A List of 181 Hot Cyber Security Topics for Research [2024]
Your computer stores your memories, contacts, and study-related materials. It’s probably one of your most valuable items. But how often do you think about its safety?
Our specialists will write a custom essay specially for you!
Cyber security is something that can help you with this. Simply put, it prevents digital attacks so that no one can access your data. Do you want to write a research paper related to the modern challenges of cyberspace? This article has all you need. In here, you’ll find:
- An overview of cyber security’s research areas.
- A selection of compelling cyber security research topics.
And don’t hesitate to contact our custom writing team in case you need any assistance!
- 🔝 Top 10 Topics
- ✅ Research Areas
- ⭐ Top 10 Cybersecurity Topics
- 🔒 Technology Security Topics
- 🖥️ Cybercrime Topics
- ⚖️ Cyber Law & Ethics Topics
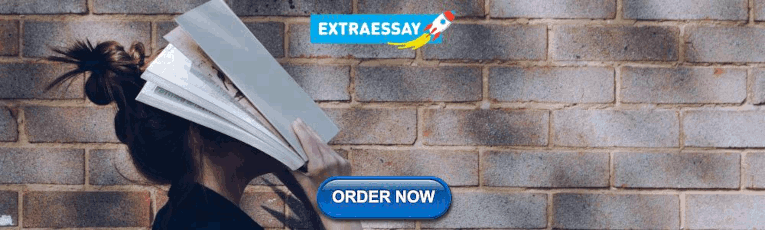
🔍 References
🔝 top 10 cyber security topics.
- How does malware work?
- The principle of zero trust access
- 3 phases of application security
- Should removable media be encrypted?
- The importance of network security
- The importance of end-user education
- Cloud security posture management
- Do biometrics ensure security of IPhones?
- Can strong passwords protect information?
- Is security in critical infrastructure important?
✅ Cyber Security Topics & Research Areas
Cyber security is a vast, constantly evolving field. Its research takes place in many areas. Among them are:

- Safe quantum and space communications . Progress in quantum technologies and space travel calls for extra layers of protection.
- Data privacy. If someone’s personal information falls into the wrong hands, the consequences can be dire. That’s why research in this area focuses on encryption techniques.
- (Inter)national cyberethics, criminology, and law. This branch analyzes how international legal frameworks work online.
- AI and IoT security . We spend more and more of our daily lives online. Additionally, our reliance on AI increases. This scientific field strives to ensure a safe continuation of this path.
As you can see, cyber security extends in various exciting directions that you can explore. Naturally, every paper needs a cover page. We know that it’s one of the more annoying parts, so it’s not a bad thing to use a title page generator for your research paper . Now, let’s move on to our cyber topics list.
⭐ Top 10 Cybersecurity Topics 2024
- Is removable media a threat?
- Blockchain security vulnerabilities
- Why should you avoid public Wi-Fi?
- How to prevent phishing attacks
- Physical security measures in banks
- Security breaches of remote working
- How does two-factor authentication work?
- How to prevent social engineering attacks
- Cybersecurity standards for automotive
- Privacy settings of social media accounts
🔒 Computer Security Topics to Research
Safe computer and network usage is crucial. It concerns not only business but also individuals. Security programs and systems ensure this protection. Explore them with one of our topics:
Just in 1 hour! We will write you a plagiarism-free paper in hardly more than 1 hour
- How do companies avoid sending out confidential information ? Sending an email to the wrong person has happened to the best of us. But what happens if the message’s contents were classified? For your paper, you can find out what technologies can prevent such slip-ups.
- What are the best ways to detect malicious activity ? Any organization’s website gets plenty of daily traffic. People log in, browse, and interact with each other. Among all of them, it might be easy for an intruder to slip in.
- Internet censorship: classified information leaks . China takes internet censorship to the next level. Its comprehensive protection policies gave the system the nickname Great Firewall of China . Discuss this technology in your essay.
- Encrypted viruses as the plague of the century . Antivirus programs are installed on almost every computer. They prevent malicious code from tampering with your data. In your paper, you can conduct a comparison of several such programs.
- What are the pros and cons of various cryptographic methods? Data privacy is becoming more and more critical. That’s why leading messaging services frequently advertise with their encryption technologies .
- What makes blockchain secure ? This technique allows anonymity and decentralization when working with cryptocurrencies . How does it work? What risks are associated with it?
- What are the advantages of SIEM ? Security Incident and Event Management helps organizations detect and handle security threats. Your essay can focus on its relevance for businesses.
- What are the signs of phishing attempts?
- Discuss unified cyber security standards in healthcare .
- Compare and contrast various forms of data extraction techniques.
- What do computers need protocols for?
- Debate the significance of frequent system updates for data security .
- What methods does HTTPS use that make it more secure than HTTP?
- The role of prime numbers in cryptography .
- What are public key certificates , and why are they useful?
- What does a VPN do?
- Are wireless internet connections less secure than LAN ones? If so, why?
- How do authentication processes work?
- What can you do with IP addresses?
- Explain the technology of unlocking your phone via facial recognition vs. your fingerprint.
- How do you prevent intrusion attempts in networks ?
- What makes Telnet vulnerable?
- What are the phases of a Trojan horse attack?
- Compare the encryption technologies of various social networks.
- Asymmetric vs. symmetric algorithms.
- How can a person reach maximum security in the computer networking world ?
- Discuss autoencoders and reveal how they work.
💾 Information Security Topics to Research
Information security’s goal is to protect the transmission and storage of data. On top of that, network security topics are at the forefront of infosec research. If you’re looking for inspiration on the subject, check out these ideas.
- What are the mechanics of password protection ? Passwords are a simple tool to ensure confidentiality. What do users and developers need to keep in mind when handling passwords?
- What are the safest ways to ensure data integrity ? Everybody wants their data to be intact. Accidental or malicious modifications of data can have dire consequences for organizations and individuals. Explore ways to avoid it.
- How can one establish non-repudiation? Non-repudiation proves the validity of your data. It’s essential in legal cases and cyber security .
- How did the advent of these new technologies impact information security ? Mobile networks have changed the way we access information. On a smartphone , everything is permanently available at your fingertips. What adverse consequences did these technologies bring?
- How do big corporations ensure that their database environment stays conflict-free? We expect our computers to always run fast and without errors. For institutions such as hospitals, a smooth workflow is vital. Discuss how it can be achieved.
- Describe solid access control methods for organizations. In a company, employees need access to different things. This means that not everyone should have an admin account. How should we control access to information ?
- Medical device cyber security. For maximum safety, it’s best to employ several measures. Protection on the hard- and software side is just a part of it. What are some other means of security?
- Write an argumentative essay on why a career in information security doesn’t require a degree.
- Pros and cons of various infosec certificates.
- Cybersecurity in cruise ship industry
- The influence of remote work on a businesses’ infosec network .
- What should everyone be aware of when it comes to safeguarding private information?
- Select a company and calculate how much budget they should allocate on cyber security.
- What are the dangers of public Wi-Fi networks ?
- How secure are cloud services ?
- Apple vs. Microsoft : whose systems offer better security?
- Why is it important to remove a USB flash drive safely?
- Is it possible to create an unguessable password?
- Intranet security : best practices.
- Does the use of biometrics increase security?
- Face recognition vs. a simple code: what are the safest locking options for smartphones ?
- How do you recover data from a broken hard drive?
- Discuss the functions and risks of cookies and cache files.
- Online privacy regulations in the US and China.
- Physical components of infosec .
- Debate security concerns regarding electronic health records .
- What are unified user profiles, and what makes them potentially risky?
🖥️ Cybercrime Topics for a Research Paper
Knowledge is one of today’s most valuable assets. Because of this, cybercrimes usually target the extraction of information. This practice can have devastating effects. Do you want to learn more about the virtual world’s dark side? This section is for you.
- Give an overview of the various types of cybercrimes today . Cybercriminals are becoming more and more inventive. It’s not easy to keep up with the new threats appearing every day. What threats are currently the most prominent?
- How does cryptojacking work, and why is it problematic? Cryptocurrency’s value explosion has made people greedy. Countries such as Iceland have become a haven for datamining. Explore these issues in your essay.
- Analyze the success rate of email frauds . You’ve probably seen irrelevant ads in your spam folder before. They often sound so silly it’s hard to believe they work. Yet, unfortunately, many people become victims of such scams.
- How did the WannaCry malware work? WannaCry was ransomware that caused global trouble in 2017. It led to financial losses in the billions. What made it so dangerous and hard to stop?
- Give famous examples of cybercrimes that targeted people instead of money . Not all cybercrimes want to generate profit. Sometimes, the reasons are political or personal. Explore several instances of such crimes in your essay. How did they pan out?

- Analyze the implications of the Cyberpunk 2077 leak. The game’s bugs and issues made many people angry. Shortly after its flop, hackers released developer CD Projekt Red’s source codes. What far-reaching consequences could this have?
- Why do hackers commit identity theft? Social media has made it easy to steal identities . Many like to display their lives online. In your paper, research what happens to the victims of identity thefts.
- Should governments punish cybercrimes like real-life crimes?
- How does ransomware work?
- Describe the phases of a DDoS attack.
- What cybercrime cases led to changes in the legislature ?
- Track the evolution of online scams.
- Online grooming: how to protect children from predators.
- Are cybercrimes “gateway crimes” that lead to real-life misbehavior?
- What are man-in-the-middle attacks?
- Big data and the rise of internet crimes.
- Are cybercrimes more dangerous to society than they are to corporations?
- Is the internet increasing the likelihood of adolescents engaging in illegal activities?
- Do the downsides of cyberlife outweigh its positives?
- Is constantly checking your crush’s Facebook page cyberstalking?
- How do you recognize your online date is a scam?
- Describe what happens during a Brute Force attack.
- What’s the difference between pharming and phishing?
- The Lehman Bank cybercrimes
- Should the punishments for cybercriminals be harsher than they are now?
- Compare various types of fraud methods .
- How do you mitigate a denial-of-service attack?
🕵️ Topics for a Research Paper on Hacking
Blinking screens and flashing lines of code: the movie industry makes hacking look fascinating. But what actually happens when someone breaks into another person’s computer’s system? Write a paper about it and find out! The following prompts allow you to dive deeper into the subject.
- Is it vital to keep shutting down online movie streaming sites? Many websites offer free movie streaming. If one of their domains gets closed down, they just open another one. Are they a threat to the industry that must be stopped? Or should cyber law enforcement rather focus on more serious crimes?
- Explore the ethical side of whistleblowing. WikiLeaks is a platform for whistleblowers. Its founder, Julian Assange, has been under arrest for a long time. Should whistleblowing be a crime? Why or why not?
- How did Kevin Mitnick’s actions contribute to the American cyber legislature? Mitnick was one of the US’s first most notorious hackers. He claimed to have broken into NORAD’s system. What were the consequences?
- Examine how GhostNet operates. GhostNet is a large organization attacking governments. Its discovery in 2009 led to a major scandal.
- Describe how an SQL injection attack unfolds. Injection attacks target SQL databases and libraries. This way, hackers gain unauthorized access to data.
- What political consequences did the attack on The Interview imply? In 2014, hackers threatened to attack theaters that showed The Interview . As a result, Sony only showed the movie online and in limited releases.
- Write about cross-site request forgery attacks. Every website tells you that logging out is a crucial step. But what can happen if you don’t do it?
- What is “Anonymous,” and what do they do?
- Is it permissible to hack a system to raise awareness of its vulnerabilities?
- Investigate the origins of the hacking culture .
- How did industrial espionage evolve into hacking?
- Is piracy destroying the music and movie industries ?
- Explain the term “cyberwarfare.”
- Contrast different types of hacking .
- Connections between political protests and hacking.
- Is it possible to create an encryption that can’t be hacked?
- The role of hackers in modern warfare .
- Can hacking be ethical?
- Who or what are white hat hackers ?
- Discuss what various types of hackers do.
- Is jailbreaking a crime?
- How does hacking a phone differ from hacking a computer?
- Is hacking your personal home devices problematic?
- What is clickjacking?
- Why would hackers target newspapers ?
- Examine the consequences society would have to bear if a hacker targeted the state.
- Compare and analyze different hacking collectives.
⚖️ Topics on Cyber Law & Ethics to Look Into
Virtual life needs rules just like the real one does. The online world brings a different set of values and issues to the table. And, naturally, cyberlife has a legal framework. That’s where researching cyber law and ethics comes into play.
- Is it ethical that governments can always access their citizens’ data? In some countries, online platforms for personal information are standard. From medical exams to debts , everything is available with a click. The system is inarguably convenient. But what about its downsides?
- Is it still morally permissible to use Spotify ? Spotify has made listening to music more accessible than ever. However, artists only receive a tiny fraction of the company’s profits. Discuss the implications of this fact.
- Should internet forums require users to display their real names? Online harassment is a widespread problem. Nicknames hide the identities of ordinary users as well as perpetrators. Can the mandatory use of real names change the situation?
- Analyze online gaming behavior from a psychological standpoint. If one wants to play online games, one needs to have a thick skin. The community can be harsh. You can dedicate your paper to exploring these behaviors. Or you might want to ponder what game publishers can do to reduce hate speech.
- What type of restrictions should sellers implement to prevent domain speculation? Some people buy domains hoping that they will sell them later for more money. This practice makes registering a new website trickier.
- Does the internet need regulations to make adult content less visible? Every computer without parental control can access pornographic websites. Most of them don’t require registration . Their contents can be disturbing, and their ads can appear anywhere. What can be done about it?
- What are cyber laws still missing in America? The US has established many laws to regulate internet usage. Select the most significant ones and explain their relevance.
- Why should cyber ethics be different from real-world norms?
- Are there instances in which illegal downloading is justified?
- The rule of law in real life vs. in cyberspace.
- Does the internet need a government?
- What is cyber terrorism, and what makes it dangerous?
- Who is responsible for online misbehavior?
- How binding are netiquettes?
- What did the implementation of the GDPR change?
- Compare and contrast Indian vs. Venezuelan internet regulations.
- What does the CLOUD entail?
- How should law enforcement adapt to online technologies?
- AI applications : ethical limits and possibilities.
- Discuss trending topics in cyber law of the past ten years.
- Should schools teach online etiquette?
- Does internet anonymity bring out the worst in people?
- Is data privacy more important than convenience and centralization?
- Debate whether bitcoins could become the currency of the future.
- How can online consumers protect themselves from fraud ?
- Is buying from websites like eBay and Craigslist more ethical than buying from other online marketplaces?
- Present RSF’s Minecraft library and discuss its moral implications.
🖱️ Cyberbullying Topics for Essays and Papers
On the web, everyone can remain anonymous. With this added comfort, bullying rises to another level. It’s a serious issue that’s getting more and more problematic. Cyber security measures can alleviate the burden. Do you want to address the problem? Have a look at our cyberbullying topics below.
Receive a plagiarism-free paper tailored to your instructions. Cut 20% off your first order!
- Cyberbullying prevention in online learning environments . Online classes increase the possibility of cyberbullying. What can teachers do to watch out for their students?
- What makes online emotional abuse particularly difficult to bear? Bullying doesn’t necessarily have to be physical to hurt. Statistics show increased suicide rates among students who were harassed online. Explore the reasons behind this phenomenon.
- How can victims of identity theft reclaim their lives? Identity theft leads not only to mental distress. Thieves also have access to credit card information and other essential assets.
- What are the best methods to stay safe online? When surfing the internet, one always has to be on one’s toes. Avoiding harassment and bullying is a particularly challenging task.
- How can parents monitor their children’s behavior on the web? Children are particularly vulnerable online. They might enter dangerous online relationships with strangers if they feel lonely. They are also more susceptible to scams. What can parents do to protect them?
- Cyberbullying among university students. Online abuse in such websites is very common. Everyone can be a potential target, regardless of age or gender. Discuss whether the structure of social networks helps to spread cyberbullying.
- What societal factors contribute to online bullying? Not everyone who uses the internet becomes an abuser. It’s possible to establish several psychological characteristics of cyberbullies. Explore them in your paper.
- Define how cyberbullying differs from in-person harassment .
- Establish a link between feminism and the fight against cyberstalking .
- The emotional consequences of physical vs. verbal abuse.
- The effects of cyberbullying and academics.
- Short vs. long-term mental health effects of internet bullying .
- What are the most widespread means of cyberbullying ?
- Should people who want to play video games online get over the fact that the community is toxic?
- Is defending the freedom of speech more important than preventing the spread of hate speech?
- Reasons and consequences of Amanda Todd’s suicide.
- The dangers of pro-ana/-mia communities for adolescents.
- What are effective strategies to cope with online harassment ?
- Would cyber communism decrease bullying?
- How enhanced cyber security measures can help reduce abuse.
- The importance of parental control mechanisms on children’s computers.
- Traditional vs. cyberbullying in children.
- Do image-heavy websites such as Tumblr and Instagram affect one’s mental state similarly to active abuse?
- What kind of people does cyber abuse affect the most, and why?
- Analyze how the stalker uses the internet in Netflix’s series You .
- Catfishing: effects and solutions.
Thanks for reading through our article. If you found it helpful, consider sharing it with your friends. We wish you good luck with your project!
Further reading:
- 220 Best Science and Technology Essay Topics to Write About
- 204 Research Topics on Technology & Computer Science
- A List of 580 Interesting Research Topics [2024 Edition]
- A List of 179 Problem Solution Essay Topics & Questions
- 193 Interesting Proposal Essay Topics and Ideas
- 226 Research Topics on Criminal Justice & Criminology
- What Is Cybersecurity?: Cisco
- Cyber Security: Research Areas: The University of Queensland, Australia
- Cybersecurity: National Institute of Standards and Technology
- What Is Information Security?: CSO Online
- Articles on Cyber Ethics: The Conversation
- What Is Cybercrime?: Kaspersky
- Types of Cybercrime and How to Protect Yourself Against Them: Security Traits
- Hacking: Computing: Encyclopedia Britannica
- Hacking News: Science Daily
- Cyberbullying and Cybersecurity: How Are They Connected?: AT&T
- Cyberbullying: What Is It and How to Stop It: UNICEF
- Current Awareness: Cyberlaw Decoded: Florida State University
- Share to Facebook
- Share to Twitter
- Share to LinkedIn
- Share to email
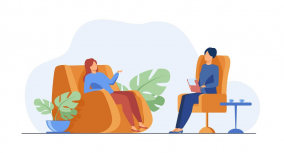
Have you ever wondered why everyone has a unique set of character traits? What is the connection between brain function and people’s behavior? How do we memorize things or make decisions? These are quite intriguing and puzzling questions, right? A science that will answer them is psychology. It’s a multi-faceted...
![research paper topics on computer security Student Exchange Program (Flex) Essay Topics [2024]](https://custom-writing.org/blog/wp-content/uploads/2021/01/student-exchange-program-284x153.jpg)
Participating in a student exchange program is a perfect opportunity to visit different countries during your college years. You can discover more about other cultures and learn a new language or two. If you have a chance to take part in such a foreign exchange, don’t miss it. Keep in...
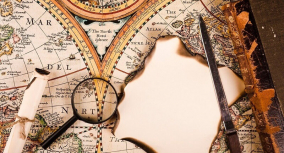
How can you define America? If you’ve ever asked yourself this question, studying US history will help you find the answer. This article will help you dive deeper into this versatile subject. Here, you will find: Early and modern US history topics to write about. We’ve also got topics for...
![research paper topics on computer security 380 Powerful Women’s Rights & Feminism Topics [2024]](https://custom-writing.org/blog/wp-content/uploads/2021/01/Womens-Rights-284x153.jpg)
Are you looking for perfect feminist topics? Then you’ve come to the right place. With our help, you can be sure to craft a great essay. Here, you can find feminist topics for discussion, feminism research topics and other ideas and questions for students. Some people think all feminists hate...
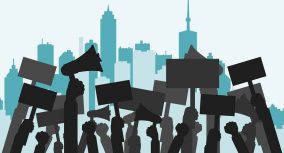
If you have an assignment in politics, look no further—this article will help you ace your paper. Here, you will find a list of unique political topics to write about compiled by our custom writing team. But that’s not all of it! Keep reading if you want to: See how to tackle political essay topics in your paper; Choose a topic that will be interesting for you to research; Refresh your knowledge of essential political concepts. Now, without further ado, let’s get started! Below, you’ll find political topics and questions for your task. 🔝 Top 10...
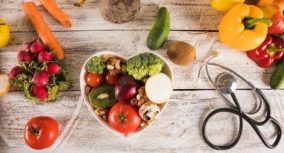
It’s not a secret that our health largely depends on nutrition. A balanced and wholesome diet improves our immune system. It lowers the risk of getting sick and makes us more productive. But if we don’t eat right, our overall well-being and performance worsen. You see, nutrition topics are more...
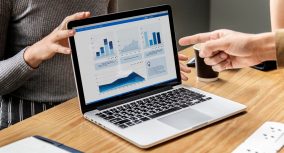
A presentation is a speech in which you explain a topic to an audience. It usually includes visuals done in a program such as PowerPoint. Teachers in schools and in colleges love to assign presentations for various reasons: It requires students to put their knowledge into practice.It teaches them how...
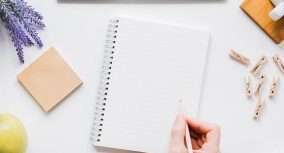
In modern societies, people do everything to live peacefully. Still, tensions often arise. We call them social issues when they start negatively impacting a specific group of people. Poverty, discrimination, and addiction are examples of such problems. We need to confront them to ensure equal treatment for everyone.
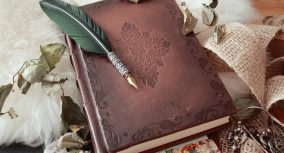
There are many ways to define popular culture. Here’s one of them: pop culture includes mainstream preferences in society within a specific time frame. It covers fashion, music, language, and even food. Pop culture is always evolving, engaging in new trends, and leaving the old ones behind.
![research paper topics on computer security 500 Sociology Questions and Topics [Examples & Tips]](https://custom-writing.org/blog/wp-content/uploads/2021/01/Sociology-Questions-284x153.jpg)
Sociology is a study that focuses on people’s interactions. It looks at structures and changes in social life. Any situation involving people can become a topic of sociology. This article is designed to help high school and college students with sociology assignments. Whether you’re writing an essay, creating a presentation,...
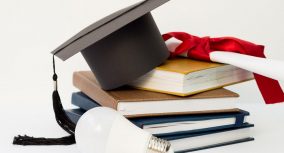
Controversial issues are the ones that evoke a variety of opinions. They often cause heated debates. And, as you can guess, controversial research topics are not easy to handle. Luckily, we’ve got you covered. This article will: help you pick a controversial question for your essay;provide you a list of...
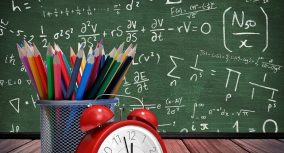
Plates break when you drop them. Glasses help you see better. Have you ever wondered why? Physics has the answer. It studies the observable as well as invisible aspects of nature. An essential part of this is examining the structure and interactions of matter.
For enquiries call:
+1-469-442-0620

60+ Latest Cyber Security Research Topics for 2024
Home Blog Security 60+ Latest Cyber Security Research Topics for 2024

The concept of cybersecurity refers to cracking the security mechanisms that break in dynamic environments. Implementing Cyber Security Project topics and cyber security thesis topics /ideas helps overcome attacks and take mitigation approaches to security risks and threats in real-time. Undoubtedly, it focuses on events injected into the system, data, and the whole network to attack/disturb it.
The network can be attacked in various ways, including Distributed DoS, Knowledge Disruptions, Computer Viruses / Worms, and many more. Cyber-attacks are still rising, and more are waiting to harm their targeted systems and networks. Detecting Intrusions in cybersecurity has become challenging due to their Intelligence Performance. Therefore, it may negatively affect data integrity, privacy, availability, and security.
This article aims to demonstrate the most current Cyber Security Topics for Projects and areas of research currently lacking. We will talk about cyber security research questions, cyber security research questions, cyber security topics for the project, best cyber security research topics, research titles about cyber security and web security research topics.
.jpg&w=3840&q=75)
List of Trending Cyber Security Research Topics for 2024
Digital technology has revolutionized how all businesses, large or small, work, and even governments manage their day-to-day activities, requiring organizations, corporations, and government agencies to utilize computerized systems. To protect data against online attacks or unauthorized access, cybersecurity is a priority. There are many Cyber Security Courses online where you can learn about these topics. With the rapid development of technology comes an equally rapid shift in Cyber Security Research Topics and cybersecurity trends, as data breaches, ransomware, and hacks become almost routine news items. In 2024, these will be the top cybersecurity trends.
A) Exciting Mobile Cyber Security Research Paper Topics
- The significance of continuous user authentication on mobile gadgets.
- The efficacy of different mobile security approaches.
- Detecting mobile phone hacking.
- Assessing the threat of using portable devices to access banking services.
- Cybersecurity and mobile applications.
- The vulnerabilities in wireless mobile data exchange.
- The rise of mobile malware.
- The evolution of Android malware.
- How to know you’ve been hacked on mobile.
- The impact of mobile gadgets on cybersecurity.
B) Top Computer and Software Security Topics to Research
- Learn algorithms for data encryption
- Concept of risk management security
- How to develop the best Internet security software
- What are Encrypting Viruses- How does it work?
- How does a Ransomware attack work?
- Scanning of malware on your PC
- Infiltrating a Mac OS X operating system
- What are the effects of RSA on network security ?
- How do encrypting viruses work?
- DDoS attacks on IoT devices
C) Trending Information Security Research Topics
- Why should people avoid sharing their details on Facebook?
- What is the importance of unified user profiles?
- Discuss Cookies and Privacy
- White hat and black hat hackers
- What are the most secure methods for ensuring data integrity?
- Talk about the implications of Wi-Fi hacking apps on mobile phones
- Analyze the data breaches in 2024
- Discuss digital piracy in 2024
- critical cyber-attack concepts
- Social engineering and its importance
D) Current Network Security Research Topics
- Data storage centralization
- Identify Malicious activity on a computer system.
- Firewall
- Importance of keeping updated Software
- wireless sensor network
- What are the effects of ad-hoc networks
- How can a company network be safe?
- What are Network segmentation and its applications?
- Discuss Data Loss Prevention systems
- Discuss various methods for establishing secure algorithms in a network.
- Talk about two-factor authentication
E) Best Data Security Research Topics
- Importance of backup and recovery
- Benefits of logging for applications
- Understand physical data security
- Importance of Cloud Security
- In computing, the relationship between privacy and data security
- Talk about data leaks in mobile apps
- Discuss the effects of a black hole on a network system.
F) Important Application Security Research Topics
- Detect Malicious Activity on Google Play Apps
- Dangers of XSS attacks on apps
- Discuss SQL injection attacks.
- Insecure Deserialization Effect
- Check Security protocols
G) Cybersecurity Law & Ethics Research Topics
- Strict cybersecurity laws in China
- Importance of the Cybersecurity Information Sharing Act.
- USA, UK, and other countries' cybersecurity laws
- Discuss The Pipeline Security Act in the United States
H) Recent Cyberbullying Topics
- Protecting your Online Identity and Reputation
- Online Safety
- Sexual Harassment and Sexual Bullying
- Dealing with Bullying
- Stress Center for Teens
I) Operational Security Topics
- Identify sensitive data
- Identify possible threats
- Analyze security threats and vulnerabilities
- Appraise the threat level and vulnerability risk
- Devise a plan to mitigate the threats
J) Cybercrime Topics for a Research Paper
- Crime Prevention.
- Criminal Specialization.
- Drug Courts.
- Criminal Courts.
- Criminal Justice Ethics.
- Capital Punishment.
- Community Corrections.
- Criminal Law.
Research Area in Cyber Security
The field of cyber security is extensive and constantly evolving. Its research covers a wide range of subjects, including:
- Quantum & Space
- Data Privacy
- Criminology & Law
- AI & IoT Security
How to Choose the Best Research Topics in Cyber Security
A good cybersecurity assignment heading is a skill that not everyone has, and unfortunately, not everyone has one. You might have your teacher provide you with the topics, or you might be asked to come up with your own. If you want more research topics, you can take references from Certified Ethical Hacker Certification, where you will get more hints on new topics. If you don't know where to start, here are some tips. Follow them to create compelling cybersecurity assignment topics.
1. Brainstorm
In order to select the most appropriate heading for your cybersecurity assignment, you first need to brainstorm ideas. What specific matter do you wish to explore? In this case, come up with relevant topics about the subject and select those relevant to your issue when you use our list of topics. You can also go to cyber security-oriented websites to get some ideas. Using any blog post on the internet can prove helpful if you intend to write a research paper on security threats in 2024. Creating a brainstorming list with all the keywords and cybersecurity concepts you wish to discuss is another great way to start. Once that's done, pick the topics you feel most comfortable handling. Keep in mind to stay away from common topics as much as possible.
2. Understanding the Background
In order to write a cybersecurity assignment, you need to identify two or three research paper topics. Obtain the necessary resources and review them to gain background information on your heading. This will also allow you to learn new terminologies that can be used in your title to enhance it.
3. Write a Single Topic
Make sure the subject of your cybersecurity research paper doesn't fall into either extreme. Make sure the title is neither too narrow nor too broad. Topics on either extreme will be challenging to research and write about.
4. Be Flexible
There is no rule to say that the title you choose is permanent. It is perfectly okay to change your research paper topic along the way. For example, if you find another topic on this list to better suit your research paper, consider swapping it out.
The Layout of Cybersecurity Research Guidance
It is undeniable that usability is one of cybersecurity's most important social issues today. Increasingly, security features have become standard components of our digital environment, which pervade our lives and require both novices and experts to use them. Supported by confidentiality, integrity, and availability concerns, security features have become essential components of our digital environment.
In order to make security features easily accessible to a wider population, these functions need to be highly usable. This is especially true in this context because poor usability typically translates into the inadequate application of cybersecurity tools and functionality, resulting in their limited effectiveness.
Writing Tips from Expert
Additionally, a well-planned action plan and a set of useful tools are essential for delving into Cyber Security Research Topics. Not only do these topics present a vast realm of knowledge and potential innovation, but they also have paramount importance in today's digital age. Addressing the challenges and nuances of these research areas will contribute significantly to the global cybersecurity landscape, ensuring safer digital environments for all. It's crucial to approach these topics with diligence and an open mind to uncover groundbreaking insights.
- Before you begin writing your research paper, make sure you understand the assignment.
- Your Research Paper Should Have an Engaging Topic
- Find reputable sources by doing a little research
- Precisely state your thesis on cybersecurity
- A rough outline should be developed
- Finish your paper by writing a draft
- Make sure that your bibliography is formatted correctly and cites your sources.
Discover the Power of ITIL 4 Foundation - Unleash the Potential of Your Business with this Cost-Effective Solution. Boost Efficiency, Streamline Processes, and Stay Ahead of the Competition. Learn More!
Studies in the literature have identified and recommended guidelines and recommendations for addressing security usability problems to provide highly usable security. The purpose of such papers is to consolidate existing design guidelines and define an initial core list that can be used for future reference in the field of Cyber Security Research Topics.
The researcher takes advantage of the opportunity to provide an up-to-date analysis of cybersecurity usability issues and evaluation techniques applied so far. As a result of this research paper, researchers and practitioners interested in cybersecurity systems who value human and social design elements are likely to find it useful. You can find KnowledgeHut’s Cyber Security courses online and take maximum advantage of them.
Frequently Asked Questions (FAQs)
Businesses and individuals are changing how they handle cybersecurity as technology changes rapidly - from cloud-based services to new IoT devices.
Ideally, you should have read many papers and know their structure, what information they contain, and so on if you want to write something of interest to others.
The field of cyber security is extensive and constantly evolving. Its research covers various subjects, including Quantum & Space, Data Privacy, Criminology & Law, and AI & IoT Security.
Inmates having the right to work, transportation of concealed weapons, rape and violence in prison, verdicts on plea agreements, rehab versus reform, and how reliable are eyewitnesses?

Mrinal Prakash
I am a B.Tech Student who blogs about various topics on cyber security and is specialized in web application security
Avail your free 1:1 mentorship session.
Something went wrong
Upcoming Cyber Security Batches & Dates

- Search Menu
- Editor's Choice
- Author Guidelines
- Submission Site
- Open Access
- About Journal of Cybersecurity
- Editorial Board
- Advertising and Corporate Services
- Journals Career Network
- Self-Archiving Policy
- Journals on Oxford Academic
- Books on Oxford Academic

Editors-in-Chief
Tyler Moore
About the journal
Journal of Cybersecurity publishes accessible articles describing original research in the inherently interdisciplinary world of computer, systems, and information security …
Latest articles
Call for Papers
Journal of Cybersecurity is soliciting papers for a special collection on the philosophy of information security. This collection will explore research at the intersection of philosophy, information security, and philosophy of science.
Find out more

High-Impact Research Collection
Explore a collection of freely available high-impact research from 2020 and 2021 published in the Journal of Cybersecurity .
Browse the collection here

Submit your paper
Join the conversation moving the science of security forward. Visit our Instructions to Authors for more information about how to submit your manuscript.

Read and Publish deals
Authors interested in publishing in Journal of Cybersecurity may be able to publish their paper Open Access using funds available through their institution’s agreement with OUP.
Find out if your institution is participating
Related Titles

Affiliations
- Online ISSN 2057-2093
- Print ISSN 2057-2085
- Copyright © 2024 Oxford University Press
- About Oxford Academic
- Publish journals with us
- University press partners
- What we publish
- New features
- Open access
- Institutional account management
- Rights and permissions
- Get help with access
- Accessibility
- Advertising
- Media enquiries
- Oxford University Press
- Oxford Languages
- University of Oxford
Oxford University Press is a department of the University of Oxford. It furthers the University's objective of excellence in research, scholarship, and education by publishing worldwide
- Copyright © 2024 Oxford University Press
- Cookie settings
- Cookie policy
- Privacy policy
- Legal notice
This Feature Is Available To Subscribers Only
Sign In or Create an Account
This PDF is available to Subscribers Only
For full access to this pdf, sign in to an existing account, or purchase an annual subscription.

Research Topics & Ideas: Cybersecurity
50 Topic Ideas To Kickstart Your Research
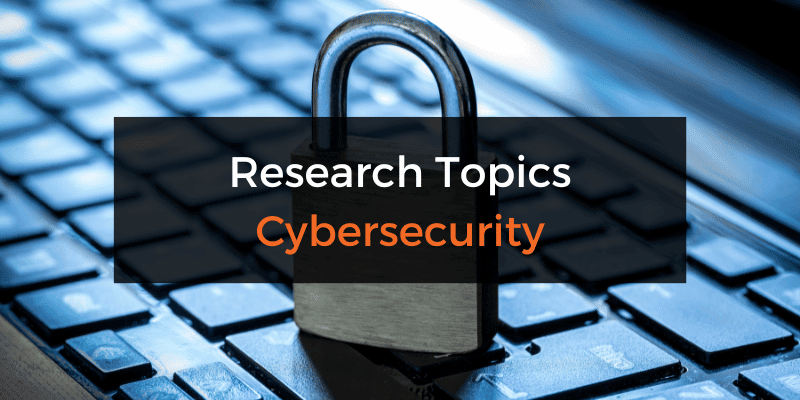
If you’re just starting out exploring cybersecurity-related topics for your dissertation, thesis or research project, you’ve come to the right place. In this post, we’ll help kickstart your research by providing a hearty list of cybersecurity-related research topics and ideas , including examples from recent studies.
PS – This is just the start…
We know it’s exciting to run through a list of research topics, but please keep in mind that this list is just a starting point . These topic ideas provided here are intentionally broad and generic , so keep in mind that you will need to develop them further. Nevertheless, they should inspire some ideas for your project.
To develop a suitable research topic, you’ll need to identify a clear and convincing research gap , and a viable plan to fill that gap. If this sounds foreign to you, check out our free research topic webinar that explores how to find and refine a high-quality research topic, from scratch. Alternatively, consider our 1-on-1 coaching service .
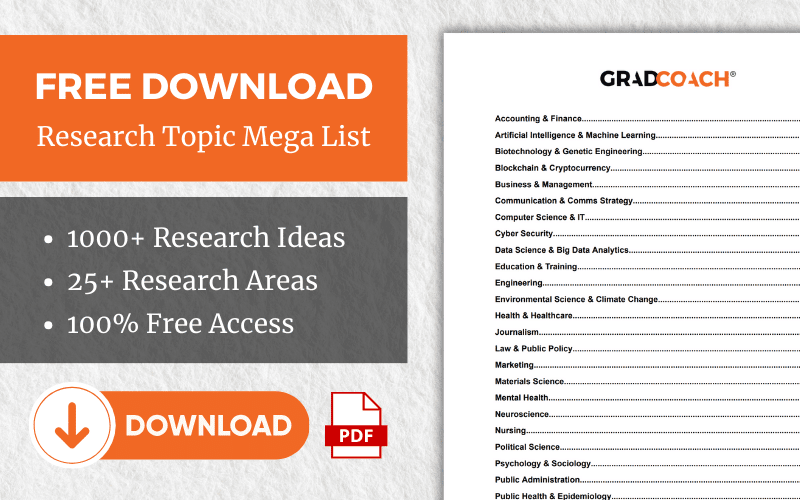
Cybersecurity-Related Research Topics
- Developing machine learning algorithms for early detection of cybersecurity threats.
- The use of artificial intelligence in optimizing network traffic for telecommunication companies.
- Investigating the impact of quantum computing on existing encryption methods.
- The application of blockchain technology in securing Internet of Things (IoT) devices.
- Developing efficient data mining techniques for large-scale social media analytics.
- The role of virtual reality in enhancing online education platforms.
- Investigating the effectiveness of various algorithms in reducing energy consumption in data centers.
- The impact of edge computing on the performance of mobile applications in remote areas.
- The application of computer vision techniques in automated medical diagnostics.
- Developing natural language processing tools for sentiment analysis in customer service.
- The use of augmented reality for training in high-risk industries like oil and gas.
- Investigating the challenges of integrating AI into legacy enterprise systems.
- The role of IT in managing supply chain disruptions during global crises.
- Developing adaptive cybersecurity strategies for small and medium-sized enterprises.
- The impact of 5G technology on the development of smart city solutions.
- The application of machine learning in personalized e-commerce recommendations.
- Investigating the use of cloud computing in improving government service delivery.
- The role of IT in enhancing sustainability in the manufacturing sector.
- Developing advanced algorithms for autonomous vehicle navigation.
- The application of biometrics in enhancing banking security systems.
- Investigating the ethical implications of facial recognition technology.
- The role of data analytics in optimizing healthcare delivery systems.
- Developing IoT solutions for efficient energy management in smart homes.
- The impact of mobile computing on the evolution of e-health services.
- The application of IT in disaster response and management.
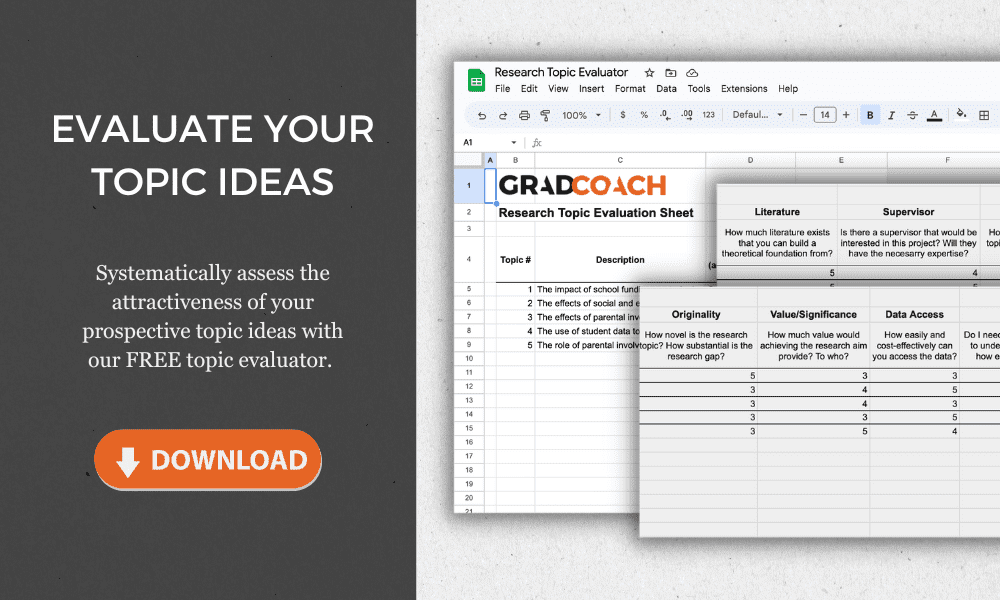
Cybersecurity Research Ideas (Continued)
- Assessing the security implications of quantum computing on modern encryption methods.
- The role of artificial intelligence in detecting and preventing phishing attacks.
- Blockchain technology in secure voting systems: opportunities and challenges.
- Cybersecurity strategies for protecting smart grids from targeted attacks.
- Developing a cyber incident response framework for small to medium-sized enterprises.
- The effectiveness of behavioural biometrics in preventing identity theft.
- Securing Internet of Things (IoT) devices in healthcare: risks and solutions.
- Analysis of cyber warfare tactics and their implications on national security.
- Exploring the ethical boundaries of offensive cybersecurity measures.
- Machine learning algorithms for predicting and mitigating DDoS attacks.
- Study of cryptocurrency-related cybercrimes: patterns and prevention strategies.
- Evaluating the impact of GDPR on data breach response strategies in the EU.
- Developing enhanced security protocols for mobile banking applications.
- An examination of cyber espionage tactics and countermeasures.
- The role of human error in cybersecurity breaches: a behavioural analysis.
- Investigating the use of deep fakes in cyber fraud: detection and prevention.
- Cloud computing security: managing risks in multi-tenant environments.
- Next-generation firewalls: evaluating performance and security features.
- The impact of 5G technology on cybersecurity strategies and policies.
- Secure coding practices: reducing vulnerabilities in software development.
- Assessing the role of cyber insurance in mitigating financial losses from cyber attacks.
- Implementing zero trust architecture in corporate networks: challenges and benefits.
- Ransomware attacks on critical infrastructure: case studies and defence strategies.
- Using big data analytics for proactive cyber threat intelligence.
- Evaluating the effectiveness of cybersecurity awareness training in organisations.
Recent Cybersecurity-Related Studies
While the ideas we’ve presented above are a decent starting point for finding a research topic, they are fairly generic and non-specific. So, it helps to look at actual studies in the cybersecurity space to see how this all comes together in practice.
Below, we’ve included a selection of recent studies to help refine your thinking. These are actual studies, so they can provide some useful insight as to what a research topic looks like in practice.
- Cyber Security Vulnerability Detection Using Natural Language Processing (Singh et al., 2022)
- Security for Cloud-Native Systems with an AI-Ops Engine (Ck et al., 2022)
- Overview of Cyber Security (Yadav, 2022)
- Exploring the Top Five Evolving Threats in Cybersecurity: An In-Depth Overview (Mijwil et al., 2023)
- Cyber Security: Strategy to Security Challenges A Review (Nistane & Sharma, 2022)
- A Review Paper on Cyber Security (K & Venkatesh, 2022)
- The Significance of Machine Learning and Deep Learning Techniques in Cybersecurity: A Comprehensive Review (Mijwil, 2023)
- Towards Artificial Intelligence-Based Cybersecurity: The Practices and ChatGPT Generated Ways to Combat Cybercrime (Mijwil et al., 2023)
- ESTABLISHING CYBERSECURITY AWARENESS OF TECHNICAL SECURITY MEASURES THROUGH A SERIOUS GAME (Harding et al., 2022)
- Efficiency Evaluation of Cyber Security Based on EBM-DEA Model (Nguyen et al., 2022)
- An Overview of the Present and Future of User Authentication (Al Kabir & Elmedany, 2022)
- Cybersecurity Enterprises Policies: A Comparative Study (Mishra et al., 2022)
- The Rise of Ransomware: A Review of Attacks, Detection Techniques, and Future Challenges (Kamil et al., 2022)
- On the scale of Cyberspace and Cybersecurity (Pathan, 2022)
- Analysis of techniques and attacking pattern in cyber security approach (Sharma et al., 2022)
- Impact of Artificial Intelligence on Information Security in Business (Alawadhi et al., 2022)
- Deployment of Artificial Intelligence with Bootstrapped Meta-Learning in Cyber Security (Sasikala & Sharma, 2022)
- Optimization of Secure Coding Practices in SDLC as Part of Cybersecurity Framework (Jakimoski et al., 2022)
- CySSS ’22: 1st International Workshop on Cybersecurity and Social Sciences (Chan-Tin & Kennison, 2022)
As you can see, these research topics are a lot more focused than the generic topic ideas we presented earlier. So, for you to develop a high-quality research topic, you’ll need to get specific and laser-focused on a specific context with specific variables of interest. In the video below, we explore some other important things you’ll need to consider when crafting your research topic.
Get 1-On-1 Help
If you’re still unsure about how to find a quality research topic, check out our Research Topic Kickstarter service, which is the perfect starting point for developing a unique, well-justified research topic.
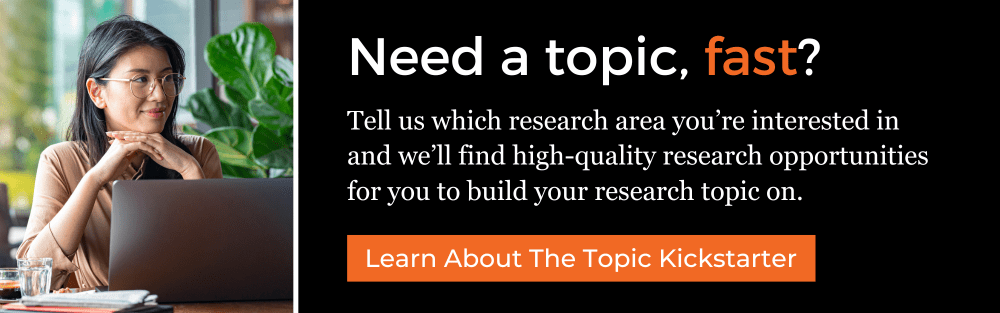
You Might Also Like:
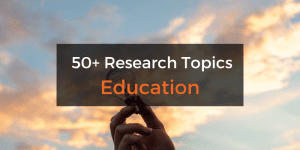
Submit a Comment Cancel reply
Your email address will not be published. Required fields are marked *
Save my name, email, and website in this browser for the next time I comment.
- Print Friendly
- Open access
- Published: 10 August 2020
Using deep learning to solve computer security challenges: a survey
- Yoon-Ho Choi 1 , 2 ,
- Peng Liu 1 ,
- Zitong Shang 1 ,
- Haizhou Wang 1 ,
- Zhilong Wang 1 ,
- Lan Zhang 1 ,
- Junwei Zhou 3 &
- Qingtian Zou 1
Cybersecurity volume 3 , Article number: 15 ( 2020 ) Cite this article
16k Accesses
20 Citations
1 Altmetric
Metrics details
Although using machine learning techniques to solve computer security challenges is not a new idea, the rapidly emerging Deep Learning technology has recently triggered a substantial amount of interests in the computer security community. This paper seeks to provide a dedicated review of the very recent research works on using Deep Learning techniques to solve computer security challenges. In particular, the review covers eight computer security problems being solved by applications of Deep Learning: security-oriented program analysis, defending return-oriented programming (ROP) attacks, achieving control-flow integrity (CFI), defending network attacks, malware classification, system-event-based anomaly detection, memory forensics, and fuzzing for software security.
Introduction
Using machine learning techniques to solve computer security challenges is not a new idea. For example, in the year of 1998, Ghosh and others in ( Ghosh et al. 1998 ) proposed to train a (traditional) neural network based anomaly detection scheme(i.e., detecting anomalous and unknown intrusions against programs); in the year of 2003, Hu and others in ( Hu et al. 2003 ) and Heller and others in ( Heller et al. 2003 ) applied Support Vector Machines to based anomaly detection scheme (e.g., detecting anomalous Windows registry accesses).
The machine-learning-based computer security research investigations during 1990-2010, however, have not been very impactful. For example, to the best of our knowledge, none of the machine learning applications proposed in ( Ghosh et al. 1998 ; Hu et al. 2003 ; Heller et al. 2003 ) has been incorporated into a widely deployed intrusion-detection commercial product.
Regarding why not very impactful, although researchers in the computer security community seem to have different opinions, the following remarks by Sommer and Paxson ( Sommer and Paxson 2010 ) (in the context of intrusion detection) have resonated with many researchers:
Remark A: “It is crucial to have a clear picture of what problem a system targets: what specifically are the attacks to be detected? The more narrowly one can define the target activity, the better one can tailor a detector to its specifics and reduce the potential for misclassifications.” ( Sommer and Paxson 2010 )
Remark B: “If one cannot make a solid argument for the relation of the features to the attacks of interest, the resulting study risks foundering on serious flaws.” ( Sommer and Paxson 2010 )
These insightful remarks, though well aligned with the machine learning techniques used by security researchers during 1990-2010, could become a less significant concern with Deep Learning (DL), a rapidly emerging machine learning technology, due to the following observations. First, Remark A implies that even if the same machine learning method is used, one algorithm employing a cost function that is based on a more specifically defined target attack activity could perform substantially better than another algorithm deploying a less specifically defined cost function. This could be a less significant concern with DL, since a few recent studies have shown that even if the target attack activity is not narrowly defined, a DL model could still achieve very high classification accuracy. Second, Remark B implies that if feature engineering is not done properly, the trained machine learning models could be plagued by serious flaws. This could be a less significant concern with DL, since many deep learning neural networks require less feature engineering than conventional machine learning techniques.
As stated in NSCAI Intern Report for Congress (2019 ), “DL is a statistical technique that exploits large quantities of data as training sets for a network with multiple hidden layers, called a deep neural network (DNN). A DNN is trained on a dataset, generating outputs, calculating errors, and adjusting its internal parameters. Then the process is repeated hundreds of thousands of times until the network achieves an acceptable level of performance. It has proven to be an effective technique for image classification, object detection, speech recognition, and natural language processing–problems that challenged researchers for decades. By learning from data, DNNs can solve some problems much more effectively, and also solve problems that were never solvable before.”
Now let’s take a high-level look at how DL could make it substantially easier to overcome the challenges identified by Sommer and Paxson ( Sommer and Paxson 2010 ). First, one major advantage of DL is that it makes learning algorithms less dependent on feature engineering. This characteristic of DL makes it easier to overcome the challenge indicated by Remark B. Second, another major advantage of DL is that it could achieve high classification accuracy with minimum domain knowledge. This characteristic of DL makes it easier to overcome the challenge indicated by Remark A.
Key observation. The above discussion indicates that DL could be a game changer in applying machine learning techniques to solving computer security challenges.
Motivated by this observation, this paper seeks to provide a dedicated review of the very recent research works on using Deep Learning techniques to solve computer security challenges. It should be noticed that since this paper aims to provide a dedicated review, non-deep-learning techniques and their security applications are out of the scope of this paper.
The remaining of the paper is organized as follows. In “ A four-phase workflow framework can summarize the existing works in a unified manner ” section, we present a four-phase workflow framework which we use to summarize the existing works in a unified manner. In “ A closer look at applications of deep learning in solving security-oriented program analysis challenges - A closer look at applications of deep learning in security-oriented fuzzing ” section, we provide a review of eight computer security problems being solved by applications of Deep Learning, respectively. In “ Discussion ” section, we will discuss certain similarity and certain dissimilarity among the existing works. In “ Further areas of investigation ” section, we mention four further areas of investigation. In “ Conclusion section, we conclude the paper.
A four-phase workflow framework can summarize the existing works in a unified manner
We found that a four-phase workflow framework can provide a unified way to summarize all the research works surveyed by us. In particular, we found that each work surveyed by us employs a particular workflow when using machine learning techniques to solve a computer security challenge, and we found that each workflow consists of two or more phases. By “a unified way”, we mean that every workflow surveyed by us is essentially an instantiation of a common workflow pattern which is shown in Fig. 1 .

Overview of the four-phase workflow
Definitions of the four phases
The four phases, shown in Fig. 1 , are defined as follows. To make the definitions of the four phases more tangible, we use a running example to illustrate each of the four phases. Phase I.(Obtaining the raw data)
In this phase, certain raw data are collected. Running Example: When Deep Learning is used to detect suspicious events in a Hadoop distributed file system (HDFS), the raw data are usually the events (e.g., a block is allocated, read, written, replicated, or deleted) that have happened to each block. Since these events are recorded in Hadoop logs, the log files hold the raw data. Since each event is uniquely identified by a particular (block ID, timestamp) tuple, we could simply view the raw data as n event sequences. Here n is the total number of blocks in the HDFS. For example, the raw data collected in Xu et al. (2009) in total consists of 11,197,954 events. Since 575,139 blocks were in the HDFS, there were 575,139 event sequences in the raw data, and on average each event sequence had 19 events. One such event sequence is shown as follows:
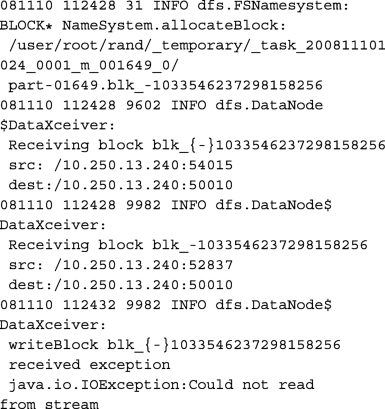
Phase II.(Data preprocessing)
Both Phase II and Phase III aim to properly extract and represent the useful information held in the raw data collected in Phase I. Both Phase II and Phase III are closely related to feature engineering. A key difference between Phase II and Phase III is that Phase III is completely dedicated to representation learning, while Phase II is focused on all the information extraction and data processing operations that are not based on representation learning. Running Example: Let’s revisit the aforementioned HDFS. Each recorded event is described by unstructured text. In Phase II, the unstructured text is parsed to a data structure that shows the event type and a list of event variables in (name, value) pairs. Since there are 29 types of events in the HDFS, each event is represented by an integer from 1 to 29 according to its type. In this way, the aforementioned example event sequence can be transformed to:
22, 5, 5, 7
Phase III.(Representation learning)
As stated in Bengio et al. (2013) , “Learning representations of the data that make it easier to extract useful information when building classifiers or other predictors.” Running Example: Let’s revisit the same HDFS. Although DeepLog ( Du et al. 2017 ) directly employed one-hot vectors to represent the event types without representation learning, if we view an event type as a word in a structured language, one may actually use the word embedding technique to represent each event type. It should be noticed that the word embedding technique is a representation learning technique.
Phase IV.(Classifier learning)
This phase aims to build specific classifiers or other predictors through Deep Learning. Running Example: Let’s revisit the same HDFS. DeepLog ( Du et al. 2017 ) used Deep Learning to build a stacked LSTM neural network for anomaly detection. For example, let’s consider event sequence {22,5,5,5,11,9,11,9,11,9,26,26,26} in which each integer represents the event type of the corresponding event in the event sequence. Given a window size h = 4, the input sample and the output label pairs to train DeepLog will be: {22,5,5,5 → 11 }, {5,5,5,11 → 9 }, {5,5,11,9 → 11 }, and so forth. In the detection stage, DeepLog examines each individual event. It determines if an event is treated as normal or abnormal according to whether the event’s type is predicted by the LSTM neural network, given the history of event types. If the event’s type is among the top g predicted types, the event is treated as normal; otherwise, it is treated as abnormal.
Using the four-phase workflow framework to summarize some representative research works
In this subsection, we use the four-phase workflow framework to summarize two representative works for each security problem. System security includes many sub research topics. However, not every research topics are suitable to adopt deep learning-based methods due to their intrinsic characteristics. For these security research subjects that can combine with deep-learning, some of them has undergone intensive research in recent years, others just emerging. We notice that there are 5 mainstream research directions in system security. This paper mainly focuses on system security, so the other mainstream research directions (e.g., deepfake) are out-of-scope. Therefore, we choose these 5 widely noticed research directions, and 3 emerging research direction in our survey:
In security-oriented program analysis, malware classification (MC), system-event-based anomaly detection (SEAD), memory forensics (MF), and defending network attacks, deep learning based methods have already undergone intensive research.
In defending return-oriented programming (ROP) attacks, Control-flow integrity (CFI), and fuzzing, deep learning based methods are emerging research topics.
We select two representative works for each research topic in our survey. Our criteria to select papers mainly include: 1) Pioneer (one of the first papers in this field); 2) Top (published on top conference or journal); 3) Novelty; 4) Citation (The citation of this paper is high); 5) Effectiveness (the result of this paper is pretty good); 6) Representative (the paper is a representative work for a branch of the research direction). Table 1 lists the reasons why we choose each paper, which is ordered according to their importance.
The summary is shown in Table 2 . There are three columns in the table. In the first column, we listed eight security problems, including security-oriented program analysis, defending return-oriented programming (ROP) attacks, control-flow integrity (CFI), defending network attacks (NA), malware classification (MC), system-event-based anomaly detection (SEAD), memory forensics (MF), and fuzzing for software security. In the second column, we list the very recent two representative works for each security problem. In the “Summary” column, we sequentially describe how the four phases are deployed at each work, then, we list the evaluation results for each work in terms of accuracy (ACC), precision (PRC), recall (REC), F1 score (F1), false-positive rate (FPR), and false-negative rate (FNR), respectively.
Methodology for reviewing the existing works
Data representation (or feature engineering) plays an important role in solving security problems with Deep Learning. This is because data representation is a way to take advantage of human ingenuity and prior knowledge to extract and organize the discriminative information from the data. Many efforts in deploying machine learning algorithms in security domain actually goes into the design of preprocessing pipelines and data transformations that result in a representation of the data to support effective machine learning.
In order to expand the scope and ease of applicability of machine learning in security domain, it would be highly desirable to find a proper way to represent the data in security domain, which can entangle and hide more or less the different explanatory factors of variation behind the data. To let this survey adequately reflect the important role played by data representation, our review will focus on how the following three questions are answered by the existing works:
Question 1: Is Phase II pervasively done in the literature? When Phase II is skipped in a work, are there any particular reasons?
Question 2: Is Phase III employed in the literature? When Phase III is skipped in a work, are there any particular reasons?
Question 3: When solving different security problems, is there any commonality in terms of the (types of) classifiers learned in Phase IV? Among the works solving the same security problem, is there dissimilarity in terms of classifiers learned in Phase IV?
To group the Phase III methods at different applications of Deep Learning in solving the same security problem, we introduce a classification tree as shown in Fig. 2 . The classification tree categorizes the Phase III methods in our selected survey works into four classes. First, class 1 includes the Phase III methods which do not consider representation learning. Second, class 2 includes the Phase III methods which consider representation learning but, do not adopt it. Third, class 3 includes the Phase III methods which consider and adopt representation learning but, do not compare the performance with other methods. Finally, class 4 includes the Phase III methods which consider and adopt representation learning and, compare the performance with other methods.

Classification tree for different Phase III methods. Here, consideration , adoption , and comparison indicate that a work considers Phase III, adopts Phase III and makes comparison with other methods, respectively
In the remaining of this paper, we take a closer look at how each of the eight security problems is being solved by applications of Deep Learning in the literature.
A closer look at applications of deep learning in solving security-oriented program analysis challenges
Recent years, security-oriented program analysis is widely used in software security. For example, symbolic execution and taint analysis are used to discover, detect and analyze vulnerabilities in programs. Control flow analysis, data flow analysis and pointer/alias analysis are important components when enforcing many secure strategies, such as control flow integrity, data flow integrity and doling dangling pointer elimination. Reverse engineering was used by defenders and attackers to understand the logic of a program without source code.
In the security-oriented program analysis, there are many open problems, such as precise pointer/alias analysis, accurate and complete reversing engineer, complex constraint solving, program de-obfuscation, and so on. Some problems have theoretically proven to be NP-hard, and others still need lots of human effort to solve. Either of them needs a lot of domain knowledge and experience from expert to develop better solutions. Essentially speaking, the main challenges when solving them through traditional approaches are due to the sophisticated rules between the features and labels, which may change in different contexts. Therefore, on the one hand, it will take a large quantity of human effort to develop rules to solve the problems, on the other hand, even the most experienced expert cannot guarantee completeness. Fortunately, the deep learning method is skillful to find relations between features and labels if given a large amount of training data. It can quickly and comprehensively find all the relations if the training samples are representative and effectively encoded.
In this section, we will review the very recent four representative works that use Deep Learning for security-oriented program analysis. We observed that they focused on different goals. Shin, et al. designed a model ( Shin et al. 2015 ) to identify the function boundary. EKLAVYA ( Chua et al. 2017 ) was developed to learn the function type. Gemini ( Xu et al. 2017 ) was proposed to detect similarity among functions. DEEPVSA ( Guo et al. 2019 ) was designed to learn memory region of an indirect addressing from the code sequence. Among these works, we select two representative works ( Shin et al. 2015 ; Chua et al. 2017 ) and then, summarize the analysis results in Table 2 in detail.
Our review will be centered around three questions described in “ Methodology for reviewing the existing works ” section. In the remaining of this section, we will first provide a set of observations, and then we provide the indications. Finally, we provide some general remarks.
Key findings from a closer look
From a close look at the very recent applications using Deep Learning for solving security-oriented program analysis challenges, we observed the followings:
Observation 3.1: All of the works in our survey used binary files as their raw data. Phase II in our survey had one similar and straightforward goal – extracting code sequences from the binary. Difference among them was that the code sequence was extracted directly from the binary file when solving problems in static program analysis, while it was extracted from the program execution when solving problems in dynamic program analysis.
*Observation 3.2: Most data representation methods generally took into account the domain knowledge.
Most data representation methods generally took into the domain knowledge, i.e., what kind of information they wanted to reserve when processing their data. Note that the feature selection has a wide influence on Phase II and Phase III, for example, embedding granularities, representation learning methods. Gemini ( Xu et al. 2017 ) selected function level feature and other works in our survey selected instruction level feature. To be specifically, all the works except Gemini ( Xu et al. 2017 ) vectorized code sequence on instruction level.
Observation 3.3: To better support data representation for high performance, some works adopted representation learning.
For instance, DEEPVSA ( Guo et al. 2019 ) employed a representation learning method, i.e., bi-directional LSTM, to learn data dependency within instructions. EKLAVYA ( Chua et al. 2017 ) adopted representation learning method, i.e., word2vec technique, to extract inter-instruciton information. It is worth noting that Gemini ( Xu et al. 2017 ) adopts the Structure2vec embedding network in its siamese architecture in Phase IV (see details in Observation 3.7). The Structure2vec embedding network learned information from an attributed control flow graph.
Observation 3.4: According to our taxonomy, most works in our survey were classified into class 4.
To compare the Phase III, we introduced a classification tree with three layers as shown in Fig. 2 to group different works into four categories. The decision tree grouped our surveyed works into four classes according to whether they considered representation learning or not, whether they adopted representation learning or not, and whether they compared their methods with others’, respectively, when designing their framework. According to our taxonomy, EKLAVYA ( Chua et al. 2017 ), DEEPVSA ( Guo et al. 2019 ) were grouped into class 4 shown in Fig. 2 . Also, Gemini’s work ( Xu et al. 2017 ) and Shin, et al.’s work ( Shin et al. 2015 ) belonged to class 1 and class 2 shown in Fig. 2 , respectively.
Observation 3.5: All the works in our survey explain why they adopted or did not adopt one of representation learning algorithms.
Two works in our survey adopted representation learning for different reasons: to enhance model’s ability of generalization ( Chua et al. 2017 ); and to learn the dependency within instructions ( Guo et al. 2019 ). It is worth noting that Shin, et al. did not adopt representation learning because they wanted to preserve the “attractive” features of neural networks over other machine learning methods – simplicity. As they stated, “first, neural networks can learn directly from the original representation with minimal preprocessing (or “feature engineering”) needed.” and “second, neural networks can learn end-to-end, where each of its constituent stages are trained simultaneously in order to best solve the end goal.” Although Gemini ( Xu et al. 2017 ) did not adopt representation learning when processing their raw data, the Deep Learning models in siamese structure consisted of two graph embedding networks and one cosine function.
*Observation 3.6: The analysis results showed that a suitable representation learning method could improve accuracy of Deep Learning models.
DEEPVSA ( Guo et al. 2019 ) designed a series of experiments to evaluate the effectiveness of its representative method. By combining with the domain knowledge, EKLAVYA ( Chua et al. 2017 ) employed t-SNE plots and analogical reasoning to explain the effectiveness of their representation learning method in an intuitive way.
Observation 3.7: Various Phase IV methods were used.
In Phase IV, Gemini ( Xu et al. 2017 ) adopted siamese architecture model which consisted of two Structure2vec embedding networks and one cosine function. The siamese architecture took two functions as its input, and produced the similarity score as the output. The other three works ( Shin et al. 2015 ; Chua et al. 2017 ; Guo et al. 2019 ) adopted bi-directional RNN, RNN, bi-directional LSTM respectively. Shin, et al. adopted bi-directional RNN because they wanted to combine both the past and the future information in making a prediction for the present instruction ( Shin et al. 2015 ). DEEPVSA ( Guo et al. 2019 ) adopted bi-directional RNN to enable their model to infer memory regions in both forward and backward ways.
The above observations seem to indicate the following indications:
Indication 3.1: Phase III is not always necessary.
Not all authors regard representation learning as a good choice even though some case experiments show that representation learning can improve the final results. They value more the simplicity of Deep Learning methods and suppose that the adoption of representation learning weakens the simplicity of Deep Learning methods.
Indication 3.2: Even though the ultimate objective of Phase III in the four surveyed works is to train a model with better accuracy, they have different specific motivations as described in Observation 3.5.
When authors choose representation learning, they usually try to convince people the effectiveness of their choice by empirical or theoretical analysis.
*Indication 3.3: Observation 3.7 indicates that authors usually refer to the domain knowledge when designing the architecture of Deep Learning model.
For instance, the works we reviewed commonly adopt bi-directional RNN when their prediction partly based on future information in data sequence.
Despite the effectiveness and agility of deep learning-based methods, there are still some challenges in developing a scheme with high accuracy due to the hierarchical data structure, lots of noisy, and unbalanced data composition in program analysis. For instance, an instruction sequence, a typical data sample in program analysis, contains three-level hierarchy: sequence–instruction–opcode/operand. To make things worse, each level may contain many different structures, e.g., one-operand instructions, multi-operand instructions, which makes it harder to encode the training data.
A closer look at applications of deep learning in defending ROP attacks
Return-oriented programming (ROP) attack is one of the most dangerous code reuse attacks, which allows the attackers to launch control-flow hijacking attack without injecting any malicious code. Rather, It leverages particular instruction sequences (called “gadgets”) widely existing in the program space to achieve Turing-complete attacks ( Shacham and et al. 2007 ). Gadgets are instruction sequences that end with a RET instruction. Therefore, they can be chained together by specifying the return addresses on program stack. Many traditional techniques could be used to detect ROP attacks, such as control-flow integrity (CFI Abadi et al. (2009) ), but many of them either have low detection rate or have high runtime overhead. ROP payloads do not contain any codes. In other words, analyzing ROP payload without the context of the program’s memory dump is meaningless. Thus, the most popular way of detecting and preventing ROP attacks is control-flow integrity. The challenge after acquiring the instruction sequences is that it is hard to recognize whether the control flow is normal. Traditional methods use the control flow graph (CFG) to identify whether the control flow is normal, but attackers can design the instruction sequences which follow the normal control flow defined by the CFG. In essence, it is very hard to design a CFG to exclude every single possible combination of instructions that can be used to launch ROP attacks. Therefore, using data-driven methods could help eliminate such problems.
In this section, we will review the very recent three representative works that use Deep Learning for defending ROP attacks: ROPNN ( Li et al. 2018 ), HeNet ( Chen et al. 2018 ) and DeepCheck ( Zhang et al. 2019 ). ROPNN ( Li et al. 2018 ) aims to detect ROP attacks, HeNet ( Chen et al. 2018 ) aims to detect malware using CFI, and DeepCheck ( Zhang et al. 2019 ) aims at detecting all kinds of code reuse attacks.
Specifically, ROPNN is to protect one single program at a time, and its training data are generated from real-world programs along with their execution. Firstly, it generates its benign and malicious data by “chaining-up” the normally executed instruction sequences and “chaining-up” gadgets with the help of gadgets generation tool, respectively, after the memory dumps of programs are created. Each data sample is byte-level instruction sequence labeled as “benign” or “malicious”. Secondly, ROPNN will be trained using both malicious and benign data. Thirdly, the trained model is deployed to a target machine. After the protected program started, the executed instruction sequences will be traced and fed into the trained model, the protected program will be terminated once the model found the instruction sequences are likely to be malicious.
HeNet is also proposed to protect a single program. Its malicious data and benign data are generated by collecting trace data through Intel PT from malware and normal software, respectively. Besides, HeNet preprocesses its dataset and shape each data sample in the format of image, so that they could implement transfer learning from a model pre-trained on ImageNet. Then, HeNet is trained and deployed on machines with features of Intel PT to collect and classify the program’s execution trace online.
The training data for DeepCheck are acquired from CFGs, which are constructed by dissembling the programs and using the information from Intel PT. After the CFG for a protected program is constructed, authors sample benign instruction sequences by chaining up basic blocks that are connected by edges, and sample malicious instruction sequences by chaining up those that are not connected by edges. Although a CFG is needed during training, there is no need to construct CFG after the training phase. After deployed, instruction sequences will be constructed by leveraging Intel PT on the protected program. Then the trained model will classify whether the instruction sequences are malicious or benign.
We observed that none of the works considered Phase III, so all of them belong to class 1 according to our taxonomy as shown in Fig. 2 . The analysis results of ROPNN ( Li et al. 2018 ) and HeNet ( Chen et al. 2018 ) are shown in Table 2 . Also, we observed that three works had different goals.
From a close look at the very recent applications using Deep Learning for defending return-oriented programming attacks, we observed the followings:
Observation 4.1: All the works ( Li et al. 2018 ; Zhang et al. 2019 ; Chen et al. 2018 ) in this survey focused on data generation and acquisition.
In ROPNN ( Li et al. 2018 ), both malicious samples (gadget chains) were generated using an automated gadget generator (i.e. ROPGadget ( Salwant 2015 )) and a CPU emulator (i.e. Unicorn ( Unicorn-The ultimate CPU emulator 2015 )). ROPGadget was used to extract instruction sequences that could be used as gadgets from a program, and Unicorn was used to validate the instruction sequences. Corresponding benign sample (gadget-chain-like instruction sequences) were generated by disassembling a set of programs. In DeepCheck ( Zhang et al. 2019 ) refers to the key idea of control-flow integrity ( Abadi et al. 2009 ). It generates program’s run-time control flow through new feature of Intel CPU (Intel Processor Tracing), then compares the run-time control flow with the program’s control-flow graph (CFG) that generates through static analysis. Benign instruction sequences are that with in the program’s CFG, and vice versa. In HeNet ( Chen et al. 2018 ), program’s execution trace was extracted using the similar way as DeepCheck. Then, each byte was transformed into a pixel with an intensity between 0-255. Known malware samples and benign software samples were used to generate malicious data benign data, respectively.
Observation 4.2: None of the ROP works in this survey deployed Phase III.
Both ROPNN ( Li et al. 2018 ) and DeepCheck ( Zhang et al. 2019 ) used binary instruction sequences for training. In ROPNN ( Li et al. 2018 ), one byte was used as the very basic element for data pre-processing. Bytes were formed into one-hot matrices and flattened for 1-dimensional convolutional layer. In DeepCheck ( Zhang et al. 2019 ), half-byte was used as the basic unit. Each half-byte (4 bits) was transformed to decimal form ranging from 0-15 as the basic element of the input vector, then was fed into a fully-connected input layer. On the other hand, HeNet ( Chen et al. 2018 ) used different kinds of data. By the time this survey has been drafted, the source code of HeNet was not available to public and thus, the details of the data pre-processing was not be investigated. However, it is still clear that HeNet used binary branch information collected from Intel PT rather than binary instructions. In HeNet, each byte was converted to one decimal number ranging from 0 to 255. Byte sequences was sliced and formed into image sequences (each pixel represented one byte) for a fully-connected input layer.
Observation 4.3: Fully-connected neural network was widely used.
Only ROPNN ( Li et al. 2018 ) used 1-dimensional convolutional neural network (CNN) when extracting features. Both HeNet ( Chen et al. 2018 ) and DeepCheck ( Zhang et al. 2019 ) used fully-connected neural network (FCN). None of the works used recurrent neural network (RNN) and the variants.
Indication 4.1: It seems like that one of the most important factors in ROP problem is feature selection and data generation.
All three works use very different methods to collect/generate data, and all the authors provide very strong evidences and/or arguments to justify their approaches. ROPNN ( Li et al. 2018 ) was trained by the malicious and benign instruction sequences. However, there is no clear boundary between benign instruction sequences and malicious gadget chains. This weakness may impair the performance when applying ROPNN to real world ROP attacks. As oppose to ROPNN, DeepCheck ( Zhang et al. 2019 ) utilizes CFG to generate training basic-block sequences. However, since the malicious basic-block sequences are generated by randomly connecting nodes without edges, it is not guaranteed that all the malicious basic-blocks are executable. HeNet ( Chen et al. 2018 ) generates their training data from malware. Technically, HeNet could be used to detect any binary exploits, but their experiment focuses on ROP attack and achieves 100% accuracy. This shows that the source of data in ROP problem does not need to be related to ROP attacks to produce very impressive results.
Indication 4.2: Representation learning seems not critical when solving ROP problems using Deep Learning.
Minimal process on data in binary form seems to be enough to transform the data into a representation that is suitable for neural networks. Certainly, it is also possible to represent the binary instructions at a higher level, such as opcodes, or use embedding learning. However, as stated in ( Li et al. 2018 ), it appears that the performance will not change much by doing so. The only benefit of representing input data to a higher level is to reduce irrelevant information, but it seems like neural network by itself is good enough at extracting features.
Indication 4.3: Different Neural network architecture does not have much influence on the effectiveness of defending ROP attacks.
Both HeNet ( Chen et al. 2018 ) and DeepCheck ( Zhang et al. 2019 ) utilizes standard DNN and achieved comparable results on ROP problems. One can infer that the input data can be easily processed by neural networks, and the features can be easily detected after proper pre-process.
It is not surprising that researchers are not very interested in representation learning for ROP problems as stated in Observation 4.1. Since ROP attack is focus on the gadget chains, it is straightforward for the researcher to choose the gadgets as their training data directly. It is easy to map the data into numerical representation with minimal processing. An example is that one can map binary executable to hexadecimal ASCII representation, which could be a good representation for neural network.
Instead, researchers focus more in data acquisition and generation. In ROP problems, the amount of data is very limited. Unlike malware and logs, ROP payloads normally only contain addresses rather than codes, which do not contain any information without providing the instructions in corresponding addresses. It is thus meaningless to collect all the payloads. At the best of our knowledge, all the previous works use pick instruction sequences rather than payloads as their training data, even though they are hard to collect.
Even though, Deep Learning based method does not face the challenge to design a very complex fine-grained CFG anymore, it suffers from a limited number of data sources. Generally, Deep Learning based method requires lots of training data. However, real-world malicious data for the ROP attack is very hard to find, because comparing with benign data, malicious data need to be carefully crafted and there is no existing database to collect all the ROP attacks. Without enough representative training set, the accuracy of the trained model cannot be guaranteed.
A closer look at applications of deep learning in achieving CFI
The basic ideas of control-flow integrity (CFI) techniques, proposed by Abadi in 2005 ( Abadi et al. 2009 ), could be dated back to 2002, when Vladimir and his fellow researchers proposed an idea called program shepherding ( Kiriansky et al. 2002 ), a method of monitoring the execution flow of a program when it is running by enforcing some security policies. The goal of CFI is to detect and prevent control-flow hijacking attacks, by restricting every critical control flow transfers to a set that can only appear in correct program executions, according to a pre-built CFG. Traditional CFI techniques typically leverage some knowledge, gained from either dynamic or static analysis of the target program, combined with some code instrumentation methods, to ensure the program runs on a correct track.
However, the problems of traditional CFI are: (1) Existing CFI implementations are not compatible with some of important code features ( Xu et al. 2019 ); (2) CFGs generated by static, dynamic or combined analysis cannot always be precisely completed due to some open problems ( Horwitz 1997 ); (3) There always exist certain level of compromises between accuracy and performance overhead and other important properties ( Tan and Jaeger 2017 ; Wang and Liu 2019 ). Recent research has proposed to apply Deep Learning on detecting control flow violation. Their result shows that, compared with traditional CFI implementation, the security coverage and scalability were enhanced in such a fashion ( Yagemann et al. 2019 ). Therefore, we argue that Deep Learning could be another approach which requires more attention from CFI researchers who aim at achieving control-flow integrity more efficiently and accurately.
In this section, we will review the very recent three representative papers that use Deep Learning for achieving CFI. Among the three, two representative papers ( Yagemann et al. 2019 ; Phan et al. 2017 ) are already summarized phase-by-phase in Table 2 . We refer to interested readers the Table 2 for a concise overview of those two papers.
Our review will be centered around three questions described in Section 3 . In the remaining of this section, we will first provide a set of observations, and then we provide the indications. Finally, we provide some general remarks.
From a close look at the very recent applications using Deep Learning for achieving control-flow integrity, we observed the followings:
Observation 5.1: None of the related works realize preventive Footnote 1 prevention of control flow violation.
After doing a thorough literature search, we observed that security researchers are quite behind the trend of applying Deep Learning techniques to solve security problems. Only one paper has been founded by us, using Deep Learning techniques to directly enhance the performance of CFI ( Yagemann et al. 2019 ). This paper leveraged Deep Learning to detect document malware through checking program’s execution traces that generated by hardware. Specifically, the CFI violations were checked in an offline mode. So far, no works have realized Just-In-Time checking for program’s control flow.
In order to provide more insightful results, in this section, we try not to narrow down our focus on CFI detecting attacks at run-time, but to extend our scope to papers that take good use of control flow related data, combined with Deep Learning techniques ( Phan et al. 2017 ; Nguyen et al. 2018 ). In one work, researchers used self-constructed instruction-level CFG to detect program defection ( Phan et al. 2017 ). In another work, researchers used lazy-binding CFG to detect sophisticated malware ( Nguyen et al. 2018 ).
Observation 5.2: Diverse raw data were used for evaluating CFI solutions.
In all surveyed papers, there are two kinds of control flow related data being used: program instruction sequences and CFGs. Barnum et al. ( Yagemann et al. 2019 ) employed statically and dynamically generated instruction sequences acquired by program disassembling and Intel ® Processor Trace. CNNoverCFG ( Phan et al. 2017 ) used self-designed algorithm to construct instruction level control-flow graph. Minh Hai Nguyen et al. ( Nguyen et al. 2018 ) used proposed lazy-binding CFG to reflect the behavior of malware DEC.
Observation 5.3: All the papers in our survey adopted Phase II.
All the related papers in our survey employed Phase II to process their raw data before sending them into Phase III. In Barnum ( Yagemann et al. 2019 ), the instruction sequences from program run-time tracing were sliced into basic-blocks. Then, they assigned each basic-blocks with an unique basic-block ID (BBID). Finally, due to the nature of control-flow hijacking attack, they selected the sequences ending with indirect branch instruction (e.g., indirect call/jump, return and so on) as the training data. In CNNoverCFG ( Phan et al. 2017 ), each of instructions in CFG were labeled with its attributes in multiple perspectives, such as opcode, operands, and the function it belongs to. The training data is generated are sequences generated by traversing the attributed control-flow graph. Nguyen and others ( Nguyen et al. 2018 ) converted the lazy-binding CFG to corresponding adjacent matrix and treated the matrix as a image as their training data.
Observation 5.4: All the papers in our survey did not adopt Phase III. We observed all the papers we surveyed did not adopted Phase III. Instead, they adopted the form of numerical representation directly as their training data. Specifically, Barnum ( Yagemann et al. 2019 ) grouped the instructions into basic-blocks, then represented basic-blocks with uniquely assigning IDs. In CNNoverCFG ( Phan et al. 2017 ), each of instructions in the CFG was represented by a vector that associated with its attributes. Nguyen and others directly used the hashed value of bit string representation.
Observation 5.5: Various Phase IV models were used. Barnum ( Yagemann et al. 2019 ) utilized BBID sequence to monitor the execution flow of the target program, which is sequence-type data. Therefore, they chose LSTM architecture to better learn the relationship between instructions. While in the other two papers ( Phan et al. 2017 ; Nguyen et al. 2018 ), they trained CNN and directed graph-based CNN to extract information from control-flow graph and image, respectively.
Indication 5.1: All the existing works did not achieve Just-In-Time CFI violation detection.
It is still a challenge to tightly embed Deep Learning model in program execution. All existing work adopted lazy-checking – checking the program’s execution trace following its execution.
Indication 5.2: There is no unified opinion on how to generate malicious sample.
Data are hard to collect in control-flow hijacking attacks. The researchers must carefully craft malicious sample. It is not clear whether the “handcrafted” sample can reflect the nature the control-flow hijacking attack.
*Observation 5.3: The choice of methods in Phase II are based on researchers’ security domain knowledge.
The strength of using deep learning to solve CFI problems is that it can avoid the complicated processes of developing algorithms to build acceptable CFGs for the protected programs. Compared with the traditional approaches, the DL based method could prevent CFI designer from studying the language features of the targeted program and could also avoid the open problem (pointer analysis) in control flow analysis. Therefore, DL based CFI provides us a more generalized, scalable, and secure solution. However, since using DL in CFI problem is still at an early age, which kinds of control-flow related data are more effective is still unclear yet in this research area. Additionally, applying DL in real-time control-flow violation detection remains an untouched area and needs further research.
A closer look at applications of deep learning in defending network attacks
Network security is becoming more and more important as we depend more and more on networks for our daily lives, works and researches. Some common network attack types include probe, denial of service (DoS), Remote-to-local (R2L), etc. Traditionally, people try to detect those attacks using signatures, rules, and unsupervised anomaly detection algorithms. However, signature based methods can be easily fooled by slightly changing the attack payload; rule based methods need experts to regularly update rules; and unsupervised anomaly detection algorithms tend to raise lots of false positives. Recently, people are trying to apply Deep Learning methods for network attack detection.
In this section, we will review the very recent seven representative works that use Deep Learning for defending network attacks. Millar et al. (2018 ); Varenne et al. (2019 ); Ustebay et al. (2019 ) build neural networks for multi-class classification, whose class labels include one benign label and multiple malicious labels for different attack types. Zhang et al. (2019 ) ignores normal network activities and proposes parallel cross convolutional neural network (PCCN) to classify the type of malicious network activities. Yuan et al. (2017 ) applies Deep Learning to detecting a specific attack type, distributed denial of service (DDoS) attack. Yin et al. (2017 ); Faker and Dogdu (2019 ) explores both binary classification and multi-class classification for benign and malicious activities. Among these seven works, we select two representative works ( Millar et al. 2018 ; Zhang et al. 2019 ) and summarize the main aspects of their approaches regarding whether the four phases exist in their works, and what exactly do they do in the Phase if it exists. We direct interested readers to Table 2 for a concise overview of these two works.
From a close look at the very recent applications using Deep Learning for solving network attack challenges, we observed the followings:
Observation 6.1: All the seven works in our survey used public datasets, such as UNSW-NB15 ( Moustafa and Slay 2015 ) and CICIDS2017 ( IDS 2017 Datasets 2019 ).
The public datasets were all generated in test-bed environments, with unbalanced simulated benign and attack activities. For attack activities, the dataset providers launched multiple types of attacks, and the numbers of malicious data for those attack activities were also unbalanced.
Observation 6.2: The public datasets were given into one of two data formats, i.e., PCAP and CSV.
One was raw PCAP or parsed CSV format, containing network packet level features, and the other was also CSV format, containing network flow level features, which showed the statistic information of many network packets. Out of all the seven works, ( Yuan et al. 2017 ; Varenne et al. 2019 ) used packet information as raw inputs, ( Yin et al. 2017 ; Zhang et al. 2019 ; Ustebay et al. 2019 ; Faker and Dogdu 2019 ) used flow information as raw inputs, and ( Millar et al. 2018 ) explored both cases.
Observation 6.3: In order to parse the raw inputs, preprocessing methods, including one-hot vectors for categorical texts, normalization on numeric data, and removal of unused features/data samples, were commonly used.
Commonly removed features include IP addresses and timestamps. Faker and Dogdu (2019 ) also removed port numbers from used features. By doing this, they claimed that they could “avoid over-fitting and let the neural network learn characteristics of packets themselves”. One outlier was that, when using packet level features in one experiment, ( Millar et al. 2018 ) blindly chose the first 50 bytes of each network packet without any feature extracting processes and fed them into neural network.
Observation 6.4: Using image representation improved the performance of security solutions using Deep Learning.
After preprocessing the raw data, while ( Zhang et al. 2019 ) transformed the data into image representation, ( Yuan et al. 2017 ; Varenne et al. 2019 ; Faker and Dogdu 2019 ; Ustebay et al. 2019 ; Yin et al. 2017 ) directly used the original vectors as an input data. Also, ( Millar et al. 2018 ) explored both cases and reported better performance using image representation.
Observation 6.5: None of all the seven surveyed works considered representation learning.
All the seven surveyed works belonged to class 1 shown in Fig. 2 . They either directly used the processed vectors to feed into the neural networks, or changed the representation without explanation. One research work ( Millar et al. 2018 ) provided a comparison on two different representations (vectors and images) for the same type of raw input. However, the other works applied different preprocessing methods in Phase II. That is, since the different preprocessing methods generated different feature spaces, it was difficult to compare the experimental results.
Observation 6.6: Binary classification model showed better results from most experiments.
Among all the seven surveyed works, ( Yuan et al. 2017 ) focused on one specific attack type and only did binary classification to classify whether the network traffic was benign or malicious. Also, ( Millar et al. 2018 ; Ustebay et al. 2019 ; Zhang et al. 2019 ; Varenne et al. 2019 ) included more attack types and did multi-class classification to classify the type of malicious activities, and ( Yin et al. 2017 ; Faker and Dogdu 2019 ) explored both cases. As for multi-class classification, the accuracy for selective classes was good, while accuracy for other classes, usually classes with much fewer data samples, suffered by up to 20% degradation.
Observation 6.7: Data representation influenced on choosing a neural network model.
Indication 6.1: All works in our survey adopt a kind of preprocessing methods in Phase II, because raw data provided in the public datasets are either not ready for neural networks, or that the quality of data is too low to be directly used as data samples.
Preprocessing methods can help increase the neural network performance by improving the data samples’ qualities. Furthermore, by reducing the feature space, pre-processing can also improve the efficiency of neural network training and testing. Thus, Phase II should not be skipped. If Phase II is skipped, the performance of neural network is expected to go down considerably.
Indication 6.2: Although Phase III is not employed in any of the seven surveyed works, none of them explains a reason for it. Also, they all do not take representation learning into consideration.
Indication 6.3: Because no work uses representation learning, the effectiveness are not well-studied.
Out of other factors, it seems that the choice of pre-processing methods has the largest impact, because it directly affects the data samples fed to the neural network.
Indication 6.4: There is no guarantee that CNN also works well on images converted from network features.
Some works that use image data representation use CNN in Phase IV. Although CNN has been proven to work well on image classification problem in the recent years, there is no guarantee that CNN also works well on images converted from network features.
From the observations and indications above, we hereby present two recommendations: (1) Researchers can try to generate their own datasets for the specific network attack they want to detect. As stated, the public datasets have highly unbalanced number of data for different classes. Doubtlessly, such unbalance is the nature of real world network environment, in which normal activities are the majority, but it is not good for Deep Learning. ( Varenne et al. 2019 ) tries to solve this problem by oversampling the malicious data, but it is better to start with a balanced data set. (2) Representation learning should be taken into consideration. Some possible ways to apply representation learning include: (a) apply word2vec method to packet binaries, and categorical numbers and texts; (b) use K-means as one-hot vector representation instead of randomly encoding texts. We suggest that any change of data representation may be better justified by explanations or comparison experiments.
One critical challenge in this field is the lack of high-quality data set suitable for applying deep learning. Also, there is no agreement on how to apply domain knowledge into training deep learning models for network security problems. Researchers have been using different pre-processing methods, data representations and model types, but few of them have enough explanation on why such methods/representations/models are chosen, especially for data representation.
A closer look at applications of deep learning in malware classification
The goal of malware classification is to identify malicious behaviors in software with static and dynamic features like control-flow graph and system API calls. Malware and benign programs can be collected from open datasets and online websites. Both the industry and the academic communities have provided approaches to detect malware with static and dynamic analyses. Traditional methods such as behavior-based signatures, dynamic taint tracking, and static data flow analysis require experts to manually investigate unknown files. However, those hand-crafted signatures are not sufficiently effective because attackers can rewrite and reorder the malware. Fortunately, neural networks can automatically detect large-scale malware variants with superior classification accuracy.
In this section, we will review the very recent twelve representative works that use Deep Learning for malware classification ( De La Rosa et al. 2018 ; Saxe and Berlin 2015 ; Kolosnjaji et al. 2017 ; McLaughlin et al. 2017 ; Tobiyama et al. 2016 ; Dahl et al. 2013 ; Nix and Zhang 2017 ; Kalash et al. 2018 ; Cui et al. 2018 ; David and Netanyahu 2015 ; Rosenberg et al. 2018 ; Xu et al. 2018 ). De La Rosa et al. (2018 ) selects three different kinds of static features to classify malware. Saxe and Berlin (2015 ); Kolosnjaji et al. (2017 ); McLaughlin et al. (2017 ) also use static features from the PE files to classify programs. ( Tobiyama et al. 2016 ) extracts behavioral feature images using RNN to represent the behaviors of original programs. ( Dahl et al. 2013 ) transforms malicious behaviors using representative learning without neural network. Nix and Zhang (2017 ) explores RNN model with the API calls sequences as programs’ features. Cui et al. (2018 ); Kalash et al. (2018 ) skip Phase II by directly transforming the binary file to image to classify the file. ( David and Netanyahu 2015 ; Rosenberg et al. 2018 ) applies dynamic features to analyze malicious features. Xu et al. (2018 ) combines static features and dynamic features to represent programs’ features. Among these works, we select two representative works ( De La Rosa et al. 2018 ; Rosenberg et al. 2018 ) and identify four phases in their works shown as Table 2 .
From a close look at the very recent applications using Deep Learning for solving malware classification challenges, we observed the followings:
Observation 7.1: Features selected in malware classification were grouped into three categories: static features, dynamic features, and hybrid features.
Typical static features include metadata, PE import Features, Byte/Entorpy, String, and Assembly Opcode Features derived from the PE files ( Kolosnjaji et al. 2017 ; McLaughlin et al. 2017 ; Saxe and Berlin 2015 ). De La Rosa et al. (2018 ) took three kinds of static features: byte-level, basic-level (strings in the file, the metadata table, and the import table of the PE header), and assembly features-level. Some works directly considered binary code as static features ( Cui et al. 2018 ; Kalash et al. 2018 ).
Different from static features, dynamic features were extracted by executing the files to retrieve their behaviors during execution. The behaviors of programs, including the API function calls, their parameters, files created or deleted, websites and ports accessed, etc, were recorded by a sandbox as dynamic features ( David and Netanyahu 2015 ). The process behaviors including operation name and their result codes were extracted ( Tobiyama et al. 2016 ). The process memory, tri-grams of system API calls and one corresponding input parameter were chosen as dynamic features ( Dahl et al. 2013 ). An API calls sequence for an APK file was another representation of dynamic features ( Nix and Zhang 2017 ; Rosenberg et al. 2018 ).
Static features and dynamic features were combined as hybrid features ( Xu et al. 2018 ). For static features, Xu and others in ( Xu et al. 2018 ) used permissions, networks, calls, and providers, etc. For dynamic features, they used system call sequences.
Observation 7.2: In most works, Phase II was inevitable because extracted features needed to be vertorized for Deep Learning models.
One-hot encoding approach was frequently used to vectorize features ( Kolosnjaji et al. 2017 ; McLaughlin et al. 2017 ; Rosenberg et al. 2018 ; Tobiyama et al. 2016 ; Nix and Zhang 2017 ). Bag-of-words (BoW) and n -gram were also considered to represent features ( Nix and Zhang 2017 ). Some works brought the concepts of word frequency in NLP to convert the sandbox file to fixed-size inputs ( David and Netanyahu 2015 ). Hashing features into a fixed vector was used as an effective method to represent features ( Saxe and Berlin 2015 ). Bytes histogram using the bytes analysis and bytes-entropy histogram with a sliding window method were considered ( De La Rosa et al. 2018 ). In ( De La Rosa et al. 2018 ), De La Rosa and others embeded strings by hashing the ASCII strings to a fixed-size feature vector. For assembly features, they extracted four different levels of granularity: operation level (instruction-flow-graph), block level (control-flow-graph), function level (call-graph), and global level (graphs summarized). bigram, trigram and four-gram vectors and n -gram graph were used for the hybrid features ( Xu et al. 2018 ).
Observation 7.3: Most Phase III methods were classified into class 1.
Following the classification tree shown in Fig. 2 , most works were classified into class 1 shown in Fig. 2 except two works ( Dahl et al. 2013 ; Tobiyama et al. 2016 ), which belonged to class 3 shown in Fig. 2 . To reduce the input dimension, Dahl et al. (2013 ) performed feature selection using mutual information and random projection. Tobiyama et al. generated behavioral feature images using RNN ( Tobiyama et al. 2016 ).
Observation 7.4: After extracting features, two kinds of neural network architectures, i.e., one single neural network and multiple neural networks with a combined loss function, were used.
Hierarchical structures, like convolutional layers, fully connected layers and classification layers, were used to classify programs ( McLaughlin et al. 2017 ; Dahl et al. 2013 ; Nix and Zhang 2017 ; Saxe and Berlin 2015 ; Tobiyama et al. 2016 ; Cui et al. 2018 ; Kalash et al. 2018 ). A deep stack of denoising autoencoders was also introduced to learn programs’ behaviors ( David and Netanyahu 2015 ). De La Rosa and others ( De La Rosa et al. 2018 ) trained three different models with different features to compare which static features are relevant for the classification model. Some works investigated LSTM models for sequential features ( Nix and Zhang 2017 ; Rosenberg et al. 2018 ).
Two networks with different features as inputs were used for malware classification by combining their outputs with a dropout layer and an output layer ( Kolosnjaji et al. 2017 ). In ( Kolosnjaji et al. 2017 ), one network transformed PE Metadata and import features using feedforward neurons, another one leveraged convolutional network layers with opcode sequences. Lifan Xu et al. ( Xu et al. 2018 ) constructed a few networks and combined them using a two-level multiple kernel learning algorithm.
Indication 7.1: Except two works transform binary into images ( Cui et al. 2018 ; Kalash et al. 2018 ), most works surveyed need to adapt methods to vectorize extracted features.
The vectorization methods should not only keep syntactic and semantic information in features, but also consider the definition of the Deep Learning model.
Indication 7.2: Only limited works have shown how to transform features using representation learning.
Because some works assume the dynamic and static sequences, like API calls and instruction, and have similar syntactic and semantic structure as natural language, some representation learning techniques like word2vec may be useful in malware detection. In addition, for the control-flow graph, call graph and other graph representations, graph embedding is a potential method to transform those features.
Though several pieces of research have been done in malware detection using Deep Learning, it’s hard to compare their methods and performances because of two uncertainties in their approaches. First, the Deep Learning model is a black-box, researchers cannot detail which kind of features the model learned and explain why their model works. Second, feature selection and representation affect the model’s performance. Because they do not use the same datasets, researchers cannot prove their approaches – including selected features and Deep Learning model – are better than others. The reason why few researchers use open datasets is that existing open malware datasets are out of data and limited. Also, researchers need to crawl benign programs from app stores, so their raw programs will be diverse.
A closer look at applications of Deep Learning in system-event-based anomaly detection
System logs recorded significant events at various critical points, which can be used to debug the system’s performance issues and failures. Moreover, log data are available in almost all computer systems and are a valuable resource for understanding system status. There are a few challenges in anomaly detection based on system logs. Firstly, the raw log data are unstructured, while their formats and semantics can vary significantly. Secondly, logs are produced by concurrently running tasks. Such concurrency makes it hard to apply workflow-based anomaly detection methods. Thirdly, logs contain rich information and complexity types, including text, real value, IP address, timestamp, and so on. The contained information of each log is also varied. Finally, there are massive logs in every system. Moreover, each anomaly event usually incorporates a large number of logs generated in a long period.
Recently, a large number of scholars employed deep learning techniques ( Du et al. 2017 ; Meng et al. 2019 ; Das et al. 2018 ; Brown et al. 2018 ; Zhang et al. 2019 ; Bertero et al. 2017 ) to detect anomaly events in the system logs and diagnosis system failures. The raw log data are unstructured, while their formats and semantics can vary significantly. To detect the anomaly event, the raw log usually should be parsed to structure data, the parsed data can be transformed into a representation that supports an effective deep learning model. Finally, the anomaly event can be detected by deep learning based classifier or predictor.
In this section, we will review the very recent six representative papers that use deep learning for system-event-based anomaly detection ( Du et al. 2017 ; Meng et al. 2019 ; Das et al. 2018 ; Brown et al. 2018 ; Zhang et al. 2019 ; Bertero et al. 2017 ). DeepLog ( Du et al. 2017 ) utilizes LSTM to model the system log as a natural language sequence, which automatically learns log patterns from the normal event, and detects anomalies when log patterns deviate from the trained model. LogAnom ( Meng et al. 2019 ) employs Word2vec to extract the semantic and syntax information from log templates. Moreover, it uses sequential and quantitative features simultaneously. Das et al. (2018 ) uses LSTM to predict node failures that occur in super computing systems from HPC logs. Brown et al. (2018 ) presented RNN language models augmented with attention for anomaly detection in system logs. LogRobust ( Zhang et al. 2019 ) uses FastText to represent semantic information of log events, which can identify and handle unstable log events and sequences. Bertero et al. (2017 ) map log word to a high dimensional metric space using Google’s word2vec algorithm and take it as features to classify. Among these six papers, we select two representative works ( Du et al. 2017 ; Meng et al. 2019 ) and summarize the four phases of their approaches. We direct interested readers to Table 2 for a concise overview of these two works.
From a close look at the very recent applications using deep learning for solving security-event-based anomaly detection challenges, we observed the followings:
Observation 8.1: Most works of our surveyed papers evaluated their performance using public datasets.
By the time we surveyed this paper, only two works in ( Das et al. 2018 ; Bertero et al. 2017 ) used their private datasets.
Observation 8.2: Most works in this survey adopted Phase II when parsing the raw log data.
After reviewing the six works proposed recently, we found that five works ( Du et al. 2017 ; Meng et al. 2019 ; Das et al. 2018 ; Brown et al. 2018 ; Zhang et al. 2019 ) employed parsing technique, while only one work ( Bertero et al. 2017 ) did not.
DeepLog ( Du et al. 2017 ) parsed the raw log to different log type using Spell ( Du and Li 2016 ) which is based a longest common subsequence. Desh ( Das et al. 2018 ) parsed the raw log to constant message and variable component. Loganom ( Meng et al. 2019 ) parsed the raw log to different log templates using FT-Tree ( Zhang et al. 2017 ) according to the frequent combinations of log words. Andy Brown et al. ( Brown et al. 2018 ) parsed the raw log into word and character tokenization. LogRobust ( Zhang et al. 2019 ) extracted its log event by abstracting away the parameters in the message. Bertero et al. (2017 ) considered logs as regular text without parsing.
Observation 8.3: Most works have considered and adopted Phase III.
Among these six works, only DeepLog represented the parsed data using the one-hot vector without learning. Moreover, Loganom ( Meng et al. 2019 ) compared their results with DeepLog. That is, DeepLog belongs to class 1 and Loganom belongs to class 4 in Fig. 2 , while the other four works follow in class 3.
The four works ( Meng et al. 2019 ; Das et al. 2018 ; Zhang et al. 2019 ; Bertero et al. 2017 ) used word embedding techniques to represent the log data. Andy Brown et al. ( Brown et al. 2018 ) employed attention vectors to represent the log messages.
DeepLog ( Du et al. 2017 ) employed the one-hot vector to represent the log type without learning. We have engaged an experiment replacing the one-hot vector with trained word embeddings.
Observation 8.4: Evaluation results were not compared using the same dataset.
DeepLog ( Du et al. 2017 ) employed the one-hot vector to represent the log type without learning, which employed Phase II without Phase III. However, Christophe Bertero et al. ( Bertero et al. 2017 ) considered logs as regular text without parsing, and used Phase III without Phase II. The precision of the two methods is very high, which is greater than 95%. Unfortunately, the evaluations of the two methods used different datasets.
Observation 8.5: Most works empolyed LSTM in Phase IV.
Five works including ( Du et al. 2017 ; Meng et al. 2019 ; Das et al. 2018 ; Brown et al. 2018 ; Zhang et al. 2019 ) employed LSTM in the Phase IV, while Bertero et al. (2017 ) tried different classifiers including naive Bayes, neural networks and random forest.
Indication 8.1: Phase II has a positive effect on accuracy if being well-designed.
Since Bertero et al. (2017 ) considers logs as regular text without parsing, we can say that Phase II is not required. However, we can find that most of the scholars employed parsing techniques to extract structure information and remove the useless noise.
Indication 8.2: Most of the recent works use trained representation to represent parsed data.
As shown in Table 3 , we can find Phase III is very useful, which can improve detection accuracy.
Indication 8.3: Phase II and Phase III cannot be skipped simultaneously.
Both Phase II and Phase III are not required. However, all methods have employed Phase II or Phase III.
Indication 8.4: Observation 8.3 indicates that the trained word embedding format can improve the anomaly detection accuracy as shown in Table 3 .
Indication 8.5: Observation 8.5 indicates that most of the works adopt LSTM to detect anomaly events.
We can find that most of the works adopt LSTM to detect anomaly event, since log data can be considered as sequence and there can be lags of unknown duration between important events in a time series. LSTM has feedback connections, which can not only process single data points, but also entire sequences of data.
As our consideration, neither Phase II nor Phase III is required in system event-based anomaly detection. However, Phase II can remove noise in raw data, and Phase III can learn a proper representation of the data. Both Phase II and Phase III have a positive effect on anomaly detection accuracy. Since the event log is text data that we can’t feed the raw log data into deep learning model directly, Phase II and Phase III can’t be skipped simultaneously.
Deep learning can capture the potentially nonlinear and high dimensional dependencies among log entries from the training data that correspond to abnormal events. In that way, it can release the challenges mentioned above. However, it still suffers from several challenges. For example, how to represent the unstructured data accurately and automatically without human knowledge.
A closer look at applications of deep learning in solving memory forensics challenges
In the field of computer security, memory forensics is security-oriented forensic analysis of a computer’s memory dump. Memory forensics can be conducted against OS kernels, user-level applications, as well as mobile devices. Memory forensics outperforms traditional disk-based forensics because although secrecy attacks can erase their footprints on disk, they would have to appear in memory ( Song et al. 2018 ). The memory dump can be considered as a sequence of bytes, thus memory forensics usually needs to extract security semantic information from raw memory dump to find attack traces.
The traditional memory forensic tools fall into two categories: signature scanning and data structure traversal. These traditional methods usually have some limitations. Firstly, it needs expert knowledge on the related data structures to create signatures or traversing rules. Secondly, attackers may directly manipulate data and pointer values in kernel objects to evade detection, and then it becomes even more challenging to create signatures and traversing rules that cannot be easily violated by malicious manipulations, system updates, and random noise. Finally, the high-efficiency requirement often sacrifices high robustness. For example, an efficient signature scan tool usually skips large memory regions that are unlikely to have the relevant objects and relies on simple but easily tamperable string constants. An important clue may hide in this ignored region.
In this section, we will review the very recent four representative works that use Deep Learning for memory forensics ( Song et al. 2018 ; Petrik et al. 2018 ; Michalas and Murray 2017 ; Dai et al. 2018 ). DeepMem ( Song et al. 2018 ) recognized the kernel objects from raw memory dumps by generating abstract representations of kernel objects with a graph-based Deep Learning approach. MDMF ( Petrik et al. 2018 ) detected OS and architecture-independent malware from memory snapshots with several pre-processing techniques, domain unaware feature selection, and a suite of machine learning algorithms. MemTri ( Michalas and Murray 2017 ) predicts the likelihood of criminal activity in a memory image using a Bayesian network, based on evidence data artefacts generated by several applications. Dai et al. (2018 ) monitor the malware process memory and classify malware according to memory dumps, by transforming the memory dump into grayscale images and adopting a multi-layer perception as the classifier.
Among these four works ( Song et al. 2018 ; Petrik et al. 2018 ; Michalas and Murray 2017 ; Dai et al. 2018 ), two representative works (i.e., ( Song et al. 2018 ; Petrik et al. 2018 )) are already summarized phase-by-phase in Table 1. We direct interested readers to Table 2 for a concise overview of these two works.
Our review will be centered around the three questions raised in Section 3 . In the remaining of this section, we will first provide a set of observations, and then we provide the indications. Finally, we provide some general remarks.
From a close look at the very recent applications using Deep Learning for solving memory forensics challenges, we observed the followings:
Observation 9.1: Most methods used their own datasets for performance evaluation, while none of them used a public dataset.
DeepMem was evaluated on self-generated dataset by the authors, who collected a large number of diverse memory dumps, and labeled the kernel objects in them using existing memory forensics tools like Volatility. MDMF employed the MalRec dataset by Georgia Tech to generate malicious snapshots, while it created a dataset of benign memory snapshots running normal software. MemTri ran several Windows 7 virtual machine instances with self-designed suspect activity scenarios to gather memory images. Dai et al. built the Procdump program in Cuckoo sandbox to extract malware memory dumps. We found that each of the four works in our survey generated their own datasets, while none was evaluated on a public dataset.
Observation 9.2: Among the four works ( Song et al. 2018 ; Michalas and Murray 2017 ; Petrik et al. 2018 ; Dai et al. 2018 ), two works ( Song et al. 2018 ; Michalas and Murray 2017 ) employed Phase II while the other two works ( Petrik et al. 2018 ; Dai et al. 2018 ) did not employ.
DeepMem ( Song et al. 2018 ) devised a graph representation for a sequence of bytes, taking into account both adjacency and points-to relations, to better model the contextual information in memory dumps. MemTri ( Michalas and Murray 2017 ) firstly identified the running processes within the memory image that match the target applications, then employed regular expressions to locate evidence artefacts in a memory image. MDMF ( Petrik et al. 2018 ) and Dai et al. (2018 ) transformed the memory dump into image directly.
Observation 9.3: Among four works ( Song et al. 2018 ; Michalas and Murray 2017 ; Petrik et al. 2018 ; Dai et al. 2018 ), only DeepMem ( Song et al. 2018 ) employed Phase III for which it used an embedding method to represent a memory graph.
MDMF ( Petrik et al. 2018 ) directly fed the generated memory images into the training of a CNN model. Dai et al. (2018 ) used HOG feature descriptor for detecting objects, while MemTri ( Michalas and Murray 2017 ) extracted evidence artefacts as the input of Bayesian Network. In summary, DeepMem belonged to class 3 shown in Fig. 2 , while the other three works belonged to class 1 shown in Fig. 2 .
Observation 9.4: All the four works ( Song et al. 2018 ; Petrik et al. 2018 ; Michalas and Murray 2017 ; Dai et al. 2018 ) have employed different classifiers even when the types of input data are the same.
DeepMem chose fully connected network (FCN) model that has multi-layered hidden neurons with ReLU activation functions, following by a softmax layer as the last layer. MDMF ( Petrik et al. 2018 ) evaluated their performance both on traditional machine learning algorithms and Deep Learning approach including CNN and LSTM. Their results showed the accuracy of different classifiers did not have a significant difference. MemTri employed a Bayesian network model that is designed with three layers, i.e., a hypothesis layer, a sub-hypothesis layer, and an evidence layer. Dai et al. used a multi-layer perception model including an input layer, a hidden layer and an output layer as the classifier.
Indication 9.1: There lacks public datasets for evaluating the performance of different Deep Learning methods in memory forensics.
From Observation 9.1, we find that none of the four works surveyed was evaluated on public datasets.
Indication 9.2: From Observation 9.2, we find that it is disputable whether one should employ Phase II when solving memory forensics problems.
Since both ( Petrik et al. 2018 ) and ( Dai et al. 2018 ) directly transformed a memory dump into an image, Phase II is not required in these two works. However, since there is a large amount of useless information in a memory dump, we argue that appropriate prepossessing could improve the accuracy of the trained models.
Indication 9.3: From Observation 9.3, we find that Phase III is paid not much attention in memory forensics.
Most works did not employ Phase III. Among the four works, only DeepMem ( Song et al. 2018 ) employed Phase III during which it used embeddings to represent a memory graph. The other three works ( Petrik et al. 2018 ; Michalas and Murray 2017 ; Dai et al. 2018 ) did not learn any representations before training a Deep Learning model.
Indication 9.4: For Phase IV in memory forensics, different classifiers can be employed.
Which kind of classifier to use seems to be determined by the features used and their data structures. From Observation 9.4, we find that the four works have actually employed different kinds of classifiers even the types of input data are the same. It is very interesting that MDMF obtained similar results with different classifiers including traditional machine learning and Deep Learning models. However, the other three works did not discuss why they chose a particular kind of classifier.
Since a memory dump can be considered as a sequence of bytes, the data structure of a training data example is straightforward. If the memory dump is transformed into a simple form in Phase II, it can be directly fed into the training process of a Deep Learning model, and as a result Phase III can be ignored. However, if the memory dump is transformed into a complicated form in Phase II, Phase III could be quite useful in memory forensics.
Regarding the answer for Question 3 at “ Methodology for reviewing the existing works ” section, it is very interesting that during Phase IV different classifiers can be employed in memory forensics. Moreover, MDMF ( Petrik et al. 2018 ) has shown that they can obtain similar results with different kinds of classifiers. Nevertheless, they also admit that with a larger amount of training data, the performance could be improved by Deep Learning.
An end-to-end manner deep learning model can learn the precise representation of memory dump automatically to release the requirement for expert knowledge. However, it still needs expert knowledge to represent data and attacker behavior. Attackers may also directly manipulate data and pointer values in kernel objects to evade detection.
A closer look at applications of deep learning in security-oriented fuzzing
Fuzzing of software security is one of the state of art techniques that people use to detect software vulnerabilities. The goal of fuzzing is to find all the vulnerabilities exist in the program by testing as much program code as possible. Due to the nature of fuzzing, this technique works best on finding vulnerabilities in programs that take in input files, like PDF viewers ( Godefroid et al. 2017 ) or web browsers. A typical workflow of fuzzing can be concluded as: given several seed input files, the fuzzer will mutate or fuzz the seed inputs to get more input files, with the aim of expanding the overall code coverage of the target program as it executes the mutated files. Although there have already been various popular fuzzers ( Li et al. 2018 ), fuzzing still cannot bypass its problem of sometimes redundantly testing input files which cannot improve the code coverage rate ( Shi and Pei 2019 ; Rajpal et al. 2017 ). Some input files mutated by the fuzzer even cannot pass the well-formed file structure test ( Godefroid et al. 2017 ). Recent research has come up with ideas of applying Deep Learning in the process of fuzzing to solve these problems.
In this section, we will review the very recent four representative works that use Deep Learning for fuzzing for software security. Among the three, two representative works ( Godefroid et al. 2017 ; Shi and Pei 2019 ) are already summarized phase-by-phase in Table 2 . We direct interested readers to Table 2 for a concise overview of those two works.
Observation 10.1: Deep Learning has only been applied in mutation-based fuzzing.
Even though various of different fuzzing techniques, including symbolic execution based fuzzing ( Stephens et al. 2016 ), tainted analysis based fuzzing ( Bekrar et al. 2012 ) and hybrid fuzzing ( Yun et al. 2018 ) have been proposed so far, we observed that all the works we surveyed employed Deep Learning method to assist the primitive fuzzing – mutation-based fuzzing. Specifically, they adopted Deep Learning to assist fuzzing tool’s input mutation. We found that they commonly did it in two ways: 1) training Deep Learning models to tell how to efficiently mutate the input to trigger more execution path ( Shi and Pei 2019 ; Rajpal et al. 2017 ); 2) training Deep Learning models to tell how to keep the mutated files compliant with the program’s basic semantic requirement ( Godefroid et al. 2017 ). Besides, all three works trained different Deep Learning models for different programs, which means that knowledge learned from one programs cannot be applied to other programs.
Observation 10.2: Similarity among all the works in our survey existed when choosing the training samples in Phase I.
The works in this survey had a common practice, i.e., using the input files directly as training samples of the Deep Learning model. Learn&Fuzz ( Godefroid et al. 2017 ) used character-level PDF objects sequence as training samples. Neuzz ( Shi and Pei 2019 ) regarded input files directly as byte sequences and fed them into the neural network model. Rajpal et al. (2017 ) also used byte level representations of input files as training samples.
Observation 10.3: Difference between all the works in our survey existed when assigning the training labels in Phase I.
Despite the similarity of training samples researchers decide to use, there was a huge difference in the training labels that each work chose to use. Learn&Fuzz ( Godefroid et al. 2017 ) directly used the character sequences of PDF objects as labels, same as training samples, but shifted by one position, which is a common generative model technique already broadly used in speech and handwriting recognition. Unlike Learn&Fuzz, Neuzz ( Shi and Pei 2019 ) and Rajpal’s work ( Rajpal et al. 2017 ) used bitmap and heatmap respectively as training labels, with the bitmap demonstrating the code coverage status of a certain input, and the heatmap demonstrating the efficacy of flipping one or more bytes of the input file. Whereas, as a common terminology well-known among fuzzing researchers, bitmap was gathered directly from the results of AFL. Heatmap used by Rajpal et al. was generated by comparing the code coverage supported by the bitmap of one seed file and the code coverage supported by bitmaps of the mutated seed files. It was noted that if there is acceptable level of code coverage expansion when executing the mutated seed files, demonstrated by more “1”s, instead of “0”s in the corresponding bitmaps, the byte level differences among the original seed file and the mutated seed files will be highlighted. Since those bytes should be the focus of later on mutation, heatmap was used to denote the location of those bytes.
Different labels usage in each work was actually due to the different kinds of knowledge each work wants to learn. For a better understanding, let us note that we can simply regard a Deep Learning model as a simulation of a “function”. Learn&Fuzz ( Godefroid et al. 2017 ) wanted to learn valid mutation of a PDF file that was compliant with the syntax and semantic requirements of PDF objects. Their model could be seen as a simulation of f ( x , θ )= y , where x denotes sequence of characters in PDF objects and y represents a sequence that are obtained by shifting the input sequences by one position. They generated new PDF object character sequences given a starting prefix once the model was trained. In Neuzz ( Shi and Pei 2019 ), an NN(Neural Network) model was used to do program smoothing, which simultated a smooth surrogate function that approximated the discrete branching behaviors of the target program. f ( x , θ )= y , where x denoted program’s byte level input and y represented the corresponding edge coverage bitmap. In this way, the gradient of the surrogate function was easily computed, due to NN’s support of efficient computation of gradients and higher order derivatives. Gradients could then be used to guide the direction of mutation, in order to get greater code coverage. In Rajpal and others’ work ( Rajpal et al. 2017 ), they designed a model to predict good (and bad) locations to mutate in input files based on the past mutations and corresponding code coverage information. Here, the x variable also denoted program’s byte level input, but the y variable represented the corresponding heatmap.
Observation 10.4: Various lengths of input files were handled in Phase II.
Deep Learning models typically accepted fixed length input, whereas the input files for fuzzers often held different lengths. Two different approaches were used among the three works we surveyed: splitting and padding. Learn&Fuzz ( Godefroid et al. 2017 ) dealt with this mismatch by concatenating all the PDF objects character sequences together, and then splited the large character sequence into multiple training samples with a fixed size. Neuzz ( Shi and Pei 2019 ) solved this problem by setting a maximize input file threshold and then, padding the smaller-sized input files with null bytes. From additional experiments, they also found that a modest threshold gived them the best result, and enlarging the input file size did not grant them additional accuracy. Aside from preprocessing training samples, Neuzz also preprocessed training labels and reduced labels dimension by merging the edges that always appeared together into one edge, in order to prevent the multicollinearity problem, that could prevent the model from converging to a small loss value. Rajpal and others ( Rajpal et al. 2017 ) used the similar splitting mechanism as Learn&Fuzz to split their input files into either 64-bit or 128-bit chunks. Their chunk size was determined empirically and was considered as a trainable parameter for their Deep Learning model, and their approach did not require sequence concatenating at the beginning.
Observation 10.5: All the works in our survey skipped Phase III.
According to our definition of Phase III, all the works in our survey did not consider representation learning. Therefore, all the three works ( Godefroid et al. 2017 ; Shi and Pei 2019 ; Rajpal et al. 2017 ) fell into class 1 shown in Fig. 2 .While as in Rajpal and others’ work, they considered the numerical representation of byte sequences. They claimed that since one byte binary data did not always represent the magnitude but also state, representing one byte in values ranging from 0 to 255 could be suboptimal. They used lower level 8-bit representation.
Indication 10.1: No alteration to the input files seems to be a correct approach. As far as we concerned, it is due to the nature of fuzzing. That is, since every bit of the input files matters, any slight alteration to the input files could either lose important information or add redundant information for the neural network model to learn.
Indication 10.2: Evaluation criteria should be chosen carefully when judging mutation.
Input files are always used as training samples regarding using Deep Learning technique in fuzzing problems. Through this similar action, researchers have a common desire to let the neural network mode learn how the mutated input files should look like. But the criterion of judging a input file actually has two levels: on the one hand, a good input file should be correct in syntax and semantics; on the other hand, a good input file should be the product of a useful mutation, which triggers the program to behave differently from previous execution path. This idea of a fuzzer that can generate semantically correct input file could still be a bad fuzzer at triggering new execution path was first brought up in Learn&Fuzz ( Godefroid et al. 2017 ). We could see later on works trying to solve this problem by using either different training labels ( Rajpal et al. 2017 ) or use neural network to do program smoothing ( Shi and Pei 2019 ). We encouraged fuzzing researchers, when using Deep Learning techniques, to keep this problem in mind, in order to get better fuzzing results.
Indication 10.3: Works in our survey only focus on local knowledge. In brief, some of the existing works ( Shi and Pei 2019 ; Rajpal et al. 2017 ) leveraged the Deep Learning model to learn the relation between program’s input and its behavior and used the knowledge that learned from history to guide future mutation. For better demonstration, we defined the knowledge that only applied in one program as local knowledge . In other words, this indicates that the local knowledge cannot direct fuzzing on other programs.
Corresponding to the problems conventional fuzzing has, the advantages of applying DL in fuzzing are that DL’s learning ability can ensure mutated input files follow the designated grammar rules better. The ways in which input files are generated are more directed, and will, therefore, guarantee the fuzzer to increase its code coverage by each mutation. However, even if the advantages can be clearly demonstrated by the two papers we discuss above, some challenges still exist, including mutation judgment challenges that are faced both by traditional fuzzing techniques and fuzzing with DL, and the scalability of fuzzing approaches.
We would like to raise several interesting questions for the future researchers: 1) Can the knowledge learned from the fuzzing history of one program be applied to direct testing on other programs? 2) If the answer to question one is positive, we can suppose that global knowledge across different programs exists? Then, can we train a model to extract the global knowledge ? 3) Whether it is possible to combine global knowledge and local knowledge when fuzzing programs?
Using high-quality data in Deep Learning is important as much as using well-structured deep neural network architectures. That is, obtaining quality data must be an important step, which should not be skipped, even in resolving security problems using Deep Learning. So far, this study demonstrated how the recent security papers using Deep Learning have adopted data conversion (Phase II) and data representation (Phase III) on different security problems. Our observations and indications showed a clear understanding of how security experts generate quality data when using Deep Learning.
Since we did not review all the existing security papers using Deep Learning, the generality of observations and indications is somewhat limited. Note that our selected papers for review have been published recently at one of prestigious security and reliability conferences such as USENIX SECURITY, ACM CCS and so on ( Shin et al. 2015 )-( Das et al. 2018 ), ( Brown et al. 2018 ; Zhang et al. 2019 ), ( Song et al. 2018 ; Petrik et al. 2018 ), ( Wang et al. 2019 )-( Rajpal et al. 2017 ). Thus, our observations and indications help to understand how most security experts have used Deep Learning to solve the well-known eight security problems from program analysis to fuzzing.
Our observations show that we should transfer raw data to synthetic formats of data ready for resolving security problems using Deep Learning through data cleaning and data augmentation and so on. Specifically, we observe that Phases II and III methods have mainly been used for the following purposes:
To clean the raw data to make the neural network (NN) models easier to interpret
To reduce the dimensionality of data (e.g., principle component analysis (PCA), t-distributed stochastic neighbor embedding (t-SNE))
To scale input data (e.g., normalization)
To make NN models understand more complex relationships depending on security problems (e.g. memory graphs)
To simply change various raw data formats into a vector format for NN models (e.g. one-hot encoding and word2vec embedding)
In this following, we do further discuss the question, “What if Phase II is skipped?", rather than the question, “Is Phase III always necessary?". This is because most of the selected papers do not consider Phase III methods (76%), or adopt with no concrete reasoning (19%). Specifically, we demonstrate how Phase II has been adopted according to eight security problems, different types of data, various models of NN and various outputs of NN models, in depth. Our key findings are summarized as follows:
How to fit security domain knowledge into raw data has not been well-studied yet.
While raw text data are commonly parsed after embedding, raw binary data are converted using various Phase II methods.
Raw data are commonly converted into a vector format to fit well to a specific NN model using various Phase II methods.
Various Phase II methods are used according to the relationship between output of security problem and output of NN models.
What if phase II is skipped?
From the analysis results of our selected papers for review, we roughly classify Phase II methods into the following four categories.
Embedding: The data conversion methods that intend to convert high-dimensional discrete variables into low-dimensional continuous vectors ( Google Developers 2016 ).
Parsing combined with embedding: The data conversion methods that constitute an input data into syntactic components in order to test conformability after embedding.
One-hot encoding: A simple embedding where each data belonging to a specific category is mapped to a vector of 0s and a single 1. Here, the low-dimension transformed vector is not managed.
Domain-specific data structures: A set of data conversion methods which generate data structures capturing domain-specific knowledge for different security problems, e.g., memory graphs ( Song et al. 2018 ).
Findings on eight security problems
We observe that over 93% of the papers use one of the above-classified Phase II methods. 7% of the papers do not use any of the above-classified methods, and these papers are mostly solving a software fuzzing problem. Specifically, we observe that 35% of the papers use a Category 1 (i.e. embedding) method; 30% of the papers use a Category 2 (i.e. parsing combined with embedding) method; 15% of the papers use a Category 3 (i.e. one-hot encoding) method; and 13% of the papers use a Category 4 (i.e. domain-specific data structures) method. Regarding why one-hot encoding is not widely used, we found that most security data include categorical input values, which are not directly analyzed by Deep Learning models.
From Fig. 3 , we also observe that according to security problems, different Phase II methods are used. First, PA, ROP and CFI should convert raw data into a vector format using embedding because they commonly collect instruction sequence from binary data. Second, NA and SEAD use parsing combined with embedding because raw data such as the network traffic and system logs consist of the complex attributes with the different formats such as categorical and numerical input values. Third, we observe that MF uses various data structures because memory dumps from memory layout are unstructured. Fourth, fuzzing generally uses no data conversion since Deep Learning models are used to generate the new input data with the same data format as the original raw data. Finally, we observe that MC commonly uses one-hot encoding and embedding because malware binary and well-structured security log files include categorical, numerical and unstructured data in general. These observations indicate that type of data strongly influences on use of Phase II methods. We also observe that only MF among eight security problems commonly transform raw data into well-structured data embedding a specialized security domain knowledge. This observation indicates that various conversion methods of raw data into well-structure data which embed various security domain knowledge are not yet studied in depth.
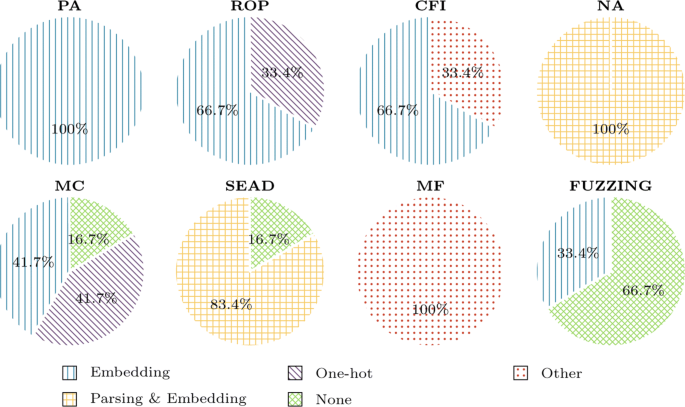
Statistics of Phase II methods for eight security problems
Findings on different data types
Note that according to types of data, a NN model works better than the others. For example, CNN works well with images but does not work with text. From Fig. 4 for raw binary data, we observe that 51.9%, 22.3% and 11.2% of security papers use embedding, one-hot encoding and Others , respectively. Only 14.9% of security papers, especially related to fuzzing, do not use one of Phase II methods. This observation indicates that binary input data which have various binary formats should be converted into an input data type which works well with a specific NN model. From Fig. 4 for raw text data, we also observe that 92.4% of papers use parsing with embedding as the Phase II method. Note that compared with raw binary data whose formats are unstructured, raw text data generally have the well-structured format. Raw text data collected from network traffics may also have various types of attribute values. Thus, raw text data are commonly parsed after embedding to reduce redundancy and dimensionality of data.
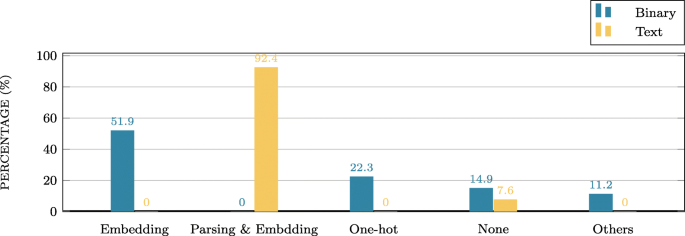
Statistics of Phase II methods on type of data
Findings on various models of NN
According to types of the converted data, a specific NN model works better than the others. For example, CNN works well with images but does not work with raw text. From Fig. 6 b, we observe that use of embedding for DNN (42.9%), RNN (28.6%) and LSTM (14.3%) models approximates to 85%. This observation indicates that embedding methods are commonly used to generate sequential input data for DNN, RNN and LSTM models. Also, we observe that one-hot encoded data are commonly used as input data for DNN (33.4%), CNN (33.4%) and LSTM (16.7%) models. This observation indicates that one-hot encoding is one of common Phase II methods to generate numerical values for image and sequential input data because many raw input data for security problems commonly have the categorical features. We observe that the CNN (66.7%) model uses the converted input data using the Others methods to express the specific domain knowledge into the input data structure of NN networks. This is because general vector formats including graph, matrix and so on can also be used as an input value of the CNN model.
From Fig. 5 b, we observe that DNN, RNN and LSTM models commonly use embedding, one-hot encoding and parsing combined with embedding. For example, we observe security papers of 54.6%, 18.2% and 18.2% models use embedding, one-hot encoding and parsing combined with embedding, respectively. We also observe that the CNN model is used with various Phase II methods because any vector formats such as image can generally be used as an input data of the CNN model.
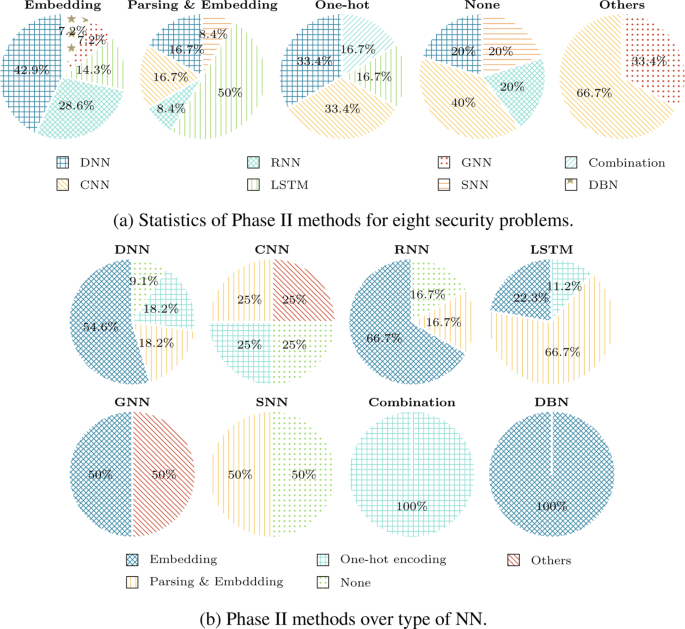
Statistics of Phase II methods for various types of NNs
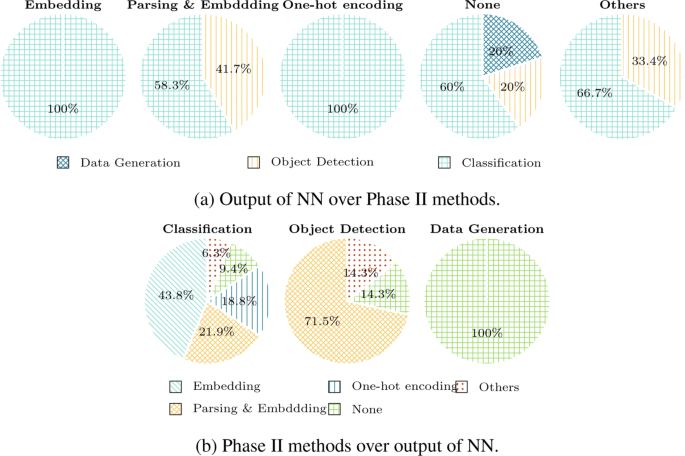
Statistics of Phase II methods for various output of NN
Findings on output of NN models
According to the relationship between output of security problem and output of NN, we may use a specific Phase II method. For example, if output of security problem is given into a class (e.g., normal or abnormal), output of NN should also be given into classification.
From Fig. 6 a, we observe that embedding is commonly used to support a security problem for classification (100%). Parsing combined with embedding is used to support a security problem for object detection (41.7%) and classification (58.3%). One-hot encoding is used only for classification (100%). These observations indicate that classification of a given input data is the most common output which is obtained using Deep Learning under various Phase II methods.
From Fig. 6 b, we observe that security problems, whose outputs are classification, commonly use embedding (43.8%) and parsing combined with embedding (21.9%) as the Phase II method. We also observe that security problems, whose outputs are object detection, commonly use parsing combined with embedding (71.5%). However, security problems, whose outputs are data generation, commonly do not use the Phase III methods. These observations indicate that a specific Phase II method has been used according to the relationship between output of security problem and use of NN models.
Further areas of investigation
Since any Deep Learning models are stochastic, each time the same Deep Learning model is fit even on the same data, it might give different outcomes. This is because deep neural networks use random values such as random initial weights. However, if we have all possible data for every security problem, we may not make random predictions. Since we have the limited sample data in practice, we need to get the best-effort prediction results using the given Deep Learning model, which fits to the given security problem.
How can we get the best-effort prediction results of Deep Learning models for different security problems? Let us begin to discuss about the stability of evaluation results for our selected papers for review. Next, we will elaborate the influence of security domain knowledge on prediction results of Deep Learning models. Finally, we will discuss some common issues in those fields.
How stable are evaluation results?
When evaluating neural network models, Deep Learning models commonly use three methods: train-test split; train-validation-test split; and k -fold cross validation. A train-test split method splits the data into two parts, i.e., training and test data. Even though a train-test split method makes the stable prediction with a large amount of data, predictions vary with a small amount of data. A train-validation-test split method splits the data into three parts, i.e., training, validation and test data. Validation data are used to estimate predictions over the unknown data. k -fold cross validation has k different set of predictions from k different evaluation data. Since k -fold cross validation takes the average expected performance of the NN model over k -fold validation data, the evaluation result is closer to the actual performance of the NN model.
From the analysis results of our selected papers for review, we observe that 40.0% and 32.5% of the selected papers are measured using a train-test split method and a train-validation-test split method, respectively. Only 17.5% of the selected papers are measured using k -fold cross validation. This observation implies that even though the selected papers show almost more than 99% of accuracy or 0.99 of F1 score, most solutions using Deep Learning might not show the same performance for the noisy data with randomness.
To get stable prediction results of Deep Learning models for different security problems, we might reduce the influence of the randomness of data on Deep Learning models. At least, it is recommended to consider the following methods:
Do experiments using the same data many time : To get a stable prediction with a small amount of sample data, we might control the randomness of data using the same data many times.
Use cross validation methods, e.g. k -fold cross validation : The expected average and variance from k -fold cross validation estimates how stable the proposed model is.
How does security domain knowledge influence the performance of security solutions using deep learning?
When selecting a NN model that analyzes an application dataset, e.g., MNIST dataset ( LeCun and Cortes 2010 ), we should understand that the problem is to classify a handwritten digit using a 28×28 black. Also, to solve the problem with the high classification accuracy, it is important to know which part of each handwritten digit mainly influences the outcome of the problem, i.e., a domain knowledge.
While solving a security problem, knowing and using security domain knowledge for each security problem is also important due to the following reasons (we label the observations and indications that realted to domain knowledge with ‘ ∗ ’):
Firstly, the dataset generation, preprocess and feature selection highly depend on domain knowledge. Different from the image classification and natural language processing, raw data in the security domain cannot be sent into the NN model directly. Researchers need to adopt strong domain knowledge to generate, extract, or clean the training set. Also, in some works, domain knowledge is adopted in data labeling because labels for data samples are not straightforward.
Secondly, domain knowledge helps with the selection of DL models and its hierarchical structure. For example, the neural network architecture (hierarchical and bi-directional LSTM) designed in DEEPVSA ( Guo et al. 2019 ) is based on the domain knowledge in the instruction analysis.
Thirdly, domain knowledge helps to speed up the training process. For instance, by adopting strong domain knowledge to clean the training set, domain knowledge helps to spend up the training process while keeping the same performance. However, due to the influence of the randomness of data on Deep Learning models, domain knowledge should be carefully adopted to avoid potential decreased accuracy.
Finally, domain knowledge helps with the interpretability of models’ prediction. Recently, researchers try to explore the interpretability of the deep learning model in security areas, For instance, LEMNA ( Guo et al. 2018 ) and EKLAVYA ( Chua et al. 2017 ) explain how the prediction was made by models from different perspectives. By enhancing the trained models’ interpretability, they can improve their approaches’ accuracy and security. The explanation for the relation between input, hidden state, and the final output is based on domain knowledge.
Common challenges
In this section, we will discuss the common challenges when applying DL to solving security problems. These challenges as least shared by the majority of works, if not by all the works. Generally, we observe 7 common challenges in our survey:
The raw data collected from the software or system usually contains lots of noise.
The collected raw is untidy. For instance, the instruction trace, the Untidy data: variable length sequences,
Hierarchical data syntactic/structure. As discussed in Section 3 , the information may not simply be encoded in a single layer, rather, it is encoded hierarchically, and the syntactic is complex.
Dataset generation is challenging in some scenarios. Therefore, the generated training data might be less representative or unbalanced.
Different for the application of DL in image classification, and natural language process, which is visible or understandable, the relation between data sample and its label is not intuitive, and hard to explain.
Availability of trained model and quality of dataset.
Finally, we investigate the availability of the trained model and the quality of the dataset. Generally, the availability of the trained models affects its adoption in practice, and the quality of the training set and the testing set will affect the credibility of testing results and comparison between different works. Therefore, we collect relevant information to answer the following four questions and shows the statistic in Table 4 :
Whether a paper’s source code is publicly available?
Whether raw data, which is used to generate the dataset, is publicly available?
Whether its dataset is publicly available?
How are the quality of the dataset?
We observe that both the percentage of open source of code and dataset in our surveyed fields is low, which makes it a challenge to reproduce proposed schemes, make comparisons between different works, and adopt them in practice. Specifically, the statistic shows that 1) the percentage of open source of code in our surveyed fields is low, only 6 out of 16 paper published their model’s source code. 2) the percentage of public data sets is low. Even though, the raw data in half of the works are publicly available, only 4 out of 16 fully or partially published their dataset. 3) the quality of datasets is not guaranteed, for instance, most of the dataset is unbalanced.
The performance of security solutions even using Deep Learning might vary according to datasets. Traditionally, when evaluating different NN models in image classification, standard datasets such as MNIST for recognizing handwritten 10 digits and CIFAR10 ( Krizhevsky et al. 2010 ) for recognizing 10 object classes are used for performance comparison of different NN models. However, there are no known standard datasets for evaluating NN models on different security problems. Due to such a limitation, we observe that most security papers using Deep Learning do not compare the performance of different security solutions even when they consider the same security problem. Thus, it is recommended to generate and use a standard dataset for a specific security problem for comparison. In conclusion, we think that there are three aspects that need to be improved in future research:
Developing standard dataset.
Publishing their source code and dataset.
Improving the interpretability of their model.
This paper seeks to provide a dedicated review of the very recent research works on using Deep Learning techniques to solve computer security challenges. In particular, the review covers eight computer security problems being solved by applications of Deep Learning: security-oriented program analysis, defending ROP attacks, achieving CFI, defending network attacks, malware classification, system-event-based anomaly detection, memory forensics, and fuzzing for software security. Our observations of the reviewed works indicate that the literature of using Deep Learning techniques to solve computer security challenges is still at an earlier stage of development.
Availability of data and materials
Not applicable.
We refer readers to ( Wang and Liu 2019 ) which systemizes the knowledge of protections by CFI schemes.
Abadi, M, Budiu M, Erlingsson Ú, Ligatti J (2009) Control-Flow Integrity Principles, Implementations, and Applications. ACM Trans Inf Syst Secur (TISSEC) 13(1):4.
Article Google Scholar
Bao, T, Burket J, Woo M, Turner R, Brumley D (2014) BYTEWEIGHT: Learning to Recognize Functions in Binary Code In: 23rd USENIX Security Symposium (USENIX Security 14), 845–860.. USENIX Association, San Diego.
Google Scholar
Bekrar, S, Bekrar C, Groz R, Mounier L (2012) A Taint Based Approach for Smart Fuzzing In: 2012 IEEE Fifth International Conference on Software Testing, Verification and Validation.. IEEE. https://doi.org/10.1109/icst.2012.182 .
Bengio, Y, Courville A, Vincent P (2013) Representation Learning: A Review and New Perspectives. IEEE Trans Pattern Anal Mach Intell 35(8):1798–1828.
Bertero, C, Roy M, Sauvanaud C, Tredan G (2017) Experience Report: Log Mining Using Natural Language Processing and Application to Anomaly Detection In: 2017 IEEE 28th International Symposium on Software Reliability Engineering (ISSRE).. IEEE. https://doi.org/10.1109/issre.2017.43 .
Brown, A, Tuor A, Hutchinson B, Nichols N (2018) Recurrent Neural Network Attention Mechanisms for Interpretable System Log Anomaly Detection In: Proceedings of the First Workshop on Machine Learning for Computing Systems, MLCS’18, 1:1–1:8.. ACM, New York.
Böttinger, K, Godefroid P, Singh R (2018) Deep Reinforcement Fuzzing In: 2018 IEEE Security and Privacy Workshops (SPW), pages 116–122.. IEEE. https://doi.org/10.1109/spw.2018.00026 .
Chen, L, Sultana S, Sahita R (2018) Henet: A Deep Learning Approach on Intel Ⓡ Processor Trace for Effective Exploit Detection In: 2018 IEEE Security and Privacy Workshops (SPW).. IEEE. https://doi.org/10.1109/spw.2018.00025 .
Chua, ZL, Shen S, Saxena P, Liang Z (2017) Neural Nets Can Learn Function Type Signatures from Binaries In: 26th USENIX Security Symposium (USENIX Security 17), 99–116.. USENIX Association. https://dl.acm.org/doi/10.5555/3241189.3241199 .
Cui, Z, Xue F, Cai X, Cao Y, Wang GG, Chen J (2018) Detection of Malicious Code Variants Based on Deep Learning. IEEE Trans Ind Inform 14(7):3187–3196.
Dahl, GE, Stokes JW, Deng L, Yu D (2013) Large-scale Malware Classification using Random Projections and Neural Networks In: IEEE International Conference on Acoustics, Speech and Signal Processing (ICASSP).. IEEE. https://doi.org/10.1109/icassp.2013.6638293 .
Dai, Y, Li H, Qian Y, Lu X (2018) A Malware Classification Method Based on Memory Dump Grayscale Image. Digit Investig 27:30–37.
Das, A, Mueller F, Siegel C, Vishnu A (2018) Desh: Deep Learning for System Health Prediction of Lead Times to Failure in HPC In: Proceedings of the 27th International Symposium on High-Performance Parallel and Distributed Computing, HPDC ’18, 40–51.. ACM, New York.
Chapter Google Scholar
David, OE, Netanyahu NS (2015) DeepSign: Deep Learning for Automatic Malware Signature Generation and Classification In: 2015 International Joint Conference on Neural Networks (IJCNN).. IEEE. https://doi.org/10.1109/ijcnn.2015.7280815 .
De La Rosa, L, Kilgallon S, Vanderbruggen T, Cavazos J (2018) Efficient Characterization and Classification of Malware Using Deep Learning In: 2018 Resilience Week (RWS).. IEEE. https://doi.org/10.1109/rweek.2018.8473556 .
Du, M, Li F (2016) Spell: Streaming Parsing of System Event Logs In: 2016 IEEE 16th International Conference on Data Mining (ICDM).. IEEE. https://doi.org/10.1109/icdm.2016.0103 .
Du, M, Li F, Zheng G, Srikumar V (2017) DeepLog: Anomaly Detection and Diagnosis from System Logs Through Deep Learning In: Proceedings of the 2017 ACM SIGSAC Conference on Computer and Communications Security, CCS ’17, 1285–1298.. ACM, New York.
Faker, O, Dogdu E (2019) Intrusion Detection Using Big Data and Deep Learning Techniques In: Proceedings of the 2019 ACM Southeast Conference on ZZZ - ACM SE ’19, 86–93.. ACM. https://doi.org/10.1145/3299815.3314439 .
Ghosh, AK, Wanken J, Charron F (1998) Detecting Anomalous and Unknown Intrusions against Programs In: Proceedings 14th annual computer security applications conference (Cat. No. 98Ex217), 259–267.. IEEE, Washington, DC.
Godefroid, P, Peleg H, Singh R (2017) Learn&Fuzz: Machine Learning for Input Fuzzing In: 2017 32nd IEEE/ACM International Conference on Automated Software Engineering (ASE).. IEEE. https://doi.org/10.1109/ase.2017.8115618 .
Google Developers (2016) Embeddings . https://developers.google.com/machine-learning/crash-course/embeddings/video-lecture .
Guo, W, Mu D, Xu J, Su P, Wang G, Xing X (2018) Lemna: Explaining deep learning based security applications In: Proceedings of the 2018 ACM SIGSAC Conference on Computer and Communications Security, pages 364–379. https://doi.org/10.1145/3243734.3243792 .
Guo, W, Mu D, Xing X, Du M, Song D (2019) { DEEPVSA }: Facilitating Value-set Analysis with Deep Learning for Postmortem Program Analysis In: 28th USENIX Security Symposium (USENIX Security 19), 1787–1804.. USENIX Association, Santa Clara, CA. https://www.usenix.org/conference/usenixsecurity19/presentation/guo .
Heller, KA, Svore KM, Keromytis AD, Stolfo SJ (2003) One Class Support Vector Machines for Detecting Anomalous Windows Registry Accesses In: Proceedings of the Workshop on Data Mining for Computer Security.. IEEE, Dallas, TX.
Horwitz, S (1997) Precise Flow-insensitive May-alias Analysis is NP-hard. ACM Trans Program Lang Syst 19(1):1–6.
Hu, W, Liao Y, Vemuri VR (2003) Robust Anomaly Detection using Support Vector Machines In: Proceedings of the international conference on machine learning, 282–289.. Citeseer, Washington, DC.
IDS 2017 Datasets (2019). https://www.unb.ca/cic/datasets/ids-2017.html .
Kalash, M, Rochan M, Mohammed N, Bruce NDB, Wang Y, Iqbal F (2018) Malware Classification with Deep Convolutional Neural Networks In: 2018 9th IFIP International Conference on New Technologies, Mobility and Security (NTMS), 1–5. https://doi.org/10.1109/NTMS.2018.8328749 .
Kiriansky, V, Bruening D, Amarasinghe SP, et al. (2002) Secure Execution via Program Shepherding In: USENIX Security Symposium, volume 92, page 84.. USENIX Association, Monterey, CA.
Kolosnjaji, B, Eraisha G, Webster G, Zarras A, Eckert C (2017) Empowering Convolutional Networks for Malware Classification and Analysis. Proc Int Jt Conf Neural Netw 2017-May:3838–3845.
Krizhevsky, A, Nair V, Hinton G (2010) CIFAR-10 (Canadian Institute for Advanced Research). https://www.cs.toronto.edu/~kriz/cifar.html .
LeCun, Y, Cortes C (2010) MNIST Handwritten Digit Database. http://yann.lecun.com/exdb/mnist/ .
Li, J, Zhao B, Zhang C (2018) Fuzzing: A Survey. Cybersecurity 1(1):6.
Li, X, Hu Z, Fu Y, Chen P, Zhu M, Liu P (2018) ROPNN: Detection of ROP Payloads Using Deep Neural Networks. arXiv preprint arXiv:1807.11110.
McLaughlin, N, Martinez Del Rincon J, Kang BJ, Yerima S, Miller P, Sezer S, Safaei Y, Trickel E, Zhao Z, Doupe A, Ahn GJ (2017) Deep Android Malware Detection In: Proceedings of the 7th ACM Conference on Data and Application Security and Privacy, 301–308. https://doi.org/10.1145/3029806.3029823 .
Meng, W, Liu Y, Zhu Y, Zhang S, Pei D, Liu Y, Chen Y, Zhang R, Tao S, Sun P, Zhou R (2019) Loganomaly: Unsupervised Detection of Sequential and Quantitative Anomalies in Unstructured Logs In: Proceedings of the Twenty-Eighth International Joint Conference on Artificial Intelligence.. International Joint Conferences on Artificial Intelligence Organization. https://doi.org/10.24963/ijcai.2019/658 .
Michalas, A, Murray R (2017) MemTri: A Memory Forensics Triage Tool Using Bayesian Network and Volatility In: Proceedings of the 2017 International Workshop on Managing Insider Security Threats, MIST ’17, pages 57–66.. ACM, New York.
Millar, K, Cheng A, Chew HG, Lim C-C (2018) Deep Learning for Classifying Malicious Network Traffic In: Pacific-Asia Conference on Knowledge Discovery and Data Mining, 156–161.. Springer. https://doi.org/10.1007/978-3-030-04503-6_15 .
Moustafa, N, Slay J (2015) UNSW-NB15: A Comprehensive Data Set for Network Intrusion Detection Systems (UNSW-NB15 Network Data Set) In: 2015 Military Communications and Information Systems Conference (MilCIS).. IEEE. https://doi.org/10.1109/milcis.2015.7348942 .
Nguyen, MH, Nguyen DL, Nguyen XM, Quan TT (2018) Auto-Detection of Sophisticated Malware using Lazy-Binding Control Flow Graph and Deep Learning. Comput Secur 76:128–155.
Nix, R, Zhang J (2017) Classification of Android Apps and Malware using Deep Neural Networks. Proc Int Jt Conf Neural Netw 2017-May:1871–1878.
NSCAI Intern Report for Congress (2019). https://drive.google.com/file/d/153OrxnuGEjsUvlxWsFYauslwNeCEkvUb/view .
Petrik, R, Arik B, Smith JM (2018) Towards Architecture and OS-Independent Malware Detection via Memory Forensics In: Proceedings of the 2018 ACM SIGSAC Conference on Computer and Communications Security, CCS ’18, pages 2267–2269.. ACM, New York.
Phan, AV, Nguyen ML, Bui LT (2017) Convolutional Neural Networks over Control Flow Graphs for Software defect prediction In: 2017 IEEE 29th International Conference on Tools with Artificial Intelligence (ICTAI), 45–52.. IEEE. https://doi.org/10.1109/ictai.2017.00019 .
Rajpal, M, Blum W, Singh R (2017) Not All Bytes are Equal: Neural Byte Sieve for Fuzzing. arXiv preprint arXiv:1711.04596.
Rosenberg, I, Shabtai A, Rokach L, Elovici Y (2018) Generic Black-box End-to-End Attack against State of the Art API Call based Malware Classifiers In: Research in Attacks, Intrusions, and Defenses, 490–510.. Springer. https://doi.org/10.1007/978-3-030-00470-5_23 .
Salwant, J (2015) ROPGadget. https://github.com/JonathanSalwan/ROPgadget .
Saxe, J, Berlin K (2015) Deep Neural Network based Malware Detection using Two Dimensional Binary Program Features In: 2015 10th International Conference on Malicious and Unwanted Software (MALWARE).. IEEE. https://doi.org/10.1109/malware.2015.7413680 .
Shacham, H, et al. (2007) The Geometry of Innocent Flesh on the Bone: Return-into-libc without Function Calls (on the x86) In: ACM conference on Computer and communications security, pages 552–561. https://doi.org/10.1145/1315245.1315313 .
Shi, D, Pei K (2019) NEUZZ: Efficient Fuzzing with Neural Program Smoothing. IEEE Secur Priv.
Shin, ECR, Song D, Moazzezi R (2015) Recognizing Functions in Binaries with Neural Networks In: 24th USENIX Security Symposium (USENIX Security 15).. USENIX Association. https://dl.acm.org/doi/10.5555/2831143.2831182 .
Sommer, R, Paxson V (2010) Outside the Closed World: On Using Machine Learning For Network Intrusion Detection In: 2010 IEEE Symposium on Security and Privacy (S&P).. IEEE. https://doi.org/10.1109/sp.2010.25 .
Song, W, Yin H, Liu C, Song D (2018) DeepMem: Learning Graph Neural Network Models for Fast and Robust Memory Forensic Analysis In: Proceedings of the 2018 ACM SIGSAC Conference on Computer and Communications Security, CCS ’18, 606–618.. ACM, New York.
Stephens, N, Grosen J, Salls C, Dutcher A, Wang R, Corbetta J, Shoshitaishvili Y, Kruegel C, Vigna G (2016) Driller: Augmenting Fuzzing Through Selective Symbolic Execution In: Proceedings 2016 Network and Distributed System Security Symposium.. Internet Society. https://doi.org/10.14722/ndss.2016.23368 .
Tan, G, Jaeger T (2017) CFG Construction Soundness in Control-Flow Integrity In: Proceedings of the 2017 Workshop on Programming Languages and Analysis for Security - PLAS ’17.. ACM. https://doi.org/10.1145/3139337.3139339 .
Tobiyama, S, Yamaguchi Y, Shimada H, Ikuse T, Yagi T (2016) Malware Detection with Deep Neural Network Using Process Behavior. Proc Int Comput Softw Appl Conf 2:577–582.
Unicorn-The ultimate CPU emulator (2015). https://www.unicorn-engine.org/ .
Ustebay, S, Turgut Z, Aydin MA (2019) Cyber Attack Detection by Using Neural Network Approaches: Shallow Neural Network, Deep Neural Network and AutoEncoder In: Computer Networks, 144–155.. Springer. https://doi.org/10.1007/978-3-030-21952-9_11 .
Varenne, R, Delorme JM, Plebani E, Pau D, Tomaselli V (2019) Intelligent Recognition of TCP Intrusions for Embedded Micro-controllers In: International Conference on Image Analysis and Processing, 361–373.. Springer. https://doi.org/10.1007/978-3-030-30754-7_36 .
Wang, Z, Liu P (2019) GPT Conjecture: Understanding the Trade-offs between Granularity, Performance and Timeliness in Control-Flow Integrity. eprint 1911.07828, archivePrefix arXiv, primaryClass cs.CR, arXiv.
Wang, Y, Wu Z, Wei Q, Wang Q (2019) NeuFuzz: Efficient Fuzzing with Deep Neural Network. IEEE Access 7:36340–36352.
Xu, W, Huang L, Fox A, Patterson D, Jordan MI (2009) Detecting Large-Scale System Problems by Mining Console Logs In: Proceedings of the ACM SIGOPS 22Nd Symposium on Operating Systems Principles SOSP ’09, 117–132.. ACM, New York.
Xu, X, Liu C, Feng Q, Yin H, Song L, Song D (2017) Neural Network-Based Graph Embedding for Cross-Platform Binary Code Similarity Detection In: Proceedings of the 2017 ACM SIGSAC Conference on Computer and Communications Security, 363–376.. ACM. https://doi.org/10.1145/3133956.3134018 .
Xu, L, Zhang D, Jayasena N, Cavazos J (2018) HADM: Hybrid Analysis for Detection of Malware 16:702–724.
Xu, X, Ghaffarinia M, Wang W, Hamlen KW, Lin Z (2019) CONFIRM: Evaluating Compatibility and Relevance of Control-flow Integrity Protections for Modern Software In: 28th USENIX Security Symposium (USENIX Security 19), pages 1805–1821.. USENIX Association, Santa Clara.
Yagemann, C, Sultana S, Chen L, Lee W (2019) Barnum: Detecting Document Malware via Control Flow Anomalies in Hardware Traces In: Lecture Notes in Computer Science, 341–359.. Springer. https://doi.org/10.1007/978-3-030-30215-3_17 .
Yin, C, Zhu Y, Fei J, He X (2017) A Deep Learning Approach for Intrusion Detection using Recurrent Neural Networks. IEEE Access 5:21954–21961.
Yuan, X, Li C, Li X (2017) DeepDefense: Identifying DDoS Attack via Deep Learning In: 2017 IEEE International Conference on Smart Computing (SMARTCOMP).. IEEE. https://doi.org/10.1109/smartcomp.2017.7946998 .
Yun, I, Lee S, Xu M, Jang Y, Kim T (2018) QSYM : A Practical Concolic Execution Engine Tailored for Hybrid Fuzzing In: 27th USENIX Security Symposium (USENIX Security 18), pages 745–761.. USENIX Association, Baltimore.
Zhang, S, Meng W, Bu J, Yang S, Liu Y, Pei D, Xu J, Chen Y, Dong H, Qu X, Song L (2017) Syslog Processing for Switch Failure Diagnosis and Prediction in Datacenter Networks In: 2017 IEEE/ACM 25th International Symposium on Quality of Service (IWQoS).. IEEE. https://doi.org/10.1109/iwqos.2017.7969130 .
Zhang, J, Chen W, Niu Y (2019) DeepCheck: A Non-intrusive Control-flow Integrity Checking based on Deep Learning. arXiv preprint arXiv:1905.01858.
Zhang, X, Xu Y, Lin Q, Qiao B, Zhang H, Dang Y, Xie C, Yang X, Cheng Q, Li Z, Chen J, He X, Yao R, Lou J-G, Chintalapati M, Shen F, Zhang D (2019) Robust Log-based Anomaly Detection on Unstable Log Data In: Proceedings of the 27th ACM Joint Meeting on European Software Engineering Conference and Symposium on the Foundations of Software Engineering, ESEC/FSE 2019, pages 807–817.. ACM, New York.
Zhang, Y, Chen X, Guo D, Song M, Teng Y, Wang X (2019) PCCN: Parallel Cross Convolutional Neural Network for Abnormal Network Traffic Flows Detection in Multi-Class Imbalanced Network Traffic Flows. IEEE Access 7:119904–119916.
Download references
Acknowledgments
We are grateful to the anonymous reviewers for their useful comments and suggestions.
This work was supported by ARO W911NF-13-1-0421 (MURI), NSF CNS-1814679, and ARO W911NF-15-1-0576.
Author information
Authors and affiliations.
The Pennsylvania State University, Pennsylvania, USA
Yoon-Ho Choi, Peng Liu, Zitong Shang, Haizhou Wang, Zhilong Wang, Lan Zhang & Qingtian Zou
Pusan National University, Busan, Republic of Korea
Yoon-Ho Choi
Wuhan University of Technology, Wuhan, China
Junwei Zhou
You can also search for this author in PubMed Google Scholar
Contributions
All authors read and approved the final manuscript.
Corresponding author
Correspondence to Peng Liu .
Ethics declarations
Competing interests.
PL is currently serving on the editorial board for Journal of Cybersecurity.
Additional information
Publisher’s note.
Springer Nature remains neutral with regard to jurisdictional claims in published maps and institutional affiliations.
Rights and permissions
Open Access This article is licensed under a Creative Commons Attribution 4.0 International License, which permits use, sharing, adaptation, distribution and reproduction in any medium or format, as long as you give appropriate credit to the original author(s) and the source, provide a link to the Creative Commons licence, and indicate if changes were made. The images or other third party material in this article are included in the article’s Creative Commons licence, unless indicated otherwise in a credit line to the material. If material is not included in the article’s Creative Commons licence and your intended use is not permitted by statutory regulation or exceeds the permitted use, you will need to obtain permission directly from the copyright holder. To view a copy of this licence, visit http://creativecommons.org/licenses/by/4.0/ .
Reprints and permissions
About this article
Cite this article.
Choi, YH., Liu, P., Shang, Z. et al. Using deep learning to solve computer security challenges: a survey. Cybersecur 3 , 15 (2020). https://doi.org/10.1186/s42400-020-00055-5
Download citation
Received : 11 March 2020
Accepted : 17 June 2020
Published : 10 August 2020
DOI : https://doi.org/10.1186/s42400-020-00055-5
Share this article
Anyone you share the following link with will be able to read this content:
Sorry, a shareable link is not currently available for this article.
Provided by the Springer Nature SharedIt content-sharing initiative
- Deep learning
- Security-oriented program analysis
- Return-oriented programming attacks
- Control-flow integrity
- Network attacks
- Malware classification
- System-event-based anomaly detection
- Memory forensics
- Fuzzing for software security
Cyber risk and cybersecurity: a systematic review of data availability
- Open access
- Published: 17 February 2022
- Volume 47 , pages 698–736, ( 2022 )
Cite this article
You have full access to this open access article
- Frank Cremer 1 ,
- Barry Sheehan ORCID: orcid.org/0000-0003-4592-7558 1 ,
- Michael Fortmann 2 ,
- Arash N. Kia 1 ,
- Martin Mullins 1 ,
- Finbarr Murphy 1 &
- Stefan Materne 2
62k Accesses
59 Citations
42 Altmetric
Explore all metrics
Cybercrime is estimated to have cost the global economy just under USD 1 trillion in 2020, indicating an increase of more than 50% since 2018. With the average cyber insurance claim rising from USD 145,000 in 2019 to USD 359,000 in 2020, there is a growing necessity for better cyber information sources, standardised databases, mandatory reporting and public awareness. This research analyses the extant academic and industry literature on cybersecurity and cyber risk management with a particular focus on data availability. From a preliminary search resulting in 5219 cyber peer-reviewed studies, the application of the systematic methodology resulted in 79 unique datasets. We posit that the lack of available data on cyber risk poses a serious problem for stakeholders seeking to tackle this issue. In particular, we identify a lacuna in open databases that undermine collective endeavours to better manage this set of risks. The resulting data evaluation and categorisation will support cybersecurity researchers and the insurance industry in their efforts to comprehend, metricise and manage cyber risks.
Similar content being viewed by others
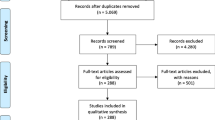
The role of artificial intelligence in healthcare: a structured literature review
Silvana Secinaro, Davide Calandra, … Paolo Biancone
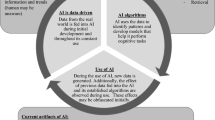
The Ethical Implications of Using Artificial Intelligence in Auditing
Ivy Munoko, Helen L. Brown-Liburd & Miklos Vasarhelyi
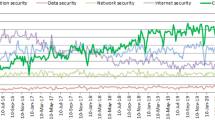
AI-Driven Cybersecurity: An Overview, Security Intelligence Modeling and Research Directions
Iqbal H. Sarker, Md Hasan Furhad & Raza Nowrozy
Avoid common mistakes on your manuscript.
Introduction
Globalisation, digitalisation and smart technologies have escalated the propensity and severity of cybercrime. Whilst it is an emerging field of research and industry, the importance of robust cybersecurity defence systems has been highlighted at the corporate, national and supranational levels. The impacts of inadequate cybersecurity are estimated to have cost the global economy USD 945 billion in 2020 (Maleks Smith et al. 2020 ). Cyber vulnerabilities pose significant corporate risks, including business interruption, breach of privacy and financial losses (Sheehan et al. 2019 ). Despite the increasing relevance for the international economy, the availability of data on cyber risks remains limited. The reasons for this are many. Firstly, it is an emerging and evolving risk; therefore, historical data sources are limited (Biener et al. 2015 ). It could also be due to the fact that, in general, institutions that have been hacked do not publish the incidents (Eling and Schnell 2016 ). The lack of data poses challenges for many areas, such as research, risk management and cybersecurity (Falco et al. 2019 ). The importance of this topic is demonstrated by the announcement of the European Council in April 2021 that a centre of excellence for cybersecurity will be established to pool investments in research, technology and industrial development. The goal of this centre is to increase the security of the internet and other critical network and information systems (European Council 2021 ).
This research takes a risk management perspective, focusing on cyber risk and considering the role of cybersecurity and cyber insurance in risk mitigation and risk transfer. The study reviews the existing literature and open data sources related to cybersecurity and cyber risk. This is the first systematic review of data availability in the general context of cyber risk and cybersecurity. By identifying and critically analysing the available datasets, this paper supports the research community by aggregating, summarising and categorising all available open datasets. In addition, further information on datasets is attached to provide deeper insights and support stakeholders engaged in cyber risk control and cybersecurity. Finally, this research paper highlights the need for open access to cyber-specific data, without price or permission barriers.
The identified open data can support cyber insurers in their efforts on sustainable product development. To date, traditional risk assessment methods have been untenable for insurance companies due to the absence of historical claims data (Sheehan et al. 2021 ). These high levels of uncertainty mean that cyber insurers are more inclined to overprice cyber risk cover (Kshetri 2018 ). Combining external data with insurance portfolio data therefore seems to be essential to improve the evaluation of the risk and thus lead to risk-adjusted pricing (Bessy-Roland et al. 2021 ). This argument is also supported by the fact that some re/insurers reported that they are working to improve their cyber pricing models (e.g. by creating or purchasing databases from external providers) (EIOPA 2018 ). Figure 1 provides an overview of pricing tools and factors considered in the estimation of cyber insurance based on the findings of EIOPA ( 2018 ) and the research of Romanosky et al. ( 2019 ). The term cyber risk refers to all cyber risks and their potential impact.
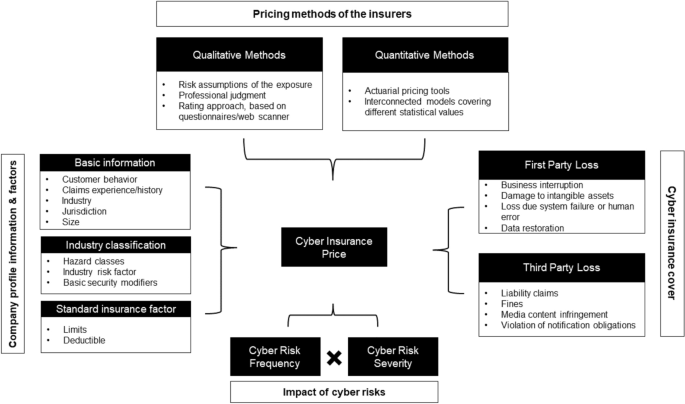
An overview of the current cyber insurance informational and methodological landscape, adapted from EIOPA ( 2018 ) and Romanosky et al. ( 2019 )
Besides the advantage of risk-adjusted pricing, the availability of open datasets helps companies benchmark their internal cyber posture and cybersecurity measures. The research can also help to improve risk awareness and corporate behaviour. Many companies still underestimate their cyber risk (Leong and Chen 2020 ). For policymakers, this research offers starting points for a comprehensive recording of cyber risks. Although in many countries, companies are obliged to report data breaches to the respective supervisory authority, this information is usually not accessible to the research community. Furthermore, the economic impact of these breaches is usually unclear.
As well as the cyber risk management community, this research also supports cybersecurity stakeholders. Researchers are provided with an up-to-date, peer-reviewed literature of available datasets showing where these datasets have been used. For example, this includes datasets that have been used to evaluate the effectiveness of countermeasures in simulated cyberattacks or to test intrusion detection systems. This reduces a time-consuming search for suitable datasets and ensures a comprehensive review of those available. Through the dataset descriptions, researchers and industry stakeholders can compare and select the most suitable datasets for their purposes. In addition, it is possible to combine the datasets from one source in the context of cybersecurity or cyber risk. This supports efficient and timely progress in cyber risk research and is beneficial given the dynamic nature of cyber risks.
Cyber risks are defined as “operational risks to information and technology assets that have consequences affecting the confidentiality, availability, and/or integrity of information or information systems” (Cebula et al. 2014 ). Prominent cyber risk events include data breaches and cyberattacks (Agrafiotis et al. 2018 ). The increasing exposure and potential impact of cyber risk have been highlighted in recent industry reports (e.g. Allianz 2021 ; World Economic Forum 2020 ). Cyberattacks on critical infrastructures are ranked 5th in the World Economic Forum's Global Risk Report. Ransomware, malware and distributed denial-of-service (DDoS) are examples of the evolving modes of a cyberattack. One example is the ransomware attack on the Colonial Pipeline, which shut down the 5500 mile pipeline system that delivers 2.5 million barrels of fuel per day and critical liquid fuel infrastructure from oil refineries to states along the U.S. East Coast (Brower and McCormick 2021 ). These and other cyber incidents have led the U.S. to strengthen its cybersecurity and introduce, among other things, a public body to analyse major cyber incidents and make recommendations to prevent a recurrence (Murphey 2021a ). Another example of the scope of cyberattacks is the ransomware NotPetya in 2017. The damage amounted to USD 10 billion, as the ransomware exploited a vulnerability in the windows system, allowing it to spread independently worldwide in the network (GAO 2021 ). In the same year, the ransomware WannaCry was launched by cybercriminals. The cyberattack on Windows software took user data hostage in exchange for Bitcoin cryptocurrency (Smart 2018 ). The victims included the National Health Service in Great Britain. As a result, ambulances were redirected to other hospitals because of information technology (IT) systems failing, leaving people in need of urgent assistance waiting. It has been estimated that 19,000 cancelled treatment appointments resulted from losses of GBP 92 million (Field 2018 ). Throughout the COVID-19 pandemic, ransomware attacks increased significantly, as working from home arrangements increased vulnerability (Murphey 2021b ).
Besides cyberattacks, data breaches can also cause high costs. Under the General Data Protection Regulation (GDPR), companies are obliged to protect personal data and safeguard the data protection rights of all individuals in the EU area. The GDPR allows data protection authorities in each country to impose sanctions and fines on organisations they find in breach. “For data breaches, the maximum fine can be €20 million or 4% of global turnover, whichever is higher” (GDPR.EU 2021 ). Data breaches often involve a large amount of sensitive data that has been accessed, unauthorised, by external parties, and are therefore considered important for information security due to their far-reaching impact (Goode et al. 2017 ). A data breach is defined as a “security incident in which sensitive, protected, or confidential data are copied, transmitted, viewed, stolen, or used by an unauthorized individual” (Freeha et al. 2021 ). Depending on the amount of data, the extent of the damage caused by a data breach can be significant, with the average cost being USD 392 million Footnote 1 (IBM Security 2020 ).
This research paper reviews the existing literature and open data sources related to cybersecurity and cyber risk, focusing on the datasets used to improve academic understanding and advance the current state-of-the-art in cybersecurity. Furthermore, important information about the available datasets is presented (e.g. use cases), and a plea is made for open data and the standardisation of cyber risk data for academic comparability and replication. The remainder of the paper is structured as follows. The next section describes the related work regarding cybersecurity and cyber risks. The third section outlines the review method used in this work and the process. The fourth section details the results of the identified literature. Further discussion is presented in the penultimate section and the final section concludes.
Related work
Due to the significance of cyber risks, several literature reviews have been conducted in this field. Eling ( 2020 ) reviewed the existing academic literature on the topic of cyber risk and cyber insurance from an economic perspective. A total of 217 papers with the term ‘cyber risk’ were identified and classified in different categories. As a result, open research questions are identified, showing that research on cyber risks is still in its infancy because of their dynamic and emerging nature. Furthermore, the author highlights that particular focus should be placed on the exchange of information between public and private actors. An improved information flow could help to measure the risk more accurately and thus make cyber risks more insurable and help risk managers to determine the right level of cyber risk for their company. In the context of cyber insurance data, Romanosky et al. ( 2019 ) analysed the underwriting process for cyber insurance and revealed how cyber insurers understand and assess cyber risks. For this research, they examined 235 American cyber insurance policies that were publicly available and looked at three components (coverage, application questionnaires and pricing). The authors state in their findings that many of the insurers used very simple, flat-rate pricing (based on a single calculation of expected loss), while others used more parameters such as the asset value of the company (or company revenue) or standard insurance metrics (e.g. deductible, limits), and the industry in the calculation. This is in keeping with Eling ( 2020 ), who states that an increased amount of data could help to make cyber risk more accurately measured and thus more insurable. Similar research on cyber insurance and data was conducted by Nurse et al. ( 2020 ). The authors examined cyber insurance practitioners' perceptions and the challenges they face in collecting and using data. In addition, gaps were identified during the research where further data is needed. The authors concluded that cyber insurance is still in its infancy, and there are still several unanswered questions (for example, cyber valuation, risk calculation and recovery). They also pointed out that a better understanding of data collection and use in cyber insurance would be invaluable for future research and practice. Bessy-Roland et al. ( 2021 ) come to a similar conclusion. They proposed a multivariate Hawkes framework to model and predict the frequency of cyberattacks. They used a public dataset with characteristics of data breaches affecting the U.S. industry. In the conclusion, the authors make the argument that an insurer has a better knowledge of cyber losses, but that it is based on a small dataset and therefore combination with external data sources seems essential to improve the assessment of cyber risks.
Several systematic reviews have been published in the area of cybersecurity (Kruse et al. 2017 ; Lee et al. 2020 ; Loukas et al. 2013 ; Ulven and Wangen 2021 ). In these papers, the authors concentrated on a specific area or sector in the context of cybersecurity. This paper adds to this extant literature by focusing on data availability and its importance to risk management and insurance stakeholders. With a priority on healthcare and cybersecurity, Kruse et al. ( 2017 ) conducted a systematic literature review. The authors identified 472 articles with the keywords ‘cybersecurity and healthcare’ or ‘ransomware’ in the databases Cumulative Index of Nursing and Allied Health Literature, PubMed and Proquest. Articles were eligible for this review if they satisfied three criteria: (1) they were published between 2006 and 2016, (2) the full-text version of the article was available, and (3) the publication is a peer-reviewed or scholarly journal. The authors found that technological development and federal policies (in the U.S.) are the main factors exposing the health sector to cyber risks. Loukas et al. ( 2013 ) conducted a review with a focus on cyber risks and cybersecurity in emergency management. The authors provided an overview of cyber risks in communication, sensor, information management and vehicle technologies used in emergency management and showed areas for which there is still no solution in the literature. Similarly, Ulven and Wangen ( 2021 ) reviewed the literature on cybersecurity risks in higher education institutions. For the literature review, the authors used the keywords ‘cyber’, ‘information threats’ or ‘vulnerability’ in connection with the terms ‘higher education, ‘university’ or ‘academia’. A similar literature review with a focus on Internet of Things (IoT) cybersecurity was conducted by Lee et al. ( 2020 ). The review revealed that qualitative approaches focus on high-level frameworks, and quantitative approaches to cybersecurity risk management focus on risk assessment and quantification of cyberattacks and impacts. In addition, the findings presented a four-step IoT cyber risk management framework that identifies, quantifies and prioritises cyber risks.
Datasets are an essential part of cybersecurity research, underlined by the following works. Ilhan Firat et al. ( 2021 ) examined various cybersecurity datasets in detail. The study was motivated by the fact that with the proliferation of the internet and smart technologies, the mode of cyberattacks is also evolving. However, in order to prevent such attacks, they must first be detected; the dissemination and further development of cybersecurity datasets is therefore critical. In their work, the authors observed studies of datasets used in intrusion detection systems. Khraisat et al. ( 2019 ) also identified a need for new datasets in the context of cybersecurity. The researchers presented a taxonomy of current intrusion detection systems, a comprehensive review of notable recent work, and an overview of the datasets commonly used for assessment purposes. In their conclusion, the authors noted that new datasets are needed because most machine-learning techniques are trained and evaluated on the knowledge of old datasets. These datasets do not contain new and comprehensive information and are partly derived from datasets from 1999. The authors noted that the core of this issue is the availability of new public datasets as well as their quality. The availability of data, how it is used, created and shared was also investigated by Zheng et al. ( 2018 ). The researchers analysed 965 cybersecurity research papers published between 2012 and 2016. They created a taxonomy of the types of data that are created and shared and then analysed the data collected via datasets. The researchers concluded that while datasets are recognised as valuable for cybersecurity research, the proportion of publicly available datasets is limited.
The main contributions of this review and what differentiates it from previous studies can be summarised as follows. First, as far as we can tell, it is the first work to summarise all available datasets on cyber risk and cybersecurity in the context of a systematic review and present them to the scientific community and cyber insurance and cybersecurity stakeholders. Second, we investigated, analysed, and made available the datasets to support efficient and timely progress in cyber risk research. And third, we enable comparability of datasets so that the appropriate dataset can be selected depending on the research area.
Methodology
Process and eligibility criteria.
The structure of this systematic review is inspired by the Preferred Reporting Items for Systematic Reviews and Meta-Analyses (PRISMA) framework (Page et al. 2021 ), and the search was conducted from 3 to 10 May 2021. Due to the continuous development of cyber risks and their countermeasures, only articles published in the last 10 years were considered. In addition, only articles published in peer-reviewed journals written in English were included. As a final criterion, only articles that make use of one or more cybersecurity or cyber risk datasets met the inclusion criteria. Specifically, these studies presented new or existing datasets, used them for methods, or used them to verify new results, as well as analysed them in an economic context and pointed out their effects. The criterion was fulfilled if it was clearly stated in the abstract that one or more datasets were used. A detailed explanation of this selection criterion can be found in the ‘Study selection’ section.
Information sources
In order to cover a complete spectrum of literature, various databases were queried to collect relevant literature on the topic of cybersecurity and cyber risks. Due to the spread of related articles across multiple databases, the literature search was limited to the following four databases for simplicity: IEEE Xplore, Scopus, SpringerLink and Web of Science. This is similar to other literature reviews addressing cyber risks or cybersecurity, including Sardi et al. ( 2021 ), Franke and Brynielsson ( 2014 ), Lagerström (2019), Eling and Schnell ( 2016 ) and Eling ( 2020 ). In this paper, all databases used in the aforementioned works were considered. However, only two studies also used all the databases listed. The IEEE Xplore database contains electrical engineering, computer science, and electronics work from over 200 journals and three million conference papers (IEEE 2021 ). Scopus includes 23,400 peer-reviewed journals from more than 5000 international publishers in the areas of science, engineering, medicine, social sciences and humanities (Scopus 2021 ). SpringerLink contains 3742 journals and indexes over 10 million scientific documents (SpringerLink 2021 ). Finally, Web of Science indexes over 9200 journals in different scientific disciplines (Science 2021 ).
A search string was created and applied to all databases. To make the search efficient and reproducible, the following search string with Boolean operator was used in all databases: cybersecurity OR cyber risk AND dataset OR database. To ensure uniformity of the search across all databases, some adjustments had to be made for the respective search engines. In Scopus, for example, the Advanced Search was used, and the field code ‘Title-ABS-KEY’ was integrated into the search string. For IEEE Xplore, the search was carried out with the Search String in the Command Search and ‘All Metadata’. In the Web of Science database, the Advanced Search was used. The special feature of this search was that it had to be carried out in individual steps. The first search was carried out with the terms cybersecurity OR cyber risk with the field tag Topic (T.S. =) and the second search with dataset OR database. Subsequently, these searches were combined, which then delivered the searched articles for review. For SpringerLink, the search string was used in the Advanced Search under the category ‘Find the resources with all of the words’. After conducting this search string, 5219 studies could be found. According to the eligibility criteria (period, language and only scientific journals), 1581 studies were identified in the databases:
Scopus: 135
Springer Link: 548
Web of Science: 534
An overview of the process is given in Fig. 2 . Combined with the results from the four databases, 854 articles without duplicates were identified.
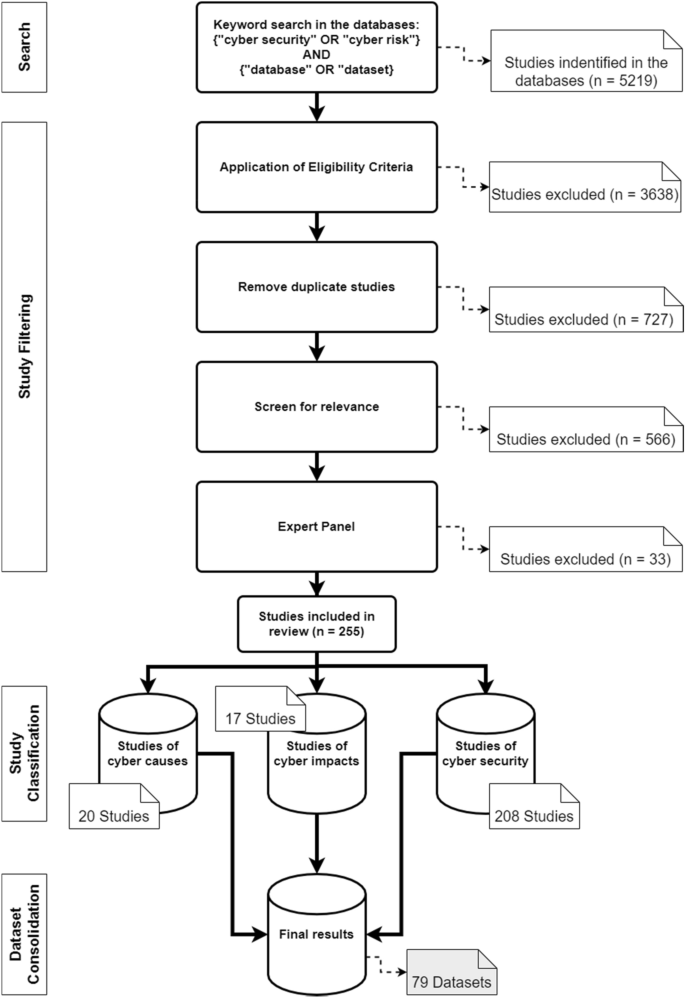
Literature search process and categorisation of the studies
Study selection
In the final step of the selection process, the articles were screened for relevance. Due to a large number of results, the abstracts were analysed in the first step of the process. The aim was to determine whether the article was relevant for the systematic review. An article fulfilled the criterion if it was recognisable in the abstract that it had made a contribution to datasets or databases with regard to cyber risks or cybersecurity. Specifically, the criterion was considered to be met if the abstract used datasets that address the causes or impacts of cyber risks, and measures in the area of cybersecurity. In this process, the number of articles was reduced to 288. The articles were then read in their entirety, and an expert panel of six people decided whether they should be used. This led to a final number of 255 articles. The years in which the articles were published and the exact number can be seen in Fig. 3 .
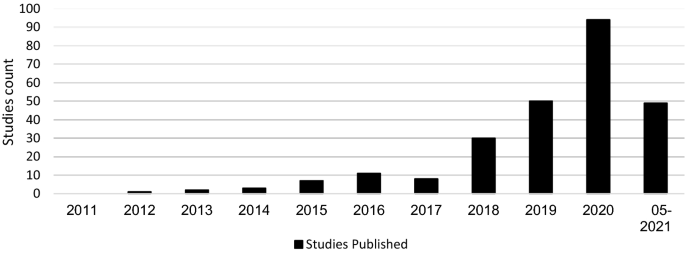
Distribution of studies
Data collection process and synthesis of the results
For the data collection process, various data were extracted from the studies, including the names of the respective creators, the name of the dataset or database and the corresponding reference. It was also determined where the data came from. In the context of accessibility, it was determined whether access is free, controlled, available for purchase or not available. It was also determined when the datasets were created and the time period referenced. The application type and domain characteristics of the datasets were identified.
This section analyses the results of the systematic literature review. The previously identified studies are divided into three categories: datasets on the causes of cyber risks, datasets on the effects of cyber risks and datasets on cybersecurity. The classification is based on the intended use of the studies. This system of classification makes it easier for stakeholders to find the appropriate datasets. The categories are evaluated individually. Although complete information is available for a large proportion of datasets, this is not true for all of them. Accordingly, the abbreviation N/A has been inserted in the respective characters to indicate that this information could not be determined by the time of submission. The term ‘use cases in the literature’ in the following and supplementary tables refers to the application areas in which the corresponding datasets were used in the literature. The areas listed there refer to the topic area on which the researchers conducted their research. Since some datasets were used interdisciplinarily, the listed use cases in the literature are correspondingly longer. Before discussing each category in the next sections, Fig. 4 provides an overview of the number of datasets found and their year of creation. Figure 5 then shows the relationship between studies and datasets in the period under consideration. Figure 6 shows the distribution of studies, their use of datasets and their creation date. The number of datasets used is higher than the number of studies because the studies often used several datasets (Table 1 ).
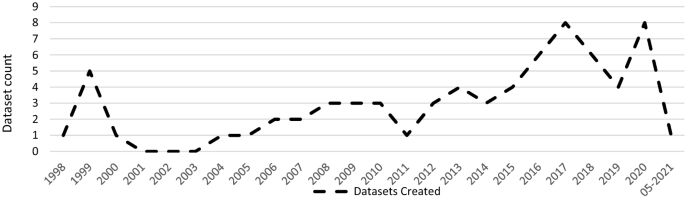
Distribution of dataset results
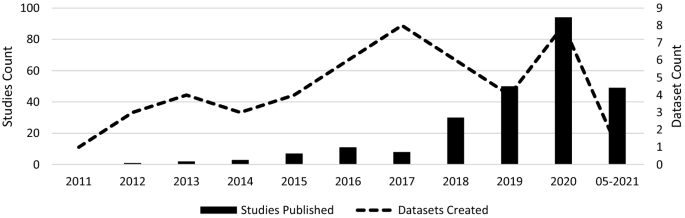
Correlation between the studies and the datasets
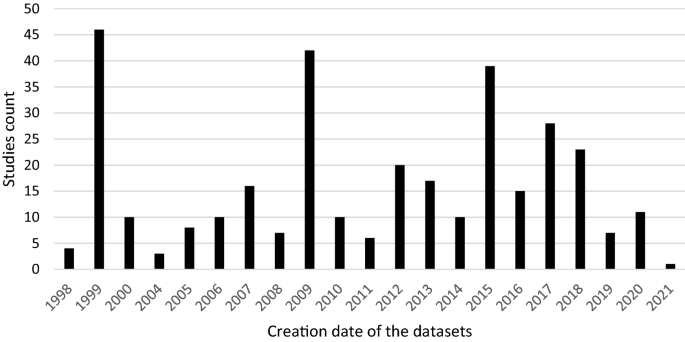
Distribution of studies and their use of datasets
Most of the datasets are generated in the U.S. (up to 58.2%). Canada and Australia rank next, with 11.3% and 5% of all the reviewed datasets, respectively.
Additionally, to create value for the datasets for the cyber insurance industry, an assessment of the applicability of each dataset has been provided for cyber insurers. This ‘Use Case Assessment’ includes the use of the data in the context of different analyses, calculation of cyber insurance premiums, and use of the information for the design of cyber insurance contracts or for additional customer services. To reasonably account for the transition of direct hyperlinks in the future, references were directed to the main websites for longevity (nearest resource point). In addition, the links to the main pages contain further information on the datasets and different versions related to the operating systems. The references were chosen in such a way that practitioners get the best overview of the respective datasets.
Case datasets
This section presents selected articles that use the datasets to analyse the causes of cyber risks. The datasets help identify emerging trends and allow pattern discovery in cyber risks. This information gives cybersecurity experts and cyber insurers the data to make better predictions and take appropriate action. For example, if certain vulnerabilities are not adequately protected, cyber insurers will demand a risk surcharge leading to an improvement in the risk-adjusted premium. Due to the capricious nature of cyber risks, existing data must be supplemented with new data sources (for example, new events, new methods or security vulnerabilities) to determine prevailing cyber exposure. The datasets of cyber risk causes could be combined with existing portfolio data from cyber insurers and integrated into existing pricing tools and factors to improve the valuation of cyber risks.
A portion of these datasets consists of several taxonomies and classifications of cyber risks. Aassal et al. ( 2020 ) propose a new taxonomy of phishing characteristics based on the interpretation and purpose of each characteristic. In comparison, Hindy et al. ( 2020 ) presented a taxonomy of network threats and the impact of current datasets on intrusion detection systems. A similar taxonomy was suggested by Kiwia et al. ( 2018 ). The authors presented a cyber kill chain-based taxonomy of banking Trojans features. The taxonomy built on a real-world dataset of 127 banking Trojans collected from December 2014 to January 2016 by a major U.K.-based financial organisation.
In the context of classification, Aamir et al. ( 2021 ) showed the benefits of machine learning for classifying port scans and DDoS attacks in a mixture of normal and attack traffic. Guo et al. ( 2020 ) presented a new method to improve malware classification based on entropy sequence features. The evaluation of this new method was conducted on different malware datasets.
To reconstruct attack scenarios and draw conclusions based on the evidence in the alert stream, Barzegar and Shajari ( 2018 ) use the DARPA2000 and MACCDC 2012 dataset for their research. Giudici and Raffinetti ( 2020 ) proposed a rank-based statistical model aimed at predicting the severity levels of cyber risk. The model used cyber risk data from the University of Milan. In contrast to the previous datasets, Skrjanc et al. ( 2018 ) used the older dataset KDD99 to monitor large-scale cyberattacks using a cauchy clustering method.
Amin et al. ( 2021 ) used a cyberattack dataset from the Canadian Institute for Cybersecurity to identify spatial clusters of countries with high rates of cyberattacks. In the context of cybercrime, Junger et al. ( 2020 ) examined crime scripts, key characteristics of the target company and the relationship between criminal effort and financial benefit. For their study, the authors analysed 300 cases of fraudulent activities against Dutch companies. With a similar focus on cybercrime, Mireles et al. ( 2019 ) proposed a metric framework to measure the effectiveness of the dynamic evolution of cyberattacks and defensive measures. To validate its usefulness, they used the DEFCON dataset.
Due to the rapidly changing nature of cyber risks, it is often impossible to obtain all information on them. Kim and Kim ( 2019 ) proposed an automated dataset generation system called CTIMiner that collects threat data from publicly available security reports and malware repositories. They released a dataset to the public containing about 640,000 records from 612 security reports published between January 2008 and 2019. A similar approach is proposed by Kim et al. ( 2020 ), using a named entity recognition system to extract core information from cyber threat reports automatically. They created a 498,000-tag dataset during their research (Ulven and Wangen 2021 ).
Within the framework of vulnerabilities and cybersecurity issues, Ulven and Wangen ( 2021 ) proposed an overview of mission-critical assets and everyday threat events, suggested a generic threat model, and summarised common cybersecurity vulnerabilities. With a focus on hospitality, Chen and Fiscus ( 2018 ) proposed several issues related to cybersecurity in this sector. They analysed 76 security incidents from the Privacy Rights Clearinghouse database. Supplementary Table 1 lists all findings that belong to the cyber causes dataset.
Impact datasets
This section outlines selected findings of the cyber impact dataset. For cyber insurers, these datasets can form an important basis for information, as they can be used to calculate cyber insurance premiums, evaluate specific cyber risks, formulate inclusions and exclusions in cyber wordings, and re-evaluate as well as supplement the data collected so far on cyber risks. For example, information on financial losses can help to better assess the loss potential of cyber risks. Furthermore, the datasets can provide insight into the frequency of occurrence of these cyber risks. The new datasets can be used to close any data gaps that were previously based on very approximate estimates or to find new results.
Eight studies addressed the costs of data breaches. For instance, Eling and Jung ( 2018 ) reviewed 3327 data breach events from 2005 to 2016 and identified an asymmetric dependence of monthly losses by breach type and industry. The authors used datasets from the Privacy Rights Clearinghouse for analysis. The Privacy Rights Clearinghouse datasets and the Breach level index database were also used by De Giovanni et al. ( 2020 ) to describe relationships between data breaches and bitcoin-related variables using the cointegration methodology. The data were obtained from the Department of Health and Human Services of healthcare facilities reporting data breaches and a national database of technical and organisational infrastructure information. Also in the context of data breaches, Algarni et al. ( 2021 ) developed a comprehensive, formal model that estimates the two components of security risks: breach cost and the likelihood of a data breach within 12 months. For their survey, the authors used two industrial reports from the Ponemon institute and VERIZON. To illustrate the scope of data breaches, Neto et al. ( 2021 ) identified 430 major data breach incidents among more than 10,000 incidents. The database created is available and covers the period 2018 to 2019.
With a direct focus on insurance, Biener et al. ( 2015 ) analysed 994 cyber loss cases from an operational risk database and investigated the insurability of cyber risks based on predefined criteria. For their study, they used data from the company SAS OpRisk Global Data. Similarly, Eling and Wirfs ( 2019 ) looked at a wide range of cyber risk events and actual cost data using the same database. They identified cyber losses and analysed them using methods from statistics and actuarial science. Using a similar reference, Farkas et al. ( 2021 ) proposed a method for analysing cyber claims based on regression trees to identify criteria for classifying and evaluating claims. Similar to Chen and Fiscus ( 2018 ), the dataset used was the Privacy Rights Clearinghouse database. Within the framework of reinsurance, Moro ( 2020 ) analysed cyber index-based information technology activity to see if index-parametric reinsurance coverage could suggest its cedant using data from a Symantec dataset.
Paté-Cornell et al. ( 2018 ) presented a general probabilistic risk analysis framework for cybersecurity in an organisation to be specified. The results are distributions of losses to cyberattacks, with and without considered countermeasures in support of risk management decisions based both on past data and anticipated incidents. The data used were from The Common Vulnerability and Exposures database and via confidential access to a database of cyberattacks on a large, U.S.-based organisation. A different conceptual framework for cyber risk classification and assessment was proposed by Sheehan et al. ( 2021 ). This framework showed the importance of proactive and reactive barriers in reducing companies’ exposure to cyber risk and quantifying the risk. Another approach to cyber risk assessment and mitigation was proposed by Mukhopadhyay et al. ( 2019 ). They estimated the probability of an attack using generalised linear models, predicted the security technology required to reduce the probability of cyberattacks, and used gamma and exponential distributions to best approximate the average loss data for each malicious attack. They also calculated the expected loss due to cyberattacks, calculated the net premium that would need to be charged by a cyber insurer, and suggested cyber insurance as a strategy to minimise losses. They used the CSI-FBI survey (1997–2010) to conduct their research.
In order to highlight the lack of data on cyber risks, Eling ( 2020 ) conducted a literature review in the areas of cyber risk and cyber insurance. Available information on the frequency, severity, and dependency structure of cyber risks was filtered out. In addition, open questions for future cyber risk research were set up. Another example of data collection on the impact of cyberattacks is provided by Sornette et al. ( 2013 ), who use a database of newspaper articles, press reports and other media to provide a predictive method to identify triggering events and potential accident scenarios and estimate their severity and frequency. A similar approach to data collection was used by Arcuri et al. ( 2020 ) to gather an original sample of global cyberattacks from newspaper reports sourced from the LexisNexis database. This collection is also used and applied to the fields of dynamic communication and cyber risk perception by Fang et al. ( 2021 ). To create a dataset of cyber incidents and disputes, Valeriano and Maness ( 2014 ) collected information on cyber interactions between rival states.
To assess trends and the scale of economic cybercrime, Levi ( 2017 ) examined datasets from different countries and their impact on crime policy. Pooser et al. ( 2018 ) investigated the trend in cyber risk identification from 2006 to 2015 and company characteristics related to cyber risk perception. The authors used a dataset of various reports from cyber insurers for their study. Walker-Roberts et al. ( 2020 ) investigated the spectrum of risk of a cybersecurity incident taking place in the cyber-physical-enabled world using the VERIS Community Database. The datasets of impacts identified are presented below. Due to overlap, some may also appear in the causes dataset (Supplementary Table 2).
Cybersecurity datasets
General intrusion detection.
General intrusion detection systems account for the largest share of countermeasure datasets. For companies or researchers focused on cybersecurity, the datasets can be used to test their own countermeasures or obtain information about potential vulnerabilities. For example, Al-Omari et al. ( 2021 ) proposed an intelligent intrusion detection model for predicting and detecting attacks in cyberspace, which was applied to dataset UNSW-NB 15. A similar approach was taken by Choras and Kozik ( 2015 ), who used machine learning to detect cyberattacks on web applications. To evaluate their method, they used the HTTP dataset CSIC 2010. For the identification of unknown attacks on web servers, Kamarudin et al. ( 2017 ) proposed an anomaly-based intrusion detection system using an ensemble classification approach. Ganeshan and Rodrigues ( 2020 ) showed an intrusion detection system approach, which clusters the database into several groups and detects the presence of intrusion in the clusters. In comparison, AlKadi et al. ( 2019 ) used a localisation-based model to discover abnormal patterns in network traffic. Hybrid models have been recommended by Bhattacharya et al. ( 2020 ) and Agrawal et al. ( 2019 ); the former is a machine-learning model based on principal component analysis for the classification of intrusion detection system datasets, while the latter is a hybrid ensemble intrusion detection system for anomaly detection using different datasets to detect patterns in network traffic that deviate from normal behaviour.
Agarwal et al. ( 2021 ) used three different machine learning algorithms in their research to find the most suitable for efficiently identifying patterns of suspicious network activity. The UNSW-NB15 dataset was used for this purpose. Kasongo and Sun ( 2020 ), Feed-Forward Deep Neural Network (FFDNN), Keshk et al. ( 2021 ), the privacy-preserving anomaly detection framework, and others also use the UNSW-NB 15 dataset as part of intrusion detection systems. The same dataset and others were used by Binbusayyis and Vaiyapuri ( 2019 ) to identify and compare key features for cyber intrusion detection. Atefinia and Ahmadi ( 2021 ) proposed a deep neural network model to reduce the false positive rate of an anomaly-based intrusion detection system. Fossaceca et al. ( 2015 ) focused in their research on the development of a framework that combined the outputs of multiple learners in order to improve the efficacy of network intrusion, and Gauthama Raman et al. ( 2020 ) presented a search algorithm based on Support Vector machine to improve the performance of the detection and false alarm rate to improve intrusion detection techniques. Ahmad and Alsemmeari ( 2020 ) targeted extreme learning machine techniques due to their good capabilities in classification problems and handling huge data. They used the NSL-KDD dataset as a benchmark.
With reference to prediction, Bakdash et al. ( 2018 ) used datasets from the U.S. Department of Defence to predict cyberattacks by malware. This dataset consists of weekly counts of cyber events over approximately seven years. Another prediction method was presented by Fan et al. ( 2018 ), which showed an improved integrated cybersecurity prediction method based on spatial-time analysis. Also, with reference to prediction, Ashtiani and Azgomi ( 2014 ) proposed a framework for the distributed simulation of cyberattacks based on high-level architecture. Kirubavathi and Anitha ( 2016 ) recommended an approach to detect botnets, irrespective of their structures, based on network traffic flow behaviour analysis and machine-learning techniques. Dwivedi et al. ( 2021 ) introduced a multi-parallel adaptive technique to utilise an adaption mechanism in the group of swarms for network intrusion detection. AlEroud and Karabatis ( 2018 ) presented an approach that used contextual information to automatically identify and query possible semantic links between different types of suspicious activities extracted from network flows.
Intrusion detection systems with a focus on IoT
In addition to general intrusion detection systems, a proportion of studies focused on IoT. Habib et al. ( 2020 ) presented an approach for converting traditional intrusion detection systems into smart intrusion detection systems for IoT networks. To enhance the process of diagnostic detection of possible vulnerabilities with an IoT system, Georgescu et al. ( 2019 ) introduced a method that uses a named entity recognition-based solution. With regard to IoT in the smart home sector, Heartfield et al. ( 2021 ) presented a detection system that is able to autonomously adjust the decision function of its underlying anomaly classification models to a smart home’s changing condition. Another intrusion detection system was suggested by Keserwani et al. ( 2021 ), which combined Grey Wolf Optimization and Particle Swam Optimization to identify various attacks for IoT networks. They used the KDD Cup 99, NSL-KDD and CICIDS-2017 to evaluate their model. Abu Al-Haija and Zein-Sabatto ( 2020 ) provide a comprehensive development of a new intelligent and autonomous deep-learning-based detection and classification system for cyberattacks in IoT communication networks that leverage the power of convolutional neural networks, abbreviated as IoT-IDCS-CNN (IoT-based Intrusion Detection and Classification System using Convolutional Neural Network). To evaluate the development, the authors used the NSL-KDD dataset. Biswas and Roy ( 2021 ) recommended a model that identifies malicious botnet traffic using novel deep-learning approaches like artificial neural networks gutted recurrent units and long- or short-term memory models. They tested their model with the Bot-IoT dataset.
With a more forensic background, Koroniotis et al. ( 2020 ) submitted a network forensic framework, which described the digital investigation phases for identifying and tracing attack behaviours in IoT networks. The suggested work was evaluated with the Bot-IoT and UINSW-NB15 datasets. With a focus on big data and IoT, Chhabra et al. ( 2020 ) presented a cyber forensic framework for big data analytics in an IoT environment using machine learning. Furthermore, the authors mentioned different publicly available datasets for machine-learning models.
A stronger focus on a mobile phones was exhibited by Alazab et al. ( 2020 ), which presented a classification model that combined permission requests and application programme interface calls. The model was tested with a malware dataset containing 27,891 Android apps. A similar approach was taken by Li et al. ( 2019a , b ), who proposed a reliable classifier for Android malware detection based on factorisation machine architecture and extraction of Android app features from manifest files and source code.
Literature reviews
In addition to the different methods and models for intrusion detection systems, various literature reviews on the methods and datasets were also found. Liu and Lang ( 2019 ) proposed a taxonomy of intrusion detection systems that uses data objects as the main dimension to classify and summarise machine learning and deep learning-based intrusion detection literature. They also presented four different benchmark datasets for machine-learning detection systems. Ahmed et al. ( 2016 ) presented an in-depth analysis of four major categories of anomaly detection techniques, which include classification, statistical, information theory and clustering. Hajj et al. ( 2021 ) gave a comprehensive overview of anomaly-based intrusion detection systems. Their article gives an overview of the requirements, methods, measurements and datasets that are used in an intrusion detection system.
Within the framework of machine learning, Chattopadhyay et al. ( 2018 ) conducted a comprehensive review and meta-analysis on the application of machine-learning techniques in intrusion detection systems. They also compared different machine learning techniques in different datasets and summarised the performance. Vidros et al. ( 2017 ) presented an overview of characteristics and methods in automatic detection of online recruitment fraud. They also published an available dataset of 17,880 annotated job ads, retrieved from the use of a real-life system. An empirical study of different unsupervised learning algorithms used in the detection of unknown attacks was presented by Meira et al. ( 2020 ).
New datasets
Kilincer et al. ( 2021 ) reviewed different intrusion detection system datasets in detail. They had a closer look at the UNS-NB15, ISCX-2012, NSL-KDD and CIDDS-001 datasets. Stojanovic et al. ( 2020 ) also provided a review on datasets and their creation for use in advanced persistent threat detection in the literature. Another review of datasets was provided by Sarker et al. ( 2020 ), who focused on cybersecurity data science as part of their research and provided an overview from a machine-learning perspective. Avila et al. ( 2021 ) conducted a systematic literature review on the use of security logs for data leak detection. They recommended a new classification of information leak, which uses the GDPR principles, identified the most widely publicly available dataset for threat detection, described the attack types in the datasets and the algorithms used for data leak detection. Tuncer et al. ( 2020 ) presented a bytecode-based detection method consisting of feature extraction using local neighbourhood binary patterns. They chose a byte-based malware dataset to investigate the performance of the proposed local neighbourhood binary pattern-based detection method. With a different focus, Mauro et al. ( 2020 ) gave an experimental overview of neural-based techniques relevant to intrusion detection. They assessed the value of neural networks using the Bot-IoT and UNSW-DB15 datasets.
Another category of results in the context of countermeasure datasets is those that were presented as new. Moreno et al. ( 2018 ) developed a database of 300 security-related accidents from European and American sources. The database contained cybersecurity-related events in the chemical and process industry. Damasevicius et al. ( 2020 ) proposed a new dataset (LITNET-2020) for network intrusion detection. The dataset is a new annotated network benchmark dataset obtained from the real-world academic network. It presents real-world examples of normal and under-attack network traffic. With a focus on IoT intrusion detection systems, Alsaedi et al. ( 2020 ) proposed a new benchmark IoT/IIot datasets for assessing intrusion detection system-enabled IoT systems. Also in the context of IoT, Vaccari et al. ( 2020 ) proposed a dataset focusing on message queue telemetry transport protocols, which can be used to train machine-learning models. To evaluate the performance of machine-learning classifiers, Mahfouz et al. ( 2020 ) created a dataset called Game Theory and Cybersecurity (GTCS). A dataset containing 22,000 malware and benign samples was constructed by Martin et al. ( 2019 ). The dataset can be used as a benchmark to test the algorithm for Android malware classification and clustering techniques. In addition, Laso et al. ( 2017 ) presented a dataset created to investigate how data and information quality estimates enable the detection of anomalies and malicious acts in cyber-physical systems. The dataset contained various cyberattacks and is publicly available.
In addition to the results described above, several other studies were found that fit into the category of countermeasures. Johnson et al. ( 2016 ) examined the time between vulnerability disclosures. Using another vulnerabilities database, Common Vulnerabilities and Exposures (CVE), Subroto and Apriyana ( 2019 ) presented an algorithm model that uses big data analysis of social media and statistical machine learning to predict cyber risks. A similar databank but with a different focus, Common Vulnerability Scoring System, was used by Chatterjee and Thekdi ( 2020 ) to present an iterative data-driven learning approach to vulnerability assessment and management for complex systems. Using the CICIDS2017 dataset to evaluate the performance, Malik et al. ( 2020 ) proposed a control plane-based orchestration for varied, sophisticated threats and attacks. The same dataset was used in another study by Lee et al. ( 2019 ), who developed an artificial security information event management system based on a combination of event profiling for data processing and different artificial network methods. To exploit the interdependence between multiple series, Fang et al. ( 2021 ) proposed a statistical framework. In order to validate the framework, the authors applied it to a dataset of enterprise-level security breaches from the Privacy Rights Clearinghouse and Identity Theft Center database. Another framework with a defensive aspect was recommended by Li et al. ( 2021 ) to increase the robustness of deep neural networks against adversarial malware evasion attacks. Sarabi et al. ( 2016 ) investigated whether and to what extent business details can help assess an organisation's risk of data breaches and the distribution of risk across different types of incidents to create policies for protection, detection and recovery from different forms of security incidents. They used data from the VERIS Community Database.
Datasets that have been classified into the cybersecurity category are detailed in Supplementary Table 3. Due to overlap, records from the previous tables may also be included.
This paper presented a systematic literature review of studies on cyber risk and cybersecurity that used datasets. Within this framework, 255 studies were fully reviewed and then classified into three different categories. Then, 79 datasets were consolidated from these studies. These datasets were subsequently analysed, and important information was selected through a process of filtering out. This information was recorded in a table and enhanced with further information as part of the literature analysis. This made it possible to create a comprehensive overview of the datasets. For example, each dataset contains a description of where the data came from and how the data has been used to date. This allows different datasets to be compared and the appropriate dataset for the use case to be selected. This research certainly has limitations, so our selection of datasets cannot necessarily be taken as a representation of all available datasets related to cyber risks and cybersecurity. For example, literature searches were conducted in four academic databases and only found datasets that were used in the literature. Many research projects also used old datasets that may no longer consider current developments. In addition, the data are often focused on only one observation and are limited in scope. For example, the datasets can only be applied to specific contexts and are also subject to further limitations (e.g. region, industry, operating system). In the context of the applicability of the datasets, it is unfortunately not possible to make a clear statement on the extent to which they can be integrated into academic or practical areas of application or how great this effort is. Finally, it remains to be pointed out that this is an overview of currently available datasets, which are subject to constant change.
Due to the lack of datasets on cyber risks in the academic literature, additional datasets on cyber risks were integrated as part of a further search. The search was conducted on the Google Dataset search portal. The search term used was ‘cyber risk datasets’. Over 100 results were found. However, due to the low significance and verifiability, only 20 selected datasets were included. These can be found in Table 2 in the “ Appendix ”.
The results of the literature review and datasets also showed that there continues to be a lack of available, open cyber datasets. This lack of data is reflected in cyber insurance, for example, as it is difficult to find a risk-based premium without a sufficient database (Nurse et al. 2020 ). The global cyber insurance market was estimated at USD 5.5 billion in 2020 (Dyson 2020 ). When compared to the USD 1 trillion global losses from cybercrime (Maleks Smith et al. 2020 ), it is clear that there exists a significant cyber risk awareness challenge for both the insurance industry and international commerce. Without comprehensive and qualitative data on cyber losses, it can be difficult to estimate potential losses from cyberattacks and price cyber insurance accordingly (GAO 2021 ). For instance, the average cyber insurance loss increased from USD 145,000 in 2019 to USD 359,000 in 2020 (FitchRatings 2021 ). Cyber insurance is an important risk management tool to mitigate the financial impact of cybercrime. This is particularly evident in the impact of different industries. In the Energy & Commodities financial markets, a ransomware attack on the Colonial Pipeline led to a substantial impact on the U.S. economy. As a result of the attack, about 45% of the U.S. East Coast was temporarily unable to obtain supplies of diesel, petrol and jet fuel. This caused the average price in the U.S. to rise 7 cents to USD 3.04 per gallon, the highest in seven years (Garber 2021 ). In addition, Colonial Pipeline confirmed that it paid a USD 4.4 million ransom to a hacker gang after the attack. Another ransomware attack occurred in the healthcare and government sector. The victim of this attack was the Irish Health Service Executive (HSE). A ransom payment of USD 20 million was demanded from the Irish government to restore services after the hack (Tidy 2021 ). In the car manufacturing sector, Miller and Valasek ( 2015 ) initiated a cyberattack that resulted in the recall of 1.4 million vehicles and cost manufacturers EUR 761 million. The risk that arises in the context of these events is the potential for the accumulation of cyber losses, which is why cyber insurers are not expanding their capacity. An example of this accumulation of cyber risks is the NotPetya malware attack, which originated in Russia, struck in Ukraine, and rapidly spread around the world, causing at least USD 10 billion in damage (GAO 2021 ). These events highlight the importance of proper cyber risk management.
This research provides cyber insurance stakeholders with an overview of cyber datasets. Cyber insurers can use the open datasets to improve their understanding and assessment of cyber risks. For example, the impact datasets can be used to better measure financial impacts and their frequencies. These data could be combined with existing portfolio data from cyber insurers and integrated with existing pricing tools and factors to better assess cyber risk valuation. Although most cyber insurers have sparse historical cyber policy and claims data, they remain too small at present for accurate prediction (Bessy-Roland et al. 2021 ). A combination of portfolio data and external datasets would support risk-adjusted pricing for cyber insurance, which would also benefit policyholders. In addition, cyber insurance stakeholders can use the datasets to identify patterns and make better predictions, which would benefit sustainable cyber insurance coverage. In terms of cyber risk cause datasets, cyber insurers can use the data to review their insurance products. For example, the data could provide information on which cyber risks have not been sufficiently considered in product design or where improvements are needed. A combination of cyber cause and cybersecurity datasets can help establish uniform definitions to provide greater transparency and clarity. Consistent terminology could lead to a more sustainable cyber market, where cyber insurers make informed decisions about the level of coverage and policyholders understand their coverage (The Geneva Association 2020).
In addition to the cyber insurance community, this research also supports cybersecurity stakeholders. The reviewed literature can be used to provide a contemporary, contextual and categorised summary of available datasets. This supports efficient and timely progress in cyber risk research and is beneficial given the dynamic nature of cyber risks. With the help of the described cybersecurity datasets and the identified information, a comparison of different datasets is possible. The datasets can be used to evaluate the effectiveness of countermeasures in simulated cyberattacks or to test intrusion detection systems.
In this paper, we conducted a systematic review of studies on cyber risk and cybersecurity databases. We found that most of the datasets are in the field of intrusion detection and machine learning and are used for technical cybersecurity aspects. The available datasets on cyber risks were relatively less represented. Due to the dynamic nature and lack of historical data, assessing and understanding cyber risk is a major challenge for cyber insurance stakeholders. To address this challenge, a greater density of cyber data is needed to support cyber insurers in risk management and researchers with cyber risk-related topics. With reference to ‘Open Science’ FAIR data (Jacobsen et al. 2020 ), mandatory reporting of cyber incidents could help improve cyber understanding, awareness and loss prevention among companies and insurers. Through greater availability of data, cyber risks can be better understood, enabling researchers to conduct more in-depth research into these risks. Companies could incorporate this new knowledge into their corporate culture to reduce cyber risks. For insurance companies, this would have the advantage that all insurers would have the same understanding of cyber risks, which would support sustainable risk-based pricing. In addition, common definitions of cyber risks could be derived from new data.
The cybersecurity databases summarised and categorised in this research could provide a different perspective on cyber risks that would enable the formulation of common definitions in cyber policies. The datasets can help companies addressing cybersecurity and cyber risk as part of risk management assess their internal cyber posture and cybersecurity measures. The paper can also help improve risk awareness and corporate behaviour, and provides the research community with a comprehensive overview of peer-reviewed datasets and other available datasets in the area of cyber risk and cybersecurity. This approach is intended to support the free availability of data for research. The complete tabulated review of the literature is included in the Supplementary Material.
This work provides directions for several paths of future work. First, there are currently few publicly available datasets for cyber risk and cybersecurity. The older datasets that are still widely used no longer reflect today's technical environment. Moreover, they can often only be used in one context, and the scope of the samples is very limited. It would be of great value if more datasets were publicly available that reflect current environmental conditions. This could help intrusion detection systems to consider current events and thus lead to a higher success rate. It could also compensate for the disadvantages of older datasets by collecting larger quantities of samples and making this contextualisation more widespread. Another area of research may be the integratability and adaptability of cybersecurity and cyber risk datasets. For example, it is often unclear to what extent datasets can be integrated or adapted to existing data. For cyber risks and cybersecurity, it would be helpful to know what requirements need to be met or what is needed to use the datasets appropriately. In addition, it would certainly be helpful to know whether datasets can be modified to be used for cyber risks or cybersecurity. Finally, the ability for stakeholders to identify machine-readable cybersecurity datasets would be useful because it would allow for even clearer delineations or comparisons between datasets. Due to the lack of publicly available datasets, concrete benchmarks often cannot be applied.
Average cost of a breach of more than 50 million records.
Aamir, M., S.S.H. Rizvi, M.A. Hashmani, M. Zubair, and J. Ahmad. 2021. Machine learning classification of port scanning and DDoS attacks: A comparative analysis. Mehran University Research Journal of Engineering and Technology 40 (1): 215–229. https://doi.org/10.22581/muet1982.2101.19 .
Article Google Scholar
Aamir, M., and S.M.A. Zaidi. 2019. DDoS attack detection with feature engineering and machine learning: The framework and performance evaluation. International Journal of Information Security 18 (6): 761–785. https://doi.org/10.1007/s10207-019-00434-1 .
Aassal, A. El, S. Baki, A. Das, and R.M. Verma. 2020. 2020. An in-depth benchmarking and evaluation of phishing detection research for security needs. IEEE Access 8: 22170–22192. https://doi.org/10.1109/ACCESS.2020.2969780 .
Abu Al-Haija, Q., and S. Zein-Sabatto. 2020. An efficient deep-learning-based detection and classification system for cyber-attacks in IoT communication networks. Electronics 9 (12): 26. https://doi.org/10.3390/electronics9122152 .
Adhikari, U., T.H. Morris, and S.Y. Pan. 2018. Applying Hoeffding adaptive trees for real-time cyber-power event and intrusion classification. IEEE Transactions on Smart Grid 9 (5): 4049–4060. https://doi.org/10.1109/tsg.2017.2647778 .
Agarwal, A., P. Sharma, M. Alshehri, A.A. Mohamed, and O. Alfarraj. 2021. Classification model for accuracy and intrusion detection using machine learning approach. PeerJ Computer Science . https://doi.org/10.7717/peerj-cs.437 .
Agrafiotis, I., J.R.C.. Nurse, M. Goldsmith, S. Creese, and D. Upton. 2018. A taxonomy of cyber-harms: Defining the impacts of cyber-attacks and understanding how they propagate. Journal of Cybersecurity 4: tyy006.
Agrawal, A., S. Mohammed, and J. Fiaidhi. 2019. Ensemble technique for intruder detection in network traffic. International Journal of Security and Its Applications 13 (3): 1–8. https://doi.org/10.33832/ijsia.2019.13.3.01 .
Ahmad, I., and R.A. Alsemmeari. 2020. Towards improving the intrusion detection through ELM (extreme learning machine). CMC Computers Materials & Continua 65 (2): 1097–1111. https://doi.org/10.32604/cmc.2020.011732 .
Ahmed, M., A.N. Mahmood, and J.K. Hu. 2016. A survey of network anomaly detection techniques. Journal of Network and Computer Applications 60: 19–31. https://doi.org/10.1016/j.jnca.2015.11.016 .
Al-Jarrah, O.Y., O. Alhussein, P.D. Yoo, S. Muhaidat, K. Taha, and K. Kim. 2016. Data randomization and cluster-based partitioning for Botnet intrusion detection. IEEE Transactions on Cybernetics 46 (8): 1796–1806. https://doi.org/10.1109/TCYB.2015.2490802 .
Al-Mhiqani, M.N., R. Ahmad, Z.Z. Abidin, W. Yassin, A. Hassan, K.H. Abdulkareem, N.S. Ali, and Z. Yunos. 2020. A review of insider threat detection: Classification, machine learning techniques, datasets, open challenges, and recommendations. Applied Sciences—Basel 10 (15): 41. https://doi.org/10.3390/app10155208 .
Al-Omari, M., M. Rawashdeh, F. Qutaishat, M. Alshira’H, and N. Ababneh. 2021. An intelligent tree-based intrusion detection model for cyber security. Journal of Network and Systems Management 29 (2): 18. https://doi.org/10.1007/s10922-021-09591-y .
Alabdallah, A., and M. Awad. 2018. Using weighted Support Vector Machine to address the imbalanced classes problem of Intrusion Detection System. KSII Transactions on Internet and Information Systems 12 (10): 5143–5158. https://doi.org/10.3837/tiis.2018.10.027 .
Alazab, M., M. Alazab, A. Shalaginov, A. Mesleh, and A. Awajan. 2020. Intelligent mobile malware detection using permission requests and API calls. Future Generation Computer Systems—the International Journal of eScience 107: 509–521. https://doi.org/10.1016/j.future.2020.02.002 .
Albahar, M.A., R.A. Al-Falluji, and M. Binsawad. 2020. An empirical comparison on malicious activity detection using different neural network-based models. IEEE Access 8: 61549–61564. https://doi.org/10.1109/ACCESS.2020.2984157 .
AlEroud, A.F., and G. Karabatis. 2018. Queryable semantics to detect cyber-attacks: A flow-based detection approach. IEEE Transactions on Systems, Man, and Cybernetics: Systems 48 (2): 207–223. https://doi.org/10.1109/TSMC.2016.2600405 .
Algarni, A.M., V. Thayananthan, and Y.K. Malaiya. 2021. Quantitative assessment of cybersecurity risks for mitigating data breaches in business systems. Applied Sciences (switzerland) . https://doi.org/10.3390/app11083678 .
Alhowaide, A., I. Alsmadi, and J. Tang. 2021. Towards the design of real-time autonomous IoT NIDS. Cluster Computing—the Journal of Networks Software Tools and Applications . https://doi.org/10.1007/s10586-021-03231-5 .
Ali, S., and Y. Li. 2019. Learning multilevel auto-encoders for DDoS attack detection in smart grid network. IEEE Access 7: 108647–108659. https://doi.org/10.1109/ACCESS.2019.2933304 .
AlKadi, O., N. Moustafa, B. Turnbull, and K.K.R. Choo. 2019. Mixture localization-based outliers models for securing data migration in cloud centers. IEEE Access 7: 114607–114618. https://doi.org/10.1109/ACCESS.2019.2935142 .
Allianz. 2021. Allianz Risk Barometer. https://www.agcs.allianz.com/content/dam/onemarketing/agcs/agcs/reports/Allianz-Risk-Barometer-2021.pdf . Accessed 15 May 2021.
Almiani, M., A. AbuGhazleh, A. Al-Rahayfeh, S. Atiewi, and Razaque, A. 2020. Deep recurrent neural network for IoT intrusion detection system. Simulation Modelling Practice and Theory 101: 102031. https://doi.org/10.1016/j.simpat.2019.102031
Alsaedi, A., N. Moustafa, Z. Tari, A. Mahmood, and A. Anwar. 2020. TON_IoT telemetry dataset: A new generation dataset of IoT and IIoT for data-driven intrusion detection systems. IEEE Access 8: 165130–165150. https://doi.org/10.1109/access.2020.3022862 .
Alsamiri, J., and K. Alsubhi. 2019. Internet of Things cyber attacks detection using machine learning. International Journal of Advanced Computer Science and Applications 10 (12): 627–634.
Alsharafat, W. 2013. Applying artificial neural network and eXtended classifier system for network intrusion detection. International Arab Journal of Information Technology 10 (3): 230–238.
Google Scholar
Amin, R.W., H.E. Sevil, S. Kocak, G. Francia III., and P. Hoover. 2021. The spatial analysis of the malicious uniform resource locators (URLs): 2016 dataset case study. Information (switzerland) 12 (1): 1–18. https://doi.org/10.3390/info12010002 .
Arcuri, M.C., L.Z. Gai, F. Ielasi, and E. Ventisette. 2020. Cyber attacks on hospitality sector: Stock market reaction. Journal of Hospitality and Tourism Technology 11 (2): 277–290. https://doi.org/10.1108/jhtt-05-2019-0080 .
Arp, D., M. Spreitzenbarth, M. Hubner, H. Gascon, K. Rieck, and C.E.R.T. Siemens. 2014. Drebin: Effective and explainable detection of android malware in your pocket. In Ndss 14: 23–26.
Ashtiani, M., and M.A. Azgomi. 2014. A distributed simulation framework for modeling cyber attacks and the evaluation of security measures. Simulation 90 (9): 1071–1102. https://doi.org/10.1177/0037549714540221 .
Atefinia, R., and M. Ahmadi. 2021. Network intrusion detection using multi-architectural modular deep neural network. Journal of Supercomputing 77 (4): 3571–3593. https://doi.org/10.1007/s11227-020-03410-y .
Avila, R., R. Khoury, R. Khoury, and F. Petrillo. 2021. Use of security logs for data leak detection: A systematic literature review. Security and Communication Networks 2021: 29. https://doi.org/10.1155/2021/6615899 .
Azeez, N.A., T.J. Ayemobola, S. Misra, R. Maskeliunas, and R. Damasevicius. 2019. Network Intrusion Detection with a Hashing Based Apriori Algorithm Using Hadoop MapReduce. Computers 8 (4): 15. https://doi.org/10.3390/computers8040086 .
Bakdash, J.Z., S. Hutchinson, E.G. Zaroukian, L.R. Marusich, S. Thirumuruganathan, C. Sample, B. Hoffman, and G. Das. 2018. Malware in the future forecasting of analyst detection of cyber events. Journal of Cybersecurity . https://doi.org/10.1093/cybsec/tyy007 .
Barletta, V.S., D. Caivano, A. Nannavecchia, and M. Scalera. 2020. Intrusion detection for in-vehicle communication networks: An unsupervised Kohonen SOM approach. Future Internet . https://doi.org/10.3390/FI12070119 .
Barzegar, M., and M. Shajari. 2018. Attack scenario reconstruction using intrusion semantics. Expert Systems with Applications 108: 119–133. https://doi.org/10.1016/j.eswa.2018.04.030 .
Bessy-Roland, Y., A. Boumezoued, and C. Hillairet. 2021. Multivariate Hawkes process for cyber insurance. Annals of Actuarial Science 15 (1): 14–39.
Bhardwaj, A., V. Mangat, and R. Vig. 2020. Hyperband tuned deep neural network with well posed stacked sparse AutoEncoder for detection of DDoS attacks in cloud. IEEE Access 8: 181916–181929. https://doi.org/10.1109/ACCESS.2020.3028690 .
Bhati, B.S., C.S. Rai, B. Balamurugan, and F. Al-Turjman. 2020. An intrusion detection scheme based on the ensemble of discriminant classifiers. Computers & Electrical Engineering 86: 9. https://doi.org/10.1016/j.compeleceng.2020.106742 .
Bhattacharya, S., S.S.R. Krishnan, P.K.R. Maddikunta, R. Kaluri, S. Singh, T.R. Gadekallu, M. Alazab, and U. Tariq. 2020. A novel PCA-firefly based XGBoost classification model for intrusion detection in networks using GPU. Electronics 9 (2): 16. https://doi.org/10.3390/electronics9020219 .
Bibi, I., A. Akhunzada, J. Malik, J. Iqbal, A. Musaddiq, and S. Kim. 2020. A dynamic DL-driven architecture to combat sophisticated android malware. IEEE Access 8: 129600–129612. https://doi.org/10.1109/ACCESS.2020.3009819 .
Biener, C., M. Eling, and J.H. Wirfs. 2015. Insurability of cyber risk: An empirical analysis. The Geneva Papers on Risk and Insurance—Issues and Practice 40 (1): 131–158. https://doi.org/10.1057/gpp.2014.19 .
Binbusayyis, A., and T. Vaiyapuri. 2019. Identifying and benchmarking key features for cyber intrusion detection: An ensemble approach. IEEE Access 7: 106495–106513. https://doi.org/10.1109/ACCESS.2019.2929487 .
Biswas, R., and S. Roy. 2021. Botnet traffic identification using neural networks. Multimedia Tools and Applications . https://doi.org/10.1007/s11042-021-10765-8 .
Bouyeddou, B., F. Harrou, B. Kadri, and Y. Sun. 2021. Detecting network cyber-attacks using an integrated statistical approach. Cluster Computing—the Journal of Networks Software Tools and Applications 24 (2): 1435–1453. https://doi.org/10.1007/s10586-020-03203-1 .
Bozkir, A.S., and M. Aydos. 2020. LogoSENSE: A companion HOG based logo detection scheme for phishing web page and E-mail brand recognition. Computers & Security 95: 18. https://doi.org/10.1016/j.cose.2020.101855 .
Brower, D., and M. McCormick. 2021. Colonial pipeline resumes operations following ransomware attack. Financial Times .
Cai, H., F. Zhang, and A. Levi. 2019. An unsupervised method for detecting shilling attacks in recommender systems by mining item relationship and identifying target items. The Computer Journal 62 (4): 579–597. https://doi.org/10.1093/comjnl/bxy124 .
Cebula, J.J., M.E. Popeck, and L.R. Young. 2014. A Taxonomy of Operational Cyber Security Risks Version 2 .
Chadza, T., K.G. Kyriakopoulos, and S. Lambotharan. 2020. Learning to learn sequential network attacks using hidden Markov models. IEEE Access 8: 134480–134497. https://doi.org/10.1109/ACCESS.2020.3011293 .
Chatterjee, S., and S. Thekdi. 2020. An iterative learning and inference approach to managing dynamic cyber vulnerabilities of complex systems. Reliability Engineering and System Safety . https://doi.org/10.1016/j.ress.2019.106664 .
Chattopadhyay, M., R. Sen, and S. Gupta. 2018. A comprehensive review and meta-analysis on applications of machine learning techniques in intrusion detection. Australasian Journal of Information Systems 22: 27.
Chen, H.S., and J. Fiscus. 2018. The inhospitable vulnerability: A need for cybersecurity risk assessment in the hospitality industry. Journal of Hospitality and Tourism Technology 9 (2): 223–234. https://doi.org/10.1108/JHTT-07-2017-0044 .
Chhabra, G.S., V.P. Singh, and M. Singh. 2020. Cyber forensics framework for big data analytics in IoT environment using machine learning. Multimedia Tools and Applications 79 (23–24): 15881–15900. https://doi.org/10.1007/s11042-018-6338-1 .
Chiba, Z., N. Abghour, K. Moussaid, A. Elomri, and M. Rida. 2019. Intelligent approach to build a Deep Neural Network based IDS for cloud environment using combination of machine learning algorithms. Computers and Security 86: 291–317. https://doi.org/10.1016/j.cose.2019.06.013 .
Choras, M., and R. Kozik. 2015. Machine learning techniques applied to detect cyber attacks on web applications. Logic Journal of the IGPL 23 (1): 45–56. https://doi.org/10.1093/jigpal/jzu038 .
Chowdhury, S., M. Khanzadeh, R. Akula, F. Zhang, S. Zhang, H. Medal, M. Marufuzzaman, and L. Bian. 2017. Botnet detection using graph-based feature clustering. Journal of Big Data 4 (1): 14. https://doi.org/10.1186/s40537-017-0074-7 .
Cost Of A Cyber Incident: Systematic Review And Cross-Validation, Cybersecurity & Infrastructure Agency , 1, https://www.cisa.gov/sites/default/files/publications/CISA-OCE_Cost_of_Cyber_Incidents_Study-FINAL_508.pdf (2020).
D’Hooge, L., T. Wauters, B. Volckaert, and F. De Turck. 2019. Classification hardness for supervised learners on 20 years of intrusion detection data. IEEE Access 7: 167455–167469. https://doi.org/10.1109/access.2019.2953451 .
Damasevicius, R., A. Venckauskas, S. Grigaliunas, J. Toldinas, N. Morkevicius, T. Aleliunas, and P. Smuikys. 2020. LITNET-2020: An annotated real-world network flow dataset for network intrusion detection. Electronics 9 (5): 23. https://doi.org/10.3390/electronics9050800 .
De Giovanni, A.L.D., and M. Pirra. 2020. On the determinants of data breaches: A cointegration analysis. Decisions in Economics and Finance . https://doi.org/10.1007/s10203-020-00301-y .
Deng, L., D. Li, X. Yao, and H. Wang. 2019. Retracted Article: Mobile network intrusion detection for IoT system based on transfer learning algorithm. Cluster Computing 22 (4): 9889–9904. https://doi.org/10.1007/s10586-018-1847-2 .
Donkal, G., and G.K. Verma. 2018. A multimodal fusion based framework to reinforce IDS for securing Big Data environment using Spark. Journal of Information Security and Applications 43: 1–11. https://doi.org/10.1016/j.jisa.2018.10.001 .
Dunn, C., N. Moustafa, and B. Turnbull. 2020. Robustness evaluations of sustainable machine learning models against data Poisoning attacks in the Internet of Things. Sustainability 12 (16): 17. https://doi.org/10.3390/su12166434 .
Dwivedi, S., M. Vardhan, and S. Tripathi. 2021. Multi-parallel adaptive grasshopper optimization technique for detecting anonymous attacks in wireless networks. Wireless Personal Communications . https://doi.org/10.1007/s11277-021-08368-5 .
Dyson, B. 2020. COVID-19 crisis could be ‘watershed’ for cyber insurance, says Swiss Re exec. https://www.spglobal.com/marketintelligence/en/news-insights/latest-news-headlines/covid-19-crisis-could-be-watershed-for-cyber-insurance-says-swiss-re-exec-59197154 . Accessed 7 May 2020.
EIOPA. 2018. Understanding cyber insurance—a structured dialogue with insurance companies. https://www.eiopa.europa.eu/sites/default/files/publications/reports/eiopa_understanding_cyber_insurance.pdf . Accessed 28 May 2018
Elijah, A.V., A. Abdullah, N.Z. JhanJhi, M. Supramaniam, and O.B. Abdullateef. 2019. Ensemble and deep-learning methods for two-class and multi-attack anomaly intrusion detection: An empirical study. International Journal of Advanced Computer Science and Applications 10 (9): 520–528.
Eling, M., and K. Jung. 2018. Copula approaches for modeling cross-sectional dependence of data breach losses. Insurance Mathematics & Economics 82: 167–180. https://doi.org/10.1016/j.insmatheco.2018.07.003 .
Eling, M., and W. Schnell. 2016. What do we know about cyber risk and cyber risk insurance? Journal of Risk Finance 17 (5): 474–491. https://doi.org/10.1108/jrf-09-2016-0122 .
Eling, M., and J. Wirfs. 2019. What are the actual costs of cyber risk events? European Journal of Operational Research 272 (3): 1109–1119. https://doi.org/10.1016/j.ejor.2018.07.021 .
Eling, M. 2020. Cyber risk research in business and actuarial science. European Actuarial Journal 10 (2): 303–333.
Elmasry, W., A. Akbulut, and A.H. Zaim. 2019. Empirical study on multiclass classification-based network intrusion detection. Computational Intelligence 35 (4): 919–954. https://doi.org/10.1111/coin.12220 .
Elsaid, S.A., and N.S. Albatati. 2020. An optimized collaborative intrusion detection system for wireless sensor networks. Soft Computing 24 (16): 12553–12567. https://doi.org/10.1007/s00500-020-04695-0 .
Estepa, R., J.E. Díaz-Verdejo, A. Estepa, and G. Madinabeitia. 2020. How much training data is enough? A case study for HTTP anomaly-based intrusion detection. IEEE Access 8: 44410–44425. https://doi.org/10.1109/ACCESS.2020.2977591 .
European Council. 2021. Cybersecurity: how the EU tackles cyber threats. https://www.consilium.europa.eu/en/policies/cybersecurity/ . Accessed 10 May 2021
Falco, G. et al. 2019. Cyber risk research impeded by disciplinary barriers. Science (American Association for the Advancement of Science) 366 (6469): 1066–1069.
Fan, Z.J., Z.P. Tan, C.X. Tan, and X. Li. 2018. An improved integrated prediction method of cyber security situation based on spatial-time analysis. Journal of Internet Technology 19 (6): 1789–1800. https://doi.org/10.3966/160792642018111906015 .
Fang, Z.J., M.C. Xu, S.H. Xu, and T.Z. Hu. 2021. A framework for predicting data breach risk: Leveraging dependence to cope with sparsity. IEEE Transactions on Information Forensics and Security 16: 2186–2201. https://doi.org/10.1109/tifs.2021.3051804 .
Farkas, S., O. Lopez, and M. Thomas. 2021. Cyber claim analysis using Generalized Pareto regression trees with applications to insurance. Insurance: Mathematics and Economics 98: 92–105. https://doi.org/10.1016/j.insmatheco.2021.02.009 .
Farsi, H., A. Fanian, and Z. Taghiyarrenani. 2019. A novel online state-based anomaly detection system for process control networks. International Journal of Critical Infrastructure Protection 27: 11. https://doi.org/10.1016/j.ijcip.2019.100323 .
Ferrag, M.A., L. Maglaras, S. Moschoyiannis, and H. Janicke. 2020. Deep learning for cyber security intrusion detection: Approaches, datasets, and comparative study. Journal of Information Security and Applications 50: 19. https://doi.org/10.1016/j.jisa.2019.102419 .
Field, M. 2018. WannaCry cyber attack cost the NHS £92m as 19,000 appointments cancelled. https://www.telegraph.co.uk/technology/2018/10/11/wannacry-cyber-attack-cost-nhs-92m-19000-appointments-cancelled/ . Accessed 9 May 2018.
FitchRatings. 2021. U.S. Cyber Insurance Market Update (Spike in Claims Leads to Decline in 2020 Underwriting Performance). https://www.fitchratings.com/research/insurance/us-cyber-insurance-market-update-spike-in-claims-leads-to-decline-in-2020-underwriting-performance-26-05-2021 .
Fossaceca, J.M., T.A. Mazzuchi, and S. Sarkani. 2015. MARK-ELM: Application of a novel Multiple Kernel Learning framework for improving the robustness of network intrusion detection. Expert Systems with Applications 42 (8): 4062–4080. https://doi.org/10.1016/j.eswa.2014.12.040 .
Franke, U., and J. Brynielsson. 2014. Cyber situational awareness–a systematic review of the literature. Computers & security 46: 18–31.
Freeha, K., K.J. Hwan, M. Lars, and M. Robin. 2021. Data breach management: An integrated risk model. Information & Management 58 (1): 103392. https://doi.org/10.1016/j.im.2020.103392 .
Ganeshan, R., and P. Rodrigues. 2020. Crow-AFL: Crow based adaptive fractional lion optimization approach for the intrusion detection. Wireless Personal Communications 111 (4): 2065–2089. https://doi.org/10.1007/s11277-019-06972-0 .
GAO. 2021. CYBER INSURANCE—Insurers and policyholders face challenges in an evolving market. https://www.gao.gov/assets/gao-21-477.pdf . Accessed 16 May 2021.
Garber, J. 2021. Colonial Pipeline fiasco foreshadows impact of Biden energy policy. https://www.foxbusiness.com/markets/colonial-pipeline-fiasco-foreshadows-impact-of-biden-energy-policy . Accessed 4 May 2021.
Gauthama Raman, M.R., N. Somu, S. Jagarapu, T. Manghnani, T. Selvam, K. Krithivasan, and V.S. Shankar Sriram. 2020. An efficient intrusion detection technique based on support vector machine and improved binary gravitational search algorithm. Artificial Intelligence Review 53 (5): 3255–3286. https://doi.org/10.1007/s10462-019-09762-z .
Gavel, S., A.S. Raghuvanshi, and S. Tiwari. 2021. Distributed intrusion detection scheme using dual-axis dimensionality reduction for Internet of things (IoT). Journal of Supercomputing . https://doi.org/10.1007/s11227-021-03697-5 .
GDPR.EU. 2021. FAQ. https://gdpr.eu/faq/ . Accessed 10 May 2021.
Georgescu, T.M., B. Iancu, and M. Zurini. 2019. Named-entity-recognition-based automated system for diagnosing cybersecurity situations in IoT networks. Sensors (switzerland) . https://doi.org/10.3390/s19153380 .
Giudici, P., and E. Raffinetti. 2020. Cyber risk ordering with rank-based statistical models. AStA Advances in Statistical Analysis . https://doi.org/10.1007/s10182-020-00387-0 .
Goh, J., S. Adepu, K.N. Junejo, and A. Mathur. 2016. A dataset to support research in the design of secure water treatment systems. In CRITIS.
Gong, X.Y., J.L. Lu, Y.F. Zhou, H. Qiu, and R. He. 2021. Model uncertainty based annotation error fixing for web attack detection. Journal of Signal Processing Systems for Signal Image and Video Technology 93 (2–3): 187–199. https://doi.org/10.1007/s11265-019-01494-1 .
Goode, S., H. Hoehle, V. Venkatesh, and S.A. Brown. 2017. USER compensation as a data breach recovery action: An investigation of the sony playstation network breach. MIS Quarterly 41 (3): 703–727.
Guo, H., S. Huang, C. Huang, Z. Pan, M. Zhang, and F. Shi. 2020. File entropy signal analysis combined with wavelet decomposition for malware classification. IEEE Access 8: 158961–158971. https://doi.org/10.1109/ACCESS.2020.3020330 .
Habib, M., I. Aljarah, and H. Faris. 2020. A Modified multi-objective particle swarm optimizer-based Lévy flight: An approach toward intrusion detection in Internet of Things. Arabian Journal for Science and Engineering 45 (8): 6081–6108. https://doi.org/10.1007/s13369-020-04476-9 .
Hajj, S., R. El Sibai, J.B. Abdo, J. Demerjian, A. Makhoul, and C. Guyeux. 2021. Anomaly-based intrusion detection systems: The requirements, methods, measurements, and datasets. Transactions on Emerging Telecommunications Technologies 32 (4): 36. https://doi.org/10.1002/ett.4240 .
Heartfield, R., G. Loukas, A. Bezemskij, and E. Panaousis. 2021. Self-configurable cyber-physical intrusion detection for smart homes using reinforcement learning. IEEE Transactions on Information Forensics and Security 16: 1720–1735. https://doi.org/10.1109/tifs.2020.3042049 .
Hemo, B., T. Gafni, K. Cohen, and Q. Zhao. 2020. Searching for anomalies over composite hypotheses. IEEE Transactions on Signal Processing 68: 1181–1196. https://doi.org/10.1109/TSP.2020.2971438
Hindy, H., D. Brosset, E. Bayne, A.K. Seeam, C. Tachtatzis, R. Atkinson, and X. Bellekens. 2020. A taxonomy of network threats and the effect of current datasets on intrusion detection systems. IEEE Access 8: 104650–104675. https://doi.org/10.1109/ACCESS.2020.3000179 .
Hong, W., D. Huang, C. Chen, and J. Lee. 2020. Towards accurate and efficient classification of power system contingencies and cyber-attacks using recurrent neural networks. IEEE Access 8: 123297–123309. https://doi.org/10.1109/ACCESS.2020.3007609 .
Husák, M., M. Zádník, V. Bartos, and P. Sokol. 2020. Dataset of intrusion detection alerts from a sharing platform. Data in Brief 33: 106530.
IBM Security. 2020. Cost of a Data breach Report. https://www.capita.com/sites/g/files/nginej291/files/2020-08/Ponemon-Global-Cost-of-Data-Breach-Study-2020.pdf . Accessed 19 May 2021.
IEEE. 2021. IEEE Quick Facts. https://www.ieee.org/about/at-a-glance.html . Accessed 11 May 2021.
Kilincer, I.F., F. Ertam, and S. Abdulkadir. 2021. Machine learning methods for cyber security intrusion detection: Datasets and comparative study. Computer Networks 188: 107840. https://doi.org/10.1016/j.comnet.2021.107840 .
Jaber, A.N., and S. Ul Rehman. 2020. FCM-SVM based intrusion detection system for cloud computing environment. Cluster Computing—the Journal of Networks Software Tools and Applications 23 (4): 3221–3231. https://doi.org/10.1007/s10586-020-03082-6 .
Jacobs, J., S. Romanosky, B. Edwards, M. Roytman, and I. Adjerid. 2019. Exploit prediction scoring system (epss). arXiv:1908.04856
Jacobsen, A. et al. 2020. FAIR principles: Interpretations and implementation considerations. Data Intelligence 2 (1–2): 10–29. https://doi.org/10.1162/dint_r_00024 .
Jahromi, A.N., S. Hashemi, A. Dehghantanha, R.M. Parizi, and K.K.R. Choo. 2020. An enhanced stacked LSTM method with no random initialization for malware threat hunting in safety and time-critical systems. IEEE Transactions on Emerging Topics in Computational Intelligence 4 (5): 630–640. https://doi.org/10.1109/TETCI.2019.2910243 .
Jang, S., S. Li, and Y. Sung. 2020. FastText-based local feature visualization algorithm for merged image-based malware classification framework for cyber security and cyber defense. Mathematics 8 (3): 13. https://doi.org/10.3390/math8030460 .
Javeed, D., T.H. Gao, and M.T. Khan. 2021. SDN-enabled hybrid DL-driven framework for the detection of emerging cyber threats in IoT. Electronics 10 (8): 16. https://doi.org/10.3390/electronics10080918 .
Johnson, P., D. Gorton, R. Lagerstrom, and M. Ekstedt. 2016. Time between vulnerability disclosures: A measure of software product vulnerability. Computers & Security 62: 278–295. https://doi.org/10.1016/j.cose.2016.08.004 .
Johnson, P., R. Lagerström, M. Ekstedt, and U. Franke. 2018. Can the common vulnerability scoring system be trusted? A Bayesian analysis. IEEE Transactions on Dependable and Secure Computing 15 (6): 1002–1015. https://doi.org/10.1109/TDSC.2016.2644614 .
Junger, M., V. Wang, and M. Schlömer. 2020. Fraud against businesses both online and offline: Crime scripts, business characteristics, efforts, and benefits. Crime Science 9 (1): 13. https://doi.org/10.1186/s40163-020-00119-4 .
Kalutarage, H.K., H.N. Nguyen, and S.A. Shaikh. 2017. Towards a threat assessment framework for apps collusion. Telecommunication Systems 66 (3): 417–430. https://doi.org/10.1007/s11235-017-0296-1 .
Kamarudin, M.H., C. Maple, T. Watson, and N.S. Safa. 2017. A LogitBoost-based algorithm for detecting known and unknown web attacks. IEEE Access 5: 26190–26200. https://doi.org/10.1109/ACCESS.2017.2766844 .
Kasongo, S.M., and Y.X. Sun. 2020. A deep learning method with wrapper based feature extraction for wireless intrusion detection system. Computers & Security 92: 15. https://doi.org/10.1016/j.cose.2020.101752 .
Keserwani, P.K., M.C. Govil, E.S. Pilli, and P. Govil. 2021. A smart anomaly-based intrusion detection system for the Internet of Things (IoT) network using GWO–PSO–RF model. Journal of Reliable Intelligent Environments 7 (1): 3–21. https://doi.org/10.1007/s40860-020-00126-x .
Keshk, M., E. Sitnikova, N. Moustafa, J. Hu, and I. Khalil. 2021. An integrated framework for privacy-preserving based anomaly detection for cyber-physical systems. IEEE Transactions on Sustainable Computing 6 (1): 66–79. https://doi.org/10.1109/TSUSC.2019.2906657 .
Khan, I.A., D.C. Pi, A.K. Bhatia, N. Khan, W. Haider, and A. Wahab. 2020. Generating realistic IoT-based IDS dataset centred on fuzzy qualitative modelling for cyber-physical systems. Electronics Letters 56 (9): 441–443. https://doi.org/10.1049/el.2019.4158 .
Khraisat, A., I. Gondal, P. Vamplew, J. Kamruzzaman, and A. Alazab. 2020. Hybrid intrusion detection system based on the stacking ensemble of C5 decision tree classifier and one class support vector machine. Electronics 9 (1): 18. https://doi.org/10.3390/electronics9010173 .
Khraisat, A., I. Gondal, P. Vamplew, and J. Kamruzzaman. 2019. Survey of intrusion detection systems: Techniques, datasets and challenges. Cybersecurity 2 (1): 20. https://doi.org/10.1186/s42400-019-0038-7 .
Kilincer, I.F., F. Ertam, and A. Sengur. 2021. Machine learning methods for cyber security intrusion detection: Datasets and comparative study. Computer Networks 188: 16. https://doi.org/10.1016/j.comnet.2021.107840 .
Kim, D., and H.K. Kim. 2019. Automated dataset generation system for collaborative research of cyber threat analysis. Security and Communication Networks 2019: 10. https://doi.org/10.1155/2019/6268476 .
Kim, G., C. Lee, J. Jo, and H. Lim. 2020. Automatic extraction of named entities of cyber threats using a deep Bi-LSTM-CRF network. International Journal of Machine Learning and Cybernetics 11 (10): 2341–2355. https://doi.org/10.1007/s13042-020-01122-6 .
Kirubavathi, G., and R. Anitha. 2016. Botnet detection via mining of traffic flow characteristics. Computers & Electrical Engineering 50: 91–101. https://doi.org/10.1016/j.compeleceng.2016.01.012 .
Kiwia, D., A. Dehghantanha, K.K.R. Choo, and J. Slaughter. 2018. A cyber kill chain based taxonomy of banking Trojans for evolutionary computational intelligence. Journal of Computational Science 27: 394–409. https://doi.org/10.1016/j.jocs.2017.10.020 .
Koroniotis, N., N. Moustafa, and E. Sitnikova. 2020. A new network forensic framework based on deep learning for Internet of Things networks: A particle deep framework. Future Generation Computer Systems 110: 91–106. https://doi.org/10.1016/j.future.2020.03.042 .
Kruse, C.S., B. Frederick, T. Jacobson, and D. Kyle Monticone. 2017. Cybersecurity in healthcare: A systematic review of modern threats and trends. Technology and Health Care 25 (1): 1–10.
Kshetri, N. 2018. The economics of cyber-insurance. IT Professional 20 (6): 9–14. https://doi.org/10.1109/MITP.2018.2874210 .
Kumar, R., P. Kumar, R. Tripathi, G.P. Gupta, T.R. Gadekallu, and G. Srivastava. 2021. SP2F: A secured privacy-preserving framework for smart agricultural Unmanned Aerial Vehicles. Computer Networks . https://doi.org/10.1016/j.comnet.2021.107819 .
Kumar, R., and R. Tripathi. 2021. DBTP2SF: A deep blockchain-based trustworthy privacy-preserving secured framework in industrial internet of things systems. Transactions on Emerging Telecommunications Technologies 32 (4): 27. https://doi.org/10.1002/ett.4222 .
Laso, P.M., D. Brosset, and J. Puentes. 2017. Dataset of anomalies and malicious acts in a cyber-physical subsystem. Data in Brief 14: 186–191. https://doi.org/10.1016/j.dib.2017.07.038 .
Lee, J., J. Kim, I. Kim, and K. Han. 2019. Cyber threat detection based on artificial neural networks using event profiles. IEEE Access 7: 165607–165626. https://doi.org/10.1109/ACCESS.2019.2953095 .
Lee, S.J., P.D. Yoo, A.T. Asyhari, Y. Jhi, L. Chermak, C.Y. Yeun, and K. Taha. 2020. IMPACT: Impersonation attack detection via edge computing using deep Autoencoder and feature abstraction. IEEE Access 8: 65520–65529. https://doi.org/10.1109/ACCESS.2020.2985089 .
Leong, Y.-Y., and Y.-C. Chen. 2020. Cyber risk cost and management in IoT devices-linked health insurance. The Geneva Papers on Risk and Insurance—Issues and Practice 45 (4): 737–759. https://doi.org/10.1057/s41288-020-00169-4 .
Levi, M. 2017. Assessing the trends, scale and nature of economic cybercrimes: overview and Issues: In Cybercrimes, cybercriminals and their policing, in crime, law and social change. Crime, Law and Social Change 67 (1): 3–20. https://doi.org/10.1007/s10611-016-9645-3 .
Li, C., K. Mills, D. Niu, R. Zhu, H. Zhang, and H. Kinawi. 2019a. Android malware detection based on factorization machine. IEEE Access 7: 184008–184019. https://doi.org/10.1109/ACCESS.2019.2958927 .
Li, D.Q., and Q.M. Li. 2020. Adversarial deep ensemble: evasion attacks and defenses for malware detection. IEEE Transactions on Information Forensics and Security 15: 3886–3900. https://doi.org/10.1109/tifs.2020.3003571 .
Li, D.Q., Q.M. Li, Y.F. Ye, and S.H. Xu. 2021. A framework for enhancing deep neural networks against adversarial malware. IEEE Transactions on Network Science and Engineering 8 (1): 736–750. https://doi.org/10.1109/tnse.2021.3051354 .
Li, R.H., C. Zhang, C. Feng, X. Zhang, and C.J. Tang. 2019b. Locating vulnerability in binaries using deep neural networks. IEEE Access 7: 134660–134676. https://doi.org/10.1109/access.2019.2942043 .
Li, X., M. Xu, P. Vijayakumar, N. Kumar, and X. Liu. 2020. Detection of low-frequency and multi-stage attacks in industrial Internet of Things. IEEE Transactions on Vehicular Technology 69 (8): 8820–8831. https://doi.org/10.1109/TVT.2020.2995133 .
Liu, H.Y., and B. Lang. 2019. Machine learning and deep learning methods for intrusion detection systems: A survey. Applied Sciences—Basel 9 (20): 28. https://doi.org/10.3390/app9204396 .
Lopez-Martin, M., B. Carro, and A. Sanchez-Esguevillas. 2020. Application of deep reinforcement learning to intrusion detection for supervised problems. Expert Systems with Applications . https://doi.org/10.1016/j.eswa.2019.112963 .
Loukas, G., D. Gan, and Tuan Vuong. 2013. A review of cyber threats and defence approaches in emergency management. Future Internet 5: 205–236.
Luo, C.C., S. Su, Y.B. Sun, Q.J. Tan, M. Han, and Z.H. Tian. 2020. A convolution-based system for malicious URLs detection. CMC—Computers Materials Continua 62 (1): 399–411.
Mahbooba, B., M. Timilsina, R. Sahal, and M. Serrano. 2021. Explainable artificial intelligence (XAI) to enhance trust management in intrusion detection systems using decision tree model. Complexity 2021: 11. https://doi.org/10.1155/2021/6634811 .
Mahdavifar, S., and A.A. Ghorbani. 2020. DeNNeS: Deep embedded neural network expert system for detecting cyber attacks. Neural Computing & Applications 32 (18): 14753–14780. https://doi.org/10.1007/s00521-020-04830-w .
Mahfouz, A., A. Abuhussein, D. Venugopal, and S. Shiva. 2020. Ensemble classifiers for network intrusion detection using a novel network attack dataset. Future Internet 12 (11): 1–19. https://doi.org/10.3390/fi12110180 .
Maleks Smith, Z., E. Lostri, and J.A. Lewis. 2020. The hidden costs of cybercrime. https://www.mcafee.com/enterprise/en-us/assets/reports/rp-hidden-costs-of-cybercrime.pdf . Accessed 16 May 2021.
Malik, J., A. Akhunzada, I. Bibi, M. Imran, A. Musaddiq, and S.W. Kim. 2020. Hybrid deep learning: An efficient reconnaissance and surveillance detection mechanism in SDN. IEEE Access 8: 134695–134706. https://doi.org/10.1109/ACCESS.2020.3009849 .
Manimurugan, S. 2020. IoT-Fog-Cloud model for anomaly detection using improved Naive Bayes and principal component analysis. Journal of Ambient Intelligence and Humanized Computing . https://doi.org/10.1007/s12652-020-02723-3 .
Martin, A., R. Lara-Cabrera, and D. Camacho. 2019. Android malware detection through hybrid features fusion and ensemble classifiers: The AndroPyTool framework and the OmniDroid dataset. Information Fusion 52: 128–142. https://doi.org/10.1016/j.inffus.2018.12.006 .
Mauro, M.D., G. Galatro, and A. Liotta. 2020. Experimental review of neural-based approaches for network intrusion management. IEEE Transactions on Network and Service Management 17 (4): 2480–2495. https://doi.org/10.1109/TNSM.2020.3024225 .
McLeod, A., and D. Dolezel. 2018. Cyber-analytics: Modeling factors associated with healthcare data breaches. Decision Support Systems 108: 57–68. https://doi.org/10.1016/j.dss.2018.02.007 .
Meira, J., R. Andrade, I. Praca, J. Carneiro, V. Bolon-Canedo, A. Alonso-Betanzos, and G. Marreiros. 2020. Performance evaluation of unsupervised techniques in cyber-attack anomaly detection. Journal of Ambient Intelligence and Humanized Computing 11 (11): 4477–4489. https://doi.org/10.1007/s12652-019-01417-9 .
Miao, Y., J. Ma, X. Liu, J. Weng, H. Li, and H. Li. 2019. Lightweight fine-grained search over encrypted data in Fog computing. IEEE Transactions on Services Computing 12 (5): 772–785. https://doi.org/10.1109/TSC.2018.2823309 .
Miller, C., and C. Valasek. 2015. Remote exploitation of an unaltered passenger vehicle. Black Hat USA 2015 (S 91).
Mireles, J.D., E. Ficke, J.H. Cho, P. Hurley, and S.H. Xu. 2019. Metrics towards measuring cyber agility. IEEE Transactions on Information Forensics and Security 14 (12): 3217–3232. https://doi.org/10.1109/tifs.2019.2912551 .
Mishra, N., and S. Pandya. 2021. Internet of Things applications, security challenges, attacks, intrusion detection, and future visions: A systematic review. IEEE Access . https://doi.org/10.1109/ACCESS.2021.3073408 .
Monshizadeh, M., V. Khatri, B.G. Atli, R. Kantola, and Z. Yan. 2019. Performance evaluation of a combined anomaly detection platform. IEEE Access 7: 100964–100978. https://doi.org/10.1109/ACCESS.2019.2930832 .
Moreno, V.C., G. Reniers, E. Salzano, and V. Cozzani. 2018. Analysis of physical and cyber security-related events in the chemical and process industry. Process Safety and Environmental Protection 116: 621–631. https://doi.org/10.1016/j.psep.2018.03.026 .
Moro, E.D. 2020. Towards an economic cyber loss index for parametric cover based on IT security indicator: A preliminary analysis. Risks . https://doi.org/10.3390/risks8020045 .
Moustafa, N., E. Adi, B. Turnbull, and J. Hu. 2018. A new threat intelligence scheme for safeguarding industry 4.0 systems. IEEE Access 6: 32910–32924. https://doi.org/10.1109/ACCESS.2018.2844794 .
Moustakidis, S., and P. Karlsson. 2020. A novel feature extraction methodology using Siamese convolutional neural networks for intrusion detection. Cybersecurity . https://doi.org/10.1186/s42400-020-00056-4 .
Mukhopadhyay, A., S. Chatterjee, K.K. Bagchi, P.J. Kirs, and G.K. Shukla. 2019. Cyber Risk Assessment and Mitigation (CRAM) framework using Logit and Probit models for cyber insurance. Information Systems Frontiers 21 (5): 997–1018. https://doi.org/10.1007/s10796-017-9808-5 .
Murphey, H. 2021a. Biden signs executive order to strengthen US cyber security. https://www.ft.com/content/4d808359-b504-4014-85f6-68e7a2851bf1?accessToken=zwAAAXl0_ifgkc9NgINZtQRAFNOF9mjnooUb8Q.MEYCIQDw46SFWsMn1iyuz3kvgAmn6mxc0rIVfw10Lg1ovJSfJwIhAK2X2URzfSqHwIS7ddRCvSt2nGC2DcdoiDTG49-4TeEt&sharetype=gift?token=fbcd6323-1ecf-4fc3-b136-b5b0dd6a8756 . Accessed 7 May 2021.
Murphey, H. 2021b. Millions of connected devices have security flaws, study shows. https://www.ft.com/content/0bf92003-926d-4dee-87d7-b01f7c3e9621?accessToken=zwAAAXnA7f2Ikc8L-SADkm1N7tOH17AffD6WIQ.MEQCIDjBuROvhmYV0Mx3iB0cEV7m5oND1uaCICxJu0mzxM0PAiBam98q9zfHiTB6hKGr1gGl0Azt85yazdpX9K5sI8se3Q&sharetype=gift?token=2538218d-77d9-4dd3-9649-3cb556a34e51 . Accessed 6 May 2021.
Murugesan, V., M. Shalinie, and M.H. Yang. 2018. Design and analysis of hybrid single packet IP traceback scheme. IET Networks 7 (3): 141–151. https://doi.org/10.1049/iet-net.2017.0115 .
Mwitondi, K.S., and S.A. Zargari. 2018. An iterative multiple sampling method for intrusion detection. Information Security Journal 27 (4): 230–239. https://doi.org/10.1080/19393555.2018.1539790 .
Neto, N.N., S. Madnick, A.M.G. De Paula, and N.M. Borges. 2021. Developing a global data breach database and the challenges encountered. ACM Journal of Data and Information Quality 13 (1): 33. https://doi.org/10.1145/3439873 .
Nurse, J.R.C., L. Axon, A. Erola, I. Agrafiotis, M. Goldsmith, and S. Creese. 2020. The data that drives cyber insurance: A study into the underwriting and claims processes. In 2020 International conference on cyber situational awareness, data analytics and assessment (CyberSA), 15–19 June 2020.
Oliveira, N., I. Praca, E. Maia, and O. Sousa. 2021. Intelligent cyber attack detection and classification for network-based intrusion detection systems. Applied Sciences—Basel 11 (4): 21. https://doi.org/10.3390/app11041674 .
Page, M.J. et al. 2021. The PRISMA 2020 statement: An updated guideline for reporting systematic reviews. Systematic Reviews 10 (1): 89. https://doi.org/10.1186/s13643-021-01626-4 .
Pajouh, H.H., R. Javidan, R. Khayami, A. Dehghantanha, and K.R. Choo. 2019. A two-layer dimension reduction and two-tier classification model for anomaly-based intrusion detection in IoT backbone networks. IEEE Transactions on Emerging Topics in Computing 7 (2): 314–323. https://doi.org/10.1109/TETC.2016.2633228 .
Parra, G.D., P. Rad, K.K.R. Choo, and N. Beebe. 2020. Detecting Internet of Things attacks using distributed deep learning. Journal of Network and Computer Applications 163: 13. https://doi.org/10.1016/j.jnca.2020.102662 .
Paté-Cornell, M.E., M. Kuypers, M. Smith, and P. Keller. 2018. Cyber risk management for critical infrastructure: A risk analysis model and three case studies. Risk Analysis 38 (2): 226–241. https://doi.org/10.1111/risa.12844 .
Pooser, D.M., M.J. Browne, and O. Arkhangelska. 2018. Growth in the perception of cyber risk: evidence from U.S. P&C Insurers. The Geneva Papers on Risk and Insurance—Issues and Practice 43 (2): 208–223. https://doi.org/10.1057/s41288-017-0077-9 .
Pu, G., L. Wang, J. Shen, and F. Dong. 2021. A hybrid unsupervised clustering-based anomaly detection method. Tsinghua Science and Technology 26 (2): 146–153. https://doi.org/10.26599/TST.2019.9010051 .
Qiu, J., W. Luo, L. Pan, Y. Tai, J. Zhang, and Y. Xiang. 2019. Predicting the impact of android malicious samples via machine learning. IEEE Access 7: 66304–66316. https://doi.org/10.1109/ACCESS.2019.2914311 .
Qu, X., L. Yang, K. Guo, M. Sun, L. Ma, T. Feng, S. Ren, K. Li, and X. Ma. 2020. Direct batch growth hierarchical self-organizing mapping based on statistics for efficient network intrusion detection. IEEE Access 8: 42251–42260. https://doi.org/10.1109/ACCESS.2020.2976810 .
Rahman, Md.S., S. Halder, Md. Ashraf Uddin, and U.K. Acharjee. 2021. An efficient hybrid system for anomaly detection in social networks. Cybersecurity 4 (1): 10. https://doi.org/10.1186/s42400-021-00074-w .
Ramaiah, M., V. Chandrasekaran, V. Ravi, and N. Kumar. 2021. An intrusion detection system using optimized deep neural network architecture. Transactions on Emerging Telecommunications Technologies 32 (4): 17. https://doi.org/10.1002/ett.4221 .
Raman, M.R.G., K. Kannan, S.K. Pal, and V.S.S. Sriram. 2016. Rough set-hypergraph-based feature selection approach for intrusion detection systems. Defence Science Journal 66 (6): 612–617. https://doi.org/10.14429/dsj.66.10802 .
Rathore, S., J.H. Park. 2018. Semi-supervised learning based distributed attack detection framework for IoT. Applied Soft Computing 72: 79–89. https://doi.org/10.1016/j.asoc.2018.05.049 .
Romanosky, S., L. Ablon, A. Kuehn, and T. Jones. 2019. Content analysis of cyber insurance policies: How do carriers price cyber risk? Journal of Cybersecurity (oxford) 5 (1): tyz002.
Sarabi, A., P. Naghizadeh, Y. Liu, and M. Liu. 2016. Risky business: Fine-grained data breach prediction using business profiles. Journal of Cybersecurity 2 (1): 15–28. https://doi.org/10.1093/cybsec/tyw004 .
Sardi, Alberto, Alessandro Rizzi, Enrico Sorano, and Anna Guerrieri. 2021. Cyber risk in health facilities: A systematic literature review. Sustainability 12 (17): 7002.
Sarker, Iqbal H., A.S.M. Kayes, Shahriar Badsha, Hamed Alqahtani, Paul Watters, and Alex Ng. 2020. Cybersecurity data science: An overview from machine learning perspective. Journal of Big Data 7 (1): 41. https://doi.org/10.1186/s40537-020-00318-5 .
Scopus. 2021. Factsheet. https://www.elsevier.com/__data/assets/pdf_file/0017/114533/Scopus_GlobalResearch_Factsheet2019_FINAL_WEB.pdf . Accessed 11 May 2021.
Sentuna, A., A. Alsadoon, P.W.C. Prasad, M. Saadeh, and O.H. Alsadoon. 2021. A novel Enhanced Naïve Bayes Posterior Probability (ENBPP) using machine learning: Cyber threat analysis. Neural Processing Letters 53 (1): 177–209. https://doi.org/10.1007/s11063-020-10381-x .
Shaukat, K., S.H. Luo, V. Varadharajan, I.A. Hameed, S. Chen, D.X. Liu, and J.M. Li. 2020. Performance comparison and current challenges of using machine learning techniques in cybersecurity. Energies 13 (10): 27. https://doi.org/10.3390/en13102509 .
Sheehan, B., F. Murphy, M. Mullins, and C. Ryan. 2019. Connected and autonomous vehicles: A cyber-risk classification framework. Transportation Research Part a: Policy and Practice 124: 523–536. https://doi.org/10.1016/j.tra.2018.06.033 .
Sheehan, B., F. Murphy, A.N. Kia, and R. Kiely. 2021. A quantitative bow-tie cyber risk classification and assessment framework. Journal of Risk Research 24 (12): 1619–1638.
Shlomo, A., M. Kalech, and R. Moskovitch. 2021. Temporal pattern-based malicious activity detection in SCADA systems. Computers & Security 102: 17. https://doi.org/10.1016/j.cose.2020.102153 .
Singh, K.J., and T. De. 2020. Efficient classification of DDoS attacks using an ensemble feature selection algorithm. Journal of Intelligent Systems 29 (1): 71–83. https://doi.org/10.1515/jisys-2017-0472 .
Skrjanc, I., S. Ozawa, T. Ban, and D. Dovzan. 2018. Large-scale cyber attacks monitoring using Evolving Cauchy Possibilistic Clustering. Applied Soft Computing 62: 592–601. https://doi.org/10.1016/j.asoc.2017.11.008 .
Smart, W. 2018. Lessons learned review of the WannaCry Ransomware Cyber Attack. https://www.england.nhs.uk/wp-content/uploads/2018/02/lessons-learned-review-wannacry-ransomware-cyber-attack-cio-review.pdf . Accessed 7 May 2021.
Sornette, D., T. Maillart, and W. Kröger. 2013. Exploring the limits of safety analysis in complex technological systems. International Journal of Disaster Risk Reduction 6: 59–66. https://doi.org/10.1016/j.ijdrr.2013.04.002 .
Sovacool, B.K. 2008. The costs of failure: A preliminary assessment of major energy accidents, 1907–2007. Energy Policy 36 (5): 1802–1820. https://doi.org/10.1016/j.enpol.2008.01.040 .
SpringerLink. 2021. Journal Search. https://rd.springer.com/search?facet-content-type=%22Journal%22 . Accessed 11 May 2021.
Stojanovic, B., K. Hofer-Schmitz, and U. Kleb. 2020. APT datasets and attack modeling for automated detection methods: A review. Computers & Security 92: 19. https://doi.org/10.1016/j.cose.2020.101734 .
Subroto, A., and A. Apriyana. 2019. Cyber risk prediction through social media big data analytics and statistical machine learning. Journal of Big Data . https://doi.org/10.1186/s40537-019-0216-1 .
Tan, Z., A. Jamdagni, X. He, P. Nanda, R.P. Liu, and J. Hu. 2015. Detection of denial-of-service attacks based on computer vision techniques. IEEE Transactions on Computers 64 (9): 2519–2533. https://doi.org/10.1109/TC.2014.2375218 .
Tidy, J. 2021. Irish cyber-attack: Hackers bail out Irish health service for free. https://www.bbc.com/news/world-europe-57197688 . Accessed 6 May 2021.
Tuncer, T., F. Ertam, and S. Dogan. 2020. Automated malware recognition method based on local neighborhood binary pattern. Multimedia Tools and Applications 79 (37–38): 27815–27832. https://doi.org/10.1007/s11042-020-09376-6 .
Uhm, Y., and W. Pak. 2021. Service-aware two-level partitioning for machine learning-based network intrusion detection with high performance and high scalability. IEEE Access 9: 6608–6622. https://doi.org/10.1109/ACCESS.2020.3048900 .
Ulven, J.B., and G. Wangen. 2021. A systematic review of cybersecurity risks in higher education. Future Internet 13 (2): 1–40. https://doi.org/10.3390/fi13020039 .
Vaccari, I., G. Chiola, M. Aiello, M. Mongelli, and E. Cambiaso. 2020. MQTTset, a new dataset for machine learning techniques on MQTT. Sensors 20 (22): 17. https://doi.org/10.3390/s20226578 .
Valeriano, B., and R.C. Maness. 2014. The dynamics of cyber conflict between rival antagonists, 2001–11. Journal of Peace Research 51 (3): 347–360. https://doi.org/10.1177/0022343313518940 .
Varghese, J.E., and B. Muniyal. 2021. An Efficient IDS framework for DDoS attacks in SDN environment. IEEE Access 9: 69680–69699. https://doi.org/10.1109/ACCESS.2021.3078065 .
Varsha, M. V., P. Vinod, K.A. Dhanya. 2017 Identification of malicious android app using manifest and opcode features. Journal of Computer Virology and Hacking Techniques 13 (2): 125–138. https://doi.org/10.1007/s11416-016-0277-z
Velliangiri, S., and H.M. Pandey. 2020. Fuzzy-Taylor-elephant herd optimization inspired Deep Belief Network for DDoS attack detection and comparison with state-of-the-arts algorithms. Future Generation Computer Systems—the International Journal of Escience 110: 80–90. https://doi.org/10.1016/j.future.2020.03.049 .
Verma, A., and V. Ranga. 2020. Machine learning based intrusion detection systems for IoT applications. Wireless Personal Communications 111 (4): 2287–2310. https://doi.org/10.1007/s11277-019-06986-8 .
Vidros, S., C. Kolias, G. Kambourakis, and L. Akoglu. 2017. Automatic detection of online recruitment frauds: Characteristics, methods, and a public dataset. Future Internet 9 (1): 19. https://doi.org/10.3390/fi9010006 .
Vinayakumar, R., M. Alazab, K.P. Soman, P. Poornachandran, A. Al-Nemrat, and S. Venkatraman. 2019. Deep learning approach for intelligent intrusion detection system. IEEE Access 7: 41525–41550. https://doi.org/10.1109/access.2019.2895334 .
Walker-Roberts, S., M. Hammoudeh, O. Aldabbas, M. Aydin, and A. Dehghantanha. 2020. Threats on the horizon: Understanding security threats in the era of cyber-physical systems. Journal of Supercomputing 76 (4): 2643–2664. https://doi.org/10.1007/s11227-019-03028-9 .
Web of Science. 2021. Web of Science: Science Citation Index Expanded. https://clarivate.com/webofsciencegroup/solutions/webofscience-scie/ . Accessed 11 May 2021.
World Economic Forum. 2020. WEF Global Risk Report. http://www3.weforum.org/docs/WEF_Global_Risk_Report_2020.pdf . Accessed 13 May 2020.
Xin, Y., L. Kong, Z. Liu, Y. Chen, Y. Li, H. Zhu, M. Gao, H. Hou, and C. Wang. 2018. Machine learning and deep learning methods for cybersecurity. IEEE Access 6: 35365–35381. https://doi.org/10.1109/ACCESS.2018.2836950 .
Xu, C., J. Zhang, K. Chang, and C. Long. 2013. Uncovering collusive spammers in Chinese review websites. In Proceedings of the 22nd ACM international conference on Information & Knowledge Management.
Yang, J., T. Li, G. Liang, W. He, and Y. Zhao. 2019. A Simple recurrent unit model based intrusion detection system with DCGAN. IEEE Access 7: 83286–83296. https://doi.org/10.1109/ACCESS.2019.2922692 .
Yuan, B.G., J.F. Wang, D. Liu, W. Guo, P. Wu, and X.H. Bao. 2020. Byte-level malware classification based on Markov images and deep learning. Computers & Security 92: 12. https://doi.org/10.1016/j.cose.2020.101740 .
Zhang, S., X.M. Ou, and D. Caragea. 2015. Predicting cyber risks through national vulnerability database. Information Security Journal 24 (4–6): 194–206. https://doi.org/10.1080/19393555.2015.1111961 .
Zhang, Y., P. Li, and X. Wang. 2019. Intrusion detection for IoT based on improved genetic algorithm and deep belief network. IEEE Access 7: 31711–31722.
Zheng, Muwei, Hannah Robbins, Zimo Chai, Prakash Thapa, and Tyler Moore. 2018. Cybersecurity research datasets: taxonomy and empirical analysis. In 11th {USENIX} workshop on cyber security experimentation and test ({CSET} 18).
Zhou, X., W. Liang, S. Shimizu, J. Ma, and Q. Jin. 2021. Siamese neural network based few-shot learning for anomaly detection in industrial cyber-physical systems. IEEE Transactions on Industrial Informatics 17 (8): 5790–5798. https://doi.org/10.1109/TII.2020.3047675 .
Zhou, Y.Y., G. Cheng, S.Q. Jiang, and M. Dai. 2020. Building an efficient intrusion detection system based on feature selection and ensemble classifier. Computer Networks 174: 17. https://doi.org/10.1016/j.comnet.2020.107247 .
Download references
Open Access funding provided by the IReL Consortium.
Author information
Authors and affiliations.
University of Limerick, Limerick, Ireland
Frank Cremer, Barry Sheehan, Arash N. Kia, Martin Mullins & Finbarr Murphy
TH Köln University of Applied Sciences, Cologne, Germany
Michael Fortmann & Stefan Materne
You can also search for this author in PubMed Google Scholar
Corresponding author
Correspondence to Barry Sheehan .
Ethics declarations
Conflict of interest.
On behalf of all authors, the corresponding author states that there is no conflict of interest.
Additional information
Publisher's note.
Springer Nature remains neutral with regard to jurisdictional claims in published maps and institutional affiliations.
Supplementary Information
Below is the link to the electronic supplementary material.
Supplementary file1 (PDF 334 kb)
Supplementary file1 (docx 418 kb), rights and permissions.
Open Access This article is licensed under a Creative Commons Attribution 4.0 International License, which permits use, sharing, adaptation, distribution and reproduction in any medium or format, as long as you give appropriate credit to the original author(s) and the source, provide a link to the Creative Commons licence, and indicate if changes were made. The images or other third party material in this article are included in the article's Creative Commons licence, unless indicated otherwise in a credit line to the material. If material is not included in the article's Creative Commons licence and your intended use is not permitted by statutory regulation or exceeds the permitted use, you will need to obtain permission directly from the copyright holder. To view a copy of this licence, visit http://creativecommons.org/licenses/by/4.0/ .
Reprints and permissions
About this article
Cremer, F., Sheehan, B., Fortmann, M. et al. Cyber risk and cybersecurity: a systematic review of data availability. Geneva Pap Risk Insur Issues Pract 47 , 698–736 (2022). https://doi.org/10.1057/s41288-022-00266-6
Download citation
Received : 15 June 2021
Accepted : 20 January 2022
Published : 17 February 2022
Issue Date : July 2022
DOI : https://doi.org/10.1057/s41288-022-00266-6
Share this article
Anyone you share the following link with will be able to read this content:
Sorry, a shareable link is not currently available for this article.
Provided by the Springer Nature SharedIt content-sharing initiative
- Cyber insurance
- Systematic review
- Cybersecurity
- Find a journal
- Publish with us
- Track your research
- Survey Paper
- Open access
- Published: 01 July 2020
Cybersecurity data science: an overview from machine learning perspective
- Iqbal H. Sarker ORCID: orcid.org/0000-0003-1740-5517 1 , 2 ,
- A. S. M. Kayes 3 ,
- Shahriar Badsha 4 ,
- Hamed Alqahtani 5 ,
- Paul Watters 3 &
- Alex Ng 3
Journal of Big Data volume 7 , Article number: 41 ( 2020 ) Cite this article
140k Accesses
236 Citations
51 Altmetric
Metrics details
In a computing context, cybersecurity is undergoing massive shifts in technology and its operations in recent days, and data science is driving the change. Extracting security incident patterns or insights from cybersecurity data and building corresponding data-driven model , is the key to make a security system automated and intelligent. To understand and analyze the actual phenomena with data, various scientific methods, machine learning techniques, processes, and systems are used, which is commonly known as data science. In this paper, we focus and briefly discuss on cybersecurity data science , where the data is being gathered from relevant cybersecurity sources, and the analytics complement the latest data-driven patterns for providing more effective security solutions. The concept of cybersecurity data science allows making the computing process more actionable and intelligent as compared to traditional ones in the domain of cybersecurity. We then discuss and summarize a number of associated research issues and future directions . Furthermore, we provide a machine learning based multi-layered framework for the purpose of cybersecurity modeling. Overall, our goal is not only to discuss cybersecurity data science and relevant methods but also to focus the applicability towards data-driven intelligent decision making for protecting the systems from cyber-attacks.
Introduction
Due to the increasing dependency on digitalization and Internet-of-Things (IoT) [ 1 ], various security incidents such as unauthorized access [ 2 ], malware attack [ 3 ], zero-day attack [ 4 ], data breach [ 5 ], denial of service (DoS) [ 2 ], social engineering or phishing [ 6 ] etc. have grown at an exponential rate in recent years. For instance, in 2010, there were less than 50 million unique malware executables known to the security community. By 2012, they were double around 100 million, and in 2019, there are more than 900 million malicious executables known to the security community, and this number is likely to grow, according to the statistics of AV-TEST institute in Germany [ 7 ]. Cybercrime and attacks can cause devastating financial losses and affect organizations and individuals as well. It’s estimated that, a data breach costs 8.19 million USD for the United States and 3.9 million USD on an average [ 8 ], and the annual cost to the global economy from cybercrime is 400 billion USD [ 9 ]. According to Juniper Research [ 10 ], the number of records breached each year to nearly triple over the next 5 years. Thus, it’s essential that organizations need to adopt and implement a strong cybersecurity approach to mitigate the loss. According to [ 11 ], the national security of a country depends on the business, government, and individual citizens having access to applications and tools which are highly secure, and the capability on detecting and eliminating such cyber-threats in a timely way. Therefore, to effectively identify various cyber incidents either previously seen or unseen, and intelligently protect the relevant systems from such cyber-attacks, is a key issue to be solved urgently.
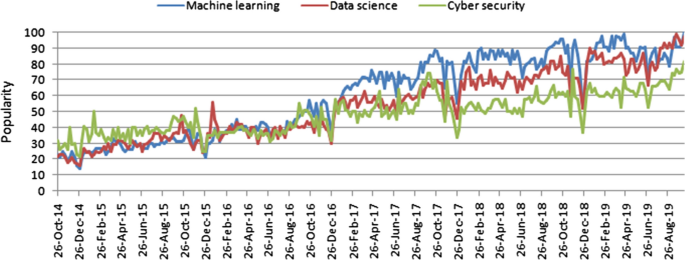
Popularity trends of data science, machine learning and cybersecurity over time, where x-axis represents the timestamp information and y axis represents the corresponding popularity values
Cybersecurity is a set of technologies and processes designed to protect computers, networks, programs and data from attack, damage, or unauthorized access [ 12 ]. In recent days, cybersecurity is undergoing massive shifts in technology and its operations in the context of computing, and data science (DS) is driving the change, where machine learning (ML), a core part of “Artificial Intelligence” (AI) can play a vital role to discover the insights from data. Machine learning can significantly change the cybersecurity landscape and data science is leading a new scientific paradigm [ 13 , 14 ]. The popularity of these related technologies is increasing day-by-day, which is shown in Fig. 1 , based on the data of the last five years collected from Google Trends [ 15 ]. The figure represents timestamp information in terms of a particular date in the x-axis and corresponding popularity in the range of 0 (minimum) to 100 (maximum) in the y-axis. As shown in Fig. 1 , the popularity indication values of these areas are less than 30 in 2014, while they exceed 70 in 2019, i.e., more than double in terms of increased popularity. In this paper, we focus on cybersecurity data science (CDS), which is broadly related to these areas in terms of security data processing techniques and intelligent decision making in real-world applications. Overall, CDS is security data-focused, applies machine learning methods to quantify cyber risks, and ultimately seeks to optimize cybersecurity operations. Thus, the purpose of this paper is for those academia and industry people who want to study and develop a data-driven smart cybersecurity model based on machine learning techniques. Therefore, great emphasis is placed on a thorough description of various types of machine learning methods, and their relations and usage in the context of cybersecurity. This paper does not describe all of the different techniques used in cybersecurity in detail; instead, it gives an overview of cybersecurity data science modeling based on artificial intelligence, particularly from machine learning perspective.
The ultimate goal of cybersecurity data science is data-driven intelligent decision making from security data for smart cybersecurity solutions. CDS represents a partial paradigm shift from traditional well-known security solutions such as firewalls, user authentication and access control, cryptography systems etc. that might not be effective according to today’s need in cyber industry [ 16 , 17 , 18 , 19 ]. The problems are these are typically handled statically by a few experienced security analysts, where data management is done in an ad-hoc manner [ 20 , 21 ]. However, as an increasing number of cybersecurity incidents in different formats mentioned above continuously appear over time, such conventional solutions have encountered limitations in mitigating such cyber risks. As a result, numerous advanced attacks are created and spread very quickly throughout the Internet. Although several researchers use various data analysis and learning techniques to build cybersecurity models that are summarized in “ Machine learning tasks in cybersecurity ” section, a comprehensive security model based on the effective discovery of security insights and latest security patterns could be more useful. To address this issue, we need to develop more flexible and efficient security mechanisms that can respond to threats and to update security policies to mitigate them intelligently in a timely manner. To achieve this goal, it is inherently required to analyze a massive amount of relevant cybersecurity data generated from various sources such as network and system sources, and to discover insights or proper security policies with minimal human intervention in an automated manner.
Analyzing cybersecurity data and building the right tools and processes to successfully protect against cybersecurity incidents goes beyond a simple set of functional requirements and knowledge about risks, threats or vulnerabilities. For effectively extracting the insights or the patterns of security incidents, several machine learning techniques, such as feature engineering, data clustering, classification, and association analysis, or neural network-based deep learning techniques can be used, which are briefly discussed in “ Machine learning tasks in cybersecurity ” section. These learning techniques are capable to find the anomalies or malicious behavior and data-driven patterns of associated security incidents to make an intelligent decision. Thus, based on the concept of data-driven decision making, we aim to focus on cybersecurity data science , where the data is being gathered from relevant cybersecurity sources such as network activity, database activity, application activity, or user activity, and the analytics complement the latest data-driven patterns for providing corresponding security solutions.
The contributions of this paper are summarized as follows.
We first make a brief discussion on the concept of cybersecurity data science and relevant methods to understand its applicability towards data-driven intelligent decision making in the domain of cybersecurity. For this purpose, we also make a review and brief discussion on different machine learning tasks in cybersecurity, and summarize various cybersecurity datasets highlighting their usage in different data-driven cyber applications.
We then discuss and summarize a number of associated research issues and future directions in the area of cybersecurity data science, that could help both the academia and industry people to further research and development in relevant application areas.
Finally, we provide a generic multi-layered framework of the cybersecurity data science model based on machine learning techniques. In this framework, we briefly discuss how the cybersecurity data science model can be used to discover useful insights from security data and making data-driven intelligent decisions to build smart cybersecurity systems.
The remainder of the paper is organized as follows. “ Background ” section summarizes background of our study and gives an overview of the related technologies of cybersecurity data science. “ Cybersecurity data science ” section defines and discusses briefly about cybersecurity data science including various categories of cyber incidents data. In “ Machine learning tasks in cybersecurity ” section, we briefly discuss various categories of machine learning techniques including their relations with cybersecurity tasks and summarize a number of machine learning based cybersecurity models in the field. “ Research issues and future directions ” section briefly discusses and highlights various research issues and future directions in the area of cybersecurity data science. In “ A multi-layered framework for smart cybersecurity services ” section, we suggest a machine learning-based framework to build cybersecurity data science model and discuss various layers with their roles. In “ Discussion ” section, we highlight several key points regarding our studies. Finally, “ Conclusion ” section concludes this paper.
In this section, we give an overview of the related technologies of cybersecurity data science including various types of cybersecurity incidents and defense strategies.
- Cybersecurity
Over the last half-century, the information and communication technology (ICT) industry has evolved greatly, which is ubiquitous and closely integrated with our modern society. Thus, protecting ICT systems and applications from cyber-attacks has been greatly concerned by the security policymakers in recent days [ 22 ]. The act of protecting ICT systems from various cyber-threats or attacks has come to be known as cybersecurity [ 9 ]. Several aspects are associated with cybersecurity: measures to protect information and communication technology; the raw data and information it contains and their processing and transmitting; associated virtual and physical elements of the systems; the degree of protection resulting from the application of those measures; and eventually the associated field of professional endeavor [ 23 ]. Craigen et al. defined “cybersecurity as a set of tools, practices, and guidelines that can be used to protect computer networks, software programs, and data from attack, damage, or unauthorized access” [ 24 ]. According to Aftergood et al. [ 12 ], “cybersecurity is a set of technologies and processes designed to protect computers, networks, programs and data from attacks and unauthorized access, alteration, or destruction”. Overall, cybersecurity concerns with the understanding of diverse cyber-attacks and devising corresponding defense strategies that preserve several properties defined as below [ 25 , 26 ].
Confidentiality is a property used to prevent the access and disclosure of information to unauthorized individuals, entities or systems.
Integrity is a property used to prevent any modification or destruction of information in an unauthorized manner.
Availability is a property used to ensure timely and reliable access of information assets and systems to an authorized entity.
The term cybersecurity applies in a variety of contexts, from business to mobile computing, and can be divided into several common categories. These are - network security that mainly focuses on securing a computer network from cyber attackers or intruders; application security that takes into account keeping the software and the devices free of risks or cyber-threats; information security that mainly considers security and the privacy of relevant data; operational security that includes the processes of handling and protecting data assets. Typical cybersecurity systems are composed of network security systems and computer security systems containing a firewall, antivirus software, or an intrusion detection system [ 27 ].
Cyberattacks and security risks
The risks typically associated with any attack, which considers three security factors, such as threats, i.e., who is attacking, vulnerabilities, i.e., the weaknesses they are attacking, and impacts, i.e., what the attack does [ 9 ]. A security incident is an act that threatens the confidentiality, integrity, or availability of information assets and systems. Several types of cybersecurity incidents that may result in security risks on an organization’s systems and networks or an individual [ 2 ]. These are:
Unauthorized access that describes the act of accessing information to network, systems or data without authorization that results in a violation of a security policy [ 2 ];
Malware known as malicious software, is any program or software that intentionally designed to cause damage to a computer, client, server, or computer network, e.g., botnets. Examples of different types of malware including computer viruses, worms, Trojan horses, adware, ransomware, spyware, malicious bots, etc. [ 3 , 26 ]; Ransom malware, or ransomware , is an emerging form of malware that prevents users from accessing their systems or personal files, or the devices, then demands an anonymous online payment in order to restore access.
Denial-of-Service is an attack meant to shut down a machine or network, making it inaccessible to its intended users by flooding the target with traffic that triggers a crash. The Denial-of-Service (DoS) attack typically uses one computer with an Internet connection, while distributed denial-of-service (DDoS) attack uses multiple computers and Internet connections to flood the targeted resource [ 2 ];
Phishing a type of social engineering , used for a broad range of malicious activities accomplished through human interactions, in which the fraudulent attempt takes part to obtain sensitive information such as banking and credit card details, login credentials, or personally identifiable information by disguising oneself as a trusted individual or entity via an electronic communication such as email, text, or instant message, etc. [ 26 ];
Zero-day attack is considered as the term that is used to describe the threat of an unknown security vulnerability for which either the patch has not been released or the application developers were unaware [ 4 , 28 ].
Beside these attacks mentioned above, privilege escalation [ 29 ], password attack [ 30 ], insider threat [ 31 ], man-in-the-middle [ 32 ], advanced persistent threat [ 33 ], SQL injection attack [ 34 ], cryptojacking attack [ 35 ], web application attack [ 30 ] etc. are well-known as security incidents in the field of cybersecurity. A data breach is another type of security incident, known as a data leak, which is involved in the unauthorized access of data by an individual, application, or service [ 5 ]. Thus, all data breaches are considered as security incidents, however, all the security incidents are not data breaches. Most data breaches occur in the banking industry involving the credit card numbers, personal information, followed by the healthcare sector and the public sector [ 36 ].
Cybersecurity defense strategies
Defense strategies are needed to protect data or information, information systems, and networks from cyber-attacks or intrusions. More granularly, they are responsible for preventing data breaches or security incidents and monitoring and reacting to intrusions, which can be defined as any kind of unauthorized activity that causes damage to an information system [ 37 ]. An intrusion detection system (IDS) is typically represented as “a device or software application that monitors a computer network or systems for malicious activity or policy violations” [ 38 ]. The traditional well-known security solutions such as anti-virus, firewalls, user authentication, access control, data encryption and cryptography systems, however might not be effective according to today’s need in the cyber industry
[ 16 , 17 , 18 , 19 ]. On the other hand, IDS resolves the issues by analyzing security data from several key points in a computer network or system [ 39 , 40 ]. Moreover, intrusion detection systems can be used to detect both internal and external attacks.
Intrusion detection systems are different categories according to the usage scope. For instance, a host-based intrusion detection system (HIDS), and network intrusion detection system (NIDS) are the most common types based on the scope of single computers to large networks. In a HIDS, the system monitors important files on an individual system, while it analyzes and monitors network connections for suspicious traffic in a NIDS. Similarly, based on methodologies, the signature-based IDS, and anomaly-based IDS are the most well-known variants [ 37 ].
Signature-based IDS : A signature can be a predefined string, pattern, or rule that corresponds to a known attack. A particular pattern is identified as the detection of corresponding attacks in a signature-based IDS. An example of a signature can be known patterns or a byte sequence in a network traffic, or sequences used by malware. To detect the attacks, anti-virus software uses such types of sequences or patterns as a signature while performing the matching operation. Signature-based IDS is also known as knowledge-based or misuse detection [ 41 ]. This technique can be efficient to process a high volume of network traffic, however, is strictly limited to the known attacks only. Thus, detecting new attacks or unseen attacks is one of the biggest challenges faced by this signature-based system.
Anomaly-based IDS : The concept of anomaly-based detection overcomes the issues of signature-based IDS discussed above. In an anomaly-based intrusion detection system, the behavior of the network is first examined to find dynamic patterns, to automatically create a data-driven model, to profile the normal behavior, and thus it detects deviations in the case of any anomalies [ 41 ]. Thus, anomaly-based IDS can be treated as a dynamic approach, which follows behavior-oriented detection. The main advantage of anomaly-based IDS is the ability to identify unknown or zero-day attacks [ 42 ]. However, the issue is that the identified anomaly or abnormal behavior is not always an indicator of intrusions. It sometimes may happen because of several factors such as policy changes or offering a new service.
In addition, a hybrid detection approach [ 43 , 44 ] that takes into account both the misuse and anomaly-based techniques discussed above can be used to detect intrusions. In a hybrid system, the misuse detection system is used for detecting known types of intrusions and anomaly detection system is used for novel attacks [ 45 ]. Beside these approaches, stateful protocol analysis can also be used to detect intrusions that identifies deviations of protocol state similarly to the anomaly-based method, however it uses predetermined universal profiles based on accepted definitions of benign activity [ 41 ]. In Table 1 , we have summarized these common approaches highlighting their pros and cons. Once the detecting has been completed, the intrusion prevention system (IPS) that is intended to prevent malicious events, can be used to mitigate the risks in different ways such as manual, providing notification, or automatic process [ 46 ]. Among these approaches, an automatic response system could be more effective as it does not involve a human interface between the detection and response systems.
- Data science
We are living in the age of data, advanced analytics, and data science, which are related to data-driven intelligent decision making. Although, the process of searching patterns or discovering hidden and interesting knowledge from data is known as data mining [ 47 ], in this paper, we use the broader term “data science” rather than data mining. The reason is that, data science, in its most fundamental form, is all about understanding of data. It involves studying, processing, and extracting valuable insights from a set of information. In addition to data mining, data analytics is also related to data science. The development of data mining, knowledge discovery, and machine learning that refers creating algorithms and program which learn on their own, together with the original data analysis and descriptive analytics from the statistical perspective, forms the general concept of “data analytics” [ 47 ]. Nowadays, many researchers use the term “data science” to describe the interdisciplinary field of data collection, preprocessing, inferring, or making decisions by analyzing the data. To understand and analyze the actual phenomena with data, various scientific methods, machine learning techniques, processes, and systems are used, which is commonly known as data science. According to Cao et al. [ 47 ] “data science is a new interdisciplinary field that synthesizes and builds on statistics, informatics, computing, communication, management, and sociology to study data and its environments, to transform data to insights and decisions by following a data-to-knowledge-to-wisdom thinking and methodology”. As a high-level statement in the context of cybersecurity, we can conclude that it is the study of security data to provide data-driven solutions for the given security problems, as known as “the science of cybersecurity data”. Figure 2 shows the typical data-to-insight-to-decision transfer at different periods and general analytic stages in data science, in terms of a variety of analytics goals (G) and approaches (A) to achieve the data-to-decision goal [ 47 ].
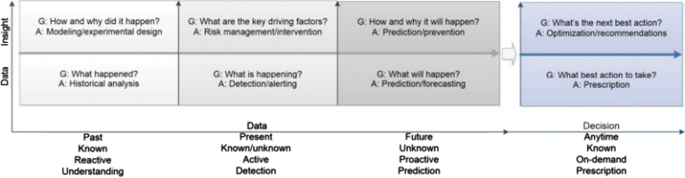
Data-to-insight-to-decision analytic stages in data science [ 47 ]
Based on the analytic power of data science including machine learning techniques, it can be a viable component of security strategies. By using data science techniques, security analysts can manipulate and analyze security data more effectively and efficiently, uncovering valuable insights from data. Thus, data science methodologies including machine learning techniques can be well utilized in the context of cybersecurity, in terms of problem understanding, gathering security data from diverse sources, preparing data to feed into the model, data-driven model building and updating, for providing smart security services, which motivates to define cybersecurity data science and to work in this research area.
Cybersecurity data science
In this section, we briefly discuss cybersecurity data science including various categories of cyber incidents data with the usage in different application areas, and the key terms and areas related to our study.
Understanding cybersecurity data
Data science is largely driven by the availability of data [ 48 ]. Datasets typically represent a collection of information records that consist of several attributes or features and related facts, in which cybersecurity data science is based on. Thus, it’s important to understand the nature of cybersecurity data containing various types of cyberattacks and relevant features. The reason is that raw security data collected from relevant cyber sources can be used to analyze the various patterns of security incidents or malicious behavior, to build a data-driven security model to achieve our goal. Several datasets exist in the area of cybersecurity including intrusion analysis, malware analysis, anomaly, fraud, or spam analysis that are used for various purposes. In Table 2 , we summarize several such datasets including their various features and attacks that are accessible on the Internet, and highlight their usage based on machine learning techniques in different cyber applications. Effectively analyzing and processing of these security features, building target machine learning-based security model according to the requirements, and eventually, data-driven decision making, could play a role to provide intelligent cybersecurity services that are discussed briefly in “ A multi-layered framework for smart cybersecurity services ” section.
Defining cybersecurity data science
Data science is transforming the world’s industries. It is critically important for the future of intelligent cybersecurity systems and services because of “security is all about data”. When we seek to detect cyber threats, we are analyzing the security data in the form of files, logs, network packets, or other relevant sources. Traditionally, security professionals didn’t use data science techniques to make detections based on these data sources. Instead, they used file hashes, custom-written rules like signatures, or manually defined heuristics [ 21 ]. Although these techniques have their own merits in several cases, it needs too much manual work to keep up with the changing cyber threat landscape. On the contrary, data science can make a massive shift in technology and its operations, where machine learning algorithms can be used to learn or extract insight of security incident patterns from the training data for their detection and prevention. For instance, to detect malware or suspicious trends, or to extract policy rules, these techniques can be used.
In recent days, the entire security industry is moving towards data science, because of its capability to transform raw data into decision making. To do this, several data-driven tasks can be associated, such as—(i) data engineering focusing practical applications of data gathering and analysis; (ii) reducing data volume that deals with filtering significant and relevant data to further analysis; (iii) discovery and detection that focuses on extracting insight or incident patterns or knowledge from data; (iv) automated models that focus on building data-driven intelligent security model; (v) targeted security alerts focusing on the generation of remarkable security alerts based on discovered knowledge that minimizes the false alerts, and (vi) resource optimization that deals with the available resources to achieve the target goals in a security system. While making data-driven decisions, behavioral analysis could also play a significant role in the domain of cybersecurity [ 81 ].
Thus, the concept of cybersecurity data science incorporates the methods and techniques of data science and machine learning as well as the behavioral analytics of various security incidents. The combination of these technologies has given birth to the term “cybersecurity data science”, which refers to collect a large amount of security event data from different sources and analyze it using machine learning technologies for detecting security risks or attacks either through the discovery of useful insights or the latest data-driven patterns. It is, however, worth remembering that cybersecurity data science is not just about a collection of machine learning algorithms, rather, a process that can help security professionals or analysts to scale and automate their security activities in a smart way and in a timely manner. Therefore, the formal definition can be as follows: “Cybersecurity data science is a research or working area existing at the intersection of cybersecurity, data science, and machine learning or artificial intelligence, which is mainly security data-focused, applies machine learning methods, attempts to quantify cyber-risks or incidents, and promotes inferential techniques to analyze behavioral patterns in security data. It also focuses on generating security response alerts, and eventually seeks for optimizing cybersecurity solutions, to build automated and intelligent cybersecurity systems.”
Table 3 highlights some key terms associated with cybersecurity data science. Overall, the outputs of cybersecurity data science are typically security data products, which can be a data-driven security model, policy rule discovery, risk or attack prediction, potential security service and recommendation, or the corresponding security system depending on the given security problem in the domain of cybersecurity. In the next section, we briefly discuss various machine learning tasks with examples within the scope of our study.
Machine learning tasks in cybersecurity
Machine learning (ML) is typically considered as a branch of “Artificial Intelligence”, which is closely related to computational statistics, data mining and analytics, data science, particularly focusing on making the computers to learn from data [ 82 , 83 ]. Thus, machine learning models typically comprise of a set of rules, methods, or complex “transfer functions” that can be applied to find interesting data patterns, or to recognize or predict behavior [ 84 ], which could play an important role in the area of cybersecurity. In the following, we discuss different methods that can be used to solve machine learning tasks and how they are related to cybersecurity tasks.
Supervised learning
Supervised learning is performed when specific targets are defined to reach from a certain set of inputs, i.e., task-driven approach. In the area of machine learning, the most popular supervised learning techniques are known as classification and regression methods [ 129 ]. These techniques are popular to classify or predict the future for a particular security problem. For instance, to predict denial-of-service attack (yes, no) or to identify different classes of network attacks such as scanning and spoofing, classification techniques can be used in the cybersecurity domain. ZeroR [ 83 ], OneR [ 130 ], Navies Bayes [ 131 ], Decision Tree [ 132 , 133 ], K-nearest neighbors [ 134 ], support vector machines [ 135 ], adaptive boosting [ 136 ], and logistic regression [ 137 ] are the well-known classification techniques. In addition, recently Sarker et al. have proposed BehavDT [ 133 ], and IntruDtree [ 106 ] classification techniques that are able to effectively build a data-driven predictive model. On the other hand, to predict the continuous or numeric value, e.g., total phishing attacks in a certain period or predicting the network packet parameters, regression techniques are useful. Regression analyses can also be used to detect the root causes of cybercrime and other types of fraud [ 138 ]. Linear regression [ 82 ], support vector regression [ 135 ] are the popular regression techniques. The main difference between classification and regression is that the output variable in the regression is numerical or continuous, while the predicted output for classification is categorical or discrete. Ensemble learning is an extension of supervised learning while mixing different simple models, e.g., Random Forest learning [ 139 ] that generates multiple decision trees to solve a particular security task.
Unsupervised learning
In unsupervised learning problems, the main task is to find patterns, structures, or knowledge in unlabeled data, i.e., data-driven approach [ 140 ]. In the area of cybersecurity, cyber-attacks like malware stays hidden in some ways, include changing their behavior dynamically and autonomously to avoid detection. Clustering techniques, a type of unsupervised learning, can help to uncover the hidden patterns and structures from the datasets, to identify indicators of such sophisticated attacks. Similarly, in identifying anomalies, policy violations, detecting, and eliminating noisy instances in data, clustering techniques can be useful. K-means [ 141 ], K-medoids [ 142 ] are the popular partitioning clustering algorithms, and single linkage [ 143 ] or complete linkage [ 144 ] are the well-known hierarchical clustering algorithms used in various application domains. Moreover, a bottom-up clustering approach proposed by Sarker et al. [ 145 ] can also be used by taking into account the data characteristics.
Besides, feature engineering tasks like optimal feature selection or extraction related to a particular security problem could be useful for further analysis [ 106 ]. Recently, Sarker et al. [ 106 ] have proposed an approach for selecting security features according to their importance score values. Moreover, Principal component analysis, linear discriminant analysis, pearson correlation analysis, or non-negative matrix factorization are the popular dimensionality reduction techniques to solve such issues [ 82 ]. Association rule learning is another example, where machine learning based policy rules can prevent cyber-attacks. In an expert system, the rules are usually manually defined by a knowledge engineer working in collaboration with a domain expert [ 37 , 140 , 146 ]. Association rule learning on the contrary, is the discovery of rules or relationships among a set of available security features or attributes in a given dataset [ 147 ]. To quantify the strength of relationships, correlation analysis can be used [ 138 ]. Many association rule mining algorithms have been proposed in the area of machine learning and data mining literature, such as logic-based [ 148 ], frequent pattern based [ 149 , 150 , 151 ], tree-based [ 152 ], etc. Recently, Sarker et al. [ 153 ] have proposed an association rule learning approach considering non-redundant generation, that can be used to discover a set of useful security policy rules. Moreover, AIS [ 147 ], Apriori [ 149 ], Apriori-TID and Apriori-Hybrid [ 149 ], FP-Tree [ 152 ], and RARM [ 154 ], and Eclat [ 155 ] are the well-known association rule learning algorithms that are capable to solve such problems by generating a set of policy rules in the domain of cybersecurity.
Neural networks and deep learning
Deep learning is a part of machine learning in the area of artificial intelligence, which is a computational model that is inspired by the biological neural networks in the human brain [ 82 ]. Artificial Neural Network (ANN) is frequently used in deep learning and the most popular neural network algorithm is backpropagation [ 82 ]. It performs learning on a multi-layer feed-forward neural network consists of an input layer, one or more hidden layers, and an output layer. The main difference between deep learning and classical machine learning is its performance on the amount of security data increases. Typically deep learning algorithms perform well when the data volumes are large, whereas machine learning algorithms perform comparatively better on small datasets [ 44 ]. In our earlier work, Sarker et al. [ 129 ], we have illustrated the effectiveness of these approaches considering contextual datasets. However, deep learning approaches mimic the human brain mechanism to interpret large amount of data or the complex data such as images, sounds and texts [ 44 , 129 ]. In terms of feature extraction to build models, deep learning reduces the effort of designing a feature extractor for each problem than the classical machine learning techniques. Beside these characteristics, deep learning typically takes a long time to train an algorithm than a machine learning algorithm, however, the test time is exactly the opposite [ 44 ]. Thus, deep learning relies more on high-performance machines with GPUs than classical machine-learning algorithms [ 44 , 156 ]. The most popular deep neural network learning models include multi-layer perceptron (MLP) [ 157 ], convolutional neural network (CNN) [ 158 ], recurrent neural network (RNN) or long-short term memory (LSTM) network [ 121 , 158 ]. In recent days, researchers use these deep learning techniques for different purposes such as detecting network intrusions, malware traffic detection and classification, etc. in the domain of cybersecurity [ 44 , 159 ].
Other learning techniques
Semi-supervised learning can be described as a hybridization of supervised and unsupervised techniques discussed above, as it works on both the labeled and unlabeled data. In the area of cybersecurity, it could be useful, when it requires to label data automatically without human intervention, to improve the performance of cybersecurity models. Reinforcement techniques are another type of machine learning that characterizes an agent by creating its own learning experiences through interacting directly with the environment, i.e., environment-driven approach, where the environment is typically formulated as a Markov decision process and take decision based on a reward function [ 160 ]. Monte Carlo learning, Q-learning, Deep Q Networks, are the most common reinforcement learning algorithms [ 161 ]. For instance, in a recent work [ 126 ], the authors present an approach for detecting botnet traffic or malicious cyber activities using reinforcement learning combining with neural network classifier. In another work [ 128 ], the authors discuss about the application of deep reinforcement learning to intrusion detection for supervised problems, where they received the best results for the Deep Q-Network algorithm. In the context of cybersecurity, genetic algorithms that use fitness, selection, crossover, and mutation for finding optimization, could also be used to solve a similar class of learning problems [ 119 ].
Various types of machine learning techniques discussed above can be useful in the domain of cybersecurity, to build an effective security model. In Table 4 , we have summarized several machine learning techniques that are used to build various types of security models for various purposes. Although these models typically represent a learning-based security model, in this paper, we aim to focus on a comprehensive cybersecurity data science model and relevant issues, in order to build a data-driven intelligent security system. In the next section, we highlight several research issues and potential solutions in the area of cybersecurity data science.
Research issues and future directions
Our study opens several research issues and challenges in the area of cybersecurity data science to extract insight from relevant data towards data-driven intelligent decision making for cybersecurity solutions. In the following, we summarize these challenges ranging from data collection to decision making.
Cybersecurity datasets : Source datasets are the primary component to work in the area of cybersecurity data science. Most of the existing datasets are old and might insufficient in terms of understanding the recent behavioral patterns of various cyber-attacks. Although the data can be transformed into a meaningful understanding level after performing several processing tasks, there is still a lack of understanding of the characteristics of recent attacks and their patterns of happening. Thus, further processing or machine learning algorithms may provide a low accuracy rate for making the target decisions. Therefore, establishing a large number of recent datasets for a particular problem domain like cyber risk prediction or intrusion detection is needed, which could be one of the major challenges in cybersecurity data science.
Handling quality problems in cybersecurity datasets : The cyber datasets might be noisy, incomplete, insignificant, imbalanced, or may contain inconsistency instances related to a particular security incident. Such problems in a data set may affect the quality of the learning process and degrade the performance of the machine learning-based models [ 162 ]. To make a data-driven intelligent decision for cybersecurity solutions, such problems in data is needed to deal effectively before building the cyber models. Therefore, understanding such problems in cyber data and effectively handling such problems using existing algorithms or newly proposed algorithm for a particular problem domain like malware analysis or intrusion detection and prevention is needed, which could be another research issue in cybersecurity data science.
Security policy rule generation : Security policy rules reference security zones and enable a user to allow, restrict, and track traffic on the network based on the corresponding user or user group, and service, or the application. The policy rules including the general and more specific rules are compared against the incoming traffic in sequence during the execution, and the rule that matches the traffic is applied. The policy rules used in most of the cybersecurity systems are static and generated by human expertise or ontology-based [ 163 , 164 ]. Although, association rule learning techniques produce rules from data, however, there is a problem of redundancy generation [ 153 ] that makes the policy rule-set complex. Therefore, understanding such problems in policy rule generation and effectively handling such problems using existing algorithms or newly proposed algorithm for a particular problem domain like access control [ 165 ] is needed, which could be another research issue in cybersecurity data science.
Hybrid learning method : Most commercial products in the cybersecurity domain contain signature-based intrusion detection techniques [ 41 ]. However, missing features or insufficient profiling can cause these techniques to miss unknown attacks. In that case, anomaly-based detection techniques or hybrid technique combining signature-based and anomaly-based can be used to overcome such issues. A hybrid technique combining multiple learning techniques or a combination of deep learning and machine-learning methods can be used to extract the target insight for a particular problem domain like intrusion detection, malware analysis, access control, etc. and make the intelligent decision for corresponding cybersecurity solutions.
Protecting the valuable security information : Another issue of a cyber data attack is the loss of extremely valuable data and information, which could be damaging for an organization. With the use of encryption or highly complex signatures, one can stop others from probing into a dataset. In such cases, cybersecurity data science can be used to build a data-driven impenetrable protocol to protect such security information. To achieve this goal, cyber analysts can develop algorithms by analyzing the history of cyberattacks to detect the most frequently targeted chunks of data. Thus, understanding such data protecting problems and designing corresponding algorithms to effectively handling these problems, could be another research issue in the area of cybersecurity data science.
Context-awareness in cybersecurity : Existing cybersecurity work mainly originates from the relevant cyber data containing several low-level features. When data mining and machine learning techniques are applied to such datasets, a related pattern can be identified that describes it properly. However, a broader contextual information [ 140 , 145 , 166 ] like temporal, spatial, relationship among events or connections, dependency can be used to decide whether there exists a suspicious activity or not. For instance, some approaches may consider individual connections as DoS attacks, while security experts might not treat them as malicious by themselves. Thus, a significant limitation of existing cybersecurity work is the lack of using the contextual information for predicting risks or attacks. Therefore, context-aware adaptive cybersecurity solutions could be another research issue in cybersecurity data science.
Feature engineering in cybersecurity : The efficiency and effectiveness of a machine learning-based security model has always been a major challenge due to the high volume of network data with a large number of traffic features. The large dimensionality of data has been addressed using several techniques such as principal component analysis (PCA) [ 167 ], singular value decomposition (SVD) [ 168 ] etc. In addition to low-level features in the datasets, the contextual relationships between suspicious activities might be relevant. Such contextual data can be stored in an ontology or taxonomy for further processing. Thus how to effectively select the optimal features or extract the significant features considering both the low-level features as well as the contextual features, for effective cybersecurity solutions could be another research issue in cybersecurity data science.
Remarkable security alert generation and prioritizing : In many cases, the cybersecurity system may not be well defined and may cause a substantial number of false alarms that are unexpected in an intelligent system. For instance, an IDS deployed in a real-world network generates around nine million alerts per day [ 169 ]. A network-based intrusion detection system typically looks at the incoming traffic for matching the associated patterns to detect risks, threats or vulnerabilities and generate security alerts. However, to respond to each such alert might not be effective as it consumes relatively huge amounts of time and resources, and consequently may result in a self-inflicted DoS. To overcome this problem, a high-level management is required that correlate the security alerts considering the current context and their logical relationship including their prioritization before reporting them to users, which could be another research issue in cybersecurity data science.
Recency analysis in cybersecurity solutions : Machine learning-based security models typically use a large amount of static data to generate data-driven decisions. Anomaly detection systems rely on constructing such a model considering normal behavior and anomaly, according to their patterns. However, normal behavior in a large and dynamic security system is not well defined and it may change over time, which can be considered as an incremental growing of dataset. The patterns in incremental datasets might be changed in several cases. This often results in a substantial number of false alarms known as false positives. Thus, a recent malicious behavioral pattern is more likely to be interesting and significant than older ones for predicting unknown attacks. Therefore, effectively using the concept of recency analysis [ 170 ] in cybersecurity solutions could be another issue in cybersecurity data science.
The most important work for an intelligent cybersecurity system is to develop an effective framework that supports data-driven decision making. In such a framework, we need to consider advanced data analysis based on machine learning techniques, so that the framework is capable to minimize these issues and to provide automated and intelligent security services. Thus, a well-designed security framework for cybersecurity data and the experimental evaluation is a very important direction and a big challenge as well. In the next section, we suggest and discuss a data-driven cybersecurity framework based on machine learning techniques considering multiple processing layers.
A multi-layered framework for smart cybersecurity services
As discussed earlier, cybersecurity data science is data-focused, applies machine learning methods, attempts to quantify cyber risks, promotes inferential techniques to analyze behavioral patterns, focuses on generating security response alerts, and eventually seeks for optimizing cybersecurity operations. Hence, we briefly discuss a multiple data processing layered framework that potentially can be used to discover security insights from the raw data to build smart cybersecurity systems, e.g., dynamic policy rule-based access control or intrusion detection and prevention system. To make a data-driven intelligent decision in the resultant cybersecurity system, understanding the security problems and the nature of corresponding security data and their vast analysis is needed. For this purpose, our suggested framework not only considers the machine learning techniques to build the security model but also takes into account the incremental learning and dynamism to keep the model up-to-date and corresponding response generation, which could be more effective and intelligent for providing the expected services. Figure 3 shows an overview of the framework, involving several processing layers, from raw security event data to services. In the following, we briefly discuss the working procedure of the framework.
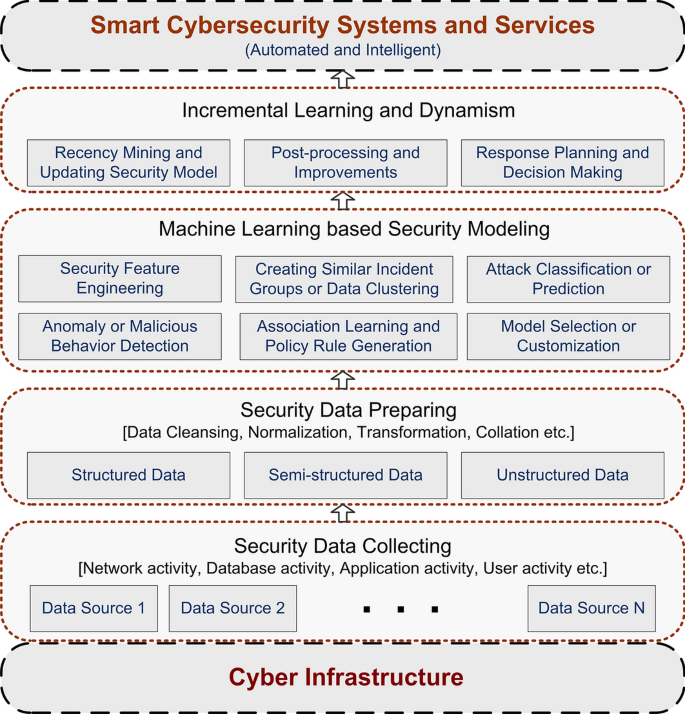
A generic multi-layered framework based on machine learning techniques for smart cybersecurity services
Security data collecting
Collecting valuable cybersecurity data is a crucial step, which forms a connecting link between security problems in cyberinfrastructure and corresponding data-driven solution steps in this framework, shown in Fig. 3 . The reason is that cyber data can serve as the source for setting up ground truth of the security model that affect the model performance. The quality and quantity of cyber data decide the feasibility and effectiveness of solving the security problem according to our goal. Thus, the concern is how to collect valuable and unique needs data for building the data-driven security models.
The general step to collect and manage security data from diverse data sources is based on a particular security problem and project within the enterprise. Data sources can be classified into several broad categories such as network, host, and hybrid [ 171 ]. Within the network infrastructure, the security system can leverage different types of security data such as IDS logs, firewall logs, network traffic data, packet data, and honeypot data, etc. for providing the target security services. For instance, a given IP is considered malicious or not, could be detected by performing data analysis utilizing the data of IP addresses and their cyber activities. In the domain of cybersecurity, the network source mentioned above is considered as the primary security event source to analyze. In the host category, it collects data from an organization’s host machines, where the data sources can be operating system logs, database access logs, web server logs, email logs, application logs, etc. Collecting data from both the network and host machines are considered a hybrid category. Overall, in a data collection layer the network activity, database activity, application activity, and user activity can be the possible security event sources in the context of cybersecurity data science.
Security data preparing
After collecting the raw security data from various sources according to the problem domain discussed above, this layer is responsible to prepare the raw data for building the model by applying various necessary processes. However, not all of the collected data contributes to the model building process in the domain of cybersecurity [ 172 ]. Therefore, the useless data should be removed from the rest of the data captured by the network sniffer. Moreover, data might be noisy, have missing or corrupted values, or have attributes of widely varying types and scales. High quality of data is necessary for achieving higher accuracy in a data-driven model, which is a process of learning a function that maps an input to an output based on example input-output pairs. Thus, it might require a procedure for data cleaning, handling missing or corrupted values. Moreover, security data features or attributes can be in different types, such as continuous, discrete, or symbolic [ 106 ]. Beyond a solid understanding of these types of data and attributes and their permissible operations, its need to preprocess the data and attributes to convert into the target type. Besides, the raw data can be in different types such as structured, semi-structured, or unstructured, etc. Thus, normalization, transformation, or collation can be useful to organize the data in a structured manner. In some cases, natural language processing techniques might be useful depending on data type and characteristics, e.g., textual contents. As both the quality and quantity of data decide the feasibility of solving the security problem, effectively pre-processing and management of data and their representation can play a significant role to build an effective security model for intelligent services.
Machine learning-based security modeling
This is the core step where insights and knowledge are extracted from data through the application of cybersecurity data science. In this section, we particularly focus on machine learning-based modeling as machine learning techniques can significantly change the cybersecurity landscape. The security features or attributes and their patterns in data are of high interest to be discovered and analyzed to extract security insights. To achieve the goal, a deeper understanding of data and machine learning-based analytical models utilizing a large number of cybersecurity data can be effective. Thus, various machine learning tasks can be involved in this model building layer according to the solution perspective. These are - security feature engineering that mainly responsible to transform raw security data into informative features that effectively represent the underlying security problem to the data-driven models. Thus, several data-processing tasks such as feature transformation and normalization, feature selection by taking into account a subset of available security features according to their correlations or importance in modeling, or feature generation and extraction by creating new brand principal components, may be involved in this module according to the security data characteristics. For instance, the chi-squared test, analysis of variance test, correlation coefficient analysis, feature importance, as well as discriminant and principal component analysis, or singular value decomposition, etc. can be used for analyzing the significance of the security features to perform the security feature engineering tasks [ 82 ].
Another significant module is security data clustering that uncovers hidden patterns and structures through huge volumes of security data, to identify where the new threats exist. It typically involves the grouping of security data with similar characteristics, which can be used to solve several cybersecurity problems such as detecting anomalies, policy violations, etc. Malicious behavior or anomaly detection module is typically responsible to identify a deviation to a known behavior, where clustering-based analysis and techniques can also be used to detect malicious behavior or anomaly detection. In the cybersecurity area, attack classification or prediction is treated as one of the most significant modules, which is responsible to build a prediction model to classify attacks or threats and to predict future for a particular security problem. To predict denial-of-service attack or a spam filter separating tasks from other messages, could be the relevant examples. Association learning or policy rule generation module can play a role to build an expert security system that comprises several IF-THEN rules that define attacks. Thus, in a problem of policy rule generation for rule-based access control system, association learning can be used as it discovers the associations or relationships among a set of available security features in a given security dataset. The popular machine learning algorithms in these categories are briefly discussed in “ Machine learning tasks in cybersecurity ” section. The module model selection or customization is responsible to choose whether it uses the existing machine learning model or needed to customize. Analyzing data and building models based on traditional machine learning or deep learning methods, could achieve acceptable results in certain cases in the domain of cybersecurity. However, in terms of effectiveness and efficiency or other performance measurements considering time complexity, generalization capacity, and most importantly the impact of the algorithm on the detection rate of a system, machine learning models are needed to customize for a specific security problem. Moreover, customizing the related techniques and data could improve the performance of the resultant security model and make it better applicable in a cybersecurity domain. The modules discussed above can work separately and combinedly depending on the target security problems.
Incremental learning and dynamism
In our framework, this layer is concerned with finalizing the resultant security model by incorporating additional intelligence according to the needs. This could be possible by further processing in several modules. For instance, the post-processing and improvement module in this layer could play a role to simplify the extracted knowledge according to the particular requirements by incorporating domain-specific knowledge. As the attack classification or prediction models based on machine learning techniques strongly rely on the training data, it can hardly be generalized to other datasets, which could be significant for some applications. To address such kind of limitations, this module is responsible to utilize the domain knowledge in the form of taxonomy or ontology to improve attack correlation in cybersecurity applications.
Another significant module recency mining and updating security model is responsible to keep the security model up-to-date for better performance by extracting the latest data-driven security patterns. The extracted knowledge discussed in the earlier layer is based on a static initial dataset considering the overall patterns in the datasets. However, such knowledge might not be guaranteed higher performance in several cases, because of incremental security data with recent patterns. In many cases, such incremental data may contain different patterns which could conflict with existing knowledge. Thus, the concept of RecencyMiner [ 170 ] on incremental security data and extracting new patterns can be more effective than the existing old patterns. The reason is that recent security patterns and rules are more likely to be significant than older ones for predicting cyber risks or attacks. Rather than processing the whole security data again, recency-based dynamic updating according to the new patterns would be more efficient in terms of processing and outcome. This could make the resultant cybersecurity model intelligent and dynamic. Finally, response planning and decision making module is responsible to make decisions based on the extracted insights and take necessary actions to prevent the system from the cyber-attacks to provide automated and intelligent services. The services might be different depending on particular requirements for a given security problem.
Overall, this framework is a generic description which potentially can be used to discover useful insights from security data, to build smart cybersecurity systems, to address complex security challenges, such as intrusion detection, access control management, detecting anomalies and fraud, or denial of service attacks, etc. in the area of cybersecurity data science.
Although several research efforts have been directed towards cybersecurity solutions, discussed in “ Background ” , “ Cybersecurity data science ”, and “ Machine learning tasks in cybersecurity ” sections in different directions, this paper presents a comprehensive view of cybersecurity data science. For this, we have conducted a literature review to understand cybersecurity data, various defense strategies including intrusion detection techniques, different types of machine learning techniques in cybersecurity tasks. Based on our discussion on existing work, several research issues related to security datasets, data quality problems, policy rule generation, learning methods, data protection, feature engineering, security alert generation, recency analysis etc. are identified that require further research attention in the domain of cybersecurity data science.
The scope of cybersecurity data science is broad. Several data-driven tasks such as intrusion detection and prevention, access control management, security policy generation, anomaly detection, spam filtering, fraud detection and prevention, various types of malware attack detection and defense strategies, etc. can be considered as the scope of cybersecurity data science. Such tasks based categorization could be helpful for security professionals including the researchers and practitioners who are interested in the domain-specific aspects of security systems [ 171 ]. The output of cybersecurity data science can be used in many application areas such as Internet of things (IoT) security [ 173 ], network security [ 174 ], cloud security [ 175 ], mobile and web applications [ 26 ], and other relevant cyber areas. Moreover, intelligent cybersecurity solutions are important for the banking industry, the healthcare sector, or the public sector, where data breaches typically occur [ 36 , 176 ]. Besides, the data-driven security solutions could also be effective in AI-based blockchain technology, where AI works with huge volumes of security event data to extract the useful insights using machine learning techniques, and block-chain as a trusted platform to store such data [ 177 ].
Although in this paper, we discuss cybersecurity data science focusing on examining raw security data to data-driven decision making for intelligent security solutions, it could also be related to big data analytics in terms of data processing and decision making. Big data deals with data sets that are too large or complex having characteristics of high data volume, velocity, and variety. Big data analytics mainly has two parts consisting of data management involving data storage, and analytics [ 178 ]. The analytics typically describe the process of analyzing such datasets to discover patterns, unknown correlations, rules, and other useful insights [ 179 ]. Thus, several advanced data analysis techniques such as AI, data mining, machine learning could play an important role in processing big data by converting big problems to small problems [ 180 ]. To do this, the potential strategies like parallelization, divide-and-conquer, incremental learning, sampling, granular computing, feature or instance selection, can be used to make better decisions, reducing costs, or enabling more efficient processing. In such cases, the concept of cybersecurity data science, particularly machine learning-based modeling could be helpful for process automation and decision making for intelligent security solutions. Moreover, researchers could consider modified algorithms or models for handing big data on parallel computing platforms like Hadoop, Storm, etc. [ 181 ].
Based on the concept of cybersecurity data science discussed in the paper, building a data-driven security model for a particular security problem and relevant empirical evaluation to measure the effectiveness and efficiency of the model, and to asses the usability in the real-world application domain could be a future work.
Motivated by the growing significance of cybersecurity and data science, and machine learning technologies, in this paper, we have discussed how cybersecurity data science applies to data-driven intelligent decision making in smart cybersecurity systems and services. We also have discussed how it can impact security data, both in terms of extracting insight of security incidents and the dataset itself. We aimed to work on cybersecurity data science by discussing the state of the art concerning security incidents data and corresponding security services. We also discussed how machine learning techniques can impact in the domain of cybersecurity, and examine the security challenges that remain. In terms of existing research, much focus has been provided on traditional security solutions, with less available work in machine learning technique based security systems. For each common technique, we have discussed relevant security research. The purpose of this article is to share an overview of the conceptualization, understanding, modeling, and thinking about cybersecurity data science.
We have further identified and discussed various key issues in security analysis to showcase the signpost of future research directions in the domain of cybersecurity data science. Based on the knowledge, we have also provided a generic multi-layered framework of cybersecurity data science model based on machine learning techniques, where the data is being gathered from diverse sources, and the analytics complement the latest data-driven patterns for providing intelligent security services. The framework consists of several main phases - security data collecting, data preparation, machine learning-based security modeling, and incremental learning and dynamism for smart cybersecurity systems and services. We specifically focused on extracting insights from security data, from setting a research design with particular attention to concepts for data-driven intelligent security solutions.
Overall, this paper aimed not only to discuss cybersecurity data science and relevant methods but also to discuss the applicability towards data-driven intelligent decision making in cybersecurity systems and services from machine learning perspectives. Our analysis and discussion can have several implications both for security researchers and practitioners. For researchers, we have highlighted several issues and directions for future research. Other areas for potential research include empirical evaluation of the suggested data-driven model, and comparative analysis with other security systems. For practitioners, the multi-layered machine learning-based model can be used as a reference in designing intelligent cybersecurity systems for organizations. We believe that our study on cybersecurity data science opens a promising path and can be used as a reference guide for both academia and industry for future research and applications in the area of cybersecurity.
Availability of data and materials
Not applicable.
Abbreviations
- Machine learning
Artificial Intelligence
Information and communication technology
Internet of Things
Distributed Denial of Service
Intrusion detection system
Intrusion prevention system
Host-based intrusion detection systems
Network Intrusion Detection Systems
Signature-based intrusion detection system
Anomaly-based intrusion detection system
Li S, Da Xu L, Zhao S. The internet of things: a survey. Inform Syst Front. 2015;17(2):243–59.
Google Scholar
Sun N, Zhang J, Rimba P, Gao S, Zhang LY, Xiang Y. Data-driven cybersecurity incident prediction: a survey. IEEE Commun Surv Tutor. 2018;21(2):1744–72.
McIntosh T, Jang-Jaccard J, Watters P, Susnjak T. The inadequacy of entropy-based ransomware detection. In: International conference on neural information processing. New York: Springer; 2019. p. 181–189
Alazab M, Venkatraman S, Watters P, Alazab M, et al. Zero-day malware detection based on supervised learning algorithms of api call signatures (2010)
Shaw A. Data breach: from notification to prevention using pci dss. Colum Soc Probs. 2009;43:517.
Gupta BB, Tewari A, Jain AK, Agrawal DP. Fighting against phishing attacks: state of the art and future challenges. Neural Comput Appl. 2017;28(12):3629–54.
Av-test institute, germany, https://www.av-test.org/en/statistics/malware/ . Accessed 20 Oct 2019.
Ibm security report, https://www.ibm.com/security/data-breach . Accessed on 20 Oct 2019.
Fischer EA. Cybersecurity issues and challenges: In brief. Congressional Research Service (2014)
Juniper research. https://www.juniperresearch.com/ . Accessed on 20 Oct 2019.
Papastergiou S, Mouratidis H, Kalogeraki E-M. Cyber security incident handling, warning and response system for the european critical information infrastructures (cybersane). In: International Conference on Engineering Applications of Neural Networks, p. 476–487 (2019). New York: Springer
Aftergood S. Cybersecurity: the cold war online. Nature. 2017;547(7661):30.
Hey AJ, Tansley S, Tolle KM, et al. The fourth paradigm: data-intensive scientific discovery. 2009;1:
Cukier K. Data, data everywhere: A special report on managing information, 2010.
Google trends. In: https://trends.google.com/trends/ , 2019.
Anwar S, Mohamad Zain J, Zolkipli MF, Inayat Z, Khan S, Anthony B, Chang V. From intrusion detection to an intrusion response system: fundamentals, requirements, and future directions. Algorithms. 2017;10(2):39.
MATH Google Scholar
Mohammadi S, Mirvaziri H, Ghazizadeh-Ahsaee M, Karimipour H. Cyber intrusion detection by combined feature selection algorithm. J Inform Sec Appl. 2019;44:80–8.
Tapiador JE, Orfila A, Ribagorda A, Ramos B. Key-recovery attacks on kids, a keyed anomaly detection system. IEEE Trans Depend Sec Comput. 2013;12(3):312–25.
Tavallaee M, Stakhanova N, Ghorbani AA. Toward credible evaluation of anomaly-based intrusion-detection methods. IEEE Transactions on Systems, Man, and Cybernetics, Part C (Applications and Reviews) 40(5), 516–524 (2010)
Foroughi F, Luksch P. Data science methodology for cybersecurity projects. arXiv preprint arXiv:1803.04219 , 2018.
Saxe J, Sanders H. Malware data science: Attack detection and attribution, 2018.
Rainie L, Anderson J, Connolly J. Cyber attacks likely to increase. Digital Life in. 2014, vol. 2025.
Fischer EA. Creating a national framework for cybersecurity: an analysis of issues and options. LIBRARY OF CONGRESS WASHINGTON DC CONGRESSIONAL RESEARCH SERVICE, 2005.
Craigen D, Diakun-Thibault N, Purse R. Defining cybersecurity. Technology Innovation. Manag Rev. 2014;4(10):13–21.
Council NR. et al. Toward a safer and more secure cyberspace, 2007.
Jang-Jaccard J, Nepal S. A survey of emerging threats in cybersecurity. J Comput Syst Sci. 2014;80(5):973–93.
MathSciNet MATH Google Scholar
Mukkamala S, Sung A, Abraham A. Cyber security challenges: Designing efficient intrusion detection systems and antivirus tools. Vemuri, V. Rao, Enhancing Computer Security with Smart Technology.(Auerbach, 2006), 125–163, 2005.
Bilge L, Dumitraş T. Before we knew it: an empirical study of zero-day attacks in the real world. In: Proceedings of the 2012 ACM conference on computer and communications security. ACM; 2012. p. 833–44.
Davi L, Dmitrienko A, Sadeghi A-R, Winandy M. Privilege escalation attacks on android. In: International conference on information security. New York: Springer; 2010. p. 346–60.
Jovičić B, Simić D. Common web application attack types and security using asp .net. ComSIS, 2006.
Warkentin M, Willison R. Behavioral and policy issues in information systems security: the insider threat. Eur J Inform Syst. 2009;18(2):101–5.
Kügler D. “man in the middle” attacks on bluetooth. In: International Conference on Financial Cryptography. New York: Springer; 2003, p. 149–61.
Virvilis N, Gritzalis D. The big four-what we did wrong in advanced persistent threat detection. In: 2013 International Conference on Availability, Reliability and Security. IEEE; 2013. p. 248–54.
Boyd SW, Keromytis AD. Sqlrand: Preventing sql injection attacks. In: International conference on applied cryptography and network security. New York: Springer; 2004. p. 292–302.
Sigler K. Crypto-jacking: how cyber-criminals are exploiting the crypto-currency boom. Comput Fraud Sec. 2018;2018(9):12–4.
2019 data breach investigations report, https://enterprise.verizon.com/resources/reports/dbir/ . Accessed 20 Oct 2019.
Khraisat A, Gondal I, Vamplew P, Kamruzzaman J. Survey of intrusion detection systems: techniques, datasets and challenges. Cybersecurity. 2019;2(1):20.
Johnson L. Computer incident response and forensics team management: conducting a successful incident response, 2013.
Brahmi I, Brahmi H, Yahia SB. A multi-agents intrusion detection system using ontology and clustering techniques. In: IFIP international conference on computer science and its applications. New York: Springer; 2015. p. 381–93.
Qu X, Yang L, Guo K, Ma L, Sun M, Ke M, Li M. A survey on the development of self-organizing maps for unsupervised intrusion detection. In: Mobile networks and applications. 2019;1–22.
Liao H-J, Lin C-HR, Lin Y-C, Tung K-Y. Intrusion detection system: a comprehensive review. J Netw Comput Appl. 2013;36(1):16–24.
Alazab A, Hobbs M, Abawajy J, Alazab M. Using feature selection for intrusion detection system. In: 2012 International symposium on communications and information technologies (ISCIT). IEEE; 2012. p. 296–301.
Viegas E, Santin AO, Franca A, Jasinski R, Pedroni VA, Oliveira LS. Towards an energy-efficient anomaly-based intrusion detection engine for embedded systems. IEEE Trans Comput. 2016;66(1):163–77.
Xin Y, Kong L, Liu Z, Chen Y, Li Y, Zhu H, Gao M, Hou H, Wang C. Machine learning and deep learning methods for cybersecurity. IEEE Access. 2018;6:35365–81.
Dutt I, Borah S, Maitra IK, Bhowmik K, Maity A, Das S. Real-time hybrid intrusion detection system using machine learning techniques. 2018, p. 885–94.
Ragsdale DJ, Carver C, Humphries JW, Pooch UW. Adaptation techniques for intrusion detection and intrusion response systems. In: Smc 2000 conference proceedings. 2000 IEEE international conference on systems, man and cybernetics.’cybernetics evolving to systems, humans, organizations, and their complex interactions’(cat. No. 0). IEEE; 2000. vol. 4, p. 2344–2349.
Cao L. Data science: challenges and directions. Commun ACM. 2017;60(8):59–68.
Rizk A, Elragal A. Data science: developing theoretical contributions in information systems via text analytics. J Big Data. 2020;7(1):1–26.
Lippmann RP, Fried DJ, Graf I, Haines JW, Kendall KR, McClung D, Weber D, Webster SE, Wyschogrod D, Cunningham RK, et al. Evaluating intrusion detection systems: The 1998 darpa off-line intrusion detection evaluation. In: Proceedings DARPA information survivability conference and exposition. DISCEX’00. IEEE; 2000. vol. 2, p. 12–26.
Kdd cup 99. http://kdd.ics.uci.edu/databases/kddcup99/kddcup99.html . Accessed 20 Oct 2019.
Tavallaee M, Bagheri E, Lu W, Ghorbani AA. A detailed analysis of the kdd cup 99 data set. In: 2009 IEEE symposium on computational intelligence for security and defense applications. IEEE; 2009. p. 1–6.
Caida ddos attack 2007 dataset. http://www.caida.org/data/ passive/ddos-20070804-dataset.xml/ . Accessed 20 Oct 2019.
Caida anonymized internet traces 2008 dataset. https://www.caida.org/data/passive/passive-2008-dataset . Accessed 20 Oct 2019.
Isot botnet dataset. https://www.uvic.ca/engineering/ece/isot/ datasets/index.php/ . Accessed 20 Oct 2019.
The honeynet project. http://www.honeynet.org/chapters/france/ . Accessed 20 Oct 2019.
Canadian institute of cybersecurity, university of new brunswick, iscx dataset, http://www.unb.ca/cic/datasets/index.html/ . Accessed 20 Oct 2019.
Shiravi A, Shiravi H, Tavallaee M, Ghorbani AA. Toward developing a systematic approach to generate benchmark datasets for intrusion detection. Comput Secur. 2012;31(3):357–74.
The ctu-13 dataset. https://stratosphereips.org/category/datasets-ctu13 . Accessed 20 Oct 2019.
Moustafa N, Slay J. Unsw-nb15: a comprehensive data set for network intrusion detection systems (unsw-nb15 network data set). In: 2015 Military Communications and Information Systems Conference (MilCIS). IEEE; 2015. p. 1–6.
Cse-cic-ids2018 [online]. available: https://www.unb.ca/cic/ datasets/ids-2018.html/ . Accessed 20 Oct 2019.
Cic-ddos2019 [online]. available: https://www.unb.ca/cic/datasets/ddos-2019.html/ . Accessed 28 Mar 2019.
Jing X, Yan Z, Jiang X, Pedrycz W. Network traffic fusion and analysis against ddos flooding attacks with a novel reversible sketch. Inform Fusion. 2019;51:100–13.
Xie M, Hu J, Yu X, Chang E. Evaluating host-based anomaly detection systems: application of the frequency-based algorithms to adfa-ld. In: International conference on network and system security. New York: Springer; 2015. p. 542–49.
Lindauer B, Glasser J, Rosen M, Wallnau KC, ExactData L. Generating test data for insider threat detectors. JoWUA. 2014;5(2):80–94.
Glasser J, Lindauer B. Bridging the gap: A pragmatic approach to generating insider threat data. In: 2013 IEEE Security and Privacy Workshops. IEEE; 2013. p. 98–104.
Enronspam. https://labs-repos.iit.demokritos.gr/skel/i-config/downloads/enron-spam/ . Accessed 20 Oct 2019.
Spamassassin. http://www.spamassassin.org/publiccorpus/ . Accessed 20 Oct 2019.
Lingspam. https://labs-repos.iit.demokritos.gr/skel/i-config/downloads/lingspampublic.tar.gz/ . Accessed 20 Oct 2019.
Alexa top sites. https://aws.amazon.com/alexa-top-sites/ . Accessed 20 Oct 2019.
Bambenek consulting—master feeds. available online: http://osint.bambenekconsulting.com/feeds/ . Accessed 20 Oct 2019.
Dgarchive. https://dgarchive.caad.fkie.fraunhofer.de/site/ . Accessed 20 Oct 2019.
Zago M, Pérez MG, Pérez GM. Umudga: A dataset for profiling algorithmically generated domain names in botnet detection. Data in Brief. 2020;105400.
Zhou Y, Jiang X. Dissecting android malware: characterization and evolution. In: 2012 IEEE Symposium on security and privacy. IEEE; 2012. p. 95–109.
Virusshare. http://virusshare.com/ . Accessed 20 Oct 2019.
Virustotal. https://virustotal.com/ . Accessed 20 Oct 2019.
Comodo. https://www.comodo.com/home/internet-security/updates/vdp/database . Accessed 20 Oct 2019.
Contagio. http://contagiodump.blogspot.com/ . Accessed 20 Oct 2019.
Kumar R, Xiaosong Z, Khan RU, Kumar J, Ahad I. Effective and explainable detection of android malware based on machine learning algorithms. In: Proceedings of the 2018 international conference on computing and artificial intelligence. ACM; 2018. p. 35–40.
Microsoft malware classification (big 2015). arXiv:org/abs/1802.10135/ . Accessed 20 Oct 2019.
Koroniotis N, Moustafa N, Sitnikova E, Turnbull B. Towards the development of realistic botnet dataset in the internet of things for network forensic analytics: bot-iot dataset. Future Gen Comput Syst. 2019;100:779–96.
McIntosh TR, Jang-Jaccard J, Watters PA. Large scale behavioral analysis of ransomware attacks. In: International conference on neural information processing. New York: Springer; 2018. p. 217–29.
Han J, Pei J, Kamber M. Data mining: concepts and techniques, 2011.
Witten IH, Frank E. Data mining: Practical machine learning tools and techniques, 2005.
Dua S, Du X. Data mining and machine learning in cybersecurity, 2016.
Kotpalliwar MV, Wajgi R. Classification of attacks using support vector machine (svm) on kddcup’99 ids database. In: 2015 Fifth international conference on communication systems and network technologies. IEEE; 2015. p. 987–90.
Pervez MS, Farid DM. Feature selection and intrusion classification in nsl-kdd cup 99 dataset employing svms. In: The 8th international conference on software, knowledge, information management and applications (SKIMA 2014). IEEE; 2014. p. 1–6.
Yan M, Liu Z. A new method of transductive svm-based network intrusion detection. In: International conference on computer and computing technologies in agriculture. New York: Springer; 2010. p. 87–95.
Li Y, Xia J, Zhang S, Yan J, Ai X, Dai K. An efficient intrusion detection system based on support vector machines and gradually feature removal method. Expert Syst Appl. 2012;39(1):424–30.
Raman MG, Somu N, Jagarapu S, Manghnani T, Selvam T, Krithivasan K, Sriram VS. An efficient intrusion detection technique based on support vector machine and improved binary gravitational search algorithm. Artificial Intelligence Review. 2019, p. 1–32.
Kokila R, Selvi ST, Govindarajan K. Ddos detection and analysis in sdn-based environment using support vector machine classifier. In: 2014 Sixth international conference on advanced computing (ICoAC). IEEE; 2014. p. 205–10.
Xie M, Hu J, Slay J. Evaluating host-based anomaly detection systems: Application of the one-class svm algorithm to adfa-ld. In: 2014 11th international conference on fuzzy systems and knowledge discovery (FSKD). IEEE; 2014. p. 978–82.
Saxena H, Richariya V. Intrusion detection in kdd99 dataset using svm-pso and feature reduction with information gain. Int J Comput Appl. 2014;98:6.
Chandrasekhar A, Raghuveer K. Confederation of fcm clustering, ann and svm techniques to implement hybrid nids using corrected kdd cup 99 dataset. In: 2014 international conference on communication and signal processing. IEEE; 2014. p. 672–76.
Shapoorifard H, Shamsinejad P. Intrusion detection using a novel hybrid method incorporating an improved knn. Int J Comput Appl. 2017;173(1):5–9.
Vishwakarma S, Sharma V, Tiwari A. An intrusion detection system using knn-aco algorithm. Int J Comput Appl. 2017;171(10):18–23.
Meng W, Li W, Kwok L-F. Design of intelligent knn-based alarm filter using knowledge-based alert verification in intrusion detection. Secur Commun Netw. 2015;8(18):3883–95.
Dada E. A hybridized svm-knn-pdapso approach to intrusion detection system. In: Proc. Fac. Seminar Ser., 2017, p. 14–21.
Sharifi AM, Amirgholipour SK, Pourebrahimi A. Intrusion detection based on joint of k-means and knn. J Converg Inform Technol. 2015;10(5):42.
Lin W-C, Ke S-W, Tsai C-F. Cann: an intrusion detection system based on combining cluster centers and nearest neighbors. Knowl Based Syst. 2015;78:13–21.
Koc L, Mazzuchi TA, Sarkani S. A network intrusion detection system based on a hidden naïve bayes multiclass classifier. Exp Syst Appl. 2012;39(18):13492–500.
Moon D, Im H, Kim I, Park JH. Dtb-ids: an intrusion detection system based on decision tree using behavior analysis for preventing apt attacks. J Supercomput. 2017;73(7):2881–95.
Ingre, B., Yadav, A., Soni, A.K.: Decision tree based intrusion detection system for nsl-kdd dataset. In: International conference on information and communication technology for intelligent systems. New York: Springer; 2017. p. 207–18.
Malik AJ, Khan FA. A hybrid technique using binary particle swarm optimization and decision tree pruning for network intrusion detection. Cluster Comput. 2018;21(1):667–80.
Relan NG, Patil DR. Implementation of network intrusion detection system using variant of decision tree algorithm. In: 2015 international conference on nascent technologies in the engineering field (ICNTE). IEEE; 2015. p. 1–5.
Rai K, Devi MS, Guleria A. Decision tree based algorithm for intrusion detection. Int J Adv Netw Appl. 2016;7(4):2828.
Sarker IH, Abushark YB, Alsolami F, Khan AI. Intrudtree: a machine learning based cyber security intrusion detection model. Symmetry. 2020;12(5):754.
Puthran S, Shah K. Intrusion detection using improved decision tree algorithm with binary and quad split. In: International symposium on security in computing and communication. New York: Springer; 2016. p. 427–438.
Balogun AO, Jimoh RG. Anomaly intrusion detection using an hybrid of decision tree and k-nearest neighbor, 2015.
Azad C, Jha VK. Genetic algorithm to solve the problem of small disjunct in the decision tree based intrusion detection system. Int J Comput Netw Inform Secur. 2015;7(8):56.
Jo S, Sung H, Ahn B. A comparative study on the performance of intrusion detection using decision tree and artificial neural network models. J Korea Soc Dig Indus Inform Manag. 2015;11(4):33–45.
Zhan J, Zulkernine M, Haque A. Random-forests-based network intrusion detection systems. IEEE Trans Syst Man Cybern C. 2008;38(5):649–59.
Tajbakhsh A, Rahmati M, Mirzaei A. Intrusion detection using fuzzy association rules. Appl Soft Comput. 2009;9(2):462–9.
Mitchell R, Chen R. Behavior rule specification-based intrusion detection for safety critical medical cyber physical systems. IEEE Trans Depend Secure Comput. 2014;12(1):16–30.
Alazab M, Venkataraman S, Watters P. Towards understanding malware behaviour by the extraction of api calls. In: 2010 second cybercrime and trustworthy computing Workshop. IEEE; 2010. p. 52–59.
Yuan Y, Kaklamanos G, Hogrefe D. A novel semi-supervised adaboost technique for network anomaly detection. In: Proceedings of the 19th ACM international conference on modeling, analysis and simulation of wireless and mobile systems. ACM; 2016. p. 111–14.
Ariu D, Tronci R, Giacinto G. Hmmpayl: an intrusion detection system based on hidden markov models. Comput Secur. 2011;30(4):221–41.
Årnes A, Valeur F, Vigna G, Kemmerer RA. Using hidden markov models to evaluate the risks of intrusions. In: International workshop on recent advances in intrusion detection. New York: Springer; 2006. p. 145–64.
Hansen JV, Lowry PB, Meservy RD, McDonald DM. Genetic programming for prevention of cyberterrorism through dynamic and evolving intrusion detection. Decis Supp Syst. 2007;43(4):1362–74.
Aslahi-Shahri B, Rahmani R, Chizari M, Maralani A, Eslami M, Golkar MJ, Ebrahimi A. A hybrid method consisting of ga and svm for intrusion detection system. Neural Comput Appl. 2016;27(6):1669–76.
Alrawashdeh K, Purdy C. Toward an online anomaly intrusion detection system based on deep learning. In: 2016 15th IEEE international conference on machine learning and applications (ICMLA). IEEE; 2016. p. 195–200.
Yin C, Zhu Y, Fei J, He X. A deep learning approach for intrusion detection using recurrent neural networks. IEEE Access. 2017;5:21954–61.
Kim J, Kim J, Thu HLT, Kim H. Long short term memory recurrent neural network classifier for intrusion detection. In: 2016 international conference on platform technology and service (PlatCon). IEEE; 2016. p. 1–5.
Almiani M, AbuGhazleh A, Al-Rahayfeh A, Atiewi S, Razaque A. Deep recurrent neural network for iot intrusion detection system. Simulation Modelling Practice and Theory. 2019;102031.
Kolosnjaji B, Zarras A, Webster G, Eckert C. Deep learning for classification of malware system call sequences. In: Australasian joint conference on artificial intelligence. New York: Springer; 2016. p. 137–49.
Wang W, Zhu M, Zeng X, Ye X, Sheng Y. Malware traffic classification using convolutional neural network for representation learning. In: 2017 international conference on information networking (ICOIN). IEEE; 2017. p. 712–17.
Alauthman M, Aslam N, Al-kasassbeh M, Khan S, Al-Qerem A, Choo K-KR. An efficient reinforcement learning-based botnet detection approach. J Netw Comput Appl. 2020;150:102479.
Blanco R, Cilla JJ, Briongos S, Malagón P, Moya JM. Applying cost-sensitive classifiers with reinforcement learning to ids. In: International conference on intelligent data engineering and automated learning. New York: Springer; 2018. p. 531–38.
Lopez-Martin M, Carro B, Sanchez-Esguevillas A. Application of deep reinforcement learning to intrusion detection for supervised problems. Exp Syst Appl. 2020;141:112963.
Sarker IH, Kayes A, Watters P. Effectiveness analysis of machine learning classification models for predicting personalized context-aware smartphone usage. J Big Data. 2019;6(1):1–28.
Holte RC. Very simple classification rules perform well on most commonly used datasets. Mach Learn. 1993;11(1):63–90.
John GH, Langley P. Estimating continuous distributions in bayesian classifiers. In: Proceedings of the eleventh conference on uncertainty in artificial intelligence. Morgan Kaufmann Publishers Inc.; 1995. p. 338–45.
Quinlan JR. C4.5: Programs for machine learning. Machine Learning, 1993.
Sarker IH, Colman A, Han J, Khan AI, Abushark YB, Salah K. Behavdt: a behavioral decision tree learning to build user-centric context-aware predictive model. Mobile Networks and Applications. 2019, p. 1–11.
Aha DW, Kibler D, Albert MK. Instance-based learning algorithms. Mach Learn. 1991;6(1):37–66.
Keerthi SS, Shevade SK, Bhattacharyya C, Murthy KRK. Improvements to platt’s smo algorithm for svm classifier design. Neural Comput. 2001;13(3):637–49.
Freund Y, Schapire RE, et al: Experiments with a new boosting algorithm. In: Icml, vol. 96, p. 148–156 (1996). Citeseer
Le Cessie S, Van Houwelingen JC. Ridge estimators in logistic regression. J Royal Stat Soc C. 1992;41(1):191–201.
Watters PA, McCombie S, Layton R, Pieprzyk J. Characterising and predicting cyber attacks using the cyber attacker model profile (camp). J Money Launder Control. 2012.
Breiman L. Random forests. Mach Learn. 2001;45(1):5–32.
Sarker IH. Context-aware rule learning from smartphone data: survey, challenges and future directions. J Big Data. 2019;6(1):95.
MacQueen J. Some methods for classification and analysis of multivariate observations. In: Fifth Berkeley symposium on mathematical statistics and probability, vol. 1, 1967.
Rokach L. A survey of clustering algorithms. In: Data Mining and Knowledge Discovery Handbook. New York: Springer; 2010. p. 269–98.
Sneath PH. The application of computers to taxonomy. J Gen Microbiol. 1957;17:1.
Sorensen T. method of establishing groups of equal amplitude in plant sociology based on similarity of species. Biol Skr. 1948;5.
Sarker IH, Colman A, Kabir MA, Han J. Individualized time-series segmentation for mining mobile phone user behavior. Comput J. 2018;61(3):349–68.
Kim G, Lee S, Kim S. A novel hybrid intrusion detection method integrating anomaly detection with misuse detection. Exp Syst Appl. 2014;41(4):1690–700.
MathSciNet Google Scholar
Agrawal R, Imieliński T, Swami A. Mining association rules between sets of items in large databases. In: ACM SIGMOD Record. ACM; 1993. vol. 22, p. 207–16.
Flach PA, Lachiche N. Confirmation-guided discovery of first-order rules with tertius. Mach Learn. 2001;42(1–2):61–95.
Agrawal R, Srikant R, et al: Fast algorithms for mining association rules. In: Proc. 20th Int. Conf. Very Large Data Bases, VLDB, 1994, vol. 1215, p. 487–99.
Houtsma M, Swami A. Set-oriented mining for association rules in relational databases. In: Proceedings of the eleventh international conference on data engineering. IEEE; 1995. p. 25–33.
Ma BLWHY. Integrating classification and association rule mining. In: Proceedings of the fourth international conference on knowledge discovery and data mining, 1998.
Han J, Pei J, Yin Y. Mining frequent patterns without candidate generation. In: ACM Sigmod Record. ACM; 2000. vol. 29, p. 1–12.
Sarker IH, Salim FD. Mining user behavioral rules from smartphone data through association analysis. In: Proceedings of the 22nd Pacific-Asia Conference on Knowledge Discovery and Data Mining (PAKDD), Melbourne, Australia. New York: Springer; 2018. p. 450–61.
Das A, Ng W-K, Woon Y-K. Rapid association rule mining. In: Proceedings of the tenth international conference on information and knowledge management. ACM; 2001. p. 474–81.
Zaki MJ. Scalable algorithms for association mining. IEEE Trans Knowl Data Eng. 2000;12(3):372–90.
Coelho IM, Coelho VN, Luz EJS, Ochi LS, Guimarães FG, Rios E. A gpu deep learning metaheuristic based model for time series forecasting. Appl Energy. 2017;201:412–8.
Van Efferen L, Ali-Eldin AM. A multi-layer perceptron approach for flow-based anomaly detection. In: 2017 International symposium on networks, computers and communications (ISNCC). IEEE; 2017. p. 1–6.
Liu H, Lang B, Liu M, Yan H. Cnn and rnn based payload classification methods for attack detection. Knowl Based Syst. 2019;163:332–41.
Berman DS, Buczak AL, Chavis JS, Corbett CL. A survey of deep learning methods for cyber security. Information. 2019;10(4):122.
Bellman R. A markovian decision process. J Math Mech. 1957;1:679–84.
Kaelbling LP, Littman ML, Moore AW. Reinforcement learning: a survey. J Artif Intell Res. 1996;4:237–85.
Sarker IH. A machine learning based robust prediction model for real-life mobile phone data. Internet of Things. 2019;5:180–93.
Kayes ASM, Han J, Colman A. OntCAAC: an ontology-based approach to context-aware access control for software services. Comput J. 2015;58(11):3000–34.
Kayes ASM, Rahayu W, Dillon T. An ontology-based approach to dynamic contextual role for pervasive access control. In: AINA 2018. IEEE Computer Society, 2018.
Colombo P, Ferrari E. Access control technologies for big data management systems: literature review and future trends. Cybersecurity. 2019;2(1):1–13.
Aleroud A, Karabatis G. Contextual information fusion for intrusion detection: a survey and taxonomy. Knowl Inform Syst. 2017;52(3):563–619.
Sarker IH, Abushark YB, Khan AI. Contextpca: Predicting context-aware smartphone apps usage based on machine learning techniques. Symmetry. 2020;12(4):499.
Madsen RE, Hansen LK, Winther O. Singular value decomposition and principal component analysis. Neural Netw. 2004;1:1–5.
Qiao L-B, Zhang B-F, Lai Z-Q, Su J-S. Mining of attack models in ids alerts from network backbone by a two-stage clustering method. In: 2012 IEEE 26th international parallel and distributed processing symposium workshops & Phd Forum. IEEE; 2012. p. 1263–9.
Sarker IH, Colman A, Han J. Recencyminer: mining recency-based personalized behavior from contextual smartphone data. J Big Data. 2019;6(1):49.
Ullah F, Babar MA. Architectural tactics for big data cybersecurity analytics systems: a review. J Syst Softw. 2019;151:81–118.
Zhao S, Leftwich K, Owens M, Magrone F, Schonemann J, Anderson B, Medhi D. I-can-mama: Integrated campus network monitoring and management. In: 2014 IEEE network operations and management symposium (NOMS). IEEE; 2014. p. 1–7.
Abomhara M, et al. Cyber security and the internet of things: vulnerabilities, threats, intruders and attacks. J Cyber Secur Mob. 2015;4(1):65–88.
Helali RGM. Data mining based network intrusion detection system: A survey. In: Novel algorithms and techniques in telecommunications and networking. New York: Springer; 2010. p. 501–505.
Ryoo J, Rizvi S, Aiken W, Kissell J. Cloud security auditing: challenges and emerging approaches. IEEE Secur Priv. 2013;12(6):68–74.
Densham B. Three cyber-security strategies to mitigate the impact of a data breach. Netw Secur. 2015;2015(1):5–8.
Salah K, Rehman MHU, Nizamuddin N, Al-Fuqaha A. Blockchain for ai: review and open research challenges. IEEE Access. 2019;7:10127–49.
Gandomi A, Haider M. Beyond the hype: big data concepts, methods, and analytics. Int J Inform Manag. 2015;35(2):137–44.
Golchha N. Big data-the information revolution. Int J Adv Res. 2015;1(12):791–4.
Hariri RH, Fredericks EM, Bowers KM. Uncertainty in big data analytics: survey, opportunities, and challenges. J Big Data. 2019;6(1):44.
Tsai C-W, Lai C-F, Chao H-C, Vasilakos AV. Big data analytics: a survey. J Big data. 2015;2(1):21.
Download references
Acknowledgements
The authors would like to thank all the reviewers for their rigorous review and comments in several revision rounds. The reviews are detailed and helpful to improve and finalize the manuscript. The authors are highly grateful to them.
Author information
Authors and affiliations.
Swinburne University of Technology, Melbourne, VIC, 3122, Australia
Iqbal H. Sarker
Chittagong University of Engineering and Technology, Chittagong, 4349, Bangladesh
La Trobe University, Melbourne, VIC, 3086, Australia
A. S. M. Kayes, Paul Watters & Alex Ng
University of Nevada, Reno, USA
Shahriar Badsha
Macquarie University, Sydney, NSW, 2109, Australia
Hamed Alqahtani
You can also search for this author in PubMed Google Scholar
Contributions
This article provides not only a discussion on cybersecurity data science and relevant methods but also to discuss the applicability towards data-driven intelligent decision making in cybersecurity systems and services. All authors read and approved the final manuscript.
Corresponding author
Correspondence to Iqbal H. Sarker .
Ethics declarations
Competing interests.
The authors declare that they have no competing interests.
Additional information
Publisher's note.
Springer Nature remains neutral with regard to jurisdictional claims in published maps and institutional affiliations.
Rights and permissions
Open Access This article is licensed under a Creative Commons Attribution 4.0 International License, which permits use, sharing, adaptation, distribution and reproduction in any medium or format, as long as you give appropriate credit to the original author(s) and the source, provide a link to the Creative Commons licence, and indicate if changes were made. The images or other third party material in this article are included in the article's Creative Commons licence, unless indicated otherwise in a credit line to the material. If material is not included in the article's Creative Commons licence and your intended use is not permitted by statutory regulation or exceeds the permitted use, you will need to obtain permission directly from the copyright holder. To view a copy of this licence, visit http://creativecommons.org/licenses/by/4.0/ .
Reprints and permissions
About this article
Cite this article.
Sarker, I.H., Kayes, A.S.M., Badsha, S. et al. Cybersecurity data science: an overview from machine learning perspective. J Big Data 7 , 41 (2020). https://doi.org/10.1186/s40537-020-00318-5
Download citation
Received : 26 October 2019
Accepted : 21 June 2020
Published : 01 July 2020
DOI : https://doi.org/10.1186/s40537-020-00318-5
Share this article
Anyone you share the following link with will be able to read this content:
Sorry, a shareable link is not currently available for this article.
Provided by the Springer Nature SharedIt content-sharing initiative
- Decision making
- Cyber-attack
- Security modeling
- Intrusion detection
- Cyber threat intelligence
- Privacy Policy
Buy Me a Coffee

Home » 500+ Cyber Security Research Topics
500+ Cyber Security Research Topics

Cybersecurity has become an increasingly important topic in recent years as more and more of our lives are spent online. With the rise of the digital age, there has been a corresponding increase in the number and severity of cyber attacks. As such, research into cybersecurity has become critical in order to protect individuals, businesses, and governments from these threats. In this blog post, we will explore some of the most pressing cybersecurity research topics, from the latest trends in cyber attacks to emerging technologies that can help prevent them. Whether you are a cybersecurity professional, a Master’s or Ph.D. student, or simply interested in the field, this post will provide valuable insights into the challenges and opportunities in this rapidly evolving area of study.
Cyber Security Research Topics
Cyber Security Research Topics are as follows:
- The role of machine learning in detecting cyber threats
- The impact of cloud computing on cyber security
- Cyber warfare and its effects on national security
- The rise of ransomware attacks and their prevention methods
- Evaluating the effectiveness of network intrusion detection systems
- The use of blockchain technology in enhancing cyber security
- Investigating the role of cyber security in protecting critical infrastructure
- The ethics of hacking and its implications for cyber security professionals
- Developing a secure software development lifecycle (SSDLC)
- The role of artificial intelligence in cyber security
- Evaluating the effectiveness of multi-factor authentication
- Investigating the impact of social engineering on cyber security
- The role of cyber insurance in mitigating cyber risks
- Developing secure IoT (Internet of Things) systems
- Investigating the challenges of cyber security in the healthcare industry
- Evaluating the effectiveness of penetration testing
- Investigating the impact of big data on cyber security
- The role of quantum computing in breaking current encryption methods
- Developing a secure BYOD (Bring Your Own Device) policy
- The impact of cyber security breaches on a company’s reputation
- The role of cyber security in protecting financial transactions
- Evaluating the effectiveness of anti-virus software
- The use of biometrics in enhancing cyber security
- Investigating the impact of cyber security on the supply chain
- The role of cyber security in protecting personal privacy
- Developing a secure cloud storage system
- Evaluating the effectiveness of firewall technologies
- Investigating the impact of cyber security on e-commerce
- The role of cyber security in protecting intellectual property
- Developing a secure remote access policy
- Investigating the challenges of securing mobile devices
- The role of cyber security in protecting government agencies
- Evaluating the effectiveness of cyber security training programs
- Investigating the impact of cyber security on the aviation industry
- The role of cyber security in protecting online gaming platforms
- Developing a secure password management system
- Investigating the challenges of securing smart homes
- The impact of cyber security on the automotive industry
- The role of cyber security in protecting social media platforms
- Developing a secure email system
- Evaluating the effectiveness of encryption methods
- Investigating the impact of cyber security on the hospitality industry
- The role of cyber security in protecting online education platforms
- Developing a secure backup and recovery strategy
- Investigating the challenges of securing virtual environments
- The impact of cyber security on the energy sector
- The role of cyber security in protecting online voting systems
- Developing a secure chat platform
- Investigating the impact of cyber security on the entertainment industry
- The role of cyber security in protecting online dating platforms
- Artificial Intelligence and Machine Learning in Cybersecurity
- Quantum Cryptography and Post-Quantum Cryptography
- Internet of Things (IoT) Security
- Developing a framework for cyber resilience in critical infrastructure
- Understanding the fundamentals of encryption algorithms
- Cyber security challenges for small and medium-sized businesses
- Developing secure coding practices for web applications
- Investigating the role of cyber security in protecting online privacy
- Network security protocols and their importance
- Social engineering attacks and how to prevent them
- Investigating the challenges of securing personal devices and home networks
- Developing a basic incident response plan for cyber attacks
- The impact of cyber security on the financial sector
- Understanding the role of cyber security in protecting critical infrastructure
- Mobile device security and common vulnerabilities
- Investigating the challenges of securing cloud-based systems
- Cyber security and the Internet of Things (IoT)
- Biometric authentication and its role in cyber security
- Developing secure communication protocols for online messaging platforms
- The importance of cyber security in e-commerce
- Understanding the threats and vulnerabilities associated with social media platforms
- Investigating the role of cyber security in protecting intellectual property
- The basics of malware analysis and detection
- Developing a basic cyber security awareness training program
- Understanding the threats and vulnerabilities associated with public Wi-Fi networks
- Investigating the challenges of securing online banking systems
- The importance of password management and best practices
- Cyber security and cloud computing
- Understanding the role of cyber security in protecting national security
- Investigating the challenges of securing online gaming platforms
- The basics of cyber threat intelligence
- Developing secure authentication mechanisms for online services
- The impact of cyber security on the healthcare sector
- Understanding the basics of digital forensics
- Investigating the challenges of securing smart home devices
- The role of cyber security in protecting against cyberbullying
- Developing secure file transfer protocols for sensitive information
- Understanding the challenges of securing remote work environments
- Investigating the role of cyber security in protecting against identity theft
- The basics of network intrusion detection and prevention systems
- Developing secure payment processing systems
- Understanding the role of cyber security in protecting against ransomware attacks
- Investigating the challenges of securing public transportation systems
- The basics of network segmentation and its importance in cyber security
- Developing secure user access management systems
- Understanding the challenges of securing supply chain networks
- The role of cyber security in protecting against cyber espionage
- Investigating the challenges of securing online educational platforms
- The importance of data backup and disaster recovery planning
- Developing secure email communication protocols
- Understanding the basics of threat modeling and risk assessment
- Investigating the challenges of securing online voting systems
- The role of cyber security in protecting against cyber terrorism
- Developing secure remote access protocols for corporate networks.
- Investigating the challenges of securing artificial intelligence systems
- The role of machine learning in enhancing cyber threat intelligence
- Evaluating the effectiveness of deception technologies in cyber security
- Investigating the impact of cyber security on the adoption of emerging technologies
- The role of cyber security in protecting smart cities
- Developing a risk-based approach to cyber security governance
- Investigating the impact of cyber security on economic growth and innovation
- The role of cyber security in protecting human rights in the digital age
- Developing a secure digital identity system
- Investigating the impact of cyber security on global political stability
- The role of cyber security in protecting the Internet of Things (IoT)
- Developing a secure supply chain management system
- Investigating the challenges of securing cloud-native applications
- The role of cyber security in protecting against insider threats
- Developing a secure software-defined network (SDN)
- Investigating the impact of cyber security on the adoption of mobile payments
- The role of cyber security in protecting against cyber warfare
- Developing a secure distributed ledger technology (DLT) system
- Investigating the impact of cyber security on the digital divide
- The role of cyber security in protecting against state-sponsored attacks
- Developing a secure Internet infrastructure
- Investigating the challenges of securing industrial control systems (ICS)
- Developing a secure quantum communication system
- Investigating the impact of cyber security on global trade and commerce
- Developing a secure decentralized authentication system
- Investigating the challenges of securing edge computing systems
- Developing a secure hybrid cloud system
- Investigating the impact of cyber security on the adoption of smart cities
- The role of cyber security in protecting against cyber propaganda
- Developing a secure blockchain-based voting system
- Investigating the challenges of securing cyber-physical systems (CPS)
- The role of cyber security in protecting against cyber hate speech
- Developing a secure machine learning system
- Investigating the impact of cyber security on the adoption of autonomous vehicles
- The role of cyber security in protecting against cyber stalking
- Developing a secure data-driven decision-making system
- Investigating the challenges of securing social media platforms
- The role of cyber security in protecting against cyberbullying in schools
- Developing a secure open source software ecosystem
- Investigating the impact of cyber security on the adoption of smart homes
- The role of cyber security in protecting against cyber fraud
- Developing a secure software supply chain
- Investigating the challenges of securing cloud-based healthcare systems
- The role of cyber security in protecting against cyber harassment
- Developing a secure multi-party computation system
- Investigating the impact of cyber security on the adoption of virtual and augmented reality technologies.
- Cybersecurity in Cloud Computing Environments
- Cyber Threat Intelligence and Analysis
- Blockchain Security
- Data Privacy and Protection
- Cybersecurity in Industrial Control Systems
- Mobile Device Security
- The importance of cyber security in the digital age
- The ethics of cyber security and privacy
- The role of government in regulating cyber security
- Cyber security threats and vulnerabilities in the healthcare sector
- Understanding the risks associated with social media and cyber security
- The impact of cyber security on e-commerce
- The effectiveness of cyber security awareness training programs
- The role of biometric authentication in cyber security
- The importance of password management in cyber security
- The basics of network security protocols and their importance
- The challenges of securing online gaming platforms
- The role of cyber security in protecting national security
- The impact of cyber security on the legal sector
- The ethics of cyber warfare
- The challenges of securing the Internet of Things (IoT)
- Understanding the basics of malware analysis and detection
- The challenges of securing public transportation systems
- The impact of cyber security on the insurance industry
- The role of cyber security in protecting against ransomware attacks
- The challenges of securing remote work environments
- Understanding the threats and vulnerabilities associated with social engineering attacks
- The impact of cyber security on the education sector
- Investigating the challenges of securing supply chain networks
- The challenges of securing personal devices and home networks
- The importance of secure coding practices for web applications
- The impact of cyber security on the hospitality industry
- The role of cyber security in protecting against identity theft
- The challenges of securing public Wi-Fi networks
- The importance of cyber security in protecting critical infrastructure
- The challenges of securing cloud-based storage systems
- The effectiveness of antivirus software in cyber security
- Developing secure payment processing systems.
- Cybersecurity in Healthcare
- Social Engineering and Phishing Attacks
- Cybersecurity in Autonomous Vehicles
- Cybersecurity in Smart Cities
- Cybersecurity Risk Assessment and Management
- Malware Analysis and Detection Techniques
- Cybersecurity in the Financial Sector
- Cybersecurity in Government Agencies
- Cybersecurity and Artificial Life
- Cybersecurity for Critical Infrastructure Protection
- Cybersecurity in the Education Sector
- Cybersecurity in Virtual Reality and Augmented Reality
- Cybersecurity in the Retail Industry
- Cryptocurrency Security
- Cybersecurity in Supply Chain Management
- Cybersecurity and Human Factors
- Cybersecurity in the Transportation Industry
- Cybersecurity in Gaming Environments
- Cybersecurity in Social Media Platforms
- Cybersecurity and Biometrics
- Cybersecurity and Quantum Computing
- Cybersecurity in 5G Networks
- Cybersecurity in Aviation and Aerospace Industry
- Cybersecurity in Agriculture Industry
- Cybersecurity in Space Exploration
- Cybersecurity in Military Operations
- Cybersecurity and Cloud Storage
- Cybersecurity in Software-Defined Networks
- Cybersecurity and Artificial Intelligence Ethics
- Cybersecurity and Cyber Insurance
- Cybersecurity in the Legal Industry
- Cybersecurity and Data Science
- Cybersecurity in Energy Systems
- Cybersecurity in E-commerce
- Cybersecurity in Identity Management
- Cybersecurity in Small and Medium Enterprises
- Cybersecurity in the Entertainment Industry
- Cybersecurity and the Internet of Medical Things
- Cybersecurity and the Dark Web
- Cybersecurity and Wearable Technology
- Cybersecurity in Public Safety Systems.
- Threat Intelligence for Industrial Control Systems
- Privacy Preservation in Cloud Computing
- Network Security for Critical Infrastructure
- Cryptographic Techniques for Blockchain Security
- Malware Detection and Analysis
- Cyber Threat Hunting Techniques
- Cybersecurity Risk Assessment
- Machine Learning for Cybersecurity
- Cybersecurity in Financial Institutions
- Cybersecurity for Smart Cities
- Cybersecurity in Aviation
- Cybersecurity in the Automotive Industry
- Cybersecurity in the Energy Sector
- Cybersecurity in Telecommunications
- Cybersecurity for Mobile Devices
- Biometric Authentication for Cybersecurity
- Cybersecurity for Artificial Intelligence
- Cybersecurity for Social Media Platforms
- Cybersecurity in the Gaming Industry
- Cybersecurity in the Defense Industry
- Cybersecurity for Autonomous Systems
- Cybersecurity for Quantum Computing
- Cybersecurity for Augmented Reality and Virtual Reality
- Cybersecurity in Cloud-Native Applications
- Cybersecurity for Smart Grids
- Cybersecurity in Distributed Ledger Technology
- Cybersecurity for Next-Generation Wireless Networks
- Cybersecurity for Digital Identity Management
- Cybersecurity for Open Source Software
- Cybersecurity for Smart Homes
- Cybersecurity for Smart Transportation Systems
- Cybersecurity for Cyber Physical Systems
- Cybersecurity for Critical National Infrastructure
- Cybersecurity for Smart Agriculture
- Cybersecurity for Retail Industry
- Cybersecurity for Digital Twins
- Cybersecurity for Quantum Key Distribution
- Cybersecurity for Digital Healthcare
- Cybersecurity for Smart Logistics
- Cybersecurity for Wearable Devices
- Cybersecurity for Edge Computing
- Cybersecurity for Cognitive Computing
- Cybersecurity for Industrial IoT
- Cybersecurity for Intelligent Transportation Systems
- Cybersecurity for Smart Water Management Systems
- The rise of cyber terrorism and its impact on national security
- The impact of artificial intelligence on cyber security
- Analyzing the effectiveness of biometric authentication for securing data
- The impact of social media on cyber security and privacy
- The future of cyber security in the Internet of Things (IoT) era
- The role of machine learning in detecting and preventing cyber attacks
- The effectiveness of encryption in securing sensitive data
- The impact of quantum computing on cyber security
- The rise of cyber bullying and its effects on mental health
- Investigating cyber espionage and its impact on national security
- The effectiveness of cyber insurance in mitigating cyber risks
- The role of blockchain technology in cyber security
- Investigating the effectiveness of cyber security awareness training programs
- The impact of cyber attacks on critical infrastructure
- Analyzing the effectiveness of firewalls in protecting against cyber attacks
- The impact of cyber crime on the economy
- Investigating the effectiveness of multi-factor authentication in securing data
- The future of cyber security in the age of quantum internet
- The impact of big data on cyber security
- The role of cybersecurity in the education system
- Investigating the use of deception techniques in cyber security
- The impact of cyber attacks on the healthcare industry
- The effectiveness of cyber threat intelligence in mitigating cyber risks
- The role of cyber security in protecting financial institutions
- Investigating the use of machine learning in cyber security risk assessment
- The impact of cyber attacks on the transportation industry
- The effectiveness of network segmentation in protecting against cyber attacks
- Investigating the effectiveness of biometric identification in cyber security
- The impact of cyber attacks on the hospitality industry
- The future of cyber security in the era of autonomous vehicles
- The effectiveness of intrusion detection systems in protecting against cyber attacks
- The role of cyber security in protecting small businesses
- Investigating the effectiveness of virtual private networks (VPNs) in securing data
- The impact of cyber attacks on the energy sector
- The effectiveness of cyber security regulations in mitigating cyber risks
- Investigating the use of deception technology in cyber security
- The impact of cyber attacks on the retail industry
- The effectiveness of cyber security in protecting critical infrastructure
- The role of cyber security in protecting intellectual property in the entertainment industry
- Investigating the effectiveness of intrusion prevention systems in protecting against cyber attacks
- The impact of cyber attacks on the aerospace industry
- The future of cyber security in the era of quantum computing
- The effectiveness of cyber security in protecting against ransomware attacks
- The role of cyber security in protecting personal and sensitive data
- Investigating the effectiveness of cloud security solutions in protecting against cyber attacks
- The impact of cyber attacks on the manufacturing industry
- The effective cyber security and the future of e-votingness of cyber security in protecting against social engineering attacks
- Investigating the effectiveness of end-to-end encryption in securing data
- The impact of cyber attacks on the insurance industry
- The future of cyber security in the era of artificial intelligence
- The effectiveness of cyber security in protecting against distributed denial-of-service (DDoS) attacks
- The role of cyber security in protecting against phishing attacks
- Investigating the effectiveness of user behavior analytics
- The impact of emerging technologies on cyber security
- Developing a framework for cyber threat intelligence
- The effectiveness of current cyber security measures
- Cyber security and data privacy in the age of big data
- Cloud security and virtualization technologies
- Cryptography and its role in cyber security
- Cyber security in critical infrastructure protection
- Cyber security in the Internet of Things (IoT)
- Cyber security in e-commerce and online payment systems
- Cyber security and the future of digital currencies
- The impact of social engineering on cyber security
- Cyber security and ethical hacking
- Cyber security challenges in the healthcare industry
- Cyber security and digital forensics
- Cyber security in the financial sector
- Cyber security in the transportation industry
- The impact of artificial intelligence on cyber security risks
- Cyber security and mobile devices
- Cyber security in the energy sector
- Cyber security and supply chain management
- The role of machine learning in cyber security
- Cyber security in the defense sector
- The impact of the Dark Web on cyber security
- Cyber security in social media and online communities
- Cyber security challenges in the gaming industry
- Cyber security and cloud-based applications
- The role of blockchain in cyber security
- Cyber security and the future of autonomous vehicles
- Cyber security in the education sector
- Cyber security in the aviation industry
- The impact of 5G on cyber security
- Cyber security and insider threats
- Cyber security and the legal system
- The impact of cyber security on business operations
- Cyber security and the role of human behavior
- Cyber security in the hospitality industry
- The impact of cyber security on national security
- Cyber security and the use of biometrics
- Cyber security and the role of social media influencers
- The impact of cyber security on small and medium-sized enterprises
- Cyber security and cyber insurance
- The impact of cyber security on the job market
- Cyber security and international relations
- Cyber security and the role of government policies
- The impact of cyber security on privacy laws
- Cyber security in the media and entertainment industry
- The role of cyber security in digital marketing
- Cyber security and the role of cybersecurity professionals
- Cyber security in the retail industry
- The impact of cyber security on the stock market
- Cyber security and intellectual property protection
- Cyber security and online dating
- The impact of cyber security on healthcare innovation
- Cyber security and the future of e-voting
- Cyber security and the role of open source software
- Cyber security and the use of social engineering in cyber attacks
- The impact of cyber security on the aviation industry
- Cyber security and the role of cyber security awareness training
- Cyber security and the role of cybersecurity standards and best practices
- Cyber security in the legal industry
- The impact of cyber security on human rights
- Cyber security and the role of public-private partnerships
- Cyber security and the future of e-learning
- Cyber security and the role of mobile applications
- The impact of cyber security on environmental sustainability
- Cyber security and the role of threat intelligence sharing
- Cyber security and the future of smart homes
- Cyber security and the role of cybersecurity certifications
- The impact of cyber security on international trade
- Cyber security and the role of cyber security auditing
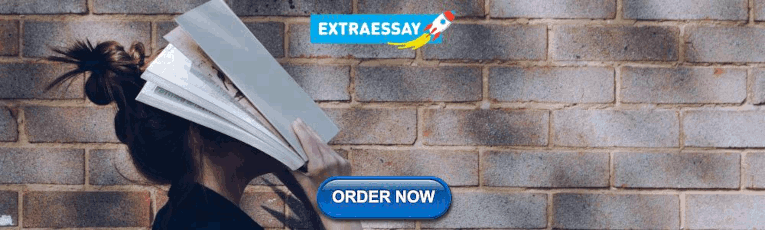
About the author
Muhammad Hassan
Researcher, Academic Writer, Web developer
You may also like

200+ Funny Research Topics

500+ Sports Research Topics

500+ Environmental Research Topics

500+ Economics Research Topics

500+ Physics Research Topics

500+ Google Scholar Research Topics
network security Recently Published Documents
Total documents.
- Latest Documents
- Most Cited Documents
- Contributed Authors
- Related Sources
- Related Keywords
A Survey on Ransomware Malware and Ransomware Detection Techniques
Abstract: is a kind of malignant programming (malware) that takes steps to distribute or hinders admittance to information or a PC framework, for the most part by scrambling it, until the casualty pays a payoff expense to the assailant. As a rule, the payoff request accompanies a cutoff time. Assuming that the casualty doesn't pay on schedule, the information is gone perpetually or the payoff increments. Presently days and assailants executed new strategies for effective working of assault. In this paper, we center around ransomware network assaults and study of discovery procedures for deliver product assault. There are different recognition methods or approaches are accessible for identification of payment product assault. Keywords: Network Security, Malware, Ransomware, Ransomware Detection Techniques
Analysis and Evaluation of Wireless Network Security with the Penetration Testing Execution Standard (PTES)
The use of computer networks in an agency aims to facilitate communication and data transfer between devices. The network that can be applied can be using wireless media or LAN cable. At SMP XYZ, most of the computers still use wireless networks. Based on the findings in the field, it was found that there was no user management problem. Therefore, an analysis and audit of the network security system is needed to ensure that the network security system at SMP XYZ is safe and running well. In conducting this analysis, a tool is needed which will be used as a benchmark to determine the security of the wireless network. The tools used are Penetration Testing Execution Standard (PTES) which is one of the tools to become a standard in analyzing or auditing network security systems in a company in this case, namely analyzing and auditing wireless network security systems. After conducting an analysis based on these tools, there are still many security holes in the XYZ wireless SMP that allow outsiders to illegally access and obtain vulnerabilities in terms of WPA2 cracking, DoS, wireless router password cracking, and access point isolation so that it can be said that network security at SMP XYZ is still not safe
A Sensing Method of Network Security Situation Based on Markov Game Model
The sensing of network security situation (NSS) has become a hot issue. This paper first describes the basic principle of Markov model and then the necessary and sufficient conditions for the application of Markov game model. And finally, taking fuzzy comprehensive evaluation model as the theoretical basis, this paper analyzes the application fields of the sensing method of NSS with Markov game model from the aspects of network randomness, non-cooperative and dynamic evolution. Evaluation results show that the sensing method of NSS with Markov game model is best for financial field, followed by educational field. In addition, the model can also be used in the applicability evaluation of the sensing methods of different industries’ network security situation. Certainly, in different categories, and under the premise of different sensing methods of network security situation, the proportions of various influencing factors are different, and once the proportion is unreasonable, it will cause false calculation process and thus affect the results.
The Compound Prediction Analysis of Information Network Security Situation based on Support Vector Combined with BP Neural Network Learning Algorithm
In order to solve the problem of low security of data in network transmission and inaccurate prediction of future security situation, an improved neural network learning algorithm is proposed in this paper. The algorithm makes up for the shortcomings of the standard neural network learning algorithm, eliminates the redundant data by vector support, and realizes the effective clustering of information data. In addition, the improved neural network learning algorithm uses the order of data to optimize the "end" data in the standard neural network learning algorithm, so as to improve the accuracy and computational efficiency of network security situation prediction.MATLAB simulation results show that the data processing capacity of support vector combined BP neural network is consistent with the actual security situation data requirements, the consistency can reach 98%. the consistency of the security situation results can reach 99%, the composite prediction time of the whole security situation is less than 25s, the line segment slope change can reach 2.3% ,and the slope change range can reach 1.2%,, which is better than BP neural network algorithm.
Network intrusion detection using oversampling technique and machine learning algorithms
The expeditious growth of the World Wide Web and the rampant flow of network traffic have resulted in a continuous increase of network security threats. Cyber attackers seek to exploit vulnerabilities in network architecture to steal valuable information or disrupt computer resources. Network Intrusion Detection System (NIDS) is used to effectively detect various attacks, thus providing timely protection to network resources from these attacks. To implement NIDS, a stream of supervised and unsupervised machine learning approaches is applied to detect irregularities in network traffic and to address network security issues. Such NIDSs are trained using various datasets that include attack traces. However, due to the advancement in modern-day attacks, these systems are unable to detect the emerging threats. Therefore, NIDS needs to be trained and developed with a modern comprehensive dataset which contains contemporary common and attack activities. This paper presents a framework in which different machine learning classification schemes are employed to detect various types of network attack categories. Five machine learning algorithms: Random Forest, Decision Tree, Logistic Regression, K-Nearest Neighbors and Artificial Neural Networks, are used for attack detection. This study uses a dataset published by the University of New South Wales (UNSW-NB15), a relatively new dataset that contains a large amount of network traffic data with nine categories of network attacks. The results show that the classification models achieved the highest accuracy of 89.29% by applying the Random Forest algorithm. Further improvement in the accuracy of classification models is observed when Synthetic Minority Oversampling Technique (SMOTE) is applied to address the class imbalance problem. After applying the SMOTE, the Random Forest classifier showed an accuracy of 95.1% with 24 selected features from the Principal Component Analysis method.
Cyber Attacks Visualization and Prediction in Complex Multi-Stage Network
In network security, various protocols exist, but these cannot be said to be secure. Moreover, is not easy to train the end-users, and this process is time-consuming as well. It can be said this way, that it takes much time for an individual to become a good cybersecurity professional. Many hackers and illegal agents try to take advantage of the vulnerabilities through various incremental penetrations that can compromise the critical systems. The conventional tools available for this purpose are not enough to handle things as desired. Risks are always present, and with dynamically evolving networks, they are very likely to lead to serious incidents. This research work has proposed a model to visualize and predict cyber-attacks in complex, multilayered networks. The calculation will correspond to the cyber software vulnerabilities in the networks within the specific domain. All the available network security conditions and the possible places where an attacker can exploit the system are summarized.
Network Security Policy Automation
Network security policy automation enables enterprise security teams to keep pace with increasingly dynamic changes in on-premises and public/hybrid cloud environments. This chapter discusses the most common use cases for policy automation in the enterprise, and new automation methodologies to address them by taking the reader step-by-step through sample use cases. It also looks into how emerging automation solutions are using big data, artificial intelligence, and machine learning technologies to further accelerate network security policy automation and improve application and network security in the process.
Rule-Based Anomaly Detection Model with Stateful Correlation Enhancing Mobile Network Security
Research on network security technology of industrial control system.
The relationship between industrial control system and Internet is becoming closer and closer, and its network security has attracted much attention. Penetration testing is an active network intrusion detection technology, which plays an indispensable role in protecting the security of the system. This paper mainly introduces the principle of penetration testing, summarizes the current cutting-edge penetration testing technology, and looks forward to its development.
Detection and Prevention of Malicious Activities in Vulnerable Network Security Using Deep Learning
Export citation format, share document.
75 Cyber Security Research Topics in 2024
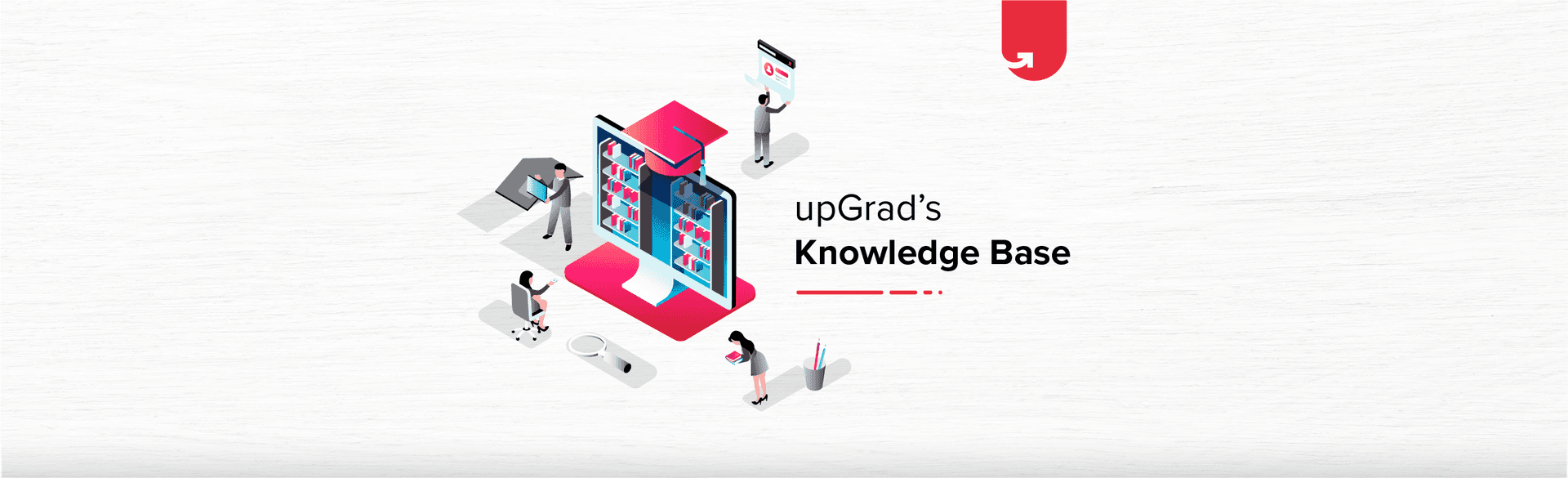
Introduction to Cybersecurity Research
Cybersecurity research aims to protect computer systems, networks, and data from unauthorised access, theft, or damage. It involves studying and developing methods and techniques to identify, understand, and mitigate cyber threats and vulnerabilities.
The field can be divided into theoretical and applied research and faces challenges such as
- Increasing complexity
- New forms of malware
- The growing sophistication of cyber attacks
On a daily basis, approximately 2,200 cyber attacks occur, with an average of one cyber attack happening every 39 seconds. This is the reason why researchers must stay up-to-date and collaborate with others in the field.
In this article, let’s discuss the different cybersecurity research topics and how they will help you become an expert in the field.
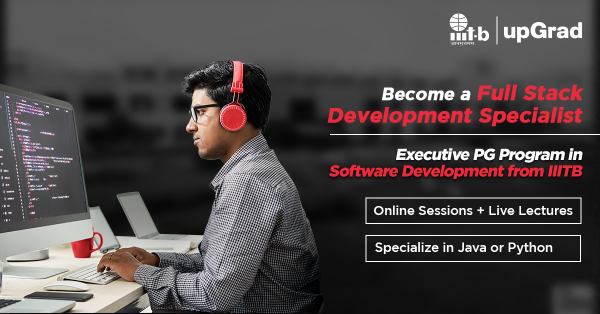
Check out our free technology courses to get an edge over the competition.
Here are some of the latest research topics in cyber security –
Emerging Cyber Threats and Vulnerabilities in 2024
Continual technological advancements lead to changes in cybersecurity trends, with data breaches, ransomware, and hacks becoming more prevalent.
- Cyber Attacks and Their Countermeasures – Discuss – This research paper will discuss various cyber attacks and their corresponding countermeasures. It aims to provide insights on how organisations can better protect themselves from cyber threats.
- Is Cryptography Necessary for Cybersecurity Applications? – Explore the role of cryptography in ensuring the confidentiality, integrity, and availability of data and information in cybersecurity. It would examine the various cryptographic techniques used in cybersecurity and their effectiveness in protecting against cyber threats.
Here are some other cyber security topics that you may consider –
- Discuss the Application of Cyber Security for Cloud-based Applications
- Data Analytics Tools in Cybersecurity
- Malware Analysis
- What Are the Behavioural Aspects of Cyber Security?
- Role of Cyber Security on Intelligent Transporation Systems
- How to Stop and Spot Different Types of Malware?
Check Out upGrad’s Software Development Courses to upskill yourself.
Machine Learning and AI in Cybersecurity Research
Machine learning and AI are research topics in cybersecurity, aiming to develop algorithms for threat detection, enhance intelligence and automate risk mitigation. However, security risks like adversarial attacks require attention.
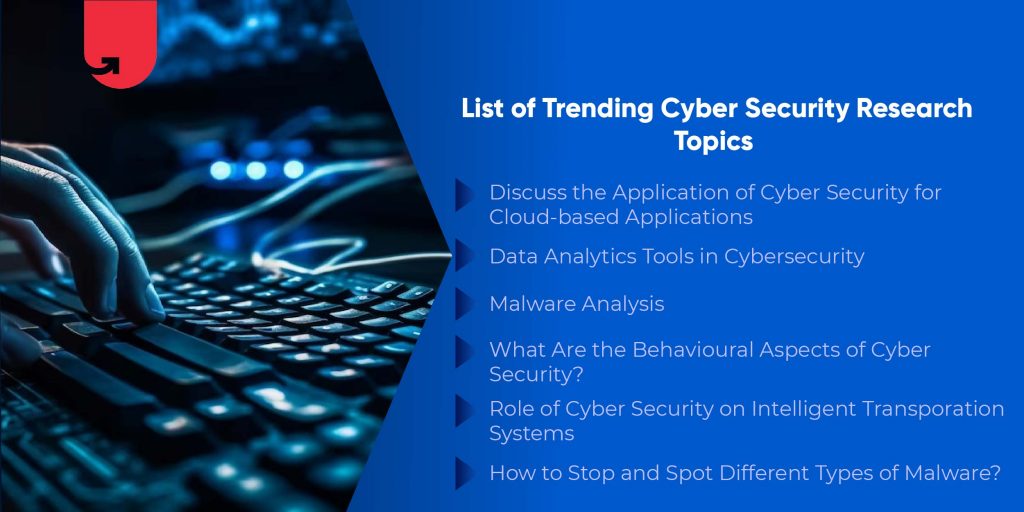
- Using AI/ML to Analyse Cyber Threats – This cyber security research paper analyses cyber threats and could include an overview of the current state of cyber threats and how AI/ML can help with threat detection and response. The paper could also discuss the challenges and limitations of using AI/ML in cybersecurity and potential areas for further research.
Here are some other topics to consider –
- Developing Cognitive Systems for Cyber Threat Detection and Response
- Developing Distributed Ai Systems to Enhance Cybersecurity
- Developing Deep Learning Architectures for Cyber Defence
- Exploring the Use of Computational Intelligence and Neuroscience in Enhancing Security and Privacy
- How is Cyber Security Relevant for Everyone? Discuss
- Discuss the Importance of Network Traffic Analysis
- How to Build an App to Break Ceasar Cipher
You can check out the Advanced Certificate Programme in Cyber Security course by upGrad, which will help students become experts in cyber security.
IoT Security and Privacy
IoT security and privacy research aim to develop secure and privacy-preserving architectures, protocols, and algorithms for IoT devices, including encryption, access control, and secure communication. The challenge is to balance security with usability while addressing the risk of cyber-attacks and compromised privacy.
- Service Orchestration and Routing for IoT – It may focus on developing efficient and secure methods for managing and routing traffic between IoT devices and services. The paper may explore different approaches for optimising service orchestration.
- Efficient Resource Management, Energy Harvesting, and Power Consumption in IoT – This paper may focus on developing strategies to improve energy use efficiency in IoT devices. This may involve investigating the use of energy harvesting technologies, optimising resource allocation and management, and exploring methods to reduce power consumption.
Here are some other cyber security project topics to consider –
- Computation and Communication Gateways for IoT
- The Miniaturisation of Sensors, Cpus, and Networks in IoT
- Big Data Analytics in IoT
- Semantic Technologies in IoT
- Virtualisation in IoT
- Privacy, Security, Trust, Identity, and Anonymity in IoT
- Heterogeneity, Dynamics, and Scale in IoT
- Consequences of Leaving Unlocked Devices Unattended
Explore our Popular Software Engineering Courses
Blockchain security: research challenges and opportunities.
Blockchain security research aims to develop secure and decentralised architectures, consensus algorithms, and privacy-preserving techniques while addressing challenges such as smart contract security and consensus manipulation. Opportunities include transparent supply chain management and decentralised identity management.
- Advanced Cryptographic Technologies in the Blockchain – Explore the latest advancements and emerging trends in cryptographic techniques used in blockchain-based systems. It could also analyse the security and privacy implications of these technologies and discuss their potential impact.
- Applications of Smart Contracts in Blockchain – Explore the various use cases and potential benefits of using smart contracts to automate and secure business processes. It could also examine the challenges and limitations of smart contracts and propose potential solutions for these issues.
Here are some other topics –
- Ensuring Data Consistency, Transparency, and Privacy in the Blockchain
- Emerging Blockchain Models for Digital Currencies
- Blockchain for Advanced Information Governance Models
- The Role of Blockchain in Future Wireless Mobile Networks
- Law and Regulation Issues in the Blockchain
- Transaction Processing and Modification in the Blockchain
- Collaboration of Big Data With Blockchain Networks
Cloud Security: Trends and Innovations in Research
Cloud security research aims to develop innovative techniques and technologies for securing cloud computing environments, including threat detection with AI, SECaaS, encryption and access control, secure backup and disaster recovery, container security, and blockchain-based solutions. The goal is to ensure the security, privacy, and integrity of cloud-based data and applications for organisations.
- Posture Management in Cloud Security – Discuss the importance of identifying and addressing vulnerabilities in cloud-based systems and strategies for maintaining a secure posture over time. This could include topics such as threat modelling, risk assessment, access control, and continuous monitoring.
- Are Cloud Services 100% Secure?
- What is the Importance of Cloud Security?
- Cloud Security Service to Identify Unauthorised User Behaviour
- Preventing Theft-of-service Attacks and Ensuring Cloud Security on Virtual Machines
- Security Requirements for Cloud Computing
- Privacy and Security of Cloud Computing
Explore Our Software Development Free Courses
Cybercrime investigations and forensics.
Cybercrime investigations and forensics involve analysing digital evidence to identify and prosecute cybercriminals, including developing new data recovery, analysis, and preservation techniques. Research also focuses on identifying cybercriminals and improving legal and regulatory frameworks for prosecuting cybercrime.
- Black Hat and White Hat Hacking: Comparison and Contrast – Explore the similarities and differences between these two approaches to hacking. It would examine the motivations and methods of both types of hackers and their impact on cybersecurity.
- Legal Requirements for Computer Forensics Laboratories
- Wireless Hacking Techniques: Emerging Technologies and Mitigation Strategies
- Cyber Crime: Current Issues and Threats
- Computer Forensics in Law Enforcement: Importance and Challenges
- Basic Procedures for Computer Forensics and Investigations
- Digital Forensic Examination of Counterfeit Documents: Techniques and Tools
- Cybersecurity and Cybercrime: Understanding the Nature and Scope
An integral part of cybercrime investigation is to learn software development. Become experts in this field with the help of upGrad’s Executive Post Graduate Programme in Software Development – Specialisation in Full Stack Development .
Cybersecurity Policy and Regulations
Cybersecurity policy and regulations research aims to develop laws, regulations, and guidelines to ensure the security and privacy of digital systems and data, including addressing gaps in existing policies, promoting international cooperation, and developing standards and best practices for cybersecurity. The goal is to protect digital systems and data while promoting innovation and growth in the digital economy.
- The Ethicality of Government Access to Citizens’ Data – Explore the ethical considerations surrounding government access to citizens’ data for surveillance and security purposes, analysing the potential risks and benefits and the legal and social implications of such access.
- The Moral Permissibility of Using Music Streaming Services – Explore the ethical implications of using music streaming services, examining issues such as intellectual property rights, artist compensation, and the environmental impact of streaming.
- Real Name Requirements on Internet Forums
- Restrictions to Prevent Domain Speculation
- Regulating Adult Content Visibility on the Internet
- Justification for Illegal Downloading
- Adapting Law Enforcement to Online Technologies
- Balancing Data Privacy With Convenience and Centralisation
- Understanding the Nature and Dangers of Cyber Terrorism
Human Factors in Cybersecurity
Human factors in cybersecurity research study how human behaviour impacts cybersecurity, including designing interfaces, developing security training, addressing user error and negligence, and examining cybersecurity’s social and cultural aspects. The goal is to improve security by mitigating human-related security risks.
- Review the Human Factors in Cybersecurity – It explores various human factors such as awareness, behaviour, training, and culture and their influence on cybersecurity, offering insights and recommendations for improving cybersecurity outcomes.
- Integrating Human Factors in Cybersecurity for Better Risk Management
- Address the Human Factors in Cybersecurity Leadership
- Human Factors in IoT Security
- Internal Vulnerabilities: the Human Factor in It Security
- Cyber Security Human Factors – the Ultimate List of Statistics and Data
In-Demand Software Development Skills
Cybersecurity education and awareness.
Cybersecurity education and awareness aims to educate individuals and organisations about potential cybersecurity threats and best practices to prevent cyber attacks. It involves promoting safe online behaviour, training on cybersecurity protocols, and raising awareness about emerging cyber threats.
- Identifying Phishing Attacks – This research paper explores various techniques and tools to identify and prevent phishing attacks, which are common types of cyber attacks that rely on social engineering tactics to trick victims into divulging sensitive information or installing malware on their devices.
- Risks of Password Reuse for Personal and Professional Accounts – Investigate the risks associated with reusing the same password across different personal and professional accounts, such as the possibility of credential stuffing attacks and the impact of compromised accounts on organisational security.
- Effective Defence Against Ransomware
- Information Access Management: Privilege and Need-to-know Access
- Protecting Sensitive Data on Removable Media
- Recognising Social Engineering Attacks
- Preventing Unauthorised Access to Secure Areas: Detecting Piggybacking and Tailgating
- E-mail Attack and Its Characteristics
- Safe Wifi Practice: Understanding VPN
With the increasing use of digital systems and networks, avoiding potential cyber-attacks is more important than ever. The 75 research topics outlined in this list offer a glimpse into the different dimensions of this important field. By focusing on these areas, researchers can make significant contributions to enhancing the security and safety of individuals, organisations, and society as a whole.
upGrad’s Master of Science in Computer Science program is one of the top courses students can complete to become experts in the field of tech and cyber security. The program covers topics such as Java Programming and other forms of software engineering which will help students understand the latest technologies and techniques used in cyber security.
The program also includes hands-on projects and case studies to ensure students have practical experience in applying these concepts. Graduates will be well-equipped to take on challenging roles in the rapidly growing field of cyber security.

Pavan Vadapalli
Something went wrong
Our Trending Software Engineering Courses
- Master of Science in Computer Science from LJMU
- Executive PG Program in Software Development Specialisation in Full Stack Development from IIIT-B
- Advanced Certificate Programme in Cyber Security from IIITB
- Full Stack Software Development Bootcamp
- Software Engineering Bootcamp from upGrad
Popular Software Development Skills
- React Courses
- Javascript Courses
- Core Java Courses
- Data Structures Courses
- ReactJS Courses
- NodeJS Courses
- Blockchain Courses
- SQL Courses
- Full Stack Development Courses
- Big Data Courses
- Devops Courses
- NFT Courses
- Cyber Security Courses
- Cloud Computing Courses
- Database Design Courses
- Crypto Courses
- Python Courses
Our Popular Software Engineering Courses
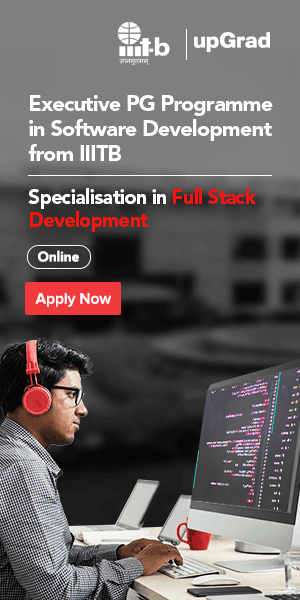
Frequently Asked Questions (FAQs)
Artificial intelligence (AI) has proved to be an effective tool in cyber defence. AI is anticipated to gain even more prominence in 2024, mainly in monitoring, resource and threat analysis, and quick response capabilities.
One area of focus is the development of secure quantum and space communications to address the increasing use of quantum technologies and space travel. Another area of research is improving data privacy.
The approach to cybersecurity is expected to change from defending against attacks to acknowledging and managing ongoing cyber risks. The focus will be on improving resilience and recovering from potential cyber incidents.
Related Programs View All
Certification
40 Hrs Live, Expert-Led Sessions
2 High-Quality Practice Exams
View Program
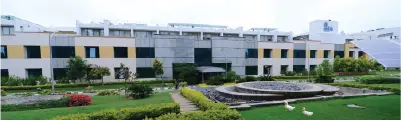
Executive PG Program
IIIT-B Alumni Status
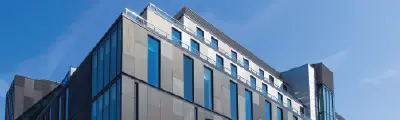
Master's Degree
40000+ Enrolled Learners
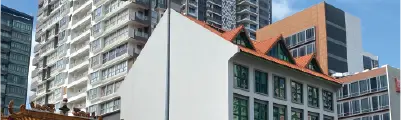
Job Assistance
32-Hr Training by Dustin Brimberry
Question Bank with 300+ Practice Qs
45 Hrs Live Expert-Led Training
Microsoft-Approved Curriculum
159+ Hours of Live Sessions
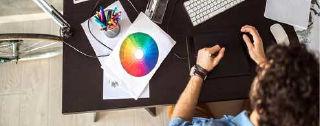
126+ Hours of Live Sessions
Fully Online
13+ Hrs Instructor-Led Sessions
Live Doubt-Solving Sessions
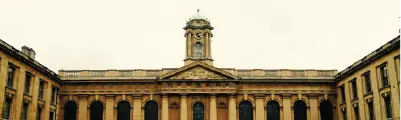
2 Unique Specialisations
300+ Hiring Partners
20+ Hrs Instructor-Led Sessions
16 Hrs Live Expert-Led Training
CLF-C02 Exam Prep Support
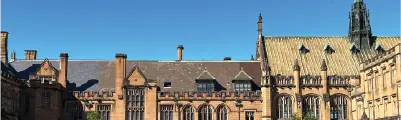
24 Hrs Live Expert-Led Training
4 Real-World Capstone Projects
17+ Hrs Instructor-Led Training
3 Real-World Capstone Projects
289 Hours of Self-Paced Learning
10+ Capstone Projects
490+ Hours Self-Paced Learning
4 Real-World Projects
690+ Hours Self-Paced Learning
Cloud Labs-Enabled Learning
288 Hours Self-Paced Learning
9 Capstone Projects
40 Hrs Live Expert-Led Sessions
2 Mock Exams, 9 Assessments
Executive PG Certification
GenAI integrated curriculum
Job Prep Support
Instructor-Led Sessions
Hands-on UI/UX
16 Hrs Live Expert-Led Sessions
12 Hrs Hand-On Practice
30+ Hrs Live Expert-Led Sessions
24+ Hrs Hands-On with Open Stack
2 Days Live, Expert-Led Sessions
34+ Hrs Instructor-Led Sessions
10 Real-World Live Projects
24 Hrs Live Expert-Led Sessions
16 Hrs Hand-On Practice
8 Hrs Instructor-Led Training
Case-Study Based Discussions
40 Hrs Instructor-Led Sessions
Hands-On Practice, Exam Support
24-Hrs Live Expert-Led Sessions
Regular Doubt-Clearing Sessions
Extensive Exam Prep Support
6 Hrs Live Expert-Led Sessions
440+ Hours Self-Paced Learning
400 Hours of Cloud Labs
15-Hrs Live Expert-Led Sessions
32 Hrs Live Expert-Led Sessions
28 Hrs Hand-On Practice
Mentorship by Industry Experts
24 Hrs Live Trainer-Led Sessions
Mentorship by Certified Trainers
GenAI Integrated Curriculum
Full Access to Digital Resources
16 Hrs Live Instructor-Led Sessions
80+ Hrs Hands-On with Cloud Labs
160+ Hours Live Instructor-Led Sessions
Hackathons and Mock Interviews
31+ Hrs Instructor-Led Sessions
120+ Hrs of Cloud Labs Access
35+ Hrs Instructor-Led Sessions
6 Real-World Live Projects
24+ Hrs Instructor-Led Training
Self-Paced Course by Nikolai Schuler
Access Digital Resources Library
300+ Hrs Live Expert-Led Training
90 Hrs Doubt Clearing Sessions
56 Hours Instructor-Led Sessions
78 Hrs Live Expert-Led Sessions
22 Hrs Live, Expert-Led Sessions
CISA Job Practice Exams
Explore Free Courses
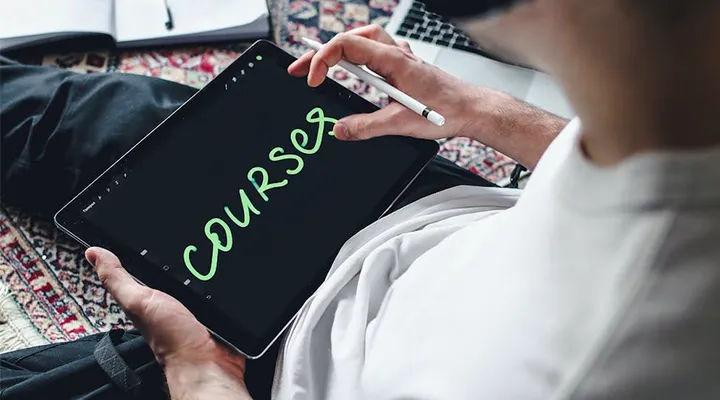
Learn more about the education system, top universities, entrance tests, course information, and employment opportunities in Canada through this course.
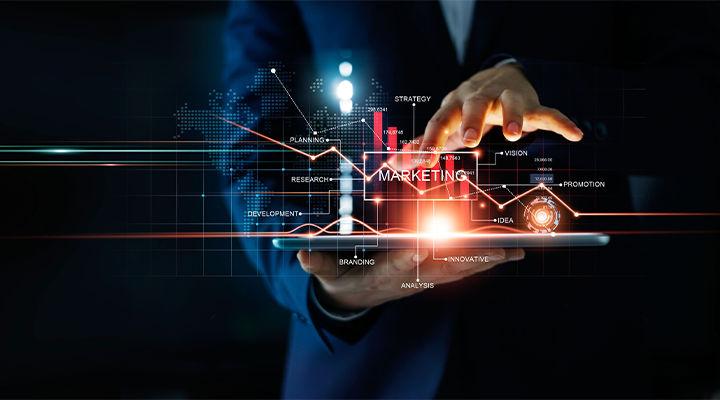
Advance your career in the field of marketing with Industry relevant free courses
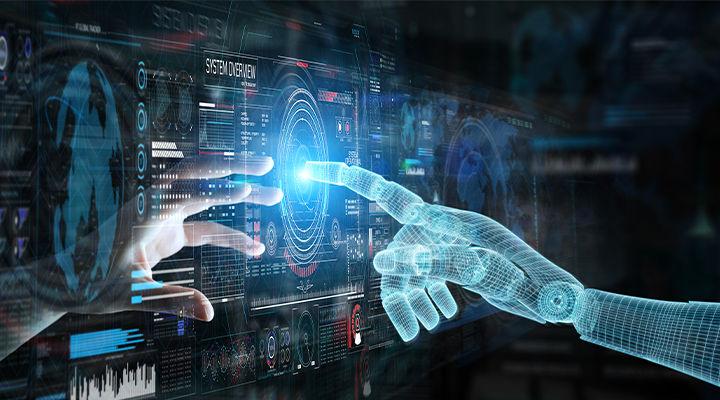
Build your foundation in one of the hottest industry of the 21st century
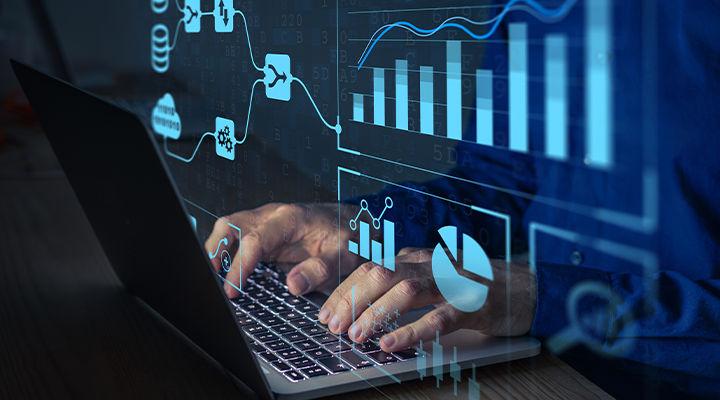
Master industry-relevant skills that are required to become a leader and drive organizational success
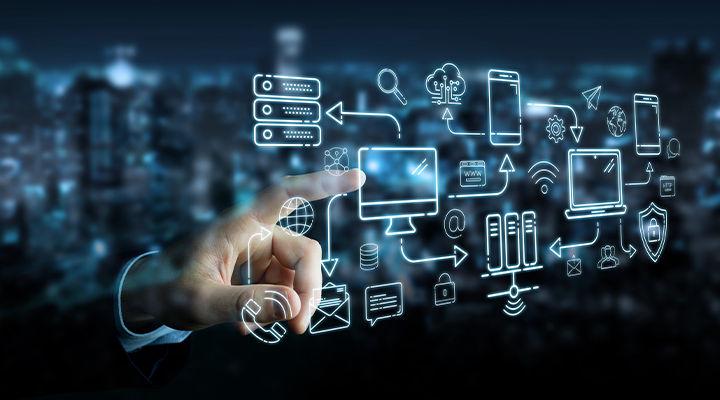
Build essential technical skills to move forward in your career in these evolving times
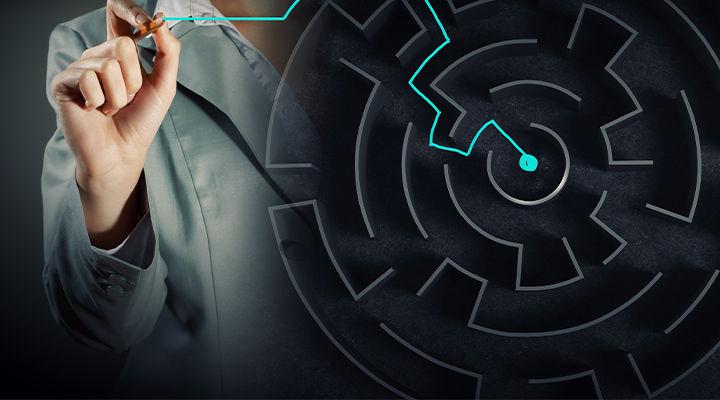
Get insights from industry leaders and career counselors and learn how to stay ahead in your career
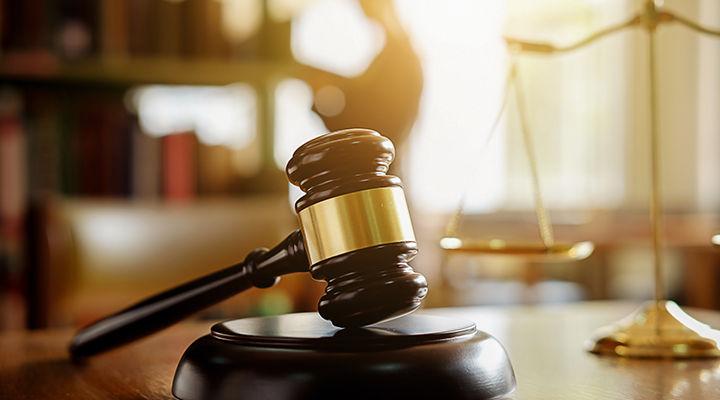
Kickstart your career in law by building a solid foundation with these relevant free courses.

Stay ahead of the curve and upskill yourself on Generative AI and ChatGPT
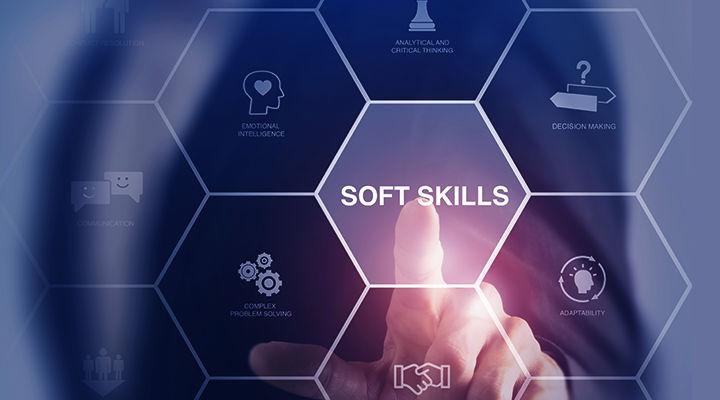
Build your confidence by learning essential soft skills to help you become an Industry ready professional.
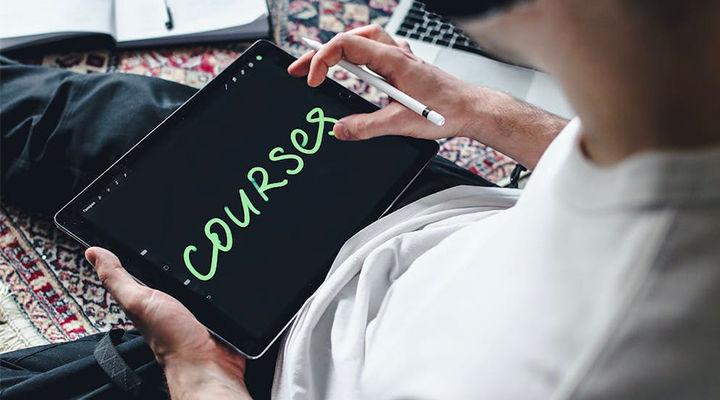
Learn more about the education system, top universities, entrance tests, course information, and employment opportunities in USA through this course.
Suggested Tutorials
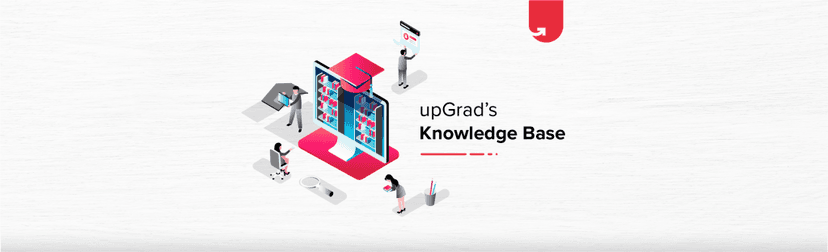
Explore Python programming with this concise tutorial, covering basics to advanced concepts for beginners and enthusiasts alike.
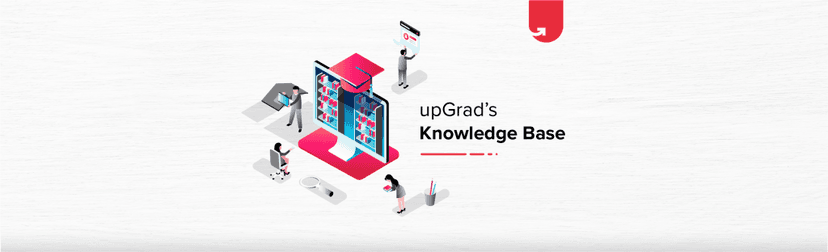
Introduction to C Programming, Learn all the C programming language concepts in this tutorial.
Suggested Blogs
![research paper topics on computer security Scrum Master Salary in India: For Freshers & Experienced [2023]](https://www.upgrad.com/__khugblog-next/image/?url=https%3A%2F%2Fd14b9ctw0m6fid.cloudfront.net%2Fugblog%2Fwp-content%2Fuploads%2F2020%2F05%2F486.png&w=3840&q=75)
by Rohan Vats
05 Mar 2024
![research paper topics on computer security SDE Developer Salary in India: For Freshers & Experienced [2024]](https://www.upgrad.com/__khugblog-next/image/?url=https%3A%2F%2Fd14b9ctw0m6fid.cloudfront.net%2Fugblog%2Fwp-content%2Fuploads%2F2020%2F05%2F482-1.png&w=3840&q=75)
by Prateek Singh
29 Feb 2024
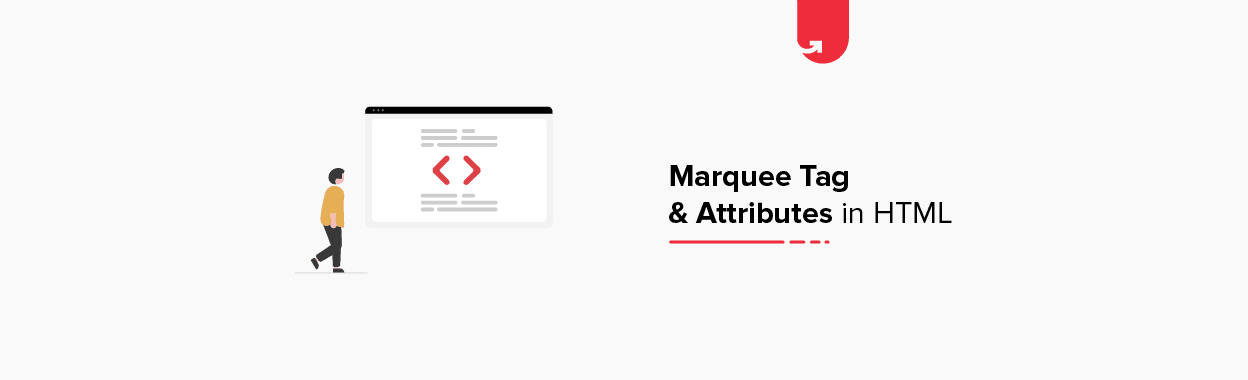
by venkatesh Rajanala
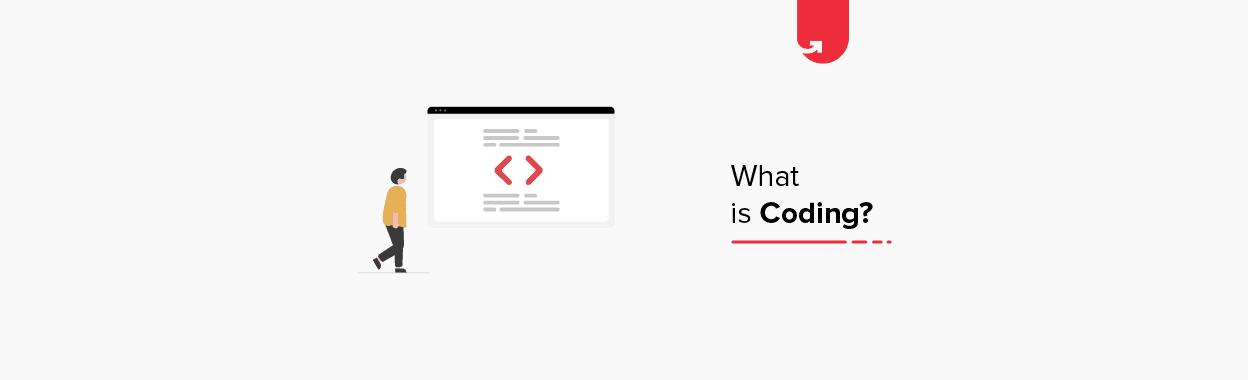
by Harish K
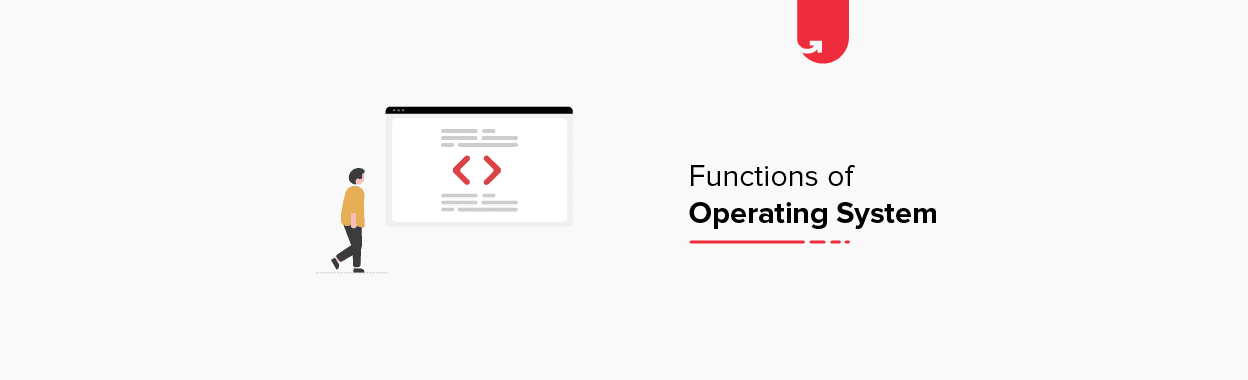
by Geetika Mathur
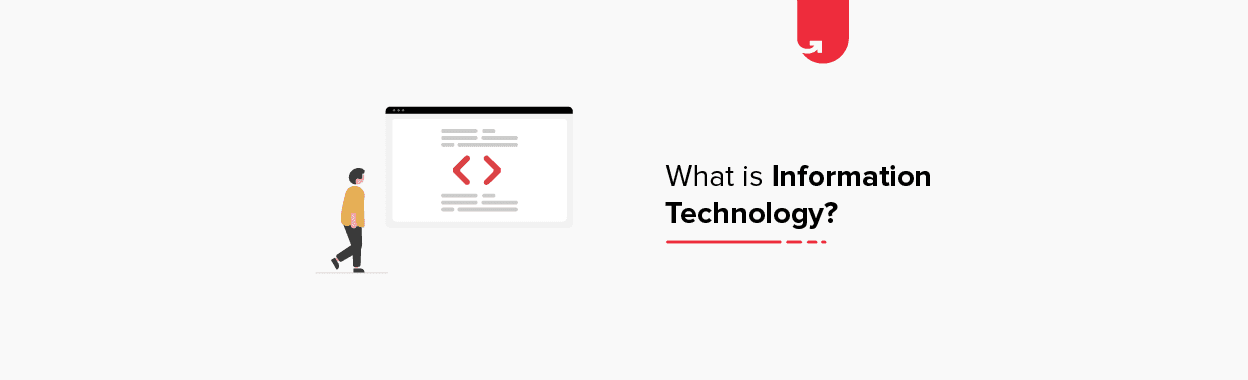
by spandita hati
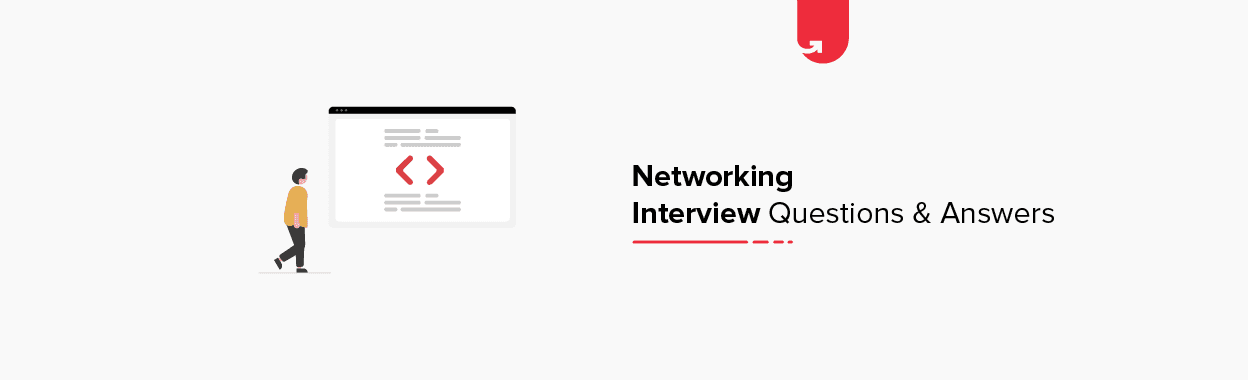
28 Feb 2024
- Write my thesis
- Thesis writers
- Buy thesis papers
- Bachelor thesis
- Master's thesis
- Thesis editing services
- Thesis proofreading services
- Buy a thesis online
- Write my dissertation
- Dissertation proposal help
- Pay for dissertation
- Custom dissertation
- Dissertation help online
- Buy dissertation online
- Cheap dissertation
- Dissertation editing services
- Write my research paper
- Buy research paper online
- Pay for research paper
- Research paper help
- Order research paper
- Custom research paper
- Cheap research paper
- Research papers for sale
- Thesis subjects
- How It Works
50 Great Cybersecurity Research Paper Topics
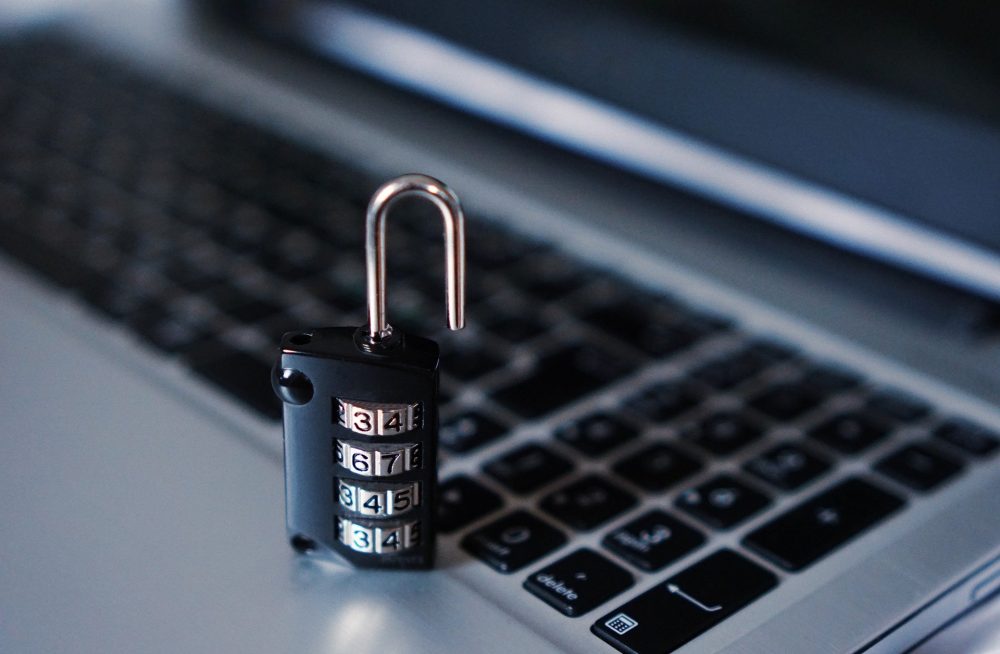
Students are required to write papers and essays on cyber security topics when pursuing programs in cyber security disciplines. These topics are technical and they require learners to inherently understand this subject. What’s more, students should have impeccable research and writing skills.
Additionally, students should choose cyber security topics to write their papers and essays carefully. As a science field, cyber security is developing rapidly and constantly. As such, learners can always find interesting topics to write papers and essays about.
Pick Cyber Security Topics From Our List
Software and computer administration cyber security topics.
- Cyber Security Research Paper Topics on Data Protection
- Cyber Security Awareness Topics
Network Security Topic Ideas
- Current and Interesting Topics in Cyber Security
Nevertheless, selecting cybersecurity topics for research shouldn’t be a rushed process. That’s because the chosen topics will influence the experience of students while writing and the grades they will score. Therefore, learners should focus on choosing topics that they will be comfortable researching and writing about.
If you’re having a hard time choosing the topics to research and write about, here are categories of some of the best cybersecurity paper topics that you can consider. We also advise you to check out capstone project topics .
The cyber security of a company can be compromised in many ways when it comes to software and computer administration. As such, software and computer administration is a great sources of cybersecurity research paper topics. Here are some of the best topics in this category.
- Evaluation of the operation of antimalware in preventing cyber attacks
- How does virus encryption work
- Is countering malware difficult because of the fast evolution of technology?
- Why should companies train their staff on cyber security?
- Why should people worry about identity theft?
- How important are software updates when it comes to cyber security?
- What causes cyber crimes?
- What are the major threats to the cyber security of social media users?
- What are the most effective methods of preventing phishing?
- What is the major threat to cyber security today and why?
These topics address issues that affect anybody or any organization that uses a computer or any device to access the internet and exchange information. As such, most people, including teachers and professors, will be impressed by papers and essays written about them.
CyberSecurity Research Paper Topics on Data Protection
Individuals and companies send and receive a lot of data every day. As such, this category has some of the best cybersecurity topics for presentation. That’s because they address issues that affect many people and organizations. Here are some of the best information security topics to consider when writing papers and essays or preparing a presentation.
- The best security measures for protecting your data
- How third-party applications can be used to access and acquire data without permission
- How to prevent the loss of data from a computer
- Can biometrics be used to prevent unauthorized data access?
- Can you protect yourself from cyber crimes by keeping personal data private?
- What should you do in case of a data breach?
- How can you secure your data with a 2-steps authentication process?
- How can public Wi-Fi or the internet be used to steal personal data?
- What information can be accessed by unauthorized persons if they hack an account?
- Can software updates help in protecting personal data?
Every computer or internet user wants to be sure that their data is safe and protected. Papers and essays that are written on these topics address issues of data protection. As such, many people will find them worth reading.
CyberSecurity Awareness Research Paper Topics
The best cyber security topics for research papers do more than just address a single issue. They also inform the readers. Here are some of the best cyber security topics for research papers that also focus on creating awareness.
- What is reverse engineering?
- How efficient are RFID security systems?
- How does the dark web propagate organized cyber crimes?
- How can steganalysis be applied?
- Analyze the best authorization infrastructures today
- How important is computer forensics in the current digital era?
- What strategies have been proven effective in preventing cyber-attacks?
- Which forensic tools are the best when it comes to detecting cyber threats?
- Can changing the password regularly help in predicting a cyber attack?
- How can you tell that you’re at risk of online identity theft?
Many people are not aware of many things that affect their cyber security. These topics are relevant because they enhance the awareness of the internet and computer users.
Most organizations today have networked systems that enhance their operations. Unfortunately, criminals have learned to target those networked systems with their criminal activities. As such, students can address some of these issues with their cyber security thesis topics. Here are interesting topics that learners can research and write about in this category.
- Evaluation of the cyber security legal framework in the U.S
- Analysis of the most difficult aspect of the administration of cyber security
- How can the possibilities of multiple threats be managed effectively?
- How does data backup help when it comes to cyber security?
- How effective is two-factor authentication?
- How should a company respond to hacking in its system?
- Which are the best cyber security protection approaches for a multinational company?
- What are the pros and cons of unified user profiles?
- What are the most important components of effective data governance?
- What motivates individuals to commit cybercrimes?
These computer security topics can be used to write papers and essays for college or even commissioned by organizations and used for presentation purposes.
Current and Interesting Topics in CyberSecurity
Some computer security research topics seek to address issues that affect society at the moment. Here are examples of such topics.
- How phishing is evolving and getting more sophisticated
- Explain the evolution of Ransomware strategies
- Explain how the cryptocurrency movement affects cybersecurity
- Cyber-Physical Attacks: How do they work?
- What are state-sponsored attacks and how do they affect cyber security at a global level?
- Discuss cyber security risks when it comes to third-party vendor relationships
- How digital advertisements are being used to collect user characteristics
- How can a person sync all their devices while ensuring their protection
- Why it’s advisable to avoid downloading files from sites that are not trusted
- Why consumers should read the terms and conditions of software before they decide to install it
Such technology security topics are trendy because they address issues that affect most people in modern society. Nevertheless, students should conduct extensive research to draft solid papers and essays on these topics.
This cyber security topic list is not exhaustive. You can contact our thesis writers if you need more ideas or help. Students have many topics to consider depending on their academic programs, interests, and instructions provided by educators or professors. Nevertheless, students should focus on choosing topics that will enable them to come up with informative and comprehensive papers. Thus, every student should choose an information security topic for which they can find relevant and supporting data.
Leave a Reply Cancel reply
Custom Essay, Term Paper & Research paper writing services
- testimonials
Toll Free: +1 (888) 354-4744
Email: [email protected]
Writing custom essays & research papers since 2008
154 exceptional cybersecurity research topics for you.
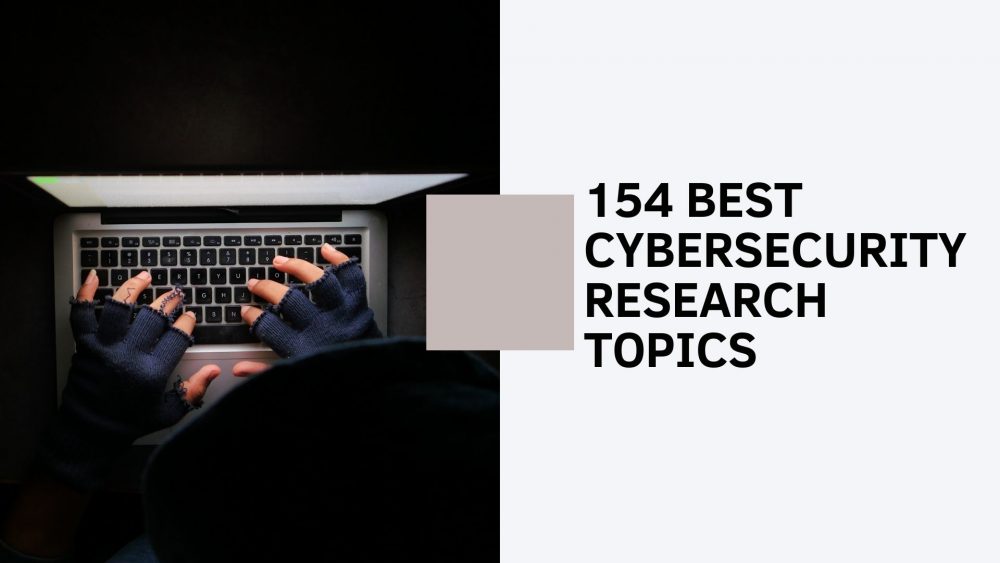
If you are studying computer science or IT-related course, you will encounter such a task. It is one of the most technical assignments, primarily in the era of advanced digital technologies. Students may not have the muscles to complete such papers on their own. That is why we provide expert help and ideas to make the process easier.
Do you want to excel in your cybersecurity paper? Here is your number one arsenal!
What You Need To Know About Cyber Security Research Topics
A cybersecurity paper deals with the practices of protecting servers, electronic systems, computers, and networks from malicious attacks. Although most students think this only applies to computers, it also applies to mobile computing and other business models.
There are various categories in cybersecurity, including:
Network security Application security Information security Operational security Disaster recovery and business continuity
Therefore, your cybersecurity topics for research should:
Examine the common security breaches in systems and networks Offer practical ways of protecting computers from such attacks Highlight the legal and ethical implications of hacking and other related practices Point out the challenges encountered in combating cybercrime
Since this is a technical paper, you should endeavor to do your research extensively to prevent rumors and unverified facts. The topics should also inform and educate people who are not conversant with cybersecurity in simple terms. Avoid using jargon at all costs, as this will make the paper difficult to read and understand.
Are you worried about where you can get professional cybersecurity topics and ideas? Well, here are a few of the most reliable sources that can furnish you with top-rated issues.
- Government legislation on cybersecurity (Acts of Parliament)
- The UN Office of Counter-Terrorism (Cybersecurity initiatives)
- The CISCO magazine
- Forbes also has excellent coverage on cybersecurity
You can find impressive topic ideas from these sources and more. Furthermore, news headlines and stories on cybersecurity can also help you gather many writing ideas. If all these prove futile, use our tip-top writing prompts below:
Quality Cyber Security Thesis Topics
- Impacts of coronavirus lockdowns on cybersecurity threats in the US
- Why ethical hacking is contributing to more harm than good
- The role of computer specialists in combating cyber threats before they occur
- Technological trends that are making it difficult to manage systems
- Are passwords reliable when protecting computer systems?
- Effects of having more than one systems administrator in a company
- Can the government shut down the dark web once and for all?
- Why should you bother about the security of your mobile device?
- Evaluate reasons why using public WIFI can be harmful to your security
- The role of cybersecurity seminars and conferences
- How universities can produce ethical computer hackers who can help the society
- How to counter-terrorism with advanced cybersecurity measures
- Impacts of teaching children how to use computers at a tender age
- Latest innovations that are a threat to cybersecurity
- The role of monitoring in combating frequent cyber attacks
- How social media is contributing to cyber attacks
- Discuss the relationship between cyberbullying and cybersecurity
- Why fingerprints may be the best method of protecting devices
- The role of YouTube in contributing to the rising number of hackers
Top Research Topics For Cyber Security For Master Thesis
- Impact of cyber threats on attaining the sustainable development goals
- Why websites are becoming easy to hack in the 21 st century
- Effects of not having an SSL certificate for a website
- Discuss the security threats associated with WordPress websites
- Impacts of frequent maintenance while the website is still running
- How computer colleges can contribute to a safe cyberspace
- Latest cyber threats to business and financial websites
- Discuss the implications of cyber threats on privacy
- The role of Facebook in advancing cyberbullying and hacking
- Is hacking becoming a global epidemic in the digital world?
- Why using Cyber Cafes may be detrimental to your digital security
- The role of systems analysts in responding to data breaches
- How cybersecurity movies are contributing to cyber threats
- Should hackers face lifetime jail imprisonment when found guilty?
- Loopholes in cyber laws that make the practice challenging to curtail
Good Thesis Topics For Cyber Security
- The relationship between privacy and data security in computing
- Why cloud computing offers a haven for computer hackers
- The role of character and human-based behavior in cybersecurity
- How to determine safe organizational security management and policy
- How the Internet of Things is promoting cyber attacks
- Effects of using cracked computer software
- Are biometrics in cybersecurity able to put off hackers?
- The role of studying mobile platform security
- Why companies should frequently monitor their firewalls
- The role of antimalware in curbing cyber attacks
- Why is Ransomware a headache to most companies handling big data?
- How does antivirus software improve the security of your computer?
- Compare and contrast between the security of UNIX and Ubuntu
- The role of data encryption technologies in ensuring system security
- Is the process of encrypting viruses safe?
Top-Grade Thesis Topics For Cyber Security
- Describe the effectiveness of cybersecurity audits on company systems
- Is it proper to conduct device synchronization?
- Why is it difficult to manage the security of an intranet?
- Discuss the effects of logging in to many devices at the same time
- Evaluate the significance of computer forensics
- How are hackers inventing new ways of breaching the systems of companies?
- Why it is necessary to review the data protection laws
- Practices that increase the vulnerability of a system to cyber attacks
- Can organizations implement impenetrable network systems?
- Why administrators should check the background of users before giving them rights and privileges
- The role of risk management cybersecurity
- Discuss the impact of reverse engineering on computing systems
- Effects of a cyber-attack on a company’s economic performance
- What legal frameworks work best for a computer company?
- The role of social engineering in cybersecurity
Information Security Research Topics
- The implication of the proliferation of the internet globally
- Innovative technologies used in keeping off hackers
- The role of information communication technologies in maintaining the security
- Are online courses on informative security practical?
- Why should people avoid sharing their details on Facebook?
- Effects of using your image on social media
- The role of pseudo names and nicknames on social media
- Discuss the implications of Wi-Fi hacking apps on mobile phones
- How to detect malicious activity on a system
- Evaluate the potential threats of conduct self-hacking on a system
- The impact of sharing personal details with hiring agencies
- How con artists lure unsuspecting applicants into giving out their details
- Effects of frequent maintenance on systems
- How to strengthen the firewall of an information system
- The role of the media in propagating security breaches to information systems
Latest Computer Security Research Topics
- Tricks that black hat hackers use to infiltrate company systems
- How children learn about cybersecurity from their parents
- The impact of watching hacking movies and TV series
- How various companies are protecting themselves from cyber attacks
- Why every company should have a systems security consultant
- Discuss the implication of digital piracy
- Threats that biometrics are bringing to digital systems
- How to block a network intrusion before it causes any effect
- Why MacOS is challenging to infiltrate, unlike Windows
- Results of two-step authentication security measures for login systems
- The role of updating computer systems during working days
- Evaluate times of the year when hackers infiltrate systems the most
- Why it isn’t easy to manage big data on the cloud
- What happens during a system breakdown and maintenance?
- Discuss the role of data synchronization in creating a backup
Network Security Research Paper Topics
- The impact of having self-configuring and decentralized network systems
- Effects of ad-hoc networks for large companies
- Discuss the role of wireless sensor networks in contributing to security breaches
- How malicious nodes join a network
- Why it is difficult to detect a passive network attack
- How active network attacks reduce a network’s performance
- Evaluate the various parameters used in network security
- Analyze how a black hole affects a network system
- Describe techniques used in detecting malicious nodes on networks
- How to improve the safety of a company network
- The role of data encryption in maintaining the security of a network
- Describe the various channels of establishing secure algorithms in a network
- How does RSA increase the safety of a particular network?
- Effective policies and procedures for maintaining network security
- The role of a unique ID and Password in securing a website
Computer Security Research Topics
- Why it is challenging to maintain endpoint security
- The role of a critical infrastructure cybersecurity
- How to create secure passwords for your computer network
- The part of scanning for malware often on your PC
- How to detect apps that invade your privacy unknowingly
- Why ordering software from the black market is a threat to security
- Safe computing techniques for first-time computer users
- The role of digital literacy in preventing hacking
- Why most online users fall to online scams
- The role of smartphones in enhancing cybersecurity threats
- Evaluate the mobile landscape concerning data security
- The implication of private email accounts in data breaches
- Sites that contain a barrel of internet criminals
- How to develop comprehensive internet security software
- How children can navigate the internet safely
Impressive Cyber Crime Research Topics
- Why cyber currencies are a threat to online security
- Why cyberbullying is rampant in the 21 st century unlike in any other time
- The impact of online persuasion campaigns on cybersecurity
- Why teenagers are victims of cyberbullying than adults
- Discuss the effects of technology evolution on cybercrime
- How online hackers collect information without the knowledge of the victim
- Traits of a robust cybersecurity system
- Practices that can help reduce cybercrime in institutions of higher learning.
- Effects of global coordinated cyber attacks
- The penalties of cyber-attack in the First Amendment
- Why the world is experiencing increased cyber attacks
- Critical concepts of cyber attacks
- Cybercriminals and enterprises
- Role of NGOs in combating cyber terrorism
- Cyberbullying in campus
World-Class Cyber Security Thesis Ideas
- Effects of the cyber-attack on Sony in 2014
- The role of globalization in enhancing cybersecurity
- How to prevent automotive software from malicious cyber attacks
- The role of cyber technology in changing the world since the 1990s
- How the private sector is essential in combating cyber threats
- Computer infrastructure protection against cyber attacks
- Impact of social networking sites on cybersecurity
- Threats that cyber-attacks pose the national security of a country
- How cyber monitoring affects ethical and legal considerations
- Factors leading to the global nature of cyber attacks
- Analyze law enforcement agencies that deal with cyber attacks
- Evaluate cyber-crime court cases
- Evolution of the cybersecurity industry
- Cyber terrorism in the US
- Implementing adequate data protection strategies
We offer paper writing help on any cybersecurity topic. Try us now!
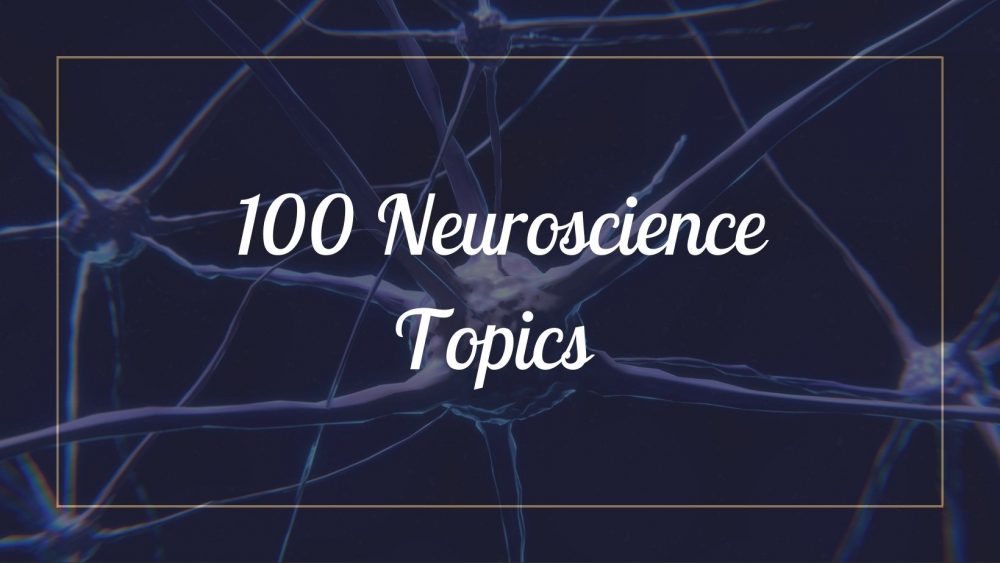
Topics in Computer and Network Security
Stanford cs 356, fall 2023.
CS 356 is graduate course that covers foundational work and current topics in computer and network security. The course consists of reading and discussing published research papers, presenting recent security work, and completing an original research project.
Course Information
Discussion: Mon/Wed 3:00–4:20 PM. Gates B12 . This course is largely based on in-person discussion rather than lecture. Attendance and participation is expected.
Instructor: Zakir Durumeric Office Hours: M/W 4:30–5:00 PM, or by appointment.
Course Assistant: Kimberly Ruth . Office hours by appointment.
Prerequisites: CS 356 is open to all graduate students as well as advanced undergraduate students. While the course has no official prerequisites, it requires a mature understanding of software systems and networks. Students are expected to have taken CS 155: Computer and Network Security or equivalent.
Topics and Readings
The tentative schedule and required readings for the class are below:
9/27 Introduction
Against security nihilism.
Blog Post. 2016. Chris Palmer.
Mining Your Ps and Qs: Detection of Widespread Weak Keys...
SEC '12 . N. Heninger, Z. Durumeric, E. Wustrow, J.A. Halderman.
How to Read a Paper
10/2 web privacy and security, the web never forgets: persistent tracking mechanisms in the....
CCS '14 . Gunes Acar, Christian Eubank, Steven Englehardt, Marc Juarez, Arvind Narayanan, Claudia Diaz.
Imperfect Forward Secrecy: How Diffie-Hellman Fails in Practice
CCS '15 . D. Adrian, K. Bhargavan, Z. Durumeric, P. Gaudry, M. Green, J.A. Halderman, N. Heninger, A. Springall, E. Thomé, L. Valenta, B. VanderSloot, E. Wustrow, S. Zanella-Beguelin, P. Zimmermann.
10/4 Usability
Alice in warningland: a large-scale field study of browser security.
SEC '13 . Devdatta Akhawe, Adrienne Porter Felt.
...no one can hack my mind”: Comparing Expert and Non-Expert Security Practices
SOUPS '15 . Iulia Ion, Rob Reeder, Sunny Consolvo.
10/9 Authentication and Phishing
The tangled web of password reuse.
NDSS '14 . Anupam Das, Joseph Bonneau, Matthew Caesar, Nikita Borisov, XiaoFeng Wang.
Detecting credential spearphishing in enterprise settings
SEC '17 . Grant Ho, Aashish Sharma, Mobin Javed, Vern Paxson, David Wagner.
10/11 Denial of Service
Inferring internet denial-of-service activity.
SEC '01 . David Moore, Geoffrey Voelker, Stefan Savage.
Understanding the Mirai Botnet
10/16 spam and ecrime, framing dependencies introduced by underground commoditization.
WEIS '15 . Kurt Thomas, Danny Huang, David Wang, Elie Bursztein, Chris Grier, Thomas Holt, Christopher Kruegel, Damon McCoy, Stefan Savage, Giovanni Vigna.
Spamalytics: An Empirical Analysis of Spam Marketing Conversion
CCS '08 . Chris Kanich, Christian Kreibich, Kirill Levchenko, Brandon Enright, Geoffrey Voelker, Vern Paxson, and Stefan Savage.
10/18 Software Attacks
Hacking blind s&p '14 . andrea bittau, adam belay, ali mashtizadeh, david mazieres, dan boneh. sok: eternal war in memory.
S&P '13 . Laszlo Szekeres, Mathias Payer, Tao Wei, Dawn Song.
10/23 Software Defenses
Native client: a sandbox for portable, untrusted x86 native code.
S&P '09 . Bennet Yee, David Sehr, Gregory Dardyk, J. Bradley Chen, Robert Muth, Tavis Ormandy, Shiki Okasaka, Neha Narula, Nicholas Fullagar.
Multiprogramming a 64 kB Computer Safely and Efficiently
SOSP '17 . Amit Levy, Bradford Campbell, Branden Ghena, Daniel B. Giffin, Pat Pannuto, Prabal Dutta, Philip Levis.
10/25 Malware and Supply Chain
Towards measuring supply chain attacks on package managers for interpreted languages.
NDSS '21 . Ruian Duan, Omar Alrawi, Ranjita Pai Kasturi, Ryan Elder, Brendan Saltaformaggio, Wenke Lee.
Before We Knew It: An Empirical Study of Zero-Day Attacks In The Real World
CCS '12 Leyla Bilge and Tudor Dumitraş.
10/30 Side Channels and Information Leakage
Timing analysis of keystrokes and timing attacks on ssh.
SEC '01 . Dawn Song, David Wagner, Xuqing Tia.
Spectre Attacks: Exploiting Speculative Execution
S&P '19 . P. Kocher, J. Horn, A. Fogh, D. Genkin, D. Gruss, W. Haas, M. Hamburg, M. Lipp, S. Mangard, T. Prescher, M. Schwarz, Y. Yarom.
11/1 Hardware
Stealthy dopant-level hardware trojans.
CHES '13 . Georg Becker, Francesco Regazzoni, Christof Paar, Wayne Burleson.
Comprehensive Experimental Analyses of Automotive Attack Surfaces
SEC '11 . Stephen Checkoway, Damon McCoy, Brian Kantor, Danny Anderson, Hovav Shacham, Stefan Savage.
W32.Stuxnet Dossier
Symantec Technical Report . Nicolas Falliere, Liam Murchu, Eric Chien.
11/8 Machine Learning
Towards evaluating the robustness of neural networks.
S&P '16 . Nicholas Carlini and David Wagner.
Outside the Closed World: On Using Machine Learning For Network Intrusion Detection
S&P '10 . Robin Sommer and Vern Paxson.
11/13 Vulnerable Populations / Security For Everyone
A stalker’s paradise: how intimate partner abusers exploit technology.
CHI '18 Diana Freed, Jackeline Palmer, Diana Minchala, Karen Levy, Thomas Ristenpart, Nicola Dell.
A11y Attacks: Exploiting Accessibility in Operating Systems
CCS '14 Yeongjin Jang, Chengyu Song, Simon Chung, Tielei Wang, Wenke Lee.
11/15 Privacy and Dark Patterns
Robust de-anonymization of large sparse datasets.
S&P '08 . Arvind Narayanan and Vitaly Shmatikov.
Dark patterns at scale: Findings from a crawl of 11K shopping websites
S&P '08 . Arunesh Mathur, Gunes Acar, Michael Friedman, Eli Lucherini, Jonathon Mayer, Marshini Chetty, Arvind Narayanan.
11/20 Thanksgiving Break
11/22 thanksgiving break, 11/27 surveillance and anonymity, keys under doormats.
MIT Technical Report '15. H. Abelson, R. Anderson, S. Bellovin, J. Benaloh, M. Blaze, W. Diffie, J. Gilmore, M. Green, S. Landau, P. Neumann, R. Rivest, J. Schiller, B. Schneier, M. Specter, D. Weitzner.
Tor: The Second-Generation Onion Router
SEC '04 . Roger Dingledine, Nick Mathewson, Paul Syverson.
11/29 Government Attacks and Disinformation
When governments hack opponents: a look at actors and technology.
SEC '14 . Bill Marczak, John Scott-Railton, Morgan Marquis-Boire, Vern Paxson.
Disinformation as Collaborative Work: Surfacing the Participatory Nature of Strategic Information Operations
CSCW '19 . Kate Starbird, Ahmer Arif, Tom Wilson.
12/4 Ethics and Problem Selection
The moral character of cryptographic work.
Phillip Rogaway.
Science, Security, and the Elusive Goal of Security as a Scientific Pursuit
S&P '17 . Cormac Herley and P.C. van Oorschot.
12/6 Final Presentations
No required reading. attendance mandatory., course structure.
This course is composed of three parts: reading and discussing foundational papers in every class, reading and presenting recent work for one class, and completing a group research project. Grading will be based on:
Readings and Discussion (30%)
We will read and discuss 1–2 papers for each class. Typically, these are formative works in an area of security. Students should come prepared to actively discuss assigned papers and to make substantive intellectual contributions. This means that you need to thoroughly read each paper ahead of time. Before each section, students will submit a short (400 word) summary and reaction for each each paper, as well as a proposal of one discussion question for class.
Students should submit the reading assignments through Gradescope by 2:30 pm on the day of each class . Paper responses should be completed individually without the assistance of LLMs (e.g., ChatGPT).
Grading will be based 20% on these written responses and 10% on in-class participation. We do not allow any late days for paper reactions, but students may skip two paper summaries and two lectures without penalty. We will take class attendance. However, participation grades are based on not only attendence, but active participation during class discussion.
Do not underestimate the amount of time required to properly read and process a research paper. Expect to spend several hours preparing for each section.
Topic Presentation (15%)
While reading formative papers helps to demonstrate how a subfield started, it oftentimes leaves us wondering how the area has evolved. To fill this gap, each student in the class will present one recent paper during the quarter topically relevant to that day's class. At the start of the quarter, students will have the opportunity to sign up for the topic/date that they want to present their paper. Stuents will have 12-15 minutes to present their paper.
Students are expected to do a literature search and to select a paper that was published in the last three years from a top-tier venue in security (e.g., IEEE Security and Privacy, USENIX Security, ACM Computer or Communication Security) or adjacent field (e.g., CHI, NSDI, ASPLOS, PLDI, etc.). Students should submit their papers to approval to the teaching staff a week prior to their presentation.
Course Project (55%)
Students will complete a quarter-long original research project in small groups (1–3 students) on a topic of their own choosing. Groups will present their work during the last two sections as well as submit a 6–10 page report, similar to the papers we read in the course.
- Project Proposal (5%). Project groups will meet with course staff to discuss their project during the third week of class and submit a one page project proposal. Written proposals are due on 10/16.
- Mid-Quarter Progress Report (5%). Submit a short (1–2 pages) progress report part way through the quarter. The report should indicate what has been accomplished, what work is remaining, obstacles the team has encountered, and any preliminary data or insights. Due 11/17.
- Class Presentation (10%). Each group will give a 10 minute class presentation during the last week of the course.
- Final Paper (35%). Groups will submit a final project report similar to the papers we read in the course. Papers should be 6–10 pages and use the USENIX LaTeX template . It may be helpful to read Writing Technical Articles if you haven't previously published any work in computer science. Due 12/8.
Students should submit all reports through Gradescope by 11:59PM on the day of each deadline.
In past offerings, well-executed projects have led to publications at top-tier security conferences and workshops. I'm happy to work with groups to publish their work.
This class has no final exam. Attendance on 12/6 is required.
This is a potential security issue, you are being redirected to https://csrc.nist.gov .
You have JavaScript disabled. This site requires JavaScript to be enabled for complete site functionality.
An official website of the United States government
Here’s how you know
Official websites use .gov A .gov website belongs to an official government organization in the United States.
Secure .gov websites use HTTPS A lock ( Lock Locked padlock icon ) or https:// means you’ve safely connected to the .gov website. Share sensitive information only on official, secure websites.
- Drafts for Public Comment
- All Public Drafts
- NIST Special Publications (SPs)
- NIST interagency/internal reports (NISTIRs)
- ITL Bulletins
- White Papers
- Journal Articles
- Conference Papers
- Security & Privacy
Applications
Technologies.
- Laws & Regulations
- Activities & Products
- News & Updates
- Cryptographic Technology
- Secure Systems and Applications
- Security Components and Mechanisms
- Security Engineering and Risk Management
- Security Testing, Validation, and Measurement
- Cybersecurity and Privacy Applications
- National Cybersecurity Center of Excellence (NCCoE)
- National Initiative for Cybersecurity Education (NICE)
Security and Privacy
- digital signatures
- key management
- lightweight cryptography
- message authentication
- post-quantum cryptography
- random number generation
- secure hashing
- cybersecurity supply chain risk management
- general security & privacy
- access authorization
- access control
- Personal Identity Verification
- public key infrastructure
- personally identifiable information
- privacy engineering
- categorization
- continuous monitoring
- controls assessment
- privacy controls
- security controls
- risk assessment
- roots of trust
- system authorization
- advanced persistent threats
- information sharing
- intrusion detection & prevention
- vulnerability management
- accessibility
- testing & validation
- acquisition
- asset management
- audit & accountability
- awareness training & education
- configuration management
- contingency planning
- incident response
- maintenance
- media protection
- patch management
- personnel security
- physical & environmental protection
- program management
- security automation
- reliability
- artificial intelligence
- cloud & virtualization
- combinatorial testing
- personal computers
- quantum information science
- smart cards
- operating systems
- communications & wireless
- cyber-physical systems
- cybersecurity education
- cybersecurity framework
- cybersecurity workforce
- industrial control systems
- Internet of Things
- mathematics
- positioning navigation & timing
- small & medium business
Laws and Regulations
- Comprehensive National Cybersecurity Initiative
- Cybersecurity Strategy and Implementation Plan
- Cyberspace Policy Review
- Executive Order 13636
- Executive Order 13702
- Executive Order 13718
- Executive Order 13800
- Executive Order 13905
- Executive Order 14028
- Executive Order 14110
- Federal Cybersecurity Research and Development Strategic Plan
- Homeland Security Presidential Directive 7
- Homeland Security Presidential Directive 12
- OMB Circular A-11
- OMB Circular A-130
- Cyber Security R&D Act
- Cybersecurity Enhancement Act
- E-Government Act
- Energy Independence and Security Act
- Federal Information Security Modernization Act
- First Responder Network Authority
- Health Insurance Portability and Accountability Act
- Help America Vote Act
- Internet of Things Cybersecurity Improvement Act
- Federal Acquisition Regulation
Activities and Products
- annual reports
- conferences & workshops
- quick start guides
- reference materials
- standards development
- financial services
- hospitality
- manufacturing
- public safety
- telecommunications
- transportation
250 Plus Cyber Security Research Topics for Students of All Levels

Cybersecurity research is all about exploring the intricate web of digital defenses. It’s essential because our digital world plays a huge role in our society, economy and well-being. Picking the right research topic becomes important since it will be the foundation for ground-breaking discoveries and defenses. Speaking of which, we have lists of over 250 impressive topics for you to write an inspiring piece like the professional Native Custom writing services providers. So, without further ado, let’s get started.
Table of Contents
Comprehensive Lists of Impressive Cyber Security Research Topics
Writing a research paper on these topics guarantees results and let you shape the different safety from creative breakthroughs. Here you go with the first list.
Interesting Cyber Security Paper Topics
Anything in computer science, including cyber security, is interesting, and so is it for our writers . Here’s the first list of cyber security research paper topics for computer science students.
- Languages for domain-specific modeling in cyber security
- To interpret the behavior of a specific hacker based on specific concerns
- A system’s perception can be dynamically adapted by using roles
- The relationship between cyber security and the Internet
- The following authentic measures ensure the security of data transmissions
- Keeping social media users safe and protected daily
- Virtual spaces are subject to legislation
- Is it possible for cyber security analysts to control crimes on the deep web?
- Identify the security risks associated with a system
- The Importance of cyber security in Ensuring the Safety of electronic payments
- A cyber-security approach to telecommunications networks
- To ensure unbeatable digital privacy, what skills must be learned?
- Knowledge and understanding of cyber security at a deep level
- A look at the contributions of remote workers from South Asia to cyber-security
- Aspects of data security that involve cybersecurity and cryptography
- E-Commerce solutions are concerned with cyber security
- A system for preventing industrial espionage and trade secret theft
- Utilize a hacker’s perception of a system to find zero errors and vulnerabilities
- Insights into the relationship between Information Technology and Cyber Security
- Critical thinking and advanced knowledge to keep the Internet safe
- A detailed analysis of vulnerabilities, architectures, and configurations
- Integrating heterogeneous DSMLs into an interoperable environment
- Several aspects of cybersecurity are covered in cybersecurity research
- A description of threats, a model of hackers, and a model of systems
- Analyzing the Attack from a Hacker’s Perspective
- The evolving nature of cyber security methods and knowledge
- Cybersecurity models that are adaptable and linked to existing ones
- Modeling framework representing different threats
- Developing and interpreting a case study from the hacker’s perspective
Best Cyber Security Thesis Topics for Research
Looking for the list of best cyber security topics for research thesis? Here you go:
- Preparing a research project or thesis for cybersecurity
- Realization of a prototype on a platform and experimentation in cyber security
- An in-depth analysis of the cyber surveillance system in terms of the data flow within it
- The modeling of maritime cyber situational awareness in the maritime domain
- An Overview of the business processes involved in Cyber Situational Awareness
- Analyzing the situation in case of a cyberattack and determining what needs to be done
- Conventionally inadequate cyber-surveillance methods
- Contributions of cyber surveillance to the Difficulties of the maritime world
- Threats and feared events related to cyber security: sources and sources of threats
- Transformation of the maritime industry through the use of digital technology
- Analysis of public policies regarding the adoption of cloud computing and big data
- The Strategic Importance of the maritime sector
- How to make a security strategy to meet the requirements of the cybersecurity information sharing act
- The security level of a system based on system modeling
- Collective intelligence for better privacy protection in connected environments
- Design and generation of tests by data alteration for transport control and monitoring systems
- Some issues around clustering: robustness, large dimensions, and intrusion detection
- Analyzing digital social media for the detection of points of view
- An effective approach to securing architecture through the use of dynamic management systems
- An overview of the security of industrial cyber-physical systems
- Preparing a company’s security strategy following the cybersecurity information sharing act
- Ransomware attacks present a variety of challenges when it comes to crisis communication
- Hardware crypto processors and units designed to perform arithmetic operations
- Lawyers who practice cyber law in the course of their practice
- Cloud Architectures and Models for an Efficient and Secure Cloud Environment
- Making post-quantum cryptography more agile and secure by accelerating and securing it
- Modeling intrusion detection systems in a formal way
- From physical models of cyber security to models that are based on deep learning
- In an untrusted cloud, how do you ensure secure computing?
- A novel approach to anomaly detection in industrial systems using online kernel learning
- Intelligent transport systems based on machine learning detect intrusions
Unique Cyber Security Research Topics
If you want to impress your professor with some unique stuff, try out these research topics in cybersecurity.
- Pervasive applications that integrate connected objects in a secure manner
- Information security is protected from criminal activity by criminal law.
- In the face of cybercrime, criminal justice must be reformed.
- The risks associated with cyberattacks should be taken into consideration.
- Enhancing security and trust in distributed networks through the use of blockchains
- Optimal security strategies for connected objects based on the use of active defense mechanisms
- A comparative analysis of 5G and 6G Trust and Reliability
- The internal security of the European Union. A study of the relationship between the law and public policy
- The application of anomaly detection to access management and identity management
- International law’s response to the use of digital technology for terrorist purposes
- Monitoring systems for industrial control systems that detect intrusions
- An overview of threats to critical wireless infrastructure, their detection, identification, and quarantine
- A security risk optimization approach to training on heterogeneous quality data
- An analysis of software vulnerabilities that can be exploited using a generic methodology
- Industrial device security characterization using safety/security models
- Analysis and evaluation of anomalous propagation in maritime cyber-physical systems
- Stream processing is used as a virtual function for Big Data surveillance and threat detection
- Cybersecurity research paper for cloud security
- In the process industry, it is necessary to control cyber-physical risks.
- A system based on machine learning techniques for the assessment of security risks and the detection of cyber intrusions
- Identifying, understanding, and securing cyber risks within an organization
- An analysis of the cyber defense policies of the United States and India in comparison
- Incorporating intrusion detection systems into the learning process under the supervision
- Is it possible to scale privacy management techniques to a multi-agent system?
Trendy Cyber Security Research Topics
Keep up with trends while you work out your research paper on cyber security with these topics or cyber security research questions
- Integration of cybersecurity and Safety to improve the resilience of banking systems
- Cybersecurity is an emerging phenomenon in communities all over the world.
- Various economic factors are impacted by the threat of cybercrime
- Investing in encrypted data: what’s at stake
- The Importance of securing the cyber-security of operators cannot be overstated
- The security of industrial equipment that is connected to the Internet
- Anomaly detection and explain ability from learning on knowledge graphs: application to cybersecurity
- Cloud security with Google Cloud: Detailed Analysis
- The extraction of information for Cybersecurity Vulnerability Management
- The English legal system concerning cybersecurity: a comparative approach
- Developing preventive practices in the domain of cyber security as part of the design process
- An analysis of how artificial intelligence systems interact with each other in the Context of cybersecurity
- A cybersecurity research paper on heterogeneous systems-on-chip cybersecurity
- Cybersecurity supervision systems must take into account business objectives and imperatives.
- Analysis and optimization of binary programs for cyber-security in a dynamic manner
Cyber Security Research Topics Related to Hacking
The only way to stay safe from hacking is to spread awareness. And who’s going to do it better than the researchers? This list of cybersecurity topics for research is your chance to delve into hacking and stuff.
- What we know and what we don’t know about hacking and hacker history
- A brief description of the types of hacking or hackers that exist
- Hacking for financial gain of a criminal nature, such as stealing credit card numbers or breaching banking systems
- Using a hacking program to hack into an Android phone
- Preventing hacking is a critical aspect of cybersecurity.
- What you need to do to get rid of threats on the Internet
- The study of hackers from a sociological perspective offers a different perspective on the phenomenon.
- Is the cloud a safe place to run operations? A detailed study of recent developments in cloud security
- The perspective of a hacker from a psychological point of view
- An in-depth analysis of how to become an ethical hacker, with a case study
- Is it true that Small and Medium Businesses are more likely to fall victim to hacking attacks?
- What is the risk of our Facebook account being hacked, and how can we prevent it from happening?
- A comparison of the psychological profiles and similarities between some of the world’s most famous hackers
- Network security research to build physical data security models
- The horrors of webcam blackmailing: The threat and effects
- Virtual vs. Physical data security? What makes them different and alike?
- What can be done about scammers who send you emails containing scams? Are there any measures that we can take to prevent this from happening?
- What are the latest cloud security threats?
- How can risk management security personnel help prevent cyber-attacks?
- An analysis of the hacking threat and the development of a revised safeguard standard
- Test for the effectiveness of attacks on a server that has been hacked before
- Ethics of hacking: The Ten Commandments
- What kind of cyber-attacks are a threat to network security?
- A case study examining the Objectives of ethical hacking
- An analysis of the psychological and sociological aspects of hacking: A definition
Computer Security Research Topics-International Controversy
Not even global organizations and the political world are safe from cyber-attacks. Following are some greatest ideas to work within this scenario.
- War In Ukraine: Can the United States be the next target of Russian hackers?
- War in Ukraine: Russian Television Hacked during Vladimir Putin’s Speech
- Analysis of the Stuxnet Attack on Iranian nuclear power plants in Detail
- In your opinion, how do you see the Wikileaks scandal from your perspective?
- WhatsApp data leaks: Understanding the consequences and raising awareness to prevent such accidents from happening in the future
- Research into one of the most notorious hacking groups on the Internet, World of Hell
- The impact of Mr. Robot on the real world of hacktivism: A look at FSociety
- Cyberspace’s Great Hacker War, the cyberspace equivalent of a gang war
- An overview of Operation Ababil, the cyberattacks that threatened the American cyber economy
- Chinese hackers against the United States attempted data breaches.
- Network security threats to large corporations
- How can government organizations prevent network attacks?
- Establishing secure algorithms in network security research
- Estonia was the victim of a cyber-attack in 2007
- During the war in Ukraine, Russian hackers have launched cyber-attacks on Ukraine.
- The accidental birth of the Brain Virus
- Mobile platform security: A case study of Pakistan phone calls data breaches
Information Security Research Paper Topics for University
- What is the role of company management in the fight against cybersecurity weaknesses?
- Using security flaws in certain operating systems to circumvent security
- Research Paper on Advanced Cyber Threats: Protecting Your Business
- Cyberattacks start with employee behavior, a potential weakness for SMEs
- Defending the public and private sectors from cybercrime
- Arranging security awareness training
- Payments and protection of personal information via electronic and digital means
- Increasing consumer confidence in e-commerce, SSL, and cybersecurity
- Accountability in the privacy sector: privacy management programs
- A list of ten tips to help you reduce the risk of a privacy breach
- Online threats related to spam and their impact on the Internet
- Does cyberspace play a role in law enforcement?
- Cyber risk management is one of the most critical aspects of IT
- Cybersecurity and Governance in the Digital Age: A Checklist
- What can we do to prevent future hacking attacks?
- An overview of the history and evolution of attacks over time
- The Importance of corporate culture in cyber security
- Organizational Indicators of Cybersecurity and IT Risks
- Risk management services provided by third parties: outsourcing to a company specializing in this field
- An Overview of the crisis management cycle in the Context of cybersecurity hazards
- The Importance of keeping up with the latest technologies and regulations cannot be overstated
- Informing employees about how to protect themselves from having their SME’s data hacked
We hope these information security research topics have guided you on the right path. You can choose any one and be sure that they’ll make you professor jawdrop and help you build your academic career.
Writing a good research paper starts with choosing a good topic. Hopefully this blog post was useful in letting you know about some good subjects to begin writing your paper. Still if you are having trouble writing one, place your order and let our experts do the magic for you.
Order Original Papers & Essays
Your First Custom Paper Sample is on Us!

Timely Deliveries

No Plagiarism & AI

100% Refund
Try Our Free Paper Writing Service
Related blogs.
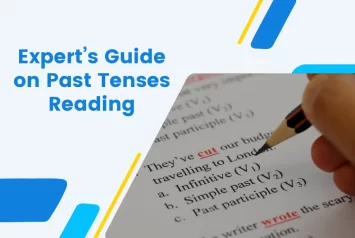
Connections with Writers and support
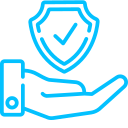
Privacy and Confidentiality Guarantee
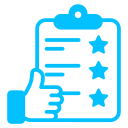
Average Quality Score
Security Management Research Paper Topics
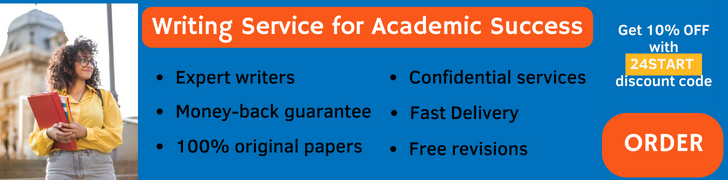
Security management research paper topics are a critical area of study for management students looking to explore the complex world of safeguarding organizational assets. Security management covers various facets, including information security, physical security, risk management, compliance, and more. The study of security management is increasingly relevant in our technology-driven world. Research within this field equips students with the knowledge to protect an organization’s information and physical resources, and the skills to respond to rapidly evolving security threats. This page provides a comprehensive list of research topics to assist students in selecting a subject that aligns with their interests and the current industry demands. The following sections will provide an in-depth look into various security management research topics, organized into ten categories with ten subjects each. Additionally, this page will offer insights into how to choose and write about these topics, along with an overview of iResearchNet’s customized writing services for those who seek professional assistance.
100 Security Management Research Paper Topics
The field of security management is as vast as it is vital in today’s global landscape. From protecting information systems to ensuring the physical safety of assets, security management plays a central role in the smooth operation of organizations across various sectors. As we dive into this comprehensive list of security management research paper topics, students will find a plethora of subjects that are both challenging and relevant. The topics are divided into ten distinct categories, each focusing on a different aspect of security management.
Academic Writing, Editing, Proofreading, And Problem Solving Services
Get 10% off with 24start discount code.
- Role of Encryption in Data Protection
- Security Protocols in Wireless Networks
- Cloud Security Management Strategies
- Biometric Security Measures
- Ethical Hacking and Defense Strategies
- Security Risks in Internet of Things (IoT)
- Mobile Application Security
- Compliance with GDPR and Other Regulations
- Social Engineering Attacks and Prevention
- Virtual Private Networks (VPNs) and Security
- Designing Secure Buildings and Facilities
- Access Control Systems and Technologies
- Surveillance and Monitoring Techniques
- Security Personnel Training and Management
- Risk Assessment for Physical Threats
- Vehicle Security and Fleet Management
- Maritime Security Protocols
- Security Measures for Public Events
- Emergency Response and Evacuation Planning
- Integration of Technology in Physical Security
- Enterprise Risk Management Strategies
- Security Policies and Compliance Auditing
- Regulatory Compliance in Different Industries
- Risk Mitigation and Disaster Recovery Planning
- Cyber Insurance and Risk Transfer
- Security Awareness and Training Programs
- Third-party Vendor Risk Management
- Financial Risk Management in Security Operations
- Implementing ISO Security Standards
- Privacy Policies and Consumer Protection
- Cyber Threat Intelligence and Analysis
- Intrusion Detection Systems and Firewalls
- Secure Software Development Lifecycle
- Incident Response and Crisis Management
- Security Considerations in E-commerce
- Protecting Against Ransomware and Malware
- Security in Social Networking Sites
- Cybersecurity in Critical Infrastructure
- Mobile Device Security in the Workplace
- Privacy vs. Security in Cyber Law
- Role of CISO (Chief Information Security Officer)
- Security Leadership and Governance
- Insider Threat Management and Mitigation
- Security Culture and Employee Behavior
- Contractual and Legal Aspects of Security
- Intellectual Property Protection
- Security Metrics and Performance Indicators
- Outsourcing Security Services
- Security Budgeting and Financial Management
- Integrating Security with Business Strategy
- Terrorism and Counterterrorism Strategies
- Security Intelligence and Law Enforcement
- Border Control and Immigration Security
- Cyber Warfare and State-sponsored Attacks
- Protection of Critical National Infrastructure
- Emergency Preparedness and Response
- Security Considerations in International Relations
- Humanitarian Security and Crisis Management
- Nuclear Security and Non-proliferation
- Global Maritime Security Issues
- Security in Hospitals and Healthcare Facilities
- Patient Data Privacy and HIPAA Compliance
- Medical Device and IoT Security
- Emergency Medical Services and Security
- Security Measures for Mental Health Facilities
- Pharmaceutical Supply Chain Security
- Bioterrorism and Public Health Security
- Security Education for Healthcare Professionals
- Medical Records Security and Management
- Telemedicine and Remote Healthcare Security
- Security Considerations in Online Retail
- Fraud Detection and Prevention Strategies
- Payment Security and PCI Compliance
- Inventory Security and Loss Prevention
- Consumer Trust and Brand Protection
- E-commerce Regulations and Compliance
- Security in Omnichannel Retailing
- Secure Customer Experience Design
- Mobile Commerce Security
- Retail Surveillance and Anti-shoplifting Techniques
- Campus Safety and Security Measures
- Cybersecurity Education and Curriculum
- Student Data Privacy and Protection
- Security in Online Learning Platforms
- Intellectual Property Rights in Academia
- Emergency Response Plans for Educational Institutions
- School Transportation Security
- Security Measures for Laboratories and Research Facilities
- Ethical Guidelines in Academic Research
- Security Considerations in International Student Exchange
- Artificial Intelligence in Security
- Quantum Computing and Cryptography
- Security Implications of 5G Technology
- Sustainable and Green Security Practices
- Human Factors in Security Design
- Blockchain for Security Applications
- Virtual and Augmented Reality Security
- Security in Autonomous Vehicles
- Integration of Smart Technologies in Security
- Ethical Considerations in Emerging Security Technologies
Security management is an ever-evolving field, reacting to both technological advancements and global socio-political changes. The above categories and topics encompass a broad spectrum of the security management domain. This comprehensive list is designed to inspire students and guide them towards a research paper that not only interests them but also contributes to the growing body of knowledge in security management. By exploring these topics, students will have the opportunity to deepen their understanding of current issues and become part of the ongoing conversation in this vital area of study.
Security Management and the Range of Research Paper Topics
Introduction to security management.
Security management has increasingly become a central concern for organizations, governments, and individuals in our interconnected and technologically driven world. Its primary focus is on safeguarding assets, information, and people by assessing risks and implementing strategies to mitigate potential threats. From the micro-level of individual privacy protection to the macro-level of national security, the concepts and practices within this field permeate almost every aspect of our daily lives. This article delves into the fundamental aspects of security management and explores the extensive range of research paper topics it offers.
Key Principles and Concepts in Security Management
- Risk Assessment and Mitigation: At the core of security management lies the process of identifying, evaluating, and minimizing risks. It involves recognizing potential vulnerabilities, assessing the likelihood of threats, and implementing measures to reduce the potential impact.
- Compliance and Regulation: Security management is also heavily influenced by various laws, regulations, and industry standards. Whether it’s GDPR for data protection or HIPAA for healthcare, compliance with these regulations is essential to avoid legal consequences.
- Physical and Cyber Security: Security management encompasses both the physical and digital realms. Physical security focuses on protecting tangible assets, such as buildings and equipment, while cyber security emphasizes safeguarding digital information.
- Human Factors: People are often considered the weakest link in security. Training, awareness, and a robust security culture are crucial in ensuring that employees and stakeholders understand and adhere to security protocols.
- Technology and Innovation: With the advent of new technologies like AI, blockchain, and IoT, security management must continuously evolve to address the unique challenges and opportunities they present.
- Global Perspectives: In a globally connected world, security management must consider international laws, cross-border data flows, and the unique risks associated with different geographical regions.
- Ethics and Social Responsibility: Ethical considerations in security management include respecting individual privacy, transparency in surveillance, and social responsibility in using technology for security purposes.
Range and Depth of Research Paper Topics
Given the complexity and multidimensionality of security management, the range of research paper topics in this field is vast. The following sections provide an insight into the various dimensions that can be explored:
- Information Security Management: Research can focus on encryption, authentication, intrusion detection, or explore the psychological aspects of social engineering attacks.
- Physical Security Management: Topics may include architectural design for security, biometrics, or the balance between security and convenience in access controls.
- Organizational Security Management: This includes leadership and governance in security, insider threats, and the alignment of security strategies with business goals.
- Global and National Security Management: Areas to explore here include counterterrorism strategies, cybersecurity policies among nations, or human rights considerations in security protocols.
- Retail and E-commerce Security Management: From payment security to fraud detection, this area explores the unique challenges in the retail and online shopping environment.
- Emerging Trends in Security Management: This invites research into the future of security management, considering technological advancements, emerging threats, and the ethical implications of new tools and techniques.
Security management is an intricate field that intertwines technological, human, organizational, and societal aspects. It continues to evolve in response to the rapidly changing global landscape marked by technological innovation, geopolitical shifts, and emerging threats. The range of research paper topics in security management reflects this diversity and offers a wealth of opportunities for students to engage with cutting-edge issues.
The ongoing development of this field requires fresh insights, innovative thinking, and a commitment to understanding the underlying principles that govern security management. By delving into any of the areas outlined above, students can contribute to this exciting and ever-changing field. Whether exploring traditional aspects like risk management or venturing into the realms of AI and blockchain, the possibilities for research are as broad and varied as the field itself.
This article provides a foundational understanding of security management and serves as a springboard for further exploration. It’s a gateway to a myriad of research avenues, each offering a unique perspective and challenge, all united by the common goal of enhancing the security and safety of our interconnected world.
How to Choose Security Management Research Paper Topics
Selecting a topic for a research paper in the field of security management is a crucial step that sets the tone for the entire research process. The breadth and depth of this field offer a wide array of possibilities, making the choice both exciting and somewhat daunting. The topic must be relevant, engaging, unique, and, most importantly, aligned with the researcher’s interests and the academic requirements. This section provides a comprehensive guide on how to choose the perfect security management research paper topic, with 10 actionable tips to simplify the process.
- Identify Your Interests: Begin by exploring areas within security management that truly intrigue you. Whether it’s cyber threats, risk management, or physical security measures, your passion for the subject will drive a more engaging research process.
- Understand the Scope: Security management spans across various sectors such as IT, healthcare, retail, and more. Assess the scope of your paper to determine which sector aligns best with your academic needs and professional goals.
- Consider the Relevance: Choose a topic that is pertinent to current trends and challenges in security management. Researching emerging threats or innovative technologies can lead to more compelling findings.
- Assess Available Resources: Ensure that there is enough accessible information and research material on the chosen topic. A topic too obscure might lead to difficulties in finding supporting evidence and data.
- Consult with Your Advisor or Mentor: An experienced academic advisor or mentor can provide valuable insights into the feasibility and potential of various topics, helping you make an informed decision.
- Balance Complexity and Manageability: Selecting a topic that is too broad can be overwhelming, while a narrow topic might lack depth. Striking the right balance ensures that you can comprehensively cover the subject within the stipulated word count and time frame.
- Consider Ethical Implications: Especially in a field like security management, ethical considerations must be at the forefront. Any topic involving human subjects, privacy concerns, or potentially sensitive information should be approached with caution and integrity.
- Align with Learning Objectives: Reflect on the specific learning outcomes of your course or program, and choose a topic that aligns with these objectives. It ensures that your research contributes to your overall academic development.
- Evaluate Potential Contributions: Think about what new insights or perspectives your research could offer to the field of security management. Choosing a topic that allows you to make a meaningful contribution can be more satisfying and impactful.
- Experiment with Preliminary Research: Before finalizing a topic, conduct some preliminary research to gauge the existing literature and potential research gaps. It can help refine your focus and provide a clearer direction.
Choosing a research paper topic in security management is a multifaceted process that requires thoughtful consideration of various factors. By following the tips outlined above, you can navigate through the complexities of this task and select a topic that resonates with your interests, aligns with academic goals, and contributes to the broader field of security management. Remember, a well-chosen topic is the foundation upon which a successful research paper is built. It’s the starting point that leads to a journey filled with discovery, analysis, and intellectual growth. Make this choice wisely, and let it be a gateway to an engaging and rewarding research experience.
How to Write a Security Management Research Paper
A. introductory paragraph.
Writing a research paper on security management requires more than just a keen interest in the subject; it demands a systematic approach, adherence to academic standards, and the ability to synthesize complex information. Security management, with its multifaceted nature encompassing physical security, cybersecurity, risk assessment, and more, offers an exciting but challenging landscape for research. In this section, we will delve into a step-by-step guide comprising 10 vital tips on how to write an effective security management research paper. These tips aim to guide you through the research, planning, writing, and revision stages, ensuring a coherent and impactful paper.
- Choose the Right Topic: Guidance: Reflect on your interests, the current trends in the field, and the available resources. Consult with mentors and refer to the previous section for more insights into selecting the perfect topic.
- Conduct Thorough Research: Guidance: Use reliable sources like academic journals, books, and reputable online resources. Gather diverse viewpoints on the topic and keep track of the sources for citation.
- Develop a Strong Thesis Statement: Guidance: The thesis should encapsulate the main argument or focus of your paper. It should be clear, concise, and specific, providing a roadmap for the reader.
- Create an Outline: Guidance: Outline the main sections, including introduction, literature review, methodology, findings, discussion, conclusion, and references. An organized structure helps maintain coherence and logical flow.
- Write a Compelling Introduction: Guidance: Begin with a hook that grabs the reader’s attention, provide background information, and conclude with the thesis statement. The introduction sets the stage for the entire paper.
- Employ the Appropriate Methodology: Guidance: Choose the research methods that align with your research question and objectives. Explain the rationale behind your choices, ensuring that they adhere to ethical standards.
- Analyze Findings and Discuss Implications: Guidance: Present your research findings in a clear and unbiased manner. Discuss the implications of the results in the context of the existing literature and real-world applications.
- Conclude with Insight: Guidance: Summarize the main findings, restate the thesis in the context of the research, and discuss the potential limitations and future research directions. The conclusion should leave the reader with something to ponder.
- Adhere to Academic Formatting: Guidance: Follow the specific formatting guidelines required by your institution or the style guide (APA, MLA, etc.). Pay attention to citations, references, headings, and overall presentation.
- Revise and Proofread: Guidance: Allocate ample time for revising content, structure, and language. Use tools or seek help from peers or professionals for proofreading to ensure grammatical accuracy and clarity.
Writing a security management research paper is a rigorous and intellectually stimulating endeavor that requires meticulous planning, research, and execution. The tips provided in this guide are meant to facilitate a well-structured and insightful paper that adheres to academic excellence. By following these guidelines, you not only develop a comprehensive understanding of security management but also contribute valuable insights to this evolving field. Remember, writing is a process of exploration, articulation, and refinement. Embrace the challenge, learn from the journey, and take pride in the scholarly contribution you make through your research paper on security management.
iResearchNet’s Custom Research Paper Services
In the complex world of security management, crafting a top-notch research paper can be a daunting task. The landscape of security management is multifaceted, encompassing areas such as cybersecurity, risk analysis, policy development, physical security, and much more. For students juggling multiple responsibilities, producing a quality research paper on these intricate subjects may seem nearly impossible. That’s where iResearchNet comes into play. Offering tailor-made solutions to your academic needs, iResearchNet is your go-to service for custom security management research papers. Below are the features that make iResearchNet the ideal choice for your academic success.
- Expert Degree-Holding Writers: At iResearchNet, we employ writers who not only hold advanced degrees but also have extensive experience in security management. Their expertise ensures that your paper is insightful, well-researched, and academically sound.
- Custom Written Works: Every research paper is crafted from scratch, tailored to your specific needs, guidelines, and preferences. Our writers work closely with you to understand your vision, making the paper uniquely yours.
- In-Depth Research: Our team engages in thorough research, using reputable sources and cutting-edge methodologies. This diligent approach guarantees a comprehensive understanding of the subject and a well-rounded paper.
- Custom Formatting: Adhering to academic standards is crucial, and our writers are skilled in various formatting styles. Whether APA, MLA, Chicago/Turabian, or Harvard, your paper will be formatted to perfection.
- Top Quality: Quality is at the core of our services. From the initial draft to the final submission, we maintain the highest standards of excellence, ensuring that your paper stands out.
- Customized Solutions: We recognize that each student’s needs are unique. Hence, our solutions are not one-size-fits-all but are customized to meet your specific requirements, timelines, and academic level.
- Flexible Pricing: Quality doesn’t have to break the bank. Our pricing structure is designed to be affordable and flexible, providing various options to fit different budgets.
- Short Deadlines: Whether you’re facing a last-minute crunch or planning ahead, our writers can accommodate tight deadlines. Even within as short as 3 hours, we deliver without compromising on quality.
- Timely Delivery: Your time is valuable, and we respect that. Our commitment to timely delivery ensures that you receive your paper well before the deadline, giving you ample time for review.
- 24/7 Support: Questions or concerns? Our support team is available around the clock. With 24/7 assistance, you can rest assured that help is always just a click away.
- Absolute Privacy: Your privacy is our priority. We employ stringent security measures to protect your personal information. With iResearchNet, your details are safe, secure, and confidential.
- Easy Order Tracking: With our user-friendly tracking system, you can easily monitor the progress of your order. Stay updated, provide feedback, and enjoy a smooth and transparent process.
- Money Back Guarantee: Your satisfaction is our goal. If, for any reason, our services do not meet your expectations, our money-back guarantee ensures that you are not at a loss.
iResearchNet’s custom security management research paper services are more than just a promise; they are a commitment to excellence, convenience, and integrity. Our blend of expert writers, personalized solutions, quality assurance, and robust support makes us the preferred choice for students across the globe. Dive into the world of security management without the stress of paper writing, knowing that iResearchNet has got your back. Embark on your academic journey with confidence and trust in a partner who understands your needs and shares your pursuit of excellence. With iResearchNet, you’re not just ordering a paper; you’re investing in your future.
Secure Your Academic Success Today
Are you feeling overwhelmed with the prospect of writing your security management research paper? Perhaps you’re struggling to find the right topic, or the research is becoming a tedious task? You don’t have to go through this alone. With iResearchNet’s specialized writing services, all your academic challenges can be turned into opportunities for success.
What sets iResearchNet apart from other writing services is not just our expertise and quality but our understanding of students’ needs. We know that every research paper is not just a task but a step towards your future career in security management. That’s why we invest our best resources to make sure your paper is nothing short of perfect. Our expert writers, meticulous research, and dedication to your satisfaction are all geared towards one goal – helping you excel.
We don’t just write papers; we create opportunities for learning and growth. When you choose iResearchNet, you’re not only receiving a top-notch research paper but also gaining access to a treasure trove of knowledge in security management. With our 24/7 support, flexible pricing, and customizable solutions, your success is no longer a distant dream but a tangible reality.
Take the step towards a brighter academic future. Don’t let the burden of research and writing hold you back from achieving your best. Click the button below to place your order and begin a collaborative journey with iResearchNet. With our secure and user-friendly platform, ordering your custom security management research paper is just a few clicks away. Empower yourself with the right partner, and let iResearchNet pave the way to your academic success.
ORDER HIGH QUALITY CUSTOM PAPER

Suggestions or feedback?
MIT News | Massachusetts Institute of Technology
- Machine learning
- Social justice
- Black holes
- Classes and programs
Departments
- Aeronautics and Astronautics
- Brain and Cognitive Sciences
- Architecture
- Political Science
- Mechanical Engineering
Centers, Labs, & Programs
- Abdul Latif Jameel Poverty Action Lab (J-PAL)
- Picower Institute for Learning and Memory
- Lincoln Laboratory
- School of Architecture + Planning
- School of Engineering
- School of Humanities, Arts, and Social Sciences
- Sloan School of Management
- School of Science
- MIT Schwarzman College of Computing
A new way to detect radiation involving cheap ceramics
Press contact :.
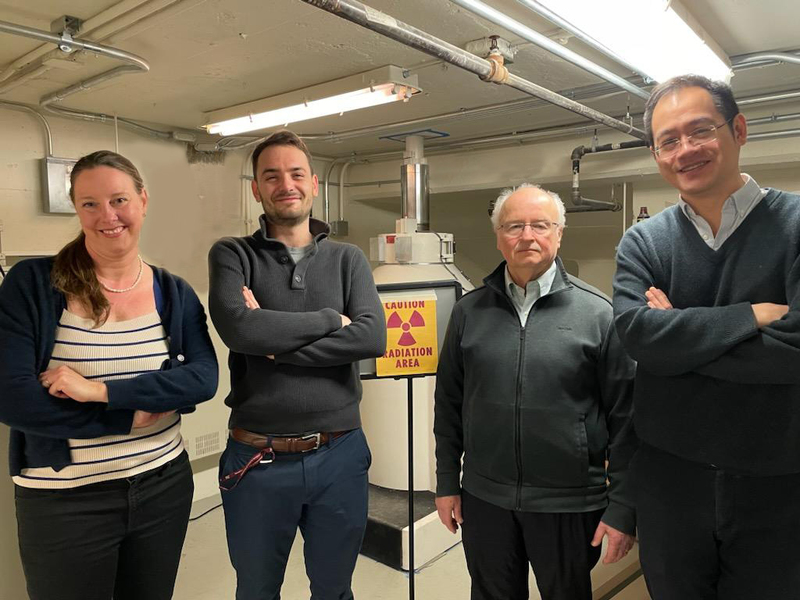
Previous image Next image
The radiation detectors used today for applications like inspecting cargo ships for smuggled nuclear materials are expensive and cannot operate in harsh environments, among other disadvantages. Now, in work funded largely by the U.S. Department of Homeland Security with early support from the U.S. Department of Energy, MIT engineers have demonstrated a fundamentally new way to detect radiation that could allow much cheaper detectors and a plethora of new applications.
They are working with Radiation Monitoring Devices , a company in Watertown, Massachusetts, to transfer the research as quickly as possible into detector products.
In a 2022 paper in Nature Materials , many of the same engineers reported for the first time how ultraviolet light can significantly improve the performance of fuel cells and other devices based on the movement of charged atoms, rather than those atoms’ constituent electrons.
In the current work, published recently in Advanced Materials , the team shows that the same concept can be extended to a new application: the detection of gamma rays emitted by the radioactive decay of nuclear materials.
“Our approach involves materials and mechanisms very different than those in presently used detectors, with potentially enormous benefits in terms of reduced cost, ability to operate under harsh conditions, and simplified processing,” says Harry L. Tuller, the R.P. Simmons Professor of Ceramics and Electronic Materials in MIT’s Department of Materials Science and Engineering (DMSE).
Tuller leads the work with key collaborators Jennifer L. M. Rupp, a former associate professor of materials science and engineering at MIT who is now a professor of electrochemical materials at Technical University Munich in Germany, and Ju Li, the Battelle Energy Alliance Professor in Nuclear Engineering and a professor of materials science and engineering. All are also affiliated with MIT’s Materials Research Laboratory
“After learning the Nature Materials work, I realized the same underlying principle should work for gamma-ray detection — in fact, may work even better than [UV] light because gamma rays are more penetrating — and proposed some experiments to Harry and Jennifer,” says Li.
Says Rupp, “Employing shorter-range gamma rays enable [us] to extend the opto-ionic to a radio-ionic effect by modulating ionic carriers and defects at material interfaces by photogenerated electronic ones.”
Other authors of the Advanced Materials paper are first author Thomas Defferriere, a DMSE postdoc, and Ahmed Sami Helal, a postdoc in MIT’s Department of Nuclear Science and Engineering.
Modifying barriers
Charge can be carried through a material in different ways. We are most familiar with the charge that is carried by the electrons that help make up an atom. Common applications include solar cells. But there are many devices — like fuel cells and lithium batteries — that depend on the motion of the charged atoms, or ions, themselves rather than just their electrons.
The materials behind applications based on the movement of ions, known as solid electrolytes, are ceramics. Ceramics, in turn, are composed of tiny crystallite grains that are compacted and fired at high temperatures to form a dense structure. The problem is that ions traveling through the material are often stymied at the boundaries between the grains.
In their 2022 paper, the MIT team showed that ultraviolet (UV) light shone on a solid electrolyte essentially causes electronic perturbations at the grain boundaries that ultimately lower the barrier that ions encounter at those boundaries. The result: “We were able to enhance the flow of the ions by a factor of three,” says Tuller, making for a much more efficient system.
Vast potential
At the time, the team was excited about the potential of applying what they’d found to different systems. In the 2022 work, the team used UV light, which is quickly absorbed very near the surface of a material. As a result, that specific technique is only effective in thin films of materials. (Fortunately, many applications of solid electrolytes involve thin films.)
Light can be thought of as particles — photons — with different wavelengths and energies. These range from very low-energy radio waves to the very high-energy gamma rays emitted by the radioactive decay of nuclear materials. Visible light — and UV light — are of intermediate energies, and fit between the two extremes.
The MIT technique reported in 2022 worked with UV light. Would it work with other wavelengths of light, potentially opening up new applications? Yes, the team found. In the current paper they show that gamma rays also modify the grain boundaries resulting in a faster flow of ions that, in turn, can be easily detected. And because the high-energy gamma rays penetrate much more deeply than UV light, “this extends the work to inexpensive bulk ceramics in addition to thin films,” says Tuller. It also allows a new application: an alternative approach to detecting nuclear materials.
Today’s state-of-the-art radiation detectors depend on a completely different mechanism than the one identified in the MIT work. They rely on signals derived from electrons and their counterparts, holes, rather than ions. But these electronic charge carriers must move comparatively great distances to the electrodes that “capture” them to create a signal. And along the way, they can be easily lost as they, for example, hit imperfections in a material. That’s why today’s detectors are made with extremely pure single crystals of material that allow an unimpeded path. They can be made with only certain materials and are difficult to process, making them expensive and hard to scale into large devices.
Using imperfections
In contrast, the new technique works because of the imperfections — grains — in the material. “The difference is that we rely on ionic currents being modulated at grain boundaries versus the state-of-the-art that relies on collecting electronic carriers from long distances,” Defferriere says.
Says Rupp, “It is remarkable that the bulk ‘grains’ of the ceramic materials tested revealed high stabilities of the chemistry and structure towards gamma rays, and solely the grain boundary regions reacted in charge redistribution of majority and minority carriers and defects.”
Comments Li, “This radiation-ionic effect is distinct from the conventional mechanisms for radiation detection where electrons or photons are collected. Here, the ionic current is being collected.”
Igor Lubomirsky, a professor in the Department of Materials and Interfaces at the Weizmann Institute of Science, Israel, who was not involved in the current work, says, “I found the approach followed by the MIT group in utilizing polycrystalline oxygen ion conductors very fruitful given the [materials’] promise for providing reliable operation under irradiation under the harsh conditions expected in nuclear reactors where such detectors often suffer from fatigue and aging. [They also] benefit from much-reduced fabrication costs.”
As a result, the MIT engineers are hopeful that their work could result in new, less expensive detectors. For example, they envision trucks loaded with cargo from container ships driving through a structure that has detectors on both sides as they leave a port. “Ideally, you’d have either an array of detectors or a very large detector, and that’s where [today’s detectors] really don’t scale very well,” Tuller says.
Another potential application involves accessing geothermal energy, or the extreme heat below our feet that is being explored as a carbon-free alternative to fossil fuels. Ceramic sensors at the ends of drill bits could detect pockets of heat — radiation — to drill toward. Ceramics can easily withstand extreme temperatures of more than 800 degrees Fahrenheit and the extreme pressures found deep below the Earth’s surface.
The team is excited about additional applications for their work. “This was a demonstration of principle with just one material,” says Tuller, “but there are thousands of other materials good at conducting ions.”
Concludes Defferriere: “It’s the start of a journey on the development of the technology, so there’s a lot to do and a lot to discover.”
This work is currently supported by the U.S. Department of Homeland Security, Countering Weapons of Mass Destruction Office. This support does not constitute an express or implied endorsement on the part of the government. It was also funded by the U.S. Defense Threat Reduction Agency.
Share this news article on:
Related links.
- Harry Tuller
- Tuller Research Group
- Materials Research Laboratory
Related Topics
- Nuclear security and policy
- Materials science and engineering
- Nuclear science and engineering
- Department of Energy (DoE)
Related Articles
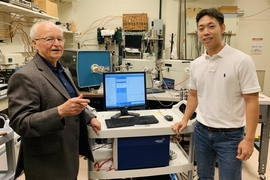
A simple way to significantly increase lifetimes of fuel cells and other devices
Harry Tuller honored for career advancing solid-state chemistry and electrochemistry
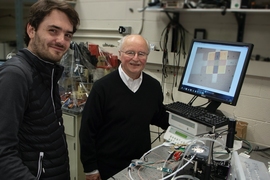
Light could boost performance of fuel cells, lithium batteries, and other devices
Previous item Next item
More MIT News
A biomedical engineer pivots from human movement to women’s health
Read full story →
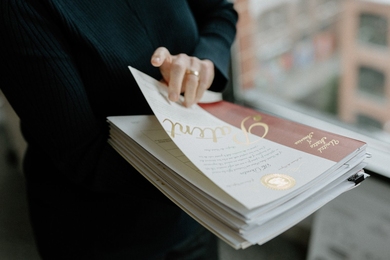
MIT tops among single-campus universities in US patents granted
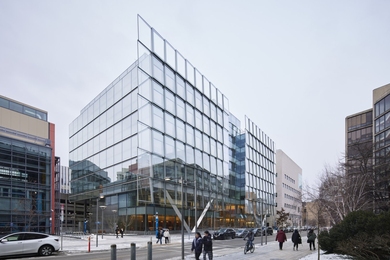
A crossroads for computing at MIT
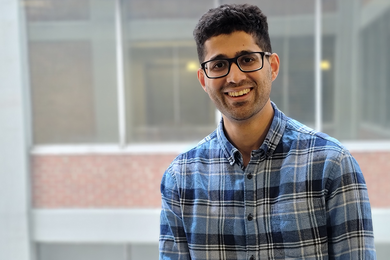
Growing our donated organ supply
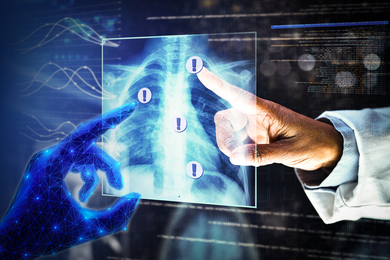
New AI method captures uncertainty in medical images
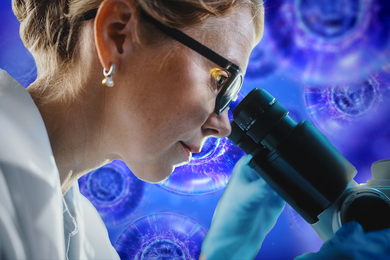
Improving drug development with a vast map of the immune system
- More news on MIT News homepage →
Massachusetts Institute of Technology 77 Massachusetts Avenue, Cambridge, MA, USA
- Map (opens in new window)
- Events (opens in new window)
- People (opens in new window)
- Careers (opens in new window)
- Accessibility
- Social Media Hub
- MIT on Facebook
- MIT on YouTube
- MIT on Instagram

Parkinson's Disease: New theory on the disease's origins and spread
The nose or the gut? For the past two decades, the scientific community has debated the wellspring of the toxic proteins at the source of Parkinson's disease. In 2003, a German pathologist, Heiko Braak, MD, first proposed that the disease begins outside the brain. More recently, Per Borghammer, MD, with Aarhus University Hospital in Denmark, and his colleagues argue that the disease is the result of processes that start in either the brain's smell center (brain-first) or the body's intestinal tract (body-first).
A new hypothesis paper appearing in the Journal of Parkinson's Disease on World Parkinson's Day unites the brain- and body-first models with some of the likely causes of the disease-environmental toxicants that are either inhaled or ingested. The authors of the new study, who include Borghammer, argue that inhalation of certain pesticides, common dry cleaning chemicals, and air pollution predispose to a brain-first model of the disease. Other ingested toxicants, such as tainted food and contaminated drinking water, lead to body-first model of the disease.
"In both the brain-first and body-first scenarios the pathology arises in structures in the body closely connected to the outside world," said Ray Dorsey, MD, a professor of Neurology at the University of Rochester Medical Center and co-author of the piece. "Here we propose that Parkinson's is a systemic disease and that its initial roots likely begin in the nose and in the gut and are tied to environmental factors increasingly recognized as major contributors, if not causes, of the disease. This further reinforces the idea that Parkinson's, the world's fastest growing brain disease, may be fueled by toxicants and is therefore largely preventable."
Different pathways to the brain, different forms of disease
A misfolded protein called alpha-synuclein has been in scientists' sights for the last 25 years as one of the driving forces behind Parkinson's. Over time, the protein accumulates in the brain in clumps, called Lewy bodies, and causes progressive dysfunction and death of many types of nerve cells, including those in the dopamine-producing regions of the brain that control motor function. When first proposed, Braak thought that an unidentified pathogen, such as a virus, may be responsible for the disease.
The new piece argues that toxins encountered in the environment, specifically the dry cleaning and degreasing chemicals trichloroethylene (TCE) and perchloroethylene (PCE), the weed killer paraquat, and air pollution, could be common causes for the formation of toxic alpha-synuclein. TCE and PCE contaminates thousands of former industrial, commercial, and military sites, most notably the Marine Corps base Camp Lejeune, and paraquat is one of the most widely used herbicides in the US, despite being banned for safety concerns in more than 30 countries, including the European Union and China. Air pollution was at toxic levels in nineteenth century London when James Parkinson, whose 269th birthday is celebrated today, first described the condition.
The nose and the gut are lined with a soft permeable tissue, and both have well established connections to the brain. In the brain-first model, the chemicals are inhaled and may enter the brain via the nerve responsible for smell. From the brain's smell center, alpha-synuclein spreads to other parts of the brain principally on one side, including regions with concentrations of dopamine-producing neurons. The death of these cells is a hallmark of Parkinson's disease. The disease may cause asymmetric tremor and slowness in movement and, a slower rate of progression after diagnosis, and only much later, significant cognitive impairment or dementia.
When ingested, the chemicals pass through the lining of the gastrointestinal tract. Initial alpha-synuclein pathology may begin in the gut's own nervous system from where it can spread to both sides of the brain and spinal cord. This body-first pathway is often associated with Lewy body dementia, a disease in the same family as Parkinson's, which is characterized by early constipation and sleep disturbance, followed by more symmetric slowing in movements and earlier dementia, as the disease spreads through both brain hemispheres.
New models to understand and study brain diseases
"These environmental toxicants are widespread and not everyone has Parkinson's disease," said Dorsey. "The timing, dose, and duration of exposure and interactions with genetic and other environmental factors are probably key to determining who ultimately develops Parkinson's. In most instances, these exposures likely occurred years or decades before symptoms develop."
Pointing to a growing body of research linking environmental exposure to Parkinson's disease, the authors believe the new models may enable the scientific community to connect specific exposures to specific forms of the disease. This effort will be aided by increasing public awareness of the adverse health effects of many chemicals in our environment. The authors conclude that their hypothesis "may explain many of the mysteries of Parkinson's disease and open the door toward the ultimate goal-prevention."
In addition to Parkinson's, these models of environmental exposure may advance understanding of how toxicants contribute to other brain disorders, including autism in children, ALS in adults, and Alzheimer's in seniors. Dorsey and his colleagues at the University of Rochester have organized a symposium on the Brain and the Environment in Washington, DC, on May 20 that will examine the role toxicants in our food, water, and air are playing in all these brain diseases.
Additional authors of the hypothesis paper include Briana De Miranda, PhD, with the University of Alabama at Birmingham, and Jacob Horsager, MD, PhD, with Aarhus University Hospital in Denmark.
- Parkinson's Research
- Chronic Illness
- Brain Tumor
- Diseases and Conditions
- Parkinson's
- Disorders and Syndromes
- Brain-Computer Interfaces
- Parkinson's disease
- Deep brain stimulation
- Homosexuality
- Dopamine hypothesis of schizophrenia
- Excitotoxicity and cell damage
Story Source:
Materials provided by University of Rochester Medical Center . Original written by Mark Michaud. Note: Content may be edited for style and length.
Journal Reference :
- E. Ray Dorsey, Briana R. De Miranda, Jacob Horsager, Per Borghammer. The Body, the Brain, the Environment, and Parkinson’s Disease . Journal of Parkinson's Disease , 2024; 1 DOI: 10.3233/JPD-240019
Cite This Page :
Explore More
- Genes for Strong Muscles: Healthy Long Life
- Brightest Gamma-Ray Burst
- Stellar Winds of Three Sun-Like Stars Detected
- Fences Causing Genetic Problems for Mammals
- Ozone Removes Mating Barriers Between Fly ...
- Parkinson's: New Theory On Origins and Spread
- Clash of Stars Solves Stellar Mystery
- Secure Quantum Computing at Home
- Ocean Currents: Collapse of Antarctic Ice ...
- Pacific Cities Much Older Than Previously ...
Trending Topics
Strange & offbeat.
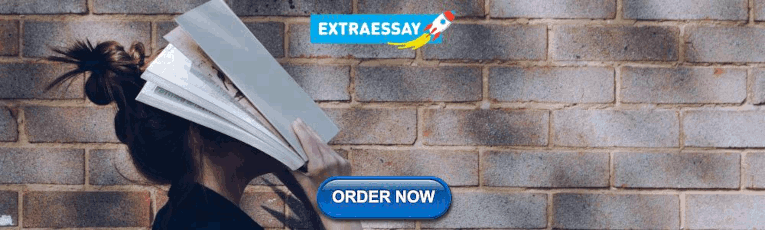
IMAGES
VIDEO
COMMENTS
204 Research Topics on Technology & Computer Science. A List of 580 Interesting Research Topics [2024 Edition] A List of 179 Problem Solution Essay Topics & Questions. 193 Interesting Proposal Essay Topics and Ideas. 226 Research Topics on Criminal Justice & Criminology.
In 2024, these will be the top cybersecurity trends. A) Exciting Mobile Cyber Security Research Paper Topics. The significance of continuous user authentication on mobile gadgets. The efficacy of different mobile security approaches. Detecting mobile phone hacking.
About the journal. Journal of Cybersecurity publishes accessible articles describing original research in the inherently interdisciplinary world of computer, systems, and information security …. Find out more. DoWNet—classification of Denial-of-Wallet attacks on serverless application traffic. The barriers to sustainable risk transfer in ...
The methodology adopted in this paper is a review of papers with keywords network security, network attacks and threats and network security measures. The aim of this paper is to critically review ...
A comprehensive list of cybersecurity-related research topics. Includes 100% free access to a webinar and research topic evaluator. ... These are actual studies, so they can provide some useful insight as to what a research topic looks like in practice. Cyber Security Vulnerability Detection Using Natural Language Processing (Singh et al., 2022 ...
Explore the latest full-text research PDFs, articles, conference papers, preprints and more on COMPUTER SECURITY. Find methods information, sources, references or conduct a literature review on ...
The article is a full research paper (i.e., not a presentation or supplement to a poster). • The article should make it apparent that AI is its primary emphasis or include AI as a large part of the methodology. For example, publications that explicitly include machine learning as a core component of their methodology/research. •
Abstract. Although using machine learning techniques to solve computer security challenges is not a new idea, the rapidly emerging Deep Learning technology has recently triggered a substantial amount of interests in the computer security community. This paper seeks to provide a dedicated review of the very recent research works on using Deep ...
Depending on the amount of data, the extent of the damage caused by a data breach can be significant, with the average cost being USD 392 million Footnote 1 (IBM Security 2020). This research paper reviews the existing literature and open data sources related to cybersecurity and cyber risk, focusing on the datasets used to improve academic ...
In a computing context, cybersecurity is undergoing massive shifts in technology and its operations in recent days, and data science is driving the change. Extracting security incident patterns or insights from cybersecurity data and building corresponding data-driven model, is the key to make a security system automated and intelligent. To understand and analyze the actual phenomena with data ...
The security of any organization begins with three principles: confidentiality, integrity, and availability. These three principles are referred to as the security triangle, or CIA, which has served as the standard for systems security since the first computer systems (see Fig. 6) (Palmieri et al., 2021). The principle of confidentiality states ...
Cybersecurity is a significant concern for businesses worldwide, as cybercriminals target business data and system resources. Cyber threat intelligence (CTI) enhances organizational cybersecurity resilience by obtaining, processing, evaluating, and disseminating information about potential risks and opportunities inside the cyber domain. This research investigates how companies can employ CTI ...
2021, for the terms Cyber Security, Computer Security, and Information Security. The y- axis depicts the relative search frequency for the term. A value of 100 is the peak popularity for the term. A value of 50 means that the term is half as popular. ... there is a need to expand research and develop novel cybersecurity methods and tools to
Cyber Security Research Topics. Cyber Security Research Topics are as follows: The role of machine learning in detecting cyber threats. The impact of cloud computing on cyber security. Cyber warfare and its effects on national security. The rise of ransomware attacks and their prevention methods.
Wireless Network Security . Wireless Router . Network Security System. The use of computer networks in an agency aims to facilitate communication and data transfer between devices. The network that can be applied can be using wireless media or LAN cable. At SMP XYZ, most of the computers still use wireless networks.
Machine learning and AI are research topics in cybersecurity, aiming to develop algorithms for threat detection, enhance intelligence and automate risk mitigation. However, security risks like adversarial attacks require attention. Using AI/ML to Analyse Cyber Threats - This cyber security research paper analyses cyber threats and could ...
The cyber security of a company can be compromised in many ways when it comes to software and computer administration. As such, software and computer administration is a great sources of cybersecurity research paper topics. Here are some of the best topics in this category. Evaluation of the operation of antimalware in preventing cyber attacks.
154 Exceptional Cybersecurity Research Topics For You. If you are studying computer science or IT-related course, you will encounter such a task. It is one of the most technical assignments, primarily in the era of advanced digital technologies. Students may not have the muscles to complete such papers on their own.
Topics in Computer and Network Security Stanford CS 356, Fall 2023. CS 356 is graduate course that covers foundational work and current topics in computer and network security. The course consists of reading and discussing published research papers, presenting recent security work, and completing an original research project.
Computer Security Resource Center. Projects; Publications Expand or Collapse Topics ... Topics Select a term to learn more about it, and to see CSRC Projects, Publications, News, Events and Presentations on that topic. ... Federal Cybersecurity Research and Development Strategic Plan;
Anything in computer science, including cyber security, is interesting, and so is it for our writers. Here's the first list of cyber security research paper topics for computer science students. Languages for domain-specific modeling in cyber security. To interpret the behavior of a specific hacker based on specific concerns.
P.O. Box 5969, Safat 13060, Kuwait University, Kuwait. Abstract. The world has become more advanced in communication, espec ially after the invention of. the Internet. A key issue facing today's ...
The range of research paper topics in security management reflects this diversity and offers a wealth of opportunities for students to engage with cutting-edge issues. The ongoing development of this field requires fresh insights, innovative thinking, and a commitment to understanding the underlying principles that govern security management. ...
In the current paper they show that gamma rays also modify the grain boundaries resulting in a faster flow of ions that, in turn, can be easily detected. And because the high-energy gamma rays penetrate much more deeply than UV light, "this extends the work to inexpensive bulk ceramics in addition to thin films," says Tuller.
A new hypothesis paper appearing in the Journal of Parkinson's Disease on World Parkinson's Day unites the brain- and body-first models with some of the likely causes of the disease-environmental ...