RESEARCH DESIGN AND METHODS
Conclusions, acknowledgments, influence of type 2 diabetes on brain volumes and changes in brain volumes : results from the women’s health initiative magnetic resonance imaging studies.
A list of participants in the WHIMS-MRI Study Group can be found in the A ppendix .
- Split-Screen
- Article contents
- Figures & tables
- Supplementary Data
- Peer Review
- Open the PDF for in another window
- Cite Icon Cite
- Get Permissions
Mark A. Espeland , R. Nick Bryan , Joseph S. Goveas , Jennifer G. Robinson , Mustafa S. Siddiqui , Simin Liu , Patricia E. Hogan , Ramon Casanova , Laura H. Coker , Kristine Yaffe , Kamal Masaki , Rebecca Rossom , Susan M. Resnick , for the WHIMS-MRI Study Group; Influence of Type 2 Diabetes on Brain Volumes and Changes in Brain Volumes : Results from the Women’s Health Initiative Magnetic Resonance Imaging Studies . Diabetes Care 1 January 2013; 36 (1): 90–97. https://doi.org/10.2337/dc12-0555
Download citation file:
- Ris (Zotero)
- Reference Manager
To study how type 2 diabetes adversely affects brain volumes, changes in volume, and cognitive function.
Regional brain volumes and ischemic lesion volumes in 1,366 women, aged 72–89 years, were measured with structural brain magnetic resonance imaging (MRI). Repeat scans were collected an average of 4.7 years later in 698 women. Cross-sectional differences and changes with time between women with and without diabetes were compared. Relationships that cognitive function test scores had with these measures and diabetes were examined.
The 145 women with diabetes (10.6%) at the first MRI had smaller total brain volumes (0.6% less; P = 0.05) and smaller gray matter volumes (1.5% less; P = 0.01) but not white matter volumes, both overall and within major lobes. They also had larger ischemic lesion volumes (21.8% greater; P = 0.02), both overall and in gray matter (27.5% greater; P = 0.06), in white matter (18.8% greater; P = 0.02), and across major lobes. Overall, women with diabetes had slightly (nonsignificant) greater loss of total brain volumes (3.02 cc; P = 0.11) and significant increases in total ischemic lesion volumes (9.7% more; P = 0.05) with time relative to those without diabetes. Diabetes was associated with lower scores in global cognitive function and its subdomains. These relative deficits were only partially accounted for by brain volumes and risk factors for cognitive deficits.
Diabetes is associated with smaller brain volumes in gray but not white matter and increasing ischemic lesion volumes throughout the brain. These markers are associated with but do not fully account for diabetes-related deficits in cognitive function.
Many interrelated factors adversely affect the brain health of individuals with type 2 diabetes, including energy dysregulation, inflammation, reduced perfusion, and increased oxidative stress and protein deposition ( 1 , 2 ). In cross-sectional studies, type 2 diabetes is often associated with smaller brain volumes and, less consistently, with greater amounts of white matter hyperintensities and other markers of cerebrovascular disease ( 3 , 4 ). Two longitudinal magnetic resonance imaging (MRI) studies have documented increased rates of total brain atrophy, which appeared to be greater among individuals with lower cognitive function, but not increased accumulations of white matter hyperintensities ( 5 , 6 ). The relative increases in atrophy associated with diabetes remained after covariate adjustment for many risk factors for cognitive dysfunction, including age, blood pressures, education, lipid levels, and BMI.
This article is organized with three aims. In a large cohort of older women, we first describe the cross-sectional associations that diabetes had with brain tissue volumes and ischemic lesion loads. We do so for the whole brain and separately for white matter, gray matter, and major lobes. Second, among the women assessed longitudinally with MRI, we examine whether changes in brain tissue and ischemic lesion volumes varied according to diabetes status. Finally, we examine associations that brain volumes and ischemic lesion volumes had with global cognitive function and its subdomains and examine the degree to which they account for diabetes-related relative deficits. There has been one report that diabetes is associated with greater adverse effects on changes in brain structure among women than men ( 5 ), perhaps because diabetes often co-occurs with relatively more vascular risk factors among women with versus without diabetes than is the case among men ( 7 ). We analyze data from the first sufficiently large cohort of women to characterize the extent to which diabetes-related brain changes account for relative cognitive deficits.
This article draws data from the MRI component of the Women’s Health Initiative (WHI) Memory Study (WHIMS-MRI). Volunteers were recruited from 14 U.S. academic centers. These women had participated in the Women’s Health Initiative Memory Study (WHIMS), an ancillary study to the WHI (which consisted of parallel, placebo-controlled, randomized clinical trials of 0.625 mg/day conjugated equine estrogens with and without 2.5 mg/day medroxyprogesterone acetate in women with a uterus or posthysterectomy) ( 8 ). At enrollment into WHIMS, women were 65 to 80 years of age and free of dementia.
WHIMS-MRI was designed to contrast MRI outcomes among women who had been assigned to active versus placebo therapy ( 9 – 11 ). Exclusion criteria included presence of pacemakers, other implants, and foreign bodies, along with other contraindications to MRI. These women’s mean age at scanning, which occurred in 2005–2007, was 78.5 (SD 3.7) years. In 2008–2010, the women were invited to return for a second MRI. Once eligibility had been reconfirmed, scans were repeated according to an identical protocol. Written, informed consent was obtained for each MRI; the National Institutes of Health and the institutional review boards of participating institutions approved the protocols and consent forms.
At WHI enrollment, women self-reported a history of diabetes when not pregnant or of diabetes treatment. Fasting blood glucose was determined for a 5% sample. During WHI follow-up, women were periodically queried about diabetes treatment, and they brought their prescription medications to clinic visits to be recorded 1, 3, 6, and 9 years after enrollment ( 12 ). Women were classified as having diabetes on the basis of self-report of diabetes, self-report of diabetes treatment, or, for those with fasting glucose measurements, levels exceeding 126 mg/dL. One woman who reported diabetes onset before the age of 30 years and who was currently taking insulin was considered to have probable type 1 diabetes and therefore excluded from this analysis.
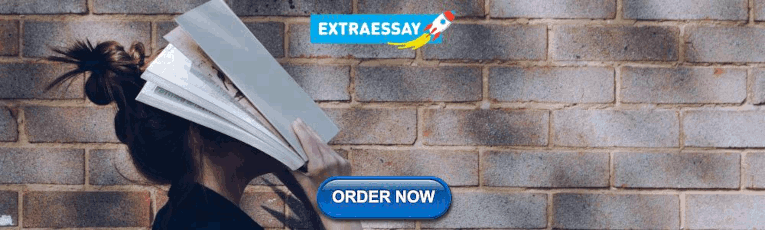
Risk factors for MRI outcomes
Risk factors for declines in structural brain MRI outcomes included in our analyses were those that may also be related to risk or co-occurrence of diabetes: age, education, race or ethnicity, BMI, waist girth, alcohol use, blood pressure, previous cardiovascular disease, previous stroke, and WHI treatment assignment. These were collected from self-reports and standardized assessments during WHI enrollment and annual follow-up visits at schedules that varied among measures. We used values from the most recent assessment before the MRI in our analyses.
MRI outcomes
Technicians trained on the study protocol collected the MRI scans. Regional brain volumes and ischemic lesion loads (i.e., volumes) were measured centrally at the WHIMS-MRI Quality Control Center (see appendix ). Standardized and validated protocols were used for obtaining and processing MRI scans and for measuring volumes ( 10 , 11 , 13 ). Series were acquired with field of view of 22 cm and matrix size of 256 × 256. They included oblique axial spin density/T2-weighted spin echo (repetition time [TR] = 3,200 ms, echo time [TE] = 30/120 ms, slice thickness = 3 mm), fluid attenuation inversion recovery (FLAIR) T2-weighted spin echo (TR = 8,000 ms, inversion time [TI] = 2,000 ms, TE = 100 ms, slice thickness = 3 mm), and axial three-dimensional spoiled gradient recalled T1-weighted gradient echo (TR = 21 ms, TE = 8 ms, flip angle = 30°, slice thickness = 1.5 mm) images from the vertex to skull base parallel to the anterior commissure–posterior commissure plane. T1-weighted volumetric MRI scans were first preprocessed according to a standardized protocol for alignment, removal of extracranial material, and segmentation of brain into gray and white parenchyma and cerebrospinal fluid. Regional volumetric measurements were obtained with an automated computer-based template warping method that summed the number of respective voxels falling within each anatomical region of interest (ROI). Intracranial volume was estimated as the total cerebral hemispheric volumes plus cerebrospinal fluid within the ventricular and sulcal spaces. After additional preprocessing steps, including histogram standardization and coregistration, the ischemic lesion segmentation component of the algorithm was applied, based on local signal features extracted from coregistered multiparametric MRI sequences (i.e., T1, proton density, T2, and FLAIR). A support vector machine classifier was first trained on expert-defined small-vessel ischemic disease (SVID) lesions in 45 cases from the Action to Control Cardiovascular Risk in Diabetes—Memory in Diabetes (ACCORD-MIND) study ( 14 ) and then used to classify SVID in new scans. For algorithm training purposes, SVID was operationally defined as a nonmass lesion having FLAIR signal greater than that of normal gray matter in a vascular distribution. The computer-assisted methodology was validated against manual segmentation, (i.e., manual drawing of ROIs) by an experienced expert neuroradiologist ( 13 ) and has been used successfully in other cohorts ( 14 – 17 ).
All supratentorial brain tissue was classified as normal or abnormal (ischemic) gray or white matter and assigned to one of 92 anatomical ROIs of the cerebrum ( 13 , 14 ). These ROIs were organized in an anatomically hierarchical system that was collapsed into anatomical regions for this analysis—total brain, gray matter, white matter, and four lobes (frontal, occipital, parietal, and temporal). We analyzed the volumes and the ischemic lesion volumes within each of these lobes and also the volume of ventricular cerebrospinal fluid.
We originally reported outcomes from 1,403 baseline MRIs on the basis of an earlier protocol for measurement ( 10 , 11 ). Baseline and follow-up MRIs were subsequently reprocessed with a refined image analysis protocol. This report is based on 1,366 of the original baseline MRIs (97.4%) and 698 follow-up MRIs of women for whom type 2 diabetes status was recorded.
Tests of cognitive function and classification of cognitive impairment
Global cognitive function was assessed with the Modified MiniMental State (3MS) examination, administered annually by trained and certified technicians from WHIMS enrollment until the first MRI ( 8 , 18 ). Possible scores ranged from 0 to 100, with a higher score reflecting better cognitive functioning. A factor analysis of WHIMS 3MS scores yielded four major components: 1 ) verbal memory and verbal fluency, 2 ) language and executive function, 3 ) orientation, and 4 ) language and praxis ( 19 ). We used the 3MS score collected most recently before the first MRI.
Statistical methods
General linear models with covariate adjustment were fitted to assess differences in volumes between women with and without diabetes at WHIMS-MRI enrollment with a model that included main effects for diabetes status, covariates, an interaction term between diabetes status, and a variable for time that took on a value of 0 at the initial MRI scan and the time between scans for the second MRI. A compound symmetry model was used for intrasubject correlations. In this model, changes in volumes occurred as a linear function of time between scans, and the rates of these changes were allowed to vary between women with and without diabetes. The advantage of this model is that it used all available data to estimate mean differences in volumes at the time of the first MRI. A supporting analysis limited to only volumes collected on the first MRI yielded similar results and is not reported. Because the distribution of ischemic lesion volumes was right skewed, an offset logarithm transformation was used to provide a more symmetrical distribution for analysis by taking the logarithm of the sum of the measured volume plus 1. Changes in volumes between the first and second MRI were computed, and differences in mean changes were described with analyses of covariance. Changes in ischemic lesion volumes were also highly skewed. We used the log-transformed values described here and applied linear regression to characterize the effect of diabetes on the second MRI measure, after adjustment for the log-transformed value of the baseline MRI. All models included age, clinic site, time from WHI enrollment, and time between scans as covariates. In addition, because a difference in intracranial volume was detected between the cross-sectional diabetes groups, this measure was also included as a covariate in their analyses.
At the first examination, standardized MRI measures were obtained from 145 women with recorded diabetes and from 1,221 without; these women comprise the cross-sectional cohort. A second standardized scan was obtained for 58 of the 145 women from the cross-sectional cohort with diabetes (40.4%) and for 640 of the women with diabetes (52.4%); this subset of women comprises the longitudinal cohort. Women for whom a second scan was not obtained (i.e., who were in the cross-sectional cohort but not the longitudinal cohort) tended to be older (mean of 79.0 vs. 78.1 years; P < 0.001), were less likely to be white (89.3 vs. 93.6%; P = 0.002), and tended to have lower 3MS scores (mean of 95.4 vs. 97.0; P < 0.001), but did not differ significantly ( P > 0.05) otherwise.
Within the cross-sectional cohort ( Table 1 ), women with diabetes were more likely to be African American, report no alcohol consumption, and have cardiovascular disease. They also had higher mean BMI and waist circumference and lower mean diastolic blood pressure. These associations were also evident among the women in the longitudinal cohort, although statistical significance was attenuated with the smaller sample size. Mean global cognitive function, as measured by the 3MS, was significantly lower among women with diabetes, as were means for each of the four components of cognitive function identified through factor analysis.
Distribution of risk factors for atrophy and cerebrovascular disease by diabetes status among women enrolled in WHIMS-MRI at the most recent assessment before first MRI
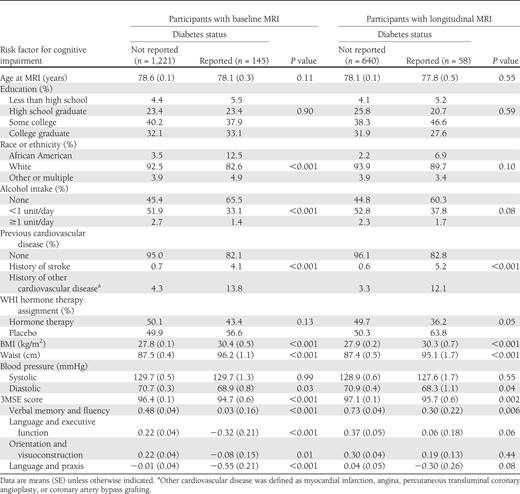
As seen in Table 2 , the initial MRI scans were collected an average of 8.0 years after enrollment in the WHI for women with and without diabetes in the cross-sectional and longitudinal cohorts. The second MRI scans were obtained an average of 4.7 years after the first for women with and without diabetes in the longitudinal cohort. In the cross-sectional cohort, the mean intracranial volume of women with diabetes was about 24 cc lower than that in women without diabetes ( P = 0.005).
Time frames and intracranial volumes for women without and with diabetes
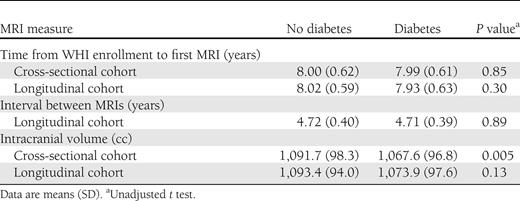
Table 3 provides mean volumes at the first MRI from general linear models with adjustment for age, clinic site, WHI treatment assignment, time from WHI enrollment, and intracranial volume. Volumes tended to be lower among women with diabetes for total brain (−0.6%; P = 0.05) and gray matter (−1.5%; P = 0.01). Among the four lobes we analyzed, differences in overall volumes between women with and without diabetes did not reach statistical significance, as seen in Table 3 . As noted in the footnote to Table 3 , we separately examined gray matter within each of the lobes and found evidence for diabetes-related deficits in gray matter within each: frontal, −1.3% ( P = 0.06); occipital, −1.4% ( P = 0.06); parietal, −2.5% ( P = 0.004); and temporal, −1.7% ( P = 0.02). Mean white matter volume was not related to diabetes overall or in any lobe. Mean ventricular volume was 7.4% larger among women with diabetes ( P = 0.05).
Covariate-adjusted relationships of regional brain volumes and ischemic lesion volumes with diabetes from analyses of all women
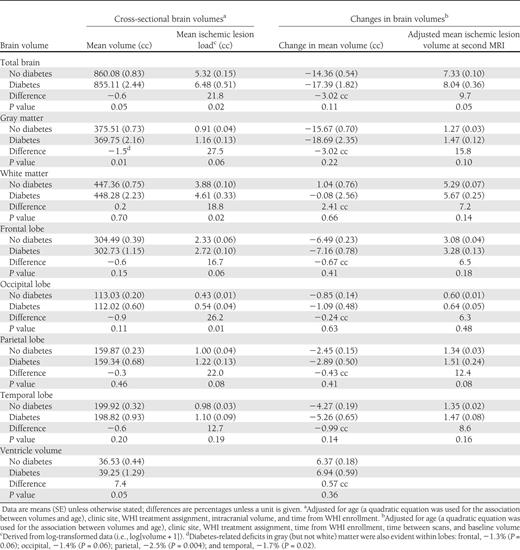
Mean ischemic lesion loads were consistently greater among women with diabetes throughout all regions and in both gray and white matter. These differences reached P < 0.05 for the total brain (21.8%; P = 0.02), white matter (18.8%; P = 0.02), and occipital lobe (26.2%; P = 0.01). Additional covariate adjustment for all factors in Table 1 did not materially affect the magnitudes of these estimated differences, which also were consistent with findings when analyses were restricted to white women (data not shown).
After similar covariate adjustment, fitted mean (SE) changes in total brain volumes between MRIs were −14.36 cc (0.54) for women without diabetes and −17.39 cc (1.82) for women with diabetes ( P = 0.11). Across regions, mean volumes tended to decrease more rapidly and ventricular volume to increase more rapidly among women with diabetes; however, no differences were statistically significant ( P > 0.05). After adjustment for baseline levels, the covariate-adjusted total brain ischemic lesion loads at the second MRI were 7.33 cc (0.10) for women without diabetes compared with 8.04 cc (0.36) for women with diabetes ( P = 0.05). This trend toward increased ischemic lesion volumes among women with diabetes was apparent in both white and gray matter and for several lobes but did not reach statistical significance for these measures ( P > 0.05).
Poorer cognitive function, as evidenced by lower 3MS scores, was correlated with smaller brain volumes ( r = −0.08; P < 0.001), larger ischemic lesion volumes ( r = 0.06; P = 0.006), greater loss in brain volume ( r = 0.10; P = 0.006), and greater increase in ischemic lesion volume ( r = 0.15; P < 0.001) in models with covariate adjustment for intracranial volume. We fitted three models with varying levels of covariate adjustment to describe relationships that diabetes had with deficits in global and domain-specific cognitive function, expressing these in standard deviation units to facilitate comparisons ( Table 4 ). After adjustment for age and WHI treatment assignment, the mean deficit for 3MS scores was 0.41 SD units (SE 0.09; ( P < 0.001), and deficits were seen in each subdomain, ranging from 0.23 SD units (0.09) for orientation to 0.40 SD units (0.09) for language and praxis (all P < 0.001). Covariate adjustment for all MRI volumes and ischemic lesion volumes (total and regional) attenuated the mean diabetes-related deficits only slightly (model 2). Additional adjustment for all other risk factors for cognitive impairment in Table 1 , further attenuated diabetes-related differences; however, these remained highly significant for all cognitive measures except orientation.
Deficits in mean 3MSE scores and principal factor scores associated with diabetes with varying levels of covariate adjustment
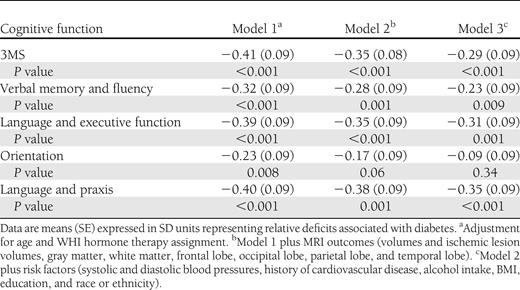
The analyses described here yielded three principal findings, which we will discuss in turn. First, in a large cohort of older women, diabetes was independently associated with significantly smaller volumes of gray matter but not white matter, significantly greater ventricular volumes, and significantly greater ischemic lesion loads throughout the brain. Second, diabetes was associated with trends toward greater progression of ischemic lesion loads and loss of total brain volumes throughout the brain but not loss of white matter during 4.7 years of average follow-up. Finally, although lower brain volumes and greater ischemic lesion volumes were all related to poorer cognitive function, these MRI measures did not fully account for the diabetes-related deficits in cognitive function. Significant diabetes-related deficits in cognitive deficits remained after adjustments for MRI measures and other factors in Table 1 .
Associations of diabetes with brain volumes and ischemic lesion loads
There are numerous reports linking diabetes with lower brain volumes later in life. In a 2006 systematic review, van Harten et al. ( 3 ) found consistent associations across many brain regions, and more recent reports add support ( 6 , 20 – 24 ). Our finding that these smaller volumes are limited to gray matter agrees with two prior reports of marked diabetes-related decrements in gray but not white matter volumes ( 25 , 26 ). The impact of diabetes on ischemic lesion loads and white matter hyperintensities has been less consistently shown, perhaps because of differences in measurement protocols and definitions ( 3 , 27 ). Certainly many shared risk factors and metabolic pathways would be expected to link diabetes with increased levels of ischemic lesion loads, including impaired perfusion and increased inflammation ( 2 , 28 ). Adjustment for BMI, waist circumference, blood pressure, previous cardiovascular disease, education, race or ethnicity, and alcohol intake did not materially affect these relationships, suggesting that the impact of diabetes on brain volumes and ischemic lesion loads in our study was not channeled through these risk factors.
Associations of diabetes with changes in brain volumes and ischemic lesion loads
Comparisons in the rates of changes in brain volumes and ischemic lesion loads between individuals with and without diabetes have been reported from two previous cohorts. The Utrecht Diabetic Encephalopathy Study collected brain MRIs 4 years apart from 55 individuals with diabetes and 28 controls with a mean age of 65 years and found greater increases in ventricular volumes but no significant differences in the rates of total brain volumes or white matter hyperintensities ( 29 ). Subgroup analyses found that the increase in ventricular volume occurred in women but not men. The Prospective Study of Pravastatin in the Elderly at Risk (PROSPER) trial collected brain MRIs 3 years apart from 89 individuals with diabetes and 438 controls with a mean age of 75 years and found increased rates of total brain atrophy but no increases in white matter hyperintensity volumes in association with diabetes ( 6 ). We found some evidence for increased rates of overall atrophy in our cohort across 4.7 years among women with diabetes, although the difference did not reach statistical significance; however, we did see stronger evidence of increased ischemic lesion loads. These differences remained after extensive covariate adjustment. Our cohort was slightly older than the previous studies, and our measurement protocol was different, which may have influenced our findings. It may also be the case that the effects of diabetes on brain structure are greater among women. Another possibility is that diabetes-related increases in ischemic lesion volumes occur later in life, which is consistent with Sonnen et al. ( 30 ), who found diabetes-related markers of cerebrovascular disease to be more pronounced among individuals in later stages of cognitive decline (i.e., with dementia).
Relationships of MRI measures with global cognitive function and its subdomains
Diabetes has been repeatedly documented to be associated with relative deficits in global and domain-specific cognitive function, similar to those that we report ( 2 , 31 ). Adverse cross-sectional and longitudinal MRI findings are also related to poorer cognitive function individuals with and without diabetes ( 29 , 32 , 33 ). Including MRI outcomes as covariates attenuated the estimated diabetes-related deficits only slightly, which is consistent with other reports ( 31 ). Inclusion of demographic markers and risk factors such as cardiovascular disease, alcohol use, waist girth, BMI, and blood pressures similarly did not fully account for these deficits. Although other candidates for mediation, such as apolipoprotein E status and proinflammatory cytokines, were not available, our findings suggest that factors not tightly linked to MRI volumes may be in play. One possibility is dysregulation of glucose metabolism. Insulin plays a central role in maintaining normal cognitive and brain function in older adults, and insulin dysregulation has been implicated in the pathophysiology of mild cognitive impairment, Alzheimer disease, and vascular dementia ( 34 , 35 ). Chronic insulin resistance and impaired glucose tolerance have been reported in mild cognitive impairment and early Alzheimer disease. Peripheral hyperinsulinemia and low brain insulin concentrations may reduce β-amyloid clearance and also promote inflammatory response. Recent observations have suggested that lower brain concentrations of insulin and reduced insulin receptors may be associated with increased incidence of Alzheimer disease; however, variable results on the effects of oral hypoglycemic agents on β-amyloid production have been reported in animal studies, and findings from recent human clinical trials have not been encouraging ( 36 , 37 – 39 ). Aggressive pharmacological management of diabetes may have mixed effects on brain structure, marginally reducing atrophy but increasing ischemic lesion volumes ( 14 ). Insulin given intranasally has shown significant promise in early mild cognitive impairment and Alzheimer disease clinical trials ( 40 ).
Limitations
Our sample is drawn from former volunteers in a trial of postmenopausal hormone therapy and may not represent general populations. Follow-up MRIs were obtained for about half of the original cohort, and women who did return differed from those who did not in several characteristics. Diabetes status for some women was based on self-report, and reliable data on duration of diabetes were not available. Our MRI protocol is different from some other studies that we cite, but it included well-validated quantitative measures of brain tissue and ischemic lesion volumes. The covariates available to us did not include some related to potential mechanisms (i.e., cholesterol levels, inflammatory markers, and insulin and glucose levels). We did not attempt to control for medication use (e.g., aspirin or statins), which likely varied with time. It is also possible that observed relationships may have been attenuated by measurement error.
Many processes adversely influence brain health and ultimately increase the risks of cognitive impairment with diabetes. Cognitive deficits emerge early in diabetes, and perhaps in prediabetes, and are maintained and may increase with time ( 31 ). In this large and diverse cohort of women, gray matter was decreased and accumulation of ischemic lesions was accelerated. Large scale measures of brain structure and changes in brain structure were modestly correlated with cognitive function but only partially explained diabetes-related deficits.
WHIMS-MRI Clinical Centers
Albert Einstein College of Medicine, Bronx, NY: Sylvia Wassertheil-Smoller, Mimi Goodwin, Richard DeNise, Michael Lipton, James Hannigan, Anthony Carpini, David Noble, Wilton Guzman; Medical College of Wisconsin, Milwaukee: Jane Morley Kotchen, Joseph Goveas, Diana Kerwin, John Ulmer, Steve Censky, Troy Flinton, Tracy Matusewic, Robert Prost; Stanford Center for Research in Disease Prevention, Stanford University, CA: Marcia L. Stefanick, Sue Swope, Anne Marie Sawyer-Glover, Susan Hartley; The Ohio State University, Columbus: Rebecca Jackson, Rose Hallarn, Bonnie Kennedy, Jill Bolognone, Lindsay Casimir, Amanda Kochis; University of California at Davis, Sacramento: John Robbins, Sophia Zaragoza, Cameron Carter, John Ryan, Denise Macias, Jerry Sonico; University of California at Los Angeles: Lauren Nathan, Barbara Voigt, Pablo Villablanca, Glen Nyborg, Sergio Godinez, Adele Perrymann; University of Florida, Gainesville/Jacksonville: Marian Limacher, Sheila Anderson, Mary Ellen Toombs, Jeffrey Bennett, Kevin Jones, Sandy Brum, Shane Chatfield, Kevin Vantrees; University of Iowa, Davenport: Jennifer Robinson, Candy Wilson, Kevin Koch, Suzette Hart, Jennifer Carroll, Mary Cherrico; University of Massachusetts, Worcester: Judith Ockene, Linda Churchill, Douglas Fellows, Anthony Serio, Sharon Jackson, Deidre Spavich; University of Minnesota, Minneapolis: Karen Margolis, Cindy Bjerk, Chip Truwitt, Margaret Peitso, Alexa Camcrena, Richard Grim, Julie Levin, Mary Perron; University of Nevada, Reno: Robert Brunner, Ross Golding, Leslie Pansky, Sandie Arguello, Jane Hammons, Nikki Peterson; University of North Carolina, Chapel Hill: Carol Murphy, Maggie Morgan, Mauricio Castillo, Thomas Beckman, Benjamin Huang; University of Pittsburgh, PA: Lewis Kuller, Pat McHugh, Carolyn Meltzer, Denise Davis, Joyce Davis, Piera Kost, Kim Lucas, Tom Potter, Lee Tarr.
WHIMS-MRI Clinical Coordinating Center
Wake Forest University Health Sciences, Winston-Salem, NC: Sally Shumaker, Mark Espeland, Laura Coker, Jeff Williamson, Debbie Felton, LeeAnn Gleiser, Steve Rapp, Claudine Legault, Maggie Dailey, Ramon Casanova, Julia Robertson, Patricia Hogan, Sarah Gaussoin, Pam Nance, Cheryl Summerville, Ricardo Peral, Josh Tan.
WHIMS-MRI Quality Control Center
University of Pennsylvania, Philadelphia: Nick Bryan, Christos Davatzikos, Lisa Desiderio.
U.S. National Institutes of Health
National Institute on Aging, Bethesda, MD: Neil Buckholtz, Susan Molchan, Susan Resnick; National Heart, Lung, and Blood Institute, Bethesda, MD, Jacques Rossouw, Linda Pottern.
A slide set summarizing this article is available online.
The Women’s Health Initiative is funded by the National Heart, Lung, and Blood Institute of the National Institutes of Health, U.S. Department of Health and Human Services. Contracts HHSN-268200464221C and N01-WH-4-4221 provided additional support. S.M.R. is supported by the Intramural Research Program, National Institute on Aging, National Institutes of Health. The Women’s Health Initiative Memory Study was funded in part by Wyeth Pharmaceuticals, Inc, St. Davids, PA. No other potential conflicts of interest relevant to this article were reported.
M.A.E. performed analyses and wrote the manuscript. R.N.B. oversaw data collection and coauthored the manuscript. J.S.G., J.G.R., M.S.S., and S.L. coauthored sections of the manuscript. P.E.H. performed analyses and coauthored sections of the manuscript. R.C., L.H.C., K.Y., K.M., and R.R. collaborated on the drafting of the manuscript, reviewed the work, and provided critical input. S.M.R. participated fully in the development and writing of this manuscript. M.A.E. is the guarantor of this work and, as such, had full access to all the data in the study and takes responsibility for the integrity of the data and the accuracy of the data analysis.
Parts of this study were presented in abstract form at the American Diabetes Association Scientific Sessions, Philadelphia, Pennsylvania, 8 – 12 June 2012.
Supplementary data
Email alerts.
- Online ISSN 1935-5548
- Print ISSN 0149-5992
- Diabetes Care
- Clinical Diabetes
- Diabetes Spectrum
- Standards of Medical Care in Diabetes
- Scientific Sessions Abstracts
- BMJ Open Diabetes Research & Care
- ShopDiabetes.org
- ADA Professional Books
Clinical Compendia
- Clinical Compendia Home
- Latest News
- DiabetesPro SmartBrief
- Special Collections
- DiabetesPro®
- Diabetes Food Hub™
- Insulin Affordability
- Know Diabetes By Heart™
- About the ADA
- Journal Policies
- For Reviewers
- Advertising in ADA Journals
- Reprints and Permission for Reuse
- Copyright Notice/Public Access Policy
- ADA Professional Membership
- ADA Member Directory
- Diabetes.org
- X (Twitter)
- Cookie Policy
- Accessibility
- Terms & Conditions
- Get Adobe Acrobat Reader
- © Copyright American Diabetes Association
This Feature Is Available To Subscribers Only
Sign In or Create an Account
- Search Menu
- The Journals of Gerontology, Series A (1995-present)
- Journal of Gerontology (1946-1994)
- Advance Articles
- Editor's Choice
- Translational articles
- Supplements
- Special Issues
- Calls for Papers
- Author Guidelines
- Biological Sciences Submission Site
- Medical Sciences Submission Site
- Why Submit to the GSA Portfolio?
- Advertising and Corporate Services
- Advertising
- Reprints and ePrints
- Sponsored Supplements
- Journals Career Network
- About The Journals of Gerontology, Series A
- About The Gerontological Society of America
- Editorial Board - Biological Sciences
- Editorial Board - Medical Sciences
- Self-Archiving Policy
- Dispatch Dates
- Terms and Conditions
- GSA Journals
- Journals on Oxford Academic
- Books on Oxford Academic

Article Contents
Conclusions, supplementary material.
- < Previous
Effects of Physical Activity Intervention on Physical and Cognitive Function in Sedentary Adults With and Without Diabetes
Address correspondence to Mark A. Espeland, PhD, Department of Biostatistical Sciences, Wake Forest School of Medicine, Medical Center Blvd, Winston-Salem, NC 27157. E-mail: [email protected]
Decision Editor: James Goodwin, MD
- Article contents
- Figures & tables
- Supplementary Data
Mark A. Espeland, Kasia Lipska, Michael E. Miller, Julia Rushing, Ronald A. Cohen, Joseph Verghese, Mary M. McDermott, Abby C. King, Elsa S. Strotmeyer, Steven N. Blair, Marco Pahor, Kieran Reid, Jamehl Demons, Stephen B. Kritchevsky, for the LIFE Study Investigators, Effects of Physical Activity Intervention on Physical and Cognitive Function in Sedentary Adults With and Without Diabetes, The Journals of Gerontology: Series A , Volume 72, Issue 6, 1 June 2017, Pages 861–866, https://doi.org/10.1093/gerona/glw179
- Permissions Icon Permissions
Type 2 diabetes mellitus may alter the effect of physical activity on physical and cognitive function.
The Lifestyle Interventions and Independence for Elders (LIFE) trial randomized controlled clinical trial of physical activity intervention (walking, resistance training, and flexibility exercises) enrolled adults aged 70–89 years who were sedentary and non-demented and who had functional limitations. Standardized measures of physical and cognitive function were collected an average of 2 years post-randomization. Differences between the intervention and control groups from 415 individuals with diabetes and 1,061 individuals without diabetes were contrasted with analyses of covariance.
At 24 months, assignment to the physical activity intervention resulted in 0.019 m/s relatively faster average 400-meter gait speeds ( p = .007 overall) both for individuals with and without diabetes (intervention × diabetes interaction p = .99). No benefits were seen on scores from a physical performance battery. Performance on cognitive tests was better among participants assigned to the physical activity intervention compared with control only for those with diabetes, particularly for global cognitive function ( p = .02) and delayed memory ( p = .005), with mean [95% confidence intervals] for benefit from physical activity intervention of 0.114 [0.007,0.111] and 0.208 [0.030,0.387] standard deviations, respectively.
Physical activity intervention improved the gait speed of older, sedentary individuals with and without diabetes. The cognitive function benefits occurred among participants with, but not without, diabetes. The mechanisms through which physical activity affects physical and cognitive function in older adults may differ for individuals by diabetes status.
One-quarter of U.S. adults who are 65 years or older have Type 2 diabetes mellitus ( 1 ). This accelerates their development of many age-related conditions and geriatric syndromes ( 2–4 ). Physical inactivity is an important component of the geriatric syndrome in diabetes ( 5 ). Sedentary lifestyle in older adults is associated with poorer control of diabetes and increased risk for comorbidities related to diabetes and insulin resistance ( 3 , 6 ). While treatment guidelines generally recommend that sedentary individuals with diabetes increase their physical activity ( 7 ), these recommendations may be of increasing importance later in life.
Declines in mobility and cognitive function are two features of the geriatric syndrome that diabetes is known to accelerate. It reduces mobility by lowering muscle quality and increasing neuropathy and peripheral vascular disease ( 8 ). It accelerates cognitive decline by disrupting energy metabolism in the brain, restricting its blood flow, and increasing atrophy and cerebrovascular disease ( 9 ). Physical activity may be expected to protect mobility and cognitive function. However, there is no clear evidence that structured physical activity programs to increase physical function benefit physical and cognitive function in older sedentary individuals with diabetes. These individuals face additional disease-related barriers towards increasing their physical activity including increased rates of hospitalization, sarcopenia, frailty, and complications such as arthritis, impaired vision, heart disease, and neuropathy that may diminish the effectiveness of interventions ( 2 , 8 ).
We conducted an exploratory analysis of data from a randomized controlled clinical trial of a physical activity intervention that included large numbers of older individuals with and without diabetes and standardized assessments of physical and cognitive function. The Lifestyle Interventions and Independence for Elders (LIFE) trial found that physical activity intervention tailored to older individuals preserved mobility, but had no overall effects on cognitive function ( 10 , 11 ). We hypothesized that the intervention may have different effects on physical and cognitive function, depending on individuals’ diabetes status.
LIFE was an eight-center, single-blinded, randomized controlled trial of an intervention to increase physical activity versus a health education control condition ( 12 , 13 , Supplementary Appendix). Participants were sedentary, aged 70–89 years, and could walk 400 meters in 15 minutes despite lower extremity functional limitations. LIFE was approved by all sites’ institutional review boards; informed consent was obtained from all participants.
Interventions
The physical activity intervention focused on walking, strength, flexibility, and balance training ( 10 , 12 ). Each week, participants were to attend two center-based visits and perform home-based activity 3–4 times, with goals of 30 minutes of walking at moderate intensity, 10 minutes of primarily lower extremity strength training with ankle weights, 10 minutes of balance training, and large muscle group flexibility exercises.
The health education group attended weekly workshops during the first 26 weeks of the intervention and monthly sessions thereafter (bi-monthly attendance was optional). Topics included travel safety, age appropriate preventive services, and nutrition, with 5–10 minutes of upper extremity stretching and flexibility exercises.
Diabetes was defined by self-report, current use of medications, or fasting glucose ≥126mg/dL at enrollment. The Short Physical Performance Battery (SPPB) consists of a 4-meter walk at usual pace, a timed repeated chair stand, and three increasingly more difficult standing balance tests ( 14 ). The total score ranges from 0 (worst) to 12 (best). Gait speed was calculated from a 400-meter walk test ( 10 ). For those who did not complete the walk, it was based on the portion of the walk that was completed.
A neuropsychological battery was administered by masked interviewers at baseline and 24 months ( 11 ). Three computer-based cognitive tests were administered at baseline and at either 18 or 30 months, depending on when participants were enrolled ( 11 , 15 ). The interviewer-administered tests included the Modified MiniMental State Exam (3MSE), a test of global cognitive function; the Wechsler Adult Intelligence Scale-III Digit Symbol Coding test (DSC), a test of psychomotor speed, attention, and working memory; and the Hopkins Verbal Learning Test-Revised (HVLT-D), a test of delayed recall. The computer tests added sensitivity for speed of processing and executive function: 1-back and 2-back tasks, the Eriksen Flanker task, and a task-switching paradigm. Higher scores reflect better performance on the 3MSE, DSC, HVLT-D, and n -back tests; lower scores reflect better performance on the Eriksen Flanker task and task-switching tests.
Self-reported demographic characteristics, medical and hospitalization history, body mass index, and medication use were collected at baseline. Physical activity (min/wk) was assessed with the Community Healthy Activities Model Program for Seniors (CHAMPS) questionnaire ( 16 ). Hospitalizations were based on self-report.
Statistical Analysis
Baseline risk factors and measures of physical and cognitive function were compared between intervention and diabetes groups using analyses of variance and logistic regression. A 1% winsorization was used to reduce the impact of extreme cognitive function scores: scores below the 1st percentile were replaced by the 1st percentile and scores above the 99th percentile were replaced by the 99th percentile. Individual scores were standardized by dividing their difference from the baseline cohort-wide mean by the standard deviation and ordered so that positive scores reflected better performance. A composite, which we loosely refer to as executive function, was formed by averaging standardized measures from the computer-administered battery ( 11 ). Analyses of covariance were used to assess mean follow-up physical and cognitive measures, with adjustment for baseline values and inclusion of age, education, sex, and race. Additional covariates were included in supporting analyses.
Our analyses were based on the 1,476 (90.3%) of 1,635 participants who provided post-randomization physical and cognitive function data. Compared with those missing assessments, participants included in analyses had faster baseline mean 400-meter gait speeds ( p < .001) and better performance on the 1-back cognitive function test ( p = .03), but did not differ markedly ( p > .05) for any other baseline factors ( Table 1 ). They also did not differ on the prevalence of diabetes ( p = .56) or intervention assignment ( p = .38).
Risk Factors and Baseline Cognitive and Physical Function Scores for Sedentary Older Adults With and Without Diabetes by Intervention Assignment: N (percent) or mean ( SD )
Notes: 3MSE = Modified MiniMental State Exam; CHAMPS = Community Healthy Activities Model Program for Seniors; DSC = Digit Symbol Coding; HVLT-D = Hopkins Verbal Learning Test-Revised; SPPB = Short Physical Performance Battery.
*Higher scores reflect better performance for the SPPB (0 to 12), 3MSE (0 to 100), DSC (0 to 133), HVLT-D (0 to 12), n -back tests (0 to 100). Higher scores reflect worse performance for the task switching and Flanker tasks.
Compared with others in our analyses, participants with diabetes were younger and had less formal education. They included a higher prevalence of males, African Americans, cardiovascular disease, and hypertension ( Table 1 ; all p < .05). They also had higher mean body mass indices and slightly lower mean 400-meter gait speeds. Their mean performances on the DSC, HVLT-D, and flanker tasks were worse. The balance between intervention groups afforded by randomization for the full LIFE cohort was maintained in the subset included in our analyses for all factors in Table 1 (all p > .05), except race/ethnicity: there were slightly more African Americans assigned to the physical activity compared with health education intervention ( p = .03).
Table 2 and Figure 1 present our primary findings. At 24 months, with covariate adjustment for baseline scores, age, education, gender, and race/ethnicity, overall mean SPPB scores did not differ between intervention groups: the mean [95% confidence interval] difference (physical activity minus health education) was 0.12 [−0.11,0.34] SPPB units ( p = .32). Overall differences in 400-meter gait speeds between intervention groups were significant ( p = .007). Physical activity intervention participants with diabetes averaged 0.019 [−0.008,0.047] m/s faster gaits; those without diabetes averaged 0.019 [0.002,0.036] m/s faster gaits. Figure 1 presents results in standard deviation units to facilitate comparisons across measures: intervention effects were similar for participants with and without diabetes, with p -values for tests of interaction p = .97 (SPPB) and p = .99 (400-meter gait speed). Analyses based on 4-meter gait yielded results similar to those for 400-meter gait.
Physical and Standardized Cognitive Function Measures at 2 Years for Participants With and Without Diabetes: Mean ( SE ) With Adjustment for Age, Education, Gender, Race/Ethnicity, and Baseline Value
Notes: 3MSE = Modified MiniMental State Exam; CI = confidence interval; DSC = Digit Symbol Coding; HE = health education; HVLT-D = Hopkins Verbal Learning Test-Revised; PA = physical activity; SD = standard deviation; SE = standard error; SPPB = Short Physical Performance Battery.
![mark espeland phd Mean [95% confidence interval] intervention effects (Physical Activity minus Health Education) in standard deviation units for physical function (Short Physical Performance Battery and 400-meter gait) and cognitive function (global composite, digit symbol coding, delayed memory, and executive function) scores with covariate adjustment for age, education, gender, race/ethnicity, and baseline scores.](https://oup.silverchair-cdn.com/oup/backfile/Content_public/Journal/biomedgerontology/72/6/10.1093_gerona_glw179/7/m_glw17901.jpeg?Expires=1714580246&Signature=zJVT8Ivk-IOqplqOI068UdS-yMJkLprM870Sjg~Va4gYNP2uF6G4U-t2NCFDbmwDczVo1SQCt6Q2CcI2v510ig6SDJ6~3nAD~E34QsUpWQjgQ5EGvcLzzXsxtQWC8bBE7LfvjVDZbYRszPQ14JKeRvPgu~unfCE7qEGajoaGe1nuQkk-uJAKDfN4Fo1s2-LVZ02B3A69mTWnCjm~-Fc6DhKNIZhvCEXG0iC9dE9yP3SByIp-SBf8SGXHMJmD47JyaOsGBaC2xZ5y7p34pNjuhth3SdAPphyNq-ddl8yO8XcgXBSKLF-LTfDx4LJW-uW1tzRt7vpsG2A3zb-9LJ3TFg__&Key-Pair-Id=APKAIE5G5CRDK6RD3PGA)
Mean [95% confidence interval] intervention effects (Physical Activity minus Health Education) in standard deviation units for physical function (Short Physical Performance Battery and 400-meter gait) and cognitive function (global composite, digit symbol coding, delayed memory, and executive function) scores with covariate adjustment for age, education, gender, race/ethnicity, and baseline scores.
There were no overall differences between intervention groups for any of the cognitive function measures ( Table 2 , all p > .50). However, as seen in Figure 1 , for each cognitive function measure, the mean differences between physical activity and health education participants were positive for participants with diabetes and negative for those without diabetes, with tests for interactions reaching statistical significance for 3MSE ( p = .02) and HVLT-D ( p = .005). For these measures, 95% confidence intervals for the mean difference between physical activity and health education participants with diabetes excluded zero: for 3MSE the mean intervention effect was 0.114 [0.007,0.222] standard deviations and for HVLT-D it was 0.208 [0.030,0.387] standard deviations. The 95% confidence intervals for intervention effects included zero among participants with diabetes for the other two cognitive function tests, and for all four tests among those without diabetes. Additional covariate adjustment for all factors in Table 1 (ie, including body mass index, prior cardiovascular disease, hypertension, CHAMPS score, and Apo-E4 genotype) attenuated the interactions for the cognitive function tests slightly. For 3MSE, the interaction no longer reached statistical significance ( p = .07). For HVLT-D, it remained highly significant ( p = .007). This additional covariate adjustment had no material impact on interactions for other measures.
Differences between participants with and without diabetes in estimated 2-year mean intervention effects ( Figure 1 ) ranged from about 0.1 to 0.3 standard deviations. When baseline cognitive functions were regressed against participants’ age, estimated slopes ranged from −0.025 SD /y for executive function to −0.043 SD /y for DSC test scores. Thus, the estimated intervention effects exceed the magnitude of cognitive decline associated with a difference of several years in age along these regression slopes.
We explored several potential correlates of intervention benefits (Supplementary Table). Attendance in physical activity and health education intervention sessions was similar for participants with and without diabetes (interaction p = .70). CHAMPS physical activity increased similarly at 12 and 24 months among physical activity intervention participants both with and without diabetes. Changes in mean body mass index and blood pressure over time were small in both intervention groups and did not vary by diabetes status. The hospitalization rate was similar between intervention groups for individuals with diabetes. It was slightly higher among physical activity compared with health education participants without diabetes, but not significantly ( p = .15). The annual hospitalization rate was negatively associated with 3MSE and HVLT-D scores (both p < .001); including this as a covariate did not alter interactions between diabetes and intervention assignment (3MSE interaction p = .03; HVLT-D interaction p = .007). Adding interactions between intervention assignment and hospitalization rates also did not affect results. Insulin use among participants with diabetes was balanced between intervention groups.
The LIFE physical activity intervention significantly increased physical activity and lowered the incidence of major mobility disability ( 10 ). During the prior LIFE pilot trial, the physical activity intervention also improved gait speeds and SPPB performance over 1 year ( 17 ), but resulted in no overall benefit for cognitive function ( 11 ). Our present analyses add the following. First, there was no difference in the effects of the physical activity intervention on 400-meter gait speeds for individuals with and without diabetes. Second, while there were no overall benefits of the intervention on cognitive function, among individuals with diabetes the physical activity intervention resulted in better global cognitive function and delayed memory. Finally, covariate adjustment for hypertension and history of cardiovascular disease did not affect results and we could not attribute differential benefits for individuals with diabetes to differences in markers of adherence (intervention session attendance, participation in physical activity), changes in body mass index, hospitalizations, or insulin use.
Physical Function
Diabetes and its primary risk factor obesity are both strongly associated with impaired mobility function and mobility disability ( 18–21 ). Many trials in persons with diabetes have assessed the effects of exercise training on metabolic control and disease risk factors, however we are aware of no reports that the response to exercise differs in persons on the basis of Type 2 diabetes status alone ( 22 ). We are also aware of no other study that evaluated the effect of exercise on the risk of mobility disability in older persons with Type 2 diabetes. A number of studies have examined the effect of exercise on changes in self-reported physical functioning in diabetes and have shown significant improvements associated with exercise ( 23 , 24 ). Most have been relatively short in duration and focused on middle-aged participants. The Action for Health in Diabetes (Look AHEAD) trial studied lifestyle intervention designed to reduce caloric intake and increase physical activity in persons with Type 2 diabetes, ages 45–76 years. Its intervention was associated with superior maintenance of self-reported physical function over 4 years ( 25 ). Mediation analysis showed that both exercise and weight loss contributed to the benefit. Gait speed was measured in a subset of participants at 8 and 9 years. The intervention benefited gait speeds in both the younger and older participants ( 25 ). In the LIFE-pilot study, obesity attenuated the benefits on gait speed and SPPB scores that the physical activity intervention provided ( 26 ). A community-based exercise intervention trial in overweight and obese older adults showed that the mobility benefits of exercise were not as well sustained unless they were also paired with weight loss ( 27 ). LIFE participants with Type 2 diabetes were heavier than nonparticipants, but had a similar experience with respect to mobility function to those without the disease. The LIFE primary results manuscript included an assessment of the comparability of intervention effects on its primary outcome, incidence of major mobility, across participants grouped by diabetes, insulin resistance, and normal, which were comparable (interaction p = .41) ( 10 ).
Cognitive Function
There is not consistent evidence that aerobic exercise benefits the cognitive function of older individuals who are not cognitively impaired ( 28 ). Evidence that physical activity interventions differentially benefit cognition among individuals with diabetes is limited and mixed. Baker, et al., reported the effects of a 6-month trial of aerobic exercise in 28 adults (ages 57–83 years) who were either newly diagnosed with diabetes (21%) or prediabetes ( 29 ). Compared to a stretching intervention, the physical activity intervention was associated with relative improvements in executive function, but not memory. The Look AHEAD trial found no cognitive benefit of 8–9 years of its lifestyle intervention in 978 adults (aged 45–76 years) with Type 2 diabetes ( 30 ). Cohort studies have reported weak associations between physical activity and better cognitive function among individuals with diabetes ( 31 , 32 ). While our findings that physical activity may benefit cognitive function in an older, inactive, and more physically vulnerable cohort with diabetes is most consistent with Baker, et al., we saw evidence of benefit for memory, and less evidence of benefit for executive function. Differences among the cohorts, timeframes, targeted physical activities, and cognitive measures among studies may account for the different findings.
The potential benefit of the LIFE physical activity intervention for cognitive function of individuals with diabetes contrasts with the lack of benefit for those without diabetes. The benefits LIFE has reported for mobility ( 10 ) and those we report for gait speed may be attributed to improvements in factors such as inflammation, neuropathy, and vascular function, pathways that cognitive and physical function share but which were not assessed in LIFE ( 33 , 34 ). It may be that cognitive deficits in individuals with diabetes are more closely associated with inflammation and vascular dysfunction than for those without diabetes, enhancing the potency of the LIFE intervention for people with diabetes. Many participants with diabetes had hypertension, heart disease, and obesity: it is possible that the benefits in cognitive function the physical activity intervention provided for these individuals reflected the contribution of a broader set of metabolic and vascular factors. It is possible that physical activity intervention benefited cognitive function by improving diabetes control ( 35 ) and that its benefits for physical function were through pathways (eg, increased strength) that did not influence cognitive function in individuals without diabetes. Another possible mechanism is through energy metabolism. Glucose is the primary source of energy for brain, however among individuals with diabetes for whom glucose-based metabolism is less reliable, the brain may adapt to have greater efficiency to draw on alternative energy sources ( 36 , 37 ). In older individuals who have less robust blood-brain barriers, it is possible that physical activity bouts may compete with the brain for glucose resources, leading to lower glucose levels in the brain that potentially counteract benefits from other pathways (eg, inflammation and vascular function) that improve physical function ( 38 ). If participants with diabetes had adapted to draw more efficiently on alternatives to glucose-based energy, perhaps they were less susceptible to this phenomenon and more able to accrue benefits from other pathways. This can account for the slightly (nonsignificantly) lower cognitive function seen in the physical activity intervention group.
Potential Mediators of Cognitive Benefits
We saw no evidence that the intervention differentially benefited individuals with diabetes due to increased adherence to the intervention, changes in weight and blood pressure, or differences in insulin use. Hospitalization rates were similar between intervention groups for participants with diabetes, but were slightly higher among physical activity intervention participants compared to health education participants among those without diabetes ( 39 ). While higher rates of hospitalization were associated with poorer levels of cognitive function during follow-up, the differential intervention effects we saw on cognition depending on diabetes status could not be attributed to differences in hospitalization rates.
Limitations
Our analyses were initiated to describe the experience of individuals with diabetes in the trial and were not a pre-specified comparison: this enhances the possibility of a chance finding. They were exploratory and require confirmation. As volunteers to a randomized clinical trial, LIFE participants may not be representative of general populations. While other trials of lifestyle interventions have reported beneficial effects on cognitive function within 2 years ( 40 , 41 ), this may be a relatively short span of time to produce cognitive benefits. The mean effect on gait speed that we report (0.019 m/s) is modest, however it falls within the range reported as minimally significant in the LIFE-P trial (0.018 to 0.027 m/s) ( 42 ). Diabetes is often underdiagnosed, however excluding participants ( N = 38) identified only through testing did not alter findings.
Based on exploratory analysis from the LIFE trial, physical activity interventions may benefit both gait speed and cognitive function in older physically vulnerable and inactive individuals with diabetes, but only gait speed among those without diabetes.
Supplementary data is available at The Journals of Gerontology, Series A: Biomedical Sciences and Medical Sciences online.
This work was supported by National Institutes of Health (UO1AG22376, U01AG022376-05A2S, P30AG028740, P30AG21332, 1P30AG031679, P30AG024827, P30AG021342, UL1RR025744, 1R24HD065688-01A1, K07AG3587) and partially supported by USDA (58-1950-7-707). Any opinions, findings, conclusion, or recommendations expressed in this publication are those of the author(s) and do not necessarily reflect the view of the United States Department of Agriculture.
Menke A Casagrande S Geiss L Cowie CC . Prevalence of trends in diabetes among adults in the United States, 1988–2012 . JAMA . 2015 ; 314 : 1021 – 1029 . doi:10.1001/jama.2015.10029
Google Scholar
Kirkman MS Briscoe VJ Clark N et al. . Diabetes in older adults: consensus report . Diabetes Care . 2012 ; 35 : 2650 – 2664 . doi:10.1111/jgs.12035
Bianchi L Zuliani G Volpato S . Physical disability in the elderly with diabetes: epidemiology and mechanisms . Curr Diab Rep . 2013 ; 13 : 824 – 830 . doi: 10.1007/s11892-013-0424-6
Araki A Ito H . Diabetes mellitus and geriatric syndromes . Geriatr Gerontol Int . 2009 ; 9 : 105 – 114 . doi: 10.1111/j.1447-0594.2008.00495.x
Abdelhafiz AH Sinclair AJ . Diabetes, nutrition, and exercise . Clin Geriatr Med . 2015 ; 31 : 439 – 451 . doi: 10.1016/j.cger.2015.04.011
Booth FW Laye MJ Roberts MD . Lifetime sedentary living accelerates some aspects of secondary aging . J Appl Physiol (1985) . 2011 ; 111 : 1497 – 1504 . doi: 10.1152/japplphysiol.00420.2011
American Diabetes Association . Foundations of care: education, nutrition, physical activity, smoking cessation, psychosocial care, and immunization . Diabetes Care . 2015 ; 38 ( Suppl ): S20 – 30 . doi:10.2337/dc15-S007
Gregg EW Beckles GL Williamson DF et al. . Diabetes and physical disability among older U.S. adults . Diabetes Care . 2000 ; 23 : 1272 – 1277 .
Biessels GJ Deary IJ Ryan CM . Cognition in diabetes: a lifespan perspective . Lancet Neurology . 2008 ; 7 : 184 – 190 .
Pahor M Guralnik JM Ambrosius WT et al. . Effect of structured physical activity on prevention of major mobility disability in older adults . JAMA . 2014 ; 311 : 2387 – 2396 . doi:10.1136/bmj.i245
Sink K Espeland MA Castro C et al. . Effect of physical activity on cognitive outcomes in sedentary older adults . JAMA . 2015 ; 314 : 781 – 790 . doi:10.1001/jama.2015.9617
Fielding RA Rejeski WJ Blair SN et al. . The Lifestyle Interventions and Independence for Elders Study: design and methods . J Gerontol A Bio Sci Med Sci . 2011 ; 66 : 1226 – 1237 . doi:10.1093/gerona/glr123
Marsh AP Lovato LC Glynn NW et al. . Lifestyle Interventions and Independence for Elders study: recruitment and baseline characteristics . J Geront A Bio Sci Med Sci . 2013 ; 68 : 1549 – 1558 . doi:10.1093/gerona/glt064
Guralnik JM Ferrucci L Simonsick EM Salive ME Wallace RB . Lower-extremity function in persons over the age of 70 years as a predictor of subsequent disability . N Engl J Med . 1995 ; 332 : 556 – 561 . doi: 10.1056/NEJM199503023320902
Espeland MA Katula JA Rushing J et al. . Performance of a computer-based assessment of cognitive function measures in two cohorts of seniors . Int J Geriatr Psychiatry . 2013 ; 28 : 1239 – 1250 . doi:10.1002/gps.3949
Stewart AL Verboncoeur CJ McLellan BY et al. . Physical activity outcomes of CHAMPS II: a physical activity promotion program for older adults . J Gerontol A Biol Sci Med Sci . 2001 ; 56 : M465 – M470 .
Pahor M Blair SN Espeland M et al. . Effects of a physical activity intervention on measures of physical performance . J Geront A Biol Sci Med Sci . 2006 ; 61 : 1157 – 1165 .
Figaro MK Kritchevsky SB Resnick HE et al. . Diabetes, inflammation, and functional decline in older adults . Diabetes Care . 2006 ; 29 : 2039 – 2045 . doi:10.2337/dc06-0245
Park SW Goodpaster BH Strotmeyer ES et al. . Accelerated loss of skeletal muscle strength in older adults with type 2 diabetes . Diabetes Care . 2007 ; 30 : 1507 – 1512 . doi:10.2337/dc06-2537
Vincent HK Vincent KR Lamb KM . Obesity and mobility disability in the older adult . Obes Rev . 2010 ; 11 : 568 – 579 . doi: 10.1111/j.1467-789X.2009.00703.x
Jensen GL Hsiao PY . Obesity in older adults: relationship to functional limitation . Curr Opin Clin Nutr Metab Care . 2010 ; 13 : 46 – 51 . doi: 10.1097/MCO.0b013e32833309cf
Reusch JE Bridenstine M Regensteiner JG . Type 2 diabetes mellitus and exercise impairment . Rev Endocr Metab Disord . 2013 ; 14 : 77 – 86 . doi: 10.1007/s11154-012-9234-4
Nicolucci A Balducci S Cardelli P et al. . Improvement of quality of life with supervised exercise training in subjects with type 2 diabetes mellitus . Arch Intern Med . 2011 ; 171 : 1951 – 1953 . doi:10.1001/archinternmed.2011.561
Rejeski WJ Ip EH Bertoni AG et al. . Lifestyle change and mobility in obese adults with type 2 diabetes . NEJM . 2012 ; 366 : 1209 – 1217 . doi:10.1056/NEJMoa1110294
Houston DK Leng X Bray GA et al. . Impact of a long-term intensive lifestyle intervention on physical function . Obesity . 2015 ; 23 : 77 – 84 . doi:10.1002/oby.20944
Manini TM Newman AB Fielding R et al. . Effects of exercise on mobility in obese and nonobese older adults . Obesity . 2010 ; 18 : 1168 – 1175 . doi:10.1038/oby.2009.317
Rejeski WJ Brubaker PH Goff DC Jr et al. . Translating weight loss and physical activity programs into the community to preserve mobility in older, obese adults in poor cardiovascular health . Arch Intern Med . 2011 ; 171 : 880 – 886 . doi: 10.1001/archinternmed.2010.522
Young J Angevaren M Rusted J Tabet N . Aerobic exercise to improve cognition in older people without known cognitive impairment . Cochrane Database Syst Rev . 2015 ; 4 : CD005381 . doi:10.1002/14651858.CD005381.pub4
Baker LD Frank LL Foster-Schubert K et al. . Aerobic exercise improves cognition for older adults with glucose intolerance, a risk factor for Alzheimer’s disease . J Alzheimers Dis . 2010 ; 22 : 569 – 579 . doi: 10.3233/JAD-2010-100768
Espeland MA Rapp SR Bray GA et al. . Long-term impact of behavioral weight loss intervention on cognitive function . J Gerontol A Biol Sci Med Sci . 2014 ; 69 : 1101 – 1108 . doi:10.1093/gerona/glu031
Colberg SR Somma CT Sechrist SR . Physical activity participation may offset some of the negative impact of diabetes on cognitive function . J Am Med Dir Assoc . 2008 ; 9 : 434 – 438 . doi: 10.1016/j.jamda.2008.03.014
Devore EE Kang JH Okereke O Grodstein F . Physical activity levels and cognitive function in women with type 2 diabetes . Am J Epidemiol . 2009 ; 170 : 1040 – 1047 . doi:10.1093/aje/kwp224
Newman AB Arnold AM Naydeck BL et al. . Successful aging: effect of subclinical cardiovascular disease . Arch Intern Med . 2003 ; 163 : 2315 – 2322 . doi:10.1001/archinte.163.19.2315
Chung HY Cesari M Anton S et al. . Molecular inflammation: underpinnings of aging and age-related diseases . Ageing Res Rev . 2009 ; 8 : 18 – 30 . doi: 10.1016/j.arr.2008.07.002
Balducci S Zanuso S Cardelli P et al. . Changes in physical fitness predict improvements in modifiable risk factors independently of body weight loss in subjects with type 2 diabetes participating in the Italian Diabetes and Exercise Study . Diabetes Care . 2012 ; 35 : 1347 – 1354 . doi:10.2337/dc11-1859
Amaral AI . Effects of hypoglycaemia on neuronal metabolism in the adult brain: role of alternative substrates to glucose . J Inherit Metab Dis . 2013 ; 36 : 621 – 634 . doi: 10.1007/s10545-012-9553-3
Sickmann HM Waagepetersen HS . Effects of diabetes on brain metabolism–is brain glycogen a significant player? Metab Brain Dis . 2015 ; 30 : 335 – 343 . doi: 10.1007/s11011-014-9546-z
De Feo P Gallai V Mazzotta G et al. . Modest decrements in plasma glucose concentration cause early impairment in cognitive function and later activation of glucose counterregulation in the absence of hypoglycemic symptoms in normal man . J Clin Invest . 1988 ; 82 : 436 – 444 . doi: 10.1172/JCI113616
Marsh AP Applegate WB Guralnik JM et al. . Hospitalization during a physical activity intervention in older adults at risk of mobility disability . J Am Geriatr Soc . 2016 ; 5 : 933 – 43 . doi:10.1111/jgs.14114
Ngandu T Lehtisalo J Solomon A et al. . A 2 year intervention of diet, exercise, cognitive training, and vascular risk monitoring versus control to prevent cognitive decline in at-risk elderly people . Lancet . 2015 ; 385 : 2255 – 2263 . doi:10.1016/S0140-6736(15)60461-5
Iuliano E di Cagno A Aquino G et al. . Effects of different types of physical activity on cognitive functions and attention in older people . Experiment Gerontol . 2015 ; 70 : 105 – 110 . doi:10.1016/j.exger.2015.07.008
Kwon S Perera S Pahor M et al. . What is a meaningful change in physical performance? Findings from a clinical trial in older adults (the LIFE-P study) . J Nutr Health Aging . 2009 ; 13 : 538 – 544 .
Author notes
- physical activity
- diabetes mellitus
- diabetes mellitus, type 2
- lack of exercise
- mental processes
- older adult
- cognitive ability
Supplementary data
Email alerts, citing articles via, looking for your next opportunity.
- Recommend to Your Librarian
Affiliations
- Online ISSN 1758-535X
- Copyright © 2024 The Gerontological Society of America
- About Oxford Academic
- Publish journals with us
- University press partners
- What we publish
- New features
- Open access
- Institutional account management
- Rights and permissions
- Get help with access
- Accessibility
- Media enquiries
- Oxford University Press
- Oxford Languages
- University of Oxford
Oxford University Press is a department of the University of Oxford. It furthers the University's objective of excellence in research, scholarship, and education by publishing worldwide
- Copyright © 2024 Oxford University Press
- Cookie settings
- Cookie policy
- Privacy policy
- Legal notice
This Feature Is Available To Subscribers Only
Sign In or Create an Account
This PDF is available to Subscribers Only
For full access to this pdf, sign in to an existing account, or purchase an annual subscription.

- < Previous
Home > USC Columbia > Public Health, Arnold School of > SPH_EPIDEMIOLOGY_BIOSTATISTICS > SPH_EPIDEMIOLOGY_BIOSTATISTICS_FACPUB > 566
Faculty Publications
Effects of a physical activity intervention on measures of physical performance: results of the lifestyle interventions and independence for elders pilot (life-p) study.
Marco Pahor Steven N. Blair , University of South Carolina - Columbia Follow Mark Espeland Roger Fielding Thomas M. Gill Jack M. Guralnik Cinzia Maraldi Michael E. Miller Anne B. Newman Walter J. Rejeski Sergei Romashkan Stephanie Studenski
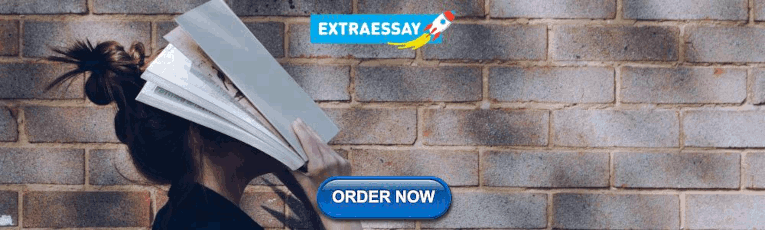
Document Type
Publication info.
The LIFE Study Investigators (Writing Group--Marco Pahor, MD, Steven N. Blair, PED, Mark Espeland, PhD, Roger Fielding, PhD, Thomas M. Gill, MD, Jack M. Guralnik, MD, PhD, Evan C. Hadley, MD, Abby C. King, PhD, Stephen B. Kritchevsky, PhD, Cinzia Maraldi, MD, Michael E. Miller, PhD, Anne B. Newman, MD, Walter J. Rejeski, PhD, Sergei Romashkan, MD, PhD, Stephanie Studenski, MD, MPH). (2006) Effects of a Physical Activity Intervention on Measures of Physical Performance: Results of the Lifestyle Interventions and Independence for Elders Pilot (LIFE-P) Study. Journal of Gerontology 61(11);1157-65.
© Journal of Gerontology 2006, Oxford University Press
Since February 10, 2014
Advanced Search
- Notify me via email or RSS
- Collections
- Disciplines
Submissions
- Submit Research
- Give us Feedback
- University Libraries
Home | About | FAQ | My Account | Accessibility Statement
Privacy Copyright

An official website of the United States government
The .gov means it’s official. Federal government websites often end in .gov or .mil. Before sharing sensitive information, make sure you’re on a federal government site.
The site is secure. The https:// ensures that you are connecting to the official website and that any information you provide is encrypted and transmitted securely.
- Publications
- Account settings
Preview improvements coming to the PMC website in October 2024. Learn More or Try it out now .
- Advanced Search
- Journal List
- HHS Author Manuscripts

Reduction in Weight and Cardiovascular Disease Risk Factors in Individuals With Type 2 Diabetes: One-Year Results of the Look AHEAD Trial
The effectiveness of intentional weight loss in reducing cardiovascular disease (CVD) events in type 2 diabetes is unknown. This report describes one-year changes in CVD risk factors in a trial designed to examine the long-term effects of an intensive lifestyle intervention on the incidence of major CVD events.
Research Design and Methods
A multi-centered randomized controlled trial of 5,145 individuals with type 2 diabetes, aged 45–74 years, with body mass index ≥25 kg/m 2 (≥27 kg/m 2 if taking insulin). An Intensive Lifestyle Intervention (ILI) involving group and individual meetings to achieve and maintain weight loss through decreased caloric intake and increased physical activity was compared to a Diabetes Support and Education (DSE) condition.
Participants assigned to ILI lost an average 8.6% of their initial weight versus 0.7% in DSE group (p<0.001). Mean fitness increased in ILI by 20.9% versus 5.8% in DSE (p<0.001). A greater proportion of ILI participants had reductions in diabetes, hypertension, and lipid-lowering medicines. Mean HbA 1 c dropped from 7.3% to 6.6% in ILI (p<0.001) versus from 7.3% to 7.2% in DSE. Systolic and diastolic pressure, triglycerides, HDL-cholesterol, and urine albumin/creatinine improved significantly more in ILI than DSE participants (all p<0.01).
Conclusions
At 1 year, ILI resulted in clinically significant weight loss in persons with type 2 diabetes. This was associated with improved diabetes control and CVD risk factors and reduced medicine use in ILI versus DSE. Continued intervention and follow-up will determine whether these changes are maintained and will reduce CVD risk.
Trial Registration
clinicaltrials.gov Identifier: {"type":"clinical-trial","attrs":{"text":"NCT00017953","term_id":"NCT00017953"}} NCT00017953
INTRODUCTION
Look AHEAD (Action for Health in Diabetes) is an NIH-funded clinical trial investigating the long-term health impact of an intensive lifestyle intervention in 5145 overweight or obese adults with type 2 diabetes. It is being conducted in 16 centers in the United States. The design and methods of this trial have been reported elsewhere ( 1 ) as have the baseline characteristics of the randomized cohort ( 2 ). Its primary objective is to determine whether cardiovascular morbidity and mortality in persons with type 2 diabetes can be reduced by long-term weight reduction, achieved by an Intensive Lifestyle Intervention (ILI) that includes diet, physical activity, and behavior modification ( 3 ). The goal of this intervention is for individuals to achieve and maintain a loss of at least 7% of initial body weight. Results of the ILI group will be compared with a usual-care condition that includes Diabetes Support and Education (DSE). Follow-up of Look AHEAD participants is ongoing and is planned to extend for up to 11.5 years. For the study to continue for this period, two feasibility criteria were set by the Look AHEAD study group based on 1-year changes: 1) a difference between ILI and DSE participants of >5 percentage points in the average percent change in weight from baseline; and 2) an average absolute percent weight loss from baseline among ILI participants (not using insulin at baseline) of >5%. This report documents the success of Look AHEAD in meeting these 1-year feasibility criteria and describes changes in the two groups at the end of the first year in fitness, cardiovascular disease risk factors, and use of medicines.
RESEARCH DESIGN AND METHODS
Participants.
For inclusion in the study, participants were 45–74 years of age (which was changed to 55–74 years during the second year of recruitment to increase the anticipated cardiovascular event rate), had a body mass index (BMI) ≥25 kg/m 2 (≥27 kg/m 2 if currently taking insulin), HbA 1 c ≤11%, blood pressure ≤160 (systolic) and ≤100 (diastolic) mm Hg and triglycerides <600 mg/dL.
Participants completed maximal graded exercise tests to assess fitness prior to randomization. The test consisted of the participant walking on a motorized treadmill at a constant self-selected walking speed (1.5, 2.0, 2.5, 3.0, 3.5, or 4.0 mph). The elevation of the treadmill was initially set at 0% grade and increased by 1.0% every minute. Heart rate and rating of perceived exertion (RPE) using the Borg 15-category scale ( 4 ) were measured during the final 10 seconds of each exercise stage and at the point of test termination. To determine eligibility at baseline, a maximal exercise test was performed. For individuals not taking prescription medicine that would affect heart rate response during exercise (e.g. beta blocker), the baseline test was considered valid if the individual achieved at least 85% of age-predicted maximal heart rate (age predicted maximal heart rate = 220 minus age) and a minimum of 4 metabolic equivalents (METS). For individuals taking prescription medicine that would affect the heart rate response during exercise, the baseline test was considered valid if the individual achieved a RPE of at least 18 and a minimum of 4 METS. Individuals not achieving these criteria were not eligible for randomization into Look AHEAD. METS at each exercise stage and at test termination were estimated from a standardized formula that incorporates walking speed and grade ( 5 ).
The goal was to recruit approximately equal numbers of men and women, with ≥33% from racial and ethnic minority groups. Informed consent was obtained from all participants before screening and at enrollment, consistent with the Helsinki Declaration and the guidelines of each center’s institutional review board. After all eligibility criteria were confirmed, participants were randomly assigned with equal probability to either ILI or the DSE comparison condition. Randomization was stratified by clinical center.
Interventions
Prior to randomization, all study participants were required to complete a 2-week run-in period which included successful self-monitoring of diet and physical activity, and they were provided an initial session of diabetes education with particular emphasis on aspects of diabetes care related to the trial such as management of hypoglycemia and foot care. The session stressed the importance of eating a healthy diet and being physically active for both weight loss and improvement of glycemic control. All individuals who smoked were encouraged to quit and were provided self-help materials and/or referral to local programs as appropriate.
The weight loss intervention prescribed in the first year has been described in detail ( 3 ). Briefly, it combines diet modification and increased physical activity and was designed to induce a minimum weight loss of 7% of initial body weight during the first year. Individual participants were encouraged to lose ≥10% of their initial body weight, with the expectation that aiming high would ensure that a greater number of participants would achieve the minimum 7% weight loss. The intervention was modeled on group behavioral programs developed for the treatment of obese patients with type 2 diabetes and included treatment components from the Diabetes Prevention Program ( 6 , 7 , 8 ) and the National Heart, Lung, and Blood Institute’s (NHLBI) clinical guidelines ( 9 ). During months 1–6, participants were seen weekly with 3 group meetings and 1 individual session per month. During months 7–12, group sessions were provided every other week and the monthly individual session was continued. Sessions were led by intervention teams that included registered dietitians, behavioral psychologists, and exercise specialists.
Caloric restriction was the primary method of achieving weight loss. The macronutrient composition of the diet was structured to enhance glycemic control and to improve CVD risk factors. It included a maximum of 30% of total calories from fat (with a maximum of 10% of total calories from saturated fat) and a minimum of 15% of total calories from protein ( 10 ). Participants were prescribed portion-controlled diets, which included the use of liquid meal replacements (provided free of charge) and frozen food entrées, as well as structured meal plans (comprised of conventional foods) for those who declined the meal replacements. Monthly reviews took place at an individual session to reassess progress.
The physical activity program prescribed in the ILI relied heavily on home-based exercise with gradual progression toward a goal of 175 minutes of moderate intensity physical activity per week. While walking was encouraged, participants were allowed to choose other types of moderate-intensity physical activity, and programs were tailored based on the results of a baseline physical fitness test and safety concerns.
The ILI included a “toolbox” approach, as used in the Diabetes Prevention Program ( 6 , 7 ), to help participants achieve and maintain the study’s weight loss and activity goals. Use of the “toolbox” was based on a pre-set algorithm and assessment of participant progress. After the first 6 months, the “toolbox” algorithm included use of a weight loss medicine (orlistat) and/or advanced behavioral strategies for individuals who had difficulty in meeting the trial’s weight or activity goals. Specific protocols were used to determine when to initiate medication or other approaches, to monitor participants, and to determine when to stop a particular intervention.
Participants assigned to DSE attended the initial pre-randomization diabetes education session (described above) and were invited to 3 additional group sessions during the first year. A standard protocol was used for conducting these sessions, which provided information and opportunities for discussing topics related to diet, physical activity, and social support. However, the DSE group was not weighed at these sessions and received no counseling in behavioral strategies for changing diet and activity.
Ongoing clinical care
All participants in the ILI and DSE groups continued to receive care for their diabetes and all other medical conditions from their own physicians. Changes in all medicines were made by the participants’ own physicians, except for temporary reductions in hyperglycemia medicines during periods of intensive weight loss intervention, which were made by the intervention sites following a standardized treatment protocol aimed at avoiding hypoglycemia.
Assessments
Anthropometry.
All participants were scheduled to attend baseline and 1-year assessments, at which measures were collected by staff members who were masked to participants’ intervention assignments. Weight and height were assessed in duplicate using a digital scale and a standard stadiometer. Seated blood pressure was measured in duplicate, using an automated device after a 5-minute rest. Participants brought all prescription medicines to the clinic to ensure recording accuracy. History of cardiovascular disease was based on self-report of myocardial infarction, stroke, transient ischemic event, percutaneous transluminal coronary angioplasty, or coronary artery bypass graft.
At 1 year, a submaximal exercise test was performed and terminated when the participant first achieved or exceeded 80% of age-predicted maximal heart rate (HR Max in beats/minute= 220 minus age). If the participant was taking a beta blocking medicine at baseline or 1-year assessment, the submaximal test was terminated at the point when the participant first reported achieving or exceeding 16 on the 15-category RPE scale. For participants not taking a beta blocking medicine, change in cardiorespiratory fitness was computed as the difference in estimated METS between points during the baseline and 1-year tests when >80% of age-predicted maximal heart rate was attained. For participants taking beta blocking medicine at either baseline or 1-year, change in cardiorespiratory fitness was computed as the difference in estimated METS between points during the baseline and 1-year tests when RPE>16 was attained.
Serum measures
The Central Biochemistry Laboratory (Northwest Lipid Research Laboratories, University of Washington, Seattle, WA.) conducted standardized analyses of shipped frozen specimens. HbA 1 c was measured by a dedicated ion exchange, high performance liquid chromatography instrument (Biorad Variant 11). Fasting serum glucose was measured enzymatically on a Hitachi 917 autoanalyzer using hexokinase and glucose-6-phosphate dehydrogenase. Total serum cholesterol and triglycerides were measured enzymatically using methods standardized to the Centers for Disease Control and Prevention Reference Methods. LDL-cholesterol was calculated by the Friedewald equation ( 11 ). HDL-cholesterol was analyzed by the treatment of whole plasma with dextran sulfate – Mg ++ to precipitate all of the apolipoprotein B-containing lipoproteins. Albumin and creatinine concentrations were measured from spot urine samples.
Participants were classified as having the metabolic syndrome using the criteria proposed by the National Cholesterol Education Program ATP III panel ( 12 ). They also were classified according to their success in meeting treatment goals published by the American Diabetes Association ( 13 ). Glycemic control was defined as HbA 1 c <7.0%; blood pressure control as systolic blood pressure <130 and diastolic blood pressure <80 mm Hg; and lipid control as LDL-cholesterol <100 mg/dL.
Statistical methods
Cross-sectional differences between participants assigned to the ILI and DSE conditions were assessed using analysis of covariance and logistic regression, with adjustment for clinical center (the sole factor used to stratify randomization). Changes in outcome measures from baseline to 1 year were compared using analysis of covariance and Mantel-Haenszel tests.
Participants’ baseline characteristics
Figure 1 describes trial enrollment. Of the 28,622 individuals who provided information during prescreening, 15,561 (54.4%) were found to be eligible for clinic visits to confirm eligibility. The most common reasons for ineligibility at this stage were related to age (13.5%), lack of diabetes (8.6%), and the likelihood that the diabetes was Type 1 (4.4%). Of the 9,045 (58.1%) who attended clinic visits, 5,145 (56.9%) were ultimately randomized: 2,570 participants were assigned to ILI and 2,575 to DSE. At this stage, individuals were most commonly ineligible due to staff judgment (7.6%), elevated blood pressure (7.0%), or incomplete behavioral run-ins (4.8%).

Enrollment of Look AHEAD participants.
At baseline, the characteristics of participants assigned to the two intervention conditions were similar ( Table 1 ). Overall, 14.0% reported a history of cardiovascular disease, 94.0% met the National Cholesterol Education program ATPIII definition for the metabolic syndrome ( 12 ), 15.3% were taking insulin, 87.5% were using diabetes medicines (including insulin), 75.3% were using anti-hypertensive medicines, and 51.0% were using lipid-lowering medicines.
Baseline characteristics of the ILI and DSE groups: mean (standard deviation) or frequency (percent).
Baseline BMI, weight, waist circumference and fitness are given by sex in Table 1 . A BMI of ≥30.0 kg/m 2 was present in 85.1% of participants.
Weight loss
The 1-year examination was attended by 2,496 (97.1%) of the ILI and 2,463 (95.7%) of the DSE participants (p=0.004). Among the factors listed in Table 1 , only the distribution of baseline insulin use significantly varied between non-attendees (21.0%) versus attendees (15.1%): p=0.04. Over the first year of the trial, the ILI group lost an average of 8.6% (standard deviation, 6.9%) of initial body weight compared with 0.7% (4.8%) in the DSE group (p<0.001). Figure 2A portrays the cumulative distribution of weight changes in the two groups. Within the ILI group, 37.8% of participants met the individual weight loss goal (≥10% of initial weight) and 55.2% met the group average goal (≥7%) compared with 3.2% and 7.0% of DSE participants, respectively. These weight losses were accompanied by greater mean reductions in waist circumference in the ILI than DSE group, with mean decreases of 6.2 (10.2) cm versus 0.5 (8.5) cm: p<0.001.

Distribution of one-year changes in percent weight and fitness (METS) among individuals grouped by intervention assignment. Dashed lines are used to indicate the percentages of ILI participants with weight losses exceeding 10%, 7%, and 5%, respectively.
In the ILI group, the average weight loss among baseline insulin users was 7.6% (7.0%) compared with 8.7% (6.9%) in non-users (p=0.002). Insulin users, compared with non-insulin users, were less likely to achieve weight losses >10% (33.5% versus 38.5%) or >7% (47.8% versus 56.4%). In the DSE group, average weight loss was 0.3% (5.1%) among insulin users versus 0.8% (4.7%) among non-insulin users.
Changes in fitness
Figure 2B illustrates the cumulative distribution of measured 1-year fitness changes in 4,246 participants who had repeat testing. Fitness tended to increase in both groups, however increases were more prevalent and tended to be larger among ILI participants; 70.1% of the ILI participants had increased fitness at 1-year compared with 46.3% of the DSE participants (p<0.001). Fitness increases averaged 20.9% (29.1%) among ILI participants compared with 5.8% (22.0%) among DSE participants (p<0.001). These changes could not be fully accounted for by changes in weight. After covariate adjustment for weight changes, the fitted mean difference in fitness increases between groups remained statistically significant (15.9% for ILI versus 10.8% for DSE, p<0.001).
Changes in medicines and cardiovascular risk factors
During the first year, use of glucose lowering medicines among ILI participants decreased from 86.5% to 78.6%, while it increased from 86.5% to 88.7% among DSE participants (p<0.001). As shown in Table 2 , despite this difference, mean fasting glucose declined more among ILI participants compared with DSE participants (p<0.001), as did mean HbA 1 c (p<0.001).
Changes in measures of diabetes control, blood pressure control, measures of lipid/lipoproteins control, albumin/creatinine, and prevalence of metabolic syndrome among participants seen at year 1: mean or percent (standard error).
As described in Table 2 , the prevalence of antihypertensive medicine use remained unchanged among ILI participants, but increased by 2.2% (0.6%) among DSE participants (p=0.02). Mean systolic and diastolic blood pressure levels declined in both groups, but reductions were significantly greater in ILI than in DSE participants (both p<0.001).
Use of lipid-lowering medicines increased in both groups; however, the increase was significantly smaller among ILI participants than in DSE participants (p<0.001). Mean levels of LDL-cholesterol declined by similar magnitudes in both groups (p=0.49). Mean HDL-cholesterol levels increased more among ILI than DSE participants (p<0.001) while mean triglyceride levels decreased more among ILI (p<0.001).
The prevalence of urine albumin/creatinine ratios ≥30.0 ug/mg decreased more among ILI participants than DSE participants (p=0.002).
Classification of participants
The percentage meeting criteria for the metabolic syndrome decreased significantly more among ILI than DSE participants (p<0.001). As shown in Table 2 , the prevalence declined from 93.6 to 78.9 in the ILI group, compared with a decline of 94.4% to 87.3% in the DSE group. The prevalence of meeting ADA goals for HbA 1 c, blood pressure, and LDL-cholesterol increased among both ILI and DSE participants ( Table 3 ). These increases were greater among ILI participants (p<0.001) for HbA 1 c and blood pressure 26.4% (1.0%) vs. 5.4% (1.07%) and 15.1% (1.1%) vs. 7.0% (1.2%) respectively (both p<0.001), but were of similar magnitudes for LDL-cholesterol. The prevalence simultaneously meeting all three goals increased from 10.8% to 23.6% among ILI participants compared with an increase from 9.5% to 16.0% among DSE participants (p<0.001).
Changes percentage of participants meeting American Diabetes Association goals for risk factors: percent (standard error).
CONCLUSIONS
The present results show that clinically significant weight loss is broadly achievable in subjects with type 2 diabetes mellitus and is associated with improved cardiovascular risk factors. At 1 year, participants in ILI achieved an average loss of 8.6% of initial body weight and a 21% improvement in cardiovascular fitness. Separate manuscripts are underway that will provide details on the relative contributions of individual strategies (e.g. meal replacement, orlistat) towards this successful outcome. Even participants on insulin lost an average of 7.6% of initial weight. The ILI was associated with an increase from 46% to 73% in the participants who met the ADA goal of HbA 1 c ≤ 7% and a doubling in the percent of individuals who met all three of the ADA goals for glycemic control, hypertension, and dyslipidemia.
Look AHEAD is the first large clinical trial to compare an intensive weight loss intervention (i.e. ILI) with a support and education group (i.e. DSE) in individuals with type 2 diabetes. As expected, participants in the ILI group had significantly greater weight loss and improvement in fitness at 1 year than those in the DSE group. Moreover, they had a significantly greater decrease in the number of medicines used to treat their diabetes and blood pressure. Despite the greater reductions in these medicines, the ILI group showed greater improvements in their glycemic control, albumin:creatinine ratio, systolic and diastolic blood pressure, triglycerides, and HDL-cholesterol than the DSE group. Changes in LDL-cholesterol were comparable in the two groups. Of particular note is that mean HbA 1 c fell from 7.2% to 6.6%. Few studies, even trials of newer diabetes medicines, have achieved levels of HbA 1 c of 6.6%. Although the DSE group had smaller benefits than ILI, it is important to recognize that these participants also experienced some improvement (not worsening), on average, in weight, fitness, and cardiovascular risk factors.
The Look AHEAD participants are of similar ethnic distribution to that observed in the National Health and Nutrition Examination Survey (NHANES) 1999–2000 ( 14 ), but their average baseline BMI was higher. Overall, they are healthier than diabetic persons in NHANES with regard to glucose, HbA 1 c, and lipid levels and are less likely to smoke. A large percentage was taking medicines for risk factors at study enrollment and many had a significant history of cardiovascular disease. Despite the level of health of the sample, fewer than half met the ADA goal for HbA 1 c and only 10% met all 3 ADA goals ( 13 ). The ILI was extremely effective in increasing the percent of participants who met these goals. At 1 year, 72.7% met the goal for HbA 1 c and 23.6% met all 3 goals, compared with only 50.8% and 16.0%, respectively, for the DSE group. The ILI also was associated with significantly greater remission of the metabolic syndrome than was the DSE intervention.
Several large clinical trials of individuals with impaired glucose tolerance ( 6 , 15 ) or hypertension ( 16 , 17 ) have achieved average weight losses of 4% to 7% at 1 year using intensive lifestyle interventions that emphasized behavior change. These weight losses were associated with marked improvement in health status. Although a number of smaller studies ( 18 , 19 ) have shown that it is possible, using strong behavioral programs, to produce significant weight loss in patients with type 2 diabetes, most studies of weight loss in such individuals have had only modest success. It appears that individuals with diabetes (especially those on insulin) may have more difficulty losing weight and then keeping it off than those without diabetes ( 20 ). For example, among adult Pima Indians receiving standard clinical care for type 2 diabetes, those treated with insulin lost less weight than those treated without drugs or with oral agents ( 21 ). The larger weight losses in Look AHEAD than in prior clinical trials may be attributable to the combination of group and individual contact, the higher physical activity goal that was prescribed, and/or the more intense dietary intervention, which included not only calorie and fat restriction but also structured meal plans, each of which has previously been associated with successful weight loss and maintenance ( 22 , 23 , 24 , 25 ). Although Look AHEAD participants using insulin achieved less average weight loss than those not on insulin (7.6% versus 8.7%), the weight loss of the participants on insulin demonstrates that use of insulin does not prevent successful weight loss. A recent meta-analysis found that the use of meal replacements increased both short and long-term average weight loss by about 2.5 kg, compared with prescription of a conventional reducing diet with the same calorie goals ( 26 ). Our findings that participants in the ILI had significant improvements in cardiovascular risk factors confirms prior studies showing that initial weight loss in type 2 diabetes is associated with improved glycemic control and cardiovascular risk factors at 1 year ( 27 , 28 ). However, the long-term impact of such weight losses remains unclear.
Estimated fitness improved in both groups over the year, but it improved significantly more in the ILI group. It is unknown how much of the improvement in either treatment group was due to measurement variability and greater familiarity with the testing procedure at the 1 year visit and how much represented physiologic change. The difference in improvement between the ILI and DSE groups, however, can be taken as a measure of the ILI treatment effect. This treatment effect persisted even after adjustment for the 1-year weight change. The changes in fitness compared favorably with those observed in prior studies with both diabetic ( 29 , 30 ) and non-diabetic ( 29 , 31 , 32 ) participants. Thus the modest increase in physical activity, primarily walking, had a very beneficial effect. This may translate into a lower rate of cardiovascular events, including mortality, as suggested in some observational studies ( 33 , 34 ).
The primary outcome of the Look AHEAD trial is the effect of weight loss on the development of cardiovascular disease. Although the difference between the ILI and DSE groups in the change in risk factors at 1 year points to the potential cardiovascular benefits of the ILI, we will need several additional years to determine whether the initial weight loss can be maintained, whether weight loss has a long-term effect on the risk factors, and whether the favorable risk factor changes translate into reduced cardiovascular events. This is critical information for establishing evidence-based recommendations with regard to weight loss for the prevention of cardiovascular disease in individuals with diabetes.
Acknowledgments
Xavier Pi-Sunyer, MD; George Blackburn, MD, PhD; Frederick L. Brancati, MD, MHS; George A. Bray, MD; Renee Bright, MS; Jeanne M. Clark, MD, MPH; Jeffrey M. Curtis, MD, MPH; Mark A. Espeland, PhD; John P. Foreyt, PhD; Kathryn Graves, MPH, RD, CDE; Steven M. Haffner, MD; Barbara Harrison, MS; James O. Hill, PhD; Edward S. Horton, MD; John Jakicic, PhD; Robert W. Jeffery, PhD; Karen C. Johnson, MD, MPH; Steven Kahn MB, ChB; David E. Kelley, MD; Abbas E. Kitabchi, MD, PhD; William C. Knowler, MD, DrPH; Cora E. Lewis, MD, MSPH; Barbara J. Maschak-Carey, MSN, CDE; Brenda Montgomery, RN, MS, CDE; David M. Nathan, MD; Jennifer Patricio, MS; Anne Peters, MD; J. Bruce Redmon, MD; Rebecca S. Reeves, DrPH, RD; Donna H. Ryan, MD; Monika Safford, MD; Brent Van Dorsten, PhD; Thomas A. Wadden, PhD; Lynne Wagenknecht, DrPH; Jacqueline Wesche-Thobaben, RN,BSN,CDE; Rena R. Wing, PhD; Susan Z. Yanovski, MD
Clinical Sites
The Johns Hopkins Medical Institutions Frederick L. Brancati, MD, MHS 1 ; Jeff Honas, MS 2 ; Lawrence Cheskin, MD 3 ; Jeanne M. Clark, MD, MPH 3 ; Kerry Stewart, EdD 3 ; Richard Rubin, PhD 3 ; Jeanne Charleston, RN; Kathy Horak, RD
Pennington Biomedical Research Center George A. Bray, MD 1 ; Kristi Rau 2 ; Allison Strate, RN 2 ; Brandi Armand, LPN 2 ; Frank L. Greenway, MD 3 ; Donna H. Ryan, MD 3 ; Donald Williamson, PhD 3 ; Amy Bachand; Michelle Begnaud; Betsy Berhard; Elizabeth Caderette; Barbara Cerniauskas; David Creel; Diane Crow; Helen Guay; Nancy Kora; Kelly LaFleur; Kim Landry; Missy Lingle; Jennifer Perault; Mandy Shipp, RD; Marisa Smith; Elizabeth Tucker
The University of Alabama at Birmingham Cora E. Lewis, MD, MSPH 1 ; Sheikilya Thomas MPH 2 ; Monika Safford, MD 3 ; Vicki DiLillo, PhD; Charlotte Bragg, MS, RD, LD; Amy Dobelstein; Stacey Gilbert, MPH; Stephen Glasser, MD; Sara Hannum, MA; Anne Hubbell, MS; Jennifer Jones, MA; DeLavallade Lee; Ruth Luketic, MA, MBA, MPH; Karen Marshall; L. Christie Oden; Janet Raines, MS; Cathy Roche, RN, BSN; Janet Truman; Nita Webb, MA; Audrey Wrenn, MAEd
Harvard Center
Massachusetts General Hospital : David M. Nathan, MD 1 ; Heather Turgeon, RN, BS, CDE 2 ; Kristina Schumann, BA 2 ; Enrico Cagliero, MD 3 ; Linda Delahanty, MS, RD 3 ; Kathryn Hayward, MD 3 ; Ellen Anderson, MS, RD 3 ; Laurie Bissett, MS, RD; Richard Ginsburg, PhD; Valerie Goldman, MS, RD; Virginia Harlan, MSW; Charles McKitrick, RN, BSN, CDE; Alan McNamara, BS; Theresa Michel, DPT, DSc CCS; Alexi Poulos, BA; Barbara Steiner, EdM; Joclyn Tosch, BA
Joslin Diabetes Center : Edward S. Horton, MD 1 ; Sharon D. Jackson, MS, RD, CDE 2 ; Osama Hamdy, MD, PhD 3 ; A. Enrique Caballero, MD 3 ; Sarah Bain, BS; Elizabeth Bovaird, BSN, RN; Ann Goebel-Fabbri, PhD; Lori Lambert, MS, RD; Sarah Ledbury, MEd, RD; Maureen Malloy, BS; Kerry Ovalle, MS, RCEP, CDE
Beth Israel Deaconess Medical Center : George Blackburn, MD, PhD 1 ; Christos Mantzoros, MD, DSc 3 ; Kristina Day, RD; Ann McNamara, RN
University of Colorado Health Sciences Center James O. Hill, PhD 1 ; Marsha Miller, MS, RD 2 ; JoAnn Phillipp, MS 2 ; Robert Schwartz, MD 3 ; Brent Van Dorsten, PhD 3 ; Judith Regensteiner, PhD 3 ; Salma Benchekroun MS; Ligia Coelho, BS; Paulette Cohrs, RN, BSN; Elizabeth Daeninck, MS, RD; Amy Fields, MPH; Susan Green; April Hamilton, BS, CCRC; Jere Hamilton, BA; Eugene Leshchinskiy; Michael McDermott, MD; Lindsey Munkwitz, BS; Loretta Rome, TRS; Kristin Wallace, MPH; Terra Worley, BA
Baylor College of Medicine John P. Foreyt, PhD 1 ; Rebecca S. Reeves, DrPH, RD 2 ; Henry Pownall, PhD 3 ; Ashok Balasubramanyam, MBBS 3 ; Peter Jones, MD 3 ; Michele Burrington, RD; Chu-Huang Chen, MD, PhD; Allyson Clark, RD; Molly Gee, MEd, RD; Sharon Griggs; Michelle Hamilton; Veronica Holley; Jayne Joseph, RD; Patricia Pace, RD: Julieta Palencia, RN; Olga Satterwhite, RD; Jennifer Schmidt; Devin Volding, LMSW; Carolyn White
University of California at Los Angeles School of Medicine Mohammed F. Saad, MD 1 ; Siran Ghazarian Sengardi, MD 2 ; Ken C. Chiu, MD 3 ; Medhat Botrous; Michelle Chan, BS; Kati Konersman, MA, RD, CDE; Magpuri Perpetua, RD
The University of Tennessee Health Science Center Karen C. Johnson, MD, MPH 1 ; Helen Lambeth, RN, BSN 2 ; Carolyn M. Gresham, RN 2 ; Abbas E. Kitabchi, MD, PhD 3 ; Stephanie A. Connelly, MD, MPH 3 ; Lynne Lichtermann, RN, BSN
University of Minnesota Robert W. Jeffery, PhD 1 ; Carolyn Thorson, CCRP 2 ; John P. Bantle, MD 3 ; J. Bruce Redmon, MD 3 ; Richard S. Crow, MD 3 ; Scott Crow, MD 3 ; Susan K Raatz, PhD, RD 3 ; Kerrin Brelje, MPH, RD; Carolyne Campbell; Jeanne Carls, MEd; Tara Carmean-Mihm, BA; Emily Finch, MA; Anna Fox, MA; Elizabeth Hoelscher, MPH, RD, CHES; La Donna James; Vicki A. Maddy, BS, RD; Therese Ockenden, RN; Birgitta I. Rice, MS, RPh CHES; Tricia Skarphol, BS; Ann D. Tucker, BA; Mary Susan Voeller, BA; Cara Walcheck, BS, RD
St. Luke’s Roosevelt Hospital Center Xavier Pi-Sunyer, MD 1 ; Jennifer Patricio, MS 2 ; Stanley Heshka, PhD 3 ; Carmen Pal, MD 3 ; Lynn Allen, MD; Diane Hirsch, RNC, MS, CDE; Mary Anne Holowaty, MS, CN
University of Pennsylvania Thomas A. Wadden, PhD 1 ; Barbara J. Maschak-Carey, MSN, CDE 2 ; Stanley Schwartz, MD 3 ; Gary D. Foster, PhD 3 ; Robert I. Berkowitz, MD 3 ; Henry Glick, PhD 3 ; Shiriki K. Kumanyika, PhD, RD, MPH 3 ; Johanna Brock; Helen Chomentowski; Vicki Clark; Canice Crerand, PhD; Renee Davenport; Andrea Diamond, MS, RD; Anthony Fabricatore, PhD; Louise Hesson, MSN; Stephanie Krauthamer-Ewing, MPH; Robert Kuehnel, PhD; Patricia Lipschutz, MSN; Monica Mullen, MS, RD; Leslie Womble, PhD, MS; Nayyar Iqbal, MD
University of Pittsburgh David E. Kelley, MD 1 ; Jacqueline Wesche-Thobaben, RN, BSN, CDE 2 ; Lewis Kuller, MD, DrPH 3 ; Andrea Kriska, PhD 3 ; Janet Bonk, RN, MPH; Rebecca Danchenko, BS; Daniel Edmundowicz, MD 3 ; Mary L. Klem, PhD, MLIS 3 ; Monica E. Yamamoto, DrPH, RD, FADA 3 ; Barb Elnyczky, MA; George A. Grove, MS; Pat Harper, MS, RD, LDN; Janet Krulia, RN, BSN, CDE; Juliet Mancino, MS, RD, CDE, LDN; Anne Mathews, MS, RD, LDN; Tracey Y. Murray, BS; Joan R. Ritchea; Jennifer Rush, MPH; Karen Vujevich, RN-BC, MSN, CRNP; Donna Wolf, MS
The Miriam Hospital/Brown Medical School Rena R. Wing, PhD 1 ; Renee Bright, MS 2 ; Vincent Pera, MD 3 ; John Jakicic, PhD 3 ; Deborah Tate, PhD 3 ; Amy Gorin, PhD 3 ; Kara Gallagher, PhD 3 ; Amy Bach, PhD; Barbara Bancroft, RN, MS; Anna Bertorelli, MBA, RD; Richard Carey, BS; Tatum Charron, BS; Heather Chenot, MS; Kimberley Chula-Maguire, MS; Pamela Coward, MS, RD; Lisa Cronkite, BS; Julie Currin, MD; Maureen Daly, RN; Caitlin Egan, MS; Erica Ferguson, BS, RD; Linda Foss, MPH; Jennifer Gauvin, BS; Don Kieffer, PhD; Lauren Lessard, BS; Deborah Maier, MS; JP Massaro, BS; Tammy Monk, MS; Rob Nicholson, PhD; Erin Patterson, BS; Suzanne Phelan, PhD; Hollie Raynor, PhD, RD; Douglas Raynor, PhD; Natalie Robinson, MS, RD; Deborah Robles; Jane Tavares, BS
The University of Texas Health Science Center at San Antonio Steven M. Haffner, MD 1 ; Maria G. Montez, RN, MSHP, CDE 2 ; Carlos Lorenzo, MD 3
University of Washington / VA Puget Sound Health Care System Steven Kahn MB, ChB 1 ; Brenda Montgomery, RN, MS, CDE 2 ; Robert Knopp, MD 3 ; Edward Lipkin, MD 3 ; Matthew L. Maciejewski, PhD 3 ; Dace Trence, MD 3 ; Terry Barrett, BS; Joli Bartell, BA; Diane Greenberg, PhD; Anne Murillo, BS; Betty Ann Richmond, MEd; April Thomas, MPH, RD
Southwestern American Indian Center, Phoenix, Arizona and Shiprock, New Mexico William C. Knowler, MD, DrPH 1 ; Paula Bolin, RN, MC 2 ; Tina Killean, BS 2 ; Jonathan Krakoff, MD 3 ; Jeffrey M. Curtis, MD, MPH 3 ; Justin Glass, MD 3 ; Sara Michaels, MD 3 ; Peter H. Bennett, MB, FRCP 3 ; Tina Morgan 3 ; Shandiin Begay, MPH; Bernadita Fallis RN, RHIT, CCS; Jeanette Hermes, MS,RD; Diane F. Hollowbreast; Ruby Johnson; Cathy Manus LPN; Maria Meacham, BSN, RN, CDE; Julie Nelson, RD; Carol Percy, RN; Patricia Poorthunder; Sandra Sangster; Nancy Scurlock, MSN, ANP-C, CDE; Leigh A. Shovestull, RD, CDE; Janelia Smiley; Katie Toledo, MS, LPC; Christina Tomchee, BA; Darryl Tonemah, PhD
University of Southern California Anne Peters, MD 1 ; Valerie Ruelas, MSW, LCSW 2 ; Siran Ghazarian Sengardi, MD 2 ; Kathryn Graves, MPH, RD, CDE; Kati Konersman, MA, RD, CDE; Sara Serafin-Dokhan
Coordinating Center
Wake Forest University Mark A. Espeland, PhD 1 ; Judy L. Bahnson, BA 2 ; Lynne Wagenknecht, DrPH 3 ; David Reboussin, PhD 3 ; W. Jack Rejeski, PhD 3 ; Alain Bertoni, MD, MPH 3 ; Wei Lang, PhD 3 ; Gary Miller, PhD 3 ; David Lefkowitz, MD 3 ; Patrick S. Reynolds, MD 3 ; Paul Ribisl, PhD 3 ; Mara Vitolins, DrPH 3 ; Michael Booth, MBA 2 ; Kathy M. Dotson, BA 2 ; Amelia Hodges, BA 2 ; Carrie C. Williams, BS 2 ; Jerry M. Barnes, MA; Patricia A. Feeney, MS; Jason Griffin, BS; Lea Harvin, BS; William Herman, MD, MPH; Patricia Hogan, MS; Sarah Jaramillo, MS; Mark King, BS; Kathy Lane, BS; Rebecca Neiberg, MS; Andrea Ruggiero, MS; Christian Speas, BS; Michael P. Walkup, MS; Karen Wall, AAS; Michelle Ward; Delia S. West, PhD; Terri Windham
Central Resources Centers
DXA Reading Center, University of California at San Francisco Michael Nevitt, PhD 1 ; Susan Ewing, MS; Cynthia Hayashi; Jason Maeda, MPH; Lisa Palermo, MS, MA; Michaela Rahorst; Ann Schwartz, PhD; John Shepherd, PhD
Central Laboratory, Northwest Lipid Research Laboratories Santica M. Marcovina, PhD, ScD 1 ; Greg Strylewicz, MS
ECG Reading Center, EPICARE, Wake Forest University School of Medicine RonaldJ. Prineas, MD, PhD 1 ; Teresa Alexander; Lisa Billings; Charles Campbell, AAS, BS; Sharon Hall; Susan Hensley; Yabing Li, MD; Zhu-Ming Zhang, MD
Diet Assessment Center, University of South Carolina, Arnold School of Public Health, Center for Research in Nutrition and Health Disparities Elizabeth J Mayer-Davis, PhD 1 ; Robert Moran, PhD
Hall-Foushee Communications, Inc Richard Foushee, PhD; Nancy J. Hall, MA
Federal Sponsors
National Institute of Diabetes and Digestive and Kidney Diseases : Barbara Harrison, MS; Van S. Hubbard, MD PhD; Susan Z. Yanovski, MD
National Heart, Lung, and Blood Institute : Lawton S. Cooper, MD, MPH; Jeffrey Cutler, MD, MPH; Eva Obarzanek, PhD, MPH, RD
Centers for Disease Control and Prevention : Edward W. Gregg, PhD; David F. Williamson, PhD; Ping Zhang, PhD
Funding and Support
This study is supported by the Department of Health and Human Services through the following cooperative agreements from the National Institutes of Health: DK57136, DK57149, DK56990, DK57177, DK57171, DK57151, DK57182, DK57131, DK57002, DK57078, DK57154, DK57178, DK57219, DK57008, DK57135, and DK56992. The following federal agencies have contributed support: National Institute of Diabetes and Digestive and Kidney Diseases; National Heart, Lung, and Blood Institute; National Institute of Nursing Research; National Center on Minority Health and Health Disparities; Office of Research on Women’s Health; and the Centers for Disease Control and Prevention. This research was supported in part by the Intramural Research Program of the National Institute of Diabetes and Digestive and Kidney Diseases.
Additional support was received from The Johns Hopkins Medical Institutions Bayview General Clinical Research Center (M01RR02719); the Massachusetts General Hospital Mallinckrodt General Clinical Research Center (M01RR01066); the University of Colorado Health Sciences Center General Clinical Research Center (M01RR00051) and Clinical Nutrition Research Unit (P30 DK48520); the University of Tennessee at Memphis General Clinical Research Center (M01RR0021140); the University of Pittsburgh General Clinical Research Center (M01RR000056 44) and NIH grant (DK 046204); and the University of Washington / VA Puget Sound Health Care System Medical Research Service, Department of Veterans Affairs; Frederic C. Bartter General Clinical Research Center (M01RR01346)
The following organizations have committed to make major contributions to Look AHEAD: Federal Express; Health Management Resources; Johnson & Johnson, LifeScan Inc.; Optifast-Novartis Nutrition; Roche Pharmaceuticals; Ross Product Division of Abbott Laboratories; and Slim-Fast Foods Company.
1 Principal Investigator
2 Program Coordinator
3 Co-Investigator
All other Look AHEAD staffs are listed alphabetically by site.
Residents Outside Moscow Protest Power Outage, Demand Heating Amid Subzero Temperatures

Residents of a Moscow region town impacted by power outages have taken to the streets, demanding that local authorities restore heat to their homes as subzero temperatures grip the region, Russian media reported Friday.
At least 21,000 people had power knocked out in their homes on Thursday morning in the town of Podolsk when a heating main burst at the Klimovsk Specialized Ammunition Plant, according to the Kommersant business daily.
The circumstances surrounding the incident at the plant in Podolsk, located some 50 kilometers south of Moscow, have not been disclosed.
The Telegram news channel Ostorozhno Moskva published a video of several local residents who gathered in the town's central square to demand the authorities restore their heating, as well as punish those responsible for the outage.
“The police arrived quickly to disrupt us. I wish they’d brought back heating as fast as they dispatched the cops,” the person recording the video could be heard saying.
A Change.org petition with identical demands was launched online, which, by Friday evening, was signed by nearly 2,000 people.
“Since more than 24 hours have passed, we ask to punish all those involved in this emergency,” the petition reads, naming Podolsk Mayor Grigory Artamonov and the heads of local utilities as the culprits.
Households in the Moscow region towns of Khimki, Balashikha, Solnechnogorsk, Lyubertsy and Elektrostal also experienced heating loss as a result of power outages, with temperatures in the area plummeting to minus 20 degrees Celsius, according to the investigative news website iStories.
The Podyom news outlet, without citing its sources, reported that prosecutors have launched an investigation into the outages.
… we have a small favor to ask.
As you may have heard, The Moscow Times, an independent news source for over 30 years, has been unjustly branded as a "foreign agent" by the Russian government. This blatant attempt to silence our voice is a direct assault on the integrity of journalism and the values we hold dear.
We, the journalists of The Moscow Times, refuse to be silenced. Our commitment to providing accurate and unbiased reporting on Russia remains unshaken. But we need your help to continue our critical mission.
Your support, no matter how small, makes a world of difference. If you can, please support us monthly starting from just 2. It's quick to set up, and you can be confident that you're making a significant impact every month by supporting open, independent journalism. Thank you.
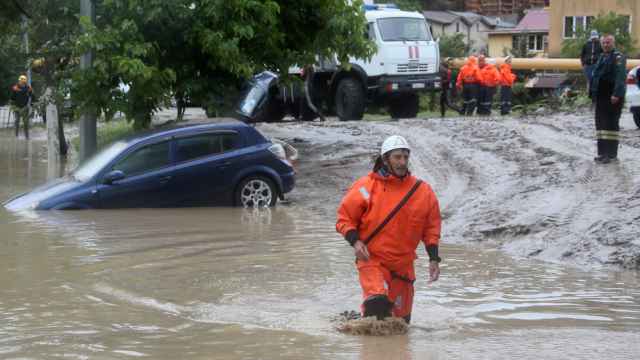
Floods Kill 8 in Southern Russia Resorts
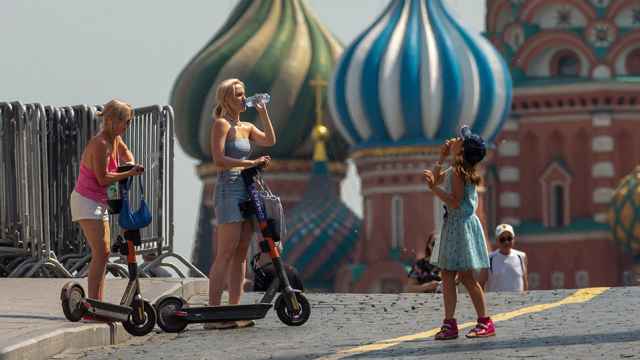
Russia’s Record-Breaking Heatwave to Continue Into July – Weather Service
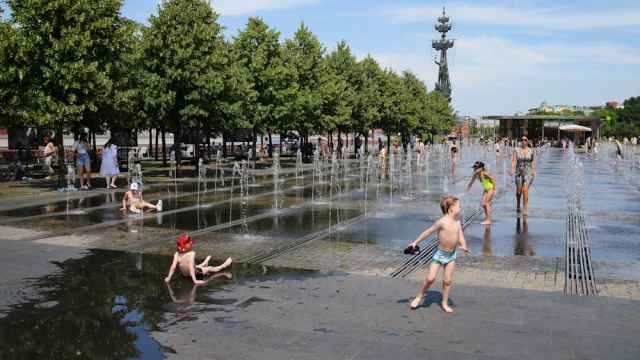
Moscow’s Sweltering Summer Breaks Heat Records
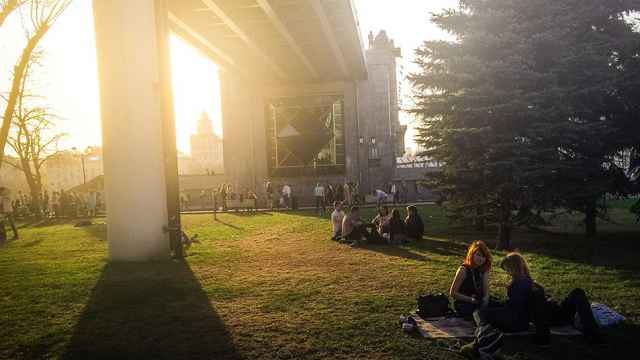
Despite 'Unusual' Sunshine, Moscow is on the Verge of Freezing
Electrostal History and Art Museum
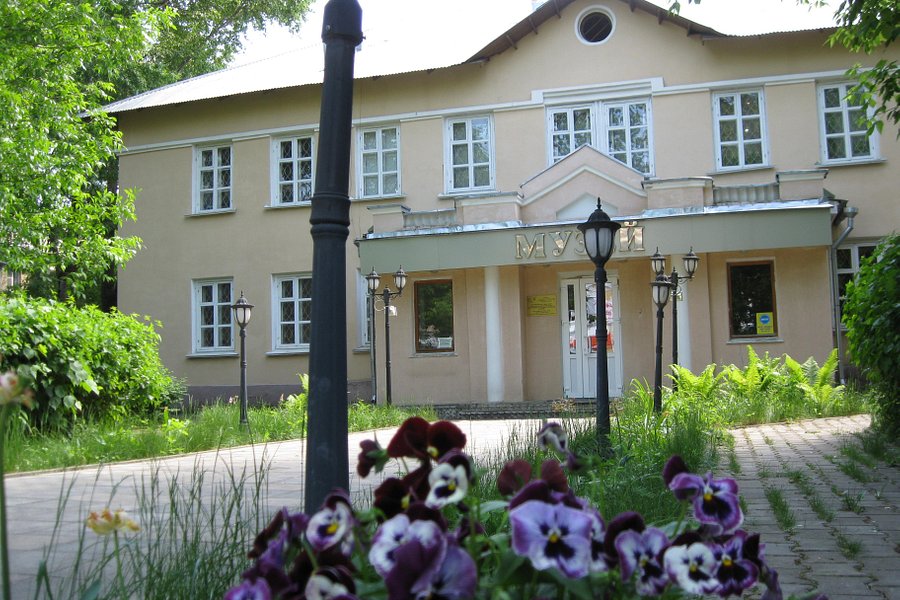
Most Recent: Reviews ordered by most recent publish date in descending order.
Detailed Reviews: Reviews ordered by recency and descriptiveness of user-identified themes such as wait time, length of visit, general tips, and location information.
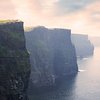
Electrostal History and Art Museum - All You Need to Know BEFORE You Go (2024)
- (0.19 mi) Elektrostal Hotel
- (1.21 mi) Yakor Hotel
- (1.27 mi) Mini Hotel Banifatsiy
- (1.18 mi) Elemash
- (1.36 mi) Hotel Djaz
- (0.07 mi) Prima Bolshogo
- (0.13 mi) Makecoffee
- (0.25 mi) Amsterdam Moments
- (0.25 mi) Pechka
- (0.26 mi) Mazhor
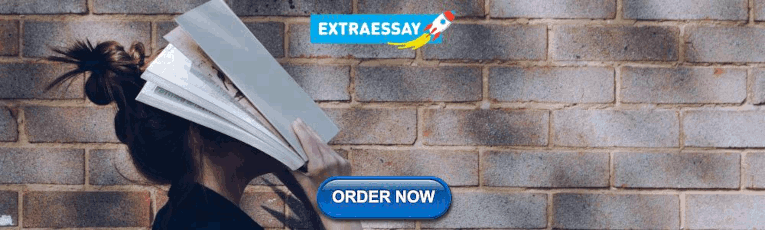
IMAGES
VIDEO
COMMENTS
About Me. I am a Professor in the Divisions of Gerontology and Geriatric Medicine and Public Health Sciences. My PhD is from the University of Rochester in Statistics. I have been at Wake Forest School of Medicine since 1986 and headed its biostatistics department for 11 years. I am a fellow of the American Statistical Association, the Society ...
Mark Espeland. Wake Forest School of Medicine. Verified email at wakehealth.edu - Homepage. biostatistics cognitive impairment aging diabetes clinical trials. Articles Cited by Public access. ... SR Rapp, MA Espeland, SA Shumaker, VW Henderson, RL Brunner, ... Jama 289 (20), 2663-2672, 2003. 1160: 2003:
Address correspondence and reprint requests to Mark Espeland, PhD, Division of Public Health Sciences, Wake Forest University School of Medicine, Medical Center Boulevard, Winston-Salem, NC 27157. E-mail: [email protected] * Authors and members of the Look AHEAD trial are listed in the appendix.
Mark A. Espeland's 633 research works with 35,906 citations and 3,806 reads, including: Latin American Initiative for Lifestyle Intervention to Prevent Cognitive Decline (LatAm-FINGERS): Study ...
Kaycee M. Sink, MD, MAS, 1 Mark A. Espeland, PhD, 2 Cynthia M. Castro, PhD, 3 Timothy Church, MD, ... Mark Espeland had full access to all of the data in the study and takes responsibility for the integrity of the data and the accuracy of the data analysis. Valerie K. Wilson, MD (Adjunct Instructor, Wake Forest School of Medicine, Winston Salem ...
Mark A. Espeland, PhD, 1 Richard E. Pratley, MD, 2 Julio Rosenstock, MD, 3 Takashi Kadowaki, MD, 4 Yutaka Seino, ... Medical writing assistance, supported financially by Boehringer Ingelheim, was provided by Giles Brooke, PhD, CMPP, of Elevate Scientific Solutions during the preparation of this manuscript.
Address correspondence to Mark A. Espeland, PhD, Department of Biostatistics and Data Science, Wake Forest School of Medicine, One Medical Center Blvd, Winston-Salem, NC 27157. E-mail: [email protected] Search for more papers by this author. Sarah A. Gaussoin MS, Sarah A. Gaussoin MS.
Mark A. Espeland, R. Nick Bryan, Joseph S. Goveas, Jennifer G. Robinson, Mustafa S. Siddiqui, Simin Liu, Patricia E. Hogan, Ramon Casanova, Laura H. Coker, Kristine Yaffe, Kamal Masaki, Rebecca Rossom, Susan M. Resnick, for the WHIMS-MRI Study Group; Influence of Type 2 Diabetes on Brain Volumes and Changes in Brain Volumes: Results from the ...
Mark A. Espeland PhD. Department of Biostatistical Sciences, School of Medicine, Wake Forest University, Winston-Salem, North Carolina. Address correspondence to Mark A. Espeland, Department of Biostatistical Sciences, Wake Forest School of Medicine, Medical Center Boulevard, Winston-Salem, NC 27157.
Cardioprotective Effects of Postmenopausal Hormone Treatment Mark A. Espeland, PhD Analyses of observational studies indicate that postmenopausal hormone treatment may be asso- ciated with a 50% reduction in the risk of incipient heart disease in postmenopausal women.1-2 Mecha- nisms that have been proposed to account for the cardioprotective benefit of hormone therapy include beneficial ...
Address correspondence to Mark A. Espeland, PhD, Department of Biostatistical Sciences, Wake Forest School of Medicine, Medical Center Boulevard, Winston-Salem, NC 27157. E-mail: [email protected] Search for more papers by this author. Jiu-Chiuan Chen MD, ScD, Jiu-Chiuan Chen MD, ScD.
Mark A. Espeland, Kasia Lipska, Michael E. Miller, Julia Rushing, Ronald A. Cohen, Joseph Verghese, Mary M. McDermott, Abby C. King, Elsa S. Strotmeyer, Steven N. Blair, Marco Pahor, Kieran Reid, Jamehl Demons, Stephen B. Kritchevsky, for the LIFE Study Investigators, Effects of Physical Activity Intervention on Physical and Cognitive Function in Sedentary Adults With and Without Diabetes, The ...
Mark A. Espeland PhD, Division of Gerontology and Geriatric Medicine, Wake Forest School of Medicine, One Medical Center Boulevard, Winston-Salem, NC 27157, USA. Email: [email protected] Search for more papers by this author. Richard E. Pratley MD, Richard E. Pratley MD.
Wake Forest Baptist Health. View Mark Espeland's profile on LinkedIn, the world's largest professional community. Mark has 1 job listed on their profile. See the complete profile on LinkedIn ...
To demonstrate how principal components analysis can be used to describe patterns of weight changes in response to an intensive lifestyle interventionPrincipal components analysis was applied to monthly percent weight changes measured on 2,485 individuals ...
Mark Espeland reports grant funding from the NIH (R01AG064440; U24AG057437; P30AG049638; R01AG050657), the Department of Health and Human Services (HHSN271201700002C), and the Alzheimer's Association (POINTER-19-611 541).
By Marco Pahor, Steven N. Blair, Mark Espeland, et al., Published on 11/01/06. Effects of a Physical Activity Intervention on Measures of Physical Performance: Results of the Lifestyle Interventions and Independence for Elders Pilot (LIFE-P) Study
About the company. In 1995 it was registered in Moscow representative office of «Granaria Food Group bv», which began to explore the potential of the Russian market. In February 1996, the company was founded by «Chaka», which started selling nuts under the brand name «Chaka» on the Russian market. In September 1998, Elektrostal (Moscow ...
Research Design and Methods. A multi-centered randomized controlled trial of 5,145 individuals with type 2 diabetes, aged 45-74 years, with body mass index ≥25 kg/m 2 (≥27 kg/m 2 if taking insulin). An Intensive Lifestyle Intervention (ILI) involving group and individual meetings to achieve and maintain weight loss through decreased caloric intake and increased physical activity was ...
Mark contributions as unhelpful if you find them irrelevant or not valuable to the article. This feedback is private to you and won't be shared publicly. Got it
Residents of a Moscow region town impacted by power outages have taken to the streets, demanding that local authorities restore heat to their homes as subzero temperatures grip the region, Russian ...
Most Recent: Reviews ordered by most recent publish date in descending order. Detailed Reviews: Reviews ordered by recency and descriptiveness of user-identified themes such as wait time, length of visit, general tips, and location information.