎
Proposition 6.5 may have important consequences since it apparently implies that for any model that is more complex than the simple 2-SBM, the two-sample problem is as difficult as the general setting of IER graphs. However, a more in-depth study may be required before a strong claim can be made in this context. For instance, one should take into account the fact that the broad literature on graph clustering and stochastic block model often require an additional assumption of balanced community size. It would be interesting to understand whether the presence of balanced communities also simplify the detection / testing problems.
In a broader context, one should note that Propositions 6.4 and 6.5 only provide minimax rates for the Frobenius and operator norm distances for these special classes of IER graphs. On the other hand, Proposition 3.1 and 3.2 demonstrate the limitation of distribution based distances when no restriction is imposed on IER model. Hence, there is a possibility that, under special models, total variation and K L 𝐾 𝐿 KL -divergence based tests are as useful or even better than Frobenius or operator norm based tests. Insights into these questions, along with minimax rates for related models such as k 𝑘 k -SBM and random dot product graphs, may provide a clear understanding of the problem of testing graphs on a common set of vertices.
6.4 Extensions
Several extensions of the two sample problem ( 2 )–( 3 ) can be studied. Earlier in this section, we have discussed the possibility of considering alternative notions of sparsity. Another interesting, and practically significant, extension is to the case of directed graphs. The problem naturally extends to this framework, and the proposed adaptive tests easily tackle this generalisation without any critical modification. For instance, in the case Ψ F subscript Ψ 𝐹 \Psi_{F} , one merely needs to define μ ^ ^ 𝜇 \widehat{\mu} and σ ^ ^ 𝜎 \widehat{\sigma} as a summation over all off-diagonal terms and the thresholds change only by constant factors. The analysis of such tests as well as the minimax lower bounds can be easily derived from our proofs. The same conclusion is true for the case of operator norm separation, particularly, when the upper bounds are derived based on Ψ o p subscript Ψ 𝑜 𝑝 \Psi_{op} and the matrix Bernstein inequality.
In this paper, we only consider the problem of identity testing, that is, P = Q 𝑃 𝑄 P=Q or d ( P , Q ) > ρ 𝑑 𝑃 𝑄 𝜌 d(P,Q)>\rho . One may also study the more general problem of closeness testing, which ignores small differences between the models, that is, one tests between the hypotheses
for some pre-specified ϵ < ρ italic-ϵ 𝜌 \epsilon<\rho . The proposed tests, which are primarily based on the principle of estimating d ( P , Q ) 𝑑 𝑃 𝑄 d(P,Q) may be easily adapted to this setting by appropriately modifying the test thresholds. However, it is not clear whether the minimax separation bounds in Theorems 4.1 and 5.1 easily extend to this setting as well.
From a practical perspective, one may face a more general problem of two sample graph testing, where the graphs are not defined on a common set of vertices and may even be of different sizes. This situation is generally hard to study, but tests for this problem are often used in many applications, where one typically computes some scalar or vector function from each graph and comments on the difference between two graph populations based on this function (Stam et al., 2007 ) . We study this principle in a recent work (Ghoshdastidar et al., 2017 ) , and propose a formal framework for testing between any two random graphs through the means of a network function f : 𝒢 ≥ n → ℳ : 𝑓 → subscript 𝒢 absent 𝑛 ℳ f:\mathcal{G}_{\geq n}\to\mathcal{M} that maps the space of graphs on at least n 𝑛 n vertices to some metric space ℳ ℳ \mathcal{M} . We argue that if the network function concentrates for some sub-class of random graphs as n → ∞ → 𝑛 n\to\infty , then one can indeed construct two sample tests based on the network function. However, such a test cannot distinguish between equivalence classes, that is, random graph models that behave identically under the mapping f 𝑓 f .
Appendix A Proof of results in Section 3
Consider the two sample problem in ( 2 )–( 3 ). Let θ 0 ∈ Ω 0 subscript 𝜃 0 subscript Ω 0 \theta_{0}\in\Omega_{0} be a particular instance satisfying the null hypothesis, and Θ 1 ⊂ Ω 1 subscript Θ 1 subscript Ω 1 \Theta_{1}\subset\Omega_{1} be a finite collection of instances satisfying ℋ 1 subscript ℋ 1 \mathcal{H}_{1} . We specify θ 0 subscript 𝜃 0 \theta_{0} and Θ 1 subscript Θ 1 \Theta_{1} later for each instance of the problem, but to prove a general lower bound, let θ 1 subscript 𝜃 1 \theta_{1} be uniformly selected from Θ 1 subscript Θ 1 \Theta_{1} . The minimax risk ( 4 ) can be bounded from below as
Let ℱ ℱ \mathcal{F} be the collection of all possible sets of 2 m 2 𝑚 2m graphs on n 𝑛 n vertices, and let F Ψ ⊂ ℱ subscript 𝐹 Ψ ℱ F_{\Psi}\subset\mathcal{F} be the sub-collection of those instances for which Ψ = 1 Ψ 1 \Psi=1 . Then, we can re-write above lower bound as
Here, ω ∈ ℱ 𝜔 ℱ \omega\in\mathcal{F} corresponds to a collection of 2 m 2 𝑚 2m graphs. The equality follows by observing that both 𝖯 θ 0 ( ⋅ ) subscript 𝖯 subscript 𝜃 0 ⋅ \mathsf{P}_{\theta_{0}}(\cdot) and 𝖤 θ 1 ∼ Unif ( Θ 1 ) [ 𝖯 θ 1 ( ⋅ ) ] subscript 𝖤 similar-to subscript 𝜃 1 Unif subscript Θ 1 delimited-[] subscript 𝖯 subscript 𝜃 1 ⋅ \mathsf{E}_{\theta_{1}\sim\text{Unif}(\Theta_{1})}[\mathsf{P}_{\theta_{1}}(\cdot)] define two measures on ℱ ℱ \mathcal{F} , and hence, the equality is due to equivalence of two definitions of total variation distance. The last step is a consequence of Cauchy-Schwarz inequality along with the observation that ∑ ω 𝖯 θ ( ω ) = 1 subscript 𝜔 subscript 𝖯 𝜃 𝜔 1 \sum_{\omega}\mathsf{P}_{\theta}(\omega)=1 for any θ 𝜃 \theta . Thus, to show that the minimax risk is larger than any η ∈ ( 0 , 1 ) 𝜂 0 1 \eta\in(0,1) , it suffices to show that for some θ 0 ∈ Ω 0 subscript 𝜃 0 subscript Ω 0 \theta_{0}\in\Omega_{0} and Θ 1 ⊂ Ω 1 subscript Θ 1 subscript Ω 1 \Theta_{1}\subset\Omega_{1} ,
A.1 Proof of Proposition 3.1
𝑝 𝛾 (p+\gamma) or ( p − γ ) 𝑝 𝛾 (p-\gamma) . Note that due to the symmetry of Q 𝑄 Q , there are exactly 2 n ( n − 1 ) / 2 superscript 2 𝑛 𝑛 1 2 2^{n(n-1)/2} elements in Θ 1 subscript Θ 1 \Theta_{1} .
𝑃 𝛾 italic-ϵ Q_{\epsilon}=P+\gamma\epsilon , where ϵ ∈ ℝ n × n italic-ϵ superscript ℝ 𝑛 𝑛 \epsilon\in\mathbb{R}^{n\times n} is symmetric with zero diagonal and off-diagonal entries as ± 1 plus-or-minus 1 \pm 1 . Recall that
where H 2 ( ⋅ , ⋅ ) superscript 𝐻 2 ⋅ ⋅ H^{2}(\cdot,\cdot) is the squared Hellinger distance. The summation is over all possible adjacency matrices and we use P ( A ) 𝑃 𝐴 P(A) to denote the probability mass at A 𝐴 A under the distribution IER ( P ) IER 𝑃 \textup{IER}(P) . We can write the above relation as
1 𝑥 2 superscript 𝑥 2 16 \sqrt{1+x}\leq 1+\frac{x}{2}-\frac{x^{2}}{16} and 1 − x ≤ 1 − x 2 − x 2 16 1 𝑥 1 𝑥 2 superscript 𝑥 2 16 \sqrt{1-x}\leq 1-\frac{x}{2}-\frac{x^{2}}{16} for all x ∈ [ − 1 , 1 ] 𝑥 1 1 x\in[-1,1] , which can be easily verified by squaring both sides. Using these bounds, we can write
Thus, ( P , Q ϵ ) ∈ Ω 1 𝑃 subscript 𝑄 italic-ϵ subscript Ω 1 (P,Q_{\epsilon})\in\Omega_{1} for every ϵ italic-ϵ \epsilon if γ > 1 n 32 δ ln ( 1 1 − ρ ) 𝛾 1 𝑛 32 𝛿 1 1 𝜌 \gamma>\frac{1}{n}\sqrt{32\delta\ln(\frac{1}{1-\rho})} . Let ρ = 1 − 1 n 𝜌 1 1 𝑛 \rho=1-\frac{1}{n} and γ = 8 δ ln n n 𝛾 8 𝛿 𝑛 𝑛 \gamma=\frac{8\sqrt{\delta\ln n}}{n} . The condition on δ 𝛿 \delta stated in the proposition ensures that γ ≤ δ 2 𝛾 𝛿 2 \gamma\leq\frac{\delta}{2} , and hence, Q ϵ subscript 𝑄 italic-ϵ Q_{\epsilon} is non-negative and ‖ Q ϵ ‖ m a x ≤ δ subscript norm subscript 𝑄 italic-ϵ 𝑚 𝑎 𝑥 𝛿 \|Q_{\epsilon}\|_{max}\leq\delta .
We now rely on the general technique for deriving lower bounds, and compute the quantity in ( 14 ) in the present case. Let ω ∈ ℱ 𝜔 ℱ \omega\in\mathcal{F} be the tuple ω = ( G 1 , … , G m , H 1 , … , H m ) 𝜔 subscript 𝐺 1 … subscript 𝐺 𝑚 subscript 𝐻 1 … subscript 𝐻 𝑚 \omega=(G_{1},\ldots,G_{m},H_{1},\ldots,H_{m}) , where we assume that the first m 𝑚 m graphs are generated from the first model, and the rest from the second model. Then
𝑝 𝛾 Q_{ij}=(p+\gamma) or ( p − γ ) 𝑝 𝛾 (p-\gamma) . Denoting the element by θ ϵ subscript 𝜃 italic-ϵ \theta_{\epsilon} , we have
Based on this, one can compute the quantity in ( 14 ) as
Pushing the summation over ω 𝜔 \omega inside the product and transforming it to a summation over every i < j 𝑖 𝑗 i<j , the above quantity corresponds to summing over possible values of ( S G ) i j subscript subscript 𝑆 𝐺 𝑖 𝑗 (S_{G})_{ij} and ( S H ) i j subscript subscript 𝑆 𝐻 𝑖 𝑗 (S_{H})_{ij} , where each of them can take the value k 𝑘 k in ( m k ) binomial 𝑚 𝑘 \binom{m}{k} ways. We obtain
1 subscript italic-ϵ 𝑖 𝑗 subscript superscript italic-ϵ ′ 𝑖 𝑗 superscript 𝛾 2 𝑝 1 𝑝 𝑚 \left(1+\frac{\epsilon_{ij}\epsilon^{\prime}_{ij}\gamma^{2}}{p(1-p)}\right)^{m} . Thus, we have
1 𝑥 𝑥 (1+x)\leq\exp(x) and cosh ( x ) ≤ exp ( x 2 / 2 ) 𝑥 superscript 𝑥 2 2 \cosh(x)\leq\exp(x^{2}/2) for all x 𝑥 x , which can be verified from Taylor series expansion, we have
1 4 superscript 1 𝜂 2 \ell_{\eta}=\sqrt{\ln(1+4(1-\eta)^{2})} . Using the value for γ = 8 δ ln n n 𝛾 8 𝛿 𝑛 𝑛 \gamma=\frac{8\sqrt{\delta\ln n}}{n} assumed earlier, one can see that both ( 14 ) and Θ 1 ⊂ Ω 1 subscript Θ 1 subscript Ω 1 \Theta_{1}\subset\Omega_{1} hold under the stated condition on m 𝑚 m , which leads to the claimed lower bound that ρ ∗ > 1 − 1 n superscript 𝜌 1 1 𝑛 \rho^{*}>1-\frac{1}{n} .
A.2 Proof of Proposition 3.2
We begin by computing the symmetric KL-divergence between two IER models. Observe that
We now swap the summation and product, and note that there are two cases — if ( i ′ , j ′ ) ≠ ( i , j ) superscript 𝑖 ′ superscript 𝑗 ′ 𝑖 𝑗 (i^{\prime},j^{\prime})\neq(i,j) , then ∑ A i ′ j ′ P i ′ j ′ A i ′ j ′ ( 1 − P i ′ j ′ ) 1 − A i ′ j ′ = 1 subscript subscript 𝐴 superscript 𝑖 ′ superscript 𝑗 ′ superscript subscript 𝑃 superscript 𝑖 ′ superscript 𝑗 ′ subscript 𝐴 superscript 𝑖 ′ superscript 𝑗 ′ superscript 1 subscript 𝑃 superscript 𝑖 ′ superscript 𝑗 ′ 1 subscript 𝐴 superscript 𝑖 ′ superscript 𝑗 ′ 1 \sum_{A_{i^{\prime}j^{\prime}}}P_{i^{\prime}j^{\prime}}^{A_{i^{\prime}j^{\prime}}}(1-P_{i^{\prime}j^{\prime}})^{1-A_{i^{\prime}j^{\prime}}}=1 , whereas if ( i ′ , j ′ ) = ( i , j ) superscript 𝑖 ′ superscript 𝑗 ′ 𝑖 𝑗 (i^{\prime},j^{\prime})=(i,j) the log term needs to be counted. Hence,
which in turn implies
𝑃 𝑝 italic-ϵ Q_{\epsilon}=P+p\epsilon , where ϵ ∈ ℝ n × n italic-ϵ superscript ℝ 𝑛 𝑛 \epsilon\in\mathbb{R}^{n\times n} is symmetric and zero everywhere except one entry above diagonal where ϵ i j = − 1 subscript italic-ϵ 𝑖 𝑗 1 \epsilon_{ij}=-1 . Note that there are ( n 2 ) binomial 𝑛 2 \binom{n}{2} such ϵ italic-ϵ \epsilon ’s and hence, this is the size of Θ 1 subscript Θ 1 \Theta_{1} . It is also obvious that S K L ( IER ( P ) , IER ( Q ϵ ) ) = ∞ 𝑆 𝐾 𝐿 IER 𝑃 IER subscript 𝑄 italic-ϵ SKL(\textup{IER}(P),\textup{IER}(Q_{\epsilon}))=\infty .
We now compute the term in ( 14 ) in this case. Note that the computation up to ( 15 ) can be done for any matrix ϵ italic-ϵ \epsilon , and in the present case, the same computation yields
1 𝛿 𝑚 𝑚 𝛿 (1+\frac{p}{1-p})^{m}\leq(1+\delta)^{m}\leq\exp(m\delta) . Hence,
Thus, ( 14 ) is satisfied when m ≤ 2 δ ln ( ( 1 − η ) n ) 𝑚 2 𝛿 1 𝜂 𝑛 m\leq\frac{2}{\delta}\ln((1-\eta)n) .
A.3 Proof of Proposition 3.3
Consider the construction in the proof of Proposition 3.1 . If γ > 0 𝛾 0 \gamma>0 , then ‖ P − Q ϵ ‖ 0 = n ( n − 1 ) subscript norm 𝑃 subscript 𝑄 italic-ϵ 0 𝑛 𝑛 1 \|P-Q_{\epsilon}\|_{0}=n(n-1) for all ( P , Q ϵ ) ∈ Θ 1 𝑃 subscript 𝑄 italic-ϵ subscript Θ 1 (P,Q_{\epsilon})\in\Theta_{1} . But, as shown in the same proof, ( 14 ) holds for γ ≤ ℓ η δ 2 m n 𝛾 subscript ℓ 𝜂 𝛿 2 𝑚 𝑛 \gamma\leq\sqrt{\frac{\ell_{\eta}\delta}{2mn}} Note that this upper bound on γ 𝛾 \gamma is strictly positive for all m < ∞ 𝑚 m<\infty , δ > 0 𝛿 0 \delta>0 and η < 1 𝜂 1 \eta<1 . Hence, the result follows.
Appendix B Proof of results in Section 4
B.1 proof of lemma 4.2.
The necessary condition is proved using the instance constructed in the proof of Proposition 3.1 . Observe that for ( P , Q ϵ ) ∈ Θ 1 𝑃 subscript 𝑄 italic-ϵ subscript Θ 1 (P,Q_{\epsilon})\in\Theta_{1} , ‖ P − Q ϵ ‖ F = γ n ( n − 1 ) > n γ 2 subscript norm 𝑃 subscript 𝑄 italic-ϵ 𝐹 𝛾 𝑛 𝑛 1 𝑛 𝛾 2 \|P-Q_{\epsilon}\|_{F}=\gamma\sqrt{n(n-1)}>\frac{n\gamma}{2} .
To prove condition (i) in the statement of the lemma, we use the bound in ( 17 ) to obtain that ( 14 ) holds for γ ≤ ℓ η δ 2 m n 𝛾 subscript ℓ 𝜂 𝛿 2 𝑚 𝑛 \gamma\leq\sqrt{\frac{\ell_{\eta}\delta}{2mn}} . We now set γ = ℓ η δ 2 m n ∧ δ 2 𝛾 subscript ℓ 𝜂 𝛿 2 𝑚 𝑛 𝛿 2 \gamma=\sqrt{\frac{\ell_{\eta}\delta}{2mn}}\wedge\frac{\delta}{2} , where the second term ensures ‖ Q ϵ ‖ m a x ≤ δ subscript norm subscript 𝑄 italic-ϵ 𝑚 𝑎 𝑥 𝛿 \|Q_{\epsilon}\|_{max}\leq\delta . The claim follows from the fact that Θ 1 ⊂ Ω 1 subscript Θ 1 subscript Ω 1 \Theta_{1}\subset\Omega_{1} if ρ < n γ 2 𝜌 𝑛 𝛾 2 \rho<\frac{n\gamma}{2} .
To prove condition (ii) in the lemma, observe that the bound in ( 16 ) equals 1 for m = 1 𝑚 1 m=1 , and hence, ( 14 ) is satisfied for all γ ≤ δ 2 𝛾 𝛿 2 \gamma\leq\frac{\delta}{2} . Setting γ = δ 2 𝛾 𝛿 2 \gamma=\frac{\delta}{2} leads to the claim.
B.2 Proof of Lemma 4.3
We assume that m 𝑚 m is even for convenience. If m > 2 𝑚 2 m>2 and odd, one may simply work with m − 1 𝑚 1 m-1 samples, and the result still holds with possible change in constants.
Preparatory computations: Ingredients for Chebyshev’s inequality
𝑃 𝑄 𝐹 2 \sigma^{2}=\mathsf{E}[\widehat{\sigma}^{2}]=\frac{m^{2}}{8}\|P+Q\|_{F}^{2} . Since all terms in μ ^ ^ 𝜇 \widehat{\mu} and σ ^ ^ 𝜎 \widehat{\sigma} are independent, one can compute their variances as
𝑃 𝑄 𝐹 1 m\|P+Q\|_{F}\geq 1 .
Controlling the type-I-error rate
Consider θ = ( P , Q ) ∈ Ω 0 𝜃 𝑃 𝑄 subscript Ω 0 \theta=(P,Q)\in\Omega_{0} . The test Ψ F subscript Ψ 𝐹 \Psi_{F} makes an error only if both events in ( 7 ) occur, that is,
It is convenient to bound both probabilities separately based on the following case analysis:
Here we bound the first term in ( 18 ) as
4 𝜂 superscript subscript 𝑡 1 2 256 𝜂 9 superscript 𝐶 ′′ \mathsf{P}_{\theta}(\Psi_{F}=1)\leq\frac{4\eta}{t_{1}^{2}}+\frac{256\eta}{9C^{\prime\prime}} , which is smaller than η 2 𝜂 2 \frac{\eta}{2} if t 1 subscript 𝑡 1 t_{1} and C ′′ superscript 𝐶 ′′ C^{\prime\prime} are large enough.
Here the error probability in ( 18 ) can be bounded using the Markov inequality.
which can be made smaller than η 2 𝜂 2 \frac{\eta}{2} by choosing t 2 subscript 𝑡 2 t_{2} large.
Thus the Type-I error rate for Ψ F subscript Ψ 𝐹 \Psi_{F} is at most η 2 𝜂 2 \frac{\eta}{2} .
Controlling the type-II-error rate
For θ = ( P , Q ) ∈ Ω 1 𝜃 𝑃 𝑄 subscript Ω 1 \theta=(P,Q)\in\Omega_{1} , we can bound the error rate as
𝑃 𝑄 𝐹 2 𝑛 𝛿 \|P+Q\|_{F}\leq 2n\delta and ρ ≥ C n δ η m 𝜌 𝐶 𝑛 𝛿 𝜂 𝑚 \rho\geq\sqrt{\frac{Cn\delta}{\eta m}} to write
Hence, by choosing C 𝐶 C large enough, we can assume that 2 σ t 1 η ≤ 2 σ t 1 η ≤ μ 2 𝜎 subscript 𝑡 1 𝜂 2 𝜎 subscript 𝑡 1 𝜂 𝜇 \frac{2\sigma t_{1}}{\sqrt{\eta}}\leq\frac{2\sigma t_{1}}{\eta}\leq\mu , and can bound the first term as
𝑃 𝑄 𝐹 subscript norm 𝑃 𝑄 𝐹 𝜌 superscript 𝐶 ′ superscript 𝜂 3 2 𝑚 \|P+Q\|_{F}\geq\|P-Q\|_{F}>\rho\geq\frac{C^{\prime}}{\eta^{3/2}m} , and so, σ ≥ C ′ 8 η 3 / 2 𝜎 superscript 𝐶 ′ 8 superscript 𝜂 3 2 \sigma\geq\frac{C^{\prime}}{\sqrt{8}\eta^{3/2}} . For large C ′ superscript 𝐶 ′ C^{\prime} , we can write t 2 η 3 / 2 ≤ σ 2 subscript 𝑡 2 superscript 𝜂 3 2 𝜎 2 \frac{t_{2}}{\eta^{3/2}}\leq\frac{\sigma}{2} . Hence, we may bound each of the last two terms in ( 19 ) by
B.3 Proof of Proposition 4.4
The proof is along the lines of the proof of Lemma 4.3 , but uses a stronger concentration inequality that helps in improving the dependence on η 𝜂 \eta at the cost of an additional ln n 𝑛 \ln n factor.
Preparation: New concentration inequality
We now state a generic concentration bound that can be applied to both μ ^ ^ 𝜇 \widehat{\mu} ( 5 ) and σ ^ 2 superscript ^ 𝜎 2 \widehat{\sigma}^{2} ( 6 ) afterwards.
Lemma B.1 (Concentration inequality for sum of “product of sums”) .
Let m 𝑚 m be even and d 𝑑 d be any positive integer. Let { X k l : 1 ≤ k ≤ m , 1 ≤ l ≤ d } conditional-set subscript 𝑋 𝑘 𝑙 formulae-sequence 1 𝑘 𝑚 1 𝑙 𝑑 \{X_{kl}:1\leq k\leq m,1\leq l\leq d\} be a collection of independent random variables with 𝖤 [ X k l ] = a l 𝖤 delimited-[] subscript 𝑋 𝑘 𝑙 subscript 𝑎 𝑙 \mathsf{E}[X_{kl}]=a_{l} , | X k l − a l | ≤ 2 subscript 𝑋 𝑘 𝑙 subscript 𝑎 𝑙 2 |X_{kl}-a_{l}|\leq 2 almost surely, and 𝖵𝖺𝗋 ( X k l ) ≤ v l 𝖵𝖺𝗋 subscript 𝑋 𝑘 𝑙 subscript 𝑣 𝑙 \mathsf{Var}(X_{kl})\leq v_{l} .
Let S l = ∑ k ≤ m / 2 X k l subscript 𝑆 𝑙 subscript 𝑘 𝑚 2 subscript 𝑋 𝑘 𝑙 S_{l}=\sum\limits_{k\leq m/2}X_{kl} and S l ′ = ∑ k > m / 2 X k l subscript superscript 𝑆 ′ 𝑙 subscript 𝑘 𝑚 2 subscript 𝑋 𝑘 𝑙 S^{\prime}_{l}=\sum\limits_{k>m/2}X_{kl} . For any ϵ ∈ ( 0 , 1 5 ) italic-ϵ 0 1 5 \epsilon\in(0,\frac{1}{5}) ,
where a = ∑ l a l 2 𝑎 subscript 𝑙 superscript subscript 𝑎 𝑙 2 a=\sqrt{\sum_{l}a_{l}^{2}} , v = ∑ l v l 2 𝑣 subscript 𝑙 superscript subscript 𝑣 𝑙 2 v=\sqrt{\sum_{l}v_{l}^{2}} ,
A similar concentration inequality also holds for the lower tail probability.
Lemma B.1 improves upon Bernstein’s inequality with respect to the allowable range of parameters m 𝑚 m and d 𝑑 d . This improvement is possible because unlike Bernstein’s inequality that only assumes | S ℓ S ℓ ′ | ≤ 1 4 m 2 subscript 𝑆 ℓ subscript superscript 𝑆 ′ ℓ 1 4 superscript 𝑚 2 |S_{\ell}S^{\prime}_{\ell}|\leq\frac{1}{4}m^{2} with probability 1, we use the fact that S ℓ S ℓ ′ subscript 𝑆 ℓ subscript superscript 𝑆 ′ ℓ S_{\ell}S^{\prime}_{\ell} is a product of sums. In the context of Proposition 4.4 , we exploit that each product term in μ ^ ^ 𝜇 \widehat{\mu} and σ ^ ^ 𝜎 \widehat{\sigma} is a product of two sums, and hence, the individual terms also concentrate. We prove the Lemma B.1 later in the section.
Target probabilities to be bounded
We now start with the proof of Proposition 4.4 . Similar to ( 18 ) and ( 19 ), we have that
for θ ∈ Ω 0 𝜃 subscript Ω 0 \theta\in\Omega_{0} , and
for θ ∈ Ω 1 𝜃 subscript Ω 1 \theta\in\Omega_{1} . The claim of the proposition follows if each term in ( 21 ) and ( 22 ) is at most η 6 𝜂 6 \frac{\eta}{6} , which we show using Lemma B.1 .
𝑃 𝑄 𝐹 𝑚 𝑛 𝛿 \sigma=\frac{m}{\sqrt{8}}\|P+Q\|_{F}\leq{mn\delta} . Furthermore, due to the stated condition on ρ 𝜌 \rho ,
subscript 𝜏 1 subscript 𝜏 2 \tau_{1}+\tau_{2} in ( 20 ) satisfies
Based on the relation between μ 𝜇 \mu and σ 𝜎 \sigma in ( 23 ), we conclude that the above quantity is smaller than μ 2 𝜇 2 \frac{\mu}{2} for large enough C 𝐶 C . Hence, due to Lemma B.1 , the first term in ( 25 ) is bounded by 4 d ϵ ≤ η 6 4 𝑑 italic-ϵ 𝜂 6 4d\epsilon\leq\frac{\eta}{6} .
subscript 𝑃 𝑖 𝑗 subscript 𝑄 𝑖 𝑗 v_{l}=P_{ij}+Q_{ij} , which gives v = a = 2 σ m 𝑣 𝑎 2 𝜎 𝑚 v=a=\frac{2\sigma}{m} . For ϵ = η 18 n 2 italic-ϵ 𝜂 18 superscript 𝑛 2 \epsilon=\frac{\eta}{18n^{2}} , we again have z ≤ c 0 σ ln ( n η ) 𝑧 subscript 𝑐 0 𝜎 𝑛 𝜂 z\leq c_{0}\sqrt{\sigma\ln(\frac{n}{\eta})} using ( 24 ), and
which is smaller than σ 2 2 superscript 𝜎 2 2 \frac{\sigma^{2}}{2} for large enough C ′ superscript 𝐶 ′ C^{\prime} . Based on this and Lemma B.1 , the second and third terms in ( 25 ) are at most η 6 𝜂 6 \frac{\eta}{6} .
We now consider θ ∈ Ω 0 𝜃 subscript Ω 0 \theta\in\Omega_{0} , where μ = 0 𝜇 0 \mu=0 . As in Section B.2 , it is convenient to distinguish two cases:
subscript 𝑃 𝑖 𝑗 subscript 𝑄 𝑖 𝑗 v_{l}=P_{ij}+Q_{ij} . Hence, a = 0 𝑎 0 a=0 and v = 2 σ m 𝑣 2 𝜎 𝑚 v=\frac{2\sigma}{m} . Let ϵ = η 12 n 2 italic-ϵ 𝜂 12 superscript 𝑛 2 \epsilon=\frac{\eta}{12n^{2}} . Due to our assumption on σ 𝜎 \sigma , we have σ ≥ ln ( n η ) 𝜎 𝑛 𝜂 \sigma\geq\ln(\frac{n}{\eta}) and so z ≤ c 0 σ ln ( n η ) 𝑧 subscript 𝑐 0 𝜎 𝑛 𝜂 z\leq c_{0}\sqrt{\sigma\ln(\frac{n}{\eta})} for some constant c 0 subscript 𝑐 0 c_{0} . We also have, for some constant c 1 subscript 𝑐 1 c_{1} ,
subscript subscript 𝐴 subscript 𝐺 𝑘 𝑖 𝑗 subscript subscript 𝐴 subscript 𝐻 𝑘 𝑖 𝑗 X_{kl}=(A_{G_{k}})_{ij}+(A_{H_{k}})_{ij} and so, a = v = 2 σ m 𝑎 𝑣 2 𝜎 𝑚 a=v=\frac{2\sigma}{m} . As in the case of the alternative hypothesis, we have
subscript 𝜏 1 subscript 𝜏 2 3 superscript 𝜎 2 4 \tau_{1}+\tau_{2}\leq\frac{3\sigma^{2}}{4} and so, the second term in ( 21 ) is at most η 6 𝜂 6 \frac{\eta}{6} . Thus, 𝖯 θ ( Ψ F ′ = 1 ) ≤ η 3 subscript 𝖯 𝜃 subscript superscript Ψ ′ 𝐹 1 𝜂 3 \mathsf{P}_{\theta}(\Psi^{\prime}_{F}=1)\leq\frac{\eta}{3} when σ ≥ ξ ln 2 ( 2 η ) ln ( n η ) 𝜎 𝜉 superscript 2 2 𝜂 𝑛 𝜂 \sigma\geq\xi\ln^{2}(\frac{2}{\eta})\ln(\frac{n}{\eta}) .
We show that the third term in ( 21 ) is smaller than η 6 𝜂 6 \frac{\eta}{6} . Observe that if t 2 > ξ subscript 𝑡 2 𝜉 t_{2}>\xi ,
subscript subscript 𝐴 subscript 𝐺 𝑘 𝑖 𝑗 subscript subscript 𝐴 subscript 𝐻 𝑘 𝑖 𝑗 X_{kl}=(A_{G_{k}})_{ij}+(A_{H_{k}})_{ij} and ϵ = η 18 n 2 italic-ϵ 𝜂 18 superscript 𝑛 2 \epsilon=\frac{\eta}{18n^{2}} as above, and observe that under the condition on σ 𝜎 \sigma , we have z ≤ ξ ln ( 2 η ) ln ( n η ) 𝑧 𝜉 2 𝜂 𝑛 𝜂 z\leq\sqrt{\xi}\ln(\frac{2}{\eta})\ln(\frac{n}{\eta}) noting that ξ > 1 𝜉 1 \xi>1 and v = a = 2 σ m 𝑣 𝑎 2 𝜎 𝑚 v=a=\frac{2\sigma}{m} . Hence,
which is smaller than the above threshold for t 2 2 ≥ 2 ξ 2 superscript subscript 𝑡 2 2 2 superscript 𝜉 2 t_{2}^{2}\geq 2\xi^{2} and so, the above probability is smaller than η 6 𝜂 6 \frac{\eta}{6} .
Hence, for t 1 subscript 𝑡 1 t_{1} and t 2 subscript 𝑡 2 t_{2} large enough, the rejection rate under null is also smaller than η 2 𝜂 2 \frac{\eta}{2} , which completes the proof of the stated result. We conclude with the proof of Lemma B.1 .
Proof of Lemma B.1 .
For the first term, we note that due to the Bernstein inequality,
noting that | X k l − a l | ≤ 2 subscript 𝑋 𝑘 𝑙 subscript 𝑎 𝑙 2 |X_{kl}-a_{l}|\leq 2 . Substituting z 𝑧 z and noting that v l ≤ v subscript 𝑣 𝑙 𝑣 v_{l}\leq v , the above bound is at most ϵ italic-ϵ \epsilon . Hence, by union bound 𝖯 ( ξ c ) ≤ 4 d ϵ 𝖯 superscript 𝜉 𝑐 4 𝑑 italic-ϵ \mathsf{P}\left(\xi^{c}\right)\leq 4d\epsilon . To deal with the other two terms in ( 26 ), we need the following claim.
The equality in (ii) follows directly from the above arguments. To prove the inequality, define the non-negative random variable Y = ( S l − 1 2 m a l ) 2 𝑌 superscript subscript 𝑆 𝑙 1 2 𝑚 subscript 𝑎 𝑙 2 Y=(S_{l}-\frac{1}{2}ma_{l})^{2} , and note that ξ l = { Y ≤ z 2 } subscript 𝜉 𝑙 𝑌 superscript 𝑧 2 \xi_{l}=\{Y\leq z^{2}\} . Hence,
since 𝖤 [ Y | ξ l ] = 𝖤 [ Y 𝟏 { X ≤ z 2 } ] 𝖯 ( ξ l ) ≤ z 2 𝖯 ( ξ l ) 𝖯 ( ξ l ) = z 2 𝖤 delimited-[] conditional 𝑌 subscript 𝜉 𝑙 𝖤 delimited-[] 𝑌 1 𝑋 superscript 𝑧 2 𝖯 subscript 𝜉 𝑙 superscript 𝑧 2 𝖯 subscript 𝜉 𝑙 𝖯 subscript 𝜉 𝑙 superscript 𝑧 2 \mathsf{E}[Y|\xi_{l}]=\frac{\mathsf{E}[Y\mathbf{1}\{X\leq z^{2}\}]}{\mathsf{P}(\xi_{l})}\leq\frac{z^{2}\mathsf{P}(\xi_{l})}{\mathsf{P}(\xi_{l})}=z^{2} . Hence, the claim. ∎
We now apply Bernstein inequality for the second term in ( 26 ) to obtain
where we use the claim to write 𝖵𝖺𝗋 ( ( S l − m a l 2 ) ( S l ′ − m a l 2 ) | ξ ) 𝖵𝖺𝗋 conditional subscript 𝑆 𝑙 𝑚 subscript 𝑎 𝑙 2 subscript superscript 𝑆 ′ 𝑙 𝑚 subscript 𝑎 𝑙 2 𝜉 \mathsf{Var}\left((S_{l}-\frac{ma_{l}}{2})(S^{\prime}_{l}-\frac{ma_{l}}{2})|\xi\right) is at most 𝖵𝖺𝗋 ( S l ) 𝖵𝖺𝗋 ( S l ′ ) = m 2 v l 2 4 𝖵𝖺𝗋 subscript 𝑆 𝑙 𝖵𝖺𝗋 subscript superscript 𝑆 ′ 𝑙 superscript 𝑚 2 superscript subscript 𝑣 𝑙 2 4 \mathsf{Var}(S_{l})\mathsf{Var}(S^{\prime}_{l})=\frac{m^{2}v_{l}^{2}}{4} , and then substitute the value of τ 1 subscript 𝜏 1 \tau_{1} . The third term in ( 26 ) can be dealt with similarly as
In the last step, we take ∑ l a l 2 v l ≤ a 2 ( max l v l ) ≤ a 2 v subscript 𝑙 superscript subscript 𝑎 𝑙 2 subscript 𝑣 𝑙 superscript 𝑎 2 subscript 𝑙 subscript 𝑣 𝑙 superscript 𝑎 2 𝑣 \sum_{l}a_{l}^{2}v_{l}\leq a^{2}(\max_{l}v_{l})\leq a^{2}v , and substitute τ 2 subscript 𝜏 2 \tau_{2} . ∎
Appendix C Proof of results in Section 5
C.1 proof of lemma 5.2.
1 𝑛 v\in\{-1,+1\}^{n} . (To be precise, Θ 1 subscript Θ 1 \Theta_{1} contains 2 n − 1 superscript 2 𝑛 1 2^{n-1} elements since v 𝑣 v and − v 𝑣 -v result in the same Q 𝑄 Q . But, for convenience, we compute the expectation by counting every model twice and divide by 2 n superscript 2 𝑛 2^{n} ). Note that Θ 1 ⊂ Ω 1 subscript Θ 1 subscript Ω 1 \Theta_{1}\subset\Omega_{1} if ‖ P − Q v ‖ o p = γ ‖ v v T − I ‖ o p = γ ( n − 1 ) > ρ subscript norm 𝑃 subscript 𝑄 𝑣 𝑜 𝑝 𝛾 subscript norm 𝑣 superscript 𝑣 𝑇 𝐼 𝑜 𝑝 𝛾 𝑛 1 𝜌 \|P-Q_{v}\|_{op}=\gamma\|vv^{T}-I\|_{op}=\gamma(n-1)>\rho .
We now compute the quantity in ( 14 ). As before, let ω ∈ ℱ 𝜔 ℱ \omega\in\mathcal{F} correspond to the tuple ω = ( G 1 , … , G m , H 1 , … , H m ) 𝜔 subscript 𝐺 1 … subscript 𝐺 𝑚 subscript 𝐻 1 … subscript 𝐻 𝑚 \omega=(G_{1},\ldots,G_{m},H_{1},\ldots,H_{m}) , where we assume that the first m 𝑚 m graphs are generated from the first model, and the rest from the second model. Then
where S G = ∑ k A G k subscript 𝑆 𝐺 subscript 𝑘 subscript 𝐴 subscript 𝐺 𝑘 S_{G}=\sum_{k}A_{G_{k}} and S H = ∑ k A H k subscript 𝑆 𝐻 subscript 𝑘 subscript 𝐴 subscript 𝐻 𝑘 S_{H}=\sum_{k}A_{H_{k}} . On the other hand, every element in Θ 1 subscript Θ 1 \Theta_{1} is characterised by v ∈ { ± 1 } n 𝑣 superscript plus-or-minus 1 𝑛 v\in\{\pm 1\}^{n} . Denoting the element by θ v subscript 𝜃 𝑣 \theta_{v} , we have
The quantity in ( 14 ) can be computed as
We now claim the following.
1 𝑙 𝑛 1 2 subscript 𝑐 0 c_{l}\leq c_{0}\left(1+\frac{l}{n-1}\right)\leq 2c_{0} .
The claim can be proved by induction. If the first bound holds for c l subscript 𝑐 𝑙 c_{l} , then
𝑙 1 c_{l+1} . ∎
For any c ≤ 2 c 0 ≤ 1 16 n 𝑐 2 subscript 𝑐 0 1 16 𝑛 c\leq 2c_{0}\leq\frac{1}{16n} ,
𝑙 1 𝑐 1 |2cS_{l}z_{l+1}+c|\leq 1 for any c ≤ 1 16 n 𝑐 1 16 𝑛 c\leq\frac{1}{16n} . The bound follows since
𝑙 1 2 1 z_{l+1}^{2}=1 . ∎
Setting c 0 = m γ 2 p subscript 𝑐 0 𝑚 superscript 𝛾 2 𝑝 c_{0}=\frac{m\gamma^{2}}{p} and using these two claims repeatedly, we bound
1 4 superscript 1 𝜂 2 \ell_{\eta}=\sqrt{\ln(1+4(1-\eta)^{2})} . Hence, the minimax risk is at least η 𝜂 \eta if γ ≤ ( ℓ η ∧ 1 32 ) p m n = 2 ℓ η ′ δ m n 𝛾 subscript ℓ 𝜂 1 32 𝑝 𝑚 𝑛 2 subscript superscript ℓ ′ 𝜂 𝛿 𝑚 𝑛 \gamma\leq(\ell_{\eta}\wedge\frac{1}{\sqrt{32}})\sqrt{\frac{p}{mn}}=2\ell^{\prime}_{\eta}\sqrt{\frac{\delta}{mn}} for ℓ η ′ subscript superscript ℓ ′ 𝜂 \ell^{\prime}_{\eta} defined in Theorem 5.1 . We now set γ = δ 2 ∧ 2 ℓ η ′ δ m n 𝛾 𝛿 2 2 subscript superscript ℓ ′ 𝜂 𝛿 𝑚 𝑛 \gamma=\frac{\delta}{2}\wedge 2\ell^{\prime}_{\eta}\sqrt{\frac{\delta}{mn}} , and obtain the stated claim by substituting this value of γ 𝛾 \gamma in the condition γ ( n − 1 ) ≥ γ n 2 > ρ 𝛾 𝑛 1 𝛾 𝑛 2 𝜌 \gamma(n-1)\geq\frac{\gamma n}{2}>\rho .
C.2 Proof of Lemma 5.3
The general approach for the proof is along the lines of Lemma 4.3 , but we now need concentration inequalities for operator norm and row sum norm.
Preparatory computations and inequalities
The concentration result for operator norm that we use is a direct consequence of the matrix Bernstein inequality (Tropp, 2012 ; Oliveira, 2009 ) . The proof, given at the end of this subsection, is similar to the concentration result for random adjacency matrices in Theorem 3.1 of Oliveira ( 2009 ) .
Lemma C.1 (Concentration of operator norm) .
0 bra 𝜏 𝑚 𝑃 evaluated-at 𝑄 𝑟 𝑜 𝑤 0<\tau\leq m\|P+Q\|_{row} , we have
We also use the following concentration result for the row sum norm, which is also proved later.
Lemma C.2 (Concentration of row sum norm) .
For any θ = ( P , Q ) 𝜃 𝑃 𝑄 \theta=(P,Q) ,
𝑃 𝑄 𝑟 𝑜 𝑤 𝜏 4 𝑚 \|P+Q\|_{row}\leq\frac{\tau}{4m} , then
Controlling the type-I error rate
We now begin the proof of Lemma 5.3 . For θ = ( P , Q ) ∈ Ω 0 𝜃 𝑃 𝑄 subscript Ω 0 \theta=(P,Q)\in\Omega_{0} , observe that 𝖤 [ S − ] = 0 𝖤 delimited-[] superscript 𝑆 0 \mathsf{E}[S^{-}]=0 and
As in Section B.2 , we distinguish between two cases.
We can write
𝑃 𝑄 𝑟 𝑜 𝑤 \|P+Q\|_{row} , we can say that the second term is bounded by ( η n ) t 1 2 / 32 superscript 𝜂 𝑛 superscript subscript 𝑡 1 2 32 (\frac{\eta}{n})^{t_{1}^{2}/32} . Since η < 1 𝜂 1 \eta<1 and n ≥ 2 𝑛 2 n\geq 2 (otherwise, we deal with empty graphs on one vertex), the above sum can be made smaller than η 2 𝜂 2 \frac{\eta}{2} by choosing t 1 subscript 𝑡 1 t_{1} large enough.
In this case, set t 2 = 2 t 1 2 subscript 𝑡 2 2 superscript subscript 𝑡 1 2 t_{2}=2t_{1}^{2} and use the last bound of Lemma C.2 to conclude that
which is at most η 2 𝜂 2 \frac{\eta}{2} for large enough t 1 subscript 𝑡 1 t_{1} . Thus, 𝖯 θ ( Ψ o p = 1 ) ≤ η 2 subscript 𝖯 𝜃 subscript Ψ 𝑜 𝑝 1 𝜂 2 \mathsf{P}_{\theta}(\Psi_{op}=1)\leq\frac{\eta}{2} for all θ ∈ Ω 0 𝜃 subscript Ω 0 \theta\in\Omega_{0} .
Controlling the type-II error rate
We now bound the Type-II error rate. For θ = ( P , Q ) ∈ Ω 1 𝜃 𝑃 𝑄 subscript Ω 1 \theta=(P,Q)\in\Omega_{1} ,
Before bounding the individual terms, we recall that the sufficient condition on ρ 𝜌 \rho implies that
For C ′ ≥ 8 t 1 2 superscript 𝐶 ′ 8 superscript subscript 𝑡 1 2 C^{\prime}\geq 8t_{1}^{2} , we can bound the third term in ( 28 ) by
The second term is similarly bounded by
𝑃 𝑄 𝑜 𝑝 𝑛 𝛿 \|P+Q\|_{op}\leq n\delta and the sufficient condition on ρ 𝜌 \rho to see that
where the last inequality holds for large C 𝐶 C . Hence, the first term in ( 28 ) is bounded by
We conclude this section with the proofs for Lemmas C.1 – C.2 .
Proof of Lemma C.1 .
which is a sum of 2 m ( n 2 ) 2 𝑚 binomial 𝑛 2 2m\binom{n}{2} independent random matrices. One can see that each of these matrices has zero mean, and its operator norm is bounded by 1 almost surely. Moreover, for each matrix, we can write
𝑃 𝑄 𝑟 𝑜 𝑤 m\|P+Q\|_{row} . Based on these observations, we use the matrix Bernstein inequality (Theorem 1.4 of Tropp ( 2012 ) or Corollary 7.1 of Oliveira ( 2009 ) ) to conclude that
𝑃 𝑄 𝑟 𝑜 𝑤 \tau\leq m\|P+Q\|_{row} . ∎
Proof of Lemma C.2 .
𝑃 𝑄 𝑟 𝑜 𝑤 d_{1}=\|P+Q\|_{row} . To prove the first inequality, we write
𝑃 𝑄 𝑟 𝑜 𝑤 d_{i}\geq\frac{1}{3}\|P+Q\|_{row} . We can use Bernstein inequality to write
subscript 𝑑 𝑖 bra 1 3 𝑃 evaluated-at 𝑄 𝑟 𝑜 𝑤 d_{i}<\frac{1}{3}\|P+Q\|_{row} , we have by the Markov inequality
subscript 𝑑 𝑖 bra 1 3 𝑃 evaluated-at 𝑄 𝑟 𝑜 𝑤 d_{i}<\frac{1}{3}\|P+Q\|_{row} , we have
Combining above bounds, we obtain the first inequality in Lemma C.2 . We prove the second inequality by observing that
𝑃 𝑄 𝑟 𝑜 𝑤 d_{1}=\|P+Q\|_{row} .
The third inequality in Lemma C.2 is proved using Markov inequality in the following way.
𝑃 𝑄 𝑟 𝑜 𝑤 \tau\geq 4m\|P+Q\|_{row} . ∎
C.3 Proof of Proposition 5.4
We note that our proof is valid for any n ≥ 2 𝑛 2 n\geq 2 . If one additionally assumes that n 𝑛 n is large enough, then the terms involving ln ( 2 η ) 2 𝜂 \ln(\frac{2}{\eta}) in the test Ψ o p ′ subscript superscript Ψ ′ 𝑜 𝑝 \Psi^{\prime}_{op} ( 13 ) and Proposition 5.4 can be replaced by absolute constants.
Preliminary computations and inequalities
We first state the concentration result of Le, Levina and Vershynin ( 2017 , Theorem 2.1) adapted to our setting. The adaptation is explicitly described later in the proof of the lemma.
Lemma C.3 (Concentration of trimmed adjacency matrix) .
Let G ∼ IER ( P ) similar-to 𝐺 IER 𝑃 G\sim\textup{IER}(P) with ‖ P ‖ m a x ≤ δ subscript norm 𝑃 𝑚 𝑎 𝑥 𝛿 \|P\|_{max}\leq\delta , where δ > 10 n 𝛿 10 𝑛 \delta>\frac{10}{n} . Consider the trimming procedure which isolates all vertices with degree larger than c n δ ln ( 2 η ) 𝑐 𝑛 𝛿 2 𝜂 cn\delta\ln(\frac{2}{\eta}) , and A G ′ subscript superscript 𝐴 ′ 𝐺 A^{\prime}_{G} be the resulting adjacency matrix. There exists an absolute constant C > 0 𝐶 0 C>0 such that with probability 1 − η 4 1 𝜂 4 1-\frac{\eta}{4} ,
The proof of Proposition 5.4 follows directly from the above lemma by setting t = 2 C 𝑡 2 𝐶 t=2C . Let θ = ( P , Q ) ∈ Ω 0 𝜃 𝑃 𝑄 subscript Ω 0 \theta=(P,Q)\in\Omega_{0} .
where the bound follows from triangle inequality and noting that P = Q 𝑃 𝑄 P=Q . Lemma C.3 states that each of the two norms can exceed C n δ ln 2 ( 2 η ) 𝐶 𝑛 𝛿 superscript 2 2 𝜂 C\sqrt{n\delta}\ln^{2}(\frac{2}{\eta}) with probability at most η 4 𝜂 4 \frac{\eta}{4} . Hence, the above probability is bounded by η 2 𝜂 2 \frac{\eta}{2} .
Now consider θ = ( P , Q ) ∈ Ω 1 𝜃 𝑃 𝑄 subscript Ω 1 \theta=(P,Q)\in\Omega_{1} and use the condition ρ ≥ 4 C n δ ln 2 ( 2 η ) 𝜌 4 𝐶 𝑛 𝛿 superscript 2 2 𝜂 \rho\geq 4C\sqrt{n\delta}\ln^{2}(\frac{2}{\eta}) . By reverse triangle inequality
Using the above lower bound for ‖ A G ′ − A H ′ ‖ o p subscript norm subscript superscript 𝐴 ′ 𝐺 subscript superscript 𝐴 ′ 𝐻 𝑜 𝑝 \|A^{\prime}_{G}-A^{\prime}_{H}\|_{op} , we get
which is also at most η 2 𝜂 2 \frac{\eta}{2} due to Lemma C.3 . Hence, Proposition 5.4 holds. We now prove Lemma C.3 .
Proof of Lemma C.3 .
Define d = n δ 𝑑 𝑛 𝛿 d=n\delta . Le, Levina and Vershynin ( 2017 , Theorem 2.1) holds for the following general regularisation process to obtain A G ′ subscript superscript 𝐴 ′ 𝐺 A^{\prime}_{G} . Consider any subset of at most 10 n d 10 𝑛 𝑑 \frac{10n}{d} vertices and reduce the weights of edges incident on them in an arbitrary way. If d ′ superscript 𝑑 ′ d^{\prime} is the maximum degree in A G ′ subscript superscript 𝐴 ′ 𝐺 A^{\prime}_{G} , then for any r ≥ 1 𝑟 1 r\geq 1 ,
with probability at least 1 − n − r 1 superscript 𝑛 𝑟 1-n^{-r} , where C ′ superscript 𝐶 ′ C^{\prime} is a constant.
Let us fix r = 6 ln ( 2 η ) 𝑟 6 2 𝜂 r=6\ln(\frac{2}{\eta}) . For any n ≥ 2 𝑛 2 n\geq 2 , n − r ≤ exp ( − 3 ln ( 2 η ) ) ≤ η 8 superscript 𝑛 𝑟 3 2 𝜂 𝜂 8 n^{-r}\leq\exp(-3\ln(\frac{2}{\eta}))\leq\frac{\eta}{8} . We claim that if c ≥ 5 𝑐 5 c\geq 5 , then with probability at least 1 − η 8 1 𝜂 8 1-\frac{\eta}{8} , G 𝐺 G has at most 10 n d = 10 δ 10 𝑛 𝑑 10 𝛿 \frac{10n}{d}=\frac{10}{\delta} vertices with degree larger than c n δ ln ( 2 η ) 𝑐 𝑛 𝛿 2 𝜂 cn\delta\ln(\frac{2}{\eta}) . Hence, after deleting all edges incident on them, d ′ = c n δ ln ( 2 η ) superscript 𝑑 ′ 𝑐 𝑛 𝛿 2 𝜂 d^{\prime}=cn\delta\ln(\frac{2}{\eta}) and the above bound suggests that with probability 1 − η 4 1 𝜂 4 1-\frac{\eta}{4}
for an appropriately defined C 𝐶 C .
We conclude the proof by showing that at most 10 δ 10 𝛿 \frac{10}{\delta} vertices can have degree larger than c n δ ln ( 2 η ) 𝑐 𝑛 𝛿 2 𝜂 cn\delta\ln(\frac{2}{\eta}) . Let c ′ = 1 2 c ln ( 2 η ) superscript 𝑐 ′ 1 2 𝑐 2 𝜂 c^{\prime}=\frac{1}{2}c\ln(\frac{2}{\eta}) . Consider any vertex set V 1 subscript 𝑉 1 V_{1} of size n 1 subscript 𝑛 1 n_{1} . The probability that every vertex in V 1 subscript 𝑉 1 V_{1} has degree larger than 2 c ′ n δ 2 superscript 𝑐 ′ 𝑛 𝛿 2c^{\prime}n\delta is bounded by
where the last step is due to Bernstein inequality and holds for c ′ ≥ 3 superscript 𝑐 ′ 3 c^{\prime}\geq 3 .
Now if more than 10 δ 10 𝛿 \frac{10}{\delta} vertices have degree larger than 2 c ′ n δ 2 superscript 𝑐 ′ 𝑛 𝛿 2c^{\prime}n\delta , then one can find such a vertex set V 1 subscript 𝑉 1 V_{1} of size n 1 ∈ [ 10 δ , n ] subscript 𝑛 1 10 𝛿 𝑛 n_{1}\in[\frac{10}{\delta},n] . But the probability that there exists such a set of size n 1 subscript 𝑛 1 n_{1} is smaller than
Recall our assumption n δ > 10 𝑛 𝛿 10 n\delta>10 , and observe that for x > 10 𝑥 10 x>10 , ln ( e x / 10 ) ≤ c ′ 8 x 𝑒 𝑥 10 superscript 𝑐 ′ 8 𝑥 \ln(ex/10)\leq\frac{c^{\prime}}{8}x . Hence, the above probability is smaller than
for c ≥ 6 𝑐 6 c\geq 6 and n ≥ 2 𝑛 2 n\geq 2 , which completes the proof. ∎
- Abbe and Sandon (2016) {binproceedings} [author] \bauthor \bsnm Abbe, \bfnm E. \binits E. and \bauthor \bsnm Sandon, \bfnm C. \binits C. ( \byear 2016). \btitle Achieving the KS threshold in the general stochastic block model with linearized acyclic belief propagation. In \bbooktitle Advances in Neural Information Processing Systems \bvolume 29. \endbibitem
- Albert and Barabási (2002) {barticle} [author] \bauthor \bsnm Albert, \bfnm R. \binits R. and \bauthor \bsnm Barabási, \bfnm A. L. \binits A. L. ( \byear 2002). \btitle Statistical mechanics of complex networks. \bjournal Reviews of Modern Physics \bvolume 74 \bpages 47-97. \endbibitem
- Arias-Castro, Bubeck and Lugosi (2015) {barticle} [author] \bauthor \bsnm Arias-Castro, \bfnm E. \binits E., \bauthor \bsnm Bubeck, \bfnm S. \binits S. and \bauthor \bsnm Lugosi, \bfnm G. \binits G. ( \byear 2015). \btitle Detecting positive correlations in a multivariate sample. \bjournal Bernoulli \bvolume 21 \bpages 209-241. \endbibitem
- Arias-Castro and Verzelen (2014) {barticle} [author] \bauthor \bsnm Arias-Castro, \bfnm E. \binits E. and \bauthor \bsnm Verzelen, \bfnm N. \binits N. ( \byear 2014). \btitle Community detection in dense random networks. \bjournal Annals of Statistics \bvolume 42 \bpages 940-969. \endbibitem
- Arias-Castro and Verzelen (2015) {barticle} [author] \bauthor \bsnm Arias-Castro, \bfnm E. \binits E. and \bauthor \bsnm Verzelen, \bfnm N. \binits N. ( \byear 2015). \btitle Community detection in sparse random networks. \bjournal Annals of Applied Probability \bvolume 25 \bpages 3465-3510. \endbibitem
- Bai and Saranadasa (1996) {barticle} [author] \bauthor \bsnm Bai, \bfnm Z. \binits Z. and \bauthor \bsnm Saranadasa, \bfnm H. \binits H. ( \byear 1996). \btitle Effect of high dimension: By an example of a two sample problem. \bjournal Statistica Sinica \bvolume 6 \bpages 311-329. \endbibitem
- Bandeira and van Handel (2016) {barticle} [author] \bauthor \bsnm Bandeira, \bfnm A. S. \binits A. S. and \bauthor \bparticle van \bsnm Handel, \bfnm R. \binits R. ( \byear 2016). \btitle Sharp nonasymptotic bounds on the norm of random matrices with independent entries. \bjournal Annals of Probability \bvolume 44 \bpages 2479-2506. \endbibitem
- Baraud (2002) {barticle} [author] \bauthor \bsnm Baraud, \bfnm Y. \binits Y. ( \byear 2002). \btitle Non-asymptotic minimax rates of testing in signal detection. \bjournal Bernoulli \bvolume 8 \bpages 577-606. \endbibitem
- Berger et al. (2005) {binproceedings} [author] \bauthor \bsnm Berger, \bfnm N. \binits N., \bauthor \bsnm Borgs, \bfnm C. \binits C., \bauthor \bsnm Chayes, \bfnm J. T. \binits J. T. and \bauthor \bsnm Saberi, \bfnm A. \binits A. ( \byear 2005). \btitle On the spread of viruses on the internet. In \bbooktitle Annual ACM-SIAM Symposium on Discrete Algorithms \bpages 301-310. \endbibitem
- Berthet and Rigollet (2013) {barticle} [author] \bauthor \bsnm Berthet, \bfnm Q. \binits Q. and \bauthor \bsnm Rigollet, \bfnm P. \binits P. ( \byear 2013). \btitle Optimal detection of sparse principal components in high dimension. \bjournal Annals of Statistics \bvolume 41 \bpages 1780-1815. \endbibitem
- Bickel and Sarkar (2016) {barticle} [author] \bauthor \bsnm Bickel, \bfnm P. J. \binits P. J. and \bauthor \bsnm Sarkar, \bfnm P. \binits P. ( \byear 2016). \btitle Hypothesis testing for automated community detection in networks. \bjournal Journal of the Royal Statistical Society: Series B (Statistical Methodology) \bvolume 78 \bpages 253-273. \endbibitem
- Bollobas, Janson and Riordan (2007) {barticle} [author] \bauthor \bsnm Bollobas, \bfnm B. \binits B., \bauthor \bsnm Janson, \bfnm S. \binits S. and \bauthor \bsnm Riordan, \bfnm O. \binits O. ( \byear 2007). \btitle The phase transition in inhomogeneous random graphs. \bjournal Random Structures & Algorithms \bvolume 31 \bpages 122. \endbibitem
- Bresler and Nagaraj (2018) {binproceedings} [author] \bauthor \bsnm Bresler, \bfnm G. \binits G. and \bauthor \bsnm Nagaraj, \bfnm D. \binits D. ( \byear 2018). \btitle Optimal Single Sample Tests for Structured versus Unstructured Network Data In \bbooktitle Conference on Learning Theory \bvolume PMLR 75 \bpages 1657-1690. \endbibitem
- Bubeck et al. (2016) {barticle} [author] \bauthor \bsnm Bubeck, \bfnm S. \binits S., \bauthor \bsnm Ding, \bfnm J. \binits J., \bauthor \bsnm Eldan, \bfnm R. \binits R. and \bauthor \bsnm Rácz, \bfnm M. Z. \binits M. Z. ( \byear 2016). \btitle Testing for high-dimensional geometry in random graphs. \bjournal Random Structures & Algorithms \bvolume 49 \bpages 503-532. \endbibitem
- Cai, Liu and Xia (2014) {barticle} [author] \bauthor \bsnm Cai, \bfnm T. T. \binits T. T., \bauthor \bsnm Liu, \bfnm W. \binits W. and \bauthor \bsnm Xia, \bfnm Y. \binits Y. ( \byear 2014). \btitle Two-sample test of high dimensional means under dependence. \bjournal Journal of the Royal Statistical Society: Series B (Statistical Methodology) \bvolume 76 \bpages 349-372. \endbibitem
- Carpentier and Nickl (2015) {barticle} [author] \bauthor \bsnm Carpentier, \bfnm A. \binits A. and \bauthor \bsnm Nickl, \bfnm R. \binits R. ( \byear 2015). \btitle On signal detection and confidence sets for low rank inference problems. \bjournal Electronic Journal of Statistics \bvolume 9 \bpages 2675-2688. \endbibitem
- Chan et al. (2014) {binproceedings} [author] \bauthor \bsnm Chan, \bfnm S. \binits S., \bauthor \bsnm Diakonikolas, \bfnm I. \binits I., \bauthor \bsnm Valiant, \bfnm G. \binits G. and \bauthor \bsnm Valiant, \bfnm P. \binits P. ( \byear 2014). \btitle Optimal Algorithms for Testing Closeness of Discrete Distributions In \bbooktitle Annual ACM-SIAM Symposium on Discrete Algorithms (SODA). \endbibitem
- Chen and Qin (2010) {barticle} [author] \bauthor \bsnm Chen, \bfnm S. X. \binits S. X. and \bauthor \bsnm Qin, \bfnm Y. L. \binits Y. L. ( \byear 2010). \btitle A two-sample test for high-dimensional data with applications to gene-set testing. \bjournal Annals of Statistics \bvolume 38 \bpages 808-835. \endbibitem
- Daskalakis, Dikkala and Kamath (2018) {binproceedings} [author] \bauthor \bsnm Daskalakis, \bfnm C. \binits C., \bauthor \bsnm Dikkala, \bfnm N. \binits N. and \bauthor \bsnm Kamath, \bfnm G. \binits G. ( \byear 2018). \btitle Testing Ising models In \bbooktitle Annual ACM-SIAM Symposium on Discrete Algorithms (SODA). \endbibitem
- Decelle et al. (2011) {barticle} [author] \bauthor \bsnm Decelle, \bfnm A. \binits A., \bauthor \bsnm Krzakala, \bfnm F. \binits F., \bauthor \bsnm Moore, \bfnm C. \binits C. and \bauthor \bsnm Zdeborová, \bfnm L. \binits L. ( \byear 2011). \btitle Asymptotic analysis of the stochastic block model for modular networks and its algorithmic applications. \bjournal Physical Review E \bvolume 84 \bpages 066106. \endbibitem
- Donoho and Jin (2004) {barticle} [author] \bauthor \bsnm Donoho, \bfnm D. \binits D. and \bauthor \bsnm Jin, \bfnm J. \binits J. ( \byear 2004). \btitle Higher criticism for detecting sparse heterogeneous mixtures. \bjournal Annals of Statistics \bvolume 32 \bpages 962-994. \endbibitem
- Donoho and Jin (2015) {barticle} [author] \bauthor \bsnm Donoho, \bfnm D. \binits D. and \bauthor \bsnm Jin, \bfnm J. \binits J. ( \byear 2015). \btitle Higher criticism for large-scale inference, Especially for Rare and Weak Effects. \bjournal Statistical Science \bvolume 30 \bpages 1-25. \endbibitem
- Gao and Lafferty (2017) {barticle} [author] \bauthor \bsnm Gao, \bfnm C. \binits C. and \bauthor \bsnm Lafferty, \bfnm J. \binits J. ( \byear 2017). \btitle Testing network structure using relations between small subgraph probabilities. \bjournal arXiv preprint \bvolume arXiv:1704.06742. \endbibitem
- Ghoshdastidar and von Luxburg (2018) {binproceedings} [author] \bauthor \bsnm Ghoshdastidar, \bfnm D. \binits D. and \bauthor \bparticle von \bsnm Luxburg, \bfnm U. \binits U. ( \byear 2018). \btitle Practical methods for graph two-sample testing. In \bbooktitle Advances in Neural Information Processing Systems \bvolume 31. \endbibitem
- Ghoshdastidar et al. (2017) {binproceedings} [author] \bauthor \bsnm Ghoshdastidar, \bfnm D. \binits D., \bauthor \bsnm Gutzeit, \bfnm M. \binits M., \bauthor \bsnm Carpentier, \bfnm A. \binits A. and \bauthor \bparticle von \bsnm Luxburg, \bfnm U. \binits U. ( \byear 2017). \btitle Two-sample tests for large random graphs using network statistics. In \bbooktitle Conference on Learning Theory \bvolume PMLR 65 \bpages 954-977. \endbibitem
- Ginestet et al. (2017) {barticle} [author] \bauthor \bsnm Ginestet, \bfnm C. E. \binits C. E., \bauthor \bsnm Li, \bfnm J. \binits J., \bauthor \bsnm Balachandran, \bfnm P. \binits P., \bauthor \bsnm Rosenberg, \bfnm S. \binits S. and \bauthor \bsnm Kolaczyk, \bfnm E. D. \binits E. D. ( \byear 2017). \btitle Hypothesis testing for network data in functional neuroimaging. \bjournal Annals of Applied Statistics \bvolume 11 \bpages 725-750. \endbibitem
- Gretton et al. (2012) {barticle} [author] \bauthor \bsnm Gretton, \bfnm A. \binits A., \bauthor \bsnm Borgwardt, \bfnm K. M. \binits K. M., \bauthor \bsnm Rasch, \bfnm M. J. \binits M. J., \bauthor \bsnm Schölkopf, \bfnm B. \binits B. and \bauthor \bsnm Smola, \bfnm A. \binits A. ( \byear 2012). \btitle A kernel two-sample test. \bjournal Journal of Machine Learning Research \bvolume 13 \bpages 723-733. \endbibitem
- Hyduke, Lewis and Palsson (2013) {barticle} [author] \bauthor \bsnm Hyduke, \bfnm D. R. \binits D. R., \bauthor \bsnm Lewis, \bfnm N. E. \binits N. E. and \bauthor \bsnm Palsson, \bfnm B. \binits B. ( \byear 2013). \btitle Analysis of omics data with genome-scale models of metabolism. \bjournal Molecular BioSystems \bvolume 9 \bpages 167-174. \endbibitem
- Ingster and Suslina (2003) {bbook} [author] \bauthor \bsnm Ingster, \bfnm Y. I. \binits Y. I. and \bauthor \bsnm Suslina, \bfnm I. A. \binits I. A. ( \byear 2003). \btitle Nonparametric goodness-of-fit testing under Gaussian models. \bseries Lecture Notes in Statistics \bvolume 169. \bpublisher Springer-Verlag New York. \endbibitem
- Klopp, Tsybakov and Verzelen (2017) {barticle} [author] \bauthor \bsnm Klopp, \bfnm O. \binits O., \bauthor \bsnm Tsybakov, \bfnm A. B. \binits A. B. and \bauthor \bsnm Verzelen, \bfnm N. \binits N. ( \byear 2017). \btitle Oracle inequalities for network models and sparse graphon estimation. \bjournal Annals of Statistics \bvolume 45 \bpages 316-354. \endbibitem
- Kondor and Pan (2016) {binproceedings} [author] \bauthor \bsnm Kondor, \bfnm R. \binits R. and \bauthor \bsnm Pan, \bfnm H. \binits H. ( \byear 2016). \btitle The multiscale Laplacian graph kernel. In \bbooktitle Advances in Neural Information Processing Systems. \endbibitem
- Landman et al. (2011) {barticle} [author] \bauthor \bsnm Landman, \bfnm B. A. \binits B. A., \bauthor \bsnm Huang, \bfnm A. J. \binits A. J., \bauthor \bsnm Gifford, \bfnm A. \binits A., \bauthor \bsnm Vikram, \bfnm D. S. \binits D. S., \bauthor \bsnm Lim, \bfnm I. A. \binits I. A., \bauthor \bsnm Farrell, \bfnm J. A. \binits J. A., \bauthor \bsnm Bogovic, \bfnm J. A. \binits J. A., \bauthor \bsnm Hua, \bfnm J. \binits J., \bauthor \bsnm Chen, \bfnm M. \binits M., \bauthor \bsnm Jarso, \bfnm S. \binits S., \bauthor \bsnm Smith, \bfnm S. A. \binits S. A., \bauthor \bsnm Joel, \bfnm S. \binits S., \bauthor \bsnm Mori, \bfnm S. \binits S., \bauthor \bsnm Pekar, \bfnm J. J. \binits J. J., \bauthor \bsnm Barker, \bfnm P. B. \binits P. B., \bauthor \bsnm Prince, \bfnm J. L. \binits J. L. and \bauthor \bsnm van Zijl, \bfnm P. C. \binits P. C. ( \byear 2011). \btitle Multi-parametric neuroimaging reproducibility: A 3-T resource study. \bjournal Neuroimage \bvolume 54 \bpages 2854-2866. \endbibitem
- Le, Levina and Vershynin (2017) {barticle} [author] \bauthor \bsnm Le, \bfnm C. M. \binits C. M., \bauthor \bsnm Levina, \bfnm E. \binits E. and \bauthor \bsnm Vershynin, \bfnm R. \binits R. ( \byear 2017). \btitle Concentration and regularization of random graphs. \bjournal Random Structures & Algorithms \bvolume 51 \bpages 538-561. \endbibitem
- Ledoit and Wolf (2002) {barticle} [author] \bauthor \bsnm Ledoit, \bfnm O. \binits O. and \bauthor \bsnm Wolf, \bfnm M. \binits M. ( \byear 2002). \btitle Some hypothesis tests for the covariance matrix when the dimension is large compared to the sample size. \bjournal Annals of Statistics \bvolume 30 \bpages 1081-1102. \endbibitem
- Lei (2016) {barticle} [author] \bauthor \bsnm Lei, \bfnm J. \binits J. ( \byear 2016). \btitle A goodness-of-fit test for stochastic block models. \bjournal Annals of Statistics \bvolume 44 \bpages 401-424. \endbibitem
- Lovász (2012) {bbook} [author] \bauthor \bsnm Lovász, \bfnm L. \binits L. ( \byear 2012). \btitle Large networks and graph limits. \bpublisher American Mathematical Society. \endbibitem
- Lu and Peng (2013) {barticle} [author] \bauthor \bsnm Lu, \bfnm L. \binits L. and \bauthor \bsnm Peng, \bfnm X. \binits X. ( \byear 2013). \btitle Spectra of edge-independent random graphs. \bjournal Electronic Journal of Combinatorics \bvolume 20 \bpages P27. \endbibitem
- Mukherjee, Pillai and Lin (2015) {barticle} [author] \bauthor \bsnm Mukherjee, \bfnm R. \binits R., \bauthor \bsnm Pillai, \bfnm N. S. \binits N. S. and \bauthor \bsnm Lin, \bfnm X. \binits X. ( \byear 2015). \btitle Hypothesis testing for high-dimensional sparse binary regression. \bjournal Annals of Statistics \bvolume 43 \bpages 352-381. \endbibitem
- Oliveira (2009) {barticle} [author] \bauthor \bsnm Oliveira, \bfnm R. I. \binits R. I. ( \byear 2009). \btitle Concentration of the adjacency matrix and of the Laplacian in random graphs with independent edges. \bjournal arXiv preprint \bvolume arXiv:0911.0600. \endbibitem
- Ramdas et al. (2015) {barticle} [author] \bauthor \bsnm Ramdas, \bfnm A. \binits A., \bauthor \bsnm Reddi, \bfnm S. \binits S., \bauthor \bsnm Poczos, \bfnm B. \binits B., \bauthor \bsnm Singh, \bfnm A. \binits A. and \bauthor \bsnm Wasserman, \bfnm L. \binits L. ( \byear 2015). \btitle Adaptivity and computation-statistics tradeoffs for kernel and distance based high dimensional two sample testing. \bjournal arXiv preprint \bvolume arXiv:1508.00655. \endbibitem
- Ryabko (2017) {binproceedings} [author] \bauthor \bsnm Ryabko, \bfnm D. \binits D. ( \byear 2017). \btitle Hypotheses testing on infinite random graphs In \bbooktitle Algorithmic Learning Theory. \endbibitem
- Shervashidze et al. (2011) {barticle} [author] \bauthor \bsnm Shervashidze, \bfnm N. \binits N., \bauthor \bsnm Schweitzer, \bfnm P. \binits P., \bauthor \bsnm van Leeuwen, \bfnm E. J. \binits E. J., \bauthor \bsnm Mehlhorn, \bfnm K. \binits K. and \bauthor \bsnm Borgwardt, \bfnm K. M. \binits K. M. ( \byear 2011). \btitle Weisfeiler-Lehman graph kernels. \bjournal Journal of Machine Learning Research \bvolume 12 \bpages 2539-2561. \endbibitem
- Stam et al. (2007) {barticle} [author] \bauthor \bsnm Stam, \bfnm C. J. \binits C. J., \bauthor \bsnm Jones, \bfnm B. F. \binits B. F., \bauthor \bsnm Nolte, \bfnm G. \binits G., \bauthor \bsnm Breakspear, \bfnm M. \binits M. and \bauthor \bsnm Scheltens, \bfnm P. \binits P. ( \byear 2007). \btitle Small-world networks and functional connectivity in Alzheimer’s disease. \bjournal Cerebral Cortex \bvolume 17 \bpages 92-99. \endbibitem
- Sun and Nobel (2008) {barticle} [author] \bauthor \bsnm Sun, \bfnm X. \binits X. and \bauthor \bsnm Nobel, \bfnm A. B. \binits A. B. ( \byear 2008). \btitle On the size and recovery of submatrices of ones in a random binary matrix. \bjournal Journal of Machine Learning Research \bvolume 9. \endbibitem
- Tang et al. (2017a) {barticle} [author] \bauthor \bsnm Tang, \bfnm M. \binits M., \bauthor \bsnm Athreya, \bfnm A. \binits A., \bauthor \bsnm Sussman, \bfnm D. L. \binits D. L., \bauthor \bsnm Lyzinski, \bfnm V. \binits V. and \bauthor \bsnm Priebe, \bfnm C. E. \binits C. E. ( \byear 2017a). \btitle A semiparametric two-sample hypothesis testing problem for random graphs. \bjournal Journal of Computational and Graphical Statistics \bvolume 26 \bpages 344-354. \bdoi 10.1080/10618600.2016.1193505 \endbibitem
- Tang et al. (2017b) {barticle} [author] \bauthor \bsnm Tang, \bfnm M. \binits M., \bauthor \bsnm Athreya, \bfnm A. \binits A., \bauthor \bsnm Sussman, \bfnm D. L. \binits D. L., \bauthor \bsnm Lyzinski, \bfnm V. \binits V. and \bauthor \bsnm Priebe, \bfnm C. E. \binits C. E. ( \byear 2017b). \btitle A nonparametric two-sample hypothesis testing problem for random graphs. \bjournal Bernoulli \bvolume 23 \bpages 1599-1630. \endbibitem
- Tropp (2012) {barticle} [author] \bauthor \bsnm Tropp, \bfnm J. A. \binits J. A. ( \byear 2012). \btitle User-friendly tail bounds for sums of random matrices. \bjournal Foundations of Computational Mathematics \bvolume 12 \bpages 389-434. \endbibitem
- Verzelen and Arias-Castro (2017) {barticle} [author] \bauthor \bsnm Verzelen, \bfnm N. \binits N. and \bauthor \bsnm Arias-Castro, \bfnm E. \binits E. ( \byear 2017). \btitle Detection and feature selection in sparse mixture Models. \bjournal Annals of Statistics \bvolume 45 \bpages 1920-1950. \endbibitem
- Vishwanathan et al. (2010) {barticle} [author] \bauthor \bsnm Vishwanathan, \bfnm S. V. N. \binits S. V. N., \bauthor \bsnm Schraudolph, \bfnm N. N. \binits N. N., \bauthor \bsnm Kondor, \bfnm R. \binits R. and \bauthor \bsnm Borgwardt, \bfnm K. M. \binits K. M. ( \byear 2010). \btitle Graph kernels. \bjournal Journal of Machine Learning Research \bvolume 11 \bpages 1201-1242. \endbibitem
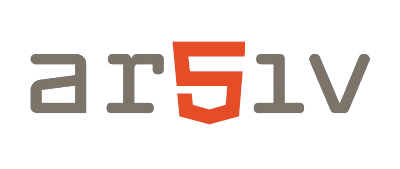
Two-sample Hypothesis Testing for Inhomogeneous Random Graphs
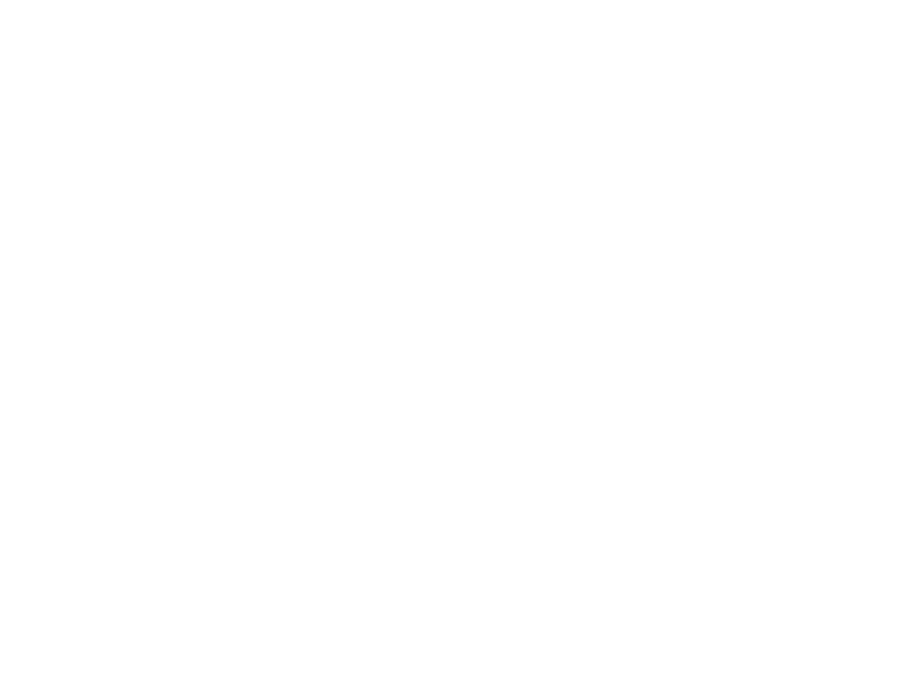
The study of networks leads to a wide range of high dimensional inference problems. In most practical scenarios, one needs to draw inference from a small population of large networks. The present paper studies hypothesis testing of graphs in this high-dimensional regime. We consider the problem of testing between two populations of inhomogeneous random graphs defined on the same set of vertices. We propose tests based on estimates of the Frobenius and operator norms of the difference between the population adjacency matrices. We show that the tests are uniformly consistent in both the "large graph, small sample" and "small graph, large sample" regimes. We further derive lower bounds on the minimax separation rate for the associated testing problems, and show that the constructed tests are near optimal.

Debarghya Ghoshdastidar
Maurilio Gutzeit
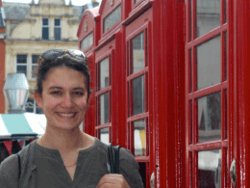
Alexandra Carpentier
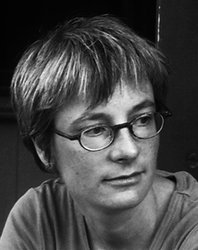
Ulrike von Luxburg
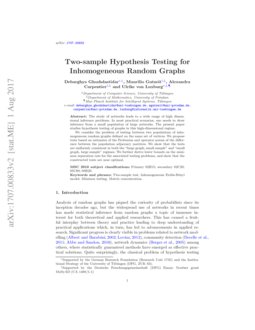
Related Research
Practical methods for graph two-sample testing, two-sample tests for large random graphs using network statistics, lost in the shuffle: testing power in the presence of errorful network vertex labels, profit-maximizing a/b tests, two-sample testing on latent distance graphs with unknown link functions, multi-level hypothesis testing for populations of heterogeneous networks, local two-sample testing over graphs and point-clouds by random-walk distributions.
Please sign up or login with your details
Generation Overview
AI Generator calls
AI Video Generator calls
AI Chat messages
Genius Mode messages
Genius Mode images
AD-free experience
Private images
- Includes 500 AI Image generations, 1750 AI Chat Messages, 30 AI Video generations, 60 Genius Mode Messages and 60 Genius Mode Images per month. If you go over any of these limits, you will be charged an extra $5 for that group.
- For example: if you go over 500 AI images, but stay within the limits for AI Chat and Genius Mode, you'll be charged $5 per additional 500 AI Image generations.
- Includes 100 AI Image generations and 300 AI Chat Messages. If you go over any of these limits, you will have to pay as you go.
- For example: if you go over 100 AI images, but stay within the limits for AI Chat, you'll have to reload on credits to generate more images. Choose from $5 - $1000. You'll only pay for what you use.
Out of credits
Refill your membership to continue using DeepAI
Share your generations with friends
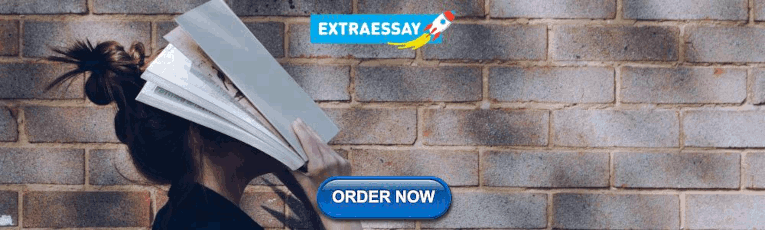
IMAGES
VIDEO
COMMENTS
studies hypothesis testing of graphs in this high-dimensional regime, where the goal is to test between two populations of inhomogeneous random graphs defined on the same set of n vertices. The size of each population m is much smaller than n, and can even be a constant as small as 1. The critical question in this context is whether the
The present paper studies hypothesis testing of graphs in this high-dimensional regime, where the goal is to test between two populations of inhomogeneous random graphs defined on the same set of n vertices. The size of each population m is much smaller than n, and can even be a constant as small as 1.
Let P P, Q Q be the population adjacencies of two sparse inhomogeneous random graph models, and d d be a suitably defined distance function. Given a population of m m graphs from each model, we derive minimax separation rates for the problem of testing P =Q P = Q against d(P,Q) >ρ d ( P, Q) > ρ. We observe that if m m is small, then the ...
Keywords and phrases: Two-sample test, Inhomogeneous Erdos-R´enyi model, Minimax testing, Matrix concentration. 1. Introduction Analysis of random graphs has piqued the curiosity of probabilists since its inception decades ago, but the widespread use of networks in recent times has made statistical inference from random graphs a topic of ...
DOI: 10.1214/19-AOS1884 Corpus ID: 88522483; Two-sample hypothesis testing for inhomogeneous random graphs @article{Ghoshdastidar2017TwosampleHT, title={Two-sample hypothesis testing for inhomogeneous random graphs}, author={Debarghya Ghoshdastidar and Maurilio Gutzeit and Alexandra Carpentier and Ulrike von Luxburg}, journal={Annals of Statistics}, year={2017}, volume={48}, pages={2208-2229 ...
AbstractWe consider a natural model of inhomogeneous random graphs that extends the classical Erdős-Rényi graphs and shares a close connection with the multiplicative coalescence, as pointed out by Aldous (Ann Probab 25:812-854, 1997). In this model, the vertices are assigned weights that govern their tendency to form edges.
@article{GhoshdastidarEtal20, title = {Two-sample Hypothesis Testing for Inhomogeneous Random Graphs}, author = {Ghoshdastidar, D. and Gutzeit, M. and Carpentier, A ...
Upload an image to customize your repository's social media preview. Images should be at least 640×320px (1280×640px for best display).
The present paper studies hypothesis testing of graphs in this high-dimensional regime, where the goal is to test between two populations of inhomogeneous random graphs defined on the same set of n vertices. The size of each population m is much smaller than n, and can even be a constant as small as 1. The critical question in this context is ...
Request PDF | On Aug 1, 2020, Debarghya Ghoshdastidar and others published Two-sample hypothesis testing for inhomogeneous random graphs | Find, read and cite all the research you need on ResearchGate
The study of networks leads to a wide range of high dimensional inference problems. In many practical applications, one needs to draw inference from one or few large sparse networks. The present paper studies hypothesi…
This paper studies hypothesis testing of graphs in the above regime. We consider the problem of testing between two populations of inhomogeneous random graphs defined on the same set of vertices.
Proceedings of The 26th International Conference on Artificial Intelligence and Statistics, PMLR 206:6903-6911, 2023.
The present paper studies hypothesis testing of graphs in this high-dimensional regime. We consider the problem of testing between two populations of inhomogeneous random graphs defined on the same set of vertices. We propose tests based on estimates of the Frobenius and operator norms of the difference between the population adjacency matrices ...
The optimal sample complexity for testing in the L r norm, for all integers r ≥ 1 is obtained, and the asymptotic distribution of the optimal tests under H 0 is derived and a method for consistently estimating their variances is developed. In this paper we study the two-sample problem for inhomogeneous Erd˝os-R´enyi (IER) random graph models in the L r norm, in the high-dimensional regime ...
Two-Sample Tests for Inhomogeneous Random Graphs in L r Norm: Optimality and Asymptotics Definition 1.1. [3] Given a symmetric matrix P(n) 2 [0;1] n with zeroes on the diagonal, a graph Gis said to be an inhomogeneous Erdos-R˝ ´enyi (IER) random graph with edge probability P(n) = ((p ij)) 2[0;1] n, de-noted as G˘IER(P(n)), if its symmetric ...
ABSTRACT. Two-sample hypothesis testing for random graphs arises naturally in neuroscience, social networks, and machine learning. In this article, we consider a semiparametric problem of two-sample hypothesis testing for a class of latent position random graphs.
Two-sample hypothesis testing for inhomogeneous random graphs @article{Ghoshdastidar2017TwosampleHT, title={Two-sample hypothesis testing for inhomogeneous random graphs}, author={Debarghya Ghoshdastidar and Maurilio Gutzeit and Alexandra Carpentier and Ulrike von Luxburg}, journal={Annals of Statistics}, year={2017}, volume={48}, pages={2208 ...
The study of networks leads to a wide range of high-dimensional inference problems. In many practical applications, one needs to draw inference from one or few large ...
from one or few large sparse networks. The present paper studies hypothesis testing of graphs in this high-dimensional regime, where the goal is to test between two populations of inhomogeneous random graphs defined on the same set of n vertices. The size of each population m is much smaller than n, and can even be a constant as small as 1.
The study of networks leads to a wide range of high dimensional inference problems. In many practical applications, one needs to draw inference from one or few large sparse networks. The present paper studies hypothesis testing of graphs in this high-dimensional regime, where the goal is to test between two populations of inhomogeneous random graphs defined on the same set of n vertices. The ...