Writing an Abstract for Your Research Paper
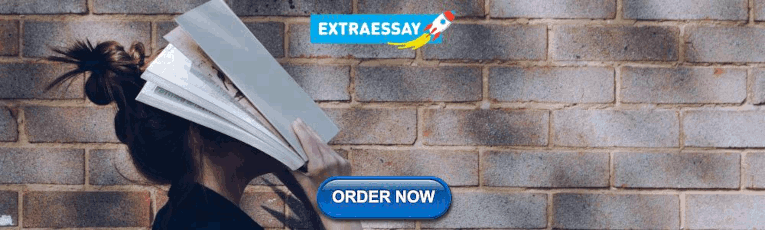
Definition and Purpose of Abstracts
An abstract is a short summary of your (published or unpublished) research paper, usually about a paragraph (c. 6-7 sentences, 150-250 words) long. A well-written abstract serves multiple purposes:
- an abstract lets readers get the gist or essence of your paper or article quickly, in order to decide whether to read the full paper;
- an abstract prepares readers to follow the detailed information, analyses, and arguments in your full paper;
- and, later, an abstract helps readers remember key points from your paper.
It’s also worth remembering that search engines and bibliographic databases use abstracts, as well as the title, to identify key terms for indexing your published paper. So what you include in your abstract and in your title are crucial for helping other researchers find your paper or article.
If you are writing an abstract for a course paper, your professor may give you specific guidelines for what to include and how to organize your abstract. Similarly, academic journals often have specific requirements for abstracts. So in addition to following the advice on this page, you should be sure to look for and follow any guidelines from the course or journal you’re writing for.
The Contents of an Abstract
Abstracts contain most of the following kinds of information in brief form. The body of your paper will, of course, develop and explain these ideas much more fully. As you will see in the samples below, the proportion of your abstract that you devote to each kind of information—and the sequence of that information—will vary, depending on the nature and genre of the paper that you are summarizing in your abstract. And in some cases, some of this information is implied, rather than stated explicitly. The Publication Manual of the American Psychological Association , which is widely used in the social sciences, gives specific guidelines for what to include in the abstract for different kinds of papers—for empirical studies, literature reviews or meta-analyses, theoretical papers, methodological papers, and case studies.
Here are the typical kinds of information found in most abstracts:
- the context or background information for your research; the general topic under study; the specific topic of your research
- the central questions or statement of the problem your research addresses
- what’s already known about this question, what previous research has done or shown
- the main reason(s) , the exigency, the rationale , the goals for your research—Why is it important to address these questions? Are you, for example, examining a new topic? Why is that topic worth examining? Are you filling a gap in previous research? Applying new methods to take a fresh look at existing ideas or data? Resolving a dispute within the literature in your field? . . .
- your research and/or analytical methods
- your main findings , results , or arguments
- the significance or implications of your findings or arguments.
Your abstract should be intelligible on its own, without a reader’s having to read your entire paper. And in an abstract, you usually do not cite references—most of your abstract will describe what you have studied in your research and what you have found and what you argue in your paper. In the body of your paper, you will cite the specific literature that informs your research.
When to Write Your Abstract
Although you might be tempted to write your abstract first because it will appear as the very first part of your paper, it’s a good idea to wait to write your abstract until after you’ve drafted your full paper, so that you know what you’re summarizing.
What follows are some sample abstracts in published papers or articles, all written by faculty at UW-Madison who come from a variety of disciplines. We have annotated these samples to help you see the work that these authors are doing within their abstracts.
Choosing Verb Tenses within Your Abstract
The social science sample (Sample 1) below uses the present tense to describe general facts and interpretations that have been and are currently true, including the prevailing explanation for the social phenomenon under study. That abstract also uses the present tense to describe the methods, the findings, the arguments, and the implications of the findings from their new research study. The authors use the past tense to describe previous research.
The humanities sample (Sample 2) below uses the past tense to describe completed events in the past (the texts created in the pulp fiction industry in the 1970s and 80s) and uses the present tense to describe what is happening in those texts, to explain the significance or meaning of those texts, and to describe the arguments presented in the article.
The science samples (Samples 3 and 4) below use the past tense to describe what previous research studies have done and the research the authors have conducted, the methods they have followed, and what they have found. In their rationale or justification for their research (what remains to be done), they use the present tense. They also use the present tense to introduce their study (in Sample 3, “Here we report . . .”) and to explain the significance of their study (In Sample 3, This reprogramming . . . “provides a scalable cell source for. . .”).
Sample Abstract 1
From the social sciences.
Reporting new findings about the reasons for increasing economic homogamy among spouses
Gonalons-Pons, Pilar, and Christine R. Schwartz. “Trends in Economic Homogamy: Changes in Assortative Mating or the Division of Labor in Marriage?” Demography , vol. 54, no. 3, 2017, pp. 985-1005.
![research in progress abstract example “The growing economic resemblance of spouses has contributed to rising inequality by increasing the number of couples in which there are two high- or two low-earning partners. [Annotation for the previous sentence: The first sentence introduces the topic under study (the “economic resemblance of spouses”). This sentence also implies the question underlying this research study: what are the various causes—and the interrelationships among them—for this trend?] The dominant explanation for this trend is increased assortative mating. Previous research has primarily relied on cross-sectional data and thus has been unable to disentangle changes in assortative mating from changes in the division of spouses’ paid labor—a potentially key mechanism given the dramatic rise in wives’ labor supply. [Annotation for the previous two sentences: These next two sentences explain what previous research has demonstrated. By pointing out the limitations in the methods that were used in previous studies, they also provide a rationale for new research.] We use data from the Panel Study of Income Dynamics (PSID) to decompose the increase in the correlation between spouses’ earnings and its contribution to inequality between 1970 and 2013 into parts due to (a) changes in assortative mating, and (b) changes in the division of paid labor. [Annotation for the previous sentence: The data, research and analytical methods used in this new study.] Contrary to what has often been assumed, the rise of economic homogamy and its contribution to inequality is largely attributable to changes in the division of paid labor rather than changes in sorting on earnings or earnings potential. Our findings indicate that the rise of economic homogamy cannot be explained by hypotheses centered on meeting and matching opportunities, and they show where in this process inequality is generated and where it is not.” (p. 985) [Annotation for the previous two sentences: The major findings from and implications and significance of this study.]](https://writing.wisc.edu/wp-content/uploads/sites/535/2019/08/Abstract-1.png)
Sample Abstract 2
From the humanities.
Analyzing underground pulp fiction publications in Tanzania, this article makes an argument about the cultural significance of those publications
Emily Callaci. “Street Textuality: Socialism, Masculinity, and Urban Belonging in Tanzania’s Pulp Fiction Publishing Industry, 1975-1985.” Comparative Studies in Society and History , vol. 59, no. 1, 2017, pp. 183-210.
![research in progress abstract example “From the mid-1970s through the mid-1980s, a network of young urban migrant men created an underground pulp fiction publishing industry in the city of Dar es Salaam. [Annotation for the previous sentence: The first sentence introduces the context for this research and announces the topic under study.] As texts that were produced in the underground economy of a city whose trajectory was increasingly charted outside of formalized planning and investment, these novellas reveal more than their narrative content alone. These texts were active components in the urban social worlds of the young men who produced them. They reveal a mode of urbanism otherwise obscured by narratives of decolonization, in which urban belonging was constituted less by national citizenship than by the construction of social networks, economic connections, and the crafting of reputations. This article argues that pulp fiction novellas of socialist era Dar es Salaam are artifacts of emergent forms of male sociability and mobility. In printing fictional stories about urban life on pilfered paper and ink, and distributing their texts through informal channels, these writers not only described urban communities, reputations, and networks, but also actually created them.” (p. 210) [Annotation for the previous sentences: The remaining sentences in this abstract interweave other essential information for an abstract for this article. The implied research questions: What do these texts mean? What is their historical and cultural significance, produced at this time, in this location, by these authors? The argument and the significance of this analysis in microcosm: these texts “reveal a mode or urbanism otherwise obscured . . .”; and “This article argues that pulp fiction novellas. . . .” This section also implies what previous historical research has obscured. And through the details in its argumentative claims, this section of the abstract implies the kinds of methods the author has used to interpret the novellas and the concepts under study (e.g., male sociability and mobility, urban communities, reputations, network. . . ).]](https://writing.wisc.edu/wp-content/uploads/sites/535/2019/08/Abstract-2.png)
Sample Abstract/Summary 3
From the sciences.
Reporting a new method for reprogramming adult mouse fibroblasts into induced cardiac progenitor cells
Lalit, Pratik A., Max R. Salick, Daryl O. Nelson, Jayne M. Squirrell, Christina M. Shafer, Neel G. Patel, Imaan Saeed, Eric G. Schmuck, Yogananda S. Markandeya, Rachel Wong, Martin R. Lea, Kevin W. Eliceiri, Timothy A. Hacker, Wendy C. Crone, Michael Kyba, Daniel J. Garry, Ron Stewart, James A. Thomson, Karen M. Downs, Gary E. Lyons, and Timothy J. Kamp. “Lineage Reprogramming of Fibroblasts into Proliferative Induced Cardiac Progenitor Cells by Defined Factors.” Cell Stem Cell , vol. 18, 2016, pp. 354-367.
![research in progress abstract example “Several studies have reported reprogramming of fibroblasts into induced cardiomyocytes; however, reprogramming into proliferative induced cardiac progenitor cells (iCPCs) remains to be accomplished. [Annotation for the previous sentence: The first sentence announces the topic under study, summarizes what’s already known or been accomplished in previous research, and signals the rationale and goals are for the new research and the problem that the new research solves: How can researchers reprogram fibroblasts into iCPCs?] Here we report that a combination of 11 or 5 cardiac factors along with canonical Wnt and JAK/STAT signaling reprogrammed adult mouse cardiac, lung, and tail tip fibroblasts into iCPCs. The iCPCs were cardiac mesoderm-restricted progenitors that could be expanded extensively while maintaining multipo-tency to differentiate into cardiomyocytes, smooth muscle cells, and endothelial cells in vitro. Moreover, iCPCs injected into the cardiac crescent of mouse embryos differentiated into cardiomyocytes. iCPCs transplanted into the post-myocardial infarction mouse heart improved survival and differentiated into cardiomyocytes, smooth muscle cells, and endothelial cells. [Annotation for the previous four sentences: The methods the researchers developed to achieve their goal and a description of the results.] Lineage reprogramming of adult somatic cells into iCPCs provides a scalable cell source for drug discovery, disease modeling, and cardiac regenerative therapy.” (p. 354) [Annotation for the previous sentence: The significance or implications—for drug discovery, disease modeling, and therapy—of this reprogramming of adult somatic cells into iCPCs.]](https://writing.wisc.edu/wp-content/uploads/sites/535/2019/08/Abstract-3.png)
Sample Abstract 4, a Structured Abstract
Reporting results about the effectiveness of antibiotic therapy in managing acute bacterial sinusitis, from a rigorously controlled study
Note: This journal requires authors to organize their abstract into four specific sections, with strict word limits. Because the headings for this structured abstract are self-explanatory, we have chosen not to add annotations to this sample abstract.
Wald, Ellen R., David Nash, and Jens Eickhoff. “Effectiveness of Amoxicillin/Clavulanate Potassium in the Treatment of Acute Bacterial Sinusitis in Children.” Pediatrics , vol. 124, no. 1, 2009, pp. 9-15.
“OBJECTIVE: The role of antibiotic therapy in managing acute bacterial sinusitis (ABS) in children is controversial. The purpose of this study was to determine the effectiveness of high-dose amoxicillin/potassium clavulanate in the treatment of children diagnosed with ABS.
METHODS : This was a randomized, double-blind, placebo-controlled study. Children 1 to 10 years of age with a clinical presentation compatible with ABS were eligible for participation. Patients were stratified according to age (<6 or ≥6 years) and clinical severity and randomly assigned to receive either amoxicillin (90 mg/kg) with potassium clavulanate (6.4 mg/kg) or placebo. A symptom survey was performed on days 0, 1, 2, 3, 5, 7, 10, 20, and 30. Patients were examined on day 14. Children’s conditions were rated as cured, improved, or failed according to scoring rules.
RESULTS: Two thousand one hundred thirty-five children with respiratory complaints were screened for enrollment; 139 (6.5%) had ABS. Fifty-eight patients were enrolled, and 56 were randomly assigned. The mean age was 6630 months. Fifty (89%) patients presented with persistent symptoms, and 6 (11%) presented with nonpersistent symptoms. In 24 (43%) children, the illness was classified as mild, whereas in the remaining 32 (57%) children it was severe. Of the 28 children who received the antibiotic, 14 (50%) were cured, 4 (14%) were improved, 4(14%) experienced treatment failure, and 6 (21%) withdrew. Of the 28children who received placebo, 4 (14%) were cured, 5 (18%) improved, and 19 (68%) experienced treatment failure. Children receiving the antibiotic were more likely to be cured (50% vs 14%) and less likely to have treatment failure (14% vs 68%) than children receiving the placebo.
CONCLUSIONS : ABS is a common complication of viral upper respiratory infections. Amoxicillin/potassium clavulanate results in significantly more cures and fewer failures than placebo, according to parental report of time to resolution.” (9)
Some Excellent Advice about Writing Abstracts for Basic Science Research Papers, by Professor Adriano Aguzzi from the Institute of Neuropathology at the University of Zurich:

Academic and Professional Writing
This is an accordion element with a series of buttons that open and close related content panels.
Analysis Papers
Reading Poetry
A Short Guide to Close Reading for Literary Analysis
Using Literary Quotations
Play Reviews
Writing a Rhetorical Précis to Analyze Nonfiction Texts
Incorporating Interview Data
Grant Proposals
Planning and Writing a Grant Proposal: The Basics
Additional Resources for Grants and Proposal Writing
Job Materials and Application Essays
Writing Personal Statements for Ph.D. Programs
- Before you begin: useful tips for writing your essay
- Guided brainstorming exercises
- Get more help with your essay
- Frequently Asked Questions
Resume Writing Tips
CV Writing Tips
Cover Letters
Business Letters
Proposals and Dissertations
Resources for Proposal Writers
Resources for Dissertators
Research Papers
Planning and Writing Research Papers
Quoting and Paraphrasing
Writing Annotated Bibliographies
Creating Poster Presentations
Thank-You Notes
Advice for Students Writing Thank-You Notes to Donors
Reading for a Review
Critical Reviews
Writing a Review of Literature
Scientific Reports
Scientific Report Format
Sample Lab Assignment
Writing for the Web
Writing an Effective Blog Post
Writing for Social Media: A Guide for Academics
- Resources Home 🏠
- Try SciSpace Copilot
- Search research papers
- Add Copilot Extension
- Try AI Detector
- Try Paraphraser
- Try Citation Generator
- April Papers
- June Papers
- July Papers

Abstract Writing: A Step-by-Step Guide With Tips & Examples
Table of Contents
Introduction
Abstracts of research papers have always played an essential role in describing your research concisely and clearly to researchers and editors of journals, enticing them to continue reading. However, with the widespread availability of scientific databases, the need to write a convincing abstract is more crucial now than during the time of paper-bound manuscripts.
Abstracts serve to "sell" your research and can be compared with your "executive outline" of a resume or, rather, a formal summary of the critical aspects of your work. Also, it can be the "gist" of your study. Since most educational research is done online, it's a sign that you have a shorter time for impressing your readers, and have more competition from other abstracts that are available to be read.
The APCI (Academic Publishing and Conferences International) articulates 12 issues or points considered during the final approval process for conferences & journals and emphasises the importance of writing an abstract that checks all these boxes (12 points). Since it's the only opportunity you have to captivate your readers, you must invest time and effort in creating an abstract that accurately reflects the critical points of your research.
With that in mind, let’s head over to understand and discover the core concept and guidelines to create a substantial abstract. Also, learn how to organise the ideas or plots into an effective abstract that will be awe-inspiring to the readers you want to reach.
What is Abstract? Definition and Overview
The word "Abstract' is derived from Latin abstractus meaning "drawn off." This etymological meaning also applies to art movements as well as music, like abstract expressionism. In this context, it refers to the revealing of the artist's intention.
Based on this, you can determine the meaning of an abstract: A condensed research summary. It must be self-contained and independent of the body of the research. However, it should outline the subject, the strategies used to study the problem, and the methods implemented to attain the outcomes. The specific elements of the study differ based on the area of study; however, together, it must be a succinct summary of the entire research paper.
Abstracts are typically written at the end of the paper, even though it serves as a prologue. In general, the abstract must be in a position to:
- Describe the paper.
- Identify the problem or the issue at hand.
- Explain to the reader the research process, the results you came up with, and what conclusion you've reached using these results.
- Include keywords to guide your strategy and the content.
Furthermore, the abstract you submit should not reflect upon any of the following elements:
- Examine, analyse or defend the paper or your opinion.
- What you want to study, achieve or discover.
- Be redundant or irrelevant.
After reading an abstract, your audience should understand the reason - what the research was about in the first place, what the study has revealed and how it can be utilised or can be used to benefit others. You can understand the importance of abstract by knowing the fact that the abstract is the most frequently read portion of any research paper. In simpler terms, it should contain all the main points of the research paper.
What is the Purpose of an Abstract?
Abstracts are typically an essential requirement for research papers; however, it's not an obligation to preserve traditional reasons without any purpose. Abstracts allow readers to scan the text to determine whether it is relevant to their research or studies. The abstract allows other researchers to decide if your research paper can provide them with some additional information. A good abstract paves the interest of the audience to pore through your entire paper to find the content or context they're searching for.
Abstract writing is essential for indexing, as well. The Digital Repository of academic papers makes use of abstracts to index the entire content of academic research papers. Like meta descriptions in the regular Google outcomes, abstracts must include keywords that help researchers locate what they seek.
Types of Abstract
Informative and Descriptive are two kinds of abstracts often used in scientific writing.
A descriptive abstract gives readers an outline of the author's main points in their study. The reader can determine if they want to stick to the research work, based on their interest in the topic. An abstract that is descriptive is similar to the contents table of books, however, the format of an abstract depicts complete sentences encapsulated in one paragraph. It is unfortunate that the abstract can't be used as a substitute for reading a piece of writing because it's just an overview, which omits readers from getting an entire view. Also, it cannot be a way to fill in the gaps the reader may have after reading this kind of abstract since it does not contain crucial information needed to evaluate the article.
To conclude, a descriptive abstract is:
- A simple summary of the task, just summarises the work, but some researchers think it is much more of an outline
- Typically, the length is approximately 100 words. It is too short when compared to an informative abstract.
- A brief explanation but doesn't provide the reader with the complete information they need;
- An overview that omits conclusions and results
An informative abstract is a comprehensive outline of the research. There are times when people rely on the abstract as an information source. And the reason is why it is crucial to provide entire data of particular research. A well-written, informative abstract could be a good substitute for the remainder of the paper on its own.
A well-written abstract typically follows a particular style. The author begins by providing the identifying information, backed by citations and other identifiers of the papers. Then, the major elements are summarised to make the reader aware of the study. It is followed by the methodology and all-important findings from the study. The conclusion then presents study results and ends the abstract with a comprehensive summary.
In a nutshell, an informative abstract:
- Has a length that can vary, based on the subject, but is not longer than 300 words.
- Contains all the content-like methods and intentions
- Offers evidence and possible recommendations.
Informative Abstracts are more frequent than descriptive abstracts because of their extensive content and linkage to the topic specifically. You should select different types of abstracts to papers based on their length: informative abstracts for extended and more complex abstracts and descriptive ones for simpler and shorter research papers.
What are the Characteristics of a Good Abstract?
- A good abstract clearly defines the goals and purposes of the study.
- It should clearly describe the research methodology with a primary focus on data gathering, processing, and subsequent analysis.
- A good abstract should provide specific research findings.
- It presents the principal conclusions of the systematic study.
- It should be concise, clear, and relevant to the field of study.
- A well-designed abstract should be unifying and coherent.
- It is easy to grasp and free of technical jargon.
- It is written impartially and objectively.
What are the various sections of an ideal Abstract?
By now, you must have gained some concrete idea of the essential elements that your abstract needs to convey . Accordingly, the information is broken down into six key sections of the abstract, which include:
An Introduction or Background
Research methodology, objectives and goals, limitations.
Let's go over them in detail.
The introduction, also known as background, is the most concise part of your abstract. Ideally, it comprises a couple of sentences. Some researchers only write one sentence to introduce their abstract. The idea behind this is to guide readers through the key factors that led to your study.
It's understandable that this information might seem difficult to explain in a couple of sentences. For example, think about the following two questions like the background of your study:
- What is currently available about the subject with respect to the paper being discussed?
- What isn't understood about this issue? (This is the subject of your research)
While writing the abstract’s introduction, make sure that it is not lengthy. Because if it crosses the word limit, it may eat up the words meant to be used for providing other key information.
Research methodology is where you describe the theories and techniques you used in your research. It is recommended that you describe what you have done and the method you used to get your thorough investigation results. Certainly, it is the second-longest paragraph in the abstract.
In the research methodology section, it is essential to mention the kind of research you conducted; for instance, qualitative research or quantitative research (this will guide your research methodology too) . If you've conducted quantitative research, your abstract should contain information like the sample size, data collection method, sampling techniques, and duration of the study. Likewise, your abstract should reflect observational data, opinions, questionnaires (especially the non-numerical data) if you work on qualitative research.
The research objectives and goals speak about what you intend to accomplish with your research. The majority of research projects focus on the long-term effects of a project, and the goals focus on the immediate, short-term outcomes of the research. It is possible to summarise both in just multiple sentences.
In stating your objectives and goals, you give readers a picture of the scope of the study, its depth and the direction your research ultimately follows. Your readers can evaluate the results of your research against the goals and stated objectives to determine if you have achieved the goal of your research.
In the end, your readers are more attracted by the results you've obtained through your study. Therefore, you must take the time to explain each relevant result and explain how they impact your research. The results section exists as the longest in your abstract, and nothing should diminish its reach or quality.
One of the most important things you should adhere to is to spell out details and figures on the results of your research.
Instead of making a vague assertion such as, "We noticed that response rates varied greatly between respondents with high incomes and those with low incomes", Try these: "The response rate was higher for high-income respondents than those with lower incomes (59 30 percent vs. 30 percent in both cases; P<0.01)."
You're likely to encounter certain obstacles during your research. It could have been during data collection or even during conducting the sample . Whatever the issue, it's essential to inform your readers about them and their effects on the research.
Research limitations offer an opportunity to suggest further and deep research. If, for instance, you were forced to change for convenient sampling and snowball samples because of difficulties in reaching well-suited research participants, then you should mention this reason when you write your research abstract. In addition, a lack of prior studies on the subject could hinder your research.
Your conclusion should include the same number of sentences to wrap the abstract as the introduction. The majority of researchers offer an idea of the consequences of their research in this case.
Your conclusion should include three essential components:
- A significant take-home message.
- Corresponding important findings.
- The Interpretation.
Even though the conclusion of your abstract needs to be brief, it can have an enormous influence on the way that readers view your research. Therefore, make use of this section to reinforce the central message from your research. Be sure that your statements reflect the actual results and the methods you used to conduct your research.
Good Abstract Examples
Abstract example #1.
Children’s consumption behavior in response to food product placements in movies.
The abstract:
"Almost all research into the effects of brand placements on children has focused on the brand's attitudes or behavior intentions. Based on the significant differences between attitudes and behavioral intentions on one hand and actual behavior on the other hand, this study examines the impact of placements by brands on children's eating habits. Children aged 6-14 years old were shown an excerpt from the popular film Alvin and the Chipmunks and were shown places for the item Cheese Balls. Three different versions were developed with no placements, one with moderately frequent placements and the third with the highest frequency of placement. The results revealed that exposure to high-frequency places had a profound effect on snack consumption, however, there was no impact on consumer attitudes towards brands or products. The effects were not dependent on the age of the children. These findings are of major importance to researchers studying consumer behavior as well as nutrition experts as well as policy regulators."
Abstract Example #2
Social comparisons on social media: The impact of Facebook on young women’s body image concerns and mood. The abstract:
"The research conducted in this study investigated the effects of Facebook use on women's moods and body image if the effects are different from an internet-based fashion journal and if the appearance comparison tendencies moderate one or more of these effects. Participants who were female ( N = 112) were randomly allocated to spend 10 minutes exploring their Facebook account or a magazine's website or an appearance neutral control website prior to completing state assessments of body dissatisfaction, mood, and differences in appearance (weight-related and facial hair, face, and skin). Participants also completed a test of the tendency to compare appearances. The participants who used Facebook were reported to be more depressed than those who stayed on the control site. In addition, women who have the tendency to compare appearances reported more facial, hair and skin-related issues following Facebook exposure than when they were exposed to the control site. Due to its popularity it is imperative to conduct more research to understand the effect that Facebook affects the way people view themselves."
Abstract Example #3
The Relationship Between Cell Phone Use and Academic Performance in a Sample of U.S. College Students
"The cellphone is always present on campuses of colleges and is often utilised in situations in which learning takes place. The study examined the connection between the use of cell phones and the actual grades point average (GPA) after adjusting for predictors that are known to be a factor. In the end 536 students in the undergraduate program from 82 self-reported majors of an enormous, public institution were studied. Hierarchical analysis ( R 2 = .449) showed that use of mobile phones is significantly ( p < .001) and negative (b equal to -.164) connected to the actual college GPA, after taking into account factors such as demographics, self-efficacy in self-regulated learning, self-efficacy to improve academic performance, and the actual high school GPA that were all important predictors ( p < .05). Therefore, after adjusting for other known predictors increasing cell phone usage was associated with lower academic performance. While more research is required to determine the mechanisms behind these results, they suggest the need to educate teachers and students to the possible academic risks that are associated with high-frequency mobile phone usage."
Quick tips on writing a good abstract
There exists a common dilemma among early age researchers whether to write the abstract at first or last? However, it's recommended to compose your abstract when you've completed the research since you'll have all the information to give to your readers. You can, however, write a draft at the beginning of your research and add in any gaps later.
If you find abstract writing a herculean task, here are the few tips to help you with it:
1. Always develop a framework to support your abstract
Before writing, ensure you create a clear outline for your abstract. Divide it into sections and draw the primary and supporting elements in each one. You can include keywords and a few sentences that convey the essence of your message.
2. Review Other Abstracts
Abstracts are among the most frequently used research documents, and thousands of them were written in the past. Therefore, prior to writing yours, take a look at some examples from other abstracts. There are plenty of examples of abstracts for dissertations in the dissertation and thesis databases.
3. Avoid Jargon To the Maximum
When you write your abstract, focus on simplicity over formality. You should write in simple language, and avoid excessive filler words or ambiguous sentences. Keep in mind that your abstract must be readable to those who aren't acquainted with your subject.
4. Focus on Your Research
It's a given fact that the abstract you write should be about your research and the findings you've made. It is not the right time to mention secondary and primary data sources unless it's absolutely required.
Conclusion: How to Structure an Interesting Abstract?
Abstracts are a short outline of your essay. However, it's among the most important, if not the most important. The process of writing an abstract is not straightforward. A few early-age researchers tend to begin by writing it, thinking they are doing it to "tease" the next step (the document itself). However, it is better to treat it as a spoiler.
The simple, concise style of the abstract lends itself to a well-written and well-investigated study. If your research paper doesn't provide definitive results, or the goal of your research is questioned, so will the abstract. Thus, only write your abstract after witnessing your findings and put your findings in the context of a larger scenario.
The process of writing an abstract can be daunting, but with these guidelines, you will succeed. The most efficient method of writing an excellent abstract is to centre the primary points of your abstract, including the research question and goals methods, as well as key results.
Interested in learning more about dedicated research solutions? Go to the SciSpace product page to find out how our suite of products can help you simplify your research workflows so you can focus on advancing science.

The best-in-class solution is equipped with features such as literature search and discovery, profile management, research writing and formatting, and so much more.
But before you go,
You might also like.

Consensus GPT vs. SciSpace GPT: Choose the Best GPT for Research
Literature Review and Theoretical Framework: Understanding the Differences

Types of Essays in Academic Writing - Quick Guide (2024)
Office of Undergraduate Research
- Office of Undergraduate Research FAQ's
- URSA Engage
- Resources for Students
- Resources for Faculty
- Engaging in Research
- Spring Poster Symposium (SPS)
- Earn Money by Participating in Research Studies
- Transcript Notation
- Student Publications
How to Write an Abstract
How to write an abstract for a conference, what is an abstract and why is it important, an abstract is a brief summary of your research or creative project, usually about a paragraph long (250-350 words), and is written when you are ready to present your research or included in a thesis or research publication..
For additional support in writing your abstract, you can contact the Office of URSA at [email protected] or schedule a time to meet with a Writing and Research Consultant at the OSU Writing Center
Main Components of an Abstract:
The opening sentences should summarize your topic and describe what researchers already know, with reference to the literature.
A brief discussion that clearly states the purpose of your research or creative project. This should give general background information on your work and allow people from different fields to understand what you are talking about. Use verbs like investigate, analyze, test, etc. to describe how you began your work.
In this section you will be discussing the ways in which your research was performed and the type of tools or methodological techniques you used to conduct your research.
This is where you describe the main findings of your research study and what you have learned. Try to include only the most important findings of your research that will allow the reader to understand your conclusions. If you have not completed the project, talk about your anticipated results and what you expect the outcomes of the study to be.
Significance
This is the final section of your abstract where you summarize the work performed. This is where you also discuss the relevance of your work and how it advances your field and the scientific field in general.
- Your word count for a conference may be limited, so make your abstract as clear and concise as possible.
- Organize it by using good transition words found on the lef so the information flows well.
- Have your abstract proofread and receive feedback from your supervisor, advisor, peers, writing center, or other professors from different disciplines.
- Double-check on the guidelines for your abstract and adhere to any formatting or word count requirements.
- Do not include bibliographic references or footnotes.
- Avoid the overuse of technical terms or jargon.
Feeling stuck? Visit the OSU ScholarsArchive for more abstract examples related to your field
Contact Info
618 Kerr Administration Building Corvallis, OR 97331
541-737-5105
Have a language expert improve your writing
Run a free plagiarism check in 10 minutes, automatically generate references for free.
- Knowledge Base
- Dissertation
- How to Write an Abstract | Steps & Examples
How to Write an Abstract | Steps & Examples
Published on 1 March 2019 by Shona McCombes . Revised on 10 October 2022 by Eoghan Ryan.
An abstract is a short summary of a longer work (such as a dissertation or research paper ). The abstract concisely reports the aims and outcomes of your research, so that readers know exactly what your paper is about.
Although the structure may vary slightly depending on your discipline, your abstract should describe the purpose of your work, the methods you’ve used, and the conclusions you’ve drawn.
One common way to structure your abstract is to use the IMRaD structure. This stands for:
- Introduction
Abstracts are usually around 100–300 words, but there’s often a strict word limit, so make sure to check the relevant requirements.
In a dissertation or thesis , include the abstract on a separate page, after the title page and acknowledgements but before the table of contents .
Instantly correct all language mistakes in your text
Be assured that you'll submit flawless writing. Upload your document to correct all your mistakes.
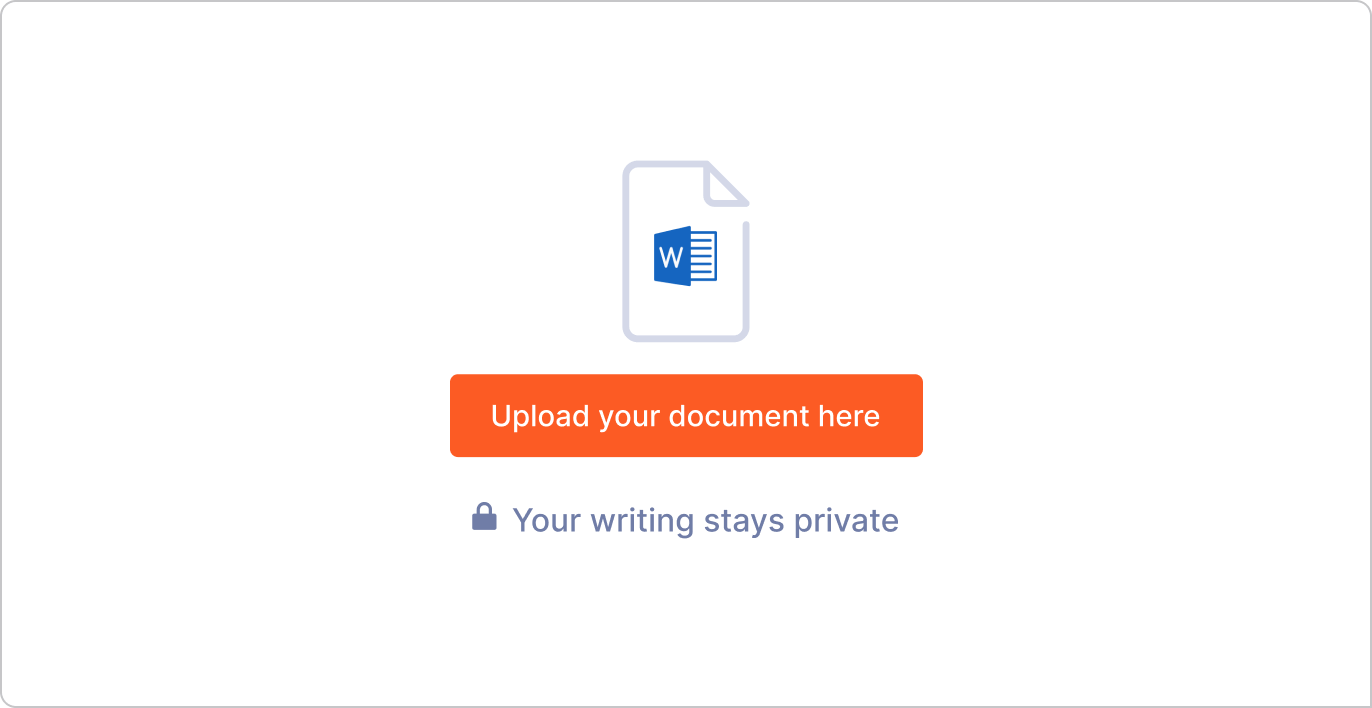
Table of contents
Abstract example, when to write an abstract, step 1: introduction, step 2: methods, step 3: results, step 4: discussion, tips for writing an abstract, frequently asked questions about abstracts.
Hover over the different parts of the abstract to see how it is constructed.
This paper examines the role of silent movies as a mode of shared experience in the UK during the early twentieth century. At this time, high immigration rates resulted in a significant percentage of non-English-speaking citizens. These immigrants faced numerous economic and social obstacles, including exclusion from public entertainment and modes of discourse (newspapers, theater, radio).
Incorporating evidence from reviews, personal correspondence, and diaries, this study demonstrates that silent films were an affordable and inclusive source of entertainment. It argues for the accessible economic and representational nature of early cinema. These concerns are particularly evident in the low price of admission and in the democratic nature of the actors’ exaggerated gestures, which allowed the plots and action to be easily grasped by a diverse audience despite language barriers.
Keywords: silent movies, immigration, public discourse, entertainment, early cinema, language barriers.
Prevent plagiarism, run a free check.
You will almost always have to include an abstract when:
- Completing a thesis or dissertation
- Submitting a research paper to an academic journal
- Writing a book proposal
- Applying for research grants
It’s easiest to write your abstract last, because it’s a summary of the work you’ve already done. Your abstract should:
- Be a self-contained text, not an excerpt from your paper
- Be fully understandable on its own
- Reflect the structure of your larger work
Start by clearly defining the purpose of your research. What practical or theoretical problem does the research respond to, or what research question did you aim to answer?
You can include some brief context on the social or academic relevance of your topic, but don’t go into detailed background information. If your abstract uses specialised terms that would be unfamiliar to the average academic reader or that have various different meanings, give a concise definition.
After identifying the problem, state the objective of your research. Use verbs like “investigate,” “test,” “analyse,” or “evaluate” to describe exactly what you set out to do.
This part of the abstract can be written in the present or past simple tense but should never refer to the future, as the research is already complete.
- This study will investigate the relationship between coffee consumption and productivity.
- This study investigates the relationship between coffee consumption and productivity.
Next, indicate the research methods that you used to answer your question. This part should be a straightforward description of what you did in one or two sentences. It is usually written in the past simple tense, as it refers to completed actions.
- Structured interviews will be conducted with 25 participants.
- Structured interviews were conducted with 25 participants.
Don’t evaluate validity or obstacles here — the goal is not to give an account of the methodology’s strengths and weaknesses, but to give the reader a quick insight into the overall approach and procedures you used.
Next, summarise the main research results . This part of the abstract can be in the present or past simple tense.
- Our analysis has shown a strong correlation between coffee consumption and productivity.
- Our analysis shows a strong correlation between coffee consumption and productivity.
- Our analysis showed a strong correlation between coffee consumption and productivity.
Depending on how long and complex your research is, you may not be able to include all results here. Try to highlight only the most important findings that will allow the reader to understand your conclusions.
Finally, you should discuss the main conclusions of your research : what is your answer to the problem or question? The reader should finish with a clear understanding of the central point that your research has proved or argued. Conclusions are usually written in the present simple tense.
- We concluded that coffee consumption increases productivity.
- We conclude that coffee consumption increases productivity.
If there are important limitations to your research (for example, related to your sample size or methods), you should mention them briefly in the abstract. This allows the reader to accurately assess the credibility and generalisability of your research.
If your aim was to solve a practical problem, your discussion might include recommendations for implementation. If relevant, you can briefly make suggestions for further research.
If your paper will be published, you might have to add a list of keywords at the end of the abstract. These keywords should reference the most important elements of the research to help potential readers find your paper during their own literature searches.
Be aware that some publication manuals, such as APA Style , have specific formatting requirements for these keywords.
It can be a real challenge to condense your whole work into just a couple of hundred words, but the abstract will be the first (and sometimes only) part that people read, so it’s important to get it right. These strategies can help you get started.
Read other abstracts
The best way to learn the conventions of writing an abstract in your discipline is to read other people’s. You probably already read lots of journal article abstracts while conducting your literature review —try using them as a framework for structure and style.
You can also find lots of dissertation abstract examples in thesis and dissertation databases .
Reverse outline
Not all abstracts will contain precisely the same elements. For longer works, you can write your abstract through a process of reverse outlining.
For each chapter or section, list keywords and draft one to two sentences that summarise the central point or argument. This will give you a framework of your abstract’s structure. Next, revise the sentences to make connections and show how the argument develops.
Write clearly and concisely
A good abstract is short but impactful, so make sure every word counts. Each sentence should clearly communicate one main point.
To keep your abstract or summary short and clear:
- Avoid passive sentences: Passive constructions are often unnecessarily long. You can easily make them shorter and clearer by using the active voice.
- Avoid long sentences: Substitute longer expressions for concise expressions or single words (e.g., “In order to” for “To”).
- Avoid obscure jargon: The abstract should be understandable to readers who are not familiar with your topic.
- Avoid repetition and filler words: Replace nouns with pronouns when possible and eliminate unnecessary words.
- Avoid detailed descriptions: An abstract is not expected to provide detailed definitions, background information, or discussions of other scholars’ work. Instead, include this information in the body of your thesis or paper.
If you’re struggling to edit down to the required length, you can get help from expert editors with Scribbr’s professional proofreading services .
Check your formatting
If you are writing a thesis or dissertation or submitting to a journal, there are often specific formatting requirements for the abstract—make sure to check the guidelines and format your work correctly. For APA research papers you can follow the APA abstract format .
Checklist: Abstract
The word count is within the required length, or a maximum of one page.
The abstract appears after the title page and acknowledgements and before the table of contents .
I have clearly stated my research problem and objectives.
I have briefly described my methodology .
I have summarized the most important results .
I have stated my main conclusions .
I have mentioned any important limitations and recommendations.
The abstract can be understood by someone without prior knowledge of the topic.
You've written a great abstract! Use the other checklists to continue improving your thesis or dissertation.
An abstract is a concise summary of an academic text (such as a journal article or dissertation ). It serves two main purposes:
- To help potential readers determine the relevance of your paper for their own research.
- To communicate your key findings to those who don’t have time to read the whole paper.
Abstracts are often indexed along with keywords on academic databases, so they make your work more easily findable. Since the abstract is the first thing any reader sees, it’s important that it clearly and accurately summarises the contents of your paper.
An abstract for a thesis or dissertation is usually around 150–300 words. There’s often a strict word limit, so make sure to check your university’s requirements.
The abstract is the very last thing you write. You should only write it after your research is complete, so that you can accurately summarize the entirety of your thesis or paper.
Avoid citing sources in your abstract . There are two reasons for this:
- The abstract should focus on your original research, not on the work of others.
- The abstract should be self-contained and fully understandable without reference to other sources.
There are some circumstances where you might need to mention other sources in an abstract: for example, if your research responds directly to another study or focuses on the work of a single theorist. In general, though, don’t include citations unless absolutely necessary.
The abstract appears on its own page, after the title page and acknowledgements but before the table of contents .
Cite this Scribbr article
If you want to cite this source, you can copy and paste the citation or click the ‘Cite this Scribbr article’ button to automatically add the citation to our free Reference Generator.
McCombes, S. (2022, October 10). How to Write an Abstract | Steps & Examples. Scribbr. Retrieved 29 April 2024, from https://www.scribbr.co.uk/thesis-dissertation/abstract/
Is this article helpful?
Shona McCombes
Other students also liked, how to write a thesis or dissertation introduction, thesis & dissertation acknowledgements | tips & examples, dissertation title page.
- Features for Creative Writers
- Features for Work
- Features for Higher Education
- Features for Teachers
- Features for Non-Native Speakers
- Learn Blog Grammar Guide Community Events FAQ
- Grammar Guide
How to Write an Abstract (With Examples)
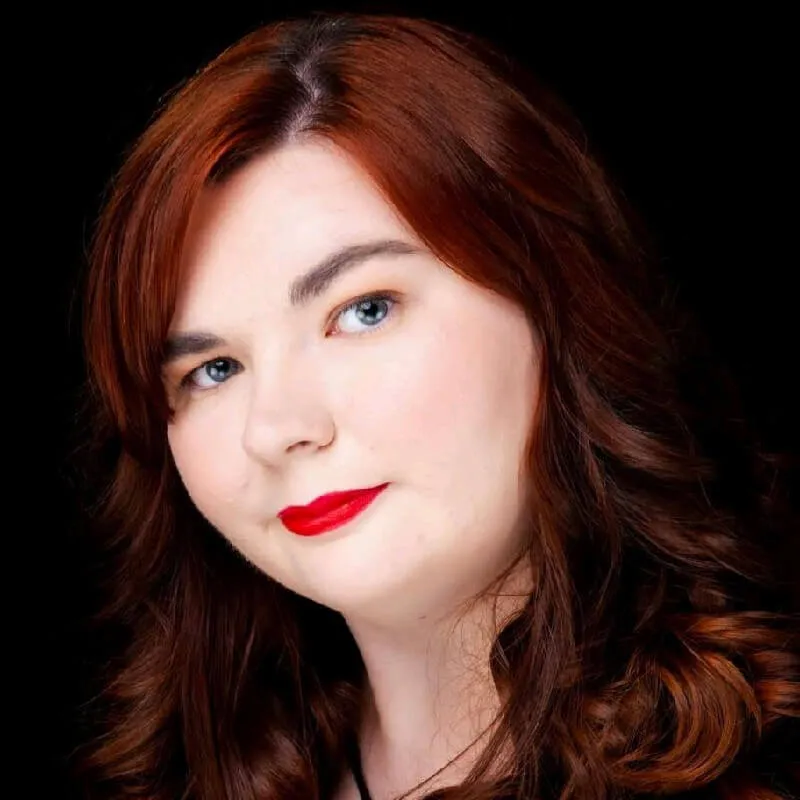
Sarah Oakley
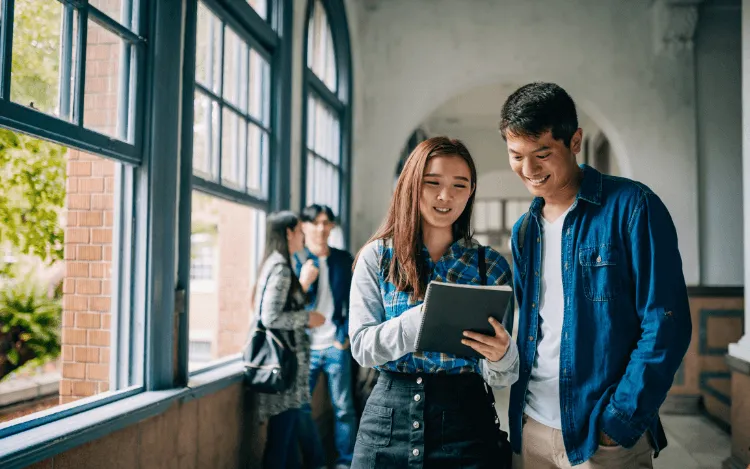
Table of Contents
What is an abstract in a paper, how long should an abstract be, 5 steps for writing an abstract, examples of an abstract, how prowritingaid can help you write an abstract.
If you are writing a scientific research paper or a book proposal, you need to know how to write an abstract, which summarizes the contents of the paper or book.
When researchers are looking for peer-reviewed papers to use in their studies, the first place they will check is the abstract to see if it applies to their work. Therefore, your abstract is one of the most important parts of your entire paper.
In this article, we’ll explain what an abstract is, what it should include, and how to write one.
An abstract is a concise summary of the details within a report. Some abstracts give more details than others, but the main things you’ll be talking about are why you conducted the research, what you did, and what the results show.
When a reader is deciding whether to read your paper completely, they will first look at the abstract. You need to be concise in your abstract and give the reader the most important information so they can determine if they want to read the whole paper.
Remember that an abstract is the last thing you’ll want to write for the research paper because it directly references parts of the report. If you haven’t written the report, you won’t know what to include in your abstract.
If you are writing a paper for a journal or an assignment, the publication or academic institution might have specific formatting rules for how long your abstract should be. However, if they don’t, most abstracts are between 150 and 300 words long.
A short word count means your writing has to be precise and without filler words or phrases. Once you’ve written a first draft, you can always use an editing tool, such as ProWritingAid, to identify areas where you can reduce words and increase readability.
If your abstract is over the word limit, and you’ve edited it but still can’t figure out how to reduce it further, your abstract might include some things that aren’t needed. Here’s a list of three elements you can remove from your abstract:
Discussion : You don’t need to go into detail about the findings of your research because your reader will find your discussion within the paper.
Definition of terms : Your readers are interested the field you are writing about, so they are likely to understand the terms you are using. If not, they can always look them up. Your readers do not expect you to give a definition of terms in your abstract.
References and citations : You can mention there have been studies that support or have inspired your research, but you do not need to give details as the reader will find them in your bibliography.
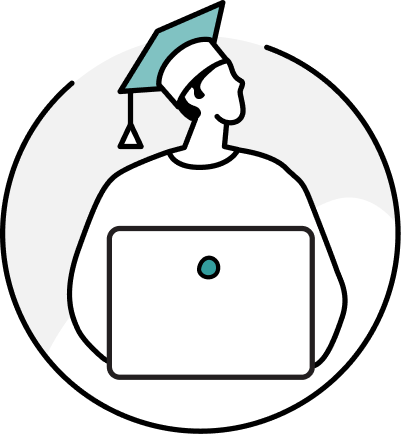
Good writing = better grades
ProWritingAid will help you improve the style, strength, and clarity of all your assignments.
If you’ve never written an abstract before, and you’re wondering how to write an abstract, we’ve got some steps for you to follow. It’s best to start with planning your abstract, so we’ve outlined the details you need to include in your plan before you write.
Remember to consider your audience when you’re planning and writing your abstract. They are likely to skim read your abstract, so you want to be sure your abstract delivers all the information they’re expecting to see at key points.
1. What Should an Abstract Include?
Abstracts have a lot of information to cover in a short number of words, so it’s important to know what to include. There are three elements that need to be present in your abstract:
Your context is the background for where your research sits within your field of study. You should briefly mention any previous scientific papers or experiments that have led to your hypothesis and how research develops in those studies.
Your hypothesis is your prediction of what your study will show. As you are writing your abstract after you have conducted your research, you should still include your hypothesis in your abstract because it shows the motivation for your paper.
Throughout your abstract, you also need to include keywords and phrases that will help researchers to find your article in the databases they’re searching. Make sure the keywords are specific to your field of study and the subject you’re reporting on, otherwise your article might not reach the relevant audience.
2. Can You Use First Person in an Abstract?
You might think that first person is too informal for a research paper, but it’s not. Historically, writers of academic reports avoided writing in first person to uphold the formality standards of the time. However, first person is more accepted in research papers in modern times.
If you’re still unsure whether to write in first person for your abstract, refer to any style guide rules imposed by the journal you’re writing for or your teachers if you are writing an assignment.
3. Abstract Structure
Some scientific journals have strict rules on how to structure an abstract, so it’s best to check those first. If you don’t have any style rules to follow, try using the IMRaD structure, which stands for Introduction, Methodology, Results, and Discussion.
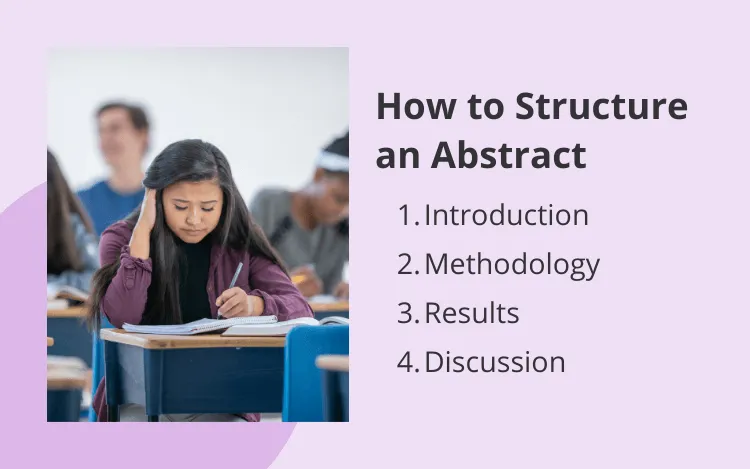
Following the IMRaD structure, start with an introduction. The amount of background information you should include depends on your specific research area. Adding a broad overview gives you less room to include other details. Remember to include your hypothesis in this section.
The next part of your abstract should cover your methodology. Try to include the following details if they apply to your study:
What type of research was conducted?
How were the test subjects sampled?
What were the sample sizes?
What was done to each group?
How long was the experiment?
How was data recorded and interpreted?
Following the methodology, include a sentence or two about the results, which is where your reader will determine if your research supports or contradicts their own investigations.
The results are also where most people will want to find out what your outcomes were, even if they are just mildly interested in your research area. You should be specific about all the details but as concise as possible.
The last few sentences are your conclusion. It needs to explain how your findings affect the context and whether your hypothesis was correct. Include the primary take-home message, additional findings of importance, and perspective. Also explain whether there is scope for further research into the subject of your report.
Your conclusion should be honest and give the reader the ultimate message that your research shows. Readers trust the conclusion, so make sure you’re not fabricating the results of your research. Some readers won’t read your entire paper, but this section will tell them if it’s worth them referencing it in their own study.
4. How to Start an Abstract
The first line of your abstract should give your reader the context of your report by providing background information. You can use this sentence to imply the motivation for your research.
You don’t need to use a hook phrase or device in your first sentence to grab the reader’s attention. Your reader will look to establish relevance quickly, so readability and clarity are more important than trying to persuade the reader to read on.
5. How to Format an Abstract
Most abstracts use the same formatting rules, which help the reader identify the abstract so they know where to look for it.
Here’s a list of formatting guidelines for writing an abstract:
Stick to one paragraph
Use block formatting with no indentation at the beginning
Put your abstract straight after the title and acknowledgements pages
Use present or past tense, not future tense
There are two primary types of abstract you could write for your paper—descriptive and informative.
An informative abstract is the most common, and they follow the structure mentioned previously. They are longer than descriptive abstracts because they cover more details.
Descriptive abstracts differ from informative abstracts, as they don’t include as much discussion or detail. The word count for a descriptive abstract is between 50 and 150 words.
Here is an example of an informative abstract:
A growing trend exists for authors to employ a more informal writing style that uses “we” in academic writing to acknowledge one’s stance and engagement. However, few studies have compared the ways in which the first-person pronoun “we” is used in the abstracts and conclusions of empirical papers. To address this lacuna in the literature, this study conducted a systematic corpus analysis of the use of “we” in the abstracts and conclusions of 400 articles collected from eight leading electrical and electronic (EE) engineering journals. The abstracts and conclusions were extracted to form two subcorpora, and an integrated framework was applied to analyze and seek to explain how we-clusters and we-collocations were employed. Results revealed whether authors’ use of first-person pronouns partially depends on a journal policy. The trend of using “we” showed that a yearly increase occurred in the frequency of “we” in EE journal papers, as well as the existence of three “we-use” types in the article conclusions and abstracts: exclusive, inclusive, and ambiguous. Other possible “we-use” alternatives such as “I” and other personal pronouns were used very rarely—if at all—in either section. These findings also suggest that the present tense was used more in article abstracts, but the present perfect tense was the most preferred tense in article conclusions. Both research and pedagogical implications are proffered and critically discussed.
Wang, S., Tseng, W.-T., & Johanson, R. (2021). To We or Not to We: Corpus-Based Research on First-Person Pronoun Use in Abstracts and Conclusions. SAGE Open, 11(2).
Here is an example of a descriptive abstract:
From the 1850s to the present, considerable criminological attention has focused on the development of theoretically-significant systems for classifying crime. This article reviews and attempts to evaluate a number of these efforts, and we conclude that further work on this basic task is needed. The latter part of the article explicates a conceptual foundation for a crime pattern classification system, and offers a preliminary taxonomy of crime.
Farr, K. A., & Gibbons, D. C. (1990). Observations on the Development of Crime Categories. International Journal of Offender Therapy and Comparative Criminology, 34(3), 223–237.
If you want to ensure your abstract is grammatically correct and easy to read, you can use ProWritingAid to edit it. The software integrates with Microsoft Word, Google Docs, and most web browsers, so you can make the most of it wherever you’re writing your paper.
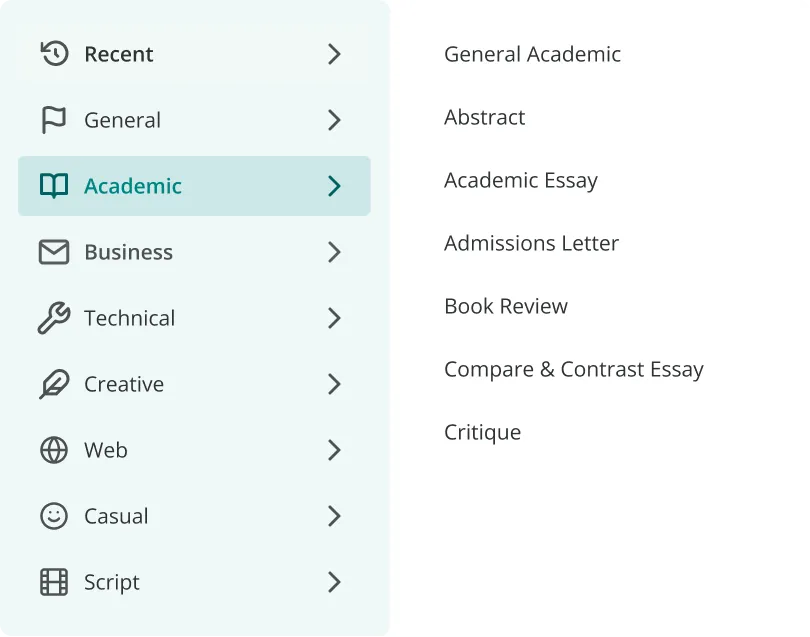
Before you edit with ProWritingAid, make sure the suggestions you are seeing are relevant for your document by changing the document type to “Abstract” within the Academic writing style section.
You can use the Readability report to check your abstract for places to improve the clarity of your writing. Some suggestions might show you where to remove words, which is great if you’re over your word count.
We hope the five steps and examples we’ve provided help you write a great abstract for your research paper.
Get started with ProWritingAid
Drop us a line or let's stay in touch via :
When you choose to publish with PLOS, your research makes an impact. Make your work accessible to all, without restrictions, and accelerate scientific discovery with options like preprints and published peer review that make your work more Open.
- PLOS Biology
- PLOS Climate
- PLOS Complex Systems
- PLOS Computational Biology
- PLOS Digital Health
- PLOS Genetics
- PLOS Global Public Health
- PLOS Medicine
- PLOS Mental Health
- PLOS Neglected Tropical Diseases
- PLOS Pathogens
- PLOS Sustainability and Transformation
- PLOS Collections
- How to Write an Abstract

Expedite peer review, increase search-ability, and set the tone for your study
The abstract is your chance to let your readers know what they can expect from your article. Learn how to write a clear, and concise abstract that will keep your audience reading.
How your abstract impacts editorial evaluation and future readership
After the title , the abstract is the second-most-read part of your article. A good abstract can help to expedite peer review and, if your article is accepted for publication, it’s an important tool for readers to find and evaluate your work. Editors use your abstract when they first assess your article. Prospective reviewers see it when they decide whether to accept an invitation to review. Once published, the abstract gets indexed in PubMed and Google Scholar , as well as library systems and other popular databases. Like the title, your abstract influences keyword search results. Readers will use it to decide whether to read the rest of your article. Other researchers will use it to evaluate your work for inclusion in systematic reviews and meta-analysis. It should be a concise standalone piece that accurately represents your research.
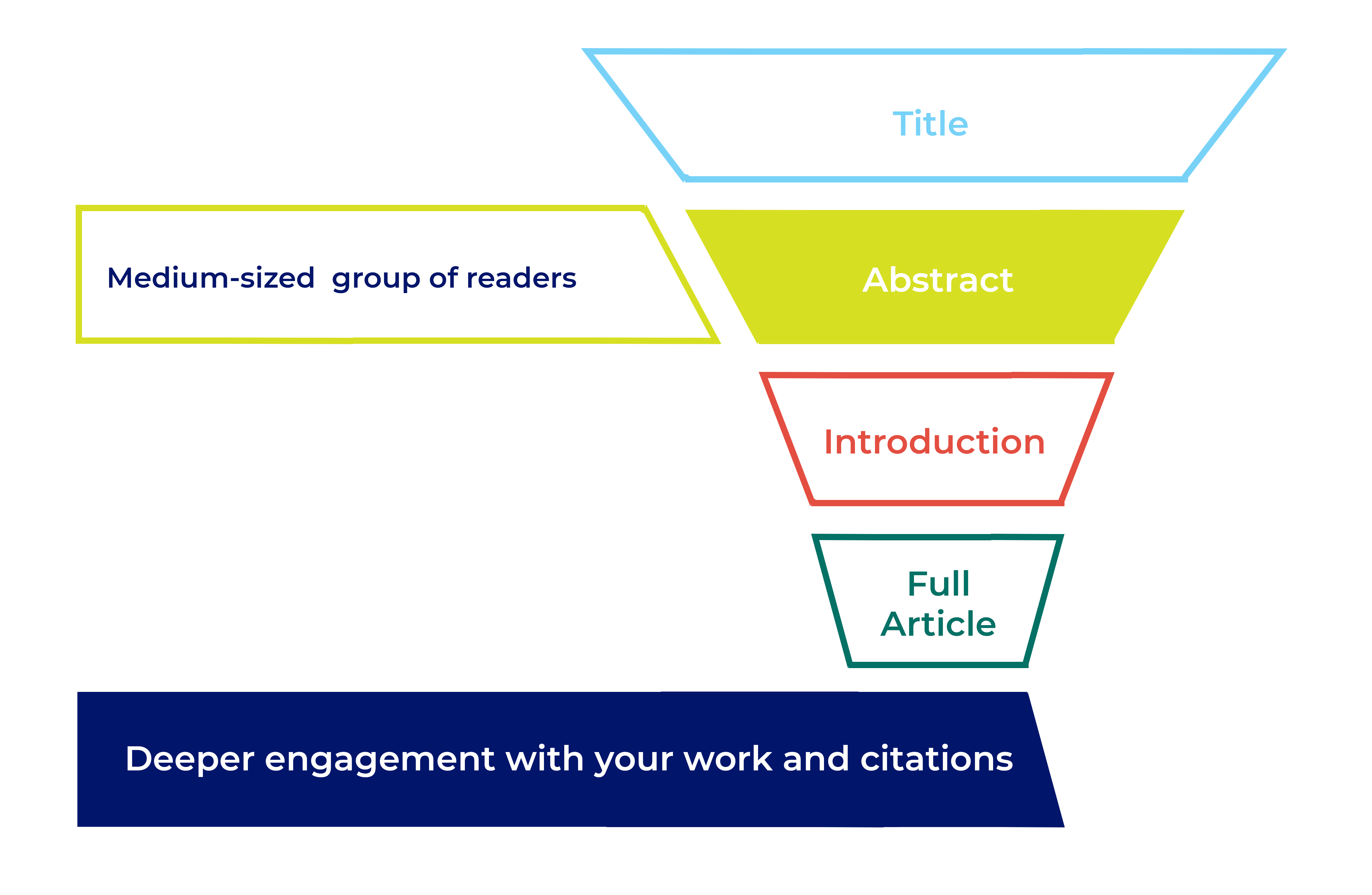
What to include in an abstract
The main challenge you’ll face when writing your abstract is keeping it concise AND fitting in all the information you need. Depending on your subject area the journal may require a structured abstract following specific headings. A structured abstract helps your readers understand your study more easily. If your journal doesn’t require a structured abstract it’s still a good idea to follow a similar format, just present the abstract as one paragraph without headings.
Background or Introduction – What is currently known? Start with a brief, 2 or 3 sentence, introduction to the research area.
Objectives or Aims – What is the study and why did you do it? Clearly state the research question you’re trying to answer.
Methods – What did you do? Explain what you did and how you did it. Include important information about your methods, but avoid the low-level specifics. Some disciplines have specific requirements for abstract methods.
- CONSORT for randomized trials.
- STROBE for observational studies
- PRISMA for systematic reviews and meta-analyses
Results – What did you find? Briefly give the key findings of your study. Include key numeric data (including confidence intervals or p values), where possible.
Conclusions – What did you conclude? Tell the reader why your findings matter, and what this could mean for the ‘bigger picture’ of this area of research.
Writing tips
The main challenge you may find when writing your abstract is keeping it concise AND convering all the information you need to.
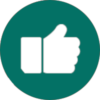
- Keep it concise and to the point. Most journals have a maximum word count, so check guidelines before you write the abstract to save time editing it later.
- Write for your audience. Are they specialists in your specific field? Are they cross-disciplinary? Are they non-specialists? If you’re writing for a general audience, or your research could be of interest to the public keep your language as straightforward as possible. If you’re writing in English, do remember that not all of your readers will necessarily be native English speakers.
- Focus on key results, conclusions and take home messages.
- Write your paper first, then create the abstract as a summary.
- Check the journal requirements before you write your abstract, eg. required subheadings.
- Include keywords or phrases to help readers search for your work in indexing databases like PubMed or Google Scholar.
- Double and triple check your abstract for spelling and grammar errors. These kind of errors can give potential reviewers the impression that your research isn’t sound, and can make it easier to find reviewers who accept the invitation to review your manuscript. Your abstract should be a taste of what is to come in the rest of your article.

Don’t
- Sensationalize your research.
- Speculate about where this research might lead in the future.
- Use abbreviations or acronyms (unless absolutely necessary or unless they’re widely known, eg. DNA).
- Repeat yourself unnecessarily, eg. “Methods: We used X technique. Results: Using X technique, we found…”
- Contradict anything in the rest of your manuscript.
- Include content that isn’t also covered in the main manuscript.
- Include citations or references.
Tip: How to edit your work
Editing is challenging, especially if you are acting as both a writer and an editor. Read our guidelines for advice on how to refine your work, including useful tips for setting your intentions, re-review, and consultation with colleagues.
- How to Write a Great Title
- How to Write Your Methods
- How to Report Statistics
- How to Write Discussions and Conclusions
- How to Edit Your Work
The contents of the Peer Review Center are also available as a live, interactive training session, complete with slides, talking points, and activities. …
The contents of the Writing Center are also available as a live, interactive training session, complete with slides, talking points, and activities. …
There’s a lot to consider when deciding where to submit your work. Learn how to choose a journal that will help your study reach its audience, while reflecting your values as a researcher…
- Privacy Policy

Home » Research Paper Abstract – Writing Guide and Examples
Research Paper Abstract – Writing Guide and Examples
Table of Contents

Research Paper Abstract
Research Paper Abstract is a brief summary of a research pape r that describes the study’s purpose, methods, findings, and conclusions . It is often the first section of the paper that readers encounter, and its purpose is to provide a concise and accurate overview of the paper’s content. The typical length of an abstract is usually around 150-250 words, and it should be written in a concise and clear manner.
Research Paper Abstract Structure
The structure of a research paper abstract usually includes the following elements:
- Background or Introduction: Briefly describe the problem or research question that the study addresses.
- Methods : Explain the methodology used to conduct the study, including the participants, materials, and procedures.
- Results : Summarize the main findings of the study, including statistical analyses and key outcomes.
- Conclusions : Discuss the implications of the study’s findings and their significance for the field, as well as any limitations or future directions for research.
- Keywords : List a few keywords that describe the main topics or themes of the research.
How to Write Research Paper Abstract
Here are the steps to follow when writing a research paper abstract:
- Start by reading your paper: Before you write an abstract, you should have a complete understanding of your paper. Read through the paper carefully, making sure you understand the purpose, methods, results, and conclusions.
- Identify the key components : Identify the key components of your paper, such as the research question, methods used, results obtained, and conclusion reached.
- Write a draft: Write a draft of your abstract, using concise and clear language. Make sure to include all the important information, but keep it short and to the point. A good rule of thumb is to keep your abstract between 150-250 words.
- Use clear and concise language : Use clear and concise language to explain the purpose of your study, the methods used, the results obtained, and the conclusions drawn.
- Emphasize your findings: Emphasize your findings in the abstract, highlighting the key results and the significance of your study.
- Revise and edit: Once you have a draft, revise and edit it to ensure that it is clear, concise, and free from errors.
- Check the formatting: Finally, check the formatting of your abstract to make sure it meets the requirements of the journal or conference where you plan to submit it.
Research Paper Abstract Examples
Research Paper Abstract Examples could be following:
Title : “The Effectiveness of Cognitive-Behavioral Therapy for Treating Anxiety Disorders: A Meta-Analysis”
Abstract : This meta-analysis examines the effectiveness of cognitive-behavioral therapy (CBT) in treating anxiety disorders. Through the analysis of 20 randomized controlled trials, we found that CBT is a highly effective treatment for anxiety disorders, with large effect sizes across a range of anxiety disorders, including generalized anxiety disorder, panic disorder, and social anxiety disorder. Our findings support the use of CBT as a first-line treatment for anxiety disorders and highlight the importance of further research to identify the mechanisms underlying its effectiveness.
Title : “Exploring the Role of Parental Involvement in Children’s Education: A Qualitative Study”
Abstract : This qualitative study explores the role of parental involvement in children’s education. Through in-depth interviews with 20 parents of children in elementary school, we found that parental involvement takes many forms, including volunteering in the classroom, helping with homework, and communicating with teachers. We also found that parental involvement is influenced by a range of factors, including parent and child characteristics, school culture, and socio-economic status. Our findings suggest that schools and educators should prioritize building strong partnerships with parents to support children’s academic success.
Title : “The Impact of Exercise on Cognitive Function in Older Adults: A Systematic Review and Meta-Analysis”
Abstract : This paper presents a systematic review and meta-analysis of the existing literature on the impact of exercise on cognitive function in older adults. Through the analysis of 25 randomized controlled trials, we found that exercise is associated with significant improvements in cognitive function, particularly in the domains of executive function and attention. Our findings highlight the potential of exercise as a non-pharmacological intervention to support cognitive health in older adults.
When to Write Research Paper Abstract
The abstract of a research paper should typically be written after you have completed the main body of the paper. This is because the abstract is intended to provide a brief summary of the key points and findings of the research, and you can’t do that until you have completed the research and written about it in detail.
Once you have completed your research paper, you can begin writing your abstract. It is important to remember that the abstract should be a concise summary of your research paper, and should be written in a way that is easy to understand for readers who may not have expertise in your specific area of research.
Purpose of Research Paper Abstract
The purpose of a research paper abstract is to provide a concise summary of the key points and findings of a research paper. It is typically a brief paragraph or two that appears at the beginning of the paper, before the introduction, and is intended to give readers a quick overview of the paper’s content.
The abstract should include a brief statement of the research problem, the methods used to investigate the problem, the key results and findings, and the main conclusions and implications of the research. It should be written in a clear and concise manner, avoiding jargon and technical language, and should be understandable to a broad audience.
The abstract serves as a way to quickly and easily communicate the main points of a research paper to potential readers, such as academics, researchers, and students, who may be looking for information on a particular topic. It can also help researchers determine whether a paper is relevant to their own research interests and whether they should read the full paper.
About the author
Muhammad Hassan
Researcher, Academic Writer, Web developer
You may also like

How to Cite Research Paper – All Formats and...

Delimitations in Research – Types, Examples and...

Research Paper Format – Types, Examples and...

Research Design – Types, Methods and Examples

Research Paper Title – Writing Guide and Example

Research Paper Introduction – Writing Guide and...
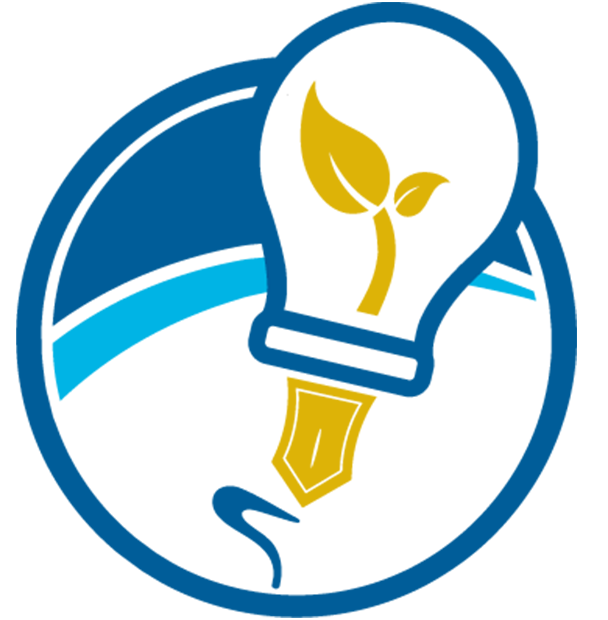
Undergraduate Research Center
The following instructions are for the Undergraduate Research Center's Undergraduate Research, Scholarship and Creative Activities Conference, however the general concepts will apply to abstracts for similar conferences. In the video to the right, Kendon Kurzer, PhD presents guidance from the University Writing Program. To see abstracts from previous URC Conferences, visit our Abstract Books Page .
What is an abstract?
An abstract is a summary of a research project. Abstracts precede papers in research journals and appear in programs of scholarly conferences. In journals, the abstract allows readers to quickly grasp the purpose and major ideas of a paper and lets other researchers know whether reading the entire paper will be worthwhile. In conferences, the abstract is the advertisement that the paper/presentation deserves the audience's attention.
Why write an abstract?
The abstract allows readers to make decisions about your project. Your sponsoring professor can use the abstract to decide if your research is proceeding smoothly. The conference organizer uses it to decide if your project fits the conference criteria. The conference audience (faculty, administrators, peers, and presenters' families) uses your abstract to decide whether or not to attend your presentation. Your abstract needs to take all these readers into consideration.
How does an abstract appeal to such a broad audience?
The audience for the abstract for the Undergraduate Research, Scholarship and Creative Activities Conference (URSCA) covers the broadest possible scope--from expert to lay person. You need to find a comfortable balance between writing an abstract that both shows your knowledge and yet is still comprehensible--with some effort--by lay members of the audience. Limit the amount of technical language you use and explain it where possible. Always use the full term before you refer to it by acronym Example: DNA double-stranded breaks (DSBs). Remember that you are yourself an expert in the field that you are writing about--don't take for granted that the reader will share your insider knowledge.
What should the abstract include?
Think of your abstract as a condensed version of your whole project. By reading it, the reader should understand the nature of your research question.
Like abstracts that researchers prepare for scholarly conferences, the abstract you submit for the Undergraduate Research, Scholarship and Creativities Conference (URSCA) will most likely reflect work still in progress at the time you write it. Although the content will vary according to field and specific project, all abstracts, whether in the sciences or the humanities, convey the following information:
- The purpose of the project identifying the area of study to which it belongs.
- The research problem that motivates the project.
- The methods used to address this research problem, documents or evidence analyzed.
- The conclusions reached or, if the research is in progress, what the preliminary results of the investigation suggest, or what the research methods demonstrate.
- The significance of the research project. Why are the results useful? What is new to our understanding as the result of your inquiry?
Whatever kind of research you are doing, your abstract should provide the reader with answers to the following questions: What are you asking? Why is it important? How will you study it? What will you use to demonstrate your conclusions? What are those conclusions? What do they mean?
SUGGESTED CONTENT STRUCTURE:
Brief Background/Introduction/Research Context: What do we know about the topic? Why is the topic important? Present Research Question/Purpose: What is the study about? Methods/Materials/Subjects/Materials: How was the study done? Results/Findings: What was discovered? Discussion/Conclusion/Implications/Recommendations What does it mean?
What if the research is in progress and I don't have results yet?
For the URSCA Conference you can write a "Promissory Abstract" which will still describe the background, purpose and how you will accomplish your study's purpose and why it is important. Phrases like "to show whether" or "to determine if" can be helpful to avoid sharing a "hoped for" result.
Stylistic considerations
The abstract should be one paragraph for the URSCA Conference and should not exceed the word limit (150-200 words). Edit it closely to be sure it meets the Four C's of abstract writing:
- Complete — it covers the major parts of the project.
- Concise — it contains no excess wordiness or unnecessary information.
- Clear — it is readable, well organized, and not too jargon-laden.
- Cohesive — it flows smoothly between the parts.
The importance of understandable language
Because all researchers hope their work will be useful to others, and because good scholarship is increasingly used across disciplines, it is crucial to make the language of your abstracts accessible to a non-specialist. Simplify your language. Friends in another major will spot instantly what needs to be more understandable. Some problem areas to look for:
- Eliminate jargon. Showing off your technical vocabulary will not demonstrate that your research is valuable. If using a technical term is unavoidable, add a non-technical synonym to help a non-specialist infer the term's meaning.
- Omit needless words—redundant modifiers, pompous diction, excessive detail.
- Avoid stringing nouns together (make the relationship clear with prepositions).
- Eliminate "narration," expressions such as "It is my opinion that," "I have concluded," "the main point supporting my view/concerns," or "certainly there is little doubt as to. . . ." Focus attention solely on what the reader needs to know.
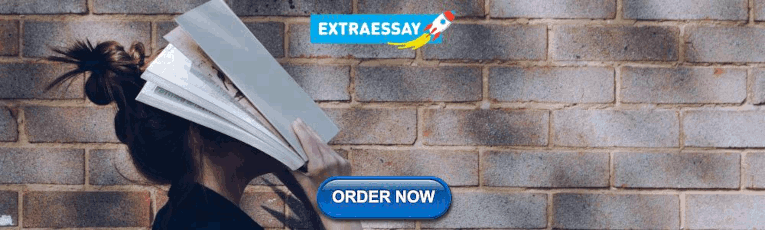
Before submitting your abstract to the URSCA Conference:
- Make sure it is within the word limit. You can start with a large draft and then edit it down to make sure your abstract is complete but also concise. (Over-writing is all too easy, so reserve time for cutting your abstract down to the essential information.).
- Make sure the language is understandable by a non-specialist. (Avoid writing for an audience that includes only you and your professor.)
- Have your sponsoring professor work with you and approve the abstract before you submit it online.
- Only one abstract per person is allowed for the URSCA Conference.
Multimedia Risk Assessment of Biodiesel - Tier II Antfarm Project
Significant knowledge gaps exist in the fate, transport, biodegradation, and toxicity properties of biodiesel when it is leaked into the environment. In order to fill these gaps, a combination of experiments has been developed in a Multimedia Risk Assessment of Biodiesel for the State of California. Currently, in the Tier II experimental phase of this assessment, I am investigating underground plume mobility of 20% and 100% additized and unadditized Soy and Animal Fat based biodiesel blends and comparing them to Ultra Low-Sulfer Diesel #2 (USLD) by filming these fuels as they seep through unsaturated sand, encounter a simulated underground water table, and form a floating lens on top of the water. Thus far, initial findings in analyzing the digital images created during the filming process have indicated that all fuels tested have similar travel times. SoyB20 behaves most like USLD in that they both have a similar lateral dispersion lens on top of the water table. In contrast, Animal Fat B100 appears to be most different from ULSD in that it has a narrower residual plume in the unsaturated sand, as well as a narrower and deeper lens formation on top of the water table.
Narrative Representation of Grief
In William Faulkner's As I Lay Dying and Kazuo Ishiguro's Never Let Me Go how can grief, an incomprehensible and incommunicable emotion, be represented in fiction? Is it paradoxical, or futile, to do so? I look at two novels that struggle with representing intense combinations of individual and communal grief: William Faulkner's As I Lay Dying and Kazuo Ishiguro's Never Let Me Go . At first glance, the novels appear to have nothing in common: Faulkner's is a notoriously bleak odyssey told in emotionally heavy stream-of-consciousness narrative, while Ishiguro's is a near-kitschy blend of a coming-of-age tale and a sci-fi dystopia. But they share a rare common thread. They do not try to convey a story, a character, an argument, or a realization, so much as they try to convey an emotion. The novels' common struggle is visible through their formal elements, down to the most basic technical aspects of how the stories are told. Each text, in its own way, enacts the trauma felt by its characters because of their grief, and also the frustration felt by its narrator (or narrators) because of the complex and guilty task of witnessing for grief and loss.
This webpage was based on articles written by Professor Diana Strazdes, Art History and Dr. Amy Clarke, University Writing Program, UC Davis. Thanks to both for their contributions.
University of Missouri
- Bias Hotline: Report bias incidents
Undergraduate Research
- How to Write An Abstract
Think of your abstract or artist statement like a movie trailer: it should leave the reader eager to learn more but knowledgeable enough to grasp the scope of your work. Although abstracts and artist statements need to contain key information on your project, your title and summary should be understandable to a lay audience.
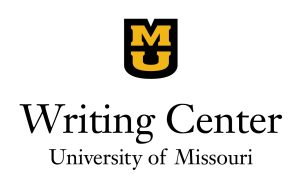
Please remember that you can seek assistance with any of your writing needs at the MU Writing Center . Their tutors work with students from all disciplines on a wide variety of documents. And they are specially trained to use the Abstract Review Rubric that will be used on the abstracts reviewed at the Spring Forum.
Types of Research Summaries
Students should submit artist statements as their abstracts. Artist statements should introduce to the art, performance, or creative work and include information on media and methods in creating the pieces. The statements should also include a description of the inspiration for the work, the meaning the work signifies to the artist, the artistic influences, and any unique methods used to create the pieces. Students are encouraged to explain the connections of the work with their inspirations or themes. The statements should be specific to the work presented and not a general statements about the students’ artistic philosophies and approaches. Effective artist statements should provide the viewer with information to better understand the work of the artists. If presentations are based on previous performances, then students may include reflections on the performance experiences and audience reactions.
Abstracts should describe the nature of the project or piece (ex: architectural images used for a charrette, fashion plates, advertising campaign story boards) and its intended purpose. Students should describe the project or problem that they addressed and limitations and challenges that impact the design process. Students may wish to include research conducted to provide context for the project and inform the design process. A description of the clients/end users may be included. Information on inspirations, motivations, and influences may also be included as appropriate to the discipline and project. A description of the project outcome should be included.
Abstracts should include a short introduction or background to put the research into context; purpose of the research project; a problem statement or thesis; a brief description of materials, methods, or subjects (as appropriate for the discipline); results and analysis; conclusions and implications; and recommendations. For research projects still in progress at the time of abstract submission, students may opt to indicate that results and conclusions will be presented [at the Forum].
Tips for writing a clear and concise abstract
The title of your abstract/statement/poster should include some language that the lay person can understand. When someone reads your title they should have SOME idea of the nature of your work and your discipline.
Ask a peer unfamiliar with your research to read your abstract. If they’re confused by it, others will be too.
Keep it short and sweet.
- Interesting eye-catching title
- Introduction: 1-3 sentences
- What you did: 1 sentence
- Why you did it: 1 sentence
- How you did it: 1 sentence
- Results or when they are expected: 2 sentences
- Conclusion: 1-3 sentences
Ideas to Address:
- The big picture your project helps tackle
- The problem motivating your work on this particular project
- General methods you used
- Results and/or conclusions
- The next steps for the project
Things to Avoid:
- A long and confusing title
- Jargon or complicated industry terms
- Long description of methods/procedures
- Exaggerating your results
- Exceeding the allowable word limit
- Forgetting to tell people why to care
- References that keep the abstract from being a “stand alone” document
- Being boring, confusing, or unintelligible!
Artist Statement
The artist statement should be an introduction to the art and include information on media and methods in creating the piece(s). It should include a description of the inspiration for the work, what the work signifies to the artist, the artistic influences, and any unique methods used to create the work. Students are encouraged to explain the connections of the work with their inspiration or theme. The artist statement (up to 300 words) should be written in plain language to invite viewers to learn more about the artist’s work and make their own interpretations. The statement should be specific to the piece(s) that will be on display, and not a general statement about the student’s artistic philosophy and approach. An effective artist statement should provide the viewer with information to better understand and experience viewing the work on display.
Research/Applied Design Abstract
The project abstract (up to 300 words) should describe the nature of the project or piece (ex: architectural images used for a charrette, fashion plates, small scale model of a theater set) and its intended purpose. Students should describe the project or problem that was addressed and limitations and challenges that impact the design process. Students may wish to include research conducted to provide context for the project and inform the design process. A description of the clients/end users may be included. Information on inspirations, motivations, and influences may also be included as appropriate to the discipline and project.
Key Considerations
- What is the problem/ big picture that your project helps to address?
- What is the appropriate background to put your project into context? What do we know? What don’t we know? (informed rationale)
- What is YOUR project? What are you seeking to answer?
- How do you DO your research? What kind of data do you collect? How do you collect it?
- What is the experimental design? Number of subjects or tests run? (quantify if you can!)
- Provide some data (not raw, but analyzed)
- What have you found? What are your results? How do you KNOW this – how did you analyze this?
- What does this mean?
- What are the next steps? What don’t we know still?
- How does this relate (again) to the bigger picture. Who should care and why? (what is your audience?)
More Resources
- Abstract Writing Presentation from University of Illinois – Chicago
- Sample Abstracts
- A 10-Step Guide to Make Your Research Paper More Effective
- Your Artist Statement: Explaining the Unexplainable
- How to Write an Artist Statement
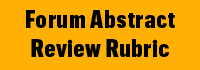
Here is the Forum Abstract Review Rubric for you and your mentor to use when writing your abstract to submit to the Spring Research & Creative Achievements Forum.

Magnum Proofreading Services
- Jake Magnum
- Jan 2, 2021
Writing an Abstract for a Research Paper: Guidelines, Examples, and Templates
There are six steps to writing a standard abstract. (1) Begin with a broad statement about your topic. Then, (2) state the problem or knowledge gap related to this topic that your study explores. After that, (3) describe what specific aspect of this problem you investigated, and (4) briefly explain how you went about doing this. After that, (5) describe the most meaningful outcome(s) of your study. Finally, (6) close your abstract by explaining the broad implication(s) of your findings.
In this article, I present step-by-step guidelines for writing an abstract for an academic paper. These guidelines are fo llowed by an example of a full abstract that follows these guidelines and a few fill-in-the-blank templates that you can use to write your own abstract.
Guidelines for Writing an Abstract
The basic structure of an abstract is illustrated below.
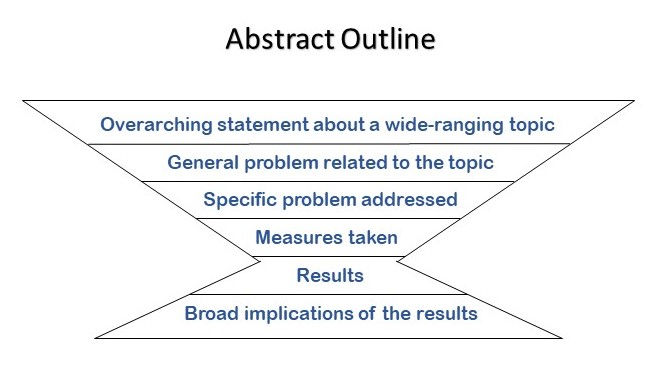
A standard abstract starts with a very general statement and becomes more specific with each sentence that follows until once again making a broad statement about the study’s implications at the end. Altogether, a standard abstract has six functions, which are described in detail below.
Start by making a broad statement about your topic.
The first sentence of your abstract should briefly describe a problem that is of interest to your readers. When writing this first sentence, you should think about who comprises your target audience and use terms that will appeal to this audience. If your opening sentence is too broad, it might lose the attention of potential readers because they will not know if your study is relevant to them.
Too broad : Maintaining an ideal workplace environment has a positive effect on employees.
The sentence above is so broad that it will not grab the reader’s attention. While it gives the reader some idea of the area of study, it doesn’t provide any details about the author’s topic within their research area. This can be fixed by inserting some keywords related to the topic (these are underlined in the revised example below).
Improved : Keeping the workplace environment at an ideal temperature positively affects the overall health of employees.
The revised sentence is much better, as it expresses two points about the research topic—namely, (i) what aspect of workplace environment was studied, (ii) what aspect of employees was observed. The mention of these aspects of the research will draw the attention of readers who are interested in them.
Describe the general problem that your paper addresses.
After describing your topic in the first sentence, you can then explain what aspect of this topic has motivated your research. Often, authors use this part of the abstract to describe the research gap that they identified and aimed to fill. These types of sentences are often characterized by the use of words such as “however,” “although,” “despite,” and so on.
However, a comprehensive understanding of how different workplace bullying experiences are associated with absenteeism is currently lacking.
The above example is typical of a sentence describing the problem that a study intends to tackle. The author has noticed that there is a gap in the research, and they briefly explain this gap here.
Although it has been established that quantity and quality of sleep can affect different types of task performance and personal health, the interactions between sleep habits and workplace behaviors have received very little attention.
The example above illustrates a case in which the author has accomplished two tasks with one sentence. The first part of the sentence (up until the comma) mentions the general topic that the research fits into, while the second part (after the comma) describes the general problem that the research addresses.
Express the specific problem investigated in your paper.
After describing the general problem that motivated your research, the next sentence should express the specific aspect of the problem that you investigated. Sentences of this type are often indicated by the use of phrases like “the purpose of this research is to,” “this paper is intended to,” or “this work aims to.”
Uninformative : However, a comprehensive understanding of how different workplace bullying experiences are associated with absenteeism is currently lacking. The present article aimed to provide new insights into the relationship between workplace bullying and absenteeism .
The second sentence in the above example is a mere rewording of the first sentence. As such, it adds nothing to the abstract. The second sentence should be more specific than the preceding one.
Improved : However, a comprehensive understanding of how different workplace bullying experiences are associated with absenteeism is currently lacking. The present article aimed to define various subtypes of workplace bullying and determine which subtypes tend to lead to absenteeism .
The second sentence of this passage is much more informative than in the previous example. This sentence lets the reader know exactly what they can expect from the full research article.
Explain how you attempted to resolve your study’s specific problem.
In this part of your abstract, you should attempt to describe your study’s methodology in one or two sentences. As such, you must be sure to include only the most important information about your method. At the same time, you must also be careful not to be too vague.
Too vague : We conducted multiple tests to examine changes in various factors related to well-being.
This description of the methodology is too vague. Instead of merely mentioning “tests” and “factors,” the author should note which specific tests were run and which factors were assessed.
Improved : Using data from BHIP completers, we conducted multiple one-way multivariate analyses of variance and follow-up univariate t-tests to examine changes in physical and mental health, stress, energy levels, social satisfaction, self-efficacy, and quality of life.
This sentence is very well-written. It packs a lot of specific information about the method into a single sentence. Also, it does not describe more details than are needed for an abstract.
Briefly tell the reader what you found by carrying out your study.
This is the most important part of the abstract—the other sentences in the abstract are there to explain why this one is relevant. When writing this sentence, imagine that someone has asked you, “What did you find in your research?” and that you need to answer them in one or two sentences.
Too vague : Consistently poor sleepers had more health risks and medical conditions than consistently optimal sleepers.
This sentence is okay, but it would be helpful to let the reader know which health risks and medical conditions were related to poor sleeping habits.
Improved : Consistently poor sleepers were more likely than consistently optimal sleepers to suffer from chronic abdominal pain, and they were at a higher risk for diabetes and heart disease.
This sentence is better, as the specific health conditions are named.
Finally, describe the major implication(s) of your study.
Most abstracts end with a short sentence that explains the main takeaway(s) that you want your audience to gain from reading your paper. Often, this sentence is addressed to people in power (e.g., employers, policymakers), and it recommends a course of action that such people should take based on the results.
Too broad : Employers may wish to make use of strategies that increase employee health.
This sentence is too broad to be useful. It does not give employers a starting point to implement a change.
Improved : Employers may wish to incorporate sleep education initiatives as part of their overall health and wellness strategies.
This sentence is better than the original, as it provides employers with a starting point—specifically, it invites employers to look up information on sleep education programs.
Abstract Example
The abstract produced here is from a paper published in Electronic Commerce Research and Applications . I have made slight alterations to the abstract so that this example fits the guidelines given in this article.
(1) Gamification can strengthen enjoyment and productivity in the workplace. (2) Despite this, research on gamification in the work context is still limited. (3) In this study, we investigated the effect of gamification on the workplace enjoyment and productivity of employees by comparing employees with leadership responsibilities to those without leadership responsibilities. (4) Work-related tasks were gamified using the habit-tracking game Habitica, and data from 114 employees were gathered using an online survey. (5) The results illustrated that employees without leadership responsibilities used work gamification as a trigger for self-motivation, whereas employees with leadership responsibilities used it to improve their health. (6) Work gamification positively affected work enjoyment for both types of employees and positively affected productivity for employees with leadership responsibilities. (7) Our results underline the importance of taking work-related variables into account when researching work gamification.
In Sentence (1), the author makes a broad statement about their topic. Notice how the nouns used (“gamification,” “enjoyment,” “productivity”) are quite general while still indicating the focus of the paper. The author uses Sentence (2) to very briefly state the problem that the research will address.
In Sentence (3), the author explains what specific aspects of the problem mentioned in Sentence (2) will be explored in the present work. Notice that the mention of leadership responsibilities makes Sentence (3) more specific than Sentence (2). Sentence (4) gets even more specific, naming the specific tools used to gather data and the number of participants.
Sentences (5) and (6) are similar, with each sentence describing one of the study’s main findings. Then, suddenly, the scope of the abstract becomes quite broad again in Sentence (7), which mentions “work-related variables” instead of a specific variable and “researching” instead of a specific kind of research.
Abstract Templates
Copy and paste any of the paragraphs below into a word processor. Then insert the appropriate information to produce an abstract for your research paper.
Template #1
Researchers have established that [Make a broad statement about your area of research.] . However, [Describe the knowledge gap that your paper addresses.] . The goal of this paper is to [Describe the purpose of your paper.] . The achieve this goal, we [Briefly explain your methodology.] . We found that [Indicate the main finding(s) of your study; you may need two sentences to do this.] . [Provide a broad implication of your results.] .
Template #2
It is well-understood that [Make a broad statement about your area of research.] . Despite this, [Describe the knowledge gap that your paper addresses.] . The current research aims to [Describe the purpose of your paper.] . To accomplish this, we [Briefly explain your methodology.] . It was discovered that [Indicate the main finding(s) of your study; you may need two sentences to do this.] . [Provide a broad implication of your results.] .
Template #3
Extensive research indicates that [Make a broad statement about your area of research.] . Nevertheless, [Describe the knowledge gap that your paper addresses.] . The present work is intended to [Describe the purpose of your paper.] . To this end, we [Briefly explain your methodology.] . The results revealed that [Indicate the main finding(s) of your study; you may need two sentences to do this.] . [Provide a broad implication of your results.] .
- How to Write an Abstract
Related Posts
How to Write a Research Paper in English: A Guide for Non-native Speakers
How to Write an Abstract Quickly
Using the Present Tense and Past Tense When Writing an Abstract
Well explained! I have given you a credit
Multi-Agent Reinforcement Learning for Energy Networks: Computational Challenges, Progress and Open Problems
The rapidly changing architecture and functionality of electrical networks and the increasing penetration of renewable and distributed energy resources have resulted in various technological and managerial challenges. These have rendered traditional centralized energy-market paradigms insufficient due to their inability to support the dynamic and evolving nature of the network. This survey explores how multi-agent reinforcement learning ( MARL ) can support the decentralization and decarbonization of energy networks and mitigate the associated challenges. This is achieved by specifying key computational challenges in managing energy networks, reviewing recent research progress on addressing them, and highlighting open challenges that may be addressed using MARL .
1 Introduction
Recent technological advancements have given rise to smart grids , electricity networks in which novel power generation, storage, and information technologies are used to monitor and manage the production, consumption, and transmission of electricity within an electrical network Nair et al. ( 2018 ); Charbonnier et al. ( 2022a ); Zhu et al. ( 2023 ); Capper et al. ( 2022b ) . Together with their potential to increase efficiency, reduce resource waste and costs, and maximize the transparency and reliability of energy supply, these technological advancements have resulted in the electrical grid’s rapidly changing architecture and functionality and have given rise to a wide range of technical and managerial challenges.
Among the key challenges is the increasing penetration of renewable energy sources ( RES ) such as solar and wind energies, which increased in volume in the last several years with the acceleration of photovoltaic ( PV )solar panels installation in private properties, and the rapidly increasing market of electrical vehicles ( EV ), which changed the demand and distribution patterns within the network and have introduced many sources of uncertainty since their power output is uncertain (hard to predict), intermittent (exhibits large fluctuations), and largely uncontrollable (not dispatchable on command) Kamboj et al. ( 2011 ); Hu et al. ( 2015 ) .
The decentralization of supply and demand raises the need to find novel ways to manage the electrical grid at two highly correlated levels of abstraction. The first focuses on maintaining the grid’s electrical quality and stability. This requires simultaneously considering a large number of consumers and producers and maintaining the supply-demand balance at fast time-scales and managing frequency, voltage, and power flow limits throughout the system. The second deals with the management and regulation of electrical markets in which various producers and consumers trade electricity.
From a computational perspective, the grid’s transition to a decentralized architecture has a tremendous effect on its management and decision-making and renders the traditional centralized methods insufficient Fähnrich et al. ( 2015 ); Roche et al. ( 2010 ) . Even if all components of the system could be controlled by one entity, centralized decision-making is highly inefficient since it requires a large spread of metering devices that continuously communicate their measurements to the centralized controller and requires high-volume data flow in order to support optimal decision-making. Moreover, due to the scale and complexity of the network, traditional methods for examining the grid’s stability become intractable.
We review methods for replacing centralized control with distributed and hierarchical models in which autonomous and semi-autonomous agents represent system components. We focus on multi-agent reinforcement learning ( MARL ) Albrecht et al. ( 2024 ) in which multiple agents learn from their interaction with the environment and with one another while aiming to maximize their expected utilities. As opposed to many common virtual MARL settings, the challenge here is in optimizing the behaviors of grid agents while considering the constraints and optimization considerations imposed by the physical electrical network.

While several surveys review the use of MARL for energy networks (e.g., Mahela et al. ( 2020 ); Charbonnier et al. ( 2022a ); Schwidtal et al. ( 2023 ); Zhu et al. ( 2023 ); Capper et al. ( 2022a ) ), this is, to the best of our knowledge, the first that is aimed at AI researchers and at highlighting the challenges they can help address. For this, we specify some of the key computational challenges of modern energy network management (Section 2 ), provide an overview of some of the current MARL methods for solving them (Section 3 ) and specify open challenges yet to be addressed toward the successful integration of MARL for energy networks (Section 4 ).
2 Key Computational Challenges in Energy Network Management
Traditionally, each entity in the electrical network had a single functionality and the network was centrally managed and controlled Zhu et al. ( 2023 ) . The recent introduction of new production and storage technologies and the emergence of new and uncertain consumption patterns have led to the grid’s decentralization and have given rise to many computational challenges in the optimization of its operation at different scales. We aim to highlight some of the key challenges that are especially suitable to be addressed using MARL.
We classify the presented problems using three main categories (see Figure 1 ). Section 2.1 considers the point of view of a single electrical component, that may either represent a single or set of devices (e.g., household) that interact with the network as one entity to optimize consumption, storage, and production. Section 2.2 considers a network manager and its need to control the electrical grid to maintain its stability. Section 2.3 broadly considers the management of the different energy markets in which energy and flexibility services are traded among different utility-maximizing entities.
We note that categorizing the challenges of energy network management is not straightforward. Many issues are interconnected and their solution is often combined. For example, ensuring that the physical system is stable requires predicting the energy consumption of various users, adjusting production, and managing electricity prices. However, once prices are set, they affect consumption, which may cause instability and raise the need to readjust the system, and so on. With modern RES s and local storage units, maintaining market and network stability becomes even more challenging.
2.1 Grid Edge Management ( GEM )
Grid Edge Management ( GEM )considers energy usage of grid-edge entities. Within this realm, recent technologies have created a major shift in the management of energy consumption within a household, referred to in the literature as Home Energy Management ( HEM ). As opposed to traditional homes which were passive consumers that only included energy-consuming appliances such as lighting appliances, washing machines, and air conditioners, modern homes have now become ‘prosumers’ – consumers who proactively manage their consumption, production and storage of energy. This includes managing heating and cooling systems, EV s, and local storage units. Thus, methods for managing a modern home or other grid-edge entities need to consider a combination of sensors, communication devices, and control algorithms that can monitor and control energy usage. The primary objective is to optimize energy consumption by minimizing cost (and sometimes carbon emission) while respecting usage requirements such as comfort.
An important characteristic of these settings is the electrical network and the details of its operation are typically abstracted and simplified. Instead, it is considered a part of the environment with which the grid-edge entities interact. Thus, for example, if a prosumer decides to turn on an appliance and increase its consumption of electricity it is typically assumed that this requirement can be satisfied and the network’s need to adjust to this new requirement is abstracted.
2.2 Power System Operation and Control ( PSOC )
Here we consider the set of activities and strategies employed to ensure the reliable, stable, and efficient operation of an electrical power network. Power in the network is divided into real power , or active power, which represents the actual energy transferred and consumed in an electrical circuit to perform ‘useful work’ (e.g., mechanical motion, heating, or light) and is the power that is bought and sold in electricity markets while reactive power , does not perform useful work but plays a crucial role in maintaining voltage levels in the electrical grid and in supporting its stability and efficiency.
To maintain system stability and reliability, efficient management of both real and reactive power is essential. Accordingly, Power System Operation and Control ( PSOC )includes various challenges that are related to both aspects including Load Balancing ( LB ), which focuses on the distribution of electrical loads across different generators and transmission lines such that no individual component is overloaded, Power Flow ( PF ), which deals with the analysis of the steady-state behavior of a power system and the distribution of real power within the network, Volt/Var Control ( VVC )which is related to the regulation of reactive power and is aimed at optimizing power quality, reducing energy losses, and ensuring grid stability, Frequency Control ( FC )which involves maintaining the power system frequency at the standard value by adjusting the generation and load, and more.
A key barrier to the integration of DER s to the electrical grid is the challenges they pose on the operation and control of the system. This is due to their intermittent and variable generation patterns, which can lead to uncertainty in power supply and unexpected effects on voltage and frequency levels and their requirement for bidirectional power flow from and to the distribution network. Moreover, although DER s can locally supply energy during a grid outage, their integration impacts the traditional protective devices and stability mechanisms designed for traditional power generation and poses safety risks that may hinder restoration efforts.
The effective integration of DER requires novel control strategies to manage the variability and intermittency and ensure grid stability, advanced methods for forecasting generation and demand, and new methods for the real-time monitoring and control of the network.
2.3 Electricity Market ( EM )
In an Electricity Market ( EM ), electricity is a commodity traded between participants that can generate, store, and consume electricity. These can be generally characterized as regulated markets , in which a single authority (e.g., a government) controls the different aspects of electricity generation, distribution, and pricing, and deregulated markets , or competitive markets , in which multiple electricity providers compete for consumers and market forces often determine prices.
The recent shift towards decentralized energy systems, in which communities of grid entities can satisfy more of their own energy needs from renewable energy generated from local sources and in which information technologies facilitate information flow Cremers et al. ( 2022 ) , has raised the need for novel structures of deregulated markets. Such markets include two levels of abstraction: wholesale and local.
In a wholesale market electricity is traded before being delivered to consumers and includes generators , which produce energy, and suppliers which are tasked with meeting consumer demands at any given time. Due to the uncertainty in supply and demand, several markets are typically maintained for different time horizons, i.e., Real-Time, Day-Ahead, etc Bose and Low ( 2019 ); Zhu et al. ( 2023 ) .
The basic setting is one in which the consumption profile of the various grid entities as well as their production and storage capabilities are fixed, and the objective is to maintain grid stability by equalizing supply and demand at all times while minimizing operational costs. This problem is typically studied as Unit Commitment ( UC )that considers which generating units in a power system should operate over a specific time horizon (typically, days) while considering factors such as generation limits, startup, and shutdown costs, minimum up and down times, and other operational constraints. Strongly related to UC is the problem of Economic Dispatch ( ED )which considers shorter horizons (typically real-time or several minutes) and the objective is to allocate the power output to meet the system demand at the lowest possible cost.
In more advanced settings, the reward-maximizing and strategic nature of grid agents is accounted for and it is possible to influence their behavior using monetary incentives and penalties. One relevant problem is Demand Response ( DR ), or demand-side management, which focuses on managing electricity demand by incentivizing customers to adjust their consumption in response to changing grid conditions. This involves designing incentives to encourage specific customer behaviors, as well as developing approaches for predicting demand patterns and coordinating customer responses. A typical goal is to promote stability by encouraging customers to reduce energy use during high-demand periods, such as hot summer days when air conditioning usage is high.
In contrast to wholesale markets which typically consider large entities, local energy markets consider smaller entities and the interaction between suppliers and end-users (residential and business consumers). Electricity traded in this market includes both that procured from generators in the wholesale electricity market and electricity generated by local generators Capper et al. ( 2022b ); Cremers et al. ( 2022 ) . Various models have been offered for organizing energy networks as transactive energy systems , in which economic and control mechanisms dictate the dynamics of the system using utility as a key operational parameter Cremers et al. ( 2022 ); Robu et al. ( 2012 ) . In general, these typically include a set of DERs, an interconnecting local network, an upstream energy market, and a digital coordination platform for sensing, communications, and control Charbonnier et al. ( 2022a ) .
An important and challenging characteristic of modern energy networks is that novel technologies enable interactions that can be used not only to support the local sharing of physical resources but also to support interactions of spatially separated entities (e.g., households in different cities) that are only connected by virtual agreements Robu et al. ( 2012 ) . Thus, local communities of anywhere between several units to several hundred houses (e.g. a village or a city neighborhood) can be formed to share energy assets, such as wind turbines or storage units, as well as the energy bill for the aggregate residual demand, i.e., the part of the demand not covered by the local generation and storage assets. At the same time, these technologies support the formation of virtual power plants by which dispersed generation and consumption units can form an aggregate that may even be large enough for participating in wholesale markets as large-scale producers and consumers. Here, finding optimal groupings within the large network and forming sustainable agreements becomes a computational challenge.
Another important challenge arises from the ability to exploit power flexibility as a commodity that can be traded Vázquez-Canteli et al. ( 2019 ) . Power flexibility can be regarded as the capability to reduce, increase, or shift electricity consumption or generation in response to an economic signal. This capability is gaining importance as a way to offset energy imbalances and help manage network constraints.
3 MARL for Energy Network Management
Many recent frameworks address the problems described in Section 2 using MARL , which is considered here as a form of ( RL ), wherein multiple agents learn to optimize their accumulated rewards while interacting with their partially known environment and with other agents. Due to space constraints, we exclude a detailed formal account of MARL and its solution methods and refer the reader to Albrecht et al. ( 2024 ) .
This general definition captures a variety of interactions and relationships that can exist between agents in collaborative, competitive, and mixed-incentive MARL settings. The complex interactions among agents may give rise to behaviors that are difficult to anticipate by simply examining each agent in isolation. Thus, despite the potential to solve complex problems across various domains, MARL faces various significant challenges Albrecht et al. ( 2024 ) . These stem from aspects such as scale, conflicting goals of self-interested agents, different partial views of the environment, the fact that agents are concurrently learning to optimize their policies while causing the environment to be non-stationary from the perspective of each agent, and the credit assignment challenge which involves determining which action and agent contributed to received rewards. All these are relevant to MARL settings in general but are particularly relevant to energy networks with the added need to account for the dynamics of the physical environment and the effect decisions may have on the functioning of the electricity network.
Hereon, we describe traditional ways for solving each of the challenges mentioned in Section 2 and how they can be modeled and solved using MARL . Notably, our account of related work is not comprehensive and is instead aimed at providing examples of research on MARL for energy systems and at setting the path to developing novel MARL methods for addressing the many unresolved challenges.
3.1 Grid Edge Management ( GEM )
Although GEM and HEM settings typically consider relatively small-scale settings, traditional methods that mostly rely on linear programming Üçtuğ and Yükseltan ( 2012 ); Amini et al. ( 2015 ) have become insufficient. This is because they are unable to account for the various forms of uncertainty related to the new technologies, such as the inability to accurately predict the generation patterns of PVs, and the need to adapt to dynamic pricing in contrast to traditional Time of Use (ToU) prices that are known in advance.
To achieve optimal performance and fully benefit from the domestic asset portfolio, GEM methods are required to consider long-horizons and to adapt and change their policy in real time. For example, the optimality of the decision of whether to store electricity in a local storage unit or sell it to the grid depends on a prediction of electricity prices and consumption volumes and on the ability to quickly adapt to unexpected events, such as major power failures.
GEM as MARL :
A common formulation for GEM and HEM considers a group of electrical entities within a grid-edge unit or household as strategic utility maximizing agents Fang et al. ( 2020 ); Charbonnier et al. ( 2022b ); Jendoubi and Bouffard ( 2023 ) . The action space 𝒜 i subscript 𝒜 𝑖 \mathcal{A}_{i} caligraphic_A start_POSTSUBSCRIPT italic_i end_POSTSUBSCRIPT of each appliance represents its ability to schedule and control the volume of power allocation for their appliance. The state space 𝒮 𝒮 \mathcal{S} caligraphic_S captures the set of power allocation schedules (e.g., electric vehicle charging, then operation the washing machine), the grid parameters (e.g., electricity price, carbon footprint, etc.), and environment and weather parameters (e.g., temperature, solar radiance, humidity, etc.). The reward function ℛ i subscript ℛ 𝑖 \mathcal{R}_{i} caligraphic_R start_POSTSUBSCRIPT italic_i end_POSTSUBSCRIPT of the i t h superscript 𝑖 𝑡 ℎ i^{th} italic_i start_POSTSUPERSCRIPT italic_t italic_h end_POSTSUPERSCRIPT appliance is typically given by:
where F i S ( s ) subscript superscript 𝐹 𝑆 𝑖 𝑠 F^{S}_{i}(s) italic_F start_POSTSUPERSCRIPT italic_S end_POSTSUPERSCRIPT start_POSTSUBSCRIPT italic_i end_POSTSUBSCRIPT ( italic_s ) refers to the user-defined satisfaction level at state s 𝑠 s italic_s (e.g., comfort), while F i C ( s ) superscript subscript 𝐹 𝑖 𝐶 𝑠 F_{i}^{C}(s) italic_F start_POSTSUBSCRIPT italic_i end_POSTSUBSCRIPT start_POSTSUPERSCRIPT italic_C end_POSTSUPERSCRIPT ( italic_s ) is the consumption cost at state s 𝑠 s italic_s , which depend on the functionalities of the different appliances. The objective is to identify an optimal control policy that maximizes the collective long-term return of all device agents within the shared environment.
Example Solution Approaches:
The GEM is a relatively small-scale setting that typically involves a single optimization objective of the grid-edge entity. For example, when used to optimize a household it includes a relatively small number of appliances with the single objective of minimizing the total utility expenses. This means that in principle such a setting can be solved by adopting a centralized fully observable model by which a single RL agent optimizes its policy defined over the joint action space of all appliances. However, since each electrical appliance may be associated with several sensors and there may be a need to consider many diverse parameters and optimization considerations (e.g., cost and carbon emission), it may become beneficial from a computational perspective to find hierarchical and decentralized control methods for such settings.
As an example, in Xu et al. ( 2020 ) a SG framework for HEM is offered which involves four types of agents that are responsible for controlling four distinct categories of appliances: non-shiftable , i.e., appliances that offer no flexibility in their operation schedules and for which demand must be met at all times (e.g., medical devices, light bulbs), power-shiftable , i.e., appliances that can adjust their power consumption (e.g., air conditioner), time-shiftable , i.e., appliances that can shift their time of operation but cannot be interrupted during their functioning (e.g., washing machine and dishwasher), and appliances that can be considered both time-shiftable and power-shiftable, including EV s for which both charging schedules and power levels can be controlled. With the expectation of non-shiftable appliances that do not have agency, each appliance is associated with an agent that determines the hour-ahead energy consumption of its appliance and an individual reward function that considers costs, dissatisfaction penalties (e.g, waiting time), etc. The suggested MARL method involves a shared neural-network-based learner (Feedforward NN) that predicts future electricity prices and solar generation and a decentralized Q-learning approach for optimizing the operation of each appliance.
As another example, the setting in Lee et al. ( 2020 ) involves multiple households equipped with a smart meter that can schedule appliances online, based on user tasks (e.g., laundry cycle) that appear according to some modeled distribution. The objective of each household is to optimize the individual accumulated reward which is a linear combination of the cost and the delay in performing the incoming tasks. The proposed SG formulation adopts a practical assumption that each household can only observe its own internal state along with the published electricity price at the previous time step. This supports scalability and privacy-preservation in a realistic setting. The solution approach is based on the commonly used Centralized Training with Decentralized Execution ( CTDE ) Lowe et al. ( 2017a ) approach by which agents jointly learn a shared critic that optimizes the Q-value that is associated with each state and action but adopt an individual actor that controls the executed policy.
3.2 Power System Operation and Control ( PSOC )
A key characteristic of the various challenges of Power System Operation and Control ( PSOC )is that they require accounting for various constraints imposed by the physical electrical networks. Accordingly, most traditional solution approaches rely on methods that can incorporate multiple constraints within the analysis and optimization process including numerical algorithms, linear and non-linear programming, and control theory. For example, the widely used methods for solving the PF equations are the Newton-Raphson and Gauss-Seidel methods which iteratively calculate bus voltages and phase angles to achieve a balanced system. Similarly, dynamic programming and Model Predictive Controllers (MPCs) are used for settings that require long-horizon decision-making such as optimal generation scheduling and energy storage management.
To account for the recent technological advancements and shifts in the network structure, new methods have been offered that apply different relaxations and heuristics and offer robust control techniques that can handle uncertainties and variations in power system parameters. For example, DC Power Flow (Linearized Power Flow) achieves computational efficiency by neglecting reactive power and assuming bus voltage magnitudes are bounded.
Another leading approach involves the decomposition of the power system into subsystems, allowing for parallel processing and computation. While these improve scalability and efficiency, they require careful handling of boundary conditions between subsystems. To demonstrate, a key aspect of VVC involves the control of capacitor banks and tap changers to keep the local voltage within an acceptable range. Such local decisions may have a sub-optimal effect on the rest of the network as adjusting the VAR value at one point of the electrical network can have negative impacts on the value at a neighboring point. Adding to this complexity in modern smart grids are the new devices and RES s connected to local distribution networks. While these can control their reactive power and participate in VVC they introduce many challenges due to their high generation and consumption uncertainty. This is added to the need to deal with scalability issues of controlling voltage in large networks, and the need to account for the locational interdependencies and frequent fluctuations that may occur unexpectedly, which is highlighted in modern electrical networks. All these have rendered the mostly rule-based traditional methods (e.g., Bob ( 2011 ); Bollen et al. ( 2015 ) ) insufficient.
A key strength of MARL models and methods for PSOC is their ability to support decentralized control strategies and effective distributed computations across different components or agents in the power system Gao et al. ( 2021 ) . Thus, even though PSOC does not typically involve strategic agents, such as in the energy markets, it is sensible to consider MARL as a way to achieve computational efficiently for problems that are challenging for conventional methods.
One such challenge is active voltage control (AVC) which refers to exploiting devices connected to an electrical network to regulate and maintain its voltage levels within desired limits. Voltage control problems have been studied for many years, but have recently come under the spotlight due to the increasing penetration of RES , and PV s in particular, that may cause voltage fluctuations. Since these new devices appear in vast numbers and are distributed across wide geographic areas, it is possible to exploit their control flexibility, together with the flexibility of other controllable devices, such as Static Var Compensators (SVCs) and On-Load Tap Changers (OLTCs) to regulate voltage throughout the network. Since AVC requires accounting for both local and global parameters and for the propagated influence decisions of nodes have on their connected nodes, and since it involves relatively less severe consequences in the case of failures and constraint violations, MARL is a suitable and promising solution approach Wang et al. ( 2021 ) .
3.2.1 PSOC as a SG
A typical model for various forms of PSOC analysis is a graph 𝒢 = ( 𝒱 , ℰ ) 𝒢 𝒱 ℰ \mathcal{G}=(\mathcal{V},\mathcal{E}) caligraphic_G = ( caligraphic_V , caligraphic_E ) , where the set of nodes 𝒱 𝒱 \mathcal{V} caligraphic_V represent the electrical components and the edges represent the relationships between them. For example, for VVC the nodes represent buses, i.e., components that facilitate the transfer of electrical energy between different components of the network, and edges ℰ ≜ 𝒱 × 𝒱 ≜ ℰ 𝒱 𝒱 \mathcal{E}\triangleq\mathcal{V}\times\mathcal{V} caligraphic_E ≜ caligraphic_V × caligraphic_V represent the connectivity between buses in a distribution network. The graph is also typically associated with an admittance matrix where 𝐘 N × N subscript 𝐘 𝑁 𝑁 \mathbf{Y}_{N\times N} bold_Y start_POSTSUBSCRIPT italic_N × italic_N end_POSTSUBSCRIPT represents the electrical current that can flow between nodes.
The network graph is used to represent the relationships between agents in a SG . As an example, in Wang et al. ( 2021 ) a Decentralized Partially-Observable MDP (Dec-POMDP) Bernstein et al. ( 2002 ); Oliehoek and Amato ( 2016 ) is offered for modeling and solving the AVC Problem for a network of PV s. A Dec-POMDP is a special case of a partially observable SG in which agents are collaborating to maximize a shared reward. In this representation, adopted from Gan et al. ( 2013 ) , the medium and low voltage distribution networks are modeled as a graph where the nodes 𝒱 𝒱 \mathcal{V} caligraphic_V are the PV s and the edges ℰ ℰ \mathcal{E} caligraphic_E are characterized by the active and reactive power injection formulas associated with the connection (i.e., transmission line) between them.
The SG formulation associates each node with an agent that controls the generation and absorption of reactive and active power, as dictated by its capacity and safety constraints. The state space represents the nodal features that are needed to compute the stable state of the network and include the set of active and reactive power of loads (consumed by the node), the active and reactive power generated by the PV s, and the voltage magnitude and phase. Observations include the current nodal state and possibly the state of the neighbors, assuming that each agent can communicate and share its local information with its neighbors defined by the network / communication graph G 𝐺 G italic_G .
To reduce computational complexity, the network can be separated into M 𝑀 M italic_M regions such that each agent can observe the nodes in its region. The objective is to control the voltage within a safety range while minimizing reactive power generation. Accordingly, the shared reward at time step t 𝑡 t italic_t is r t = − 1 | V | ∑ i ∈ V l v ( v i ) − α ⋅ l q ( q P V ) superscript 𝑟 𝑡 1 𝑉 subscript 𝑖 𝑉 subscript 𝑙 𝑣 subscript 𝑣 𝑖 ⋅ 𝛼 subscript 𝑙 𝑞 superscript q 𝑃 𝑉 r^{t}=-\frac{1}{|V|}\sum_{i\in V}l_{v}(v_{i})-\alpha\cdot l_{q}({\emph{q}^{PV})} italic_r start_POSTSUPERSCRIPT italic_t end_POSTSUPERSCRIPT = - divide start_ARG 1 end_ARG start_ARG | italic_V | end_ARG ∑ start_POSTSUBSCRIPT italic_i ∈ italic_V end_POSTSUBSCRIPT italic_l start_POSTSUBSCRIPT italic_v end_POSTSUBSCRIPT ( italic_v start_POSTSUBSCRIPT italic_i end_POSTSUBSCRIPT ) - italic_α ⋅ italic_l start_POSTSUBSCRIPT italic_q end_POSTSUBSCRIPT ( q start_POSTSUPERSCRIPT italic_P italic_V end_POSTSUPERSCRIPT ) where l v ( ⋅ ) subscript 𝑙 𝑣 ⋅ l_{v}(\cdot) italic_l start_POSTSUBSCRIPT italic_v end_POSTSUBSCRIPT ( ⋅ ) represents the violation of voltage constraints and l q subscript 𝑙 𝑞 l_{q} italic_l start_POSTSUBSCRIPT italic_q end_POSTSUBSCRIPT represents the reactive power generation loss.
Variations of this model include an individual reward function for each agent that is locally computed based on the local measurements of the node and the neighboring nodes Gao et al. ( 2021 ) . Another variation uses a Constrained MDP (CMDP) that associates a cost to constraint violations and sets a budget that maintains nodal voltage profiles within a desirable range Wang et al. ( 2019 ) .
3.2.2 Solution Approaches
A prominent paradigm in MARL is that of Centralized Training Decentralized Execution (CTDE) Lowe et al. ( 2017b ) by which agents expedite training by sharing their insights gained during training, i.e., sharing their leaned value functions, but operating according to individual policies. This approach is particularly relevant to PSOC settings in which agents are locally deployed, but their experiences and learned policies are relevant to nodes across the network. Accordingly, many PSOC frameworks adopt CTDE Hu et al. ( 2022 ); Chen et al. ( 2022 ); Liu and Wu ( 2021 ); Liu et al. ( 2021 ); Gao et al. ( 2021 ); Cao et al. ( 2021 ) . The key differences between the approaches lie in the way the physical constraints are accounted for, the way scale is addressed, and the information that is assumed to be available for decision-making.
For example, in Hu et al. ( 2022 ) , the authors address the dual problem of PF and VVC at two different time scales. At a slower rate, PF is resolved using a mixed-integer nonlinear programming method, while a model-free MARL approach works at high rate and aims to minimize voltage deviations of inverters and charging stations in the network. In Chen et al. ( 2022 ) a physics-shielding mechanism penalizes agents for selecting actions that do not conform to a set of constraints. As the set of constraints is global, the centralized training parameters are shared between agents by sharing the learned critic that maintains the action values and is used to regulate the training process. In Liu et al. ( 2021 ) the network is partitioned into distinct, interconnected subnetworks, where each subnetwork is given agency over all of its photovoltaic ( PV )reactive power values. The reward for each agent in the environment is defined locally as the weighted sum of power loss and voltage violation of all the buses in the subnetwork.
3.3 Electricity Markets
As opposed to traditional regulated markets where the role of each participant was distinct and fixed, modern energy market structures need to account for the dynamic nature of the market in which agents can control their consumption, production, and storage profiles in response to market signals and can interact with other agents and affect the grid’s dynamics Bose and Low ( 2019 ); Pinson ( 2023 ); Capper et al. ( 2022a ); Charbonnier et al. ( 2022a ) .
Most traditional formulations of energy market problems such as UC , ED and DR were adequate for centralized control of generation units and for unidirectional energy flow from transition to distribution. Solution methods for these problems typically rely on optimization methods such as mixed-integer linear programming (MILP) that are solved together with an interactive examination of the feasibility of the result using PSOC methods Saravanan et al. ( 2013 ); Zheng et al. ( 2014 ) . With the emergence of novel smart grid structures and technologies that allow bidirectional energy flow and with the integration of active devices at the distribution leves, such methods are no longer sufficient.
Optimizing modern deregulated energy markets requires considering two perspectives. On the one hand, mechanism design is needed for the formation of economic mechanisms and protocols by which network agents can interact Rosenschein and Zlotkin ( 1994 ); Wooldridge ( 2009 ); Shoham and Leyton-Brown ( 2008 ); Leyton-Brown and Shoham ( 2022 ) . This involves mechanisms such as auctions and monetary incentives to induce individual self-interested behaviors that maximize some global objective. On the other hand, there is a need to optimize the policies of the network agents given the underlying structure and mechanisms of interactions.
Importantly, unlike traditional marketplaces in which a key challenge is the predication of the behavior of strategic agents, here there is the additional need to account for the high number of heterogeneous participants and the various constraints imposed by the physics of the underlying electrical network and their impact on the market. Moreover, with the recent shift towards decentralized energy systems and the new information technologies, small-scale communities can coordinate their operation and satisfy more of their energy needs from local resources Cremers et al. ( 2022 ) . This raises the need to support various possibilities of local energy trading. However, despite various attempts at supporting these interactions, many governmental initiatives have failed due to the inability to support these highly dynamic and complex settings Schwidtal et al. ( 2023 ); Capper et al. ( 2022b ) . Our focus is on harnessing MARL to achieve computational savings via distributed computations and to account for complex systems with strategic agents.
3.3.1 EM as a SG
Energy markets have many different forms and consider different optimization challenges: some consider network constraints while others rely on a post-process analysis to examine whether violations occur, some account for the cooperative and competitive nature of grid agents and their ability to adapt to the market dynamics while others use a fixed behavior (e.g., fixed consumption profile). This variety yielded many ways to model EM s as SG s Zhu et al. ( 2023 ) .
Within this variety, many market settings, including UC , DR and ED , can be modeled using two types of agents: a Grid Manager ( GM )that is responsible for managing the network and its markets and typically aims to maintain the network’s stability while minimizing operation costs, and Grid Agent ( GA )s that represent market participants that aim to optimize their rewards while considering user-specific constraints and preferences (e.g., comfort, degradation costs, etc.).
Assumption that control of generation is centralized, these settings can be solved in principle using a single-agent MDP . However, even in such settings, it is often helpful to consider a decentralized formulation such as a Dec-POMDP Bernstein et al. ( 2002 ); Oliehoek and Amato ( 2016 ) to achieve distributed computation by representing each generation unit as a reward-maximizing agent. The challenge becomes finding a formulation that minimizes the information shared by agents to achieve optimal performance. In decentralized settings with self-interested agents modeled as a SG there is the extra need to guarantee that the interaction mechanisms are incentive-compatible in that they induce truthful reports by agents and that they achieve stability and social welfare Shoham and Leyton-Brown ( 2008 ); Leyton-Brown and Shoham ( 2022 ) . This trade-off between local and global objectives is at the heart of MARL and multi-agent AI research.
3.3.2 Solution Approaches
In general, it is challenging to design RL and MARL solutions for problems that involve non-stationary and partially observable environments with self-interested agents Hu et al. ( 2023 ) . This is especially true for energy networks where demand and supply are hard to predict, there are many coupling constraints to consider, and the system is distributed. Thus, while this research area is on the rise Zhu et al. ( 2023 ) , there is currently limited work on using MARL for solving many of the EM problems and there are many open challenges for the integration of MARL for these problems.
One recent example is Charbonnier et al. ( 2022b ) which presents a distributed RL approach where agents collaborate (and share information) to regulate their consumption and optimize costs. Another example is the use of distributed training with distributed execution (DTDE) approach in which agents jointly estimate the total power demand using a distributed communication protocol, locally decide their power generation value, and use another distributed computation to assess the total cost, which serves as the reward signal in the learning process Hu et al. ( 2023 ) . While this trend is on the rise Zhu et al. ( 2023 ) , there are many open challenges for the integration of MARL for these problems.
4 Research Gaps and Open Challenges
To promote the use of MARL for energy networks we highlight the following research gaps that should be addressed.
Gap 1 – Inconsistent and non-unified energy network problem definitions: While various frameworks have been suggested, they typically address a specific aspect of the system. This, together with the lack of standard definitions for energy networks and solution evaluation criteria, prevents the unified and standardized evaluation of proposed MARL approaches and hinders research progress.
Gap 2 – Limited robustness, scalability and generalisability of MARL solutions: While a range of MARL-based strategies for energy network management have been developed, it is unclear whether these will scale and perform well beyond the specific case studies they were trained for. One current limitation is the reliance on strong simplifying assumptions, such as that the environment is stationarity, whereas energy networks and markets are non-stationary and unpredictable. Also, evaluations of the suggested frameworks are typically done on small-scale settings which are far from realistic. Overcoming these challenges is key to the adoption of such methods by power system operators, especially given the stringent power sector reliability requirements.
Gap 3 – Limited real-world data: MARL methods typically require vast amounts of data and many environment interactions to train efficiently and to avoid any bias during training. However, a limited volume of data can be currently collected from real-world energy networks and used for training. This limits the generalisability and ability of the applied approaches to produce efficient policies, which is the specific advantage we seek in applying MARL to energy systems. This urges the need to find robust and scalable MARL solutions that work with limited data and to find ways to produce large volumes of high-quality data.
Gap 4 – Lack of standardized simulations. One way to address settings with limited data is by creating high-fidelity simulators that allow generating high-quality data. This is challenging in energy networks due to the need to account for both the strategic behavior of agents, as well as for the dynamics of the physical system. This is exacerbated by the integration of RESs and DERs that are highly uncertain and hard to predict and accurately model. Thus, while several recent MARL environments have been created to model certain aspects of grid management (e.g., Pigott et al. ( 2022 ); Vázquez-Canteli et al. ( 2019 ) ), they do not offer a unified perspective on the physical considerations of the network together with the management of its induced market dynamics.
5 Conclusion
Recent advancements in MARL research and the availability of cost-effective computing have promoted the application of MARL methods to the effective management of energy systems. While this has resulted in many publications over the last decade, we believe many of the potential contributions are yet to be explored. A key insight from our exploration is that most work so far has been performed by power system researchers who use existing and possibly non-optimal MARL frameworks to solve complex energy network problems. On the flip side, a key barrier for AI researchers to become involved in these problems is that they typically lack the necessary domain knowledge and expertise to fully understand the critical challenges of energy network management. We hope that our review here will facilitate future collaboration efforts between researchers from both fields and will help unlock the full potential of using MARL to promote more efficient and sustainable energy networks.
- Albrecht et al. [2024] Stefano V. Albrecht, Filippos Christianos, and Lukas Schäfer. Multi-Agent Reinforcement Learning: Foundations and Modern Approaches . MIT Press, 2024.
- Amini et al. [2015] MH Amini, Justin Frye, Marija D Ilić, and O Karabasoglu. Smart residential energy scheduling utilizing two stage mixed integer linear programming. In 2015 North American Power Symposium (NAPS) , pages 1–6. IEEE, 2015.
- Bernstein et al. [2002] Daniel S Bernstein, Robert Givan, Neil Immerman, and Shlomo Zilberstein. The complexity of decentralized control of markov decision processes. Mathematics of operations research , 27(4):819–840, 2002.
- Bob [2011] Uluski Bob. Volt/var control and optimization concepts and issues, 2011.
- Bollen et al. [2015] M Bollen, S Bharamirad, A Khodaei, J Meyer, R Langella, JF Hasler, F Zavoda, and J LIU. Volt-var control and power quality (cigre/cired c4.24). In International Conference on Electricity Distribution , 2015.
- Bose and Low [2019] Subhonmesh Bose and Steven H Low. Some emerging challenges in electricity markets. Smart grid control: Overview and research opportunities , 2019.
- Cao et al. [2021] Di Cao, Junbo Zhao, Weihao Hu, Fei Ding, Qi Huang, Zhe Chen, and Frede Blaabjerg. Data-Driven Multi-Agent Deep Reinforcement Learning for Distribution System Decentralized Voltage Control With High Penetration of PVs. 12(5), 2021.
- Capper et al. [2022a] Timothy Capper, Anna Gorbatcheva, Mustafa A Mustafa, Mohamed Bahloul, Jan Marc Schwidtal, Ruzanna Chitchyan, Merlinda Andoni, Valentin Robu, Mehdi Montakhabi, Ian J Scott, et al. Peer-to-peer, community self-consumption, and transactive energy: A systematic literature review of local energy market models. Renewable and Sustainable Energy Reviews , 2022.
- Capper et al. [2022b] Timothy Capper, Anna Gorbatcheva, Mustafa A. Mustafa, Mohamed Bahloul, Jan Marc Schwidtal, Ruzanna Chitchyan, Merlinda Andoni, Valentin Robu, Mehdi Montakhabi, Ian J. Scott, Christina Francis, Tanaka Mbavarira, Juan Manuel Espana, and Lynne Kiesling. Peer-to-peer, community self-consumption, and transactive energy: A systematic literature review of local energy market models. Renewable and Sustainable Energy Reviews , 2022.
- Charbonnier et al. [2022a] Flora Charbonnier, Thomas Morstyn, and Malcolm D. McCulloch. Coordination of resources at the edge of the electricity grid: Systematic review and taxonomy. Applied Energy , 318:119188, 2022.
- Charbonnier et al. [2022b] Flora Charbonnier, Thomas Morstyn, and Malcolm D. McCulloch. Scalable multi-agent reinforcement learning for distributed control of residential energy flexibility. Applied Energy , May 2022.
- Chen et al. [2022] Pengcheng Chen, Shichao Liu, Xiaozhe Wang, and Innocent Kamwa. Physics-Shielded Multi-Agent Deep Reinforcement Learning for Safe Active Voltage Control with Photovoltaic/Battery Energy Storage Systems. 2022.
- Cremers et al. [2022] Sho Cremers, Valentin Robu, Daan Hofman, Titus Naber, Kawin Zheng, and Sonam Norbu. Efficient methods for approximating the shapley value for asset sharing in energy communities. In ACM International Conference on Future Energy Systems , 2022.
- Fähnrich et al. [2015] Klaus-Peter Fähnrich, Stefan Kühne, and Axel Hummel. Multi-agent-based simulation of decentralized energy systems. In International Conference on Green Materials and Environmental Engineering; Advances in Engineering Research , 2015.
- Fang et al. [2020] Xiaohan Fang, Jinkuan Wang, Chunhui Yin, Yinghua Han, and Qiang Zhao. Multiagent Reinforcement Learning With Learning Automata for Microgrid Energy Management and Decision Optimization. In Chinese Control And Decision Conference (CCDC) , 2020.
- Gan et al. [2013] Lingwen Gan, Na Li, Ufuk Topcu, and Steven H Low. Optimal power flow in tree networks. In 52nd IEEE Conference on Decision and Control . IEEE, 2013.
- Gao et al. [2021] Yuanqi Gao, Wei Wang, and Nanpeng Yu. Consensus Multi-Agent Reinforcement Learning for Volt-VAR Control in Power Distribution Networks. 2021.
- Hansen et al. [2004] Eric A Hansen, Daniel S Bernstein, and Shlomo Zilberstein. Dynamic programming for partially observable stochastic games. In AAAI , 2004.
- Hu et al. [2015] Junjie Hu, Arshad Saleem, Shi You, Lars Nordström, Morten Lind, and Jacob Østergaard. A multi-agent system for distribution grid congestion management with electric vehicles. Engineering Applications of Artificial Intelligence , 38:45–58, 2015.
- Hu et al. [2022] Daner Hu, Zhenhui Ye, Yuanqi Gao, Zuzhao Ye, Yonggang Peng, and Nanpeng Yu. Multi-Agent Deep Reinforcement Learning for Voltage Control With Coordinated Active and Reactive Power Optimization. 2022.
- Hu et al. [2023] Chengfang Hu, Guanghui Wen, Shuai Wang, Junjie Fu, and Wenwu Yu. Distributed multiagent reinforcement learning with action networks for dynamic economic dispatch. 2023.
- Jendoubi and Bouffard [2023] Imen Jendoubi and François Bouffard. Multi-agent hierarchical reinforcement learning for energy management. Applied Energy , 332:120500, 2023.
- Kamboj et al. [2011] Sachin Kamboj, Willett Kempton, and Keith S Decker. Deploying power grid-integrated electric vehicles as a multi-agent system. In The 10th International Conference on Autonomous Agents and Multiagent Systems-Volume 1 , 2011.
- Lee et al. [2020] Joash Lee, Wenbo Wang, and Dusit Niyato. Demand-Side Scheduling Based on Multi-Agent Deep Actor-Critic Learning for Smart Grids. In 2020 IEEE International Conference on Communications, Control, and Computing Technologies for Smart Grids (SmartGridComm) , 2020.
- Leyton-Brown and Shoham [2022] Kevin Leyton-Brown and Yoav Shoham. Essentials of game theory: A concise multidisciplinary introduction . Springer Nature, 2022.
- Liu and Wu [2021] Haotian Liu and Wenchuan Wu. Online Multi-Agent Reinforcement Learning for Decentralized Inverter-Based Volt-VAR Control. 2021.
- Liu et al. [2021] Hangyue Liu, Cuo Zhang, Qingmian Chai, Ke Meng, Qinglai Guo, and Zhao Yang Dong. Robust Regional Coordination of Inverter-Based Volt/Var Control via Multi-Agent Deep Reinforcement Learning. 12(6), 2021.
- Lowe et al. [2017a] Ryan Lowe, Aviv Tamar, Jean Harb, OpenAI Pieter Abbeel, and Igor Mordatch. Multi-agent actor-critic for mixed cooperative-competitive environments. Advances in neural information processing systems , 30, 2017.
- Lowe et al. [2017b] Ryan Lowe, Yi Wu, Aviv Tamar, Jean Harb, Abbeel Pieter, and Igor Mordatch. Multi-Agent Actor-Critic for Mixed Cooperative-Competitive Environments. In Advances in Neural Information Processing Systems . Curran Associates, Inc., 2017.
- Mahela et al. [2020] Om Prakash Mahela, Mahdi Khosravy, Neeraj Gupta, Baseem Khan, Hassan Haes Alhelou, Rajendra Mahla, Nilesh Patel, and Pierluigi Siano. Comprehensive overview of multi-agent systems for controlling smart grids. CSEE Journal of Power and Energy Systems , 2020.
- Nair et al. [2018] Arun Sukumaran Nair, Tareq Hossen, Mitch Campion, Daisy Flora Selvaraj, Neena Goveas, Naima Kaabouch, and Prakash Ranganathan. Multi-agent systems for resource allocation and scheduling in a smart grid. Technology and Economics of Smart Grids and Sustainable Energy , 2018.
- Oliehoek and Amato [2016] Frans A Oliehoek and Christopher Amato. A concise introduction to decentralized POMDPs . Springer, 2016.
- Pigott et al. [2022] Aisling Pigott, Constance Crozier, Kyri Baker, and Zoltan Nagy. Gridlearn: Multiagent reinforcement learning for grid-aware building energy management. Electric Power Systems Research , 2022.
- Pinson [2023] Pierre Pinson. What may future electricity markets look like?, 2023.
- Robu et al. [2012] Valentin Robu, Ramachandra Kota, Georgios Chalkiadakis, Alex Rogers, and Nicholas Jennings. Cooperative virtual power plant formation using scoring rules. In The Conference of the Association for the Advancement of Artificial Intelligence (AAAI) , 2012.
- Roche et al. [2010] Robin Roche, Benjamin Blunier, Abdellatif Miraoui, Vincent Hilaire, and Abder Koukam. Multi-agent systems for grid energy management: A short review. In IEEE Industrial Electronics Society , 2010.
- Rosenschein and Zlotkin [1994] Jeffrey S Rosenschein and Gilad Zlotkin. Rules of encounter: designing conventions for automated negotiation among computers . MIT press, 1994.
- Saravanan et al. [2013] Balasubramanian Saravanan, Siddharth Das, Surbhi Sikri, and DP Kothari. A solution to the unit commitment problem—a review. Frontiers in Energy , 2013.
- Schwidtal et al. [2023] J.M. Schwidtal, P. Piccini, M. Troncia, R. Chitchyan, M. Montakhabi, C. Francis, A. Gorbatcheva, T. Capper, M.A. Mustafa, M. Andoni, V. Robu, M. Bahloul, I.J. Scott, T. Mbavarira, J.M. España, and L. Kiesling. Emerging business models in local energy markets: A systematic review of peer-to-peer, community self-consumption, and transactive energy models. Renewable and Sustainable Energy Reviews , 179, 2023.
- Shapley [1953] Lloyd S Shapley. Stochastic games. Proceedings of the national academy of sciences , 1953.
- Shoham and Leyton-Brown [2008] Yoav Shoham and Kevin Leyton-Brown. Multiagent systems: Algorithmic, game-theoretic, and logical foundations . Cambridge University Press, 2008.
- Üçtuğ and Yükseltan [2012] Fehmi Görkem Üçtuğ and Ergün Yükseltan. A linear programming approach to household energy conservation: Efficient allocation of budget. Energy and Buildings , 49, 2012.
- Vázquez-Canteli et al. [2019] José R. Vázquez-Canteli, Jérôme Kämpf, Gregor Henze, and Zoltan Nagy. Citylearn v1.0: An openai gym environmfent for demand response with deep reinforcement learning. Association for Computing Machinery, 2019.
- Wang et al. [2019] Wei Wang, Nanpeng Yu, Yuanqi Gao, and Jie Shi. Safe off-policy deep reinforcement learning algorithm for volt-var control in power distribution systems. IEEE Transactions on Smart Grid , 2019.
- Wang et al. [2021] Jianhong Wang, Wangkun Xu, Yunjie Gu, Wenbin Song, and Tim C Green. Multi-agent reinforcement learning for active voltage control on power distribution networks. Advances in Neural Information Processing Systems , 2021.
- Wooldridge [2009] Michael Wooldridge. An introduction to multiagent systems . John wiley & sons, 2009.
- Xu et al. [2020] Xu Xu, Youwei Jia, Yan Xu, Zhao Xu, Songjian Chai, and Chun Sing Lai. A Multi-Agent Reinforcement Learning-Based Data-Driven Method for Home Energy Management. 11(4), 2020.
- Zheng et al. [2014] Qipeng P Zheng, Jianhui Wang, and Andrew L Liu. Stochastic optimization for unit commitment—a review. IEEE Transactions on Power Systems , 30(4):1913–1924, 2014.
- Zhu et al. [2023] Ziqing Zhu, Ze Hu, Ka Wing Chan, Siqi Bu, Bin Zhou, and Shiwei Xia. Reinforcement learning in deregulated energy market: A comprehensive review. Applied Energy , 2023.
Thank you for visiting nature.com. You are using a browser version with limited support for CSS. To obtain the best experience, we recommend you use a more up to date browser (or turn off compatibility mode in Internet Explorer). In the meantime, to ensure continued support, we are displaying the site without styles and JavaScript.
- View all journals
- My Account Login
- Explore content
- About the journal
- Publish with us
- Sign up for alerts
- Open access
- Published: 30 April 2024
Microbiome confounders and quantitative profiling challenge predicted microbial targets in colorectal cancer development
- Raúl Y. Tito ORCID: orcid.org/0000-0001-9660-7621 1 , 2 na1 ,
- Sara Verbandt 3 na1 ,
- Marta Aguirre Vazquez 3 ,
- Leo Lahti ORCID: orcid.org/0000-0001-5537-637X 1 , 4 ,
- Chloe Verspecht 1 , 2 ,
- Verónica Lloréns-Rico 1 , 2 , 5 ,
- Sara Vieira-Silva ORCID: orcid.org/0000-0002-4616-7602 1 , 6 , 7 ,
- Janine Arts 8 ,
- Gwen Falony 1 , 2 , 6 ,
- Evelien Dekker 9 ,
- Joke Reumers ORCID: orcid.org/0000-0001-5434-6515 10 ,
- Sabine Tejpar ORCID: orcid.org/0000-0003-3281-8643 3 na1 &
- Jeroen Raes ORCID: orcid.org/0000-0002-1337-041X 1 , 2 na1
Nature Medicine ( 2024 ) Cite this article
Metrics details
- Colon cancer
- Diagnostic markers
Despite substantial progress in cancer microbiome research, recognized confounders and advances in absolute microbiome quantification remain underused; this raises concerns regarding potential spurious associations. Here we study the fecal microbiota of 589 patients at different colorectal cancer (CRC) stages and compare observations with up to 15 published studies (4,439 patients and controls total). Using quantitative microbiome profiling based on 16S ribosomal RNA amplicon sequencing, combined with rigorous confounder control, we identified transit time, fecal calprotectin (intestinal inflammation) and body mass index as primary microbial covariates, superseding variance explained by CRC diagnostic groups. Well-established microbiome CRC targets, such as Fusobacterium nucleatum , did not significantly associate with CRC diagnostic groups (healthy, adenoma and carcinoma) when controlling for these covariates. In contrast, the associations of Anaerococcus vaginalis , Dialister pneumosintes , Parvimonas micra , Peptostreptococcus anaerobius , Porphyromonas asaccharolytica and Prevotella intermedia remained robust, highlighting their future target potential. Finally, control individuals (age 22–80 years, mean 57.7 years, standard deviation 11.3) meeting criteria for colonoscopy (for example, through a positive fecal immunochemical test) but without colonic lesions are enriched for the dysbiotic Bacteroides2 enterotype, emphasizing uncertainties in defining healthy controls in cancer microbiome research. Together, these results indicate the importance of quantitative microbiome profiling and covariate control for biomarker identification in CRC microbiome studies.
Colorectal cancer (CRC) incidence is steadily increasing 1 , especially in people under 50 years 2 . It is estimated that approximately 16 and approximately 14 individuals per 100,000 people in the United States and Belgium, respectively, die every year from CRC 3 . As medical interventions can effectively reduce CRC progression and associated mortality, it is imperative to identify individuals at increased risk.
Colonoscopies with polypectomy of adenomas reduce up to 90% of CRC risk 4 . Early identification of individuals with polyps would reduce the global burden of CRC. Yet, ascertainment of patients at an increased risk remains challenging, highlighting the need for population-wide screening.
Microbiota shifts have been associated with a wide array of disease phenotypes 5 . Some bacterial markers, such as Fusobacterium , have been reported enriched in lesions and stools of patients with CRC 6 , 7 , 8 , 9 , 10 , 11 , 12 , 13 , 14 across developing and developed countries 15 , suggesting a potential role for microbiome-based diagnostics and/or prognostics.
Although microbiome profiles are affected by multiple variables that may confound or compound biological phenomena, covariate control is far from standard. For example, moisture content, a proxy for transit time, remains uncontrolled despite showing the biggest explanatory power for overall gut microbiota variation in multiple cohorts 16 , 17 . Intestinal inflammation, measured as fecal calprotectin 18 , 19 that reflects increased neutrophil shedding into the intestinal lumen 20 , is more sensitive than fecal occult blood for identifying patients with CRC 21 , thus a potential untapped target for molecular stool CRC-screening 19 .
Relative microbiome profiling (RMP, taxon abundances are expressed in percentages) remains the dominant approach in microbiome research. However, given issues with compositionality 22 and interpretation of relative profiles 23 , the use of experimental and quantitative approaches is increasingly recommended 23 , 24 , 25 . This reduces both false-positive and false-negative rates in downstream analyses, thereby lowering the risk of erroneous interpretation of microbiome associations, and allows focusing clinical programs on biologically relevant targets 25 . Although quantitative microbiome profiling (QMP) facilitates normalized comparisons across different samples or conditions 24 , 25 , so far, no QMP CRC microbiota studies were performed.
In this Article, we address these two gaps in CRC microbiota studies: (1) to quantitively characterize the microbiota profile associated with malignant colonic transformation and (2) to identify microbiota covariates that may obscure biological phenomena behind microbiota-CRC associations. To this end, we examined the microbial profiles of 589 Belgian patients from Universitair Ziekenhuis Leuven (UZL) who warranted colonoscopies based on clinical presentations, including patients with CRC, and compared these to existing published datasets (total n = 4,439 patients and controls). To the best of our knowledge, this is the first large scale study of the gut microbiota across colonic cancer developmental stages that combines QMP analysis with extensive analysis of microbiota covariates to disentangle disease-associated from confounder-based signals to identify taxa specifically associated with CRC.
Intestinal inflammation is higher in patients with colorectal tumors
We recruited 650 volunteers referred for colonoscopy and colonic resections at UZL between 2017 and 2018 who provided a stool sample before the colonic procedure. Most participants were from the Flemish region of Belgium. For this study, cancer developmental stages were defined as diagnosis groups, and we classified participants into three groups according to a thorough colonoscopy and clinical assessment: (1) patients without evidence of colonic lesions (CTLs, n = 205), (2) patients with polyps (considering polyps as a precancerous lesion; n < 10 and size between 6 and 10 mm) (ADE, n = 337) and (3) patients with CRC ( n = 47; 2 (4%) stage 0, 14 (30%) stage I, 13 (28%) stage II, 11 (23%) stage III, 3 (6%) stage IV and 4 (9%) of undetermined stage). We excluded patients outside these criteria, as well as those with insufficient clinical and molecular data. The final Leuven CRC Progression Microbiome (LCPM) study cohort consisted of 589 patients. The most frequent indications for colonoscopy were either a positive fecal immunochemical test (FIT) or adenoma surveillance. Other indications included familial risk, abdominal symptoms and change in bowel habits (Fig. 1a and Supplementary Table 1 ). The study was registered at clinicaltrials.gov (NCT02947607).
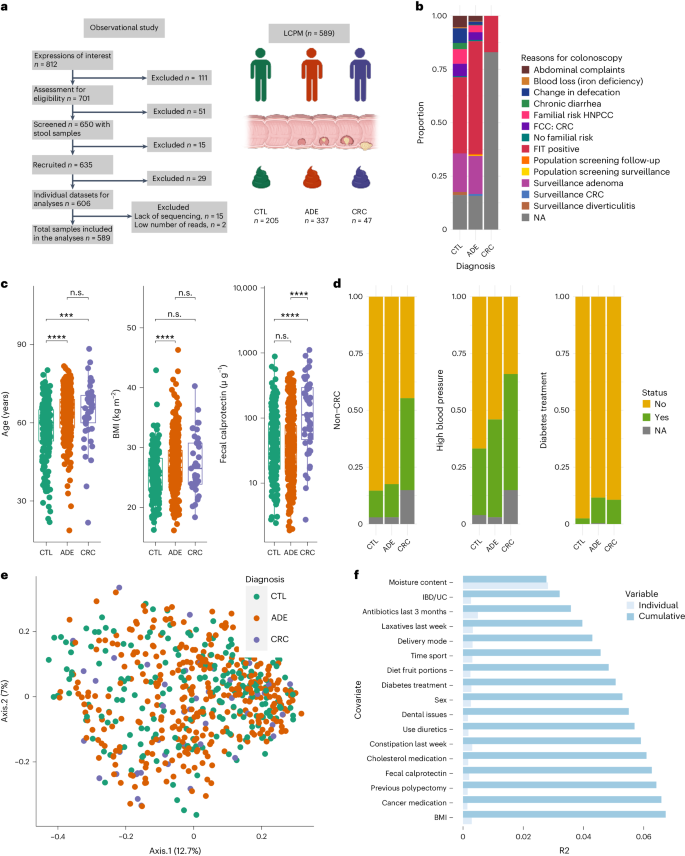
a , STROBE flowchart and cohort size. CTL represents patients without colonic lesions, ADE denotes patients with colonic polyps and CRC refers to patients with colorectal tumors (generated in BioRender.com ). b , Colonoscopy referral reasons for patients of the LCPM cohort: positive FIT, adenoma surveillance, familial risk cancer (FCC), hereditary nonpolyposis CRC (HNPCC) and changes in defecation. NA, denotes the proportion of patients without information. c , Age, BMI and calprotectin are associated with diagnosis groups. The patients without lesions were younger ( n = 589, two-sided KW test χ 2 = 35.77, adjusted P = 2.6 × 10 −7 ; phD tests) and had lower BMI ( n = 553, two-sided KW test χ 2 = 15.73, adjusted P = 1.9 × 10 −3 ; phD tests), while patients with tumors had higher fecal calprotectin levels ( n = 583, two-sided KW test χ 2 = 29.43, adjusted P = 3.0 × 10 −6 ; phD tests, adjusted *** P <0.001, ** P <0.01, * P <0.05 and n.s., non-significant P > 0.05; Supplementary Table 3 ). The box plot center represents the median value whiskers extend from the quartiles to the last data point within 1.5 times of the interquartile range, with outliers beyond. d , Previous non-CRC cancer, high blood pressure and diabetes treatment are associated with the distribution of diagnosis groups. The patients with CRC have a higher proportion of previous cancer (47.5% versus 15.0 % and 12.1%, two-sided CS test, CV effect size of 0.24, χ 2 = 31.65, d.f. of 2, adjusted P = 1.98 × 10 −2 ) and high blood pressure (60.0% versus 44.3% and 30.5%, CV of 0.17, two-sided CS test, χ 2 = 16.55, d.f. of 2, adjusted P = 1.98 × 10 −2 ) while the CTL group has the lowest proportion of patients with diabetes treatment (2.4% versus 10.3 and 10.6, two-sided CV effect size of 0.15, CS test, χ 2 = 13.79, d.f. of 2, adjusted P = 1.98 × 10 −2 ). e , PCoA on BCD representing QMP species-level microbiota variation in the LCPM cohort ( n = 589), PCoA1 (Axis.1) and PCoA2 (Axis.2) respectively explained 12.7% and 7% of the variance. Each dot represents one sample, colored by assigned diagnosis group. f , Cumulative effect sizes of significant covariates on microbiota community variation (cumulative bars; stepwise dbRDA on BCD) as compared to individual effect sizes (R 2 ) assuming covariate independence in the LCPM cohort ( n = 589; Supplementary Table 5 ). UC, ulcerative colitis.
Source data
We collected an extensive set of 165 universal metadata variables (nonspecific for any of the three groups) from each participant. After curation, we excluded variables that were colinear (if Pearson | r | > 0.8, we kept the variable with fewer missing data) or had incomplete data collection (variables missing more than 20% of the values). The final set consisted of 95 high-quality variables (Supplementary Table 2 ).
To identify metadata variables associated with diagnosis groups, we applied two statistical approaches: (1) nonparametric Kruskal–Wallis (KW) test and its η 2 effect size (Supplementary Table 3 ) for all numerical variables and (2) chi-square (CS) tests and Cramer’s V effect size (CV) (Supplementary Table 4 ) for categorical variables, followed by the Benjamini–Hochberg method for multiple testing correction (adjusted P ). We found eight variables associated with diagnosis groups (false discovery rate <5%), namely: age, body mass index (BMI), calprotectin, reported hours of sleep, previous cancer (including CRC), dental status (complete, partial and so on), diabetes treatment and high blood pressure (Supplementary Tables 3 and 4 ). The CTL patients were younger ( n = 589, KW test, η 2 = 0.058, χ 2 = 35.77, adjusted P = 2.6 × 10 −7 ; post hoc Dunn (phD) tests, adjusted P < 0.05 for CTL versus ADE or CRC groups), had a lower BMI ( n = 553, KW test, η 2 = 0.023, χ 2 = 15.73, adjusted P = 1.9 × 10 −3 ; phD tests, adjusted P < 0.05 for CTL versus ADE) and reported fewer hours of sleep than participants from the other two diagnosis groups ( n = 557, KW test, η 2 = 0.019, χ 2 = 13.41, adjusted P = 4.6 × 10 −3 ; phD tests, adjusted P < 0.05 for CTL versus ADE; Fig. 1 ; see Supplementary Table 3 for full results). Moisture content, an important microbiota covariate 16 , was not significant across diagnosis groups ( n = 589, KW test, η 2 = −0.001, χ 2 = 1.32, adjusted P = 7.0 × 10 −1 ).
The calprotectin levels were positively associated with malignant transformation. The patients with CRC showed higher intestinal inflammation, measured by fecal calprotectin 18 , 26 (Fig. 1a and Supplementary Table 3 ). Specifically, CRC exhibited higher levels (219.42 µg g −1 , range 2.74–1,114.42, n = 47) compared to ADE (70.24 µg g −1 , range 1.87–487.21, n = 337) or CTL (73.25 µg g −1 , range 2.42–884.82, n = 202) (Fig. 1a , N = 583, KW test, η 2 = 0.047, χ 2 = 29.43, adjusted P = 3.0 × 10 −6 ; phD tests, adjusted P < 0.05 for CRC versus CTL and CRC versus ADE). We also observed increased fecal calprotectin in patients reporting previous cancers (primarily breast and prostate cancer) (Wilcoxon ranksum (WR) test, W = 11,067, adjusted P = 4.1 × 10 −3 ), consumption of cancer medication (WR test, W = 3,671, adjusted P < 0.05), heartburn complaints (WR test, W = 11,067, adjusted P = 1.0 × 10 −10 ) and lower dietary fiber (WR test, W = 20,964, adjusted P = 3.3 × 10 −2 ).
The history of chronic diseases was distinct across diagnosis groups. The patients with CRC showed higher proportions of previous non-CRC cancer (47.5% versus 15.0 % and 12.1%, CS test, CV of 0.24, χ 2 = 31.65, d.f. of 2, adjusted P = 1.98 × 10 −2 ) and high blood pressure (60.0% versus 44.3% and 30.5%, CS test, CV of 0.17, χ 2 = 16.55, d.f. of 2, adjusted P = 1.98 × 10 −2 ) (Fig. 1b and Supplementary Table 4 ). The CTL group had the lowest diabetes treatment (2.4% versus 10.3% and 10.6%, CS test, CV of 0.15, χ 2 = 13.79, d.f. of 2, adjusted P = 1.98 × 10 −2 ) (Fig. 1b and Supplementary Table 4 ) and mostly complete dental sets (53.3% versus 35.2% and 32.5%, CS test, CV of 0.03, χ 2 = 30.78, d.f. of 10, adjusted P = 1.98 × 10 −2 ) (Supplementary Table 4 ).
Known confounders, not diagnosis groups, explain overall microbiota variation across CRC developmental stages
The influence of microbiota covariates and the quantitative amplitude of observed microbiota shifts are understudied in CRC. We combined sequencing data with flow cytometry measurements of fecal microbial load 23 to generate QMP data from our study cohort. 23 We studied the QMP variation in the context of the 94 potential covariates mentioned above (the 95th being microbial load) using established procedures 17 .
A principal coordinate analysis (PCoA; Fig. 1c ) on a species-level Bray–Curtis dissimilarity (BCD) matrix revealed no significant separation between diagnosis groups. Furthermore, no difference in total microbial load was found between groups ( n = 589, KW test, χ 2 = 0.68, adjusted P = 8.2 × 10 −1 ). Distance-based redundancy analysis (dbRDA) revealed 24 microbiota covariates associated with microbial variation in this cohort (Fig. 1d and Supplementary Table 5 ). We identified 17 nonredundant covariates that jointly explained 6.7% of microbiota compositional variation (Supplementary Table 5 ).
Consistent with previous reports 16 , 17 , moisture content exhibited the highest explanatory value (2.8%) of all covariates ( n = 589, stepwise dbRDA, R 2 = 2.8%, adjusted P = 2 × 10 −3 ). Intestinal bowel disease/ulcerative colitis (IBD/UC) status, a CRC-risk factor, possibly associated with its microbial dysbiotic community and intestinal inflammation 27 , was the second largest covariate. IBD/UC explained 0.4% of the microbiota variation ( n = 569, stepwise dbRDA, R 2 = 0.4%, adjusted P = 2 × 10 −3 ). Other top microbiota covariates included antibiotics and laxatives use (Fig. 1d ). Delivery mode (cesarean or natural birth) explained 0.3% variation ( n = 533, stepwise dbRDA, R 2 = 0.3%, adjusted P = 2 ×10 −3 ), although it is probably confounded by diet in this cohort (proportion of dietary vegetables; CS test, χ 2 = 33.09, d.f. of 14, P = 2.8 × 10 −3 , adjusted P < 0.05). Intestinal inflammation (fecal calprotectin) explained 0.2% ( n = 583, stepwise dbRDA, R 2 = 0.2%, adjusted P = 2.6 × 10 −2 ). In contrast with our previous study in the Flemish population (Flemish Gut Flora Project, FGFP) 17 , age did not explain microbiota variation ( n = 589, univariate dbRDA, R 2 = 0.2%, adjusted P = 5.9 × 10 −2 ). Surprisingly, the cancer diagnosis group (CTL, ADE and CRC), as a covariate, was not associated with microbial variation ( n = 589, univariate dbRDA, R 2 = 0.2%, adjusted P = 0.22; Supplementary Table 5 ).
Fusobacterium association with CRC stages disappears when controlling for confounders or when using QMP
Microbiota signals can be specific to taxonomic groups and, thus, not reflected in broad community shifts. While a multitude of microbial associations have been reported in CRC studies using RMP 6 , 7 , 8 , 13 , we used QMP to identify species whose absolute abundance associated with diagnosis groups. The comparisons were limited to the 138 species with a prevalence of greater than 5% in at least one of the diagnosis groups of the LCPM cohort (Supplementary Table 6 ). Only eight species showed significant differential abundance (absolute or relative) among diagnosis groups: Anaerococcus vaginalis ( Anaerococcus obesiensis ), Alistipes onderdonkii , Dialister pneumosintes , Fusobacterium nucleatum , Parvimonas micra , Peptostreptococcus anaerobius , Porphyromonas asaccharolytica and Prevotella intermedia (KW test, adjusted P < 0.05; Fig. 2a,b and Supplementary Table 7 ). While Fusobacterium nucleatum has been consistently associated with colorectal lesions across cohorts of diverse backgrounds 13 , 14 , in the LCPM cohort, Fusobacterium nucleatum absolute abundance was positively correlated with high fecal calprotectin levels (Spearman’s rank and Kendall’s tau correlations, adjusted P < 0.05; Fig. 2c , Extended Data Fig. 1 and Supplementary Table 8 ) and cancer progression (diagnosis groups) (KW test, η 2 = 0.010, adjusted P = 1.84 × 10 −5 ; phD test adjusted P = 8.80 × 10 −1 for CTL versus ADE, adjusted P = 3.84 × 10 −7 for CTL versus CRC and adjusted P = 3.84 × 10 −7 for ADE versus CRC; Fig. 2c and Supplementary Table 7 ). However, after deconfounding for calprotectin only or combined BMI, moisture content and calprotectin, and neither absolute nor relative Fusobacterium nucleatum abundance were associated with diagnosis (generalized linear model analysis of variance (ANOVA), n = 547, P > 0.05; Extended Data Fig. 2 ).
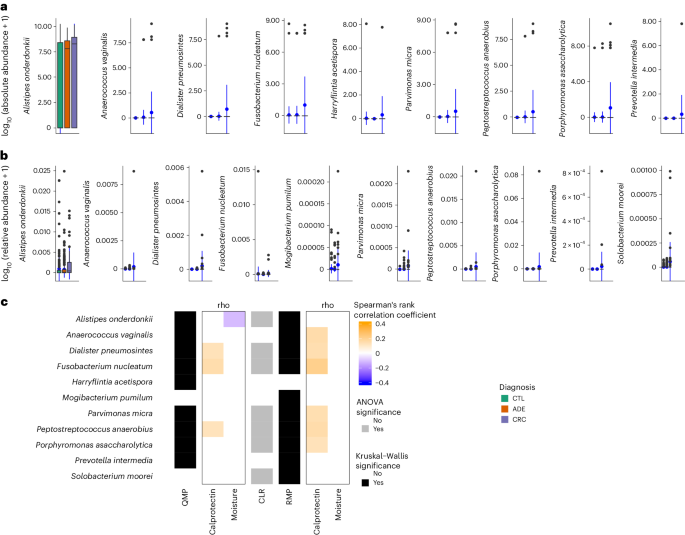
a , Nine species were identified with differential absolute abundance across diagnosis groups ( n = 589, KW test, adjusted P < 0.05; Supplementary Table 7 ). b , Ten species were identified with differential relative abundance across diagnosis groups ( n = 589, KW test, adjusted P < 0.05; Supplementary Table 7 ). The center of the box plot represents the median value of the data, and the whiskers extend from the quartiles to the last data point within 1.5 times of the interquartile range, with outliers beyond. The blue circles represent the mean. c , Biomarkers associations and their confounders. Species Spearman’s rank correlation with calprotectin levels and moisture proportions using QMP (first rho column panel) and RMP (second rho column panel) data. The effect size of the associations between species and calprotectin, moisture and diagnosis variables for QMP and RMP ( n = 589, Spearman’s rank correlation comparison, adjusted P < 0.05). Significant associations were tested using two-sided KW tests for QMP and RMP data and ANOVA for CLR data. The associations for Harryflintia acetispora , Parvimonas micra and Prevotella intermedia are sensitive to bias by the extreme values (absolute abundance) in the higher range. Removing these values leads to loss of significance. As rank-based approaches were used, it is not clear if this loss is due to the strength of the signal or the loss of power.
Multiple established CRC microbial markers are associated with transit time, intestinal inflammation and body mass index but not with CRC stages
The association of Fusobacterium abundance with fecal calprotectin urged us to investigate the influence of this confounder on previously reported CRC-associated genera, adding moisture content since it is the top microbiome covariate, and BMI, which showed differences among diagnosis groups.
To this end, we compiled a list of 89 CRC species-level markers from ten published cohorts 6 , 9 , 11 , 13 , 14 , 28 , 29 , 30 , 31 (including 1,633 samples) and 67 genera-level markers from 15 cohorts 6 , 7 , 8 , 9 , 11 , 12 , 13 , 14 , 15 , 28 , 29 , 30 , 31 , 32 (representing 4,439 samples). We used this compiled list of taxa as a criterion to test whether the CRC association of these taxa in our cohort is influenced by the target covariates. To reduce the impact of distinct statistical treatments, we downloaded the microbial profiles of nine out of ten studies at species level from the curated MetagenomicData 33 resource and analyzed them using the statistical component of our pipeline.
Spearman correlation between taxa abundances and the three focus covariates revealed strong associations between microbial targets and these confounders at the species (Extended Data Fig. 3a ) and genus level (Fig. 3b ). Most of these associations were replicated in an independent population cohort (FGFP), suggesting these associations are robust and not specifically linked to CRC (Extended Data Fig. 3 ). Moisture content, the known major covariate in microbiome studies 17 , is unsurprisingly associated with many taxa validated in both cohorts.
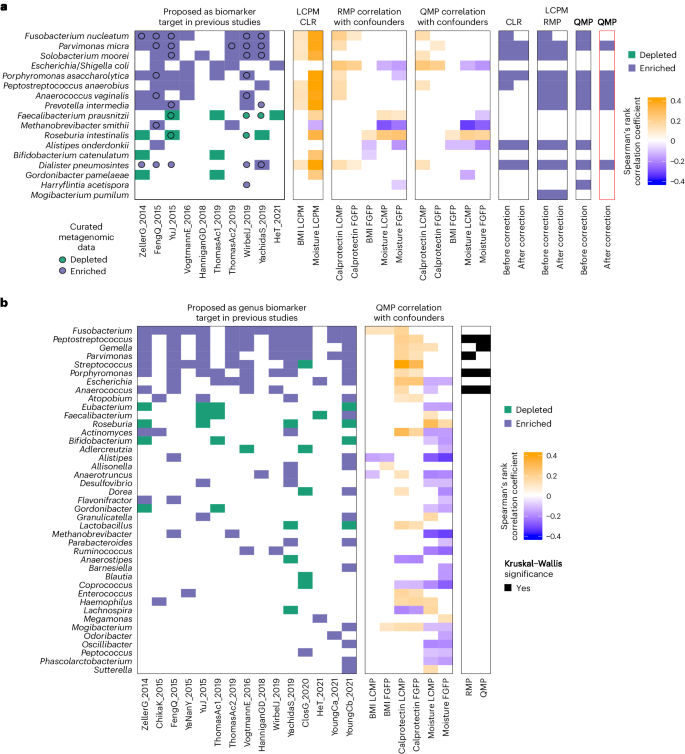
a , b , Species ( a ) and genera ( b ) previously reported in association with CRC (blue and green represent enrichment or depletion; the squares indicate reported in corresponding publications, while circles represent our reanalysis of the MetaPhlAn 3.0 profiles generated from the curatedMetagenomicData 33 of these cohorts using the statistical part of our pipeline). Graphic representation of Spearman’s rank correlation of pairwise analysis of fecal calprotectin, BMI, and moisture values against absolute species abundance (QMP) and RMP from the LCPM ( N = 589) and FGFP ( N = 1,045) cohorts (adjusted P < 0.05, Supplementary Table 8 ). The species enriched or depleted in relation to CRC diagnosis groups were tested using QMP, CLR and RMP data before ( n = 589, two-sided KW test and Spearman’s rank correlation comparison, adjusted P < 0.05) and after controlling for microbiota covariates (before adjustment for BMI, calprotectin and moisture; generalized linear model ANOVA, adjusted P < 0.05).
As we compiled the CRC-associated taxa from non-QMP studies, we conducted analyses using both RMP and QMP to assess whether confounder associations influence quantitative association of biomarkers or targets to diagnosis groups in LCPM. We found only 8% (6 out of 89) and 10% (9 out of 89) of species previously associated with CRC using QMP and RMP replicating after confounder control. Anaerococcus vaginalis , Dialister pneumosintes , Parvimonas micra , Peptostreptococcus anaerobius , Prevotella intermeia and Porphyromonas asaccharolytica , were identified by controlled QMP and RMP. Controlled QMP excluded Fusobacterium nucleatum and Alistipes onderdonkii , suggesting previous associations of these two species may be spurious (Fig. 3a ).
We identified eight species previously linked to CRC (that is, using QMP and/or RMP), including Fusobacterium nucleatum and Peptostreptococcus anaerobius , to be associated with inflammation (Fig. 3 and Supplementary Tables 8 and 9 ). This association was previously reported for only three out of the eight taxa above ( Escherichia , Fusobacterium and Streptococcus ) 24 . Further validation of this association was conducted using the FGFP (Extended Data Fig. 3 and Supplementary Tables 8 and 9 ).
Recognizing that inflammation is a risk factor, not a requirement, for CRC progression, we further investigated markers associated with diagnosis groups in relation to inflammatory status. To this end, we focused on a subset of 340 samples, which, regardless of their CRC status, exhibited normal levels of calprotectin (fecal calprotectin under 50 μg g −1 (ref. 34 )), indicating no evidence of local inflammation (112 CTL, 216 ADE and 12 CRC). Assessment of the 89 CRC species-level markers mentioned above confirmed that the association of three of the six replicating species ( Anaerococcus vaginalis , Prevotella intermedia and Porphyromonas asaccharolytica) is independent of intestinal inflammation (Supplementary Table 10 ).
Colonoscopy patients, with or without CRC, exhibit an excess of the Bacteroides2 enterotype
To study the LCPM cohort in a population context, we enterotyped participants using Dirichlet multinomial mixtures (DMM) on a genus matrix against the background of microbial variation as observed in the FGFP samples ( n = 1,045) 17 . Consistent with previous description of the Flemish population 23 , we identified four community types based on selecting the optimal number of clusters using the Bayesian Information Criterion (Fig. 4a,b and Extended Data Fig. 4 ), ‘Bacteroides1’ (Bact1), ‘Bacteroides2’ (Bact2), ‘Prevotella’ (Prev) and ‘Ruminococcaceae’ (Rum). The enterotype distribution was different between LCPM and FGFP (CS test, χ 2 = 34.3, d.f. of 3, adjusted P = 1.7 × 10 −7 ), but no differences were observed among diagnosis groups within the LCPM cohort (pairwise CS tests, adjusted P > 0.1). Pairwise comparisons of the prevalence of the dysbiotic Bact2 enterotype in the LCPM cohort diagnosis groups revealed that compared to the FGFP population, this enterotype was enriched in all CRC diagnosis groups (test of equal or given proportions, FGFP versus CTL: χ 2 = 15.09, d.f. of 1, adjusted P = 1.1 × 10 −4 ; FGFP versus ADE: χ 2 = 18.93, d.f. of 1, adjusted P = 2.4 × 10 −5 ; and FGFP versus CRC: χ 2 = 4.34, d.f. of 1, adjusted P = 3.4 × 10 −2 ). Although dysbiosis and CRC development were previously linked 13 , 35 , the high prevalence of this enterotype in the LCPM, even in samples from patients free of lesions, is unexpected. Consistent with previous reports 24 , 25 , the Bact2 enterotype in this group exhibited all hallmarks of dysbiosis: low cell count, low richness, higher calprotectin values, reduced butyrate producers and increased proinflammatory bacteria.
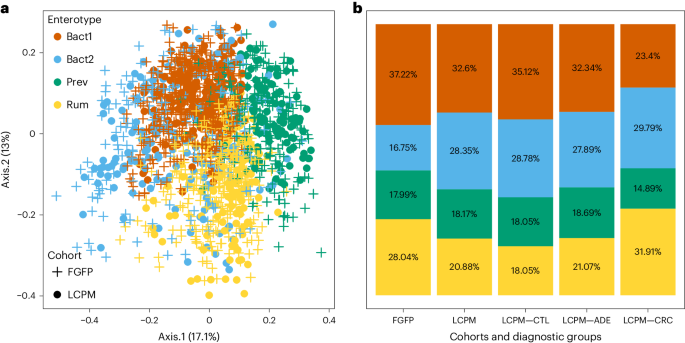
a , PCoA of interindividual differences (BCD) in relative microbiota profiles of the LCPM cohort ( n = 589 samples) using a cross-section of the Flemish population ( n = 1,045 samples) as a background dataset. PCoA1 (Axis.1) and PCoA2 (Axis.2) respectively explained 13% and 17.1% of the variance of microbiota at the genus level. b , Enterotype distribution across the FGFP, LCPM and LCPM diagnosis groups (CTL, ADE and CRC), increased prevalence of the Bact2 enterotype in the three groups from the LCPM cohort ( n = 589) as compared to FGFP samples ( n = 1,045); pairwise two-sided test of equal or given proportions ( P < 0.05).
Additional categorical variables appeared associated with the Bact2 enterotype. They included antibiotic consumption (CS test, χ 2 = 30.78, d.f. of 3, adjusted P = 2.1 × 10 −2 ), current treatment with anti-inflammatory medications (CS test, χ 2 = 30.78, d.f. of 3, adjusted P = 2.1 × 10 −2 ), diabetes treatment (CS test, χ 2 = 30.78, d.f. of 3, adjusted P = 3.3 × 10 −2 ), recent diarrhea (last week) (CS test, χ 2 = 30.78, d.f. of 3, adjusted P = 2.1 × 10 −2 ), history of gallstones (CS test, χ 2 = 30.78, d.f. of 3, adjusted P = 4.7 × 10 −2 ) and recent use of laxatives (last week) ( χ 2 = 30.78, d.f. of 3, adjusted P = 4.2 × 10 −2 ) (Supplementary Table 11 ).
While associations between the gut microbiota and CRC have been extensive, this is the first study using QMP and extensive metadata collection to systematically investigate microbiota covariates that potentially are masking or creating spurious associations between specific taxa and malignant transformation.
At first glance, this study yielded a gut microbial profile partially consistent with previous reports of CRC-associated taxa. Further analysis, however, suggested that many of the previously reported associations, including those of prominent biomarkers, such as Fusobacterium (nucleatum), are confounded by microbiota covariates. A total of 17 of 94 variables explained 6.7% of the observed variation. Of those, the moisture content had highest explanatory power (2.7%), greater than eight times that of the next covariate (IBD status). The explanatory power of fecal calprotectin was lower (0.2%) but significant; age and, most importantly, diagnosis groups were not.
Some associations were complex in nature. For example, BMI, consistent with previous reports, showed an association with both microbial composition 17 , 25 and cancer progression 36 , while others, such as age, suggested to modify the BMI-association with cancer progression 37 , were not significant in this cohort.
Inflammation is a known risk factor for CRC 38 , but its effect size in shaping the cancer-associated microbiota is yet to be described. Fecal calprotectin is a well-documented marker of intestinal local inflammation 39 , 40 and has been associated with cancer progression, probably having an effect on tumor development rather than on tumor initiation 41 . We observed participants with normal and elevated fecal calprotectin levels within each diagnosis group and covariate-controlled analysis of the LCPM cohort revealed that 8 and 19 CRC-associated markers, at the species and genus levels, respectively, associated with fecal calprotectin rather than with the diagnosis group. We replicated these observations in an independent cohort of apparently healthy individuals (FGFP).
High levels of fecal calprotectin have been associated with intestinal inflammatory pathologies 19 . However, when removing patients with IBD from our analysis, CRC diagnosis groups remained not significant, and the significance of Fusobacterium nucleatum , among other six species, was unaltered after differential abundance analysis. In patients with CRC, increased levels of fecal calprotectin (>50 µg g −1 stool 18 , 26 ) are directly associated with tumor presence, as the level decreases after tumor resection 42 . Here, fecal calprotectin was increased in CRC, consistent with previous associations between malignant transformation, local inflammation 43 and advanced tumor stages (T3 and T4) 42 . No difference in calprotectin levels was observed between CTL and ADE (mean 73.25 versus 70.24 µg g −1 ), suggesting that although no lesions are visible in the colon of the CTL group, they have a detectable level of local inflammation. The potential effect of local inflammation in shaping the colonic microbiota in the context of malignant transformation, or its potential confounding effect, remains largely obscure, as most studies surveying the association between gut microbiota and CRC, including meta-analysis 13 , 14 , do not control for local inflammation.
We argue that strict control of covariates is a must in any microbiota analysis assessing potential clinical associations, as for example, three of the species with repeated CRC association 11 , 13 , 14 , 28 , 29 , 30 , 32 , Escherichia coli , Fusobacterium nucleatun and Parvimonas micra , exhibit association with local inflammation, unfortunately uncontrolled for in previous studies, that may or may not be associated with cancer progression.
Fusobacterium nucleatum is one of the species that attracts more attention as there is a substantial body of work linking it to CRC 44 . In this study, Fusobacterium was enriched in patients with CRC. However, this apparent association disappears when the analysis is covariate controlled. Our study suggests that the association of Fusobacterium nucleatum to cancer may be driven by its association to intestinal inflammatory conditions; there are no differences in the abundance of Fusobacterium nucleatum across diagnostic groups once calprotectin is controlled for. These results suggest reassessment of the diagnostic utility of this marker. At the same time, our results do not mean that Fusobacterium nucleatum is not linked to CRC; they rather suggest that the reasons behind this association might be less straightforward than originally considered. They, thus, present a cautionary tale of the importance to control for covariates as the microbiome field moves forward. Given that inflammation is a risk factor for CRC but not a requirement 41 , potential use of Fusobacterium nucleatum as a marker of CRC development could fail to identify those cases of inflammation-independent cancer progression. While not yet commercialized, there are already publications proposing the use of microbial markers, including Fusobacterium nucleatum , for CRC screening 7 , 45 , which, in light of our results, raises concerns as uncontrolled variables may be obscuring actual biological mechanisms. We present evidence that purported CRC biomarkers, even those replicated in multiple studies, may suffer from the compounding or confounding effect of covariates, which in addition to the use of nonquantitative signals, may result in misleading conclusions on what diagnostic signals really mean—complicating the path towards potential clinical applications.
BMI, in combination or independent of inflammation, has been independently associated with changes in the gut microbiota 46 , which in turn are associated with increased risk of CRC 47 . Yet, microbial dysbiosis by itself does not explain the higher risk of colon cancer observed in the obese population 48 , indicating that the underlying process that associates obesity and CRC is more complex and demands further investigation.
Among four described gut enterotypes, the Bact2 enterotype is defined as a dysbiotic microbial profile 24 , 25 . Bact2 enrichment is observed in obesity 25 and in conditions such as PSC (Primary sclerosing cholangitis) and IBD 24 , further supporting the potential disease association of this enterotype. The analysis of the LCPM cohort revealed an excess of the Bact2 enterotype across all diagnosis subgroups, regardless of BMI.
Increased Bact2 prevalence in the no-lesions group compared to FGFP is particularly striking. While patients in the CTL group have no observable lesions, they may be considered at increased risk for colorectal perturbations based on clinical referrals (blood loss in the stool, familiar risk to colonic lesion and so on) that warranted colonoscopies—something that might also be reflected by their Bact2 enterotype. Of importance, ‘healthy’ biopsies included in CRC microbiome studies are often selected using colonoscopies with a negative result as the main criterium, posing a potential problem, as no other markers of colonic health are considered to qualify these healthy individuals. The reasons for the appearance of Bact2 in the no-lesion group are multifold, but these findings suggest that such individuals, while representing a useful category for biomarker discovery, may harbor an unhealthy gut ecosystem, from a microbial point of view.
There is a plethora of variables identified as modifiers of the gut microbiota. Yet, covariate control is far from standard and notably absent from most association studies. As intestinal microbial taxa are being nominated as potential biomarkers of malignant transformation, it is imperative to explore the influence of microbiota covariates as potential confounders or compounders of observed associations. Rather than denying previous associations, our analysis emphasizes the need for covariate-controlled analysis for any microbiota study aiming to establish clinical associations, as these covariates by themselves may explain most of the stool microbiota variation, independent of CRC status.
Out of the multiple taxa previously associated with CRC, six species remain significant after strict control of covariates in this quantitative cohort. Without denying other potential biomarkers, further studies are warranted on Anaerococcus vaginalis , Dialister pneumosintes , Parvimonas micra , Peptostreptococcus anaerobius , Prevotella intermedia and Porphyromonas asaccharolytica , as their reported association to CRC 6 , 7 is robust enough to remain independent of the method. Our data present a strong argument in favor of revisiting potential microbial associations with clinical phenotypes to ensure that the purported associations are not driven by uncontrolled covariates warranting further follow up of the mechanisms underlying these associations. Refining the approaches to discover microbial biomarkers will undoubtedly impact the microbiota field, facilitating the path towards the much-coveted clinical applications.
Limitations
We aim to identify taxa associated with malignant colonic transformation. While our cohort includes a set of participants without lesions, we make no claim that these are healthy controls, as there is an apparent increased incidence of gut dysbiosis in this group. Considering that all participants in this study had a medical need for a colonoscopy, there is an implicit increased risk to CRC. Thus, the present study cannot rule out that the group without polyps is undergoing potential molecular or cellular changes that are not detectable via colonoscopy. In addition, as this is a cross-sectional study, the term cancer progression is an extrapolation of what is seen at cancer development stages (operationalized here as diagnosis groups). We cannot rule out potential particularities of our cohort that may be contributing to our observations, as most studies do not report sufficient metadata for us to compare across cohorts. It is important to consider that certain taxonomic groups may not even be represented in current databases, and specific microbial species may require longer hypervariable regions or alternative sequencing approaches to achieve accurate species-level identification. Nonetheless, the V4 region for our cohort seems to be able to resolve species taxonomy of the biomarkers previously associated with CRC, as we show for the case of Fusobacterium .
Furthermore, it has been proposed that the potential diagnostic value of colonic microbial profiles goes beyond bacteria, as fungal and viral species have been proposed as CRC biomarkers 49 . We recognize that multidomain approaches to discover CRC biomarkers and longitudinal prospective studies to better study the dynamics of cancer progression are warranted to comprehensively inform cancer detection and treatment.
Participant recruitment
The LCPM project was an observational cross-sectional survey for which procedures were approved by the medical ethics committee of the UZL (ethical approval number S57084). Between 2017 and 2018, we recruited patients through the study nurse following a standardized procedure. Briefly, we invited patients scheduled for lower gastrointestinal endoscopy or abdominal surgery for CRC removal at the UZL were invited. After explaining the research project and if they expressed their agreement, participants signed an informed consent, and no compensation was offered. A set of stool sample collection material was provided.
Each patient completed an extensive questionnaire containing information about the date of sample collection, the consistency of the stool, diet, antibiotics usage, clinical symptoms or disease among other variables 17 , as well as an extensive medical and clinical questionnaire using the Websurvey service of KU Leuven.
As a validation cohort we included the FGFP 17 , a population-wide microbiota monitoring effort, representing one of the largest and best characterized fecal microbiota database currently available. Its extensive metadata including health and lifestyle allowed the identification of 69 factors associated with microbiota variation (microbiota covariates). The QMP transformation was conducted in parallel, with the same protocol, for both the FGFP and the LCPM cohorts.
CRC status classification
We invited patients referred for colonoscopy or colectomy to participate in the study. Those that consented were instructed to collect a stool sample at home, which was kept frozen using a sample kit provided by the research team. Upon completion of the medically necessary procedures (colonoscopy or colon resection), we stratified study participants into three diagnosis groups according to their clinical phenotype: (1) patients without evidence of lesions, (2) patients with polyps ( n < 10 and size between 6 and 10 mm) (ADE) and (3) patients with CRC. Patients whose clinical presentation did not fit any of these three groups were excluded from the study. Once the participants were included in the corresponding groups, extensive metadata was collected from their medical records as stated in the informed consent.
Sample collection
The stool samples of patients from UZL were collected as part of the LCPM project using aliquot ready mat without any buffer or preservative (Supplementary Fig. 1 ). The samples were kept at −20 °C freezers at the patients’ homes and brought to our laboratory on icepacks. Upon arrival, samples were stored in the Raes’ Lab at −80 °C until further analysis. Each stool sample had a temperature logger to make sure that, during the storage at home or transport to the laboratory, low stable temperature was maintained.
Stool sample analyses
Microbial load measurement by flow cytometry.
We determined microbial loads of stool samples of LCPM patients following published procedures 23 . We performed cell counting for all other samples in triplicate. Briefly, we dissolved 0.2 g frozen (−80 °C) aliquots in physiological solution to a total volume of 100 ml (8.5 g l −1 NaCl; VWR International). Subsequently, the slurry was diluted 1,000 times. The samples were filtered using a sterile syringe filter (pore size of 5 μm; Sartorius Stedim Biotech). Next, we stained 1 ml of the microbial cell suspension obtained with 1 μl SYBR Green I (1:100 dilution in dimethylsulfoxide; shaded for 15 min of incubation at 37 °C; 10,000 concentrate, Thermo Fisher Scientific) and monitored fluorescence events using the FL1 533/530 nm and FL3 >670 nm optical detectors of the C6 Accuri flow cytometer (BD Biosciences). In addition, forward and sideward scattered light was collected. The BD Accuri CFlow (v.1.0.264.21) software was used to gate and separate the microbial fluorescence events on the FL1/FL3 density plot from background events Supplementary Fig. 2 . A threshold value of 2,000 was applied on the FL1 channel. We evaluated the gated fluorescence events on the forward and sideward density plot, as to exclude remaining background events. We kept instrument and gating settings identical for all samples as described previously 24 . Based on the exact weight of the aliquots analyzed, we converted cell counts to microbial loads per gram of fecal material.
Fecal moisture content
We determined moisture content as the percentage of mass loss after lyophilization from 0.2 g frozen aliquots of nonhomogenized fecal material (−80 °C) as described previously 24 .
Fecal calprotectin measurement
We quantified fecal calprotectin concentrations using the fCAL ELISA Kit (Buhlmann). For patients and FGFP participants, we conducted analyses on frozen fecal material (−80 °C) as described previously 24 .
Microbiota phylogenetic profiling
Dna extraction and sequencing data preprocessing.
The fecal microbiota profile of the FGFP cohort was described previously 17 . For fecal DNA extraction and microbiota profiling of the new cohort, we followed the same protocols 17 .
The bacterial profiling was carried out as described previously 50 . Briefly, we extracted nucleic acids from frozen fecal aliquots using the MagAttract PowerMicrobiome DNA/RNA kit (Qiagen). We modified the manufacturer’s protocol by the addition of a heating step at 90 °C for 10 min after vortexing and excluding the steps where DNA is removed. For bacterial and archaeal characterization, we used 16S ribosomal RNA primers 515F (5′-GTGYCAGCMGCCGCGGTAA-3′) and 806R (5′-GGACTACNVGGGTWTCTAAT-3′) targeting the V4 region. These primers were modified to contain a barcode sequence between each primer and the Illumina adapter sequences to produce dual-barcoded libraries from the extracted DNA (dilution 1:10) in triplicate. Deep sequencing was performed on a MiSeq platform (2 × 250 paired end (PE) reads, Illumina). We randomized all samples and negative controls (polymerase chain reaction (PCR) and extraction controls) taken along for sequencing. After demultiplexing with sdm as part of the LotuS pipeline (v. 1.60) 51 without allowing for mismatches, we further analyzed fastq sequences per sample using DADA2 pipeline (v. 1.6) 52 . Briefly, we removed the primer sequences and the first ten nucleotides after the primer. After merging paired sequences and removing chimeras, we assigned taxonomy using formatted Silva set ‘SLV_nr99_v138.1’. We performed taxonomic assignments at the domain, class, order, family, genus and species levels were performed using the ‘assignTaxonomy’ function from the DADA2 R library, by a naive Bayesian classifier method with a minimum bootstrap confidence of 50, using the ‘silva_nr99_v138.1_wSpecies_train_set.fa.gz’ training database (Extended Data Fig. 5 ). Deep sequencing was performed on a MiSeq platform from the DADA2 R library with the formatted Silva SSU database ‘silva_species_assignment_v138.1.fa.gz’ to obtain species assignments for the amplicon sequence variants (ASVs). We labeled any unassigned ASVs at any taxonomic level, with the prefix ‘uc’ along with the assigned taxonomic level (not species level) to avoid the lack of labels.
Before the analyses, we removed sequences annotated to the class Chloroplast, family mitochondria or unknown archaea and bacteria from eukaryotic origin. phyloseq (v. 1.36.0) 53 and MicroViz (v. 0.11.0) 54 libraries were used for data curation and figure generation.
For the relative microbiome matrix, we transformed ASV counts to relative abundances. In other words, we divided ASV counts by the total counts of ASV per sample. We agglomerated ASV to species level using the phyloseq (v. 1.36.0) 53 function ‘tax_glom’.
We agglomerated ASV to the species level, and the abundance matrix was centered log-ratio (CLR)-transformed using ‘codaSeq.clr ’ in the CoDaSeq (v. 0.99.6) 55 using the minimum proportional abundance detected for each taxon for the imputation of zeros.
Workflow Assessment
We conducted a workflow assessment using (1) a commercial mock community, ZymoBIOMICS Gut, and (2) two Fusobacterium species: Fusobacterium hwasookii (THCT14E2) and Fusobacterium nucleatum (DSM 20482T). The assessment followed our standard methods, involving the amplification, sequencing and analysis of the extracted DNA. This evaluation aimed to assess the performance of our full methodology, as depicted in Extended Data Fig. 6 .
Quality control assessment for amplicon sequencing data (16S rRNA) using RMP
In short, we sequenced all samples in six MiSeq runs (Extended Data Fig. 7a ). Per each run, we used a set of internal controls to identify: 1) Technical variation within and between runs 1) Contamination events during the DNA extraction, 2) Contamination events during the amplification and sequencing procedures and, 3) Carry-over contamination at the sequencing facility and barcode crosstalk.
We amplified all samples, including biological material (stool samples), positive controls (DNA from a stool sample previously profiled and RS: nonhuman gut bacteria strain ‘ Runella slithyformis’ ), negative controls (negative control of extraction (NCE) and negative control during PCR (NCP)) in triplicate using a unique barcode combination, while omitting several barcode combinations to control for primer synthesis cross contamination. We used Runella slithyformis in duplicate within each sequencing library to detect barcode crosstalk during the sequencing procedure (Extended Data Fig. 7b ). This genus is not detected in human gut samples; therefore, we expected no Runella slithyformis reads in any of the stool samples analyzed. We determined technical variation based on the BCD of positive control samples (Extended Data Fig. 7c ). Finally, we included NCEs along the whole process from extraction to bioinformatic analysis. For amplification and sequencing contamination 56 , we used NCP and NCE (Extended Data Fig. 7d and Supplementary Table 12 ), and for carry-over contamination events, we used a different set of barcode combinations in consecutive MiSeq runs 56 .
We built the QMP matrix as described previously 23 . In brief, we downsized samples to even sampling depth, defined as the ratio between sampling size (16S rRNA gene copy number-corrected sequencing depth) and microbial load (the average total cell counts per gram of frozen fecal material; Supplementary Table 2 ). We imputed 16S rRNA genome copies (GC) numbers using RasperGade16S (v. 0.0.1) 57 , a new tool that utilizes a heterogeneous pulsed evolution model for predicting 16S rRNA GC. It not only predicts the GC but also provides confidence estimates for the predictions 57 . We used a minimum rarefied read count of less than 150 for QMP analyses. We converted rarefied ASV abundances into numbers of cells per gram. The QMP matrices had a final size of 589 samples for the study cohort and 1,045 samples for the FGFP validation cohort 17 . We agglomerate the QMP matrix at ASV level to species level using the phyloseq (v. 1.36.0) 53 function ‘tax_glom’. We used the resulting species QMP matrix for the main analysis.
Statistical analysis
We performed all statistical analyses with R (Version 4.2.1, RStudio v.2022.12.0 + 353, 86_64-apple-darwin17.0 (64-bit)) and packages phyloseq (v. 1.36.0) 53 , vegan (v. 2.6.2) 58 , coin(v. 1.4.2) 59 , effectsize (v. 0.8.3), vcd(1.4.11) 60 , DirichletMultinomial(v. 1.34.0) 61 , pairwiseAdonis (v. 0.4.1) and microbiome (v. 1.14.0) 62 . We used nonparametric statistical tests for robust comparisons among unbalanced groups. For multiple testing, we corrected all P values using the Benjamini–Hochberg method (reported as adjusted P ) as appropriate on lists ( n > 1) of features (for example, taxa–metadata or metadata–metadata associations) and also when performing multiple pairwise group ( n > 2) comparisons (for example, KW test with phD test).
Fecal microbiota derived features and visualization
We visualized microbiota interindividual variation by PCoA using BCD on the species QMP matrix 24 , 25 . All the rest of the microbiota derived features were calculated based on QMP. We determined the contribution of metadata variables to microbiota community variation (effect size) of each of 94 variables by dbRDA on a species-level BCD with the capscale function in the vegan package 58 . We visualized absolute abundance species as log10 (abundance +1). This was the same for relative abundance.
Microbiota and physiological features associations
We excluded from analyzes any taxa unclassified at the species level or present in less than 5% of samples per each diagnosis group (Supplementary Table 6 ). We used Spearman correlations for rank–order correlations, between continuous variables complemented by Kendall’s tau correlation, including species abundances, calprotectin values and moisture content. We used the Mann–Whitney U -test to test median differences of continuous variables between two different groups. For more than two groups, for example, for differential abundance analysis for QMP and RMP taxa versus diagnosis groups, we used the KW test with phD test. For differential abundance analysis among diagnosis groups and bacteria species abundances from CLR transformed data, we performed an ANOVA test.
We evaluated statistical differences in the proportions of categorical variables (enterotypes) between patient groups using pairwise CS tests. We tested for deconfounded microbiota contributions to the diagnosis groups variable by using a nested model comparison (ANOVA) of generalized linear models as follows:
[alternative model] glm1 = rank(abundance) + rank(calprotectin) + rank(moisture) + rank(BMI) + diagnosis, where the diagnosis groups were recoded as 1, 2 and 3 for patients without evidence of CTLs, patients with polyps and patients with CRC, respectively. We treated this variable as a continuous variable, translating the directional increase in disease progression, from healthy to lesions, in the colonic mucosa. For the nested model comparison, we used taxa abundances (quantitative or relative) as explanatory variables, the diagnosis groups variable as response variable and BMI, fecal calprotectin and moisture as covariates. Additionally, we employed rank-transformed modeling to perform nonparametric testing on data that is not normally distributed, such as species abundances.
Previous reported CRC microbial markers
To compile a list of published CRC markers that would define taxa that should be tested against covariates in our data set, we conducted a PubMed search query using the keywords ‘CRC AND microbiome AND stool AND human AND biomarkers’. We found ten studies that met our inclusion criteria, namely: (1) a sample size minimum of 60 and (2) the CRC biomarker described at the species level, with statistical significance, in the main text of the publication. We included this list of published biomarkers in our correlation analysis between taxa and the three main covariates (fecal calprotectin, BMI and moisture) within the LCPM cohort. A similar procedure was followed at the genus level, which included 15 studies found in our PubMed search.
CRC microbial markers identification
We performed differential abundance analyzes on nine different CRC shotgun datasets as part of ‘curatedMetagenomicData’ 33 using MetaPhlAn 3.0 profiles to compare the results while controlling for potential differences arising from the classification tools and statistical methods used in each independent study. The results of the meta-analysis are presented in Extended Data Fig. 8 and Supplementary Table 13 .
Enterotyping and visualization
Using the genus matrix (agglomerated and downsized to 10,000 reads), we enterotyped and calculated observed genus richness 53 , as already reported for previous studies 24 , 25 . For enterotyping (or community typing) based on the DMM approach we used R as described previously 61 . We performed enterotyping on a combined genus-level abundance RMP matrix including LCPM samples compiled with 1,045 samples originating from the FGFP 17 . The optimal number of Dirichlet components based on the Bayesian information criterion was four. The four clusters were named ‘Bact1’, ‘Bact2’, ‘Prev’ and ‘Rum’, as described previously 23 .
Reporting summary
Further information on research design is available in the Nature Portfolio Reporting Summary linked to this article.
Data availability
Raw amplicon sequencing data and metadata reported in this study have been deposited in European Nucleotide Archive with accession code EGAS00001007413 . FGFP 16S rRNA gene sequencing data and metadata are available at the European Genome-phenome Archive ( EGAS00001003296 ). The diagnosis metadata and processed microbiome data required for the reanalysis are provided as Supplementary Tables 1 and 14 , respectively. Formatted Silva set ‘SLV_nr99_v138.1’ files were downloaded from Zenodo via https://zenodo.org/records/4587955/files/silva_nr99_v138.1_wSpecies_train_set.fa.gz?download=1 (silva_nr99_v138.1_wSpecies_train_set.fa.gz) 63 and https://zenodo.org/records/4587955/files/silva_species_assignment_v138.1.fa.gz?download=1 (silva_species_assignment_v138.1.fa.gz) 63 . The nine CRC cohort MetaPhlAn 3.0 profiles were collected from curatedMetagenomicData, study names: FengQ_2015, HanniganGD_2017, ThomasAM_2018a, ThomasAM_2018b, VogtmannE_2016, WirbelJ_2018, YachidaS_2019 and YuJ_2015, ZellerG_2014 ( https://doi.org/10.18129/B9.bioc.curatedMetagenomicData ). Source data are provided with this paper.
Code availability
Analysis codes are available via Github at https://github.com/raeslab/QMP-Microbiome-CRC-confounders .
Yang, L. et al. Changes in colorectal cancer incidence by site and age from 1973 to 2015: a SEER database analysis. Aging Clin. Exp. Res. 33 , 1–10 (2020).
CAS Google Scholar
Keum, N. & Giovannucci, E. Global burden of colorectal cancer: emerging trends, risk factors and prevention strategies. Nat. Rev. Gastroenterol. Hepatol. 16 , 713–732 (2019).
Article PubMed Google Scholar
Araghi, M. et al. Global trends in colorectal cancer mortality: projections to the year 2035. Int. J. Cancer https://doi.org/10.1002/ijc.32055 (2018).
Rex, D. K. & Eid, E. Considerations regarding the present and future roles of colonoscopy in colorectal cancer prevention. Clin. Gastroenterol. Hepatol. 6 , 506–514 (2008).
Gupta, V. K. et al. A predictive index for health status using species-level gut microbiome profiling. Nat. Commun. 11 , 4635 (2020).
Article CAS PubMed PubMed Central Google Scholar
Yachida, S. et al. Metagenomic and metabolomic analyses reveal distinct stage-specific phenotypes of the gut microbiota in colorectal cancer. Nat. Med. 25 , 968–976 (2019).
Young, C. et al. Microbiome analysis of more than 2,000 NHSbowel cancer screening programme samples shows the potential to improve screening accuracy. Clin. Cancer Res. 27 , 2246–2254 (2021).
Clos-Garcia, M. et al. Integrative analysis of fecal metagenomics and metabolomics in colorectal cancer. Cancers https://doi.org/10.3390/cancers12051142 (2020).
Article PubMed PubMed Central Google Scholar
Yu, Y. N. et al. Berberine may rescue Fusobacterium nucleatum- induced colorectal tumorigenesis by modulating the tumor microenvironment. Oncotarget 6 , 32013–32026 (2015).
Yu, T. C. et al. Fusobacterium nucleatum promotes chemoresistance to colorectal cancer by modulating autophagy. Cell 170 , 548–563.e16 (2017).
He, T., Cheng, X. & Xing, C. The gut microbial diversity of colon cancer patients and the clinical significance. Bioengineered 12 , 7046–7060 (2021).
Kasai, C. et al. Comparison of human gut microbiota in control subjects and patients with colorectal carcinoma in adenoma: terminal restriction fragment length polymorphism and next-generation sequencing analyses. Oncol. Rep. 35 , 325–333 (2016).
Article CAS PubMed Google Scholar
Thomas, A. M. et al. Metagenomic analysis of colorectal cancer datasets identifies cross-cohort microbial diagnostic signatures and a link with choline degradation. Nat. Med. https://doi.org/10.1038/s41591-019-0405-7 (2019).
Wirbel, J. et al. Meta-analysis of fecal metagenomes reveals global microbial signatures that are specific for colorectal cancer. Nat. Med. https://doi.org/10.1038/s41591-019-0406-6 (2019).
Young, C. et al. The colorectal cancer-associated faecal microbiome of developing countries resembles that of developed countries. Genome Med. 13 , 1–13 (2021).
Article Google Scholar
Vandeputte, D. et al. Stool consistency is strongly associated with gut microbiota richness and composition, enterotypes and bacterial growth rates. Gut 65 , 57–62 (2016).
Falony, G. et al. Population-level analysis of gut microbiome variation. Science 352 , 560–564 (2016).
Poullis, A., Foster, R., Shetty, A., Fagerhol, M. K. & Mendall, M. A. Bowel inflammation as measured by fecal calprotectin: a link between lifestyle factors and colorectal cancer risk. Cancer Epidemiol. Biomarkers Prev. https://doi.org/10.1158/1055-9965.EPI-03-0160 (2004).
Högberg, C., Karling, P., Rutegård, J. & Lilja, M. Diagnosing colorectal cancer and inflammatory bowel disease in primary care: the usefulness of tests for faecal haemoglobin, faecal calprotectin, anaemia and iron deficiency. A prospective study. Scand. J. Gastroenterol. 52 , 69–75 (2017).
Schreuders, E. H., Grobbee, E. J., Spaander, M. C. W. & Kuipers, E. J. Advances in fecal tests for colorectal cancer screening. Curr. Treat. Options Gastroenterol. 14 , 152–162 (2016).
Røseth, A. G. et al. Faecal calprotectin: a novel test for the diagnosis of colorectal cancer? Scand. J. Gastroenterol. 28 , 1073–1076 (1993).
Gloor, G. B., Macklaim, J. M., Pawlowsky-Glahn, V. & Egozcue, J. J. Microbiomedatasets are compositional: and this is not optional. Front. Microbiol . 8 , 2224 (2017).
Vandeputte, D. et al. Quantitative microbiome profiling links gut community variation to microbial load. Nature 551 , 507–511 (2017).
Vieira-Silva, S. et al. Quantitative microbiome profiling disentangles inflammation-and bile duct obstruction-associated microbiota alterations across PSC/IBD diagnoses. Nat. Microbiol . 4 , 1826–1831(2019).
Vieira-Silva, S. et al. Statin therapy is associated with lower prevalence of gut microbiota dysbiosis. Nature https://doi.org/10.1038/s41586-020-2269-x (2020).
Tibble, J. A. & Bjarnason, I. Fecal calprotectin as an index of intestinal inflammation. Drugs Today https://doi.org/10.1358/dot.2001.37.2.614846 (2001).
Quaglio, A. E. V., Grillo, T. G., De Oliveira, E. C. S., Di Stasi, L. C. & Sassaki, L. Y. Gut microbiota, inflammatory bowel disease and colorectal cancer. World J. Gastroenterol. 28 , 4053–4060 (2022).
Zeller, G. et al. Potential of fecal microbiota for early-stage detection of colorectal cancer. Mol. Syst. Biol. 10 , 766 (2014).
Feng, Q. et al. Gut microbiome development along the colorectal adenoma–carcinoma sequence. Nat. Commun. 6 , 6528 (2015).
Vogtmann, E. et al. Colorectal cancer and the human gut microbiome: reproducibility with whole-genome shotgun sequencing. PLoS ONE 11 , e0155362 (2016).
Hannigan, G. D., Duhaime, M. B., Ruffin, M. T., Koumpouras, C. C. & Schloss, P. D. Diagnostic potential and interactive dynamics of the colorectal cancer virome. mBio 9 , e02248-18 (2018).
Yu, J. et al. Metagenomic analysis of faecal microbiome as a tool towards targeted non-invasive biomarkers for colorectal cancer. Gut 66 , 70–78 (2017).
Pasolli, E. et al. Accessible, curated metagenomic data through ExperimentHub. Nat. Methods 14 , 1023–1024 (2017).
Bjarnason, I. The use of fecal calprotectin in inflammatory bowel disease. Gastroenterol. Hepatol. 13 , 53–56 (2017).
Google Scholar
Dai, Z. et al. Multi-cohort analysis of colorectal cancer metagenome identified altered bacteria across populations and universal bacterial markers. Microbiome https://doi.org/10.1186/s40168-018-0451-2 (2018).
Zheng, R. et al. Body mass index (BMI) trajectories and risk of colorectal cancer in the PLCO cohort. Br. J. Cancer 119 , 130–132 (2018).
Carr, P. R. et al. Association of BMI and major molecular pathological markers of colorectal cancer in men and women. Am. J. Clin. Nutr. https://doi.org/10.1093/ajcn/nqz315 (2020).
Rutter, M. et al. Severity of inflammation is a risk factor for colorectal neoplasia in ulcerative colitis. Gastroenterology 126 , 451–459 (2004).
Costa, F. et al. Role of faecal calprotectin as non-invasive marker of intestinal inflammation. Digest. Liver Dis. 35 , 642–647 (2003).
Article CAS Google Scholar
Konikoff, M. R. & Denson, L. A. Role of fecal calprotectin as a biomarker of intestinal inflammation in inflammatory bowel disease. Inflamm. Bowel Dis. https://doi.org/10.1097/00054725-200606000-00013 (2006).
Terzić, J., Grivennikov, S., Karin, E. & Karin, M. Inflammation and colon cancer. Gastroenterology 138 , 2101–2114 (2010).
Lehmann, F. S. et al. Clinical and histopathological correlations of fecal calprotectin release in colorectal carcinoma. World J. Gastroenterol. https://doi.org/10.3748/wjg.v20.i17.4994 (2014).
Pathirana, W. G. W., Chubb, S. P., Gillett, M. J., & Vasikaran, S. D. Faecal calprotectin. Clin. Biochem. Rev. https://doi.org/10.1097/mpg.0000000000001847 (2018).
Bullman, S. et al. Analysis of Fusobacterium persistence and antibiotic response in colorectal cancer. Science 358 , 1443–1448 (2017).
Osman, M. A. et al. Parvimonas micra , Peptostreptococcus stomatis , Fusobacterium nucleatum and Akkermansia muciniphila as a four-bacteria biomarker panel of colorectal cancer. Sci. Rep. 11 , 1–12 (2021).
Turnbaugh, P. J. et al. A core gut microbiome in obese and lean twins. Nature 457 , 480–484 (2009).
Moghaddam, A. A., Woodward, M. & Huxley, R. Obesity and risk of colorectal cancer: a meta-analysis of 31 studies with 70,000 events. Cancer Epidemiol. Biomarkers Prev. 16 , 2533–2547 (2007).
Greathouse, K. L. et al. Gut microbiome meta-analysis reveals dysbiosis is independent of body mass index in predicting risk of obesity-associated CRC. BMJ Open Gastroenterol. https://doi.org/10.1136/bmjgast-2018-000247 (2019).
Liu, N. N. et al. Multi-kingdom microbiota analyses identify bacterial–fungal interactions and biomarkers of colorectal cancer across cohorts. Nat. Microbiol. 7 , 238–250 (2022).
Tito, R. Y. et al. Population-level analysis of Blastocystis subtype prevalence and variation in the human gut microbiota. Gut https://doi.org/10.1136/gutjnl-2018-316106 (2018).
Hildebrand, F., Tadeo, R., Voigt, A. Y., Bork, P. & Raes, J. LotuS: an efficient and user-friendly OTU processing pipeline. Microbiome 2 , 30 (2014).
Callahan, B. J. et al. DADA2: high-resolution sample inference from Illumina amplicon data. Nat. Methods 13 , 581–583 (2016).
McMurdie, P. J. & Holmes, S. phyloseq: an R package for reproducible interactive analysis and graphics of microbiome census data. PLoS ONE 8 , e61217 (2013).
Barnett, D., Arts, I. & Penders, J. microViz: an R package for microbiome data visualization and statistics. J. Open Source Softw. 6 , 3201 (2021).
Gloor, G. B., Wu, J. R., Pawlowsky-Glahn, V. & Egozcue, J. J. It’s all relative: analyzing microbiome data as compositions. Ann. Epidemiol. 26 , 322–329 (2016).
Seitz, V. et al. A new method to prevent carry-over contaminations in two-step PCR NGS library preparations. Nucleic Acids Res. https://doi.org/10.1093/nar/gkv694 (2015).
Gao, Y. & Wu, M. Accounting for 16S rRNA copy number prediction uncertainty and its implications in bacterial diversity analyses. ISME Commun. 3 , 59–67 (2023).
Oksanen, F. J. et al. Vegan: Community Ecology Package. R package Version 2.4-3 https://CRAN.R-project.org/package=vegan (2017).
Hothorn, T., Hornik, K., Van De Wiel, M. A. & Zeileis, A. A Lego system for conditional inference. Am. Stat. https://doi.org/10.1198/000313006×118430 (2006).
Friendly, M. & Institute, S. A. S. Visualizing Categorical Data (SAS Institute, 2000).
Holmes, I., Harris, K. & Quince, C. Dirichlet multinomial mixtures: generative models for microbial metagenomics. PLoS ONE 7 , e30126 (2012).
Shetty, S. A. & Lahti, L. Microbiome data science. J. Biosci. 44 , 1–6 (2019).
McLaren, M. R. & Callahan, B. J. Silva 138.1 prokaryotic SSU taxonomic training data formatted for DADA2. Zenodo https://doi.org/10.5281/zenodo.4587955 (2021).
Download references
Acknowledgements
We thank all study participants and the different staff members involved in the recruitment and execution of this project. We acknowledge L. Rymenans for her contribution to sample analysis. R.Y.T., S.V. and V.L.R. are funded by postdoctoral fellowships from the Research Fund–Flanders (1234321N, 12R6119N and 12V9421N, respectively). This work was funded by the Innovatie door Wetenschap en Technologie project ‘CRC_µBiome: characterization of human and microbial genetic components in premalignant adenoma and colorectal cancer’. The Raes lab is supported by Vlaams Instituut voor Biotechnologie (VIB), KU Leuven and the Rega Institute for Medical Research. The funders had no role in study design, data collection and analysis, decision to publish or preparation of the manuscript.
Author information
These authors contributed equally: Raúl Y. Tito, Sara Verbandt, Sabine Tejpar, Jeroen Raes.
Authors and Affiliations
Laboratory of Molecular Bacteriology, Department of Microbiology and Immunology, Rega Institute, Katholieke Universiteit Leuven, Leuven, Belgium
Raúl Y. Tito, Leo Lahti, Chloe Verspecht, Verónica Lloréns-Rico, Sara Vieira-Silva, Gwen Falony & Jeroen Raes
Center for Microbiology, Vlaams Instituut voor Biotechnologie, Leuven, Belgium
Raúl Y. Tito, Chloe Verspecht, Verónica Lloréns-Rico, Gwen Falony & Jeroen Raes
Digestive Oncology, Department of Oncology, Katholieke Universiteit Leuven, Leuven, Belgium
Sara Verbandt, Marta Aguirre Vazquez & Sabine Tejpar
Department of Computing, University of Turku, Turku, Finland
Systems Biology of Host–Microbiome Interactions Laboratory, Principe Felipe Research Center (CIPF), Valencia, Spain
Verónica Lloréns-Rico
Institute of Medical Microbiology and Hygiene and Research Center for Immunotherapy, University Medical Center of the Johannes Gutenberg-University Mainz, Mainz, Germany
Sara Vieira-Silva & Gwen Falony
Institute of Molecular Biology, Mainz, Germany
Sara Vieira-Silva
Oncology, Janssen Pharmaceutica NV, Beerse, Belgium
Janine Arts
Department of Gastroenterology and Hepatology, Amsterdam University Medical Centers, Amsterdam, the Netherlands
Evelien Dekker
Therapeutics Discovery, Janssen Pharmaceutica NV, Beerse, Belgium
Joke Reumers
You can also search for this author in PubMed Google Scholar
Contributions
This study was conceived by J.A., S.T., J. Reumers and J. Raes. The experiments were designed by R.Y.T. and J. Raes. The data were collected and curated by S.V., M.A.V., L.L., J. Reumers, V.L.R., S.V.S., G.F. and S.T. The molecular data were generated by C.V. and R.Y.T. The statistical analyses were planned and executed by R.Y.T. and J. Raes R.Y.T. and J. Raes drafted the manuscript. All authors revised the article and approved the final version for publication.
Corresponding author
Correspondence to Jeroen Raes .
Ethics declarations
Competing interests.
J.A. and J. Reumers are employees of Janssen Pharmaceutica NV. J. Raes and R.T. are inventors on the patent application WO2017109059A1 in the name of VIB VZW, Katholieke Universiteit Leuven, KU Leuven R&D and Universiteit Gent covering methods for detecting the presence or assessing the risk of development of inflammatory arthritis disease. J. Raes, S.V.S. and G.F. are inventors on the patent application PCT/EP2018/084920 in the name of VIB VZW, KAtholieke Universiteit Leuven, KU Leuven Research and Development and Vrije Universiteit Brussel covering microbiome features associated with inflammation described in Vieira-Silva et al. Nature Microbiology 2019. The other authors declare no competing interests.
Peer review
Peer review information.
Nature Medicine thanks Ruixin Zhu and the other, anonymous, reviewer(s) for their contribution to the peer review of this work. Primary Handling editor: Alison Farrell, in collaboration with the Nature Medicine team.
Additional information
Publisher’s note Springer Nature remains neutral with regard to jurisdictional claims in published maps and institutional affiliations.
Extended data
Extended data fig. 1 association of intestinal inflammation with fusobacterium nucleatum ..
Intestinal calprotectin levels associate Fusobacterium nucleatum absolute ( a) and relative ( b ) abundance in the LCMP. Two-sided Spearman rank correlation (adjP <0.05) and ‘x’ axes are log 10 transformed just for plotting. To rule out that the observed association is driven by a few samples with high abundance of Fusobacterium nucleatum, panel a has an insert of the plot removing samples with Fusobacterium nucleatum values above 1E8 cells per gram of stool. Best-fitting regression line in blue and 95% confidence interval shown in grey shading.
Extended Data Fig. 2 Fusobacterium nucleatum abundances before and after correction for intestinal calprotectin across diagnosis groups.
Absolute abundance of Fusobacterium nucleatum before ( a ) and after ( b ) correcting for intestinal calprotectin. Relative abundance of Fusobacterium nucleatum before ( c ) and after ( d ) correcting for intestinal calprotectin. The whiskers extend from the quartiles to the last data point within 1.5× of the interquartile range, with outliers beyond. The ‘y’ axes for (a) are log 10 transformed values (absolute abundance +1). The whiskers extend from the quartiles to the last data point within 1.5× of the interquartile range, with outliers beyond.
Extended Data Fig. 3 Spearman correlation between species abundance and microbiota covariates in the LCPM and FGFP cohorts.
Two-sided Spearman’s rank correlation comparison between absolute species abundance (QMP) and relative abundance (RMP) from the LCPM (N = 589 samples) and FGFP (N = 1045 samples) cohorts and a, BMI b, faecal calprotectin and c, moisture content values. Spearman correlation adjP < 0.05 (QMP and RMP, Supplementary Table 8 ).
Extended Data Fig. 4 Enterotype stratification by DMM community typing.
a , Identification of optimal number of clusters (Dirichlet components) in the LCPM cohort (n = 589) complemented with 1045 samples from the FGFP cohort, based on the Bayesian Information Criterion (BIC). b , Barplot representation of the average relative abundance of a few representative genera split into the four enterotypes identified by DMM community typing on the combined LCPM and FGFP cohorts (n = 1634).
Extended Data Fig. 5 Taxa assignation performance of the V4 amplicon marker in the LCPM.
a , Bootstrap values distribution across different ranks, b , Proportion of ASVs assigned from species to phylum, c , Proportion of ASVs assigned from species to phylum to each sample. The whiskers extend from the quartiles to the last data point within 1.5× of the interquartile range, with outliers beyond. The figure below (Panel a) illustrates our taxa assignation performance, showing that more than half of the ASVs were assigned to species level with bootstrap values above 80. Panel b shows the ASV assignation proportions from phylum (100%) to species level (50%). A comparison of proportions of ASVs assigned from each sample at different taxonomic levels revealed no significant differences in the distributions of assigned ASVs per sample across diagnosis groups, as indicated in panel c (KW test, p-values > 0.05). The center of the box plot represents the median value of the data, and the whiskers extend from the quartiles to the last data point within 1.5× of the interquartile range, with outliers beyond.
Extended Data Fig. 6 Performance of our methodology in small communities and isolated microorganisms.
a , Species composition of the ZymoBIOMICS gut controls, ten successfully identified species and b , two Fusobacterium species: Fusobacterium hwasookii (THCT14E2) and Fusobacterium nucleatum (DSM 20482T) were successfully identified using our methodology.
Extended Data Fig. 7 Quality control assessment for amplicon sequencing data (V4 16S rRNA gene).
a , The obtained reads for each sample are shown after processing with DADA2 (red and orange dashed lines represent 10, 000 and 1,000 reads, respectively; NCP: PCR negative control, NCE: DNA extraction Negative control, PC: positive control, and RS: Runella slithyformis control). b , Sequencing controls reveal the absence of barcode crosstalk. RS sequences serve as a marker for barcode crosstalk during sequencing. The absence of RS sequences in the samples without RS (no_RS) ruled out barcode crosstalk during the sequencing or PCR setup procedures. c , BCD among technical replicates demonstrating reproducibility. Pairwise comparisons between PC samples within and among MiSeq runs showed values under 0.2 (depicted by the pointed blue line). The center of the box plot represents the median value of the data, and the whiskers extend from the quartiles to the last data point within 1.5× of the interquartile range, with outliers beyond. d , Species composition of negative controls is presented, indicating the relative abundance and prevalence of the top 20 species. None of the species detected with differential abundance using QMP, RMP or CLR were found as background contaminants. Non-significant differences in bacteria composition were observed among DNA sequencing runs (Padj > 0.05, pairwiseAdonis test). A full list of detected species is available in Supplementary Table 12 . Of note, DI18R24 is not shown as the negative controls (NCE and NCP) did not produce reads.
Extended Data Fig. 8 Species and genera associated with CRC on a subset of the curatedMetagenomicData.
After performing our differential abundance procedure on the MataPhalAn 3.0 profiles downloaded from the curatedMetagenomicData, 108 species ( a ) and 63 genera ( b ) were identified across the 9 metagenomics datasets.
Supplementary information
Supplementary information.
Supplementary Figs. 1 and 2 and Tables 1–14.
Reporting Summary
Supplementary tables 1–14.
Supplementary Table 1. Reasons for the colonoscopy referral of the LCPM cohort. Supplementary Table 2. LCMP cohort variable names, 95 variables plus enterotypes. Supplementary Table 3. Associations between continuous variables and cancer progression (KW test with phD tests. N is specified for each test, and statistical significance was derived from two-sided testing and adjusted for multiple testing (adjusted P , Benjamini–Hochberg method)). Supplementary Table 4. Associations between categorical variables and cancer progression (two-sided CS test; statistical significance was derived from two-sided testing and adjusted for multiple testing (adjusted P , Benjamini–Hochberg method)). Supplementary Table 5. Microbiome variation in the LCMP cohort. Independent and cumulative contribution of metadata variables to species-level microbiome variation (dbRDA and stepwise dbRDA; false discovery rate by Benjamini–Hochberg). Cumulative explanatory power and significance level of the included variables are reported. Supplementary Table 6. List of species excluded and included from the analysis. Supplementary Table 7. Differences in absolute (QMP) and relative (RMP) species abundances over diagnostic groups LCMP cohort ( n = 589, KW, phD test; statistical significance was derived from two-sided testing and adjusted for multiple testing (adjusted P , Benjamini–Hochberg method)). Supplementary Table 8. Associations between species abundances (QMP and RMP) and BMI, intestinal calprotectin and moisture in the LCPM cohort ( n = 589, Spearman and Kendall’s tau; statistical significance was derived from two-sided testing and adjusted for multiple testing (adjusted P , Benjamini–Hochberg method)). Supplementary Table 9. Associations between species abundances (QMP and RMP) and BMI, intestinal calprotectin and moisture in the FGFP cohort ( n = 1,045, Spearman; statistical significance was derived from two-sided testing and adjusted for multiple testing (adjusted P , Benjamini–Hochberg method)). Supplementary Table 10. Differences in absolute (QMP) and relative (RMP) species abundances over diagnostic groups in the LCMP cohort subset with normal levels of fecal calprotectin ( n = 340 (112 PWoL, 216 PWP and 12 PWT, KW and adjusted for multiple testing (adjusted P , Benjamini–Hochberg method)). Supplementary Table 11. Associations between categorical variables and enterotype distribution (two-sided CS test; statistical significance was derived from two-sided testing and adjusted for multiple testing (adjusted P , Benjamini–Hochberg method)). Supplementary Table 12. Full list of the species detected in the negative controls (NCE and NCP). Supplementary Table 13. Differences in relative abundances of species profiles from MetaPhlAn 3.0 between CRC and controls from nine published CRC cohorts from the curatedMetagenomicData ( n = 1,254, two-sided Wilcoxon signed-rank test and adjusted for multiple testing (adjusted P , Benjamini–Hochberg method)). Supplementary Table 14. Absolute taxonomic abundances at species level in the LCMP cohort ( n = 589).
Source Data Fig. 1
Statistical source data.
Source Data Fig. 2
Source data fig. 3, source data fig. 4, rights and permissions.
Open Access This article is licensed under a Creative Commons Attribution 4.0 International License, which permits use, sharing, adaptation, distribution and reproduction in any medium or format, as long as you give appropriate credit to the original author(s) and the source, provide a link to the Creative Commons licence, and indicate if changes were made. The images or other third party material in this article are included in the article’s Creative Commons licence, unless indicated otherwise in a credit line to the material. If material is not included in the article’s Creative Commons licence and your intended use is not permitted by statutory regulation or exceeds the permitted use, you will need to obtain permission directly from the copyright holder. To view a copy of this licence, visit http://creativecommons.org/licenses/by/4.0/ .
Reprints and permissions
About this article
Cite this article.
Tito, R.Y., Verbandt, S., Aguirre Vazquez, M. et al. Microbiome confounders and quantitative profiling challenge predicted microbial targets in colorectal cancer development. Nat Med (2024). https://doi.org/10.1038/s41591-024-02963-2
Download citation
Received : 18 November 2022
Accepted : 29 March 2024
Published : 30 April 2024
DOI : https://doi.org/10.1038/s41591-024-02963-2
Share this article
Anyone you share the following link with will be able to read this content:
Sorry, a shareable link is not currently available for this article.
Provided by the Springer Nature SharedIt content-sharing initiative
Quick links
- Explore articles by subject
- Guide to authors
- Editorial policies
Sign up for the Nature Briefing newsletter — what matters in science, free to your inbox daily.

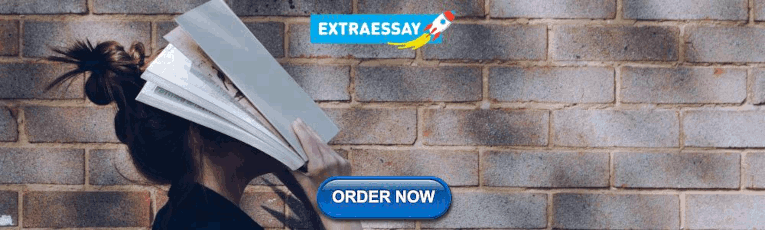
IMAGES
VIDEO
COMMENTS
3. Focus: Explain what you intend to do to solve the problem. Normally, you would now describe what you did to accomplish your research goal. However, if you have not yet carried out your research, you have nothing to report. As such, you should instead explain what you intend to do to accomplish your goal.
Step 2: Methods. Next, indicate the research methods that you used to answer your question. This part should be a straightforward description of what you did in one or two sentences. It is usually written in the past simple tense, as it refers to completed actions.
Definition and Purpose of Abstracts An abstract is a short summary of your (published or unpublished) research paper, usually about a paragraph (c. 6-7 sentences, 150-250 words) long. A well-written abstract serves multiple purposes: an abstract lets readers get the gist or essence of your paper or article quickly, in order to decide whether to….
Informative Abstract Example 1. Emotional intelligence (EQ) has been correlated with leadership effectiveness in organizations. Using a mixed-methods approach, this study assesses the importance of emotional intelligence on academic performance at the high school level. The Emotional Intelligence rating scale was used, as well as semi ...
You can, however, write a draft at the beginning of your research and add in any gaps later. If you find abstract writing a herculean task, here are the few tips to help you with it: 1. Always develop a framework to support your abstract. Before writing, ensure you create a clear outline for your abstract.
Follow these five steps to format your abstract in APA Style: Insert a running head (for a professional paper—not needed for a student paper) and page number. Set page margins to 1 inch (2.54 cm). Write "Abstract" (bold and centered) at the top of the page. Place the contents of your abstract on the next line.
An abstract is a brief summary of your research or creative project, usually about a paragraph long (250-350 words), and is written when you are ready to present your research or included in a thesis or research publication. ... Social Science Example . Office of Undergraduate Research. Contact Info. 618 Kerr Administration Building Corvallis ...
How to Write an Abstract | Steps & Examples. Published on 1 March 2019 by Shona McCombes.Revised on 10 October 2022 by Eoghan Ryan. An abstract is a short summary of a longer work (such as a dissertation or research paper).The abstract concisely reports the aims and outcomes of your research, so that readers know exactly what your paper is about.
5. How to Format an Abstract. Most abstracts use the same formatting rules, which help the reader identify the abstract so they know where to look for it. Here's a list of formatting guidelines for writing an abstract: Stick to one paragraph. Use block formatting with no indentation at the beginning.
Write your paper first, then create the abstract as a summary. Check the journal requirements before you write your abstract, eg. required subheadings. Include keywords or phrases to help readers search for your work in indexing databases like PubMed or Google Scholar. Double and triple check your abstract for spelling and grammar errors.
7th edition. Reading and Understanding Abstracts. Abstracts are short summaries of scientific research articles. This guide will explain how understanding them not only saves time but also helps you conduct better research and write more effectively. Abstracts Are Snapshots. Abstracts provide a snapshot of a study.
Research Paper Abstract. Research Paper Abstract is a brief summary of a research paper that describes the study's purpose, methods, findings, and conclusions. It is often the first section of the paper that readers encounter, and its purpose is to provide a concise and accurate overview of the paper's content.
A good abstract is one that is clear, concise, and critical; it needs to be informative, providing a succinct overview of how the study was conducted, what it found, and what it means for practice.An abstract must be critical, in that implications and conclusions derived from the results of the study emerge logically from the findings and do not overestimate or underestimate the meaning of the ...
An abstract is a summary of a research project. Abstracts precede papers in research journals and appear in programs of scholarly conferences. In journals, the abstract allows readers to quickly grasp the purpose and major ideas of a paper and lets other researchers know whether reading the entire paper will be worthwhile.
Although abstracts and artist statements need to contain key information on your project, your title and summary should be understandable to a lay audience. Please remember that you can seek assistance with any of your writing needs at the MU Writing Center. Their tutors work with students from all disciplines on a wide variety of documents.
There are six steps to writing a standard abstract. (1) Begin with a broad statement about your topic. Then, (2) state the problem or knowledge gap related to this topic that your study explores. After that, (3) describe what specific aspect of this problem you investigated, and (4) briefly explain how you went about doing this.
Here are the basic steps to follow when writing an abstract: 1. Write your paper. Since the abstract is a summary of a research paper, the first step is to write your paper. Even if you know what you will be including in your paper, it's always best to save your abstract for the end so you can accurately summarize the findings you describe in ...
10 Ways to Make the Most of Your RIP Presentation. 1. Present early and often. Better to reconsider your design before submitting the IRB, collecting data, or writing the manuscript. 2. Present weeks or months before key deadlines. You'll be more willing to incorporate major changes and have time to present again. 3.
research and format for dissemination (e.g., manuscript for publication, poster, or platform presen - tation). For example, if the research is complete, the abstract should be the last section written ... Additionally, conferences may have special sections for research-in-progress abstracts for pharmacy trainees, including students and ...
Concisely describe how your results pertain to your study aim or hypothesis. Statements such as "to be completed" or "to be presented" are not acceptable. Remember to report non-significant differences too. Usually the longest section, 3-8 sentences.
Example Abstract of Research in Progress The Effects of Antiretroviral Therapy on the Cardiovascular and Metabolic Health of Mice Presenter(s): Priscilla Ajala Author(s): Priscilla Ajala, Taylor Kress, and Eric Belin de Chantemele Faculty Sponsor(s): Eric Belin de Chantemele, PhD Affiliation(s): Department of Chemistry and Physics, Department of Vascular Biology Center
Evaluative Study Abstract Sample. PLEASE NOTE: Do not include the field names - Purpose, Methods, Results, and Conclusion - in the body of your abstract. Effect of carvedilol or atenolol combined with a renin-angiotesin blocker on glycemic control. Purpose: Beta-blockers decrease cardiovascular risk in patients with hypertension and ...
Poster Abstract Examples Descriptive Report Poster Abstract PLEASE NOTE: Do not include the field names - Purpose, Methods, Results, and Conclusion - in the body of your abstract. Title: Assessing pharmacist competency for processing adult chemotherapy orders in a community hospital Purpose: The avoidance of errors in the processing of chemotherapy orders is an important component
Abstract. The rapidly changing architecture and functionality of electrical networks and the increasing penetration of renewable and distributed energy resources have resulted in various technological and managerial challenges. ... From left to right: (a) an example energy network (b) optimizing the policy of a single prosumer: Section 2.1 (c ...
Despite substantial progress in cancer microbiome research, recognized confounders and advances in absolute microbiome quantification remain underused; this raises concerns regarding potential ...
In this research, we are presenting a precise spectral adjustment procedure for light-emitting diode (LED) and other multilight-source solar simulators. Applying the procedure on an LED-based solar simulator, we achieve excellent agreement in a measurement comparison conducted with Fraunhofer ISE CalLab, using a dual-junction solar cell as test ...